Cooperative Target Tracking Control of Multiple Robots
具有弱通讯的多智能体分布式自适应协同跟踪控制
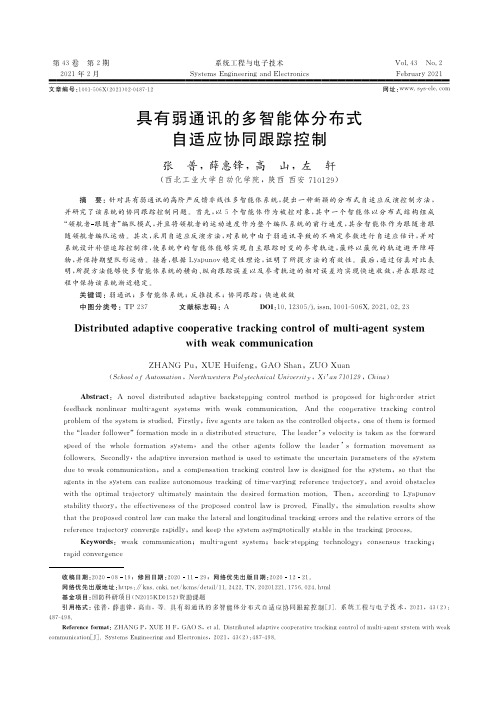
(犛犮犺狅狅犾狅犳 犃狌狋狅犿犪狋犻狅狀,犖狅狉狋犺狑犲狊狋犲狉狀犘狅犾狔狋犲犮犺狀犻犮犪犾犝狀犻狏犲狉狊犻狋狔,犡犻’犪狀710129,犆犺犻狀犪)
犃犫狊狋狉犪犮狋:A noveldistributedadaptivebacksteppingcontrol methodisproposedforhighorderstrict feedback nonlinear multiagentsystems with weak communication.And the cooperativetracking control problemofthesystemisstudied.Firstly,fiveagentsaretakenasthecontrolledobjects,oneofthemisformed the “leaderfollower”formation modeinadistributedstructure.Theleader’svelocityistakenastheforward speedofthe wholeformation system,andthe otheragentsfollow theleader’sformation movementas followers.Secondly,theadaptiveinversionmethodisusedtoestimatetheuncertainparametersofthesystem duetoweakcommunication,andacompensationtrackingcontrollawisdesignedforthesystem,sothatthe agentsinthesystemcanrealizeautonomoustrackingoftimevaryingreferencetrajectory,andavoidobstacles withtheoptimaltrajectoryultimately maintainthedesiredformation motion.Then,accordingtoLyapunov stabilitytheory,theeffectivenessoftheproposedcontrollawisproved.Finally,thesimulationresultsshow thattheproposedcontrollawcanmakethelateralandlongitudinaltrackingerrorsandtherelativeerrorsofthe referencetrajectoryconvergerapidly,andkeepthesystemasymptoticallystableinthetrackingprocess.
模型不确定下无人艇协同目标跟踪控制

第40卷第12期2023年12月控制理论与应用Control Theory&ApplicationsV ol.40No.12Dec.2023模型不确定下无人艇协同目标跟踪控制高胜男1,2,彭周华1,4†,王丹1,李铁山3(1.大连海事大学船舶电气工程学院,辽宁大连116026;2.北方工业大学电气与控制工程学院,北京100144;3.电子科技大学自动化工程学院,四川成都611731;4.水路交通控制全重实验室,辽宁大连116026)摘要:本文针对感知信息不完全、模型参数不确定、海洋环境扰动下的多无人艇系统,文章提出了分布式分层协同目标估计与控制结构.在通信层级,本文设计了基于单向通信拓扑的分布式扩张状态观测器,实现了对非合作目标速度与位置的分布式估计;在控制层级,设计了仅需要相对视距距离的降维扩张状态观测器,不仅实现了对未知动力学不确定性的估计,而且简化了观测器的结构.本文设计了基于降维扩张状态观测器的抗干扰位置跟踪控制律,提高了协同目标跟踪的抗干扰能力.文章采用级联系统稳定性分析证明了闭环系统是输入状态稳定的.此外,本文证明了闭环系统内跟踪误差是一致最终有界的.仿真结果验证了所提方法的有效性.关键词:无人艇;分布式扩张状态观测器;协同目标跟踪;抗干扰控制引用格式:高胜男,彭周华,王丹,等.模型不确定下无人艇协同目标跟踪控制.控制理论与应用,2023,40(12): 2198–2208DOI:10.7641/CTA.2023.30229Cooperative target tracking by multiple unmanned surface vehiclessubject to model uncertaintiesGAO Sheng-nan1,2,PENG Zhou-hua1,4†,WANG Dan1,LI Tie-shan3(1.School of Marine Electrical Engineering,Dalian Maritime University,Dalian Liaoning116026,China;2.School of Electrical and Control Engineering,North China University of Technology,Beijing100144,China;3.School of Automation Engineering,University of Electronic Science and Technology,Chengdu Sichuan611731,China;4.State Key Laboratory of Maritime Technology and Safety,Dalian Liaoning116026,China)Abstract:For the multiple unmanned surface vehicle system with incomplete sensing information,the model parameters uncertainty,and the marine environment disturbance,a distributed hierarchical cooperative target estimation and tracking control structure is proposed.At the communication level,a distributed extended state observer based on the unidirectional communication topology is designed.The speed and location of noncooperative target are estimated.At the control level,a reduced-order extended state observer requiring only relative distance is designed,while not only realizes the synchronous estimation of the unknown dynamic uncertainty,but also simplifies the structure of the observer.In this paper, an anti-disturbance position tracking control law based on the reduced-order extended state observer is designed to improve the anti-interference ability of the cooperative target tracking control system is improved.The input-to-state stability of the closed-loop system is analyzed via cascade theory.Besides,the tracking errors are uniformly ultimately bounded.Simulations verify the effectiveness of the proposed control method.Key words:unmanned surface vehicles;distributed extended state observer;cooperative target tracking;antidisturbance controlCitation:GAO Shengnan,PENG Zhouhua,WANG Dan,et al.Cooperative target tracking by multiple unmanned surface vehicles subject to model uncertainties.Control Theory&Applications,2023,40(12):2198–2208收稿日期:2023−04−19;录用日期:2023−11−22.†通信作者.E-mail:***************.cn.本文责任编委:胡德文.大连市基础重大项目(2023JJ11CG008),国家自然科学基金项目(51979020,51909021,51939001,52071044,52301408,61976033),国家青年拔尖人才计划项目(36261402),辽宁省教育厅高等学校基本科研项目(LJKQZ2021007),辽宁省兴辽英才计划项目(XLYC2007188)资助.Supported by the Key Basic Research of Dalian(2023JJ11CG008),National Natural Science Foundation of China(51979020,51909021,51939001, 52071044,52301408,61976033),the Top-notch Young Talents Program of China(36261402),the Basic Scientific Research in Colleges and Unive-rsities of Liaoning Provincial Education Department(LJKQZ2021007)and the Liaoning Revitalization Talents Program(XLYC2007188).第12期高胜男等:模型不确定下无人艇协同目标跟踪控制21991引言近年来,由于无人艇(unmanned surface vehicle, USV)在侦察搜索、反潜作战、环境监测与船只补给中的重要应用,其运动控制受到了越来越多的关注[1–2].根据受控无人艇数量的不同,可以分为单无人艇运动控制和多无人艇协同运动控制.多无人艇协同作业可以完成单一无人艇不能高效完成或无法完成的复杂任务,在军事和民用领域中或的广泛应用[2].根据运动场景的不同,协同运动控制技术主要分为协同轨迹跟踪[3]、协同路径跟踪[4]、协同给目标包围[5]以及协同目标跟踪[6–7].其中,协同目标跟踪控制技术在对非合作目标进行探测、识别、捕获、跟踪过程中作用突出,典型应用包括“蜂群”跟踪、协同目标打击与海上溢油围捕等,成为当前备受关注的研究热点之一[8].目前,针对单无人艇的目标跟踪问题,国内外科研人员已取得较为丰富的研究成果[9–20].文献[9]采用平行接近制导方法,解决了单无人艇在高速航行的情况下跟踪单个移动目标.文献[10]针对复杂海洋环境扰动下海洋航行器的单个目标跟踪问题,设计了一种基于神经网络的目标跟踪控制器,提高了控制器的鲁棒性.文献[11]针对控制输入增益函数部分已知的单目标无人艇跟踪问题,提出了一种仅需相对距离和相对角度信息的有限时间收敛无人艇控制器.文献[12]设计了一种基于传感器和通信系统的平行接近制导方法,通过跟踪位于目标附近的虚拟目标,实现了无人艇跟踪高速直线运动的目标.值得注意的是,上述研究成果是在目标速度已知的前提下得到的,但在实际应用中,目标速度往往是不可获得的.因此,国内外学者针对速度未知的单无人艇目标跟踪问题展开一系列研究.文献[13]提出了一种仅基于测量距离的切换逻辑控制策略来解决目标跟踪问题.文献[14]提出了一种基于视距测量信息的无人艇三维目标跟踪控制器,采用神经网络自适应控制技术对系统的不确定性进行补偿.文献[15]研究了时变距离下的无人艇单目标跟踪问题,采用有限时间收敛的观测器观测未知的目标速度,实现了时变距离下无人艇单目标的跟踪控制.文献[16]针对目标动态未知的无人艇目标跟踪问题,提出了基于神经网络与动态面控制技术的目标跟踪控制方法.文献[17]提出了基于径向基函数和神经网络的目标跟踪控制器,解决了运动学和动力学同时存在不确定项的跟踪控制问题.考虑控制输入受限问题,文献[18]提出了基于饱和函数的控制器.文献[19]针对未知动态的单无人艇目标跟踪问题,提出了仅采用视距制导距离和角度信息的目标跟踪方法.文献[20]设计了基于滑模控制的领航–跟随目标跟踪控制器,解决了目标速度未知的跟踪控制问题.但随着海上作业任务日益多样,任务难度逐渐提高,一对一的目标跟踪技术会限制无人艇在具有挑战性任务中的应用,例如多无人艇协同跟踪水下潜艇、对特定目标进行协同救援、海区遂行破障等任务,因此研究群体对单体的协同目标跟踪控制,具有较强工程应用前景[21].近年来,多无人艇协同目标跟踪控制取得了一些重要成果[7,22],如文献[7]针对时变海洋环境扰动下的目标跟踪问题,提出了分散式领导–跟随控制方法;文献[22]提出了基于零空间方法的控制器,实现了水面目标的捕获任务.值得注意的是,文献[7]和文献[22]实现了目标速度已知情形下多无人艇协同目标的跟踪控制任务.但在实际应用中,跟踪目标往往是速度难以获得的非合作目标,只能基于局部信息和探测信息完成对非合作目标的估计任务,这给协同目标跟踪控制带来了挑战[20].不仅如此,由于模型参数不确定与海洋环境扰动,跟随无人艇动力学模型含有非线性不确定项.扩张状态观测器作为处理包括内部模型不确定和外部扰动等非线性系统的有力工具,已经被广泛应用到工程领域中[23–30],如文献[20]和文献[26–27]采用了扩张状态观测器对目标的未知速度进行估计,分别实现了单无人艇目标环绕与跟踪任务.值得注意的是,上述文献[20,26–27]中基于扩张状态观测器的估计方法均应用于单无人艇系统,而对于存在感知信息不完全的多无人艇系统,目标位置信息可能仅对部分个体局部已知,跟随无人艇只能基于局部信息和探测信息完成对目标的跟踪任务,这给协同跟踪控制方法的设计带来了挑战[20].因此,如何构建分布式协同估计方法来获取未知的目标动态,为多无人艇协同目标跟踪控制方法的设计奠定基础,是亟待解决的关键问题.基于以上几点,本章研究了感知信息不完全、模型参数不确定、海洋环境扰动下的多无人艇分布式目标状态估计与协同跟踪问题,提出了分布式分层协同目标估计与控制结构.首先,在通信层级,设计了基于单向通信拓扑的分布式扩张状态观测器(ESO),实现了对非合作目标速度与位置的分布式估计.随后,在控制层级,本文设计了仅需要相对距离的降维ESO,不仅实现了对由模型参数不确定与海洋环境扰动造成的未知动力学不确定性的准确估计,而且简化了观测器结构,降低了计算量;设计了基于降维ESO的抗干扰位置跟踪控制律,提高了协同目标跟踪的抗扰能力.文章采用级联系统稳定性分析证明了闭环系统是输入状态稳定的.此外,本文证明了闭环系统内跟踪误差是一致最终有界的.仿真结果验证了所提方法的有效性.所提方法的优点如下:第一,与现有研究成果[9–20]提出的无人艇目标跟踪控制方法相比,本章针对多无人艇系统,构建了一种分布式分层协同目标估计与控制结构,实现了多无人艇协同目标的跟踪控制;2200控制理论与应用第40卷第二,与现有无人艇协同目标跟踪方法[7,20]局限于目标速度已知相比,本章设计了分布式ESO,仅利用获知的目标位置信息,实现了对非合作目标速度与位置的分布式观测;第三,与现有研究成果[23–31]中的ESO 相比,本章采用了降维ESO,不仅实现了对未知动力学的准确估计,提高了抗扰能力,而且简化了观测器的结构,降低了计算量.2问题描述2.1图论在欠驱动无人艇运动控制方法中,可以由式G ={V ,E}来描述欠驱动跟随无人艇与目标无人艇之间的通信关系.其中V ={n 1,···,n N }表示节点集合,用来表示无人艇节点.E ={(n i ,n j )∈V ×V}表示边集合,用来代表无人艇之间是否存在通信.定义一个邻接矩阵A =[a ij ]∈R N ×N 来描述无人艇之间的通信关系,如果(i,j )∈E ,则a ij =1,反之则为a ij =0.定义d i =∑j ∈Na ij 为式G 的度,表示图G 中与n i 有通信的边的条数,所组成的矩阵为度矩阵,记作D =diag {d 1,···,d 2}.则图G 的拉普拉斯矩阵可表示为L =D−A .定义目标无人艇的邻接矩阵A 0=diag {a i0},则有H =L +A 0.如果对于任意(i,j )∈E 有(j,i )∈E ,即欠驱动无人艇i 与欠驱动无人艇j 之间的信息传递不区分方向,则图G 称为无向图,反之则为有向图.如果有向图G 至少存在一个根节点,即该节点到其他任意节点都存在路径,则称图G 包含有向生成树.针对本文所考虑的多无人艇系统通信拓扑,有下列假设和引理.假设1有向图G 中存在有向生成树,根节点能够获得目标无人艇信息.引理1如果图G 是一个有向图,则存在正定矩阵G 和Z ,使得G =Z H +H T Z ,其中,Z =diag {z i }=diag {1h i}(i =1,···,N ),h i =H −11N [32].2.2无人艇运动数学模型考虑如图1所示的由N 艘欠驱动跟随无人艇和单艘目标无人艇组成的多无人艇系统.无人艇在水平面上的运动,可以由一个地球坐标X E −X E 和一个无人艇本体坐标系X B −X B 来描述.目标无人艇的运动学模型可以表示为[33]˙x0=u 0cos ψ0−υ0sin ψ0,˙y 0=u 0sin ψ0+υ0cos ψ0,˙ψ0=r 0,(1)其中:x 0,y 0,ψ0分别表示目标无人艇在地球坐标系下X E 轴坐标、Y E 轴坐标和艏摇角;u 0,v 0,r 0分别表示目标无人艇在无人艇本体坐标系下的纵向速度、横漂速度和艏摇角速度.欠驱动跟随无人艇的运动学和动力学模型可以分别表示为˙x i =u i cos ψi −υi sin ψi ,˙y i =u i sin ψi +υi cos ψi ,˙ψi =r i (2)与m ui ˙u i =f ui (u i ,v i ,r i )+τui +τwui ,m vi ˙v i =f vi (u i ,v i ,r i )+τwvi ,m ri ˙ri =f ri (u i ,v i ,r i )+τri +τwri ,(3)其中:x i ,y i ,ψi 分别表示第i 个跟随无人艇在地球坐标系下X E 轴坐标、Y E 轴坐标和艏摇角(角度正方向如图1所示);u i ,v i ,r i 分别表示第i 个跟随无人艇在无人艇本体坐标系下的纵向速度、横漂速度和艏摇角速度;m ui ,m vi ,m ri 分别表示第i 个跟随无人艇在纵向、横漂、艏摇3个方向的质量与惯量;τui ,τRi 表示第i 个跟随无人艇纵向方向的推力和艏摇方向上的力矩;τwui ,τwvi ,τwri 表示第i 个跟随无人艇外部海洋环境扰动.