An Adaptive Channel Estimation Technique in MIMO OFDM Systems
自适应控制和参数估计-纽约大学教授孙静课件
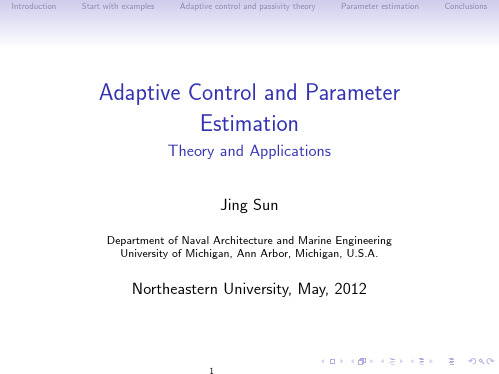
for any r, i.e., x − xm → 0 for any reference input r.
11
Introduction
Start with examples
Adaptive control and passivity theory
Parameter estimation
Conclusions
2
Introduction
Start with examples
Adaptive control and passivity theory
Parameter estimation
Conclusions
Some definitions:
Control system: an interconnection of components forming a system configuration that will provide a desired system response (Modern Control Systems, Richard Dorf). To adapt: to change (oneself) so that one’s behavior will conform to new or changed circumstances (Webster dictionary). Adaptive control:
Achieve high performance Take advantage of available on-line computation resources
6
Introduction
Start with examples
Adaptive control and passivity theory
Adaptive Finite-Time Control of Nonlinear Systems
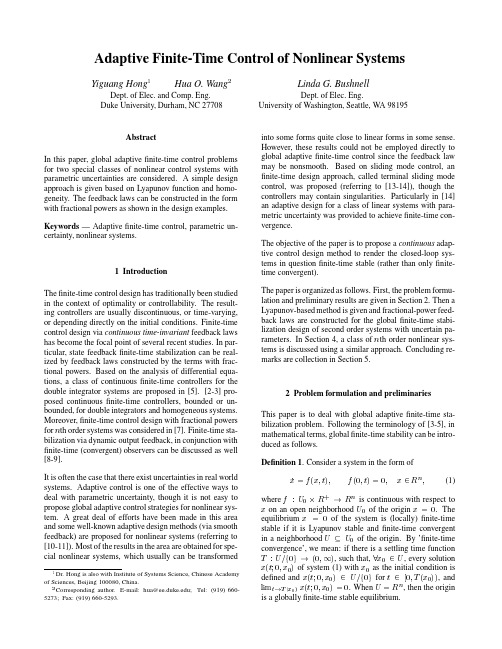
Adaptive Finite-Time Control of Nonlinear SystemsYiguang Hong Hua O.Wang Dept.of Elec.and Comp.Eng.Duke University,Durham,NC27708Linda G.BushnellDept.of Elec.Eng.University of Washington,Seattle,W A98195AbstractIn this paper,global adaptivefinite-time control problems for two special classes of nonlinear control systems with parametric uncertainties are considered.A simple design approach is given based on Lyapunov function and homo-geneity.The feedback laws can be constructed in the form with fractional powers as shown in the design examples. Keywords—Adaptivefinite-time control,parametric un-certainty,nonlinear systems.1IntroductionThefinite-time control design has traditionally been studied in the context of optimality or controllability.The result-ing controllers are usually discontinuous,or time-varying, or depending directly on the initial conditions.Finite-time control design via continuous time-invariant feedback laws has become the focal point of several recent studies.In par-ticular,state feedbackfinite-time stabilization can be real-ized by feedback laws constructed by the terms with frac-tional powers.Based on the analysis of differential equa-tions,a class of continuousfinite-time controllers for the double integrator systems are proposed in[5].[2-3]pro-posed continuousfinite-time controllers,bounded or un-bounded,for double integrators and homogeneous systems. Moreover,finite-time control design with fractional powers for th order systems was considered in[7].Finite-time sta-bilization via dynamic output feedback,in conjunction with finite-time(convergent)observers can be discussed as well [8-9].It is often the case that there exist uncertainties in real world systems.Adaptive control is one of the effective ways to deal with parametric uncertainty,though it is not easy to propose global adaptive control strategies for nonlinear sys-tem.A great deal of efforts have been made in this area and some well-known adaptive design methods(via smooth feedback)are proposed for nonlinear systems(referring to [10-11]).Most of the results in the area are obtained for spe-cial nonlinear systems,which usually can be transformed Dr.Hong is also with Institute of Systems Science,Chinese Academy of Sciences,Beijing100080,China.Corresponding author.E-mail:hua@;Tel:(919)660-5273;Fax:(919)660-5293.into some forms quite close to linear forms in some sense. However,these results could not be employed directly to global adaptivefinite-time control since the feedback law may be nonsmooth.Based on sliding mode control,an finite-time design approach,called terminal sliding mode control,was proposed(referring to[13-14]),though the controllers may contain singularities.Particularly in[14] an adaptive design for a class of linear systems with para-metric uncertainty was provided to achievefinite-time con-vergence.The objective of the paper is to propose a continuous adap-tive control design method to render the closed-loop sys-tems in questionfinite-time stable(rather than onlyfinite-time convergent).The paper is organized as follows.First,the problem formu-lation and preliminary results are given in Section2.Then a Lyapunov-based method is given and fractional-power feed-back laws are constructed for the globalfinite-time stabi-lization design of second order systems with uncertain pa-rameters.In Section4,a class of th order nonlinear sys-tems is discussed using a similar approach.Concluding re-marks are collection in Section5.2Problem formulation and preliminariesThis paper is to deal with global adaptivefinite-time sta-bilization problem.Following the terminology of[3-5],in mathematical terms,globalfinite-time stability can be intro-duced as follows.Definition1.Consider a system in the form of(1) where is continuous with respect to on an open neighborhood of the origin.Theequilibrium of the system is(locally)finite-time stable if it is Lyapunov stable andfinite-time convergent in a neighborhood of the origin.By’finite-time convergence’,we mean:if there is a settling time function,such that,,every solution of system(1)with as the initial condition is defined and for,and.When,then the origin is a globallyfinite-time stable equilibrium.Then we give the formulation of our problem.Definition2.Consider the following nonlinear system(2) where and are smooth with and, and is an uncertain parameter vector.The problem of global adaptivefinite-time stabilization is tofind a continu-ous time-invariant state feedback and an updat-ing law,such that1.the equilibrium of the closed-loop systemis globally stable,and2.For any initial condition,converges to infinite time,or in other words,isfinite-time convergent.Next,let us introduce the concept about homogeneity(re-ferring to[6][12]),which is useful in the following stabi-lization analysis.Definition3.Dilation is a mapping,depending on dilation coefficients,which assigns to every real a diffeomorphismwhere are suitable coordinates on and are positive real numbers.A function is called homogeneous of degreewith respect to dilation,if there existssuch thatA vectorfield is called homo-geneous of degree with respect to dilationif there exists such thatwhere.System is called homoge-neous if its vectorfield is homogeneous.In the sequel,where no confusion arises,we will simply use”with respect to dilation”instead of”with respect to dilation”.The two following lemmas can be found in[2-3][6]: Lemma1.Consider the nonlinear system described in(1). Suppose there is a function defined in a neighbor-hood of the origin,real numbers and,such that is positive definite on and is negative semidefinite(along the tra-jectory)on.Then the origin of system(1)isfinite-time stable.Lemma2.Assume system is homogeneous of degree with respect to the dilation, is continuous and is its asymptotically stable equi-librium.Then,the equilibrium of this system is globally finite-time stable.The following lemmas are quite straightforward,but,for convenience,the proofs are still given.Lemma3.A function is positive definite and homo-geneous of degree with respect to the dilation. If is a continuous function such that,for anyfixed ,,,as. Then is locally positive definite.Proof:With the conditions,there is a neighborhood of the origin,with small enough, such that,for any,Therefore,holds when.Lemma4.If the solution of system(1),for any initial condition,converges to,and there is a neighborhood of such that of system (1),for any initial condition,converges to0infinite time,then of system(1),for any initial condition ,converges to0infinite time.This lemma shows that global convergence and localfinite-time convergence implies globalfinite-time convergence. The fact is based on that global convergence yields that any solution will enter the given set infinite time.With the preliminary results,we will study our problem in the sequel.3Adaptivefinite-time control of second order systemsIn the section,we consider second order nonlinear systems of the form(3)where,are smooth with,and denotes the parameter vector,which may be un-certain.Adaptivefinite-time control design via continuous feedback is quite difficult.The existing results on adaptive(asymp-totic)control cannot be used directly,though their ideas very helpful([10-11]).Consider system(4)which can befinite-time stabilized in different ways([4] [6]).In fact,assume that is a homogeneous func-tion of degree with dilation.Then, with the feedback,the closed-loop system will be homoge-neous of degree,which is negative,and therefore,it isfinite-time stable according to Lemma2.Moreover,be-causefinite-time stability implies asymptotic stability,from [12],for any real number,there is a Lyapunov function of homogeneity degree,with the same dilation as that of,such that is positive definite and radially unbounded(i.e.,as), and(5)is negative definite along the trajectory of the closed-loop system.Then the main result of this section can be put as follows: Theorem1.Assume and defined as above(for system(4)).Then the global adaptivefinite-time stabiliza-tion problem of system(3)can be solved by the control law(6) with an updating law(7) whereProof:The proof procedure can be given in following four steps:Step1:Since denotes the uncertain parameters,we have to use the estimate instead of in the control design. Therefore,taking yields(8)where the function and.Note thatis the update law.Note that if and only if.Therefore,according to Definition2,the adaptivefinite-time stabilization prob-lem can be solved for system(8)under certain feedback laws implies that the problem can be solved for system(3) with the same feedback.Hence,we will focus on system (8).For simplicity,letWith the discussion just before the theorem,we haveis negative definite.In addition,is homogeneous of degree with dilation since both system (4)and are homogeneous with this dilation.Step2:The control law(6)can be rewritten with respect to as follows:Thus,Then the derivative of along system(8)isTake,which is positive definite with re-spect to.Then,which is negative defi-nite,and homogeneous of degree with respect to dilation as pointed out in Step1.This directly means that the overall closed-loop system is Lyapunov stable(that is,thefirst condition in Definition2).Following the standard analysis about adaptive control(for example,Krstic,et al.1995),we have that,for system(8)under the adaptive control law,is bounded(say,)and converges to zero.Step3:Following the above discussion,we consider,where denotes the term .Clearly,whereNote that,for any,which is a higher degree term of.Therefore,for any fixed and,Step4:Invoking Lemma3,there is a neighborhoodof such that when,we haveis negative definite and also homogeneous of degree .Consider the definition of,it is not difficult to see that there are positive constants and such thatTherefore(9)is negative definite when.This inequality leads to the conclusion that converges to0infinite time when. Then,according to Lemma4,converges to0,which is the second condition of Definition2.Remark1.Note that when is known,the system isfinite-time stabilizable by the feedbackTheorem1implies that the adaptive control law can be con-structed once and are given for system(4).How-ever,we have not discussed the construction of them there. In what follows,we consider how to construct the adap-tivefinite-time stabilizing feedback laws.Two different laws are given for illustration.For simplicity,in the ex-amples,we assume that and withodd integers,Example1.For system(4),[3]gave afinite-time controllerand a homogeneous Lyapunov function of the closed-loop systemwhere,andClearly,and satisfy the conditions required in Theorem 1,which have been shown in[3].Sincefrom Theorem1,adaptivefinite-time control for system(3) can be constructed as:with an updating lawwith and.For illustration,we consider a specific simple systemThus,with and,the updating law isand the control lawIn comparison,with taking,the control law is a con-ventional adaptive feedback law:with.Example2.We propose another adaptive control law for finite-time design.Take a Lyapunov candidate function where.It is easy to see that the function is homo-geneous(of degree with respect to)and positive definite.With the method of[7],it is not difficult to obtain that the derivative of along the system(4)is negative definite withfinite-time controllerwhere is suitably large.Therefore,both and satisfy the conditions required in Theorem1.Thus,system(3)under the adaptive control lawand an updating lawis globallyfinite-time stable if.4.Adaptivefinite-time control of th order systemsFollowing the idea proposed for second order systems in the previous section,we can similarly propose a globalfinite-time adaptive control method for th order nonlinear sys-tems of the form:(10)where are odd positive integers,and is uncertain parameter vector.Remark2.Please note that the class of systems in the form of(10)is different from the class of systems considered in the last section even for the case of.In the case when is known,we have the result from[7]: Lemma5.For system of form(11)thefinite-time feedback law can be constructed as ,where andwith,renders the system(11)finite-time stable and homogeneous of degree with respect to dilation:ifwith some odd integers andsome suitable constants.In addition, its Lyapunov function can be constructed in a way:andwhereThen an adaptive control law is proposed for the global finite-time stability of the closed-loop system,following a procedure similar to that proposed in Section3. Theorem2.Thefinite-time adaptive control law can be constructed with(12) where as given in(11),and with an updating law(13)Proof:In fact,with an analysis similar to the above section, considering Lyapunov function and,we have the updating law of the form:Note thatwhich is negative definite with respect to.Therefore,as discussed in Step2in the proof of Theorem1,we have the overall system is Lyapunov stable,and moreover,is bounded(say,)and converges to zero.Then similar to Step3in the proof of Theorem1,we con-siderwithIt is not difficult to see that is higher degree with re-spect to with the dilation.Therefore,lo-calfinite-time convergence can be obtained.Then invoking Lemma4leads to the conclusion of the theorem.Remark3.For the system(10)with parametric uncer-tainty,the proposed globalfinite-time adaptive control will not only keeps the state of the considered systemfinite-time convergent and the overall system with Lyapunov stable in the case of unknown parameters,but also consists with thefinite-time design when there is no uncertain parameter. Remark4.An adaptive control method with terminal slid-ing mode was proposed in[14]for a special class of the systems in form of(10).In addition,their controllers may contain singularity as mentioned in[13-14]4ConclusionsAdaptivefinite-time control design with fractional powers is studied for two classes of uncertain nonlinear systems.A simple design approach is given based on Lyapunov func-tion and homogeneity.However,due to the presence of un-certainty,the estimation of the settling time remains a dif-ficult task.Control design with fractional powers is found to be viable for realizing desired system dynamic behavior. In fact,the two class of nonlinear systems are special classes of the systems of a generalized parametric strict-feedback form,whose adaptive control law can be constructed by the well-known backstepping method([10]).However,since backstepping method could not used tofinite-time design directly,the adaptivefinite-time design for this generalized systems will be studied.Acknowledgment The authors wish to express their gratitude to an anomynous reviewer for the many construc-tive suggestions on the paper.This research has been sup-ported in part by the U.S.Army Research Office Grants DAAG55-98-0002,DAAH04-96-0448and DAAD19-00-01-0504,and by the NSF of China.References[1]S.Bhat and D.Bernstein,Lyapunov analysis offinite-time differential equations,Proc of ACC,Seattle,W A,1995,pp.1831-1832.[2]S.Bhat and D.Bernstein,Finite-time stability of homo-geneous systems,Proc.of ACC,Albuquerque,NM, 1995,pp.2513-2514.[3]S.Bhat and D.Bernstein,Continuousfinite-time stabi-lization of the translational and rotational double in-tegrators,IEEE Trans.Automatic Control.,vol.43, 1998,pp.678-682.[4]S.Bhat and D.Bernstein,Finite-time stability of con-tinuous autonomous systems,SIAM J.Control and Optimization,vol.38,2000,pp.751-766.[5]V.Haimo,Finite time controllers,SIAM J.Control andOptimization,vol.24,1986,pp.760-770.[6]H.Hermes,Homogeneous coordinates and continuousasymptotically stabilizing feedback controls,in Dif-ferential Equations,Stability and Control,(S.Elaydi ed.),New York:Marcel Dekkere,1991,pp.249-260.[7]Y.Hong,Finite-time stabilization and stabilizability ofcontrollable systems,preprint.[8]Y.Hong,J.Huang,and Y.Xu,On an output feedbackfinite-time stabilization problem,IEEE Trans.Auto-matic Control,vol.46,2001,pp.305-309.[9]Y.Hong,G.Yang,L.Bushnell,and H.Wang,Globalfinite-time control:from state feedback to output feedback,Proc.of IEEE CDC,Sydney,Australia, 2000.[10]M.Krstic,L.Kanellakopoulos,and P.Kokotovic,Nonlinear and Adaptive Control Systems Design, John Wiley,New York,1995.[11]R.Marino,and P.Tomei,Nonlinear Control Design:Geometric,Adaptive,Robust,New York,Prentice Hall,1995.[12]L.Rosier,Homogeneous Lyapunov function for ho-mogeneous continuous vectorfield,Syst.Contr.Lett., vol.19,1992,pp.467-473.[13]Y.Wu,X.Yu,and Z.Man,Terminal sliding modecontrol for nonlinear systems,Syst.Contr.Lett.,vol.34,1998,pp.281-287.[14]X.Yu and Z.Man,Model reference adaptive controlsystems with terminal sliding modes,Int.J.of Con-trol,vol.64,1996,pp.1165-1176.。
channel Estimation techniques based on pilot arrangement in ofdm systems
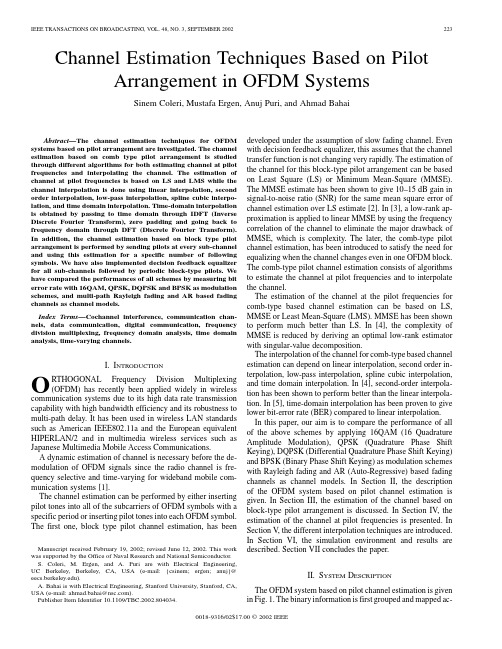
Channel Estimation Techniques Based on Pilot Arrangement in OFDM SystemsSinem Coleri,Mustafa Ergen,Anuj Puri,and Ahmad BahaiAbstract—The channel estimation techniques for OFDM systems based on pilot arrangement are investigated.The channel estimation based on comb type pilot arrangement is studied through different algorithms for both estimating channel at pilot frequencies and interpolating the channel.The estimation of channel at pilot frequencies is based on LS and LMS while the channel interpolation is done using linear interpolation,second order interpolation,low-pass interpolation,spline cubic interpo-lation,and time domain interpolation.Time-domain interpolation is obtained by passing to time domain through IDFT(Inverse Discrete Fourier Transform),zero padding and going back to frequency domain through DFT(Discrete Fourier Transform). In addition,the channel estimation based on block type pilot arrangement is performed by sending pilots at every sub-channel and using this estimation for a specific number of following symbols.We have also implemented decision feedback equalizer for all sub-channels followed by periodic block-type pilots.We have compared the performances of all schemes by measuring bit error rate with16QAM,QPSK,DQPSK and BPSK as modulation schemes,and multi-path Rayleigh fading and AR based fading channels as channel models.Index Terms—Cochannel interference,communication chan-nels,data communication,digital communication,frequency division multiplexing,frequency domain analysis,time domain analysis,time-varying channels.I.I NTRODUCTIONO RTHOGONAL Frequency Division Multiplexing (OFDM)has recently been applied widely in wireless communication systems due to its high data rate transmission capability with high bandwidth efficiency and its robustness to multi-path delay.It has been used in wireless LAN standards such as American IEEE802.11a and the European equivalent HIPERLAN/2and in multimedia wireless services such as Japanese Multimedia Mobile Access Communications.A dynamic estimation of channel is necessary before the de-modulation of OFDM signals since the radio channel is fre-quency selective and time-varying for wideband mobile com-munication systems[1].The channel estimation can be performed by either inserting pilot tones into all of the subcarriers of OFDM symbols with a specific period or inserting pilot tones into each OFDM symbol. The first one,block type pilot channel estimation,has beenManuscript received February19,2002;revised June12,2002.This work was supported by the Office of Naval Research and National Semiconductor. S.Coleri,M.Ergen,and A.Puri are with Electrical Engineering, UC Berkeley,Berkeley,CA,USA(e-mail:{csinem;ergen;anuj}@ ).A.Bahai is with Electrical Engineering,Stanford University,Stanford,CA, USA(e-mail:ahmad.bahai@).Publisher Item Identifier10.1109/TBC.2002.804034.developed under the assumption of slow fading channel.Even with decision feedback equalizer,this assumes that the channel transfer function is not changing very rapidly.The estimation of the channel for this block-type pilot arrangement can be based on Least Square(LS)or Minimum Mean-Square(MMSE). The MMSE estimate has been shown to give10–15dB gain in signal-to-noise ratio(SNR)for the same mean square error of channel estimation over LS estimate[2].In[3],a low-rank ap-proximation is applied to linear MMSE by using the frequency correlation of the channel to eliminate the major drawback of MMSE,which is complexity.The later,the comb-type pilot channel estimation,has been introduced to satisfy the need for equalizing when the channel changes even in one OFDM block. The comb-type pilot channel estimation consists of algorithms to estimate the channel at pilot frequencies and to interpolate the channel.The estimation of the channel at the pilot frequencies for comb-type based channel estimation can be based on LS, MMSE or Least Mean-Square(LMS).MMSE has been shown to perform much better than LS.In[4],the complexity of MMSE is reduced by deriving an optimal low-rank estimator with singular-value decomposition.The interpolation of the channel for comb-type based channel estimation can depend on linear interpolation,second order in-terpolation,low-pass interpolation,spline cubic interpolation, and time domain interpolation.In[4],second-order interpola-tion has been shown to perform better than the linear interpola-tion.In[5],time-domain interpolation has been proven to give lower bit-error rate(BER)compared to linear interpolation.In this paper,our aim is to compare the performance of all of the above schemes by applying16QAM(16Quadrature Amplitude Modulation),QPSK(Quadrature Phase Shift Keying),DQPSK(Differential Quadrature Phase Shift Keying) and BPSK(Binary Phase Shift Keying)as modulation schemes with Rayleigh fading and AR(Auto-Regressive)based fading channels as channel models.In Section II,the description of the OFDM system based on pilot channel estimation is given.In Section III,the estimation of the channel based on block-type pilot arrangement is discussed.In Section IV,the estimation of the channel at pilot frequencies is presented.In Section V,the different interpolation techniques are introduced. In Section VI,the simulation environment and results are described.Section VII concludes the paper.II.S YSTEM D ESCRIPTIONThe OFDM system based on pilot channel estimation is given in Fig.1.The binary information is first grouped and mapped ac-0018-9316/02$17.00©2002IEEEFig.1.Baseband OFDM system.cording to the modulation in “signal mapper.”After inserting pi-lots either to all sub-carriers with a specific period or uniformly between the information data sequence,IDFT block is used totransform the data sequence oflength into time do-mainsignalis the DFT length.Following IDFT block,guard time,which is chosen to be larger than the expected delay spread,is inserted to prevent inter-symbol interference.This guard time includes the cyclically extended part of OFDM symbol in order to eliminate inter-carrier interference (ICI).The resultant OFDM symbol is given asfollows:(2)wherewill pass through the frequency selective timevarying fading channel with additive noise.The received signal is givenby:(3)whereis Additive White Gaussian Noise (AWGN)andcan be represented by[5]:is the total number of propagationpaths,thpath,is theis delay spreadindex,th path delay normalized by the sampling time.Atthe receiver,after passing to discrete domain through A/D and low pass filter,guard time isremoved:for(5)Thenis sent to DFT block for the followingoperation:(6)Assuming there is no ISI,[8]shows the relation of theresulting,(7)where(8)Then the binary information data is obtained back in “signal demapper”block.III.C HANNEL E STIMATION B ASED ON B LOCK -T YPEP ILOT A RRANGEMENT In block-type pilot based channel estimation,OFDM channel estimation symbols are transmitted periodically,in which all sub-carriers are used as pilots.If the channel is constant during the block,there will be no channel estimation error since the pi-lots are sent at all carriers.The estimation can be performed by using either LS or MMSE [2],[3].If inter symbol interference is eliminated by the guard in-terval,we write (7)in matrixnotation.........is Gaussian and uncorre-lated with the channelnoise (11)COLERI et al.:CHANNEL ESTIMATION TECHNIQUES BASED ON PILOT ARRANGEMENT IN OFDM SYSTEMS225whereand.and represents the noisevariance.When the channel is slow fading,the channel estimation in-side the block can be updated using the decision feedback equal-izer at each sub-carrier.Decision feedback equalizer fortheThe channel response attheis used to find the estimated trans-mittedsignal(14)is mapped to the binary data through“signaldemapper”and then obtained back through“signal mapper”as.