Estimating Vector Autoregressions with Panel Data
stata误差修正模型命令

Stata误差修正模型命令简介误差修正模型(Error Correction Model,ECM)是一种用于描述时间序列数据之间长期和短期关系的经济模型。
它是自回归移动平均模型(ARMA)和协整关系的结合,可以用于分析变量之间的长期均衡关系和短期调整速度。
Stata是一款功能强大的统计分析软件,提供了许多用于估计和分析误差修正模型的命令。
本文将介绍Stata中常用的误差修正模型命令及其使用方法。
命令介绍vecintrovecintro命令用于估计向量自回归(Vector Autoregression,VAR)模型,并进行协整检验。
在估计VAR之前,我们需要先检验变量之间是否存在协整关系。
vecintro命令可以帮助我们进行协整检验并选择适当的滞后阶数。
使用示例:vecintro y x1 x2, lags(1/4)其中,y表示因变量,x1和x2表示自变量。
lags(1/4)表示选择滞后阶数为1至4。
vecrankvecrank命令用于估计向量错误修正模型(Vector Error Correction Model,VECM)。
VECM是一种描述协整关系和短期调整速度的模型。
使用示例:vecrank y x1 x2, lags(1/4) rank(2)其中,y表示因变量,x1和x2表示自变量。
lags(1/4)表示选择滞后阶数为1至4,rank(2)表示选择协整关系的阶数为2。
vecvec命令用于估计向量错误修正模型,并进行残差诊断和模型拟合优度检验。
使用示例:vec y x1 x2, lags(1/4) rank(2)其中,y表示因变量,x1和x2表示自变量。
lags(1/4)表示选择滞后阶数为1至4,rank(2)表示选择协整关系的阶数为2。
常用参数lags在估计误差修正模型时,我们需要选择合适的滞后阶数。
Stata中的误差修正模型命令通常都提供了lags参数来指定滞后阶数范围。
使用示例:vec y x, lags(1/4)上述示例中的lags参数指定了滞后阶数范围为1至4。
马尔可夫区制转换向量自回归模型
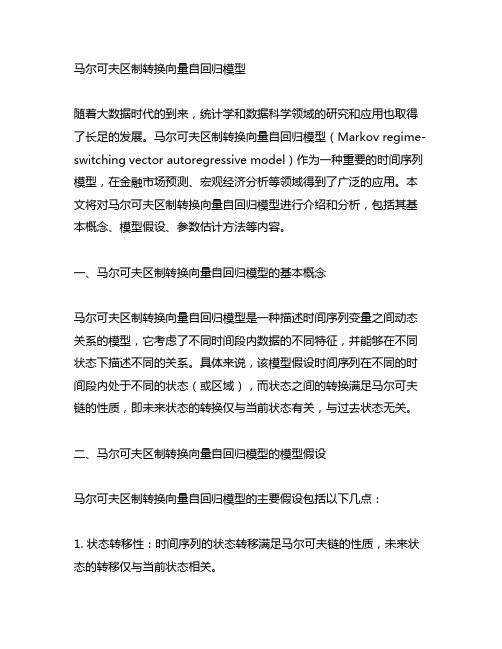
马尔可夫区制转换向量自回归模型随着大数据时代的到来,统计学和数据科学领域的研究和应用也取得了长足的发展。
马尔可夫区制转换向量自回归模型(Markov regime-switching vector autoregressive model)作为一种重要的时间序列模型,在金融市场预测、宏观经济分析等领域得到了广泛的应用。
本文将对马尔可夫区制转换向量自回归模型进行介绍和分析,包括其基本概念、模型假设、参数估计方法等内容。
一、马尔可夫区制转换向量自回归模型的基本概念马尔可夫区制转换向量自回归模型是一种描述时间序列变量之间动态关系的模型,它考虑了不同时间段内数据的不同特征,并能够在不同状态下描述不同的关系。
具体来说,该模型假设时间序列在不同的时间段内处于不同的状态(或区域),而状态之间的转换满足马尔可夫链的性质,即未来状态的转换仅与当前状态有关,与过去状态无关。
二、马尔可夫区制转换向量自回归模型的模型假设马尔可夫区制转换向量自回归模型的主要假设包括以下几点:1. 状态转移性:时间序列的状态转移满足马尔可夫链的性质,未来状态的转移仅与当前状态相关。
2. 向量自回归性:时间序列变量之间的关系可以用向量自回归模型描述,即当前时间点的向量可以由过去时间点的向量线性组合而成。
3. 区制转换性:时间序列的状态在不同时期具有不同的动态特征,模型需要考虑不同状态下的向量自回归关系。
以上假设为马尔可夫区制转换向量自回归模型的基本假设,这些假设使得模型能够较好地描述时间序列数据的动态演化。
三、马尔可夫区制转换向量自回归模型的参数估计方法马尔可夫区制转换向量自回归模型的参数估计是一个重要且复杂的问题,一般可以通过以下几种方法进行估计:1. 极大似然估计:假设时间序列的概率分布形式,通过最大化似然函数来得到模型参数的估计值。
这种方法需要对概率分布进行合理的假设,并且通常需要通过迭代算法来求解。
2. 贝叶斯方法:利用贝叶斯统计理论,结合先验分布和似然函数,通过马尔科夫链蒙特卡洛(MCMC)等方法得到模型参数的后验分布,进而得到参数的估计值。
eviews操作实例-向量自回归模型VAR和VEC
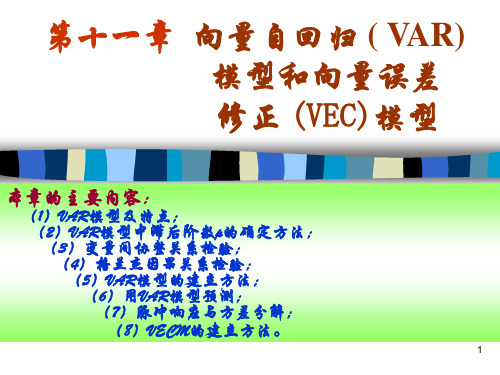
-5.4324 -5.7557
5% 临界值
-2.9202 -2.9202 -2.9202
模型形式 (C t p)
(c 0 3) (c 0 0) (c 0 0)
DW值
1.6551 1.9493 1.8996
结论
LGDPt ~I(1) LCt ~I( 1)
LIt~I(1)
注 C为位移项, t为趋势,p为滞后阶数。
yNt
的最大p阶滞后变量为解释变量的方程组模型,方程组模 型中共有N个方程。显然,VAR模型是由单变量AR模型推广到 多变量组成的“向量”自回归模型。
对于两个变量(N=2),Yt ( yt xt )T 时,VAR(2)模型为
2
Yt iYti Ut 1Yt1 2Yt2 Ut i 1
6
用矩阵表示:
xt
121 yt1
122xt1
221yt2
222xt2
u2t
显然,方程组左侧是两个第t期内生变量;右侧分 别是两个1阶和两个2阶滞后应变量做为解释变量,且 各方程最大滞后阶数相同,都是2。这些滞后变量与随 机误差项不相关(假设要求)。
7
由于仅有内生变量的滞后变量出现在等式的 右侧,故不存在同期相关问题,用“LS”法估计 参数,估计量具有一致和有效性。而随机扰动列 向量的自相关问题可由增加作为解释应变量的滞 后阶数来解决。
3
政策分析。但实际中,这种模型的效果并不令人满 意。
联立方程组模型的主要问题:
(1)这种模型是在经济理论指导下建立起来的结构模型 。遗憾的是经济理论并不未明确的给出变量之间的动态关 系。
(2)内生、外生变量的划分问题较为复杂; (3)模型的识别问题,当模型不可识别时,为达到可识别 的目的,常要将不同的工具变量加到各方程中,通常这种 工具变量的解释能力很弱; (4)若变量是非平稳的(通常如此),则会违反假设, 带来更严重的伪回归问题。
VAR模型和VEC模型

关于其它识别方法:
王中昭制作
• Eviews5.1版本结出了5个评价标准的结果(见下页解释)。 例如利用实例的文件aL3得(在VAR模型估计结果窗 口中点view再选取lag structure , lag length Criteria得 到),根据金融理论,货币效应时滞在一年左右,所 以选择最大4阶,也可以结合模型检验来确定。
方程,滞后项数为b的VAR模型,k=a2ba 。
检验的方法是主观地定出滞后期上限Q,对滞后长度b=1,2,…,Q,
分别求出AIC和SC,则对应的AIC和SC的同时最小值(不是取绝对 值)即为滞后期b(以模型总的AIC和SC为判断标准,不是以单个 方程的AIC和SC),可以进一步结合模型统计检验来确定b。此法 有一定的主观性。
王中昭制作
• 假设系统处于均衡状态,如果由于某种原因,破 坏了均衡,系统对该干扰作出反映,偏离均衡然后恢 复均衡,这个过程用脉冲响应函数来描述。
• 脉冲响应函数是度量来自于每个方程的随机误差项 的一个标准差新信息(见新信息解释)冲击时被解释 变量的响应程度和持续时间。例如假定某个方程的随 机误差项在第t期发生突变,而后各期重新恢复平静, 这时脉冲响应测量表示的是各期(t,t+1,t+2…)的被 解释变量对该冲击的反应。例如VAR(1):Yt=c+θYt+e ,则 其 1 中 tYt yy1 2tt ,则Yt c(cYt2et1)et
aL3.wf1
2、建模。 王中昭制作 在选择滞后项时,应用信息准则,根据金融理论, 货币效应时滞在一年左右,所以我们选择最大4阶。
滞后期 b=1 b=2 b=3 b=4
AIC 39.56 39.43 39.14 38.95
SC
贝叶斯矢量自回归模型与随机波动率和时变参数的分析软件说明书
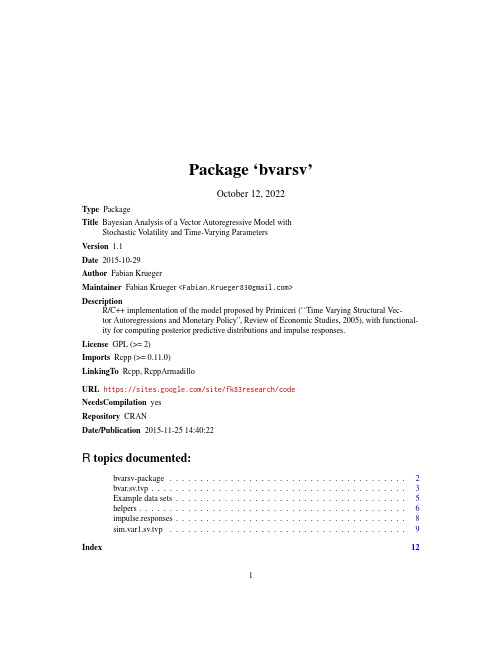
Package‘bvarsv’October12,2022Type PackageTitle Bayesian Analysis of a Vector Autoregressive Model withStochastic V olatility and Time-Varying ParametersVersion1.1Date2015-10-29Author Fabian KruegerMaintainer Fabian Krueger<**************************>DescriptionR/C++implementation of the model proposed by Primiceri(``Time Varying Structural Vec-tor Autoregressions and Monetary Policy'',Review of Economic Studies,2005),with functional-ity for computing posterior predictive distributions and impulse responses.License GPL(>=2)Imports Rcpp(>=0.11.0)LinkingTo Rcpp,RcppArmadilloURL https:///site/fk83research/codeNeedsCompilation yesRepository CRANDate/Publication2015-11-2514:40:22R topics documented:bvarsv-package (2)p (3)Example data sets (5)helpers (6)impulse.responses (8)p (9)Index1212bvarsv-packagebvarsv-package Bayesian Analysis of a Vector Autoregressive Model with StochasticVolatility and Time-Varying ParametersDescriptionR/C++implementation of the Primiceri(2005)model,which allows for both stochastic volatility and time-varying regression parameters.The package contains functions for computing posterior predictive distributions and impulse responses from the model,based on an input data set.DetailsPackage:bvarsvType:PackageVersion: 1.0Date:2014-08-14License:GPL(>=2)URL:https:///site/fk83research/codeAuthor(s)Fabian Krueger<**************************>,based on Matlab code by Dimitris Korobilis(see Koop and Korobilis,2010).ReferencesThe code incorporates the recent corrigendum by Del Negro and Primiceri(2015),which points to an error in the original MCMC algorithm of Primiceri(2005).Del Negro,M.and Primicerio,G.E.(2015).‘Time Varying Structural Vector Autoregressions and Monetary Policy:A Corrigendum’,Review of Economic Studies82,1342-1345.Koop,G.and D.Korobilis(2010):‘Bayesian Multivariate Time Series Methods for Empirical Macroeconomics’,Foundations and Trends in Econometrics3,267-358.Accompanying Matlab code available at https:///site/dimitriskorobilis/matlab.Primiceri,G.E.(2005):‘Time Varying Structural Vector Autoregressions and Monetary Policy’, Review of Economic Studies72,821-852.Examples##Not run:#Load US macro datadata(usmacro)#Estimate trivariate model using Primiceri s prior choices(default settings)set.seed(5813)bv<p(usmacro)##End(Not run)p Bayesian Analysis of a Vector Autoregressive Model with StochasticVolatility and Time-Varying ParametersDescriptionBayesian estimation of theflexible V AR model by Primiceri(2005)which allows for both stochasticvolatility and time drift in the model parameters.Usagep(Y,p=1,tau=40,nf=10,pdrift=TRUE,nrep=50000,nburn=5000,thinfac=10,itprint=10000,save.parameters=TRUE,k_B=4,k_A=4,k_sig=1,k_Q=0.01,k_S=0.1,k_W=0.01,pQ=NULL,pW=NULL,pS=NULL)ArgumentsY Matrix of data,where rows represent time and columns are different variables.Y must have at least two columns.p Lag length,greater or equal than1(the default)tau Length of the training sample used for determining prior parameters via leastsquares(LS).That is,data in Y[1:tau,]are used for estimating prior parame-ters via LS;formal Bayesian analysis is then performed for data in Y[(tau+1):nrow(Y),].nf Number of future time periods for which forecasts are computed(integer,1orgreater,defaults to10).pdrift Dummy,indicates whether or not to account for parameter drift when simulatingforecasts(defaults to TRUE).nrep Number of MCMC draws excluding burn-in(defaults to50000)nburn Number of MCMC draws used to initialize the sampler(defaults to5000).Thesedraws do not enter the computation of posterior moments,forecasts etc.thinfac Thinning factor for MCMC output.Defaults to10,which means that the fore-cast sequences(fc.mdraws,fc.vdraws,fc.ydraws,see below)contain onlyevery tenth draw of the original sequence.Set thinfac to one to obtain the fullMCMC sequence.itprint Print every itprint-th iteration.Defaults to10000.Set to very large value toomit printing altogether.save.parametersIf set to TRUE,parameter draws are saved in lists(these can be very large).De-faults to TRUE.k_B,k_A,k_sig,k_Q,k_W,k_S,pQ,pW,pSQuantities which enter the prior distributions,see the links below for details.Defaults to the exact values used in the original article by Primiceri.ValueBeta.postmean Posterior means of coefficients.This is an array of dimension[M,Mp+1,T],where T denotes the number of time periods(=number of rows of Y),and Mdenotes the number of system variables(=number of columns of Y).The sub-matrix[,,t]represents the coefficient matrix at time t.The intercept vector isstacked in thefirst column;the p coefficient matrices of dimension[M,M]areplaced next to it.H.postmean Posterior means of error term covariance matrices.This is an array of dimension[M,M,T].The submatrix[,,t]represents the covariance matrix at time t.Q.postmean,S.postmean,W.postmeanPosterior means of various covariance matrices.fc.mdraws Draws for the forecast mean vector at various horizons(three-dimensional array,where thefirst dimension corresponds to system variables,the second to forecasthorizons,and the third to MCMC draws).Note:The third dimension will beequal to nrep/thinfac,apart from possible rounding issues.fc.vdraws Draws for the forecast covariance matrix.Design similar to fc.mdraws,ex-cept that thefirst array dimension contains the lower-diagonal elements of theforecast covariance matrix.fc.ydraws Simulated future observations.Design analogous to fc.mdraws.Beta.draws,H.drawsMatrices of parameter draws,can be used for computing impulse responses lateron(see impulse.responses),and accessed via the helper function parameter.draws.These outputs are generated only if save.parameters has been set to TRUE.Author(s)Fabian Krueger,based on Matlab code by Dimitris Korobilis(see Koop and Korobilis,2010).In-corporates the corrigendum by Del Negro and Primiceri(2015),which points to an error in the original MCMC algorithm of Primiceri(2005).ReferencesDel Negro,M.and Primicerio,G.E.