图1多无人艇协同目标跟踪示意图Fig.1Cooperative target tracking of multiple USVs本文的控制目标是设计一种分布式分层协同单目标估计与控制结构,使得运动学和动力学可分别由式(2)–(3)表示的欠驱动跟随无人艇能够对运动学由式(1)表示的非合作目标无人艇进行跟踪.3分布式分层协同目标估计与控制结构设计本节目标是设计感知信息不完全下的分布式分层协同目标估计与控制结构.由文献[34]可知,无人艇之间保持相对运动角度变化运动,系统可观测.因此,首先在通信层级建立了基于单向通信拓扑的分布式ESO,实现了对非合作目标无人艇位置和速度的分布式估计.随后,在控制层级,结合视距制导原理,设第12期高胜男等:模型不确定下无人艇协同目标跟踪控制2201计了仅需要相对视距距离的ESO,不仅实现了对由内部模型不确定和外部海洋环境扰动造成动力学不确定性的准确估计,而且简化了观测器的结构.最后,基于降维ESO,设计了抗干扰位置跟踪控制律,用于每个跟随无人艇跟踪非合作目标,不仅实现了编队模式下的协同目标跟踪,而且提高了协同目标跟踪的抗扰性.所设计的多无人艇分布式分层协同单目标估计与控制结构框图如图2所示.图2分布式分层协同目标估计与控制结构图Fig.2The distributed hierarchical coordinated target estimation and control structure3.1通信层设计首先,定义(ˆx 0i ,ˆy 0i )和(ˆu 0i ,ˆv 0i )分别为第i 个跟随无人艇在地球坐标系下对非合作目标无人艇位置和速度的估计.为实现对非合作目标无人艇位置和速度的估计,设计如下分布式ESO˙ˆu 0i =−ck o ˆu (N ∑j =1a ij (ˆx 0i −ˆx 0j )+a 0i (ˆx 0i −x 0)),˙ˆx 0i =−ck o ˆx (N ∑j =1a ij (ˆx 0i −ˆx 0j )+a 0i (ˆx 0i −x 0))+ˆu 0i ,˙ˆv 0i =−ck o ˆv (N ∑j =1a ij (ˆy 0i −ˆy 0j )+a 0i (ˆy 0i −y 0)),˙ˆy 0i =−ck o ˆy (N ∑j =1a ij (ˆy 0i −ˆy 0j )+a 0i (ˆy 0i −y 0))+ˆv 0i ,(4)式中:k oˆx ∈R +,k ˆuo ∈R +,k ˆy o ∈R +,k ˆv o ∈R +,c ∈R +表示分布式ESO 的增益.为了后续分析简便,定义p 0=[x 0y 0]T ,ˆp 0i =[ˆx 0i ˆy 0i ]T ,ˆω0i =[ˆu 0i ˆv 0i ]T .采用所设计的分布式ESO,仅利用邻居位置信息便可估计目标位置与速度信息.随后,定义如下估计误差˜x 0i =ˆx 0i −x 0,˜y 0i =ˆy 0i −y 0,˜u 0i =ˆu 0i −˙x 0与˜v 0i =ˆv 0i −˙y 0,则误差动态可表示为˙˜u 0i =−ck o ˆu (N ∑j =1a ij (˜x 0i −˜x 0j )+a 0i ˜x 0i )−¨x 0,˙˜x 0i =−ck o ˆx (N ∑j =1a ij (˜x 0i −˜x 0j )+a 0i ˜x 0i )+˜u 0i ,˙˜v 0i =−ck o ˆv (N ∑j =1a ij (˜y 0i −˜y 0j )+a 0i ˜y 0i )−¨y 0,˙˜y 0i =−ck o ˆy (N ∑j =1a ij (˜x 0i −˜y 0j )+a 0i ˜y 0i )+˜v 0i ,(5)定义χi =[˜x 0i ˜u 0i ˜y 0i ˜v 0i ]T ,χ=[χ1···χN ]T ,1N =[11···1]T N ,¨p0=[¨x 00¨y 00]T ,则上述式(5)可以整理为˙χ=(I N ⊗A −c H ⊗F C )χ−1N ⊗(B ¨p 0),(6)式中A =10000000001000,(7)B =[0101]T ,C =[1000]和F =[k o ˆx k o ˆuk o ˆy k o ˆv ]T.存在正定对称矩阵P 与Q 使得A T P +P A −P C T CP +Q 0.2202控制理论与应用第40卷3.2控制层设计3.2.1相对动态方程定义第i 个跟随无人艇与第i 个由分布式ESO 产生的虚拟点之间视距距离与角度为{ρi =√(ˆy 0i −y i −∆yi )2+(ˆx 0i −x i −∆xi )2,βi =atan2(ˆy 0i −y i −∆yi ,ˆx 0i −x i −∆xi ),(8)其中:∆xi 与∆yi 表示设计的第i 个虚拟点与目标无人艇之间的期望偏差.可以得到位置跟踪误差e ρi 与角度跟踪误差e βi 为{e ρi =ρi −l i ,e βi =βi −ψi −θi ,(9)式中:l i 为期望距离;θsi 为侧滑角.随后,定义速度跟踪误差e ui 与e ri 为{e ui =u i −αui ,e ri =r i −αri ,(10)式中αui 与αri 为虚拟控制律并将在下面进行设计.根据式(2)–(3),可以求得位置误差、角度误差和速度误差的动态方程,如下所示:˙e ρi =˙ˆx 0i cos βi +˙ˆy 0i sin βi −˙l i −u i −v i sin (βi −ψi )+2u i sin 2βi −ψi 2,˙e βi =1ρi(−˙ˆx 0i sin βi +˙ˆy 0i cos βi −r i −˙θi −v i cos (βi −ψi )+u i sin (βi −ψi )),˙e ui =f ui (u i ,v i ,r i )−˙αui +τwui m ui +τui m ui ,˙e ri =f ri (u i ,v i ,r i )−˙αri +τwri m ri +τri m ri,(11)为了方便分析,将上述动态方程简化为如下形式:˙e ρi =ζui (·)−αui ,˙eβi =ζri (·)−αri ,˙e ui =g ui(·)+τuim ui ,˙e ri =g ri (·)+τri m ri,(12)式中ζui (·)=˙ˆx 0i cos βi +˙ˆy 0i sin βi −˙l i −u i +αui −v i sin (βi −ψi )+2u i sin 2βi −ψi 2,ζri (·)=1ρi (−˙ˆx 0i sin βi +˙ˆy 0i cos βi −v i cos (βi −ψi )+u i sin (βi −ψi ))−˙θi −r i +αri ,g ui (·)=f ui (u i ,v i ,r i )−˙αui +τwui m ui ,g ri (·)=f ri (u i ,v i ,r i )−˙αri +τwri m ri.(13)3.2.2降维ESO 设计由于侧滑角未知、模型参数未知以及海洋环境扰动等因素,函数ζui ,ζri ,g ui 与g ri 是不可测的.为了估计上述未知函数,设计如下降维ESO˙s ρi =−k o ρi s ρi −(k o ρi )2e ρi +k o ρi αui ,ˆζui =s ρi +k o ρi e ρi ,˙s βi =−k o βi s βi −(k o βi )2e βi +k o βi αri ,ˆζri =s βi +k o βi e βi ,˙s ui =−k o ui s ui −(k o ui )2e ui −k o ui τui m ui ,ˆg ui =s ui +k o ui e ui ,˙s vi =−k o ri s ri −(k o ri )2e ri −k o ri τri m ri ,ˆg ri=s ri+k o ri e ri,(14)式中s ρi ,s βi ,s ui 和s ri 为降维ESO 的辅助状态;k oρi ,k o βi ,k o ui 和k o ri 为降维ESO 的增益;ˆζui ,ˆζri ,ˆg ui 和ˆg ri 分别为ζui ,ζri ,g ui 和g ri 的估计值.本文中,位置跟踪控制律的设计是基于以下假设成立的基础上.假设2非线性函数ζui ,ζri ,g ui 和g ri 的导数˙ζui ,˙ζri ,˙g ui ,˙g ri 是有界的,满足∥˙ζui ∥ ζui ∗,∥˙ζri ∥ ζri ∗,||˙g ui ∥ g ∗ui 和||˙g ri ∥ g ∗ri ,其中ζ∗ui ∈R +,ζ∗ri ∈R +,g ∗ui ∈R +与g ∗ri ∈R +.定义上述未知函数的估计误差为˜ζui =ˆζui −ζui ,˜ζri =ˆζri −ζri ,˜g ui =ˆg ui −g ui 与˜g ri =ˆg ri −g ri .则误差动态为 ˙˜ζui =−k o ρi s ρi −(k o ρi )2e ρi +k o ρi ζui −˙ζui =−k o ρi ˜ζui −˙ζui ,˙˜ζri =−k o βi s βi −(k o βi )2e βi +k o βi ζri −˙ζri =−k o βi ˜ζri −˙ζri ,˙˜g ui =−k o ui s ui −(k o ui )2e ui +k o ui g ui −˙g ui =−k o ui ˜g ui −˙g ui ,˙˜g ri =−k o ri s ri −(k o ri )2e ri +k o ri g ri −˙g ri =−k o ri ˜g ri −˙g ri ,(15)3.2.3位置跟踪控制律设计为了稳定跟踪误差e ρi ,e βi ,e ui 与e ri ,本部分在动力学层设计基于降维ESO 的抗干扰位置跟踪控制律,如下所示:αui =c ρi e ρi Πρi +ˆζui ,αri =c βi e βi Πβi+ˆζri ,τui ={−c ui e ui Πui −ˆg ui }m ui ,τri ={−c ri e ri Πri−ˆg ri }m ri ,(16)式中:c ρi ∈R +,c βi ∈R +,c ui ∈R +,c ri ∈R +,且Πρi=第12期高胜男等:模型不确定下无人艇协同目标跟踪控制2203√e2ρi +∆2ρi,Πβi=√e2βi+∆2βi,Πui=√e2ui+∆2ui,Πri =√e2ri+∆2ri,满足∆ρi∈R+,∆βi∈R+,∆ui∈R+与∆ri∈R+,将式(16)代入式(12)中,可得误差动态如下所示:˙eρi=−cρi eρiΠρi−˜ζui,˙eβi=−cβi eβiΠβi−˜ζri,˙e ui=−c ui e uiΠui−˜g ui,˙e ri=−c ri e riΠri−˜g ri,(17)4稳定性分析4.1分布式ESO误差子系统稳定性分析引理2在满足假设1的前提下,如果存在F=P−1C T与c>1mini=1,···,N{h i g i},则分布式ESO误差子系统(5)以¨p0为输入,以χ为状态,是输入状态稳定的.证考虑如下李雅普诺夫函数Vχ=12χT(Z⊗P)χ,(18)对Vχ进行求导,根据式(5)可得˙V 1=12χT(Z⊗(A T P+P A)−cG⊗(P C T CP))χ−χT((Z1N)⊗(P B¨p0)).(19)为了简化证明,根据文献[35],引入状态转换概念可得¯χ=(U T⊗I N)χ,基于状态转换,式(19)可整理为˙V χ=12N∑i=1z i¯χi T((A T P+P A)−cq i g i P C T CP)ׯχi−χT((Z1N)⊗(P B¨p0))−12mini=1···N(z i)×λmin(Q)∥¯χ∥2+mini=1,···,N(z i)∥¯χ∥∥P B∥∥¨p0∥,由∥χ∥ 2∥P B∥∥¨p0∥θ1λmin(Q)可得˙Vχ −12mini=1···N(z i)λmin(Q)(1−θ1)||¯χ||2,其中0<θ1<1.综上所述,分布式ESO误差子系统(5)是输入状态稳定的,有||χ|| max{γ¯χ(∥χ(0)∥, t),σ¨p0(∥¨p0∥)},式中γ¯χ为KL函数,且σ¨p0为K函数,如下表示:σ¨p0(s)=√λmax(P)λmax(Z)λmin(P)λmin(Z)2∥P B∥θ1λmin(Q)s.(20)4.2降维ESO误差子系统稳定性分析引理3在满足假设1和假设2的前提下,降维ESO误差子系统(15)以˙ζu,˙ζr,˙g u与˙g r为输入,˜ζu,˜ζr,˜g u与˜g r为状态,是输入状态稳定的.证建立如下李雅普诺夫函数:V gi=12N∑i=1{˜ζ2ui+˜ζ2ri+˜g2ui+˜g2ri},(21)对其进行求导,根据式(15)可得˙Vgi=∑Ni=1{−k oρi˜ζ2ui−˙ζui˜ζui−k oβi˜ζ2ri−˙ζri˜ζri−k oui˜g2ui−˙g ui˜g ui−k o ri˜g2ri−˙g ri˜g ri}−k min∥E g∥2+∥ξ∥∥E g∥,(22)其中:k min=min{||k oρi||,||k oβi||,||k o ui||,||k o ri||};E g=[E g1···E gN]T,E gi=[˜ζu,˜ζr,˜g u,˜g r]T,k oρi=[k oρ1···k oρN]T,k oβi=[k oβ1···k oβN]T,k oui=[k ou1···k o uN]T,k ori=[k or1···k o rN]T,ξ=[ξ1···ξN]T,ξi=[∥˙ζu∥∥˙ζr∥∥˙g u∥∥˙g r∥]T,˜ζu=[˜ζu1···˜ζuN]T,˜ζr=[˜ζr1···˜ζrN]T,˜g u=[˜g u1···˜g uN]T,˜g r=[˜g r1···˜g rN]T,˜g r=[˜g r1···˜g rN]T,˙ζu=[˙ζu1···˙ζuN]T,˙ζr=[˙ζr1···˙ζrN]T,˙g u=[˙g u1···˙g uN]T,˙g r=[˙g r1···˙g rN]T.由∥E g∥∥˙ζu∥θ2k min+∥˙ζr∥θ2k min+∥˙g u∥θ2k min+∥˙g r∥θ2k min∥ξ∥θ2k min,可得˙V gi −k min(1−θ2)∥E g∥2,其中0<θ2<1.综上所述,降维ESO误差子系统(15)是输入状态稳定的,且有∥E g∥ max{γE g(∥E g(0)∥,t),σξ(∥ξ∥)},式中γE g为KL函数,σξ(s)=∥ξ∥θ2k mins为K函数.证毕.4.3跟踪误差子系统稳定性分析引理4在满足假设1和假设2的前提下,跟踪误差子系统(17)以˜ζu,˜ζr,˜g u与˜g r为输入,eρ,eβ,e u和e r为状态,是输入状态稳定的.2204控制理论与应用第40卷证建立如下李雅普诺夫函数:V ei =12N ∑i =1{e 2ρi+e 2βi +e 2ui +e 2ri },(23)对其进行求导,根据式(17)可得:˙V ei =−N ∑i =1{c ρi (e ρi )2Πρi +c βi (e βi )2Πβi+c ui (e ui )2Πui +c ri (e ri )2Πri−e ui ˜g ui −e ri ˜g ri −e ρi ˜ζui −e βi ˜ζri },定义c min =min {∥c ρ∥,∥c β∥,∥c u ∥,∥c r ∥},c ρ=[c ρ1···c ρN ],c β=[c β1···c βN ],c u =[c u 1···c uN ],c r =[c r 1···c rN ],E e =[e ρe βe u e r ]T ,∆max =max {∥∆ρ∥,∥∆β∥,∥∆u ∥,∥∆r ∥},e ρ=[e ρ1···e ρN ],e β=[e β1···e βN ],e u =[e u 1···e uN ],e r =[e r 1···e rN ],∆ρ=[∆ρ1···∆ρN ],∆β=[∆β1···∆βN ],∆u =[∆u 1···∆uN ],∆r =[∆r 1···∆rN ],ϖ=[∥˜ζu ∥∥˜ζr ∥∥˜g u ∥∥˜g r ∥]T ,(24)可得˙V ei −c min ∥E e ∥2√∥E e ∥2+∆2max+∥ϖ∥∥E e ∥,由∥E e ∥∥˜ζu ∥θ3c min +∥˜ζr ∥θ3c min +∥˜g u ∥θ3c min +∥˜g r ∥∥θ3c min ∥ϖ∥θ3c min,有˙V ei −c min (1−θ3)∥E e ∥2,其中0<θ3<1.可得跟踪误差子系统(17)是输入状态稳定的,且跟踪误差e ρ,e β,e u ,e r 是有界的,得∥E e ∥ max {γE e (∥E e (0)∥,t ),σϖ(∥ϖ∥)},其中γE e 为KL 函数,σϖ(s )=∥ϖ∥θ3c mins 为K 函数.以下定理给出闭环系统稳定性结论.定理1考虑由非合作目标无人艇(1)和跟随无人艇(2)(3)组成的无人艇系统.满足假设1与假设2的前提下,采用分布式ESO(4),降维ESO(14),抗干扰位置跟踪控制律(16),则由子系统(5),子系统(15)和子系统(17)组成的闭环系统是输入状态稳定的,此外,系统中所有误差均为一致最终有界的.证毕.证引理2指出分布式ESO 误差子系统(5)是以¨p 0为输入,以χ为状态,是输入状态稳定的.引理3指出降维ESO 误差子系统(15)以˙ζu ,˙ζr ,˙g u 与˙g r 为输入,˜ζu ,˜ζr ,˜g u 与˜g r 为状态,是输入状态稳定的.引理4指出跟踪误差子系统(17)以˜ζu ,˜ζr ,˜g u 与˜g r 为输入,e ρ,e β,e u和e r 为状态,是输入状态稳定的.应用文献[36]中级联系统稳定性定理可得由子系统(5),子系统(15)和子系统(17)组成的级联系统以˜ζu ,˜ζr ,˜g u ,˜g r ,e ρ,e β,e u 和e r 为状态,以˙ζu ,˙ζr ,˙g u 与˙g r 为输入,是输入状态稳定的,即存在KL 函数γE b 和K 函数σϕ使得∥E b ∥ max {γE b (∥E b (0)∥,t ),σϕ(∥ϕ∥)},式中:E b =[˜ζu ˜ζr ˜g u ˜g r e ρe βe u e r ]T ;ϕ=[˙ζu ˙ζr ˙g u ˙g r ˜ζu ˜ζr ˜g u ˜g r b u b r ]T .由假设2可知,˙ζu ,˙ζr ,˙g u ,˙g r 均有界,且界值为ζ∗u ,ζ∗r ,g ∗u ,g ∗r .因此,闭环系统误差信号是一致最终有界的.证毕.5仿真验证考虑一个由六艘跟随无人艇和单艘目标无人艇组成的多无人艇网络系统,它们之间的通信拓扑关系如图3所示。
Adaptive Trajectory Tracking Control of Skid-Steered Mobile Robots
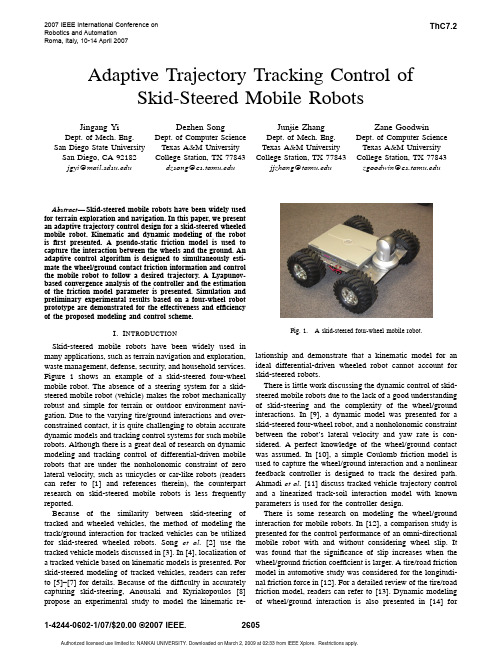
1-4244-0602-1/07/$20.00 ©2007 IEEE.
2605
Authorized licensed use limited to: NANKAI UNIVERSITY. Downloaded on March 2, 2009 at 02:33 from IEEE Xplore. Restrictions apply.
