Personal and Ubiquitous Computing manuscript No. (will be inserted by the editor) A Taxonom
阿兰·麦席森·图灵

阿兰·麦席森·图灵百科名片图灵年轻时的照片图灵是英国著名的数学家和逻辑学家,被称为计算机科学之父、人工智能之父,是计算机逻辑的奠基者,提出了“图灵机”和“图灵测试”等重要概念。
人们为纪念其在计算机领域的卓越贡献而设立“图灵奖”。
中文名:阿兰·麦席森·图灵外文名:Alan Mathison Turing 国籍:英国民族:英格兰出生地:英国伦敦出生日期:1912年6月23日逝世日期:1954年6月7日职业:数学家及计算机逻辑学家毕业院校:剑桥大学国王学院信仰:科学主要成就:提出“图灵机”概念提出“图灵测试”概念开创非线性力学破解德国密码系统Enigma 代表作品:《论数字计算在决断难题中的应用》,《机器能思考吗?》目录[隐藏]人物履历人物生平人物大事年表图灵奖图灵机图灵实验人物履历人物生平人物大事年表图灵奖图灵机图灵实验[编辑本段]人物履历阿兰·麦席森·图灵(Alan Mathison Turing,1912.6.23—1954.6.7),英国数学家、逻辑学家,被称为计算机科学之父、人工智能之父。
1931年图灵进入剑桥大学国王学院,毕业后到美国普林斯顿大学攻读博士学位,二战爆发后回到剑桥,后曾协助军方破解德国的著名密码系统Enigma,帮助盟军取得了二战的胜利。
阿兰·麦席森·图灵,1912年生于英国伦敦,1954年死于英国的曼彻斯特,他是计算机逻辑的奠基者,许多人工智能的重要方法也源自于这位伟大的科学家。
他对计算机的重要贡献在于他提出的有限状态自动机也就是图灵机的概念,对于人工智能,它提出了重要的衡量标准“图灵测试”,如果有机器能够通过图灵测试,那他就是一个完全意义上的智能机,和人没有区别了。
他杰出的贡献使他成为计算机界的第一人,现在人们为了纪念这位伟大的科学家将计算机界的最高奖定名为“图灵奖”。
上中学时,他在科学方面的才能就已经显示出来,这种才能仅仅限于非文科的学科上,他的导师希望这位聪明的孩子也能够在历史和文学上有所成就,但是都没有太大的建树。
Ubiquitous Computing
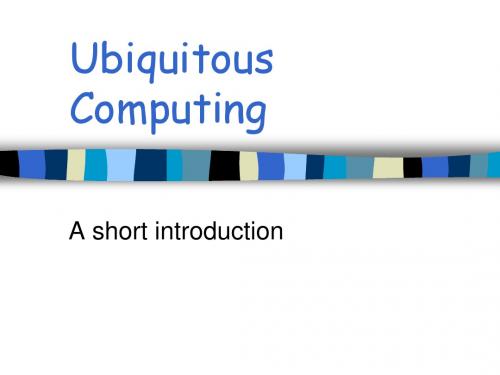
A short introduction
How to achieve ubiquity?
Make computing available beyond desktop
Make it mobile and connected Instrument the person Instrument the physical surroundings
It is always smaller than what came before. Most think of the new technology as “just a toy” The new dominant use was almost completely absent before. Technology spread increases
Information Appliances
Universal Devices vs. Specialized Devices
– E.g., Swiss Army Knife vs. Butcher, Butter, Steak, Bread knife
Different design constraints based on intended use, enhances ease of use
– Has expanded the use and impact of computers in society – Allows integration of processing, communication, and sensors
Many cheap and self-supporting intelligent devices
蒂姆伯纳斯 李

人物评价
万维网创始人
蒂姆·伯纳斯·李 2004年4月15日,在芬兰的埃斯波市,芬兰技术奖基金会将全球最大的技术类奖“千年技术 奖”授予了现年49岁的英国物理学家蒂姆·伯纳斯-李(Tim Berners-Lee)。这位万维网(World Wide Web)的发明 人在成为世界上首位“千年技术奖”得主的同时,也获得了生平最大的一笔100万欧元的奖金。当人们每天打开 电脑,感叹着互联网强大的同时,很少有人想到这一切竟是由一人之力创造的。 1955年6月8日,伯纳斯-李出生 在英国伦敦的西南部,他的父母都是英国计算机界的名人,曾参与了英国第一台商用计算机的研制工作,他从小 便耳濡目染。在牛津大学的女王学院学习期间,他就用从旧货商店花7美元买回的电视机,与M6800处理器、烙 铁、电路板组装出了自己的第一台电脑。
其它
因为伯纳斯-李曾经在英国东多塞特郡居住,他于2001年接受郡古迹信托会的名誉保护人的职位。 他与他的 妻子和两个孩子住在美国波士顿。
个人荣誉
个人荣誉
1994年,他成为万维网名人堂的成员之一。 1995年,他赢得凯尔贝基金会的“年度青年发明家”奖项。同 年,他获得了ACM软件系统奖。 1997年,在英女皇寿辰上,为表扬他在“全球计算机网络”方面作出的贡献, 他获颁大英帝国官佐勋章。 1998年,他获得艾塞克斯大学荣誉博士学位。 1999年,他入选时代杂志20世纪最重 要的100个人物。 2000年3月,他获得公开大学荣誉博士学位。 2001年,他入选美国文理科学院。 2002年,他 入选BBC最伟大的100名英国人。 2003年,为表扬他对万维网发展的开创性贡献,他获颁计算机历史博物馆研究 奖。 2004年4月15日,他获芬兰技术学院颁发千禧技术奖。奖金高达一百万欧元,奖项在同年6月15日由芬兰总 统向他颁发。同年7月16日,因获颁大英帝国爵级司令,他获封为爵士。 2004年21日,他获得兰开斯特大学荣誉 科学博士学位。 2005年1月27日,因他的成就,与他展示出英国人的特质,“羞怯、决心、敏锐的幽默感及良好 的适应能力”,他入选2004年最伟大的英国人。 2007年,他获得成就学院金盘奖。同年,他与艾伯特·霍夫曼一 起并列每日电讯报“最伟大的100名在生天才”榜首。同年6月13日,他获颁功绩勋章。 2008年,为表扬他“构 思出万维网,并进一步发展万维网”,他获得了电气电子工程师学会詹姆斯·克拉克·麦克斯韦奖。 2008年12月2 日,他获得曼切斯特大学荣誉博士学位。 2009年4月21日,他获得马德里技术大学荣誉博士学位。同年4月28日, 他获选为美国国家科学院外籍院士。同年6月8日,因他的终生成就,他获得威比奖。颁奖仪式于纽约市举行。同 年10月,他获得阿姆斯特丹自由大学荣誉博士学位。 2011年3月30日,他获得米哈伊尔·戈尔巴乔夫“改变世界 的人”奖。同年5月26日,他获得哈佛大学荣誉科学博士学位。同年,他入选电气电子工程师学会人工智能名人 堂。 2012年,他入选互联网名人堂。同年7月27日,他在2012年夏季奥林匹克运动会开幕典礼受到了表扬。 2017年,他因“发......
