The Equivalence between Model Predictive Control and Anti-Windup Control Schemes
《翻译研究入门理论与应用》总结笔记
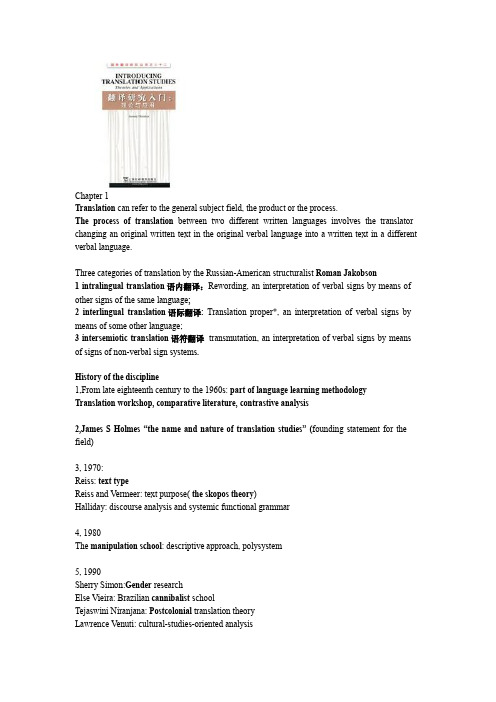
Chapter1Translation can refer to the general subject field,the product or the process.The process of translation between two different written languages involves the translator changing an original written text in the original verbal language into a written text in a different verbal language.Three categories of translation by the Russian-American structuralist Roman Jakobson1intralingual translation语内翻译:Rewording,an interpretation of verbal signs by means of other signs of the same language;2interlingual translation语际翻译:Translation proper*,an interpretation of verbal signs by means of some other language;3intersemiotic translation语符翻译transmutation,an interpretation of verbal signs by means of signs of non-verbal sign systems.History of the discipline1,From late eighteenth century to the1960s:part of language learning methodology Translation workshop,comparative literature,contrastive analysis2,James S Holmes“the name and nature of translation studies”(founding statement for the field)3,1970:Reiss:text typeReiss and Vermeer:text purpose(the skopos theory)Halliday:discourse analysis and systemic functional grammar4,1980The manipulation school:descriptive approach,polysystem5,1990Sherry Simon:Gender researchElse Vieira:Brazilian cannibalist schoolTejaswini Niranjana:Postcolonial translation theoryLawrence Venuti:cultural-studies-oriented analysisHolmes’s map of translation studiesThe objectives of the pure areas of research:1,descriptive translation theory:the description of the phenomena of translation2,translation theory:the establishment of general principles to explain and predict such phenomenaPure:theoretical and descriptiveDTS:descriptive translation studies1,product-oriented DTS:existing translations,text(diachronic or synchronic)2,function-oriented DTS:the function of translations in the recipient sociocultural situation (socio-translation studies or cultural-studies-oriented translation)3,process-oriented DTS:the psychology of translation(later think-aloud protocols)Relation between Theoretical and descriptiveThe results of DTS research can be fed into the theoretical branch to evolve either a general theory of translation or,more likely,partial theories of translation.Partial theories1,Medium-restricted theories:translation by machine and humans2,Area-restricted theories:3,Rank-restricted theories:the level of word,sentence or text4,Text-type restricted theories:discourse types or genres5,Time-restricted theories:6,Problem-restricted theories:Applied branch of Holmes’s framework:translator training,translation aids and translation criticism.Translation policy:the translation scholar advising on the place of translation in societyChapter2translation theory before the twentieth centuryLiteral vs.free debateCicero(first century BCE):I did not hold it necessary to render word for word,but I preserved the general style and force of the language.Horace:producing an aesthetically pleasing and creative text in the TL.St Jerome:I render not word for word,but sense for sense.Martin Luther:1,non-literal or non-accepted translation came to be seen and used as a weapon against the Church.2,his infusion of the Bible with the language of ordinary people and his consideration of translation in terms focusing on the TL and the TT reader were crucial.“Louis Kelly:Fidelity: to both the words and the perceived senseSpirit:1, creative energy or inspiration of a text or language, proper to literature; 2, the Holy Spirit.Truth: content17 century:Early attempts at systematic translation theoryCowley: imitationCounter the inevitable loss of beauty in translation by using our wit or invention to create new beauty;he has ‘taken, left out and added what I please’John Dryden reduces all translation to three categories: the triadic model(约翰 德莱顿的三分法:“直译”、意译”与“仿译”) 1, metaphrase: word for word translation2, paraphrase : sense for sense translation3, imitation : forsake both words and senseEtienne Dolet: a French humanist, burned at the stake for his addition to his translation of one of Plato’s dialogues.Five principles:① The translator must perfectly understand the sense and material of the original author,although he should feel free to clarify obscurities.②The translator should have a perfect knowledge of both SL and TL , so as not to lessen the majesty of the language.③The translator should avoid word-for-word renderings.④The translator should avoid Latinate and unusual forma .⑤The translator should assemble and liaise words eloquently to avoid clumsiness.Alexander Fraser TytlerTL-reader-oriented definition of a good translation: That, in which the merit of the original work is so completely transfused into another language, as to be as distinctly apprehended, and as strongly felt, by a native of the country to which that language belongs, as it is by those who speak the language of the original work.Three general rules:I. That the Translation should give a complete transcript of the ideas of the original work.II. That the style and manner of writing should be of t he same character with that of the original.III. That the Translation should have all the ease of original composition.—— A. F. Tytler: Essay on the Principles of TranslationTytler ranks his three laws in order of comparative importance:Ease of composition would be sacrificed if necessary for manner,and a departure would be made from manner in the interests of sense.Friedrich Schleiermacher:the founder of modern Protestant theology and of modern hermeneuticsHermeneutics:a Romantic approach to interpretation based not on absolute truth but on the individual’s inner feeling and understanding.2types of translators:1,Dolmetscher:who translates commercial texts;2,Ubersetzer:who works on scholarly and artistic texts.2translation methods:1,translator leaves the reader in peace,as much as possible,and moves the author towards him. Alienating method2,translator leaves the writer alone,as much as possible,and moves the reader towards the writer. Naturalizing methodThe status of the ST and the form of the TLFrancis Newman:emphasize the foreignness of the workMatthew Arnold:a transparent translation method(led to the devaluation of translation and marginalization of translation)Chapter3Equivalence and equivalent effectRoman Jakobson:the nature of linguistic meaningSaussure:the signifier(能指)the spoken and written signalThe signified(所指)the concept signifiedThe signifier and signified form the linguistic sign,but that sign is arbitrary or unmotivated.1,There is ordinarily no full equivalence between code-units.Interlingual translation involves substituting messages in one language not for separate code-units but for entire messages in some other language.2,for the message to be equivalent in ST and TT,the code-unit will be different since they belong to two different sign systems which partition reality differently.3,the problem of meaning and equivalence thus focuses on differences in the structure and terminology of languages rather than on any inability of one language to render a message that has been written in another verbal language.4,cross-linguistic differences center around obligatory grammatical and lexical forms.They occur at the level of gender,aspect and semantic fields.Eugene Nida1,an orthographic word has a fixed meaning and towards a functional definition of meaning in which a word acquires meaning through its context and can produce varying responses accordingto culture.2,meaning is broke down into a,linguistic meaning,b,referential meaning(the denotative ‘dictionary’meaning指称,字面)and c,emotive meaning(connotative隐含).3,techniques to determine the meaning of different linguistic itemsA,analyze the structure of wordsB,differentiate similar words in relaxed lexical fields3techniques to determine the meaning of different linguistic items1,Hierarchical structuring,differentiates series of words according to their level,2,Techniques of componential analysis(成分分析法)identify and discriminate specific features of a range of related words.3,Semantic structure analysis:Discriminate the sense of a complex semantic termChomsky:Generative-transformational model:analyze sentences into a series of related levels governed by rules.3features1,phrase-structure rules短语结构规则generate an underlying or deep structure which is2,transformed by transformational rules转换规则relating one underlying structure to another, to produce3,a final surface structure,which itself is subject to形态音位规则phonological and morphemic rules.The most basic of such structures are kernel sentences,which are simple,active,declarative sentences that require the minimum of transformation.Three-stage system of translationAnalysis:the surface structure of the ST is analyzed into the basic elements of the deep structure Transfer:these are transferred in the translation processRestructuring:these are transferred in the translation process and then restructured semantically and stylistically into the surface structure of the TT.Back-transformation回归转换(Kernels are to be obtained from the ST structure by a reductive process)Four types of functional class:events,objects,abstracts and relationals.Kernels are the level at which the message is transferred into the receptor language before being transformed into the surface structure in three stages:literal transfer,minimal transfer最小单位转换and literary transfer.Formal equivalence:focuses attention on the message itself,in both form and content,the message in the receptor language should match as closely as possible the different elements in the source language.Gloss translations释译Dynamic equivalence is based on what Nida calls the principle of equivalent effect,where the relationship between receptor and message should be substantially the same as that which existed between the original receptors and the message.Four basic requirements of a translation1,making sense2,conveying the spirit and manner of the original3,having a natural and easy form of expression4,producing a similar response.NewmarkCommunicative translation attempts to produce on its reader an effect as close as possible to that obtained on the readers of the original.Semantic translation attempts to render,as closely as the semantic and syntactic structures of the second language allow,the exact contextual meaning of the original.Literal translation is held to be the best approach in both communicative translation and semantic translation.One of the difficulties encountered by translation studies in systematically following up advances in theory may indeed be partly attributable to the overabundance of terminology.Werner KollerCorrespondence:contrastive linguistics,compares two language systems and describes contrastively differences and similarities.Saussure’s langue(competence in foreign language) Equivalence:equivalent items in specific ST-TT pairs and contexts.Saussure’s parole (competence in translation)Five types of equivalenceDenotative equivalenceConnotative equivalenceText-normative equivalencePragmatic equivalence(communicative equivalence)Formal equivalence(expressive equivalence,the form and aesthetics of the text)A checklist for translationally relevant text analysis:Language functionContent characteristicsLanguage-stylistic characteristicsFormal-aesthetic characteristicsPragmatic characteristicsTertium comparationi in the comparison of an ST and a TTChapter5functional theories of translationKatharina Reiss:Text TypeBuilds on the concept of equivalence but views the text,rather than the word or sentence as the level at which communication is achieved and at which equivalence must be sought.Four-way categorization of the functions of language(Karl Buhler,three)1,plain communication of facts,transmit information and content,informative text2,creative composition,expressive text3,inducing behavioral responses,operative text4,audiomedial text,supplement the other three functions with visual images,music,etc.Different translation methods for different texts1,transmit the full referentical or conceptual content of the ST in plain prose without redundancy and with the use of explicitation when required.2,transmit the aesthetic and artistic form of the ST,using the identifying method,with the translator adopting the standpoint of the ST author.3,produce the desired response in the TT receiver,employing the adaptive method,creating an equivalent effect among TT readers.4,supplementing written words with visual images and music.Intralinguistic and extralinguistic instruction criteria1,intralinguistic criteria:semantic,lexical,grammatical and stylistic features2,extralinguistic criteria:situation,subject field,time,place,receiver,sender and affective implications(humor,irony,emotion,etc.)Holz-Manttari:Translational actionTakes up concepts from communication theory and action theoryTranslation action views translation as purpose-driven,outcome oriented human interaction and focuses on the process of translation as message-transmitter compounds involving intercultural transfer.Interlingual translation is described as translational action from a source text and as a communicative process involving a series of roles and players.The initiatorThe commissionerThe ST producerThe TT producerThe TT userThe TT receiverContent,structured by what are called tectonics,is divided into a)factual information and b) overall communicative strategy.Form,structured by texture,is divided into a)terminology and b)cohesive elements.Value:place of translation,at least the professional non-literary translation within its sociocultural context,including the interplay between the translator and the initiating institution.Vermeer:Skopos theorySkopos theory focuses above all on the purpose of the translation,which determines the translation methods and strategies that are to be employed in order to produce a functionally adequate result(TT,translatum).Basic rules of the theory:1,a translatum is determined by its skopos;2,a TT is an offer of information in a target culture and TL concerning an offer of information in a source culture and SL.3,a TT does not initiate an offer of information in a clearly reversible way4a TT must be internally coherent5a TT must be coherent with the ST6the five rules above stand in hierarchical order,with the skopos rule predominating.The coherence rule,internally coherent,the TT must be interpretable as coherent with the TT receiver’s situation.The fidelity rule,coherent with the ST,there must be coherence between the translatum and the ST.1,the ST information received by the translator;2,the interpretation the translator makes of this information;3,the information that is encoded for the TT receivers.Intratextual coherence intertextual coherenceAdequacy comes to override equivalence as the measure of the translational action. Adequacy:the relations between ST and TT as a consequence of observing a skopos during the translation process.In other words,if the TT fulfills the skopos outlined by the commission,it is functionally and communicatively adequate.Criticisms:1,valid for non-literary texts2,Reiss’s text type approach and Vermeer’s skopos theory are considering different functional phenomena3,insufficient attention to the linguistic nature of the ST nor to the reproduction of microlevel features in the TT.Christiane Nord:translation-oriented text analysisExamine text organization at or above sentence level.2basic types of translation product:1,documentary translation:serves as a document of a source culture communication between the author and the ST recipient.2,instrumental translation:the TT receiver read the TT as though it were an ST written in their own language.Aim:provide a model of ST analysis which is applicable to all text types and translation situations.Three aspects of functionalist approaches that are particularly useful in translator training1,the importance of the translation commission(translation brief)2,the role of ST analysis3,the functional hierarchy of translation problems.1,compare ST and TT profiles defined in the commission to see where the two texts may diverge Translation brief should include:The intended text functions;The addressees(sender and recipient)The time and place of text receptionThe medium(speech and writing)The motive(why the ST was written and why it is being translated)2,intratextual factors for the ST analysisSubject matterContent:including connotation and cohesionPresuppositions:real-world factors of the communicative situation presumed to be known to the participants;Composition:microstructure and macrostructure;Non-verbal elements:illustrations,italics,etc.;Lexic:including dialect,register and specific terminology;Sentence structure;Suprasegemtal features:stress,rhythm and stylistic punctuationIt does not matter which text-linguistic model is used3,the intended function of the translation should be decided(documentary or instrumental) Those functional elements that will need to be adapted to the TT addressee’s situation have to be determinedThe translation type decides the translation style(source-culture or target culture oriented)The problems of the text can then be tackled at a lower linguistic levelChapter6discourse and register analysis approachesText analysis:concentrate on describing the way in which texts are organized(sentence structure,cohesion,etc.)Discourse analysis looks at the way language communicates meaning and social and power relations.Halliday’s model of discourse analysis,based on systemic functional grammarStudy of language as communication,seeing meaning in the writer’s linguistic choices and systematically relating these choices to a wider sociocultural framework.Relation of genre and register to languageGenre:the conventional text type that is associated with a specific communicative function Variables of Register:1,field:what is being written about,e.g.a delivery2,tenor:who is communicating and to whom,e.g.a sales representative to a customer3,mode:the form of communication,e.g.written.Each is associated with a strand of meaning:Metafunctions:概念功能(ideational function)、人际功能(interpersonal function)和语篇功能(textual function)Realized by the lexicogrammar:the choices of wording and syntactic structureField--ideational meaning—transitivity patternsTenor—interpersonal meaning—patterns of modalityMode—textual meaning—thematic and information structures and cohesion及物性系统(transitivity)情态系统(modality)、主位结构(theme structure)和信息结构(information structure)。
Evaluation of Recommendations__Rating-Prediction and Ranking