(2015).‘Time Varying Structural Vector Autoregressions and Monetary Policy:A Corrigendum’,Review of Economic Studies82,1342-1345.Koop,G.and D.Korobilis(2010):‘Bayesian Multivariate Time Series Methods for Empirical Macroeconomics’,Foundations and Trends in Econometrics3,267-358.Accompanying Matlab code available at https:///site/dimitriskorobilis/matlab.Primiceri,G.E.(2005):‘Time Varying Structural Vector Autoregressions and Monetary Policy’, Review of Economic Studies72,821-852.Example data sets5See AlsoThe helper functions predictive.density and predictive.draws provide simple access to the forecast distribution produced by p.Impulse responses can be computed using im-pulse.responses.For detailed examples and explanations,see the accompanying pdffile hosted at https:///site/fk83research/code.Examples##Not run:#Load US macro datadata(usmacro)#Estimate trivariate BVAR using default settingsset.seed(5813)bv<p(usmacro)##End(Not run)Example data sets US Macroeconomic Time SeriesDescriptionInflation rate,unemployment rate and treasury bill interest rate for the US,as used by Primiceri (2005).Whereas usmacro covers the time period studied by Primiceri(1953:Q1to2001:Q3), usmacro.update updates the data until2015:Q2.FormatMultiple time series(mts)object,series names:‘inf’,‘une’,and‘tbi’.SourceInflation data provided by Federal Reserve Bank of Philadelphia(2015):‘Real-Time Data Research Center’,https:///research-and-data/real-time-center/real-time-data/ data-files/p Accessed:2015-10-29.The inflation rate is the year-over-year log growth rate of the GDP price index.We use the2001:Q4vintage of the price index for usmacro,and the2015:Q3 vintage for usmacro.update.Unemployment and Treasury Bill:Federal Reserve Bank of St.Louis(2015):‘Federal Reserve Economic Data’,/fred2/.Accessed:2015-10-29.The two series have the identifiers‘UNRATE’and‘TB3MS’.For each quarter,we compute simple averages over three monthly observations.Disclaimer:Please note that the providers of the original data cannot take responsibility for the data posted here,nor can they answer any questions about ers should consult their respective websites for the official and most recent version of the data.ReferencesPrimiceri,G.E.(2005):‘Time Varying Structural Vector Autoregressions and Monetary Policy’, Review of Economic Studies72,821-852.Examples##Not run:#Load and plot datadata(usmacro)plot(usmacro)##End(Not run)helpers Helper Functions to Access BVAR Forecast Distributions and Param-eter DrawsDescriptionFunctions to extract a univariate posterior predictive distribution from a modelfit generated by p.Usagepredictive.density(fit,v=1,h=1,cdf=FALSE)predictive.draws(fit,v=1,h=1)parameter.draws(fit,type="lag1",row=1,col=1)Argumentsfit List,modelfit generated by pv Index for variable of interest.Must be in line with the specification of fit.h Index for forecast horizon of interest.Must be in line with the specification offit.cdf Set to TRUE to return cumulative distribution function,set to FALSE to return probability density functiontype Character string,used to specify output for function parameter.draws.Setting to"intercept"returns parameter draws for the intercept vector.Setting to oneof"lag1",...,"lagX",(where X is the lag order used in fit)returns parameterdraws from the autoregressive coefficient matrices.Setting to"vcv"returnsdraws for the elements of the residual variance-covariance matrix.row,col Row and column index for the parameter for which parameter.draws should return posterior draws.That is,the function returns the row,col element of thematrix specified by type.Note that col is irrelevant if type="intercept"hasbeen chosen.Valuepredictive.density returns a function f(z),which yields the value(s)of the predictive density at point(s)z.This function exploits conditional normality of the model,given the posterior draws of the parameters.predictive.draws returns a list containing vectors of MCMC draws,more specifically:y Draws from the predictand itselfm Mean of the normal distribution for the predictand in each drawv Variance of the normal distribution for the predictand in each drawBoth outputs should be closely in line with each other(apart from a small amount of sampling noise),see the link below for details.parameter.draws returns posterior draws for a single(scalar)parameter of the modelfitted by p.The output is a matrix,with rows representing MCMC draws,and columns repre-senting time.Author(s)Fabian KruegerSee AlsoFor examples and background,see the accompanying pdffile hosted at https://sites.google.com/site/fk83research/code.Examples##Not run:#Load US macro datadata(usmacro)#Estimate trivariate BVAR using default settingsset.seed(5813)bv<p(usmacro)#Construct predictive density function for the second variable(inflation),one period ahead f<-predictive.density(bv,v=2,h=1)#Plot the density for a grid of valuesgrid<-seq(-2,5,by=0.05)plot(x=grid,y=f(grid),type="l")#Cross-check:Extract MCMC sample for the same variable and horizonsmp<-predictive.draws(bv,v=2,h=1)#Add density estimate to plotlines(density(smp),col="green")##End(Not run)8impulse.responses impulse.responses Compute Impulse Response Function from a Fitted ModelDescriptionComputes impulse response functions(IRFs)from a modelfit produced by p.The IRF describes how a variable responds to a shock in another variable,in the periods following the shock.To enable simple handling,this function computes IRFs for only one pair of variables that must be specified in advance(see impulse.variable and response.variable below).Usageimpulse.responses(fit,impulse.variable=1,response.variable=2,t=NULL,nhor=20,scenario=2,draw.plot=TRUE) Argumentsfit Modelfit produced by p,with the option save.parameters set to TRUE.impulse.variableVariable which experiences the shock.response.variableVariable which(possibly)responds to the shock.t Time point from which parameter matrices are to be taken.Defaults to most recent time point.nhor Maximal time between impulse and response(defaults to20).scenario If1,there is no orthogonalizaton,and the shock size corresponds to one unit of the impulse variable.If scenario is either2(the default)or3,the errorterm variance-covariance matrix is orthogonalized via Cholesky decomposition.For scenario=2,the Cholesky decomposition of the error term VCV matrix attime point t is used.scenario=3is the variant used in Del Negro and Primiceri(2015).Here,the diagonal elements are set to their averages over time,whereasthe off-diagonal elements are specific to time t.See the notes below for furtherinformation.draw.plot If TRUE(the default):Produces a plot showing the5,25,50,75and95percent quantiles of the simulated impulse responses.ValueList of two elements:contemporaneousContemporaneous impulse responses(vector of simulation draws).irf Matrix of simulated impulse responses,where rows represent simulation draws, and columns represent the number of time periods after the shock(1infirstcolumn,nhor in last column).NoteIf scenario is set to either2or3,the Cholesky transform(transpose of chol)is used to produce the orthogonal impulse responses.See Hamilton(1994),Section11.4,and particularly Equation[11.4.22].As discussed by Hamilton,the ordering of the system variables matters,and should beconsidered carefully.The magnitude of the shock(impulse)corresponds to one standard deviation of the error term.If scenario=1,the function simply outputs the matrices of the model’s moving average represen-tation,see Equation[11.4.1]in Hamilton(1994).The scenario considered here may be unrealistic, in that an isolated shock may be unlikely.The magnitude of the shock(impulse)corresponds to one unit of the error term.Further supporting information is available at https:///site/FK83research/ code.Author(s)Fabian KruegerReferencesHamilton,J.D.