Robust Experimental Design for Multivariate Generalized Linear Models
基于RF-NSGA-Ⅱ的建筑能耗多目标优化

第38卷第2期2021年4月Vol.38No.2Apr.2021土木工程与管理学报Journal of Civil Engineering and Management基于RF-NSGA-II的建筑能耗多目标优化吴贤国1,杨赛1,王成龙2,王洪涛2,陈虹宇3,朱海军2,王雷1(1.华中科技大学土木与水利工程学院,湖北武汉430074;2.中建三局集团有限公司,湖北武汉430064;3.南洋理工大学土木工程与环境学院,新加坡639798)摘要:本文提出一种随机森林-带精英策略的非支配排序遗传算法(RF-NSGA-II)相结合的方法,基于建筑外围护结构设计优选进行建筑能耗多目标优化。
首先通过建立BIM模型导入DesignBuilder软件,利用正交试验获取建筑外维护结构主要参数与对应能耗的模拟样本集,再将样本数据集用于RF模型预测中进行训练,将训练好的RF回归函数作为适应度函数并作为优化目标之一。
在此基础上引入室内热舒适度函数为另一个适应度函数,采用NSGA-II进行多目标优化,得到Pareto前沿解集并从中利用理想点法得到一组多目标最优解。
通过本研究可以得出:利用RF对建筑能耗进行预测精度高,所得预测效果好,通过NSGA-II算法和理想点法可以获得满足建筑能耗和舒适度要求的外围护设计参数最优取值,结果与实际相符,效果良好。
对实现低建筑能耗和高热舒适度优化具有参考价值。
关键词:建筑能耗模拟;围护结构;随机森林;遗传算法;热舒适度;多目标优化中图分类号:TU201.5文献标识码:A文章编号:2095-0985(2021)02-0001-08Multi-objective Optimization of Building Energy Consumption Based on RF-NSGA-II WU Xianguo1,YANG Sai1,WANG Chenglong2,WANG Hongtao2,CHEN Hongyu3,ZHU Haijun2,WANG Lei(1.School of Civil and Hydraulic Engineering,Huazhong University of Science and Technology,Wuhan430074,China; 2.China Construction Third Engineering Bureau Group Co Ltd, Wuhan430064,China; 3.School of Civil and Environmental Engineering,Nanyang Technological University,Singapore639798,Singapore)Abstract:This paper proposes a combination method of random forest and non-dominated sorting genetic algorithm with elite strategy(RF-NSGA-II),which is based on the optimization of building envelope structure design for multi-objective optimization of building energy consumption.Firstly,by establishing a BIM model and importing it into DesignBuilder software,we use orthogonal experiments to obtain a simulation sample set of the main parameters of the maintenance structure outside the building and the corresponding energy consumption.And then we use the sample data set in the RF model prediction for training and take the trained RF regression function as a fitness function and one of the optimization goals.On this basis,the indoor thermal comfort function is introduced as another fitness function,and NSGA-II is used for multi-objective optimization,and the Pareto frontier solution set is obtained,and a set of multi-objective optimal solutions are obtained by using the ideal point method.Through this research,it can be concluded that the prediction accuracy of building energy consumption by RF is used,and the obtained prediction effect is good.The optimal envelope design parameters can be obtained to meet the requirements of building energy consumption and comfort through NS-GA-II algorithm and ideal point method.The result is consistent with the actual value and the effect is good.It has reference value to realize the optimization of low building energy consumption and high收稿日期:2020-10-05修回日期:2020-12-09作者简介:吴贤国(1964—),女,湖北武汉人,博士,教授,研究方向为土木工程施工及管理(Email:wxg0220@)通讯作者:王成龙(1990—),男,湖北武汉人,工程师,研究方向为土木工程施工及管理(Email:*****************)基金项目:国家重点研发计划(2016YFC0800208);国家自然科学基金(51378235;71571078;51308240)・2・土木工程与管理学报2021年thermal comfort.Key words:building energy consumption simulation;envelope structure;random forest;genetic algorithm;thermal comfort;multi-objective optimization能源消耗一直是世界各国备受关注的问题,其中建筑能耗问题同样需要引起重视。
Robust Control

Robust ControlRobust control is a concept that is essential in the field of engineering, particularly in the design and implementation of control systems for various applications. It involves the ability of a control system to maintain stable and satisfactory performance despite uncertainties and variations in the system or external disturbances. This is crucial in ensuring the safety, reliability, and efficiency of complex engineering systems such as aircraft, automotive, robotics, and industrial processes. One of the key challenges in robust control is dealing with uncertainties in the system dynamics, parameters, and disturbances. These uncertainties can arise from various sources such as variations in operating conditions, environmental factors, component tolerances, and modeling errors. In traditional control design, the controller is designed based on a precise mathematical model of the system, which may not accurately capture all the uncertainties. As a result, the controller may perform poorly or even become unstable when the actual system deviates from the ideal model. To address these challenges, robust control techniques have been developed to ensure that the control system can effectively handle uncertainties and variations. One approach is to use robust control design methods such as H-infinity control, mu-synthesis, and loop-shaping to explicitly account for uncertainties and optimize the system performance under these conditions. These methods allow engineers to design controllers that are more resilient to variations and disturbances, providing a higher level of stability and performance across a wide range of operating conditions. Another important aspect of robust control is the consideration of practical implementation issues such as sensor noise, actuator saturation, and communication delays. These factors can significantly affect the performance of a control system and may lead to instability or poor control performance if not properly addressed. Robust control techniques can be used to design controllers that are inherently robust to these practical limitations, ensuring that the control system can maintain stable and satisfactory performance in real-world applications. From a practical perspective, robust control is crucial in ensuring the safety and reliability of critical engineering systems. For example, in the aerospace industry, robust control techniques are used to design flight controlsystems that can effectively handle uncertainties and variations in the aircraft dynamics, ensuring stable and safe flight under various operating conditions. Similarly, in automotive applications, robust control is essential for designing vehicle stability control systems that can maintain stability and handling performance in the presence of uncertainties and disturbances. Furthermore, robust control is also important in the field of robotics, where control systems must be able to adapt to variations in the environment, sensor noise, and uncertainties in the robot dynamics. By employing robust control techniques, engineers can design robotic control systems that are more resilient to these uncertainties, enabling robots to perform tasks with a higher level of accuracy and reliability. In conclusion, robust control is a critical aspect of control system design, particularly in the context of complex engineering systems where uncertainties and variations are inevitable. By employing robust control techniques, engineers can design control systems that are more resilient to uncertainties, variations, and practical limitations, ensuring the safety, reliability, and performance of critical engineering systems. As technology continues to advance and engineering systems become more complex, the importance of robust control will only continue to grow, making it an essential area of research and development in the field of control engineering.。
基于动态规划提取信号小波脊和瞬时频率
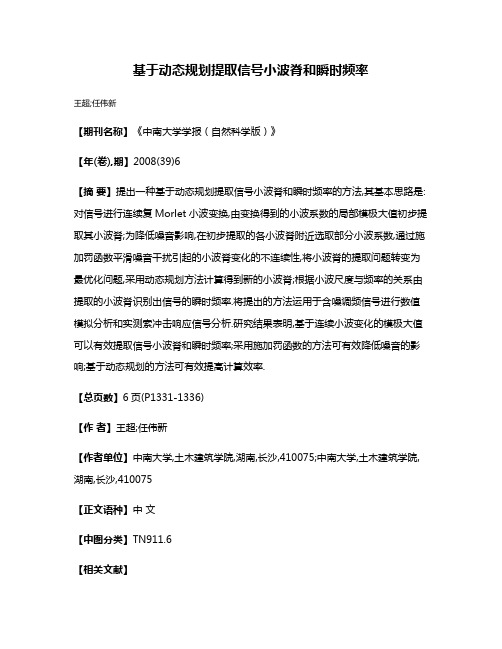
基于动态规划提取信号小波脊和瞬时频率
王超;任伟新
【期刊名称】《中南大学学报(自然科学版)》
【年(卷),期】2008(39)6
【摘要】提出一种基于动态规划提取信号小波脊和瞬时频率的方法,其基本思路是:对信号进行连续复Morlet小波变换,由变换得到的小波系数的局部模极大值初步提取其小波脊;为降低噪音影响,在初步提取的各小波脊附近选取部分小波系数,通过施加罚函数平滑噪音干扰引起的小波脊变化的不连续性,将小波脊的提取问题转变为最优化问题,采用动态规划方法计算得到新的小波脊;根据小波尺度与频率的关系由提取的小波脊识别出信号的瞬时频率.将提出的方法运用于含噪调频信号进行数值模拟分析和实测索冲击响应信号分析.研究结果表明,基于连续小波变化的模极大值可以有效提取信号小波脊和瞬时频率;采用施加罚函数的方法可有效降低噪音的影响;基于动态规划的方法可有效提高计算效率.
【总页数】6页(P1331-1336)
【作者】王超;任伟新
【作者单位】中南大学,土木建筑学院,湖南,长沙,410075;中南大学,土木建筑学院,湖南,长沙,410075
【正文语种】中文
【中图分类】TN911.6
【相关文献】
1.基于小波脊的UM71信号瞬时特征提取 [J], 林王仲;戴云陶
2.基于改进小波脊提取算法的数字信号瞬时频率估计方法 [J], 汪赵华;郭立;李辉
3.基于二进小波变换的实信号的多尺度Hilbert变换和瞬时频率提取 [J], 蔡毓;刘贵忠;侯兴松
4.基于SWT的自适应多脊提取的滚动轴承瞬时频率估计 [J], 李延峰;韩振南;王志坚;武学峰
5.基于最大坡度法提取非平稳信号小波脊线和瞬时频率 [J], 刘景良;任伟新;王超;黄文金
因版权原因,仅展示原文概要,查看原文内容请购买。
德国艺术设计教育模式探究——以汉堡美术学院为例
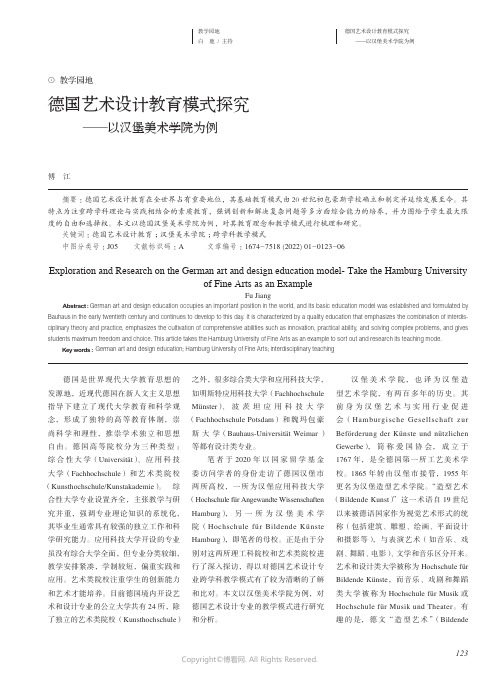
教学园地白 鹿 / 主持德国艺术设计教育模式探究 —以汉堡美术学院为例⊙ 教学园地德国艺术设计教育模式探究—以汉堡美术学院为例傅 江德国是世界现代大学教育思想的发源地,近现代德国在新人文主义思想指导下建立了现代大学教育和科学观念,形成了独特的高等教育体制,崇尚科学和理性,推崇学术独立和思想自由。
德国高等院校分为三种类型:综合性大学(Universität )、应用科技大学(Fachhochschule )和艺术类院校(Kunsthochschule/Kunstakademie )。
综合性大学专业设置齐全,主张教学与研究并重,强调专业理论知识的系统化,其毕业生通常具有较强的独立工作和科学研究能力。
应用科技大学开设的专业虽没有综合大学全面,但专业分类较细,教学安排紧凑,学制较短,偏重实践和应用。
艺术类院校注重学生的创新能力和艺术才能培养。
目前德国境内开设艺术和设计专业的公立大学共有24所,除了独立的艺术类院校(Kunsthochschule )摘要:德国艺术设计教育在全世界占有重要地位,其基础教育模式由20世纪初包豪斯学校确立和制定并延续发展至今。
其特点为注重跨学科理论与实践相结合的素质教育,强调创新和解决复杂问题等多方面综合能力的培养,并力图给予学生最大限度的自由和选择权。
本文以德国汉堡美术学院为例,对其教育理念和教学模式进行梳理和研究。
关键词:德国艺术设计教育;汉堡美术学院;跨学科教学模式中图分类号:J05 文献标识码:A 文章编号:1674-7518 (2022) 01-0123-06Exploration and Research on the German art and design education model- Take the Hamburg Universityof Fine Arts as an ExampleFu JiangAbstract : German art and design education occupies an important position in the world, and its basic education model was established and formulated by Bauhaus in the early twentieth century and continues to develop to this day. It is characterized by a quality education that emphasizes the combination of interdis-ciplinary theory and practice, emphasizes the cultivation of comprehensive abilities such as innovation, practical ability, and solving complex problems, and gives students maximum freedom and choice. This article takes the Hamburg University of Fine Arts as an example to sort out and research its teaching mode. Key words : German art and design education; Hamburg University of Fine Arts; interdisciplinary teaching之外,很多综合类大学和应用科技大学,如明斯特应用科技大学(Fachhochschule Münster )、波茨坦应用科技大学(Fachhochschule Potsdam )和魏玛包豪斯大学(Bauhaus-Universität Weimar )等都有设计类专业。
Adaptive tracking control of uncertain MIMO nonlinear systems with input constraints
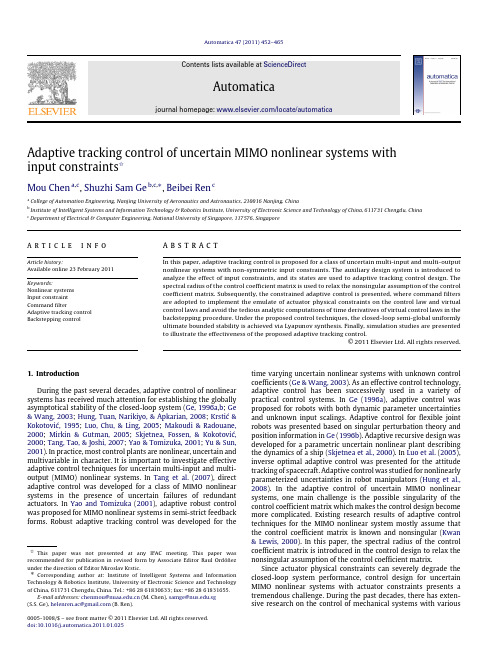
article
info
abstract
In this paper, adaptive tracking control is proposed for a class of uncertain multi-input and multi-output nonlinear systems with non-symmetric input constraints. The auxiliary design system is introduced to analyze the effect of input constraints, and its states are used to adaptive tracking control design. The spectral radius of the control coefficient matrix is used to relax the nonsingular assumption of the control coefficient matrix. Subsequently, the constrained adaptive control is presented, where command filters are adopted to implement the emulate of actuator physical constraints on the control law and virtual control laws and avoid the tedious analytic computations of time derivatives of virtual control laws in the backstepping procedure. Under the proposed control techniques, the closed-loop semi-global uniformly ultimate bounded stability is achieved via Lyapunov synthesis. Finally, simulation studies are presented to illustrate the effectiveness of the proposed adaptive tracking control. © 2011 Elsevier Ltd. All rights reserved.
