Connected rigidity matroids and unique realizations of graphs
The study of the properties of metal complexes

The study of the properties of metalcomplexesMetal complexes are compounds formed by metal ions and ligands. They have unique properties that make them important in various fields like medicine, material science, nanotechnology, and environmental studies. Understanding the properties of metal complexes is crucial for designing new compounds with specific functions. In this article, we will discuss the important properties of metal complexes and their applications.Ligand Exchange ReactionsLigand exchange reactions are the most important properties of metal complexes. In these reactions, a ligand replaces another ligand from the metal ion, which results in the formation of a new complex. The rate of the ligand exchange reaction depends on the steric and electronic factors. Steric factors such as the size of the ligand and the geometry of the metal complex affect the rate of the reaction. Electronic factors such as the charge and the electronegativity of the ligand also play a crucial role.Applications: Ligand exchange reactions are important in catalysis and bioinorganic chemistry. Many catalysts use metal complexes because of their ability to undergo ligand exchange reactions. In bioinorganic chemistry, metal complexes play a crucial role in the transport and storage of metals in the body.Redox PropertiesRedox properties refer to the ability of a metal complex to undergo oxidation-reduction reactions. In these reactions, the metal ion changes its oxidation state, resulting in the formation of a new complex. The redox potential of a metal complex depends on the ligands and the metal ion. The presence of strong field ligands like cyanide and carbon monoxide increases the redox potential of the metal complex.Applications: Redox properties of metal complexes are important in electrochemistry and catalysis. In electrochemistry, metal complexes are used as mediators in the redoxreactions. In catalysis, many reactions are driven by the redox properties of metal complexes.Optical PropertiesOptical properties refer to the ability of a metal complex to exhibit color and luminescence. The color of a metal complex depends on the nature of the ligands and the metal ion. The presence of d-orbitals in the metal ion gives rise to the color of the complex. The luminescence of a metal complex depends on the energy gap between the ground and excited states.Applications: Optical properties of metal complexes are important in materials science, biology, and medicine. In materials science, metal complexes are used as dyes, pigments, and sensors. In biology, metal complexes are used as probes to study biological processes. In medicine, metal complexes are used as imaging agents and anticancer drugs.Structural PropertiesStructural properties refer to the geometry and bonding of a metal complex. The geometry of a metal complex is determined by the coordination number, the ligands, and the metal ion. The bonding in a metal complex can be classified as covalent, ionic, and dative.Applications: Structural properties of metal complexes are important in catalysis, material science, and environmental studies. In catalysis, the geometry and bonding of metal complexes determine their catalytic activity. In material science, the structure of metal complexes determines their thermal stability and mechanical properties. In environmental studies, the structure of metal complexes plays a crucial role in their toxicology and biodegradation.In conclusion, the study of the properties of metal complexes is crucial for their understanding and applications. The properties of metal complexes like ligand exchange reactions, redox properties, optical properties, and structural properties make them important in various fields like medicine, material science, nanotechnology, andenvironmental studies. Further research in this field will lead to the development of new compounds with specific functions.。
【国家自然科学基金】_乳腺图像_基金支持热词逐年推荐_【万方软件创新助手】_20140802
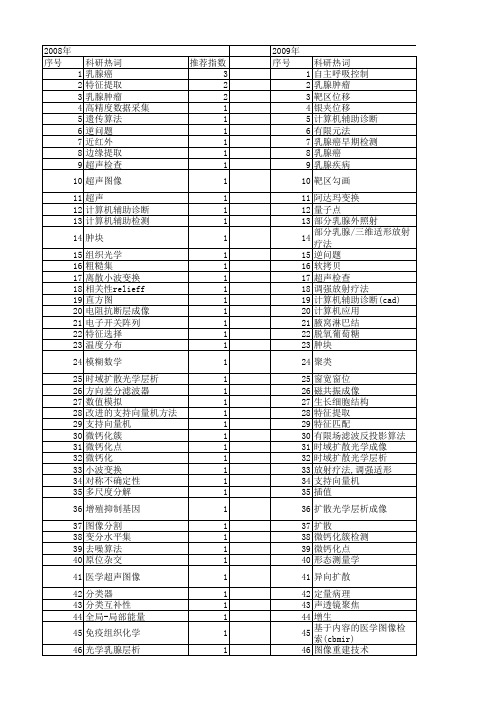
1 1 1 1 1 1 1 1 1 1 1 1 1 1 1 1 1 1 1 1 1 1 1 1 1
53 54 55 56 57 58 59 60 61 62 63 64 65 66 67 68 69 70 71 72 73 74 75 76 77 78 79 80 81 82 83 84 85 86
科研热词 自主呼吸控制 乳腺肿瘤 靶区位移 银夹位移 计算机辅助诊断 有限元法 乳腺癌早期检测 乳腺癌 乳腺疾病 靶区勾画 阿达玛变换 量子点 部分乳腺外照射 部分乳腺/三维适形放射疗法 逆问题 软拷贝 超声检查 调强放射疗法 计算机辅助诊断(cad) 计算机应用 腋窝淋巴结 脱氧葡萄糖 肿块 聚类 窗宽窗位 磁共振成像 生长细胞结构 特征提取 特征匹配 有限场滤波反投影算法 时域扩散光学成像 时域扩散光学层析 放射疗法,调强适形 支持向量机 插值 扩散光学层析成像 扩散 微钙化簇检测 微钙化点 形态测量学 异向扩散 定量病理 声透镜聚焦 增生 基于内容的医学图像检索(cbmir) 图像重建技术 图像处理,计算机辅助 图像分割技术 图像分割 可视化 发射型计算机 双视角乳腺x线图像
小波融合 1 小波变换 1 多普勒特征 1 多尺度几何分析 1 多尺度 1 增强高通滤波 1 图像引导 1 图像分析仪 1 图像分割 1 噪声功率谱 1 去噪后增强 1 原发性淋巴瘤 1 卵巢癌 1 医学图像 1 分类器融合 1 分类 1 分形维参数 1 光学乳腺层析成像 1 代价敏感 1 乳腺超声影像学报告及数据系统 1 乳腺超声图像的影像报告与数据系统 1 乳腺超声 1 乳腺肿瘤/放射疗法 1 乳腺肿块 1 乳腺结节 1 乳腺数字图像 1 乳腺x光图像 1 乳腺 1 s期细胞比率 1 roi检测 1 normalized cut 1 multi-agent 1 dna含量 1 cascade结构 1
用于均相FRET检测的纳米稀土荧光生物标记材料的研制项目通过评审

在 国家 9 3项 目、国家 自然科 学 7 基金委重大研究计划 、中科 院 “ 引进 海
外 杰 出 人 才 ” 百 人 计 划 等 项 目的 支 持
下, 由合肥物质科 学研 究院智能所刘 锦 淮研 究员和 黄行 九研究 员领 导的课 题
组 首 次 制 备 了 具 有 蛋 形 水 母 状 的
土 荧光生物 标记材 料 的制备 、表面修
饰 、 物 偶 联 、 谱 性 质 以及 生ቤተ መጻሕፍቲ ባይዱ 应 用 生 光
将原本三 维 ( D)或二维 (D)无序 3 2 的导 电聚合物 结构降 为一 维 ( D)有 1
检测等方面 的研究 , 取得 了系列创新性
研究成果 : ( ) 制 了一 类 新 颖 的稀 土 发 光 I研 纳米材料 , 巧妙 地 将 时 间分 辨 技 术 和 荧
具 有 简 单 器 件 工 艺 的 高 性 能 双 侧 栅 晶
体 、 比优化聚合环境 , 功实现 了吡 参 成 咯 单体在 含纳米 孔 的金 属有机 框架 中 的高度有序聚合 。 该 小组在世界上 率先使用含有 仅
lm 大 小 一 维 孔 道 的金 属 有 机 框 架 为 n
用 水 中,只 要微量 浓度 即产生 毒性 效
读者 服 务 卡 编 号 0 2 1 2 1 -
件、 纳米传感器 、分子机器 等器件 中获
得应用 。
读者服务卡编 号 03 2 口
yAI O 勃姆石) i /eO4 - O H( @SO2 3 空心 磁 F
性 微 球 ,能 够 高 效 地 去 除水 中 的 P 2、 b+
cu2 、 Hg2 + +
元研究员主持完成 。 审委 员会 一致认 评
为 , 项 目研 究 处 于 国 际前 沿 领 域 , 该 项
DBN-Hinton-简洁
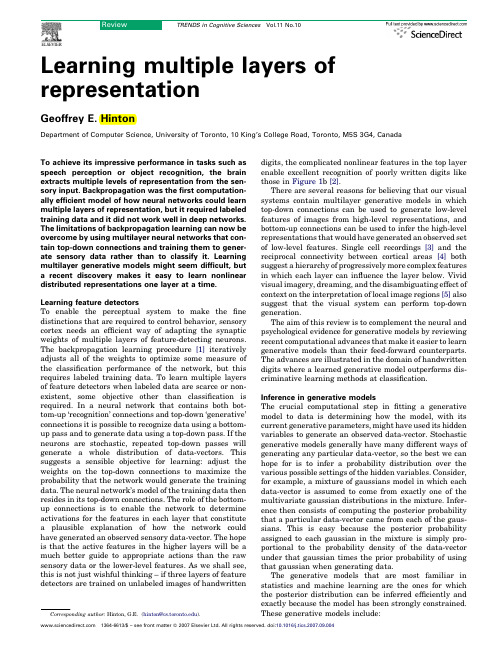
Learning multiple layers of representationGeoffrey E.HintonDepartment of Computer Science,University of Toronto,10King’s College Road,Toronto,M5S 3G4,CanadaTo achieve its impressive performance in tasks such as speech perception or object recognition,the brain extracts multiple levels of representation from the sen-sory input.Backpropagation was the first computation-ally efficient model of how neural networks could learn multiple layers of representation,but it required labeled training data and it did not work well in deep networks.The limitations of backpropagation learning can now be overcome by using multilayer neural networks that con-tain top-down connections and training them to gener-ate sensory data rather than to classify it.Learning multilayer generative models might seem difficult,but a recent discovery makes it easy to learn nonlinear distributed representations one layer at a time.Learning feature detectorsTo enable the perceptual system to make the fine distinctions that are required to control behavior,sensory cortex needs an efficient way of adapting the synaptic weights of multiple layers of feature-detecting neurons.The backpropagation learning procedure [1]iteratively adjusts all of the weights to optimize some measure of the classification performance of the network,but this requires labeled training data.To learn multiple layers of feature detectors when labeled data are scarce or non-existent,some objective other than classification is required.In a neural network that contains both bot-tom-up ‘recognition’connections and top-down ‘generative’connections it is possible to recognize data using a bottom-up pass and to generate data using a top-down pass.If the neurons are stochastic,repeated top-down passes will generate a whole distribution of data-vectors.This suggests a sensible objective for learning:adjust the weights on the top-down connections to maximize the probability that the network would generate the training data.The neural network’s model of the training data then resides in its top-down connections.The role of the bottom-up connections is to enable the network to determine activations for the features in each layer that constitute a plausible explanation of how the network could have generated an observed sensory data-vector.The hope is that the active features in the higher layers will be a much better guide to appropriate actions than the raw sensory data or the lower-level features.As we shall see,this is not just wishful thinking –if three layers of feature detectors are trained on unlabeled images of handwrittendigits,the complicated nonlinear features in the top layer enable excellent recognition of poorly written digits like those in Figure 1b [2].There are several reasons for believing that our visual systems contain multilayer generative models in which top-down connections can be used to generate low-level features of images from high-level representations,and bottom-up connections can be used to infer the high-level representations that would have generated an observed set of low-level features.Single cell recordings [3]and the reciprocal connectivity between cortical areas [4]both suggest a hierarchy of progressively more complex features in which each layer can influence the layer below.Vivid visual imagery,dreaming,and the disambiguating effect of context on the interpretation of local image regions [5]also suggest that the visual system can perform top-down generation.The aim of this review is to complement the neural and psychological evidence for generative models by reviewing recent computational advances that make it easier to learn generative models than their feed-forward counterparts.The advances are illustrated in the domain of handwritten digits where a learned generative model outperforms dis-criminative learning methods at classification.Inference in generative modelsThe crucial computational step in fitting a generative model to data is determining how the model,with its current generative parameters,might have used its hidden variables to generate an observed data-vector.Stochastic generative models generally have many different ways of generating any particular data-vector,so the best we can hope for is to infer a probability distribution over the various possible settings of the hidden variables.Consider,for example,a mixture of gaussians model in which each data-vector is assumed to come from exactly one of the multivariate gaussian distributions in the mixture.Infer-ence then consists of computing the posterior probability that a particular data-vector came from each of the gaus-sians.This is easy because the posterior probability assigned to each gaussian in the mixture is simply pro-portional to the probability density of the data-vector under that gaussian times the prior probability of using that gaussian when generating data.The generative models that are most familiar in statistics and machine learning are the ones for which the posterior distribution can be inferred efficiently and exactly because the model has been strongly constrained.These generative modelsinclude:TRENDS in Cognitive Sciences Vol.11No.10Corresponding author:Hinton,G.E.(hinton@ ).1364-6613/$–see front matter ß2007Elsevier Ltd.All rights reserved.doi:10.1016/j.tics.2007.09.004Factor analysis –in which there is a single layer of gaussian hidden variables that have linear effects on the visible variables (see Figure 2).In addition,independent gaussian noise is added to each visible variable [6–8].Given a visible vector,it is impossible to infer the exact state of the factors that generated it,but it is easy to infer the mean and covariance of the gaussian posterior distribution over the factors,and this is sufficient to enable the parameters of the model to be improved. Independent components analysis –which generalizes factor analysis by allowing non-gaussian hidden vari-ables,but maintains tractable inference by eliminating the observation noise in the visible variables and using the same number of hidden and visible variables.These restrictions ensure that the posterior distribution collapses to a single point because there is only one setting of the hidden variables that can generate each visible vector exactly [9–11].Mixture models –in which each data-vector is assumed to be generated by one of the component distributions in the mixture and it is easy to compute the density under each of the component distributions.If factor analysis is generalized to allow non-gaussian hidden variables,it can model the development of low-level visual receptive fields [12].However,if the extra con-straints used in independent components analysis are not imposed,it is no longer easy to infer,or even to represent,the posterior distribution over the hidden vari-ables.This is because of a phenomenon known as explain-ing away [13](see Figure 3b).Multilayer generative modelsGenerative models with only one hidden layer are much too simple for modeling the high-dimensional and richly struc-tured sensory data that arrive at the cortex,but they have been pressed into service because,until recently,it was too difficult to perform inference in the more complicated,multilayer,nonlinear models that are clearly required.There have been many attempts to develop multilayer,nonlinear models [14–18].In Bayes nets (also called belief nets),which have been studied intensively in artificial intelligence and statistics,the hidden variables typically have discrete values.Exact inference is possible if every variable only has a few parents.This can occur in Bayes nets that are used to formalize expert knowledge in limited domains [19],but for more densely connected Bayes nets,exact inference is generally intractable.It is important to realize that if some way can be found to infer the posterior distribution over the hidden variables for each data-vector,learning a multilayer generative model is relatively straightforward.Learning is also straightforward if we can get unbiased samples from the posterior distribution.In this case,we simply adjust the parameters so as to increase the probability that the sampled states of the hidden variables in each layerwouldFigure 1.(a)The generative model used to learn the joint distribution of digit images and digit labels.(b)Some test images that the network classifies correctly even though it has never seen thembefore.Figure 2.The generative model used in factor analysis.Each real-valued hidden factor is chosen independently from a gaussian distribution,N (0,1),with zero mean and unit variance.The factors are then linearly combined using weights (W jk )and gaussian observation noise with mean (m i )and standard deviation (s i )is added independently to each real-valued variable (i ).TRENDS in Cognitive Sciences Vol.11No.10429generate the sampled states of the hidden or visible variables in the layer below.In the case of the logistic belief net shown in Figure 3a,which will be a major focus of this review,the learning rule for each training case is a version of the delta rule [20].The inferred state,h i ,of the ‘postsynaptic’unit,i ,acts as a target value and the prob-ability,ˆhi ,of activating i given the inferred states,h j ,of all the ‘presynaptic’units,j ,in the layer above acts as a prediction:D w ji /h j ðh i Àˆhi Þ(Equation 1)where D w ji is the change in the weight on the connectionfrom j to i .If i is a visible unit,h i is replaced by the actual state of i in the training example.If training vectors are selected with equal probability from the training set and the hidden states are sampled from their posterior distribution given the training vector,the learning rule in Equation 1has a positive expected effect on the probability that the gen-erative model would produce exactly the N training vectors if it was run N times.Approximate inference for multilayer generative modelsThe generative model in Figure 3a is defined by the weights on its top-down,generative connections,but it also has bottom-up,recognition connections that can be used to perform approximate inference in a single,bottom-up pass.The inferred probability that h j =1is s (S i h i r ij ).This inference procedure is fast and simple,but it is incorrect because it ignores explaining away.Surprisingly,learning is still possible with incorrect inference because there is a more general objective function that the learning rule in Equation 1is guaranteed to improve [21,22].Instead of just considering the log probability of gen-erating each training case,we can also take the accuracy ofthe inference procedure into account.Other things being equal,we would like our approximate inference method to be as accurate as possible,and we might prefer a model that is slightly less likely to generate the data if it enables more accurate inference of the hidden representations.So it makes sense to use the inaccuracy of inference on each training case as a penalty term when maximizing the log probability of the observed data.This leads to a new objective function that is easy to maximize and is a ‘vari-ational’lower-bound on the log probability of generating the training data [23].Learning by optimizing a vari-ational bound is now a standard way of dealing with the intractability of inference in complex generative models [24–27].An approximate version of this type of learning has been proposed as a model of learning in sensory cortex (Box 1),but it is slow in deep networks if the weights are initialized randomly.A nonlinear module with fast exact inferenceWe now turn to a different type of model called a ‘restricted Boltzmann machine’(RBM)[28](Figure 4a).Despite its undirected,symmetric connections,the RBM is the key to finding an efficient learning procedure for deep,directed,generative models.Images composed of binary pixels can be modeled by using the hidden layer of an RBM to model the higher-order correlations between pixels [29].To learn a good set of feature detectors from a set of training images,we start with zero weights on the symmetric connections between each pixel i and each feature detector j .Then we repeatedly update each weight,w ij ,using the difference between two measured,pairwise correlations D w i j ¼e ð<v i h j >data À<v i h i >recon Þ(Equation 2)where e is a learning rate,<v i h j >data is the frequency with which pixel i and feature detector j are on togetherwhenFigure 3.(a)A multilayer belief net composed of logistic binary units.To generate fantasies from the model,we start by picking a random binary state of 1or 0for each top-level unit.Then we perform a stochastic downwards pass in which the probability,ˆhi ,of turning on each unit,i ,is determined by applying the logistic function s (x )=1/(1+exp(Àx ))to the total input S j h j w ji that i receives from the units,j ,in the layer above,where h j is the binary state that has already been chosen for unit j .It is easy to give each unit an additional bias,but to simplify this review biases will usually be ignored.r ij is a recognition weight.(b)An illustration of ‘explaining away’in a simple logistic belief net containing two independent,rare,hidden causes that become highly anticorrelated when we observe the house jumping.The bias of À10on the earthquake unit means that,in the absence of any observation,this unit is e 10times more likely to be off than on.If the earthquake unit is on and the truck unit is off,the jump unit has a total input of 0,which means that it has an even chance of being on.This is a much better explanation of the observation that the house jumped than the odds of e À20,which apply if neither of the hidden causes is active.But it is wasteful to turn on both hidden causes to explain the observation because the probability of them both happening is approximately e À20.430TRENDS in Cognitive Sciences Vol.11No.10the feature detectors are being driven by images from the training set,and <v i h j >recon is the corresponding frequency when the feature detectors are being driven by recon-structed images.A similar learning rule can be used for the biases.Given a training image,we set the binary state,h j ,of each feature detector to be 1with probabilityp ðh j ¼1Þ¼s ðb j þXiv i w i j Þ(Equation 3)where s ( )is the logistic function,b j is the bias of j and v i isthe binary state of pixel i .Once binary states have been chosen for the hidden units we produce a ‘reconstruction’of the training image by setting the state of each pixel to be 1with probabilityp ðv i ¼1Þ¼s ðb i þXjh j w i j Þ(Equation 4)The learned weights and biases directly determine theconditional distributions p (h j v )and p (v j h )using Equations 3and 4.Indirectly,the weights and biases define the joint and marginal distributions p (v ,h ),p (v )and p (h ).Sampling from the joint distribution is difficult,but it can be done by using ‘alternating Gibbs sampling’.This starts with a random image and then alternates between updating all of the features in parallel using Equation 3and updating all of the pixels in parallel using Equation 4.After Gibbs sampling for sufficiently long,the network reaches ‘thermal equilibrium’.The states of pixels and feature detectors still change,but the probability of finding the system in any particular binary configuration does not.By observing the fantasies on the visible units at thermal equilibrium,we can see the distribution over visible vectors that the model believes in.The RBM has two major advantages over directed models with one hidden layer.First,inference is easy because there is no explaining away:given a visible vector,the posterior distribution over hidden vectors factorizes into a product of independent distributions for each hidden unit.So to get a sample from the posterior we simply turn on each hidden unit with a probability given by Equation 3.Box 1.The wake-sleep algorithmFor the logistic belief net shown in Figure 3a,it is easy to improve the generative weights if the network already has a good set of recognition weights.For each data-vector in the training set,the recognition weights are used in a bottom-up pass that stochastically picks a binary state for each hidden unit.Applying the learning rule in Equation 1will then follow the gradient of a variational bound on how well the network generates the training data [22].It is not so easy to compute the derivatives of the bound with respect to the recognition weights,but there is a simple,approx-imate learning rule that works well in practice.If we generate fantasies from the model by using the generative weights in a top-down pass,we know the true causes of the activities in each layer,so we can compare the true causes with the predictions made by the approximate infererence procedure and adjust the recognition weights,r ij ,to maximize the probability that the predictions are correct:D r i j /h i h j Às ðXih i r i j Þ (Equation 5)The combination of approximate inference for learning the gen-erative weights,and fantasies for learning the recognition weights isknown as the ‘wake-sleep’algorithm [22].Figure 4.(a)Two separate restricted Boltzmann machines (RBMs).The stochastic,binary variables in the hidden layer of each RBM are symmetrically connected to the stochastic,binary variables in the visible layer.There are no connections within a layer.The higher-level RBM is trained by using the hidden activities of the lower RBM as data.