Evaluation of Recommendations: Rating-Prediction and RankingHarald Steck∗Netflix Inc.Los Gatos,Californiahsteck@netflABSTRACTThe literature on recommender systems distinguishes typi-cally between two broad categories of measuring recommen-dation accuracy:rating prediction,often quantified in terms of the root mean square error(RMSE),and ranking,mea-sured in terms of metrics like precision and recall,among others.In this paper,we examine both approaches in de-tail,andfind that the dominating difference lies instead in the training and test data considered:rating prediction is concerned with only the observed ratings,while ranking typi-cally accounts for all items in the collection,whether the user has rated them or not.Furthermore,we show that predict-ing observed ratings,while popular in the literature,only solves a(small)part of the rating prediction task for any item in the collection,which is a common real-world prob-lem.The reasons are selection bias in the data,combined with data sparsity.We show that the latter rating-prediction task involves the prediction task’Who rated What’as a sub-problem,which can be cast as a classification or ranking problem.This suggests that solving the ranking problem is not only valuable by itself,but also for predicting the rating value of any item.Categories and Subject DescriptorsH.2.8[Database Management]:Database Applications—Data MiningKeywordsRecommender Systems;Selection Bias;Rating Prediction; Ranking1.INTRODUCTIONThe idea of recommender systems(RS)is to automatically suggest items to each user that s/he mayfind appealing,e.g.,∗Part of this work was done while at Bell-Labs,Alcatel-Lucent,Murray Hill,New Jersey.Permission to make digital or hard copies of part or all of this work for personal or classroom use is granted without fee provided that copies are not made or distributed for profit or commercial advantage,and that copies bear this notice and the full ci-tation on thefirst page.Copyrights for third-party components of this work must be honored.For all other uses,contact the owner/author(s).Copyright is held by the author/owner(s).RecSys’13,October12–16,2013,Hong Kong,China.ACM978-1-4503-2409-0/13/10./10.1145/2507157.2507160.see[2]for an overview.Prior to a user study or deployment, offline testing on historical data provides a time and cost efficient initial assessment of an RS.A meaningful offline test ideally provides a good approximation to the utility function to be optimized by the deployed system(e.g.,user satisfaction,increase in sales).Recommendation accuracy is an important part of such an offline metric.The literature on recommender systems typically distin-guishes between two ways of measuring recommendation ac-curacy:rating prediction,often measured in terms of the root mean square error(RMSE);and ranking,which is mea-sured in terms of metrics like precision and recall,among others.In this paper,we examine both kinds of accuracy measures in detail and identify different variants. Concerning rating prediction,the literature has focused mainly on predicting the rating values for those items that a user has deliberately chosen to rate.This kind of data can be collected easily,and is hence readily available for offline training and testing of recommender systems.Moreover,the root mean square error(RMSE),the most popular accuracy metric in the recommender literature,can easily be evalu-ated on the user-item pairs that actually have a rating value in the data.The objective of common real-world rating prediction tasks, however,is often different from this scenario:typically,the goal is to predict the rating value for any item in the collec-tion,independent of the fact if a user rates it or not.The reason for the difference is that the ratings are missing not at random(MNAR)in the data sets typically collected[14,13, 22,23,17].For instance,on the Netflix web site,a personal-ized rating value is predicted for any video in the collection, as to provide the user with an indication of enjoyment if s/he watches it.These two variants of rating prediction motivated us to examine it in more detail.In thefirst part of this paper,we identify three variants of rating prediction,and show how they differ from each other in terms of answers they provide to the recommendation problem and in terms of the degree of difficulty to solve them.Moreover,we show that many real-world rating prediction tasks require an additional sub-problem to be solved:as to which user deliberately chooses to assign a rating to which item in the collection(also known as’Who rated What’[1]).This sub-problem may be cast as a classification or ranking problem.In the second part of this paper,we focus on ranking for solving real-world recommendation tasks.As just mo-tivated,ranking may not only be an important sub-problem of many real-world rating-prediction tasks,but ranking is arelevant approach on its own–for instance,when the objec-tive is to select a small subset of items from the entire collec-tion(top N recommendations).We examine three variants of ranking:ranking of all items in the collection,ranking of only those items that the user has not rated yet,and ranking of only those items that the user has already rated. Our experiments provide strong empirical evidence that the difference in ranking accuracy due to these variants is con-siderably larger than the difference due to different ranking metrics.This suggests that the appropriate choice of train-ing and testing protocol,e.g.,which user-item pairs to con-sider,may be more important than the ranking metric for solving a given real-world ranking problem.The main contributions of this paper can be summarized as follows:1.we identify three variants of rating prediction.Weshow that the variant that is the main focus in the literature solves only a part of many real-world rating-prediction problems.This suggests further research is needed to develop rating prediction approaches for the (various)real-world tasks.2.concerning ranking,wefind that in practice the choiceof an appropriate training and testing protocol makesa bigger difference than the choice of ranking metric.3.when comparing rating prediction with ranking,wefind that their main difference is not caused by the different metrics(e.g.,RMSE vs.ranking metrics).Instead,it is due to the user-item pairs that are taken into account:only the subset of user-item pairs wherea rating is observed in the data(e.g.,for RMSE),vs.all user-item pairs–whether the user deliberately chose to assign a rating value to an item or not.As main causes,we identify selection bias in the data combined with data sparsity.This paper is organized into two main parts:first,we examine rating prediction,which we model as a two-stage problem:the user’s deliberate choice as to which item to rate,and which rating value to assign(Section2.1).This leads immediately to three variants of rating prediction(Sec-tion2.2),and we derive their general relationships and dif-ferences in Section2.3.In Section2.4,we discuss general implications on modeling the two-stage rating process,while Section2.5justifies a particular model approach.In the sec-ond part of this paper(Section3),we outline three variants of ranking protocols(Section3.1),which is analogous to the variants for rating prediction.In Section3.2,we briefly review various ranking metrics,so that we are ready to com-pare the effects of ranking protocols and ranking metrics in our experiments in Section3.3.Wefinish with our Conclu-sions.2.RATING PREDICTIONThe root mean square error(RMSE)is by far the most popular accuracy measure in the recommender literature.It is commonly associated with the objective of rating predic-tion,i.e.,predicting the rating value that a user would assign to an item which s/he has not rated yet.In this section,we examine this objective in detail,and identify three variants with important differences.2.1Modeling the Decision to RateThere has been recent work on the fact that ratings are missing not at random(MNAR)in the data typically col-lected in recommender system applications[14,13,22,23, 17].The main reason for this selection bias in the observed data is that the users are free to deliberately choose which items to rate.This motivates us to model the rating-prediction task as shown in Figure1(left):In this graphical model,U denotes the random variable concerning users,and takes values u, where u∈{1,...,n}denotes user IDs,and n is the num-ber of users.Similarly,I is the random variable concerning items,taking values i∈{1,...,m},where m is the number of items in the collection.The decision if a user u deliberately chooses to rate item i is represented by the random variableC,which takes values c∈{c+,c−},where c+denotes that the user chooses to rate the item,while c−means that the user does not deliberately choose to rate the item.Finally,R is the random variable regarding rating values,taking val-ues r.For instance,r∈{1,...,5}in case of ratings on a scale from1to5stars like in the Netflix Prize competition[3]or MovieLens data[5].Note that C depends on the user and the item,such that this model is able to capture any reason that depends on u and i for choosing to give a rating(e.g.,the item’s popu-larity[23,17]).This is more general than the approaches presented in[13],where this decision does not depend on the user-item pair directly,but only on the rating value itself. The dependence on the rating value as a decision-criteria whether to provide a rating is included as a special case in the model in Figure1:using theory on graphical models,the graph in Figure1is Markov-equivalent to the graph where the orientation of the edge between C and R is reversed. Markov-equivalence means that both graphical models rep-resent the same probability distribution(even though the graphs look different).The reason why the edge can be re-versed is that both nodes C and R in the graph have the same parents,namely U and I.This is a key difference to the graphs used in[13],where C is not connected to the user or item.Given that all random variables U,I,C,and R are dis-crete,we assume the most general probability distribution, the multinomial distribution.Note that this may be too general a model assumption as to learn a“good”model in practice,but in thefirst part of this paper,we are concerned with general insights that we can obtain without resorting to a more specific model.2.2Rating-Prediction VariantsRating prediction is concerned with predicting the rating value r that a user u assigns to an item i.It can be cast as predicting the probability of each rating value r that user u assigns to each item i,denoted by p(r u,i)=p(r|u,i).Based on the graphical model in Figure1,this can be decomposed as follows:p(r|u,i)(1)=p(r|c+,u,i)(2)p(c+|u,i)+p(r|c−,u,i)(3)p(c−|u,i)(1) This equation shows that there are three variants of rating prediction,i.e.,three probabilities concerning rating value r. Additionally,Eq.1also shows that these terms are linked via two terms regarding the probability that the user de-marginalization:Figure 1:When a user (node U )provides a rating value (node R )for an item (node I ),the user typ-ically has the freedom to deliberately choose which item(s)to rate.The user’s implicit decision as to whether rate an item or not,is represented by node C in the graphical model.The graph on the right hand side is obtained by marginalizing out U and I .liberately chooses to rate an item (’Who rated What’[1]),which is discussed at the end of this sub-section.We first discuss the three variants of rating prediction:1.p (r |u,i ):This is the probability that user u assigns rat-ing value r to item i in the collection.It is important that there is no restriction on which item i it is;it can be any item in the collection.The ability to make an accurate rating prediction for any item i is extremely useful in practical applications,as it allows one to find the items with the highest (predicted)enjoyment value from the entire collection .2.p (r |c +,u,i ):This is the probability that user u assigns rating value r to item i under the condition that the user deliberately chooses to rate this item.This con-dition may not hold for any item i in the collection.In fact,due to data sparsity,it may apply to only a (small)subset of items ,as shown in the next section.For instance,it is commonly observed in practice that users tend to assign ratings mainly to items they like or know [14,13,22,23,17].The reason is that the observed data is collected by allowing users to choose which items to rate.As a consequence,this conditional probability is learned when optimizing RMSE or MAE on the observed data.3.p (r |c −,u,i ):This is the probability that user u would assign rating value r to item i under the condition that the user would not deliberately choose to rate this item.In practice,this rating value could be elicited,for instance,by providing the user with some reward for completing this task.These ratings are typically costly to elicit,and burdensome for the user to provide.For this reason,ratings under this condition are typically not available,which obviously makes it difficult to es-timate this probability distribution accurately.Due to data sparsity,this distribution applies to the vast ma-jority of user-item pairs,as discussed in more detail in the next section.In the rare case that they were collected,for instance in the Yahoo!Music data [13],it becomes obvious that this conditional distribution of rating values can be very different from the rating distribution under the condition that the user delib-erately chose an item to rate.For instance,compare Figures 2(a)and (b)in [13],or Figure 1in [17].The decomposition in Eq.1also shows that,in addition,the two conditional probabilities p (r |c +,u,i )and p (r |c −,u,i )have to be estimated as to obtain valid rating predictions for any item in the collection.These probabilities capture whether user u deliberately chooses to rate item i or not.This shows their importance for rating prediction of any item,and motivates further research beyond the few works conducted so far,like the KDD Cup 2007,titled ’Who rated What’[1];there,one of the insights was that it was difficult to make accurate predictions as to which user deliberately chooses to rate which items.2.3Relationship of the three VariantsThis section presents our main results concerning rating prediction,i.e.,a general relationship between the three vari-ants of rating prediction.2.3.1Marginal ProbabilitiesA necessary condition for accurate personalized rating pre-diction is that the average (and hence unpersonalized)rating predictions have to be accurate on average as well.This is outlined in detail in the following.To this end,let us con-sider p (r ),which is the probability of rating value r averaged over all users u and items i .Based on our graphical model in Figure 1,this can be derived by marginalizing over u and i in Eq.1:p (r )=c,u,ip (r |c,u,i )·p (c |u,i )·p (u )·p (i )(2)=cp (r |c )·p (c )(3)=p (r |c +)·p (c +)+p (r |c −)·p (c −)(4)=p (r |c −)+p (c +){p (r |c +)−p (r |c −)}(5)≈p (r |c −)(6)The line-by-line comments are as follows:equality (2)in the first line follows from the definition of p (r )in terms of the graphical model in Figure 1.In equality (3),the random variables U and I are marginalized out from the probability distribution,so that we obtain the marginal dis-tribution over C and R ,which is also represented in Figure 1(right).This is valid [11]because the class of graphi-cal models representing probability distributions from the exponential family,like the multinomial distribution used here,is closed when marginalizing out over a variable that is connected with all its edges to the same clique 1in the graph,which is obviously the case in Figure 1.Equality (4)re-states the previous line,using c ∈{c +,c −},and equality (5)uses p (c −)=1−p (c +).The interesting approximation in the last line is accurate due the sparsity of the data ,which is typical for recommender applications:based on the graph,we havep (c +)=u,ip (c +|u,i )·p (u )·p (i ).(7)Any accurate prediction for p (c +)has to be close to the empirical estimate ˆp (c +),which is given byˆp (c +)=#ratings|U |·|I |=data sparsity1A clique is a completely connected sub-graph.where#ratings is the number of ratings in the data; |U|=n and|I|=m denote the number of users and items, respectively.Hence,p(c+)equals the data sparsity.In typ-ical applications the data sparsity is in the single-digit per-cent range,and often even one or more orders of magnitudes lower.For instance,it was about1%in the Netflix Prize data[3],which may be considered a relatively dense data set compared to other recommender applications.Hence, the approximation in Eq.6is accurate up to a few percent in general.This is orders of magnitudes more accurate than any real-world rating-prediction accuracy,e.g.,see RMSE for the Netflix Prize data[3]or MovieLens data[5].We hence arrive atConclusion1:Due to data sparsity,the rating prediction variants1.and3.,as outlined in Section2.2,have to be closely related.In contrast,variant2.is not required to be similar to variants1.or3.This suggests that there is a difference between the main focus of the literature(variant 2.),and many real-world rating-prediction problems(variants1.and3.).2.3.2Average RatingsThe average of the predicted rating values of a recom-mender system has an important impact on its accuracy in terms of RMSE(and similarly for MAE).This is outlined in the following.Analogous to Eq.(2)-(6),one obtains for the average rating value:E[R]=r,c,u,ir·p(r|c,u,i)·p(c|u,i)·p(u)·p(i)(8) =E[R|c−]+p(c+){E[R|c+]−E[R|c−]}(9)≈E[R|c−](10) where E[·]denotes the(conditional)expectation/average of the random variable R,i.e.,the rating values.Not sur-prisingly,this confirms the previous relationships among the three variants of rating prediction:the average rating value of variants1.and3.has to be approximately the same due to data sparsity,while the average rating of variant2.is not required to be similar.In fact,there is strong empirical evidence,for instance, provided by the Yahoo!Music data2[13],suggesting that these average rating values can actually be very different: Figures2(a)and(b)in[13]show that,on a rating scale from1to5,we have:•E[R|c−]≈E[R]≈1.8:this is the average rating when users where asked to rate songs that were randomly selected by Yahoo!(instead of selected by the users).These rating data may hence be considered as(approx-imately)fulfilling the missing at random condition[18, 12],such that its rating distribution provides an unbi-ased estimate of the(unknown)true distribution.•E[R|c+]≈2.9:this is the average rating when users were free to deliberately choose which items to rate.Evidently,this value is significantly larger than1.8, suggesting a strong selection bias.2The actual Yahoo!Music data set was not available to us, so that we had to resort to the histograms published in[13].This difference in the average rating is extremely large–when compared to typical improvements in terms of RMSE, as outlined in the following.In the Netflix Prize data,the winning approach achieved RMSE≈0.86,which was so dif-ficult to achieve that it required about three years of research work.In comparison,when simply predicting the average rating for all users and all items,one achieves RMSE≈1.0. This shows that an improvement in RMSE of0.14(on a rat-ing scale of1to5)may look small,but is actually very large. The numbers for MovieLens data are slightly different,but also here,the improvement of a sophisticated approach over a simple approach in terms of RMSE is much less than1. This shows that a difference in the average predicted rat-ing of about1can easily dominate over the improvement of a more accurate recommender system.This becomes clear from the following thought experiment:Let us assume we train a recommender system on available rating data where the users were free to choose which items to rate.This is the typical scenario for collecting data.Let its RMSE(on a test set)be given by RMSE0;its average predicted rating value will be close to the average rating value in the training data, E[R|c+].This recommender system will hence be accurate for variant2.Now,let us consider the common real-world task of rating-prediction for any/each item in the collection (variant1):in this case,the(unknown)true average rating is E[R];now,the previously trained recommender system has a bias b=E[R|c+]−E[R].This results in a degraded RMSE:RMSE1=RMSE2+ing the numerical values from above,this suggests that RMSE1 1.Inter-estingly,this is worse than RMSE≈1,which can be achieved by an(unpersonalized)recommender system that predicts the average rating E[R].Even though the value E[R]may be unknown in many practical applications,it may also be possible to determine its value with some additional efforts, for instance,by running a truly randomized experiment with a small subset of the users.This leads us to the following conclusion:Conclusion2:A recommender system with a low RMSE concerning the rating-prediction variant2,is not guaranteed to achieve a low RMSE regarding rating-prediction tasks1 or3.Among these three variants,variant1refers to a common real-world rating-prediction task,like e.g.,on the Netflix web site,which provides a personalized rating prediction for any video on its website.While many excellent solutions have been developed for variant2in the literature,this conclusion suggests that additional research is needed as to develop accurate prediction models for variant1.2.4Implications for ModelingConclusions1and2above show that a key challenge in building real-world recommender systems is that rating distributions p(R|U,I)(variant1)or p(R|c−,U,I)(variant 3)are relevant for the user experience in many real-world applications;in contrast,the data that are readily avail-able in large quantities follow the distribution p(R|c+,U,I) (i.e.,variant2).Given the practical importance of variants 1and3,it is crucial to build recommender systems that account for the items(and their ratings)that a user has not rated.Developing solutions for these new objectives of rating-prediction is an interesting area for future work.In the following,we outline a Bayesian approach that uses an informative prior distribution that incorporates the rat-ing distributions of the items that were not rated by a user.There are different ways of defining this prior distribution.First,one may run an experiment and elicit ratings for ran-dom items from users,like in the Yahoo!Music data.This provides a good estimate of the ratings concerning items that a user would not have rated otherwise.But it is also a costly experiment,and puts a burden onto users.Especially,if the items to rate are movies,it would be very time-consuming for users to watch random movies as they might not enjoy them.Second,one may use a prior distribution with a small number of free parameters,which can be then tuned to achieve the desired result,e.g.,by cross-validation.Such an approach was outlined in [22]for rating data,and a prob-abilistic version with prior distributions in [25].The lat-ter paper also shows that several rating values assigned to the same item i by a user u ,i.e.,a distribution of rating values p (R |u,i ),is equivalent to using the average rating ¯r u,i =E[R |u,i ]= r r ·p (r |u,i )when optimizing the least squares objective function.3This allows one to parameter-ize the prior distribution in terms of its mean.In [22],a single mean rating value is used for all users and items.It is an interesting result that,when this mean rating value is optimized as to achieve the best ranking 4performance on the Netflix test set,a mean rating value of about 2is found.This appears like a reasonable value for approximating the (unknown)mean rating value for the items that a user did not rate,as it agrees well with the results found for the Ya-hoo!Music data.Hence,this approach may provide a way for approximating relevant parameters of the (otherwise un-known)rating distribution concerning the items that a user did not rate.The approach in [22]is summarized in the fol-lowing section;it will also be used in our experiments later in this paper.2.5Model and TrainingIn this section,we briefly review the low-rank matrix-factorization (MF)model named AllRank in [22],which was introduced as a point-wise ranking approach that accounts for all (unrated)items for each user.However,one can also view it from the perspective of rating prediction:the ob-served ratings in the data (i.e.,p (r |c +,u,i )),are comple-mented by imputed ratings with low values (as to approxi-mate the unknown p (r |c −,u,i )).As a result,an approxima-tion to p (r |u,i )is achieved,which applies to any item in the collection.For comparison,also the standard MF approach of minimizing RMSE on the observed ratings is discussed,and denoted by MF-RMSE.The matrix of predicted ratings ˆR∈R m ×n ,where m de-notes the number of items,and n the number of users,is modeled asˆR=r 0+P Q ,(11)with matrices P ∈R m ×j 0and Q ∈R n ×j 0,where the rank j 0 m,n ;and r 0∈R is a (global)offset.3Note that this holds only for optimization/maximum-a-posteriori estimates,but does not apply for estimating the full posterior distribution,e.g.,by means of Markov Chain Monte Carlo.4Note that,in contrast to RMSE,(some)ranking measures can be applied to the entire collection of items,and hence account for both items with and without ratings assigned by the user.For computationally efficient training,the square error (with the usual L2-norm regularization)is optimized: all uall iW i,u ·R o&i i,u −ˆRi,u 2+λj 0j =1P 2i,j +Q 2u,j,(12)where ˆRi,u denotes the ratings predicted by the model in Eq.11;and R o&ii,uequals the actual rating value in the training data if observed for item i and user u ;otherwise thevalue R o&ii,u=r 0∈R is imputed.The key is in the training weights,W i,u =1if R obsi,u observedw 0otherwise.