Geometric Modeling

Geometric ModelingGeometric modeling is a fundamental concept in the field of computer graphics and design. It involves the creation and manipulation of digital representations of objects and environments using geometric shapes and mathematical equations. This process is essential for various applications, including animation, virtual reality, architectural design, and manufacturing. Geometric modeling plays a crucial role in bringing creative ideas to life and enabling the visualization of complex concepts. In this article, we will explore the significance of geometric modeling from multiple perspectives, including its technical aspects, creative potential, and real-world applications. From a technical standpoint, geometric modeling relies on mathematical principles to define and represent shapes, surfaces, and volumes in a digital environment. This involves the use of algorithms to generate and manipulate geometric data, enabling the creation of intricate and realistic 3D models. The precision and accuracy of geometric modeling are essential for engineering, scientific simulations, and industrial design. Engineers and designers utilize geometric modeling software to develop prototypes, analyze structural integrity, and simulate real-world scenarios. The ability to accurately model physical objects and phenomena in a virtual space is invaluable for testing and refining concepts before they are realized in the physical world. Beyond its technical applications, geometric modeling also offers immense creative potential. Artists and animators use geometric modeling tools to sculpt, texture, and animate characters and environments for films, video games, and virtual experiences. The ability to manipulate geometric primitives and sculpt organic forms empowers creatives to bring their imaginations to life in stunning detail. Geometric modeling software provides a canvas for artistic expression, enabling artists to explore new dimensions of creativity and visual storytelling. Whether it's crafting fantastical creatures or architecting futuristic cityscapes, geometric modeling serves as a medium for boundless creativity and artistic innovation. In the realm of real-world applications, geometric modeling has a profound impact on various industries and disciplines. In architecture and urban planning, geometric modeling software is used to design and visualize buildings, landscapes, and urban developments. This enables architects and urban designers toconceptualize and communicate their ideas effectively, leading to the creation of functional and aesthetically pleasing spaces. Furthermore, geometric modelingplays a critical role in medical imaging and scientific visualization, allowing researchers and practitioners to study complex anatomical structures and visualize scientific data in meaningful ways. The ability to create accurate and detailed representations of biological and physical phenomena contributes to advancementsin healthcare, research, and education. Moreover, geometric modeling is integral to the manufacturing process, where it is used for product design, prototyping,and production. By creating digital models of components and assemblies, engineers can assess the functionality and manufacturability of their designs, leading tothe development of high-quality and efficient products. Geometric modeling also facilitates the implementation of additive manufacturing technologies, such as 3D printing, by providing the digital blueprints for creating physical objects layer by layer. This convergence of digital modeling and manufacturing technologies is revolutionizing the production landscape and enabling rapid innovation across various industries. In conclusion, geometric modeling is a multifaceteddiscipline that intersects technology, creativity, and practicality. Its technical foundations in mathematics and algorithms underpin its applications in engineering, design, and scientific research. Simultaneously, it serves as a creative platform for artists and animators to realize their visions in virtual spaces. Moreover,its real-world applications extend to diverse fields such as architecture, medicine, and manufacturing, where it contributes to innovation and progress. The significance of geometric modeling lies in its ability to bridge the digital and physical worlds, facilitating the exploration, creation, and realization of ideas and concepts. As technology continues to advance, geometric modeling will undoubtedly play an increasingly pivotal role in shaping the future of design, visualization, and manufacturing.。
基于Roberts边缘检测的面向对象建筑物信息提取

2 2 R br 算 法描 述 . o et s 多尺度分 割采 用 的是 一种 基 于 区域合 并 的分 割方
式, 分割结果 表 现在 不 同的层 面上 , 些层 面 的信 息 由 这 选 择 的尺度 但 形 状 的观察 并不适 合 , 物 的空 问信息 在边 缘检 测 所 而地 得 到 的影像 结果 中 同样 可以体 现 出来 , 同时生 成 了每 个
择分 割尺度 。选择 分割尺 度 的过 程 繁琐 而复 杂 , 分 割 在
形状 标 准 由光滑 度 与 紧致度 两个 参数 组 成 。光 滑
度标 准 的作 用是 将 影 像 对 象 的边 界 光 滑 度 进 行 优 化 , 在数 据 异质 性 较 高 的情 况 下 可 防止 对 象 边 缘 的破 碎 , 并且 生 成非 紧致 的对 象 。紧致度 标 准 的作 用是 将 影 像 对 象 的紧致 度 进行 优 化 , 影 像 数 据 光 谱 反 差 较 弱 并 当 且 需要 将 紧致 对 象 与非 紧致 对 象 分 开 时 , 使 用 紧 致 需 度 标准 。光 滑 度标 准 与 紧致 度 标 准 并 不 冲 突 , 具 体 在
尺度 ; 最后 , 根据分割得到的影像对象选取建筑物的特
征 组合 成 知识 体 系进 行 分类 提 取 。
2 R br 尺度参数计 算模型 oe s t
2 1 分 割 尺度 .
估 算方 法, 利用 R b, 检 测得到边缘影像 并选取样本计 算 出参数 估值 , 而确定最优分割尺度 。实验表明 。 方法 实 oe s 从 该
现 过程 简单 , 能快速地确 定最优 分割尺度参数 , 高 了建筑物提 取的效 率且精度 高。 提
关 键 词 : 向 对 象 ; 筑 物 提 取 ; 割 尺 度 ; o e s 缘 检 测 面 建 分 Rb, 边
西门子PLM软件Parasolid 产品介绍说明书

Siemens PLM Software ParasolidSummaryParasolid® software is the world’s premier 3D geometric modeling component, selected by leading application vendors and end-user organizations spanning multiple industries as their preferred platform for delivering innovative 3D solutions with unparalleled modeling power, versatility and interoperability. A key offering within Siemens PLM Software’s PLM Components family of software products, Parasolid is tar-geted at a broad range of applications across the product lifecycle and provides robust, high-quality functionality that is easy to use and cost-effective to implement.World-class geometric modeling for demanding 3D applications Parasolid supports solid modeling, facet modeling, generalized cellular modeling, direct modeling and freeform surface/sheet modeling within an integrated framework. Parasolid is available in three commercial packages: Designer, Editor and Communicator – each of which is offered with convergent modeling technology as an option – and is also available to the aca-demic community via an Educator package. The functional scope and typical application at each level are outlined below. The table on the next page summarizes the corre-sponding functionality.Parasolid Designer delivers the full power of Parasolid functionality for unlimited creation, manipulation, interrogation and storage of 3D models. Over 900 object-based API functions provide the most comprehensive and robust 3D modeling platform for demanding 3D applications. Parasolid Editor provides an extended subset of Parasolid functionality that is ideal for analysis, manufacturing and other downstream applications that need to easily manipulate, edit, repair or simplify 3D models without the need for advanced modeling operations.Parasolid benefits• Provides ideal foundationfor innovative 3D applica-tion development• Reduces development costsand risks by providing aproven 3D modelingsolution• Ensures state-of-the-artquality and robustness• Convergent modeling tech-nology seamlesslyintegrates classic b-rep andfacet b-rep modeling opera-tions in a unifiedarchitecture• Offers world-class technicalsupport for rapidtime-to-market• Enables instantcompatibility with otherParasolid-based applicationsthrough translation-freeexchange of 3D data/plmcomponentsParasolidPLM COMPONENTSParasolid Communicator comprises versatile base functionality, including interoper a bility, visualization and data interro g ation capabili-ties, that provides a platform for applications to consume existing 3D models.Parasolid Educator, complementing the above commercial packages, provides academic institutions with the full power of Parasolid functionality for teaching,research and industrial collaboration.Parasolid facts• Fully integrated modeling of 3D curves, surfaces and solids with over 900 API functions • Modeling foundation for hundreds of the world’s leading CAD, CAM and CAE applications• Corporate standard forSiemens’ NX™, Solid Edge®, Femap™ and Teamcenter® software solutions • Used in over 3.5 million seats of application soft-ware globally• Licensed by 170 software vendors for integration into more than 350 applications • Provides industry-leading robustness with over a mil-lion quality tests run daily • Provides unmatched two-way data compatibility via Parasolid native XT format Parasolid usageParasolid is the component of choice for both cloud-based solutions and traditional stand-alone workstations. Parasolid is deployed across a wide range of PLM application domains, including:• Mechanical CAD • CAM/manufacturing • CAE/validation • AEC• Visualization • Data exchange • Interoperability • Knowledge-based engineering • CMM/inspection • CNC/machine tools • Corporate R&D • Academic R&DPLM COMPONENTSFoundation capabilitiesParasolid is built on critical foundation capabilities that enable Parasolid to be deployed successfully in a wide variety of software applications. Enabled across all relevant functionality, Parasolid foundation capabilities include:• Tolerant modeling for intrinsically reliable modeling with imported data• Convergent modeling technology, available as a licensed option, seamlessly integrates classic b-rep and facet b-rep modeling operations in a unified architecture• Attributes and callbacks for application-specific character-istics and behavior• Session and partitioned rollback for flexible history and undo/redo implementation• Data management and tracking for managing models and associated data as they evolve• Thread safety and symmetric multi-processing support for optimal performance on multi-processor machines• Model storage in forwards and backwards compatible native XT format• .NET Binding to integrate Parasolid into .NET applications written in C#• Broad platform coverage including comprehensive support for Windows, Linux, Unix and MacGetting startedParasolid is delivered with a compre h ensive set of documen-tation and developer resources, including a complete Jumpstart Kit of tools that promote easy integration of Parasolid into new and existing applications:• Full Product Documentation Suite in html and pdf formats • Parasolid Workshop prototypingenvironment for Windows• Example Application Resourcesto get you up and running• Code Example Suite illustratesbest implementation practice• Parasolid ‘Getting Started’ Guideanswers your questions• Parasolid Overview summarizesParasolid capabilities• Parasolid API Training Materialsto educate the team Support, training and consultingParasolid has a renowned technical support, training and consulting team, dedicated to helping customers achieve the best possible implementation by providing expert advice on all matters related to Parasolid usage.Responsive telephone and email support is backed by an online support center that provides round-the-clock access to frequent product updates, as well as customer-specific issue reporting and tracking.In addition, specialized training and consulting services are available that can be tailored to customer requirements. Whether you are starting fresh, extending an existing appli-cation or transitioning from other modeling technology, the Parasolid support, training and consulting team is with you every step of the way.Interoperability productsThe Parasolid product suite is augmented by a range of add-on products that provide high-quality interoperability with third-party CAD data. These include Parasolid Bodyshop, a specialized tool for boosting the success of 3D data exchange by cleaning and repairing imported models, and Parasolid Translator toolkits for converting model data between Parasolid and other major standard and proprietary CAD formats, including STEP, IGES, Catia V4, Catia V5, Pro/ Engineer and ACIS(SAT).Siemens PLM Software partners with Tech Soft 3D to offer Hoops Exchange. This highly-integrated and industry-proven 3D data collaboration solution for Parasolid provides high performance import, export, healing and visualization toolsfor a wide range of 3D file formats.Siemens PLM SoftwareAmericas +1 314 264 8499Europe +44 (0) 1276 413200Asia-Pacific +852 2230 3308/plmrespective holders.5661-Y7 3/16 BConvergent modeling: facetmodel of knee joint withb-rep surgical guide, mod-eled in single architecture.。
一种虚拟手术二维力觉交互装置的力学模型
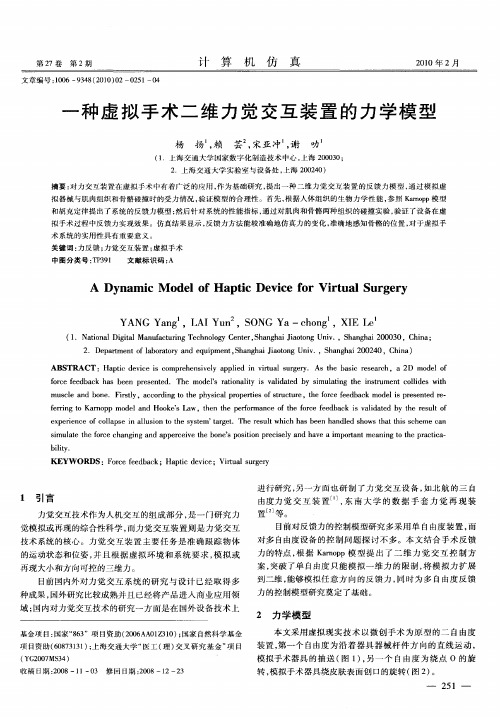
2 eat n fa oa r adeup n,h nhi io n nv , h nhi 0 20 hn ) .D p r met brt y n q i ol o metS ag a J t gU i S aga 2 0 4 ,C ia ao .