(15)Since the decision feedback equalizer has to assume that thedecisions are correct,the fast fading channel will cause thecomplete loss of estimated channel parameters.Therefore,asthe channel fading becomes faster,there happens to be a com-promise between the estimation error due to the interpolationand the error due to loss of channel tracking.For fast fadingchannels,as will be shown in simulations,the comb-type basedchannel estimation performs much better.IV.C HANNEL E STIMATION AT P ILOT F REQUENCIES INC OMB-T YPE P ILOT A RRANGEMENTIn comb-type pilot based channel estimation,the(16)where th pilotcarrier value.Wedefine as the fre-quency response of the channel at pilot sub-carriers.The esti-mate of the channel at pilot sub-carriers based on LS estimationis givenby:(17)where th pilotsub-carrier respectively.Since LS estimate is susceptible to noise and ICI,MMSEis proposed while compromising complexity.Since MMSEincludes the matrix inversion at each iteration,the simplifiedlinear MMSE estimator is suggested in[6].In this simplifiedversion,the inverse is only need to be calculated once.In[4],the complexity is further reduced with a low-rank approxima-tion by using singular valuedecomposition.Fig.2.Pilot arrangement.V.I NTERPOLATION T ECHNIQUES IN C OMB-T YPEP ILOT A RRANGEMENTIn comb-type pilot based channel estimation,an efficient in-terpolation technique is necessary in order to estimate channelat data sub-carriers by using the channel information at pilotsub-carriers.The linear interpolation method is shown to perform betterthan the piecewise-constant interpolation in[7].The channelestimation at thedata-carrier(18)The second-order interpolation is shown to fit better than linearinterpolation[4].The channel estimated by second-order inter-polation is givenby:226IEEE TRANSACTIONS ON BROADCASTING,VOL.48,NO.3,SEPTEMBER 2002TABLE IS IMULATION PARAMETERSinterpolation (spline function in MATLAB )produces a smooth and continuous polynomial fitted to given data points.The time domain interpolation is a high-resolution interpolation based on zero-padding and DFT/IDFT [8].After obtaining the estimatedchannelpoints with the followingmethod:(22)VI.S IMULATIONA.Description of Simulation1)System Parameters:OFDM system parameters used in the simulation are indicated in Table I:We assume to have perfect synchronization since the aim is to observe channel estimation performance.Moreover,we have chosen the guard interval to be greater than the maximum delay spread in order to avoid inter-symbol interference.Simulations are carried out for different signal-to-noise (SNR)ratios and for different Doppler spreads.2)Channel Model:Two multi-path fading channel models are used in the simulations.The 1st channel model is the ATTC (Advanced Television Technology Center)and the Grande Al-liance DTV laboratory’s ensemble E model,whose static case impulse response is givenby:(24)whereis chosen to be close to 1in order to satisfy the assumption that channel impulse response does not change within one OFDM symbol duration.In thesimulations,COLERI et al.:CHANNEL ESTIMATION TECHNIQUES BASED ON PILOT ARRANGEMENT IN OFDM SYSTEMS227Fig. 5.BPSK modulation with Rayleigh fading (channel 1,Doppler freq.70Hz).Fig.6.QPSK modulation with Rayleigh fading (channel 1,Doppler freq.70Hz).The channel estimation at pilot frequencies is performed by using either LS or LMS.Then all of the possible interpola-tion techniques (linear interpolation,second order interpolation,low-pass interpolation,spline cubic interpolation,and time do-main interpolation)are applied to LS estimation result to inves-tigate the interpolation effects and linear interpolation is applied to LMS estimation results to compare with the LS overall esti-mation results.B.Simulation ResultsThe legends “linear,second-order,low-pass,spline,time do-main”denote interpolation schemes of comb-type channel es-timation with the LS estimate at the pilot frequencies,“block type”shows the block type pilot arrangement with LS estimate at the pilot frequencies and without adjustment,“decision feed-back”means the block type pilot arrangement with LS estimate at the pilot frequencies and with decision feedback,and“LMS”Fig.7.16QAM modulation with Rayleigh fading (channel 1,Doppler freq.70Hz).Fig.8.DQPSK modulation with Rayleigh fading (channel 1,Doppler freq.70Hz).is for the linear interpolation scheme for comb-type channel es-timation with LMS estimate at the pilot frequencies.Figs.5–8give the BER performance of channel estimation algorithms for different modulations and for Rayleigh fading channel,with static channel response given in (23),Doppler fre-quency 70Hz and OFDM parameters given in Table I.These re-sults show that the block-type estimation and decision feedback BER is 10–15dB higher than that of the comb-type estimation type.This is because the channel transfer function changes so fast that there are even changes for adjacent OFDM symbols.The comb-type channel estimation with low pass interpola-tion achieves the best performance among all the estimation techniques for BPSK,QPSK,and 16QAM modulation.The per-formance among comb-type channel estimation techniques usu-ally ranges from the best to the worst as follows:low-pass,spline,time-domain,second-order and linear.The result was ex-pected since the low-pass interpolation used in simulation does the interpolation such that the mean-square error between the228IEEE TRANSACTIONS ON BROADCASTING,VOL.48,NO.3,SEPTEMBER2002Fig.9.16QAM modulation with AR fading(channel1).Fig.10.16QAM modulation with Rayleigh fading(channel2,Doppler freq.70Hz).interpolated points and their ideal values is minimized.Theseresults are also consistent with those obtained in[4]and[5].DQPSK modulation based channel estimation shows almostthe same performance for all channel estimation techniques ex-cept the decision-feedback method.This is expected because di-viding two consecutive data sub-carriers in signal de-mappereliminates the time varying fading channel effect.The errorin estimation techniques result from the additive white noise.The BER performance of DQPSK for all estimation types ismuch better than those with modulations QPSK and16QAMand worse than those with the BPSK modulation for high SNR.The effect of fading on the block type and LMS estimationcan be observed from Fig.9for autoregressive channel modelwith different fading parameters.As the fading factor“COLERI et al.:CHANNEL ESTIMATION TECHNIQUES BASED ON PILOT ARRANGEMENT IN OFDM SYSTEMS229worse than that of the best estimation.Therefore,some perfor-mance degradation can be tolerated for higher data bit rate for low Doppler spread channels although low-pass interpolation comb-type channel estimation is more robust for Doppler fre-quency increase.A CKNOWLEDGMENTThe authors are grateful to Prof.P.Varaiya for his help.R EFERENCES[1] A.R.S.Bahai and B.R.Saltzberg,Multi-Carrier Digital Communica-tions:Theory and Applications of OFDM:Kluwer Academic/Plenum, 1999.[2]J.-J.van de Beek,O.Edfors,M.Sandell,S.K.Wilson,and P.O.Bor-jesson,“On channel estimation in OFDM systems,”in Proc.IEEE45th Vehicular Technology Conf.,Chicago,IL,Jul.1995,pp.815–819.[3]O.Edfors,M.Sandell,J.-J.van de Beek,S.K.Wilson,and P.O.Br-jesson,“OFDM channel estimation by singular value decomposition,”IEEE mun.,vol.46,no.7,pp.931–939,Jul.1998.[4]M.Hsieh and C.Wei,“Channel estimation for OFDM systems based oncomb-type pilot arrangement in frequency selective fading channels,”IEEE Trans.Consumer Electron.,vol.44,no.1,Feb.1998.[5]R.Steele,Mobile Radio Communications.London,England:PentechPress Limited,1992.[6]U.Reimers,“Digital video broadcasting,”IEEE Commun.Mag.,vol.36,no.6,pp.104–110,June1998.[7]L.J.Cimini,“Analysis and simulation of a digital mobile channel usingorthogonal frequency division multiplexing,”IEEE mun., vol.33,no.7,pp.665–675,Jul.1985.[8]Y.Zhao and A.Huang,“A novel channel estimation method for OFDMMobile Communications Systems based on pilot signals and transform domain processing,”in Proc.IEEE47th Vehicular Technology Conf., Phoenix,USA,May1997,pp.2089–2093.[9] A.V.Oppenheim and R.W.Schafer,Discrete-Time Signal Processing,New Jersey:Prentice-Hall Inc.,1999.[10]“Digital video broadcasting(DVB):Framing,channel coding and mod-ulation for digital terrestrial television,”,Draft ETSI EN300744V1.3.1 (2000-08).[11]Y.Li,“Pilot-symbol-aided channel estimation for OFDM in wirelesssystems,”IEEE Trans.Vehicular Technol.,vol.49,no.4,Jul.2000.Sinem Coleri is a Ph.D.student in the Department of Electrical Engineering and Computer Science at University of California,Berkeley.She received her B.S.from Bilkent University in June2000.Her research interests include com-munication theory,adhoc networks,and mobile IP.Mustafa Ergen is a Ph.D.student in the Department of Electrical Engineering and Computer Science at University of California,Berkeley.He received his M.S.degree from University of California Berkeley in May2002and his B.S. degree from Middle East Technical University as a METU Valedictorian in June 2000.His research interests are in wireless networks and communication theory.Anuj Puri received his Ph.D.from the University of California,Berkeley in December1995.He was with Bell Labs of Lucent Technologies until December 1998.Since then he has been with the Department of Electrical Engineering and Computer Sciences at UC Berkeley.His interests are in wireless networks and embedded systems.Ahmad Bahai received his M.S.degree in electrical engineering from Impe-rial College,University of London in1988and his Ph.D.degree in electrical engineering from University of California at Berkeley in1993.From1992to 1994he worked as a member of technical staff in the wireless communications division of TCSI.He joined AT&T Bell Laboratories in1994where he was Technical Manager of Wireless Communication Group in Advanced Commu-nications Technology Labs until1997.He has been involved in design of PDC, IS-95,GSM,and IS-136terminals and base stations,as well as ADSL and Cable modems.He is one of the inventors of Multi-carrier CDMA(OFDM)concept and proposed the technology for the third generation wireless systems.He was the co-founder and Chief Technical Officer of ALGOREX Inc.and currently is the Chief Technology Officer of National Semiconductor,wireless division.He is an adjunct/consulting professor at Stanford University and UC Berkeley.His research interests include adaptive signal processing and communication theory. He is the author of more than30papers and reports and his book on Multi-Car-rier Digital Communications is published by Kluwer/Plenum.Dr.Bahai holds five patents in the Communications and Signal Processing field and currently serves as an editor of IEEE Communication Letters.。
3.2自适应均衡技术(Adaptive Equalization Techniques)

§3-2 自适应均衡技术(Adaptive Equalization Techniques)Review :分集有哪两层含义?合并的方式有哪几种?各自的基本思想是什么?分集是如何分类的?一、均衡基础(Fundamentals of Equalization )====》 如何补偿信道的多径衰落?均衡本质:产生与信道相反的特性,用来抵消信道的时变多径传播特性引起的码间串扰。
均衡不用增加传输功率和带宽,即可改善移动通信链路的传输质量。
均衡重在消除码间串扰,而分集重在消除深度衰落的影响。
均衡适用于信号不可分离多径且时延扩展远大于符号宽度的情况。
均衡有两个基本途径:1、频域均衡,它使包括均衡器在内的整个系统的总传输函数满足无失真传输的条件(即H(w)=1)。
它往往是分别校正幅频特性和群时延特性,模拟通信(序列均衡)通常采用这种频域均衡法。
2、时域均衡,就是直接从时间响应考虑,使包括均衡器在内的整个系统的冲激响应满足无码间串扰的条件(即h(t)=)(t δ)。
数字移动通信面临的信号是时变信号,因此通常采用这种时域均衡法,来达到整个系统无码间串扰。
随着码率的提高,时域均衡愈来愈复杂,研究热点逐步转入频域均衡。
均衡器常被放在接收机的基带或中频部分实现二、均衡原理图3-8 均衡器的实现框图如果x (t )是原始基带号,f (t )是等效的基带冲激响应,即综合反映了发射机、信道和接收机的射频、中频部分的总的传输特性,g (t )是发射机、信道、接收机的射频、中频部分和均衡器四者的等效冲激响应。
均衡器的期望输出值为原始信息x (t )。
假定n b (t )= 0,则g (t )必须满足下式:)()()()(t t h t f t g eq δ=⊗=-------(3-8)其频域表达式如下:1)()(=f F f H eq -------(3-9)式(3-9)的物理意义:将经过信道后的信号中频率衰落大的频谱部分进行增强,衰落小的部分进行削弱,以使所收到频谱的各部分衰落趋于平坦,相位趋于线性。
ChannelEstimation
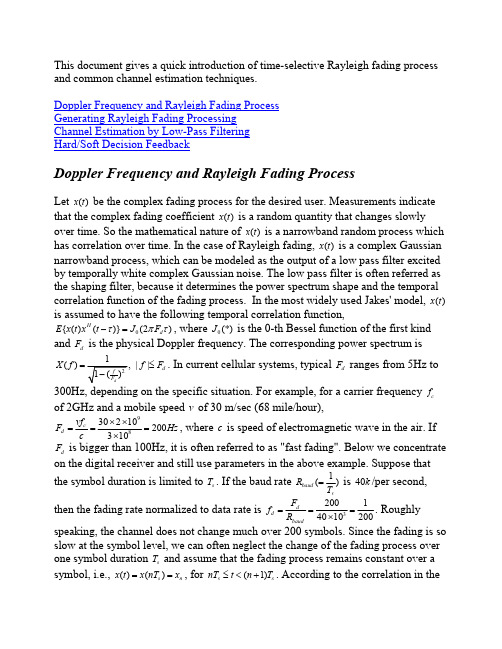
This document gives a quick introduction of time-selective Rayleigh fading process and common channel estimation techniques.Doppler Frequency and Rayleigh Fading Process Generating Rayleigh Fading Processing Channel Estimation by Low-Pass Filtering Hard/Soft Decision FeedbackDoppler Frequency and Rayleigh Fading ProcessLet ()x t be the complex fading process for the desired user. Measurements indicate that the complex fading coefficient ()x t is a random quantity that changes slowly over time. So the mathematical nature of ()x t is a narrowband random process which has correlation over time. In the case of Rayleigh fading, ()x t is a complex Gaussian narrowband process, which can be modeled as the output of a low pass filter excited by temporally white complex Gaussian noise. The low pass filter is often referred as the shaping filter, because it determines the power spectrum shape and the temporal correlation function of the fading process. In the most widely used Jakes' model, ()x t is assumed to have the following temporal correlation function,0{()()}(2)H d E x t x t J F τπτ−=, where 0(*)J is the 0-th Bessel function of the first kind and d Fis the physical Doppler frequency. The corresponding power spectrum is()||d X f f F =≤. In current cellular systems, typical d F ranges from 5Hz to300Hz, depending on the specific situation. For example, for a carrier frequency c f of 2GHz and a mobile speed v of 30 m/sec (68 mile/hour),9830210200310c d vf F Hz c××===×, where c is speed of electromagnetic wave in the air. If d F is bigger than 100Hz, it is often referred to as "fast fading". Below we concentrateon the digital receiver and still use parameters in the above example. Suppose that the symbol duration is limited to s T . If the baud rate 1()baud s R T =is 40k /per second, then the fading rate normalized to data rate is 320014010200dd baud F f R ===×. Roughly speaking, the channel does not change much over 200 symbols. Since the fading is so slow at the symbol level, we can often neglect the change of the fading process over one symbol duration s T and assume that the fading process remains constant over asymbol, i.e., ()()s n x t x nT x ==, for (1)s s nT t n T ≤<+. According to the correlation in thecontinuous case, the correlation function is 0{}(2)H n n m d E x x J f m π−=, whered d d s baudF f F T R == is the normalized Doppler frequency. The power spectrum of n xis ()||d X f f f =≤. At last, we point out that Jakes’ model in fact is mathematically derived rather than synthesized from field measurement. While Jakes’ model does fit some field measurement, other models from measurementcampaign might be more accurate in specific cases. For example, another normalized correlation function appears in a contribution to IEEE 802.16 specification for broadband wireless access, whose power spectrum is24()1 1.720.785,||d X f f f f f =−+≤.Generating Jakes’ Rayleigh Fading ProcessingA common method to generate n x is to sum up several sinusoids, as first suggested inJakes’ book. However, this method in fact generates a deterministic process and not a truly random process. Nevertheless, it is still widely used due to its simplicity. Here are the C++ implementation and COM implementation of a modified Jakes’ model proposed in this paper by P. Dent et al . Another way is to pass white complex Gaussian noise n uthrough the following shaping filter, ()||d h f f f =≤,which is the square root of the power spectrum of n x . This filter is highly nonlinearand approximation has to be sought. Since the shaping filter has infinite impulseresponse, it is natural to seek an IIR filter for approximation. JTC recommends using a 32 order IIR filter to approximate ()h f with normalized fading rate d f of 12andthen generating slower fading process by interpolating the output from the IIR filter.A simpler method is to use an autoregressive (AR) filter to approximate ()h f , whose coefficients can be calculated from the correlation function of n x via the Yuler-Walker equation.Channel Estimation by Low-Pass FilteringThe model for flat fading channel can be expressed as, n n n n y x d v =+, where n y is the received signal and n v is the complex additive white Gaussian noise. n d is the n thtransmitted symbol and in this documentation is limited to BPSK signals taking values of 1±. We first assume that the transmitted data is known to the receiver, forexample, n d is either a known pilot symbol or a highly reliable decision. So we canalternatively write the model as H H n n n n n y d x v d =+, where we want to recover thenarrowband process n x buried in white noise H n n v d .Figure 1: Channel Estimation via Low-pass FilteringThe engineering approach is low-pass filtering of the modulation-free signal H n n y d . Low-pass filtering itself is a well studied problem, both mathematically andpractically. If the temporal correlation of the fading process is known, Wiener filter is the optimal filter. Particularly, if the fading process is generated by the IIR filter or AR filter, a Kalman filter can carry out the estimation recursively, which is computationally efficient. Note that temporal correlation is a function of thenormalized Doppler frequency and is unknown unless the vehicle speed is known. If the vehicle speed is unknown, adaptive algorithms can be used to extract the narrowband process n x from the background noise. A popular adaptive filter is thelinear predictor, where LMS or RLS is used to minimize the prediction error. There are also methods to estimate the coefficients of the AR filter when Kalman filtering is carried out. Approaches above are pursuing the optimality of the channel estimator by adapting the low-pass filter to the bandwidth/shape of the shaping filter. As a practical matter, the low-pass filter can be designed as a fixed filter to handle the maximum Doppler frequency possible, for example, a brick-wall low-pass filter with bandwidth max f . This worst-case design has been shown numerically to be robust invarious situations and the performance degradation from "optimal" filters is minimal. The reason is that typically the maximum normalized Doppler frequency is fairlysmall (for example, 1in the first paragraph) and the fixed low-pass filter can200average over sufficient number of symbols to obtain accurate channel estimate for detection. An "optimal" filter could have averaged over even more symbols to further improve the channel estimate, but bit-error-rate is dominated by the additive noisevn and will not be significantly lowered by the better channel estimate. On the other hand, if the bandwidth of the fixed low-pass filter is smaller than the true normalized Doppler frequency, the channel estimate can not keep up with channel variation and the BER degradation is more severe. Since channel estimation only incurs moderate complexity, most cellular systems employ coherent demodulation to take advantage of its 2-3dB gain over non-coherent demodulation.A useful BER expression can be found in the Appendix C of "Digital Communications" by John G. Proakis, which takes into account the impact of channel estimation error when maximal ratio combining is used. This article by me further analyzes the impact of channel estimation error in the presence of strong co-channel interference.Hard/Soft Decision FeedbackUp to now, we have assumed that the symbold is somehow known and we use n dnto remove the modulation fromy. While we can insert many pilot symbols to makenthis assumption true, the overhead consumes a lot of energy and bandwidth. A more attractive approach is to use decisions as addition pilot symbols as follows. Suppose the transmission is in blocks. After the initial channel estimate is carried out, the data block is detected. While we might make wrong symbol decisions, we still remove the modulation with these decisions and re-estimate the channel using the low-pass filter. Hopefully the correct decisions can outweigh wrong decisions and output of the low-pass filter is a better channel estimate than the initial channel estimate. This better channel estimate in turn can lead to better decisions. This procedure can be repeated several times. As found out by many practitioners, this iterative hard decision feedback method does provide decent BER even if the number of known pilot symbols is quite small. In fact, if the shaping filter is an IIR/AR filter and the channel estimator is a Kalman filter, it can be formally proved that each iteration can increase the likelihood of the demodulated symbol sequence, which justifies the validity of this intuitive idea of hard decision feedback.Figure 2: Iterative Receiver with Soft Decision FeedbackIn a fast fading environment, further improvement can be obtained by using "soft" decisions. That is, if the decision is unreliable, it is discounted in re-estimating the channel. For BPSK signals, the implementation is to multiply n y with {}H n E d , the expected value of the (conjugate of the) symbol. The rationale is that the closer the signal is to the decision boundary, the more unreliable the decision is and the smaller the amplitude of expected value of the symbol. So by using soft decision (the expected value) rather than hard decision, an unreliable decision has less impact in re-estimating the channel. Similar to the hard decision feedback case, if the shaping filter is an IIR/AR filter and the channel estimator is a Kalman filter, it can be formally proved that each iteration can increase the a posteriori probability of the channel estimate, which justifies the validity of this idea of soft decision feedback. Interested readers are referred to Chapter 4 of my thesis for the proof. Through extensive simulations we find that when the fading is slow (say, 150d f <), hard decision feedback has similar BER performance as soft decision feedback, whereas if the fading is fast, soft decision feedback is better than hard decision feedback. Our conjecture is that when fading is slow, the channel estimator can average over many symbols, so the effect of a few wrong decisions can be averaged out. However, if the fading is fast, the channel estimator can only average over limited number of symbols and the effect of wrong decisions is much harder to be averaged out.Consequently soft decision feedback is more favorable in a fast fading environment.A rigorous proof of why soft decision back is better still remains elusive, because it needs to deal with the convergence property of the expectation-maximization algorithm.from the Previous StageSoft Limiter。