深度学习专家:尤舒亚·本吉奥(Yoshua Bengio)人物简介
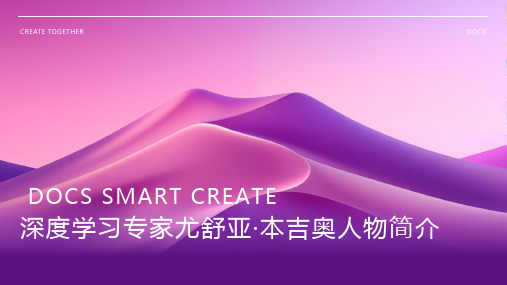
2000年代开始研究自然语言处理领域
• 提出循环神经网络(RNN)模型
• 应用于机器翻译和情感分析任务
2010年代开始研究强化学习领域
• 提出深度强化学习模型
• 应用于机器人控制和游戏智能任务
⌛️
03
尤舒亚·本吉奥在工业界的合作与影响
尤舒亚·本吉奥与科技公司的合作经历
与IBM合作研究深度学习和人工智能
DOCS
倡导跨学科合作和学术交流
• 加强深度学习领域与其他学科领域的合作
• 支持国际学术交流和合作研究
关注深度学习技术在教育和医疗等领域的应用
• 利用深度学习技术改进教育方法和教学质量
• 利用深度学习技术提高医疗诊断和治疗水平
CREATE TOGETHER
谢谢观看
THANK YOU FOR WATCHING
• 与IBM合作开发IBM Watson自然语言处理系统
• 与谷歌合作开发Google Assistant智能语音助手
将深度学习技术应用于强化学习领域
• 与谷歌合作开发AlphaGo围棋智能程序
• 与特斯拉合作研究自动驾驶技术
尤舒亚·本吉奥对工业界深度学习发展的影响与推动作用
提高深度学习技术的社会影响力
• 引入梯度消失和梯度爆炸概念
1990年代提出卷积神经网络(CNN)模型
• 应用于图像识别和语音识别领域
• 取得显著的研究成果
尤舒亚·本吉奥在深度学习算法与模型方面的创新
01
提出深度信念网络(DBN)模型
• 结合受限玻尔兹曼机(RBM)和反向传播算法
• 应用于图像识别和语音识别领域
02
提出生成对抗网络(GAN)模型
注重跨学科合作和学术交流
丘奇-图灵论题、超计算和人工智能

丘奇-图灵论题、超计算和人工智能如果我们认可丘奇-图灵论题和相似性原则,那么人就是图灵机。
所有目前的人工智能工作都是建立在这个认同之上的。
丘奇-图灵论题和洪加威相似性原则是战斗在第一线的研究者的工作假设。
丘奇-图灵论题说的是能行性(effectiveness),而相似性原则则是说的效率(efficiency)。
多依奇在他的科普著作里提到,他认为人类有史以来有4个伟大理论:达尔文进化论、波普尔证伪理论、量子理论和计算理论。
他的工作把量子理论和计算理论整合到一起。
但他不认为人脑是量子计算机,这点有别于彭罗斯。
自然科学和唯物论、经验论的关系要比唯心论、理性论的关系更近,背后都有一条归约主义路线图(reductionist),从生物到化学,再到物理。
一个有机体最终被归约到物理化学过程,一个活的、有意识的生物最终会被归约到神经网络过程。
这是自顶向下的思路。
近来也有自底向上的思路,例如细胞自动机,利用很简单的几条规则,就可展示很复杂的行为。
沃尔夫勒姆在他的《新科学》(A New Kind of Science )一书中提到的这种现象其实早就被数学家康韦(John Conway)观察到,他设计了《生命游戏》(Game of Life ),企图利用细胞自动机来说明确定性和自由意志的问题。
高德纳在评论康韦的工作时说:所有规则都是确定性的,但游戏的演进过程却给人一种自主性的感觉。
高老喜欢阅读英国女作家塞耶斯(Dorothy Sayers),她更偏爱写剧本而不是小说,她说剧本给了演员发挥再创作的机会,这个再创作就是自由意志。
高老说量子力学为自由意志提供了空间,也使得上帝可以操纵世界而不违反物理定律。
所谓“太阳底下没啥是新鲜的”,但几条简单规则展示的行为却无法解释。
如果考虑数学定理证明,我们可以说勾股定理不新鲜——毕竟从简单几何公理不用费太大力气就可证明。
但我们敢说黎曼猜想也不新鲜或者庞加莱猜想的证明也不新鲜因为所有结果不都是可以从起始点(那几条数论公理)推出吗?这似乎模糊了柏拉图主义(实在论)和构造主义的边界。
普林斯顿 结构生物 类脑计算 经费

《普林斯顿:结构生物与类脑计算的探索与发展》一、引言在当今科技飞速发展的时代,结构生物学和类脑计算作为两大前沿交叉学科,对人类社会和科学技术发展产生了深远的影响。
作为这一领域的先锋,普林斯顿大学一直致力于对结构生物与类脑计算进行探索与发展。
本文将从普林斯顿大学的视角出发,全面评估和解析普林斯顿在这一领域的最新研究成果,并共享对结构生物与类脑计算的个人理解和观点。
二、普林斯顿:结构生物的前沿研究1. 普林斯顿在结构生物领域的权威地位作为一所拥有丰富研究资源和顶尖科研团队的顶尖大学,普林斯顿在结构生物领域拥有显著的研究优势。
在高分辨率结构生物学、蛋白质折叠与组装、大分子相互作用等方面,普林斯顿都有着卓越的研究成果和学术地位。
2. 普林斯顿的结构生物研究成果通过对普林斯顿在结构生物学领域的研究成果进行深入探讨,我们可以发现,普林斯顿在解析高分辨率蛋白质结构、研究生命大分子的结构与功能、探索生命活动的分子机制等方面取得了突破性进展。
这些成果不仅为生命科学领域的发展提供了重要的理论和实践支持,同时也为人类社会的生物医药、食品安全等方面带来了巨大的影响和推动力。
三、普林斯顿:类脑计算的前沿研究1. 普林斯顿在类脑计算领域的研究方向作为类脑计算领域的佼佼者,普林斯顿在神经科学、人工智能、认知科学等领域的研究成果备受关注。
通过对普林斯顿在类脑计算领域的研究方向进行全面评估,我们可以发现,普林斯顿在神经元信号传导模型、神经网络结构与功能的模拟、认知计算理论等方面取得了令人瞩目的成就。
2. 普林斯顿的类脑计算研究成果普林斯顿在类脑计算领域的研究成果不仅涉及基础理论研究,还包括脑-机器接口技术、人工智能系统的优化与应用、大规模脑网络行为建模等领域。
这些成果为人工智能、神经科学、认知计算等领域的交叉研究提供了重要的理论和技术支持,推动了类脑计算技术的迅速发展和应用。
四、结构生物与类脑计算的交叉研究1. 普林斯顿在结构生物与类脑计算交叉研究的探索普林斯顿在结构生物与类脑计算交叉研究的探索成果丰硕,涉及蛋白质与神经元的相互作用、大脑认知功能的生物结构基础、类脑计算技术在生物医学领域的应用等诸多领域。
曼纽尔 布卢姆
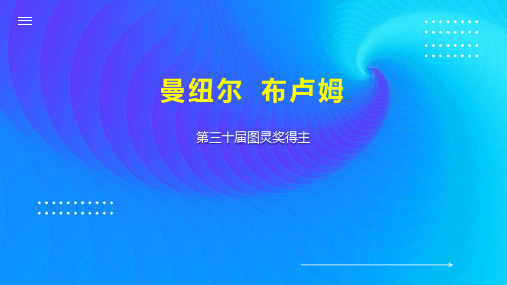
主要贡献
1.计算机复杂性理论
曼纽尔·布卢姆尤里斯·哈特马尼斯理查德·斯特恩斯19Байду номын сангаас5年度的图灵奖授予加州大学伯克利分校的计算机 科学家曼纽尔·布卢姆(Manuel Blum)。布卢姆是计算复杂性理论的主要奠基人之一。在此之前,已有5位科学 家因为在计算复杂性理论方面的贡献而获此殊荣,他们是:拉宾(1976年)、库克(1982年)、卡普(1985年)、 哈特马尼斯和(1993年)。在图灵奖34年的历史上,这是获奖科学家最多的一个分支领域,由此可见计算复杂性 作为基础性分支在计算机科学中的突出地位及其重要性。
其它活动
曼纽尔·布卢姆曼纽尔·布卢姆精彩演讲2002年10月18日,由微软亚洲研究院和国家自然科学基金委员会联 合举办的第四届“二十一世纪的计算”大型国际学术研讨会在北大百年纪念堂举行,本次会议主题为“高信度计 算”。ManuelBlum博士做了关于“慵懒的密码学专家们是如何做人工智能的”精彩演讲。
谢谢观看
到2005年秋天为止,为了从国家科学基金会(NSF)赢得价值560万美元的信息技术研究(ITR)的承认, Blum全家把他们在计算机科学理论方面的专门技术与几个理论工作组的同事们共享,其中包括 GuyBlelloch,DanielSleator和副教授RamamoorthiRavi。该项基金是为了帮助支持算法的自适应、分解与集成 中心,能够使其受这560万美元资助的“阿拉丁(Aladdin)计划”更广为人知。其目标是把运算法则以一个更及 时的方式送到那些潜在的用户手中。 Blum是美国国家科学院所选出的,认为在该年独创研究方面做出了卓越、 持续成就的72名新成员之一,他在国家科学院的这一当选,使卡内基梅隆大学的成员数量增加到了7名,其他几 位分别是JohnR.Anderson,StephenFienberg和 JamesMcClelland,DanaScott,RobertGriffiths以及 LincolnWolfenstein。
我所知道的计算流体力学大牛们
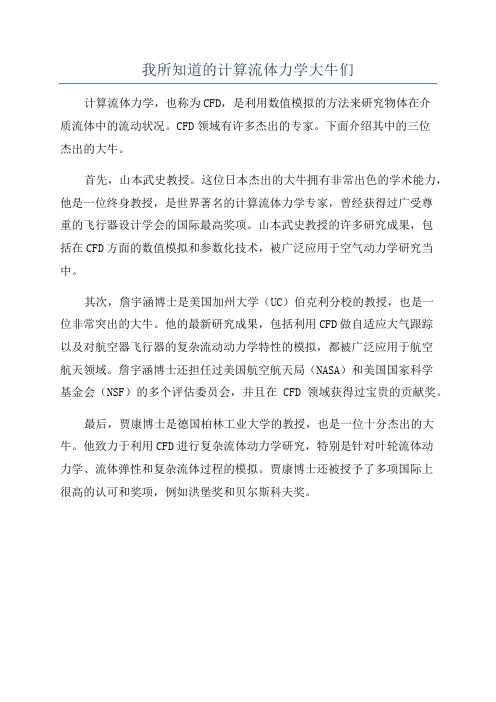
我所知道的计算流体力学大牛们
计算流体力学,也称为CFD,是利用数值模拟的方法来研究物体在介
质流体中的流动状况。
CFD领域有许多杰出的专家。
下面介绍其中的三位
杰出的大牛。
首先,山本武史教授。
这位日本杰出的大牛拥有非常出色的学术能力,他是一位终身教授,是世界著名的计算流体力学专家,曾经获得过广受尊
重的飞行器设计学会的国际最高奖项。
山本武史教授的许多研究成果,包
括在CFD方面的数值模拟和参数化技术,被广泛应用于空气动力学研究当中。
其次,詹宇涵博士是美国加州大学(UC)伯克利分校的教授,也是一
位非常突出的大牛。
他的最新研究成果,包括利用CFD做自适应大气跟踪
以及对航空器飞行器的复杂流动动力学特性的模拟,都被广泛应用于航空
航天领域。
詹宇涵博士还担任过美国航空航天局(NASA)和美国国家科学
基金会(NSF)的多个评估委员会,并且在CFD领域获得过宝贵的贡献奖。
最后,贾康博士是德国柏林工业大学的教授,也是一位十分杰出的大牛。
他致力于利用CFD进行复杂流体动力学研究,特别是针对叶轮流体动
力学、流体弹性和复杂流体过程的模拟。
贾康博士还被授予了多项国际上
很高的认可和奖项,例如洪堡奖和贝尔斯科夫奖。
10大杰出计算机学者2024
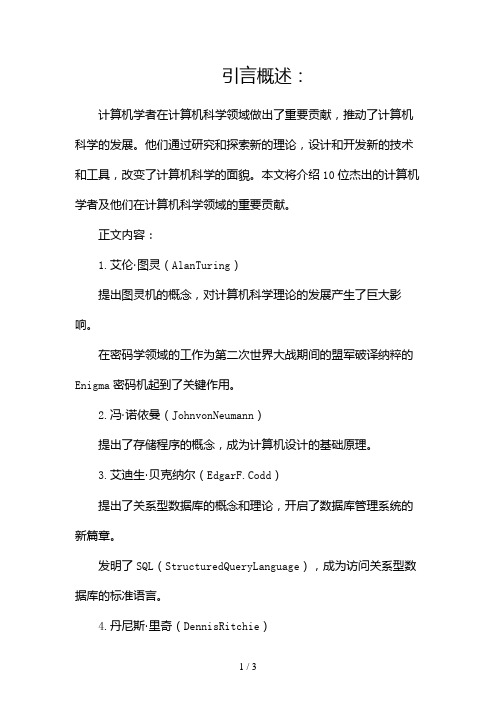
引言概述:计算机学者在计算机科学领域做出了重要贡献,推动了计算机科学的发展。
他们通过研究和探索新的理论,设计和开发新的技术和工具,改变了计算机科学的面貌。
本文将介绍10位杰出的计算机学者及他们在计算机科学领域的重要贡献。
正文内容:1.艾伦·图灵(AlanTuring)提出图灵机的概念,对计算机科学理论的发展产生了巨大影响。
在密码学领域的工作为第二次世界大战期间的盟军破译纳粹的Enigma密码机起到了关键作用。
2.冯·诺依曼(JohnvonNeumann)提出了存储程序的概念,成为计算机设计的基础原理。
3.艾迪生·贝克纳尔(EdgarF.Codd)提出了关系型数据库的概念和理论,开启了数据库管理系统的新篇章。
发明了SQL(StructuredQueryLanguage),成为访问关系型数据库的标准语言。
4.丹尼斯·里奇(DennisRitchie)发明了C语言,成为一种广泛应用的编程语言。
联合发明了UNIX操作系统,对操作系统的发展做出了重大贡献。
5.约翰·麦卡锡(JohnMcCarthy)提出了的概念,并发明了Lisp编程语言。
在领域的研究,尤其是专家系统和自动推理方面,为该领域的发展奠定了基础。
6.艾伦·凯(AlanKay)提出了面向对象的编程思想,开创了面向对象编程的先河。
设计了Smalltalk编程语言,对图形用户界面和面向对象编程的发展产生了深远影响。
7.登·霍普金斯(DonHopkins)提出了窗口系统的概念,对图形用户界面的发展起到了重要作用。
设计了Smalltalk/V编程语言,成为早期图形用户界面的标志性编程语言。
8.斯蒂芬·沃尔夫勒姆(StephenWolfram)提出了元胞自动机的概念,对复杂系统的研究有重要意义。
创立了独立的计算平台Mathematica,为科学计算和数据分析提供了强大的工具。
9.蒂姆·伯纳斯李(TimBernersLee)发明了万维网,改变了人们获取信息和交流的方式。
Ubiquitous CSCL的概念模型与关键技术要素
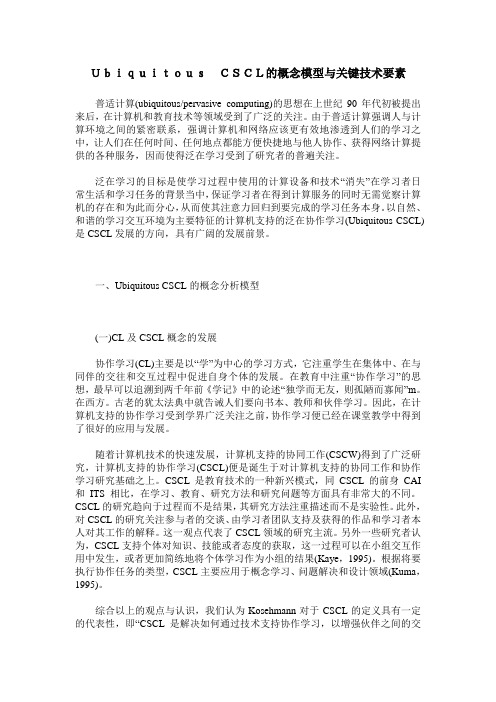
UbiquitousCSCL的概念模型与关键技术要素普适计算(ubiquitous/pervasive computing)的思想在上世纪90年代初被提出来后,在计算机和教育技术等领域受到了广泛的关注。
由于普适计算强调人与计算环境之间的紧密联系,强调计算机和网络应该更有效地渗透到人们的学习之中,让人们在任何时间、任何地点都能方便快捷地与他人协作、获得网络计算提供的各种服务,因而使得泛在学习受到了研究者的普遍关注。
泛在学习的目标是使学习过程中使用的计算设备和技术“消失”在学习者日常生活和学习任务的背景当中,保证学习者在得到计算服务的同时无需觉察计算机的存在和为此而分心,从而使其注意力回归到要完成的学习任务本身。
以自然、和谐的学习交互环境为主要特征的计算机支持的泛在协作学习(Ubiquitous CSCL)是CSCL发展的方向,具有广阔的发展前景。
一、Ubiquitous CSCL的概念分析模型(一)CL及CSCL概念的发展协作学习(CL)主要是以“学”为中心的学习方式,它注重学生在集体中、在与同伴的交往和交互过程中促进自身个体的发展。
在教育中注重“协作学习”的思想,最早可以追溯到两千年前《学记》中的论述“独学而无友,则孤陋而寡闻”m。
在西方。
古老的犹太法典中就告诫人们要向书本、教师和伙伴学习。
因此,在计算机支持的协作学习受到学界广泛关注之前,协作学习便已经在课堂教学中得到了很好的应用与发展。
随着计算机技术的快速发展,计算机支持的协同工作(CSCW)得到了广泛研究,计算机支持的协作学习(CSCL)便是诞生于对计算机支持的协同工作和协作学习研究基础之上。
CSCL是教育技术的一种新兴模式,同CSCL的前身CAI 和ITS相比,在学习、教育、研究方法和研究问题等方面具有非常大的不同。
CSCL的研究趋向于过程而不是结果,其研究方法注重描述而不是实验性。
此外,对CSCL的研究关注参与者的交谈、由学习者团队支持及获得的作品和学习者本人对其工作的解释。
巴伦博伊姆简介
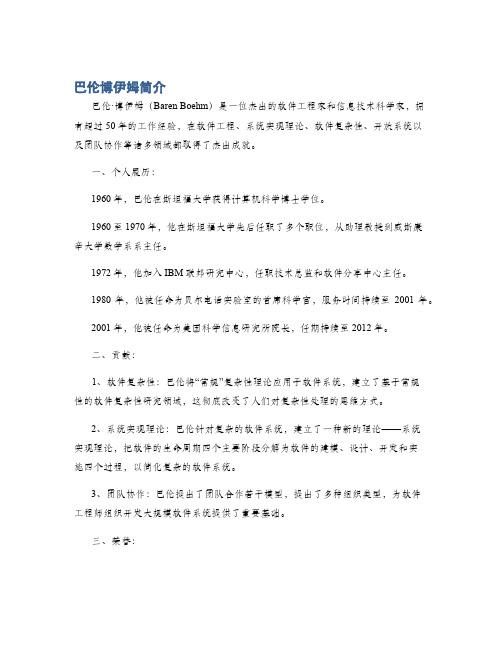
巴伦博伊姆简介巴伦·博伊姆(Baren Boehm)是一位杰出的软件工程家和信息技术科学家,拥有超过50年的工作经验,在软件工程、系统实现理论、软件复杂性、开放系统以及团队协作等诸多领域都取得了杰出成就。
一、个人履历:1960年,巴伦在斯坦福大学获得计算机科学博士学位。
1960至1970年,他在斯坦福大学先后任职了多个职位,从助理教授到威斯康辛大学数学系系主任。