ABSTRA CT : Ha tc d v c s o p e e ie y a le n vrua ure y. As t e b sc e e r h, a 2D d l f pi e i e i c m r h nsv l pp id i it ls g r h a i r s a c mo e o
fri g t a n p d la d Ho k ’ L w,t e h e fr n e o e f re  ̄e b c s v l ae y te r s l o e rn K r o p mo e n o eS a o h n te p roma c ft o c h d a k i ai td b h e u t f d
( .N t n l ii l n fc r gT c n l yC n r S a g a Jatn n . h n h i 0 0 0 C ia 1 a o a D gt ua t n e h o g e t , h n h i i o gU i ,S a g a 2 0 3 , hn ; i a Ma u i o e o v
A na i o e f Ha i v c o r ua r e y Dy m c M d lo ptc De i e f r Vit lSu g r
YANG n ,LAIYu , S Ya g n ONG Ya — c o g h n ,XI L E e
blt. i y i
rdmulti 1.1 软件包用户指南:分析 RD 设计中的多个阈值或评分说明书
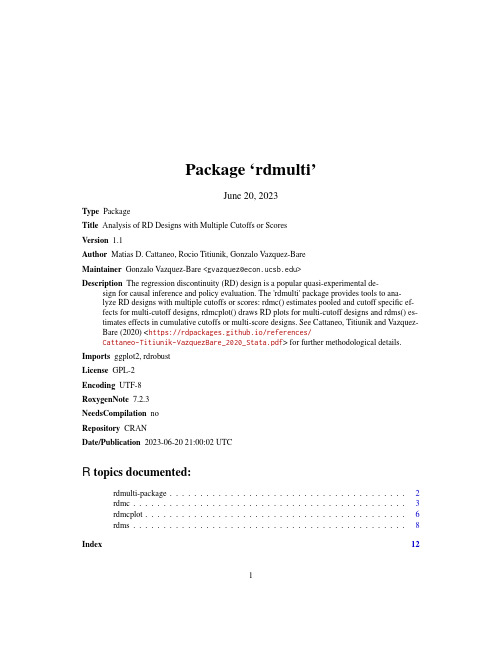
Package‘rdmulti’June20,2023Type PackageTitle Analysis of RD Designs with Multiple Cutoffs or ScoresVersion1.1Author Matias D.Cattaneo,Rocio Titiunik,Gonzalo Vazquez-BareMaintainer Gonzalo Vazquez-Bare<******************.edu>Description The regression discontinuity(RD)design is a popular quasi-experimental de-sign for causal inference and policy evaluation.The'rdmulti'package provides tools to ana-lyze RD designs with multiple cutoffs or scores:rdmc()estimates pooled and cutoff specific ef-fects for multi-cutoff designs,rdmcplot()draws RD plots for multi-cutoff designs and rdms()es-timates effects in cumulative cutoffs or multi-score designs.See Cattaneo,Titiunik and Vazquez-Bare(2020)<https://rdpackages.github.io/references/Cattaneo-Titiunik-VazquezBare_2020_Stata.pdf>for further methodological details. Imports ggplot2,rdrobustLicense GPL-2Encoding UTF-8RoxygenNote7.2.3NeedsCompilation noRepository CRANDate/Publication2023-06-2021:00:02UTCR topics documented:rdmulti-package (2)rdmc (3)rdmcplot (6)rdms (8)Index1212rdmulti-package rdmulti-package rdmulti:analysis of RD Designs with multiple cutoffs or scoresDescriptionThe regression discontinuity(RD)design is a popular quasi-experimental design for causal infer-ence and policy evaluation.The rdmulti package provides tools to analyze RD designs with multiple cutoffs or scores:rdmc()estimates pooled and cutoff-specific effects in multi-cutoff de-signs,rdmcplot()draws RD plots for multi-cutoff RD designs and rdms()estimates effects in cumulative cutoffs or multi-score designs.For more details,and related Stata and R packages useful for analysis of RD designs,visit https://rdpackages.github.io/.Author(s)Matias Cattaneo,Princeton University.<**********************>Rocio Titiunik,Princeton University.<**********************>Gonzalo Vazquez-Bare,UC Santa Barbara.<******************.edu>ReferencesCalonico,S.,M.D.Cattaneo,M.Farrell and R.Titiunik.(2017).rdrobust:Software for Regres-sion Discontinuity Designs.Stata Journal17(2):372-404.Calonico,S.,M.D.Cattaneo,and R.Titiunik.(2014).Robust Data-Driven Inference in the Regression-Discontinuity Design.Stata Journal14(4):909-946.Calonico,S.,M.D.Cattaneo,and R.Titiunik.(2015).rdrobust:An R Package for Robust Non-parametric Inference in Regression-Discontinuity Designs.R Journal7(1):38-51.Cattaneo,M.D.,L.Keele,R.Titiunik and G.Vazquez-Bare.(2016).Interpreting Regression Dis-continuity Designs with Multiple Cutoffs.Journal of Politics78(4):1229-1248.Cattaneo,M.D.,L.Keele,R.Titiunik and G.Vazquez-Bare.(2020).Extrapolating Treatment Effects in Multi-Cutoff Regression Discontinuity Designs.Journal of the American Statistical As-sociation116(536):1941,1952.Cattaneo,M.D.,R.Titiunik and G.Vazquez-Bare.(2020).Analysis of Regression Discontinuity Designs with Multiple Cutoffs or Multiple Scores.Stata Journal20(4):866-891.Keele,L.and R.Titiunik.(2015).Geographic Boundaries as Regression Discontinuities.Political Analysis23(1):127-155rdmc3 rdmc Analysis of RD designs with multiple cutoffsDescriptionrdmc()analyzes RD designs with multiple cutoffs.Usagerdmc(Y,X,C,fuzzy=NULL,derivvec=NULL,pooled_opt=NULL,verbose=FALSE,pvec=NULL,qvec=NULL,hmat=NULL,bmat=NULL,rhovec=NULL,covs_mat=NULL,covs_list=NULL,covs_dropvec=NULL,kernelvec=NULL,weightsvec=NULL,bwselectvec=NULL,scaleparvec=NULL,scaleregulvec=NULL,masspointsvec=NULL,bwcheckvec=NULL,bwrestrictvec=NULL,stdvarsvec=NULL,vcevec=NULL,nnmatchvec=NULL,cluster=NULL,level=95,plot=FALSE,conventional=FALSE)ArgumentsY outcome variable.X running variable.4rdmcC cutoff variable.fuzzy specifies a fuzzy design.See rdrobust()for details.derivvec vector of cutoff-specific order of derivatives.See rdrobust()for details.pooled_opt options to be passed to rdrobust()to calculate pooled estimand.verbose displays the output from rdrobust for estimating the pooled estimand.pvec vector of cutoff-specific polynomial orders.See rdrobust()for details.qvec vector of cutoff-specific polynomial orders for bias estimation.See rdrobust()for details.hmat matrix of cutoff-specific bandwidths.See rdrobust()for details.bmat matrix of cutoff-specific bandwidths for bias estimation.See rdrobust()fordetails.rhovec vector of cutoff-specific values of rho.See rdrobust()for details.covs_mat matrix of covariates.See rdrobust()for details.covs_list list of covariates to be used in each cutoff.covs_dropvec vector indicating whether collinear covariates should be dropped at each cutoff.See rdrobust()for details.kernelvec vector of cutoff-specific kernels.See rdrobust()for details.weightsvec vector of length equal to the number of cutoffs indicating the names of the vari-ables to be used as weights in each cutoff.See rdrobust()for details.bwselectvec vector of cutoff-specific bandwidth selection methods.See rdrobust()for de-tails.scaleparvec vector of cutoff-specific scale parameters.See rdrobust()for details.scaleregulvec vector of cutoff-specific scale regularization parameters.See rdrobust()fordetails.masspointsvec vector indicating how to handle repeated values at each cutoff.See rdrobust()for details.bwcheckvec vector indicating the value of bwcheck at each cutoff.See rdrobust()for de-tails.bwrestrictvec vector indicating whether computed bandwidths are restricted to the range orrunvar at each cutoff.See rdrobust()for details.stdvarsvec vector indicating whether variables are standardized at each cutoff.See rdrobust() for details.vcevec vector of cutoff-specific variance-covariance estimation methods.See rdrobust() for details.nnmatchvec vector of cutoff-specific nearest neighbors for variance estimation.See rdrobust() for details.cluster cluster ID variable.See rdrobust()for details.level confidence level for confidence intervals.See rdrobust()for details.plot plots cutoff-specific estimates and weights.conventional reports conventional,instead of robust-bias corrected,p-values and confidenceintervals.rdmc5Valuetau pooled estimatese.rb robust bias corrected standard error for pooled estimatepv.rb robust bias corrected p-value for pooled estimateci.rb.l left limit of robust bias corrected CI for pooled estimateci.rb.r right limit of robust bias corrected CI for pooled estimatehl bandwidth to the left of the cutoff for pooled estimatehr bandwidth to the right of the cutofffor pooled estimateNhl sample size within bandwidth to the left of the cutoff for pooled estimateNhr sample size within bandwidth to the right of the cutoff for pooled estimateB vector of bias-corrected estimatesV vector of robust variances of the estimatesCoefs vector of conventional estimatesW vector of weights for each cutoff-specific estimateNh vector of sample sizes within bandwidthCI robust bias-corrected confidence intervalsH matrix of bandwidthsPv vector of robust p-valuesrdrobust.resultsresults from rdrobust for pooled estimatecfail Cutoffs where rdrobust()encountered problemsAuthor(s)Matias Cattaneo,Princeton University.<**********************>Rocio Titiunik,Princeton University.<**********************>Gonzalo Vazquez-Bare,UC Santa Barbara.<******************.edu>ReferencesCattaneo,M.D.,R.Titiunik and G.Vazquez-Bare.