I. I NTRODUCTION Skid-steered mobile robots have been widely used in many applications, such as terrain navigation and exploration, waste management, defense, security, and household services. Figure 1 shows an example of a skid-steered four-wheel mobile robot. The absence of a steering system for a skidsteered mobile robot (vehicle) makes the robot mechanically robust and simple for terrain or outdoor environment navigation. Due to the varying tire/ground interactions and overconstrained contact, it is quite challenging to obtain accurate dynamic models and tracking control systems for such mobile robots. Although there is a great deal of research on dynamic modeling and tracking control of differential-driven mobile robots that are under the nonholonomic constraint of zero lateral velocity, such as unicycles or car-like robots (readers can refer to [1] and references therein), the counterpart research on skid-steered mobile robots is less frequently reported. Because of the similarity between skid-steering of tracked and wheeled vehicles, the method of modeling the track/ground interaction for tracked vehicles can be utilized for skid-steered wheeled robots. Song et al. [2] use the tracked vehicle models discussed in [3]. In [4], localization of a tracked vehicle based on kinematic models is presented. For skid-steered modeling of tracked vehicles, readers can refer to [5]–[7] for details. Because of the difficulty in accurately capturing skid-steering, Anousaki and Kyriakopoulos [8] propose an experimental study to model the kinematic re-
合作雷达信号干扰分析

合作雷达信号干扰分析发布时间:2023-07-10T06:40:59.574Z 来源:《科技新时代》2023年6期作者:高钰,贾润熙,王永虎,郑安良[导读] 在航天测控领域中,对于空间内合作目标的跟踪测量任务通常由多台跟踪测量雷达组成的雷达链接力完成,雷达链中合作雷达测量任务弧段存在交叉现象。
酒泉卫星发射中心酒泉 735700摘要:在航天测控领域中,对于空间内合作目标的跟踪测量任务通常由多台跟踪测量雷达组成的雷达链接力完成,雷达链中合作雷达测量任务弧段存在交叉现象。
合作雷达也因复杂的电磁环境而极大地提高了跟踪测量任务的难度。
本文针对雷达链跟踪任务中出现目标丢失的问题进行分析研究,提出改进的方法和对策,提高雷达链中合作雷达的捕获跟踪性能。
关键词:合作雷达;干扰;跟踪性能Analysis of Cooperative Radar Signal Jammingjirunxi,gaoyu,wangyonghu,zhenganliang63623army jiuquan chinaAbstract:In the field of space TT&C, the tracking and measurement tasks of cooperative targets in space are usually completed by a radar chain composed of multiple tracking and measurement radars, and the measurement task arcs of cooperative radars in the radar chain are crossed. Because of the complex electromagnetic environment, the task of tracking and measurement of cooperative radar is very difficult. In this paper, the problem of target loss in radar chain tracking task is analyzed and studied, and the improved methods and countermeasures are proposed to improve the acquisition and tracking performance of cooperative radar in radar chain.Key words:Cooperative radar;Jamming;Tracking performance1 引言由于雷达链中合作雷达通常采用相参应答方式工作,且工作点频、脉冲宽度、雷达重复周期等基本参数设置相同,因此在多台雷达跟踪任务中,容易产生多个反射、应答回波信号交会干扰的影响,合作雷达信号相互干扰,电磁情况复杂,严重影响雷达的捕获和跟踪,以至任务弧段内跟踪目标的丢失。
考虑行车能见度状况的车辆队列协作控制
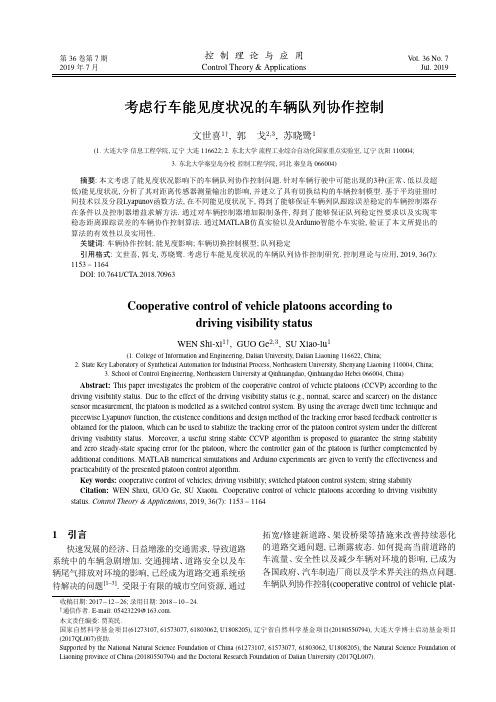
控制理论与应用
Control Theory & Applica车能见度状况的车辆队列协作控制
文世喜1†, 郭 戈2,3, 苏晓鹭1
(1. 大连大学 信息工程学院, 辽宁 大连 116622; 2. 东北大学 流程工业综合自动化国家重点实验室, 辽宁 沈阳 110004;
3. 东北大学秦皇岛分校 控制工程学院, 河北 秦皇岛 066004)
摘要: 本文考虑了能见度状况影响下的车辆队列协作控制问题. 针对车辆行驶中可能出现的3种(正常、低以及超 低)能见度状况, 分析了其对距离传感器测量输出的影响, 并建立了具有切换结构的车辆控制模型. 基于平均驻留时 间技术以及分段Lyapunov函数方法, 在不同能见度状况下, 得到了能够保证车辆列队跟踪误差稳定的车辆控制器存 在条件以及控制器增益求解方法. 通过对车辆控制器增加限制条件, 得到了能够保证队列稳定性要求以及实现零 稳态距离跟踪误差的车辆协作控制算法. 通过MATLAB仿真实验以及Ardunio智能小车实验, 验证了本文所提出的 算法的有效性以及实用性.
关键词: 车辆协作控制; 能见度影响; 车辆切换控制模型; 队列稳定 引用格式: 文世喜, 郭戈, 苏晓鹭. 考虑行车能见度状况的车辆队列协作控制研究. 控制理论与应用, 2019, 36(7): 1153 – 1164 DOI: 10.7641/CTA.2018.70963
Cooperative control of vehicle platoons according to
1 引言
快速发展的经济、日益增涨的交通需求, 导致道路 系统中的车辆急剧增加. 交通拥堵、道路安全以及车 辆尾气排放对环境的影响, 已经成为道路交通系统亟 待解决的问题[1–3]. 受限于有限的城市空间资源, 通过
高速铁路移动闭塞和多智能体协调控制文献综述
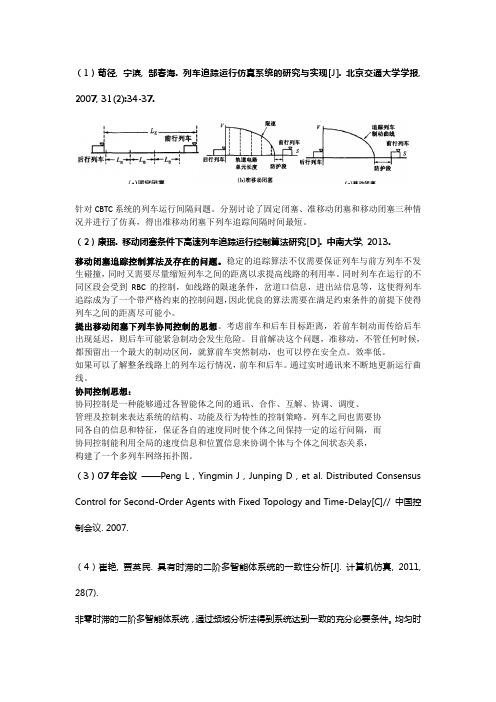
(1)荀径, 宁滨, 郜春海. 列车追踪运行仿真系统的研究与实现[J]. 北京交通大学学报, 2007, 31(2):34-37.针对CBTC系统的列车运行间隔问题。
分别讨论了固定闭塞、准移动闭塞和移动闭塞三种情况并进行了仿真,得出准移动闭塞下列车追踪间隔时间最短。
(2)康珉. 移动闭塞条件下高速列车追踪运行控制算法研究[D]. 中南大学, 2013.移动闭塞追踪控制算法及存在的问题。
稳定的追踪算法不仅需要保证列车与前方列车不发生碰撞,同时又需要尽量缩短列车之间的距离以求提高线路的利用率。
同时列车在运行的不同区段会受到RBC的控制,如线路的限速条件,岔道口信息,进出站信息等,这使得列车追踪成为了一个带严格约束的控制问题,因此优良的算法需要在满足约束条件的前提下使得列车之间的距离尽可能小。
提出移动闭塞下列车协同控制的思想。
考虑前车和后车目标距离,若前车制动而传给后车出现延迟,则后车可能紧急制动会发生危险。
目前解决这个问题,准移动,不管任何时候,都预留出一个最大的制动区间,就算前车突然制动,也可以停在安全点。
效率低。
如果可以了解整条线路上的列车运行情况,前车和后车。
通过实时通讯来不断地更新运行曲线。
协同控制思想:协同控制是一种能够通过各智能体之间的通讯、合作、互解、协调、调度、管理及控制来表达系统的结构、功能及行为特性的控制策略。
列车之间也需要协同各自的信息和特征,保证各自的速度同时使个体之间保持一定的运行问隔,而协同控制能利用全局的速度信息和位置信息来协调个体与个体之间状态关系,构建了一个多列车网络拓扑图。
(3)07年会议——Peng L , Yingmin J , Junping D , et al. Distributed Consensus Control for Second-Order Agents with Fixed Topology and Time-Delay[C]// 中国控制会议. 2007.(4)崔艳, 贾英民. 具有时滞的二阶多智能体系统的一致性分析[J]. 计算机仿真, 2011, 28(7).非零时滞的二阶多智能体系统,通过频域分析法得到系统达到一致的充分必要条件。
文献——精选推荐
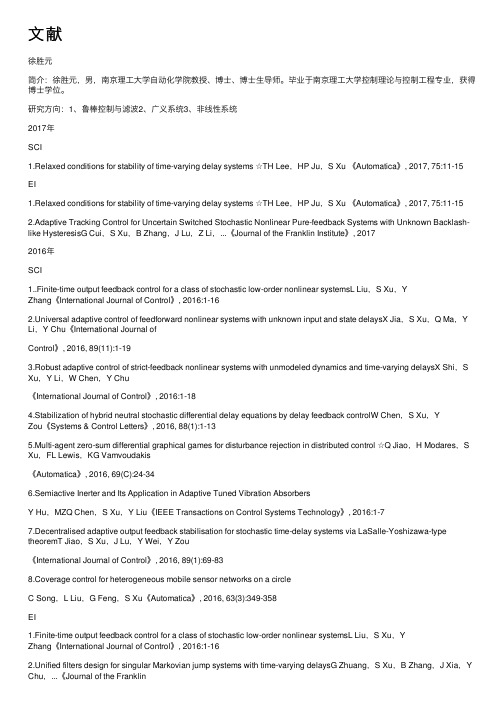
⽂献徐胜元简介:徐胜元,男,南京理⼯⼤学⾃动化学院教授、博⼠、博⼠⽣导师。
毕业于南京理⼯⼤学控制理论与控制⼯程专业,获得博⼠学位。
研究⽅向:1、鲁棒控制与滤波2、⼴义系统3、⾮线性系统2017年SCI1.Relaxed conditions for stability of time-varying delay systems ☆TH Lee,HP Ju,S Xu 《Automatica》, 2017, 75:11-15EI1.Relaxed conditions for stability of time-varying delay systems ☆TH Lee,HP Ju,S Xu 《Automatica》, 2017, 75:11-152.Adaptive Tracking Control for Uncertain Switched Stochastic Nonlinear Pure-feedback Systems with Unknown Backlash-like HysteresisG Cui,S Xu,B Zhang,J Lu,Z Li,...《Journal of the Franklin Institute》, 20172016年SCI1..Finite-time output feedback control for a class of stochastic low-order nonlinear systemsL Liu,S Xu,YZhang《International Journal of Control》, 2016:1-162.Universal adaptive control of feedforward nonlinear systems with unknown input and state delaysX Jia,S Xu,Q Ma,Y Li,Y Chu《International Journal ofControl》, 2016, 89(11):1-193.Robust adaptive control of strict-feedback nonlinear systems with unmodeled dynamics and time-varying delaysX Shi,S Xu,Y Li,W Chen,Y Chu《International Journal of Control》, 2016:1-184.Stabilization of hybrid neutral stochastic differential delay equations by delay feedback controlW Chen,S Xu,YZou《Systems & Control Letters》, 2016, 88(1):1-135.Multi-agent zero-sum differential graphical games for disturbance rejection in distributed control ☆Q Jiao,H Modares,S Xu,FL Lewis,KG Vamvoudakis《Automatica》, 2016, 69(C):24-346.Semiactive Inerter and Its Application in Adaptive Tuned Vibration AbsorbersY Hu,MZQ Chen,S Xu,Y Liu《IEEE Transactions on Control Systems Technology》, 2016:1-77.Decentralised adaptive output feedback stabilisation for stochastic time-delay systems via LaSalle-Yoshizawa-type theoremT Jiao,S Xu,J Lu,Y Wei,Y Zou《International Journal of Control》, 2016, 89(1):69-838.Coverage control for heterogeneous mobile sensor networks on a circleC Song,L Liu,G Feng,S Xu《Automatica》, 2016, 63(3):349-358EI1.Finite-time output feedback control for a class of stochastic low-order nonlinear systemsL Liu,S Xu,YZhang《International Journal of Control》, 2016:1-162.Unified filters design for singular Markovian jump systems with time-varying delaysG Zhuang,S Xu,B Zhang,J Xia,Y Chu,...《Journal of the FranklinInstitute》, 2016, 353(15):3739-37683.Improvement on stability conditions for continuous-time T–S fuzzy systemsJ Chen,S Xu,Y Li,Z Qi,Y Chu《Journal of the Franklin Institute》, 2016, 353(10):2218-22364.Universal adaptive control of feedforward nonlinear systems with unknown input and state delaysX Jia,S Xu,Q Ma,Y Li,Y Chu《International Journal ofControl》, 2016, 89(11):1-195.H∞ Control with Transients for Singular Systems Z Feng,J Lam,S Xu,S Zhou 《Asian Journal of Control》, 2016,18(3):817-8272015年SCI1.Pinning control for cluster synchronisation of complex dynamical networks withsemi-Markovian jump topologyTH Lee,Q Ma,S Xu,HP Ju《International Journal of Control》, 2015, 88(6):1223-12352..Anti-disturbance control for nonlinear systems subject to input saturation via disturbance observer ☆Y Wei,WX Zheng,S Xu《Systems & ControlLetters》, 2015, 85:61-693.Exact tracking control of nonlinear systems with time delays and dead-zone inputZ Zhang,S Xu,B Zhang《Automatica》, 2015, 52(52):272-276EI1.Further studies on stability and stabilization conditions for discrete-time T–S systems with the order relation information of membership functionsJ Chen,S Xu,Y Li,Y Chu,Y Zou《Journal of the Franklin Institute》, 2015, 352(12):5796-5809 .2 .Stability analysis of random systems with Markovian switching and its application T Jiao,J Lu,Y Li,Y Chu,SXu《Journal of the Franklin Institute》, 2015, 353(1):200-220 3.Exact tracking control of nonlinear systems with time delays and dead-zone inputZ Zhang,S Xu,B Zhang《Automatica》, 2015, 52(52):272-2764.Event-triggered average consensus for multi-agent systems with nonlinear dynamics and switching topologyD Xie,S Xu,Y Chu,Y Zou《Journal of the Franklin Institute》, 2015, 352(3):1080-1098葛树志简介:葛树志,男,汉族,1963年9⽉20⽇⽣于⼭东省安丘县景芝的葛家彭旺村。
制造业信息化名词解释
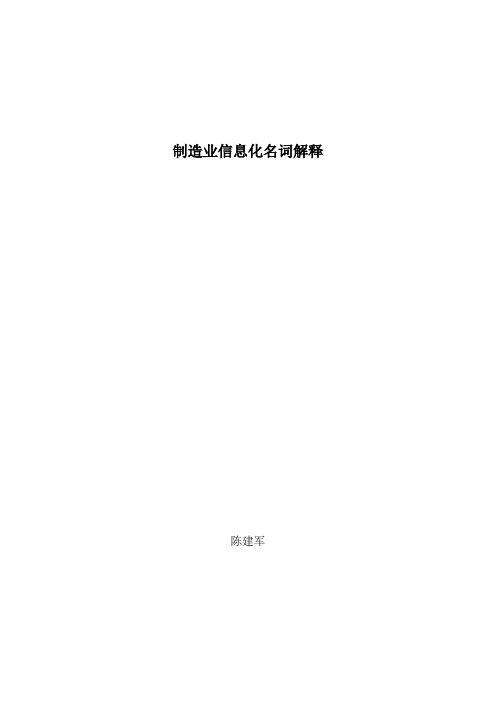
制造业信息化名词解释陈建军目次1 PLC (4)2 SFC (4)3 QQS (4)4 AM (4)5 CAD (5)6 CAE (5)7 CAM (6)8 CAPP (6)9 CE (6)10 CSCW (6)11 DCS (7)12 FMS (7)13JIT (8)14 LP (8)15 CIMS (9)16 VM (9)17 PDM (10)18 PLM (10)19 MES (11)20 BPM(BPE) (14)21 BPR (15)22 APS (15)23 OA (16)24 MIS (16)25 MRP (17)26 SCM (18)27DRP (18)28 EC (19)29 CRM (19)30 SAP (20)31 ERP (21)32 BOM (21)33 EIP (22)34 DBMS (22)35 SOA (22)36 SaaS (24)37 Gantt Chart (24)38 BI (24)39 TQCSE (25)40KPI (27)41 KRA (29)42 ASP (29)43 B/S (30)44 C/S (30)1 PLCProgrammable logic Controller可编程操纵器PLC是一种专门为在工业情形下应用而设计的数字运算操作的电子装配。
它采取能够编制法度榜样的储备器,用来在其内部储备履行逻辑运算、次序运算、计时、计数和算术运算等操作的指令,并能经由过程数字式或仿照式的输入和输出,操纵各类类型的机械或临盆过程。
PLC及其有关的外围设备都应当按易于与工业操纵体系形成一个整体,易于扩大其功能的原则而设计。
2 SFCShop Floor Control System现场主动化体系3 QQSquality and quantity system计质量治理体系4 AMAgile Manufacturing灵敏制造代表了制造业全球化信息化成长的最新时期,它重要经由过程灵敏化企业组织、并行工程情形、全球运算机收集或国度信息差不多举措措施,在全球范畴内实现企业间的动态联盟和拟实制造,使全球化临盆体系或企业群能灵敏开创出新产品,响应市场,博得竞争。
AUV浮力调节装置的设计及试验
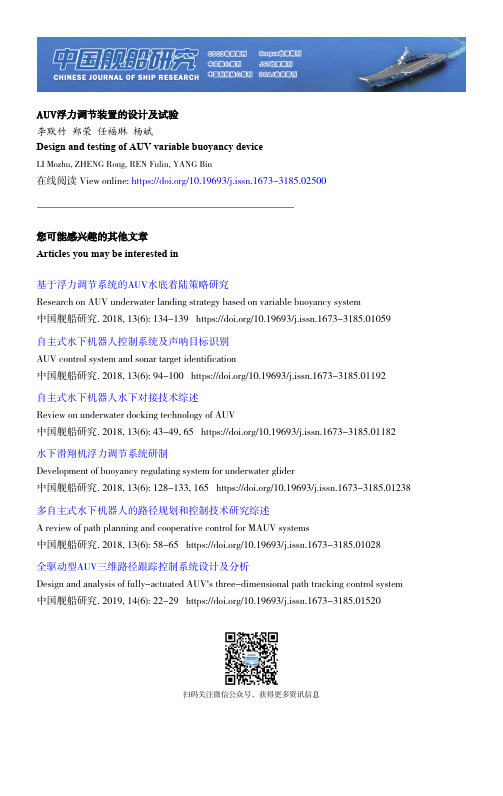
AUV浮力调节装置的设计及试验李默竹 郑荣 任福琳 杨斌Design and testing of AUV variable buoyancy deviceLI Mozhu, ZHENG Rong, REN Fulin, YANG Bin在线阅读 View online: https:///10.19693/j.issn.1673-3185.02500您可能感兴趣的其他文章Articles you may be interested in基于浮力调节系统的AUV水底着陆策略研究Research on AUV underwater landing strategy based on variable buoyancy system中国舰船研究. 2018, 13(6): 134-139 https:///10.19693/j.issn.1673-3185.01059自主式水下机器人控制系统及声呐目标识别AUV control system and sonar target identification中国舰船研究. 2018, 13(6): 94-100 https:///10.19693/j.issn.1673-3185.01192自主式水下机器人水下对接技术综述Review on underwater docking technology of AUV中国舰船研究. 2018, 13(6): 43-49, 65 https:///10.19693/j.issn.1673-3185.01182水下滑翔机浮力调节系统研制Development of buoyancy regulating system for underwater glider中国舰船研究. 2018, 13(6): 128-133, 165 https:///10.19693/j.issn.1673-3185.01238多自主式水下机器人的路径规划和控制技术研究综述A review of path planning and cooperative control for MAUV systems中国舰船研究. 2018, 13(6): 58-65 https:///10.19693/j.issn.1673-3185.01028全驱动型AUV三维路径跟踪控制系统设计及分析Design and analysis of fully-actuated AUV's three-dimensional path tracking control system中国舰船研究. 2019, 14(6): 22-29 https:///10.19693/j.issn.1673-3185.01520扫码关注微信公众号,获得更多资讯信息网络首发地址:https:///kcms/detail/42.1755.TJ.20211221.1554.003.html期刊网址:引用格式:李默竹, 郑荣, 任福琳, 等. AUV 浮力调节装置的设计及试验[J]. 中国舰船研究, 2021, 16(增刊 1): 11–18.LI M Z, ZHENG R, REN F L, et al. Design and testing of AUV variable buoyancy device[J]. Chinese Journal of Ship Re-search, 2021, 16(Supp 1): 11–18.AUV浮力调节装置的设计及试验扫码阅读全文李默竹*1,2,郑荣1,2,任福琳1,2,杨斌1,21 中国科学院沈阳自动化研究所 机器人学国家重点实验室,辽宁 沈阳 1100162 中国科学院机器人与智能制造创新研究院,辽宁 沈阳 110169摘 要:[目的]自主式水下航行器(AUV )在使用过程中受不同水域密度差异的影响,需相应计算和调整其配平状态及控制参数,而增加浮力调节装置可以使AUV 具备更好的自主调节能力,适应更多的应用场景。
Adaptive cooperative tracking control of higher-order nonlinear systems with
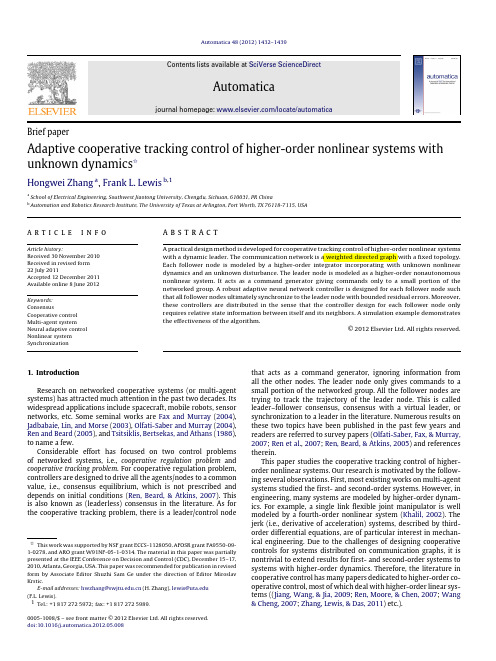
Contents lists available at SciVerse ScienceDirect
Automatica
journal homepage: /locate/automatica
Brief paper
Article history: Received 30 November 2010 Received in revised form 22 July 2011 Accepted 12 December 2011 Available online 8 June 2012 Keywords: Consensus Cooperative control Multi-agent system Neural adaptive control Nonlinear system Synchronization
✩ This work was supported by NSF grant ECCS-1128050, AFOSR grant FA9550-091-0278, and ARO grant W91NF-05-1-0314. The material in this paper was partially presented at the IEEE Conference on Decision and Control (CDC), December 15–17, 2010, Atlanta, Georgia, USA. This paper was recommended for publication in revised form by Associate Editor Shuzhi Sam Ge under the direction of Editor Miroslav Krstic. E-mail addresses: hwzhang@ (H. Zhang), lewis@ (F.L. Lewis). 1 Tel.: +1 817 272 5972; fax: +1 817 272 5989.