1972年,他加入IBM联邦研究中心,任职技术总监和软件分享中心主任。
1980年,他被任命为贝尔电话实验室的首席科学官,服务时间持续至2001年。
2001年,他被任命为美国科学信息研究所院长,任期持续至2012年。
二、贡献:1、软件复杂性:巴伦将“常规”复杂性理论应用于软件系统,建立了基于常规性的软件复杂性研究领域,这彻底改变了人们对复杂性处理的思维方式。
2、系统实现理论:巴伦针对复杂的软件系统,建立了一种新的理论——系统实现理论,把软件的生命周期四个主要阶段分解为软件的建模、设计、开发和实施四个过程,以简化复杂的软件系统。
3、团队协作:巴伦提出了团队合作若干模型,提出了多种组织类型,为软件工程师组织开发大规模软件系统提供了重要基础。
三、荣誉:巴伦获得过多项杰出奖项,包括:1993年诺贝尔经济学奖,1997年萨缪尔·米勒卓越贡献奖,1999年在计算机科学领域的科学与文化奖,2007年国家科学院科学与工程院的终身成就奖,2013年IEEE安迪·科拉工程成就奖,2014年德拉特美国国家学会院士奖,2015年贝尔实验室实施成就奖,2016年美国国家学会院士奖,2017年Turing Award等。
用计算感知社会——记西北工业大学普适与智能计算研究所所长、洪堡学者於志文
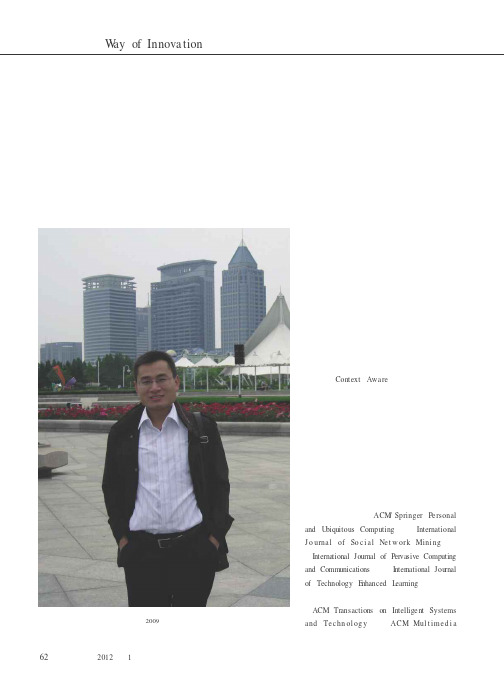
6科学中国人年第期【创新之路】W ay of Innovation当历史的车轮驶进二十一世纪,科技以前所未有的速度发展着,人类的生活也发生了重大的变革。
随着嵌入式设备、无线传感网络、移动计算等快速发展,集成感知、计算和通信能力的普适智能系统被广泛部署,并逐步融入到人类的日常生活环境。
人类生活在由通信网、互联网、传感网等相互融合所形成的混合网络环境中。
在这样的环境中,仅仅依靠传统的“感觉器官”,已经无法满足人类对社会的感知需要。
普适计算技术,特别是传感技术和情境感知(Context Aware )技术正以前所未有的方式增强着我们收集、分析和利用数据的广度和深度。
基于此,西北工业大学普适与智能计算研究所所长於志文教授将“社会感知计算”带入了我们的生活。
於志文,教授,博士生导师,洪堡学者,西北工业大学计算机学院副院长,普适与智能计算研究所所长,主要从事普适计算、移动互联网、社会感知计算等领域的研究工作。
担任《ACM /Springer Personal and U biquitous Computing 》、《International J o urnal of So ci al Net w ork Mini ng 》、《International Journal of Pervasive Computing and Communications 》、《International Jou rnal of Technology E nhanced L earning 》等国际期刊和《中国计算机学会通讯》编委,担任《ACM Transactions on Intelligent SystemsT y 》、《M M 用计算感知社会——记西北工业大学普适与智能计算研究所所长、洪堡学者於志文年在青岛本刊记者吴应清220121and echn ol og AC ul t imed i a2009Systems Journal》、《M ultimedia Tools and Applications》(Springer出版社)、《Pervasive and Mobile Computing》(El s ev ie r出版社)等国际期刊客座编辑。
冯 诺伊曼

冯诺伊曼冯诺伊曼:计算机科学之父冯·诺伊曼(John von Neumann),匈牙利裔美国物理学家、数学家、计算机科学家,被誉为计算机科学之父。
他对计算机的架构和设计做出了巨大的贡献,被认为是现代计算机的奠基人之一。
在他的领导下,冷战时期的美国在计算机科学和高级技术领域取得了巨大的进展。
本文将介绍冯·诺伊曼的生平事迹、对计算机科学的贡献以及他的遗产。
冯·诺伊曼于1903年12月28日出生在匈牙利的布达佩斯。
早年他展示出了惊人的数学天赋,在17岁时进入布达佩斯科技大学学习化学工程。
然而,他很快发现对数学的兴趣超过了对化学工程的兴趣,于是决定转而攻读数学课程。
他在多个领域都有出色的成就,包括数学、物理学和化学等。
冯·诺伊曼的科学生涯开始于20世纪30年代。
他在普林斯顿高等研究院(Institute for Advanced Study)担任教授,并与一些世界闻名的数学家如艾克曼(Hermann Weyl)和哥德尔(Kurt Gödel)等人合作。
在与这些杰出的数学家合作期间,冯·诺伊曼发展出了许多重要的理论,这些理论对后来的计算机科学产生了深远的影响。
冯·诺伊曼最重要的贡献之一是对计算机体系结构的提出。
他在上世纪40年代中期提出了存储程序的概念,意味着计算机可以像执行指令一样执行数据。
他设计了一种具有存储器、运算器和控制器的计算机结构,这种结构被称为冯·诺伊曼体系结构。
这一体系结构成为了现代计算机设计的基础,影响了后来无数计算机的制造。
冯·诺伊曼还是早期的计算机软件开发的先驱之一。
他与他的团队合作开发了一种可以执行复杂算法的机器,这种机器被称为ENIAC (电子数字积分计算机)。
他们还开发了EDVAC(电子离散变量自动计算机),该计算机采用了存储程序的概念,并具有更高的可编程性和灵活性。
冯·诺伊曼对计算机科学的贡献不仅限于计算机体系结构和软件开发。
人工智能原理名人文献

人工智能原理名人文献
以下是一些与人工智能原理相关的重要文献和名人:
1. "Computing Machinery and Intelligence" - 艾伦·图灵(Alan Turing)- 发表于1950年,提出了著名的图灵测试和思考机器智能的问题。
2. "A Proposal for the Dartmouth Summer Research Project on Artificial Intelligence" - 约翰·麦卡锡(John McCarthy)等合著- 1956年提出了人工智能领域的名称,标志着人工智能正式成为一个独立学科。
3. "Perceptrons: An Introduction to Computational Geometry" - 弗兰克·罗森布拉特(Frank Rosenblatt)- 发表于1958年,引入了感知机模型,这是神经网络的早期形式。
4. "Artificial Intelligence: A Modern Approach" - 斯图尔特·拉塞尔(Stuart Russell)和彼得·诺维格(Peter Norvig)- 这本教材出版于1995年,并成为人工智能领域的经典参考书之一。
5. "Deep Learning" - 亚历山大·库兹涅佐夫(Alexey Kuznetsov)等合著- 该书于2015年发表,详细介绍了深度学习的基本原理和算法。
计算机与人工智能之父艾伦图灵生平简介
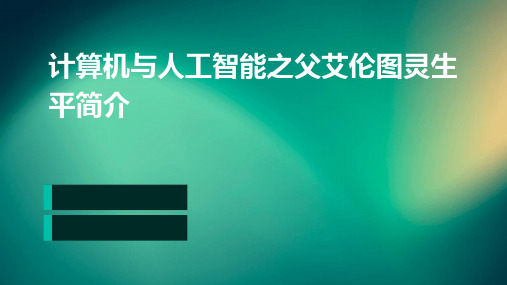
图灵的贡献不仅在于理论创新,也在 于实际应用。他的思想和发明为人类 带来了巨大的便利和福祉,提高了人 类的生活质量。
THANKS FOR WATCHING
感谢您的观看
艾伦·图灵的职业生涯
1938年,艾伦·图灵发表了题为《论 可计算数及其在判定问题中的应用》 的论文,提出了著名的图灵机的概念 。
战后,他加入了曼彻斯特大学计算机 实验室,并参与了世界上第一台电子 计算机的研制工作。
二战期间,他加入了英国政府密码破 译部门,并设计了一种名为“图灵炸 弹机”的密码破译机器。
03 图灵在人工智能领域的贡 献
图灵关于机器智能的思想Fra bibliotek01图灵认为机器可以像人一样思考 ,并提出了一种名为“图灵测试 ”的方法来评估机器智能的水平 。
02
图灵认为机器智能的发展需要依 靠算法和程序,并致力于设计和 开发能够实现人工智能的算法和 程序。
图灵对人工智能发展的影响
图灵的机器智能思想对人工智能领域 产生了深远的影响,推动了人工智能 技术的快速发展。
图灵对后世科技发展的影响
引领科技革命
图灵的贡献不仅限于计算机科学和人工智能领域,他的思想对整个科技领域的 发展产生了深远影响,推动了科技革命的进程。
启发科学家和工程师
图灵的思想和成就激励了无数科学家和工程师投身于科技研究和创新,推动了 科技的快速发展。
图灵对社会的贡献和影响
促进社会发展
图灵的贡献不仅在于科技领域,也在 于他对社会发展的推动。他的思想对 现代社会的各个方面产生了深远影响, 如教育、经济、文化等。
图灵提出的“图灵测试”是一种评估人工智能是否具有人类智能的方法。该测试通过比较人类评委对 机器生成的内容和人类生成的内容的区分能力,来评估机器是否能够表现出与人类相似的智能水平。
10大杰出计算机学者
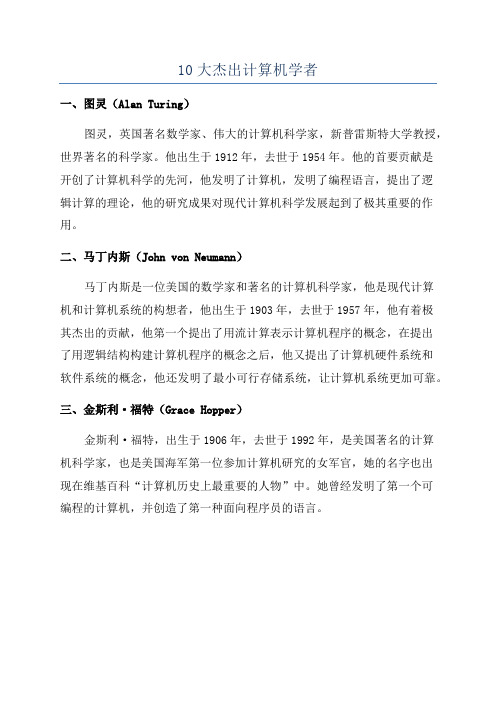
10大杰出计算机学者
一、图灵(Alan Turing)
图灵,英国著名数学家、伟大的计算机科学家,新普雷斯特大学教授,世界著名的科学家。
他出生于1912年,去世于1954年。
他的首要贡献是
开创了计算机科学的先河,他发明了计算机,发明了编程语言,提出了逻
辑计算的理论,他的研究成果对现代计算机科学发展起到了极其重要的作用。
二、马丁内斯(John von Neumann)
马丁内斯是一位美国的数学家和著名的计算机科学家,他是现代计算
机和计算机系统的构想者,他出生于1903年,去世于1957年,他有着极
其杰出的贡献,他第一个提出了用流计算表示计算机程序的概念,在提出
了用逻辑结构构建计算机程序的概念之后,他又提出了计算机硬件系统和
软件系统的概念,他还发明了最小可行存储系统,让计算机系统更加可靠。
三、金斯利·福特(Grace Hopper)
金斯利·福特,出生于1906年,去世于1992年,是美国著名的计算
机科学家,也是美国海军第一位参加计算机研究的女军官,她的名字也出
现在维基百科“计算机历史上最重要的人物”中。
她曾经发明了第一个可
编程的计算机,并创造了第一种面向程序员的语言。
斯蒂芬 库克
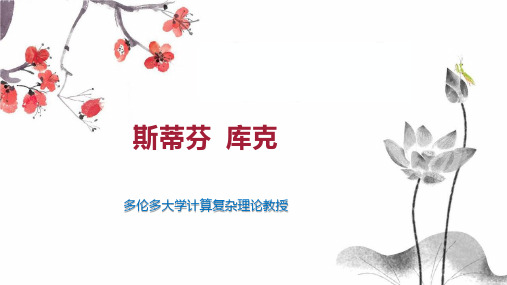
个人成就
ቤተ መጻሕፍቲ ባይዱ
复杂性规约
颁奖相关
复杂性规约
斯蒂芬·库克库克在建立NP完全性理论时,为研究复杂性类之间的关系提出的方法,叫“复杂性归 约”(complexity reduction),用以比较问题的计算难度。库克所用的归约方法是多项式时间图灵归约,有时 直接把它叫做库克归约。其要点如下:假设所考虑的问题都已编码成字母表∑上的语言(实例的集合)。设Ll、L2 是∑上的两个语言,若存在以上:为oracle集的多项式时间图灵机M,其接受的语言为Ll,则称L1,多项式时间 图灵归约到L2,记为i1≤PTL2。这时,对x是否属于L1的判别可转化为至多,|x|的多项式个元素是否属于i2的判 别,因此L2∈p便导致L1∈p。从这种相对的意义上说,i1的计算不比L2难。≤;可以是定义在任何语言类D上的 一种二元前序关系,如果存在L∈D,对于任何L'∈D,都有L'≤PtL,则L就是D中(在多项式时间图灵归约下)“最 困难的”,称其为D-T完全的。
人物介绍
人物介绍
斯蒂芬·库克NP完全性理论的奠基人斯蒂芬·A·库克(Stephen A. Cook,1939年-),计算机科学家, 计算复杂性理论的重要研究者。
1971年,在他的论文《The Complexity of Theorem Proving Procedures》,他整理了NP完备性的目标, 亦产生了库克定理——布尔可满足性问题是NP完备的证明。
个人经历
少年时光 大学生涯
研究项目 著名论文
少年时光
斯蒂芬·库克实际上是美国科学家,1939年12月14日生于纽约州的布法罗(Buffalo),他的父亲是一名
斯蒂芬·库克化学家,在著名的联合碳化物公司工作,同时在布法罗大学任教,有一份不错的收入。但库克 的父亲喜欢农村的恬静生活和清新空气,因此在库克10岁时全家迁居到纽约州克拉伦斯的一个奶牛场。在这里, 少年库克可以与牛羊为伴,还学会了挤奶。在乡村中学,库克的数学成绩比较好,但那时他并没有梦想当数学家。 库克的另一个爱好是下棋,这帮助他发展了逻辑思维能力。在克拉克伦斯,当时出现了一位传奇式的英雄,那就 是威尔逊·格莱特巴郝(Wilson Greatbatch),他发明了可植入式心脏起搏器,挽救了世界上无数人的性命,使 他远近闻名。库克对这位发明家也很敬仰和崇拜,暑假时曾到他手下去打工,帮他焊晶体管电路板。当时晶体管 问世不久,是新鲜事物,库克对神奇的晶体管也很有兴趣,想当个电气工程师。
介绍图灵的英语作文
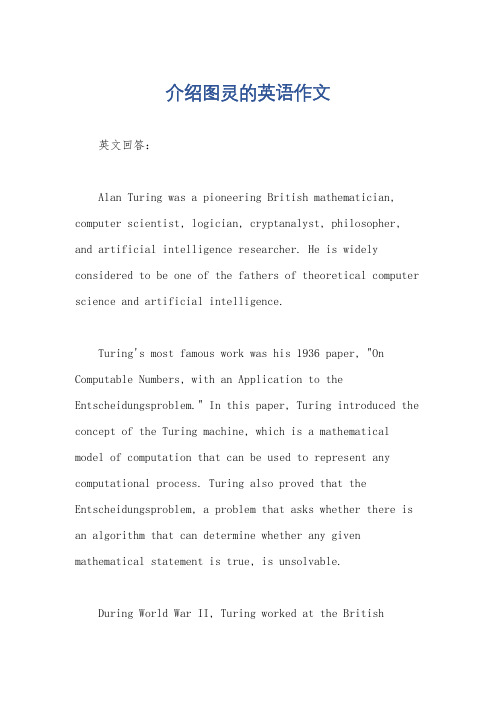