(2020).Analysis of Regression Discontinuity Designs with Multiple Cutoffs or Multiple Scores.Stata Journal,forthcoming.Examples#Toy datasetX<-runif(1000,0,100)C<-c(rep(33,500),rep(66,500))Y<-(1+X+(X>=C))*(C==33)+(.5+.5*X+.8*(X>=C))*(C==66)+rnorm(1000)#rdmc with standard syntaxtmp<-rdmc(Y,X,C)rdmcplot RD plots with multiple cutoffs.Descriptionrdmcplot()RD plots with multiple cutoffs.Usagerdmcplot(Y,X,C,nbinsmat=NULL,binselectvec=NULL,scalevec=NULL,supportmat=NULL,pvec=NULL,hmat=NULL,kernelvec=NULL,weightsvec=NULL,covs_mat=NULL,covs_list=NULL,covs_evalvec=NULL,covs_dropvec=NULL,ci=NULL,col_bins=NULL,pch_bins=NULL,col_poly=NULL,lty_poly=NULL,col_xline=NULL,lty_xline=NULL,nobins=FALSE,nopoly=FALSE,noxline=FALSE,nodraw=FALSE)ArgumentsY outcome variable.X running variable.C cutoff variable.nbinsmat matrix of cutoff-specific number of bins.See rdplot()for details.binselectvec vector of cutoff-specific bins selection method.See rdplot()for details.scalevec vector of cutoff-specific scale factors.See rdplot()for details.supportmat matrix of cutoff-specific support conditions.See rdplot()for details..pvec vector of cutoff-specific polynomial orders.See rdplot()for details.hmat matrix of cutoff-specific bandwidths.See rdplot()for details.kernelvec vector of cutoff-specific kernels.See rdplot()for details.weightsvec vector of cutoff-specific weights.See rdplot()for details.covs_mat matrix of covariates.See rdplot()for details.covs_list list of of covariates to be used in each cutoff.covs_evalvec vector indicating the evaluation point for additional covariates.See rdrobust() for details.covs_dropvec vector indicating whether collinear covariates should be dropped at each cutoff.See rdrobust()for details.ci adds confidence intervals of the specified level to the plot.See rdrobust()for details.col_bins vector of colors for bins.pch_bins vector of characters(pch)type for bins.col_poly vector of colors for polynomial curves.lty_poly vector of lty for polynomial curves.col_xline vector of colors for vertical lines.lty_xline vector of lty for vertical lines.nobins omits bins plot.nopoly omits polynomial curve plot.noxline omits vertical lines indicating the cutoffs.nodraw omits plot.Valueclist list of cutoffscnum number of cutoffsX0matrix of X values for control unitsX1matrix of X values for treated unitsYhat0estimated polynomial for control unitsYhat1estimated polynomial for treated unitsXmean bin average of X valuesYmean bin average for Y valuesCI_l lower end of confidence intervalsCI_r upper end of confidence intervalscfail Cutoffs where rdrobust()encountered problems8rdmsAuthor(s)Matias Cattaneo,Princeton University.<**********************>Rocio Titiunik,Princeton University.<**********************>Gonzalo Vazquez-Bare,UC Santa Barbara.<******************.edu>ReferencesCattaneo,M.D.,R.Titiunik and G.Vazquez-Bare.(2020).Analysis of Regression Discontinuity Designs with Multiple Cutoffs or Multiple Scores.Stata Journal,forthcoming.Examples#Toy datasetX<-runif(1000,0,100)C<-c(rep(33,500),rep(66,500))Y<-(1+X+(X>=C))*(C==33)+(.5+.5*X+.8*(X>=C))*(C==66)+rnorm(1000)#rdmcplot with standard syntaxtmp<-rdmcplot(Y,X,C)rdms Analysis of RD designs with cumulative cutoffs or two running vari-ablesDescriptionrdms()analyzes RD designs with cumulative cutoffs or two running variables.Usagerdms(Y,X,C,X2=NULL,zvar=NULL,C2=NULL,rangemat=NULL,xnorm=NULL,fuzzy=NULL,derivvec=NULL,pooled_opt=NULL,pvec=NULL,qvec=NULL,hmat=NULL,bmat=NULL,rdms9 rhovec=NULL,covs_mat=NULL,covs_list=NULL,covs_dropvec=NULL,kernelvec=NULL,weightsvec=NULL,bwselectvec=NULL,scaleparvec=NULL,scaleregulvec=NULL,masspointsvec=NULL,bwcheckvec=NULL,bwrestrictvec=NULL,stdvarsvec=NULL,vcevec=NULL,nnmatchvec=NULL,cluster=NULL,level=95,plot=FALSE,conventional=FALSE)ArgumentsY outcome variable.X running variable.C vector of cutoffs.X2if specified,second running variable.zvar if X2is specified,treatment indicator.C2if specified,second vector of cutoffs.rangemat matrix of cutoff-specific ranges for the running variable.xnorm normalized running variable to estimate pooled effect.fuzzy specifies a fuzzy design.See rdrobust()for details.derivvec vector of cutoff-specific order of derivatives.See rdrobust()for details.pooled_opt options to be passed to rdrobust()to calculate pooled estimand.pvec vector of cutoff-specific polynomial orders.See rdrobust()for details.qvec vector of cutoff-specific polynomial orders for bias estimation.See rdrobust() for details.hmat matrix of cutoff-specific bandwidths.See rdrobust()for details.bmat matrix of cutoff-specific bandwidths for bias estimation.See rdrobust()for details.rhovec vector of cutoff-specific values of rho.See rdrobust()for details.covs_mat matrix of covariates.See rdplot()for details.covs_list list of of covariates to be used in each cutoff.10rdms covs_dropvec vector indicating whether collinear covariates should be dropped at each cutoff.See rdrobust()for details.kernelvec vector of cutoff-specific kernels.See rdrobust()for details.weightsvec vector of length equal to the number of cutoffs indicating the names of the vari-ables to be used as weights in each cutoff.See rdrobust()for details.bwselectvec vector of cutoff-specific bandwidth selection methods.See rdrobust()for de-tails.scaleparvec vector of cutoff-specific scale parameters.See rdrobust()for details.scaleregulvec vector of cutoff-specific scale regularization parameters.See rdrobust()fordetails.masspointsvec vector indicating how to handle repeated values at each cutoff.See rdrobust()for details.bwcheckvec vector indicating the value of bwcheck at each cutoff.See rdrobust()for de-tails.bwrestrictvec vector indicating whether computed bandwidths are restricted to the range orrunvar at each cutoff.See rdrobust()for details.stdvarsvec vector indicating whether variables are standardized at each cutoff.See rdrobust() for details.vcevec vector of cutoff-specific variance-covariance estimation methods.See rdrobust() for details.nnmatchvec vector of cutoff-specific nearest neighbors for variance estimation.See rdrobust() for details.cluster cluster ID variable.See rdrobust()for details.level confidence level for confidence intervals.See rdrobust()for details.plot plots cutoff-specific and pooled estimates.conventional reports conventional,instead of robust-bias corrected,p-values and confidenceintervals.ValueB vector of bias-corrected coefficientsV variance-covariance matrix of the estimatorsCoefs vector of conventional coefficientsNh vector of sample sizes within bandwidth at each cutoffCI bias corrected confidence intervalsH bandwidth used at each cutoffPv vector of robust p-valuesAuthor(s)Matias Cattaneo,Princeton University.<**********************>Rocio Titiunik,Princeton University.<**********************>Gonzalo Vazquez-Bare,UC Santa Barbara.<******************.edu>rdms11ReferencesCattaneo,M.D.,R.Titiunik and G.Vazquez-Bare.(2020).Analysis of Regression Discontinuity Designs with Multiple Cutoffs or Multiple Scores.Stata Journal,forthcoming.Examples#Toy dataset:cumulative cutoffsX<-runif(1000,0,100)C<-c(33,66)Y<-(1+X)*(X<C[1])+(0.8+0.8*X)*(X>=C[1]&X<C[2])+(1.2+1.2*X)*(X>=C[2])+rnorm(1000) #rmds:basic syntaxtmp<-rdms(Y,X,C)Index_PACKAGE(rdmulti-package),2rdmc,2,3rdmcplot,2,6rdms,2,8rdmulti-package,2rdmulti_package(rdmulti-package),212。
基于小波极限学习机的分解炉温控系统建模
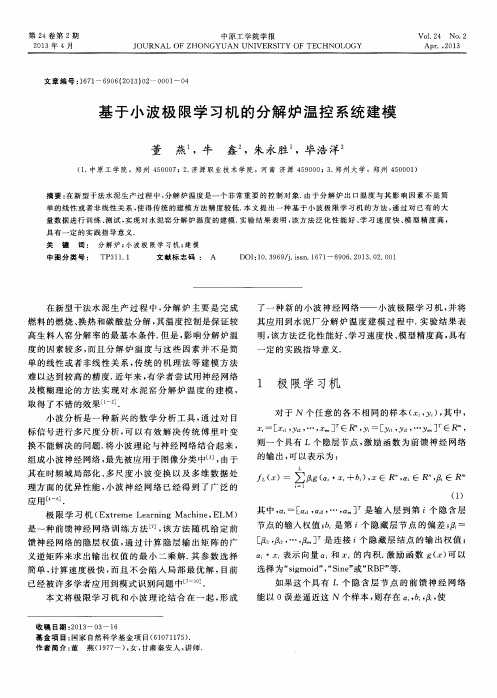
如果 这个具 有 L 个 隐 含 层 节 点 的前 馈 神 经 网络
能 以 0误 差逼 近这 N 个 样本 , 则存 在 a , b , , 使
收 稿 日期 : 2 0 1 3 —0 3 —1 6 基金项 目: 国家 自然 科 学 基 金 项 目( 6 1 0 7 1 1 7 5 ) .
摘要 : 在新型干法水泥生产过程 中, 分 解 炉 温 度 是 一 个 非 常 重 要 的控 制 对 象 . 由 于 分 解 炉 出 口温 度 与 其 影 响 因 素 不 是 简
单的线性或者非线性关 系 , 使得传统的建模方法精度较低. 本 文 提 出 一 种 基 于 小 波 极 限 学 习机 的 方 法 , 通 过 对 已 有 的大
一
标信 号进 行多 尺度 分 析 , 可 以有效 解 决 传 统 傅 里 叶 变
换不 能解 决 的 问题 . 将 小波理 论 与神经 网络结合 起来 ,
I x 1 , Y 2 , …, z ] ∈R , Y 一E y 1 , Y 2 , …Y ] ∈R ,
基 于小 波极 限 学 习机 的分 解 炉 温 控 系统 建 模
董 燕 ,牛 鑫 ,朱 永 胜 ,毕 浩 洋。
( 1 . 中原 工 学 院 ,郑 州 4 5 0 0 0 7 ;2 . 济 源 职 业 技 术 学 院 ,河 南 济 源 4 5 9 0 0 0 ; 3 . 郑州 大 学 ,郑 州 4 5 0 0 0 1 )
了一种 新 的 小波 神 经 网络—— 小 波 极 限学 习 机 , 并将
其应 用到 水泥 厂分 解 炉温 度 建 模 过程 中. 实 验 结 果表
明, 该方法 泛化 性能 好 、 学 习速 度快 、 模型 精度 高 , 具有
非数字,非物理——南加州建筑学院机器人工作室
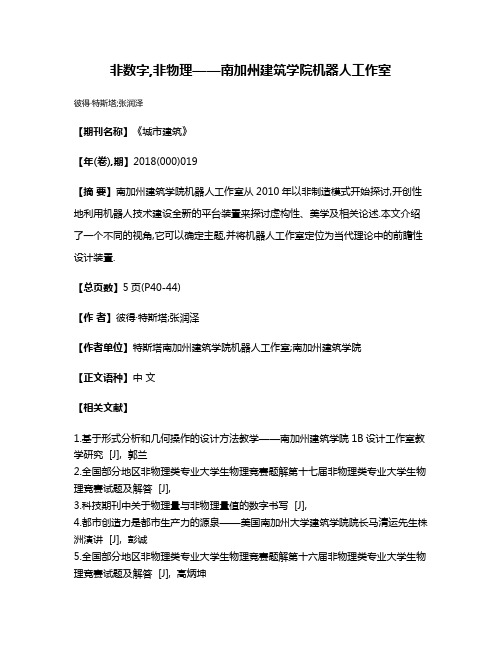
非数字,非物理——南加州建筑学院机器人工作室
彼得·特斯塔;张润泽
【期刊名称】《城市建筑》
【年(卷),期】2018(000)019
【摘要】南加州建筑学院机器人工作室从2010年以非制造模式开始探讨,开创性地利用机器人技术建设全新的平台装置来探讨虚构性、美学及相关论述.本文介绍了一个不同的视角,它可以确定主题,并将机器人工作室定位为当代理论中的前瞻性设计装置.