基于避障路径规划的无人直升机空地跟踪控制
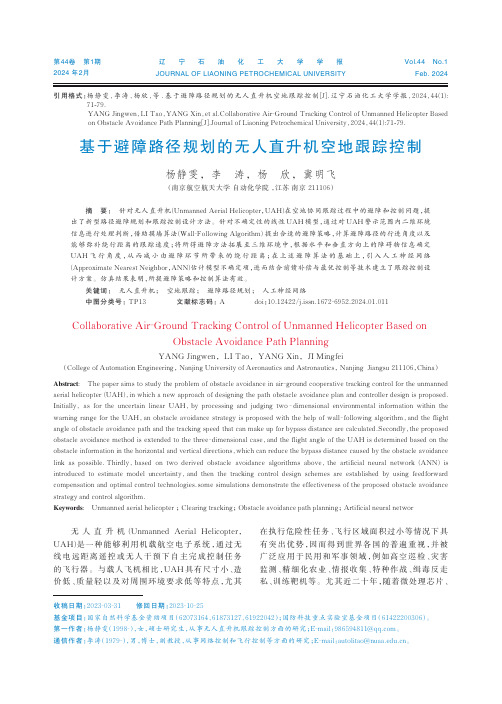
第44卷 第1期2024 年2月辽宁石油化工大学学报JOURNAL OF LIAONING PETROCHEMICAL UNIVERSITYVol.44 No.1Feb. 2024引用格式:杨静雯,李涛,杨欣,等.基于避障路径规划的无人直升机空地跟踪控制[J].辽宁石油化工大学学报,2024,44(1): 71-79.YANG Jingwen,LI Tao,YANG Xin,et al.Collaborative Air⁃Ground Tracking Control of Unmanned Helicopter Based on Obstacle Avoidance Path Planning[J].Journal of Liaoning Petrochemical University,2024,44(1):71-79.基于避障路径规划的无人直升机空地跟踪控制杨静雯,李涛,杨欣,冀明飞(南京航空航天大学自动化学院,江苏南京 211106)摘要: 针对无人直升机(Unmanned Aerial Helicopter,UAH)在空地协同跟踪过程中的避障和控制问题,提出了新型路径避障规划和跟踪控制设计方法。
针对不确定性的线性UAH模型,通过对UAH警示范围内二维环境信息进行处理判断,借助摸墙算法(Wall⁃Following Algorithm) 提出合适的避障策略,计算避障路径的行进角度以及能够弥补绕行距离的跟踪速度;将所得避障方法拓展至三维环境中,根据水平和垂直方向上的障碍物信息确定UAH飞行角度,从而减小由避障环节所带来的绕行距离;在上述避障算法的基础上,引入人工神经网络(Approximate Nearest Neighbor,ANN)估计模型不确定项,进而结合前馈补偿与最优控制等技术建立了跟踪控制设计方案。
仿真结果表明,所提避障策略和控制算法有效。
关键词: 无人直升机; 空地跟踪; 避障路径规划; 人工神经网络中图分类号: TP13 文献标志码: A doi:10.12422/j.issn.1672⁃6952.2024.01.011Collaborative Air⁃Ground Tracking Control of Unmanned Helicopter Based onObstacle Avoidance Path PlanningYANG Jingwen,LI Tao,YANG Xin,JI Mingfei(College of Automation Engineering, Nanjing University of Aeronautics and Astronautics, Nanjing Jiangsu 211106,China)Abstract: The paper aims to study the problem of obstacle avoidance in air⁃ground cooperative tracking control for the unmanned aerial helicopter (UAH),in which a new approach of designing the path obstacle avoidance plan and controller design is proposed. Initially, as for the uncertain linear UAH,by processing and judging two⁃dimensional environmental information within the warning range for the UAH,an obstacle avoidance strategy is proposed with the help of wall⁃following algorithm,and the flight angle of obstacle avoidance path and the tracking speed that can make up for bypass distance are calculated.Secondly,the proposed obstacle avoidance method is extended to the three⁃dimensional case,and the flight angle of the UAH is determined based on the obstacle information in the horizontal and vertical directions,which can reduce the bypass distance caused by the obstacle avoidance link as possible.Thirdly,based on two derived obstacle avoidance algorithms above,the artificial neural network (ANN) is introduced to estimate model uncertainty,and then the tracking control design schemes are established by using feedforward compensation and optimal control technologies.some simulations demonstrate the effectiveness of the proposed obstacle avoidance strategy and control algorithm.Keywords: Unmanned aerial helicopter ; Clearing tracking; Obstacle avoidance path planning; Artificial neural networ无人直升机(Unmanned Aerial Helicopter,UAH)是一种能够利用机载航空电子系统,通过无线电远距离遥控或无人干预下自主完成控制任务的飞行器。
供应链管理的优化:提升运营效率与客户满意度

供应链管理的优化:提升运营效率与客户满意度引言在当今竞争激烈的商业环境中,供应链管理的优化对于企业的成功至关重要。
供应链管理是一个涉及到物流、采购、生产和销售等各个环节的复杂系统,它的高效运作直接关系到企业的运营效率与客户满意度。
本文将从不同的角度探讨供应链管理的优化策略,帮助企业提升运营效率并提升客户满意度。
理解供应链管理什么是供应链管理?供应链管理是指对企业的物流和资源流动过程进行计划、组织、协调和控制的一系列管理活动。
它涵盖了从原材料采购到最终产品销售的整个流程。
供应链管理的核心目标是通过优化流程和资源配置,提高企业的运营效率和降低成本。
供应链管理的重要性一个高效的供应链管理可以为企业带来诸多优势。
首先,它能够缩短产品的交付周期,满足客户的需求,并提高客户满意度。
其次,通过优化库存管理和供应商选择,供应链管理可以帮助企业降低库存成本和采购成本。
此外,供应链管理还可以提高企业的生产效率和资源利用率,从而提高企业的竞争力。
优化供应链管理的策略精确需求预测与库存管理供应链管理的第一步是准确地预测客户需求,并合理安排产品库存。
通过使用先进的预测模型和分析工具,企业可以预测客户需求的变化趋势,从而减少产品的过剩和缺货现象。
此外,采用合理的库存管理方法,如Just-In-Time ( JIT)和Vendor-Managed Inventory( VMI ),可以帮助企业降低库存水平并提高资金利用效率。
加强供应链协作与沟通供应链管理涉及众多参与者,包括供应商、生产商和分销商等。
为了实现供应链的高效运作,这些参与者之间需要加强合作与沟通。
通过建立稳定的合作关系,并使用适当的信息技术工具,企业可以实现供应链各环节之间的实时数据共享,提高信息流畅度和业务响应速度。
自动化与数字化技术的应用在现代供应链管理中,自动化和数字化技术的应用越来越重要。
企业可以利用物联网、大数据分析和人工智能等技术来实现供应链的自动化和智能化管理。
商业综合体招商运营成功的流程和要点
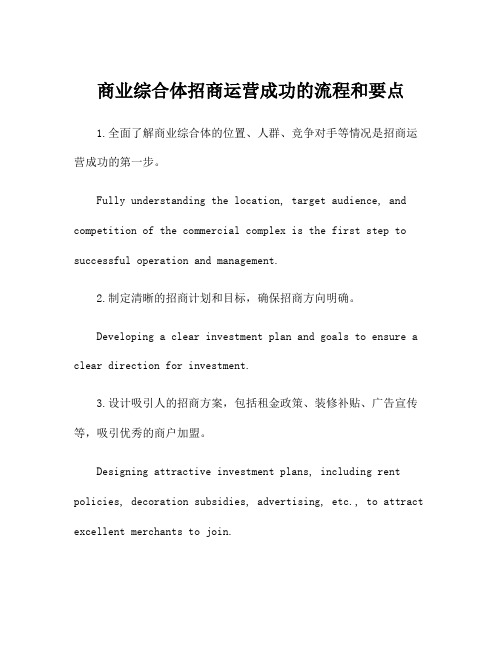
商业综合体招商运营成功的流程和要点1.全面了解商业综合体的位置、人群、竞争对手等情况是招商运营成功的第一步。
Fully understanding the location, target audience, and competition of the commercial complex is the first step to successful operation and management.2.制定清晰的招商计划和目标,确保招商方向明确。
Developing a clear investment plan and goals to ensure a clear direction for investment.3.设计吸引人的招商方案,包括租金政策、装修补贴、广告宣传等,吸引优秀的商户加盟。
Designing attractive investment plans, including rent policies, decoration subsidies, advertising, etc., to attract excellent merchants to join.4.与各行业的知名品牌进行洽谈合作,吸引知名品牌商家入驻商业综合体。
Negotiating with well-known brands in various industries to attract them to settle in the commercial complex.5.确保商业综合体内的消费氛围和商户结构与项目定位相匹配。
Ensuring that the consumer atmosphere and merchant structure within the commercial complex match the project positioning.6.进行市场调研,了解潜在商户的需求和市场脉搏,为他们提供有针对性的合作方案。
壁面爬行机器人研究与发展
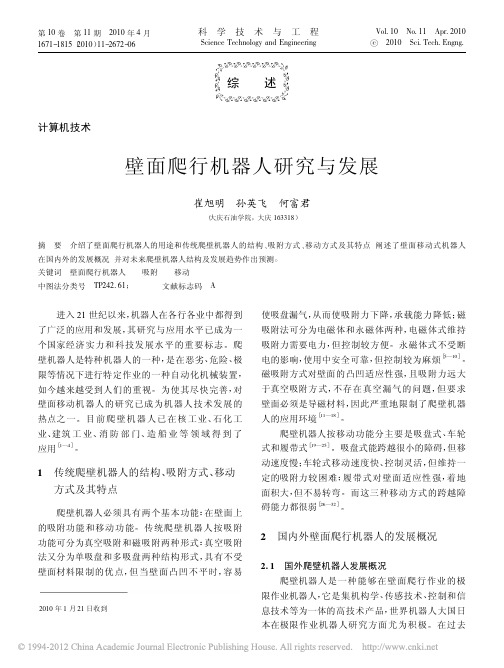
随着机器人技术的出现和发展以及自我保护 意识的增强,人们迫切希望能用机器人代替人工进 行高空作业。爬壁机器人的使用可以大大降低高 层建筑的清洗成本和工业中高空高危设施的维护
11 期
崔旭明,等:壁面爬行机器人研究与发展
2010 年 1 月 21 日收到
使吸盘漏气,从而使吸附力下降,承载能力降低;磁 吸附法可分为电磁体和永磁体两种,电磁体式维持 吸附力需要电力,但控制较方便。永磁体式不受断 电的影响,使用中安全可靠,但控制较为麻烦[5—10]。 磁吸附方式对壁面的凸凹适应性强,且吸附力远大 于真空吸附 方 式,不 存 在 真 空 漏 气 的 问 题,但 要 求 壁面必须是导磁材料,因此严重地限制了爬壁机器 人的应用环境[11—18]。
在移动机器人中,轮式和履带式移动方式已获 得广泛的应用,但是足式移动方式具有轮式和履带 式所没有的优点,足式移动方式的机器人可以相对 较容易地跨过比较大的障碍,并且机器人的足所具 有的大量的自由度可以使机器人的运动更加灵活, 对凸凹不平的地形适应能力更强,足式机器人的立 足点是离散 的,跟 壁 面 接 触 的 面 积 小,可 以 在 可 达 到的范围内选择最优支撑点,即使在表面极度不规 则的情况下,通过严格选择足的支撑点,也能够行走 自如。正是由于足式结构多样、运动灵活,适应于各 种形状的壁面上[74—76],而且能够跨越障碍物,因此足 式结构将在爬壁机器人上有着较好的应用前景。 3. 3 驱动设备
早在 1966 年,在本大阪府立大学工学部任讲师 的西亮,就利用电风扇进气侧低压空气产生的负压 作为吸附力制作了一台垂直壁面移动机器人的原 理样机,这被 看 作 是 爬 壁 机 器 人 研 究 的 开 端[46—48]。 日本应用技术研究所研制出了车轮式磁吸附爬壁 机器人,它 可 以 吸 附 在 各 种 大 型 构 造 物 如 油 罐、球 形煤气罐、船 舶 等 的 壁 面,代 替 人 进 行 检 查 或 修 理 等作业。这种爬壁机器人靠磁性车轮对壁面产生 吸附力,其主要特征是:行走稳定速度快,最大速度 可达 9 m / min,适用于各种形状的壁面,且不损坏壁 面的油漆。1989 年日本东京工业大学的宏油茂男 研究开发了吸盘式磁吸附爬壁机器人,吸盘与壁面 之间有一个很小的倾斜角度,这样吸盘对壁面的吸 力仍然很 大,每 个 吸 盘 分 别 由 一 个 电 动 机 来 驱 动, 与壁面线接触的吸盘旋转,爬壁机器人就随着向前 移动,这种吸附机构的吸附力可以达到很大[49—53]。
机载雷达目标跟踪与航迹信息提取
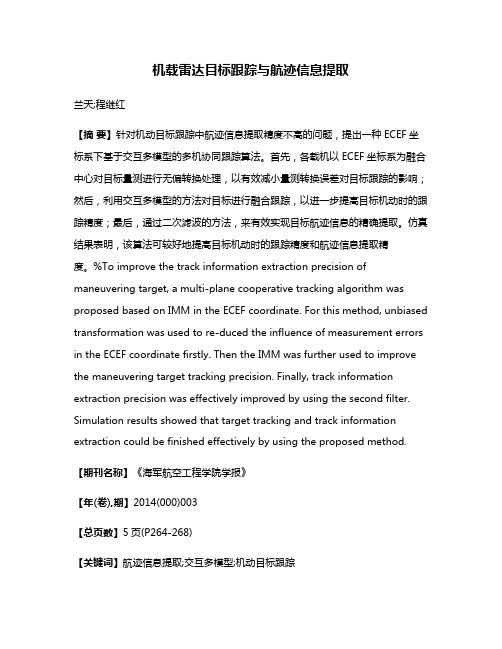
机载雷达目标跟踪与航迹信息提取兰天;程继红【摘要】针对机动目标跟踪中航迹信息提取精度不高的问题,提出一种ECEF坐标系下基于交互多模型的多机协同跟踪算法。
首先,各载机以ECEF坐标系为融合中心对目标量测进行无偏转换处理,以有效减小量测转换误差对目标跟踪的影响;然后,利用交互多模型的方法对目标进行融合跟踪,以进一步提高目标机动时的跟踪精度;最后,通过二次滤波的方法,来有效实现目标航迹信息的精确提取。
仿真结果表明,该算法可较好地提高目标机动时的跟踪精度和航迹信息提取精度。
%To improve the track information extraction precision of maneuvering target, a multi-plane cooperative tracking algorithm was proposed based on IMM in the ECEF coordinate. For this method, unbiased transformation was used to re-duced the influence of measurement errors in the ECEF coordinate firstly. Then the IMM was further used to improve the maneuvering target tracking precision. Finally, track information extraction precision was effectively improved by using the second filter. Simulation results showed that target tracking and track information extraction could be finished effectively by using the proposed method.【期刊名称】《海军航空工程学院学报》【年(卷),期】2014(000)003【总页数】5页(P264-268)【关键词】航迹信息提取;交互多模型;机动目标跟踪【作者】兰天;程继红【作者单位】海军装备部西安军事代表局,太原030006;海军航空工程学院科研部,山东烟台264001【正文语种】中文【中图分类】TN958.93机载雷达航迹信息提取是当前信息化综合作战、传感器组网作战和多平台一体化联合作战的重要研究课题[1]。
针对机动目标跟踪的雷达发射波形选择

和信噪比等方面决定。因此要提高系统的整体跟踪 性能,一方面需要选择良好的跟踪算法以及与目标 实际运动状态尽可能接近的状态模型,另一方面需 要选择合理的发射波形和参数估计方法。这两方面 相辅相成,缺一不可。跟踪算法方面,交互多模型 (Interacting Multiple Model, IMM)算法因为其在运 算量和跟踪性能等方面的优势,得到了广泛的研究 与应用 [1−4] ,是目前机动目标跟踪领域的主流算法。 本文在IMM算法的基础上,重点讨论针对机动目标 跟踪的发射波形选择问题。 近年来,针对目标跟踪的发射波形选择得到了
1
引言
众所周知,影响雷达系统跟踪性能的因素主要 有两个方面:一是对目标运动状态的预测精度,这 主要由跟踪算法及其利用的状态模型决定;二是对 目标运动状态的观测精度,这主要由雷达发射波形
2013-11-07 收到,2014-01-17 改回 国家自然科学基金(61271291, 61201285),新世纪优秀人才支持计划 (NCET-09-0630) , 陕 西 省 自 然 科 学 基 础 研 究 计 划 项 目 (2012JM 8015),陕西省教育厅专项计划项目(12JK0530, 12JK0557)和中国博 士后科学基金(2013M542329)资助课题 *通信作者:靳标 jinpuresky@
第 36 卷第 8 期 2014 年 8 月
电 子 与 信 息 学 报 Journal of Electronics & Information Technology
Vol.36No.8 Aug. 2014
针对机动目标跟踪的雷达发射波形选择
靳
①
标
*①
纠
②
博
①
苏
涛
①
浅析无人机在武警部队中的应用

浅析无人机在武警部队中的应用曾强凯梁志贤周进军(武警警官学院学员7大队19队四川·成都610213)摘要在未来复杂战场环境中,随着无人机技术的快速发展,多无人机协同对多目标点进行侦查和实施打击是未来战争的必然选择。
本文介绍了无人机产生的背景及其在军事领域的发展历程,重点分析了无人机在武警部队应用中可能出现的问题,总结出可使用群智能优化算法对无人机进行任务分配和路径规划的解决方法。
关键词无人机群智能任务分配路径规划1无人机在武警部队的应用背景人民武装警察部队担负国家赋予的安全保卫任务以及防卫作战、抢险救灾、参加国家经济建设等任务。
这些任务的共同特点是具有突发性、事件涉及区域较广、人员数量较多、不确定因数较多、危险系数较大,而事发区域兵力分散较广,不能第一时间掌握现场情况,有效控制现场局面,也就给我方指挥人员在短时间内根据现地地形制定出一套确实可行的实施方案增加了非常大的难度。
无人机以其机动性好、连续性强、零伤亡、隐身性能好、高超速过载、寿命费用相对较低等诸多特点,可以在复杂、恶劣、狭小的环境中作业,并在近几年的局部战争中表现优异,得到一致好评。
通过多无人机协同编队对事件发生区域的先期侦查,可以迅速判断事件的性质和初步情况,然后将情况反馈给现场指挥员,指挥员根据收集到的信息可以更加快速准确的制定出可行方案并付诸实施,有效控制了人员伤亡率、大大提高了事件处置的效率。
对未来部队在更加多元化、复杂化的战场环境中处置任务具有十分重要的意义。
2无人机在军用方面的发展历程无人机最早启用于20世纪40年代,二战中将无人靶机用于训练防空炮手,成为近代无人机使用的趋势的先河;随着电子技术的进步,无人机在担任侦察任务的角色上开始展露它的弹性和重要性,20世纪55年代到74年的越南战争,海湾战争乃至北约空袭南斯拉夫的过程中,无人机都被频繁的用于执行军事任务;1982年以色列IAI航空工业公司首创以无人机担任其他角色的军事任务;在黎巴嫩战争时期,侦察者无人机曾在以色列陆军和以色列空军的服役中担任重要战斗角色;以色列国防军主要用无人机进行侦查,情报收集,跟踪和通讯;20世纪90年代,海湾战争后,无人机开始飞速发展和广泛运用。
航空专业术语缩写英汉
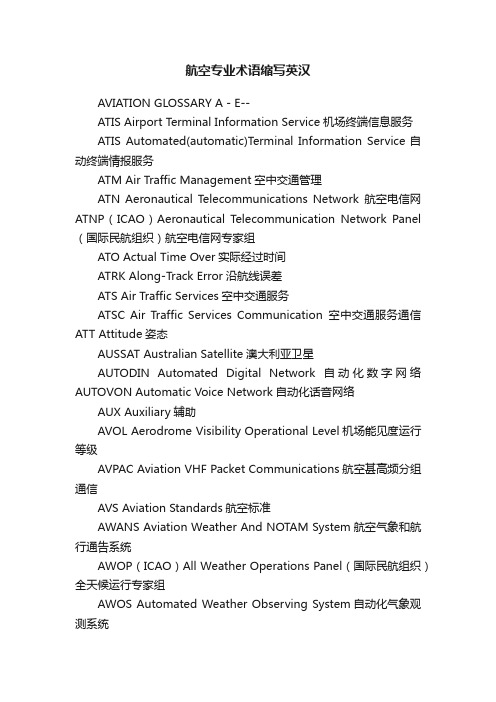
航空专业术语缩写英汉AVIATION GLOSSARY A-E--ATIS Airport Terminal Information Service机场终端信息服务ATIS Automated(automatic)Terminal Information Service自动终端情报服务ATM Air Traffic Management空中交通管理ATN Aeronautical Telecommunications Network航空电信网ATNP(ICAO)Aeronautical Telecommunication Network Panel (国际民航组织)航空电信网专家组ATO Actual Time Over实际经过时间ATRK Along-Track Error沿航线误差ATS Air Traffic Services空中交通服务ATSC Air Traffic Services Communication空中交通服务通信ATT Attitude姿态AUSSAT Australian Satellite澳大利亚卫星AUTODIN Automated Digital Network自动化数字网络AUTOVON Automatic Voice Network自动化话音网络AUX Auxiliary辅助AVOL Aerodrome Visibility Operational Level机场能见度运行等级AVPAC Aviation VHF Packet Communications航空甚高频分组通信AVS Aviation Standards航空标准AWANS Aviation Weather And NOTAM System航空气象和航行通告系统AWOP(ICAO)All Weather Operations Panel(国际民航组织)全天候运行专家组AWOS Automated Weather Observing System自动化气象观测系统AWP Aviation Weather Processor航空气象处理器AWS Aviation Weather Service航空气象服务AZ Azimuth transmitter方位台BBARO Barometric气压BAZ Back Azimuth后方位,背航道BER Basic Encoding Rules基本编码规则BER Bit Error Rate误码率BIT Built-In-Test机内测试BITE Built-In-Test Equipment机内测试设备BOP Bit Oriented Protocol面向位的协议BPS bits per second每秒传送位数;每秒比特数BPSK Biphase Shift Keying两相相移键控BRITE Bright Radar Indicator Tower Equipment塔台高亮度雷达显示设备BRL Bearing Range Line方位距离线BSU Beam Steering Unit天线方位控制组件BUEC Backup Emergency Communications备用紧急通信C通信C-Band Approx.5,000MHz C波段C/A(CA)Code Course Acquisition Code粗获码(民用的)C/I Carrier-to-Interference Ratio信号干扰比C/N Carrier-to-Noise Ratio信噪比CA Conflict Alert冲突告警CA GPS Course-Acquisition Code粗捕获码(民用码)CA/MSAW Conflict Alert/Minimum Safe Altitude Warning冲突告警/最低安全高度警告CAA Civil Aviation Administration,Civil Aeronautical Authority,Civil Aviation Authority民航局CAAC General Administration of Civil Aviation of China中国民用航空总局CAASD Center for Advanced Aviation System Development(The MITRE Corporation)(MITRE公司)高级航行系统开发中心CAB Civil Aeronautical Bureau民航局CARF Central Altitude Reservation Function中央飞行高度保留功能CARs Civil Air Regulations民用航空规则CASITAF CNS/ATM implementation task force新航行系统实施特别工作组CAT Category仪表着陆等级CATⅠCategoryⅠ一类仪表着陆CATⅡCategoryⅡ二类仪表着陆CATⅢa CategoryⅢa三类a级仪表着陆CATⅢb CategoryⅢb三类b级仪表着陆CATⅢc CategoryⅢc三类c级仪表着陆CATC Civil Aviation Training Center民航培训中心CATMAC Co-operative Air Traffic Management Concept空中交通管理合作方案CBA Cost/Benefit Analysis成本效益分析C-BAND The frequency range between4000and8000MHz4000到8000MHz频段CBI Computer Based Instruction计算机基本指令CBT Computer-Based Training计算机辅助训练CC Connection Confirm联接确认CCA Continental Control Area大陆管制区CCC蜂窝式CNS概念CCD Consolidated Cab Display综合机舱显示器CCIR International Radio Consultative Committee国际无线电咨询委员会CCITT International Telegraph and Telephone ConsultativeCommittee国际电报电话咨询委员会CCP Contingency Command Post应急指挥站CCWS Common controller workstation通用管制员工作站CD Common Digitizer通用数字化仪设备CDC Computer Display Channel计算机显示通道CDI Course Deviation Indicator偏航指示器CDM Code division multiplex码分复用CDM Continuous Delta Modulation连续增量调制CDMA Code Division Multiple Access码分多址CDT Controlled Departure Times管制离场时间CDTI Cockpit Display of Traffic Information驾驶舱交通信息显示CDU Control Display Unit控制显示组件CEP Circular error probability圆概率误差CERAC Combined Center Radar Approach Control雷达进近管制联合中心CFCC Central Flow Control Computer中央流量管制计算机CFCF Central Flow Control Facility中央流量管制设施(功能) CFDPS Compact Flight Data Processing System小型飞行数据处理系统CFWP Central Flow Weather Processor中央流量气象处理机CFWSU Central Flow Weather Service Unit中央流量气象服务单元(组件)CHI Computer Human Interface机人接口CIDIN Common ICAO Data Interchange Network国际民航组织公用数据交换网CIS Cooperative independent surveillance合作式独立监视CLAM Cleared Level Adherence Monitoring放行高度保持监视CLB Climb爬升CLK Clock时钟CLNP Connectionless Network Protocol无连接网络规程(协议)CLR Clear清除CMC Central Maintenance Computer中央维护计算机CMD Command命令CMS Cabin Management System机舱管理系统CMU Communications Management Unit通信管理单元CNDB Customized Navigation Database用户导航数据库CNS Consolidated NOTAM System综合航行通告系统CNS/ATM Communication Navigation,Surveillance/AirTraffic Management通信导航监视/空中交通管理CODEC Coder/Decoder编码器/解码器COM/MET/OPS Communication/Meteorology/Operations通信/气象/运行COMLO Compass Locator罗盘定位器;罗盘示位信标COMM Communication通信COMP Compressor压缩器COMSEC Communications Security通信保安CON Continuous连续CONUS Continental,Contiguous,or Conterminous United States美国大陆本部(四十八州)COP Change Over Point转换点COP Character Oriented Protocol面向字符协议COTS Commercial Off-the-Shelf商业货架产品供应CPDLC Controller Pilot Data Link Communications管制员驾驶员数据链通信CPFSK Continuous Phase Frequency Shift Keying连续相位频移键控CR Connection Request联接申请CRA Conflict Resolution Advisory冲突解脱咨询CRC Cyclic Redundant Check循环冗余校验CRCO Central Route Charges Office中央航路收征费办公室CRM C Reference Model C参考模式CRM Collision Risk Modeling碰撞危险模型CRM Crew Resource Management机组人员安排CRT Cathode Ray Tube阴极射线管CRZ Cruise巡航CSA Standard Accurate Channel标准精度通道CSE Course Setting Error航线设定误差CSMA Carrier Sense Multiple Access(datalink protocol)载波侦听多址访问C/SOIT Communication/Surveillance Operational Implementation Team通信监视运行实施小组(美国)CTA Calculated Time of Arrival计算到达时间CTA Control Area管制区CTAS Central Tracon Automation System中央终端雷达进近管制自动系统CTL Control控制CTMO Central traffic Management Organization中央交通流量管理组织CTMO Centralized Traffic Management Organization中央交通管理组织CTOL Conventional Take Off and Landing常规起飞着陆CTR Control zone管制地带CTS Control Tracking Station控制跟踪站CU Control Unit控制单元C§W Control and Warning控制和告警CW Carrier Wave载波CWI Continuous Wave Interference连续波干扰CWP Central Weather Processor中央气象处理器CWSU Center Weather Service Unit中央气象服务单元DD/A Digital-to-Analog数/模转换DABS Discrete Addressable Beacon System离散寻址信标系统DADC Digital Air Data Computer数字大气数据计算机D-ATIS Digital Automatic