(b)The composite generative model produced by composing the two RBMs.Note that the connections in the lower layer of the composite generative model are directed.The hidden states are still inferred by using bottom-up recognition connections,but these are no longer part of the generative model.TRENDS in Cognitive Sciences Vol.11No.10431Second,as we shall see,it is easy to learn deep directed networks one layer at a time by stacking yer-by-layer learning does not work nearly as well when the individual modules are directed,because each directed module bites off more than it can chew:it tries to learn hidden causes that are marginally independent.This is generally beyond its abilities so it settles for a generative model in which independent causes generate a poor approximation to the data distribution.Learning many layers of features by composing RBMs After an RBM has been learned,the activities of its hidden units(when they are being driven by data)can be used as the‘data’for learning a higher-level RBM.To understand why this is a good idea,it is helpful to consider decompos-ing the problem of modeling the data distribution,P0,into two subproblems by picking a distribution,P1,that is easier to model than P0.Thefirst subproblem is to model P1and the second subproblem is to model the transform-ation from P1to P0.P1is the distribution obtained by applying p(h j v)to the data distribution to get the hidden activities for every data-vector in the training set.P1is easier for an RBM to model than P0because it is obtained from P0by allowing an RBM to settle towards a distri-bution that it can model perfectly:its equilibrium distri-bution.The RBM’s model of P1is p(h),the distribution over hidden vectors when the RBM is sampling from its equi-librium distribution.The RBM’s model of the transform-ation from P1to P0is p(v j h).After thefirst RBM has been learned,we keep p(v j h)as part of the generative model and we keep p(h j v)as a quick way of performing inference,but we throw away our model of P1and replace it by a better model that is obtained, recursively,by treating P1as the training data for the second-level RBM.This leads to the composite generative model shown in Figure4b.To generate from this model we need to get an equilibrium sample from the top-level RBM, but then we simply perform a single downwards pass through the bottom layer of weights.So the composite model is a curious hybrid whose top two layers form an undirected associative memory and whose lower layers form a directed generative model.It is shown in reference [30]that if the second RBM is initialized appropriately,the gain from building a better model of P1always outweighs the loss that comes from the fact that p(h j v)is no longer the correct way to perform inference in the composite genera-tive model shown in Figure4b.Adding another hidden layer always improves a variational bound on the log probability of the training data unless the top-level RBM is already a perfect model of the data it is trained on. Modeling images of handwritten digitsFigure1a shows a network that was used to model the joint distribution of digit images and their labels.It was learned one layer at a time and the top-level RBM was trained using‘data’-vectors that were constructed by concatenat-ing the states of ten winner-take-all label units with500 binary features inferred from the image.After greedily learning one layer of weights at a time,all the weights were fine-tuned using a variant of the wake-sleep algorithm(see reference[30]for details).Thefine-tuning significantly improves the ability of the model to generate images that resemble the data,but without the initial layer-by-layer learning,thefine-tuning alone is hopelessly slow.The model was trained to generate both a label and an image,but it can be used to classify new images.First,the recognition weights are used to infer binary states for the 500feature units in the second hidden layer,then alter-nating Gibbs sampling is applied to the top two layers with these500features heldfixed.The probability of each label is then represented by the frequency with which it turns ing an efficient version of this method,the network significantly outperforms both backpropagation and sup-port vector machines[31]when trained on the same data [30].A demonstration of the model generating and recog-nizing digit images is at my homepage(www.cs.toronto. edu/$hinton).Instead offine-tuning the model to be better at generating the data,backpropagation can be used to fine-tune it to be better at discrimination.This works extremely well[2,20].The initial layer-by-layer learning finds features that enable good generation and then the discriminativefine-tuning slightly modifies these features to adjust the boundaries between classes.This has the great advantage that the limited amount of information in the labels is used only for perturbing features,not for creating them.If the ultimate aim is discrimination it is possible to use autoencoders with a single hidden layer instead of restricted Boltzmann machines for the unsuper-vised,layer-by-layer learning[32].This produces the best results ever achieved on the most commonly used bench-mark for handwritten digit recognition[33].Modeling sequential dataThis review has focused on static images,but restricted Boltzmann machines can also be applied to high-dimen-sional sequential data such as video sequences[34]or the joint angles of a walking person[35].The visible and hidden units are given additional,conditioning inputs that come from previous visible frames.The conditioning inputs have the effect of dynamically setting the biases of the visible and hidden units.These conditional restricted Boltzmann machines can be composed by using the sequence of hidden activities of one as the training data for the next.This creates multilayer distributed repres-entations of sequences that are far more powerful than the representations learned by standard methods such as hidden Markov models or linear dynamical systems[34]. Concluding remarksA combination of three ideas leads to a novel and effective way of learning multiple layers of representation.Thefirst idea is to learn a model that generates sensory data rather than classifying it.This eliminates the need for large amounts of labeled data.The second idea is to learn one layer of representation at a time using restricted Boltz-mann machines.This decomposes the overall learning task into multiple simpler tasks and eliminates the infer-ence problems that arise in directed generative models. The third idea is to use a separatefine-tuning stage to improve the generative or discriminative abilities of the composite model.432TRENDS in Cognitive Sciences Vol.11No.10 Versions of this approach are currently being applied to tasks as diverse as denoising images[36,37],retrieving documents[2,38],extracting opticalflow[39],predicting the next word in a sentence[40]and predicting what movies people will like[41].Bengio and Le Cun[42]give further reasons for believing that this approach holds great promise for artificial intelligence applications,such as human-level speech and object recognition,that have proved too difficult for shallow methods like support vector machines[31]that cannot learn multiple layers of representation.The initial successes of this approach to learning deep networks raise many ques-tions(see Box2).There is no concise definition of the types of data for which this approach is likely to be successful,but it seems most appropriate when hidden variables generate richly structured sensory data that provide plentiful infor-mation about the states of the hidden variables.If the hidden variables also generate a label that contains little information or is only occasionally observed,it is a bad idea to try to learn the mapping from sensory data to labels using discriminative learning methods.It is much more sensiblefirst to learn a generative model that infers the hidden variables from the sensory data and then to learn the simpler mapping from the hidden variables to the labels.AcknowledgementsI thank Yoshua Bengio,David MacKay,Terry Sejnowski and my past and present postdoctoral fellows and graduate students for helping me to understand these ideas,and NSERC,CIAR,CFI and OIT for support.References1Rumelhart, D.E.et al.(1986)Learning representations by back-propagating errors.Nature323,533–5362Hinton,G.E.and Salakhutdinov,R.R.(2006)Reducing the dimensionality of data with neural networks.Science313,504–507 3Lee,T.S.et al.(1998)The role of the primary visual cortex in higher level vision.Vision Res.38,2429–24544Felleman,D.J.and Van Essen,D.C.(1991)Distributed hierarchical processing in the primate cerebral cortex.Cereb.Cortex1,1–475Mumford, D.(1992)On the computational architecture of the neocortex.II.The role of cortico-cortical loops.Biol.Cybern.66, 241–2516Dayan,P.and Abbott,L.F.(2001)Theoretical Neuroscience: Computational and Mathematical Modeling of Neural Systems,MIT Press7Roweis,S.and Ghahramani,Z.(1999)A unifying review of linear gaussian models.Neural Comput.11,305–3458Marks,T.K.and Movellan,J.R.(2001)Diffusion networks,products of experts,and factor analysis.In Proceedings of the International Conference on Independent Component Analysis(Lee,T.W.et al., eds),pp.481–485,/article/marks01diffusion.html9Bell,A.J.and Sejnowski,T.J.(1995)An information-maximization approach to blind separation and blind deconvolution.Neural Comput.7,1129–115910Hyva¨rinen,A.et al.(2001)Independent Component Analysis,Wiley 11Bartlett,M.S.et al.(2002)Face recognition by independent component analysis.IEEE Trans.Neural Netw.13,1450–146412Olshausen, B.A.and Field, D.(1996)Emergence of simple-cell receptivefield properties by learning a sparse code for natural images.Nature381,607–60913Pearl,J.(1988)Probabilistic Inference in Intelligent Systems:Networks of Plausible Inference,Morgan Kaufmann14Lewicki,M.S.and Sejnowski,T.J.(1997)Bayesian unsupervised learning of higher order structure.In Advances in Neural Information Processing Systems(Vol.9)(Mozer,M.C.et al.,eds),pp.529–535,MIT Press15Hoyer,P.O.and Hyva¨rinen,A.(2002)A multi-layer sparse coding network learns contour coding from natural images.Vision Res.42, 1593–160516Portilla,J.et al.(2004)Image denoising using Gaussian scale mixtures in the wavelet domain.IEEE Trans.Image Process.12, 1338–135117Schwartz,O.et al.(2006)Soft mixer assignment in a hierarchical generative model of natural scene statistics.Neural Comput.18,2680–271818Karklin,Y.and Lewicki,M.S.(2003)Learning higher-order structures in natural work14,483–49919Cowell,R.G.et al.(2003)Probabilistic Networks and Expert Systems, Springer20O’Reilly,R.C.(1998)Six principles for biologically based computational models of cortical cognition.Trends Cogn.Sci.2,455–46221Hinton,G.E.and Zemel,R.S.(1994)Autoencoders,minimum description length,and Helmholtz free energy.Adv.Neural Inf.Process.Syst.6,3–1022Hinton,G.E.et al.(1995)The wake-sleep algorithm for self-organizing neural networks.Science268,1158–116123Neal,R.M.and Hinton,G.E.(1998)A new view of the EM algorithm that justifies incremental,sparse and other variants.In Learning in Graphical Models(Jordan,M.I.,ed.),pp.355–368,Kluwer Academic Publishers24Jordan,M.I.et al.(1999)An introduction to variational methods for graphical models.Mach.Learn.37,183–23325Winn,J.and Jojic,N.(2005)LOCUS:Learning object classes with unsupervised segmentation,Tenth IEEE International Conference on Computer Vision(Vol.1),pp.756–763,IEEE Press26Bishop, C.M.(2006)Pattern Recognition and Machine Learning, Springer27Bishop,C.M.et al.(2002)VIBES:a variational inference engine for Bayesian networks.Adv.Neural Inf.Process.Syst.15,793–80028Hinton,G.E.(2007)Boltzmann Machines,Scholarpedia29Hinton,G.E.(2002)Training products of experts by minimizing contrastive divergence.Neural Comput.14,1711–1800Box2.Questions for future researchHow might this type of algorithm be implemented in cortex?Inparticular,is the initial perception of sensory input closelyfollowed by a reconstruction that uses top-down connections?Computationally,the learning procedure for restricted Boltzmannmachines does not require a‘pure’reconstruction.All that isrequired is that there are two phases that differ in the relativebalance of bottom-up and top-down influences,with synapticpotentiation in one phase and synaptic depression in the other.Can this approach deal adequately with lateral connections andinhibitory interneurons?Currently,there is no problem inallowing lateral interactions between the visible units of a‘semirestricted’Boltzmann machine[30,43].Lateral interactionsbetween the hidden units can be added when these become thevisible units of the higher-level,semirestricted Boltzmann ma-chine.This makes it possible to learn a hierarchy of undirectedMarkov random fields,each of which has directed connections tothe field below as suggested in ref.[44].This is a more powerfultype of generative model because each level only needs toprovide a rough specification of the states at the level below:Thelateral interactions at the lower level can settle on the fine detailsand ensure that they obey learned constraints.Can we understand the representations that are learned in thedeeper layers?In a generative model,it is easy to see what adistributed pattern of activity over a whole layer means:simplygenerate from it to get a sensory input vector(e.g.an image).It ismuch harder to discover the meaning of activity in an individualneuron in the deeper layers because the effects of that activitydepend on the states of all the other nonlinear neurons.The factthe some neurons in the ventral stream can be construed as facedetectors is intriguing,but I can see no good reason to expectsuch simple stories to be generally applicable.TRENDS in Cognitive Sciences Vol.11No.10433 。
Graph Regularized Nonnegative Matrix
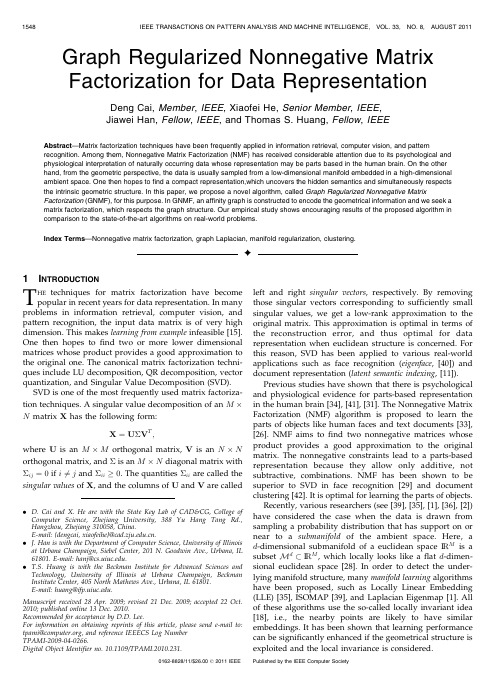
Ç
1 INTRODUCTION
HE
techniques for matrix factorization have become popular in recent years for data representation. In many problems in information retrieval, computer vision, and pattern recognition, the input data matrix is of very high dimension. This makes learning from example infeasible [15]. One then hopes to find two or more lower dimensional matrices whose product provides a good approximation to the original one. The canonical matrix factorization techniques include LU decomposition, QR decomposition, vector quantization, and Singular Value Decomposition (SVD). SVD is one of the most frequently used matrix factorization techniques. A singular value decomposition of an M Â N matrix X has the following form: X ¼ UÆVT ; where U is an M Â M orthogonal matrix, V is an N Â N orthogonal matrix, and Æ is an M Â N diagonal matrix with Æij ¼ 0 if i 6¼ j and Æii ! 0. The quantities Æii are called the singular values of X, and the columns of U and V are called
Finding community structure in networks using the eigenvectors of matrices
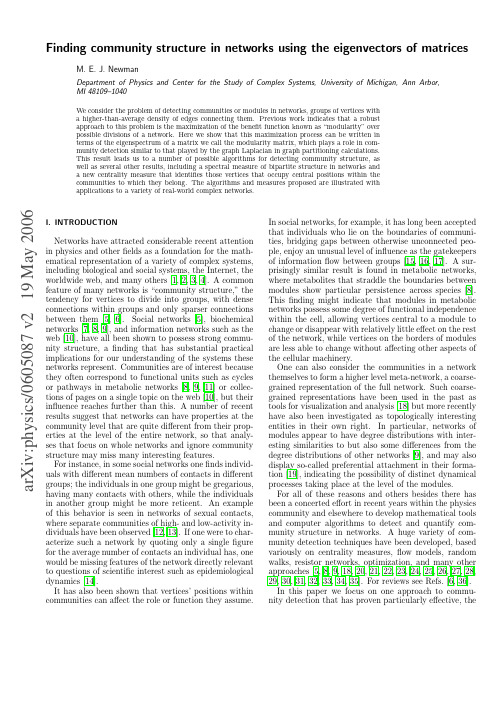
M. E. J. Newman
Department of Physics and Center for the Study of Complex Systems, University of Michigan, Ann Arbor, MI 48109–1040
We consider the problem of detecting communities or modules in networks, groups of vertices with a higher-than-average density of edges connecting them. Previous work indicates that a robust approach to this problem is the maximization of the benefit function known as “modularity” over possible divisions of a network. Here we show that this maximization process can be written in terms of the eigenspectrum of a matrix we call the modularity matrix, which plays a role in community detection similar to that played by the graph Laplacian in graph partitioning calculations. This result leads us to a number of possible algorithms for detecting community structure, as well as several other results, including a spectral measure of bipartite structure in neteasure that identifies those vertices that occupy central positions within the communities to which they belong. The algorithms and measures proposed are illustrated with applications to a variety of real-world complex networks.
Geometric Modeling
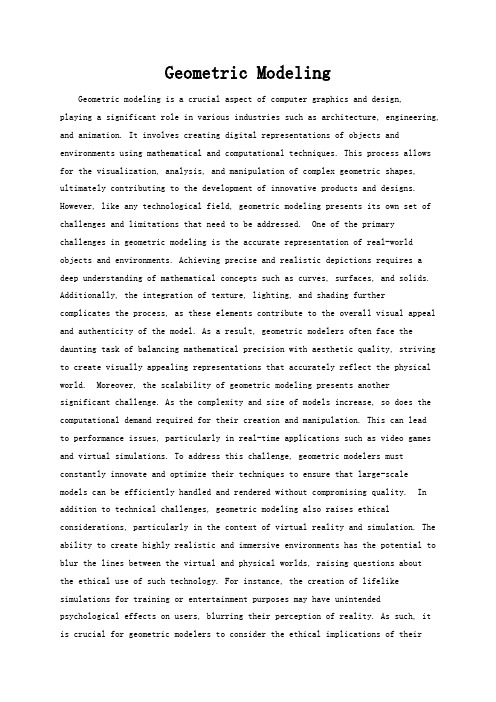
Geometric ModelingGeometric modeling is a crucial aspect of computer graphics and design,playing a significant role in various industries such as architecture, engineering, and animation. It involves creating digital representations of objects and environments using mathematical and computational techniques. This process allows for the visualization, analysis, and manipulation of complex geometric shapes, ultimately contributing to the development of innovative products and designs. However, like any technological field, geometric modeling presents its own set of challenges and limitations that need to be addressed. One of the primary challenges in geometric modeling is the accurate representation of real-world objects and environments. Achieving precise and realistic depictions requires a deep understanding of mathematical concepts such as curves, surfaces, and solids. Additionally, the integration of texture, lighting, and shading furthercomplicates the process, as these elements contribute to the overall visual appeal and authenticity of the model. As a result, geometric modelers often face the daunting task of balancing mathematical precision with aesthetic quality, striving to create visually appealing representations that accurately reflect the physical world. Moreover, the scalability of geometric modeling presents anothersignificant challenge. As the complexity and size of models increase, so does the computational demand required for their creation and manipulation. This can leadto performance issues, particularly in real-time applications such as video games and virtual simulations. To address this challenge, geometric modelers must constantly innovate and optimize their techniques to ensure that large-scale models can be efficiently handled and rendered without compromising quality. In addition to technical challenges, geometric modeling also raises ethical considerations, particularly in the context of virtual reality and simulation. The ability to create highly realistic and immersive environments has the potential to blur the lines between the virtual and physical worlds, raising questions aboutthe ethical use of such technology. For instance, the creation of lifelike simulations for training or entertainment purposes may have unintended psychological effects on users, blurring their perception of reality. As such, itis crucial for geometric modelers to consider the ethical implications of theirwork and strive to use their skills responsibly. Despite these challenges, the field of geometric modeling continues to evolve, driven by advancements in technology and the increasing demand for realistic digital representations. Innovations such as 3D scanning and printing have revolutionized the way geometric models are created, allowing for the direct conversion of physical objects into digital form. Additionally, the integration of artificial intelligence and machine learning has the potential to streamline the modeling process, automating repetitive tasks and enabling more efficient creation of complex geometries. Ultimately, the future of geometric modeling holds great promise, as it continues to push the boundaries of what is possible in the digital realm. By addressing the challenges and ethical considerations inherent to the field, geometric modelers can harness the full potential of their craft, contributing to the creation of captivating virtual worlds, groundbreaking designs, and innovative technological solutions. As technology continues to advance, the role of geometric modeling will only become more prominent, shaping the way we interact with and perceive the world around us.。
An Overview of Recent Progress in the Study of Distributed Multi-agent Coordination
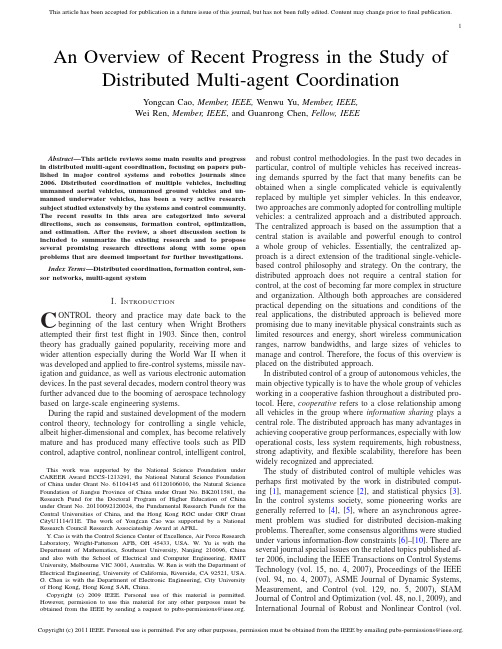
An Overview of Recent Progress in the Study of Distributed Multi-agent CoordinationYongcan Cao,Member,IEEE,Wenwu Yu,Member,IEEE,Wei Ren,Member,IEEE,and Guanrong Chen,Fellow,IEEEAbstract—This article reviews some main results and progress in distributed multi-agent coordination,focusing on papers pub-lished in major control systems and robotics journals since 2006.Distributed coordination of multiple vehicles,including unmanned aerial vehicles,unmanned ground vehicles and un-manned underwater vehicles,has been a very active research subject studied extensively by the systems and control community. The recent results in this area are categorized into several directions,such as consensus,formation control,optimization, and estimation.After the review,a short discussion section is included to summarize the existing research and to propose several promising research directions along with some open problems that are deemed important for further investigations.Index Terms—Distributed coordination,formation control,sen-sor networks,multi-agent systemI.I NTRODUCTIONC ONTROL theory and practice may date back to thebeginning of the last century when Wright Brothers attempted theirfirst testflight in1903.Since then,control theory has gradually gained popularity,receiving more and wider attention especially during the World War II when it was developed and applied tofire-control systems,missile nav-igation and guidance,as well as various electronic automation devices.In the past several decades,modern control theory was further advanced due to the booming of aerospace technology based on large-scale engineering systems.During the rapid and sustained development of the modern control theory,technology for controlling a single vehicle, albeit higher-dimensional and complex,has become relatively mature and has produced many effective tools such as PID control,adaptive control,nonlinear control,intelligent control, This work was supported by the National Science Foundation under CAREER Award ECCS-1213291,the National Natural Science Foundation of China under Grant No.61104145and61120106010,the Natural Science Foundation of Jiangsu Province of China under Grant No.BK2011581,the Research Fund for the Doctoral Program of Higher Education of China under Grant No.20110092120024,the Fundamental Research Funds for the Central Universities of China,and the Hong Kong RGC under GRF Grant CityU1114/11E.The work of Yongcan Cao was supported by a National Research Council Research Associateship Award at AFRL.Y.Cao is with the Control Science Center of Excellence,Air Force Research Laboratory,Wright-Patterson AFB,OH45433,USA.W.Yu is with the Department of Mathematics,Southeast University,Nanjing210096,China and also with the School of Electrical and Computer Engineering,RMIT University,Melbourne VIC3001,Australia.W.Ren is with the Department of Electrical Engineering,University of California,Riverside,CA92521,USA.G.Chen is with the Department of Electronic Engineering,City University of Hong Kong,Hong Kong SAR,China.Copyright(c)2009IEEE.Personal use of this material is permitted. However,permission to use this material for any other purposes must be obtained from the IEEE by sending a request to pubs-permissions@.and robust control methodologies.In the past two decades in particular,control of multiple vehicles has received increas-ing demands spurred by the fact that many benefits can be obtained when a single complicated vehicle is equivalently replaced by multiple yet simpler vehicles.In this endeavor, two approaches are commonly adopted for controlling multiple vehicles:a centralized approach and a distributed approach. The centralized approach is based on the assumption that a central station is available and powerful enough to control a whole group of vehicles.Essentially,the centralized ap-proach is a direct extension of the traditional single-vehicle-based control philosophy and strategy.On the contrary,the distributed approach does not require a central station for control,at the cost of becoming far more complex in structure and organization.Although both approaches are considered practical depending on the situations and conditions of the real applications,the distributed approach is believed more promising due to many inevitable physical constraints such as limited resources and energy,short wireless communication ranges,narrow bandwidths,and large sizes of vehicles to manage and control.Therefore,the focus of this overview is placed on the distributed approach.In distributed control of a group of autonomous vehicles,the main objective typically is to have the whole group of vehicles working in a cooperative fashion throughout a distributed pro-tocol.Here,cooperative refers to a close relationship among all vehicles in the group where information sharing plays a central role.The distributed approach has many advantages in achieving cooperative group performances,especially with low operational costs,less system requirements,high robustness, strong adaptivity,andflexible scalability,therefore has been widely recognized and appreciated.The study of distributed control of multiple vehicles was perhapsfirst motivated by the work in distributed comput-ing[1],management science[2],and statistical physics[3]. In the control systems society,some pioneering works are generally referred to[4],[5],where an asynchronous agree-ment problem was studied for distributed decision-making problems.Thereafter,some consensus algorithms were studied under various information-flow constraints[6]–[10].There are several journal special issues on the related topics published af-ter2006,including the IEEE Transactions on Control Systems Technology(vol.15,no.4,2007),Proceedings of the IEEE (vol.94,no.4,2007),ASME Journal of Dynamic Systems, Measurement,and Control(vol.129,no.5,2007),SIAM Journal of Control and Optimization(vol.48,no.1,2009),and International Journal of Robust and Nonlinear Control(vol.21,no.12,2011).In addition,there are some recent reviewsand progress reports given in the surveys[11]–[15]and thebooks[16]–[23],among others.This article reviews some main results and recent progressin distributed multi-agent coordination,published in majorcontrol systems and robotics journals since2006.Due to space limitations,we refer the readers to[24]for a more completeversion of the same overview.For results before2006,thereaders are referred to[11]–[14].Specifically,this article reviews the recent research resultsin the following directions,which are not independent but actually may have overlapping to some extent:1.Consensus and the like(synchronization,rendezvous).Consensus refers to the group behavior that all theagents asymptotically reach a certain common agreementthrough a local distributed protocol,with or without predefined common speed and orientation.2.Distributed formation and the like(flocking).Distributedformation refers to the group behavior that all the agents form a pre-designed geometrical configuration throughlocal interactions with or without a common reference.3.Distributed optimization.This refers to algorithmic devel-opments for the analysis and optimization of large-scaledistributed systems.4.Distributed estimation and control.This refers to dis-tributed control design based on local estimation aboutthe needed global information.The rest of this article is organized as follows.In Section II,basic notations of graph theory and stochastic matrices are introduced.Sections III,IV,V,and VI describe the recentresearch results and progress in consensus,formation control, optimization,and estimation.Finally,the article is concludedby a short section of discussions with future perspectives.II.P RELIMINARIESA.Graph TheoryFor a system of n connected agents,its network topology can be modeled as a directed graph denoted by G=(V,W),where V={v1,v2,···,v n}and W⊆V×V are,respectively, the set of agents and the set of edges which directionallyconnect the agents together.Specifically,the directed edgedenoted by an ordered pair(v i,v j)means that agent j can access the state information of agent i.Accordingly,agent i is a neighbor of agent j.A directed path is a sequence of directed edges in the form of(v1,v2),(v2,v3),···,with all v i∈V.A directed graph has a directed spanning tree if there exists at least one agent that has a directed path to every other agent.The union of a set of directed graphs with the same setof agents,{G i1,···,G im},is a directed graph with the sameset of agents and its set of edges is given by the union of the edge sets of all the directed graphs G ij,j=1,···,m.A complete directed graph is a directed graph in which each pair of distinct agents is bidirectionally connected by an edge,thus there is a directed path from any agent to any other agent in the network.Two matrices are used to represent the network topology: the adjacency matrix A=[a ij]∈R n×n with a ij>0if (v j,v i)∈W and a ij=0otherwise,and the Laplacian matrix L=[ℓij]∈R n×n withℓii= n j=1a ij andℓij=−a ij,i=j, which is generally asymmetric for directed graphs.B.Stochastic MatricesA nonnegative square matrix is called(row)stochastic matrix if its every row is summed up to one.The product of two stochastic matrices is still a stochastic matrix.A row stochastic matrix P∈R n×n is called indecomposable and aperiodic if lim k→∞P k=1y T for some y∈R n[25],where 1is a vector with all elements being1.III.C ONSENSUSConsider a group of n agents,each with single-integrator kinematics described by˙x i(t)=u i(t),i=1,···,n,(1) where x i(t)and u i(t)are,respectively,the state and the control input of the i th agent.A typical consensus control algorithm is designed asu i(t)=nj=1a ij(t)[x j(t)−x i(t)],(2)where a ij(t)is the(i,j)th entry of the corresponding ad-jacency matrix at time t.The main idea behind(2)is that each agent moves towards the weighted average of the states of its neighbors.Given the switching network pattern due to the continuous motions of the dynamic agents,coupling coefficients a ij(t)in(2),hence the graph topologies,are generally time-varying.It is shown