介绍图灵的英语作文英文回答:Alan Turing was a pioneering British mathematician, computer scientist, logician, cryptanalyst, philosopher, and artificial intelligence researcher. He is widely considered to be one of the fathers of theoretical computer science and artificial intelligence.Turing's most famous work was his 1936 paper, "On Computable Numbers, with an Application to the Entscheidungsproblem." In this paper, Turing introduced the concept of the Turing machine, which is a mathematical model of computation that can be used to represent any computational process. Turing also proved that the Entscheidungsproblem, a problem that asks whether there is an algorithm that can determine whether any given mathematical statement is true, is unsolvable.During World War II, Turing worked at the Britishcodebreaking center at Bletchley Park. He played a key role in the development of the Bombe, a machine that was used to break the German Enigma code. Turing's work at Bletchley Park is credited with shortening the war by at least two years.After the war, Turing worked on the development of the first electronic computers. He also developed the Turing test, a test designed to determine whether a machine can exhibit intelligent behavior. Turing's work on artificial intelligence laid the foundation for the development of modern artificial intelligence systems.Turing died in 1954 at the age of 41. He was posthumously pardoned in 2013 for his conviction of gross indecency, a charge that was related to his homosexuality. In 2019, Turing was named one of the 50 greatest Britons in history by the BBC.中文回答:图灵是一位开创性的英国数学家、计算机科学家、逻辑学家、密码分析学家、哲学家和人工智能研究员。
人工智能安全专家:布鲁斯·施奈尔(Bruce Schneier)人物简介
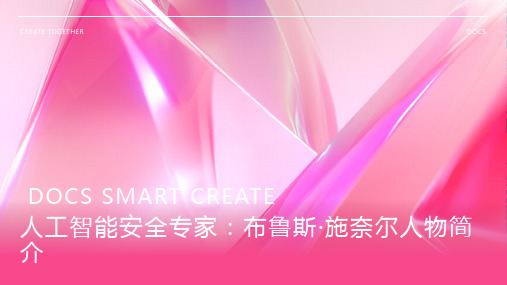
• 如何制定合适的隐私保护政策和法规
布鲁斯·施奈尔在网络安全和基础设施保护方面的贡献
网络安全的重要性
基础设施保护的重要性
• 布鲁斯·施奈尔认为,网络安全是保护信息系统安全的关
• 布鲁斯·施奈尔强调,基础设施保护是国家安全的重要组
键
成部分
• 网络安全可以防止网络攻击和数据泄露
主要成就
• 参与制定了美国政府的密码政策
• 为多家企业提供网络安全咨询和解决方案
• 创立了著名的安全会议“Schneier on Security”
布鲁斯·施奈尔在人工智能安全领域的影响和贡献
人工智能安全领域的影响
主要贡献
• 布鲁斯·施奈尔是人工智能安全领域的先驱之一
• 提出了人工智能安全的基本概念和框架
02
中期思想
• 随着人工智能技术的发展,布鲁斯·施奈尔开始关注人工
智能安全
• 他对人工智能系统的安全和隐私保护进行了深入研究
03
后期思想
• 布鲁斯·施奈尔在后期更加关注网络安全和基础设施保护
• 他强调了网络安全和基础设施保护对于国家安全和社会
稳定的重要性
布鲁斯·施奈尔对人工智能安全领域的影响
观点影响
布鲁斯·施奈尔在行
业发展中的角色和地
位
• 角色
• 布鲁斯·施奈尔是人工智能安全领域的先驱之一
• 他为人工智能安全领域的发展提供了宝贵的意见和建议
• 地
CREATE TOGETHER
谢谢观看
THANK YOU FOR WATCHING
DOCS
CREATE TOGETHER
DOCS
DOCS SMART CREATE
查仁裕个人简历

查仁裕个人简历查仁裕,出生于1990年5月15日,现年31岁,中国最著名的人工智能科学家之一。
他以其在机器学习和深度学习领域的杰出贡献而闻名,被誉为现代科技领域的瑰宝。
教育背景查仁裕于2008年进入北京理工大学计算机科学与技术专业学习,他在校期间表现出超凡的才华和对计算机科学的浓厚兴趣。
他在大学期间参加了多个项目团队,展现了出色的领导才能和团队合作精神。
毕业后,他获得了学士学位,并继续攻读硕士学位。
研究生阶段在攻读硕士学位期间,查仁裕对机器学习产生了浓厚的兴趣,并在导师的指导下开始深入研究。
他的研究成果引起了学术界的广泛关注,他的论文被多个国际会议和期刊接受发表。
在研究生阶段,他还积极参与各种学术交流活动,并与国际知名学者展开合作研究。
博士学位与卓越成果毕业后,查仁裕决定继续攻读博士学位,他进入中国科学院计算技术研究所,加入了国内最出色的人工智能研究团队。
在博士阶段,他领导了一项重要的研究项目,通过深入研究神经网络算法,提出了一种新颖的深度学习模型,并在多个国际机器学习比赛中取得了卓越成绩。
学术影响力查仁裕的研究成果在学术界和工业界产生了巨大的影响力。
他的论文被广泛引用,他的研究成果为人工智能领域的发展做出了重要贡献。
他还担任多个人工智能国际会议的程序委员会委员,积极参与学术交流和合作。
他的演讲和讲座也备受关注,在世界各地的学术机构和研究中心都受到热烈欢迎。
企业经历除了学术研究,查仁裕还积极参与企业实践。
他曾在一家人工智能初创公司担任高级算法工程师,在该公司的研发团队中提供技术支持。
他的才华和实践经验使他成为了该公司最受尊重和推崇的员工之一。
公益事业查仁裕不仅在学术和工业界有杰出的贡献,他还十分关注社会公益事业。
他积极参与各种公益活动,为贫困地区的学生提供教育支持,为人工智能教育普及做出了积极努力。
他还与多个非营利组织合作,致力于推动人工智能技术的公平和可持续发展。
未来展望作为人工智能领域的翘楚,查仁裕将继续努力,为该领域的进一步发展贡献自己的力量。
- 1、下载文档前请自行甄别文档内容的完整性,平台不提供额外的编辑、内容补充、找答案等附加服务。
- 2、"仅部分预览"的文档,不可在线预览部分如存在完整性等问题,可反馈申请退款(可完整预览的文档不适用该条件!)。
- 3、如文档侵犯您的权益,请联系客服反馈,我们会尽快为您处理(人工客服工作时间:9:00-18:30)。
Personal and Ubiquitous Computing manuscript No. (will be inserted by the editor)A Taxonomy for Resource Discovery Koen Vanthournout,Geert Deconinck,Ronnie Belmans Katholieke Universiteit Leuven,Belgium,Department Electrical Engineering(ESAT),e-mail:Koen.Vanthournout@esat.kuleuven.ac.be,http://www.esat.kuleuven.ac.beThe date of receipt and acceptance will be inserted by the editorAbstract Resource discovery systems become more and more important as distributed systems grow and as their pool of resources becomes more variable.As such, an increasing amount of networked systems provide a discovery service.This paper provides a taxonomy for resource discovery systems by defining their design as-pects.This allows comparison of the designs of the de-ployed discovery services and is intended as an aid to sys-tem designers when selecting an appropriate mechanism. The surveyed systems are divided into four classes that are separately described.Finally,we identify a hiatus in the design space and point out genuinely distributed resource discovery systems that support dynamic and mobile resources and use attribute-based naming as a main direction for future research in this area.1IntroductionA large and growing number of modern computing sys-tems are composed of a diverse multitude of networked components,that cooperate to accomplish the applica-tion’s targets.Examples are:peer-to-peerfile sharing networks,GRID computing,the ambient environment [13],LAN plug-and-play,etc.,or:Gnutella[14],CAN [25],Globus[9],CORBA[15],UPnP[8],Jini[4],etc1.The enabling technology for all these systems is re-source sharing.But before resources can be shared,po-tential users must have the ability to locate them.This can be accomplished by either manual configuration or discovery at-runtime of the resources(from here on named resource discovery(RD)).The larger the system grows,the more cumbersome manual configurations are. 1A complete list of all systems that were surveyed for this paper can be found in Table2(legend in Table3).Mind that this list is not exhaustive and only includes IP-based resource discovery systems.And if the resource pool is variable and not static,man-ual configuration is ruled out completely.Since the cur-rent trend is towards such larger,complexer and more variable systems,resource discovery becomes an ever more important service,worth special attention.Because of it indispensable nature,all current net-worked systems that contain some level of self-config-uration,already provide a RD service.For instance,a GRID-client’s computational task is automatically dis-tributed to a varying pool of available processing units, in a plug-and-play environment an arriving laptop au-tomaticallyfinds the local printer,afile is located in a peer-to-peer network where nodes continuously enter and leave,etc.Although the resource discovery systems used in these examples pursue comparable tasks,the di-versity of the applied strategies is as large as the different types of applications that require it.The aim of this paper is to provide a taxonomy for the different strategies that are used to tackle IP-based resource discovery.This is accomplished by defining the design space of RD systems.After clarifying the termi-nology,used in this paper,the relevant design aspects