Terminal Information Service数字自动终端信息服务DA Decision Addressing beacon system决断寻址信标系统DA Demand Assignment按需分配DA/H Decision Altitude(Height)决断高度DARC Direct Access Radar Channel直接存取雷达信道DARP Dynamic Air Route Planning动态航线计划DARPS Dynamic Aircraft(Air)Route Planning Study动态飞机航线计划研究DC Departure Clearance离场放行许可DC Direct Current直流(电)DCC Display Channel Complex显示通道组合DCIU Data Control Interface Unit数据控制接口单元DCL Departure Clearance Delivery起飞许可传送DCPC Direct Controller Pilot Communication管制员驾驶员直接通信DES Data Encryption Standard数据加密标准DF Direction Finder测向器DFCS Digital Flight Control System数字飞行控制系统DFDAU Digital Flight Data Acquisition Unit数字飞行数据采集单元DGCA Director-General Civil Aviation民航局长DGNSS Differential Global Navigation Satellite System差分全球导航卫星系统DGPS Differential Global Positioning System差分全球定位系统DH Decision Height决断高度DIP Diplexer双工器DL Data Link数据链DLAC Data Link Applications Coding数据链应用编码DLAS Differential GNSS Instrument Approach System差分GNSS仪表进近系统DLK data link数据链DLORT FAA Data Link Operational Requirements Team FAA 数据链运行要求工作组DMAP ICAO Data Link Mobile Applications Panel(proposed) 国际民航组织数据链移动应用专家组(建议)DME Distance Measuring Equipment测距设备DME/N Distance Measuring Equipment/Normal标准测距设备DME/P Distance Measuring Equipment/Precision精密测距设备DMU Data Management Unit数据管理单元DO(DOC)Document记录(文件)DOD Department of Defense(美国)国防部DOP Dilution of Precision精度扩散因子DOT Department of Transportation(美国)运输部DOTS Dynamic Ocean Tracking System动态海洋跟踪系统DP Disconnect Request分离拆线请求DPF Data Processing Function数据处理功能D8PSK Differential Eight-Phase Shift Keying差分8相移键控DPSK Differential Phase Shift Keying差分相移键控DRMS Distance Root Mean Square距离均方根值DRN Document Release Notice文件发放通告DSB-AM Double Sideband Amplitude双边带调幅DSDU Data Signal Display Unit数据信号显示单元DSP Departure Sequencing Program起飞排序计划;离港排序计划DT Data数据DTE Data Terminal Equipment数据终端设备DT&E Development Test and Evaluation开发测试和评估DTF Data Test Facility数据检测设备DTG待飞距离DTN Data Transport Network数据传输网络DUAT Direct User Access Terminal用户直接存取终端DVOR Doppler Very high frequency Omni-directional Range 多普勒甚高频全向信标EEANPG European Air Navigation Planning Group欧洲航行规划小组E-DARC Enhanced Direct Access Radar Channel增强的直接存取雷达信道EARTS En route Automated Radar Tracking System航路自动化雷达跟踪系统EASIE Enhanced ATM and Mode S Implementation in Europe 欧洲S模式和增强的空中交通管理实施项目EATCHIP European ATC Harmonization Implementation Program 欧洲空中交通管制协调实施计划EATMS European Air Traffic Management System欧洲空中交通管理系统ECAC European Civil Aviation Conference欧洲民航会议ECEF 地心地固坐标EDCT Estimated Departure Clearance Time预计离港起飞放行时间EET Estimated Elapsed Time预计经过时间EFAS En route Flight Advisory Service航路飞行咨询服务EFAS Extended Final Approach Segment扩展最后进近段EFIS Electronic Flight Instrument System电子飞行仪表系统EFC Expect Further Clearance预期进一步放行许可EFIS Electronic Flight Information System电子飞行情报系统EGNOS European global navigation overlay system欧洲全球导航重迭系统EHSI Electronic Horizontal Situation Indicator电子平面状态显示器EIRP Equivalent Isotropic Radiate Power等效各向同性辐射功率EISA Extended Industry Standard Architecture扩展的工业标准结构EL Elevation Transmitter仰角台ELOD En route sector Load航路扇区负载管制飞机数量ELT Emergency Locator Transmitter紧急示位发射机EMC Electromagnetic Compatibility电磁兼容EMI Electromagnetic Interference电磁干扰ENRI Electronic Navigation Research Institute(日本)电子导航研究所EOF Emergency Operations Facility应急运行设施EPA Environmental Protection Agency环境保护署ER Error误差ERL Environmental Research Laboratories环境研究实验室ERM En Route Metering航路计量管制ERN Earth Referenced Navigation大地参考导航ERP Effective Radiated Power有效幅射功率ES End System终端系统ESA European Space Agency欧洲航天局ESCAN Electronic Scanning(radar antenna)ESMMC Enhanced SMMC增强的系统维护监视台ESP En route Spacing Program航路间隔计划EST Estimated message预计信息ETA Estimated Time of Arrival预计到达时间ETB Estimated Time of Boundary预计边界时间ETD Estimated Time of Departure预计离港时间ETG Enhanced Target Generator增强的显示目标产生器ETN Estimated Time of Entry预计进入时间ETO Estimated Time Over预计飞越时间ETSI European Telecommunications Standards Institute欧洲电信标准学会EU European Union欧洲联盟EURATN European ATN欧洲航空电信网EUROCAE European Organization for Civil Aviation Electronics欧洲民用航空电子学组织EUROCONTROL European Organization for the Safety of Air Navigation欧洲航行安全组织(欧安局)EVS Enhanced Vision System增强视景系统EWAS En-route Weather Advisory Service航路气象咨询服务FF&E Facilities and Equipment设施和设备F,E&D Facilities,Engineering,and Development设施、工程和开发FAA Federal Aviation Administration(美国)联邦航空局FAATC FAA Technical Center(美国)联邦航空局技术中心FAF Final Approach Fix最终进近坐标FANS ICAO Future Air Navigation Systems(国际民航组织)未来航行系统FANS Special Committee on Future Air Navigation Systems 未来航行系统特别委员会FANS(Phase II)Special Committee for the Monitor-ing and Co-ordination of Develop-ment and Transition Planningfor the Future Air Navigation System未来航行系统监督、协调发展与过渡规划专门委员会FAR Federal Aviation Regulation联邦航空条例FAS Final Approach Segment最后进近段FASID Facilities And Services Implementation Document设施和服务实施文件FCC Flight Communication Center飞行通信中心FCC Federal Communication Commission联邦通信委员会FCC Flight Control Computer飞行控制计算机FDAU Flight Data Acquisition Unit飞行数据收集单元FDDI Fiber Distributed Data Interface光纤分布数据接口FDEP Flight Data Entry and Printout飞行数据输入和输出FDI Fault Detection and Isolation故障检测和隔离FDIO Flight Data Input/Output飞行数据输入/输出FDM Frequency Division Multiplex频分复用FDMA Frequency Division Multiple Access频分多址FDP Flight Data Processor飞行数据处理器FDPS Flight Data Processing System飞行数据处理系统FDR Flight Data Recorder飞行数据记录仪FEATS Future European ATS System Concept未来欧洲空中交通服务系统方案FEATS ICAO Future European Air Traffic Management System 国际民航组织未来欧洲空中交通管理系统FEC Forward Error Correction前向纠错FGC Flight Guidance Computer飞行引导计算机FGCC Federal Geodetic Control Committee联邦大地测量管理委员会FI Flight Inspection飞机校验FIC Flight Information Center飞行信息中心FIFO First In-First Out先入先出FIFO Flight Inspection Field Office飞行检查现场办事处FIR Flight Information Region飞行情报区FIS Flight Information Services飞行情报服务FISA Automatic Flight Information Service自动飞行信息服务FL Flight Level飞行高度层FLIR Forward Looking Infra-red Detection前视红外线探测FM Frequency Modulation调频FMC Flight Management Computer飞行管理计算机FMEA Failure Mode Effects Analysis故障模式效果分析FMS Flight Management System飞行管理系统FMS Frequency Management System频率管理系统FMSG Frequency Management Study Group频率管理研究组FMU Flight Management Unit飞行管理组件FMU Flow Management Unit流量管理单元FOC Full Operation Capability全运行能力FOM Figure of Merit性能指数FPA Flight Path Angle航迹倾角FPD Flight Plan Data飞行计划数据FPS Military Primary Radar军用一次雷达FREQ Frequency频率FRP Federal Radio navigation Plan联邦无线电导航计划(美国)FS Functional Statement功能描述FSAS Flight Service Automation System飞行服务自动化系统FSDPS Flight Service Data Processing System飞行服务数据处理系统FSK Frequency Shift Keying频移键控FSP Flight Strip Printer飞行进程单打印机FSS Flight Service Station飞行服务站FSTN Federal Security Telephone Network联邦政府保安电话网络FT Functional Test功能测试FTE Flight Technical Error飞行技术误差FY Fiscal Year财政年度;会计年度GGA General Aviation通用航空GA Ground annta地面天线Gatelink Datalink for packed aircraft网关数据链路GADS Generic Aircraft Display System通用航空器显示系统GAIT Ground-based Augmentation and Integrity Technique陆基增强和完好性技术GAO Government Accounting Office(联邦)政府会计署GBA Geostationary broadcast area静止卫星广播区域GCAS Ground Collision Avoidance System地面防撞系统GCS Ground Controlled Approach地面控制系统GDLP Ground Data Link Processor地面数据链处理器GDOP Geometic Dilution of Position位置几何扩散因子GDOP Geometry Dilution of Precision精度几何扩散因子GEO Geostationary静地的GEO Geostationary Earth Orbit相对地球静止轨道静止卫星GES Ground Earth Station地面地球站GFE Government-Furnished Equipment政府提供的设备GHz Giga hertz千兆赫兹GIB GNSS integrity broadcast全球导航卫星系统完好性数据广播GIC GNSS Integrity Channel全球卫星导航系统完好性通道GICB Ground-initiated Comm-B地面启动的B类通信GIRU Ground Interrogator Receiver Unit地面应答机接收单元GIS Geographical Information System地理信息系统GLONASS Global Orbit Navigation Satellite System全球轨道导航卫星系统(俄罗斯)GLS GPS Landing System GPS着陆系统GM Guidance Material指导材料GMC Ground Movement Control地面活动管制GMSK Gaussian Minimum Shift KeyingGMT Greenwich Mean Time格林威治时间GNAS General NAS综合国家空域系统GND Ground地GNE Gross Navigational Error总导航误差GNR Global Navigation Receiver全球导航接收机GNSS Global Navigation Satellite System全球导航卫星系统GNSSP ICAO Global Navigation Satellite Systems Panel国际民航组织全球卫星导航系统专家组GPSSU Global Positioning System Sensor Unit全球定位系统(GPS)传感器组件GOES Geostationary Operational Environmental Satellite静地运行环境卫星GOS Grade of Service服务等级GOSEP Government Open Systems Interconnection Profile政府开放系统互联结构GOSIP Government Open systems Implementation Profile政府开放系统实施结构GP Glide-Path下滑道GPIP Glide-Path Intercept Point下滑道截获点GPIWP Glide Path Intercept Waypoint滑行道切入点GPO/GPI General Purpose Output/General Purpose Input通用输出/通用输入GPS Global Positioning System全球定位系统GPWS Ground Proximity Warming System近地告警系统GREPECAS Caribean/South American Planning and Implementation Regional Group加勒比/南美洲计划和实施区域小组GRS Ground-Reference Station地面基准站GRS80Geodetic-Reference System-80大地基准系统-80GS(G/S)Glide Slope下滑坡度GS Ground Speed地速GSA General Services Administration综合服务管理局(联邦政府下属)GSL General Support Laboratory综合保障实验室GSM Global System(or Mobile)Communication全球通信系统GWS Graphic Weather Service图形气象服务HH Homing radio beacon归航无线电信标HARN High Accuracy Reference Network高精度参考网HAT Height Above Touchdown高于接地点的高度HCI Human Computer Interface人机接口HDD Head Down Display下视显示器HDG Heading航向HDOP Horizontal Dilution Of Precision精度水平扩散因子HEMP High Altitude Electromagnetic Pulse高空电磁脉冲HEO High Elliptical Orbit高椭圆率轨道HF High Frequency(3-30MHz)高频HFDL High Frequency Data Link高频数据链HGA High Gain Antenna高增益天线HIRF High Intensity Radiated Fields高强度辐射场HIWAS Hazardous In-flight Weather Advisory Service飞行时遇危险天气的咨询服务HMI Human Machine Interface人机接口HPA high power amplifier高功率放大器HPF Horizontal Position Fix Error水平位置坐标误差HSI Horizontal Situation Indicator水平位置指示器HUD Head-up Display平视显示仪HUI Head up DisplayHVAC Heating,Ventilating,And air Conditioning加热,通风和空调Hybird GNSS/ILS Precision Approach/Landing based on combination of GNSS localizer and ILS glide path基于GNSS 航向和ILS下滑道组合的精密进近/着陆系统Hz Hertz赫兹IIA5International Alopabet5国际字母表第5号码IACA International Air Carrier Association国际航空公司协会IACSP International Aeronautical Communication Service Provider国际航空通信业务提供者IAF Initial Approach Fix初始进近点(坐标)IAG International Association of Geodetical国际测地协会IAIN International Association of Institutes of Navigation国际导航学会联合会IAOPA International Council of Aircraft Owner and Pilot Associations航空器企业主和驾驶员协会国际委员会IAP Instrument Approach Procedure仪表进近程序IAR Intersection of Air Routes航路交叉点IAS Indicated Air Speed指示空速IASC Inter Area Speech Circuit区域间话音线路IATA International Air Transport Association国际航空运输协会IBAC International Business Aviation Council国际商业航空委员会ICAO International Civil Aviation Organization国际民航组织ICCAI(A)International Co-ordination Council of Aerospace Industries Associations国际宇航工业联合会合作委员会ICD Interface Control Document接口控制文件ICO Interim Circle Orbit中高度圆轨道ICSS Integrated Communications Switching System综合通信转换系统ID Identifier(Identification)标识码(编码、识别标志)ID Instrument Departure仪表离场IDSG ICAO Internet Working Standards Drafting Group国际民航组织网间标准起草小组IEEE Institute of Electrical and Electronic Engineers电气和电子工程师学会IF Intermediate approach Fix中间进近定位点IFALPA International Federation of Airline Pilots Associations 航空公司驾驶员协会国际联合会IFATCA International Federation of Air TrafficControllers'Associations空中交通管制员协会国际联合会IFCN Interfacility Flow Control Network设施(单位)间流量管制网络IFF敌我识别器IFM Integrated Flow Management综合流量管理IFR Instrument Flight Rules仪表飞行规则IFRB International Frequency Registration Board国际频率注册委员会IFSS International Flight Service Station国际飞行服务站IBM International Business Machines(美国)国际商用机器公司ILA International Law Association国际法律协会ILS Instrument Landing System仪表着陆系统IMA Integrated Modular Avionics集成化模块式航空电子设备IMAWP Initial Missed Approach Waypoint起始复飞航路点IMC Instrument Meteorological Conditions仪表气象条件IMCS Interim MCS过渡性监控/管制软件IMO International Maritime Organization国际海事组织IMS Integrity Monitoring System完好性监视系统IN Information Need信息需求INMARSAT International Marine Satellite Organization国际移动卫星组织(原名国际海事卫星组织)INS Inertial Navigation System惯性导航系统INS Insert插入INTNET Integrated Data Communications Network集成化数据通信网络I/O input/output输入/输出IOACG Informal Indian Ocean Air Traffic Services Coordinating Group非正式印度洋空中交通服务协调小组IOC Initial Operational Capability初始运行能力IOD GPS Issue of Data全球定位系统数据发布ION Institute of Navigation导航学会IOR Indian Ocean Region印度洋区域IOT§E Initial Operational Test and Evaluation初始运行测试和评估IP Internetwork Protocol网络间协议IPACG Informal Pacific Air Traffic Control Coordination Group 非正式太平洋空中交通管制协调小组IRS Inertial Reference System惯性参考系统ISA International Standard Atmosphere国际标准大气ISDN Integrated Service Digital Network综合业务数字网络ISNS国际卫星导航服务ISO International Organization for Standardization国际标准化组织ISPACG Informal South Pacific ATS Co-Ordination Group非正式南太平洋空中交通服务协调小组ISSS Initial Sector Suite Subsystem起始扇区管制席位分系统ITU International Telecommunication Union国际电信联盟ITWS Integrated T erminal Weather Service综合终端气象服务IVAD Integrate Voice and Data综合话音和数据(通信数据链)IVRS Interim Voice Response System过渡性话音响应系统IWP Interim Working Party临时工作组JAWS Joint Airport Weather Studies联合机场气象研究JCAB Japan Civil Aviation Bureau日本民航局JAWS Joint Airport Weather Studies联合机场气象研究JPO Joint GPS Planning Office联合GPS规划办公室JSS Joint Surveillance System联合监视系统Kbps Kilo bits per second千位每秒KDP Key Decision Point关键性决定点kHz Kilohertz千赫KLAAS Kinematics Local Area Augmentation System动态地面局域增强系统KLADGNSS Kinematics Local Area Differential GNSS 动态地局域差分GNSSkW Kilowatt千瓦kWh Kilowatt hour千瓦小时LL11575.42MHz L-Band carrier L1频率L21227.6MHz L-Band carrier L2频率LAAS Local Area Augmentation System局域增强系统LACAC Latin American Civil Aviation Commission拉丁美洲民航委员会LADGNSS Local Area Differential GNSS局域差分全球卫星导航系统LADS局域差分系统LAN Local Area Network局域网LAT/LONG Latitude/LongitudeLat/Long Reference Waypoint经/纬度经/纬度参考点L-Band Approx1,500MHz L波段(1500兆赫附近频段)LCD Liquid Crystal Display液晶显示LCN Local Communications Network局域通信网络LDGPS Local Differential GPS本地差分GPSLEO Low Earth Orbit近地轨道、低高度轨道LGA Low Gain Antenna低增益天线LCN Local Communications NetworkLLWSA(S)Low Level Wind Shear Alert System低空风切变报警系统LMM Locator Middle Marker航向中指点标LNA Low Noise Amplifier低噪音放大器LNAV Lateral Navigation侧向导航LOC Localize Transmitter(Localizer)航向台发信机LOM Locator Outer Marker航向外指点标LON Longitude经度LORAN-C Long Range Navigation System罗兰-C导航系统LPC Linear Predicative Coding线性预测编码LRR Long Range Radar远程雷达LRU