【总页数】5页(P40-44)
【作者】彼得·特斯塔;张润泽
【作者单位】特斯塔南加州建筑学院机器人工作室;南加州建筑学院
【正文语种】中文
【相关文献】
1.基于形式分析和几何操作的设计方法教学——南加州建筑学院1B设计工作室教学研究 [J], 郭兰
2.全国部分地区非物理类专业大学生物理竞赛题解第十七届非物理类专业大学生物理竞赛试题及解答 [J],
3.科技期刊中关于物理量与非物理量值的数字书写 [J],
4.都市创造力是都市生产力的源泉——美国南加州大学建筑学院院长马清运先生株洲演讲 [J], 彭诚
5.全国部分地区非物理类专业大学生物理竞赛题解第十六届非物理类专业大学生物理竞赛试题及解答 [J], 高炳坤
因版权原因,仅展示原文概要,查看原文内容请购买。
基于Robei EDA工具的多功能可重构机器人设计
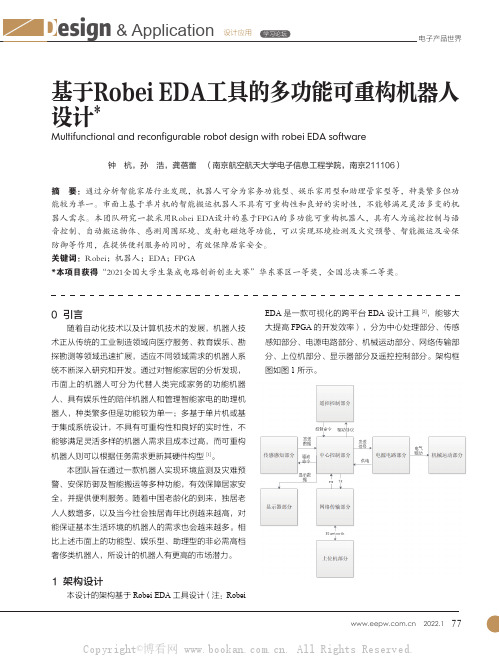
5 图像处理
图像处理功能由 CCD 基于 FPGA 实现,主要有两
种功能:轨迹识别及显示、复杂循迹。以下将详细介绍
各个功能的实现。
5.1 轨迹识别及显示
传感系统中的 CCD 模块使用 FPGA 实现,本功能
基于 FPGA 驱动 TS1401 线性 CCD 采集轨迹信息二值
化后输出至上位机,以显示轨迹。
4 算法系统
4.1 机器人运动算法 本作品设计的机器人运动部分采用 4WD 的麦克纳
姆轮,每个麦克纳姆轮上有若干倾斜 45° 的小轮子,在 转动时产生相比机器人倾斜 45° 的摩擦力,4 个轮胎转 动配合即可实现平移、原地旋转等运动。其运动原理如 图 5 所示,其中红色代表轮子向前转,蓝色代表轮子向 后转。
Design & Application 设计应用 学习论坛
电子产品世界
基于Robei EDA工具的多功能可重构机器人 设计*
Multifunctional and reconfigurable robot design with robei EDA software
钟 杭,孙 浩,龚蓓蕾 (南京航空航天大学电子信息工程学院,南京211106)
机器人轮胎选用麦克纳姆轮。由于独特的机械结构, 4 个麦克纳姆轮通过矢量相加可实现机器人平移、原地 旋转等特殊运动。 3.4 电磁炮部分
该部分主要包含逆变升压模块、继电器模块、超级 电容模块和电磁线圈模块。
首先从继电器模块中引出两根信号线给 FPGA, FPGA 可根据手柄和语音控制继电器模块闭合张开以控 制 12 V 电源,通过逆变升压模块给超级电容充电,然 后控制继电器将 12 V 电源断开,此时电能被存储在超 级电容之中。然后再次由 FPGA 控制电磁线圈闭合,使 电能从电容中释放,此时变化的电流通过线圈生成磁场。 根据法拉第电磁感应定律和楞次定律,线圈中将产生排 斥方向的洛伦兹力带动炮管中的金属弹射出。为了保护 电路和隔离,使用可控硅和续流二极管等器件。
Optimal Designs for Mixed-Effects Models with Random Nested Factors

Optimal Designs for Mixed-Effects Models with Random Nested FactorsB RUCE E. A NKENMAN, ankenman@Phone: (847) 491-5674; Fax: (847) 491-8005A NA I VELISSE A VILES, ivelisse@Northwestern UniversityDepartment of Industrial Engineering and Management Sciences2145 Sheridan Rd. MEAS C210, Evanston, IL 60208J OSE C. P INHEIRO, jcp@Bell Laboratories, Lucent Technologies600 Mountain Ave. Room 2C-258, Murray Hill, NJ 07974ABSTRACTThe problem of experimental design for the purpose of estimating the fixed effects and the variance components corresponding to random nested factors is a widely applicable problem in industry. Random nested factors arise from quantity designations such as lot or batch and from sampling and measurement procedures. We introduce a new class of designs, called assembled designs, where all the nested factors are nested under the treatment combinations of the crossed factors. We provide parameters and notation for describing and enumerating assembled designs. Using maximum likelihood estimation and the D-optimality criterion, we show that, for most practical situations, designs that are as balanced as possible are optimal for estimating both fixed effects and variance components in a mixed-effects model.KEYWORDS: Assembled Designs, Crossed and Nested Factors, D-Optimality, Experimental Design, Fixed and Random Effects, Hierarchical Nested Design, Maximum Likelihood, Nested Factorials, Variance Components.1. IntroductionIn many experimental settings, different types of factors affect the measured response. The factors of primary interest can usually be set independently of each other and thus are called crossed factors. For example, crossed factors are factors like temperature and pressure where each level of temperature can be applied independently of the level of pressure. These effects are often modeled as fixed effects. Nested factors cannot be set independently because the level of one factor takes on a different meaning when other factors are changed. Random nested factors arise from quantity designations such as lot or batch and from sampling and measurement procedures that are often inherent in the experimentation. The variances of the random effects associated with nested factors are called variance components since they are components of the random variation of the response. Batch-to-batch variation and sample-to-sample variation are examples of variance components.The nested or hierarchical nested design (HND), which is used in many sampling and testing situations, is a design where the levels of each factor are nested within the levels of another factor. Balanced HNDs for estimating variance components have an equal number of observations for each branch at a given level of nesting. Not only do these designs tend to require a large number of observations, but they also tend to produce precise estimates of certain variance components and poor estimates of others. Some articles that address these issues are Bainbridge (1965) and, more recently, Smith and Beverly (1981) and Naik and Khattree (1998). These articles use unbalanced HNDs, called staggered nested designs, to spread the information in the experiment more equally among the variance components. A staggered nested design only branches once at eachlevel of nesting and, thus, there is only one degree of freedom for estimating each variance component. Of course, if a staggered nested design is replicated n times then each variance component will have n degrees of freedom for estimation. Goldsmith and Gaylor (1970) address the optimality of unbalanced HNDs. Delgado and Iyer (1999) extend this work to obtain nearly optimal HNDs for the estimation of variance components using a limit argument combined with numerical optimization.When the fixed effects of crossed factors and variance components from nested random factors appear in the same model, there are many analysis techniques available for estimation and inference (see Searle, Casella, and McCulloch, 1992; Khuri, Matthew, and Sinha, 1998; or Pinheiro and Bates, 2000). However, only limited work has been done to determine what experimental designs should be used when both crossed factors and variance components occur in a single experiment. Smith and Beverly (1981) introduced the idea of a nested factorial, which is an experimental design where some factors appear in factorial relationships and others in nested relationships. They propose placing staggered nested designs at each treatment combination of a crossed factorial design and called the resulting designs staggered nested factorials.Ankenman, Liu, Karr, and Picka (1998) introduced what they call split factorials, which split a fractional factorial design into subexperiments. A different nested design is used for each subexperiment, but within a subexperiment all design points have the same nested design. The nested designs in a split factorial only branch at a single level and thus, the effect is to study a different variance component in each subexperiment.The general problem of experimental design for the purpose of estimating both crossed factor effects and variance components is a quite broad and widely applicable problem in industry. Some examples could be:1)Chemical or pharmaceutical production where certain reactor settings or catalysts are the crossed factors and raw material lot-to-lot variation, reactor batch-to-batch variation, and sample-to-sample variation are the variance components. In this case, knowing the size of the variance components may help to determine the most economical size for a batch.2)A molding process where machine settings such as mold zone temperatures or mold timings are the crossed factors and shift-to-shift variation, part-to-part variation, and measurement-to-measurement variation are the variance components. Knowing which variation source is largest could help to focus quality improvement efforts.3)Concrete mixing where recipe factors such as the size of the aggregate used in the concrete and the ratio of water to cement powder are crossed factors and batch-to-batch variation and sample-to-sample variation are the variance components. Knowing about the variance components can help engineers to understand how variation in the properties of the concrete will change throughout large concrete structures.The purpose of this paper is to provide experimental design procedures for the estimation of the fixed effects of crossed factors as well as variance components associated with nested factors that arise from sampling and measurement procedures. We introduce a special class of nested factorials, called assembled designs, where all the nested factors are random and nested under the treatment combinations of the crossed factors. The class of assembled designs includes both the split factorials and thestaggered nested factorial designs. In Section 2, we provide parameters and notation for describing and enumerating assembled designs. In Section 3, we describe a linear mixed-effects model, which can be used to analyze assembled designs. The fixed effects and the variance components are estimated using maximum likelihood (ML). We present expressions for the information matrix for the fixed effects and the variance components in assembled designs with two variance components in Section 4. In Section 5, we provide theorems which show that under most practical situations, the design which is the most balanced (i.e., spreading the observations as uniformly as possible between the branches in the nested designs) is D-optimal for estimating both fixed effects and two variance components. In Section 6, we show with examples how to obtain the D-optimal design for the requirements of an experiment. Conclusions and discussion are then presented in Section 7.2. Assembled DesignsAn assembled design is a crossed factor design that has an HND placed at each design point of the crossed factor design. If the assembled design has the same number of observations at each design point, it can be described by the following parameters: r , the number of design points in the crossed factor design; n , the number of observations at each design point; q , the number of variance components; and s , the number of different HNDs used. For the special case of only two variance components (q =2), we will use the terms batch and sample to refer to the higher and lower level of random effects,respectively. Also for this case, we define B T as the total number of batches; B j as the number of batches in the j th HND; and r j as the number of design points that contain the j th HND. Thus, T sj j j B B r =∑=1We will use a simplified version of the concrete experiment in Jaiswal, Picka, Igusa,Karr, Shah, Ankenman, and Styer (2000) to illustrate the concepts of and introduce the notation for assembled designs. The objective of the concrete experiment is to determine the effects of certain crossed factors on the permeability of concrete and the variability of the permeability from batch-to-batch and from sample-to-sample. The design has three two-level factors (Aggregate Grade, Water to Cement (W/C) Ratio, and Max Size of Aggregate) and two variance components (q=2), Batch and Sample. The design (Figure1) has a total of 20 batches (B T =20).In Figure 1, each vertex of the cube represents one of the eight possible concrete recipes or design points (r=8) that can be made using the two levels of Grade, W/C Ratio,and Max Size. Thus, the front upper left-hand vertex represents a concrete recipe with the low level of Grade, the low level of W/C Ratio, and the high level of Max Size. The branching structure coming from each vertex represents batches and test samples to be made from that recipe. There are four samples per recipe (n=4).In the context of an assembled design, HNDs that are attached to the crossed factor design points will be referred to as structures . In the concrete experiment, there are twoFigure 1: An Assembled Design (B T = 20, r = 8, q = 2, n = 4, s = 2).Grade W/C RatioMax SizeLevel 2Level 2Level 2Level 1Level 1Level 1Guide to StructuresStructure 1Structure 2different structures (s=2). Structure 1 consists of three batches (B 1=3) where two samples are cast from one of the batches and one sample is cast from each of the other two batches. Structure 1 appears at four of the design points, so r 1=4. Structure 2appears at the other four design points (r 2=4) and consists of two batches (B 2=2) and two samples cast from each batch.In order to compare all possible assembled designs with the same parameter values,we will begin by discussing what structures are available for building the assembled designs. Let N represent the number of structures available for a given n and let k be the number of batches. The number of structures available for given n and k , with two variance components (q =2) is∑∑∑∑∑ −−++−=++−= = −−= −−−=−−−−−−=)3()(2)(11231322121111221231k k i i n i i i i n i i k n i k i n i i k i i n i i k k k k k k k N ,where x called floor[x ], gives the greatest integer less than or equal to x . The total number of structures is then, ∑==nk k N N 1. Figure 2 shows the number of possible structures that are available for use in assembled designs for various values of n and q =2and provides some examples.In a structure, the total number of degrees of freedom is equal to the number of observations and each degree of freedom can be thought of as a piece of information with a cost equal to that of a single observation. For a single structure, the degrees of freedom available for estimating the first variance component equals its number of branches minus one. More generally, the degrees of freedom associated with the i th nested random factor equals the number of branches at the i th level of nesting minus the number of branches at the (i -1)st level of nesting. Figure 3 shows an example of calculations for degrees offreedom for an HND with q =4. (Note that an HND can also be thought of as an assembled design with one structure and one design point.)The notation for a single q -level structure consists of q –1 different sets of parentheses/brackets. The i th nested random factor in a q-stage hierarchical design (i =1,…,q -1) is represented by a specific set of parentheses including as many elements as the number of i th nested random factor branches. For the q th variance component, the number of observations at the last level of nesting is specified. For uniqueness of equivalent nested designs, the elements at the last level of nesting as well as the number of elements at the i th level of nesting, i =1,…,q -1, are specified in descending order,starting from the q th level of nesting and ending with the first level of nesting.Notation can be understood easier by examples. Figure 4 shows the notation for the two structures in the concrete experiment. Structure 1 has three batches and thus has notation (2,1,1), where each element refers to the number of samples cast from eachFigure 3: Degrees of Freedom for an HND (q =4).22334557611n# of Structures Structures 715All available structures Some examplesFigure 2: The Number of Possible Structures (HNDs) for n =[2,7] and q =2. EUDQFKHV/ / / /batch. Structure 2 consists of two batches and two samples cast from each batch and thus has notation (2,2). Note that for q=2, there is just one set of parenthesis. Figure 5 shows an example of notation for a structure with four variance components.Figure 2 shows that as n increases, there are more available structures and it follows that, for a given number of design points, r , there are more potential assembled designs which have the same number of observations at each design point (n ).Building on the notation used for individual structures, the notation for an assembled design is ,} structure with points @{design structure 1∑=sj j j where