(13)In AllRank [22],the weight assigned to the imputedratings is positive ,i.e.,w 0>0[22],and the imputed rat-ing value is lower than the observed average rating in the data.This captures the fact that the (unknown)probability p (r |c −,u,i )is larger for lower rating values,compared to the observed probabilities p (r |c +,u,i ).In contrast,MF-RMSE is obtained for w 0=0.This seemingly small difference has the important effect that AllRank is trained on a combi-nation of both distributions,p (r |c −,u,i )and p (r |c +,u,i ),geared towards approximating p (r |u,i ).In contrast,MF-RMSE is optimized towards p (r |c +,u,i ).Due to this differ-ence,in [22]AllRank was found to achieve a considerably larger top-N hit-rate or recall when ranking all items in the collection,compared to various state-of-the-art approaches optimized on the observed ratings only,see results in [22]and compare to [10,4].Alternating least squares can be used for computationally efficient training of AllRank [22]and MF-RMSE.We found the following values for the tuning parameters in Eq.12for the Netflix data (see also [22])to yield the best results:r 0=2,w 0=0.005and λ=0.04for AllRank;and λ=0.07for MF-RMSE (and w 0=0);we use rank j o =50for both approaches.While there are several state-of-the-art approaches,e.g.,[6,9,10,16,19],that achieve a lower RMSE on observed rat-ings than MF-RMSE does,note that their test performances on all (unrated)items is quite similar to each other,e.g.,see [10,4].This is interesting,as some of these approaches,like [16,19,10,20],actually account in some sense for the MNAR nature of the data–but in the context of observed ratings (minimizing RMSE).Note that AllRank does not only apply to explicit feedback data,but can also be used for implicit feedback data,similar to [8,15].3.RANKINGIn this section,we examine ranking as a means for assess-ing recommendation accuracy.Ranking is a useful approach when the recommendation task is,for each user,to pick a small number,say N ,of items from among all available items in the collection.We divide the ranking task into two parts in this section:ranking protocols and ranking metrics,as outlined and compared to each other in the following.3.1Ranking ProtocolsWith ranking protocols,we refer to the fact as to which items are ranked.One may distinguish between two slightly。
云南省高考英语一轮复习训练:23
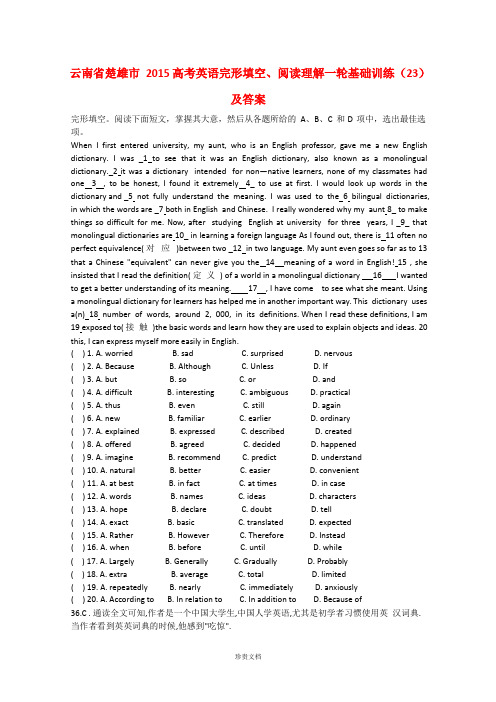
云南省楚雄市2015 高考英语完形填空、阅读理解一轮基础训练(23)及答案完形填空。
阅读下面短文,掌握其大意,然后从各题所给的A、B、C 和D 项中,选出最佳选项。
When I first entered university, my aunt, who is an English professor, gave me a new English dictionary. I was _1 to see that it was an English dictionary, also known as a monolingual dictionary._2 it was a dictionary intended for non—native learners, none of my classmates had one 3 , to be honest, I found it extremely 4_ to use at first. I would look up words in the dictionary and _5 not fully understand the meaning. I was used to the 6 bilingual dictionaries, in which the words are _7 both in English and Chinese. I really wondered why my aunt 8_ to make things so difficult for me. Now, after studying English at university for three years, I _9_ that monolingual dictionaries are 10_ in learning a foreign language As I found out, there is 11 often no perfect equivalence( 对应)between two _12 in two language. My aunt even goes so far as to 13 that a Chinese "equivalent" can never give you the 14 meaning of a word in English! 15 , she insisted that I read the definition( 定义) of a world in a monolingual dictionary 16 I wanted to get a better understanding of its meaning. 17 , I have come to see what she meant. Using a monolingual dictionary for learners has helped me in another important way. This dictionary uses a(n) 18 number of words, around 2, 000, in its definitions. When I read these definitions, I am 19 exposed to( 接触)the basic words and learn how they are used to explain objects and ideas. 20 this, I can express myself more easily in English.( ) 1. A. worried B. sad C. surprised D. nervous( ) 2. A. Because B. Although C. Unless D. If( ) 3. A. but B. so C. or D. and( ) 4. A. difficult B. interesting C. ambiguous D. practical( ) 5. A. thus B. even C. still D. again( ) 6. A. new B. familiar C. earlier D. ordinary( ) 7. A. explained B. expressed C. described D. created( ) 8. A. offered B. agreed C. decided D. happened( ) 9. A. imagine B. recommend C. predict D. understand( ) 10. A. natural B. better C. easier D. convenient( ) 11. A. at best B. in fact C. at times D. in case( ) 12. A. words B. names C. ideas D. characters( ) 13. A. hope B. declare C. doubt D. tell( ) 14. A. exact B. basic C. translated D. expected( ) 15. A. Rather B. However C. Therefore D. Instead( ) 16. A. when B. before C. until D. while( ) 17. A. Largely B. Generally C. Gradually D. Probably( ) 18. A. extra B. average C. total D. limited( ) 19. A. repeatedly B. nearly C. immediately D. anxiously( ) 20. A. According to B. In relation to C. In addition to D. Because of36.C . 通读全文可知,作者是一个中国大学生,中国人学英语,尤其是初学者习惯使用英汉词典.当作者看到英英词典的时候,他感到"吃惊".珍贵文档37.B.前后两句在意义上是让步关系,所以用although 引导让步状语从句.而A 项because 引导原因状语从句;Unless 和if'引导条件状语从句.38.A.此空格处是一个并列连词,连接前后两句.而前后两句之间存在的是转折关系,所以用but.39.A.最初用英英词典,不习惯,所以作者感觉用起来"很难difficult".interesting 是"有趣味的意思",根据下文not fully understand the meaning 不可能是这个答案;ambiguous 是"不明确的";practical 是"实用的",这两个词也不符合语境.40.C.句子中有否定词not,有副词fully,所以用still,表示"仍然不能够完全"的意思.41.B.be used to 表示"习惯……,对作者来说习惯了双语词典,或者说是英汉词典.这里指双语词典的使用对他来说是熟悉.42.A.此句是一个in which 引导的定语从句,其中words 是主语,根据常识在词典中单词的意思是被"解释出来"的,所以用explained.43.C.我真想知道我姑妈为什么决定这么为难我.其他三个词虽然都跟动词不定式,但是在这里意思都不恰当.44.D.过去不理解,经过一段时间后,"明白了understand";imagine 是"想象"的意思; recommend 是"推荐"的意思;predict 是"预测"的意思.45.B.此句中monolingual dictionaries 和bilingual dictionaries 比较,A 和D 项没用比较级, better 强调更好,更实用,而easier 则不可能.46.B.常见的情况是实际上两种语言之间的两个词没有完全的对应.in fact 表示"实际上, 事实上".at best 是"至多;充其量";at times 是"有时";in case 是"万一,以防", 显然A,C,D 项不合语境. 47.A.词典上重点的内容应该是单词.48.B.我姑妈甚至还声称汉语意思决不能给出一个英语单词的确切意思.49.A.前面的a Chinese equivalent 和the meaning of a word in English 对应,所表明还是对应不准确的问题,所以用exact.50.C.此空后面的句子和前面表示的是一种"因果"关系,所以用therefore.51.A.表示"当……时候",用when 引导时间状语从句.而before 通常指"在……之前"; until 指"直到……时候";while 指"在……的同时".52.C.该句的谓语have come to see 是表示"变化过程",所以用gradually 表示"渐渐地".53.D.从后面的around 2,000 可知词典中用于解释词义的词是限制在2,000 左右.54.A.在有限的范围内,查阅阅读释义的话,就会反复接触基本词汇,学会这些词汇怎样用来解释事物和观点,所以用repeatedly 表示"反复地,经常地"55.D.后面主句部分I can express myself more easily in English.表达的是结果,此处表达的应该是原因,说明使用这种词典所带来的好处.阅读下列短文, 从给的四个选项(A、B、C 和D) 中, 选出最佳选项。
1-Discrete-time+MPC+for+Beginners+
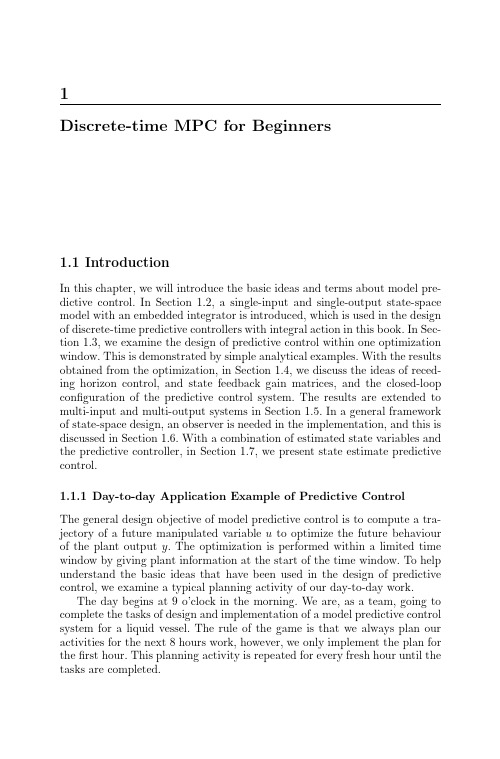
1Discrete-time MPC for Beginners1.1IntroductionIn this chapter,we will introduce the basic ideas and terms about model pre-dictive control.In Section1.2,a single-input and single-output state-space model with an embedded integrator is introduced,which is used in the design of discrete-time predictive controllers with integral action in this book.In Sec-tion1.3,we examine the design of predictive control within one optimization window.This is demonstrated by simple analytical examples.With the results obtained from the optimization,in Section1.4,we discuss the ideas of reced-ing horizon control,and state feedback gain matrices,and the closed-loop configuration of the predictive control system.The results are extended to multi-input and multi-output systems in Section1.5.In a general framework of state-space design,an observer is needed in the implementation,and this is discussed in Section1.6.With a combination of estimated state variables and the predictive controller,in Section1.7,we present state estimate predictive control.1.1.1Day-to-day Application Example of Predictive ControlThe general design objective of model predictive control is to compute a tra-jectory of a future manipulated variable u to optimize the future behaviour of the plant output y.The optimization is performed within a limited time window by giving plant information at the start of the time window.To help understand the basic ideas that have been used in the design of predictive control,we examine a typical planning activity of our day-to-day work.The day begins at9o’clock in the morning.We are,as a team,going to complete the tasks of design and implementation of a model predictive control system for a liquid vessel.The rule of the game is that we always plan our activities for the next8hours work,however,we only implement the plan for thefirst hour.This planning activity is repeated for every fresh hour until the tasks are completed.21Discrete-time MPC for BeginnersGiven the amount of background work that we have completed for9o’clock,we plan ahead for the next8hours.Assume that the work tasks are divided into modelling,design,simulation and pleting these tasks will be a function of various factors,such as how much effort we will put in,how well we will work as a team and whether we will get some additional help from others.These are the manipulated variables in the planning problem.Also,we have our limitations,such as our ability to understand the design problem,and whether we have good skills of computer hardware and software engineering. These are the hard and soft constraints in the planning.The background information we have already acquired is paramount for this planning work.After everything is considered,we determine the design tasks for the next 8hours as functions of the manipulated variables.Then we calculate hour-by-hour what we need to do in order to complete the tasks.In this calculation, based on the background information,we will take our limitations into con-sideration as the constraints,andfind the best way to achieve the goal.The end result of this planning gives us our projected activities from9o’clock to 5o’clock.Then we start working by implementing the activities for thefirst hour of our plan.At10o’clock,we check how much we have actually done for thefirst hour.This information is used for the planning of our next phase of activities. Maybe we have done less than we planned because we could not get the correct model or because one of the key members went for an emergency meeting.Nevertheless,at10o’clock,we make an assessment on what we have achieved,and use this updated information for planning our activities for the next8hours.Our objective may remain the same or may change.The length of time for the planning remains the same(8hours).We repeat the same planning process as it was at9o’clock,which then gives us the new projected activities for the next8hours.We implement thefirst hour of activities at 10o’clock.Again at11o’clock,we assess what we have achieved again and use the updated information for the plan of work for the next8hours.The planning and implementation process is repeated every hour until the original objective is achieved.There are three key elements required in the planning.Thefirst is the way of predicting what might happen(model);the second is the instrument of assessing our current activities(measurement);and the third is the instrument of implementing the planned activities(realization of control).The key issues in the planning exercise are:1.the time window for the planning isfixed at8hours;2.we need to know our current status before the planning;3.we take the best approach for the8hours work by taking the constraintsinto consideration,and the optimization is performed in real-time with a moving horizon time window and with the latest information available. The planning activity described here involves the principle of MPC.In this example,it is described by the terms that are to be used frequently in the1.1Introduction3 following:the moving horizon window,prediction horizon,receding horizon control,and control objective.They are introduced as below.1.Moving horizon window:the time-dependent window from an arbitrarytime t i to t i+T p.The length of the window T p remains constant.In this example,the planning activity is performed within an8-hour window, thus T p=8,with the measurement taken every hour.However,t i,which defines the beginning of the optimization window,increases on an hourly basis,starting with t i=9.2.Prediction horizon:dictates how‘far’we wish the future to be predictedfor.This parameter equals the length of the moving horizon window,T p.3.Receding horizon control:although the optimal trajectory of future controlsignal is completely described within the moving horizon window,the actual control input to the plant only takes thefirst sample of the control signal,while neglecting the rest of the trajectory.4.In the planning process,we need the information at time t i in order topredict the future.This information is denoted as x(t i)which is a vec-tor containing many relevant factors,and is either directly measured or estimated.5.A given model that will describe the dynamics of the system is paramountin predictive control.A good dynamic model will give a consistent and accurate prediction of the future.6.In order to make the best decision,a criterion is needed to reflect the ob-jective.The objective is related to an error function based on the difference between the desired and the actual responses.This objective function is often called the cost function J,and the optimal control action is found by minimizing this cost function within the optimization window.1.1.2Models Used in the DesignThere are three general approaches to predictive control design.Each ap-proach uses a unique model structure.In the earlier formulation of model predictive control,finite impulse response(FIR)models and step response models were favoured.FIR model/step response model based design algo-rithms include dynamic matrix control(DMC)(Cutler and Ramaker,1979) and the quadratic DMC formulation of Garcia and Morshedi(1986).The FIR type of models are appealing to process engineers because the model structure gives a transparent description of process time delay,response time and gain.However,they are limited to stable plants and often require large model orders.This model structure typically requires30to60impulse re-sponse coefficients depending on the process dynamics and choice of sampling intervals.Transfer function models give a more parsimonious description of process dynamics and are applicable to both stable and unstable plants.Rep-resentatives of transfer function model-based predictive control include the predictive control algorithm of Peterka(Peterka,1984)and the generalized41Discrete-time MPC for Beginnerspredictive control(GPC)algorithm of Clarke and colleagues(Clarke et al., 1987).The transfer function model-based predictive control is often considered to be less effective in handling multivariable plants.A state-space formulation of GPC has been presented in Ordys and Clarke(1993).Recent years have seen the growing popularity of predictive control de-sign using state-space design methods(Ricker,1991,Rawlings and Muske, 1993,Rawlings,2000,Maciejowski,2002).In this book,we will use state-space models,both in continuous time and discrete time for simplicity of the design framework and the direct link to the classical linear quadratic regulators. 1.2State-space Models with Embedded IntegratorModel predictive control systems are designed based on a mathematical model of the plant.The model to be used in the control system design is taken to be a state-space model.By using a state-space model,the current information required for predicting ahead is represented by the state variable at the current time.1.2.1Single-input and Single-output SystemFor simplicity,we begin our study by assuming that the underlying plant is a single-input and single-output system,described by:x m(k+1)=A m x m(k)+B m u(k),(1.1) y(k)=C m x m(k),(1.2)where u is the manipulated variable or input variable;y is the process output; and x m is the state variable vector with assumed dimension n1.Note that this plant model has u(k)as its input.Thus,we need to change the model to suit our design purpose in which an integrator is embedded.Note that a general formulation of a state-space model has a direct term from the input signal u(k)to the output y(k)asy(k)=C m x m(k)+D m u(k).However,due to the principle of receding horizon control,where a current information of the plant is required for prediction and control,we have im-plicitly assumed that the input u(k)cannot affect the output y(k)at the same time.Thus,D m=0in the plant model.Taking a difference operation on both sides of(1.1),we obtain thatx m(k+1)−x m(k)=A m(x m(k)−x m(k−1))+B m(u(k)−u(k−1)).1.2State-space Models with Embedded Integrator 5Let us denote the difference of the state variable byΔx m (k +1)=x m (k +1)−x m (k );Δx m (k )=x m (k )−x m (k −1),and the difference of the control variable byΔu (k )=u (k )−u (k −1).These are the increments of the variables x m (k )and u (k ).With this transfor-mation,the difference of the state-space equation is:Δx m (k +1)=A m Δx m (k )+B m Δu (k ).(1.3)Note that the input to the state-space model is Δu (k ).The next step is to connect Δx m (k )to the output y (k ).To do so,a new state variable vector is chosen to be x (k )= Δx m (k )T y (k ) T ,where superscript T indicates matrix transpose.Note thaty (k +1)−y (k )=C m (x m (k +1)−x m (k ))=C m Δx m (k +1)=C m A m Δx m (k )+C m B m Δu (k ).(1.4)Putting together (1.3)with (1.4)leads to the following state-space model:x (k +1) Δx m (k +1)y (k +1) =A A m o T m C m A m 1 x (k ) Δx m (k )y (k ) +B B m C m B mΔu (k )y (k )=C o m 1 Δx m (k )y (k ),(1.5)where o m =n 1 00...0 .The triplet (A,B,C )is called the augmented model,which will be used in the design of predictive control.Example 1.1.Consider a discrete-time model in the following form:x m (k +1)=A m x m (k )+B m u (k )y (k )=C m x m (k )(1.6)where the system matrices areA m = 1101 ;B m = 0.51;C m = 10 .Find the triplet matrices (A,B,C )in the augmented model (1.5)and calcu-late the eigenvalues of the system matrix,A ,of the augmented model.61Discrete-time MPC for BeginnersSolution.From (1.5),n 1=2and o m =[00].The augmented model for this plant is given byx (k +1)=Ax (k )+BΔu (k )y (k )=Cx (k ),(1.7)where the augmented system matrices are A = A m o T m C m A m 1 =⎡⎣110010111⎤⎦;B = B m C m B m =⎡⎣0.510.5⎤⎦;C = o m 1 = 001 .The characteristic equation of matrix A is given by ρ(λ)=det(λI −A )=det λI −A m o T m −C m A m (λ−1)=(λ−1)det(λI −A m )=(λ−1)3.