A10GbsBiCMOSAdaptiveCableEqualizer_slicer_DETAIL
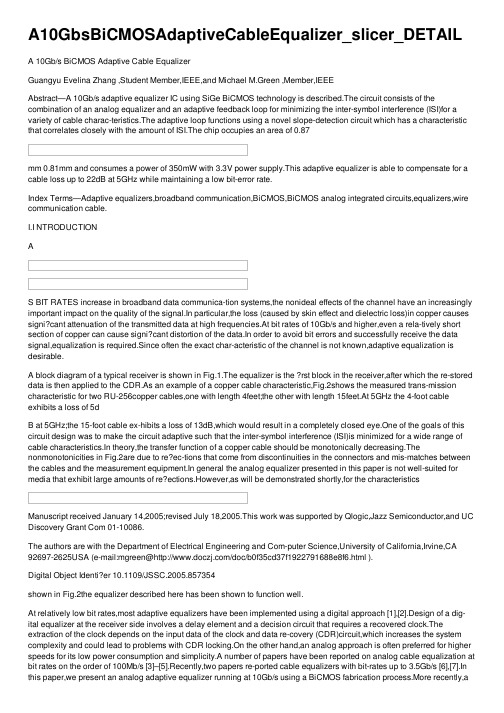
A10GbsBiCMOSAdaptiveCableEqualizer_slicer_DETAIL A 10Gb/s BiCMOS Adaptive Cable EqualizerGuangyu Evelina Zhang ,Student Member,IEEE,and Michael M.Green ,Member,IEEEAbstract—A 10Gb/s adaptive equalizer IC using SiGe BiCMOS technology is described.The circuit consists of the combination of an analog equalizer and an adaptive feedback loop for minimizing the inter-symbol interference (ISI)for a variety of cable charac-teristics.The adaptive loop functions using a novel slope-detection circuit which has a characteristic that correlates closely with the amount of ISI.The chip occupies an area of 0.87mm 0.81mm and consumes a power of 350mW with 3.3V power supply.This adaptive equalizer is able to compensate for a cable loss up to 22dB at 5GHz while maintaining a low bit-error rate.Index Terms—Adaptive equalizers,broadband communication,BiCMOS,BiCMOS analog integrated circuits,equalizers,wire communication cable.I.I NTRODUCTIONAS BIT RATES increase in broadband data communica-tion systems,the nonideal effects of the channel have an increasingly important impact on the quality of the signal.In particular,the loss (caused by skin effect and dielectric loss)in copper causes signi?cant attenuation of the transmitted data at high frequencies.At bit rates of 10Gb/s and higher,even a rela-tively short section of copper can cause signi?cant distortion of the data.In order to avoid bit errors and successfully receive the data signal,equalization is required.Since often the exact char-acteristic of the channel is not known,adaptive equalization is desirable.A block diagram of a typical receiver is shown in Fig.1.The equalizer is the ?rst block in the receiver,after which the re-stored data is then applied to the CDR.As an example of a copper cable characteristic,Fig.2shows the measured trans-mission characteristic for two RU-256copper cables,one with length 4feet;the other with length 15feet.At 5GHz the 4-foot cable exhibits a loss of 5dB at 5GHz;the 15-foot cable ex-hibits a loss of 13dB,which would result in a completely closed eye.One of the goals of this circuit design was to make the circuit adaptive such that the inter-symbol interference (ISI)is minimized for a wide range of cable characteristics.In theory,the transfer function of a copper cable should be monotonically decreasing.The nonmonotonicities in Fig.2are due to re?ec-tions that come from discontinuities in the connectors and mis-matches between the cables and the measurement equipment.In general the analog equalizer presented in this paper is not well-suited for media that exhibit large amounts of re?ections.However,as will be demonstrated shortly,for the characteristicsManuscript received January 14,2005;revised July 18,2005.This work was supported by Qlogic,Jazz Semiconductor,and UC Discovery Grant Com 01-10086.The authors are with the Department of Electrical Engineering and Com-puter Science,University of California,Irvine,CA 92697-2625USA (e-mail:mgreen@/doc/b0f35cd37f1922791688e8f6.html ).Digital Object Identi?er 10.1109/JSSC.2005.857354shown in Fig.2the equalizer described here has been shown to function well.At relatively low bit rates,most adaptive equalizers have been implemented using a digital approach [1],[2].Design of a dig-ital equalizer at the receiver side involves a delay element and a decision circuit that requires a recovered clock.The extraction of the clock depends on the input data of the clock and data re-covery (CDR)circuit,which increases the system complexity and could lead to problems with CDR locking.On the other hand,an analog approach is often preferred for higher speeds for its low power consumption and simplicity.A number of papers have been reported on analog cable equalization at bit rates on the order of 100Mb/s [3]–[5].Recently,two papers re-ported cable equalizers with bit-rates up to 3.5Gb/s [6],[7].InCMOS equalizer operating at 10Gb/s was presented [8]where more design effort was needed to overcome the gain limitations of CMOS.An analog FIR approach to 10Gb/s equalization was presented in [9].A BiCMOS process was chosen for this design due to boththehighand high intrinsic gain of the bipolar transistors.In particular,it was critical that the slicer used in the adaptive feedback loop (explained in Section III)exhibited high gain in order to restore the logic levels.This paper,which is an expanded version of the paper pre-sented in [10],is organized as follows.Section II discusses the principle of operation for the equalizer and adaptive loop.Section III describes the circuit design details for eachblock.Measurement results of prototypes are presented in Section IV .Finally,conclusions are drawn in Section V .II.P RINCIPLE OF O PERATIONTo understand the equalizer’s operation,we ?rst consider the operation of a simpli?ed linear circuit,shown in Fig.3oper-ating at a bit rate normalized to 1b/s.A rudimentary cable model shown in Fig.3(a),consisting of four RC sections,is used for this illustration.As mentioned in the previous section,this medium exhibits a monotonically decreasing transfer function.Thus,we would expect the ISI to be dominated by the attenu-ation of short (i.e.,1UI)pulses.In other words,the worst ISI would come from an isolated logic “1”of duration 1UI sur-rounded by strings of logic “0.”This is illustrated in Fig.4,where the response of the Fig.3(a)cable model,withset to 0.188,is shown for three different input pulse widths:1s,2s,and 3s.Clearly the worst case ISI comes from the shortest pulse width.Thus,our approach for designing the equalizer and adaptation loop is to reduce the ISI of a singlepulse,described as follows.0018-9200/$20.00?2005IEEEFig.1.Block diagram of broadband receiver including equalizer.TABLE IO PTIMUM T RANSIENT C HARACTERISTICS OF E QUALIZER FOR T HREE D IFFERENT C ABLE MODELSFig.2.S for two copper cables.As shown in Fig.3(b),thesignal from the cable is applied to an LC bandpass ?lter centered at 0.5Hz witha of 2.The equalizeroutput consists of a weighted sum of the originalsignal from thecableand the bandpassoutput .As illus-trated in Fig.3(b),weightingparameterdetermines the proportion of each signal that is summed at the output.Inparticular,if the input signal has a DC voltageswingbe.A sketch of the response at each node to a 1-s input pulse is shown in Fig.3(c).The linear equal-izer ’s transfer function is givenby(1)At DC,the transfer functiongivesfrequency (i.e.,0.5Hz),the transferfunctiongivesThe equalizer ’s operation can be illustrated in the time domain by considering its response to a 1-s unit interval pulse.This is shown in the curve labeled “cable output ”in Fig.5.As mentioned earlier,since the cable exhibits nearly unity gain at DC and signi ?cant attenuation at the Nyquist rate of 0.5Hz,the output pulsewidth is reduced.The equalizer operates by reducing the DC voltage swing while maintaining the gain at 0.5Hz,resulting in an increased pulse width.This is illustrated in Fig.5,where the equalizer pulse response is shown for threedifferent valuesof .In thiscasegives zero ISI.Note that the stage that follows this equalizer would require suf ?cient gain in order to restore the appropriate DC logic swing.We now consider a group of three different cable characteris-tics [parameterized by different valuesofin the Fig.3(a)cable model]and for each characteristic determine the valueof such that the ISI is zero.In doing this we can observe the equalizer ’s behavior at the optimum settings and identify a suitable method for adaptation.The cable pulse responses are shown in Fig.6(a).The resulting equalizer output wave-forms are shown in Fig.6(b),where for each ofthevalues,weightingparamter was set to its optimumvalue such that the pulsewidth for each waveform is exactly 1s.Table I lists the characteristics of each waveform.Note from this table that although the voltage swings and slew rates vary widely (more than 50%over the rangeof ),these values track each other,resulting in transition times that show much less variation (less than 15%).Thus,it is feasible to use this equalizer output transi-tion time as a rough measurement of ISI to be used in the adap-tive loop,where the optimum transition time is approximately 60%of the unit interval.(This optimum transition time value depends on the of the LC tank circuit;for this example a of 2was chosen because that is approximately the same value asFig.3.Linear circuit normalized to 1b/s forillustration.Fig.4.Pulse responses of cable.that of the LC tank used in the transistor-level implementation described in the next section.)The above analysis was based on a simpli ?ed linear circuit.As will be described in the following sections,the actual cir-cuit realization,based on current-mode logic (CML)techqniues,is of course nonlinear.However,the primary concern for min-imizing the ISI is to set precisely the timing of the zero cross-ings.In the vicinity of the zero crossings,CML circuitry in fact behaves nearly linearly.As will be shown shortly,the analysis given here does indeed apply to the actual transistor-level cir-cuitry.III.C IRCUIT D ESIGNThe block diagram of the adaptive equalizer is shown in Fig.7.The feedforward path consists of three parts:1)equalizer ? lter,which functions as a high-pass ?lter whose character-istics can be adjusted and whose operation was described in the previous section;2)slicer,which restores the full logic levels after the equalizer ?lter;and 3)output driver,which is used to drive an external50load.The feedback path consists of two slope detectors and an integrator,used to compare the difference between the transition times of the equalizer ?lter output and the slicer output.Each part will be described in detail asfollows.Fig.5.Pulse response of RC ladder (for C =0:125)and linear equalizer output for three different values of .A.Feedforward PathThe equalizer ?lter schematic is shown in Fig.8.The input data is applied to a peaked ampli ?er which resonates at thewith bandwidth of 9GHz (“slow ”path).Theoutputsand are applied to the mixer.The output of the mixer is thus the weighted sumofand with the weighting determinedby.Fig.9shows the magnitude transfer function for three different valuesof,exhibiting a maximum equal-ization of 22dB (de ?ned as the ratio of the gain at 5GHz to the DC gain).To demonstrate that this equalizer behaves like the linear pro-totype presented in Section II,the following simulation is per-formed.An input pulse with 100ps pulsewidth is applied to the(a)(b)Fig.6.(a)Pulse response of three different cable characteristics.(b)Pulse response of equalizer with optimum value of chosen for each cablecharacteristic.Fig.7.Adaptive equalizer blockcable 1followed by the equalizer ?lter.Byadjusting ,the output transitiontimevaries accordingly.The output pulsewidth varies directly with the value of the control voltageas shown in Fig.10.Notethatps corresponds to zero ISI,consistent with the results given in Section II.We observe that the pulses in Fig.10(which come from the transistor-level realization of the equalizer)look quite different from the pulses in Fig.5(which come from the simple linear model of the equal-izer).The reason for this difference is that the circuit realization is nonlinear due to the saturating characteristics of the CML blocks.However,in the vicinity of the zero crossings,the CML blocks behave linearly.Thus,the pulse widths of the Fig.10waveforms are consistent with those of Fig.5.1Thecable model used in this simulation is based on a behavioral model thatapproximates the characteristic in Fig.2corresponding to a length of 15feet.The relationbetweenand can be characterized by coef ?cientwith units of ps/mV .Simulation gives a valueof approximately 1.5ps/mV;i.e.,if is increased by 1mV ,the equalizer transition time decreases by 1.5ps.B.Feedback PathAs discussed in Section II,the feedback path functions to set the transition time of the equalizer ?lter output to a ?xed value of approximately 60ps so that the ISI is minimized.To accomplish this,the equalizer ?lter output is applied to the input of a slicer.The slicer,shown in Fig.11,is implemented by two cascaded CML buffers.The ?rst buffer exhibits fast transition time and corrects the signal amplitude.The second buffer is capacitively loaded so that it produces an output with a ?xed transition time of 60ps,independent of the input transition time.Thus,eachFig.8.Equalizer ?lterschematic.Fig.9.Magnitude plot of equalizer output for three different controlvoltages.Fig.10.Relation between output pulsewidth and transition time.transition at the equalizer ?lter output (where the transition time varies)always results in a transition at the slicer output with a 60ps transition time.In order to compare the transition times of these two signals a transition time detector is required.The transition time detector plays a critical role in the equal-izer circuit performance.Traditionally,such detectors are made up of a high-pass ?lter and recti ?er [4].However,the design ofaFig.11.Slicer schematic.recti ?er that operates at 10Gb/s is dif ?cult.In this circuit a dif-ferent approach was used,where the detection is performed by a single stage.As illustrated in Fig.12(a),theinputsand are applied to the gates of a CMOS differential pair.For a slow transition of either polarity,the source-coupled node voltage is minimum when the differential input is 0.Thus,the response to any input transition is a negative pulse whose amplitude is de-termined by the differential pair design.However,a faster tran-sition will have a smaller pulse amplitude due to the capacitance that is always present at the source-coupled node.Fig.12(b)shows 100ps input pulses with two different transition times;Fig.12(c)shows the corresponding detector output waveforms.The energy contained in each negative pulse is nearly propor-tional to the input transition time.In the feedback path,two detectors —one connected to the equalizer ?lter output,the other connected to the slicerFig.12.(a)Transition detector circuit.(b)Input waveforms.(c)Outputwaveforms.Fig.13.Schematic of integrator with common-mode feedback circuit.output —give output pulses whose energy is proportional to the input transition time.The pulses from both detector outputs are then applied to the differential inputs of an integrator (shown in Fig.13,including the common-mode feedback circuit);the integratoroutput,which is fed back to the equalizer,responds to the difference in transition time between the de-tector inputs.When the adaptive loop reachessteady-state,will be set such that the equalizer output will exhibitthe same transition time as the slicer output,thus minimizing the ISI.Although this circuit should function as a transition time detector,it is also sensitive to the signal levels of the input.In particular,if there is any mismatch in the DC levels between the two detector outputs,there will be a steady-state error in the equalization.Fortunately,the nonlinearity of the equalizer itself maintains a logic level that is independent of the equalizer settings,which can be observed in Fig.10.Thus,care needs to be taken only in ensuring that the logic levels of the input and output of the transition detectors are well-matched.The transferfunctionof the Fig.13integrator is givenbyFig.14.Operation of slicer and integrator.whereand .The integratortimeis set to 75ns.To illustrate the operation of the slicer and integrator,simulation was performed by varying theequalizer transitiontimeand observing the control voltage.The simulation results are shown in Fig.14.The combination of detector and integrator can be characterized by detectorgainwith units of mV/ps,where is proportional to the transi-tion density.SimulationgivesmV/ps for this circuit。
ADAPTIVE WAVELET PACKET IMAGE CODING USING AN ESTIMATION-QUANTIZATION FRAMEWORK
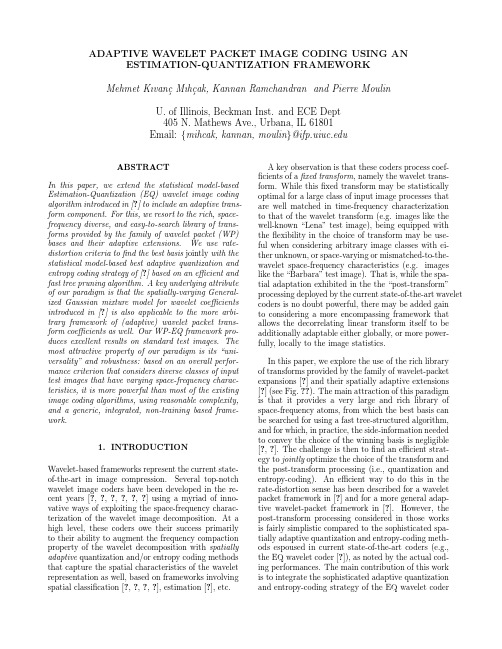