in[9],[10]that consensus is achieved if the underlying directed graph has a directed spanning tree in some jointly fashion in terms of a union of its time-varying graph topologies.The idea behind consensus serves as a fundamental principle for the design of distributed multi-agent coordination algo-rithms.Therefore,investigating consensus has been a main research direction in the study of distributed multi-agent co-ordination.To bridge the gap between the study of consensus algorithms and many physical properties inherited in practical systems,it is necessary and meaningful to study consensus by considering many practical factors,such as actuation,control, communication,computation,and vehicle dynamics,which characterize some important features of practical systems.This is the main motivation to study consensus.In the following part of the section,an overview of the research progress in the study of consensus is given,regarding stochastic network topologies and dynamics,complex dynamical systems,delay effects,and quantization,mainly after2006.Several milestone results prior to2006can be found in[2],[4]–[6],[8]–[10], [26].A.Stochastic Network Topologies and DynamicsIn multi-agent systems,the network topology among all vehicles plays a crucial role in determining consensus.The objective here is to explicitly identify necessary and/or suffi-cient conditions on the network topology such that consensus can be achieved under properly designed algorithms.It is often reasonable to consider the case when the network topology is deterministic under ideal communication chan-nels.Accordingly,main research on the consensus problem was conducted under a deterministicfixed/switching network topology.That is,the adjacency matrix A(t)is deterministic. Some other times,when considering random communication failures,random packet drops,and communication channel instabilities inherited in physical communication channels,it is necessary and important to study consensus problem in the stochastic setting where a network topology evolves according to some random distributions.That is,the adjacency matrix A(t)is stochastically evolving.In the deterministic setting,consensus is said to be achieved if all agents eventually reach agreement on a common state. In the stochastic setting,consensus is said to be achieved almost surely(respectively,in mean-square or in probability)if all agents reach agreement on a common state almost surely (respectively,in mean-square or with probability one).Note that the problem studied in the stochastic setting is slightly different from that studied in the deterministic setting due to the different assumptions in terms of the network topology. Consensus over a stochastic network topology was perhaps first studied in[27],where some sufficient conditions on the network topology were given to guarantee consensus with probability one for systems with single-integrator kinemat-ics(1),where the rate of convergence was also studied.Further results for consensus under a stochastic network topology were reported in[28]–[30],where research effort was conducted for systems with single-integrator kinematics[28],[29]or double-integrator dynamics[30].Consensus for single-integrator kine-matics under stochastic network topology has been exten-sively studied in particular,where some general conditions for almost-surely consensus was derived[29].Loosely speaking, almost-surely consensus for single-integrator kinematics can be achieved,i.e.,x i(t)−x j(t)→0almost surely,if and only if the expectation of the network topology,namely,the network topology associated with expectation E[A(t)],has a directed spanning tree.It is worth noting that the conditions are analogous to that in[9],[10],but in the stochastic setting. In view of the special structure of the closed-loop systems concerning consensus for single-integrator kinematics,basic properties of the stochastic matrices play a crucial role in the convergence analysis of the associated control algorithms. Consensus for double-integrator dynamics was studied in[30], where the switching network topology is assumed to be driven by a Bernoulli process,and it was shown that consensus can be achieved if the union of all the graphs has a directed spanning tree.Apparently,the requirement on the network topology for double-integrator dynamics is a special case of that for single-integrator kinematics due to the difference nature of thefinal states(constantfinal states for single-integrator kinematics and possible dynamicfinal states for double-integrator dynamics) caused by the substantial dynamical difference.It is still an open question as if some general conditions(corresponding to some specific algorithms)can be found for consensus with double-integrator dynamics.In addition to analyzing the conditions on the network topology such that consensus can be achieved,a special type of consensus algorithm,the so-called gossip algorithm[31],[32], has been used to achieve consensus in the stochastic setting. The gossip algorithm can always guarantee consensus almost surely if the available pairwise communication channels satisfy certain conditions(such as a connected graph).The way of network topology switching does not play any role in the consideration of consensus.The current study on consensus over stochastic network topologies has shown some interesting results regarding:(1) consensus algorithm design for various multi-agent systems,(2)conditions of the network topologies on consensus,and(3)effects of the stochastic network topologies on the con-vergence rate.Future research on this topic includes,but not limited to,the following two directions:(1)when the network topology itself is stochastic,how to determine the probability of reaching consensus almost surely?(2)compared with the deterministic network topology,what are the advantages and disadvantages of the stochastic network topology,regarding such as robustness and convergence rate?As is well known,disturbances and uncertainties often exist in networked systems,for example,channel noise,commu-nication noise,uncertainties in network parameters,etc.In addition to the stochastic network topologies discussed above, the effect of stochastic disturbances[33],[34]and uncertain-ties[35]on the consensus problem also needs investigation. Study has been mainly devoted to analyzing the performance of consensus algorithms subject to disturbances and to present-ing conditions on the uncertainties such that consensus can be achieved.In addition,another interesting direction in dealing with disturbances and uncertainties is to design distributed localfiltering algorithms so as to save energy and improve computational efficiency.Distributed localfiltering algorithms play an important role and are more effective than traditional centralizedfiltering algorithms for multi-agent systems.For example,in[36]–[38]some distributed Kalmanfilters are designed to implement data fusion.In[39],by analyzing consensus and pinning control in synchronization of complex networks,distributed consensusfiltering in sensor networks is addressed.Recently,Kalmanfiltering over a packet-dropping network is designed through a probabilistic approach[40]. Today,it remains a challenging problem to incorporate both dynamics of consensus and probabilistic(Kalman)filtering into a unified framework.plex Dynamical SystemsSince consensus is concerned with the behavior of a group of vehicles,it is natural to consider the system dynamics for practical vehicles in the study of the consensus problem. Although the study of consensus under various system dynam-ics is due to the existence of complex dynamics in practical systems,it is also interesting to observe that system dynamics play an important role in determining thefinal consensus state.For instance,the well-studied consensus of multi-agent systems with single-integrator kinematics often converges to a constantfinal value instead.However,consensus for double-integrator dynamics might admit a dynamicfinal value(i.e.,a time function).These important issues motivate the study of consensus under various system dynamics.As a direct extension of the study of the consensus prob-lem for systems with simple dynamics,for example,with single-integrator kinematics or double-integrator dynamics, consensus with general linear dynamics was also studied recently[41]–[43],where research is mainly devoted tofinding feedback control laws such that consensus(in terms of the output states)can be achieved for general linear systems˙x i=Ax i+Bu i,y i=Cx i,(3) where A,B,and C are constant matrices with compatible sizes.Apparently,the well-studied single-integrator kinematics and double-integrator dynamics are special cases of(3)for properly choosing A,B,and C.As a further extension,consensus for complex systems has also been extensively studied.Here,the term consensus for complex systems is used for the study of consensus problem when the system dynamics are nonlinear[44]–[48]or with nonlinear consensus algorithms[49],[50].Examples of the nonlinear system dynamics include:•Nonlinear oscillators[45].The dynamics are often as-sumed to be governed by the Kuramoto equation˙θi=ωi+Kstability.A well-studied consensus algorithm for(1)is given in(2),where it is now assumed that time delay exists.Two types of time delays,communication delay and input delay, have been considered in the munication delay accounts for the time for transmitting information from origin to destination.More precisely,if it takes time T ij for agent i to receive information from agent j,the closed-loop system of(1)using(2)under afixed network topology becomes˙x i(t)=nj=1a ij(t)[x j(t−T ij)−x i(t)].(7)An interpretation of(7)is that at time t,agent i receives information from agent j and uses data x j(t−T ij)instead of x j(t)due to the time delay.Note that agent i can get its own information instantly,therefore,input delay can be considered as the summation of computation time and execution time. More precisely,if the input delay for agent i is given by T p i, then the closed-loop system of(1)using(2)becomes˙x i(t)=nj=1a ij(t)[x j(t−T p i)−x i(t−T p i)].(8)Clearly,(7)refers to the case when only communication delay is considered while(8)refers to the case when only input delay is considered.It should be emphasized that both communication delay and input delay might be time-varying and they might co-exist at the same time.In addition to time delay,it is also important to consider packet drops in exchanging state information.Fortunately, consensus with packet drops can be considered as a special case of consensus with time delay,because re-sending packets after they were dropped can be easily done but just having time delay in the data transmission channels.Thus,the main problem involved in consensus with time delay is to study the effects of time delay on the convergence and performance of consensus,referred to as consensusabil-ity[52].Because time delay might affect the system stability,it is important to study under what conditions consensus can still be guaranteed even if time delay exists.In other words,can onefind conditions on the time delay such that consensus can be achieved?For this purpose,the effect of time delay on the consensusability of(1)using(2)was investigated.When there exists only(constant)input delay,a sufficient condition on the time delay to guarantee consensus under afixed undirected interaction graph is presented in[8].Specifically,an upper bound for the time delay is derived under which consensus can be achieved.This is a well-expected result because time delay normally degrades the system performance gradually but will not destroy the system stability unless the time delay is above a certain threshold.Further studies can be found in, e.g.,[53],[54],which demonstrate that for(1)using(2),the communication delay does not affect the consensusability but the input delay does.In a similar manner,consensus with time delay was studied for systems with different dynamics, where the dynamics(1)are replaced by other more complex ones,such as double-integrator dynamics[55],[56],complex networks[57],[58],rigid bodies[59],[60],and general nonlinear dynamics[61].In summary,the existing study of consensus with time delay mainly focuses on analyzing the stability of consensus algo-rithms with time delay for various types of system dynamics, including linear and nonlinear dynamics.Generally speaking, consensus with time delay for systems with nonlinear dynam-ics is more challenging.For most consensus algorithms with time delays,the main research question is to determine an upper bound of the time delay under which time delay does not affect the consensusability.For communication delay,it is possible to achieve consensus under a relatively large time delay threshold.A notable phenomenon in this case is that thefinal consensus state is constant.Considering both linear and nonlinear system dynamics in consensus,the main tools for stability analysis of the closed-loop systems include matrix theory[53],Lyapunov functions[57],frequency-domain ap-proach[54],passivity[58],and the contraction principle[62]. Although consensus with time delay has been studied extensively,it is often assumed that time delay is either constant or random.However,time delay itself might obey its own dynamics,which possibly depend on the communication distance,total computation load and computation capability, etc.Therefore,it is more suitable to represent the time delay as another system variable to be considered in the study of the consensus problem.In addition,it is also important to consider time delay and other physical constraints simultaneously in the study of the consensus problem.D.QuantizationQuantized consensus has been studied recently with motiva-tion from digital signal processing.Here,quantized consensus refers to consensus when the measurements are digital rather than analog therefore the information received by each agent is not continuous and might have been truncated due to digital finite precision constraints.Roughly speaking,for an analog signal s,a typical quantizer with an accuracy parameterδ, also referred to as quantization step size,is described by Q(s)=q(s,δ),where Q(s)is the quantized signal and q(·,·) is the associated quantization function.For instance[63],a quantizer rounding a signal s to its nearest integer can be expressed as Q(s)=n,if s∈[(n−1/2)δ,(n+1/2)δ],n∈Z, where Z denotes the integer set.Note that the types of quantizers might be different for different systems,hence Q(s) may differ for different systems.Due to the truncation of the signals received,consensus is now considered achieved if the maximal state difference is not larger than the accuracy level associated with the whole system.A notable feature for consensus with quantization is that the time to reach consensus is usuallyfinite.That is,it often takes afinite period of time for all agents’states to converge to an accuracy interval.Accordingly,the main research is to investigate the convergence time associated with the proposed consensus algorithm.Quantized consensus was probablyfirst studied in[63], where a quantized gossip algorithm was proposed and its convergence was analyzed.In particular,the bound of theconvergence time for a complete graph was shown to be poly-nomial in the network size.In[64],coding/decoding strate-gies were introduced to the quantized consensus algorithms, where it was shown that the convergence rate depends on the accuracy of the quantization but not the coding/decoding schemes.In[65],quantized consensus was studied via the gossip algorithm,with both lower and upper bounds of the expected convergence time in the worst case derived in terms of the principle submatrices of the Laplacian matrix.Further results regarding quantized consensus were reported in[66]–[68],where the main research was also on the convergence time for various proposed quantized consensus algorithms as well as the quantization effects on the convergence time.It is intuitively reasonable that the convergence time depends on both the quantization level and the network topology.It is then natural to ask if and how the quantization methods affect the convergence time.This is an important measure of the robustness of a quantized consensus algorithm(with respect to the quantization method).Note that it is interesting but also more challenging to study consensus for general linear/nonlinear systems with quantiza-tion.Because the difference between the truncated signal and the original signal is bounded,consensus with quantization can be considered as a special case of one without quantization when there exist bounded disturbances.Therefore,if consensus can be achieved for a group of vehicles in the absence of quantization,it might be intuitively correct to say that the differences among the states of all vehicles will be bounded if the quantization precision is small enough.However,it is still an open question to rigorously describe the quantization effects on consensus with general linear/nonlinear systems.E.RemarksIn summary,the existing research on the consensus problem has covered a number of physical properties for practical systems and control performance analysis.However,the study of the consensus problem covering multiple physical properties and/or control performance analysis has been largely ignored. In other words,two or more problems discussed in the above subsections might need to be taken into consideration simul-taneously when studying the consensus problem.In addition, consensus algorithms normally guarantee the agreement of a team of agents on some common states without taking group formation into consideration.To reflect many practical applications where a group of agents are normally required to form some preferred geometric structure,it is desirable to consider a task-oriented formation control problem for a group of mobile agents,which motivates the study of formation control presented in the next section.IV.F ORMATION C ONTROLCompared with the consensus problem where thefinal states of all agents typically reach a singleton,thefinal states of all agents can be more diversified under the formation control scenario.Indeed,formation control is more desirable in many practical applications such as formationflying,co-operative transportation,sensor networks,as well as combat intelligence,surveillance,and reconnaissance.In addition,theperformance of a team of agents working cooperatively oftenexceeds the simple integration of the performances of all individual agents.For its broad applications and advantages,formation control has been a very active research subject inthe control systems community,where a certain geometric pattern is aimed to form with or without a group reference.More precisely,the main objective of formation control is to coordinate a group of agents such that they can achievesome desired formation so that some tasks can befinished bythe collaboration of the agents.Generally speaking,formation control can be categorized according to the group reference.Formation control without a group reference,called formationproducing,refers to the algorithm design for a group of agents to reach some pre-desired geometric pattern in the absenceof a group reference,which can also be considered as the control objective.Formation control with a group reference,called formation tracking,refers to the same task but followingthe predesignated group reference.Due to the existence of the group reference,formation tracking is usually much morechallenging than formation producing and control algorithmsfor the latter might not be useful for the former.As of today, there are still many open questions in solving the formationtracking problem.The following part of the section reviews and discussesrecent research results and progress in formation control, including formation producing and formation tracking,mainlyaccomplished after2006.Several milestone results prior to 2006can be found in[69]–[71].A.Formation ProducingThe existing work in formation control aims at analyzingthe formation behavior under certain control laws,along with stability analysis.1)Matrix Theory Approach:Due to the nature of multi-agent systems,matrix theory has been frequently used in thestability analysis of their distributed coordination.Note that consensus input to each agent(see e.g.,(2))isessentially a weighted average of the differences between the states of the agent’s neighbors and its own.As an extensionof the consensus algorithms,some coupling matrices wereintroduced here to offset the corresponding control inputs by some angles[72],[73].For example,given(1),the controlinput(2)is revised as u i(t)= n j=1a ij(t)C[x j(t)−x i(t)], where C is a coupling matrix with compatible size.If x i∈R3, then C can be viewed as the3-D rotational matrix.The mainidea behind the revised algorithm is that the original controlinput for reaching consensus is now rotated by some angles. The closed-loop system can be expressed in a vector form, whose stability can be determined by studying the distribution of the eigenvalues of a certain transfer matrix.Main research work was conducted in[72],[73]to analyze the collective motions for systems with single-integrator kinematics and double-integrator dynamics,where the network topology,the damping gain,and C were shown to affect the collective motions.Analogously,the collective motions for a team of nonlinear self-propelling agents were shown to be affected by。
Consensus and Cooperation in Networked Multi-Agent Systems
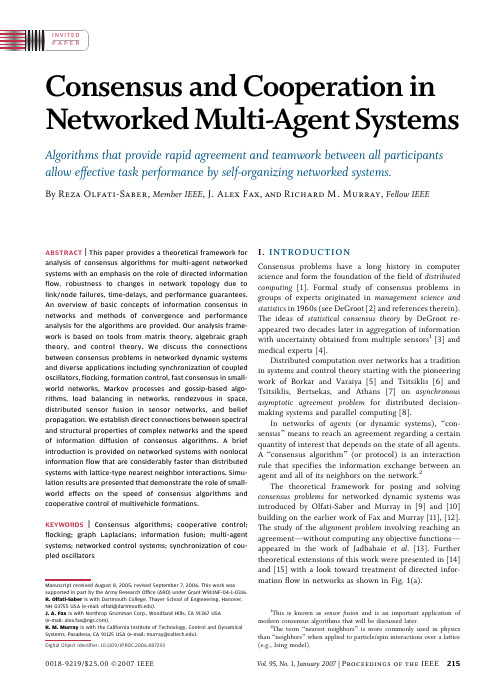
Consensus and Cooperation in Networked Multi-Agent SystemsAlgorithms that provide rapid agreement and teamwork between all participants allow effective task performance by self-organizing networked systems.By Reza Olfati-Saber,Member IEEE,J.Alex Fax,and Richard M.Murray,Fellow IEEEABSTRACT|This paper provides a theoretical framework for analysis of consensus algorithms for multi-agent networked systems with an emphasis on the role of directed information flow,robustness to changes in network topology due to link/node failures,time-delays,and performance guarantees. An overview of basic concepts of information consensus in networks and methods of convergence and performance analysis for the algorithms are provided.Our analysis frame-work is based on tools from matrix theory,algebraic graph theory,and control theory.We discuss the connections between consensus problems in networked dynamic systems and diverse applications including synchronization of coupled oscillators,flocking,formation control,fast consensus in small-world networks,Markov processes and gossip-based algo-rithms,load balancing in networks,rendezvous in space, distributed sensor fusion in sensor networks,and belief propagation.We establish direct connections between spectral and structural properties of complex networks and the speed of information diffusion of consensus algorithms.A brief introduction is provided on networked systems with nonlocal information flow that are considerably faster than distributed systems with lattice-type nearest neighbor interactions.Simu-lation results are presented that demonstrate the role of small-world effects on the speed of consensus algorithms and cooperative control of multivehicle formations.KEYWORDS|Consensus algorithms;cooperative control; flocking;graph Laplacians;information fusion;multi-agent systems;networked control systems;synchronization of cou-pled oscillators I.INTRODUCTIONConsensus problems have a long history in computer science and form the foundation of the field of distributed computing[1].Formal study of consensus problems in groups of experts originated in management science and statistics in1960s(see DeGroot[2]and references therein). The ideas of statistical consensus theory by DeGroot re-appeared two decades later in aggregation of information with uncertainty obtained from multiple sensors1[3]and medical experts[4].Distributed computation over networks has a tradition in systems and control theory starting with the pioneering work of Borkar and Varaiya[5]and Tsitsiklis[6]and Tsitsiklis,Bertsekas,and Athans[7]on asynchronous asymptotic agreement problem for distributed decision-making systems and parallel computing[8].In networks of agents(or dynamic systems),B con-sensus[means to reach an agreement regarding a certain quantity of interest that depends on the state of all agents.A B consensus algorithm[(or protocol)is an interaction rule that specifies the information exchange between an agent and all of its neighbors on the network.2 The theoretical framework for posing and solving consensus problems for networked dynamic systems was introduced by Olfati-Saber and Murray in[9]and[10] building on the earlier work of Fax and Murray[11],[12]. The study of the alignment problem involving reaching an agreement V without computing any objective functions V appeared in the work of Jadbabaie et al.[13].Further theoretical extensions of this work were presented in[14] and[15]with a look toward treatment of directed infor-mation flow in networks as shown in Fig.1(a).Manuscript received August8,2005;revised September7,2006.This work was supported in part by the Army Research Office(ARO)under Grant W911NF-04-1-0316. R.Olfati-Saber is with Dartmouth College,Thayer School of Engineering,Hanover,NH03755USA(e-mail:olfati@).J.A.Fax is with Northrop Grumman Corp.,Woodland Hills,CA91367USA(e-mail:alex.fax@).R.M.Murray is with the California Institute of Technology,Control and Dynamical Systems,Pasadena,CA91125USA(e-mail:murray@).Digital Object Identifier:10.1109/JPROC.2006.8872931This is known as sensor fusion and is an important application of modern consensus algorithms that will be discussed later.2The term B nearest neighbors[is more commonly used in physics than B neighbors[when applied to particle/spin interactions over a lattice (e.g.,Ising model).Vol.95,No.1,January2007|Proceedings of the IEEE2150018-9219/$25.00Ó2007IEEEThe common motivation behind the work in [5],[6],and [10]is the rich history of consensus protocols in com-puter science [1],whereas Jadbabaie et al.[13]attempted to provide a formal analysis of emergence of alignment in the simplified model of flocking by Vicsek et al.