(1994):Time Series Analysis,Princeton University Press.Del Negro,M.and Primicerio,G.E.(2015).‘Time Varying Structural Vector Autoregressions and Monetary Policy:A Corrigendum’,Review of Economic Studies82,1342-1345.Supplementary material available at /content/82/4/1342/suppl/DC1(ac-cessed:2015-11-17).Examples##Not run:data(usmacro)set.seed(5813)#Run BVAR;save parametersfit<p(usmacro,save.parameters=TRUE)#Impulse responsesimpulse.responses(fit)##End(Not run)p Simulate from a VAR(1)with Stochastic Volatility and Time-VaryingParametersDescriptionSimulate from a V AR(1)with Stochastic V olatility and Time-Varying ParametersUsagep(B0=NULL,A0=NULL,Sig0=NULL,Q=NULL,S=NULL,W=NULL,t=500,init=1000)ArgumentsB0Initial values of mean parameters:Matrix of dimension[M,M+1],where the first column holds the intercept vector and the other columns hold the matrixoffirst-order autoregressive coefficients.By default(NULL),B0corresponds toM=2uncorrelated zero-mean processes with moderate persistence(first-orderautocorrelation of0.6).A0Initial values for(transformed)error correlation parameters:Vector of length0.5∗M∗(M−1).Defaults to a vector of zeros.Sig0Initial values for log error term volatility parameters:Vector of length M.De-faults to a vector of zeros.Q,S,W Covariance matrices for the innovation terms in the time-varying parameters (B,A,Sig).The matrices are symmetric,with dimensions equal to the numberof elements in B,A and Sig,respectively.Default to diagonal matrices withvery small terms(1e-10)on the main diagonal.This corresponds to essentiallyno time variation in the parameters and error term matrix elements.t Number of time periods to simulate.init Number of draws to initialize simulation(to decrease the impact of starting val-ues).Valuedata Simulated data,with rows corresponding to time and columns corresponding to the M system variables.Beta Array of dimension[M,M+1,t].Submatrix[,,l]holds the parameter matrix for time period l.H Array of dimension[M,M,t].Submatrix[,,l]holds the error term covariancematrix for period l.NoteThe choice of‘reasonable’values for the elements of Q,S and W requires some care.If the elements of these matrices are too large,parameter variation can easily become excessive.Too large elements of Q can lead the parameter matrix B into regions which correspond to explosive processes.Too large elements in S and(especially)W may lead to excessive error term variances.Author(s)Fabian KruegerReferencesPrimiceri,G.E.(2005):‘Time Varying Structural Vector Autoregressions and Monetary Policy’, Review of Economic Studies72,821-852.p11See Alsop can be used tofit a model on data generated by p.This can be a useful way to analyze the performance of the estimation methods.Examples##Not run:#Generate data from a model with moderate time variation in the parameters#and error term variancesset.seed(5813)sim<p(Q=1e-5*diag(6),S=1e-5*diag(1),W=1e-5*diag(2))#Plot both seriesmatplot(sim$data,type="l")#Plot AR(1)parameters of both equationsmatplot(cbind(sim$Beta[1,2,],sim$Beta[2,3,]),type="l")##End(Not run)Index∗datasetsExample data sets,5∗forecasting methodsp,3p,9∗helpershelpers,6∗impulse response analysisimpulse.responses,8∗packagebvarsv-package,2p,3,5–8,11bvarsv(bvarsv-package),2bvarsv-package,2chol,9Example data sets,5helpers,6impulse.responses,4,5,8parameter.draws,4,6,7parameter.draws(helpers),6predictive.density,5,7predictive.density(helpers),6 predictive.draws,5,7predictive.draws(helpers),6p,9,11usmacro(Example data sets),512。
generalized autoregressive score模型 -回复
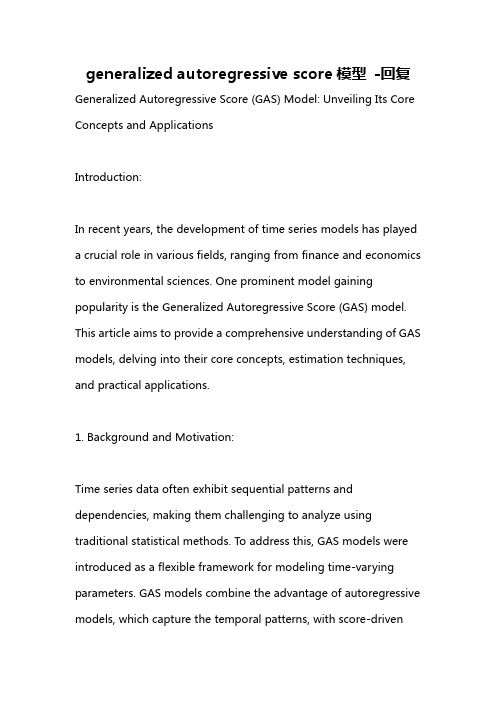
generalized autoregressive score模型-回复Generalized Autoregressive Score (GAS) Model: Unveiling Its Core Concepts and ApplicationsIntroduction:In recent years, the development of time series models has played a crucial role in various fields, ranging from finance and economics to environmental sciences. One prominent model gaining popularity is the Generalized Autoregressive Score (GAS) model. This article aims to provide a comprehensive understanding of GAS models, delving into their core concepts, estimation techniques, and practical applications.1. Background and Motivation:Time series data often exhibit sequential patterns and dependencies, making them challenging to analyze using traditional statistical methods. To address this, GAS models were introduced as a flexible framework for modeling time-varying parameters. GAS models combine the advantage of autoregressive models, which capture the temporal patterns, with score-drivenmodeling principles enabling non-linear and dynamic parameter estimation.2. Core Concepts:The core concept of GAS models lies in the scoring function, which measures the discrepancy between the actual and predicted values of the parameter. The scoring function acts as a driving force, updating the parameter based on the information obtained from the current observation. This score-driven approach allows the model to adapt to changes in the data, making it well-suited for modeling time-varying parameters.3. Estimation Techniques:The estimation of GAS models involves two main steps: defining the scoring function and selecting the appropriate distributional assumption for the error term. The choice of the scoring function depends on the nature of the parameter being estimated. Common scoring functions include the Expectation-Maximization (EM) algorithm, the Fisher scoring algorithm, and the Stochastic Approximation Expectation-Maximization (SAEM) algorithm. Thesetechniques ensure that the parameter estimation is efficient and accurate.4. Applications:The GAS model has found applications in various fields due to its flexibility and adaptability. Here are some prominent applications:4.1. Financial Econometrics: GAS models have been extensively used in modeling and forecasting financial time series, such as stock prices, exchange rates, and volatility. Their ability to capture time-varying parameters and adjust to changing market conditions makes them valuable tools for risk management, portfolio optimization, and option pricing.4.2. Macroeconomics: In macroeconomic analysis, GAS models have been employed to estimate time-varying parameters in economic models. By capturing the changing dynamics of key macroeconomic variables, such as inflation rates and GDP growth, these models enable policymakers to make informed decisions and forecast future trends accurately.4.3. Environmental Sciences: GAS models have been successfully applied to model and predict biophysical processes, such as climate variables and ecological systems. By accounting fortime-varying parameters, these models enhance the understanding of complex environmental processes and aid in developing strategies for sustainable resource management.4.4. Engineering and Control Systems: GAS models have also found applications in engineering and control systems, wheretime-varying parameters are prevalent. These models help in predicting system behavior and optimizing control policies, contributing to improved performance and efficiency in various engineering domains.Conclusion:The Generalized Autoregressive Score (GAS) model represents a powerful and flexible framework for modeling time-varying parameters in time series data. By combining autoregressive models with score-driven principles, GAS models adapt to changing patterns and provide accurate parameter estimation.From finance and economics to environmental sciences and engineering, the applications of GAS models are widespread. Researchers and practitioners continue to explore the potential of this innovative modeling approach, paving the way for advancements in various domains.。
马尔可夫区制转换向量自回归模型
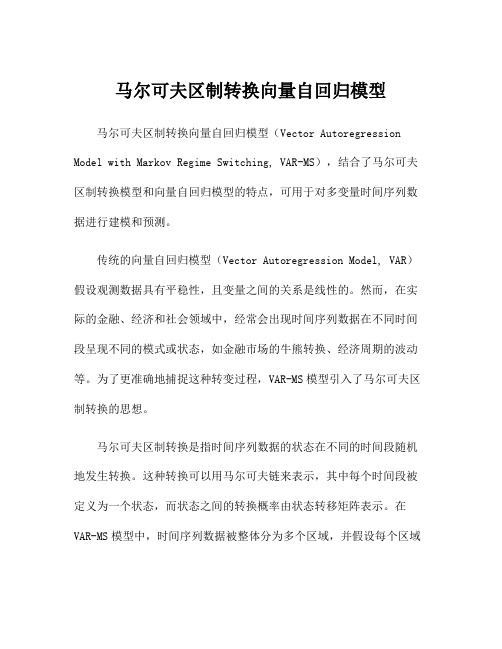
马尔可夫区制转换向量自回归模型马尔可夫区制转换向量自回归模型(Vector Autoregression Model with Markov Regime Switching, VAR-MS),结合了马尔可夫区制转换模型和向量自回归模型的特点,可用于对多变量时间序列数据进行建模和预测。
传统的向量自回归模型(Vector Autoregression Model, VAR)假设观测数据具有平稳性,且变量之间的关系是线性的。
然而,在实际的金融、经济和社会领域中,经常会出现时间序列数据在不同时间段呈现不同的模式或状态,如金融市场的牛熊转换、经济周期的波动等。
为了更准确地捕捉这种转变过程,VAR-MS模型引入了马尔可夫区制转换的思想。
马尔可夫区制转换是指时间序列数据的状态在不同的时间段随机地发生转换。
这种转换可以用马尔可夫链来表示,其中每个时间段被定义为一个状态,而状态之间的转换概率由状态转移矩阵表示。
在VAR-MS模型中,时间序列数据被整体分为多个区域,并假设每个区域内的数据服从一个固定的向量自回归模型。
根据当前的状态,根据转移概率矩阵,模型会在不同的区域之间进行切换。