(1.8)Therefore,the augmented state-space model has three eigenvalues at λ=1.Among them,two are from the original integrator plant,and one is from the augmentation of the plant model.1.2.2MATLAB Tutorial:Augmented Design ModelTutorial 1.1.The objective of this tutorial is to demonstrate how to obtain a discrete-time state-space model from a continuous-time state-space model,and form the augmented discrete-time state-space model.Consider a continuous-time system has the state-space model:˙x m (t )=⎡⎣010301010⎤⎦x m (t )+⎡⎣113⎤⎦u (t )y (t )= 010 x m (t ).(1.9)Step by Step1.Create a new file called extmodel.m.We form a continuous-time state vari-able model;then this continuous-time model is discretized using MATLAB function ‘c2dm’with specified sampling interval Δt .2.Enter the following program into the file:Ac =[010;301;010];Bc=[1;1;3];Cc=[010];Dc=zeros(1,1);Delta_t=1;[Ad,Bd,Cd,Dd]=c2dm(Ac,Bc,Cc,Dc,Delta_t);1.3Predictive Control within One Optimization Window7 3.The dimensions of the system matrices are determined to discover thenumbers of states,inputs and outputs.The augmented state-space model is produced.Continue entering the following program into thefile: [m1,n1]=size(Cd);[n1,n_in]=size(Bd);A_e=eye(n1+m1,n1+m1);A_e(1:n1,1:n1)=Ad;A_e(n1+1:n1+m1,1:n1)=Cd*Ad;B_e=zeros(n1+m1,n_in);B_e(1:n1,:)=Bd;B_e(n1+1:n1+m1,:)=Cd*Bd;C_e=zeros(m1,n1+m1);C_e(:,n1+1:n1+m1)=eye(m1,m1);4.Run this program to produce the augmented state variable model for thedesign of predictive control.1.3Predictive Control within One Optimization Window Upon formulation of the mathematical model,the next step in the design of a predictive control system is to calculate the predicted plant output with the future control signal as the adjustable variables.This prediction is described within an optimization window.This section will examine in detail the opti-mization carried out within this window.Here,we assume that the current time is k i and the length of the optimization window is N p as the number of samples.For simplicity,the case of single-input and single-output systems is consideredfirst,then the results are extended to multi-input and multi-output systems.1.3.1Prediction of State and Output VariablesAssuming that at the sampling instant k i,k i>0,the state variable vector x(k i)is available through measurement,the state x(k i)provides the current plant information.The more general situation where the state is not directly measured will be discussed later.The future control trajectory is denoted by Δu(k i),Δu(k i+1),...,Δu(k i+N c−1),where N c is called the control horizon dictating the number of parameters used to capture the future control trajectory.With given information x(k i), the future state variables are predicted for N p number of samples,where N p is called the prediction horizon.N p is also the length of the optimization window.We denote the future state variables asx(k i+1|k i),x(k i+2|k i),...,x(k i+m|k i),...,x(k i+N p|k i),81Discrete-time MPC for Beginnerswhere x (k i +m |k i )is the predicted state variable at k i +m with given current plant information x (k i ).The control horizon N c is chosen to be less than (or equal to)the prediction horizon N p .Based on the state-space model (A,B,C ),the future state variables are calculated sequentially using the set of future control parameters:x (k i +1|k i )=Ax (k i )+BΔu (k i )x (k i +2|k i )=Ax (k i +1|k i )+BΔu (k i +1)=A 2x (k i )+ABΔu (k i )+BΔu (k i +1)...x (k i +N p |k i )=A N p x (k i )+A N p −1BΔu (k i )+A N p −2BΔu (k i +1)+...+A N p −N c BΔu (k i +N c −1).From the predicted state variables,the predicted output variables are,by substitutiony (k i +1|k i )=CAx (k i )+CBΔu (k i )(1.10)y (k i +2|k i )=CA 2x (k i )+CABΔu (k i )+CBΔu (k i +1)y (k i +3|k i )=CA 3x (k i )+CA 2BΔu (k i )+CABΔu (k i +1)+CBΔu (k i +2)...y (k i +N p |k i )=CA N p x (k i )+CA N p −1BΔu (k i )+CA N p −2BΔu (k i +1)+...+CA N p −N c BΔu (k i +N c −1).(1.11)Note that all predicted variables are formulated in terms of current state variable information x (k i )and the future control movement Δu (k i +j ),where j =0,1,...N c −1.Define vectors Y = y (k i +1|k i )y (k i +2|k i )y (k i +3|k i )...y (k i +N p |k i )T ΔU = Δu (k i )Δu (k i +1)Δu (k i +2)...Δu (k i +N c −1) T ,where in the single-input and single-output case,the dimension of Y is N p and the dimension of ΔU is N c .We collect (1.10)and (1.11)together in a compact matrix form asY =F x (k i )+ΦΔU,(1.12)where F =⎡⎢⎢⎢⎢⎢⎣CACA 2CA 3...CA N p ⎤⎥⎥⎥⎥⎥⎦;Φ=⎡⎢⎢⎢⎢⎢⎣CB 00...0CAB CB 0...0CA 2B CAB CB ...0...CA N p −1B CA N p −2B CA N p −3B ...CA N p −N c B⎤⎥⎥⎥⎥⎥⎦.1.3Predictive Control within One Optimization Window9 1.3.2OptimizationFor a given set-point signal r(k i)at sample time k i,within a prediction horizon the objective of the predictive control system is to bring the predicted output as close as possible to the set-point signal,where we assume that the set-point signal remains constant in the optimization window.This objective is then translated into a design tofind the‘best’control parameter vectorΔU such that an error function between the set-point and the predicted output is minimized.Assuming that the data vector that contains the set-point information isR T s=N p11 (1)r(k i),we define the cost function J that reflects the control objective asJ=(R s−Y)T(R s−Y)+ΔU T¯RΔU,(1.13) where thefirst term is linked to the objective of minimizing the errors between the predicted output and the set-point signal while the second term reflects the consideration given to the size ofΔU when the objective function J is made to be as small as possible.¯R is a diagonal matrix in the form that¯R=rw I N c×N c(r w≥0)where r w is used as a tuning parameter for the desired closed-loop performance.For the case that r w=0,the cost function (1.13)is interpreted as the situation where we would not want to pay any attention to how large theΔU might be and our goal would be solely to make the error(R s−Y)T(R s−Y)as small as possible.For the case of large r w,the cost function(1.13)is interpreted as the situation where we would carefully consider how large theΔU might be and cautiously reduce the error (R s−Y)T(R s−Y).Tofind the optimalΔU that will minimize J,by using(1.12),J is ex-pressed asJ=(R s−F x(k i))T(R s−F x(k i))−2ΔU TΦT(R s−F x(k i))+ΔU T(ΦTΦ+¯R)ΔU.(1.14) From thefirst derivative of the cost function J:∂J∂ΔU=−2ΦT(R s−F x(k i))+2(ΦTΦ+¯R)ΔU,(1.15) the necessary condition of the minimum J is obtained as∂J∂ΔU=0,from which wefind the optimal solution for the control signal asΔU=(ΦTΦ+¯R)−1ΦT(R s−F x(k i)),(1.16)101Discrete-time MPC for Beginnerswith the assumption that (ΦT Φ+¯R)−1exists.The matrix (ΦT Φ+¯R )−1is called the Hessian matrix in the optimization literature.Note that R s is a data vector that contains the set-point information expressed asR s =N p [111...1]T r (k i )=¯Rs r (k i ),where¯Rs =N p [111...1]T .The optimal solution of the control signal is linked to the set-point signal r (k i )and the state variable x (k i )via the following equation:ΔU =(ΦT Φ+¯R )−1ΦT (¯R s r (k i )−F x (k i )).(1.17)Example 1.2.Suppose that a first-order system is described by the state equa-tion:x m (k +1)=ax m (k )+bu (k )y (k )=x m (k ),(1.18)where a =0.8and b =0.1are scalars.Find the augmented state-space model.Assuming a prediction horizon N p =10and control horizon N c =4,calcu-late the components that form the prediction of future output Y ,and the quantities ΦT Φ,ΦT F and ΦT ¯Rs .Assuming that at a time k i (k i =10for this example),r (k i )=1and the state vector x (k i )=[0.10.2]T ,find the optimal solution ΔU with respect to the cases where r w =0and r w =10,and compare the results.Solution.The augmented state-space equation is Δx m (k +1)y (k +1) = a 0a 1 Δx m (k )y (k ) + b b Δu (k )y (k )= 01 Δx m (k )y (k ).(1.19)Based on (1.12),the F and Φmatrices take the following forms:F =⎡⎢⎢⎢⎢⎢⎢⎢⎢⎢⎢⎢⎢⎢⎢⎣CA CA 2CA 3CA 4CA 5CA 6CA 7CA 8CA 9CA 10⎤⎥⎥⎥⎥⎥⎥⎥⎥⎥⎥⎥⎥⎥⎥⎦;Φ=⎡⎢⎢⎢⎢⎢⎢⎢⎢⎢⎢⎢⎢⎢⎢⎣CB 000CAB CB 00CA 2B CAB CB 0CA 3B CA 2B CAB CB CA 4B CA 3B CA 2B CAB CA 5B CA 4B CA 3B CA 2B CA 6B CA 5B CA 4B CA 3B CA 7B CA 6B CA 5B CA 4B CA 8B CA 7B CA 6B CA 5B CA 9B CA 8B CA 7B CA 6B ⎤⎥⎥⎥⎥⎥⎥⎥⎥⎥⎥⎥⎥⎥⎥⎦.1.3Predictive Control within One Optimization Window11 The coefficients in the F andΦmatrices are calculated as follows:CA=s11CA2=s21CA3=s31... CA k=s k1,(1.20)where s1=a,s2=a2+s1,...,s k=a k+s k−1,andCB=g0=bCAB=g1=ab+g0CA2B=g2=a2b+g1...CA k−1B=g k−1=a k−1b+g k−2CA k B=g k=a k b+g k−1.(1.21) With the plant parameters a=0.8and b=0.1,N p=10and N c=4,we calculate the quantitiesΦTΦ=⎡⎢⎢⎣1.15411.04070.91160.7726 1.04070.95490.84750.7259 0.91160.84750.76750.6674 0.77260.72590.66740.5943⎤⎥⎥⎦ΦT F=⎡⎢⎢⎣9.23253.21478.32592.76847.29272.33556.18111.9194⎤⎥⎥⎦;ΦT¯R s=⎡⎢⎢⎣3.21472.76842.33551.9194⎤⎥⎥⎦.Note that the vectorΦT¯R s is identical to the last column in the matrixΦT F. This is because the last column of F matrix is identical to¯R s.At time k i=10,the state vector x(k i)=[0.10.2]T.In thefirst case,the error between predicted Y and R s is reduced without any consideration to the magnitude of control ly,r w=0.Then,the optimalΔU is found through the calculationΔU=(ΦTΦ)−1(ΦT R s−ΦT F x(k i))=7.2−6.400T.We note that without weighting on the incremental control,the last two ele-mentsΔu(k i+2)=0andΔu(k i+3)=0,while thefirst two elements have a rather large magnitude.Figure1.1a shows the changes of the state variables where we can see that the predicted output y has reached the desired set-point121Discrete-time MPC for Beginners(a)State variables with no weight onΔu(b)State variables with weight on Δuparison of optimal solutions.Key:line (1)Δx m ;line (2)y 1while the Δx m decays to zero.To examine the effect of the weight r w on the optimal solution of the control,we let r w =10.The optimal solution of ΔU is given below,where I is a 4×4identity matrix,ΔU =(ΦT Φ+10×I )−1(ΦT R s −ΦT F x (k i ))(1.22)= 0.12690.10340.08290.065 T .With this choice,the magnitude of the first two control increments is signifi-cantly reduced,also the last two components are no longer zero.Figure 1.1b shows the optimal state variables.It is seen that the output y did not reach the set-point value of 1,however,the Δx m approaches zero.An observation follows from the comparison study.It seems that if we want the control to move cautiously,then it takes longer for the control signal to reach its steady state (i.e.,the values in ΔU decrease more slowly),because the optimal control energy is distributed over the longer period of future time.We can verify this by increasing N c to 9,while maintaining r w =10.The result shows that the magnitude of the elements in ΔU is reducing,but they are significant for the first 8elements:ΔU T = 0.12270.09930.07900.06140.04630.03340.02270.01390.0072 .In comparison with the case where N c =4,we note that when N c =9,the first four parameters in ΔU are slightly different from the previous case.Example 1.3.There is an alternative way to find the minimum of the cost function via completing the squares.This is an intuitive approach,also the minimum of the cost function becomes a by-product of the approach.Find the optimal solution for ΔU by completing the squares of the cost func-tion J (1.14).1.3Predictive Control within One Optimization Window 13Solution.From (1.14),by adding and subtracting the term(R s −F x (k i ))T Φ(ΦT Φ+¯R)−1ΦT (R s −F x (k i ))to the original cost function J ,its value remains unchanged.This leads toJ =(R s −F x (k i ))T (R s −F x (k i )) −2ΔU T ΦT (R s −F x (k i ))+ΔU T (ΦT Φ+¯R )ΔU + (R s −F x (k i ))T Φ(ΦT Φ+¯R)−1ΦT (R s −F x (k i ))−(R s −F x (k i ))T Φ(ΦT Φ+¯R)−1ΦT (R s −F x (k i )),(1.23)where the quantities under the .are the completed ‘squares’:J 0= ΔU −(ΦT Φ+¯R )−1ΦT (R s −F x (k i )) T ×(ΦT Φ+¯R ) ΔU −(ΦT Φ+¯R )−1ΦT (R s −F x (k i )) .(1.24)This can be easily verified by opening the squares.Since the first and last terms in (1.23)are independent of the variable ΔU (sometimes,we call this a decision variable),and (ΦT Φ+¯R)is assumed to be positive definite,then the minimum of the cost function J is achieved if the quantity J 0equals zero,i.e.,ΔU =(ΦT Φ+¯R)−1ΦT (R s −F x (k i )).(1.25)This is the optimal control solution.By substituting this optimal solution into the cost function (1.23),we obtain the minimum of the cost asJ min =(R s −F x (k i ))T (R s −F x (k i ))−(R s −F x (k i ))T Φ(ΦT Φ+¯R)−1ΦT (R s −F x (k i )).1.3.3MATLAB Tutorial:Computation of MPC GainsTutorial 1.2.The objective of this tutorial is to produce a MATLAB function for calculating ΦT Φ,ΦT F ,ΦT ¯Rs .The key here is to create F and Φmatrices.Φmatrix is a Toeplitz matrix,which is created by defining its first column,and the next column is obtained through shifting the previous column.Step by Step1.Create a new file called mpcgain.m.2.The first step is to create the augmented model for MPC design.The input parameters to the function are the state-space model (A p ,B p ,C p ),prediction horizon N p and control horizon N c .Enter the following program into the file:141Discrete-time MPC for Beginnersfunction[Phi_Phi,Phi_F,Phi_R,A_e,B_e,C_e]=mpcgain(Ap,Bp,Cp,Nc,Np);[m1,n1]=size(Cp);[n1,n_in]=size(Bp);A_e=eye(n1+m1,n1+m1);A_e(1:n1,1:n1)=Ap;A_e(n1+1:n1+m1,1:n1)=Cp*Ap;B_e=zeros(n1+m1,n_in);B_e(1:n1,:)=Bp;B_e(n1+1:n1+m1,:)=Cp*Bp;C_e=zeros(m1,n1+m1);C_e(:,n1+1:n1+m1)=eye(m1,m1);3.Note that the F and P hi matrices have special forms.By taking advantageof the special structure,we obtain the matrices.4.Continue entering the program into thefile:n=n1+m1;h(1,:)=C_e;F(1,:)=C_e*A_e;for kk=2:Nph(kk,:)=h(kk-1,:)*A_e;F(kk,:)=F(kk-1,:)*A_e;endv=h*B_e;Phi=zeros(Np,Nc);%declare the dimension of PhiPhi(:,1)=v;%first column of Phifor i=2:NcPhi(:,i)=[zeros(i-1,1);v(1:Np-i+1,1)];%Toeplitz matrixendBarRs=ones(Np,1);Phi_Phi=Phi’*Phi;Phi_F=Phi’*F;Phi_R=Phi’*BarRs;5.Type into the MATLAB Work Space with Ap=0.8,Bp=0.1,Cp=1,Nc=4and Np=10.Run this MATLAB function by typing[Phi_Phi,Phi_F,Phi_R,A_e,B_e,C_e]=mpcgain(Ap,Bp,Cp,Nc,Np);paring the results with the answers from Example1.2.If it is identicalto what was presented there,then your program is correct.7.Varying the prediction horizon and control horizon,observe the changesin these matrices.8.CalculateΔU by assuming the information of initial condition on x andr.The inverse of matrix M is calculated in MATLAB as inv(M).9.Validate the results in Example1.2.1.4Receding Horizon Control 151.4Receding Horizon ControlAlthough the optimal parameter vector ΔU contains the controls Δu (k i ),Δu (k i +1),Δu (k i +2),...,Δu (k i +N c −1),with the receding horizon control principle,we only implement the first sample of this sequence,i.e.,Δu (k i ),while ignoring the rest of the sequence.When the next sample period arrives,the more recent measurement is taken to form the state vector x (k i +1)for calculation of the new sequence of control signal.This procedure is repeated in real time to give the receding horizon control law.Example 1.4.We illustrate this procedure by continuing Example 1.2,where a first-order system with the state-space descriptionx m (k +1)=0.8x m (k )+0.1u (k )is used in the computation.We will consider the case r w =0.The initial conditions are x (10)=[0.10.2]T and u (9)=0.Solution.At sample time k i =10,the optimal control was previously com-puted as Δu (10)=7.2.Assuming that u (9)=0,then the control signal to the plant is u (10)=u (9)+Δu (10)=7.2and with x m (10)=y (10)=0.2,we calculate the next simulated plant state variablex m (11)=0.8x m (10)+0.1u (10)=0.88.(1.26)At k i =11,the new plant information is Δx m (11)=0.88−0.2=0.68and y (11)=0.88,which forms x (11)= 0.680.88 T .Then we obtainΔU =(ΦT Φ)−1(ΦT R s −ΦT F x (11))= −4.24−0.960.00000.0000 T .This leads to the optimal control u (11)=u (10)+Δu (11)=2.96.This new control is implemented to obtainx m (12)=0.8x m (11)+0.1u (11)=1.(1.27)At k i =12,the new plant information is Δx m (12)=1−0.88=0.12and y (12)=1,which forms x (12)= 0.121 .We obtainΔU =(ΦT Φ)−1(ΦT R s −ΦT F x (11))= −0.960.0000.00000.0000 T .This leads to the control at k i =12as u (12)=u (11)−0.96=2.By imple-menting this control,we obtain the next plant output asx m (13)=ax m (12)+bu (12)=1.(1.28)The new plant information is Δx m (13)=1−1=0and y (13)=1.From this information,we obtain。
结构方程模型 交叉效度

结构方程模型交叉效度英文回答:Structural equation modeling (SEM) is a statistical method used to test and estimate the relationships between observed and latent variables. Cross-validity is an important aspect of SEM, as it helps to ensure that the measures used to assess latent variables are valid and reliable.There are two main types of cross-validity:Convergent validity assesses the extent to which different measures of the same latent variable are correlated with each other.Discriminant validity assesses the extent to which different measures of different latent variables are not correlated with each other.Cross-validity is essential for ensuring that the results of an SEM analysis are valid and reliable. If the measures used to assess latent variables are not valid or reliable, then the results of the analysis will be biased and inaccurate.There are a number of ways to assess cross-validity. One common method is to use a confirmatory factor analysis (CFA). A CFA is a statistical model that tests the relationships between observed and latent variables. By examining the fit of the CFA model, researchers can assess the convergent and discriminant validity of the measures used to assess latent variables.Another method for assessing cross-validity is to use a multitrait-multimethod (MTMM) analysis. A MTMM analysis compares the correlations between different measures of the same latent variable with the correlations betweendifferent measures of different latent variables. By comparing these correlations, researchers can assess the convergent and discriminant validity of the measures used to assess latent variables.Cross-validity is an important aspect of SEM, and it is essential for ensuring the validity and reliability of the results of an SEM analysis. Researchers should carefully assess the cross-validity of the measures used in their SEM analyses to ensure that the results are accurate and reliable.中文回答:结构方程模型(SEM)是一种用于检验和估计观测变量和潜在变量之间关系的统计方法。
加速寿命试验三参数威布尔分布的极小变异-极大似然估计

装备环境工程第20卷第5期·12·EQUIPMENT ENVIRONMENTAL ENGINEERING2023年5月加速寿命试验三参数威布尔分布的极小变异-极大似然估计马小兵,刘宇杰,王晗(北京航空航天大学 可靠性与系统工程学院,北京 100191)摘要:目的在加速试验中,对寿命服从三参数威布尔分布的产品进行可靠性评估与寿命预测,解决形状参数小于1时传统方法难以计算的问题。
方法利用三参数威布尔分布与指数分布之间的转换关系,以变异系数误差最小为优化目标,在确定最优位置参数估计值的基础上,应用拟极大似然方法估计分布模型中的其余参数,建立极小变异–极大似然估计(MV-MLE)。
根据加速寿命试验中失效机理不变的原则,在失效机理等同条件下,将该方法推广至多应力水平下的可靠寿命评估。
结果在单一应力与多应力水平下,通过仿真模拟验证了所提方法的有效性。
与传统方法相比,在小样本条件下,所提方法可提高形状参数(机理等同性参数)估计精度40%以上。
结论所提方法对于三参数威布尔分布的参数估计和寿命评估具有较高精度,能够有效克服传统方法的不足,在加速寿命试验评估中具有良好的应用效果。
关键词:三参数威布尔分布;变异系数;加速寿命试验;机理等同性;可靠性评估;寿命预测中图分类号:TB114 文献标识码:A 文章编号:1672-9242(2023)05-0012-07DOI:10.7643/ issn.1672-9242.2023.05.003Minimum Variation-Maximum Likelihood Estimation of Three-parameterWeibull Distribution under Accelerated Life TestMA Xiao-bing, LIU Yu-jie, WANG Han(School of Reliability and Systems Engineering, Beihang University, Beijing 100191, China)ABSTRACT: The work aims to estimate the reliability and predict the lifetime of the products subject to three-parameter Weibull distribution under accelerated life test, so as to solve the problem that the traditional methods are difficult to complete the calculation when the shape parameter is less than 1. Through the conversion relationship between three-parameter Weibull distribution and exponential distribution, the best estimated value of the location parameter was determined with the error of co-efficient of variation as the optimization objective. Then, the analogue maximum likelihood method was used to estimate the remaining parameters of the Weibull distribution, based on which the minimum variation-maximum likelihood estimation收稿日期:2023–04–13;修订日期:2023–05–04Received:2023-04-13;Revised:2023-05-04基金项目:国家自然科学基金(72201019,52075020);可靠性与环境工程技术重点实验室项目(6142004210105);国防技术基础项目(JSZL2018601B004)Fund:The National Natural Science Foundation of China (72201019, 52075020); Reliability and Environmental Engineering Science & Tech-nology Laboratory (6142004210105); Basic Technical Research Project of China (JSZL2018601B004).作者简介:马小兵(1978—),男,博士。
第一部分听力(共两节,满分30分)

9. what has Barbara got in her suitcase?
A. shoes
B. stones
C. books
听第8段材料,回答第10至12题。
10.Who is making the telephone call?
A.Thomas Brothers.
A so B .much C. that D. it
34. _it’s no use having ideas only .