ADAPTIVE W A VELET PACKET IMAGE CODING USING AN ESTIMATION-QUANTIZATION FRAMEWORK Mehmet Kıvan¸c Mıh¸c ak,Kannan Ramchandran and Pierre MoulinU.of Illinois,Beckman Inst.and ECE Dept405N.Mathews Ave.,Urbana,IL61801Email:{mihcak,kannan,moulin}@ABSTRACTIn this paper,we extend the statistical model-based Estimation-Quantization(EQ)wavelet image coding algorithm introduced in[?]to include an adaptive trans-form component.For this,we resort to the rich,space-frequency diverse,and easy-to-search library of trans-forms provided by the family of wavelet packet(WP) bases and their adaptive extensions.We use rate-distortion criteria tofind the best basis jointly with the statistical model-based best adaptive quantization and entropy coding strategy of[?]based on an efficient and fast tree pruning algorithm.A key underlying attribute of our paradigm is that the spatially-varying General-ized Gaussian mixture model for wavelet coefficients introduced in[?]is also applicable to the more arbi-trary framework of(adaptive)wavelet packet trans-form coefficients as well.Our WP-EQ framework pro-duces excellent results on standard test images.The most attractive property of our paradigm is its“uni-versality”and robustness:based on an overall perfor-mance criterion that considers diverse classes of input test images that have varying space-frequency charac-teristics,it is more powerful than most of the existing image coding algorithms,using reasonable complexity, and a generic,integrated,non-training based frame-work.1.INTRODUCTIONWavelet-based frameworks represent the current state-of-the-art in image compression.Several top-notch wavelet image coders have been developed in the re-cent years[?,?,?,?,?,?]using a myriad of inno-vative ways of exploiting the space-frequency charac-terization of the wavelet image decomposition.At a high level,these coders owe their success primarily to their ability to augment the frequency compaction property of the wavelet decomposition with spatially adaptive quantization and/or entropy coding methods that capture the spatial characteristics of the wavelet representation as well,based on frameworks involving spatial classification[?,?,?,?],estimation[?],etc.A key observation is that these coders process coef-ficients of afixed transform,namely the wavelet trans-form.While thisfixed transform may be statistically optimal for a large class of input image processes that are well matched in time-frequency characterizationto that of the wavelet transform(e.g.images like the well-known“Lena”test image),being equipped with theflexibility in the choice of transform may be use-ful when considering arbitrary image classes with ei-ther unknown,or space-varying or mismatched-to-the-wavelet space-frequency characteristics(e.g.images like the“Barbara”test image).That is,while the spa-tial adaptation exhibited in the the“post-transform”processing deployed by the current state-of-the-art wavelet coders is no doubt powerful,there may be added gainto considering a more encompassing framework that allows the decorrelating linear transform itself to be additionally adaptable either globally,or more power-fully,locally to the image statistics.In this paper,we explore the use of the rich libraryof transforms provided by the family of wavelet-packet expansions[?]and their spatially adaptive extensions [?](see Fig.??).The main attraction of this paradigmis that it provides a very large and rich library of space-frequency atoms,from which the best basis canbe searched for using a fast tree-structured algorithm, and for which,in practice,the side-information neededto convey the choice of the winning basis is negligible [?,?].The challenge is then tofind an efficient strat-egy to jointly optimize the choice of the transform and the post-transform processing(i.e.,quantization and entropy-coding).An efficient way to do this in the rate-distortion sense has been described for a wavelet packet framework in[?]and for a more general adap-tive wavelet-packet framework in[?].However,the post-transform processing considered in those worksis fairly simplistic compared to the sophisticated spa-tially adaptive quantization and entropy-coding meth-ods espoused in current state-of-the-art coders(e.g., the EQ wavelet coder[?]),as noted by the actual cod-ing performances.The main contribution of this workis to integrate the sophisticated adaptive quantization and entropy-coding strategy of the EQ wavelet coderof[?]with the powerful adaptive wavelet packet ba-sis framework of[?,?]to formulate a powerful uni-versal framework that subsumes existing wavelet cod-ing paradigms,and that can handle diverse classes of input images.The idea of combining a power-ful coding scheme with adaptive wavelet packet ba-sis has been applied in[?];it is a zero-tree based wavelet image coder and it tries to capture the depen-dencies between different bands.However,the image coding algorithm presented in this paper is not only faster,but also produces better results,since it em-ploys spatial dependencies within a certain subband. Our simulation results indicate that there is appre-ciable performance gain for certain test images(e.g., the“Barbara”and“Goldhill”images)but negligible gain for others(e.g.,the“Lena”image).However, we emphasize that the power of our framework lies in its universality at reasonable computational cost, i.e.we can handle arbitrary image classes,with the satisfaction of strictly outperforming the correspond-ing(fixed)wavelet-based coding framework,at a rea-sonable increase in complexity,thanks to fast tree-pruning algorithms.Our test results indicate the uni-versal promise of our framework.FrequencyTime FrequencyTimeFrequencyTime(a)(b)(c) Figure1:Different tree tilings on the time-frequency plane:(a)Basis generated by wavelet tree(b)Ex-ample of a basis generated by wavelet packets(c)Ex-ample of a basis generated by a space-frequency tree. Notice that in(b),no spatial segmentation in the bases selection is allowed.2.THE TREE STRUCTURES2.1.The Frequency Tree Structure-Wavelet PacketsThe complete subband decomposition of a discrete signal set can be represented by“the frequency tree structure”,formed by recursivelyfiltering and down-sampling on both low and high frequency branches of the tree up to a certain depth(Fig.??).A spe-cific choice of a decomposition topology,correspond-ing to any pruned subtree of the original frequency tree,would represent an orthonormal basis to expand the input signal.The set of all such orthonormal ba-sis form“wavelet packets”[?].In2D manipulations, each parent would potentially have4children,each of which denoted as LL,LH,HL and HH.(a)(b)(c)LF : Low freqency branchHF : High frequency branchLFLFLFLFLFLFLFLFLFLFLF LFLFLFHFHFHFHFHFHFHFHFHFHFHFHFHFHFHFHFHFHFLFLF LFLFFigure2:Tree structures in1D(a)The frequency tree structure up to depth2,(b)The wavelet tree structure of depth2,(c)Arbitrary wavelet packet trees with a maximum depth of2.2.2.The Space-Frequency Tree StructureThe“space-frequency tree”provides a broader set of basis for expansion than does the frequency tree by adding the option of splitting in spatial domain,i.e. spatial adaptation to the frequency tree structure.In 2D manipulations,each parent of the space-frequency tree has up to8children,4of which are the frequency children discussed in the previous subsection and the other4are the space children.The spatial children are the1st,2nd,3rd and4th spatial quadrants(Fig.??). Space-frequency bases are constructed from the space-frequency tree structure,by either not splitting,or splitting in the frequency domain(that is low-pass and high-passfiltering followed by downsampling)or splitting in the spatial domain from the middle both vertically and horizontally.3.IMAGE CODING ALGORITHMS3.1.EQ CoderIn this work,the so-called Estimation-Quantization (EQ)coder has been used at each subband separately. It has been developed recently in[?].It is based on an independent“infinite”mixture model which accu-rately captures the space-frequency characterizationchild1st quarter 2nd quarter child 3rd quarter child 4th quarterchild ABC DLL childLH childHL childHH childBCDASPACE CHILDREN FREQUENCY CHILDRENPARENT NODEFigure 3:Parent node,frequency children and space children at a particular node of the space-frequency tree structure.within each pression efficiency is com-bined with speed.The image wavelet coefficients are modeled as be-ing drawn from an independent Generalized Gaussian distribution field,of fixed unknown shape for each sub-band,having zero mean and unknown slowly spatially-varying variances.The EQ framework consists of first finding the Maximum-Likelihood estimate of the indi-vidual spatially-varying coefficient field variances based on causal and quantized spatially-varying contexts;followed by applying an off-line Rate-Distortion op-timized quantization/entropy coding strategy,imple-mented as a fast lookup table,that is optimally matched to variance estimates.In the estimation step above,the variance estimates are obtained from a causal “quan-tized”spatial neighborhood set,which is available at the decoder simultaneously.This set can also include cross-scale spatial-tree coefficients to enrich the infor-mation of the domain.The quantization framework is potentially very general,can range from simple uni-form scalar quantization to entropy-constrained scalar quantization,with the advantage that this can be done “off-line”based on a unit variance R-D quantization table.In this work,deadzone quantizers have been used.The EQ paradigm also allows dynamic switch-ing between forward and backward adaptation,the decision is made based on the reliability of causal pre-diction contexts.It is based on pixel-level adaptivity and hence pro-duces a very accurate mixture field separation.The scheme elegantly adapts itself to all target bitrates,aided by the dynamic partitioning of the data into the forward and backward operation modes and the flexibility of the GGD model.Moreover,it combines theoretical elegance with a significance improvement in speed since it allows the off-line predesign of R-D optimized quantizer/entropy coding tables.Unlike the coding schemes used in zero-tree based wavelet image coders,it captures the correlations within eachsubband and thus it is inherently more suitable to be used in an adaptive wavelet packet based image coding framework.3.2.Wavelet Packet-EQ and Space Frequency-EQ AlgorithmsThe new WP-EQ (Wavelet Packet-EQ)and SF-EQ(Space Frequency-EQ)algorithms,we propose,com-bine the frequency and space-frequency tree pruning algorithms [?,?]with the EQ technique [?].Un-like other spatially adaptive quantization schemes [?,?],which capture the spatial characteristics of the transform coefficients by exploiting the correlations between different subbands,the EQ framework can naturally be integrated to the tree-pruning algorithms,since it is based on spatial adaptation within each sub-band [?].In WP-EQ the frequency tree and in SF-EQ the space-frequency tree is grown up to a certain depth as explained in the previous section.Then,having found the rate-distortion cost at each node using the EQ coder,in a bottom-to-top fashion,the cost of each parent node is compared with the sum of the costs of its frequency children for WP-EQ;the sum of the costs of its space children and the sum of the costs of its frequency children separately for SF-EQ.For WP-EQ,the decision at each node is either to split or not to split,whereas for SF-EQ the decision at each node is either not to split,or to split in frequency or to split in space.Having found the optimal tree struc-ture,the transform coefficients at the leaves of the tree are encoded using the EQ coder.The pseuocode de-scriptions of the WP-EQ and SF-EQ algorithms are presented in Table 1.The decoder,simply finds the quantized coefficients in the leave nodes and then in a bottom-to-top fashion the coefficients are decoded.The cost of sending a description of the winning basis (optimal tree structure)was shown to be negligibly small in [?,?]for all practical scenarios.Post-transform processing used in this work is not only faster,but also more powerful than most of the other tree-pruning algorithms proposed up to now [?,?],as shown by the performance of our algorithms.Furthermore,the reasonable complexity needed to find the optimal solution could be reduced using faster,near-optimal heuristics.Instead of using the actual coder to find the optimal tree structure,good esti-mates of rate-distortion values at each node can be employed to prune the tree.4.EXPERIMENTAL RESULTSIn this section,we present and compare the resultsof the wavelet packet-EQ(WP-EQ)and the space frequency-EQ(SF-EQ)algorithms with the best known results in the literature[?].Table??presents PSNR results for the“Lena”,“Barbara”and“Goldhill”im-ages.We used trees of maximum depth5and biorthog-onal10/18filters.The cost of sending the overhead information is included in the results presented and shown to be negligible.For arbitrary tree structures,itis not clear how to employ the cross-scale dependencies within the EQ framework,thus the EQ coder was usedin the“parent-off”mode,that is we did not exploit the correlation between different subbands for variance es-timation.We anticipate that further,yet incremental, gains might be possible when the correlation betweendifferent subbands is exploited in the EQ coder.Note that for“Lena”,the optimal wavelet-packet tree struc-ture turns out to be exactly the wavelet tree;whereas for“Barbara”and“Goldhill”,the optimal wavelet-packet and space-frequency tree structures are fairlydifferent from the wavelet tree structure.The results presented are either superior to the most powerful im-age coders in the literature or marginally close to the best result reported,for any standard image that has been worked on.Since these three images are good ex-amples of different image textures,it is a good indica-tion of the fact that our algorithms perform extremely well for images from diversefields.5.CONCLUSIONSA new image coding scheme has been presented,whichis the extension of the statistical model-based Estimation-Quantization framework to include an adaptive trans-form component,which consists of rich,space-frequency diverse family of bases.The proposed adaptive trans-form systems employ a wavelet-packet tree and a space-frequency tree respectively.The coders are robust todifferent image characteristics and perform either the best or very close to the best for any type of image that we have tested.The usage of wavelet packets in the EQ framework clearly augments the coding perfor-mance over wavelet tree-EQ algorithm in a substantial amount,whereas spatial segmentation(SF-EQ algo-rithm)does not increase the performance over WP-EQin a comparable amount.We believe that this is dueto the fact that the gains which can be obtained by exploiting spatial correlation have mostly been used by the EQ coder’s spatially adaptive scheme.We be-lieve that better spatial segmentation schemes within the space-frequency framework can be found and leave this issue for future research.The universality and robustness of our algorithms appears to be the most attractive feature,compared to other state-of-the-art image coding algorithms which perform very well for a certain class of images but somewhat worse for other classes of images.6.REFERENCES[1]M.Vetterli and J.Kova˘c evi´c,Wavelets and Subband Cod-ing,Prentice-Hall,Englewood Cliffs,NJ,1995.[2]R.Coifman,Y.Meyer,S.Quake,and V.Wickerhauser,“Signal Processing with wave packets,”Numerical Algo-rithms Research Group,Yale University,1990.[3]K.Ramchandran and M.Vetterli,“Best Wavelet PacketBases In A Rate-Distortion Sense,”IEEE Trans.Image Proc.,Vol.7,No6,pp.June1998.[4]Z.Xiong,K.Ramchandran and M.T.Orchard,“WaveletPacket Image Coding Using Space-Frequency Quantiza-tion,”IEEE Trans.Image Proc.,2(2):160-175,April 1993.[5] C.Herley,Z.Xiong,K.Ramchandran and M.T.Or-chard,“Joint Space-Frequency Segmentation Using Bal-anced Wavelet Packet Trees for Least-Cost Image Repre-sentation,”IEEE Trans.Image Proc.,Vol.6,No9,pp.1213-1230,September1997.[6]Z.Xiong,K.Ramchandran and M.T.Orchard,“Space-Frequency Quantization for Wavelet Image Coding,”IEEE Trans.Image Proc.,Vol.6,No5,pp.677-693,May1997.[7]S.LoPresto,K.Ramchandran,and M.Orchard,“ImageCoding based on Mixture Modeling of Wavelet Coefficients and a fast Estimation-Quantization Frameqork,”In Pro-ceedings of the Data Compression Conference,Snowbird UT,1997.[8]I.Daubechies,“Ten Lectures on Wavelets,”SIAM,Philadelphia,PA,1992.[9]I.Daubechies,“The wavelet transform,time-frequency lo-calization and signal analysis,”IEEE rm.Th., Vol.36,pp.961-1005,September1990.[10]R.L.Joshi,H.Jafarkhani,J.H.Kasner,T.R.Fischer,N.Farvardin,M.W.Marcellin,and R.H.Bamberger,“Com-parison of different methods of Classif.in subband coding of images,”IEEE Trans.Image Proc.,Vol.6,No11,pp.1473-1486,November1997[11] C.Chrysafis and A.Ortega,“Efficient context-based en-tropy coding for lossy wavelet image compression,”DCC, Data Compression Conference,Snowbird,UT,1997. [12]I.Balasingham, A.Fuldseth,and T.A.Ramstad,“OnOptimal Tiling of the Spectrum in Subband Image Com-pression,”In Proceedings of the IEEE International Con-ference on Image Processing,Santa Barbara,CA,1997.[13] A.Fuldseth,I.Balasingham and T.A.Ramstad,“EfficientCoding of the Classif.Table in Low Bit Rate Subband Im-age Coding by Use of Hierarchical Enumeration,”In Pro-ceedings of the IEEE International Conference on Image Processing,Santa Barbara,CA,1997.[14] A.Said,W.A.Pearlman,“A new fast and efficient imagecodec based on set of partitioning in hierarchical trees”, IEEE Trans.on Circuits and Systems for Video technol-ogy,vol.6,no.3,pp.243-250,June1996.[15]UCLA Image Communications Lab:PSNR Results,/˜ipl/psnr results.html1.Grow the full tree up to a certain depth andfind the transform coefficients at each nodeof the tree.e the EQ coder[?]at each node to generatethe rate and distortion values for thatparticular node given a quality factor,thereby computing the cost for that node.(The cost for a particular node is expressedas Rate+QualityF actor∗Distortion.)3.Initialize i←maximumdepth.At depthi,assign the minimum cost as the node’soriginal cost which was found by the EQcoder.4.i←i−1,if i<0go to step7.5.For all the nodes at depth i,make the SPLITdecision:if(Original Cost of The Parent Node<Sum ofThe Minimum Costs of Children)thenDO NOT SPLIT the parent node and assign theminimum cost of the parent as its originalcost which was found by the EQ coder,storethe split decision.elseSPLIT the parent node.Assign the minimumcost of the parent as the sum of the minimumcosts of its children in case of WP-EQ,whereas in case of SF-EQ the the minimum costof the parent is the smallest of‘‘the sum ofthe minimum costs of its frequency children’’and‘‘the sum of the minimum costs of itsspace children’’.Store the split decisionaccordingly.6.Go to Step 4.7.The split decisions at each node of the treeare found for a certain quality factor in arate-distortion ing these splitdecisions fix the optimal tree structureand quantize the transform coefficients atthe nodes where there should be no split.Produce the bits from the quantized data.(Quantization and production of the bits isagain done by the EQ coder[?]).Table1:Pseudocode Description of the Encoder Part of the Wavelet Packet-EQ algorithmRate(bpp) 1.000.500.25LENASubband Based Classif.41.14dB37.69dB34.31dB using VQ[?]Context Based[?]40.97dB37.52dB34.57dB Subband Based Classif.40.26dB37.18dB34.08dB with PVQ[?][?]Wavelet tree-EQ40.88dB37.69dB34.57dB (parent on)[?]Said-Pearlman[?]40.46dB37.21dB34.11dB WP-EQ(parent off)40.80dB37.66dB34.49dB SF-EQ(parent off)40.87dB37.70dB34.55dBBARBARASubband Based Classif.—–—–—–using VQ[?]Context Based[?]37.61dB32.64dB28.75dB Subband Based Classif.38.35dB33.89dB30.12dB with PVQ[?][?]Wavelet tree-EQ37.65dB32.87dB28.48dB (parent on)[?]Said-Pearlman[?]36.47dB33.07dB29.36dB WP-EQ(parent off)38.51dB33.87dB30.00dB SF-EQ(parent off)38.57dB33.89dB30.07dBGOLDHILLSubband Based Classif.—–—–—–using VQ[?]Context Based[?]36.90dB33.53dB30.80dB Subband Based Classif.—–33.46dB30.86dB with PVQ[?][?]Wavelet tree-EQ36.96dB33.44dB30.76dB (parent on)[?]Said-Pearlman[?]36.55dB33.19dB30.70dB WP-EQ(parent off)37.11dB33.61dB30.95dB SF-EQ(parent off)37.22dB33.70dB31.05dB Table2:Performances of the best wavelet and subband coding based image coders compared to our image coder algorithms’performances.The PSNR value is found from the distortion between the original image and the decoded image at different bit rates。
一种基于压缩感知的信道估计方法

一种基于压缩感知的信道估计方法作者:苏子业来源:《价值工程》2020年第24期摘要:利用水声信道稀疏特性,提出了一种基于压缩感知的信道估计方法。
首先对基于零前缀正交频分复用(zeros-Padded orthogonal frequency division multiplexing,ZP-OFDM)的水声通信系统接收端信号进行两次多普勒频移补偿并建立了离散信号模型,接着在传统正交匹配追踪(orthogonal matching pursuit,OMP)算法的框架下提出了一种改进的算法,该算法依据上次迭代中残差值和观测值的比例,加入相对应的加权矩阵以减小异常样本对本次迭代结果的影响,然后在所提算法的基础上,结合频域过采样的方法估计出水声信道参数。
仿真结果表明,改进的算法性能优于传统OMP算法,且更加有效的提高系统可靠性和有效性。
Abstract: Exploiting the sparse channel in underwater acoustic (UWA) communication, an improved channel estimation method based on compressed sensing was proposed. Frist of all, a received discrete signal model was established in zeros Padded-orthogonal frequency division multiplexing (ZP-OFDM) UWA communication system after compensating for the Doppler shift two times. Secondly an improved algorithm was proposed based on the structure of orthogonal matching pursuit (OMP), where an corresponding weighted matrix was added to decrease the impact of the outliers in this iteration by the ratio of the residuals and measurements in the last iteration. Then the improved algorithm and frequency domain oversampling method was jointly to have channel parameters estimated. The simulation results verify that the improved algorithm outperforms the traditional OMP algorithm, and the improved algorithm can enhance the system's reliability better.關键词:零前缀正交频分复用;频域过采样;改进正交匹配追踪算法Key words: zeros Padded-orthogonal frequency division multiplexing (ZP-OFDM);frequency domain oversampling;improved orthogonal matching pursuit (OMP)algorithm中图分类号:G353.1 ; ; ; ; ; ; ; ; ; ; ; ; ; ; ; ; ; ; 文献标识码:A ; ; ; ; ; ; ; ; ; ; ; ; ; ; ; ; ;文章编号:1006-4311(2020)24-0210-030 ;引言水声信道是双选择性信道,但信道的大多数能量仅仅存在于少数的时延点和多普勒频移因子上,即水声信道是典型的稀疏信道[1]。
自适应短波通信系统跳频信号时差定位方法

计算机仿真2021年1月第38卷第1期文章编号:1006 -9348(2021 )01 -0162 -05自适应短波通信系统跳频信号时差定位方法孙沙沙,王鹏(山东科技大学电子信息工程学院,山东青岛266590)摘要:短波通信在拓宽应用广泛性与普适性的同时,也存在信号跳频与难以监管问题。
以自适应短波通信系统为研究对象,提出一种跳频信号时差定位方法。
分析自适应短波通信系统的天波传输信道电离层特性、短波传播形式与跳频信号特点,利用离散频谱与傅里叶基,构建基于平坦衰落信道的跳频信号定位模型,根据架构的模型似然函数与互相关函数矩阵,获取 目标方位定位最大似然估计,实现跳频信号时差定位。
采用三个信号接收站与一个控制处理中心构成自适应短波通信系 统,经过外场试验环境,展开信号源信号与无人机遥控跳频信号的时差定位试验,依据对比实验结果可知,所提方法能够有 效定位目标信号,且定位精度具有显著的优越性,验证了方法的正确性与可行性,并为日后的研究工作提供了一定的参考 价值。
关键词:自适应短波;通信系统;跳频信号;时差定位;最大似然估计中图分类号:TN97 文献标识码:BTime Difference Positioning Method for Frequency HoppingSignal of Adaptive Shortwave Communication SystemSUN Sha - sha,WANG Peng(College of Information and Electrical Engineering,Shandong University of Science and Technology,Qingdao Shandong266590, China)A B S T R A C T:Generally,there are some defects in shortwave communication,such as signal frequency modulationand difficulty in supervision.In this regard,a novel time difference location method for frequency hopping signal i s proposed in this paper.The ionospheric characteristics,short wave propagation forms and frequency hopping signal characteristics of the sky wave transmission channel in the self- adaptive shortwave communication system were analyzed.The location model of frequency hopping signal based on f l a t fading channel was founded via established by the use of discrete spectrum and Fourier basis.Based on the model likelihood function and cross - correlation function matrix of the architecture,the maximum likelihood estimation of the target azimuth location was obtained,thus achieving the time difference location method of frequency hopping signal.The self- adaptive shortwave communication system consisted of three signal receiving stations and a control and processing center.The time difference location t e s t of signal source signal and U A V remote frequency hopping signal was carried out for verifying the effectiveness of the method in our work.The results show that the method can effectively locate the target signal,and has high positioning accuracy,showing the correctness and feasibility of the method,and providing a theoretical basis for future research work.K E Y W O R D S:Self-adaptive shortwave;Communication system;Frequency hopping signal;Time difference positioning;Maximum likelihood estimation基金项目:国家自然科学青年项目(61801270)收稿日期:2020-03 -19—162—1引言短波通信飞速发展,应用价值与日俱增,在远洋商业活 动、军事行动、国际组织行为以及人道主义救援等活动中得 到广泛应用。
M.2135 Guidelines for evaluation of radio interface technologies for IMT-Advanced
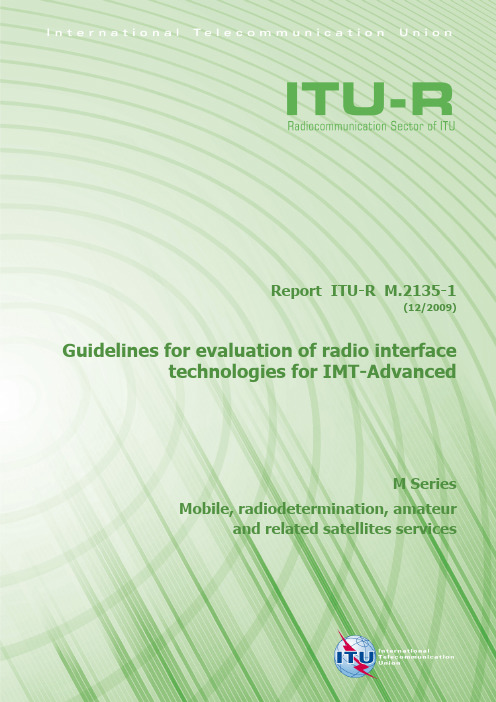
This Report provides guidelines for both the procedure and the criteria (technical, spectrum and service) to be used in evaluating the proposed IMT-Advanced radio interface technologies (RITs) or Sets of RITs (SRITs) for a number of test environments and deployment scenarios for evaluation. These test environments are chosen to simulate closely the more stringent radio operating environments. The evaluation procedure is designed in such a way that the overall performance of the candidate RIT/SRITs may be fairly and equally assessed on a technical basis. It ensures that the overall IMT-Advanced objectives are met.