and the values they take are discussed(Sect.3).This is followed in Sect.4with a overview of the large clusters of existing RD systems and the indication of the hiatuses in the design space.2TerminologyTo understand resource discovery,one mustfirst clearly understand what is meant by‘resources’.The dictionary gives us following definition:A source of supply,support,or aid,esp.one thatcan be readily drawn upon when needed.We discuss resources in the context of resource discovery as an enabling step for networked resource sharing.Thus, we can transform above definition to:2Koen Vanthournout,Geert Deconinck,Ronnie BelmansDefinition1A resource is any source of supply,sup-port,or aid a component in a networked environment can readily draw upon when needed.Examples are:files,measurements,CPU-cycles,mem-ory,printing,control-devices,forums,online shops,etc. This definition requires further specification,since dif-ferent systems support different types of resources.A classification of resources is presented in Sect.3.7.Definition2Resource discovery is the ability to locate resources that comply to a set of requirements given in a query.Sect.3elaborates on the available mechanisms for re-source discovery.The process of resource discovery involves three main actors:resource providers,resource users and the RD service itself.–Definition3A resource provider is any networked entity that allows sharing of its resources.–Definition4A resource user is any networked en-tity that uses shared resources.–Definition5A resource discovery service is the ser-vice that returns the location of matching resources in response to a query with requirements.Multiple types of actors can be exerted by one single entity in a system,e.g,a resource provider can at the same time be resource user or a discovery service can be implemented by the resource users and providers them-selves,without third party interference(see Sect.3.1). It always remains possible,however,to logically divide a single entity into the actors it’s composed of.The term node is used throughout this paper to indi-cate a network-enabled device.Such a device can contain any of the above actors.3Relevant Design AspectsResource discovery systems can be categorized by dif-ferent design aspects.Each of these aspects represents a design choice for which several solutions are possible. None of these solutions can be entitled as‘the best’so-lution.Depending on the envisioned target application and environment,different choices may prove optimal. Therefore,a classification into a design space will not allow an absolute ranking,but rather permits the iden-tification and comparison of clusters of RD systems with equal targets.It is also an aid to the designers of new systems and helps identifying hiatuses and potential new approaches.A list of the design aspects can be found in Table1.3.1The Service ProviderA designer is presented with two options when selecting how to provide a RD service.First choice is to implement Table1resource discovery design aspectsdesign aspectservice providerconstructionforeknowledgearchitectureregistrationdiscoverysupported resourcesnaming and queriesit as a third party service,i.e.,as an entity,distinctly sep-arate from the resource providers and users.This usu-ally takes the form of a server or collection of servers that gather information on the available resources and use this information to respond to queries of users.The third party type of RD system is the most common one. It is the traditional well-established way to provide ser-vices and allows deterministic and centrally controllable systems.Examples of systems like these are:DNS[19], CORBA[15],Napster[22],etc.(see Table2for a full list and Table3for the legend of Table2).It does occur however,that there is no organization willing or allowed to take the responsibility of providing a centralized RD service.An infamous example is mp3file sharing,but also GRIDs with resources owned by various adminis-trative organizations[18]and ambient intelligence appli-cations[13]fall into this category.The alternative to a third party service is a genuinely distributed system,i.e., the RD service is distributed across all involved resource providers and users,without any central or coordinating components.Examples of genuinely distributed systems are:Gnutella[14],Freenet[6],Tapestry[31],CAN[25], etc.Mind though,that also third party systems can be distributed,although these systems act as a single entity to the outside world.DNS,LDAP[17]and CORBA are only a few examples.Afinal note must be made on systems like Saluta-tion[7]and Jini[4],since these are systems that support both models:in the presence of a RD server,this server provides a third party service,but in absence of such a server,the system automatically switches to genuinely distributed operation(more on this subject in Sect.3.2).3.2ConstructionA networked distributed system constructs an overlay network on top of the actual communication layer.This overlay network is a directed graph:vertices represent network nodes and an edge represents the knowledge of a node about another node.Two alternatives exist for the construction of such an overlay network:they can be manually configured or they can grow by means of self-organization.A Taxonomy for Resource Discovery–Manually Configured Networks:Third partytems that extend beyond the LAN-level aremanually configured,i.e.,a human administrator responsible for providing each server with thetion and function of the other components andand providers must know the address of a server.put differently,a human administrator mustthe overlay network graph:the differentmust be informed of the edges that leave them.manual configuration allows more control andterministic behavior,it is cumbersome to scale.large organizational and human resources required maintain the DNS system can serve as an example.