the design points need to be ordered in some way. For assembled designs, we order the design points so that all rows with the same structure are in adjacent rows. This order is called design order .Recall that r j is the number of design points with structure j . The notation in design order would be ∑=−−++s j j j j R ,R R j 111},,21{@ structure , where ∑==jh h j r R 1 and .00=R Figure 6 shows that structures in the concrete experiment were assigned to the design points using the interaction ABC and it also shows the comparison of design order and standard order (see Myers and Montgomery 1995, p. 84) for a two-level factorial. TheseFigure 5: Notation for HND (q = 4).Figure 4: Concrete Experiment; Notation for HND (q =2).Notation for this Structure:{[(2,2,1),(3),(1)],[(3,2,2),(2,1)]}n =19lot batch samplemeasurement q =4(2,2)(2,1,1)batch ; sampleStructure 1Structure 2orderings are for convenience in describing the experiment and in manipulating the expressions of the model and analysis. When conducting the experiment, the order of observations should be randomly determined whenever possible.In an assembled design, the number of degrees of freedom is equal to the total number of observations. Thus, an assembled design with nr observations has a total of nr degrees of freedom. There are r degrees of freedom for estimating the fixed effects including the constant. There are nr -r degrees of freedom left for estimating variance components. We will designate the number of degrees of freedom for estimating the i th variance component as d i . When q=2, ()r B r B r B r d T sj j s j j j s j j j −=−=−=∑∑∑===11111 and d 2=nr-d 1-r=nr-B T .3. Analysis of Assembled Designs3.1. Model and Variance StructureThe linear mixed-effects model used to represent the response in an assembled design with nr observations and q variance components is,∑=+=q i i i 1u Z X y ,(1)Figure 6: Concrete Experiment (A=Grade, B=W/C Ratio, and C=Max Size);Comparison of Standard Order and Design Order.D esignO rder S ta ndard O rderA B C A B C S tructure11----24++--36+-+-47-++-52+--+63-+-+75--++88++++,5,8}(2,2)@{2,3,4,6,7}(2,1,1)@{1 :Order Standard in Notation ,7,8}(2,2)@{5,6,2,3,4}(2,1,1)@{1 :Order Design in Notation ++where y is a vector of nr observations, X is the fixed-effects design matrix, is a vector of r unknown coefficients including the constant term, Z i is an indicator matrix associated with the i th variance component, u i is a vector of normally distributed independent random effects associated with the i th variance component such that ()I 0u 2 i i N a . Let V be the nr ×nr variance-covariance matrix of the observations.Assume that the variance components do not depend on the crossed factor levels. Then,∑=′==qi i i i Var 12 Z Z y V .(2)Let X D be the full rank r r × design matrix (including the constant column) for a single replicate of a crossed factor design, where rows are ordered in design order. Also let the observations in X be ordered such that X =X D §1n , where 1n is an n -length vector of ones and § represents the Kronecker product. This ordering in X gives rise to Z-matrices that have the form ,1it rt i Z Z ⊕== where ⊕ refers to the Kronecker sum and Z it isthe indicator matrix related to the observations associated with variance component i for the treatment combination t . For the fixed effects, X t is the portion of the X matrix associated with the t th treatment combination. X t is a n ×r matrix where all n rows are identical. Let t D X ′ represent the row of X D corresponding to the t th treatment combination, then X t = t D X ′§1n . Let V t be the n ×n variance-covariance matrix associated with the treatment combination t , then , 12∑=′=qi it it i t Z Z V which relates to (2). Thus, Vcan be written as: .1t rt V V ⊕==Consider the case of two variance components (q =2) and denote by 21σ the batchvariance and by 22σ the sample variance. Z 2, the sample indicator matrix, is the identity matrix of order nr . Z 1t has n rows and as many columns as the number of batches used with treatment combination t . Z 1, the batch indicator matrix for an assembled design, has as many rows as total number of samples (nr ) and as many columns as total number of batches (B T ). As an example, recall that in the concrete experiment (introduced in Section 2) there are eight design points (r =8), two structures (s =2), and four observations at each design point (n =4). Recall that Structure 1 is (2,1,1) and Structure 2 is (2,2).Thus, based on treatment combinations: Z 2=I 32, Z 2t =I 4, t =1,2, (8). and ,10100101,100010001001181716151413121111817161514131211=========Z 00Z 00000000Z 00000000Z 0000000Z 0000000Z 00000000Z 00000000Z Z Z Z Z Z Z Z Z Z 3.2. Analysis TechniquesDifferent estimation methods have been proposed for the analysis of linear mixed-effects models. Currently, the two most commonly used methods are maximum likelihood (ML) and restricted maximum likelihood (REML). The method of maximum likelihood prescribes the maximization of the likelihood function over the parameter space, conditional on the observed data. Such optimization involves iterative numerical methods, which only became widely applicable with recent advances in computer technology. REML estimation is effectively ML estimation based on the likelihood of the ordinary least-squares residuals of the response vector y regressed on the X matrix ofthe fixed effects. Because it takes into account the loss of degrees of freedom due to the estimation of the fixed effects, REML estimates of variance components tend to be less biased than the corresponding ML estimates. In this paper, we will use ML estimation for the fixed effects and variance components, because of the greater simplicity of the associated asymptotic variance-covariance matrices (which are used to determine the D-optimal designs). Since ML and REML estimators are asymptotically equivalent, we expect that the D-optimal designs for ML will be at least close to optimal for REML.Conditional on the variance components, the estimation of the fixed effects is a generalized least squares (GLS) problem, with solution ()y V X X V X111ˆ−−−′′= (see Thiel, 1971, p. 238 for further details). In general, the variance components need to be estimated from the data, in which case the GLS methodology becomes equivalent to ML.It follows from the model assumptions that, conditional on the variance components,the variance-covariance matrix of the fixed-effects estimators is ().11−−′X V X In practice,the unknown variance components are replaced by their ML estimates. In this case,under the assumption of normality, the variance-covariance matrix ofˆ is the inverse of the information matrix corresponding to (see Searle, Casella, and McCulloch, 1992, p.252-254). Because of the independence of the observations at different treatmentcombinations, ,)()(11∑=−=′=rt t Inf Inf X V X where t t t t Inf X V X 1)(−′=. It thenfollows that ()()()()∑∑=−=−′⊗′=⊗′⊗′⊗=rt n t n t t rt n ttn t Inf 11111)(1V 1X X 1X V 1X D D D D .Since n t n 1V 11−′ is a scalar,.)(11∑=−′′=rt t t n t n Inf D D X X 1V 1 (3)There are only asymptotic results available for the variance-covariance matrix of the variance component estimators. Denoting the vector of ML estimators by 2ˆσ, the asymptotic variance-covariance matrix of q variance-components estimators for an assembled design is (see Searle, Casella, and McCulloch, 1992, p. 253):()()()()()111111111111111112222122ˆˆˆˆ−−−−−−−−−′′′′′′′′≈=qq q q q q q q q tr tr tr tr Var Var Z Z V Z Z V Z Z V Z Z V Z Z V Z Z V Z Z V Z Z Vσσσ,where tr () indicates the trace function of a matrix. The information matrix of the variance components for treatment combination t is()()()()′′′′′′′′=−−−−−−−−qt qt t qt qt t t t t qt qt t qt qt t t t t t t t t t t t tr tr tr tr Inf Z Z V Z Z V Z Z V Z Z V Z Z V Z Z V Z Z V Z Z V 1111111111111111221)( .The information matrix of variance-components estimators is ∑==rt t Inf Inf 122)()( .4. Information Matrix for Two Variance ComponentsAssembled designs are a very large class of designs, many of which are too complicated for practical use. Since the most likely assembled designs to be used in practice are the most simple, we will study assembled designs for two variance components (q =2) in detail. Detailed study of assembled designs with more than two variance components is left for future research.The simplest assembled design with r =1 design point and s =1 structure is equivalent to an HND. Hence, it is fundamental to study a single HND or the j th structure. For notational simplification, we will use B instead of j B to represent the number of batches in a structure for the case of q =2, r =1, and s =1. Using the notation established in Section3 for HNDs, any structure with B batches and q =2 levels of nesting can be represented by()B B m m m m ,,,,121− , where i m is the number of samples in batch i and 1+≥i im m .To develop the information matrix for fixed effects at a single design point, the expressions in terms of m i ’s for a treatment combination t are:[]i i m m Bi nt t t t t t t I J I Z Z Z Z Z Z V 2221122112122221121+=+′=′+′=⊕=,where J n is an n ×n square matrix with all elements equal to one. Note that for q =2,n t t I Z Z =′22. Also,++−=⊕=−i i m m i Bi tm I J V 2221222221111) ( and from (3), it can be shown that the information for fixed-effects estimator (in this case just the mean) of a structure at a design point given n , B , and q =2 is∑∑==−−+=++−=′′=′=B i i i Bi i i i tt n t n t t t t m mm m m Inf 1212212221222222111. ) ( ) (D D X X 1V 1X V X (4)Note that for a single design point (r =1), X D t =1, thus and ) (t Inf are scalars.The asymptotic results for the variance-covariance matrix of the variance-components estimators for ML (q =2) are:()()()()()()()()1211121112121112)(2ˆ−−−−−′′′≈tt t t t t tt tt t tr tr tr tr Var V Z Z V Z Z V Z Z V σand()()()()()()()()()+−+−++++=′′′=∑∑∑∑====−−−−Bi i i i i B i i i Bi iiB i i i t t t t t t t t t t t m m m m m m m m m m tr tr tr tr Inf 1222121212242211121112121112)1()1())1(1()1()1()1(21)(21ττττττσV Z Z V Z Z V Z Z V σ(5)where τ is defined as the variance ratio 2221σσ. Expressions in terms of the i m ’s in (5)are derived in Appendix 1.The information matrix for []′2 is block diagonal (see Searle, Casella, andMcCulloch, 1992, p. 239) and, therefore, the estimators for the fixed effects and the variance components are asymptotically uncorrelated.5. Optimal Assembled DesignsAs n increases, there become a large number of assembled designs that are essentially equivalent according to their parameters. In Section 4, expressions were provided for the asymptotic variance-covariance matrices of the estimates from assembled designs. In this section, designs are compared in terms of their ability to accurately estimate the fixed effects and the variance components. There are many criteria that have been used to compare experimental designs. These optimality criteria are based on minimizing in some sense the variance of the estimates of the fixed effects and variance components.The D-optimality criterion is possibly the best known and most widely accepted (see Myers and Montgomery, 1995, p. 364 and Pukelsheim, 1993, p. 136). A design is D-optimal if it minimizes the determinant of the variance-covariance matrix of the estimates, often called the generalized variance of the estimates. Because no closed form expressions are available for the variance-covariance matrix of the maximum likelihood estimates in a linear mixed-effects model, we rely on the asymptotic results of Section 4and investigate (approximate) D-optimal assembled designs using the asymptotic variance-covariance matrices. Equivalently, we will then seek the assembled design that maximizes the determinant of the information matrix of the fixed effects and the variance components. Because the information matrix is block diagonal, its determinant is theproduct of the determinant of the fixed-effects information matrix and the determinant of the variance-components information matrix. It follows that if the same design that maximizes the determinant of fixed-effects information matrix also maximizes the determinant of the variance-components information matrix, then that design is D-optimal.Recall that for q =2, any HND with B batches can be represented by ()B B m m m m ,,,,121−= m , where i m is the number of samples in batch i, i=1,2,…,B and 1+≥i i m m . Define M B as the set of all feasible and non-trivial HNDs,{}1;,,2,1;,,,,M 1121B −<=≥∈=++−n B B i m m m m m m m i i i B B ),(6)where )+ denotes the set of positive integers (i.e., at least a sample is taken per produced batch). Note that, by definition, n m Bi i =∑=1. We consider the HNDs where n B = or1−=n B to be trivial, since there is only one HND for each of these cases and thus theymust be optimal.5.1. Fixed-Effects OptimalityFor a single HND, the D-optimality criterion for the fixed effects is the determinant of the matrix defined in (4). Since (4) is a scalar, it is the D-optimality criterion. The D-optimal HND for fixed effects can be found for any choice of n and B by solving Problem I : n m m m Bi i Bi i i=+∑∑==∈112122M subject to max Bσσm .Problem I is non-linear and has implicit integer constraints. A solution to Problem I is found by comparing any given HND with another that subtracts one observation from 1m and adds one observation to B m . By the definition of M B in (6), 1+≥i i m m , 1m and B m are respectively the maximum and minimum number of samples per batch in m . Theorem。
robust design

Carbon Fiber Screw, Rivet, Welding Bonding
LOAD TYPE Launch vehicle thrust Transient Thermal
Source: Klein, M., Schueller, G.I., et.al.,Probabilistic Approach to Structural Factors of Safety in Aerospace, Proceedings of the CNES Spacecraft Structures and Mechanical Testing Conference, Paris, June 1994, Cepadues Edition, Toulouse, 1994.
. . .
. . .
Design variables. Large changes in mean values are allowed.
. . .
Vibration Acoustics Aerodynamics Impacts Controls
Allowed design interval
From Analysis to Simulation
Stochastic Methods DOE and Response Surface Optimization
Optimization
Automated modifications of the analysis model parameters to achieve a desired objective while satisfying specified design requirements Wide range of methods
露天矿边坡破坏概率计算混合遗传算法

露天矿边坡破坏概率计算混合遗传算法
陈昌富;龚晓南
【期刊名称】《工程地质学报》
【年(卷),期】2002(010)003
【摘要】将数值优化中的Powell法引入遗传算法中,提出了一种新型的混合遗传算法,并针对新疆雅满苏露天铁矿上盘边坡,提出了一种新的露天矿边坡破坏概率分析方法,计算结果表明本文方法准确可靠,克服了传统方法易陷于局部最优解的缺点.【总页数】4页(P305-308)
【作者】陈昌富;龚晓南
【作者单位】湖南大学岩土工程研究所,长沙,410082;浙江大学岩土工程研究所,杭州,310027;浙江大学岩土工程研究所,杭州,310027
【正文语种】中文
【中图分类】U416.1
【相关文献】
1.Montle Carlo法在某露天矿边坡破坏概率计算中的应用 [J], 李海蒙;蔡美峰;乔兰
2.基于遗传算法的露天矿边坡检测传感网络优化 [J], 王焱;孙雁鸣;佟维妍
3.基于混合萤火虫遗传算法的云计算中的任务调度优化 [J], 孟庆岩;王晶晶
4.基于遗传算法的移动边缘计算混合关键任务卸载 [J], 邓添;沈艳;史奎锐
5.计算周期序列k-错线性复杂度的混合遗传算法 [J], 牛志华;苑璨;孔得宇
因版权原因,仅展示原文概要,查看原文内容请购买。
室外场景CSIFT的关键点检测和特征匹配
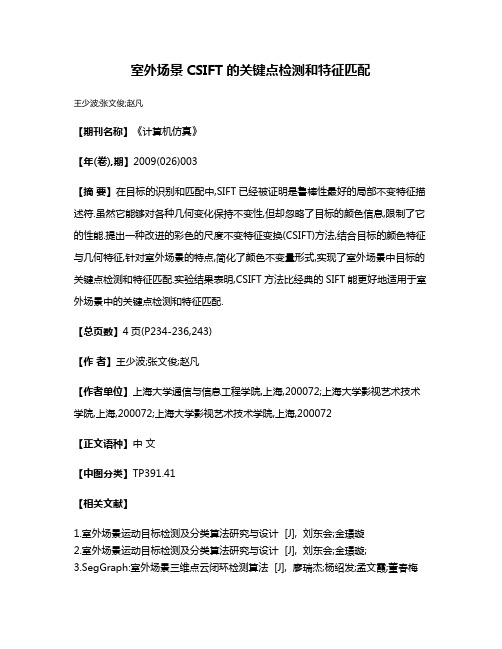
室外场景CSIFT的关键点检测和特征匹配
王少波;张文俊;赵凡
【期刊名称】《计算机仿真》
【年(卷),期】2009(026)003
【摘要】在目标的识别和匹配中,SIFT已经被证明是鲁棒性最好的局部不变特征描述符.虽然它能够对各种几何变化保持不变性,但却忽略了目标的颜色信息,限制了它的性能.提出一种改进的彩色的尺度不变特征变换(CSIFT)方法,结合目标的颜色特征与几何特征,针对室外场景的特点,简化了颜色不变量形式,实现了室外场景中目标的关键点检测和特征匹配.实验结果表明,CSIFT方法比经典的SIFT能更好地适用于室外场景中的关键点检测和特征匹配.