[16].The setup in [10]was originally created with the vision of de-signing agent-based amorphous computers [17],[18]for collaborative information processing in ter,[10]was used in development of flocking algorithms with guaranteed convergence and the capability to deal with obstacles and adversarial agents [19].Graph Laplacians and their spectral properties [20]–[23]are important graph-related matrices that play a crucial role in convergence analysis of consensus and alignment algo-rithms.Graph Laplacians are an important point of focus of this paper.It is worth mentioning that the second smallest eigenvalue of graph Laplacians called algebraic connectivity quantifies the speed of convergence of consensus algo-rithms.The notion of algebraic connectivity of graphs has appeared in a variety of other areas including low-density parity-check codes (LDPC)in information theory and com-munications [24],Ramanujan graphs [25]in number theory and quantum chaos,and combinatorial optimization prob-lems such as the max-cut problem [21].More recently,there has been a tremendous surge of interest V among researchers from various disciplines of engineering and science V in problems related to multia-gent networked systems with close ties to consensus prob-lems.This includes subjects such as consensus [26]–[32],collective behavior of flocks and swarms [19],[33]–[37],sensor fusion [38]–[40],random networks [41],[42],syn-chronization of coupled oscillators [42]–[46],algebraic connectivity 3of complex networks [47]–[49],asynchro-nous distributed algorithms [30],[50],formation control for multirobot systems [51]–[59],optimization-based co-operative control [60]–[63],dynamic graphs [64]–[67],complexity of coordinated tasks [68]–[71],and consensus-based belief propagation in Bayesian networks [72],[73].A detailed discussion of selected applications will be pre-sented shortly.In this paper,we focus on the work described in five key papers V namely,Jadbabaie,Lin,and Morse [13],Olfati-Saber and Murray [10],Fax and Murray [12],Moreau [14],and Ren and Beard [15]V that have been instrumental in paving the way for more recent advances in study of self-organizing networked systems ,or swarms .These networked systems are comprised of locally interacting mobile/static agents equipped with dedicated sensing,computing,and communication devices.As a result,we now have a better understanding of complex phenomena such as flocking [19],or design of novel information fusion algorithms for sensor networks that are robust to node and link failures [38],[72]–[76].Gossip-based algorithms such as the push-sum protocol [77]are important alternatives in computer science to Laplacian-based consensus algorithms in this paper.Markov processes establish an interesting connection between the information propagation speed in these two categories of algorithms proposed by computer scientists and control theorists [78].The contribution of this paper is to present a cohesive overview of the key results on theory and applications of consensus problems in networked systems in a unified framework.This includes basic notions in information consensus and control theoretic methods for convergence and performance analysis of consensus protocols that heavily rely on matrix theory and spectral graph theory.A byproduct of this framework is to demonstrate that seem-ingly different consensus algorithms in the literature [10],[12]–[15]are closely related.Applications of consensus problems in areas of interest to researchers in computer science,physics,biology,mathematics,robotics,and con-trol theory are discussed in this introduction.A.Consensus in NetworksThe interaction topology of a network of agents is rep-resented using a directed graph G ¼ðV ;E Þwith the set of nodes V ¼f 1;2;...;n g and edges E V ÂV .TheFig.1.Two equivalent forms of consensus algorithms:(a)a networkof integrator agents in which agent i receives the state x j of its neighbor,agent j ,if there is a link ði ;j Þconnecting the two nodes;and (b)the block diagram for a network of interconnecteddynamic systems all with identical transfer functions P ðs Þ¼1=s .The collective networked system has a diagonal transfer function and is a multiple-input multiple-output (MIMO)linear system.3To be defined in Section II-A.Olfati-Saber et al.:Consensus and Cooperation in Networked Multi-Agent Systems216Proceedings of the IEEE |Vol.95,No.1,January 2007neighbors of agent i are denoted by N i ¼f j 2V :ði ;j Þ2E g .According to [10],a simple consensus algorithm to reach an agreement regarding the state of n integrator agents with dynamics _x i ¼u i can be expressed as an n th-order linear system on a graph_x i ðt Þ¼X j 2N ix j ðt ÞÀx i ðt ÞÀÁþb i ðt Þ;x i ð0Þ¼z i2R ;b i ðt Þ¼0:(1)The collective dynamics of the group of agents following protocol (1)can be written as_x ¼ÀLx(2)where L ¼½l ij is the graph Laplacian of the network and itselements are defined as follows:l ij ¼À1;j 2N i j N i j ;j ¼i :&(3)Here,j N i j denotes the number of neighbors of node i (or out-degree of node i ).Fig.1shows two equivalent forms of the consensus algorithm in (1)and (2)for agents with a scalar state.The role of the input bias b in Fig.1(b)is defined later.According to the definition of graph Laplacian in (3),all row-sums of L are zero because of P j l ij ¼0.Therefore,L always has a zero eigenvalue 1¼0.This zero eigenvalues corresponds to the eigenvector 1¼ð1;...;1ÞT because 1belongs to the null-space of L ðL 1¼0Þ.In other words,an equilibrium of system (2)is a state in the form x üð ;...; ÞT ¼ 1where all nodes agree.Based on ana-lytical tools from algebraic graph theory [23],we later show that x Ãis a unique equilibrium of (2)(up to a constant multiplicative factor)for connected graphs.One can show that for a connected network,the equilibrium x üð ;...; ÞT is globally exponentially stable.Moreover,the consensus value is ¼1=n P i z i that is equal to the average of the initial values.This im-plies that irrespective of the initial value of the state of each agent,all agents reach an asymptotic consensus regarding the value of the function f ðz Þ¼1=n P i z i .While the calculation of f ðz Þis simple for small net-works,its implications for very large networks is more interesting.For example,if a network has n ¼106nodes and each node can only talk to log 10ðn Þ¼6neighbors,finding the average value of the initial conditions of the nodes is more complicated.The role of protocol (1)is to provide a systematic consensus mechanism in such a largenetwork to compute the average.There are a variety of functions that can be computed in a similar fashion using synchronous or asynchronous distributed algorithms (see [10],[28],[30],[73],and [76]).B.The f -Consensus Problem and Meaning of CooperationTo understand the role of cooperation in performing coordinated tasks,we need to distinguish between un-constrained and constrained consensus problems.An unconstrained consensus problem is simply the alignment problem in which it suffices that the state of all agents asymptotically be the same.In contrast,in distributed computation of a function f ðz Þ,the state of all agents has to asymptotically become equal to f ðz Þ,meaning that the consensus problem is constrained.We refer to this con-strained consensus problem as the f -consensus problem .Solving the f -consensus problem is a cooperative task and requires willing participation of all the agents.To demonstrate this fact,suppose a single agent decides not to cooperate with the rest of the agents and keep its state unchanged.Then,the overall task cannot be performed despite the fact that the rest of the agents reach an agree-ment.Furthermore,there could be scenarios in which multiple agents that form a coalition do not cooperate with the rest and removal of this coalition of agents and their links might render the network disconnected.In a dis-connected network,it is impossible for all nodes to reach an agreement (unless all nodes initially agree which is a trivial case).From the above discussion,cooperation can be infor-mally interpreted as B giving consent to providing one’s state and following a common protocol that serves the group objective.[One might think that solving the alignment problem is not a cooperative task.The justification is that if a single agent (called a leader)leaves its value unchanged,all others will asymptotically agree with the leader according to the consensus protocol and an alignment is reached.However,if there are multiple leaders where two of whom are in disagreement,then no consensus can be asymptot-ically reached.Therefore,alignment is in general a coop-erative task as well.Formal analysis of the behavior of systems that involve more than one type of agent is more complicated,partic-ularly,in presence of adversarial agents in noncooperative games [79],[80].The focus of this paper is on cooperative multi-agent systems.C.Iterative Consensus and Markov ChainsIn Section II,we show how an iterative consensus algorithm that corresponds to the discrete-time version of system (1)is a Markov chainðk þ1Þ¼ ðk ÞP(4)Olfati-Saber et al.:Consensus and Cooperation in Networked Multi-Agent SystemsVol.95,No.1,January 2007|Proceedings of the IEEE217with P ¼I À L and a small 90.Here,the i th element of the row vector ðk Þdenotes the probability of being in state i at iteration k .It turns out that for any arbitrary graph G with Laplacian L and a sufficiently small ,the matrix P satisfies the property Pj p ij ¼1with p ij !0;8i ;j .Hence,P is a valid transition probability matrix for the Markov chain in (4).The reason matrix theory [81]is so widely used in analysis of consensus algorithms [10],[12]–[15],[64]is primarily due to the structure of P in (4)and its connection to graphs.4There are interesting connections between this Markov chain and the speed of information diffusion in gossip-based averaging algorithms [77],[78].One of the early applications of consensus problems was dynamic load balancing [82]for parallel processors with the same structure as system (4).To this date,load balancing in networks proves to be an active area of research in computer science.D.ApplicationsMany seemingly different problems that involve inter-connection of dynamic systems in various areas of science and engineering happen to be closely related to consensus problems for multi-agent systems.In this section,we pro-vide an account of the existing connections.1)Synchronization of Coupled Oscillators:The problem of synchronization of coupled oscillators has attracted numer-ous scientists from diverse fields including physics,biology,neuroscience,and mathematics [83]–[86].This is partly due to the emergence of synchronous oscillations in coupled neural oscillators.Let us consider the generalized Kuramoto model of coupled oscillators on a graph with dynamics_i ¼ Xj 2N isin ð j À i Þþ!i (5)where i and !i are the phase and frequency of the i thoscillator.This model is the natural nonlinear extension of the consensus algorithm in (1)and its linearization around the aligned state 1¼...¼ n is identical to system (2)plus a nonzero input bias b i ¼ð!i À"!Þ= with "!¼1=n P i !i after a change of variables x i ¼ð i À"!t Þ= .In [43],Sepulchre et al.show that if is sufficiently large,then for a network with all-to-all links,synchroni-zation to the aligned state is globally achieved for all ini-tial states.Recently,synchronization of networked oscillators under variable time-delays was studied in [45].We believe that the use of convergence analysis methods that utilize the spectral properties of graph Laplacians willshed light on performance and convergence analysis of self-synchrony in oscillator networks [42].2)Flocking Theory:Flocks of mobile agents equipped with sensing and communication devices can serve as mobile sensor networks for massive distributed sensing in an environment [87].A theoretical framework for design and analysis of flocking algorithms for mobile agents with obstacle-avoidance capabilities is developed by Olfati-Saber [19].The role of consensus algorithms in particle-based flocking is for an agent to achieve velocity matching with respect to its neighbors.In [19],it is demonstrated that flocks are networks of dynamic systems with a dynamic topology.This topology is a proximity graph that depends on the state of all agents and is determined locally for each agent,i.e.,the topology of flocks is a state-dependent graph.The notion of state-dependent graphs was introduced by Mesbahi [64]in a context that is independent of flocking.3)Fast Consensus in Small-Worlds:In recent years,network design problems for achieving faster consensus algorithms has attracted considerable attention from a number of researchers.In Xiao and Boyd [88],design of the weights of a network is considered and solved using semi-definite convex programming.This leads to a slight increase in algebraic connectivity of a network that is a measure of speed of convergence of consensus algorithms.An alternative approach is to keep the weights fixed and design the topology of the network to achieve a relatively high algebraic connectivity.A randomized algorithm for network design is proposed by Olfati-Saber [47]based on random rewiring idea of Watts and Strogatz [89]that led to creation of their celebrated small-world model .The random rewiring of existing links of a network gives rise to considerably faster consensus algorithms.This is due to multiple orders of magnitude increase in algebraic connectivity of the network in comparison to a lattice-type nearest-neighbort graph.4)Rendezvous in Space:Another common form of consensus problems is rendezvous in space [90],[91].This is equivalent to reaching a consensus in position by a num-ber of agents with an interaction topology that is position induced (i.e.,a proximity graph).We refer the reader to [92]and references therein for a detailed discussion.This type of rendezvous is an unconstrained consensus problem that becomes challenging under variations in the network topology.Flocking is somewhat more challenging than rendezvous in space because it requires both interagent and agent-to-obstacle collision avoidance.5)Distributed Sensor Fusion in Sensor Networks:The most recent application of consensus problems is distrib-uted sensor fusion in sensor networks.This is done by posing various distributed averaging problems require to4In honor of the pioneering contributions of Oscar Perron (1907)to the theory of nonnegative matrices,were refer to P as the Perron Matrix of graph G (See Section II-C for details).Olfati-Saber et al.:Consensus and Cooperation in Networked Multi-Agent Systems218Proceedings of the IEEE |Vol.95,No.1,January 2007implement a Kalman filter [38],[39],approximate Kalman filter [74],or linear least-squares estimator [75]as average-consensus problems .Novel low-pass and high-pass consensus filters are also developed that dynamically calculate the average of their inputs in sensor networks [39],[93].6)Distributed Formation Control:Multivehicle systems are an important category of networked systems due to their commercial and military applications.There are two broad approaches to distributed formation control:i)rep-resentation of formations as rigid structures [53],[94]and the use of gradient-based controls obtained from their structural potentials [52]and ii)representation of form-ations using the vectors of relative positions of neighboring vehicles and the use of consensus-based controllers with input bias.We discuss the later approach here.A theoretical framework for design and analysis of distributed controllers for multivehicle formations of type ii)was developed by Fax and Murray [12].Moving in formation is a cooperative task and requires consent and collaboration of every agent in the formation.In [12],graph Laplacians and matrix theory were extensively used which makes one wonder whether relative-position-based formation control is a consensus problem.The answer is yes.To see this,consider a network of self-interested agents whose individual desire is to minimize their local cost U i ðx Þ¼Pj 2N i k x j Àx i Àr ij k 2via a distributed algorithm (x i is the position of vehicle i with dynamics _x i ¼u i and r ij is a desired intervehicle relative-position vector).Instead,if the agents use gradient-descent algorithm on the collective cost P n i ¼1U i ðx Þusing the following protocol:_x i ¼Xj 2N iðx j Àx i Àr ij Þ¼Xj 2N iðx j Àx i Þþb i (6)with input bias b i ¼Pj 2N i r ji [see Fig.1(b)],the objective of every agent will be achieved.This is the same as the consensus algorithm in (1)up to the nonzero bias terms b i .This nonzero bias plays no role in stability analysis of sys-tem (6).Thus,distributed formation control for integrator agents is a consensus problem.The main contribution of the work by Fax and Murray is to extend this scenario to the case where all agents are multiinput multioutput linear systems _x i ¼Ax i þBu i .Stability analysis of relative-position-based formation control for multivehicle systems is extensively covered in Section IV.E.OutlineThe outline of the paper is as follows.Basic concepts and theoretical results in information consensus are presented in Section II.Convergence and performance analysis of consensus on networks with switching topology are given in Section III.A theoretical framework for cooperative control of formations of networked multi-vehicle systems is provided in Section IV.Some simulationresults related to consensus in complex networks including small-worlds are presented in Section V.Finally,some concluding remarks are stated in Section VI.RMATION CONSENSUSConsider a network of decision-making agents with dynamics _x i ¼u i interested in reaching a consensus via local communication with their neighbors on a graph G ¼ðV ;E Þ.By reaching a consensus,we mean asymptot-ically converging to a one-dimensional agreement space characterized by the following equation:x 1¼x 2¼...¼x n :This agreement space can be expressed as x ¼ 1where 1¼ð1;...;1ÞT and 2R is the collective decision of the group of agents.Let A ¼½a ij be the adjacency matrix of graph G .The set of neighbors of a agent i is N i and defined byN i ¼f j 2V :a ij ¼0g ;V ¼f 1;...;n g :Agent i communicates with agent j if j is a neighbor of i (or a ij ¼0).The set of all nodes and their neighbors defines the edge set of the graph as E ¼fði ;j Þ2V ÂV :a ij ¼0g .A dynamic graph G ðt Þ¼ðV ;E ðt ÞÞis a graph in which the set of edges E ðt Þand the adjacency matrix A ðt Þare time-varying.Clearly,the set of neighbors N i ðt Þof every agent in a dynamic graph is a time-varying set as well.Dynamic graphs are useful for describing the network topology of mobile sensor networks and flocks [19].It is shown in [10]that the linear system_x i ðt Þ¼Xj 2N ia ij x j ðt ÞÀx i ðt ÞÀÁ(7)is a distributed consensus algorithm ,i.e.,guarantees con-vergence to a collective decision via local interagent interactions.Assuming that the graph is undirected (a ij ¼a ji for all i ;j ),it follows that the sum of the state of all nodes is an invariant quantity,or P i _xi ¼0.In particular,applying this condition twice at times t ¼0and t ¼1gives the following result¼1n Xix i ð0Þ:In other words,if a consensus is asymptotically reached,then necessarily the collective decision is equal to theOlfati-Saber et al.:Consensus and Cooperation in Networked Multi-Agent SystemsVol.95,No.1,January 2007|Proceedings of the IEEE219average of the initial state of all nodes.A consensus algo-rithm with this specific invariance property is called an average-consensus algorithm [9]and has broad applications in distributed computing on networks (e.g.,sensor fusion in sensor networks).The dynamics of system (7)can be expressed in a compact form as_x ¼ÀLx(8)where L is known as the graph Laplacian of G .The graph Laplacian is defined asL ¼D ÀA(9)where D ¼diag ðd 1;...;d n Þis the degree matrix of G with elements d i ¼Pj ¼i a ij and zero off-diagonal elements.By definition,L has a right eigenvector of 1associated with the zero eigenvalue 5because of the identity L 1¼0.For the case of undirected graphs,graph Laplacian satisfies the following sum-of-squares (SOS)property:x T Lx ¼12Xði ;j Þ2Ea ij ðx j Àx i Þ2:(10)By defining a quadratic disagreement function as’ðx Þ¼12x T Lx(11)it becomes apparent that algorithm (7)is the same as_x ¼Àr ’ðx Þor the gradient-descent algorithm.This algorithm globallyasymptotically converges to the agreement space provided that two conditions hold:1)L is a positive semidefinite matrix;2)the only equilibrium of (7)is 1for some .Both of these conditions hold for a connected graph and follow from the SOS property of graph Laplacian in (10).Therefore,an average-consensus is asymptotically reached for all initial states.This fact is summarized in the following lemma.Lemma 1:Let G be a connected undirected graph.Then,the algorithm in (7)asymptotically solves an average-consensus problem for all initial states.A.Algebraic Connectivity and Spectral Propertiesof GraphsSpectral properties of Laplacian matrix are instrumen-tal in analysis of convergence of the class of linear consensus algorithms in (7).According to Gershgorin theorem [81],all eigenvalues of L in the complex plane are located in a closed disk centered at Áþ0j with a radius of Á¼max i d i ,i.e.,the maximum degree of a graph.For undirected graphs,L is a symmetric matrix with real eigenvalues and,therefore,the set of eigenvalues of L can be ordered sequentially in an ascending order as0¼ 1 2 ÁÁÁ n 2Á:(12)The zero eigenvalue is known as the trivial eigenvalue of L .For a connected graph G , 290(i.e.,the zero eigenvalue is isolated).The second smallest eigenvalue of Laplacian 2is called algebraic connectivity of a graph [20].Algebraic connectivity of the network topology is a measure of performance/speed of consensus algorithms [10].Example 1:Fig.2shows two examples of networks of integrator agents with different topologies.Both graphs are undirected and have 0–1weights.Every node of the graph in Fig.2(a)is connected to its 4nearest neighbors on a ring.The other graph is a proximity graph of points that are distributed uniformly at random in a square.Every node is connected to all of its spatial neighbors within a closed ball of radius r 90.Here are the important degree information and Laplacian eigenvalues of these graphsa Þ 1¼0; 2¼0:48; n ¼6:24;Á¼4b Þ 1¼0; 2¼0:25; n ¼9:37;Á¼8:(13)In both cases, i G 2Áfor all i .B.Convergence Analysis for Directed Networks The convergence analysis of the consensus algorithm in (7)is equivalent to proving that the agreement space characterized by x ¼ 1; 2R is an asymptotically stable equilibrium of system (7).The stability properties of system (7)is completely determined by the location of the Laplacian eigenvalues of the network.The eigenvalues of the adjacency matrix are irrelevant to the stability analysis of system (7),unless the network is k -regular (all of its nodes have the same degree k ).The following lemma combines a well-known rank property of graph Laplacians with Gershgorin theorem to provide spectral characterization of Laplacian of a fixed directed network G .Before stating the lemma,we need to define the notion of strong connectivity of graphs.A graph5These properties were discussed earlier in the introduction for graphs with 0–1weights.Olfati-Saber et al.:Consensus and Cooperation in Networked Multi-Agent Systems220Proceedings of the IEEE |Vol.95,No.1,January 2007。
【国家自然科学基金】_示踪法_基金支持热词逐年推荐_【万方软件创新助手】_20140730

1 1 1 1 1 1 1 1 1 1 1 1 1 1 1 1 1 1 1 1 1 1 1 1 1 1 1 1 1 1 1 1 1 1 1 1 1 1 1 1 1 1 1 1 1 1 1 1 1 1 1 1 1 1
53 54 55 56
丹江口 三峡贯通 22na 137cs示踪技术
107 108 109 110 111 112 113 114 115 116 117
2010年 序号 1 2 3 4 5 6 7 8 9 10 11 12 13 14 15 16 17 18 19 20 21 22 23 24 25 26 27 28 29 30 31 32 33 34 35 36 37 38 39 40 41 42 43 44 45 46 47 48 49 50 51 52
推荐指数 3 3 3 2 2 2 2 2 2 2 2 2 2 1 1 1 1 1 1 1 1 1 1 1 1 1 1 1 1 1 1 1 1 1 1 1 1 1 1 1 1 1 1 1 1 1 1 1 1 1 1 1
2009年 序号 1 2 3 4 5 6 7 8 9 10 11 12 13 14 15 16 17 18 19 20 21 22 23 24 25 26 27 28 29 30 31 32 33 34 35 36 37 38 39 40 41 42 43 44 45 46 47 48 49 50 51 52
2008年 序号 1 2 3 4 5 6 7 8 9 10 11 12 13 14 15 16 17 18 19 20 21 22 23 24 25 26 27 28 29 30 31 32 33 34 35 36 37 38 39 40 41 42 43 44 45 46 47 48 49 50 51 52
Parallel and Distributed Computing and Systems
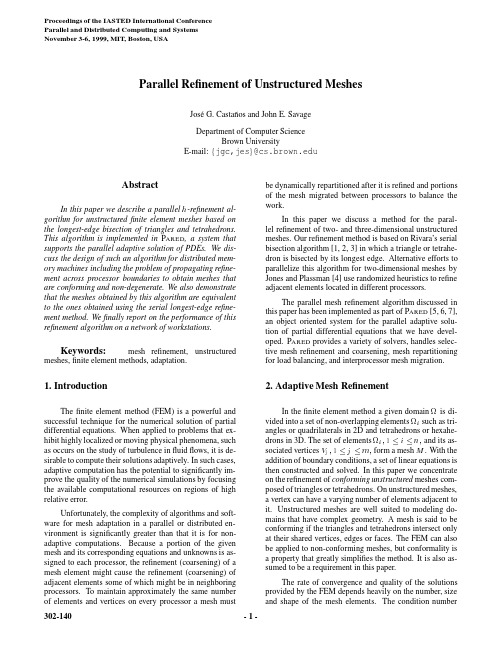