VAR-MS模型可以用以下的数学表达式表示:Y_t = μ_Z + A_ZY_{t-1} + ε_t其中,Y_t是一个n维向量,表示时间t时刻的观测数据;μ_Z是一个n维向量,表示在状态为Z时的截距项;A_Z是一个n×n的矩阵,表示在状态为Z时的系数矩阵;ε_t是一个n维向量,表示误差项,满足ε_t ∼ N(0, Σ_Z),其中Σ_Z是在状态为Z时的协方差矩阵。
VAR-MS模型的参数估计通常采用最大似然估计或贝叶斯估计方法。
在实际应用中,首先需要通过一些判别方法(如似然比检验或信息准则)来确定马尔可夫区制转换的状态数。
然后,使用EM算法或Gibbs采样等方法来估计模型的参数和状态序列。
VAR-MS模型在金融和经济领域具有广泛的应用。
2018考研:金融硕士考研——清华经院祝林老师个人介绍

2018考研:金融硕士考研——清华经院祝林老师个人介绍尽管人们都说考研需要完成一系列的工作量,历时长、任务繁重,但只要你花了足够多的时间、足够多的精力、以科学合理而行之有效的办法得相关信息,我们便可以说,考研其实并不难,而只是“会者不难、难者不会”。
现凯程教育为2018考研考生们分享相关重要的备考信息点拨。
祝林经济系助理教授办公室伟伦楼555个人简介研究成果研究项目清华大学经济管理学院助理教授。
祝林博士2004年获中国科学技术大学统计学学士学位;2006年获印第安纳大学伯明顿分校数学硕士学位;2012年获印第安纳大学伯明顿分校经济学博士学位。
他的主要研究兴趣包括部分识别计量模型的点集推断,半参数/非参数计量模型的估计与推断,时间序列计量经济学、金融计量经济学。
讲授课程有:计量经济学2(本科生课程)、经济学中的数学方法(研究生课程)、发表论文"AutomaticSpecificationTestingForVectorAutoregressionsandMultivariateNonlinearTimeSeriesM odels"(jointwithJ.C.Escanciano,I.N.Lobato),forthcominginJournalofBusiness&EconomicStatistics工作论文“InferencesinSemiparametricPartiallyIdentifiedModels:AnEmpiricalProcessApproach”(2012,withJ.C.Escanciano)“ASimpleData-DrivenEstimatorfortheSemiparametricSampleSelectionModel,”(R&R2012,withJ.C.Escanciano) “ASemiparametricTestforDriftSpecificationintheDiffusionModel,”(2010)会议SymposiuminFinancialEconometrics,IndianaUniversity,Poster “ASemiparametricTestforDriftSpecificationintheDiffusionModel,”March2009 EconometricsWorkshop,IndianaUniversity,“InferencesinSemiparametricTwo-StepPartiallyIdentifiedModels:AnEmpiricalProcessApproach,”December2011InternationalConferenceonMicroeconomicDataandEmpiric清华大学经济管理学院助理教授。
空间滞后模型和空间自回归模型

空间滞后模型和空间自回归模型空间滞后模型(Spatial Lag Model)和空间自回归模型(Spatial Autoregressive Model)是空间计量经济学中常用的两种模型,用于分析空间数据中的空间依赖性。
空间滞后模型是一种描述因变量与其邻近地区的自变量之间的依赖关系的模型。
它假设一个地区的因变量取决于该地区的自身特征以及其邻近地区的特征。
换句话说,该模型认为一个地区的因变量受到其邻近地区因变量的影响。
空间滞后模型可以用以下公式表示:Y = ρWy + Xβ + ε。
其中,Y是因变量,Wy是空间权重矩阵,ρ是空间滞后参数,X是自变量矩阵,β是自变量系数,ε是误差项。
空间滞后模型考虑了空间上的依赖性,可以用来解释因变量的空间聚集现象。
空间自回归模型是一种描述因变量与其邻近地区的因变量之间的依赖关系的模型。
它假设一个地区的因变量取决于该地区的自身特征以及其邻近地区的因变量。
换句话说,该模型认为一个地区的因变量受到其邻近地区因变量的影响。
空间自回归模型可以用以下公式表示:Y = ρWY + Xβ +ε。
其中,Y是因变量,W是空间权重矩阵,ρ是空间自回归参数,X是自变量矩阵,β是自变量系数,ε是误差项。
空间自回归模型考虑了空间上的依赖性,可以用来解释因变量的空间自相关现象。
这两种模型都考虑了空间上的依赖性,但是它们的依赖关系不同。
空间滞后模型是因变量与邻近地区的自变量之间的依赖关系,而空间自回归模型是因变量与邻近地区的因变量之间的依赖关系。
在实际应用中,选择使用哪种模型取决于具体问题和数据的特征。
总结起来,空间滞后模型和空间自回归模型是两种常用的空间计量经济学模型,用于分析空间数据中的空间依赖性。
它们都考虑了因变量与邻近地区之间的依赖关系,但是依赖关系的对象不同,一个是自变量,一个是因变量。
stata障碍度模型命令
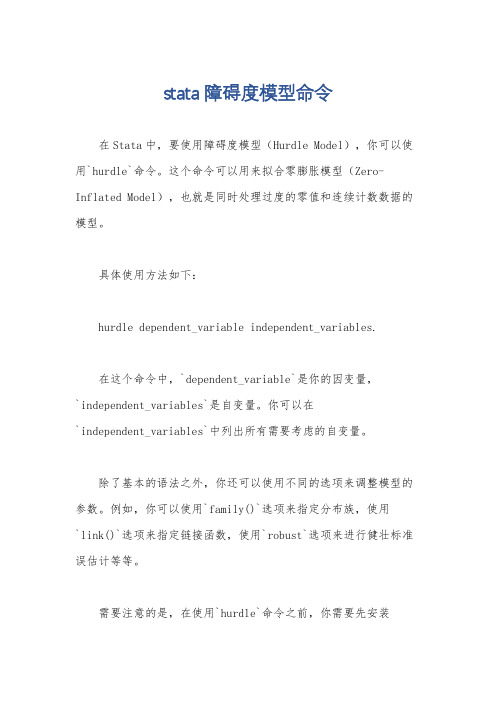
stata障碍度模型命令
在Stata中,要使用障碍度模型(Hurdle Model),你可以使用`hurdle`命令。
这个命令可以用来拟合零膨胀模型(Zero-Inflated Model),也就是同时处理过度的零值和连续计数数据的模型。
具体使用方法如下:
hurdle dependent_variable independent_variables.
在这个命令中,`dependent_variable`是你的因变量,
`independent_variables`是自变量。
你可以在
`independent_variables`中列出所有需要考虑的自变量。
除了基本的语法之外,你还可以使用不同的选项来调整模型的参数。
例如,你可以使用`family()`选项来指定分布族,使用
`link()`选项来指定链接函数,使用`robust`选项来进行健壮标准误估计等等。
需要注意的是,在使用`hurdle`命令之前,你需要先安装
`hurdle`命令,可以使用以下命令安装:
ssc install hurdle.
总的来说,通过使用`hurdle`命令,你可以在Stata中拟合障碍度模型,同时处理过度的零值和连续计数数据,从而进行更加全面和准确的统计分析。
四参数回归计算 程序
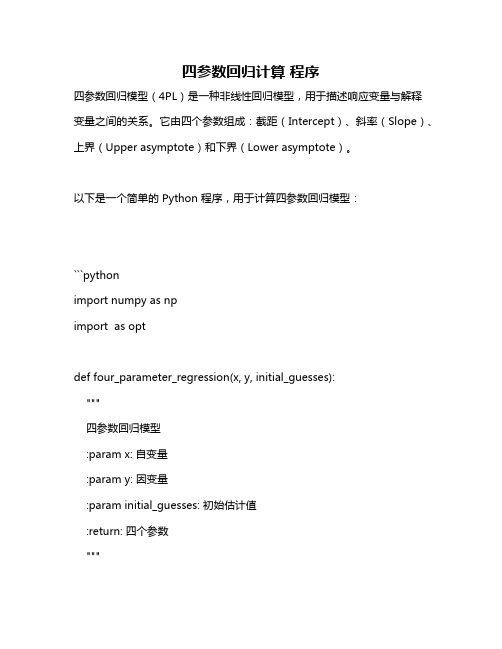
四参数回归计算程序四参数回归模型(4PL)是一种非线性回归模型,用于描述响应变量与解释变量之间的关系。
它由四个参数组成:截距(Intercept)、斜率(Slope)、上界(Upper asymptote)和下界(Lower asymptote)。
以下是一个简单的 Python 程序,用于计算四参数回归模型:```pythonimport numpy as npimport as optdef four_parameter_regression(x, y, initial_guesses):"""四参数回归模型:param x: 自变量:param y: 因变量:param initial_guesses: 初始估计值:return: 四个参数"""定义非线性回归模型def non_linear_model(params, x, y):intercept, slope, upper_asymptote, lower_asymptote = params y_predicted = (slope (1 - (x / upper_asymptote)) intercept) / (1 + (x / upper_asymptote)) + lower_asymptotereturn y_predicted - y定义优化器,使用BFGS方法bounds = [(0, None), (0, None), (None, None), (None, None)]result = (non_linear_model, initial_guesses, args=(x, y),bounds=bounds)提取参数intercept = [0]slope = [1]upper_asymptote = [2]lower_asymptote = [3]return intercept, slope, upper_asymptote, lower_asymptote```使用方法:1. 准备数据:将自变量 `x` 和因变量 `y` 存储在 NumPy 数组中。
stock_watson_jep2001vars
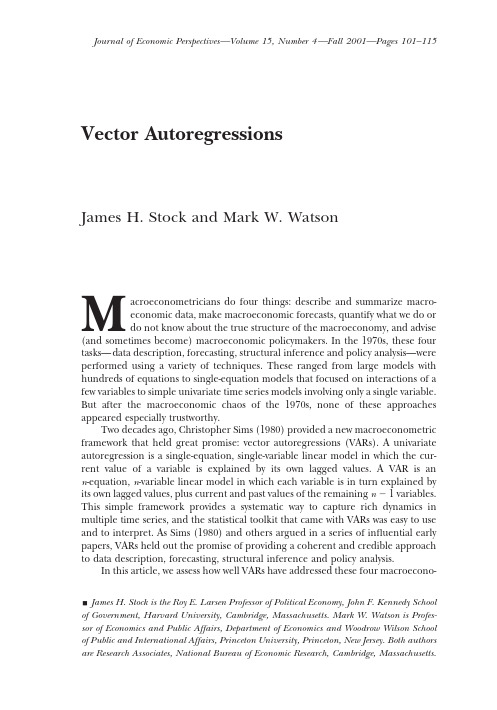
Journal of Economic Perspectives—Volume15,Number4—Fall2001—Pages101–115 Vector AutoregressionsJames H.Stock and Mark W.WatsonM acroeconometricians do four things:describe and summarize macro-economic data,make macroeconomic forecasts,quantify what we do ordo not know about the true structure of the macroeconomy,and advise (and sometimes become)macroeconomic policymakers.In the1970s,these four tasks—data description,forecasting,structural inference and policy analysis—were performed using a variety of techniques.These ranged from large models with hundreds of equations to single-equation models that focused on interactions of a few variables to simple univariate time series models involving only a single variable. But after the macroeconomic chaos of the1970s,none of these approaches appeared especially trustworthy.Two decades ago,Christopher Sims(1980)provided a new macroeconometric framework that held great promise:vector autoregressions(VARs).A univariate autoregression is a single-equation,single-variable linear model in which the cur-rent value of a variable is explained by its own lagged values.A VAR is an n-equation,n-variable linear model in which each variable is in turn explained by its own lagged values,plus current and past values of the remaining nϪ1variables. This simple framework provides a systematic way to capture rich dynamics in multiple time series,and the statistical toolkit that came with VARs was easy to use and to interpret.As Sims(1980)and others argued in a series of influential early papers,VARs held out the promise of providing a coherent and credible approach to data description,forecasting,structural inference and policy analysis.In this article,we assess how well VARs have addressed these four macroecono-y James H.Stock is the Roy rsen Professor of Political Economy,John F.Kennedy School of Government,Harvard University,Cambridge,Massachusetts.Mark W.Watson is Profes-sor of Economics and Public Affairs,Department of Economics and Woodrow Wilson School of Public and International Affairs,Princeton University,Princeton,New Jersey.Both authors are Research Associates,National Bureau of Economic Research,Cambridge,Massachusetts.102Journal of Economic Perspectivesmetric tasks.1Our answer is“it depends.”In data description and forecasting,VARs have proven to be powerful and reliable tools that are now,rightly,in everyday use. Structural inference and policy analysis are,however,inherently more difficult because they require differentiating between correlation and causation;this is the “identification problem,”in the jargon of econometrics.This problem cannot be solved by a purely statistical tool,even a powerful one like a VAR.Rather,economic theory or institutional knowledge is required to solve the identification(causation versus correlation)problem.A Peek Inside the VAR ToolkitWhat,precisely,is the effect of a100-basis-point hike in the federal funds interest rate on the rate of inflation one year hence?How big an interest rate cut is needed to offset an expected half percentage point rise in the unemployment rate?How well does the Phillips curve predict inflation?What fraction of the variation in inflation in the past40years is due to monetary policy as opposed to external shocks?Many macroeconomists like to think they know the answers to these and similar questions,perhaps with a modest range of uncertainty.In the next two sections,we take a quantitative look at these and related questions using several three-variable VARs estimated using quarterly U.S.data on the rate of price inflation(t),the unemployment rate(u t)and the interest rate(R t,specifically,the federal funds rate)from1960:I–2000:IV.2First,we construct and examine these models as a way to display the VAR toolkit;criticisms are reserved for the next section.VARs come in three varieties:reduced form,recursive and structural.A reduced form VAR expresses each variable as a linear function of its own past values,the past values of all other variables being considered and a serially uncor-related error term.Thus,in our example,the VAR involves three equations: current unemployment as a function of past values of unemployment,inflation and the interest rate;inflation as a function of past values of inflation,unemployment and the interest rate;and similarly for the interest rate equation.Each equation is estimated by ordinary least squares regression.The number of lagged values to include in each equation can be determined by a number of different methods, and we will use four lags in our examples.3The error terms in these regressions are the“surprise”movements in the variables after taking its past values into account. If the different variables are correlated with each other—as they typically are in 1Readers interested in more detail than provided in this brief tutorial should see Hamilton’s(1994) textbook or Watson’s(1994)survey article.