–Self-organizing Networks:Self-organizingbecome interesting when the size of the networklarge,if human configurations are too expensiveif no central authority is available to coordinate con-figuration.Drawback is the increased network traffic, required for the self-organization and maintenance of the overlay net and the complexity of the algorithms.Examples are Gnutella,Freenet,CAN,etc.–Hybrids:Also hybrid solutions are possible.This can be a means to add redundancy to a system and to increase its robustness.Examples are Salutation and Jini,where the absence of a(manually configured) RD server doesn’t imply system failure,since the resource providers and users will automatically self-organize into a complete graph(see Sect.3.4),though this increases the network load significantly.Another class of hybrid systems are those that use multicast or broadcast to self-organize on LAN-level and man-ual configuration to interconnect these self-organized subgraphs at WAN level(e.g.,Ninja SSDS[10]and Uniframe[27]).3.3ForeknowledgeMost systems,even self-organizing,require some config-uration per node,prior to its entrance in the system.The information contained in this node specific configuration is referred to as foreknowledge and is closely related to the method of overlay network construction:–Nodes in manually configured systems require a list of all components they need to interact with.The foreknowledge composes of a list of well-known ad-dresses.–Self-organizing systems that operate at WAN level, usually require at least the address of one random active ing the knowledge of the overlay net-work edges leaving this node,the new node canfind its location.Many require on top of this a unique identifier,though this can often be avoided by usinga hash function on the node’s network-address.–Self-organizing systems that employ multicast or broadcast usually need no foreknowledge,but are lim-ited to operate at LAN level.a tree graph,a ring-shaped regular graph,a2-dimensional Cartesian regular graph,a random graph and a complete graph.3.4ArchitectureThe nodes of a distributed RD system organize into an overlay network,as mentioned before.The architecture of these overlay structures can be visualized by graphs and thus can be categorized by using graph theory[12, 2](see Fig.3.4for graphical representations):–Trivial Graph:A graph of order0or1(0or1ver-tices),is a trivial graph.Order0graphs are logically of no interest to us.Trivial graphs of order1,on the contrary,are the purely centralized systems.The RD system consists of a single server,registering all re-sources and answering all queries.These systems may have scaling difficulties and are prone to single points of failures.Mind that trivial graph servers may still be internally duplicated.Trivial means here that the server has a single point of access.–Tree Graph:Systems organized as trees scale well and allow for backtracking as a search algorithm,but high level node failures affect large portions of the system.They are especially suited for directory sys-tems,e.g.,DNS,LDAP,etc.P-Grid[1]is an exam-ple of a self-organizing system that constructs a tree graph.To overcome the problems associated with high level failures,P-Grid uses node redundancy.–Regular Graph:If nodes are structured in an or-dered lattice and if adjacent nodes are interconnected, the graph is regular.(See[2]for how to measure regu-larity by using clustering coefficients).Self-organizing networks that grow into a regular structure allow4Koen Vanthournout,Geert Deconinck,Ronnie Belmansfor optimized search algorithms2(e.g.,Plaxton rout-ing[23],used by Tapestry).–Random Graph:Systems exhibiting random graph behavior include the manually configured systems, where administrators can interconnect nodes as they please,e.g.,CORBA,and self-organizing networks where the number and nature of links is not prede-fined,e.g.,Gnutella and Freenet.It should be noted, though,that many networks that seem completely random atfirst sight,do have an emergent struc-ture:they are scale free networks[2].The distribu-tion of the number of links per node of scale free net-works follows a power law.Both Gnutella and Freenet exhibit this behavior.Main consequences are an in-creased resilience to random failures and shorter path lengths,but also a larger susceptibility to directed attacks.–Complete Graph:In this case all nodes know each other.Multicast and broadcast systems without cen-tral server(e.g.,Jini)and replicated server RD sys-tems(e.g.,NetSolve[5])are examples.3.5Resource RegistrationBefore requests for resources can be made,a reference to them must be stored on a place where these refer-ences can be predictably accessed.The different tech-niques used are:–Local registration only:Only the resource pro-viders are aware of the resources they share.This implies that a resource can only be located by im-mediate interrogation of the provider.Clearly,this is an inefficient method.If only replicable resources are offered(see Sect.3.7),improvements can be made by replication and caching(e.g.,Freenet).–References:References to the location of a resource are stored on a predictable place in a regular graph structure.Typically this is realized by using hash identifiers for both nodes and resources.References are then stored at the node whose identifier matches the resource’s identifier closest.–Registration at the local server:Resource pro-viders announce their resources to the local server (in case of trivial graphs to the only server).This in-formation may then be stored only locally or locally and at replicated servers.–Manual registration:Resources are registered at RD servers by means of configurationfiles.This is only useful for long-term stable resources,e.g.,URL names stored at DNS servers.2Notable also is‘small world’behavior[30,2]:if nodes in a highly regular structure maintain few links to far parts of the lattice,the average path lengths drop strongly,while the regularity is still preserved.Chord,for instance,uses this technique to optimize search.3.6Query RoutingIf a resource user wants to access a resource and thusfirst needs to locate it,a query with the user’s requirements is composed and send to that user’s local contact point to the RD system.The latter is the local server or,in case of a genuinely distributed system,the RD module on the node itself.This query must then be routed in the graph structure towards the node that knows the location of a matching resource.The strategies are:–Central server or local replicated server:In case of a single local server or a replicated server infrastructure,routing is limited to the single query send from resource users to the local server,where the information is readily available.