_don’t worry .peter can show you ________to turn an idea into an act .
第二部分 英语知识运用(共两节,满分45分)
第一节 单项填空(共15小题;每小题1分,满分15分)
从A、B、C、D四个选项中,选出可以填入空白处的最佳选项,并在答题卡上将该项涂黑。
例:We _______ last night, but we went to the concert instead.
25.We were astonished _______ the temple still in its original condition.
A.finding B.to find C.find D.to be found
26.Doctors say that exercise is important for health, but it _______ be regular exercise.
A. it has a large sitting room.
B. it has good furniture
C. ir has a big kitchen.
超实用高考英语复习:专题03 利用词汇复现和逻辑关系进行解题+名校真题针对性训练 (原卷版)
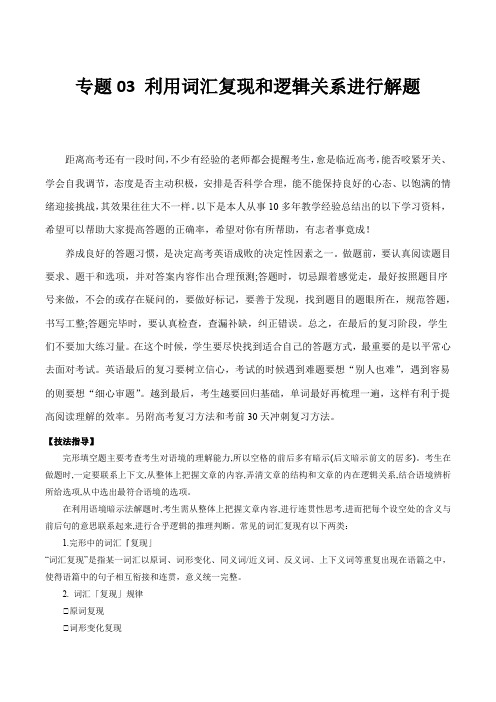
专题03 利用词汇复现和逻辑关系进行解题距离高考还有一段时间,不少有经验的老师都会提醒考生,愈是临近高考,能否咬紧牙关、学会自我调节,态度是否主动积极,安排是否科学合理,能不能保持良好的心态、以饱满的情绪迎接挑战,其效果往往大不一样。
以下是本人从事10多年教学经验总结出的以下学习资料,希望可以帮助大家提高答题的正确率,希望对你有所帮助,有志者事竟成!养成良好的答题习惯,是决定高考英语成败的决定性因素之一。
做题前,要认真阅读题目要求、题干和选项,并对答案内容作出合理预测;答题时,切忌跟着感觉走,最好按照题目序号来做,不会的或存在疑问的,要做好标记,要善于发现,找到题目的题眼所在,规范答题,书写工整;答题完毕时,要认真检查,查漏补缺,纠正错误。
总之,在最后的复习阶段,学生们不要加大练习量。
在这个时候,学生要尽快找到适合自己的答题方式,最重要的是以平常心去面对考试。
英语最后的复习要树立信心,考试的时候遇到难题要想“别人也难”,遇到容易的则要想“细心审题”。
越到最后,考生越要回归基础,单词最好再梳理一遍,这样有利于提高阅读理解的效率。
另附高考复习方法和考前30天冲刺复习方法。
【技法指导】完形填空题主要考查考生对语境的理解能力,所以空格的前后多有暗示(后文暗示前文的居多)。
考生在做题时,一定要联系上下文,从整体上把握文章的内容,弄清文章的结构和文章的内在逻辑关系,结合语境辨析所给选项,从中选出最符合语境的选项。
在利用语境暗示法解题时,考生需从整体上把握文章内容,进行连贯性思考,进而把每个设空处的含义与前后句的意思联系起来,进行合乎逻辑的推理判断。
常见的词汇复现有以下两类:1.完形中的词汇『复现」“词汇复现”是指某一词汇以原词、词形变化、同义词/近义词、反义词、上下义词等重复出现在语篇之中,使得语篇中的句子相互衔接和连贯,意义统一完整。
2. 词汇「复现」规律①原词复现①词形变化复现①同义/近义词复现①反义词复现①上下义词复现【示例】2018 全国| 卷完形填空:During my second year at the city college, I was told that the education department was offering a “free” course, called Thinking Chess, for three credits.I 41 jumped at the idea of taking the class because, after all, who doesn't want to 42 a few dollars? More than that, I'd always wanted to learn chess. And, even if l weren't 43 excited enough about free credits, news about our 44 instructor was appealing enough to me. He was an international grandmaster, which 45 meant I would be learning from one of the game’s 46 I could hardly wait to 47 meet him. I managed to get an A in that 53 and learned life lessons that have served me well beyond the 54 classroom .42. A. waste B.earn C. save D pay46.A.fastest B. easiest C. best D rarest53. A.game B. presentation C.course D,experiment【答案分析】近义词复现。
SiC单晶加工参数优化及表面粗糙度预测

SiC 单晶加工参数优化及表面粗糙度预测李伦1,2,杨少东1,2,李济顺1,2,陈稳1,2(1.河南科技大学河南省机械设计及传动系统重点实验室,河南洛阳471003;2.河南科技大学机电工程学院,河南洛阳471003)来稿日期:2020-01-02基金项目:河南省高等学校重点科研项目计划(17A460013)作者简介:李伦,(1969-),男,河南镇平人,博士研究生,副教授,主要研究方向:硬脆材料加工技术与机械设计仿真分析;杨少东,(1992-),男,河南唐河人,硕士研究生,主要研究方向:硬脆材料加工技术1引言碳化硅(SiC )单晶具有耐高温、导热性强、高电子饱和漂移率、低介电常数、抗冲击强和硬度高等特点,成为航空航天、半导体和微电子等领域制作高频、高温、高功率光电器件的理想材料[1-2]。
电子和光学器件应用SiC 单晶片高性能的同时,对其表面质量具有严格的要求。
SiC 单晶切割作为晶片生产制造过程中的重要工序,晶片表面质量对后续加工成本和晶片的性能具有显著影响[3-4]。
因此,研究线锯切割SiC 单晶的加工参数对线锯锯切力、晶片表面粗糙度的影响规律、以及预测晶片表面粗糙度都具有十分重要的意义。
国内外学者对金刚石线锯切割SiC 单晶等硬脆材料加工参数和锯切力、晶片表面粗糙度的研究有大量的文献报道。
文献[5]利用固结磨料金刚石线锯对SiC 单晶进行切割实验,发现工件进给速度对晶片表面粗糙度及亚表面损伤层影响最大。
文献[6]基于压痕断裂力学分析了硬脆材料表面粗糙度与加工参数的数学模型,结果表明晶片表面粗糙度随工件进给速度增大而增大,随线锯速摘要:因独特的共价键晶体结构,SiC 单晶具有较高的硬度和脆性,是典型的难加工材料。
以横向超声激励线锯的方法对SiC 单晶进行切割,采用正交实验设计,并引入灰色关联分析法研究切割过程中锯切力、晶片表面粗糙度等多目标与主要加工参数之间的影响关系,以及获得线锯加工最优参数组合,即工件进给速度0.025mm/min 、超声振幅1.8μm 、线锯速度1.6m/s 、工件转速16r/min 为最优加工参数组合,并通过实验进行验证。
句子的翻译1

2021/3/11
3
Hypotaxis vs Parataxis
Hypotaxis 形合: The dependent or subordinate construction or relationship of clauses arranged with connectives;
2021/3/11
24
二、比较下列译文。译文a与b哪一种较好?为什么?
1.中国社会主义建设的航船将乘风破浪地驶向现 代化的光辉彼岸。
Domains under heaven, after a long period of division, tends to unite; after a long period of union, tends to divide. This has been so since antiquity. When the rule of the Zhou Dynasty weakened, seven contending kingdoms sprang up*, warring one with another until the kingdom of Qin prevailed and possessed the empire*.
2021/3/11
19
农民缺乏训练,许多农场生产力低下,这就 使得大多数农民处于贫穷的困境。
Inadequate training for farmers and the low productivity of many farmers place the majority of country dwellers in a disadvantageous position in their own countries.
2024届上海市嘉定区高三上学期一模英语试题

2024届上海市嘉定区高三上学期一模英语试题一、听力选择题1. Who is the woman?A.She’s a teacher.B.She’s a student.C.She’s an assistant.2. What’s the weather like now?A.Sunny.B.Windy.C.Rainy.3. What did Smith offer her?A.Smith offered her a room.B.Smith offered her a computer.C.Smith offered her a job as a typist.4. What are the speakers mainly talking about?A.A painting.B.A photo.C.An exhibition.5. Where is the man’s envelope now?A.In his room.B.At the post office.C.At the front desk.二、听力选择题6. 听下面一段较长对话,回答以下小题。
1. What did David do last night?A.He played volleyball.B.He watched television.C.He read the newspaper.2. What time will the match on Saturday afternoon start?A.At 2:30.B.At 3:00.C.At 3:30.3. What will Lisa do first?A.Talk with her mom.B.Give David a call.C.Take a piano lesson.7. 听下面一段较长对话,回答以下小题。
1. What is the woman dissatisfied with about the island?A.The food.B.The hotel.C.The beach.2. What do we know about the woman?A.She lost her way several times.B.She met some unfriendly locals.C.She missed home-cooked meals.3. What does the woman advise the man to do in the end?A.Go to the island of Gozo.B.Taste the local food.C.Visit the churches.8. 听下面一段较长对话,回答以下小题。
冲压模英文翻译原文
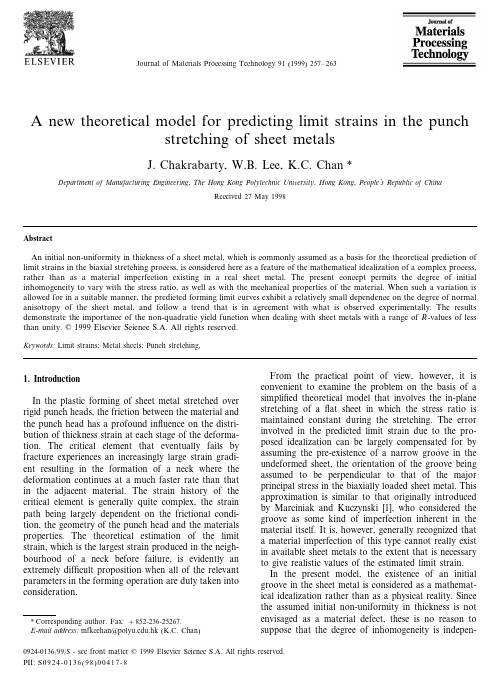
Journal of Materials Processing Technology91(1999)257–263A new theoretical model for predicting limit strains in the punchstretching of sheet metalsJ.Chakrabarty,W.B.Lee,K.C.Chan*Department of Manufacturing Engineering,The Hong Kong Polytechnic Uni6ersity,Hong Kong,People’s Republic of ChinaReceived27May1998AbstractAn initial non-uniformity in thickness of a sheet metal,which is commonly assumed as a basis for the theoretical prediction of limit strains in the biaxial stretching process,is considered here as a feature of the mathematical idealization of a complex process, rather than as a material imperfection existing in a real sheet metal.The present concept permits the degree of initial inhomogeneity to vary with the stress ratio,as well as with the mechanical properties of the material.When such a variation is allowed for in a suitable manner,the predicted forming limit curves exhibit a relatively small dependence on the degree of normal anisotropy of the sheet metal,and follow a trend that is in agreement with what is observed experimentally.The results demonstrate the importance of the non-quadratic yield function when dealing with sheet metals with a range of R-values of less than unity.©1999Elsevier Science S.A.All rights reserved.Keywords:Limit strains;Metal sheets;Punch stretching,1.IntroductionIn the plastic forming of sheet metal stretched over rigid punch heads,the friction between the material and the punch head has a profound influence on the distri-bution of thickness strain at each stage of the deforma-tion.The critical element that eventually fails by fracture experiences an increasingly large strain gradi-ent resulting in the formation of a neck where the deformation continues at a much faster rate than that in the adjacent material.The strain history of the critical element is generally quite complex,the strain path being largely dependent on the frictional condi-tion,the geometry of the punch head and the materials properties.The theoretical estimation of the limit strain,which is the largest strain produced in the neigh-bourhood of a neck before failure,is evidently an extremely difficult proposition when all of the relevant parameters in the forming operation are duly taken into consideration.From the practical point of view,however,it is convenient to examine the problem on the basis of a simplified theoretical model that involves the in-plane stretching of aflat sheet in which the stress ratio is maintained constant during the stretching.The error involved in the predicted limit strain due to the pro-posed idealization can be largely compensated for by assuming the pre-existence of a narrow groove in the undeformed sheet,the orientation of the groove being assumed to be perpendicular to that of the major principal stress in the biaxially loaded sheet metal.This approximation is similar to that originally introduced by Marciniak and Kuczynski[1],who considered the groove as some kind of imperfection inherent in the material itself.It is,however,generally recognized that a material imperfection of this type cannot really exist in available sheet metals to the extent that is necessary to give realistic values of the estimated limit strain.In the present model,the existence of an initial groove in the sheet metal is considered as a mathemat-ical idealization rather than as a physical reality.Since the assumed initial non-uniformity in thickness is not envisaged as a material defect,these is no reason to suppose that the degree of inhomogeneity is indepen-*Corresponding author.Fax:+852-236-25267.E-mail address:mfkcchan@.hk(K.C.Chan)0924-0136/99/$-see front matter©1999Elsevier Science S.A.All rights reserved. PII:S0924-0136(98)00417-8J.Chakrabarty et al./Journal of Materials Processing Technology91(1999)257–263 258dent of the material properties and the stress ratio.As a matter of fact,it will be more realistic to allow the inhomogeneity factor to vary with the relevant physical parameters in an appropriate manner.This means that, for a given planar stress–strain curve of the material, the inhomogeneity factor is a function of the ratio of the applied stresses as well as of the R-value of the sheet metal.The observed discrepancies between the theoretical and experimental limit strains can be mini-mized when suitable variations of the inhomogeneity factor are included in the theoretical framework. Following the work of Marciniak and Kuczynski[1], the theoretical estimation of limit strains in anisotropic sheets has been studied by a number of investigators in the past[2–12],on the basis of the M–K model which assumes the inhomogeneity factor to constitute some kind of material defect.Although the significance of the non-quadratic yield function has been recognized by some authors in relation to low R-value materials,it has been tacitly assumed in the previous investigations that the hypothesis of work equivalence proposed by Hill[13]is universally acceptable for representing the effective stress–strain behaviour of anisotropic materi-als.On the other hand,one of the present authors[14] has demonstrated clearly the appropriateness of the hypothesis of strain equivalence in expressing the hard-ening response of engineering materials.The theoretical curves corresponding to different R-values,according to the present analysis are found to be relatively close to one another,whilst exhibiting a trend that is in accord with the available experimental results.A useful experimental technique for obtaining the forming-limit diagram has been described by Raghavan[15].2.Yield criterion andflow ruleIt is assumed at the outset that the variation of the R-value in the plane of the sheet is small,so that the anisotropy may be regarded as rotationally symmetric about the normal to the plane.It is convenient in this case to express the yield criterion in terms of the uniform R-value and the current uniaxial yield stress|¯in the plane of the sheet.The starting point of the model is Hill’s1979non-quadratic yield criterion[16], which in terms of the principal stresses|1and|2in the plane of the sheet assumes the form:|1+|2 1+m+(1+2R) |1−|2 1+m=2(1+R)|¯1+m(1) where m\0for the yield function to be convex.The yield criterion(Eq.(1))predicts(|b/|¯)1+m=(1+R)/ 2m,where|b denotes the yield stress in equi-biaxial tension.The yield criterion is also capable of explaining an anomalous behaviour observed in low R-value mate-rials[17].Theflow rule associated with Eq.(1)accord-ing to the usual normality rule[18],may be written as: d m1+d m2|1+|2 m=d m1−d m2(1+2R) |1+|2 m=d u(1+R)|¯m(2)which is supplemented by the fact that the signs of d m1+d m2and d m1−d m2are identical to those of|1+|2 and|1−|2,respectively.The increment of plastic work per unit volume corresponding to Eq.(1)and Eq.(2)is given by:2d w=(|1+|2)(d m1+d m2)+(|1−|2)(d m1−d m2) =2|¯d uindicating that the non-negative scalar d u is equal to the equivalent strain increment d m¯in accordance with the hypothesis of work equivalence.The expression for d m¯in terms of the principal components of the strain increment is shown easily to be:2(d m¯)1+v=(1+R){ d m1+d m2 1+v+(1+2R)−1 d m1−d m2 1+v}(3)where v=1/m.For m=v=1,this expression reduces to that corresponding to the quadratic yield function. When the hypothesis of strain equivalence is consid-ered,d u may be regarded as one of the unknowns of the problem.The expression for the equivalent strain increment in this case differs from that for an isotropic material only by a constant factor that depends on the R-value[13],and can be written in the form:d m¯=1+R2!2(d m1+d m2)2+(d m1−d m2)21+R+R2"12(4)for all values of R.The expressions in R in Eq.(4)are such that d m¯equals the lonqitudinal strain increment in the case of a uniaxial tension acting in the plane of the sheet.The parameters m and R occurring in Eq.(1)and Eq.(2)cannot be independent of one another,since m must be unity for an isotropic material(R=1).Available experimental results tend to suggest that it is generally a good approximation to take:m=R(R51),m=1(R]1)It is evident that a wide range of m values cannot be associated with a given R-value when the non-quadratic yield function is appropriate.3.Analysis for the forming limitFollowing Marciniak and Kuczynski[1],it is as-sumed that there is an initial discontinuity in the sheet thickness in the form of a narrow groove,as shown in Fig.1,the direction of the major principal stress|1 being considered as perpendicular to the groove.The initial inhomogeneity factor p B1is defined in such aJ.Chakrabarty et al./Journal of Materials Processing Technology91(1999)257–263259 way that the initial sheet thicknesses outside and insidethe groove are t0and p t0,respectively.The principalstress ratio|%2/|%1outside the groove is maintainedconstant throughout the biaxial stretching,the corre-sponding stress ratio|%2/|%1inside the groove beingallowed to vary in a manner that depends on theapplied stress ratio|%2/|%1for a given material.It isconvenient to introduce dimensionless variables h and idefined as:h=|1−|2|1+|2,i=|%1−|%2|%1+|%2(5)specifying suitable measures of the stress ratios outside and inside the groove.The strain ratio m2/m1outside the groove is taken to be constant,but the ratio m2/m1of the principal strains inside the groove varies continuously during the process.The strain component parallel to the groove is assumed to be continuous,so that the equality:d m2=d m%2holds at each stage.If the compressive thickness strain increment is denoted by d m outside the groove and by d m%inside the groove,then by the condition of plastic incompressibility:d m=d m1+d m2,d m%=d m%1+d m%2The difference between the thickness strain increments inside and outside the groove may be written as:d m−d m%=(d m1+d m2)−(d m%1+d m%2)=(d m1−d m2)−(d m%1−d m%2)(6) in view of the continuity of the minor principal strain components across the groove.As the loading contin-ues,the strain ratio within the groove changes continu-ously until d m%2/d m%1tends to zero,and the subsequent deformation in the groove occurs under condition of plane strain.Considering the associatedflow rule(Eq.(2))in terms of the unprimed and primed components separately:d m−d m=(1+2R)h m d m,d m%−d m%=(1+2R)i m d m%in view of Eq.(5).Substituting these relations into Eq.(6),the ratio of the thickness strain increment is ob-tained asd md m%=1−(1+2R)i m1−(1+2R)h m(7)Writing the yield criterion(Eq.(1))separately for the regions outside and inside the groove,and dividing oneby the other,it is shown easily that:|1+|2|%1+|%21+m!1+(1+2R)h1+m1+(1+2R)h1+m"=|¯|¯%1+m(8)in view of Eq.(5),the current uniaxial yield stress of the material within the groove being denoted by|¯%.The flow rule(Eq.(2))also furnishes the relations(1+r)d md u=|1+|2|¯m,(1+R)d m%d u%=|%1+|%2|¯%m(9)when applied to the material outside and inside the groove.The expressions in the parentheses are obtained directly from Eq.