ϭ400ln(P t/P tϪ1),where P t is the chain-weighted GDP price 2The inflation data are computed astindex and u t is the civilian unemployment rate.Quarterly data on u t and R t are formed by taking quarterly averages of their monthly values.3Frequently,the Akaike(AIC)or Bayes(BIC)information criteria are used;for a discussion,see Lu¨tkepohl(1993,chapter4).James H.Stock and Mark W.Watson103 macroeconomic applications—then the error terms in the reduced form model will also be correlated across equations.A recursive VAR constructs the error terms in each regression equation to be uncorrelated with the error in the preceding equations.This is done by judiciously including some contemporaneous values as regressors.Consider a three-variable VAR,ordered as1)inflation,2)the unemployment rate,and3)the interest rate. In thefirst equation of the corresponding recursive VAR,inflation is the dependent variable,and the regressors are lagged values of all three variables.In the second equation,the unemployment rate is the dependent variable,and the regressors are lags of all three variables plus the current value of the inflation rate.The interest rate is the dependent variable in the third equation,and the regressors are lags of all three variables,the current value of the inflation rate plus the current value of the unemployment rate.Estimation of each equation by ordinary least squares produces residuals that are uncorrelated across equations.4Evidently,the results depend on the order of the variables:changing the order changes the VAR equations,coefficients,and residuals,and there are n!recursive VARs representing all possible orderings.A structural VAR uses economic theory to sort out the contemporaneous links among the variables(Bernanke,1986;Blanchard and Watson,1986;Sims,1986). Structural VARs require“identifying assumptions”that allow correlations to be interpreted causally.These identifying assumptions can involve the entire VAR,so that all of the causal links in the model are spelled out,or just a single equation,so that only a specific causal link is identified.This produces instrumental variables that permit the contemporaneous links to be estimated using instrumental vari-ables regression.The number of structural VARs is limited only by the inventiveness of the researcher.In our three-variable example,we consider two related structural VARs.Each incorporates a different assumption that identifies the causal influence of monetary policy on unemployment,inflation and interest rates.Thefirst relies on a version of the“Taylor rule,”in which the Federal Reserve is modeled as setting the interest rate based on past rates of inflation and unemployment.5In this system,the Fed sets the federal funds rate R according to the ruleR tϭr*ϩ1.5͑tϪ*͒Ϫ1.25͑utϪu*͒ϩlagged values of R,,uϩt, where r*is the desired real rate of interest,t and ut are the average values of inflation and unemployment rate over the past four quarters,*and u*are the target values of inflation and unemployment,andt is the error in the equation. This relationship becomes the interest rate equation in the structural VAR.4In the jargon of VARs,this algorithm for estimating the recursive VAR coefficients is equivalent to estimating the reduced form,then computing the Cholesky factorization of the reduced form VAR covariance matrix;see Lu¨tkepohl(1993,chapter2).5Taylor’s(1993)original rule used the output gap instead of the unemployment rate.Our version uses Okun’s Law(with a coefficient of2.5)to replace the output gap with unemployment rate.104Journal of Economic PerspectivesThe equation error,t,can be thought of as a monetary policy“shock,”sinceit represents the extent to which actual interest rates deviate from this Taylor rule.This shock can be estimated by a regression with R tϪ1.5tϩ1.25ut as the dependent variable,and a constant and lags of interest rates,unemployment andinflation on the right-hand side.The Taylor rule is“backward looking”in the sense that the Fed reacts to pastinformation(t and ut are averages of the past four quarters of inflation and unemployment),and several researchers have argued that Fed behavior is more appropriately described by forward-looking behavior.Because of this,we consider another variant of the model in which the Fed reacts to forecasts of inflation and unemployment four quarters in the future.This Taylor rule has the same form as the rule above,but witht and ut replaced by four-quarter ahead forecasts com-puted from the reduced form VAR.Putting the Three-Variable VAR Through Its PacesThe different versions of the inflation-unemployment-interest rate VAR are put through their paces by applying them to the four macroeconometric tasks. First,the reduced form VAR and a recursive VAR are used to summarize the comovements of these three series.Second,the reduced form VAR is used to forecast the variables,and its performance is assessed against some alternative benchmark models.Third,the two different structural VARs are used to estimate the effect of a policy-induced surprise move in the federal funds interest rate on future rates of inflation and unemployment.Finally,we discuss how the structural VAR could be used for policy analysis.Data DescriptionStandard practice in VAR analysis is to report results from Granger-causality tests,impulse responses and forecast error variance decompositions.These statistics are computed automatically(or nearly so)by many econometrics packages(RATS, Eviews,TSP and others).Because of the complicated dynamics in the VAR,these statistics are more informative than are the estimated VAR regression coefficients or R2statistics,which typically go unreported.Granger-causality statistics examine whether lagged values of one variable help to predict another variable.For example,if the unemployment rate does not help predict inflation,then the coefficients on the lags of unemployment will all be zero in the reduced-form inflation equation.Panel A of Table1summarizes the Granger-causality results for the three-variable VAR.It shows the p-values associated with the F-statistics for testing whether the relevant sets of coefficients are zero.The unemployment rate helps to predict inflation at the5percent significance level (the p-value is0.02,or2percent),but the federal funds interest rate does not(the p-value is0.27).Inflation does not help to predict the unemployment rate,but the federal funds rate does.Both inflation and the unemployment rates help predict the federal funds interest rate.Table1VAR Descriptive Statistics for(,u,R)A.Granger-Causality TestsRegressorDependent Variable in Regressionu R0.000.310.00 u0.020.000.00 R0.270.010.00 B.Variance Decompositions from the Recursive VAR Ordered as,u,RB.i.Variance Decomposition ofForecast HorizonForecastStandard ErrorVariance Decomposition(Percentage Points)u R10.9610000 4 1.3488102 8 1.758217112 1.9782162B.ii.Variance Decomposition of uForecast HorizonForecastStandard ErrorVariance Decomposition(Percentage Points)u R10.231990 40.640982 80.7978211 120.92166618B.iii.Variance Decomposition of RForecast HorizonForecastStandard ErrorVariance Decomposition(Percentage Points)u R10.85219794 1.84950418 2.4412602812 2.63165925Notes:denotes the rate of price inflation,u denotes the unemploy-ment rate and R denotes the Federal Funds interest rate.The entriesin Panel A show the p-values for F-tests that lags of the variable in therow labeled Regressor do not enter the reduced form equation for thecolumn variable labeled Dependent Variable.The results were com-puted from a VAR with four lags and a constant term over the1960:I–2000:IV sample period.Vector Autoregressions105106Journal of Economic PerspectivesImpulse responses trace out the response of current and future values of each of the variables to a one-unit increase in the current value of one of the VAR errors, assuming that this error returns to zero in subsequent periods and that all other errors are equal to zero.The implied thought experiment of changing one error while holding the others constant makes most sense when the errors are