All rights reserved. No part of this publication may be reproduced, by any means whatsoever, without written permission of ITU.
Rep. ITU-R M.2135-1
1
Adaptive+MIMO+switching+for+Mobile+WiMAX+OFDMA+Systems+Performance+Trade+off
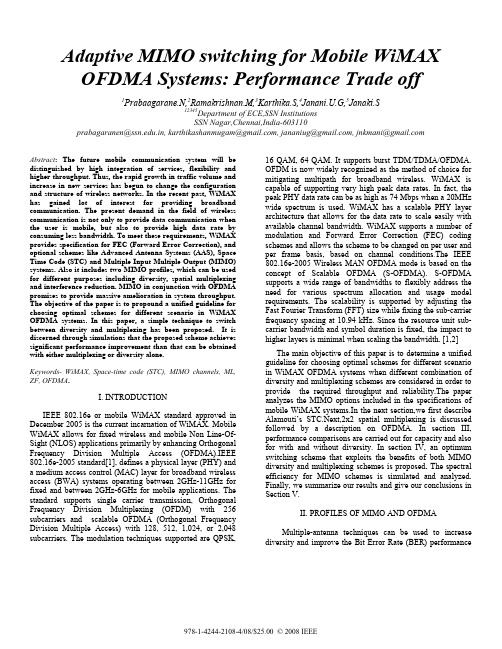
Adaptive MIMO switching for Mobile WiMAX OFDMA Systems: Performance Trade off 1Prabaagarane.N,2Ramakrishnan.M,3Karthika.S,4Janani.U.G,5Janaki.S12345Department of ECE,SSN InstitutionsSSN Nagar,Chennai,India-603110prabagaranen@.in,karthikashanmugam@, jananiug@, jnkmani@Abstract: The future mobile communication system will be distinguished by high integration of services, flexibility and higher throughput. Thus, the rapid growth in traffic volume and increase in new services has begun to change the configuration and structure of wireless networks. In the recent past, WiMAX has gained lot of interest for providing broadband communication. The present demand in the field of wireless communication is not only to provide data communication when the user is mobile, but also to provide high data rate by consuming less bandwidth. To meet these requirements, WiMAX provides specification for FEC (Forward Error Correction), and optional schemes like Advanced Antenna Systems (AAS), Space Time Code (STC) and Multiple Input Multiple Output (MIMO) systems. Also it includes two MIMO profiles, which can be used for different purposes including diversity, spatial multiplexing and interference reduction. MIMO in conjunction with OFDMA promises to provide massive amelioration in system throughput. The objective of the paper is to propound a unified guideline for choosing optimal schemes for different scenario in WiMAX OFDMA systems. In this paper, a simple technique to switch between diversity and multiplexing has been proposed. It is discerned through simulations that the proposed scheme achieves significant performance improvement than that can be obtained with either multiplexing or diversity alone.Keywords- WiMAX, Space-time code (STC), MIMO channels, ML, ZF, OFDMA.I. INTRODUCTIONIEEE 802.16e or mobile WiMAX standard approved in December 2005 is the current incarnation of WiMAX. Mobile WiMAX allows for fixed wireless and mobile Non Line-Of-Sight (NLOS) applications primarily by enhancing Orthogonal Frequency Division Multiple Access (OFDMA).IEEE 802.16e-2005 standard[1], defines a physical layer (PHY) and a medium access control (MAC) layer for broadband wireless access (BWA) systems operating between 2GHz-11GHz for fixed and between 2GHz-6GHz for mobile applications. The standard supports single carrier transmission, Orthogonal Frequency Division Multiplexing (OFDM) with 256 subcarriers and scalable OFDMA (Orthogonal Frequency Division Multiple Access) with 128, 512, 1,024, or 2,048 subcarriers. The modulation techniques supported are QPSK, 16 QAM, 64 QAM. It supports burst TDM/TDMA/OFDMA. OFDM is now widely recognized as the method of choice for mitigating multipath for broadband wireless. WiMAX is capable of supporting very high peak data rates. In fact, the peak PHY data rate can be as high as 74 Mbps when a 20MHz wide spectrum is used. WiMAX has a scalable PHY layer architecture that allows for the data rate to scale easily with available channel bandwidth. WiMAX supports a number of modulation and Forward Error Correction (FEC) coding schemes and allows the scheme to be changed on per user and per frame basis, based on channel conditions.The IEEE 802.16e-2005 Wireless MAN OFDMA mode is based on the concept of Scalable OFDMA (S-OFDMA). S-OFDMA supports a wide range of bandwidths to flexibly address the need for various spectrum allocation and usage model requirements. The scalability is supported by adjusting the Fast Fourier Transform (FFT) size while fixing the sub-carrier frequency spacing at 10.94 kHz. Since the resource unit sub-carrier bandwidth and symbol duration is fixed, the impact to higher layers is minimal when scaling the bandwidth. [1,2] The main objective of this paper is to determine a unified guideline for choosing optimal schemes for different scenario in WiMAX OFDMA systems when different combination of diversity and multiplexing schemes are considered in order to provide the required throughput and reliability.The paper analyzes the MIMO options included in the specifications of mobile WiMAX systems.In the next section,we first describe Alamouti’s STC.Next,2x2 spatial multiplexing is discussed followed by a description on OFDMA. In section III, performance comparisons are carried out for capacity and also for with and without diversity. In section IV, an optimum switching scheme that exploits the benefits of both MIMO diversity and multiplexing schemes is proposed. The spectral efficiency for MIMO schemes is simulated and analyzed. Finally, we summarize our results and give our conclusions in Section V.II. PROFILES OF MIMO AND OFDMAMultiple-antenna techniques can be used to increase diversity and improve the Bit Error Rate (BER) performanceof wireless systems, in accession to increasing the cell range, data rate through spatial multiplexing, and reduce interference from other users. Multiple-antenna techniques include MIMO diversity, and MIMO multiplexing. Diversity is traditionally obtained by repeating the transmitted symbols in time, in frequency or in space using multiple antennas at the receiver to achieve diversity gain. In addition to diversity gain, one can achieve spatial multiplexing gain, realized by transmitting independent information using individual antennas. The enormous values of the spatial multiplexing gain that can be achieved by MIMO techniques have had a major impact on the introduction of MIMO technology in wireless systems.For wireless communication systems, a novel direction proposed to resolve capacity requirements in the challenging radio environment is the exploitation of Multi Element Array (MEA) at both transmitter and receiver sides. The WiMAX Forum has selected two different multiple antenna profiles for use on the downlink. One of them is based on the Space Time Code (STC) proposed by Alamouti for transmit diversity and the other is a 2x2 spatial multiplexing scheme. These profiles can also be used on the uplink, but their implementation is only optional. [3]A.Alamouti’s Transmit DiversityA diversity scheme refers to a method for improving the reliability of a message signal by utilizing two or more communication channels with different characteristics. Diversity plays an important role in combating fading and co-channel interference and avoiding error bursts.Diversity schemes are more robust at low SNR conditions.A mobile user located far away from the base station benefits from diversity schemes. The first multiple antenna profile is the simple STC scheme proposed by Alamouti [4] for transmit diversity. In the IEEE 802.16e-2005 specifications, this scheme is referred to as Matrix A. Originally, Alamouti’s transmit diversity was proposed to avoid the use of receive diversity and keep the subscriber stations simple [3]. In OFDMA-based WiMAX systems, Alamouti scheme is applied subcarrier by subcarrier. Let (s1, s2) represent a group of two consecutive symbols in the input data stream to be transmitted. During a first symbol period t1, transmit (Tx) antenna 1 transmits symbol s1 and Tx antenna 2 transmits symbol s2. Next, during the second symbol period t2, Tx antenna 1 transmits symbol s2* and Tx antenna 2 transmits symbol -s1*. Denoting the channel response from Tx1 to the receiver (Rx) by h1 and the channel response from Tx2 to the receiver by h2, the received signal samples corresponding to the symbol periods t1 and t2 can be written as:r1 = h1 s1 + h2 s2+ n1r2 = h1 s2*- h2 s1* + n2 where n1 and n2 are additive noise terms.The receiver computes the following signals to estimate the symbols s1 and s2:x1 = h1* r1 - h2 r2*x2 = h2* r1 + h1 r2*These expressions clearly show that x1 and x2 can be sent to a threshold detector to estimate symbols s1 and s2 without interference from the other symbol. Alamouti’s transmit diversity can also be combined with MRC when 2 antennas are used at the receiver. Thus the resulting 2x2 system benefits from fourth-order diversity.Diversity gain is the increase in SNR by employing multiple antennas or the reduction in transmitted power when a diversity scheme is introduced without a performance loss. [3]B. Spatial MultiplexingIn spatial multiplexing, a high rate signal is split into multiple lower rate streams and each stream is transmitted from a different transmit antenna in the same frequency channel. Multiplexing schemes are more robust at low SNR conditions.A mobile user located close to the base station benefits from multiplexing schemes.Spatial multiplexing scheme is implemented using VBLAST architecture. The second multiple antenna profile included in WiMAX systems is the 2x2 MIMO technique based on matrix B = (s1,s2)T. This system performs spatial multiplexing and does not offer any diversity gain from the transmitter side. But it does offer a diversity gain of 2 on the receiver side when detected using maximum-likelihood (ML) detection. The symbols transmitted by Tx1 and Tx2 in parallel are denoted as s1 and s2 respectively. Denoting by h ji the channel response from Txi to Rxj (i,j = 1, 2), the signals received by the two Rx antennas are given by[3]r1 = h11 s1 + h12 s2+ n1r2 = h21 s1 + h22 s2+ n2which can be written in matrix form as:Maximum Likelihood and Zero Forcing detectors are usedto retrieve the transmitted symbols s1 and s2.III. PERFORMANCE ANALYSISFigure 1 show the performance of Alamouti 2x2 system with 16 QAM (ML detector) and 2x2 Spatial multiplexingwith QPSK modulation (ZF and ML detector) for 1 million samples for Rayleigh channel. Since Alamouti scheme has fourth-order diversity and the 2x2 spatial multiplexing has second-order diversity, the former obviously has better lower average BER than the latter. It can be observed that spatial multiplexing with ML detector performs better than ZF detector since ML benefits from diversity order of 2. Also, the ML detection results in optimal detection of the received samples. Another interesting observation is that the slope of the Alamouti scheme is twice as large as that of spatial multiplexing with ML receiver. At high SNR values, this scheme with 16 QAM and ML detection achieves better performance than 2x2 spatial multiplexing MIMO system with QPSK and ML detection. In accession to that, to swell the system capacity, the MIMO options needs to be selected as a function of channel SNR in the same way as modulation andcoding.Figure 1. Comparison of Alamouti (2x2) with 2x2 Spatial Multiplexing forRayleigh channelIV. ADAPTIVE MIMO SWITCHINGFor a fixed rate, either multiplexing or diversity is chosen based on the instantaneous channel state and the decision is conveyed to the transmitter via a low-rate feedback channel. The choice of multiplexing or diversity is dependent on the matrix channel, the constellation and the desired rate. The following assumptions are made for switching between diversity and multiplexing [6].1. The low-rate feedback channel from the receiverto the transmitter is assumed to be error-free and has zero-delay.2. We consider only the frequency-flat channelswhere the channel is known perfectly to the receiver but not to the transmitter.3. Error correction coding is not considered.4. The overall data rate of diversity andmultiplexing is fixedFigure 2 shows downlink adaptive MIMO switching where the transmitter switches between spatial multiplexing and diversity based on feedback from the receiver. The input data stream is encoded using the channel encoder where theredundant data is added to the input data for error correction atthe receiver.Figure 2. Downlink Adaptive MIMO SwitchingWhen the channel is slowly varying, the receiver computes theoptimum signaling technique and sends this information to the transmitter. Based on the Channel State Information (CSI), the Link Adaptation (LA) decision unit selects the modulation scheme and code rate and the MIMO mode. The transmitter then employs the corresponding spatial signaling method for next channel use. Low-bandwidth feedback channels are present in most cellular systems for the purpose of power control.Optimum performance for a fixed rate system is obtained by choosing the signaling scheme with lowest bit error rate. The throughput is optimized through link adaptation, which selects a constellation and a code rate as a function of the channel. This concept is called Adaptive Modulation and Coding (AMC). The basic idea is to measure the channel quality (for instance by estimating the received power or the received SNR) at the mobile station. If the channel variations are sufficiently slow so that they are essentially constant, the channel quality measurement can be fed back to the Base Station (BS) with estimation error and delay that do not significantly degrade performance. The BS can then adapt the modulation and coding schemes to the channel and optimize the overall spectral efficiency.V. PERFORMANCE TRADEOFFFigures 3 and 4 elucidate the performance of 2x2 diversity system with 16 QAM, 2x2 spatial multiplexing system with 4 QAM and optimal MIMO switching scheme for 100 samples and 10000 iterations for AWGN and Rayleigh channels respectively. It is observed from the figure that spatial multiplexing performs well at low SNR but slightly worst than MIMO diversity at higher SNR. This is due to the fact that spatial multiplexing has a diversity order of 2 whereas MIMO diversity has a diversity order of 4. It can be inferred that optimal selection scheme outperforms both diversity and multiplexing because it switches between both the schemes according to CSI. Figure 5 shows the spectral efficiency for 10 frame transmissions for various modulation schemes and code rates. The SNR thresholds are computed for a system using MIMO matrix A - diversity. Based on the SNR thresholds, AMC consists of using the modulation/coding combinationthat leads to the highest throughput. For instance, 16-QAM with code rate ½ provides the same spectral efficiency of 2.5 bits/symbol as that of 64-QAM with code rate 1/3 at a lower SNR threshold. Therefore, 16 QAM with code rate ½ can be used instead of 64 QAM with code rate 1/3. From the analysis, it can be concluded that increase in spectral efficiency as a function of SNR with respect to the user location will result in an increase in the cell capacity. Also, it can be divulged that higher level modulation cannot be employed for the users near a cell edge typically having low SNR values. In other words, multiplexing cannot be used for these users.Figure 3.Switching between diversity and multiplexing – AWGN ChannelFigure 4. Switching between diversity and multiplexing–Rayleigh ChannelFigure 5. Operating SNR thresholds for adaptive modulation and coding(L=10)V.CONCLUSIONIn this paper, the performance of MIMO diversity and MIMO multiplexing schemes has been evaluated by incorporating the PHY layer specifications according toWiMAX IEEE 802.16e. The primary focus was to assay WiMAX system with adaptive MIMO switching over AWGN and Rayleigh channels to mitigate ISI, multipath interference and multiuser interference. The channel model considered throughout this work was built on the classical understanding of fading, Doppler shift and delay spread. The transmitted substreams are corrupted by multipath and multiuser interference, in accession to AWGN at the front end of the receiver. The main benefits and design challenges of adaptive modulation and coding, and multiple antenna techniques such as transmit/receive diversity and spatial multiplexing are discussed. Several simulations were carried out to evaluate the performance of MIMO systems. Investigations were carried out on the BER of downlink with the propounded optimal switching scheme.It is discerned that the use of OFDMA in MIMO systems led to a significant cell range extension on the downlink in accession to efficient power to carrier groups assigned to distant users. Also, MIMO - OFDMA system can perform well in frequency selective fading environment as the substreams of that particular subcarrier which undergoes deep fade alone is lost. Employing OFDMA in MIMO eased the deployment of networks with a frequency reuse factor of 1 and thus eliminating the need for frequency planning. The investigation of simulation results reveal that by employing adaptive MIMO switching, a considerable amelioration in system throughput can be achieved by employing multiplexing in accession to bringing down the average BER by diversity, which was the prime objective of this paper.REFERENCES[1] IEEE 802.16-2005:IEEE Standard for Local and metropolitan areanetworks-Part16: Air Interface for Fixed and Mobile Broadband Wireless Access Systems – Amendment 2:Physical Layer and Medium Access Layers for Combined Fixed and Mobile Operation in Licensed Bands, February 2006. [2] [3] Bertrand Muquet, Ezio Biglieri and Hikmet Sari, “MIMO LinkAdaptation in Mobile WiMAX Systems,” in Proc. IEEE Wireless Communications and Networking Conference (WCNC 2007).[4] S.M. Alamouti, “A Simple Transmit Diversity Technique for WirelessCommunications”, IEEE Journal on Selected Areas in Communications, vol.16, no.8, pp.1451-1458, October 1998.[5] George Tsoulos, ‘MIMO System Technology for WirelessCommunications’, Taylor and Francis group, pp. 103-107.[6] R.W. Heath Jr. and A.J. Paulraj, “Switching Between Diversity andMultiplexing in MIMO Systems”, IEEE Transactions On Communications, Vol. 53, No. 6, pp. 962-968, June 2005.。
adaptive filter
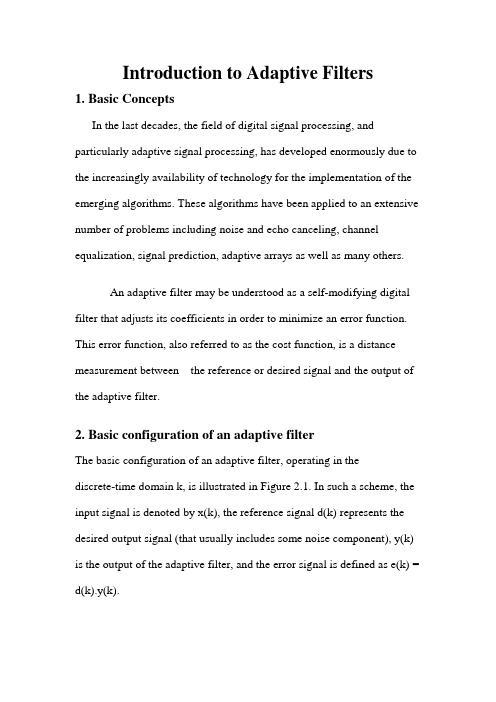
Introduction to Adaptive Filters1. Basic ConceptsIn the last decades, the field of digital signal processing, and particularly adaptive signal processing, has developed enormously due to the increasingly availability of technology for the implementation of the emerging algorithms. These algorithms have been applied to an extensive number of problems including noise and echo canceling, channel equalization, signal prediction, adaptive arrays as well as many others.An adaptive filter may be understood as a self-modifying digital filter that adjusts its coefficients in order to minimize an error function. This error function, also referred to as the cost function, is a distance measurement between the reference or desired signal and the output of the adaptive filter.2. Basic configuration of an adaptive filterThe basic configuration of an adaptive filter, operating in thediscrete-time domain k, is illustrated in Figure 2.1. In such a scheme, the input signal is denoted by x(k), the reference signal d(k) represents the desired output signal (that usually includes some noise component), y(k) is the output of the adaptive filter, and the error signal is defined as e(k) = d(k).y(k).Fig. 2.1 Basic block diagram of an adaptive filter.The error signal is used by the adaptation algorithm to update the adaptive filter coefficient vector w(k) according to some performance criterion. In general, the whole adaptation process aims at minimizing some metric of the error signal, forcing the adaptive filter output signal to approximate the reference signal in a statistical sense.Fig. 2.2Channel equalization configuration of an adaptive filter: The output signal y(k) estimates the transmitted signal s(k).Fig. 2.3 Predictor configuration of an adaptive filter: The output signaly(k) estimates the presentinput sample s(k) based on past values of this same signal. Therefore, when the adaptive filter output y(k) approximates the reference, the adaptive filter operates as a predictor system.3. Adaptation algorithmSeveral optimization procedures can be employed to adjust the filter coefficients, including, for instance, the least mean-square (LMS) and its normalized version, the data-reusing (DR) including the affine projection (AP), and the recursive least-squares (RLS) algorithms. All these schemes are discussed in Section 2.3, emphasizing their main convergence and implementation characteristics. The remaining of the book focuses on the RLS algorithms, particularly, those employing QR decomposition, which achieve excellent overall convergence performance.3.1 Error MeasurementsAdaptation of the filter coefficients follows a minimization procedure of aparticular objective or cost function. This function is commonly defined as a norm of the error signal e(k). The three most commonly employed norms are the mean-square error (MSE), the instantaneous square error (ISE), and the weighted least-squares (WLS), which are introduced below.3.2 The mean-square errorThe MSE is defined as(k) = E[e2(k)] = E[|d(k)−y(k)|2].Where R and p are the input-signal correlation matrix and thecross-correlation vector between the reference signal and the input signal, respectively, and are defined asR = E[x(k)x T(k)],p = E[d(k)x T(k)].Note, from the above equations, that R and p are not represented as a function of the iteration k or not time-varying, due to the assumed stationarity of the input and reference signals.From Equation (2.5), the gradient vector of the MSE function with respect to the adaptive filter coefficient vector is given byThe so-called Wiener solution w o, that minimizes the MSE cost function, is obtained by equating the gradient vector in Equation (2.8) to zero. Assuming that R is non-singular, one gets that3.3 The instantaneous square errorThe MSE is a cost function that requires knowledge of the error function e(k) at all time k. For that purpose, the MSE cannot be determined precisely in practice and is commonly approximated by other cost functions. The simpler form to estimate the MSE function is to work with the ISE given byIn this case, the associated gradient