(1)and its primed counterpart.At a generic stage of the biaxial stretching,let the thickness of the sheet outside and inside the groove become t and t%,respectively.Since t|1=t%|%1by the condition of force equilibrium in the direction perpen-dicular to the groove:t%t=(|1+|2)+(|1−|2)(|%1+|%2)+(|%1−|%2)=1+h1+i(|1+|2)(|%1+|%2)where t=t0exp(−m)and t%=p t0exp(−m%),in terms of the initial thickness of the material outside the groove, and the thickness strains inside and outside the groove. Thus:|1+|2|%1+|%2=p1+i1+hexp(m−m%)(10)Combining Eq.(10)and Eq.(8)to eliminate the stress components,and adopting the Swift power law of hardening in the form:|¯=|0(m¯)n,|¯%=|0(c+m¯%)nwhere|0,c and n are material constants,one of the governing equations for the solution to the limit strain problem is obtained as:1+(1+2R)i1+m1+(1+2R)h1+m=!p1+i1+hm¯% exp(m−m%)"1+m(11) Consideringfirst the hypothesis of work equivalent,for which d u=d m¯and d u%=d m¯%,it follows from Eq.(9)and the yield criterion(Eq.(1))thatFig.1.The geometry of a groove.J.Chakrabarty et al./Journal of Materials Processing Technology91(1999)257–263 260d m¯d m =(1+R)!1+(1+2R)h1+m2(1+R)"m1+m,d m¯%d m%=(1+R)!1+(1+2R)i1+m2(1+R)"m1+m(12)If,on the other hand,the hypothesis of strain equiva-lence is adopted,it follows from Eq.(2)and Eq.(4),as well as their primed couterparts,that:d m¯d u =|1+|2|¯m'3+(1+2R)2h2m3+(1+2R)2,d m¯%d u%=|%1+|%2|¯m'3+(1+2R)2i2m3+(1+2R)2These relations are now combined with Eq.(9)to give the required differential relations as:d m¯d m =(1+R)'3+(1+2R)2h2m3+(1+2R)2,d m¯%d m%=(1+R)'3+(1+2R)2i2m3+(1+2R)2(13)Eqs.(7)and(11)and either Eq.(12)or Eq.(13)form aset of four equations forfinding the four unknowns i,m, m¯and m¯%as functions of m¯%,when,h,p,R,m,c and n are given.As explained earlier,a realistic prediction of thelimit strain would require p to vary with the materialproperties and the stress ratio outside the groove.Thesimplest way to allow such a variation is to stipulatethat p is a linear function of h,and depends on theyield stress ratio|¯/|b according to a power law.Sincethe limiting value of the thickness strain is found toapproach the Swift instability strain when m2tends tozero,it is reasonable to assume that:1−p 1−p0=1−hh0|¯|bv(14)where p0is a constant and m is a suitable exponent. The above expression implies that p=1in plane strain,which corresponds to h=h0=(1+2R)−1/m. The constant p0is identified readily as the inhomo-geneity factor in equi-biaxial tension(h0=0)in the case of an isotropic material(h0=|¯).Inserting the value of h0,and using the expression for|¯/|b that follows from Eq.(1),in the special case m=2can bewritten as:p=1−(1−p0)2m 2m1+R21+m 1−(1+2R)−1mh n(15)where m=1for R]1,and m=R for R51.When h=0,Eq.(15)implies that p exceeds p0not only for R]1but also for R B1.The only unknown parameter that appears in Eq.(15)is p0,which can be determined from the measured value of the limit strain for an isotropic sheet under balanced biaxial tension.4.Evaluation of limit strainsFor any given material,for which the mechanical properties are characterised by c,n,R,and m,the analysis can be carried out numerically in a straight-forward manner for a selected value of a and an appropriate value of p0.It is found to be convenient to use selected values of the strain ratio z=m2/m1outside the groove as the basis of the computational work,the relationship between r and a being:h=h01−z1+z1m,h=(1+2R)−1m(16) in view of theflow rule(Eq.(2)).Eq.(15)for the initial inhomogeneity factor may now be written in terms of r as:p=1−(1−p0)2m1+R21+m!1− 1−z1+z1m"(17)When R1,it is neccessary to set=1,and the expres-sion in the curly bracket of Eq.(17)then reduces to 2z/(1+z).The parameter i,which varies continuously during the development of the neck,must be regarded as one of the unknowns of the problem.The numerical analysis can be reduced to the solu-tion of three simultaneousfirst order differential equa-tions involving m%as the independent variable,and m%,i and m¯%as the dependent ing Eqs.(7),(11) and(12)and Eq.(13),the set of three governing differ-ential equations can be written asd md m%=1+z2z[1−(1+2R)i m](18) d m¯%d m%=(1+R)x(19) d id m%=(1+i)[1+(1+2R)i1+m]! 1−n(1+R)c+m¯%n[1−(1+2R)i m]−1−1+z2z1−nkc+k m"(20) where:x=!1+(1+2R)i1+m2(1+R)"m1+m(21a) according to the hypothesis of work equivalence,and:x=12!3+(1+2R)2i2m2+R+R2"12(21b)according to the hypothesis of strain equivalence,while k denotes the expression on the right-hand side of either Eq.(12)or Eq.(13).Thus:J .Chakrabarty et al ./Journal of Materials Processing Technology 91(1999)257–263261k =1+R 2m 11+m!1+h 01−z1+z1+m m"m 1+m(22a)according to the hypothesis of work equivalence,and:k =1+R 1+z'1+z +z 21+R +R 2(22b)according to the hypothesis of strain equivalence.The differential equation for i ,expressed by Eq.(18),is obtained easily by the logarithmic differentiation of Eq.(11)with respect to m %,using Eqs.(7),(12)and (13)and Eq.(16).The integration of the set of differential equations Eqs.(18)–(20)can be carried out numerically using the initial conditions m =m ¯%=0and i =i 0=0when m %=0,where i 0is given by Eq.(11)and Eq.(16)as the solution of the equation:1+(1+2R )i01+m (1+i )1+m=p 1+m !1+h 01−z 1+z1m"−(1+m )!1+h 01−z 1+z1+m m"(23)The inhomogeneity factor p is expressed by Eq.(15)in terms of p 0,R ,and m .Once the thickness strain e is known at any stage,the corresponding values of princi-pal surface strains m 1and m 2follow from the relation:m 1=m 1+z,m 2=mz 1+z(24)During the continued straining of the material,thedeformation of the material within the groove proceeds at a much faster rate than that outside the groove,and the strain increment ratio d m /d m %decreases rapidly.The limiting state is characterised by d m /d m %tending to zero,defining a state of incremental plane-strain of the mate-rial in the groove d m 2=0in view of the coninuity of the strain component parallel to the groove.The solution is therefore terminated when i is sufficiently close to the limiting value h 0.In the special case of h =h 0,then i =h 0throughout the deformation,and the limiting state then corresponds to plastic instability of the mate-rial in the groove,giving m =m %=n for all values of R ,the assumed inhomogeneity factor being exactly unity under conditions of plane-strain.Over a range of stress ratios in the neighbourhood of the plane-strain state (z =0),some deformation would occur in the groove with i =h 0before the material outside the groove reaches the yield point.This changes the initial condition for the problem of simultaneous straining of the material inside and outside the groove leading to the limiting state,which is attained when i approaches h 0again after undergoing some variation [3].When the R -value is sufficiently small,the quadratic yield function is found to give unusually high limit strains [3],whilst the more appropriate non-quadratic yield function seems to predict exceptionally low valuesFig.2.Predicted limit strains based on a constant inhomogeneity factor (p =0.98).of the limit-strain [4].It is reasonable,therefore,to consider a different criterion for failure that would apply in situations where the M–K model seems to be unsatisfactory.As a simple alternative criterion,it is stipulated that the load acting in the direction of the major principal stress attains a stationary value in the limiting state,the initial inhomogeneity in the sheet being assumed to be absent.This condition furnishes the relation d |1/|1=d m 1at the onset of failure,and in view of the assumed constancy of the stress ratio:d |¯|¯=d m 1,or1|¯d |¯d m ¯=m 1m Using the strain-hardening law |¯=|0(c +m ¯)n ,and re-membering that m ¯=k (1+z )m 1,the limit strains in this case are obtained as:m 1=n −ck (1+z ),m 2=zm 1(25)where k is given by Eqs.(22a)and (22b).The above formula includes the plane-strain case (z =0).The ma-jor limit-strain,according to Eq.(25)differs from n by an amount that vanishes when c =0.The effect of the hardening hypothesis,appearing through the constant k ,would be negligible for the usual values of c .5.Discussion of resultsIn order to estimate the influence of the hardening hypothesis on the magnitude of the limit strain,the first part of the numerical computation has been based on a constant inhomogeneity factor p =p 0.In the case of a plane sheet under biaxial stretching,the equivaluent plastic strain at instability depends on the choice of hardening hypothesis,but the principal surface strains at instability are unaffected [12].The forming limit curves for several R -values are plotted in Fig.2,using p =0.98,n =0.2and c =0.01.It is evident that theJ.Chakrabarty et al./Journal of Materials Processing Technology91(1999)257–263 262hardening hypothesis is without effect on the limitcurves except for the one corresponding to R=0.5.For this particular value of R,the quadratic yieldcriterion,which is inappropriate for low R-value ma-terials,gives exceedingly high values of the limitstrain,the derived curve being in agreement with thatreported by Sowerby and Duncan[3].The non-quadratic yield function,on the other hand,givesvery low values of the limit strain when R=0.5,forboth the hypotheses of the work and strain equiva-lence.It is apparent,therefore,that the M–K modelneeds to be modified for low R-value materials insuch as way that the extreme sensitivity of the limitstrain on the choice of yield function is eliminated.The results of the computation based on an inhomo-geneity factor that varies with the stress ratio accord-ing to Eq.(17)are displayed in Fig.3,using the same values of p0and n as those used in the deriva-tion of Fig.2.The initial part of the limit curve for each value of R]1is based on the maximum load criterion and is given by Eq.(25).The latter model for the sheet failure,which is found to be appropriate for all values of z when the R-value of the material is less than about0.72,is represented by a horizontal solid line in Fig.3.The limit curves falling below this line,derived on the basis of the modified M–K model,do not seem to be realistic,since the predicted limit strain decreases with increasing values of z.The trend exhibited by the limit strain curves represented by the proposed theoretical model is more in accord with experimental curves.If the value of p0is Eq.(17)is selected appropriately in any particular situa-tion,quantitative agreement between the theoretical and experimental curves can be achieved.Fig.4 shows the variation of the parameter i,which latter is a measure of the stress ratio within the groove,as the thickness strain increases to the limiting value in the particular case of balanced biaxial tension(z=1).Fig.4.Variation of i with the thickness strain. Similar variations of i with m are noted for other values of z\0.6.ConclusionsThe M–K model for the theoretical estimation of limit strains is modified in this work to allow a varia-tion of the material inhomogeneity with the stress ra-tio as well as with the R-value of the material.The equation that defines the variation is such that the inhomogeneity factor has its smallest value under bal-anced biaxial tension,whilst becomong unity in the plane-strain state.The general trend exhibited by the limit strain curves,accdording to the modified M–K model,is found to be more in accord with the experi-mental limit curves.Since neither the quardatic yield function nor the non-quadratic yield function satisfac-torily predicts the limit strain for low R-value materi-als on the basis of the M–K model,a stationary load criterion is proposed in this paper to define a lower bound on the limit strain,the range of applicability of which increases with decreasing R-values. References[1]Z.Marciniak,K.Kuczynski,Int.J.Mech.Sci.9(1967)609.[2]Z.Marciniak,K.Kuczynski,T.Pakora,Int.J.Mech.Sci.15(1973)789.[3]R.Sowerby,J.L.Duncan,Int.J.Mech.Sci.13(1971)217.[4]A.Parmar,P.B.Mellor,Int.J.Mech.Sci.20(1978)707.[5]K.W.Neale,E.Chater,Int J.Mech.Sci.22(9)(1980)563.[6]C.C.Chu,Int.J.Solids Struct.18(1984)205.[7]K.S.Chan,Metall.Trans.16A(1985)629.[8]J.Lian,F.Barlat,B.Baudelet,Int.J.Plast.5(1989)131.[9]A.Graf,W.F.Hosford,Metall.Trans.21A(1990)87.[10]L.Zhao,R.Sowerby,M.R.Sklad,Int.J.Mech.Sci.38(1996)1307.[11]J.Chakrabarty,in:Proceedings of the32nd InternationalMATADOR Conference,Manchester,UK,(1997),p.373.Fig.3.Predicted limit strains based on an inhomogeneity factor that varies with the stress ratio(p0=0.98).J.Chakrabarty et al./Journal of Materials Processing Technology91(1999)257–263263[12]W.B.Lee,K.C.Chan,Textures Microstruct.14–18(1991)1221.[13]R.Hill,The Mathematical Theory of Plasticity,Clarendon Press,Oxford,1950.[14]J.Chakrabarty,Int.J.Mech.Sci.12(1970)169.[15]K.S.Raghavan,Metall.Trans.26A(1995)2075.[16]R.Hill,Math.Proc.Cam.Phil.Soc.85(1979)179.[17]J.Woodthorpe,R.Pearce,Int.J.Mech.Sci.12(1970)341.[18]J.Chakrabarty,Theory of Plasticity,McGraw–Hill,New York,1987..。
大学生反刍思维和述情障碍的关系:孤独感和社交焦虑的多重中介作用
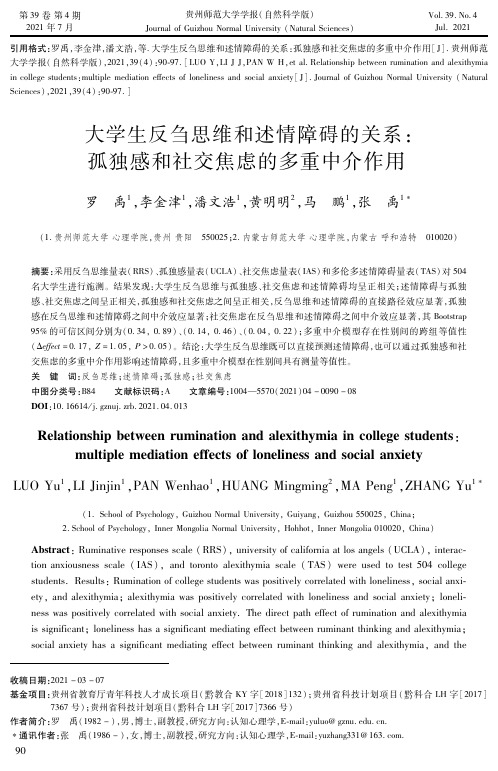
担忧等负性情绪的体验[16-18]。赵燕[19]研究显示, 社交焦虑已成为大学生精神疾病的一个重要心理 威胁因素。 马 俊 军 等[9]研 究 发 现,作 为 负 性 反 应 风格的反刍思维与社交焦虑存在正相关关系。反 刍思维作为一种非适应性反应风格,是引发、保持 和增强社交焦虑的重要因素 。 [17] 高反刍思维个体 会不断回忆人际交往过程中的消极体验,产生负性 自我评价和社交焦虑[20-21]。严重社交焦虑症障碍 患者普遍表 现 出 高 水 平 的 述 情 障 碍 问 题 [22],高 社 交焦虑被 试 存 在 明 显 的 述 情 障 碍 特 征[15]。 据 此, 本研究提出假设 H1:社交焦虑在反刍思维和述情 障碍的之间起中介作用。
0 引言
述情障碍 (Alexithymia),又 称 情 感 表 达 不 能, 表现为个体无法识别自身和他人的情绪、描述情绪 困难以及缺乏想象力等状况,是一种反映个体在情 绪认知和 情 绪 调 节 方 面 存 在 缺 陷 的 人 格 特 征[1]。 述情障碍个体在面对压力事件时因无法有效进行 情绪调节,可能会产生抑郁、躯体化、偏执等精神问 题[2]。张春雨等[3]研究显示:受文化氛围影响,中 国个体的情绪表达普遍含蓄、内向,易出现述情障 碍;受性别社会化影响,男性述情障碍得分普遍高 于女性,男性会更依赖自身的情绪感受,进而压抑 负性情绪,而不是表达自身的情绪。
90
Байду номын сангаас
第 4期
罗 禹,李金津,潘文浩,等:大学生反刍思维和述情障碍的关系:孤独感和社交焦虑的多重中介作用
人工智能原理MOOC习题集及答案北京大学王文敏课件
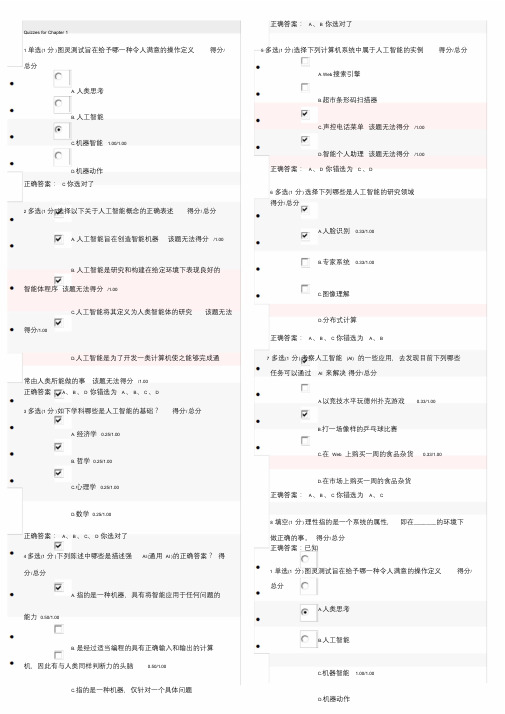
正确答案:A、B 你选对了Quizzes for Chapter 11 单选(1 分)图灵测试旨在给予哪一种令人满意的操作定义得分/ 5 多选(1 分)选择下列计算机系统中属于人工智能的实例得分/总分总分A. Web搜索引擎A. 人类思考B.超市条形码扫描器B. 人工智能C.声控电话菜单该题无法得分/1.00C.机器智能 1.00/1.00D.智能个人助理该题无法得分/1.00正确答案:A、D 你错选为C、DD.机器动作正确答案: C 你选对了6 多选(1 分)选择下列哪些是人工智能的研究领域得分/总分2 多选(1 分)选择以下关于人工智能概念的正确表述得分/总分A.人脸识别0.33/1.00A. 人工智能旨在创造智能机器该题无法得分/1.00B.专家系统0.33/1.00B. 人工智能是研究和构建在给定环境下表现良好的智能体程序该题无法得分/1.00C.图像理解C.人工智能将其定义为人类智能体的研究该题无法D.分布式计算得分/1.00正确答案:A、B、C 你错选为A、BD.人工智能是为了开发一类计算机使之能够完成通7 多选(1 分)考察人工智能(AI) 的一些应用,去发现目前下列哪些任务可以通过AI 来解决得分/总分常由人类所能做的事该题无法得分/1.00正确答案:A、B、D 你错选为A、B、C、DA.以竞技水平玩德州扑克游戏0.33/1.003 多选(1 分)如下学科哪些是人工智能的基础?得分/总分B.打一场像样的乒乓球比赛A. 经济学0.25/1.00C.在Web 上购买一周的食品杂货0.33/1.00B. 哲学0.25/1.00D.在市场上购买一周的食品杂货C.心理学0.25/1.00正确答案:A、B、C 你错选为A、CD.数学0.25/1.008 填空(1 分)理性指的是一个系统的属性,即在_________的环境下正确答案:A、B、C、D 你选对了做正确的事。
得分/总分正确答案:已知4 多选(1 分)下列陈述中哪些是描述强AI (通用AI )的正确答案?得1 单选(1 分)图灵测试旨在给予哪一种令人满意的操作定义得分/ 分/总分总分A. 指的是一种机器,具有将智能应用于任何问题的A.人类思考能力0.50/1.00B.人工智能B. 是经过适当编程的具有正确输入和输出的计算机,因此有与人类同样判断力的头脑0.50/1.00C.机器智能 1.00/1.00C.指的是一种机器,仅针对一个具体问题D.机器动作正确答案: C 你选对了D.其定义为无知觉的计算机智能,或专注于一个狭2 多选(1 分)选择以下关于人工智能概念的正确表述得分/总分窄任务的AIA. 人工智能旨在创造智能机器该题无法得分/1.00B.专家系统0.33/1.00B. 人工智能是研究和构建在给定环境下表现良好的C.图像理解智能体程序该题无法得分/1.00D.分布式计算C.人工智能将其定义为人类智能体的研究该题无法正确答案:A、B、C 你错选为A、B得分/1.00 7 多选(1 分)考察人工智能(AI) 的一些应用,去发现目前下列哪些任务可以通过AI 来解决得分/总分D.人工智能是为了开发一类计算机使之能够完成通A.以竞技水平玩德州扑克游戏0.33/1.00常由人类所能做的事该题无法得分/1.00正确答案:A、B、D 你错选为A、B、C、DB.打一场像样的乒乓球比赛3 多选(1 分)如下学科哪些是人工智能的基础?得分/总分C.在Web 上购买一周的食品杂货0.33/1.00A. 经济学0.25/1.00D.在市场上购买一周的食品杂货B. 哲学0.25/1.00正确答案:A、B、C 你错选为A、CC.心理学0.25/1.008 填空(1 分)理性指的是一个系统的属性,即在_________的环境下D.数学0.25/1.00 做正确的事。
统计建模中的分类响应回归模型及glmcat包的教程说明书