【总页数】4页(P234-236,243)
【作者】王少波;张文俊;赵凡
【作者单位】上海大学通信与信息工程学院,上海,200072;上海大学影视艺术技术学院,上海,200072;上海大学影视艺术技术学院,上海,200072
【正文语种】中文
【中图分类】TP391.41
【相关文献】
1.室外场景运动目标检测及分类算法研究与设计 [J], 刘东会;金璟璇
2.室外场景运动目标检测及分类算法研究与设计 [J], 刘东会;金璟璇;
3.SegGraph:室外场景三维点云闭环检测算法 [J], 廖瑞杰;杨绍发;孟文霞;董春梅
4.基于PointNet++的室外场景三维点云多目标检测方法 [J], 吴登禄;薛喜辉;张东文;付展宏
5.移动视点下室外场景的实时阴影检测 [J], 邹星明; 刘艳丽
因版权原因,仅展示原文概要,查看原文内容请购买。
- 1、下载文档前请自行甄别文档内容的完整性,平台不提供额外的编辑、内容补充、找答案等附加服务。
- 2、"仅部分预览"的文档,不可在线预览部分如存在完整性等问题,可反馈申请退款(可完整预览的文档不适用该条件!)。
- 3、如文档侵犯您的权益,请联系客服反馈,我们会尽快为您处理(人工客服工作时间:9:00-18:30)。
Robust Experimental Design for Multivariate Generalized Linear ModelsHovav A.D ROR and David M.S TEINBERGDepartment of Statistics and Operations ResearchRaymond and Beverly Sackler Faculty of Exact SciencesT el Aviv UniversityRamat Aviv69978Israel(hovavdror@;dms@post.tau.ac.il)A simple heuristic is proposed for constructing robust experimental designs for multivariate generalizedlinear models.The method is based on clustering a set of local optimal designs.A method forfindinglocal D-optimal designs using available resources is also introduced.Clustering,with its simplicity andminimal computation needs,is demonstrated to outperform more complex and sophisticated methods.KEY WORDS:Binary response;Clustering;D-optimal;Design of experiments;Logit;Poisson.1.INTRODUCTIONEfficient experimental designs for generalized linear models (GLMs)depend on the unknown coefficients;therefore—unlike experimental designs for linear models—two experiments hav-ing the same model but different coefficient values will typi-cally require different designs.For any given set of values for the model parameters,there is an experimental design that is lo-cally optimal.However,because there is uncertainty about the values,one should look for an experimental design that per-forms well all over the uncertainty space,giving higher priority to regions of higher likelihood within that space.Previous work on experimental designs for GLMs is focused mainly on locally optimal designs for a simple linear effect and one design variable(see,e.g.,Abdelbasit and Plackett1983; Ford,Torsney,and Wu1992;Mathew and Sinha2001).Most extensions(e.g.,Sitter and Torsney1995a,b)are limited to two factors or tofirst-order models that do not contain inter-actions.The limited amount of research is due in part to the fact thatfinding locally optimal designs for GLMs,and even more so for high-order multivariate models,is far from a trivial task.Section2describes a fast,simple method forfinding local D-optimal designs for these complex cases.The ability tofind locally optimal designs still leaves the serious problem of how to take into account uncertainty with respect to model coefficients.Different attitudes toward de-sign robustness for univariate GLMs have been expressed by Abdelbasit and Plackett(1983),Sitter(1992),Hedayat,Yan, and Pezzuto(1997),and Chaloner and Larntz(1989).Of these approaches,the latter should be emphasized for sug-gesting a Bayesian experimental design.Literature on mul-tivariate robust designs for GLMs is scarce and includes an unpublished manuscript by Chipman and Welch(avail-able at http://ace.acadiau.ca/math/chipmanh/papers/chipman-d-opt.ps)that suggests a minimax approach and an article by Robinson and Khuri(2003)that evokes the idea of using so-called“quantile dispersion”graphs.Khuri,Mukherjee,Sinha, and Ghosh(2004,p.42)surveyed design issues for GLMs and noted that“the research on designs for generalized linear mod-els is still very much in developmental stage.Not much work has been accomplished either in terms of theory or in terms of computational methods to evaluate the optimal design when the dimension of the design space is high.The situation when one has several covariates...demand[s]extensive work to eval-uate“optimal or at least efficient designs.”Recently Woods, Lewis,Eccleston,and Russell(2006)delivered much of the sought-after results by proposing a method forfinding multi-variate compromise designs that allow for uncertainty in the link function,the linear predictor,or the model parameters.In this article we suggest a simple heuristic capable offind-ing designs that are robust to most parameters an experimenter might consider,including(similar to Woods et al.2006)uncer-tainty in the coefficient values,in the linear predictor equation, and in the link pared with Bayesian designs,such as those of Chaloner and Larntz(1989),or the compromise de-signs of Woods et al.(2006),the suggested procedure requires considerably shorter computation time and is easier to imple-ment,requiring only the ability tofind locally optimal designs and a K-means clustering procedure(MacQueen1967).The process allows rapid exploration of various designs,enabling the procedure to outperform the existing alternatives.Given a set of local D-optimal designs,the core of the method proposed is to combine them into a set of location vectors and use K-means clustering to derive a robust design,as motivated by the following examples.1.1Example1Assume a logistic model with the linear predictorη=β0+βx x+βy y+βxy xy having uncertainty aboutβ0modeled as a uniform distribution over the region[0,2]withβx=βy=2,βxy=.2.Figure1shows the local D-optimal designs for this model,for25different equally spaced values ofβ0from the feasible region.Each local D-optimal design has four support points.It can be seen that different values ofβ0result in a small change of the location of these support points,and as a result there is a clear©2006American Statistical Association andthe American Society for Quality TECHNOMETRICS,NOVEMBER2006,VOL.48,NO.4DOI10.1198/004017006000000318520ROBUST DESIGN FOR GENERALIZED LINEAR MODELS521Figure 1.Proximity of 25Local D-Optimal Designs for a Logistic Model With Intercept Value Uncertainty.partition of the local designs’points to four clusters.Desiring an efficient experimental design without knowing any further information,it seems reasonable to place one point at the “mid-dle”of each cluster.1.2Example 2Woods et al.(2006)noted that the local D-optimal design for the centroid of the β’s uncertainty space often may be an ef-ficient compromise design.A preference for clustering can be justified only if it remains an efficient method even in condi-tions where using the best local D-optimal design fails to per-form well.Continuing Example 1but assuming a larger uncertainty re-gion for β0causes the four clusters to overlap.Figure 2dis-plays local D-optimal designs for 25different values of β0from [0,15]with βx =βy =10and βxy =.2.The filled points in the figure show the local D-optimal design for the centroid of the feasible region,β0=7.5.Figure 2.An Illustration of the Shortcoming of the Best Local D-Optimal Design.It is seen that the local D-optimal design for the centroid of the beta space has two support points on the diagonal whose distance from each other is smaller than the range of diagonal point shifts for other possible values of β0.Coverage of the design space through clustering has better potential for creating a robust design than the parameter space centroid or any other local D-optimal design.2.FINDING LOCAL D–OPTIMAL DESIGNS The procedure suggested in this article assumes the ability to easily construct local optimal designs.The assumption is far from being trivial,because common packages such as “gosset”(Hardin and Sloane 1993),the statistical toolbox in MATLAB (MathWorks Inc.),JMP,or the SAS Optex procedure were not designed to be used with GLMs.Finding an exact local D-optimal design for GLMs requires finding a choice of n support points that will maximize the determinant of the information matrix.For linear models,the information matrix is simply F F ,where F is the regression matrix.For GLMs,the information matrix also depends on a weight matrix and can be represented as F WF (see,e.g.,Atkinson and Donev 1992).The weights are given by W =V −1(µ)(d µ/d η)2,where V is the variance function;µis a vec-tor with row values,µi ,being the expected response for the experimental configuration expressed by the row F i of the re-gression matrix;η=F βis the linear predictor;βis the vector of p unknown coefficients;and the relation between µi and ηi is expressed through a given link function.For example,for a Poisson model with a log link,the diagonal elements of W are w ii =µi =exp (F i β),and for a binary response with the logit link,w ii =µi (1−µi )=exp (F i β)/(1+exp (F i β))2.Thus,given the values of β,we can compute the values of the diagonal matrix W for any candidate set of design points.Thus,local D-optimal designs for generalized linear models canbe found by setting ˜F=F √W and using a row-exchange algo-rithm,such as that of Federov (1972),to find an n point subset of F that maximizes the determinant of the information ma-trix ˜F˜F .For multivariate problems,a good candidate set may be of enormous size,causing common computer algorithms to mal-function or preventing their implementation.To overcome this obstacle,sequential methods may be used.Begin with a rough grid chosen at random or from a low-discrepancy sequence.[A short description of low-discrepancy (also known as quasi-random)sequences is given in the App.]For this candidate set,calculate the regression matrix and find a D-optimal e the result to create a new candidate set,with each support point of the D-optimal design found being the center of a new random or quasi-random sequence.To avoid large candidate sets,limit the size of the sequence around each point so that the number of candidate points from all sequences will be reasonably small;in the examples presented herein,we used 50normally distributed points around each candidate.Create a rule for adjusting the search radius around the points;for instance,reduce the search radius according to the largest distance between points in the new design compared with the previous step,but no less than 30%of the last search radius used.Create a stopping rule in accordance with the accuracy desired.TECHNOMETRICS,NOVEMBER 2006,VOL.48,NO.4522HOVAV A.DROR AND DAVID M.STEINBERGTo give an idea of the effectiveness of the procedure just described,it takes less than1second to produce a16-point local D-optimal design accurate to2decimal places for the 5-variable Poisson model containing2interactions used in puter run times presented in this article were mea-sured using a desktop PC with a2.4-GHz Celeron processor. An implementation of the algorithm and procedures for ex-amples from the following sections are available at http://www. math.tau.ac.il/˜dms/GLM_Design.3.CLUSTERING VERSUS BAYESIAN DESIGNS Chaloner and Larntz(1989)discussed construction of Bayes-ian-optimal designs for a one-variable(two parameters)logistic regression,where the probability of success for an observation at x∈[−1,1]is p(x;θ,µ)=1/[1+exp{−θ(x−µ)}].Their criterion for Bayesian D-optimality is to maximize the average log determinant of the normalized information matrix;the ex-pectation is taken according to a prior distribution on the coeffi-cients(µ,θ).Their method requires that the number of design points be specified,and so they repeated the optimization us-ing the simplex algorithm of Nelder and Mead(1965)starting with2design points and increasing the number steadily up to 20points.They then chose the design that optimized the crite-rion on the smallest number of design points.They illustrated their method withµandθuniformly distributed on an interval and with three different intervals for each parameter. Chaloner and Larntz(1989)demonstrated that as the uncer-tainty increases,so does the minimum number of support points required to attain the optimal value.However,in a1987tech-nical report,they also showed that out of three intervals exam-ined forµ,only for the widest interval,when it is distributed uniformly on[−1,1],is the Bayesian design significantly more efficient than the best local D-optimal design.A design based on clustering yields similar results and has three support points for the examples where the Bayesian design has three support points.It is more interesting to evaluate the effectiveness of a design based on clustering for the examples in which the Bayesian design proved superior to the centroid local D-optimal design,that is,forµ∼U[−1,1].As discussed by Chaloner and Larntz(1989),the choice of interval forθhas only a small in-fluence on thefinal design and its efficiency,and we display the results whenθ∼U[6,8].Their optimal Bayesian design uses seven support points with a reported value of−4.5783for the average log of the information matrix determinant.We used K-means clustering over100local D-optimal designs corresponding to coefficients ofθandµset by a Niederreiter(1988)quasi-random sequence over the described intervals.Similar to Chaloner and Larntz(1989),we increased the value of K,the number of support points,from2to20.Fig-ure3shows the mean value of the log of the determinant matrix when estimated using the same100local designs.Similar to the reported result,the criterion seems to reach a stable value for a design with seven support points,and that value seems to be better than the one stated by Chaloner and Larntz. Averaging over100designs may be insufficient for a precise evaluation,and using the same coefficient values to create the cluster and to estimate its performance may create a bias.There-fore,we reevaluated the7-support point design(createdthroughFigure3.Mean Value of the Log of the Determinant Matrix Estimated Over a Rough Grid.The dotted line represents the Chaloner and Larntz (1989)reported value.100local D-optimal designs)using10,000local D-optimal de-signs,with their coefficients again determined by a Niederreiter sequence.The criterion value given by this more thorough eval-uation confirmed the validity of the rough estimation.Its value is−4.25,higher than the value reported for the Bayesian de-sign.One of the advances of the work of Chaloner and Larntz (1989)over previous approaches is to create designs without the requirement that the points be equally spaced,and with the possibility of a different number of observations at each point. Like their work,a design created by clustering is not restricted to equally spaced points,but it does put equal weight on all of the support points.For a given set of points,it is possible to improve the design using sequential quadratic programming to adjust the weights.For the given example,this leads to only a minor improvement in the criterion value,to−4.23.Even though creating a robust design using clustering was found to be superior in this example,Bayesian designs would be expected to be generally better.If clustering normally does not fall much from Bayesian designs,then it has clear advan-tages over them:simplicity of creation and the need for consid-erably less computational resources.Unlike Bayesian design, extending the clustering procedure to multivariate problems is almost trivial and is considered next.4.CLUSTERING VERSUS MULTIVARIATECOMPROMISE DESIGNSWoods et al.(2006)provided a method forfinding exact de-signs for experiments in which there are several explanatory variables.They used simulated annealing tofind(as in Chaloner and Larntz1989)a design with a given number of support points that maximizes the average log determinant of the nor-malized information matrix.They noted that evaluating the in-tegral is too computationally intensive for incorporation within a search algorithm,and thus they averaged over a partial set chosen to represent the model space.Their method allows cre-ation of compromise designs with uncertainty in the link func-tion,the linear predictor,and the model parameters.TECHNOMETRICS,NOVEMBER2006,VOL.48,NO.4ROBUST DESIGN FOR GENERALIZED LINEAR MODELS523Woods et al.