Proceedings of the IASTED International ConferenceParallel and Distributed Computing and SystemsNovember3-6,1999,MIT,Boston,USAParallel Refinement of Unstructured MeshesJos´e G.Casta˜n os and John E.SavageDepartment of Computer ScienceBrown UniversityE-mail:jgc,jes@AbstractIn this paper we describe a parallel-refinement al-gorithm for unstructuredfinite element meshes based on the longest-edge bisection of triangles and tetrahedrons. This algorithm is implemented in P ARED,a system that supports the parallel adaptive solution of PDEs.We dis-cuss the design of such an algorithm for distributed mem-ory machines including the problem of propagating refine-ment across processor boundaries to obtain meshes that are conforming and non-degenerate.We also demonstrate that the meshes obtained by this algorithm are equivalent to the ones obtained using the serial longest-edge refine-ment method.Wefinally report on the performance of this refinement algorithm on a network of workstations.Keywords:mesh refinement,unstructured meshes,finite element methods,adaptation.1.IntroductionThefinite element method(FEM)is a powerful and successful technique for the numerical solution of partial differential equations.When applied to problems that ex-hibit highly localized or moving physical phenomena,such as occurs on the study of turbulence influidflows,it is de-sirable to compute their solutions adaptively.In such cases, adaptive computation has the potential to significantly im-prove the quality of the numerical simulations by focusing the available computational resources on regions of high relative error.Unfortunately,the complexity of algorithms and soft-ware for mesh adaptation in a parallel or distributed en-vironment is significantly greater than that it is for non-adaptive computations.Because a portion of the given mesh and its corresponding equations and unknowns is as-signed to each processor,the refinement(coarsening)of a mesh element might cause the refinement(coarsening)of adjacent elements some of which might be in neighboring processors.To maintain approximately the same number of elements and vertices on every processor a mesh must be dynamically repartitioned after it is refined and portions of the mesh migrated between processors to balance the work.In this paper we discuss a method for the paral-lel refinement of two-and three-dimensional unstructured meshes.Our refinement method is based on Rivara’s serial bisection algorithm[1,2,3]in which a triangle or tetrahe-dron is bisected by its longest edge.Alternative efforts to parallelize this algorithm for two-dimensional meshes by Jones and Plassman[4]use randomized heuristics to refine adjacent elements located in different processors.The parallel mesh refinement algorithm discussed in this paper has been implemented as part of P ARED[5,6,7], an object oriented system for the parallel adaptive solu-tion of partial differential equations that we have devel-oped.P ARED provides a variety of solvers,handles selec-tive mesh refinement and coarsening,mesh repartitioning for load balancing,and interprocessor mesh migration.2.Adaptive Mesh RefinementIn thefinite element method a given domain is di-vided into a set of non-overlapping elements such as tri-angles or quadrilaterals in2D and tetrahedrons or hexahe-drons in3D.The set of elements and its as-sociated vertices form a mesh.With theaddition of boundary conditions,a set of linear equations is then constructed and solved.In this paper we concentrate on the refinement of conforming unstructured meshes com-posed of triangles or tetrahedrons.On unstructured meshes, a vertex can have a varying number of elements adjacent to it.Unstructured meshes are well suited to modeling do-mains that have complex geometry.A mesh is said to be conforming if the triangles and tetrahedrons intersect only at their shared vertices,edges or faces.The FEM can also be applied to non-conforming meshes,but conformality is a property that greatly simplifies the method.It is also as-sumed to be a requirement in this paper.The rate of convergence and quality of the solutions provided by the FEM depends heavily on the number,size and shape of the mesh elements.The condition number(a)(b)(c)Figure1:The refinement of the mesh in using a nested refinement algorithm creates a forest of trees as shown in and.The dotted lines identify the leaf triangles.of the matrices used in the FEM and the approximation error are related to the minimum and maximum angle of all the elements in the mesh[8].In three dimensions,the solid angle of all tetrahedrons and their ratio of the radius of the circumsphere to the inscribed sphere(which implies a bounded minimum angle)are usually used as measures of the quality of the mesh[9,10].A mesh is non-degenerate if its interior angles are never too small or too large.For a given shape,the approximation error increases with ele-ment size(),which is usually measured by the length of the longest edge of an element.The goal of adaptive computation is to optimize the computational resources used in the simulation.This goal can be achieved by refining a mesh to increase its resolution on regions of high relative error in static problems or by re-fining and coarsening the mesh to follow physical anoma-lies in transient problems[11].The adaptation of the mesh can be performed by changing the order of the polynomi-als used in the approximation(-refinement),by modifying the structure of the mesh(-refinement),or a combination of both(-refinement).Although it is possible to replace an old mesh with a new one with smaller elements,most -refinement algorithms divide each element in a selected set of elements from the current mesh into two or more nested subelements.In P ARED,when an element is refined,it does not get destroyed.Instead,the refined element inserts itself into a tree,where the root of each tree is an element in the initial mesh and the leaves of the trees are the unrefined elements as illustrated in Figure1.Therefore,the refined mesh forms a forest of refinement trees.These trees are used in many of our algorithms.Error estimates are used to determine regions where adaptation is necessary.These estimates are obtained from previously computed solutions of the system of equations. After adaptation imbalances may result in the work as-signed to processors in a parallel or distributed environ-ment.Efficient use of resources may require that elements and vertices be reassigned to processors at runtime.There-fore,any such system for the parallel adaptive solution of PDEs must integrate subsystems for solving equations,adapting a mesh,finding a good assignment of work to processors,migrating portions of a mesh according to anew assignment,and handling interprocessor communica-tion efficiently.3.P ARED:An OverviewP ARED is a system of the kind described in the lastparagraph.It provides a number of standard iterativesolvers such as Conjugate Gradient and GMRES and pre-conditioned versions thereof.It also provides both-and -refinement of meshes,algorithms for adaptation,graph repartitioning using standard techniques[12]and our ownParallel Nested Repartitioning(PNR)[7,13],and work mi-gration.P ARED runs on distributed memory parallel comput-ers such as the IBM SP-2and networks of workstations.These machines consist of coarse-grained nodes connectedthrough a high to moderate latency network.Each nodecannot directly address a memory location in another node. In P ARED nodes exchange messages using MPI(Message Passing Interface)[14,15,16].Because each message has a high startup cost,efficient message passing algorithms must minimize the number of messages delivered.Thus, it is better to send a few large messages rather than many small ones.This is a very important constraint and has a significant impact on the design of message passing algo-rithms.P ARED can be run interactively(so that the user canvisualize the changes in the mesh that results from meshadaptation,partitioning and migration)or without directintervention from the user.The user controls the systemthrough a GUI in a distinguished node called the coordina-tor,.This node collects information from all the other processors(such as its elements and vertices).This tool uses OpenGL[17]to permit the user to view3D meshes from different angles.Through the coordinator,the user can also give instructions to all processors such as specify-ing when and how to adapt the mesh or which strategy to use when repartitioning the mesh.In our computation,we assume that an initial coarse mesh is given and that it is loaded into the coordinator.The initial mesh can then be partitioned using one of a num-ber of serial graph partitioning algorithms and distributed between the processors.P ARED then starts the simulation. Based on some adaptation criterion[18],P ARED adapts the mesh using the algorithms explained in Section5.Af-ter the adaptation phase,P ARED determines if a workload imbalance exists due to increases and decreases in the num-ber of mesh elements on individual processors.If so,it invokes a procedure to decide how to repartition mesh el-ements between processors;and then moves the elements and vertices.We have found that PNR gives partitions with a quality comparable to those provided by standard meth-ods such as Recursive Spectral Bisection[19]but which(b)(a)Figure2:Mesh representation in a distributed memory ma-chine using remote references.handles much larger problems than can be handled by stan-dard methods.3.1.Object-Oriented Mesh RepresentationsIn P ARED every element of the mesh is assigned to a unique processor.V ertices are shared between two or more processors if they lie on a boundary between parti-tions.Each of these processors has a copy of the shared vertices and vertices refer to each other using remote ref-erences,a concept used in object-oriented programming. This is illustrated in Figure2on which the remote refer-ences(marked with dashed arrows)are used to maintain the consistency of multiple copies of the same vertex in differ-ent processors.Remote references are functionally similar to standard C pointers but they address objects in a different address space.A processor can use remote references to invoke meth-ods on objects located in a different processor.In this case, the method invocations and arguments destined to remote processors are marshalled into messages that contain the memory addresses of the remote objects.In the destina-tion processors these addresses are converted to pointers to objects of the corresponding type through which the meth-ods are invoked.Because the different nodes are inher-ently trusted and MPI guarantees reliable communication, P ARED does not incur the overhead traditionally associated with distributed object systems.Another idea commonly found in object oriented pro-gramming and which is used in P ARED is that of smart pointers.An object can be destroyed when there are no more references to it.In P ARED vertices are shared be-tween several elements and each vertex counts the number of elements referring to it.When an element is created, the reference count of its vertices is incremented.Simi-larly,when the element is destroyed,the reference count of its vertices is decremented.When the reference count of a vertex reaches zero,the vertex is no longer attached to any element located in the processor and can be destroyed.If a vertex is shared,then some other processor might have a re-mote reference to it.In that case,before a copy of a shared vertex is destroyed,it informs the copies in other processors to delete their references to itself.This procedure insures that the shared vertex can then be safely destroyed without leaving dangerous dangling pointers referring to it in other processors.Smart pointers and remote references provide a simple replication mechanism that is tightly integrated with our mesh data structures.In adaptive computation,the struc-ture of the mesh evolves during the computation.During the adaptation phase,elements and vertices are created and destroyed.They may also be assigned to a different pro-cessor to rebalance the work.As explained above,remote references and smart pointers greatly simplify the task of creating dynamic meshes.4.Adaptation Using the Longest Edge Bisec-tion AlgorithmMany-refinement techniques[20,21,22]have been proposed to serially refine triangular and tetrahedral meshes.One widely used method is the longest-edge bisec-tion algorithm proposed by Rivara[1,2].This is a recursive procedure(see Figure3)that in two dimensions splits each triangle from a selected set of triangles by adding an edge between the midpoint of its longest side to the opposite vertex.In the case that makes a neighboring triangle,,non-conforming,then is refined using the same algorithm.This may cause the refinement to prop-agate throughout the mesh.Nevertheless,this procedure is guaranteed to terminate because the edges it bisects in-crease in length.Building on the work of Rosenberg and Stenger[23]on bisection of triangles,Rivara[1,2]shows that this refinement procedure provably produces two di-mensional meshes in which the smallest angle of the re-fined mesh is no less than half of the smallest angle of the original mesh.The longest-edge bisection algorithm can be general-ized to three dimensions[3]where a tetrahedron is bisected into two tetrahedrons by inserting a triangle between the midpoint of its longest edge and the two vertices not in-cluded in this edge.The refinement propagates to neigh-boring tetrahedrons in a similar way.This procedure is also guaranteed to terminate,but unlike the two dimensional case,there is no known bound on the size of the small-est angle.Nevertheless,experiments conducted by Rivara [3]suggest that this method does not produce degenerate meshes.In two dimensions there are several variations on the algorithm.For example a triangle can initially be bisected by the longest edge,but then its children are bisected by the non-conforming edge,even if it is that is not their longest edge[1].In three dimensions,the bisection is always per-formed by the longest edge so that matching faces in neigh-boring tetrahedrons are always bisected by the same com-mon edge.Bisect()let,and be vertices of the trianglelet be the longest side of and let be the midpoint ofbisect by the edge,generating two new triangles andwhile is a non-conforming vertex dofind the non-conforming triangle adjacent to the edgeBisect()end whileFigure3:Longest edge(Rivara)bisection algorithm for triangular meshes.Because in P ARED refined elements are not destroyed in the refinement tree,the mesh can be coarsened by replac-ing all the children of an element by their parent.If a parent element is selected for coarsening,it is important that all the elements that are adjacent to the longest edge of are also selected for coarsening.If neighbors are located in different processors then only a simple message exchange is necessary.This algorithm generates conforming meshes: a vertex is removed only if all the elements that contain that vertex are all coarsened.It does not propagate like the re-finement algorithm and it is much simpler to implement in parallel.For this reason,in the rest of the paper we will focus on the refinement of meshes.5.Parallel Longest-Edge RefinementThe longest-edge bisection algorithm and many other mesh refinement algorithms that propagate the refinement to guarantee conformality of the mesh are not local.The refinement of one particular triangle or tetrahedron can propagate through the mesh and potentially cause changes in regions far removed from.If neighboring elements are located in different processors,it is necessary to prop-agate this refinement across processor boundaries to main-tain the conformality of the mesh.In our parallel longest edge bisection algorithm each processor iterates between a serial phase,in which there is no communication,and a parallel phase,in which each processor sends and receives messages from other proces-sors.In the serial phase,processor selects a setof its elements for refinement and refines them using the serial longest edge bisection algorithms outlined earlier. The refinement often creates shared vertices in the bound-ary between adjacent processors.To minimize the number of messages exchanged between and,delays the propagation of refinement to until has refined all the elements in.The serial phase terminates when has no more elements to refine.A processor informs an adjacent processor that some of its elements need to be refined by sending a mes-sage from to containing the non-conforming edges and the vertices to be inserted at their midpoint.Each edge is identified by its endpoints and and its remote ref-erences(see Figure4).If and are sharedvertices,(a)(c)(b)Figure4:In the parallel longest edge bisection algo-rithm some elements(shaded)are initially selected for re-finement.If the refinement creates a new(black)ver-tex on a processor boundary,the refinement propagates to neighbors.Finally the references are updated accord-ingly.then has a remote reference to copies of and lo-cated in processor.These references are included in the message,so that can identify the non-conforming edge and insert the new vertex.A similar strategy can be used when the edge is refined several times during the re-finement phase,but in this case,the vertex is not located at the midpoint of.Different processors can be in different phases during the refinement.For example,at any given time a processor can be refining some of its elements(serial phase)while neighboring processors have refined all their elements and are waiting for propagation messages(parallel phase)from adjacent processors.waits until it has no elements to refine before receiving a message from.For every non-conforming edge included in a message to,creates its shared copy of the midpoint(unless it already exists) and inserts the new non-conforming elements adjacent to into a new set of elements to be refined.The copy of in must also have a remote reference to the copy of in.For this reason,when propagates the refine-ment to it also includes in the message a reference to its copies of shared vertices.These steps are illustrated in Figure4.then enters the serial phase again,where the elements in are refined.(c)(b)(a)Figure5:Both processors select(shaded)mesh el-ements for refinement.The refinement propagates to a neighboring processor resulting in more elements be-ing refined.5.1.The Challenge of Refining in ParallelThe description of the parallel refinement algorithm is not complete because refinement propagation across pro-cessor boundaries can create two synchronization prob-lems.Thefirst problem,adaptation collision,occurs when two(or more)processors decide to refine adjacent elements (one in each processor)during the serial phase,creating two(or more)vertex copies over a shared edge,one in each processor.It is important that all copies refer to the same logical vertex because in a numerical simulation each ver-tex must include the contribution of all the elements around it(see Figure5).The second problem that arises,termination detection, is the determination that a refinement phase is complete. The serial refinement algorithm terminates when the pro-cessor has no more elements to refine.In the parallel ver-sion termination is a global decision that cannot be deter-mined by an individual processor and requires a collabora-tive effort of all the processors involved in the refinement. Although a processor may have adapted all of its mesh elements in,it cannot determine whether this condition holds for all other processors.For example,at any given time,no processor might have any more elements to re-fine.Nevertheless,the refinement cannot terminate because there might be some propagation messages in transit.The algorithm for detecting the termination of parallel refinement is based on Dijkstra’s general distributed termi-nation algorithm[24,25].A global termination condition is reached when no element is selected for refinement.Hence if is the set of all elements in the mesh currently marked for refinement,then the algorithmfinishes when.The termination detection procedure uses message ac-knowledgments.For every propagation message that receives,it maintains the identity of its source()and to which processors it propagated refinements.Each prop-agation message is acknowledged.acknowledges to after it has refined all the non-conforming elements created by’s message and has also received acknowledgments from all the processors to which it propagated refinements.A processor can be in two states:an inactive state is one in which has no elements to refine(it cannot send new propagation messages to other processors)but can re-ceive messages.If receives a propagation message from a neighboring processor,it moves from an inactive state to an active state,selects the elements for refinement as spec-ified in the message and proceeds to refine them.Let be the set of elements in needing refinement.A processor becomes inactive when:has received an acknowledgment for every propa-gation message it has sent.has acknowledged every propagation message it has received..Using this definition,a processor might have no more elements to refine()but it might still be in an active state waiting for acknowledgments from adjacent processors.When a processor becomes inactive,sends an acknowledgment to the processors whose propagation message caused to move from an inactive state to an active state.We assume that the refinement is started by the coordi-nator processor,.At this stage,is in the active state while all the processors are in the inactive state.ini-tiates the refinement by sending the appropriate messages to other processors.This message also specifies the adapta-tion criterion to use to select the elements for refinement in.When a processor receives a message from,it changes to an active state,selects some elements for refine-ment either explicitly or by using the specified adaptation criterion,and then refines them using the serial bisection algorithm,keeping track of the vertices created over shared edges as described earlier.When itfinishes refining its ele-ments,sends a message to each processor on whose shared edges created a shared vertex.then listens for messages.Only when has refined all the elements specified by and is not waiting for any acknowledgment message from other processors does it sends an acknowledgment to .Global termination is detected when the coordinator becomes inactive.When receives an acknowledgment from every processor this implies that no processor is re-fining an element and that no processor is waiting for an acknowledgment.Hence it is safe to terminate the refine-ment.then broadcasts this fact to all the other proces-sors.6.Properties of Meshes Refined in ParallelOur parallel refinement algorithm is guaranteed to ter-minate.In every serial phase the longest edge bisectionLet be a set of elements to be refinedwhile there is an element dobisect by its longest edgeinsert any non-conforming element intoend whileFigure6:General longest-edge bisection(GLB)algorithm.algorithm is used.In this algorithm the refinement prop-agates towards progressively longer edges and will even-tually reach the longest edge in each processor.Between processors the refinement also propagates towards longer edges.Global termination is detected by using the global termination detection procedure described in the previous section.The resulting mesh is conforming.Every time a new vertex is created over a shared edge,the refinement propagates to adjacent processors.Because every element is always bisected by its longest edge,for triangular meshes the results by Rosenberg and Stenger on the size of the min-imum angle of two-dimensional meshes also hold.It is not immediately obvious if the resulting meshes obtained by the serial and parallel longest edge bisection al-gorithms are the same or if different partitions of the mesh generate the same refined mesh.As we mentioned earlier, messages can arrive from different sources in different or-ders and elements may be selected for refinement in differ-ent sequences.We now show that the meshes that result from refining a set of elements from a given mesh using the serial and parallel algorithms described in Sections4and5,re-spectively,are the same.In this proof we use the general longest-edge bisection(GLB)algorithm outlined in Figure 6where the order in which elements are refined is not spec-ified.In a parallel environment,this order depends on the partition of the mesh between processors.After showing that the resulting refined mesh is independent of the order in which the elements are refined using the serial GLB al-gorithm,we show that every possible distribution of ele-ments between processors and every order of parallel re-finement yields the same mesh as would be produced by the serial algorithm.Theorem6.1The mesh that results from the refinement of a selected set of elements of a given mesh using the GLB algorithm is independent of the order in which the elements are refined.Proof:An element is refined using the GLBalgorithm if it is in the initial set or refinementpropagates to it.An element is refinedif one of its neighbors creates a non-conformingvertex at the midpoint of one of its edges.Therefinement of by its longest edge divides theelement into two nested subelements andcalled the children of.These children are inturn refined by their longest edge if one of their edges is non-conforming.The refinement proce-dure creates a forest of trees of nested elements where the root of each tree is an element in theinitial mesh and the leaves are unrefined ele-ments.For every element,let be the refinement tree of nested elements rooted atwhen the refinement procedure terminates. Using the GLB procedure elements can be se-lected for refinement in different orders,creating possible different refinement histories.To show that this cannot happen we assume the converse, namely,that two refinement histories and generate different refined meshes,and establish a contradiction.Thus,assume that there is an ele-ment such that the refinement trees and,associated with the refinement histories and of respectively,are different.Be-cause the root of and is the same in both refinement histories,there is a place where both treesfirst differ.That is,starting at the root,there is an element that is common to both trees but for some reason,its children are different.Be-cause is always bisected by the longest edge, the children of are different only when is refined in one refinement history and it is not re-fined in the other.In other words,in only one of the histories does have children.Because is refined in only one refinement his-tory,then,the initial set of elements to refine.This implies that must have been refined because one of its edges became non-conforming during one of the refinement histo-ries.Let be the set of elements that are present in both refinement histories,but are re-fined in and not in.We define in a similar way.For each refinement history,every time an ele-ment is refined,it is assigned an increasing num-ber.Select an element from either or that has the lowest number.Assume that we choose from so that is refined in but not in.In,is refined because a neigh-boring element created a non-conforming ver-tex at the midpoint of their shared edge.There-fore is refined in but not in because otherwise it would cause to be refined in both sequences.This implies that is also in and has a lower refinement number than con-。
Electronic transport in two dimensional graphene
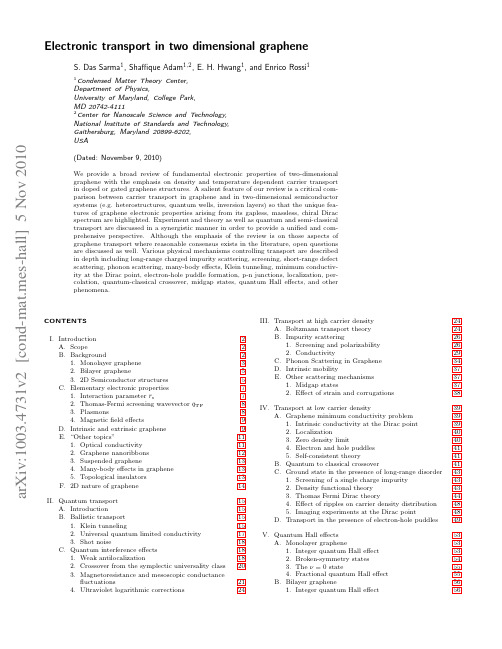
arXiv:1003.4731v2 [cond-mat.mes-hall] 5 Nov 2010
(Dated: November 9, 2010)
We provide a broad review of fundamental electronic properties of two-dimensional graphene with the emphasis on density and temperature dependent carrier transport in doped or gated graphene structures. A salient feature of our review is a critical comparison between carrier transport in graphene and in two-dimensional semiconductor systems (e.g. heterostructures, quantum wells, inversion layers) so that the unique features of graphene electronic properties arising from its gapless, massless, chiral Dirac spectrum are highlighted. Experiment and theory as well as quantum and semi-classical transport are discussed in a synergistic manner in order to provide a unified and comprehensive perspective. Although the emphasis of the review is on those aspects of graphene transport where reasonable consensus exists in the literature, open questions are discussed as well. Various physical mechanisms controlling transport are described in depth including long-range charged impurity scattering, screening, short-range defect scattering, phonon scattering, many-body effects, Klein tunneling, minimum conductivity at the Dirac point, electron-hole puddle formation, p-n junctions, localization, percolation, quantum-classical crossover, midgap states, quantum Hall effects, and other phenomena.