A tutorial on fitting Generalized Linear Models for categoricalresponses with the glmcat packageLorena León ∗Jean Peyhardi †Catherine Trottier ‡AbstractIn statistical modeling,there is a wide variety of regression models for categorical responses.Yet,no software encapsulates all of these models in a standardized format.We introduce and illustrate the utility of glmcat,the R package we developed to estimate generalized linear models implemented under the unified specification (r,F,Z ),where r represents the ratio of probabilities (reference,cumulative,adjacent,or sequential),F the cumulative cdf function for the linkage,and Z the design matrix.We present the properties of the four families of models,which must be investigated when selecting the components r ,F ,and Z .The functions are user-friendly and fairly intuitive;offering the possibility to choose from a large range of models through a combination (r,F,Z ).Introduction to the (r,F,Z)methodology:A generalized linear model is characterized by three components:1)the random component that defines the conditional cdf of the response variable Y i given the realization of the explanatory variables x i ;2)the systematic component which is determined by the linear predictor η(that specifies the linear entry of the independent variables),and 3)the link function g that relates the expected response and the linear predictor.The random component of a GLM for a categorical response with J categories is the multinomial cdf with vector of probabilities (π1,...,πJ )where πr =1.The linear predictor (η1,...,ηJ −1)can be written as the product of the design matrix Z and the unknown parameter vector β.The link function which characterizes this model is given by the equation g (π)=Zβ,with J −1equations g j =ηj .Peyhardi,Trottier,and Guédon (2015)proposed to write the link function asg j =F −1◦r j ⇔r j =F (ηj )j =1,2,...,J −1(1)where F is a cumulative cdf function and r =(r 1,...,r J −1)is a transformation of the expected value vector.In the following,we will describe in more details the components (r,F,Z)and their modalities.Ratio of probabilities rThe linear predictor is not directly related to the expectation πinstead they are related through a par-ticular transformation r of the vector πwhich is called the ratio.Peyhardi,Trottier,and Guédon (2015)proposed four ratios that gather the alternatives to model categorical response data:Cumulative SequentialAdjacentReference r j (π)π1+...+πjπjπj +...+πJ πjπj +πj +1πj πj +πJ Yordinalnominal∗Universitéde Montpellier,**********************†Universitéde Montpellier,*****************************‡Universitéde Montpellier,**********************************Each component r j(π)can be viewed as a(conditional)probability.For the reference ratio,each category j is compared to the reference category J.For the adjacent ratio,each category j is compared to its adjacent category j+1.For the cumulative ratio,the probabilities of categories are cumulated.For the sequential ratio,each category j is compared to its following category,j+1,...,J.The adjacent,cumulative and sequential ratios all rely on an ordering assumption among categories.The reference ratio is devoted to nominal responses.Cumulative cdf function FThe cumulative cdf functions(distributions)available in glmcat tofit the models are:logistic,normal, Cauchy,Student(with any df),Gompertz and Gumbel.The logistic and normal distributions are the sym-metric distributions most commonly used to define link functions in generalized linear models.However,for specific scenarios,the use of other distributions may result in a more accuratefit.An example is presented by Bouscasse,Joly,and Peyhardi(2019),where the employment of the Student cdf leaded to a betterfit for a modeling exercise on travel choice data.For the asymmetric case,the Gumbel and Gompertz distributions are the most commonly used.Design Matrix ZIt is possible to impose restrictions on the thresholds,or on the effects of the covariates,for example,for them to vary or not according to the response categories.•Constraints on the effects:It is plausible for a predictor to have specific level of impact on the different categories of the response. Thus,the J−1linear predictors are of the form:ηj=αj+x′δj withβ=(α1,...,αJ−1,δ′1,...,δ′J−1).And, its associated design matrix is:Z c=1x t......1x t(J−1)×(J−1)(1+p)(2)\end{equation}Another case is to constrain the effects of the covariates to be constant across the response categories.Therefore,there is only a global effect that is not specific to the response categories,this is known as the parallelism assumption,for which the constrained space is represented by:Z p=1x t......1x t(J−1)×(J−1+p)(3)Thefirst case(Z c)is named by Peyhardi,Trottier,and Guédon(2015)as the complete design,whereas the second(Z p)as the parallel design.These two matrices are sufficient to define all the classical models.A third option is to consider both kind of effects,complete and parallel,this in known as partial parallel designZ=1x t k x t l.........1x t k x t l(J−1)×((J−1)(1+K)+L)(4)\end{equation}•Constraints on the intercepts:For the particular case of the cumulative ratio the equidistant constraint considers that the distances between adjacent intercepts are the same for all the pairs(j,j+1),therefore we can write the intercepts asαj=α1+(j−1)θ(5) this restriction implies that only two parameters(α1,thefirst threshold,and,θthe spacing)have to be estimated regardless the number of categories.All the classical models for categorical response data,can be written as an(r,F,Z)triplet,as examples:•The multinomimal model≡(Reference,Logistic,Complete)•The odds parallel logit model≡(Cumulative,Logistic,parallel)•The parallel hazard model≡(Sequential,Gompertz,parallel)•The continuation ratio logit model≡(Sequential,Logistic,Complete)•The adjacent logit model≡(Adjacent,Logistic,Complete)Fitting(r,F,Z)with the glmcat packageFamily of reference modelsWe used the223observations of the boy’s disturbed dreams benchmark dataset drawn from a study that cross-classified boys by their age x and the severity of their disturbed dreams y(Maxwell1961).The data is available as the object DisturbedDreams in the package glmcat.For more information see the manual entry for the DisturbedDreams data:help(DisturbedDreams).#devtools::load_all()library(GLMcat)data("DisturbedDreams")summary(DisturbedDreams)##Age Level##Min.:6.00Not.severe:100##1st Qu.:8.50Severe.1:42##Median:10.50Severe.2:41##Mean:10.96Very.severe:40##3rd Qu.:12.50##Max.:14.50We willfit the model(Reference,Logistic,Complete)to the DisturbedDreams data using the function glmcat.We save thefitted glmcat model in the object mod_ref_log_c and we print it by simply typing its name:DisturbedDreams$Level<-as.factor(as.character(DisturbedDreams$Level))mod_ref_log_c<-glmcat(formula=Level~Age,ratio="reference",cdf="logistic",ref_category="Very.severe",data=DisturbedDreams)The most common R functions which describe different model features are available for the objects in glmcat •The summary of the object:summary(mod_ref_log_c)##Level~Age##ratio cdf nobs niter logLik##Model info:reference logistic2235-277.1345##Estimate Std.Error z value Pr(>|z|)##(Intercept)Not.severe-2.454440.84559-2.9030.0037**##(Intercept)Severe.1-0.554640.89101-0.6220.5336##(Intercept)Severe.2-1.124640.91651-1.2270.2198##Age Not.severe0.309990.07804 3.9727.13e-05***##Age Severe.10.059970.085820.6990.4847##Age Severe.20.112280.08684 1.2930.1960##---##Signif.codes:0’***’0.001’**’0.01’*’0.05’.’0.1’’1•The number or observations:nobs(mod_ref_log_c)##[1]223•The coefficients of the modelcoef(mod_ref_log_c)##[,1]##(Intercept)Not.severe-2.45443827##(Intercept)Severe.1-0.55463962##(Intercept)Severe.2-1.12464112##Age Not.severe0.30998759##Age Severe.10.05997162##Age Severe.20.11228063•The LogLikelihoodlogLik(mod_ref_log_c)##’log Lik.’-277.1345(df=6)•Information criteriaAIC(mod_ref_log_c)##[1]566.2691BIC(mod_ref_log_c)##[1]586.7121It is possible to do predictions in glmcat using the function predict_glmcat.We are going to predict the response for3random observations:#Random observationsset.seed(13)ind<-sample(x=1:nrow(DisturbedDreams),size=3)#Probabilitiespredict(mod_ref_log_c,newdata=DisturbedDreams[ind,],type="prob")##Not.severe Severe.1Severe.2Very.severe##[1,]0.53920490.15833210.17218260.1302804##[2,]0.18322070.27325190.21150460.3320229##[3,]0.29964140.23918790.21100340.2501674#Linear predictorpredict(mod_ref_log_c,newdata=DisturbedDreams[ind,],type="linear.predictor")##Not.severe Severe.1Severe.2##[1,] 1.42040660.195005660.2788668##[2,]-0.5945128-0.19480988-0.4509573##[3,]0.1804562-0.04488083-0.1702558Now we illustrate how to predict in a set of new observations.Suppose we want to predict the severity of dreams for3individuals whose ages are5,9.5and15respectively:#New data#Age<-c(5,9.5,15)#predict(mod_ref_log_c,newdata=Age,type="prob")Assume that we are interested in making the effect of the predictor variable parallel,to that end,we type the name of the predictor variable as the input for the parameter parallel.The model tofit corresponds to the triplet(Reference,Logistic,parallel):#DisturbedDreams$Level<-as.factor(as.character(DisturbedDreams$Level))#mod2<-glmcat(#formula=Level~Age,cdf="logistic",#parallel="Age",ref_category="Very.severe",#data=DisturbedDreams#)#summary(mod2)#logLik(mod2)Another variation of the reference model is obtained at changing the cdf function.Let’s nowfit the model (Reference,Student(0.5),Complete):#DisturbedDreams$Level<-as.factor(as.character(DisturbedDreams$Level))#mod3<-glmcat(#formula=Level~Age,ref_category="Very.severe",#data=DisturbedDreams,cdf=list("student",0.5)#)#summary(mod3)#logLik(mod3)Family of adjacent modelsThe equivalence between(Adjacent,Logistic,Complete)and(Reference,Logistic,Complete)models is shown by comparing the associated LogLikelihood of both models:logLik(mod_ref_log_c)#recall(ref,logit,com)##’log Lik.’-277.1345(df=6)mod_adj_log_c<-glmcat(formula=Level~Age,ratio="adjacent",data=DisturbedDreams,cdf="logistic")##Warning in glmcat(formula=Level~Age,ratio="adjacent",data=##DisturbedDreams,:The response variable is not defined as an ordered variable.##Recall that the the reference ratio is appropiate for nominal responses,while##for ordinal responses the ratios to use are cumulative,sequential or adjacent.logLik(mod_adj_log_c)##’log Lik.’-279.5628(df=4)summary(mod_adj_log_c)##Level~Age##ratio cdf nobs niter logLik##Model info:adjacent logistic2235-279.5628##Estimate Std.Error z value Pr(>|z|)##(Intercept)Not.severe-0.236110.33657-0.7020.48297##(Intercept)Severe.1-1.018750.33535-3.0380.00238**##(Intercept)Severe.2-0.954640.31524-3.0280.00246**##Age0.097300.02405 4.0455.23e-05***##---##Signif.codes:0’***’0.001’**’0.01’*’0.05’.’0.1’’1Remark that despite the fact that the LogLikelihoods are equal,the parameters estimations are different (α=α′).Defining the matrix A T as follows:A T=100−1100−11we can check that A T∗α=α′.Note:The adjacent models are stable under the reverse permutation.(Adjacent,Cauchy,Complete)mod_adj_cau_c<-glmcat(formula=Level~Age,ratio="adjacent",cdf="cauchy",categories_order=c("Not.severe","Severe.1","Severe.2","Very.severe"),data=DisturbedDreams)##Warning in glmcat(formula=Level~Age,ratio="adjacent",cdf="cauchy",: ##The response variable is not defined as an ordered variable.Recall that the the ##reference ratio is appropiate for nominal responses,while for ordinal responses ##the ratios to use are cumulative,sequential or adjacent.logLik(mod_adj_cau_c)##’log Lik.’-280.116(df=4)summary(mod_adj_cau_c)##Level~Age##ratio cdf nobs niter logLik##Model info:adjacent cauchy2236-280.116##Estimate Std.Error z value Pr(>|z|)##(Intercept)Not.severe-0.180050.28499-0.6320.527526##(Intercept)Severe.1-0.832970.29215-2.8510.004356**##(Intercept)Severe.2-0.783600.26287-2.9810.002874**##Age0.080080.02083 3.8450.000121***##---##Signif.codes:0’***’0.001’**’0.01’*’0.05’.’0.1’’1(Adjacent,Cauchy,Complete)with reversed ordermod_adj_cau_c_rev<-glmcat(formula=Level~Age,ratio="adjacent",cdf="cauchy",categories_order=c("Very.severe","Severe.2","Severe.1","Not.severe"),data=DisturbedDreams)##Warning in glmcat(formula=Level~Age,ratio="adjacent",cdf="cauchy",: ##The response variable is not defined as an ordered variable.Recall that the the ##reference ratio is appropiate for nominal responses,while for ordinal responses ##the ratios to use are cumulative,sequential or adjacent.logLik(mod_adj_cau_c_rev)##’log Lik.’-280.116(df=4)summary(mod_adj_cau_c_rev)##Level~Age##ratio cdf nobs niter logLik##Model info:adjacent cauchy2236-280.116##Estimate Std.Error z value Pr(>|z|)##(Intercept)Very.severe0.783600.26287 2.9810.002874**##(Intercept)Severe.20.832970.29215 2.8510.004356**##(Intercept)Severe.10.180050.284990.6320.527526##Age-0.080080.02083-3.8450.000121***##---##Signif.codes:0’***’0.001’**’0.01’*’0.05’.’0.1’’1The LogLikelihoods of the last two models are the same,this is because the Cauchy cdf is symmetric;for non symmetric distributions this is not longer true.Note that if the Gumbel cdf is used with the reverse order,then,its LogLikelihood is equal to the model using Gompertz as the cdf,this is because the Gumbel cdf is the symmetric of the Gompertz cdf.Otherwise,the parameter estimations are reversed: (Adjacent,Gumbel,parallel)adj_gumbel_p<-glmcat(formula=Level~Age,ratio="adjacent",cdf="gumbel",categories_order=c("Not.severe","Severe.1","Severe.2","Very.severe"),parallel=c("(Intercept)","Age"),data=DisturbedDreams)##Warning in glmcat(formula=Level~Age,ratio="adjacent",cdf="gumbel",:##The response variable is not defined as an ordered variable.Recall that the the##reference ratio is appropiate for nominal responses,while for ordinal responses##the ratios to use are cumulative,sequential or adjacent.logLik(adj_gumbel_p)##’log Lik.’-284.0416(df=2)summary(adj_gumbel_p)##Level~Age##ratio cdf nobs niter logLik##Model info:adjacent gumbel2235-284.0416##Estimate Std.Error z value Pr(>|z|)##(Intercept)-0.280230.20340-1.3780.168##Age0.083850.01909 4.3921.13e-05***##---##Signif.codes:0’***’0.001’**’0.01’*’0.05’.’0.1’’1(Adjacent,Gompertz,parallel)adj_gompertz_rev<-glmcat(formula=Level~Age,ratio="adjacent",cdf="gompertz",categories_order=c("Very.severe","Severe.2","Severe.1","Not.severe"),parallel=c("(Intercept)","Age"),data=DisturbedDreams)##Warning in glmcat(formula=Level~Age,ratio="adjacent",cdf="gompertz",:##The response variable is not defined as an ordered variable.Recall that the the##reference ratio is appropiate for nominal responses,while for ordinal responses##the ratios to use are cumulative,sequential or adjacent.logLik(adj_gompertz_rev)##’log Lik.’-284.0416(df=2)summary(adj_gompertz_rev)##Level~Age##ratio cdf nobs niter logLik##Model info:adjacent gompertz2235-284.0416##Estimate Std.Error z value Pr(>|z|)##(Intercept)0.280230.20340 1.3780.168##Age-0.083850.01909-4.3921.13e-05***##---##Signif.codes:0’***’0.001’**’0.01’*’0.05’.’0.1’’1Family of sequential modelsThe sequential ratio,which assumes a binary process at each transition,higher levels can be reached only if previous levels where reached at a earlier stage.(Sequential,Normal,Complete)seq_probit_c<-glmcat(formula=Level~Age,ratio="sequential",cdf="normal",data=DisturbedDreams)##Warning in glmcat(formula=Level~Age,ratio="sequential",cdf="normal",:##The response variable is not defined as an ordered variable.Recall that the the##reference ratio is appropiate for nominal responses,while for ordinal responses##the ratios to use are cumulative,sequential or adjacent.logLik(seq_probit_c)##’log Lik.’-280.5465(df=4)summary(seq_probit_c)##Level~Age##ratio cdf nobs niter logLik##Model info:sequential normal2236-280.5465##Estimate Std.Error z value Pr(>|z|)##(Intercept)Not.severe-1.203130.27969-4.3021.69e-05***##(Intercept)Severe.1-1.413470.28345-4.9876.14e-07***##(Intercept)Severe.2-0.983930.28252-3.4830.000496***##Age0.097520.02414 4.0395.36e-05***##---##Signif.codes:0’***’0.001’**’0.01’*’0.05’.’0.1’’1Family of cumulative models(Cumulative,Logistic,Complete)cum_log_co<-glmcat(formula=Level~Age,cdf="logistic",ratio="cumulative",data=DisturbedDreams)##Warning in glmcat(formula=Level~Age,cdf="logistic",ratio=##"cumulative",:The response variable is not defined as an ordered variable.##Recall that the the reference ratio is appropiate for nominal responses,while##for ordinal responses the ratios to use are cumulative,sequential or adjacent.logLik(cum_log_co)##’log Lik.’-278.4682(df=4)summary(cum_log_co)##Level~Age##ratio cdf nobs niter logLik##Model info:cumulative logistic2236-278.4682##Estimate Std.Error z value Pr(>|z|)##(Intercept)Not.severe-2.606390.56166-4.6403.48e-06***##(Intercept)Severe.1-1.781570.54641-3.2600.00111**##(Intercept)Severe.2-0.777140.53923-1.4410.14953##Age0.218750.04949 4.4209.86e-06***##---##Signif.codes:0’***’0.001’**’0.01’*’0.05’.’0.1’’1The function glmcat has special features for the cumulative models.The option for the thresholds to be equidistant is a characteristic of interest for the family of cumulative models:(Cumulative,Logistic,Equidistant)cum_log_co_e<-glmcat(formula=Level~Age,cdf="logistic",ratio="cumulative",data=DisturbedDreams,parallel="Age",threshold="equidistant",)##Warning in glmcat(formula=Level~Age,cdf="logistic",ratio=##"cumulative",:The response variable is not defined as an ordered variable.