uncorre-lated across equations,so impulse responses are typically calculated for recursive and structural VARs.The impulse responses for the recursive VAR,orderedt,u t,R t,are plotted in Figure1.Thefirst row shows the effect of an unexpected1percentage point increase in inflation on all three variables,as it works through the recursive VAR system with the coefficients estimated from actual data.The second row shows the effect of an unexpected increase of1percentage point in the unemployment rate, and the third row shows the corresponding effect for the interest rate.Also plotted areϮ1standard error bands,which yield an approximate66percent confidence interval for each of the impulse responses.These estimated impulse responses show patterns of persistent common variation.For example,an unexpected rise in inflation slowly fades away over24quarters and is associated with a persistent increase in unemployment and interest rates.The forecast error decomposition is the percentage of the variance of the error made in forecasting a variable(say,inflation)due to a specific shock(say,the error term in the unemployment equation)at a given horizon(like two years).Thus,the forecast error decomposition is like a partial R2for the forecast error,by forecast horizon.These are shown in Panel B of Table1for the recursive VAR.They suggest considerable interaction among the variables.For example,at the12-quarter horizon,75percent of the error in the forecast of the federal funds interest rate is attributed to the inflation and unemployment shocks in the recursive VAR. ForecastingMultistep-ahead forecasts,computed by iterating forward the reduced form VAR,are assessed in Table2.Because the ultimate test of a forecasting model is its out-of-sample performance,Table2focuses on pseudo out-of-sample forecasts over the period from1985:I to2000:IV.It examines forecast horizons of two quarters, four quarters and eight quarters.The forecast h steps ahead is computed by estimating the VAR through a given quarter,making the forecast h steps ahead, reestimating the VAR through the next quarter,making the next forecast and so on through the forecast period.6As a comparison,pseudo out-of-sample forecasts were also computed for a univariate autoregression with four lags—that is,a regression of the variable on lags 6Forecasts like these are often referred to as pseudo or“simulated”out-of-sample forecasts to emphasize that they simulate how these forecasts would have been computed in real time,although,of course,this exercise is conducted retrospectively,not in real time.Our experiment deviates slightly from what would have been computed in real time because we use the current data,which includes later revisions made to the inflation and unemployment data by statistical agencies,rather than the data available in real time.of its own past values—and for a random walk (or “no change”)forecast.Inflation rate forecasts were made for the average value of inflation over the forecast period,while forecasts for the unemployment rate and interest rate were made for the final quarter of the forecast period.Table 2shows the root mean square forecast error for each of the forecasting methods.(The mean squared forecast error is computed as the average squared value of the forecast error over the 1985–2000out-of-sample period,and the resulting square root is the root mean squared forecast error reported in the table.)Table 2indicates that the VAR either does no worse than or improves upon the univariate autoregression and that both improve upon the random walk forecast.Structural InferenceWhat is the effect on the rates of inflation and unemployment of a surprise 100basis–point increase in the federal funds interest rate?Translated into VAR jargon,Figure 1Impulse Responses in the Inflation-Unemployment-Interest Rate RecursiveVAR James H.Stock and Mark W.Watson 107this question becomes:What are the impulse responses of the rates of inflation and unemployment to the monetary policy shock in a structural VAR?The solid line in Figure 2plots the impulse responses computed from our model with the backward-looking Taylor rule.It shows the inflation,unemploy-ment and real interest rate (R t Ϫt )responses to a 1percentage point shock in the nominal federal funds rate.The initial rate hike results in the real interest rate exceeding 50basis points for six quarters.Although inflation is eventually reduced by approximately 0.3percentage points,the lags are long,and most of the action occurs in the third year after the contraction.Similarly,the rate of unemployment rises by approximately 0.2percentage points,but most of the economic slowdown is in the third year after the rate hike.How sensitive are these results to the specific identifying assumption used in this structural VAR—that the Fed follows the backward-looking Taylor rule?As it happens,very sensitive.The dashed line in Figure 2plots the impulse responses computed from the structural VAR with the forward-looking Taylor rule.The impulse responses in real interest rates are broadly similar under either rule.However,in the forward-looking model the monetary shock produces a 0.5per-centage point increase in the unemployment rate within a year,and the rate of inflation drops sharply at first,fluctuates,then leaves a net decline of 0.5percent-age points after six years.Under the backward-looking rule,this 100basis-point rate hike produces a mild economic slowdown and a modest decline in inflation several years hence;under the forward-looking rule,by this same action the Fed wins a major victory against inflation at the cost of a swift and sharp recession.Policy AnalysisIn principle,our small structural VAR can be used to analyze two types of policies:surprise monetary policy interventions and changing the policy rule,like shifting from a Taylor rule (with weight on both unemployment and inflation)to an explicit inflation targeting rule.Table 2Root Mean Squared Errors of Simulated Out-Of-Sample Forecasts,1985:1–2000:IVForecastHorizonInflation RateUnemployment Rate Interest Rate RW AR VAR RW AR VAR RW AR VAR 2quarters0.820.700.680.340.280.290.790.770.684quarters0.730.650.630.620.520.53 1.36 1.25 1.078quarters 0.750.750.75 1.120.950.78 2.18 1.92 1.70Notes:Entries are the root mean squared error of forecasts computed recursively for univariate and vector autoregressions (each with four lags)and a random walk (“no change”)model.Results for the random walk and univariate autoregressions are shown in columns labeled RW and AR,respectively.Each model was estimated using data from 1960:I through the beginning of the forecast period.Forecasts for the inflation rate are for the average value of inflation over the period.Forecasts for the unemployment rate and interest rate are for the final quarter of the forecast period.108Journal of Economic PerspectivesIf the intervention is an unexpected movement in the federal funds interest rate,then the estimated effect of this policy on future rates of inflation and unemployment is summarized by the impulse response functions plotted in Figure2.This might seem a somewhat odd policy,but the same mechanics can be used to evaluate a more realistic intervention,such as raising the federal funds rate by 50basis points and sustaining this increase for one year.This policy can be engineered in a VAR by using the right sequence of monetary policy innovations to hold the federal funds interest rate at this sustained level for four quarters,taking into account that in the VAR,actions on interest rates in earlier quarters affect those in later quarters (Sims,1982;Waggoner and Zha,1999).Analysis of the second type of policy—a shift in the monetary rule itself—is more complicated.One way to evaluate a new policy rule candidate is to ask what would be the effect of monetary and nonmonetary shocks on the economy under the new rule.Since this question involves all the structural disturbances,answering Figure 2Impulse Responses of Monetary Policy Shocks for Different Taylor Rule IdentifyingAssumptionsNotes:The solid line is computed with the backward-looking Taylor rule;the dashed line,with the forward-looking Taylor rule.Vector Autoregressions 109110Journal of Economic Perspectivesit requires a complete macroeconomic model of the simultaneous determination of all the variables,and this means that all of the causal links in the structural VAR must be specified.In this case,policy analysis is carried out