北京大学科技成果——拉普拉斯域光学乳腺影像系统(LD-DOT)

北京大学科技成果——拉普拉斯域光学乳腺影像系
统(LD-DOT)
项目概述
拉普拉斯域光学乳腺影像系统(LD-DOT)是一种基于漫反射光成像原理的功能成像手段。
有别于基于形态学进行诊断的传统医学成像手段,它利用不同病变的乳腺组织对光的漫反射程度的不同,通过得到双乳全局血管微循环特征参数的定量分布来诊断组织病理属性(正常/良性/恶性)以及分布范围,探测深度可达6厘米以上,扫描过程安全快速,结果直接客观,是一种经济高效的诊断乳腺病变的无创方法。
应用范围
本系统可用于例行的乳腺检查、乳腺癌筛查,提供定量的生理信息可帮助医生对患者进行早期的乳腺癌诊断,准确区分正常组织与良性、恶性肿瘤。
由于该系统便携程度高,不仅适用于医院、体检中心筛查,也适用于流动医疗工作站。
另外,系统技术也可扩展到脑血管相关研究,如中风监控、脑活动跟踪等。
技术优势
系统技术先进性主要体现在:
(1)成本低廉成像质量高
DOT领域的自主技术创新(美国专利8649010),克服传统DOT 技术以及常用影像学手段无法兼顾成像质量和成本的局限;
(2)准确性高
由定量生理信息的检测来形成诊断依据,灵敏度,特异性均高于其他影像学方法,不存在读图差异;
(3)无创且无放射性
使用对人体无害的低能量近红外光,适用不同年龄段广大人群密集跟踪检查;
(4)便携度高,对环境无特殊要求。
研究阶段
该技术前期已在新加坡国家肿瘤中心进行了临床验证,目前正在制造第二代样机,并与相关三甲医院达成人体实验合作意向,处于中试早期阶段。
设备目标市场定位于体检机构、高端美容院、社区医院等。
Anomaly Detection A Survey(综述)
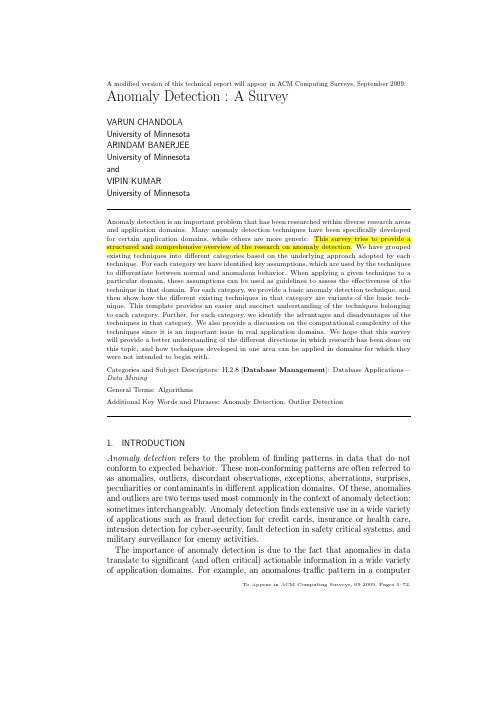
A modified version of this technical report will appear in ACM Computing Surveys,September2009. Anomaly Detection:A SurveyVARUN CHANDOLAUniversity of MinnesotaARINDAM BANERJEEUniversity of MinnesotaandVIPIN KUMARUniversity of MinnesotaAnomaly detection is an important problem that has been researched within diverse research areas and application domains.Many anomaly detection techniques have been specifically developed for certain application domains,while others are more generic.This survey tries to provide a structured and comprehensive overview of the research on anomaly detection.We have grouped existing techniques into different categories based on the underlying approach adopted by each technique.For each category we have identified key assumptions,which are used by the techniques to differentiate between normal and anomalous behavior.When applying a given technique to a particular domain,these assumptions can be used as guidelines to assess the effectiveness of the technique in that domain.For each category,we provide a basic anomaly detection technique,and then show how the different existing techniques in that category are variants of the basic tech-nique.This template provides an easier and succinct understanding of the techniques belonging to each category.Further,for each category,we identify the advantages and disadvantages of the techniques in that category.We also provide a discussion on the computational complexity of the techniques since it is an important issue in real application domains.We hope that this survey will provide a better understanding of the different directions in which research has been done on this topic,and how techniques developed in one area can be applied in domains for which they were not intended to begin with.Categories and Subject Descriptors:H.2.8[Database Management]:Database Applications—Data MiningGeneral Terms:AlgorithmsAdditional Key Words and Phrases:Anomaly Detection,Outlier Detection1.INTRODUCTIONAnomaly detection refers to the problem offinding patterns in data that do not conform to expected behavior.These non-conforming patterns are often referred to as anomalies,outliers,discordant observations,exceptions,aberrations,surprises, peculiarities or contaminants in different application domains.Of these,anomalies and outliers are two terms used most commonly in the context of anomaly detection; sometimes interchangeably.Anomaly detectionfinds extensive use in a wide variety of applications such as fraud detection for credit cards,insurance or health care, intrusion detection for cyber-security,fault detection in safety critical systems,and military surveillance for enemy activities.The importance of anomaly detection is due to the fact that anomalies in data translate to significant(and often critical)actionable information in a wide variety of application domains.For example,an anomalous traffic pattern in a computerTo Appear in ACM Computing Surveys,092009,Pages1–72.2·Chandola,Banerjee and Kumarnetwork could mean that a hacked computer is sending out sensitive data to an unauthorized destination[Kumar2005].An anomalous MRI image may indicate presence of malignant tumors[Spence et al.2001].Anomalies in credit card trans-action data could indicate credit card or identity theft[Aleskerov et al.1997]or anomalous readings from a space craft sensor could signify a fault in some compo-nent of the space craft[Fujimaki et al.2005].Detecting outliers or anomalies in data has been studied in the statistics commu-nity as early as the19th century[Edgeworth1887].Over time,a variety of anomaly detection techniques have been developed in several research communities.Many of these techniques have been specifically developed for certain application domains, while others are more generic.This survey tries to provide a structured and comprehensive overview of the research on anomaly detection.We hope that it facilitates a better understanding of the different directions in which research has been done on this topic,and how techniques developed in one area can be applied in domains for which they were not intended to begin with.1.1What are anomalies?Anomalies are patterns in data that do not conform to a well defined notion of normal behavior.Figure1illustrates anomalies in a simple2-dimensional data set. The data has two normal regions,N1and N2,since most observations lie in these two regions.Points that are sufficiently far away from the regions,e.g.,points o1 and o2,and points in region O3,are anomalies.Fig.1.A simple example of anomalies in a2-dimensional data set. Anomalies might be induced in the data for a variety of reasons,such as malicious activity,e.g.,credit card fraud,cyber-intrusion,terrorist activity or breakdown of a system,but all of the reasons have a common characteristic that they are interesting to the analyst.The“interestingness”or real life relevance of anomalies is a key feature of anomaly detection.Anomaly detection is related to,but distinct from noise removal[Teng et al. 1990]and noise accommodation[Rousseeuw and Leroy1987],both of which deal To Appear in ACM Computing Surveys,092009.Anomaly Detection:A Survey·3 with unwanted noise in the data.Noise can be defined as a phenomenon in data which is not of interest to the analyst,but acts as a hindrance to data analysis. Noise removal is driven by the need to remove the unwanted objects before any data analysis is performed on the data.Noise accommodation refers to immunizing a statistical model estimation against anomalous observations[Huber1974]. Another topic related to anomaly detection is novelty detection[Markou and Singh2003a;2003b;Saunders and Gero2000]which aims at detecting previously unobserved(emergent,novel)patterns in the data,e.g.,a new topic of discussion in a news group.The distinction between novel patterns and anomalies is that the novel patterns are typically incorporated into the normal model after being detected.It should be noted that solutions for above mentioned related problems are often used for anomaly detection and vice-versa,and hence are discussed in this review as well.1.2ChallengesAt an abstract level,an anomaly is defined as a pattern that does not conform to expected normal behavior.A straightforward anomaly detection approach,there-fore,is to define a region representing normal behavior and declare any observation in the data which does not belong to this normal region as an anomaly.But several factors make this apparently simple approach very challenging:—Defining a normal region which encompasses every possible normal behavior is very difficult.In addition,the boundary between normal and anomalous behavior is often not precise.Thus an anomalous observation which lies close to the boundary can actually be normal,and vice-versa.—When anomalies are the result of malicious actions,the malicious adversaries often adapt themselves to make the anomalous observations appear like normal, thereby making the task of defining normal behavior more difficult.—In many domains normal behavior keeps evolving and a current notion of normal behavior might not be sufficiently representative in the future.—The exact notion of an anomaly is different for different application domains.For example,in the medical domain a small deviation from normal(e.g.,fluctuations in body temperature)might be an anomaly,while similar deviation in the stock market domain(e.g.,fluctuations in the value of a stock)might be considered as normal.Thus applying a technique developed in one domain to another is not straightforward.—Availability of labeled data for training/validation of models used by anomaly detection techniques is usually a major issue.—Often the data contains noise which tends to be similar to the actual anomalies and hence is difficult to distinguish and remove.Due to the above challenges,the anomaly detection problem,in its most general form,is not easy to solve.In fact,most of the existing anomaly detection techniques solve a specific formulation of the problem.The formulation is induced by various factors such as nature of the data,availability of labeled data,type of anomalies to be detected,etc.Often,these factors are determined by the application domain inTo Appear in ACM Computing Surveys,092009.4·Chandola,Banerjee and Kumarwhich the anomalies need to be detected.Researchers have adopted concepts from diverse disciplines such as statistics ,machine learning ,data mining ,information theory ,spectral theory ,and have applied them to specific problem formulations.Figure 2shows the above mentioned key components associated with any anomaly detection technique.Anomaly DetectionTechniqueApplication DomainsMedical InformaticsIntrusion Detection...Fault/Damage DetectionFraud DetectionResearch AreasInformation TheoryMachine LearningSpectral TheoryStatisticsData Mining...Problem CharacteristicsLabels Anomaly Type Nature of Data OutputFig.2.Key components associated with an anomaly detection technique.1.3Related WorkAnomaly detection has been the topic of a number of surveys and review articles,as well as books.Hodge and Austin [2004]provide an extensive survey of anomaly detection techniques developed in machine learning and statistical domains.A broad review of anomaly detection techniques for numeric as well as symbolic data is presented by Agyemang et al.[2006].An extensive review of novelty detection techniques using neural networks and statistical approaches has been presented in Markou and Singh [2003a]and Markou and Singh [2003b],respectively.Patcha and Park [2007]and Snyder [2001]present a survey of anomaly detection techniques To Appear in ACM Computing Surveys,092009.Anomaly Detection:A Survey·5 used specifically for cyber-intrusion detection.A substantial amount of research on outlier detection has been done in statistics and has been reviewed in several books [Rousseeuw and Leroy1987;Barnett and Lewis1994;Hawkins1980]as well as other survey articles[Beckman and Cook1983;Bakar et al.2006].Table I shows the set of techniques and application domains covered by our survey and the various related survey articles mentioned above.12345678TechniquesClassification Based√√√√√Clustering Based√√√√Nearest Neighbor Based√√√√√Statistical√√√√√√√Information Theoretic√Spectral√ApplicationsCyber-Intrusion Detection√√Fraud Detection√Medical Anomaly Detection√Industrial Damage Detection√Image Processing√Textual Anomaly Detection√Sensor Networks√Table parison of our survey to other related survey articles.1-Our survey2-Hodge and Austin[2004],3-Agyemang et al.[2006],4-Markou and Singh[2003a],5-Markou and Singh [2003b],6-Patcha and Park[2007],7-Beckman and Cook[1983],8-Bakar et al[2006]1.4Our ContributionsThis survey is an attempt to provide a structured and a broad overview of extensive research on anomaly detection techniques spanning multiple research areas and application domains.Most of the existing surveys on anomaly detection either focus on a particular application domain or on a single research area.[Agyemang et al.2006]and[Hodge and Austin2004]are two related works that group anomaly detection into multiple categories and discuss techniques under each category.This survey builds upon these two works by significantly expanding the discussion in several directions. We add two more categories of anomaly detection techniques,viz.,information theoretic and spectral techniques,to the four categories discussed in[Agyemang et al.2006]and[Hodge and Austin2004].For each of the six categories,we not only discuss the techniques,but also identify unique assumptions regarding the nature of anomalies made by the techniques in that category.These assumptions are critical for determining when the techniques in that category would be able to detect anomalies,and when they would fail.For each category,we provide a basic anomaly detection technique,and then show how the different existing techniques in that category are variants of the basic technique.This template provides an easier and succinct understanding of the techniques belonging to each category.Further, for each category we identify the advantages and disadvantages of the techniques in that category.We also provide a discussion on the computational complexity of the techniques since it is an important issue in real application domains.To Appear in ACM Computing Surveys,092009.6·Chandola,Banerjee and KumarWhile some of the existing surveys mention the different applications of anomaly detection,we provide a detailed discussion of the application domains where anomaly detection techniques have been used.For each domain we discuss the notion of an anomaly,the different aspects of the anomaly detection problem,and the challenges faced by the anomaly detection techniques.We also provide a list of techniques that have been applied in each application domain.The existing surveys discuss anomaly detection techniques that detect the sim-plest form of anomalies.We distinguish the simple anomalies from complex anoma-lies.The discussion of applications of anomaly detection reveals that for most ap-plication domains,the interesting anomalies are complex in nature,while most of the algorithmic research has focussed on simple anomalies.1.5OrganizationThis survey is organized into three parts and its structure closely follows Figure 2.In Section2we identify the various aspects that determine the formulation of the problem and highlight the richness and complexity associated with anomaly detection.We distinguish simple anomalies from complex anomalies and define two types of complex anomalies,viz.,contextual and collective anomalies.In Section 3we briefly describe the different application domains where anomaly detection has been applied.In subsequent sections we provide a categorization of anomaly detection techniques based on the research area which they belong to.Majority of the techniques can be categorized into classification based(Section4),nearest neighbor based(Section5),clustering based(Section6),and statistical techniques (Section7).Some techniques belong to research areas such as information theory (Section8),and spectral theory(Section9).For each category of techniques we also discuss their computational complexity for training and testing phases.In Section 10we discuss various contextual anomaly detection techniques.We discuss various collective anomaly detection techniques in Section11.We present some discussion on the limitations and relative performance of various existing techniques in Section 12.Section13contains concluding remarks.2.DIFFERENT ASPECTS OF AN ANOMALY DETECTION PROBLEMThis section identifies and discusses the different aspects of anomaly detection.As mentioned earlier,a specific formulation of the problem is determined by several different factors such as the nature of the input data,the availability(or unavailabil-ity)of labels as well as the constraints and requirements induced by the application domain.This section brings forth the richness in the problem domain and justifies the need for the broad spectrum of anomaly detection techniques.2.1Nature of Input DataA key aspect of any anomaly detection technique is the nature of the input data. Input is generally a collection of data instances(also referred as object,record,point, vector,pattern,event,case,sample,observation,entity)[Tan et al.2005,Chapter 2].Each data instance can be described using a set of attributes(also referred to as variable,characteristic,feature,field,dimension).The attributes can be of different types such as binary,categorical or continuous.Each data instance might consist of only one attribute(univariate)or multiple attributes(multivariate).In To Appear in ACM Computing Surveys,092009.Anomaly Detection:A Survey·7 the case of multivariate data instances,all attributes might be of same type or might be a mixture of different data types.The nature of attributes determine the applicability of anomaly detection tech-niques.For example,for statistical techniques different statistical models have to be used for continuous and categorical data.Similarly,for nearest neighbor based techniques,the nature of attributes would determine the distance measure to be used.Often,instead of the actual data,the pairwise distance between instances might be provided in the form of a distance(or similarity)matrix.In such cases, techniques that require original data instances are not applicable,e.g.,many sta-tistical and classification based techniques.Input data can also be categorized based on the relationship present among data instances[Tan et al.2005].Most of the existing anomaly detection techniques deal with record data(or point data),in which no relationship is assumed among the data instances.In general,data instances can be related to each other.Some examples are sequence data,spatial data,and graph data.In sequence data,the data instances are linearly ordered,e.g.,time-series data,genome sequences,protein sequences.In spatial data,each data instance is related to its neighboring instances,e.g.,vehicular traffic data,ecological data.When the spatial data has a temporal(sequential) component it is referred to as spatio-temporal data,e.g.,climate data.In graph data,data instances are represented as vertices in a graph and are connected to other vertices with ter in this section we will discuss situations where such relationship among data instances become relevant for anomaly detection. 2.2Type of AnomalyAn important aspect of an anomaly detection technique is the nature of the desired anomaly.Anomalies can be classified into following three categories:2.2.1Point Anomalies.If an individual data instance can be considered as anomalous with respect to the rest of data,then the instance is termed as a point anomaly.This is the simplest type of anomaly and is the focus of majority of research on anomaly detection.For example,in Figure1,points o1and o2as well as points in region O3lie outside the boundary of the normal regions,and hence are point anomalies since they are different from normal data points.As a real life example,consider credit card fraud detection.Let the data set correspond to an individual’s credit card transactions.For the sake of simplicity, let us assume that the data is defined using only one feature:amount spent.A transaction for which the amount spent is very high compared to the normal range of expenditure for that person will be a point anomaly.2.2.2Contextual Anomalies.If a data instance is anomalous in a specific con-text(but not otherwise),then it is termed as a contextual anomaly(also referred to as conditional anomaly[Song et al.2007]).The notion of a context is induced by the structure in the data set and has to be specified as a part of the problem formulation.Each data instance is defined using following two sets of attributes:To Appear in ACM Computing Surveys,092009.8·Chandola,Banerjee and Kumar(1)Contextual attributes.The contextual attributes are used to determine thecontext(or neighborhood)for that instance.For example,in spatial data sets, the longitude and latitude of a location are the contextual attributes.In time-series data,time is a contextual attribute which determines the position of an instance on the entire sequence.(2)Behavioral attributes.The behavioral attributes define the non-contextual char-acteristics of an instance.For example,in a spatial data set describing the average rainfall of the entire world,the amount of rainfall at any location is a behavioral attribute.The anomalous behavior is determined using the values for the behavioral attributes within a specific context.A data instance might be a contextual anomaly in a given context,but an identical data instance(in terms of behavioral attributes)could be considered normal in a different context.This property is key in identifying contextual and behavioral attributes for a contextual anomaly detection technique.TimeFig.3.Contextual anomaly t2in a temperature time series.Note that the temperature at time t1is same as that at time t2but occurs in a different context and hence is not considered as an anomaly.Contextual anomalies have been most commonly explored in time-series data [Weigend et al.1995;Salvador and Chan2003]and spatial data[Kou et al.2006; Shekhar et al.2001].Figure3shows one such example for a temperature time series which shows the monthly temperature of an area over last few years.A temperature of35F might be normal during the winter(at time t1)at that place,but the same value during summer(at time t2)would be an anomaly.A similar example can be found in the credit card fraud detection domain.A contextual attribute in credit card domain can be the time of purchase.Suppose an individual usually has a weekly shopping bill of$100except during the Christmas week,when it reaches$1000.A new purchase of$1000in a week in July will be considered a contextual anomaly,since it does not conform to the normal behavior of the individual in the context of time(even though the same amount spent during Christmas week will be considered normal).The choice of applying a contextual anomaly detection technique is determined by the meaningfulness of the contextual anomalies in the target application domain. To Appear in ACM Computing Surveys,092009.Anomaly Detection:A Survey·9 Another key factor is the availability of contextual attributes.In several cases defining a context is straightforward,and hence applying a contextual anomaly detection technique makes sense.In other cases,defining a context is not easy, making it difficult to apply such techniques.2.2.3Collective Anomalies.If a collection of related data instances is anomalous with respect to the entire data set,it is termed as a collective anomaly.The indi-vidual data instances in a collective anomaly may not be anomalies by themselves, but their occurrence together as a collection is anomalous.Figure4illustrates an example which shows a human electrocardiogram output[Goldberger et al.2000]. The highlighted region denotes an anomaly because the same low value exists for an abnormally long time(corresponding to an Atrial Premature Contraction).Note that that low value by itself is not an anomaly.Fig.4.Collective anomaly corresponding to an Atrial Premature Contraction in an human elec-trocardiogram output.As an another illustrative example,consider a sequence of actions occurring in a computer as shown below:...http-web,buffer-overflow,http-web,http-web,smtp-mail,ftp,http-web,ssh,smtp-mail,http-web,ssh,buffer-overflow,ftp,http-web,ftp,smtp-mail,http-web...The highlighted sequence of events(buffer-overflow,ssh,ftp)correspond to a typical web based attack by a remote machine followed by copying of data from the host computer to remote destination via ftp.It should be noted that this collection of events is an anomaly but the individual events are not anomalies when they occur in other locations in the sequence.Collective anomalies have been explored for sequence data[Forrest et al.1999; Sun et al.2006],graph data[Noble and Cook2003],and spatial data[Shekhar et al. 2001].To Appear in ACM Computing Surveys,092009.10·Chandola,Banerjee and KumarIt should be noted that while point anomalies can occur in any data set,collective anomalies can occur only in data sets in which data instances are related.In contrast,occurrence of contextual anomalies depends on the availability of context attributes in the data.A point anomaly or a collective anomaly can also be a contextual anomaly if analyzed with respect to a context.Thus a point anomaly detection problem or collective anomaly detection problem can be transformed toa contextual anomaly detection problem by incorporating the context information.2.3Data LabelsThe labels associated with a data instance denote if that instance is normal or anomalous1.It should be noted that obtaining labeled data which is accurate as well as representative of all types of behaviors,is often prohibitively expensive. Labeling is often done manually by a human expert and hence requires substantial effort to obtain the labeled training data set.Typically,getting a labeled set of anomalous