##Recall that the the reference ratio is appropiate for nominal responses,while##for ordinal responses the ratios to use are cumulative,sequential or adjacent.logLik(cum_log_co_e)##’log Lik.’-278.892(df=3)summary(cum_log_co_e)##Level~Age##ratio cdf nobs niter logLik##Model info:cumulative logistic2236-278.892##Estimate Std.Error z value Pr(>|z|)##(Intercept)Not.severe-2.637690.56148-4.6982.63e-06***##(Intercept)distance0.903660.0886010.199<2e-16***##Age0.219950.04947 4.4468.75e-06***##---##Signif.codes:0’***’0.001’**’0.01’*’0.05’.’0.1’’1If we have a preliminary idea of the coefficients of the model,we can specify an initialization vector through the parameter beta_init:cum_log_c<-glmcat(formula=Level~Age,cdf=list("student",0.8),ratio="cumulative",data=DisturbedDreams,control=control_glmcat(beta_init=coef(cum_log_co)))##Warning in glmcat(formula=Level~Age,cdf=list("student",0.8),ratio=##"cumulative",:The response variable is not defined as an ordered variable.##Recall that the the reference ratio is appropiate for nominal responses,while##for ordinal responses the ratios to use are cumulative,sequential or adjacent.logLik(cum_log_c)##’log Lik.’-280.5428(df=4)summary(cum_log_c)##Level~Age##ratio cdf nobs niter logLik##Model info:cumulative student2237-280.5428##Estimate Std.Error z value Pr(>|z|)##(Intercept)Not.severe-2.152580.56970-3.7780.000158***##(Intercept)Severe.1-1.391690.52035-2.6750.007483**##(Intercept)Severe.2-0.071410.57835-0.1230.901740##Age0.179090.04957 3.6130.000302***##---##Signif.codes:0’***’0.001’**’0.01’*’0.05’.’0.1’’1The equivalence between the(Cumulative,Gompertz,parallel)and(Sequential,Gompertz,parallel)mod-els has been demonstrated by Lääräand Matthews(1985)and it is hereby tested using the functions:cum_gom_p<-glmcat(formula=Level~Age,cdf="gompertz",ratio="cumulative",data=DisturbedDreams,parallel="Age")##Warning in glmcat(formula=Level~Age,cdf="gompertz",ratio=##"cumulative",:The response variable is not defined as an ordered variable.##Recall that the the reference ratio is appropiate for nominal responses,while##for ordinal responses the ratios to use are cumulative,sequential or adjacent.logLik(cum_gom_p)##’log Lik.’-280.0788(df=4)summary(cum_gom_p)##Level~Age##ratio cdf nobs niter logLik##Model info:cumulative gompertz2236-280.0788##Estimate Std.Error z value Pr(>|z|)##(Intercept)Not.severe-1.887810.36046-5.2371.63e-07***##(Intercept)Severe.1-1.335150.35206-3.7920.000149***##(Intercept)Severe.2-0.785510.34252-2.2930.021828*##Age0.124340.03009 4.1333.59e-05***##---##Signif.codes:0’***’0.001’**’0.01’*’0.05’.’0.1’’1seq_gom_p<-glmcat(formula=Level~Age,cdf="gompertz",ratio="sequential",data=DisturbedDreams,parallel="Age")##Warning in glmcat(formula=Level~Age,cdf="gompertz",ratio=##"sequential",:The response variable is not defined as an ordered variable.##Recall that the the reference ratio is appropiate for nominal responses,while##for ordinal responses the ratios to use are cumulative,sequential or adjacent.logLik(seq_gom_p)##’log Lik.’-280.0788(df=4)summary(seq_gom_p)##Level~Age##ratio cdf nobs niter logLik##Model info:sequential gompertz2236-280.0788##Estimate Std.Error z value Pr(>|z|)##(Intercept)Not.severe-1.887810.36046-5.2371.63e-07***##(Intercept)Severe.1-2.191800.36851-5.9482.72e-09***##(Intercept)Severe.2-1.646260.35822-4.5964.31e-06***##Age0.124340.03009 4.1333.59e-05***##---##Signif.codes:0’***’0.001’**’0.01’*’0.05’.’0.1’’1ConclusionThe models for categorical response data have been evolved in differentfields of research under different names.Some of them are fairly similar or are even the same.Until recently,there was no methodology that encompassed these models in a comparable scheme.glmcat is based on the new specification of a generalized linear model given by the(r,F,Z)-triplet,which groups together all the proposed methodologies for modelling categorical responses.glmcat offers a full picture of the spectrum of models where the user has three components to combine in order to obtain a model that meets the specifications of the problem. ReferencesBouscasse,Hélène,Iragaël Joly,and Jean Peyhardi.2019.“A new family of qualitative choice models:An application of reference models to travel mode choice.”Transportation Research Part B:Methodological 121(C):74–91.Läärä,E.,and J.N.S.Matthews.1985.“The equivalence of two models for ordinal data.”Biometrika72(1):206–7.https:///10.1093/biomet/72.1.206.Maxwell,A.E.1961.Analyzing Qualitative Data.Methuen.Peyhardi,J.,C.Trottier,and Y.Guédon.2015.“A new specification of generalized linear models for categorical responses.”Biometrika102(4):889–906.https:///10.1093/biomet/asv042.。
prediction-error method
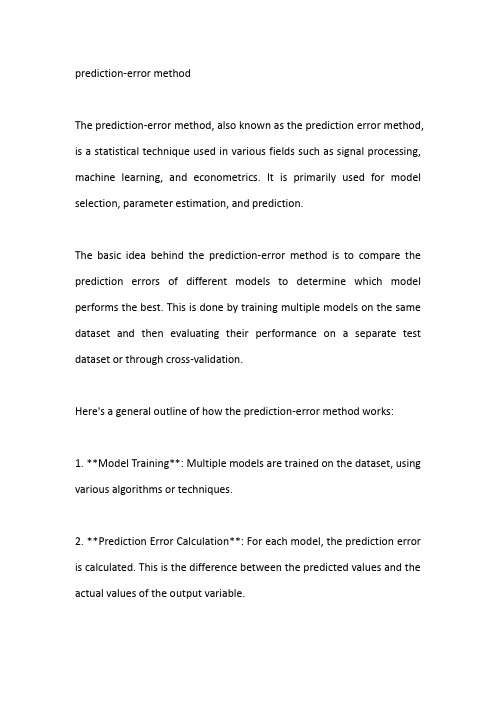
prediction-error methodThe prediction-error method, also known as the prediction error method, is a statistical technique used in various fields such as signal processing, machine learning, and econometrics. It is primarily used for model selection, parameter estimation, and prediction.The basic idea behind the prediction-error method is to compare the prediction errors of different models to determine which model performs the best. This is done by training multiple models on the same dataset and then evaluating their performance on a separate test dataset or through cross-validation.Here's a general outline of how the prediction-error method works:1. **Model Training**: Multiple models are trained on the dataset, using various algorithms or techniques.2. **Prediction Error Calculation**: For each model, the prediction error is calculated. This is the difference between the predicted values and the actual values of the output variable.3. **Model Comparison**: The models are compared based on their prediction errors. The model with the smallest prediction error is considered the best performing model.4. **Model Selection**: The model with the lowest prediction error is selected as the final model for prediction or further analysis.The prediction-error method is particularly useful when the goal is to find a model that is not only accurate but also parsimonious, meaning it has a relatively simple structure with fewer parameters. By focusing on minimizing prediction error, this method aims to find a balance between model complexity and performance.It's important to note that the prediction-error method is just one approach to model selection and evaluation. Other factors such as interpretability, computational efficiency, and domain-specific requirements may also influence the choice of model.。
高中英语必修四期末测试题(新人教版必修4)
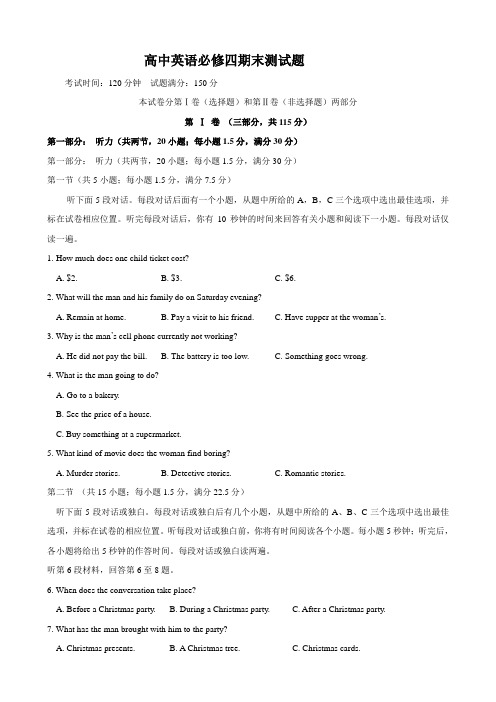
高中英语必修四期末测试题考试时间:120分钟试题满分:150分本试卷分第Ⅰ卷(选择题)和第Ⅱ卷(非选择题)两部分第Ⅰ卷(三部分,共115分)第一部分:听力(共两节,20小题;每小题1.5分,满分30分)第一部分:听力(共两节,20小题;每小题1.5分,满分30分)第一节(共5小题;每小题1.5分,满分7.5分)听下面5段对话。
每段对话后面有一个小题,从题中所给的A,B,C三个选项中选出最佳选项,并标在试卷相应位置。
听完每段对话后,你有10秒钟的时间来回答有关小题和阅读下一小题。
每段对话仅读一遍。
1. How much does one child ticket cost?A. $2.B. $3.C. $6.2. What will the man and his family do on Saturday evening?A. Remain at home.B. Pay a visit to his friend.C. Have supper at the woman’s.3. Why is the man’s cell phone currently not working?A. He did not pay the bill.B. The battery is too low.C. Something goes wrong.4. What is the man going to do?A. Go to a bakery.B. See the price of a house.C. Buy something at a supermarket.5. What kind of movie does the woman find boring?A. Murder stories.B. Detective stories.C. Romantic stories.第二节(共15小题;每小题1.5分,满分22.5分)听下面5段对话或独白。
高三英语 备考冲刺 必抓的八篇文章

高三备考冲刺,必抓的八篇文章高三备考在完成英语基础知识的复习总结之后,便进入了冲刺阶段。
冲刺阶段是英语知识及技能的灵活运用及输出阶段,是复习的最高境界。
其主要分为阅读和写作;阅读要求熟练运用语言知识获取、归纳、总结信息;写作则是语言知识的输出,是知识内化后的输出;是对词汇、语法、句式的恰到好处的表达。
高考试卷中有八篇与阅读相关的文章,即完形填空1篇、阅读理解5篇、短文改错1篇、写作1篇共115分(全国II卷)。
因此,我们说高考英语得阅读者得天下。
下面就完形填空、阅读理解、短文改错及写作的备考进行分述:完形填空【题型特点及考纲要求】1. 考查目的完形填空是对考生英语语言综合运用能力的考查,既考查考生对语篇的理解能力,包括篇章阅读理解、获取和分析信息的能力,又在语篇层面上考查学生在一定的语境中准确、恰当、得体运用词汇的能力。
2. 题型特点(1)体裁和题材:高考完形填空大多选择夹叙夹议的议论文或有一定故事情节和相对完整的故事片断的记叙文。
题材大都富有教育意义,能给考生以启迪,类似人生感悟的心灵鸡汤的小短文,语言地道,文笔优美。
(2)考点设置:完形填空设题完全不同于单项填空,以篇章语义为主,所设4个选项从语法角度考虑都正确。
错误项只能通过语义、语境、常识、逻辑和搭配来排除。
因而,高考完形填空设点以实词为主,其中最多是动词(包括非谓语动词、短语动词、情态动词)和名词,其次是形容词和副词,再次是连词和介词。
【完形填空应试技巧指南】首先,仔细审题,明确大意。
首先要以很快的速度浏览全文,掌握文章的主旨,不要急于看选项。
浏览全文时要重点了解文中所叙述的人物、时间、地点、事件,即who,when,where,what。
完形填空命题的原则一般是第一句话不挖空,目的是使读者进入语境,因此一定要认真阅读这句话。
例如:Why is a space left between the rails of a railway line where one piece joins the next?这句话从铁轨之间的缝隙引出了问题。
高考英语复习九月完形填空选编(一)1

陕西商南县2017高考英语九月完形填空选编(一)完型填空。
阅读下面短文,掌握其大意,然后从以下题所给的A、B、C、D四个选项中,选出最佳选项。
Most parents, I suppose, have had the experience of reading a bedtime story __1__ their children. And they must have realized how difficult it is to write a __2__ children’s book. Either the author has aimed (定目标) too __3__, so that children can’t follow what is in his ( or more often, her ) story, __4__ the story seems to be talking to the readers.The best chil dren’s books are __5__ very difficult nor very simple, and satisfy(令人满意的) the __6__ who hears the story and the adult(成年人) who __7__ it. Unfortunately(不幸的是), there are in fact few books like this, __8__ the problem of finding the right bedtime story is not __9__ to solve. This may be why many of the books regarded as __10__ of children’s literature(文学) were in fact written for __11__ “Alice in Wonderland” is perhaps the most obvious(明显)of this.Children, left for themselves, often __12__ the worst possible interest in literature. Just leave a child in a bookshop or a __13__ and he will more willingly choose the books written in an unimaginative (并非想象的) way, or have a look at the most children’s comics(连环图书), full of the stories and jokes which are the rejections of teachers and righting-thinking parents.Perhaps we parents should stop __14__ to brainwash(洗脑)children into accepting(接受)our taste in literature. After all, children and adults are so __15__ that we parents should not expect that they will enjoy the same books. So I suppose we’ll just have to compromise(妥协)over the bedtime story.名师点评本文说明了写一本供孩子读的好书并非一件容易的事,并且告诫家长不要一味强迫孩子接受大人的观点,因为孩子和大人在兴趣方面不尽相同。
风险预警体系
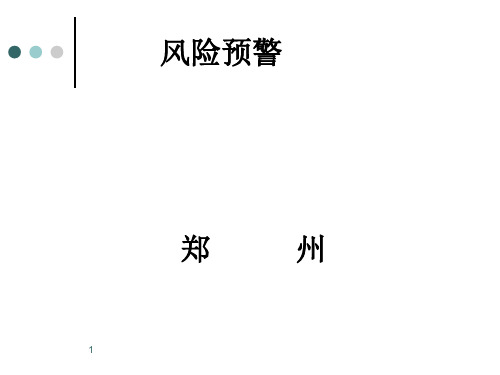
6A. FDA finds sample in compliance 样本 合格
“Release Notice” sent to U.S. Customs and importer “放行文件”交给海关和进口
商
7A. Importer responds to “Notice of Detention and Hearing”进口商做出
反应
8A. FDA holds hearing on detained product 1F6DA听取扣押产品的听证
6B. FDA finds sample is violative不合格
“Notice of Detention and Hearing” sent to U.S. Customs and importer “扣留和听
合要求
9B. Importer submits application to recondition 提出整改申请
10A. FDA collects follow-up sample FDA接受追加样本
11C. FDA approves importer’s reconditioning proposal FDA允许
将“进行许可”交给海关和进口商
15
4B. FDA wants sample 需样本 “Notice of Sampling” sent to U.S. Customs and importer “取样证明”交给
海关和进口商
5. FDA/U.S. Customs collects physical sample FDA/海关取样
预警内容:货物品种、生产商/承运人、进口商、地区及国家、原产国;
(必要时可参照国外检查信息及出口国提供的资料证明)
- 1、下载文档前请自行甄别文档内容的完整性,平台不提供额外的编辑、内容补充、找答案等附加服务。
- 2、"仅部分预览"的文档,不可在线预览部分如存在完整性等问题,可反馈申请退款(可完整预览的文档不适用该条件!)。
- 3、如文档侵犯您的权益,请联系客服反馈,我们会尽快为您处理(人工客服工作时间:9:00-18:30)。
1
Author to whom all correspondence should be addressed. Email address: nikolaou@. Phone: (713) 743-4309. Fax (713) 743-4323.
1.
Introduction
Controller design for linear or nonlinear processes with actuator saturation nonlinearities has long been studied within various contexts. Kothare (1997a) provides a good discussion on the various approaches that have appeared in literature. There are two distinct classes of structures that handle input saturation nonlinearities: (a) On-line optimization based control structures, such as Model Predictive Control (MPC), and (b) anti-windup bumpless transfer (AWBT) controllers that have a closed form and do not perform online optimization. Controllers in the first class rely on a process model and on-line solution of a constrained optimization problem that minimizes an objective over a future horizon. If properly designed, such controllers can provide optimality, robustness, and other desirable properties. However, because of the time needed to perform the on-line optimization, controllers in the first class are usually implemented on relatively slow processes. Controllers in the second class completely bypass on-line optimization, therefore they are inherently faster and can be used on faster processes. The AWBT controller design approach is based on the following two-step design paradigm: Firstly, a linear controller is designed ignoring input constraints. In the next step, an anti-windup scheme is added to compensate for the adverse effects of the constraints on closed-loop performance. There have been many heuristic techniques for the design of AWBT controllers, virtually all of which can be summarized into a structure that includes a saturation nonlinearity in the forward path and a linear transfer function in the feedback path. Campo (1997) and Kothare et al. (1994) unified all the existing AWBT schemes and developed a general framework for studying stability and robustness issues. The importance of that work lies in that model uncertainty can be taken into account systematically and theory exists to analyze the closed-loop system for stability and robustness. However, their analysis is also based on the standard conic sector nonlinear stability theory. Therefore, the results could be potentially conservative. The design of AWBT controllers for SISO systems relies on a mix of intuitive and rigorous arguments, which become difficult to use in the MIMO case. As pointed by Doyle et al. (1987), for MIMO controllers, the saturation may cause a change in the plant input direction resulting in disastrous consequences. Through an example, Doyle et al. (1987) showed that all anti-windup schemes of the time failed to work on MIMO systems. Recently, Kothare et al. (1997b) described three performance requirements that should be incorporated in a multi-objective multivariable AWBT controller synthesis framework. Although promising lines for designing an AWBT controller using dynamic output feedback and one-step design were outlined, many of the details like "recovery of linear performance" need to be worked out. Model Predictive Control (MPC) is a discrete-time technique in which the control action is obtained by repeatedly solving an on-line optimization problem at each time step. The flexibility of MPC has been useful in addressing various implementation issues that traditionally have been problematic. From a practical viewpoint, the most important feature of MPC is its ability to naturally and explicitly handle multivariable input and output constraints by direct incorporation into the on-line optimization. The issues of stability and robustness of MPC are now a fairly well understood topics (Rawlings and Muske, 1993; Nikolaou, 1998). For linear plants the MPC problem is usually reduced to a quadratic program (QP) which can be solved efficiently (Cutler et al., 1980; García et al., 1986). Nonlinear plants, however, lead to nonlinear programs (NLP) which are in general non-convex and very demanding computationally (Biegler et al., 1991; Mayne et al., 1991; Mayne et al., 1990). Evidence of the difficulty and effort involved in controlling a real experimental system by solving the general NLP is shown in (Kreshenbaum et al., 1994). The MPC strategy was first exploited and successfully employed on linear plants, especially in the process industries, where relatively slow sampling times made extensive on-line intersample computation feasible. But for a fast process the implementation of MPC may be quite difficult and sometime infeasible. Both MPC and AWBT controllers have their own advantages and disadvantages. However, to the best of our knowledge, no clear relationship between these two control schemes has established. In this work, we rigorously show that there is a direct relationship between MPC and AWBT control. In fact, we show that the general structure of AWBT controllers emerges naturally from the structure of MPC with quadratic objective, input constraints, and plant model structure affine in the input variables. This realization is important for a number of reasons, including the following: • It provides theoretical justification for the empirical realization that virtually all heuristically developed AWBT control structures follow a similar pattern involving a nonlinear (saturation) block and linear transfer functions (Kothare, 1997a).