Line Replaceable Unit在线替换部件LSB Least Significant Bit最低有效位LVA Large Vertical Aperture大垂直孔径MMALSR Medium-intensity Approach Lighting System with Runway alignment indicator lights中级亮度进近照明系统,并有跑道对准线显示灯MAP Missed Approach Point复飞点MAR Minimally Attended Radar需低度护理的雷达MASPS Minimum Aeronautical System Standards最低航空系统标准MASPS Minimum Aircraft(Aviation)System Performance Specification(Standards)最小飞机(航空)系统性能标准扩展频谱MAWP Missed Approached WaypointMB Market Beacon指点标MBI Message Block Identifier信息块指示器mbps、Mbit/s mega bits per second兆位每秒MCA Minimum Crossing Altitude最低穿越高度MCC Maintenance Control Center维护控制中心MCDU Multifunction Control Display Unit多功能控制显示单元MCI Mode C intruder装有C模式应答器的入侵飞机MCS Master Control Station主控站MCS Monitoring/Control Software监控/管制软件MDA Minimum Descent Altitude最低下降高度MDT Maintenance Data Terminal维护数据终端MEA Minimum En-route Altitude最低航路高度MED Manual Entry Device人工输入器MET Meteorology气象METAR Meteorological Report of Aerodrome Conditions机场条件气象报告MFCP Multifunction Control Display Panel多功能控制显示面板MFDU Multifunction Display Unit多功能显示单元MHz Megahertz兆赫MIDANPIRG Middle East Air Navigation Planning and Implementation Regional Group中东地区航行规划<请合法使用í?t>实施小组MIFR Master International Frequency Registration国际频率注册管理站(员)MIL Military军方MKR Marker指点标MLS Microwave Landing System微波着陆系统MMALS Multi-Mode Approach and Landing System多模式进近和着陆系统MMI Man-Machine Interface人机接口MMR Multi-Mode Receiver多模式接收机MMS Maintenance Management System维护管理系统MMW Millimeter Wave毫米波MNPS Minimum Navigation Performance Specification最低导航性能规范MNPSA MNPS airspace最低导航性能规范空域MNT Mach Number Technique马赫数技术MOCA Minimum Obstruction Clearance Altitudes最低超障净空高度Mode S specific services S模式特定业务MODEM Modulator-Demodulator调制解调器MOPR Minimum Operational Performance Requirements最低运行性能要求MOPS Minimum Operational Performance Standards最低运行性能规范MORA Minimum Off-Route Altitude<请合法使用软件>低偏离航路高度MOS Metal-Oxide Semiconductor金属-氧化物半导体MOU Memorandum Of Understanding备忘录MPS Maintenance Processor Subsystem维护处理机分系统MRA Minimum Reception Altitude最低接受高度MRT Multi-Radar Tracking多雷达跟踪MRT-VU Multi-Radar Tracking using Variable Update采用变化更新的多雷达跟踪MSA Minimum Sector Altitude最低扇区高度MSAT移动业务卫星系统(美国的)MSAW Minimum Safe Altitude Warning最低安全高度警告MSCP Mobile Satellite Service Provider移动卫星业务提供者MSE Mean Square Error均方误差MSK Minimum Shift Keying最小移频键控MSL Mean Sea Level平均海平面MSP Mode S specific protocol S模式特别协议(规程)MSU Mode Select Unit模式选择单元MTBA Mean Time Between Alarm(Warning)平均告警间隔时间MTBF Mean Time Between Failure平均故障间隔时间MTBO Mean Time Between Outage平均故障停工间隔时间MTBR Mean Time Between Repairs平均故障修复间隔时间MTBUR Mean Time Between Unit Replacements平均更换故障单元间隔时间MTBW Mean Time Between Warning平均告警间隔时间MTD Maintenance Terminal Display维护终端显示器MTI Moving Target Indicator活动目标指示器MTM Module Test and Maintenance模块测试和维护MTMIU Module Test and Maintenance Bus Interface Unit模块测试和维护总线接口单元MTN MEGA Transport Network MEGA运输网络MTSAT Multi-Functional Transport Satellite多功能传送卫星(日本)MTTDA Mean Time To Dispatch Alert平均签派告警时间MTTF Mean Time To Failure平均故障时间MTTM Mean Time To Maintenance平均维护时间MTTR Mean Time to Repair维平均修时间MTTR Mean Time to Restore平均恢复时间MU Management Unit管理单元MUS Minimum Use Specification最低应用规格MUX Multiplexer复用器MN Multisensor Navigation多传感器导航MUX Multiplexer多工器,复用器MWARA Major World Air Route Area世界主要航路区MWO Meteorological Watch Office气象观测台NN Navigation导航NA Not Applicable不可用NACK Negative Acknowledgment出错通知NAD North American Datum北美数据(基准)NADIN National Airspace Data Interchange Network国家空域数据交换网NAGU咨询报告NAILS National Airspace Integrated Logistics Support国家空域综合后勤保障NAPA国家公共管理科学院(美国)Nanosecond One billionth of a second十亿分之一秒NAR National Airspace Review国家空域审议NARACS National Radio Communications System国家无线电通信系统NAS National Airspace System国家空域系统NASA National Aeronautics and Space Administration国家航空和宇航局(美国)NASNET National Airspace System Network国家空域系统网络NASP National Airport System Plan国家航空港系统计划NASPALS NAS Precision Approach and Landing System国家空域系统进近和着陆系统NAT North Atlantic北大西洋地区NAT ADSG North Atlantic Automatic Dependent Surveillance Development Group北大西洋自动相关监视开发小组NAT ATS North Atlantic Air Traffic Services北大西洋空中交通服务NAT SPG North Atlantic Systems Planning Group北大西洋系统规划组NATCOM National Communications Center,Kansas City, Missouri国家通信中心(位于密苏里州堪萨斯城)NATS National Air Traffic Service国家空中交通服务NAV NavigationNAVAID Navigational Aid导航设施NAVAID Radio Aid to Navigation无线电助(导)收设备NAVD North American Vertical Datum北美垂直向数据NCA National Control Authority国家指挥当局NAWP National Aviation Weather Processor国家航空气象处理机NCC Network Control Center网络控制中心NCD No-Computed Data无算出数据NCIU NEXRAD communications interface unit改进型气象雷达通信接口单元NCP Network Control processor网络控制处理器NCS Network Coordinating Station网络协调台NDB Nondirectional Radio Beacon无方向信标NEAN North European ADS-B Network北欧ADS-B网络NEOF National Emergency Operations Facilities国家应急指挥设施NEXRAD Next Generation Weather Radar改进型气象雷达;下一代气象雷达NGRS National Geodetic Reference System国家测地参考系统NGS National Geodetic Survey国家测地勘察NICS NAS Interfacility Communications System国家空域系统设施间的通信系统NIST National Institute of Standards and Technology国家标准和技术研究所NLES导航岸站NM(NMI)Nautical Mile海里,节NMC National Meteorological Center国家气象中心(美国) NMCE Network Monitoring and Control Equipment网络监控设备NMDPS Network Management Data Process System网络管理数据处理系统NMS Navigation Management System导航管理系统NOAA National Oceanic and Atmospheric Administration国家海洋和大气局(美国)NOPAC North Pacific北太平洋NOTAM Notice to Airmen航行通告NPA Non-Precision Approach非精密进近NPDU Network Protocol Data Unit网络协议数据单元NPLAS National Plan of Integrated Airport Systems国家综合航空港系统的计划NRC National Research Committee国家科学研究委员会(美国的)NSAP Network Service Access Point网络服务访问点NSB National Secure Bureau国家保安局(美国的)NSC Network Service Centre网络服务中心NSDU Network Service Data Unit网络服务数据单元NSE Navigation System Error导航系统误差NSSF NAS Simulation Support Facility国家空域系统的仿真保障设施NTLA National Telecommunications Information Agency国家电信资料署(美国)NTSB National Transportation Safety Board国家运输安全委员会(美国)NWS National Weather Service国家气象服务(美国)OO&M Operation and Maintenance运行和维修O.R.Operational Requirement运营要求OACC Oceanic Area Control Center海洋区域管制中心OAS Obstacle Assessment Surface障碍物评价面OAS Oceanic Automation System海洋自动化系统OCA Obstacle Clearance Altitude超障净高度OCA Oceanic Control Area海洋管制区OCH Obstacle Clearance Height超障高OCM Oceanic Clearance Message海洋放行许可信息OCP ICAO Obstacle Clearance Panel国际民航组织超障净空专家组OCS运行控制系统OCS Obstacle Clearance Surface超障面ODALS Omnidirectional Approach Lighting System全向进近灯光系统ODAPS Oceanic Display and Planning System远洋飞行显示和规划系统ODF Oceanic Development Facility海洋开发设施ODIAC Operational Development of Initial Air-ground data Communications早期空-地数据通信运行开发ODL Oceanic Data Link海洋数据链OEM Original Equipment Manufacturer原始设备制造商OFDPS Offshore Flight Data Processing System近海飞行数据处理系统OLAN Onboard Local Area Network机载局域网OLDI On-line Data Interchange联机数据交换OMB Office of Management and Budget国家管理和预算局(美国) OMEGA A navigation system that uses two high-powered transmitter grounds stations to broadcast a continuous wave signal.奥米加导航系统ODALS Omnidirectional Approach Lighting System全向进近灯光系统OOOI Out-Off-On-In滑出-起飞-接地-停靠门位OP Operational 运行。
朱俊威-浙江工业大学信息工程学院
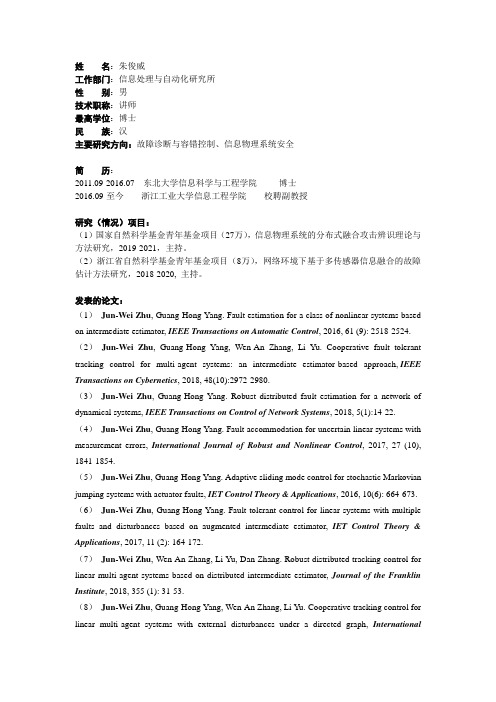
姓名:朱俊威工作部门:信息处理与自动化研究所性别:男技术职称:讲师最高学位:博士民族:汉主要研究方向:故障诊断与容错控制、信息物理系统安全简历:2011.09-2016.07 东北大学信息科学与工程学院博士2016.09-至今浙江工业大学信息工程学院校聘副教授研究(情况)项目:(1)国家自然科学基金青年基金项目(27万),信息物理系统的分布式融合攻击辨识理论与方法研究,2019-2021,主持。
(2)浙江省自然科学基金青年基金项目(8万),网络环境下基于多传感器信息融合的故障估计方法研究,2018-2020, 主持。
发表的论文:(1)Jun-Wei Zhu, Guang-Hong Yang. Fault estimation for a class of nonlinear systems based on intermediate estimator, IEEE Transactions on Automatic Control, 2016, 61 (9): 2518-2524. (2)Jun-Wei Zhu, Guang-Hong Yang, Wen-An Zhang, Li Yu. Cooperative fault tolerant tracking control for multi-agent systems: an intermediate estimator-based approach, IEEE Transactions on Cybernetics, 2018, 48(10):2972-2980.(3)Jun-Wei Zhu, Guang-Hong Yang. Robust distributed fault estimation for a network of dynamical systems, IEEE Transactions on Control of Network Systems, 2018, 5(1):14-22.(4)Jun-Wei Zhu, Guang-Hong Yang. Fault accommodation for uncertain linear systems with measurement errors, International Journal of Robust and Nonlinear Control, 2017, 27 (10), 1841-1854.(5)Jun-Wei Zhu, Guang-Hong Yang. Adaptive sliding mode control for stochastic Markovian jumping systems with actuator faults, IET Control Theory & Applications, 2016, 10(6): 664-673. (6)Jun-Wei Zhu, Guang-Hong Yang. Fault tolerant control for linear systems with multiple faults and disturbances based on augmented intermediate estimator, IET Control Theory & Applications, 2017, 11 (2): 164-172.(7)Jun-Wei Zhu, Wen-An Zhang, Li Yu, Dan Zhang. Robust distributed tracking control for linear multi-agent systems based on distributed intermediate estimator, Journal of the Franklin Institute, 2018, 355 (1): 31-53.(8)Jun-Wei Zhu, Guang-Hong Yang, Wen-An Zhang, Li Yu. Cooperative tracking control for linear multi-agent systems with external disturbances under a directed graph, InternationalJournal of Systems Science, 2017, 48 (13): 2683-2691.(9)Jun-Wei Zhu, Guang-Hong Yang. Fault accommodation for linear systems with time varying delay, International Journal of Systems Science, 2017, 48 (2): 316-323.(10)Jun-Wei Zhu, Guang-Hong Yang. Robust H∞ dynamic output feedback synchronization for complex dynamical networks with disturbances, Neurocomputing, 2016, 175 (part A): 287-292.研究生培养等教学情况:主讲《计算机控制技术》、《系统辨识》等留学生课程。
- 1、下载文档前请自行甄别文档内容的完整性,平台不提供额外的编辑、内容补充、找答案等附加服务。
- 2、"仅部分预览"的文档,不可在线预览部分如存在完整性等问题,可反馈申请退款(可完整预览的文档不适用该条件!)。
- 3、如文档侵犯您的权益,请联系客服反馈,我们会尽快为您处理(人工客服工作时间:9:00-18:30)。
Target Tracking Control of Multiple RobotsZongyao Wang,and Dongbing Gu,Senior Member,IEEEAbstract—This paper focuses on the problem of moving tar-get tracking with a group of mobile robots.Each robot in the group has a pan/tilt camera to detect the target and has limited communication capability to communicate with neighbor robots. The problem is solved by separating it into two parts.One part is the estimation of target position and another is theflocking control of multiple robots moving toward the estimated position. In the target estimation part,we propose to use a novel distributed Kalmanfilter to estimate the target position.The distributed Kalmanfilter is deduced based on a standard Kalmanfilter by modeling the neighbor’s information as one of measurements.In the motion control part,a distributedflocking algorithm is devel-oped.It is used to track the estimated target and avoid collision.In both parts,only local communication between neighbor robots is required.Finally,the tracking algorithms are simulated with2-D and3-D robots to verify their performance.The simulation results provide afirm conclusion that the proposed algorithms are able to track a moving target.A group of real ground mobile robots is used to test the proposed algorithm.The experiment results show that multiple robots are able to cooperate to track the target under the proposed algorithms and the tracking result outperforms the result produced by individual robots without cooperation.Index Terms—Cooperative tracking control,distributed Kalmanfilter,distributed processing,flocking control.I.I NTRODUCTIONT HE collective and cooperation behavior of living beings, such asflocks of bird,shoals offish,herds of wildebeest, has certain advantages,including avoiding predators,increas-ing the chance offinding food,saving energy,etc.Inspired by biological behaviors,multi-robot systems have became an active research area in the past few years.The multi-robot system can be defined as a robot group in which the robots can communicate with others and can cooperatively implement different kinds of tasks.Multi-robot systems have the potential in numerous applications,such as military surveillance,space exploration,cooperative classification,rescue and search,etc. In this paper,we will investigate a multi-robot system,in which each robot is equipped with a local vision camera to detect a moving target and tracks the target.The neighborManuscript received February1,2010;revised September10,2010and February3,2011;accepted April9,2011.Date of publication April21,2011; date of current version March30,2012.Z.Wang is with the Global institute of Management and Economics, Dongbei University of Finance and Economics,Dalian116025,China(e-mail: qianxilong@).D.Gu is with the School of Computer Science and Electronic Engineering, University of Essex,CO43SQ Colchester,U.K.