data instances which cover all possible type of anomalous behavior is more difficult than getting labels for normal behavior.Moreover,the anomalous behavior is often dynamic in nature,e.g.,new types of anomalies might arise,for which there is no labeled training data.In certain cases,such as air traffic safety, anomalous instances would translate to catastrophic events,and hence will be very rare.Based on the extent to which the labels are available,anomaly detection tech-niques can operate in one of the following three modes:2.3.1Supervised anomaly detection.Techniques trained in supervised mode as-sume the availability of a training data set which has labeled instances for normal as well as anomaly class.Typical approach in such cases is to build a predictive model for normal vs.anomaly classes.Any unseen data instance is compared against the model to determine which class it belongs to.There are two major is-sues that arise in supervised anomaly detection.First,the anomalous instances are far fewer compared to the normal instances in the training data.Issues that arise due to imbalanced class distributions have been addressed in the data mining and machine learning literature[Joshi et al.2001;2002;Chawla et al.2004;Phua et al. 2004;Weiss and Hirsh1998;Vilalta and Ma2002].Second,obtaining accurate and representative labels,especially for the anomaly class is usually challenging.A number of techniques have been proposed that inject artificial anomalies in a normal data set to obtain a labeled training data set[Theiler and Cai2003;Abe et al.2006;Steinwart et al.2005].Other than these two issues,the supervised anomaly detection problem is similar to building predictive models.Hence we will not address this category of techniques in this survey.2.3.2Semi-Supervised anomaly detection.Techniques that operate in a semi-supervised mode,assume that the training data has labeled instances for only the normal class.Since they do not require labels for the anomaly class,they are more widely applicable than supervised techniques.For example,in space craft fault detection[Fujimaki et al.2005],an anomaly scenario would signify an accident, which is not easy to model.The typical approach used in such techniques is to 1Also referred to as normal and anomalous classes.To Appear in ACM Computing Surveys,092009.Anomaly Detection:A Survey·11 build a model for the class corresponding to normal behavior,and use the model to identify anomalies in the test data.A limited set of anomaly detection techniques exist that assume availability of only the anomaly instances for training[Dasgupta and Nino2000;Dasgupta and Majumdar2002;Forrest et al.1996].Such techniques are not commonly used, primarily because it is difficult to obtain a training data set which covers every possible anomalous behavior that can occur in the data.2.3.3Unsupervised anomaly detection.Techniques that operate in unsupervised mode do not require training data,and thus are most widely applicable.The techniques in this category make the implicit assumption that normal instances are far more frequent than anomalies in the test data.If this assumption is not true then such techniques suffer from high false alarm rate.Many semi-supervised techniques can be adapted to operate in an unsupervised mode by using a sample of the unlabeled data set as training data.Such adaptation assumes that the test data contains very few anomalies and the model learnt during training is robust to these few anomalies.2.4Output of Anomaly DetectionAn important aspect for any anomaly detection technique is the manner in which the anomalies are reported.Typically,the outputs produced by anomaly detection techniques are one of the following two types:2.4.1Scores.Scoring techniques assign an anomaly score to each instance in the test data depending on the degree to which that instance is considered an anomaly. Thus the output of such techniques is a ranked list of anomalies.An analyst may choose to either analyze top few anomalies or use a cut-offthreshold to select the anomalies.2.4.2Labels.Techniques in this category assign a label(normal or anomalous) to each test instance.Scoring based anomaly detection techniques allow the analyst to use a domain-specific threshold to select the most relevant anomalies.Techniques that provide binary labels to the test instances do not directly allow the analysts to make such a choice,though this can be controlled indirectly through parameter choices within each technique.3.APPLICATIONS OF ANOMALY DETECTIONIn this section we discuss several applications of anomaly detection.For each ap-plication domain we discuss the following four aspects:—The notion of anomaly.—Nature of the data.—Challenges associated with detecting anomalies.—Existing anomaly detection techniques.To Appear in ACM Computing Surveys,092009.。
IEEE 519-1992 谐波分析指南说明书

Harmonic Analysis and IEEE 1992 GuidelinesIntroductionIEEE 519-1992 provides guidelines for applying limits to the level of harmonic distortion that a utilitycustomer may inject into the power system. This is a concern, since Adjustable Frequency Drives (AFDs) can contribute significant harmonic distortion to a power system. The guidelines pertain to percentharmonic current and voltage distortion at the point of common coupling (PCC), which is defined as the point where the utility connects to multiple customers.Although many customers and system designers interpret the PCC to be at the AFD input or various locations within the 480V distribution, this is not consistent with the intent of IEEE guidelines. There are no limits recommended for individual loads, only for the overall system. Customers and system designers can choose the point of analysis (POA) where they desire, but it may add substantial filtering costs if the POA is downstream of the PCC.Current distortion drawn through an impedance (transformer, cable resistance) causes voltagedistortion.The distorted current will also cause additional heating of the input cables and the transformer. Excessive voltage distortion is a concern, since it may cause interference with other electronic equipment and additional motor heating.IEEE 519-1992 recommends different limits on Individual Harmonics (I h ) and Total Demand Distortion (TDD), depending on the I SC /I L ratio. I SC is the short circuit current at the PCC, and I L is the maximum demand load current (fundamental) at the PCC. More current distortion is allowed at higher I SC /I L ratios, since voltage distortion decreases as the ratio increases.The voltage distortion guidelines for IEEE-1992 (at 480V) remain the same as IEEE 519-1981:∙ 3% — Special systems (i.e. hospitals or universities) ∙ 5% — General systems∙ 10% —Dedicated systems (AFDs only)Application Note AP04014002EHarmonic Analysis and IEEE 1992 GuidelinesEffective July 20142 EATON CORPORATION The best way to estimate AFD harmonic contribution to an electrical system is to perform a harmonicanalysis based on known system characteristics. An individual AFD may meet the IEEE guidelines in one system and not meet the guidelines in another system depending on the pre-existing characteristics of the specific system.Some AFD vendors, upon seeing a specification requirement for IEEE 519-1992, will simply add a line reactor. This is the wrong approach, since some systems will not require a line reactor and others will not benefit sufficiently to meet the guidelines (or the specification).For a free computerized harmonic analysis of AFD contribution to system harmonics or for additional information, contact your local Eaton sales office. A one-line drawing of the electrical distribution system and specification criteria will be required. A harmonic analysis worksheet for required data is attached.Any additional harmonic mitigation equipment requirements will be determined during the analysis. If there are harmonic constraints during AFD operation on a standby generator, a separate analysis will be required for the generator, and assumptions on load-shedding strategies during generator operationshould be provided. Several data runs may be required to evaluate various harmonic mitigation methods. Resultant recommendations may include 1%, 3%, or 5% line reactors, phase-shifting transformers, filters, or CPX9000 Clean Power.Helpful Facts∙ Harmonics are supply system dependent. As the short circuit amps available (SCA) increase, %voltage distortion decreases, and % current distortion increases.∙ For each PCC or POA analysis required, provide SCA and IL (load current) values for that point. SCAs used must be without motor contribution to SCA.∙Current distortion percentages are dependent on overall system loading. As linear loads (non-harmonic loads such as AC motors on line power) increase, the percent current distortion decreases through dilution.∙ % current distortion is the same across a transformer. Voltage distortion percentage is lower on the primary than on the secondary, assuming the harmonic loads are on the secondary. ∙A harmonic analysis is only as accurate as the assumptions made for the analysis!Harmonic Analysis Data Worksheet(Use separate sheets if necessary. Provide a 1-line drawing or sketch.)lated voltage distortion and higher calculated current distortion on the transformer secondary.Distribution Transformer(s) Data:Generator Data:Describe load-shedding scheme for generator operation in “AFD Data” below.AFD Data:kVAImpedanceX/R Ratio#1#2kW kVAVoltsX"dI L (amps)#1#2AFD hpType (SVX9000,Clean Power, etc.)Quantity Operated on Line / GeneratorDesired, existing or specified line reactor or isolation transformer (none, 1% / 3% / 5%)//////Application Note AP04014002E Effective July 2014Harmonic Analysis and IEEE 1992 Guidelines3 EATON CORPORATION Application Note AP04014002E Harmonic Analysis and IEEE 1992 Guidelines Effective July 2014Additional HelpIn the US or Canada: please contact the Technical Resource Center at 1-877-ETN-CAREor 1-877-326-2273 option 2, option 6.All other supporting documentation is located on the Eaton web site at /DrivesEaton1000 Eaton BoulevardCleveland, OH 44122 USA© 2014 EatonAll Rights ReservedPrinted in USAPublication No.AP04014002EJuly 2014Eaton is a registered trademarkof Eaton Corporation.All other trademarks are propertyof their respective owners。
Tikhonov吉洪诺夫正则化

Tikhonov regularizationFrom Wikipedia, the free encyclopediaTikhonov regularization is the most commonly used method of of named for . In , the method is also known as ridge regression . It is related to the for problems.The standard approach to solve an of given as,b Ax =is known as and seeks to minimize the2bAx -where •is the . However, the matrix A may be or yielding a non-unique solution. In order to give preference to a particular solution with desirable properties, the regularization term is included in this minimization:22xb Ax Γ+-for some suitably chosen Tikhonov matrix , Γ. In many cases, this matrix is chosen as the Γ= I , giving preference to solutions with smaller norms. In other cases, operators ., a or a weighted ) may be used to enforce smoothness if the underlying vector is believed to be mostly continuous. This regularizationimproves the conditioning of the problem, thus enabling a numerical solution. An explicit solution, denoted by , is given by:()b A A A xTTT 1ˆ-ΓΓ+=The effect of regularization may be varied via the scale of matrix Γ. For Γ=αI , when α = 0 this reduces to the unregularized least squares solution providedthat (A T A)−1 exists.Contents••••••••Bayesian interpretationAlthough at first the choice of the solution to this regularized problem may look artificial, and indeed the matrix Γseems rather arbitrary, the process can be justified from a . Note that for an ill-posed problem one must necessarily introduce some additional assumptions in order to get a stable solution.Statistically we might assume that we know that x is a random variable with a . For simplicity we take the mean to be zero and assume that each component isindependent with σx. Our data is also subject to errors, and we take the errorsin b to be also with zero mean and standard deviation σb. Under these assumptions the Tikhonov-regularized solution is the solution given the dataand the a priori distribution of x, according to . The Tikhonov matrix is then Γ=αI for Tikhonov factor α = σb/ σx.If the assumption of is replaced by assumptions of and uncorrelatedness of , and still assume zero mean, then the entails that the solution is minimal . Generalized Tikhonov regularizationFor general multivariate normal distributions for x and the data error, one can apply a transformation of the variables to reduce to the case above. Equivalently,one can seek an x to minimize22Q P x x b Ax -+-where we have used 2P x to stand for the weighted norm x T Px (cf. the ). In the Bayesian interpretation P is the inverse of b , x 0 is the of x , and Q is the inverse covariance matrix of x . The Tikhonov matrix is then given as a factorization of the matrix Q = ΓT Γ. the ), and is considered a . This generalized problem can be solved explicitly using the formula()()010Ax b P A QPA A x T T-++-[] Regularization in Hilbert spaceTypically discrete linear ill-conditioned problems result as discretization of , and one can formulate Tikhonov regularization in the original infinite dimensional context. In the above we can interpret A as a on , and x and b as elements in the domain and range of A . The operator ΓΓ+T A A *is then a bounded invertible operator.Relation to singular value decomposition and Wiener filterWith Γ= αI , this least squares solution can be analyzed in a special way viathe . Given the singular value decomposition of AT V U A ∑=with singular values σi , the Tikhonov regularized solution can be expressed asb VDU xT =ˆ where D has diagonal values22ασσ+=i i ii Dand is zero elsewhere. This demonstrates the effect of the Tikhonov parameteron the of the regularized problem. For the generalized case a similar representation can be derived using a . Finally, it is related to the :∑==qi iiT i i v bu f x1ˆσwhere the Wiener weights are 222ασσ+=i i i f and q is the of A .Determination of the Tikhonov factorThe optimal regularization parameter α is usually unknown and often in practical problems is determined by an ad hoc method. A possible approach relies on the Bayesian interpretation described above. Other approaches include the , , , and . proved that the optimal parameter, in the sense of minimizes:()()[]21222ˆTTXIX XX I Tr y X RSSG -+--==αβτwhereis the and τ is the effective number .Using the previous SVD decomposition, we can simplify the above expression:()()21'22221'∑∑==++-=qi iiiqi iiub u ub u y RSS ασα()21'2220∑=++=qi iiiub u RSS RSS ασαand∑∑==++-=+-=qi iqi i i q m m 12221222ασαασστRelation to probabilistic formulationThe probabilistic formulation of an introduces (when all uncertainties are Gaussian) a covariance matrix C M representing the a priori uncertainties on the model parameters, and a covariance matrix C D representing the uncertainties on the observed parameters (see, for instance, Tarantola, 2004 ). In the special case when these two matrices are diagonal and isotropic,and, and, in this case, the equations of inverse theory reduce to theequations above, with α = σD / σM .HistoryTikhonov regularization has been invented independently in many differentcontexts. It became widely known from its application to integral equations from the work of and D. L. Phillips. Some authors use the term Tikhonov-Phillips regularization . The finite dimensional case was expounded by A. E. Hoerl, who took a statistical approach, and by M. Foster, who interpreted this method as a - filter. Following Hoerl, it is known in the statistical literature as ridge regression .[] References•(1943). "Об устойчивости обратных задач [On the stability of inverse problems]". 39 (5): 195–198.•Tychonoff, A. N. (1963). "О решении некорректно поставленных задач и методе регуляризации [Solution of incorrectly formulated problems and the regularization method]". Doklady Akademii Nauk SSSR151:501–504.. Translated in Soviet Mathematics4: 1035–1038. •Tychonoff, A. N.; V. Y. Arsenin (1977). Solution of Ill-posed Problems.Washington: Winston & Sons. .•Hansen, ., 1998, Rank-deficient and Discrete ill-posed problems, SIAM •Hoerl AE, 1962, Application of ridge analysis to regression problems, Chemical Engineering Progress, 58, 54-59.•Foster M, 1961, An application of the Wiener-Kolmogorov smoothing theory to matrix inversion, J. SIAM, 9, 387-392•Phillips DL, 1962, A technique for the numerical solution of certain integral equations of the first kind, J Assoc Comput Mach, 9, 84-97•Tarantola A, 2004, Inverse Problem Theory (), Society for Industrial and Applied Mathematics,•Wahba, G, 1990, Spline Models for Observational Data, Society for Industrial and Applied Mathematics。
Principles_of_Algebraic_Geometr_Griffiths_Harris
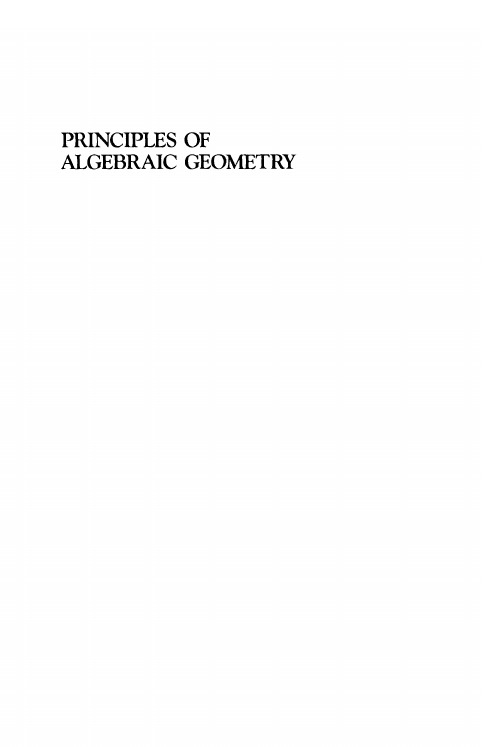
Includes bibliographical references.
1. Geometry, Algebraic. I. Harris, Joseph,
1951- jointauthor.il. Title.
QA564.G64
516'.35
78-6993
ISBN 0-471-05059-8
Printed in the United States of America
A number of principles guided the preparation of the book. One was to develop only that general machinery necessary to study the concrete geometric questions and special classes of algebraic varieties around which the presentation was centered.
No part of this publication may be reproduced, stored in a retrieval system or transmitted in any form or by any means, electronic, mechanical, photocopying, recording, scanning or otherwise, except as permitted under Sections 107 or 108 of the 1976 United States Copyright Act, without either the prior written permission of the Publisher, or authorization through payment of the appropriate per-copy fee to the Copyright Clearance Center, 222 Rosewood Drive, Danvers, MA 01923, (978) 750-8400, fax (978) 750-4470. Requests to the Publisher for permission should be addressed to the Permissions Department, John Wiley & Sons, Inc., 111 River Street, Hoboken, NJ 07030, (201) 748-6011, fax (201) 748-6008.
Euler Parameters in Computational Kinematics
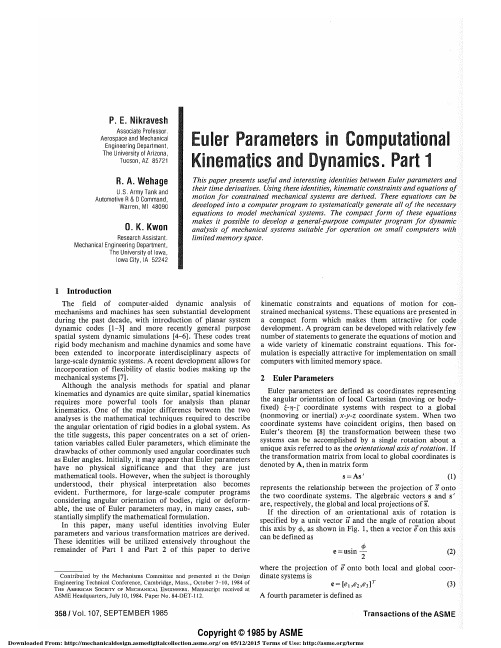
Associate Professor. Aerospace and Mechanical Engineering Department, The University of Arizona, Tucson, AZ 85721
Euler Parameters in Computational Kinematics and Dynamics. Part 1
The field of computer-aided dynamic analysis of mechanisms and machines has seen substantial development during the past decade, with introduction of planar system dynamic codes [1-3] and more recently general purpose spatial system dynamic simulations [4-6]. These codes treat rigid body mechanism and machine dynamics and some have been extended to incorporate interdisciplinary aspects of large-scale dynamic systems. A recent development allows for incorporation of flexibility of elastic bodies making up the mechanical systems [7], Although the analysis methods for spatial and planar kinematics and dynamics are quite similar, spatial kinematics requires more powerful tools for analysis than planar kinematics. One of the major differencs between the two analyses is the mathematical techniques required to describe the angular orientation of rigid bodies in a global system. As the title suggests, this paper concentrates on a set of orientation variables called Euler parameters, which eliminate the drawbacks of other commonly used angular coordinates such as Euler angles. Initially, it may appear that Euler parameters have no physical significance and that they are just mathematical tools. However, when the subject is thoroughly understood, their physical interpretation also becomes evident. Furthermore, for large-scale' computer programs considering angular orientation of bodies, rigid or deformable, the use of Euler parameters may, in many cases, substantially simplify the mathematical formulation. In this paper, many useful identities involving Euler parameters and various transformation matrices are derived. These identities will be utilized extensively throughout the remainder of Part 1 and Part 2 of this paper to derive
- 1、下载文档前请自行甄别文档内容的完整性,平台不提供额外的编辑、内容补充、找答案等附加服务。
- 2、"仅部分预览"的文档,不可在线预览部分如存在完整性等问题,可反馈申请退款(可完整预览的文档不适用该条件!)。
- 3、如文档侵犯您的权益,请联系客服反馈,我们会尽快为您处理(人工客服工作时间:9:00-18:30)。
Egerv´a ry Research Groupon Combinatorial OptimizationTechnical reportSTR-2002-12.Published by the Egrerv´a ry Research Group,P´a zm´a ny P.s´e t´a ny1/C, H–1117,Budapest,Hungary.Web site:www.cs.elte.hu/egres.ISSN1587–4451. Connected rigidity matroids andunique realizations of graphsBill Jackson and Tibor Jord´a nMarch28,2003EGRES Technical Report No.2002-121 Connected rigidity matroids and unique realizationsof graphsBill Jackson and Tibor Jord´a nAbstractA d-dimensional framework is a straight line embedding of a graph G inR d.We shall only consider generic frameworks,in which the co-ordinates ofall the vertices of G are algebraically independent.Two frameworks for G areequivalent if corresponding edges in the two frameworks have the same length.A framework is a unique realization of G in R d if every equivalent frameworkcan be obtained from it by a rigid congruence of R d.Bruce Hendrickson provedthat if G has a unique realization in R d then G is(d+1)-connected and redun-dantly rigid.He conjectured that every realization of a(d+1)-connected andredundantly rigid graph in R d is unique.This conjecture is true for d=1butwas disproved by Robert Connelly for d≥3.We resolve the remaining opencase by showing that Hendrickson’s conjecture is true for d=2.As a corol-lary we deduce that every realization of a6-connected graph as a2-dimensionalgeneric framework is a unique realization.Our proof is based on a new inductivecharacterization of3-connected graphs whose rigidity matroid is connected.1IntroductionWe shall considerfinite graphs without loops,multiple edges or isolated vertices.A d-dimensional framework is a pair(G,p),where G=(V,E)is a graph and p is a map from V to R d.We consider the framework to be a straight line embedding of G in R d in which the length of an edge uv∈E is given by the Euclidean distance between the points p(u)and p(v).Two frameworks(G,p)and(G,q)are equivalent if corresponding edges of the two frameworks have the same length.We say that two frameworks(G,p), (G,q)are congruent if there is a rigid congruence(i.e.translation or rotation)of R d which maps p(v)onto q(v)for each v∈V.We shall say that(G,p)is a unique realization of G in R d if every framework which is equivalent to(G,p)is congruent to(G,p).The unique realization problem is to decide whether a given realization School of Mathematical Sciences,Queen Mary,University of London,Mile End Road,London E1 4NS,England.e-mail:B.Jackson@ Supported by the Royal Society/Hungarian Academy of Science Exchange Programme.Department of Operations Research,E¨o tv¨o s University,P´a zm´a ny P´e ter s´e t´a ny1/C,1117Bu-dapest,Hungary.e-mail:jordan@cs.elte.hu Supported by the Hungarian Scientific Research Fund grant no.T037547,F034930,and FKFP grant no.0143/2001.is unique.Saxe[14]proved that this problem is NP-hard.We obtain a problem of different type,however,if we exclude‘degenerate’cases.A framework(G,p)is said to be generic if the coordinates of all the points are algebraically independent over the rationals.In what follows we shall consider the unique realization problem for generic frameworks.A simple necessary condition for unique realization of generic frameworks is rigidity. Intuitively,this means that if we think of a d-dimensional framework(G,p)as a collection of bars and joints where points correspond to joints and each edge to a rigid bar joining its end-points,then the framework is rigid if it has no non-trivial continuous deformations.It is known[18]that rigidity is a generic property,that is,the rigidity of(G,p)depends only on the graph G,if(G,p)is generic.We say that the graph G is rigid in R d if every generic realization of G in R d is rigid.(A combinatorial definition for the rigidity of G in R2will be given in Section2of this paper.We refer the reader to[18,19]for a formal definition and detailed survey of the rigidity of d-dimensional frameworks.)The necessary condition of rigidity was strengthened by Hendrickson[9]as follows.A graph G is2-rigid in R d if deleting any edge of G results in a graph which is rigid in R d.(Other authors have used the terms redundantly rigid and edge birigid for 2-rigid.)By using methods from differential topology,Hendrickson proved that the 2-rigidity of G is a stronger necessary condition for the unique realizability of a generic framework(G,p).Hendrickson[9]also pointed out that the(d+1)-connectivity of G is another necessary condition for a d-dimensional framework(G,p)to be a unique realization of G:if G has at least d+2vertices and has a vertex separator of size d,then we can obtain an equivalent framework to(G,p)by reflecting G along this separator. Summarising we haveTheorem1.1.