vector with respect to the coefficient vector is determined asThis vector can be seen as a noisy estimate of the MSE gradient vector defined in Equation (2.8) or as a precise gradient of the ISE function,which, in its own turn, is a noisy estimate of the MSE cost function seen in Section 2.2.1.3.4 The weighted least-squaresAnother objective function is the WLS function given bywhere 0_⎣ < 1 is the so-called forgetting factor. The parameter ⎣ k−i emphasizes the most recent error samples (where i ≈k) in the composition of the deterministic cost function ⎩D(k), giving to this function the ability of modeling non-stationary processes. In addition, since the WLS function is based on several error samples, its stochastic nature reduces in time, being significantly smaller than the noisy ISE nature as k increases.2.3 Adaptation AlgorithmsIn this section, a number of schemes are presented to find the optimal filter solution for the error functions seen in Section 2.2. Each scheme constitutes an adaptation algorithm that adjusts the adaptive filter coefficients in order to minimize the associated error norm.The algorithms seen here can be grouped into three families, namely the LMS, the DR, and the RLS classes of algorithms. Each group presents particular characteristics of computational complexity and speed ofconvergence, which tend to determine the best possible solution to an application at hand.2.3.1 LMS and normalized-LMS algorithmsDetermining the Wiener solution for the MSE problem requires inversion of matrix R, which makes Equation (2.9) hard to implement in real time. One can then estimate the Wiener solution, in a computationally efficient manner, iteratively adjusting the coefficient vector w at each time instant k, in such a manner that the resulting sequence w(k) converges to the desired w o solution, possibly in a sufficiently small number of iterations. The LMS algorithm is summarized in Table 2.1, where the superscripts . and H denote the complex-conjugate and the Hermitian operations, respectively.The LMS algorithm is very popular and has been widely used due to its extreme simplicity. Its convergence speed, however, is highly dependent on the condition number p of the input-signal autocorrelation matrix[1–3],defined as the ratio between the maximum and minimum Eigen values of this matrix.In the NLMS algorithm, when = 0, one has w(k) = w(k−1) and theupdating halts. When υ= 1, the fastest convergence is attained at the price of a higher misadjustment then the one obtained for 0 <υ< 1.2.3.2 Data-reusing LMS algorithmsAs remarked before, the LMS algorithm estimates the MSE function with the current ISE value, yielding a noisy adaptation process. In this algorithm, information from each time sample k is disregarded in future coefficient updates. DR algorithms [9–11] employ present and past samples of the reference and input signals to improve convergence characteristics of the overall adaptation process.As a generalization of the previous idea, the AP algorithm [13–15] is among the prominent adaptation algorithms that allow trade-off between fast convergence and low computational complexity. By adjusting the number of projections, or alternatively, the number of data reuses, one obtains adaptation processes ranging from that of the NLMS algorithm to that of the sliding-window RLS algorithm [16, 17].2.3.3 RLS-type algorithmsThis subsection presents the basic versions of the RLS family of adaptive algorithms. Importance of the expressions presented here cannot be overstated for they allow an easy and smooth reading of the forthcoming chapters.The RLS-type algorithms have a high convergence speed which is independent of the Eigen value spread of the input correlation matrix. These algorithms are also very useful in applications where the environment is slowly varying.The price of all these benefits is a considerable increase in the computational complexity of the algorithms belonging to the RLS family.The main advantages associated to the QR-decomposition RLS(QRD-RLS) algorithms, as opposed to their conventional RLS counterpart, are the possibility of implementation in systolic arrays and the improved numerical behavior in limited precision environment.2.5 ConclusionIt was verified how adaptive algorithms are employed to adjust the coefficients of a digital filter to achieve a desired time-varying performance in several practical situations. Emphasis was given on the description of several adaptation algorithms. In particular, the LMS and the NLMS algorithms were seen as iterative schemes for optimizing the ISE, an instantaneous approximation of the MSE objective function. Data-reuse algorithms introduced the concept of utilizing data from past time samples, resulting in a faster convergence of the adaptive process. Finally, the RLS family of algorithms, based on the WLS function, was seen as the epitome of fast adaptation algorithms, which use all available signal samples to perform the adaptation process. In general, RLSalgorithms are used whenever fast convergence is necessary, for input signals with a high Eigen value spread, and when the increase in the computational load is tolerable. A detailed discussion on the RLS family of algorithms based on the QR decomposition, which also guarantees good numerical properties in finite-precision implementations, constitutes the main goals of this book. Practical examples of adaptive system identification and channel equalization were presented, allowing one to visualize convergence properties, such as misadjustment, speed, and stability, of several distinct algorithms discussed previously.。
METHODS AND SYSTEMS FOR ADAPTIVE CHANNEL ESTIMATIO
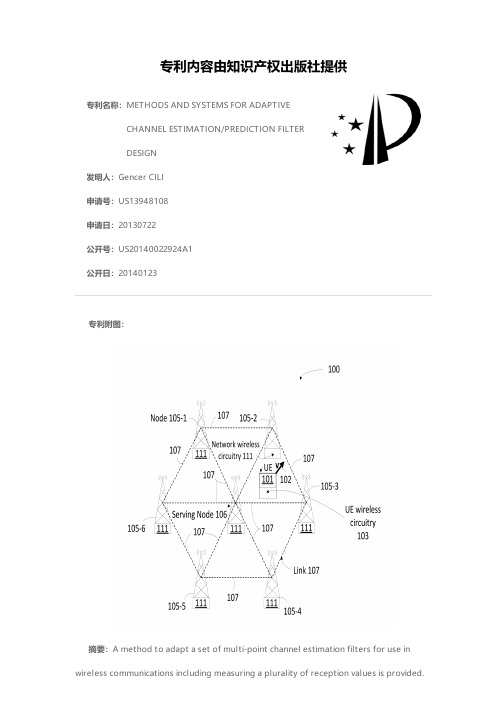
专利名称:METHODS AND SYSTEMS FOR ADAPTIVECHANNEL ESTIMATION/PREDICTION FILTERDESIGN发明人:Gencer CILI申请号:US13948108申请日:20130722公开号:US20140022924A1公开日:20140123专利内容由知识产权出版社提供专利附图:摘要:A method to adapt a set of multi-point channel estimation filters for use in wireless communications including measuring a plurality of reception values is provided.Each reception value included in the plurality of reception values associated with one of the network nodes. The method includes sorting the plurality of reception values according to magnitude and determining a select reception value. The method includes selecting a particular network node from the set of network nodes based on the reception value. The reception value is within a threshold from the select reception value. The method includes adjusting the length of a multi-point channel estimation filter associated with the particular network node. The adjusting the length is based on a lookup table and on the reception value associated with the particular network node, according to some embodiments. User equipment in a wireless network adapted for the above method is also provided.申请人:Apple Inc.地址:Cupertino CA US国籍:US更多信息请下载全文后查看。
一种基于rls自适应滤波跟踪的信道估计方法

2019年第10期信息通信2019 (总第202期)INFORMATION&COMMUNICATIONS(Sum.No202)一种基于RLS自适应滤波跟踪的信道估计方法杨慧霞打唐加山S卢美玲打章歆羡打卓干兵I(1.南京邮电大学通信与信息工程学院;2.南京邮电大学理学院,江苏南京210003)摘要:对于快时变信道⑴,基扩展模型(Basic Expansion Model,BEM)能彳艮好地捕捉信道的时变特性,并能有效模拟信道的传输情况,进而常用于信道建模。
本文提出了一种基于RLS自适应滤波跟踪的信道估计方法。
自适应滤波器本身有一个重要的算法,即递归最小二乘(Recursive least squares,RLS)算法。
文章利用RLS自适应滤波算法对BEM基系数g 进行跟踪,并将其自适应的调整大小,然后对信道响应进行估计。
为验证所提方法的性能,本文对所提算法与LS配合插值算法进行仿真对比。
仿真结果表明,所提方法相较LS算法有很好的估计精度。
关键词:基扩展模型;递归最小二乘;估计精度中图分类号:TN713文献标识码:A 文章编号:1673-1131(2019)10-0004-04Adaptive Channel estimation based on RLSYang Huixia,Tang Jiashan,Lu Meiling,Zhang Xinxian,Zhuo Ganbing (Naming University ofPosts and Telecommunications,College of T elecommunication and Information EnginneringjNanjing^10003,China) Abstract:for fast time-varying channels[1],the basic expansion model(Basic Expansion Model,BEM)can capture the time-varying characteristics of the channel and effectively simulate the transmission of the channel,so it is often used in channel modeling.In this papei;a channel estimation method based on RLS adaptive filtering tracking is proposed.Adaptive filter itself h as an important algorithm,that is,Recursive least Squares(Recursive least squares,RLS).In this paper,the RLS adaptive filtering algorithm is used to track the BEM base coefEicient g,and it is adaptive.The size should be adjusted,and then the channel response is estimated.In order to verify the performance of t he proposed method,the proposed algorithm is simulated and compared with the LS interpolation algorithm.The simulation results show that the proposed method has better estimation accuracy than the LS algorithm.Keywords:basic expansion model;Recursive least Squares;estimation accuracy0引言OFDM(正交频分复用)凭借其高速数据传输能力、高频谱利用率、抗多径干扰、灵活调制方式等优点,已成为高速无线通信系统的主要传输技术。
Seamless rate adaptation for wireless networking

4Hale Waihona Puke 7put of type II HARQ is much higher than type I HARQ, we only discuss type II HARQ in this paper and use HARQ as type II HARQ unless otherwise specified. In HARQ, the incremental redundancy is usually encoded by either a rate compatible code, e.g. punctured Turbo code [13] or fountain code, e.g. Raptor code [19]. However, both of them only have a limited rate dynamic range. To cover a typical range, from 0.5 bit/s/Hz to 6 bit/s/Hz, one way is to use AMC. This will fall back to the dilemma. The other way is to fix the modulation and use a low rate mother code for puncturing. The problem in punctured Turbo code is that a low rate mother code encounters throughput loss at high end [14]. In Raptor code, which is originally designed for binary erasure channel (BEC), a 0.9 outer LDPC code is needed before inner LT code for Gaussian channel [21]. In addition, the overhead of LT code in Gaussian channel is larger than that in BEC. Thus, the outer coding rate and larger overhead make it saturate at a lower transmission rate than punctured Turbo code. We will show this through simulation. To tackle these issues, we need a receiver rate adaptation approach, which can be seamless. By seamless, we mean that the rate adjustment is rateless, smooth and spread on the typical range. The sender continuously generates and transmits coded symbols for a data block. The receiver collects symbols until the data block can be correctly decoded and delivers back an ACK for the transmission of next block. In addition, since we cannot guarantee an accurate channel estimation, the system performance should be insensitive to the channel estimation error. In order to achieve the desired seamless adaptation, we propose a rate compatible modulation (RCM) which is motivated by the analysis of conventional modulation. RCM incrementally generates symbols with fine-grained bit energy allocation. Collecting symbols can progressively accumulate the transmission power until meeting the requirement, which is rateless. Unlike conventional modulation, the generated symbols are directly placed on each dimension of the constellation to modulate signals, so that the symbol locates in the same domain with channel noise. This makes it possible for smooth degradation. The constellation in RCM is fixed and very dense (23 × 23 QAM). By fixing the constellation, there is no need to make decision on modulation selection and avoid the dilemma as mentioned above. Dense constellation contains large amount information and it will not be easily saturated. Therefore, it covers a broad dynamic range. Based on RCM, we design and implement a rate adaptation system, called SRA (seamless rate adaptation), on orthogonal frequency division multiplexing (OFDM) physical layer, and evaluate its performance on a software radio platform. Results show that, while SRA excels others in all four test scenarios, it shows greatest advantage in non-lineof-sight scenario, achieving over 80% gain over conventional 802.11 rate adaptation which is pure AMC, 28.8% and 43.8% improvement on Turbo code and Raptor code based HARQ with AMC. The rest of the paper is organized as follows. Section 2 provides the recent works on receiver rate adaptation. Section 3 presents the motivation and design of RCM, then introduces the modulation and demodulation process and its complexity. Section 4 evaluates RCM through Matlab simulations. Section 5 describes the implementation and evalu-
Signal Processing in Cognitive Radio
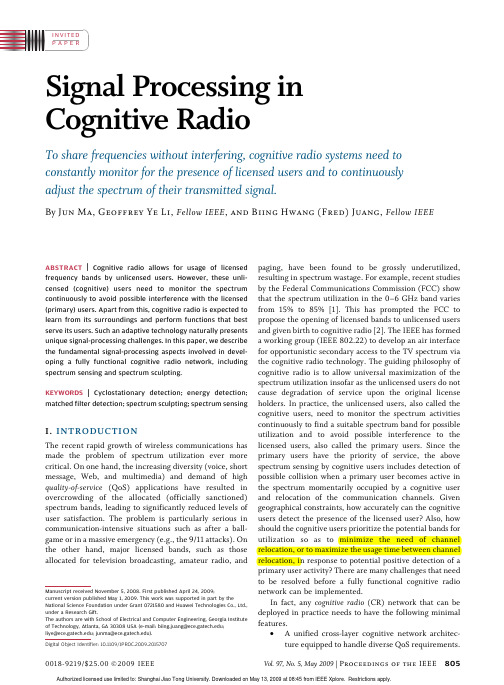
Signal Processing in Cognitive RadioTo share frequencies without interfering,cognitive radio systems need to constantly monitor for the presence of licensed users and to continuously adjust the spectrum of their transmitted signal.By Jun Ma,Geoffrey Ye Li,Fellow IEEE,and Biing Hwang(Fred)Juang,Fellow IEEEABSTRACT|Cognitive radio allows for usage of licensed frequency bands by unlicensed users.However,these unli-censed(cognitive)users need to monitor the spectrum continuously to avoid possible interference with the licensed (primary)users.Apart from this,cognitive radio is expected to learn from its surroundings and perform functions that best serve its users.Such an adaptive technology naturally presents unique signal-processing challenges.In this paper,we describe the fundamental signal-processing aspects involved in devel-oping a fully functional cognitive radio network,including spectrum sensing and spectrum sculpting.KEYWORDS|Cyclostationary detection;energy detection; matched filter detection;spectrum sculpting;spectrum sensingI.INTRODUCTIONThe recent rapid growth of wireless communications has made the problem of spectrum utilization ever more critical.On one hand,the increasing diversity(voice,short message,Web,and multimedia)and demand of high quality-of-service(QoS)applications have resulted in overcrowding of the allocated(officially sanctioned) spectrum bands,leading to significantly reduced levels of user satisfaction.The problem is particularly serious in communication-intensive situations such as after a ball-game or in a massive emergency(e.g.,the9/11attacks).On the other hand,major licensed bands,such as those allocated for television broadcasting,amateur radio,and paging,have been found to be grossly underutilized, resulting in spectrum wastage.For example,recent studies by the Federal Communications Commission(FCC)show that the spectrum utilization in the0–6GHz band varies from15%to85%[1].This has prompted the FCC to propose the opening of licensed bands to unlicensed users and given birth to cognitive radio[2].The IEEE has formed a working group(IEEE802.22)to develop an air interface for opportunistic secondary access to the TV spectrum via the cognitive radio technology.The guiding philosophy of cognitive radio is to allow universal maximization of the spectrum utilization insofar as the unlicensed users do not cause degradation of service upon the original license holders.In practice,the unlicensed users,also called the cognitive users,need to monitor the spectrum activities continuously to find a suitable spectrum band for possible utilization and to avoid possible interference to the licensed users,also called the primary users.Since the primary users have the priority of service,the above spectrum sensing by cognitive users includes detection of possible collision when a primary user becomes active in the spectrum momentarily occupied by a cognitive user and relocation of the communication channels.Given geographical constraints,how accurately can the cognitive users detect the presence of the licensed user?Also,how should the cognitive users prioritize the potential bands for utilization so as to minimize the need of channel relocation,or to maximize the usage time between channel relocation,in response to potential positive detection of a primary user activity?There are many challenges that need to be resolved before a fully functional cognitive radio network can be implemented.In fact,any cognitive radio(CR)network that can be deployed in practice needs to have the following minimal features.•A unified cross-layer cognitive network architec-ture equipped to handle diverse QoS requirements.Manuscript received November5,2008.First published April24,2009;current version published May1,2009.This work was supported in part by theNational Science Foundation under Grant0721580and Huawei Technologies Co.,Ltd.,under a Research Gift.The authors are with School of Electrical and Computer Engineering,Georgia Instituteof Technology,Atlanta,GA30308USA(e-mail:biing.juang@;liye@;junma@).Digital Object Identifier:10.1109/JPROC.2009.2015707Vol.97,No.5,May2009|Proceedings of the IEEE805 0018-9219/$25.00Ó2009IEEE•Efficient spectrum sensing techniques that provide continuous monitoring of the presence of multi-carriers in the CR network.•Dynamic spectrum access methods that adapt to the fluctuating nature of the CR network andallocate bandwidth accordingly.•Adaptive spectrum sculpting at the transmitter end that causes minimal or no interference to theprimary users occupying adjacent bands.To build a highly adaptive radio technology that learns from the environment to best serve its users,novel signa-processing techniques that are channel-aware and cogni-tive need to be developed.In[3],fundamental issues specific to cognitive radio,including radio-scene analysis, channel-state estimation and predictive modeling,transmit-power control,and dynamic spectrum management,have been first investigated,presenting a big picture of cognitive radio.In[4],signal-processing issues in the context of spectrum sensing implementation in CR networks have been investigated.In this paper,we focus on the signal processing aspects demanded of each of the above-mentioned features in CR networks.More precisely,we investigate spectrum sensing and spectrum sculpting in the context of cognitive radio.The rest of this paper is organized as follows.In Section II,we formulate the problem of spectrum sensing in CR networks and describe the basic techniques that may be employed for detection of the primary signal.These techniques require a different amount of knowledge of the primary signal characteristics and are applicable to different scenarios.In order that the primary signal is detected as quickly as possible,it is sometimes necessary to employ more than one cognitive detector for cooperative spectrum sensing.In Section III,we describe cooperative detection techniques to boost the overall detection capability of a CR network.In Section IV,we discuss the problem of adaptive spectrum sculpting that is a necessary functionality in operational CR networks.In particular,we investigate the multicarrier techniques and transform-domain communication system(TDCS)that can be used in the physical layer to accomplish this objective.We conclude this paper with a summary in Section V.II.SPECTRUM SENSING:BASIC TECHNIQUESAs secondary users,CR operators are allowed to utilize a licensed band only when they do not cause interference to the primary users.Spectrum sensing aims at monitoring the usage and characteristics of the covered spectral band(s)and is thus required by CR users both before and during the use of licensed spectrum bands.In this section,we first formulate the problem of spectrum sensing in CR and then describe the basic spectrum sensing techniques,including energy detection,cyclostationary detection,pilot-based coherent detection,and some other detection techniques.A.Spectrum HolesCR is designed to identify and scavenge the spectrum holes in the licensed spectrum bands.A spectrum hole is defined as a licensed spectrum band that can be used by CR users without interfering the primary or licensed users. Generally spectrum holes can be broadly divided into two categories:temporal spectrum holes and spatial spectrum holes,which are shown in Fig.1(a)and1(b),respectively.A temporal spectrum hole means that there is no primary transmission over the spectrum band of interest during the time of sensing(over a reasonable period); hence,this band can be utilized by CR users in the current time slot.For the temporal spectrum holes,as indicated in Fig.1(a),the secondary users are located in the coverage area of the primary transmission.Consequently,it is relatively easy to detect the presence or absence of the primary user activity since CR users only need to have a similar detection sensitivity as regular primary receivers and,more importantly,identifying the presence of a primary signal is much easier than demodulating and decoding it.Therefore,spectrum sensing in this case does not pose a onerous demand on signal processing.A spatial spectrum hole exists when the spectrum band of interest is occupied by the primary transmission only in a restricted area;hence,this band can be utilized by CR users well outside this area[5].1In contrast with the utilization of temporal spectrum holes,secondary users utilizing spatial spectrum holes work outside the coverage of the primary transmission,as indicated in Fig.1(b).Since there are no primary receivers outside the coverage area,secondary communication over the licensed band is allowed if only the secondary transmit-ter does not interfere with the primary transmission and reception within the coverage area.To accomplish this, the secondary transmitter has to successfully detect the presence of the primary signal at any location where the secondary transmission may cause intolerable interfer-ence to the possible nearby primary receiver.Since the secondary users fall outside the coverage area of the primary transmission,detection of the primary signal in this case is a challenging task.Here we elaborate the stringent signal processing requirements for detection of spatial spectrum holes from a geographic perspective as in[6]and[7].Denote P p and P s as the transmit powers of the primary and the secondary transmitters,respectively,P n as the noise power at the primary and the secondary receivers,R as the maximum distance between the primary transmitter and the coverage edge,and D as the minimum distance between the secondary transmitter and the coverage edge.Further defineÀmin as the lowest signal-to-noise ratio(SNR)level at the primary receiver that guarantees successful primary signal reception.In order for the 1In theory,the area is defined not necessarily in the geographical sense but in the communication sense;a covered area or coverage is where the link between a receiver and the target transmitter is sustainable.Ma et al.:Signal Processing in Cognitive Radio806Proceedings of the IEEE|Vol.97,No.5,May2009primary receivers located the furthest from the primary transmitter to be able to detect the primary signal,we must haveP p L ðR ÞP n!