(e-mail:dgu@). Color versions of one or more of thefigures in this paper are available online at .Digital Object Identifier10.1109/TIE.2011.2146211robots interact with each other to share target information.All the robots in the group gradually reach to an agreement about the target position.Finally,all the robots use the estimated target position as a tracking destination to control their motions toflock cohesively.A multi-robot system can be considered as a mobile sensor network.When a sensor network is used to track a moving object,the task of local data aggregation in the network presents a new set of challenges[1]–[8].Although the sensor network covers large spatial area,the moving target only can be viewed by a small number of sensor nodes while most sensor nodes cannot observe the moving target[9]–[11].Cooperative esti-mation of the target position between neighbor sensor nodes and coordinated motion control of multiple robots toward the estimated target position are the challenging issues investigated in this paper.The target tracking control problem is normally solved by separating it into two parts.One part is the estimation of target position and another is theflocking control of multiple robots moving toward the estimated position.In the target estimation part,variants of distributed Kalmanfilters(DKF) have been developed[12]–[14].In these algorithms,multiple measurements and their covariance matrices in the information form of DKF can be expressed as the sum of measurements and covariance matrices of individual sensors.The consensus algorithm developed in[12],[13]can be used to approximate the sums and implement the Kalmanfilter in a distributed way. The main advantage of these DKF s is that the estimates in all sensor nodes will eventually converge to the estimate of centralized Kalmanfilter.However,they are no longer optimal in each step in terms of minimizing estimated error due to the heuristic use of consensus iteration inside of the algorithm, i.e.,the error covariance of these DKF s is an approximation to the optimal error covariance.In the case discussed in this paper,only small number of sensor nodes can detect the moving targets.Most nodes do not have direct measurements and have to use the results of motion model prediction as their estimates. The error covariance approximation could deteriorate the esti-mation result of the entire group.There are also other ad hoc applications of consensus algo-rithms in Kalmanfilters.In[15],a consensus Kalmanfilter with purpose of optimizing two design parameters,the Kalman gain and the consensus matrix,was proposed.In[16],a distributed adaptive algorithm was designed and analyzed.The presence of measurement noises and of packet losses was considered.Each node of the network locally computes adaptive weights that guarantee to minimize the estimation error variance.Decentral-ized conditions on the weights,which ensure the stability of the0278-0046/$26.00©2011IEEEestimates throughout the overall network,were also considered. In[4],the problem was solved by communicating estimates between neighbors and then forming a weighted average as the new estimate.The weights were optimized to yield a small estimation error covariance.The minimization can be done offline,thus allowing only estimates to be communicated. This paper focuses on the Kalmanfilter tracking problem where only part of sensor nodes can observe the moving target. The difference with aforementioned approaches is that the neighbor’s information is modeled as additional measurement in this paper.This modeling strategy results in an implicit consensus algorithm to track the target cooperatively for those robot which can detect the target.For the robots which cannot detect the target,they simply use the neighbor’s information to update its estimated result so that the estimates of small number of robots can be propagated over the entire network through local communication.By modeling the measurements and estimates from neighbors as measurement equations in a local sensor node,a DKF is deduced from the standard Kalmanfilter.The proposed DKF is able to propagate the local information over the entire network.With the estimated target position,mobile robots are required to move toward to the target.Or we can say they are required to move as aflocking group to track the target.We develop aflocking algorithm for the robots to track the target.The flocking algorithm includes a cohesion control component and a separation control component.The cohesion control can keep the group cohesive,and consequently,each robot can communi-cate with at least one neighbors.The key information to achieve the cohesion control is the use of estimated target position as the tracking target for each robot.The separation control is to avoid collision between neighbor robots.When the robots move close enough,they use the potential function approach to separate each other.A collision avoiding potential function is developed for the separation control.The recent work in[17]employed path following strategy for formation control,in[18]employed sliding-mode strategy for formation control,and in[19]em-ployed a vision-guided control for two roboticfishes to track a target.They are different with the proposed work in this paper. The outline of this paper is arranged in the following order. Section II introduces a DKF with a setting of sensor networks. Section III develops the proposed DKF.Section IV describes theflocking algorithm.Section V presents simulation results of 2-D and3-D robots.A group of three real robots is tested and the results are provided in Section VI.A conclusion is given in Section VII.II.K ALMAN F ILTER W ITH M ULTIPLE S ENSORSThe Kalmanfilter is used to estimate the state of a dynamic system based on the sensor measurement.The sensor mea-surement is related to the estimated state,the measurement model,and the system model.The Kalmanfilter is an optimal estimator in the case of Gaussian noise.In the following,a standard Kalmanfilter with multiple sensors is revisited.This is a centralized Kalmanfilter(CKF)where all sensors send their readings to a centralized unit to execute a standard Kalmanfilter to estimate the target position.Consider a discrete-time linear time-invariant system defined by a system equationx t+1=Ax t+Bw t(1) where x t is the system state and w t is the system disturbance. w t is assumed to be Gaussian random vector and its covariancematrix Q is time invariant and Q≥0.The subscript t is the time index.Assuming N robots are used,each of which has a sensor and the measurement is y i t at time t.For robot i,the measurement equation is defined asy i t=C i x t+v i t(2) where v i t is the measurement noise and is assumed to be Gaussian random vector with a time-invariant covariance ma-trix R i>0.Multiple measurements are stacked into a measurement vec-tor and the compact measurement equation isy t=Cx t+v t(3) wherey t=⎡⎢⎣y1t...y N t⎤⎥⎦,C=⎡⎣C1...C N⎤⎦,v t=⎡⎢⎣v1t...v N t⎤⎥⎦.R is the covariance matrix of v tR=diag[R1,...,R N].Following[20],the standard Kalmanfilter consists of two steps.The time update step in the Kalmanfilter¯x t+1=Aˆx t¯P t+1=AP t A T+BQB T.(4) And the measurement update step in the Kalmanfilterˆx t+1=¯x t+1+K t+1(y t+1−C¯x t+1)P t+1=¯P t+1−K t+1C¯P t+1(5) where thefilter gain isK t+1=¯P t+1C T(C¯P t+1C T+R)−1andˆx0and P0are initial state and covariance.In the following presentation,we call(¯x t+1,¯P t+1)as the predicted result and (ˆx t+1,P t+1)as the estimated result.The evolution of covariance in the above two steps can be integrated into a Ricatti difference equation(RDE)P t+1=AP t A T+BQB T−AP t C T(CP t C T+R)−1CP t A T.(6) For Q≥0,R>0,and P0≥0,if[A,C]is detectable and [A,Q1/2]is stabilizable,the following results are available:thefollowing algebraic Ricatti equation(ARE)exists a unique nonnegative definite symmetrical solution P∞P∞=AP∞A T+BQB T−AP∞C T(CP∞C T+R)−1CP∞A T.(7) The iteration of RDE converges to the solution of ARE. The property of[A,C]is detectable guarantees that nonneg-ative symmetric P t is bounded.The property of[A,Q1/2]is stabilizable guarantees that P t is asymptotic stable.If the covariance¯P t increases,i.e.,the predicted state¯x t becomes worse,it will change thefilter gain accordingly and result in an estimated state which relies more on the measure-ment than the predicted state.If the measurement covariance R increases,i.e.,the measurement y t becomes worse,it will change thefilter gain accordingly and result in an estimated state which relies less on the measurement result than the predicted state.We will use the informationfilter form[21]to deduce the new DKF.The time update step of the CKF in the informa-tion form is the same as in(4).The measurement update step of the CKF in the information form can be written asˆx t+1=¯x t+1+¯P t+1Ni=1(C i)T(R i)−1y i t+1−C i¯x t+1(P t+1)−1=(¯P t+1)−1+Ni=1(C i)T(R i)−1C i.(8) III.DKFIt is not scalable and robust for a robot to receive the measurements from all other robots in order to use a CKF to estimate the result.A DKF advocates each robot only communicates with its neighbors to exchange information and executes DKF using its own measurement and neighbor’s information.In our proposed DKF,the exchanged information between neighbors includes the sensor measurement y i t and the predicted result(¯x i t+1,¯P i t+1).The target state equation is still the same as in(1)in our proposed DKF.However,the measurement equation needs to be reformulated to include the neighbor’s information.The local sensor measurement equation is the same as in (2).The predicted state in time update stepˆx j t(j∈N i)from one of its neighbors is viewed as a measurement for robot i.An additional measurement equation is required for this measurementz ij t=¯x j t.This measurement equation can be further written asz ij t=x t+u ij t(9) where u ij t=¯x j t−x t can be viewed as the measurement noisewith a covarianceS ij t=E¯x j t−x tT¯x j t−x t=¯P j t.By combining the sensor measurement equation(2)and the information from neighbors(9),thefinal measurement equation of robot i can be written asz i t=H i x t+u i t(10) where z i t=⎡⎢⎢⎢⎢⎢⎢⎢⎢⎢⎣y i ty i1t...y iN itz i1t...z iN it⎤⎥⎥⎥⎥⎥⎥⎥⎥⎥⎦,H i=⎡⎢⎢⎢⎢⎢⎢⎢⎢⎢⎣C iC i1...C iN iI...I⎤⎥⎥⎥⎥⎥⎥⎥⎥⎥⎦,and u i t=⎡⎢⎢⎢⎢⎢⎢⎢⎢⎢⎣v i tv i1t...v iN itu i1t...u iN i t⎤⎥⎥⎥⎥⎥⎥⎥⎥⎥⎦witha covarianceU i=diag[R i,R i1,...,R iN i,S i1,...,S iN i]=diagR i,R i1,...,R iN i,¯P i1t,...,¯P iN it.The measurement updating step is deduced from(8)as follows:ˆx i t+1=¯x i t+1+¯P i t+1(H i)T(U i)−1z i t+1−H i¯x i t+1=¯x i t+1+¯P i t+1j∈N i∪i(C j)T(R j)−1×y j t+1−C j¯x i t+1+¯P i t+1j∈N i¯P jt+1−1ׯx j t+1−¯x i t+1P i t+1−1=¯P i t+1−1+(H i)T(U i)−1H i=¯P i t+1−1+j∈N i∪i(C j)T(R j)−1C j+j∈N i¯P jt+1−1.(11)There are three terms inˆx i t+1.Thefirst term¯x i t+1is the predicted state.The second term¯P i t+1j∈N i∪i(C j)T(R j)−1 (y j t+1−C j¯x i t+1)is the contributions from local measure-ment y i t+1and neighbor measurements y j t+1.The third term¯P it+1j∈N i(¯P j t+1)−1(¯x j t+1−¯x i t+1)is the difference between local predicted state and neighbor predicted state.It is the third term that plays a consensus role,i.e., it reduces the estimation difference between neighbor ro-bots.This consensus term is weighted with an adaptive gain ¯P it+1j∈N i(¯P j t+1)−1.If the neighbor’s predicted state is not good,the predicted covariance¯P j t+1is large and the adaptive gain is small.Thus,the corresponding consensus term is small. If the neighbor’s predicted state is very good,the predicted covariance¯P j t+1is small and the adaptive gain is large.Thus, the corresponding consensus term is large.The inverse covariance (P i t +1)−1also includes three terms.The first term (¯P i t +1)−1is the local prediction.The second term j ∈N i ∪i (C j )T (R j )−1C jis the contributions from local andneighbor covariances.The third term j ∈N i (¯P j t +1)−1is the neighbor’s predicted covariances.The addition of the third term can reduce the local estimated covariance P i t +1.If there is no local measurement,but there is at least one of measurements or predicted states from neighbors,then [A,H i ]is detectable.The conclusions about the RDE and RDE of the CKF described in the previous section are true for the proposed DKF .Thus,the robots which cannot detect the target can still maintain a stable estimated result.The DKF algorithm is summarized in the following table:Algorithm 1Distributed Kalman FilterInitialize P i0,ˆx i 0,t =0Repeat:Time update step:updates ¯x i t +1and ¯P i t +1using (4)Communication step:sends (y i t +1,C i ,R i ,¯x i t +1,¯P i t +1)to its neighbors receives (y j t +1,C j ,R j ,¯x j t +1,¯P j t +1)from its neighborsMeasurement update step:updates ˆx i t +1and P it +1using (11)EndIV .D ISTRIBUTED F LOCKING A LGORITHMIn this section,we will use the estimated target position as a tracking destination and design a flocking controller to enable the entire group to track the target.The artificial potential function approach is used to design the flocking controller.The robot model we used is a kinematic model˙q i =p i(12)where q i is the robot state vector in 2-D or 3-D space and p i isthe robot control vector in 2-D or 3-D space.The first component in the flocking controller is tracking control.The estimated target position plays a key role in this component.When all the robots move toward the target,they will have potential to converge to the target and achieve the cohesive property.The cohesive potential function of robot i isdefined as H c ( q i −ˆxi )=(1/2) q i −ˆx i 2,where q i −ˆx i is the Euclidian norm of q i −ˆxi .In the flocking control,adjacent robots are required to keep a specific distance.If the distance between adjacent robots is too small,they attempt to separate.The robot communica-tion range is limited,the interaction will disappear between neighbors when their distance is larger than the communication range.Based on these requirements,the second component in the flocking controller is separating control.Let H s (r ij )denote the separating potential function between robot i and j where r ij = q i −q j .The potential function of robot i should be nonnegative.We use ∇H s (r ij )to denote the gradient of H s (r ij )with respect to q i.Fig.1.Fuzzy input membershipsets.Putting two control components together,the controller of robot i is designed asp i=−j∈N i∇H s(r ij)−k(q i−ˆx i)(14)where k is a positive gain.Finally,the DKF andflocking control algorithm are summarized in the following table:Algorithm2Distributed Tracking AlgorithmInitialize P i0,ˆx i0,q i0,t=0Loops:Communication step:Robot i sends q i t and(y i t+1,C i,R i,¯x i t+1,¯P i t+1)to its neighborsRobot i receives q j t and(y j t+1,C j,R j,¯x j t+1,¯P j t+1) from its neighborsEstimation step:Robot i estimates the stateˆx i t+1and covariance P i t+1using DKFControl step:Robot i computes p i t using(14)EndV.S IMULATIONSIn our simulations,the pan/tilt camera is simulated with a sensing range of3m.The view of the camera is40◦and it can pan from-170◦to170◦to increase the view.The pan speed of the camera is0.8rad/s.The view of the camera could be blocked by neighbors or obstacles.The camera will not stop scanning unless it detects a target.Also,Gaussian noise is added to the measurement.A.2-D Flocking TrackingIn thefirst simulation,the target moved along a sine-shape trajectory.The60robots were randomly placed in a7m×7m area.The robot communication range was selected as C=1.2m and the expected distance between robots was D= 1m.The robots used the DKF to estimate the position of the target and used theflocking controller toflock and track the estimated target.Fig.3shows the tracking trajectories.The flocking network of thefinal simulation stage is shown in Fig.3 as well.We can see that almost all the individuals maintained the specific distance with their neighbors.An obstacle was placed near the target trajectory.It can be seen that the trajectories of theflocking robots were slightly changed due to the obstacle avoidance maneuvers.But the flocking robots can still keep cohesive and pass through the obstacle.The process of60robots avoiding the obstacle is shown in Fig.4.Fig.4(a)shows theflocking system was moving to the obstacle.At t=115s,theflocking system just encountered the obstacle.Some robots detected the obstacle and moved around it.In Fig.4(b),one of the robots was pushed away from theflocking system and it came back in Fig.4(c).Fig.3.Sixty robots tracking a sinetrajectory.Fig. 4.Sixty robots tracking the target and avoiding the obstacle.