(2006,sec.5)gave an example of creating a16-point compromise design across a parameter space.Theydescribed a crystallography experiment aimed at modeling howfour explanatory variables(rate of agitation during mixing,vol-ume of composition,temperature,and evaporation rate)affectthe probability that a new product is formed.They recom-mended that when the suggested ranges for the unknowns,βi,are not large,the local D-optimal design for the centroid of theparameter space will be used.Otherwise,a compromise designbased on a coverage design performs better.The superiority ofthe compromise design created using a coverage set is demon-strated with a parameter space as described in Table1,which isbased on parameter space B3in table1of the original article.A design’s performance was evaluated using the median andminimum efficiency relative to10,000local D-optimal designscreated for random parameter vectors from the parameter space.The efficiency of a design was calculated as(|M C|/|M L|)1/p, where p is the number of unknown coefficients and M C andM L are the information matrices for the evaluated and localD-optimal designs.A standard24factorial design performedpoorly for the example with a median efficiency value of.07and a minimum of.003.Before creating a design using clustering,we examined thecompatibility of our assessments to those given by Woods et al.(2006).We created10,000local D-optimal designs using theprocedure described in Section2.The values of the10,000pa-rameter vectors were produced by a base2Niederreiter quasi-random sequence with212as a seed.This procedure enablesrecreation of the exact parameter vectors used here and at thesame time promises more uniform coverage of the parameterspace than can be achieved by random sampling.We then usedthese designs to evaluate the median and minimum efficiencyof the coverage design of Woods et al.(2006).The results werecompatible with those reported by Woods et al.:a median of.415(vs..41),and a minimum of.113(vs..12).The procedure of Woods et al.(2006)requires their specialalgorithm and a good problem-dependent choice of numeroustuning parameters,and is computer-intensive.The current tim-ing for their algorithm(see /staff/woods/glm_design;Woods2006)is roughly1.5minutes on a strongercomputer than we used,and7minutes on our computer.Weproceeded in an attempt to create an alternative design by clus-tering.First,we created local D-optimal designs for100parame-ter values,continuing the Niederreiter quasi-random sequenceused so far to ensure the use of different locally optimal de-signs for creating the composite design and assessing its ef-ficiency.This preparation work took less than1minute.Wethen gathered the1,600resulting points and applied K-meansclustering,as implemented in MATLAB(MathWorks Inc.)toTable1.Coefficient Ranges From the Woodset al.(2006)Crystallography ExperimentParameter Rangeβ0[−3,3]β1[4,10]β2[5,11]β3[−6,0]β4[−2.5,3.5]choose16representative points as our design.Often optimaldesign points are found on the boundary of the design region;thus we used the sum of the absolute differences as a distancemeasure,so that each cluster is represented by the component-wise median of its points.Each time that clustering is performed,a slightly different de-sign emerges.This is due to the random choice of initial clustercentroid positions.Thus we summarize the design performancethrough the median(and minimum)efficiencies averaged over50identical clustering runs,using the notation mean[95%CI],where“CI”denotes confidence interval.Clustering was found to have results comparable to thoseof the Woods et al.(2006)composite design,with medianefficiency of.40[.38,.42]and minimum efficiency of.091 [.06,.12].The time it took to create the composite design was negligible:.25seconds[.16,.33],in addition to the1-minutepreparation phase forfinding100local D-optimal designs.Better results can be obtained by repeating the cluster-ing process numerous times.Similar to Chaloner and Larntz(1989)and Woods et al.(2006),we chose the cluster withthe highest average log determinant of the information ma-trix.Averaging was done on the rough grid of100parametervectors used to create the local D-optimal designs.Indeed,re-peated clustering improved the results;the median efficiencygrew to.423[.416,.430],and the minimum efficiency was.096[.06,.13],requiring only25seconds to choose the design.Furthermore,because clustering is very fast,we can easilyexamine the effect of different choices for the number of sup-port points.Figure4displays the result of clustering done withdifferent numbers of support points.For each number of sup-port points,we used clustering only once,based on the100lo-cal D-optimal designs.We approximated the efficiency usingthe same locally optimal designs.Given the local designs,pro-ducing the data for thefigure took only20seconds.From Figure4,we see that the median efficiency reachesa stable value around or slightly above the previous numberof16support points,but the minimum efficiency continues togrow and stabilizes for only30support points or more.ThisFigure4.The Effect of Different Choices for the Number of Support Points on the Approximated Efficiency(medianefficiency; minimum efficiency).TECHNOMETRICS,NOVEMBER2006,VOL.48,NO.4524HOVAV A.DROR AND DAVID M.STEINBERGshows that a design with more support points may be advised. In fact,Woods et al.(2006)stated that in the crystallography experiment,48observations will be run,with the16-point de-sign replicated3times.Woods(2006)reported that computa-tion time for a24-point design is about twice as long as that needed for their16-point composite design,which may be why they used replicates rather than considering the option of adding new support points.Creating a48-point design using the algo-rithm of Woods et al.(2006)required more than100minutes on our computer.Given this,we chose,as before,the best design out of100 repetitions for a48-means clustering.As expected,the median did not change much:.423[.415,.432],but the minimum effi-ciency increased to.177[.141,.213].The increase in the min-imal value is of great importance,as we discuss in Section6. In addition,it is found that efficiency estimation based on100 local D-optimal designs is quite accurate,so one can produce both a compromise design and an estimate of its efficiency dis-tribution based on a small sample of local designs that is easy to obtain.Producing the48-point design that exceeds the efficiency of the results of Woods et al.(2006),requires only an additional 72[63,80]seconds,and,combined with the preparation phase, takes only2.5minutes,compared with the100minutes needed to generate a48-point design with the procedure of Woods et al. (2006)on our computer.The proposed method is simpler,both conceptually and computationally.Not only is it faster and of higher efficiency,but,due to the production of local D-optimal design,it facilitates the possibility of evaluating the efficiency of the design and comparing the efficiency of designs with dif-ferent numbers of support points.Without this unique trait,an algorithm produces a design with no measure of its effective-ness,and the importance of using a48-point design might not have been recognized.5.ROBUSTNESS FOR LINEAR PREDICTORSAND LINK FUNCTIONSThe method of Woods et al.(2006)forfinding compro-mise designs allows uncertainty not only in the model para-meters,but also in the link function and the choice of the linear predictor.In section6of their article they give an ex-ample with two explanatory variables in which there is un-certainty as to whether afirst-order model or a model with the interaction term is more appropriate,and also uncertainty about the link function:probit versus the asymmetric com-plementary log–log(CLL).The values of the model parame-ters wereβ=(3.0,1.6,4.1) for thefirst-order model andβ= (1.2,1.7,5.4,−1.7) when considering a model with the inter-action term.The results given are for designs with six observa-tions.Woods et al.(2006)showed that for this example,all of the four locally optimal designs perform poorly for some of the possible characteristics,with thefirst-order local D-optimal de-signs insufficient for any estimation of the interaction term.A compromise design created for the same problem enables es-timation of all four models with efficiency of at least.64.Ta-ble2is a reproduction of table3of Woods et al.(2006),addingTable2.Efficiencies of a Design Produced by Clustering,the Woods et al.(2006)Compromise Design,and Four Local Optimal Designs d i Reproduced From Table3of the Original ArticleDesignModel Clustering Woods d3d4d5d6 Probit No interaction.75.77 1.00.34.99.30 Interaction.81.800 1.000.97 CLL No interaction.64.64.99.24 1.00.11 Interaction.85.860.970 1.00a column with the efficiency achieved by clustering the four lo-cal D-optimal designs.It is seen that the performance of the Woods et al.(2006) compromise design and the design created by clustering the local D-optimal designs is very similar;both achieve at least moderate efficiency for all four models.Besides demonstrating the heuristic qualities of clustering, this example is useful for demonstrating limitations of its use. Three of the local D-optimal designs included a replicate of the point[1,−1],and so had onlyfive support points for a six-point design.This poses an obstacle for clustering,because although the best design may put higher weight on this support point than on the other design points,the output of the clustering pro-cedure includes any single point only once,and if seeking a six-point design,it is likely to replace the replication of the existing point by adding a different point with inferior contribution.To overcome this obstacle,we slightly jittered the points of the local D-optimal designs.Indeed,clustering the jittered design points puts two points very close to[1,−1]and is an easy way to overcome the limitation.6.WAVE SOLDERING EXAMPLEWu and Hamada(2000,chap.13,p.563)discussed a two-level factorial experiment to study the number of defects in a wave soldering process.We now consider how our approach could be applied to such an experiment,focusing on the number of solder-joint defects andfive continuous process variables: prebake temperature,flux density,conveyer speed,cooling time,and solder temperature.Like Wu and Hamada(2000),we use a Poisson model for the defect counts.Thefirst step in our algorithm is to define an a priori distribution for the model parameters.Here we consider the common situation when no previous experimental data are available for this task.As an alternative,we suggest construct-ing the prior distribution in collaboration with an expert from the factory.The expert is asked to estimate the number of de-fects for different possible settings of thefive factors;his es-timates are analyzed as if they were experimental results,and the uncertainty modeled is presented to the expert for approval. We consider a case in which the expert believes that afirst-order model would be sufficient,but also specifies two pairs of factors that have possible interaction effects.Both afirst-order model and a model with two cross-product terms were constructed from the analysis of the thought experiment.The estimations are presented in Table3,for factor levels coded to[−1,1]. Remark1.Note that the standard errors(SEs)are much larger for the model that contains interactions,even though bothTECHNOMETRICS,NOVEMBER2006,VOL.48,NO.4ROBUST DESIGN FOR GENERALIZED LINEAR MODELS 525Table 3.Prior Coefficient Estimates for Two Models for the WaveSoldering ExampleFirst-order With interactions TermEstimateSEEstimate SEIntercept −1.52.21−2.35.69x 1−4.30.20−5.53.94x 2−1.79.16−2.99.82x 3−3.39.24−3.95.59x 4−.28.32−.86.54x 5.23.30.41.36x 1x 2−2.07 1.32x 1x 3−1.13.98NOTE:SE,standard error.models were estimated from the same data.This phenomenon of having less precise estimates for more complex models is common.Remark 2.Our analysis did not take into account the cor-relation of coefficients,but this can be easily addressed,if de-sired,in sampling the parameter vectors used to generate local D-optimal designs.Efficiency was estimated using 20,000local D-optimal de-signs,half for the first-order model and the other half for a model with the suspected interactions.For each model,local D-optimal designs were found for 10,000coefficient vectors sampled from the normal distribution through a quasi-random sequence.6.1Clustering versus a Full Factorial DesignThe median efficiency of a full factorial experiment with 32points is <.1and,as shown in Figure 5,its distribution has 2peaks,originating from the 2models considered.(The full factorial has higher efficiency for the first-order model.)The efficiency can be greatly improved using clustering.As a prepa-ration phase,we created a set of 200local D-optimal designs,100for each model,with parameters taken from a quasi-random sequence in accordance with the normal distributionassumed.Figure 5.A Full Factorial Design Efficiencies Histogram for 2Consid-ered Models With 10,000Representative Model ParametersEach.Figure 6.The Effect of Different Choices for the Number of Support Points on the Approximated Efficiency(medianefficiency;minimum efficiency).The next step was to choose a good number of support points.We repeated the process used with the crystallography experi-ment,clustering only once for each of a set of possible support point numbers,and evaluating the efficiency only roughly over the same set of parameter vectors.Remark 3.For our purposes,it is sufficient to cluster only once for any tested number of support points without any repeti-tions,as was done in the production of Figure 6.But lack of rep-etition causes some of the cases studied to perform very poorly,due to a bad random choice of the initial K cluster centroids when performing the K -means clustering procedure.Hence the graph is not smooth,and the “dips”observed at around 15and 30support points are likely to be an effect of a poor clustering solution related to the random initial choice of centroids,not to a real problem with these design ing this graph,we choose the desired value for K ;then it is important to repeat the clustering process numerous times,to ensure high efficiency.Remark 4.It should be assumed that when the unknown pa-rameters’uncertainty is distributed normally,the true minimum efficiency should approach 0.Thus the values of the lower curve in Figure 6are not representative for the minimum values.But we argue that the lower curve is still a good indicator for the expected change in small efficiency quantiles.It is seen that the median efficiency is stable for any choice of more than 30support points.If we now choose,for exam-ple,a design with 48support points,then the median efficiency (as evaluated with the comprehensive database of 20,000local D-optimal designs)is.65.Figure 7displays a histogram of local efficiencies for a 48-run design achieved by clustering.6.2Clustering versus Centroid DesignAs noted by Woods et al.(2006),the local D-optimal design for the centroid of the parameter space is often a sufficiently robust design.When there is more than one model,as in our example,there is no single centroid.Still,given a strong re-lationship between the two examined models,one of the twoTECHNOMETRICS,NOVEMBER 2006,VOL.48,NO.4。