as follows:a structural VAR is estimated in which all the equations are identified,then a new model is formed by replacing the monetary policy paring the impulse responses in the two models shows how the change in policy has altered the effects of monetary and nonmonetary shocks on the variables in the model.How Well Do VARs Perform the Four Tasks?We now turn to an assessment of VARs in performing the four macroecono-metric tasks,highlighting both successes and shortcomings.Data DescriptionBecause VARs involve current and lagged values of multiple time series,they capture comovements that cannot be detected in univariate or bivariate models. Standard VAR summary statistics like Granger-causality tests,impulse response functions and variance decompositions are well-accepted and widely used methods for portraying these comovements.These summary statistics are useful because they provide targets for theoretical macroeconomic models.For example,a theoretical model that implied that interest rates should Granger-cause inflation but unem-ployment should not would be inconsistent with the evidence in Table1.Of course,the VAR methods outlined here have some limitations.One is that the standard methods of statistical inference(such as computing standard errors for impulse responses)may give misleading results if some of the variables are highly persistent.7Another limitation is that,without modification,standard VARs miss nonlinearities,conditional heteroskedasticity and drifts or breaks in parameters.ForecastingSmall VARs like our three-variable system have become a benchmark against which new forecasting systems are judged.But while useful as a benchmark,small VARs of two or three variables are often unstable and thus poor predictors of the future(Stock and Watson,1996).State-of-the-art VAR forecasting systems contain more than three variables and allow for time-varying parameters to capture important drifts in coefficients(Sims, 1993).However,adding variables to the VAR creates complications,because the number of VAR parameters increases as the square of the number of variables:a nine-variable,four-lag VAR has333unknown coefficients(including the inter-7Bootstrap methods provide some improvements(Kilian,1999)for inference about impulse responses, but treatments of this problem that are fully satisfactory theoretically are elusive(Stock,1997;Wright, 2000).James H.Stock and Mark W.Watson111 cepts).Unfortunately,macroeconomic time series data cannot provide reliable estimates of all these coefficients without further restrictions.One way to control the number of parameters in large VAR models is to impose a common structure on the coefficients,for example using Bayesian meth-ods,an approach pioneered by Litterman(1986)(six variables)and Sims(1993) (nine variables).These efforts have paid off,and these forecasting systems have solid real-time track records(McNees,1990;Zarnowitz and Braun,1993). Structural InferenceIn our three-variable VAR in the previous section,the estimated effects of a monetary policy shock on the rates of inflation and unemployment(summarized by the impulse responses in Figure2)depend on the details of the presumed mone-tary policy rule followed by the Federal Reserve.Even modest changes in the assumed rule resulted in substantial changes in these impulse responses.In other words,the estimates of the structural impulse responses hinge on detailed institu-tional knowledge of how the Fed sets interest rates.8Of course,the observation that results depend on assumptions is hardly new. The operative question is whether the assumptions made in VAR models are any more compelling than in other econometric models.This is a matter of heated debate and is thoughtfully discussed by Leeper,Sims and Zha(1996),Christiano, Eichenbaum and Evans(1999),Cochrane(1998),Rudebusch(1998)and Sims (1998).Below are three important criticisms of structural VAR modeling.9 First,what really makes up the VAR“shocks?”In large part,these shocks,like those in conventional regression,reflect factors omitted from the model.If these factors are correlated with the included variables,then the VAR estimates will contain omitted variable bias.For example,officials at the Federal Reserve might scoff at the idea that they mechanically followed a Taylor rule,or any other fixed-coefficient mechanical rule involving only a few variables;rather,they suggest that their decisions are based on a subtle analysis of very many macroeconomic factors,both quantitative and qualitative.These considerations,when omitted from the VAR,end up in the error term and(incorrectly)become part of the estimated historical“shock”used to estimate an impulse response.A concrete example of this in the VAR literature involves the“price puzzle.”Early VARs showed an odd result: inflation tended to increase following monetary policy tightening.One explanation for this(Sims,1992)was that the Fed was looking forward when it set interest rates and that simple VARs omitted variables that could be used to predict future inflation.When these omitted variables intimated an increase in inflation,the Fed tended to increase interest rates.Thus,these VAR interest rate shocks presaged 8In addition,the institutional knowledge embodied in our three-variable VAR is rather naı¨ve;for example,the Taylor rule was designed to summarize policy in the Greenspan era,not the full sample in our paper.9This list hits only the highlights;other issues include the problem of“weak instruments”discussed in Pagan and Robertson(1998)and the problem of noninvertible representations discussed in Hansen and Sargent(1991)and Lippi and Reichlin(1993).112Journal of Economic Perspectivesincreases in inflation.Because of omitted variables,the VAR mistakenly labeled these increases in interest rates as monetary shocks,which led to biased impulse responses.Indeed,Sims’s explanation of the price puzzle has led to the practice of including commodity prices in VARs to attempt to control for predicted future inflation.Second,policy rules change over time,and formal statistical tests reveal widespread instability in low-dimensional VARs(Stock and Watson,1996).Con-stant parameter structural VARs that miss this instability are improperly identified. For example,several researchers have documented instability in monetary policy rules(for example,Bernanke and Blinder,1992;Bernanke and Mihov,1998; Clarida,Gali and Gertler,2000;Boivin,2000),and this suggests misspecification in constant coefficient VAR models(like our three-variable example)that are esti-mated over long sample periods.Third,the timing conventions in VARs do not necessarily reflect real-time data availability,and this undercuts the common method of identifying restrictions based on timing assumptions.For example,a common assumption made in struc-tural VARs is that variables like output and inflation are sticky and do not respond “within the period”to monetary policy shocks.This seems plausible over the period of a single day,but becomes less plausible over a month or quarter.In this discussion,we have carefully distinguished between recursive and structural VARs:recursive VARs use an arbitrary mechanical method to model contemporaneous correlation in the variables,while structural VARs use economic theory to associate these correlations with causal relationships.Unfortunately,in the empirical literature the distinction is often murky.It is tempting to develop economic“theories”that,conveniently,lead to a particular recursive ordering of the variables,so that their“structural”VAR simplifies to a recursive VAR,a structure called a“Wold causal chain.”We think researchers yield to this temptation far too often.Such cobbled-together theories,even if superficially plausible,often fall apart on deeper inspection.Rarely does it add value to repackage a recursive VAR and sell it as structural.Despite these criticisms,we think it is possible to have credible identifying assumptions in a VAR.One approach is to exploit detailed institutional knowledge. An example of this is the study by Blanchard and Perotti(1999)of the macroeco-nomic effects offiscal policy.They argue that the tax code and spending rules impose tight constraints on the way that taxes and spending vary within the quarter, and they use these constraints to identify the exogenous changes in taxes and spending necessary for causal analysis.Another example is Bernanke and Mihov (1998),who use a model of the reserves market to identify monetary policy shocks.A different approach to identification is to use long-run restrictions to identify shocks;for example,King,Plosser,Stock and Watson(1991)use the long-run neutrality of money to identify monetary shocks.However,assumptions based on the infinite future raise questions of their own(Faust and Leeper,1997).A constructive approach is to recognize explicitly the uncertainty in the assumptions that underlie structural VAR analysis and see what inferences,or range of inferences,still can be made.For example,Faust(1998)and Uhlig(1999)。