–Query forwarding:if a node is located in a graph and it fails to match a query,it must forward the query to one or more nodes,based on some metric or rule.For instance,Freenet uses hash identifiers to select the next node a query will be forwarded to(caches enlarge the probability of this being a suc-cessful strategy).The most popular query techniques are:–Flooding:Used by some systems that deploya random graph structure,flooding burdens thecommunication network heavily and requires lim-ited hop-distance settings and special precautionsfor loops.Yetflooding guarantees that the nodewith the requested knowledge will be reached bythe shortest path.The best-known system withflooding is probably Gnutella,but also theCORBA traders use it.Multicast or broadcastLAN-discovery systems that operate withoutserver,can also be included in this category.–Backtracking:The single biggest advantage oftree graph structures is that they allow backtrack-ing search algorithms.–Regular structure routing:The effort of im-plementing the complex algorithms that allownodes to self-organize into a regular lattice hasbut one goal:predictable locations of resource ref-erences and a method to route towards those lo-cations.A multitude of techniques are used forthis purpose(see the footnotes to Table2).Almost all used query forwarding strategies fall into one of the above mentioned sub-categories.An over-view of alternative query forwarding algorithms for RD systems can be found in[18].3.7Supported ResourcesAs defined in Sect.2,resources include everything that could be useful for an entity within a networked system that requires resource sharing and thus resource discov-ery.This includes both services and information.ButA Taxonomy for Resource Discovery5Fig.2The classes of resourcesservices and information are not clearly delineated cate-gories of resources,since they often overlap.E.g.,a mea-surement device provides a service that delivers informa-tion.Better is to sort them in classes by rate of change (see Figure 2):–Fixed resources:These are resources that have both a fixed location and fixed properties.Examples are network printers with fixed properties (color,resolu-tion,etc.),weather stations with a static set of mea-surement devices,etc.–Replicable resources:Basically,replicable resour-ces are fixed resources combined with replicated files.In the case of replicated files,each file will have a sin-gle set of identifiers and the resource discovery service will return the location of one of the copies.–Mobile resources:The class of mobile resources contains resources with a fixed location,replicable resources and resources with a variable location.Ex-amples of the latter are laptops,wireless devices,etc.–Dynamic resources:Dynamic resources are resour-ces that have a fixed location,but whose identifiers can vary.Servers or PC’s that offer their (idle)com-putational resources are an example:the current load and the available amount of CPU time,memory and disk space vary.–Mobile &dynamic resources:This class embraces all resources,delineated above.Some of the surveyed systems target a specific set of resources,but could include other types with few or even no adaptations.Nevertheless,the values in Table 2refer to the resources the system was specifically designed for and not to what could be included.3.8Resource Naming and QueriesReferences to resources are composed of two parts:the location and the name of the resource.Mind that ‘name’could be as large as an XML file.Queries for resour-ces are matched to these names.Consequently,the used naming mechanism defines largely the types of resources that can be served by the RD system and the ease by which those resources can be pare the cum-bersome task of locating resources (e.g.,web pages)by means of clear text boolean queries to the deterministic alternative of assigning values to a selection of predefined attributes.–Unique identifiers and hashes:If a resource has a unique name,it is easier to locate.And,as shown before,such an identifier can be used to define and re-trieve a fixed node where a reference to the resource’s location can be stored.Identifiers like these are usu-ally obtained by using a hash function on the clear text name of the resource.Its main problem is that this system does not allow for changing properties to be reflected in the naming system and thus excludes dynamic resources.Or at least,dynamic properties of resources are not reflected in the RD system.–String naming:While hashes allow no search on specific terms in the resource name/description,clear text naming does allow more complex queries:boolean expressions,‘sound as’queries,etc.–Directories:Directory naming systems build on scal-able hierarchical name spaces,with as most famous examples DNS and LDAP.Most RD systems with directory naming employ the DNS or LDAP syntax or a variant on one of these two.