[9]If a generic framework(G,p)is a unique realization of G in R d then either G is the complete graph with at most d+1vertices,or the following conditions hold:(a)G is(d+1)–connected,and(b)G is2-rigid.Hendrickson[7,8,9]conjectured that conditions(a)and(b)are sufficient to guar-antee that any generic framework(G,p)is a unique realization of G.This conjecture is easy to prove for d=1since G is rigid in R if and only if G is connected;G is 2-rigid in R if and only if G is2-edge-connected;and(G,p)is a unique generic real-ization of G in R if and only if G is2-connected.On the other hand,Connelly[3]has shown that Hendrickson’s conjecture is false for d≥3.We shall settle the remaining case by showing that the conjecture is true for d=2.As a corollary we deduce that unique realizability is also a generic property,that is to say the unique realizability of a2-dimensional generic framework(G,p)depends only on the graph G.Following Connelly[3],we say that a graph G is globally rigid in R d if every generic realization of G in R d is a unique realization.Our solution of the conjecture implies that G is globally rigid in R2if and only if G is a complete graph on at most three vertices or G is3-connected and2-rigid.Our proof of the conjecture is based on an inductive construction for all3-connected 2-rigid graphs.We shall show that every graph in this family can be built up from K4(which is globally rigid)by an appropriate sequence of operations,where each of the two operations we use preserves global rigidity.One operation is edge addition:we add a new edge connecting some pair of non-adjacent vertices.The other is1-extension:we subdivide an edge uv by a new vertex z,and add a new edge zw for some w=u,v.Clearly,thefirst operation preserves global rigidity.So does the second.This fact follows from a deep result of Connelly [4](see also[2],[8]),who developed a sufficient condition for the global rigidity of a generic framework in terms of the rank of its‘stress matrix’.Based on this condition, he proved that if G is globally rigid in R2and G is obtained from G by a1-extension, then G is also globally rigid in R2.In what follows we shall assume that d=2.In this case both conditions in Hendrick-son’s conjecture can be characterized(and efficiently tested)by purely combinatorial methods.This is straightforward for3-connectivity.In the case of2-rigidity,the com-binatorial characterization and algorithm are based on the following result of Laman [11].For a graph(G,E)and a subset X⊆V let i G(X)(or simply i(X)when it is obvious to which graph we are referring)denote the number of edges in the subgraph induced by X in G.The graph G is said to be minimally rigid if G is rigid,and G−e is not rigid for all e∈E.Theorem1.2.[11]A graph G=(V,E)is minimally rigid in R2if and only if |E|=2|V|−3andi(X)≤2|X|−3for all X⊂V with|X|≥2.(1) Note that a graph is rigid if and only if it has a minimally rigid spanning subgraph. It can be seen from Theorem1.2that a2-rigid graph G=(V,E)will have at least four vertices and at least2|V|−2edges.We call graphs which are2-rigid and have this minimum number of edges M-circuits.Motivated by Hendrickson’s conjecture, Connelly conjectured(see e.g.[6,p.99],[18,p.188])in the1980’s that all3-connected M-circuits can be obtained from K4by1-extensions.It is easy to see that the1-extension operation preserves3-connectivity and that it creates an M-circuit from an M-circuit.The other direction is more difficult.It is equivalent to saying that every 3-connected M-circuit on at leastfive vertices has a vertex of degree three which can be“suppressed”by the inverse operation to1-extension,so that the resulting graph is a smaller3-connected M-circuit.The inverse operation to1-extension is called splitting off:it chooses a vertex v of degree three in a graph G,deletes v(and the edges incident to v)and adds a new edge connecting two non-adjacent neighbours of v.If G is a3-connected M-circuit with at leastfive vertices and at least one of the splittings of v results in a3-connected M-circuit,then we say that the vertex v is feasible.It can be seen that each M-circuit G has at least four vertices of degree three.It is not true,however,that each vertex of degree three in G is feasible.The existence of such a vertex was verified by Berg and the second named author[1]in their recent solution to Connelly’s conjecture.In this paper we shall show that every3-connected2-rigid graph can be obtained from K4by edge additions and1-extensions by extending the methods in[1].We show that every3-connected2-rigid graph G on at leastfive vertices either contains an edge e such that G−e is3-connected and2-rigid,or a vertex v of degree three such that splitting offv in G results in a graph which is3-connected and2-rigid. The structure of the paper is as follows.In Section2we review elementary results on rigidity:we define the rigidity matroid of a graph and use it to give combinatorial definitions for when a graph is rigid,2-rigid or an M-circuit.In Section3we char-acterize M-connected graphs(graphs with a connected rigidity matroid).Section4 describes and extends lemmas from[1]on splitting offin M-circuits.In Section5, we use the concept of an ear decomposition of a matroid to extend the splitting offtheorem of[1]from M-circuits to M-connected graphs.We use this in Section6to obtain our above mentioned recursive construction for3-connected2-rigid graphs and hence solve Hendrickson’s conjecture.2Rigid graphs and the rigidity matroidIn this section we prove a number of preliminary lemmas and basic results,most of which are known.Our goal is to make the paper self-contained and to give a unified picture of these frequently used statements.Our proofs are based on Laman’s theorem and use only graph theoretical arguments.Some of these results can be found in[6,12,16,18,19].Let G=(V,E)be a graph.Let S be a non-empty subset of E,and H be the subgraph of G induced by edge set S.We say that S is independent ifi H(X)≤2|X|−3for all X⊆V(H)with|X|≥2.(2) The empty set is also defined to be independent.The rigidity matroid M(G)=(E,I) is defined on the edge set of G byI={S⊆E:S is independent in G}.To see that M(G)is indeed a matroid,we shall verify that the following three matroid axioms are satisfied.(For basic matroid definitions not given here the reader may consult the book[13].)(M1)∅∈I,(M2)if Y⊂X∈I then Y∈I,(M3)for every E ⊆E the maximal independent subsets of E have the same cardi-nality.Let G=(V,E)be a graph.For X,Y,Z⊂V,let E(X)be the set of edges of G[X], d(X,Y)=|E(X∪Y)−(E(X)∪E(Y))|,and d(X,Y,Z)=|E(X∪Y∪Z)−(E(X)∪E(Y)∪E(Z))|.We define the degree of X by d(X)=d(X,V−X).The degree of a vertex v is simply denoted by d(v).We shall need the following equalities,which are easy to check by counting the contribution of an edge to each of their two sides.Lemma2.1.Let G be a graph and X,Y⊆V(G).Theni(X)+i(Y)+d(X,Y)=i(X∪Y)+i(X∩Y).(3) Lemma2.2.Let G be a graph and X,Y,Z⊆V(G).Theni(X)+i(Y)+i(Z)+d(X,Y,Z)=i(X∪Y∪Z)+i(X∩Y)+i(X∩Z)+i(Y∩Z)−i(X∩Y∩Z).We say that the graph H=(V,F)is M-independent if F is independent in M(H). We call a set X⊆V critical if i(X)=2|X|−3holds.Lemma2.3.Let H=(V,F)be M-independent and let X,Y⊂V be critical sets in H with|X∩Y|≥2.Then X∩Y and X∪Y are also critical,and d(X,Y)=0. Proof:Since H is M-independent,(2)holds.By(3)we have2|X|−3+2|Y|−3=i(X)+i(Y)=i(X∩Y)+i(X∪Y)−d(X,Y)≤2|X∩Y|−3+2|X∪Y|−3−d(X,Y)=2|X|−3+2|Y|−3−d(X,Y).Thus d(X,Y)=0and equality holds everywhere.Therefore X∩Y and X∪Y are also critical.•Lemma2.4.Let G=(V,E )be a graph with|E |≥1and let F⊆E be a maximal independent subset of E .Then|F|=min{ti=1(2|X i|−3)}(4)where the minimum is taken over all collections of subsets{X1,X2,...,X t}of V(G) such that{E(X1),E(X2),...,E(X t)}partitions E .Proof:Since F is independent,we have|F∩E(X i)|≤2|X i|−3for all1≤i≤t. Thus|F|≤ t i=1(2|X i|−3)for any collection of subsets{X1,X2,...,X t}satisfying the hypothesis of the lemma.To see that equality can be attained,let H be the subgraph of G induced by F.Consider the maximal critical sets X1,X2,...,X t in H.By Lemma2.3we have |X i∩X j|≤1for all1≤i<j≤t.Since every single edge of F induces a critical set, it follows that{E H(X1),E H(X2),...,E H(X t)}is a partition of F.Thus|F|=t1|E H(X i)|=t1(2|X i|−3).To complete the proof we show that{E G(X1),E G(X2),...,E G(X t)}is a partition of E .Choose uv∈E −F.Since F is a maximal independent subset of E ,F+uv is dependent.Thus there exists a set X⊆V such that u,v∈X and i H(X)=2|X|−3. Hence X is a critical set in H.This implies that X⊆X i and hence uv∈E G(X i)forsome1≤i≤t.•It follows from the definition of independence that M(G)satisfies axioms(M1)and (M2).Lemma2.4implies that M(G)also satisfies(M3).It also determines the rank function of M(G),which we shall denote by r G or simply by r.Corollary2.5.First Let G=(V,E)be a graph.Then M(G)is a matroid,in which the rank of a non-empty set E ⊆E of edges is given byr(E )=min{ti=1(2|X i)|−3)}where the minimum is taken over all collections of subsets{X1,X2,...,X t}of G such that{E(X1),E(X2),...,E(X t)}partitions E .We say that a graph G=(V,E)is rigid if r(E)=2|V|−3in(M)(G).The graph G is minimally rigid if it is rigid and|E|=2|V|−3.Thus,if G is rigid and H=(V,E ) is a spanning subgraph of G,then H is minimally rigid if and only if E is a base in M(G).Theorem1.2ensures that these definitions agree with the intuitive definitions for rigidity given in Section1.A k-separation of a graph H=(V,E)is a pair(H1,H2)of edge-disjoint subgraphs of G each with at least k+1vertices such that H=H1∪H2and|V(H1)∩V(H2)|=k. The graph H is said to be k-connected if it has at least k+1vertices and has no j-separation for all0≤j≤k−1.If(H1,H2)is a k-separation of H,then we say that V(H1)∩V(H2)is a k-separator of H.For X⊆V let N(X)denote the set of neighbours of X(that is,N(X):={v∈V−X:uv∈E for some u∈X}).2.1Minimally rigid graphsWefirst investigate the connectivity properties of minimally rigid graphs.Lemma2.6.Let G=(V,E)be minimally rigid with|V|≥3.Then(a)G is2-connected.(b)for every∅=X⊂V we have d(X)≥2and if d(X)=2holds then either|X|=1 or|V−X|=1,Proof:Suppose that for some v∈V the graph G−v is disconnected and let A∪B be a partition of V−v with d(A,B)=0.Then(2)gives|E|=2|V|−3= i(A+v)+i(B+v)≤2(|A|+1)−3+2(|B|+1)−3=2(|A|+|B|+1)−4=2|V|−4, a contradiction.This proves(a).Using(a),we have d(X)≥2for every∅=X⊂V.Suppose|X|,|V−X|≥2.By (2)we obtain|E|=i(X)+i(V−X)+d(X)≤2|X|−3+2|V−X|−3+d(X)= 2|V|−6+d(X)=|E|−3+d(X).This implies d(X)≥3and proves(b).•Let v be a vertex in a graph G with d(v)=3and N(v)={u,w,z}.Recall that the operation splitting offmeans deleting v(and the edges incident to v)and adding anew edge,say uw,connecting two non-adjacent vertices of N(v).The resulting graphis denoted by G uwv and we say that the splitting is made on the pair uv,wv.Note thatv can be split offin at most three different ways.Let G=(V,E)be minimally rigid and let v be a vertex with d(v)=3.Splittingoffv on the pair uv,wv is said to be suitable if G uwv is minimally rigid.We also call avertex v suitable if there is an suitable splitting at v.We shall show that every vertexof degree three in a minimally rigid graph is suitable.Lemma2.7.Let G=(V,E)be minimally rigid and let X,Y,Z⊂V be critical setsin G with|X∩Y|=|X∩Z|=|Y∩Z|=1and X∩Y∩Z=∅.Then X∪Y∪Z iscritical,and d(X,Y,Z)=0.Proof:Since G is minimally rigid and our sets are critical,Lemma 2.2gives2|X|−3+2|Y|−3+2|Z|−3+d(X,Y,Z)=i(X)+i(Y)+i(Z)+d(X,Y,Z)≤i(X∪Y∪Z)≤2(|X∪Y∪Z|)−3=2(|X|+|Y|+|Z|−3)−3=2|X|−3+2|Y|−3+2|Z|−3.Hence d(X,Y,Z)=0and equality holds everywhere.Thus X∪Y∪Z is critical.•Lemma2.8.Let v be a vertex in an minimally rigid graph G=(V,E).(a)If d(v)=2then G−v is minimally rigid.(b)If d(v)=3then v is suitable.Proof:Part(a)follows easily from(2)and from the definition of minimally rigidgraphs.To prove(b)let N(v)={u,w,z}.It is easy to see that splitting offv on thepair uv,wv is not suitable if and only if there exists a critical set X⊂V withu,w∈X and v,z/∈X.Also observe that no critical set Z⊆V−v can satisfyd(v,Z)≥3,since otherwise E(G[Z∪{v}])is not independent in G,contradictingthe fact that G is minimally rigid.Thus if v is not suitable then there existmaximal critical sets X uw,X uz,X wz⊂V−v each containing precisely two neighbours ({u,w},{u,z},{wz},resp.)of v.By Lemma 2.3and the maximality of thesesets we must have|X uw∩X uz|=|X uw∩X wz|=|X uz∩X wz|=1.Thus,by Lemma2.7the set Y:=X uw∪X uz∪X wz is also critical.Since N(v)⊆Y,we have d(v,Y)≥3.This is impossible by our previous observation.Therefore v is suitable.•The minimally rigid graph K4−e shows that among the three possible splittings at a vertex of degree three there may be only one which is suitable.We now define the reverse operations of vertex deletion and vertex splitting usedin Lemma2.8.The operation0-extension adds a new vertex v and two edges vu,vwwith u=w.The operation1-extension subdivides an edge uw by a new vertex vand adds a new edge vz for some z=u,w.An extension is either a0-extension or a1-extension.The next lemma follows easily from(2).Lemma2.9.Let G be minimally rigid and let G be obtained from G by an extension.Then G is minimally rigid.Theorem2.10.Let G=(V,E)be minimally rigid and let G =(V ,E )be a mini-mally rigid subgraph of G.Then G can be obtained from G by a sequence of extensions. Proof:We shall prove that G can be obtained from G by a sequence of vertex splittings and deletions of vertices(of degree two).The theorem will then follow since these are the inverse operations of extensions.The proof is by induction on|V−V |.Since G is minimally rigid,it must be an induced subgraph of G.Thus the theorem holds trivially when|V−V |=0.Now suppose that Y=V−V =∅.Since G and G are minimally rigid,it is easy to see that|E−E |=2|Y|holds.Therefore,if|Y|=1,then we must have d(v)=2for the unique vertex v∈Y.Hence,by Lemma2.8(a),G−v is a minimally rigid subgraph of G which contains G and has|V(G−v)−V |<|V−V |,and the theorem follows by induction.Thus we may assume that|Y|≥2.Claim2.11.If|Y|≥2then v∈Y d(v)≤4|Y|−3.Proof:Since|V |≥2and|V−V |≥2,we can apply Lemma2.6(b)to deduce that d(Y)≥3.Since i(Y)+d(Y)=|E−E |=2|Y|,we obtainv∈Yd(v)=2i(Y)+d(Y)=4|Y|−d(Y)≤4|Y|−3.•It follows from Claim2.11(and from the fact that the minimum degree in G is at least two)that there is a vertex v∈Y with2≤d(v)≤3.Now Lemma2.8impliesthat either G−v or,for some edges vu,vw,G uwv is a minimally rigid proper subgraphof G which contains G .As above,the theorem follows by induction.•By choosing G to be an arbitrary edge of G we obtain the following constructive characterization of minimally rigid graphs(called the Henneberg or Henneberg-Laman construction,c.f.[10,11]).Corollary2.12.G=(V,E)is minimally rigid if and only if G can be obtained from K2by a sequence of extensions.Theorem2.13.Let G1=(V1,E1)and G2=(V2,E2)be two minimally rigid graphs with|V1∩V2|≥2.Then G1∪G2is rigid.Moreover,if G1∩G2is minimally rigid then G1∪G2is minimally rigid as well.Proof:Let F be a maximal independent set in M(G1∩G2).Let K t be the complete graph with vertex set V(G1∩G2)and F be a basis of M(K t)containing F .Let H be a minimally rigid spanning subgraph of G2+(F−F )which contains F.Such an H exists,since G2,and hence G2+(F−F ),is rigid.(To see that F and H exist we use the fact that any independent set in a matroid can be extended to a basis.) Now Theorem2.10implies that H can be obtained by a sequence of extensions from (V1∩V2,F).The same sequence of extensions,applied to G1,yields a minimally rigid spanning subgraph of G1∪G2by Lemma2.9.This proves that G1∪G2is rigid.2.2M-circuits and2-rigid graphs9 The second assertion follows from the fact that if G1∩G2is minimally rigid then F=F and H=G2.•Corollary2.14.Let G1=(V1,E1)and G2=(V2,E2)be two rigid graphs with|V1∩V2|≥2.Then G1∪G2is rigid.Let G=(V,E)be a graph.Since every edge of G induces a rigid subgraph of G,Corollary2.14implies that the maximal rigid subgraphs R1,R2,...,R t(called the rigid components of G)of G are pairwise edge-disjoint and E(R1),E(R2),...,E(R t) is a partition of E.Thus a graph is rigid if and only if it has precisely one rigid component.2.2M-circuits and2-rigid graphsGiven a graph G=(V,E),a subgraph H=(W,C)is said to be an M-circuit in G if C is a circuit(i.e.a minimal dependent set)in M(G).In particular,G is an M-circuit if E is a circuit in M(G).For example,K4,K3,3plus an edge,and K3,4 are all ing(2)we may deduce:Lemma2.15.Let G=(V,E)be a graph.The following statements are equivalent.(a)G is an M-circuit.(b)|E|=2|V|−2and G−e is minimally rigid for all e∈E.(c)|E|=2|V|−2and i(X)≤2|X|−3for all X⊆V with2≤|X|≤|V|−1.We shall need the following elementary properties of M-circuits which can be de-rived in a similar way to Lemma2.6.Lemma2.16.[1,Lemma2.4]Let H=(V,E)be an M-circuit.(a)For every∅=X⊂V we have d(X)≥3and if d(X)=3holds then either|X|=1 or|V−X|=1,(b)If X⊂V is critical with|X|≥3then H[X]is2-connected.Let H=(V,E)be a2-connected graph and suppose that(H1,H2)is a2-separationof G with V(H1)∩V(H2)={a,b}.For1≤i≤2,let Hi =H i+ab if ab∈E(H i)and otherwise put Hi =H i.We say that H1,H2are the cleavage graphs obtained bycleaving G along{a,b}.The inverse operation of cleaving is2-sum:given two graphs H1=(V1,E1)and H2=(V2,E2)with V1∩V2=∅and two designated edges u1v1∈E1 and u2v2∈E2,the2-sum of H1and H2(along the edge pair u1v1,u2v2),denoted by H1⊕2H2,is the graph obtained from H1−u1v1and H2−u2v2by identifying u1with u2and v1with v2.We shall use the following results on2-sums and2-separations.Lemma2.17.[1,Lemma4.1]Let G1=(V1,E1)and G2=(V2,E2)be M-circuits and let u1v1∈E1and u2v2∈E2.Then the2-sum G1⊕2G2along the edge pair u1v1, u2v2is an M-circuit.Lemma2.18.[1,Lemma4.2]Let G=(V,E)be an M-circuit and let G and G be the graphs obtained from G by cleaving G along a2-separator.Then G and G are both M-circuits.Recall that a graph G is2-rigid if G has at least two edges and G−e is rigid for all e∈E.M-circuits are examples of(minimally)2-rigid graphs.Note also that a graph G is2-rigid if and only if G is rigid and each edge of G belongs to a circuit in M(G)i.e.an M-circuit of G.It follows from Theorem2.13that any two maximal2-rigid subgraphs of a graph G=(V,E)can have at most one vertex in common,and hence are edge-disjoint. Defining a2-rigid component of G to be either a maximal2-rigid subgraph of G,or a subgraph induced by an edge which belongs to no M-circuit of G,we deduce that the2-rigid components of G partition E.Since each2-rigid component is rigid,this partition is a refinement of the partition of E given by the rigid components of G. We shall need two elementary lemmas on2-rigidity.Lemma2.19.If G is2-rigid and G is obtained from G by an edge addition or a 1-extension,then G is2-rigid.Proof:This follows from the definition of2-rigidity and the facts that edge additions, 0-extensions and1-extensions preserve rigidity.•Lemma2.20.If G is2-rigid and{u,v}is a2-separator in G then d(u),d(v)≥4. Proof:Suppose d(u)≤3.Then we can choose an edge e incident to u such that G−e is not2-connected.By Lemma2.6(a),G−e is not rigid.This contradicts the 2-rigidity of G.•3Graphs with a connected rigidity matroidGiven a matroid M=(E,I),we define a relation on E by saying that e,f∈E are related if e=f or if there is a circuit C in M with e,f∈C.It is well-known that this is an equivalence relation.The equivalence classes are called the components of M.If M has at least two elements and only one component then M is said to be connected.If M has components E1,E2,...,E t and M i is the matroid restriction of M onto E i then M=M1⊕M2...⊕M t is the direct sum of the M i’s.We say that a graph G=(V,E)is M-connected if M(G)is connected.For example, K3,m is M-connected for all m≥4.The M-components of G are the subgraphs of G induced by the components of M(G).Since the M-circuits of G are2-rigid, every M-circuit of G is contained in one of the2-rigid components of G.Thus the partition of E(G)given by the M-components is a refinement of the partition given by the2-rigid components and hence a further refinement of the partition given by the rigid components.Furthermore,M(G)can be expressed as the direct sum of the rigidity matroids of the rigid components of G,the2-rigid components of G,or the M-components of G.Lemma3.1.Suppose that G is M-connected.Then G is2-rigid.Proof:G is rigid,since otherwise G has at least two rigid components and hence at least two M-components.Since M(G)is connected,every edge e is contained in a circuit of M(G).Thus G is2-rigid.•The main result of this section characterizes which2-rigid graphs are M-connected. We say that a graph G is nearly3-connected if G can be made3-connected by adding at most one new edge.Theorem3.2.Suppose that G is nearly3-connected and2-rigid.Then G is M-connected.Proof:For a contradiction suppose that G is not M-connected and let H1,H2,...,H q be the M-components of G.Let S i=V(H i)−∪j=i V(H j)denote the set of vertices belonging to no other M-component than H i,and let P i=V(H i)−S i for1≤i≤q. Let n i=|V(H i)|,s i=|S i|,p i=|P i|.Clearly,n i=s i+p i and|V|= q i=1s i+|∪q i=1P i|. Moreover,we have q i=1p i≥2|∪q i=1P i|.Since every edge of G is in some M-circuit, and every M-circuit has at least four vertices,we have that n i≥4for1≤i≤q.Furthermore,since G is nearly3-connected,p i≥2for all1≤i≤q,and p i≥3for all but at most two M-components.Let us choose a basis B i in each rigidity matroid M(H i).Using the above inequal-ities we have|∪q i=1B i|=qi=1|B i|=qi=1(2n i−3)=2qi=1n i−3q≥(2qi=1s i+qi=1p i)+qi=1p i−3q≥2|V|+3q−2−3q=2|V|−2.Since r(M(G))=2|V|−3,this implies that∪qi=1B i contains a circuit,contradictingthe fact that the B i’s are bases for the M(H i)’s and M(G)=⊕qi=1M(H i).•A graph G is birigid if G−v is rigid for all v∈V(G).It was shown by Servatius [15,Theorem2.2](using a similar argument to our proof of Theorem3.2)that every birigid graph is M-connected.Theorem3.2extends this result,since birigid graphs are clearly3-connected and2-rigid.The wheels(on at least5vertices)are3-connected 2-rigid graphs which are not birigid.This shows that the extension is proper.We need the following results to complete our characterization of M-connected graphs.Thefirst two lemmas follow from Lemmas2.17and2.18,respectively. Lemma3.3.Suppose G1and G2are M-connected.Then G1⊕2G2is M-connected. Lemma3.4.Suppose G1and G2are obtained from G by cleaving G along a2-separator.If G is M-connected then G1and G2are also M-connected.Let G=(V,E)be a2-connected graph,c≥3be an integer,and let(X1,X2,...,X c) be cyclically ordered subsets of V satisfying(by taking X c+1=X1):(i)|X i∩X j|=1,for|i−j|=1,and X i∩X j=∅for|i−j|≥2,and(ii){E(X1),E(X2),...,E(X c)}is a partition of E.Then we say that(X1,X2,...,X c)is a polygon(of size c)in G.It is easy to see that if u and v are distinct vertices with{u}=X i−1∩X i and{v}=X j∩X j+1,for some 1≤i,j≤c,then either{u,v}is a2-separator in G or i=j and X i={u,v}.Lemma3.5.Suppose that G=(V,E)has a polygon of size c.Then(a)G is not M-connected.(b)If c≥4then G is not rigid.Proof:Let X1,X2,...,X c be a polygon and let E i=E(X i)for1≤i≤c.Note that E1,E2,...,E c is a partition of ing the polygon structure we obtainr(E)≤ci=1r(E i)≤ci=1(2|X i|−3)=2|V|+2c−3c=2|V|−c.(5)Thus for c≥4we have r(E)≤2|V|−4,and hence G is not rigid.This proves(b). To prove(a)suppose that G is M-connected.Then G is rigid and r(E)=2|V|−3. By(b)this yields c=3.Moreover,equality must hold everywhere in(5).Thus r(E)= c i=1r(E i).Thus M(G)is the direct sum of its restrictions to the sets E i. This contradicts the fact that M(G)is a connected matroid.•We say that a2-separator{x1,x2}crosses another2-separator{y1,y2}in a graph G,if x1and x2are in different components of G−{y1,y2}.It is easy to see that if {x1,x2}crosses{y1,y2}then{y1,y2}crosses{x1,x2}.Thus,we can say that these 2-separators are crossing.It is also easy to see that crossing2-separators induce a polygon of size four in G.Thus Lemma3.5(a)has the following corollary:Lemma3.6.Suppose that G is rigid(and hence2-connected).Then there are no crossing2-separators in G.Let G=(V,E)be a2-connected graph with no crossing2-separators.The cleav-age units of G are the graphs obtained by recursively cleaving G along each of its 2-separators.Since G has no crossing2-separators this sequence of operations is uniquely defined and results in a unique set of graphs each of which have no2-separators.Thus each cleavage unit of G is either3-connected or else a complete graph on three vertices.The stronger hypothesis that G has no polygons will imply that each cleavage unit of G is a3-connected graph.In this case,an equivalent def-inition for the cleavage units is tofirst construct the augmented graphˆG from G by adding all edges uv for which{u,v}is a2-separator of G and uv∈E,and then take the cleavage units to be the maximal3-connected subgraphs ofˆG.(These definitions are a special case of a general decomposition theory for2-connected graphs due to Tutte[17].)。