Àmin (1)where L ðÁÞdenotes the power loss for a given distance,including the path loss,shadowing,and multipath fading.Define¼P p L ðR ÞP n Àmin(2)which is the power margin factor of the primary system.Under the worst case that the primary receiver lies on the coverage edge,the furthest ðR Þfrom the primary transmitter,and the nearest ðD Þfrom the secondary transmitter,as indicated in Fig.1(b),the received SNR at the primary receiver is given by¼P p L ðR ÞP s L ðD ÞþP n:(3)For to be above Àmin ,it is required thatD !D min¼L À1P p L ðR ÞP s Àmin ÀP nP s ¼L À1ð À1ÞP nP s(4)Fig.1.Spectrum holes for secondary communication.(a)Temporal spectrum holes and (b)spatial spectrum holes.Ma et al.:Signal Processing in Cognitive RadioVol.97,No.5,May 2009|Proceedings of the IEEE807where LÀ1ðÁÞdenotes the inverse function of LðÁÞ. According to(4),D min increases with the transmit power of the secondary transmitter P s and decreases with the power margin factor of the primary system .Equation(4) also indicates that,to avoid intolerable interference with the primary transmission,any secondary transmitter with transmit power P s must successfully detect the presence of primary signal when it is RþD min away from the primary transmitter.In other words,there exists a protection area for the primary transmission in which the presence of primary signal must be successfully detected by secondary transmitters to avoid interfering with the primary trans-mission.As indicated in Fig.1(b),the protection area of primary transmission contains and is larger than the primary transmission coverage.Since the secondary users are required to detect the presence of primary signal well outside the primary transmission coverage,the detection of spatial spectrum holes entails advanced spectrum sensing techniques.Generally,secondary users utilizing spatial spectrum holes must have a much higher detection sensitivity than regular primary receivers.B.Primary Signal DetectionWhether for the detection of temporal or spatial spectrum holes,spectrum sensing in CR involves deciding whether the primary signal is present or not from the observed signals.It can be formulated as the following two hypotheses:yðtÞ¼iðtÞþwðtÞ;H0sðtÞþiðtÞþwðtÞ;H1&(5)where yðtÞis the received signal at the CR user,sðtÞis the primary signal,iðtÞis interference,2and wðtÞis the additive white Gaussian noise(AWGN).In(5),H0and H1denote the hypotheses corresponding to the absence and presence of the primary signal,respectively.Thus from the observa-tion yðtÞ,the CR user needs to decide between H0and H1.For different licensed band(s),primary signals have different characteristics.The802.22wireless regional-area network(WRAN)is developed to work in licensed TV bands; therefore the primary signal is the Advanced Television Systems Committee(ATSC)digital TV signal or the wireless microphone signal.For CR networks to utilize the temporally idle spectrum bands allocated to the3G cellular mobile communication system,the primary signal may be direct spread code-division multiple access(DS-CDMA) signal or orthogonal frequency-division multiplexing (OFDM)signal for its long-term evaluation(LTE)version. In this paper,we do not restrict the primary signal to any waveform.Instead,we exploit the characteristics of the primary signal that are generally known to the public for spectrum sensing.Up to now,various spectrum sensing techniques have been proposed to utilize the characteristics or the a priori knowledge of the primary signal.In the rest of this section,we will focus on the basic spectrum sensing techniques that can be implemented at an individual CR user.Cooperative spectrum sensing techni-ques will be addressed in the next section.C.Energy DetectionEnergy detection[8]is the simplest spectrum sensing technique,which is shown in Fig.2.An energy detector (ED)simply treats the primary signal as noise and decides on the presence or absence of the primary signal based on the energy of the observed signal.Since it does not need any a priori knowledge of the primary signal,the ED is robust to the variation of the primary signal.Moreover,the ED does not involve complicated signal processing and has low complexity.In practice,energy detection is especially suitable for wide-band spectrum sensing.In this case,the simulta-neous sensing of a number of subbands can be realized by simply scanning the power spectral density(PSD)of the received wide-band signal.In practice,it is advisable to complete wide-band spectrum sensing via two stages.In the first stage,low-complexity energy detection is applied to search for possible idle subbands;in the second stage, more advanced spectrum sensing techniques with a higher detection sensitivity and thereby higher complexity,such as cyclostationary detection,are applied to the subband candidates to determine whether they are actually available for secondary usage.Performance Analysis:As indicated in Fig.2,the spectral component on each spectrum subband of interest is obtained from the fast Fourier transform(FFT)of the sampled received signal.Then the test statistics of the ED is obtained as the observed energy summation within M consecutive segments,i.e.,3Y¼P Mm¼1WðmÞj j2;H0P Mm¼1SðmÞþWðmÞj j2;H1&(6)where SðmÞand WðmÞdenote the spectral components of the received primary signal and the white noise on the subband2The difference between interference and noise is that interference is undesired man-made colored signal while noise is white and statistically Gaussian.When interference from various sources in the environment approximates Gaussian and white,it is regarded asnoise.Fig.2.Schematic representation of the energy detector over a spectrum subband of interest.3To facilitate analysis,here we ignore the interference component in the received signal.Ma et al.:Signal Processing in Cognitive Radio808Proceedings of the IEEE|Vol.97,No.5,May2009of interest in the m th segment,respectively.The decision of the ED regarding the subband of interest is given by^¼H 1;if Y >H 0;if Y G&(7)where the threshold is chosen to satisfy a target false-alarm probability.4Without loss of generality,we assume the noise W ðm Þis white complex Gaussian with zero mean and variance two.Define the instantaneous SNR of the received primary signal within the current M segments as¼12M X M m ¼1S ðm Þj j 2:(8)Then the test statistics of the ED Y follows a central chi-square distribution with 2M degrees of freedom under H 0,and a noncentral chi-square distribution with 2M degrees of freedom and a noncentrality parameter ¼P Mm ¼1j S ðm Þj 2¼2M H 1,i.e.,f Y ðY Þ$ 22M ;H 0 22M ð Þ;H 1&(9)where f Y ðY Þdenotes the probability density function (pdf)of Y and 2M and 2M ð Þdenote a central and noncentral chi-square distribution,respectively.Thus the false-alarm probability P F ¼P ðY > jH 0Þcan be expressed as [9]P F ¼ÀM ; 2ÀÁÀðM Þ(10)where ÀðÁÞand ÀðÁ;ÁÞdenote the gamma function and the upper incomplete gamma function [10],respectively.Given the target false-alarm probability,the threshold can be uniquely determined based on (10).Once is determined,the detection probability P D ¼P ðY > jH 1Þcan be obtained by [9]P D ¼Z þ10P ðY > jH 1; Þf ð Þd ¼Z þ10Q M ðffiffiffi p ;ffiffiffip Þf ð Þd(11)where Q M ðÁ;ÁÞis the generalized Marcum Q -function and f ð Þis the pdf of .In [9],a closed-form expression of the detection probability of the ED over general Nakagami fading channel has been derived.Advanced Power Spectrum Estimation Techniques:As indicated in Fig.2,energy-detection-based wide-band spectrum sensing can monitor multiple subbands simulta-neously by scanning the estimated power spectrum of the received wide-band signal.5Obviously,an accurate power spectrum estimate is vital to successful detection of idle subbands.Over a wide spectrum band of interest,some subbands may be occupied by licensed services with significantly different transmit powers and the others may be unoccupied and filled with random noise only.As a result,the power spectrum estimator must have a high spectral dynamic range (SDR),which is defined as the ratio of the maximum and the minimum spectral powers that are distinguishable by this estimator [11].The ED shown in Fig.2applies the simplest periodogram spectral estimator [12].In the following,we will investigate more advanced nonparametric power spectrum estimation techniques that can be applied to improve the performance of energy detection.Here we refer to [11]and investigate power spectral estimation from the perspective of filter bank.The principle of filter bank power spectrum estimation is shown in Fig.3.Suppose there are altogether N subbands in the whole spectrum band of interest.As indicated in the figure,the i th ð0 i N À1Þsubfilter of the filter bank h i ðn Þ¼h ðn Þe j 2 f i n is utilized to extract the spectral component of the received signal over the i th subband with the normalized center frequency f i ¼i =N ,where h ðn Þ,the low-pass filter used to realize the zeroth subband,is called the prototype filter of the filter bank.Obviously,the selection of the prototype filter determines the accuracy of spectral estimation.More specifically,the magnitude of the side lobes of the prototype filter determines the amount of power leakage from the neighboring subbands to the subband of interest and,hence,determines the SDR of the power spectrum estimator.Therefore,in order to improve the performance of power spectrum estimation,the side lobes of the prototype filter should be reduced.4The threshold defines an operating point on the B receiver operating curve [over two performance parameters V the false-alarm probability and the missed detection probability.Here,the false-alarm probability is customarily chosen as the primary operating specification.5Generally speaking,the received stochastic signal at the CR user is nonstationary,i.e.,its statistics varies with time.In practice,the received signal is usually sectioned and analyzed burst by burst,with each burst short enough to ensure pseudostationary and yet long enough to produce an accurate spectral estimate [3].For the classical periodogram spectral estimator,the signal energy on the i th subband is estimated by^Sðfi Þ¼X NÀ1n¼0yðnÞwðnÞeÀj2 f i n2¼X NÀ1n¼0wðnÞe j2 f i n yðNÀ1ÀnÞ2(12)where wðnÞdenotes a symmetric window function, i.e.,wðnÞ¼wðNÀ1ÀnÞ,0n NÀ1.Equation(12) indicates that the periodogram spectral estimator is actually a filter-bank-based one with the prototype filter being the window function wðnÞ.For the simple FFT-based power spectrum estimator in Fig.2,a rectangular window is implied, which,as the prototype filter,has large side lobes and therefore inevitably results in a limited SDR of the estimator.To improve the performance of periodogram spectral estimator,various window functions with small side lobes have been proposed[13]to preprocess the received signal before FFT operation,and this is called tapering in the literature.Though tapering effectively reduces the bias of the power spectrum estimate of a stochastic signal,it unfortu-nately increases its variance because of the information loss caused by the truncation of the time-domain windowing.To mitigate such information loss,it has been proposed to utilize multiple tapers,or prototype filters,in power spectrum estimation so as to reduce the variance of the estimate.This is called multitaper spectral estimation[14].In the multitaper method,a special family of sequences known as the Slepian sequences are usually applied as the tapers for spectral estimation.The Slepian sequences have two basic character-istics:first,their Fourier transforms have the maximal energy concentration in the main lobe,which means the least power leakage when applied for spectral estimation;secondly,they are orthogonal to each other,which means that the estimate outputs of different estimation entities employing different tapers are uncorrelated if only the variation of the signal spectrum over each subband is negligible.As a result, averaging these estimates will result in a minimum variance. Due to the two characteristics of the Slepian sequences,the multitaper spectral estimation has been shown nearly optimal in the sense that it almost achieves the Cramer–Rao bound for a nonparametric spectral estimator[15].Therefore,the multitaper spectral estimation has been recommended by Haykin in[3]as a promising power spectrum estimation technique for energy-detection-based wide-band spectrum sensing.Although the multitaper spectral estimation has nearly optimal performance,it involves high implementation complexity.Recently,filter bank multicarrier communica-tion techniques,including the OFDM offset quadrature amplitude modulation(OQAM),cosine modulated multi-tone(CMT),and filtered multitone(FMT),have been proposed for the physical layer of CR systems[16].Thesefilter bank multicarrier techniques provide a high flexibility in adapting the spectrum shape of the transmitted signal in accordance with the available licensed bands and,at the same time,can better mitigate the mutual interference between the primary and secondary users than the conventional OFDM technique,as will be discussed in detail in Section IV. Since the prototype filters of these filter bank multicarrier techniques have small side lobes,these filter banks can be utilized for accurate power spectral estimation in wide-band spectrum sensing.It has been shown in[11]that such filter bank power spectrum estimators can achieve a similar SDR as the multitaper method with a lower complexity.More importantly,since the filter bank has already been exploited as the physical layer of CR systems for multicarrier communication,it can be utilized for spectrum sensing without any additional cost.Limitations of Energy Detection:So far,we have assumed that the noise power is exactly known for the ED.However, this assumption may be invalid under certain environments. The noise usually consists of the local thermal noise and the environment noise.In practice,the local thermal noise changes over time because of temperature variations at the receiver;the environment noise,which is an aggregation of random signals from various sources in the environment,also varies with time.As a result,though the central limit theorem is invoked to justify the Gaussian nature of noise,it is practically impossible to know the current noise power exactly.Moreover,the existence of variable in-band interference makes the situation even worse.As a result, such noise and interference power uncertainty severely degrades the performance of energy detection.Denote 2n and 2e to be the actual noise and interference power and its estimate,respectively.Suppose there is an x dB uncertainty in the noise and interference power estimation. Then 2e may take any value between 2n10Àðx=10Þand 2n10x=10,i.e., 2e2½10Àðx=10Þ 2n;10x=10 2n .Therefore,the primary signal can be always detected only when the power of the received signal is greater than the threshold 2T¼10x=10 2e.Under the worst case that 2e¼10x=10 2n,2T¼102x=10 2n,and,as a result,the ED will fail to detect the presence of the primary signal if the power of the received primary signal is smaller thanð 2TÀ 2nÞ.That is,there is an SNR wall for the ED given byw¼2TÀ 2n2n¼102x10À1:(13)In other words,for all received SNRs smaller than w,there exists a possibility that the ED is not able to distinguish between the two hypotheses H0and H1,no matter how many samples the ED utilizes.In[17],bounds on the SNR wall of general detectors based on the2k th moments have been pre-sented for a slightly more general noise uncertainty model.Ma et al.:Signal Processing in Cognitive Radio810Proceedings of the IEEE|Vol.97,No.5,May2009Even if the actual noise and interference power is known exactly,the performance of energy detection is still limited by its inability to differentiate the primary signal from the interference and noise,especially when it comes to low-power primary signals such as spread spectrum signals.While the ED is a good option when the CR user knows nothing about the primary signal or when complex-ity is the main concern,more complicated and accurate spectrum sensing techniques that exploit the primary signal characteristics should be employed to achieve a better detection performance.This is particularly meaningful in CR networks since the primary signal has certain signatures,such as modulation,coding,and pilot symbols, that can be extracted to improve the detection capability.In the rest of this section,we will investigate various spectrum sensing techniques that utilize different characteristics of the primary signal and are able to differentiate the primary signal from the interference and noise.D.Cyclostationary DetectionMan-made signals are generally nonstationary.Some of them are cyclostationary,i.e.,their statistics exhibit period-icity,which may be caused by modulation and coding or even be intentionally produced to aid channel estimation and synchronization.Such periodicity can be utilized for detection of a random signal with a particular modulation type in a background of noise and other modulated signals. This is called cyclostationary detection.Mathematically, cyclostationary detection is realized by analyzing the cyclic autocorrelation function(CAF)[18]of the received signal, or,equivalently,its two-dimensional spectrum correlation function(SCF)[19]since the spectrum redundancy caused by periodicity in the modulated signal results in correlation between widely separated frequency components[19],[20].As a spectrum sensing scheme in CR,cyclostationary detection is especially appealing because it is capable of differentiating the primary signal from the interference and noise.Due to its noise rejection property,cyclosta-tionary detection works even in very low SNR region, where the traditional signal detection method,such as the ED,fails.In[4],cyclostationary detectors have been demonstrated to enhance the detection capability,espe-cially in the presence of noise power uncertainty.In[21], joint cyclostationary detection and optimal data fusion has been considered to improve the overall detection perfor-mance of CR networks.The FCC has suggested cyclosta-tionary detectors as a useful alternative to enhance the detection sensitivity in CR networks[1].Consider a typical digitally modulated signal of the form sðtÞ¼XnaðnÞgðtÀnT0Àt0Þ(14)where T0is the symbol period,t0is an unknown timing offset,and gðtÞis the shaping pulse.For simplicity,assume that the sequence aðnÞis stationary with zero mean and variance 2a;then the time-varying autocorrelation func-tion(TVAF)of sðtÞis defined asR sðt; Þ¼E sðtþ ÞsÃðtÞf g¼Xn2agðtþ ÀnT0Àt0ÞgÃðtÀnT0Àt0Þ¼X¼k=T0R ð Þe j2 t(15) whereR ð Þ¼2a e j2 t0T0ÂRGÃðfþ ÞGðfÞe j2 f df; ¼kT00;otherwise8<:(16)and GðfÞis the Fourier transform of gðtÞ.The function R ð Þis called the cyclic autocorrelation function and is called cyclic frequency.As indicated in (16),the CAF at a given cyclic frequency determines the correlation between spectral components of the signal separated in frequency by an amount of .In general[19], the CAF of cyclostationary signals is nonzero only for integer multiples of a fundamental cyclic frequency 0. For the signal model given in(14), 0¼1=T0.Thus,given T0,the CAF can be utilized to determine the presence or absence of the primary signal by evaluating the values of R ð Þat corresponding cyclic frequencies.In practice,cyclostationary detection can be imple-mented in discrete time domain.Let yðnÞ,0n NÀ1, denote the sampled received signal at the CR user;then the discrete-time CAF of the received signal at a cyclic frequency can be estimated as[18]b R ½l ¼1NÀlXNÀlÀ1n¼0yðnþlÞyÃðnÞeÀj2 n;0l LÀ1(17)where L is the number of lags.Then a vector is constructed asb r¼Re b R ð1ÞÈÉ;Re b R ð2ÞÈÉ;...;Re b R ðLÞÈÉÂIm b R ð1ÞÈÉ;Im b R ð2ÞÈÉ;...;Im b R ðLÞÈÉÃ(18)where Re f:g and Im f:g refer to the real and imaginary parts of a complex number,respectively.In[18],the statistical characteristics of b r under H0and H1for a sufficiently large N have been investigated;based on that, an algorithm has been developed to perform CAF-based cyclostationary detection.Ma et al.:Signal Processing in Cognitive Radio Vol.97,No.5,May2009|Proceedings of the IEEE811。
DRAM efficient adaptive MCMC
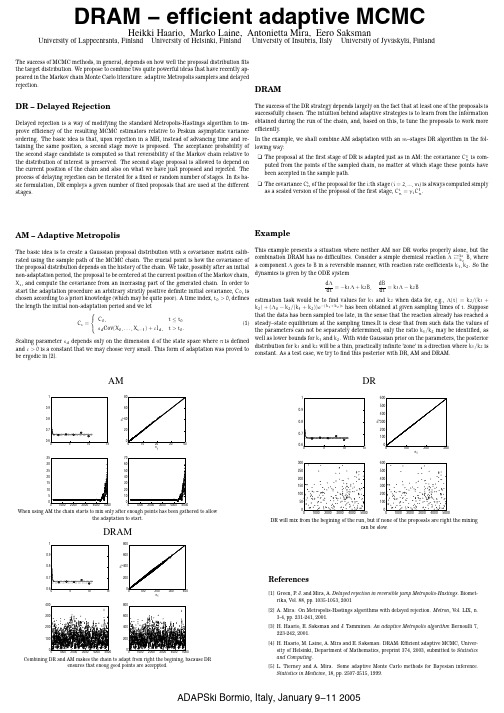
DRAM
The success of the DR strategy depends largely on the fact that at least one of the proposals is successfully chosen. The intuition behind adaptive strategies is to learn from the information obtained during the run of the chain, and, based on this, to tune the proposals to work more efficiently. In the example, we shall combine AM adaptation with an m–stages DR algorithm in the following way: K The proposal at the first stage of DR is adapted just as in AM: the covariance C 1 n is computed from the points of the sampled chain, no matter at which stage these points have been accepted in the sample path. K The covariance Ci n of the proposal for the i:th stage (i = 2, ..., m) is always computed simply 1 as a scaled version of the proposal of the first stage, Ci n = γ i Cn .