(a)t=107s;(b)t=115s;(c)t=126s;(d)t=140s.Finally,theflocking system passed the obstacle and continuedthe tracking.Fig.5shows the estimated results of all the robots.The solidline denotes the true trajectory of the target and the dotted linesare the estimated results of the robots.At the beginning,therobots did not reach to an agreement about the target position.Particularly at thefirst wave crest of the sine trajectory,the dif-ferences between the estimates were enormous.Gradually,allthe estimates asymptotically approximated to a single trajectorywhich was close to the true trajectory.B.3-D Flocking TrackingIn a3-D space,a pan/tilt camera with the sensing range3m issimulated.In the x–y plane,the view of the camera is40◦.Thecamera can rotate360◦around z-axis with a speed0.8rad/s.The camera can detect the target with a tilt view of−15◦∼15◦. The60robots were randomly placed in a7m×7m×7marea.The robot communication range and the expected distancebetween robots were the same as that in the2-D simulation.Fig.6shows the robot trajectories.Fig.5.Targetestimation.Fig.6.Sixty robots track a target in3-Dspace.Fig.7.Target estimates of the60robots in3-D space.The results of the target estimation are shown in Fig.7.The solid line denotes the true trajectory of the target.The dashed lines are the estimated results of the robots.The estimated trajectories are very close to the trueone.Fig.8.Sixty robots track the target and avoid the obstacles.(a)t=20s;(b)t=40s;(c)t=60s;(d)t=80s;(e)t=100s;(f)t=110s;(g)t=115s;and(h)t=120s.In the second simulation,we tested the capability of obstacle avoidance.The process of60robots avoiding the obstacle is shown in Fig.8.The solid line in Fig.8(a)is the trajectory of the target.The two cylinders were placed in the arena to act as the obstacles.The video was taken from t=20s. Fig.8(a)shows that theflocking system kept cohesive and moved to the obstacle.Theflocking system encountered the first obstacle at t=40s[Fig.8(b)].Some robots detected the obstacle and moved around it.In Fig.8(c),theflocking pattern was changed due to the potential force from thefirst obstacle. Fig.8(d)shows that theflocking system encountered the second obstacle and was“nipped”by the two obstacles.Because the gap between two obstacles was not wide enough to let the entire flocking system pass through,some of the robots were pushed away from theflocking system andflew around the obstacles [Fig.8(e)and(f)].In Fig.8(g),the robots rejoined together and theflocking system became cohesive again.Finally,the flocking system passed through the obstacles and continued the tracking[Fig.8(h)].During the tracking,no robot collided with the obstacles.Fig.9.Minimum distance between the robots.To prove that there was no collision,the minimal distance among the robots was calculated and shown in Fig.9.During the whole process,the minimal distance kept a stable level around0.9m and no collision happened at any time.There were two small valleys at t=60s and t=110s which were the moments of encountering thefirst and second obstacle.VI.E XPERIMENTSA group of three wifibots was used to test the proposed algo-rithm in our robotic arena of University of Essex.The wifibots are networked robots which can use wireless communication to exchange information with neighbors.The TCP/IP protocol was used to implement the communication between robots. A3-D racking system(VICON)was used to provide robot positions to each of the robots.It also provides the ground true target trajectory for analysis.The on-board camera can pan from−170to170◦to increase the viewfield.A feedback control loop was used to control the camera pan angle.The control objective was to keep the target in the middle of the image.The target was also a wifibot and detected by using the on-board pan/tilt camera of other three tracking robots.To simplify the vision processing,the target wifibot was equipped with a red marker.The on-board camera only detected the red marker by using color threshold techniques.The image obtained by the on-board camera was in RGB(red,green,blue)space.Because an image in HSV(hue,saturation,value)space is more sensitive to colors,the RGB image was converted to the HSV image.It is known that different colors have different hue degrees in HSV images.A band-passfilter was used tofilter the red color.For the purpose of de-noise,an erosion algorithm was applied to erase the high-frequency component in the image.Finally,we got an image with the target only.The mean position of all the pixels of target was calculated.To measure the distance to the target,the width of the target image was also calculated during the process.Tofind the relationship between the distance and the width of the target image,we recorded the distances from the robot to the target and also recorded the corresponding width of thetarget Fig.10.Target estimation of three robots withoutcooperation.Fig.11.Target estimation of three robots with cooperation.image.It was found that the relationship between the distance and the image width can befitted to a logarithm functiond ir=−590log(α/195)+300whereαis the width of the target image.In thefirst test,three robots used the standard Kalmanfilter, i.e.,they did not exchange their information.Fig.10shows the estimated results of the target position of all three robots. It can be seen that the differences between three estimates were enormous.Due to the disagreement,it made theflocking control more difficult.During the testing,theflocking network easily collapsed because robots tracked“different targets”.In the second test,three robot used the developed DKF, i.e.,they exchanged their information for tracking cooperation. Fig.11shows the estimated results of the target position of all three robots.The solid line denotes the true trajectory of the target;the dashed lines are the estimated results of threeand(d)t=100s.Fig.13.Robot trajectories.pared with thefirst testing result,the disagreement of estimates was reduced.All the estimates asymptotically approximated to a single trajectory which was close to the true trajectory.Fig.12shows the video snapshots at time t=0s,t=37, t=72,and t=100s to demonstrate theflocking behavior. At the beginning,three robots were placed randomly in the robot arena.The target shown in Fig.12(a)was moving along a predefined trajectory.Three robots used the cameras and the DKF to estimate the target position.At t=37s,three robots had reached to an agreement about the target position,the robots cooperatively tracked the target,and all the three robots were moving to the estimated position.After t=72s,three robots gradually caught up with the target.The trajectories of three robots are shown in Fig.13.The solid line represents thetrajectory of the target.The other three dashed lines are theFig.16.Camera detectability(a)individual robots and(b)cooperative robots. Fig.16(a)and cooperative robots in Fig.16(b).It demonstrates a clear improvement of the view coverage with the proposed algorithm.VII.C ONCLUSIONThis paper presents a cooperative target tracking and coor-dinated control approach to multi-robot systems.A DKF is developed to estimate the target position cooperatively.Mul-tiple robots provide a wider view of the system and therefore improve the estimation result.The proposed DKF is deduced from the standard Kalmanfilter and only requires the local communication between neighbor robots.Aflocking algorithm based on the estimated target position is used to control the robot group to track the target and keep the group connected. Substantial work has been done to implement the algorithms in real robots.A group of three robots was used to test the algorithm performance.The experiment results show that the proposed DKF can provide the estimated result of target position with an accuracy of average250mm in our experiment. Our further work will focuses on implementation of the proposed algorithm on3-D real robots.Also,the theoretical analysis of the proposed algorithm should be conducted.R EFERENCES[1]A.Ribeiro,G.Giannakis,and S.Roumeliotis,“SOI-KF:DistributedKalmanfiltering with low-cost communications using the sign of inno-vations,”IEEE Trans.Signal Process.,vol.54,no.12,pp.4782–4795, Dec.2006.[2]Y.Sheng,X.Hu,and P.Ramanathan,“Distributed particlefilter withGMM approximation for multiple targets localization and tracking in wireless sensor networks,”in Proc.4th Int.Symp.Inf.Process.Sensor Netw.,Apr.2005,pp.181–188.[3]F.Zhao,J.Shin,and J.Reich,“Information-driven dynamic sensor col-laboration for tracking applications,”IEEE Signal Process.Mag.,vol.19, no.2,pp.61–72,Mar.2002.[4]P.Alriksson and A.Rantzer,“Experimental evaluation of a distributedKalmanfilter algorithm,”in Proc.46th IEEE Conf.Decision Control, Dec.2007,pp.5499–5504.[5]B.Zhu,B.Sinopoli,K.Poolla,and S.Sastry,“Estimation over wirelesssensor networks,”in Proc.Amer.Control Conf.,Jul.2007,pp.2732–2737.[6]S.Aeron,V.Saligrama,and D.Castanon,“Energy efficient policies fordistributed target tracking in multihop sensor networks,”in Proc.45th IEEE Conf.Decision Control,Dec.2006,pp.380–385.[7]A.Speranzon,C.Fischione,and K.Johansson,“Distributed and collabo-rative estimation over wireless sensor networks,”in Proc.45th IEEE Conf.Decision Control,Dec.2006,pp.1025–1030.[8]B.Sinopoli,L.Schenato,M.Franceschetti,K.Poolla,M.Jordan,andS.Sastry,“Kalmanfiltering with intermittent observations,”IEEE Trans.Autom.Control,vol.49,no.9,pp.1453–1464,Sep.2004.[9]V.Gupta,T.Chang,B.Hassibi,and R.Murray,“On a stochastic sensorselection algorithm with applications in sensor scheduling and sensor coverage,”Automatica,vol.44,pp.1295–1303,2008.[10]H.Medeiros,J.Park,and A.Kak,“Distributed object tracking using acluster-based Kalmanfilter in wireless camera networks,”IEEE J.Sel.Topics Signal Process.,vol.2,no.4,pp.448–463,Aug.2008.[11]U.Khan and J.Moura,“Distributing the Kalmanfilter for large-scalesystems,”IEEE Trans.Signal Process.,vol.56,no.10,pp.4919–4935, Oct.2008.[12]D.P.Spanos,R.Olfati-Saber,and R.M.Murray,“Dynamic consensuson mobile networks,”in Proc.16th IFAC World Congr.,Prague,Czech Republic,Apr.2005.[13]R.Olfati-Saber,“Distributed Kalmanfiltering for sensor networks,”inProc.46th IEEE Conf.Decision Control,Dec.2007,pp.5492–5498. [14]R.Rahman,M.Alanyali,and V.Saligrama,“Distributed tracking in mul-tihop sensor networks with communication delays,”IEEE Trans.Signal Process.,vol.55,no.9,pp.4656–4668,Sep.2007.[15]R.Carli,A.Chiuso,L.Schenato,and S.Zampieri,“Distributed Kalmanfiltering using consensus strategies,”in Proc.46th IEEE Conf.Decision Control,Dec.2007,pp.5486–5491.[16]A.Speranzon,C.Fischione,B.Johansson,and K.Johansson,“Adaptivedistributed estimation over wireless sensor networks with packet losses,”in Proc.46th IEEE Conf.Decision Control,Dec.2007,pp.5472–5477.[17]J.Ghommam and F.Mnif,“Coordinated path-following control for agroup of underactuated surface vessels,”IEEE Trans.Ind.Electron., vol.56,no.10,pp.3951–3963,Oct.2009.[18]M.Defoort,T.Floquet,A.Kokosy,and W.Perruquetti,“Sliding-mode for-mation control for cooperative autonomous mobile robots,”IEEE Trans.Ind.Electron.,vol.55,no.11,pp.3944–3953,Nov.2008.[19]Y.Hu,W.Zhao,and L.Wang,“Vision-based target tracking and collisionavoidance for two autonomous roboticfish,”IEEE Trans.Ind.Electron., vol.56,no.5,pp.1401–1410,May2009.[20]B.Anderson and J.Moore,Optimal Filtering.Englewood Cliffs,NJ:Prentice-Hall,1979.[21]B.Rao and H.Durrant-Whyte,“Fully decentralized algorithm for multi-sensor Kalmanfiltering,”Proc.Inst.Elect.Eng.—Control Theory Appl., vol.138,no.5,pp.413–420,Sep.1991.Zongyao Wang received the B.Sc.degree intelecommunication from Harbin Engineering Uni-versity,Harbin,China,the M.Sc.degree in electron-ics,and the Ph.D.degree in robotics from Universityof Essex,Colchester,U.K.He is a Lecturer with the Global institute ofManagement and Economics,Dongbei University ofFinance and Economics,Dalian,China.His researchinterest includes multi-agent systems,distributed ro-botflocking,sensor network,and consensus algo-rithm.He has the experience of teaching and research in U.K.as a Graduate Teaching Assistant.He published15academic papers in international conferences andjournals.Dongbing Gu received the B.Sc.and M.Sc.de-grees in automatic control from Beijing Instituteof Technology,Beijing,China,and the Ph.D.de-grees in computer science from University of Essex,Colchester,U.K.He joined University of Essex in2000as a Lec-turer.He was an academic visitor in robotics re-search group at Oxford University,Oxford,U.K.,during1996–1997.He is currently a Reader with theSchool of Computer Science and Electronic Engi-neering,University of Essex.He has published over 100papers in international journals and conferences.His current research inter-ests include multi-agent systems,wireless sensor networks,distributed control algorithms,distributed information fusion,cooperative control,reinforcement learning,and model predictive control.His research has been supported by EU, EPSRC,and the Royal Society.。