–Attributes:The most powerful naming system is the use of attributes:resources are described by means of a number of predefined attributes that take a value.Attributes allow for extensive queries with predictable results,as opposed to ad-hoc queries used with clear text naming.Attributes can be used for the full range of resources,even for descriptions of dynamic resour-ces.Note that the most commonly used attribute sys-tem is XML (used by UPnP,Ninja SSDS,UniFrame,UDDI,etc.).4The Main Classes of RD SystemsTable 2gives an overview of the RD systems that were surveyed for this paper and lists the values they take for the several design aspects.The legend to Table 2can be found in Table 3.Table 3also serves as an overview of the design aspects and their values.This list of RD6Koen Vanthournout,Geert Deconinck,Ronnie Belmans Table2Resource discovery systems and their properties(legend in Table3),sorted by class(from top to bottom):P2P RD systems,multicast discovery RD systems,distributed third party RD systems and centralized RD systems Name Prov Constr Forekn Arch Res Reg Routing Sup Res NamingChord[11]GD SO ID+RN Reg3Ref@N Route Repl HashTapestry[31]GD SO ID+RN Reg4Ref@N Route Repl HashPastry[26]GD SO ID+RN Reg5Ref@N Route Repl HashCAN[25]GD SO RN Reg6Ref@N Route Repl HashP-Grid[1]GD SO RN Tree Ref@N BT Repl HashFreenet[6]GD SO RN Rand Res@N QF Repl HashGnutella[14]GD SO RN Rand None Flooding Repl StringSalutation[7]Both SO None Compl Node/Serv Flood/Serv Mob Attr Jini[4]Both SO None Compl/Triv Node/Serv Flood/Serv Mob AttrUPnP[8]3P SO None Triv Serv Serv M/D AttrSLP[16]3P SO None Triv Serv Serv Mob Dir Ninja SSDS[10]3P SO/Man No/WKAddr Tree Loc Serv BT Mob AttrUniFrame[27]3P SO/Man No/WKAddr Rand Loc Serv Flooding Fix AttrNetSolve[5]3P Man WKAddr Compl Loc Serv Repl Serv Dyn AttrUDDI[28]3P Man WKAddr Compl Loc Serv Repl Serv Fix AttrRCDS[20]3P Man WKAddr Compl Loc Serv Repl Serv Repl DirCORBA7[15]3P Man WKAddr Rand Loc Serv Flooding Dyn AttrGlobe[29]3P Man WKAddr Tree Loc Serv BT Mob HashDNS[19]3P Man WKAddr Tree Man BT Fix DirLDAP[17]3P Man WKAddr Tree Man BT Fix Dir Matchmaking[24]3P Man WKAddr Triv Serv Serv Dyn AttrNapster[22]3P Man WKAddr Triv Serv Serv Repl StringSuperWeb[3]3P Man WKAddr Triv Serv Serv Dyn AttrNinf[21]3P Man WKAddr Triv Man Serv Dyn AttrGlobus8[9]3P Man WKAddr Triv Man Serv Fix Attrsystems in Table2is not exhaustive,but contains the four classes presented here.4.1Centralized Third Party RD SystemsCentralized RD systems are the systems that have a unique contact point for their users.They are manu-ally configured systems with a trivial graph.The distinct advantage of centralized RD systems is that resource registrations,updates and queries require no(time con-suming)routing,which is reflected in the resources they support:this class represents the largest portion of the 3The regular graph for Chord is built from a circular iden-tifier space.4The regular graph for Tapestry is built from a Plaxton Mesh[23].5The regular graph for Pastry is built from a circular iden-tifier space.6The regular graph for CAN is built from a d-dimensional Cartesian identifier space.7The CORBA object implementing its resource discovery is the Trading Object Service.8The component of GLOBUS that provides the RD service is the MDS(Metacomputing Directory Service).The MDS builds on LDAP and enhances it with attribute-based search.attributes using systems that support dynamic resour-ces.Immediate drawback is scalability and the single point of failure created by the trivial graph.4.2Distributed Third Party RD SystemsThe single point of failure in centralized RD systems can be overcome by replication and multiple contact points to the system.Scalability can be improved by spreading the resource references on several servers.To-gether,these systems make the class of distributed third party systems(identified by requiring manual configu-ration and by a graph of larger complexity than triv-ial).Long established and offering the most straight for-ward billing,these systems are well-developed.This is reflected in their diversity.Indeed,many combinations of techniques are available and together they cover all types of resources.4.3Multicast RD SystemsThe use of multicast(or broadcast)offers a clean and straightforward method to implement resource discov-ery with minimal administration effort(no foreknowl-A Taxonomy for Resource Discovery7 Table3Legend to Table2Prov Service Provider GD Genuinely Distributed3P Third PartyBoth3P,if absent GDConstr Construction SO Self-OrganizingMan Manual configurationSO/Man@LAN level:SO,@WAN level:ManForekn Foreknowledge RN Random NodeID+RN Unique Identifier and RNWKAddr Well-known AddressesNo/WKAddr@LAN level:None,@WAN level:WKAddr Arch Architecture Triv Trivial graphRand Random graphTree Tree graphReg Regular graphCompl Complete graphRes Reg Resource Registration None Local registration onlyRef@N Reference at node with closest IDRes@N Resource cached at nodes with a closer IDServ Registration at unique serverLoc Serv Registration at local serverMan Manual configurationRouting Query Routing Flooding Flood queryBT BacktrackingRoute Route to node with closest IDQF Query forwardingServ Query central serverRepl Serv Query local replicated serverFlood/Serv if GD:Flooding,if3P:ServSup Res Supported Resources Fix Fixed resourcesRepl Replicated resourcesMob Mobile resourcesDyn Dynamic resourcesM/D Mobile&Dynamic resourcesNaming Resource Naming and Queries Hash Hash IDString String naming and queriesDir DirectoriesAttr Attributesedge is needed)and is especially useful if mobile resour-ces are to be handled.Its use,however,is limited by its very enabling technology.The system’s size is restricted to multicast supporting LANs as internet-wide multi-cast is no option yet.Ninja and UniFrame overcome this problem by combining it with a distributed third party approach.However,the drawback of this solution is the manual configurations that are associated with third party RD bination of a genuinely dis-tributed approach(see Sect.4.4)with multicast would be an interesting approach to overcome this,but,as far as the authors know,no such system exists.4.4P2P SystemsGenuinely distributed systems are commonly referred to as peer-to-peer systems(P2P)9,though this might be a confusing term.Nevertheless,P2P is widely accepted and recognized and thus will be used in the remaining of this paper.Two classes of P2P are available:–Random P2P RD Systems:Thefirst class of P2P systems contains systems like Gnutella and Freenet.Using nofixed rules and variating metrics,nodes build a list of other nodes,starting from the location 9Although Napster is commonly accepted as a P2P sys-tem,it is not included here.Napster does use peer-to-peer file transfer,but the RD mechanism is a centralized one.。