一种改进的自适应局部噪声消除滤波算法
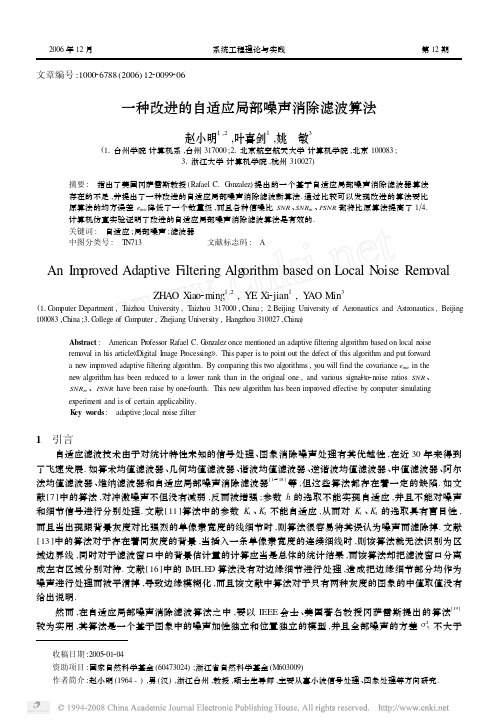
2006年12月系统工程理论与实践第12期 文章编号:100026788(2006)1220099206一种改进的自适应局部噪声消除滤波算法赵小明1,2,叶喜剑1,姚 敏3(11台州学院计算机系,台州317000;21北京航空航天大学计算机学院,北京100083;31浙江大学计算机学院,杭州310027)摘要: 指出了美国冈萨雷斯教授(Rafael C.G onzalez )提出的一个基于自适应局部噪声消除滤波器算法存在的不足,并提出了一种改进的自适应局部噪声消除滤波新算法.通过比较可以发现改进的算法要比原算法的均方误差e mse 降低了一个数量级,而且各种信噪比SNR 、SNR m 、PSNR 都将比原算法提高了1Π4.计算机仿真实验证明了改进的自适应局部噪声消除滤波算法是有效的.关键词: 自适应;局部噪声;滤波器中图分类号: T N713 文献标志码: A An Im proved Adaptive Filtering Alg orithm based on Local N oise Rem ovalZHAO X iao 2ming 1,2,YE X i 2jian 1,Y AO Min 3(11C omputer Department ,T aizhou University ,T aizhou 317000,China ;21Beijing University of Aeronautics and Astronautics ,Beijing 100083,China ;31C ollege of C omputer ,Zhejiang University ,Hangzhou 310027,China )Abstract : American Profess or Rafael C.G onzalez once mentioned an adaptive filtering alg orithm based on local noiserem oval in his article 《Digital Image Processing 》.This paper is to point out the defect of this alg orithm and put forwarda new improved adaptive filtering alg orithm.By comparing this tw o alg orithms ,y ou will find the covariance e mse in thenew alg orithm has been reduced to a lower rank than in the original one ,and various signal 2to 2noise ratios SNR 、SNR m 、PSNR have been raise by one 2fourth.This new alg orithm has been improved effective by computer simulatingexperiment and is of certain applicability.K ey w ords : adaptive ;local noise ;filter收稿日期:2005201204资助项目:国家自然科学基金(60473024);浙江省自然科学基金(M603009) 作者简介:赵小明(1964-),男(汉),浙江台州,教授,硕士生导师,主要从事小波信号处理、图象处理等方向研究.1 引言自适应滤波技术由于对统计特性未知的信号处理、图象消除噪声处理有其优越性,在近30年来得到了飞速发展.如算术均值滤波器、几何均值滤波器、谐波均值滤波器、逆谐波均值滤波器、中值滤波器、阿尔法均值滤波器、维纳滤波器和自适应局部噪声消除滤波器[1~18]等,但这些算法都存在着一定的缺陷.如文献[7]中的算法,对冲激噪声不但没有减弱,反而被增强;参数h 的选取不能实现自适应,并且不能对噪声和细节信号进行分别处理.文献[11]算法中的参数K 1、K 2不能自适应,从而对K 1、K 2的选取具有盲目性,而且当出现跟背景灰度对比强烈的单像素宽度的线细节时,则算法很容易将其误认为噪声而滤除掉.文献[13]中的算法对于存在着同灰度的背景,当插入一条单像素宽度的连续细线时,则该算法就无法识别为区域边界线,同时对于滤波窗口中的背景估计量的计算应当是总体的统计结果,而该算法却把滤波窗口分离成左右区域分别对待.文献[16]中的I MF LE D 算法没有对边缘细节进行处理,造成把边缘细节部分均作为噪声进行处理而被平滑掉,导致边缘模糊化,而且该文献中算法对于只有两种灰度的图象的中值取值没有给出说明.然而,在自适应局部噪声消除滤波算法之中,要以IEEE 会士、美国著名教授冈萨雷斯提出的算法[19]较为实用,其算法是一个基于图象中的噪声加性独立和位置独立的模型,并且全部噪声的方差σ2η不大于去噪滤波器所在的像素点的局部方差σ2L,在这样的假设前提下,建立了一种自适应、局部噪声消除滤波器.其中加性独立是指噪声项中的噪声相互独立,互不干扰,且可以叠加在一起,两个输入之和的响应等于两个响应之和;位置独立是指图象中任一点的响应只取决于在该点的输入值,而与该点的位置无关;而均值给出了计算均值的区域中灰度平均值的度量,而方差给出了这个区域的平均对比度的度量.如滤波器作用于局部区域Sxy,滤波器在中心化区域中任何点(x,y)上的滤波器响应基于以下4个量:1)g(x,y)为噪声图象在点(x,y)上的灰度值;2)σ2η为干扰f(x,y)以形成g(x,y)的噪声方差;3)m L为在S x,y上像素点的局部均值;4)σ2L为在S x,y上像素点的局部方差.其中(x,y)为像素点坐标;Sxy为作用于图像局部区域内的自适应滤波器所在的m×n矩形窗口;f(x,y)为原未加噪声时图像中(x,y)点所在像素的灰度值.假如^f(x,y)为任意点(x,y)滤波后图象的灰度值,则滤波器的预期性能如下:1)如果σ2η为零,滤波器应该简单地返回g(x,y)的值,即在零噪声的情况下g(x,y)等同于f(x,y);2)如果局部方差与σ2η是高相关的,那么滤波器返回一个g(x,y)的近似值,即高局部方差与边缘相关,应保留边缘;3)如果两个方差相等,则滤波器返回区域S xy上像素的算术均值.这种情况发生在局部面积与全部图象有相同特性的条件下,此时局部噪声可以简单地用求平均来降低.基于这些假设的自适应局部澡声消除滤波算法中的^f可表示为:^f(x,y)=g(x,y)-[g(x,y)-m L]σ2ηΠσ2L,(1)在(1)式中唯一需要知道的或要估计的量是全局噪声的方差σ2η,其他参数需要从Sxy中的各个像素点(x, y)计算得到,在这里滤波器窗口已被中心化.在(1)式中,假设σ2η≤σ2L,而模型中的噪声是加性和位置独立的,因此,这是一个合理的假设,因为Sxy是g (x,y)的子集.然而在实际中很少有确切的σ2η的知识,结果很可能违反假设条件,由于这个原因,常对(1)式构建一个测试,以便当条件σ2ηΠσ2L发生时,把比率设置为1,这使该滤波器成为非线性的,但是它可以防止由于缺乏图象噪声方差的知识而产生的无意义结果,即负灰度值,依赖于mL值.2 冈萨雷斯滤波算法存在的局限尽管冈萨雷斯自适应、局部噪声消除滤波器是一个较实用的算法,但通过对其研究分析,发现(1)式自适应局部噪声消除滤波算法存在下列缺点:1)滤波图象像素灰度值^f对于小的局部方差σ2L过于敏感.令^f′|σL=2[g(x,y)-m L]σ2ηΠσ3L,当σL→0时,则^f′|σL →∞,此时方差σ2L微小的变化,都导致滤波图象像素灰度值^f的剧烈变化.2)(1)式自适应滤波器算法所得到的图象不够平滑;3)缺少对于强噪声弱边缘的处理.因为(1)式滤波器只有针对弱噪声强边缘情况的处理,即(1)式由于对于小的局部方差σ2L过于敏感,只针对σ2η≤σ2L的情况下进行处理.而对于σ2η>σ2L的情况下(1)式的自适应滤波器则无能为力.其原因为:当噪声图象中噪声的几率(P oise)增大时,噪声方差σ2η也随着增大,如图1(a)所示.当处于弱噪声强边缘图象情形下,随着强边缘图象的噪声的几率的增大,噪声将使图象的强边缘越来越模糊,结果边缘的界限越来越不清晰,从而噪声使得强边缘图象的局部方差逐渐减小,如图1(b)所示.当处于强噪声弱边缘图象情形下,随着弱边缘图象的噪声的几率的增大,噪声将使图象的灰度平坦区域越来越少,从而噪声使得弱边缘图象的局部方差逐渐增大,如图1(c)所示.但值得注意的是,当噪声的几率逐渐增大时,最终图象的噪声方差σ2η,与弱噪声强边缘图象和强噪声弱边缘图象的局部方差σ2L都将收敛于一个实数值,而不可能无限的增大或减小.当然,随着图象的噪声001系统工程理论与实践2006年12月图1 噪声与噪声方差之间的关系图2 全局方差与局部噪声方差的关系的不断增加,图象的原有的有用信息将逐渐被噪声覆盖,有用信息在噪声图象中所占的比重将不断地下降.再进一步,到达极端情形,可以预见图象的原有信息将完全被噪声所覆盖,这时候局部图象与全局图象有着相同的特性,从而图象的局部方差σ2L 将等同于噪声方差σ2η.这一概念的具体描述如图2所示.图2(a )描述了弱噪声强边缘图象的局部方差变化,图2(b )描述了强噪声弱边缘图象的局部方差变化,图中的虚线为y =x 的函数曲线.通过以上分析可知,当|ση-σL |值趋向于0时,则图象局部区域S xy 为噪声的可能性越大;同样,当|ση-σL |值越大,则图象局部区域S xy 为噪声的可能性越小.本文根据冈萨雷斯滤波算法存在的不足,提出一种改进的自适应局部噪声消除滤波算法.3 改进的自适应局部噪声消除滤波算法在上述已有的假设与分析的前提下,本文提出了一种新的自适应、局部噪声消除滤波器算法,对(1)式的自适应滤波器算法进行了改进,改进后的滤波算法应具有的预期性能为:1)如果σ2η为零,滤波器应该简单地返回g (x ,y )的值,即在零噪声的情况下g (x ,y )等同于f (x ,y );2)如果局部方差与σ2η高相关,那么滤波器要返回一个g (x ,y )的近似值,即高局部方差与边缘相关,应保留边缘;3)如果两个方差相等,则滤波器返回区域S xy 上像素的算术均值.这种情况发生在局部面积与全部图象有相同特性的条件下,此时局部噪声可以简单地用求平均来降低.4)如果两个方差越接近,则自适应滤波器所在的局部区域存在噪声的可能性越大,则此点应当更多地被滤波平滑掉.5)要求滤波图象像素灰度值^f 对于小的局部方差σ2L 平缓变化,同时兼有针对强噪声弱边缘图象和弱噪声强边缘图象的自适应消除噪声的能力.基于以上改进的应有的预期性能和假设下的前提下,改进后的自适应滤波算法中的^f 可表示为:^f (x ,y )=g (x ,y )-[g (x ,y )-m L ][exp (ση)-1]|ση-σL |,(2) 由于把原来置于分母的σ2L 转变成指数形式,消除了复原图象像素灰度值^f 对于小的局部方差σ2L 的敏感性.令^f ′|σL=-[g (x ,y )-m L ][exp (ση)-1]|ση-σL |ln[exp (ση)-1]sgn[σL -ση],当σL →0时,则^f ′|σL→[g (x ,y )-m L ][exp (ση)-1]σηln[exp (ση)-1](常数),此时方差σ2L 微小的变化,不会导致滤波图象像素灰度值^f 的剧烈变化.与此同时带来的好处是比原来的滤波器算法增添了对于强噪声弱边缘图象的去噪处理能力.而图象的去噪中一个关键参数为自适应滤波器区域中局部方差σ2L ,改进后的算法较之已有的滤波算法降低了对σ2L 的敏感性.也就意味着利用改进后的自适应滤波算法得到的滤波图像比原算法得到的滤波图象具有更平滑性.改进后的自适应滤波算法及操作步骤如下:1)采集噪声图象g 的灰度数据,并得出图象的坐标范围M 和N ;2)计算噪声方差σ2η;101第12期一种改进的自适应局部噪声消除滤波算法3)确定滤波模板S xy 的尺寸;4)赋初值x =-1;5)令x =x +1;6)判断x >M ?成立则退出滤波计算;7)令y =0;8)求取经定位(x ,y )的像素点的灰度值g (x ,y );9)求取经定位的像素点所在滤波窗口S xy 中的局部均值m L ;10)求取经定位的像素点所在滤波窗口S xy 中的局部方差σ2L ;11)用自适应滤波公式(2)计算滤波后图象像素灰度值;12)令y =y +1;13)判断y >N ?成立则转入第5)步,不成立则转入第8)步.4 两滤波算法结果比较分析衡量一个滤波器消除噪声图象质量的好坏通常有两种方法:主观评判法和客观评判法.411 主观评判结果图3 仿真实验结果为了验证本文提出的改进的自适应局部噪声消除滤波算法的有效性、优越性,利用Matlab ,对Lena 图象分别用原自适应局部噪声消除滤波算法和改进的自适应局部噪声消除滤波算法进行计算机仿真实验.实验采用3×3滤波模板,并选取了均值为0,发生概率为015%的高斯(gaussian )噪声作为图象的噪声污染模型.实验结果如图3所示.从滤波后的图象中可以非常明显地看出,图3(c )中的Lena 的左侧鼻子和嘴角两侧的白色噪声在图3(d )中已经消失;图3(d )中的Lena 的额角要比图3(c )显然得更平滑.通过图象对比可以知道,本文提出的自适应局部噪声消除滤波算法确实要比原有算法的消除噪声能力来得优越.412 客观评判结果客观准则是对滤波后的图象与原始未加噪声图象的误差进行定量计算,一般是对整个图象或图象中一个指定的区域进行某种平均计算,以得到均方误差[2].设一个原始图象为{f (i ,j ),0≤i ≤M -1,0≤j ≤N -1},相应的滤波后的图象为{^f (i ,j ),0≤i ≤M -1,0≤j ≤N -1},误差图象为{e (i ,j )=f (i ,j )-^f (i ,j ),0≤i ≤M -1,0≤j ≤N -1},那么均方误差可表示为:e mse =1MN ∑M -1i =0∑N -1j =0e 2(i ,j ),(3) 信噪比可表示为:SNR =10lg ∑M -1i =0∑N -1j =0f 2(i ,j )∑M -1i =0∑N -1j =0[f (i ,j )-^f (i ,j )]2.(4) 信噪比是用分贝表示压缩图象的定量性能评价,是最常用的一种图象质量比较方法.此外,还有另一种信噪比表示方法,它先对原始图象进行去均值处理,然后再计算信噪比,其表达式为:f (i ,j )=1MN ∑M -1i =0∑N -1j =0f (i ,j ),(5)201系统工程理论与实践2006年12月SNR m =10lg ∑M -1i =0∑N -1j =0[f (i ,j )- f (i ,j )]2∑M -1i =0∑N -1j =0[f (i ,j )-^f (i ,j )]2.(6) 而在文献[2]中,比较图象质量的最常用的方法是峰值信噪比(PS NR ).设f max =2K -1,K 是表示一个像素点用所的2进制位数,则PSNR =10lg MNf 2max∑M -1i =0∑N -1j =0[f (i ,j )-^f (i ,j )]2.(7) 在许多采集的视频序列和图象的应用中,常取K =8,本文也将用K =8,并直接将f max =255代入上式.通过编程计算,得到新算法与原算法的图象在多种图象质量标准下的比较数据如下.两种算法的图象质量标准的数据比较均方误差e mse信噪比SNR 信噪比SNR m 信噪比PSNR 原滤波算法0.002518.482812.293826.0206新滤波算法0.0007537723.689817.500931.2276从上表数据可以看出,新的自适应滤波算法的均方误差e mse 要比原自适应滤波算法小得多,降低了一个数量级;而各种信噪比SNR 、SNR m 、PSNR 都比原来滤波算法要高出1Π4左右.结果可以表明,本文提出的自适应、局部噪声消除滤波器算法要比原算法确实来得优越.5 结论通过理论分析和仿真实验的主、客观评判结果都表明:本文提出的改进的自适应局部噪声消除滤波算法对于小的局部方差的鲁棒抗噪性能要优于原有的算法,并且可以使用该算法来处理强噪声弱边缘图象.同时,采用改进后的算法进行滤波所得的图象的均方误差减小是相当可观的,而且各种信噪比的提升均达到1Π4左右.因此,本文提出的改进的自适应局部噪声消除滤波算法在实际应用中具有较大的推广价值.参考文献:[1] 尚久铨.卡尔曼滤波法在结构动态参数估计中的应用[J ].地震工程与工程振动,1991,6.Shang Jiuquan.Application of K alman filter method to dynamic parameter estimation of structures[J ].Earthquake Engineering and Engineering Vibration ,1991,6.[2] 吴森堂,张水祥,陈海尔.一类结构随机跳变系统的自适应滤波方法[J ].北京航空航天大学学报,2002,28(3):287-290.Wu Sentang ,Zhang Shuixiang ,Chen Haier.Approach for adaptive filter of systems with random changing structure s[J ].Journal of Beijing University of Aeronautics and Astronautics ,2002,28(3):287-290.[3] 刘广军,吴晓平,郭晶.一种数值稳定的次优并行Sage 自适应滤波器[J ].测绘学报,2002,31(4):8-10.Liu G uangjun ,Wu X iaoping ,G uo Jing.A numerically stable sub 2optimal parallel Sage adaptive filter [J ].Acta G eodaetica et Cartographica S inica ,2002,31(4):8-10.[4] 魏瑞轩,韩崇昭,张宗麟.鲁棒总体均方最小自适应滤波:算法与分析[J ].电子学报,2002,30(7)1023-1026.Wei Ruixuan ,Han Chongzhao ,Zhang Z onglin ,et al.R obust total least mean square adaptive filter :Alg orithm and analysis[J ].Acta E lectronica S inica ,2002,30(7):1023-1026.[5] 高鹰,谢胜利.基于矩阵广义逆递推的自适应滤波算法[J ].电子学报,2002,30(7):1032-1034.G ao Y ing ,X ie Shengli.An adaptive filtering alg orithm Based on recursionof generalized inverse matrix[J ].Acta E lectronica S inica ,2002,30(7):1032-1034.[6] 赵昕,李杰.一类加权全局迭代参数卡尔曼滤波算法[J ].计算力学学报,2002,19(4).Zhao X in ,Li Jie.A weighted globaliteration parametric K alman filter alg orithm[J ].Chinese Journal of C omputational Mechanics ,301第12期一种改进的自适应局部噪声消除滤波算法401系统工程理论与实践2006年12月2002,19(4).[7] 景晓军,李剑峰,熊玉庆.静止图像的一种自适应平滑滤波算法[J].通信学报,2002,23(10).Jing X iaojun,Li Jian feng,X iong Y uqing.An adaptive sm ooth filter alg orithms of still images[J].Journal of China Inatitute ofC ommunications,2002,23(10).[8] 郭业才,赵俊渭,陈华伟,王峰.基于高阶累积量符号相干累积自适应滤波算法[J].系统仿真学报,2002,14(10).G uo Y ecai,Zhao Junwei,Chen Huawei,Wang Feng.An adaptive filtering alg orithm of higher2order cumulant2based signed coherentintegration[J].Journal of System S imulation,2002,14(10).[9] 高鹰,谢胜利.一种基于三阶累积量的准则及自适应滤波算法[J].电子与信息学报,2002,24(9).G ao Y ing,X ie Shengli.A third2order cumulant2based criterion and adaptive filtering alg orithm[J].Journal of E lectronics andIn formation T echnology,2002,24(9).[10] 高为广,何海波.自适应抗差联邦滤波算法[J].测绘学院学报,2004,21(1).G ao Weiguang,He Haibo.Adaptive robust federated filtering[J].Journal of Institute of Surveying and Mapping,2004,21(1).[11] 单永高,范影乐,庞全.基于差分的细节保留自适应滤波算法[J].计算机工程与科学,2004,26(7).Shan Y onggao,Fan Y ingle,Pang Quan.A difference2based detail2preserving adaptive filter alg orithm[J].C omputer Engineering &Science,2004,26(7).[12] 张恒,雷志辉,丁晓华.一种改进的中值滤波算法[J].中国图象图形学报,2004,9(4).Zhang Heng,Lei Zhihui,Ding X iaohua.An improved method of median filter[J].Journal of Image and G raphics,2004,9(4). [13] 李剑峰,乐光新,尚勇.基于改进型D2S证据理论的决策层融合滤波算法[J].电子学报,2004,32(7).Li Jian feng,Y ue G uangxin,Shang Y ong.Decision2level fusion filtering alg orithm based on advanced D2S theory of evidence[J].Acta E lectronica S inica,2004,32(7).[14] 孔祥玉,魏瑞轩,,马红光.一种稳定的总体最小二乘自适应滤波算法[J].西安交通大学学报,2004,38(8).K ong X iangyu,Wei Ruixuan,Han Chongzhao,et al.S table total least mean square adaptive filter alg orithm[J].Journal of X i′an Jiaotong University,2004,38(8).[15] Chen J,Sato Y,T amura S.Orientation space filtering for multiple orientation line segmentation[J].IEEE T rans Pattern Analysis andMachine Intelligence,2000,22(5):417-429.[16] 曲延锋,徐健,李卫军,等.有效去除图像中脉冲噪声的新型滤波算法[J].计算机辅助设计与图形学学报,2003,15(4):397-401.Qu Y an feng,Xu Jian,Li Weijun,et al.New effective filtering alg orithm for the rem oval of impulse noise from images[J].Journal ofC omputer2aided Design&C omputer G raphics,2003,15(4):397-401.[17] 朱虹,李永盛,乐静,等.一种肾切片图像的肾小球边界增强滤波算法[J].中国生物医学工程学报,2003,22(4):370-373.Zhu H ong,Li Y ongsheng,Le Jing,et al.A filter alg orithm for edge emphasis of glomerulusin the image of kidney tissue slices[J].Chinese Journal of Biomedical Engineering,2003,22(4):370-373.[18] 赵乘麟.一种新的保边缘滤波算法[J].电子与信息学报,2003,25(11):1581-1584.Zhao Chenglin.A new edge2reserve filtering alg orithm[J].Journal of E lectronics and In formation T echnology,2003,25(11):1581 -1584.[19] 冈萨雷斯(美).数字图象处理(第二版)[M].北京:电子工业出版社,2003.Rafael C.G onzalez Digital Image Processing[M].Second Edition.Beijing:Publishing H ouse of E lectronics Industry,2003.。