自相关的相关估计方法

自相关的相关估计方法自相关是一种衡量时间序列数据中各个时刻之间相关性的方法。
在时间序列分析中,自相关函数(ACF)被广泛用于研究数据的自相关性,并且可以通过自相关函数进行相关估计。
自相关函数的定义是指一个时间序列与其自身在不同滞后期的相关性。
简单来说,自相关系数表示一个时间序列在不同时间滞后下的相关性强度。
自相关函数可以用于寻找时间序列中的周期性和重复模式,以及预测未来趋势和波动。
在进行自相关估计时,需要首先计算自相关函数。
常用的自相关函数估计方法有以下几种:1. 样本自相关函数(Sample Autocorrelation Function,SACF):样本自相关函数是通过计算实际观测值之间的相关性来估计自相关函数。
这种方法是最直接的估计方法,通常用于较短的时间序列。
2. 经验自相关函数(Empirical Autocorrelation Function,EACF):经验自相关函数是通过对时间序列进行拟合,得到拟合残差序列的自相关来估计自相关函数。
这种方法是一种非参数估计方法,不需要对时间序列的分布做出假设。
3. 动态自相关函数(Dynamic Autocorrelation Function,DACF):动态自相关函数是通过建立动态模型,计算模型的残差序列的自相关来估计自相关函数。
这种方法通常适用于具有长期相关性的时间序列。
4. 超前影响函数(Impulse Response Function,IRF):超前影响函数是通过对外生冲击变量的动态影响进行估计,来估计系统自相关函数。
这种方法通常用于估计多变量时间序列模型的自相关。
自相关函数的估计结果可以用于判断时间序列是否存在相关性、相关性的强度和方向,以及预测未来的变化趋势。
通过自相关函数的分析,可以为时间序列建立适当的模型,以进一步分析和预测时间序列的特征和行为。
总结起来,自相关函数是一种用于估计时间序列中各个时刻之间相关性的方法。
通过计算自相关函数,可以了解时间序列的自相关性和相关性的强度。
优化机器学习模型的集成方法与技巧
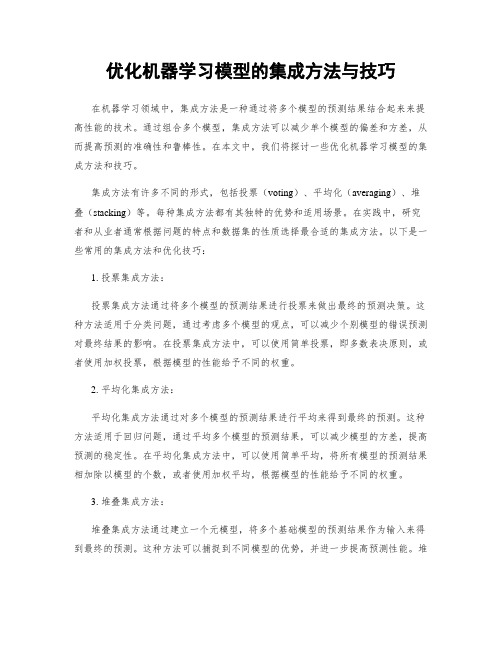
优化机器学习模型的集成方法与技巧在机器学习领域中,集成方法是一种通过将多个模型的预测结果结合起来来提高性能的技术。
通过组合多个模型,集成方法可以减少单个模型的偏差和方差,从而提高预测的准确性和鲁棒性。
在本文中,我们将探讨一些优化机器学习模型的集成方法和技巧。
集成方法有许多不同的形式,包括投票(voting)、平均化(averaging)、堆叠(stacking)等。
每种集成方法都有其独特的优势和适用场景。
在实践中,研究者和从业者通常根据问题的特点和数据集的性质选择最合适的集成方法。
以下是一些常用的集成方法和优化技巧:1. 投票集成方法:投票集成方法通过将多个模型的预测结果进行投票来做出最终的预测决策。
这种方法适用于分类问题,通过考虑多个模型的观点,可以减少个别模型的错误预测对最终结果的影响。
在投票集成方法中,可以使用简单投票,即多数表决原则,或者使用加权投票,根据模型的性能给予不同的权重。
2. 平均化集成方法:平均化集成方法通过对多个模型的预测结果进行平均来得到最终的预测。
这种方法适用于回归问题,通过平均多个模型的预测结果,可以减少模型的方差,提高预测的稳定性。
在平均化集成方法中,可以使用简单平均,将所有模型的预测结果相加除以模型的个数,或者使用加权平均,根据模型的性能给予不同的权重。
3. 堆叠集成方法:堆叠集成方法通过建立一个元模型,将多个基础模型的预测结果作为输入来得到最终的预测。
这种方法可以捕捉到不同模型的优势,并进一步提高预测性能。
堆叠集成方法需要更多的计算资源和时间,但通常可以获得更好的性能。
在堆叠集成方法中,基础模型可以使用不同的算法,例如决策树、支持向量机、神经网络等。
除了选择适当的集成方法,还有一些技巧可以进一步优化机器学习模型的集成:1. 多样化基模型:为了提高集成方法的性能,基础模型应该具有多样性。
这意味着使用不同的算法、不同的特征子集或不同的训练数据来训练基础模型。
多样性可以增加模型的学习能力,提高集成的鲁棒性。
多项式回归与响应面分析的原理及应用
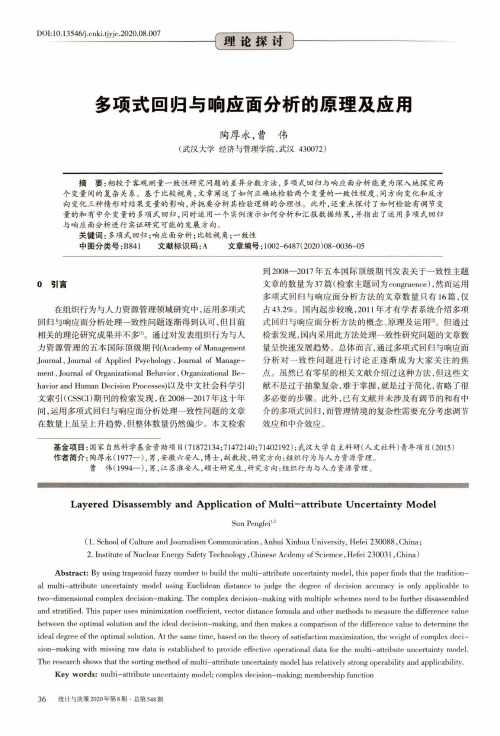
D01:10.13546/ki.tjyjc.2020.08.007 广他~----------------!理论探讨多项式回归与响应面分析的原理及应用陶厚永,曹伟(武汉大学经济与管理学院,武汉430072)摘要:相较于客观测量一致性研究问题的差异分数方法,多项式回归与响应面分析能更为深入地探究两 个变量间的复杂关系。
基于比较视角,文章阐述了如何正确地检验两个变量的一致性程度、同方向变化和反方 向变化三种情形对结果变量的影响,并扼要分析其检验逻辑的合理性。
此外,还重点探讨了如何检验有调节变 量的和有中介变量的多项式回归,同时运用一个实例演示如何分析和汇报数据结果,并指出了运用多项式回归 与响应面分析进行实证研究可能的发展方向。
关键词:多项式回归;响应面分析;比较视角;一致性中图分类号:B841 文献标识码:A 文章编号:1002-6487(2020)08-0036-050引言在组织行为与人力资源管理领域研究中,运用多项式 回归与响应面分析处理一致性问题逐渐得到认可,但目前 相关的理论研究成果并不多'通过对发表组织行为与人 力资源管理的五本国际顶级期刊(Academy of Management Joumal、JournalofAppliedPsychology、JoumalofManage—ment、JoumaIofOrganizationalBehavior、OrganizationalBe—havior and Human Decision Processes)以及中文社会科学引 文索引(CSSCI)期刊的检索发现,在2008—2017年这十年 间,运用多项式回归与响应面分析处理一致性问题的文章 在数量上虽呈上升趋势,但整体数量仍然偏少。
本文检索到2008—2017年五本国际顶级期刊发表关于一致性主题 文章的数量为37篇(检索主题词为congruence),然而运用 多项式回归与响应面分析方法的文章数量只有16篇,仅 占43.2%。
Autoregressive模型

Autoregressive模型简介Autoregressive模型是一种时间序列预测模型,它假设当前时间点的值与前一时间点的值有关。
该模型的核心思想是利用过去的观测值来预测未来的观测值,通过建立当前时刻的观测值与一系列过去观测值之间的关系,来进行预测。
模型原理Autoregressive模型的基本原理是利用自相关的概念来描述当前时间点的观测值与过去时间点的观测值之间的关系。
模型的一般形式为:其中,Y<sub>t</sub>表示当前时间点的观测值,Y<sub>t-1</sub>表示前一时间点的观测值,ε<sub>t</sub>表示误差项,p表示历史观测值的阶数。
φ<sub>1</sub>, φ<sub>2</sub>, ...,φ<sub>p</sub> 是参数,它们表示该模型的自回归系数。
在实际应用中,我们需要先确定模型的阶数p,然后通过最小二乘法或其他参数估计方法,获得模型的自回归系数。
模型应用Autoregressive模型广泛应用于时间序列预测领域,在经济学、气象学、金融学等领域都有重要应用。
在经济学中,Autoregressive模型被用于预测国民经济指标、股票价格等,从而为政策制定和投资决策提供参考。
在气象学中,Autoregressive模型被用于天气预报,通过分析过去的气象观测数据,预测未来的天气状况。
在金融学中,Autoregressive模型被用于时间序列分析、股票价格预测等,为投资者提供决策参考。
模型优缺点Autoregressive模型的优点包括:- 提供了一种简单而有效的时间序列预测方法。
- 基于自回归系数的估计,可以为数据提供解释性。
- 可以对多个时间序列进行建模,捕捉它们之间的动态关系。
然而,Autoregressive模型也存在一些缺点:- 需要确定模型的阶数,选择合适的阶数对模型的拟合效果有很大影响。
Vector Autoregressive Models
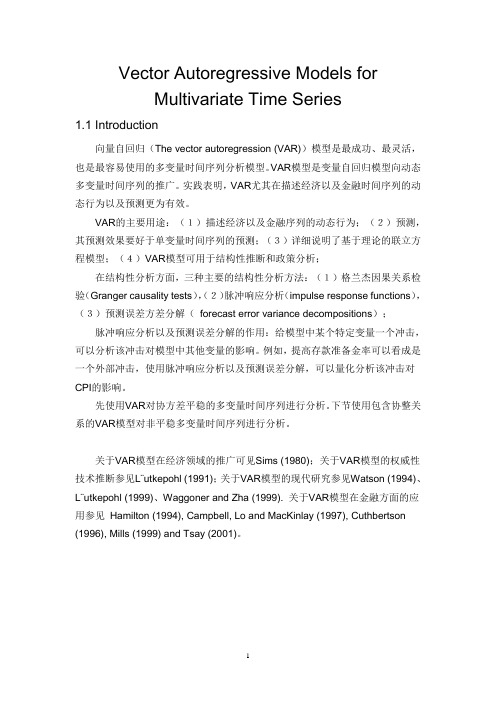
Vector Autoregressive Models forMultivariate Time Series1.1 Introduction向量自回归(The vector autoregression (VAR))模型是最成功、最灵活,也是最容易使用的多变量时间序列分析模型。
VAR模型是变量自回归模型向动态多变量时间序列的推广。
实践表明,VAR尤其在描述经济以及金融时间序列的动态行为以及预测更为有效。
VAR的主要用途:(1)描述经济以及金融序列的动态行为;(2)预测,其预测效果要好于单变量时间序列的预测;(3)详细说明了基于理论的联立方程模型;(4)VAR模型可用于结构性推断和政策分析;在结构性分析方面,三种主要的结构性分析方法:(1)格兰杰因果关系检验(Granger causality tests),(2)脉冲响应分析(impulse response functions),(3)预测误差方差分解(forecast error variance decompositions);脉冲响应分析以及预测误差分解的作用:给模型中某个特定变量一个冲击,可以分析该冲击对模型中其他变量的影响。
例如,提高存款准备金率可以看成是一个外部冲击,使用脉冲响应分析以及预测误差分解,可以量化分析该冲击对CPI的影响。
先使用VAR对协方差平稳的多变量时间序列进行分析。
下节使用包含协整关系的VAR模型对非平稳多变量时间序列进行分析。
关于VAR模型在经济领域的推广可见Sims (1980);关于VAR模型的权威性技术推断参见L¨utkepohl (1991);关于VAR模型的现代研究参见Watson (1994)、L¨utkepohl (1999)、Waggoner and Zha (1999). 关于VAR模型在金融方面的应用参见Hamilton (1994), Campbell, Lo and MacKinlay (1997), Cuthbertson (1996), Mills (1999) and Tsay (2001)。
Stata:面板向量自回归PVAR
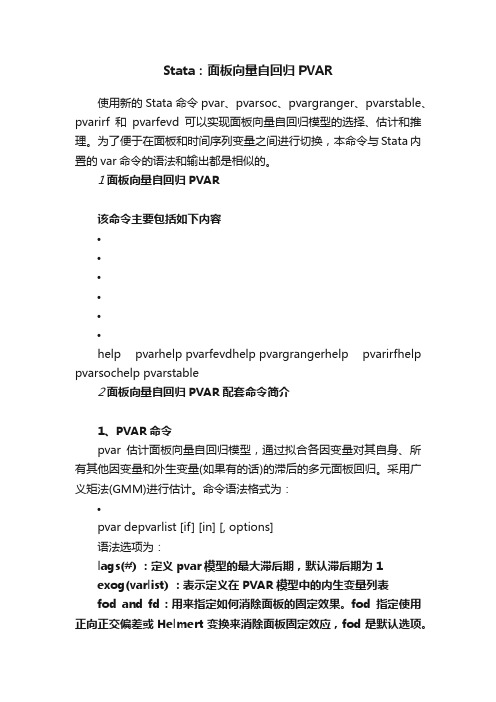
Stata:面板向量自回归PVAR使用新的Stata命令pvar、pvarsoc、pvargranger、pvarstable、pvarirf和pvarfevd可以实现面板向量自回归模型的选择、估计和推理。
为了便于在面板和时间序列变量之间进行切换,本命令与Stata内置的var命令的语法和输出都是相似的。
1面板向量自回归PVAR该命令主要包括如下内容••••••help pvarhelp pvarfevdhelp pvargrangerhelp pvarirfhelp pvarsochelp pvarstable2面板向量自回归PVAR配套命令简介1、PVAR命令pvar估计面板向量自回归模型,通过拟合各因变量对其自身、所有其他因变量和外生变量(如果有的话)的滞后的多元面板回归。
采用广义矩法(GMM)进行估计。
命令语法格式为:•pvar depvarlist [if] [in] [, options]语法选项为:lags(#) :定义pvar模型的最大滞后期,默认滞后期为1exog(varlist) :表示定义在PVAR模型中的内生变量列表fod and fd:用来指定如何消除面板的固定效果。
fod指定使用正向正交偏差或Helmert变换来消除面板固定效应,fod是默认选项。
fd规定了使用一阶差分而不是正向正交偏差来消除特定于面板的固定效应。
td:表示减去模型中每个变量在估计之前的横截面均值。
这可以用于在任何其他转换之前从所有变量中删除固定时间的效果。
gmmstyle指定使用Holtz-Eakin、Newey和Rosen(1988)提出的“GMM-style”工具。
gmmopts(options)覆盖pvar运行的默认gmm选项。
可以使用depvarlist中的变量名作为方程名分别访问模型中的每个方程。
vce(vcetype[, independent])指定报告的标准误差类型overid指定要报告Hansen的J统计量的过度识别限制。
向量自回归时间序列介绍
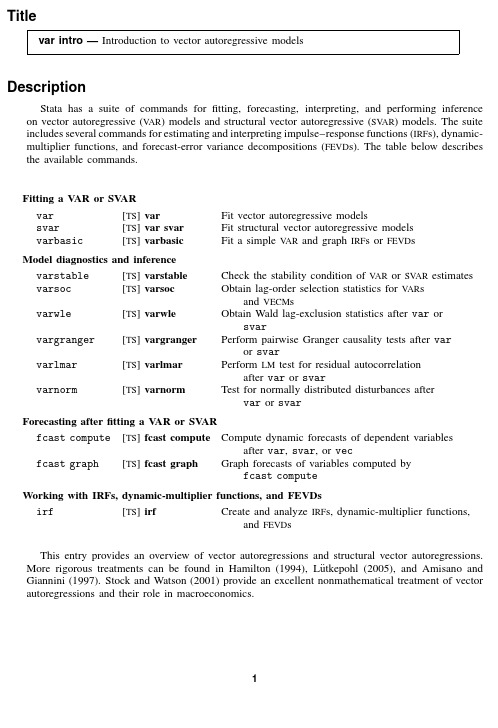
var intro — Introduction to vector autoregressive models
Description
Stata has a suite of commands for fitting, forecasting, interpreting, and performing inference on vector autoregressive (VAR) models and structural vector autoregressive (SVAR) models. The suite includes several commands for estimating and interpreting impulse–response functions (IRFs), dynamicmultiplier functions, and forecast-error variance decompositions (FEVDs). The table below describes the available commands.
E(ut) = 0, E(utut) = Σ, and E(utus) = 0 for t = s
There are K2 × p + K × (M (s + 1) + 1) parameters in the equation for yt, and there are {K × (K + 1)}/2 parameters in the covariance matrix Σ. One way to reduce the number of parameters is to specify an incomplete VAR, in which some of the A or B matrices are set to zero. Another way is to specify linear constraints on some of the coefficients in the VAR.
- 1、下载文档前请自行甄别文档内容的完整性,平台不提供额外的编辑、内容补充、找答案等附加服务。
- 2、"仅部分预览"的文档,不可在线预览部分如存在完整性等问题,可反馈申请退款(可完整预览的文档不适用该条件!)。
- 3、如文档侵犯您的权益,请联系客服反馈,我们会尽快为您处理(人工客服工作时间:9:00-18:30)。
Estimating Vector Autoregressions with Panel Data
Author(s): Douglas Holtz-Eakin, Whitney Newey, Harvey S. Rosen
Source: Econometrica, Vol. 56, No. 6 (Nov., 1988), pp. 1371-1395
Published by: The Econometric Society
Stable URL: /stable/1913103
Accessed: 24/11/2009 02:57
Your use of the JSTOR archive indicates your acceptance of JSTOR's Terms and Conditions of Use, available at
/page/info/about/policies/terms.jsp. JSTOR's Terms and Conditions of Use provides, in part, that unless you have obtained prior permission, you may not download an entire issue of a journal or multiple copies of articles, and you may use content in the JSTOR archive only for your personal, non-commercial use.
Please contact the publisher regarding any further use of this work. Publisher contact information may be obtained at
/action/showPublisher?publisherCode=econosoc.
Each copy of any part of a JSTOR transmission must contain the same copyright notice that appears on the screen or printed page of such transmission.
JSTOR is a not-for-profit service that helps scholars, researchers, and students discover, use, and build upon a wide range of content in a trusted digital archive. We use information technology and tools to increase productivity and facilitate new forms of scholarship. For more information about JSTOR, please contact support@.
The Econometric Society is collaborating with JSTOR to digitize, preserve and extend access to Econometrica.。