Map Learning with Uninterpreted Sensors and Effectors
基于深度学习的传感器信号处理技术研究
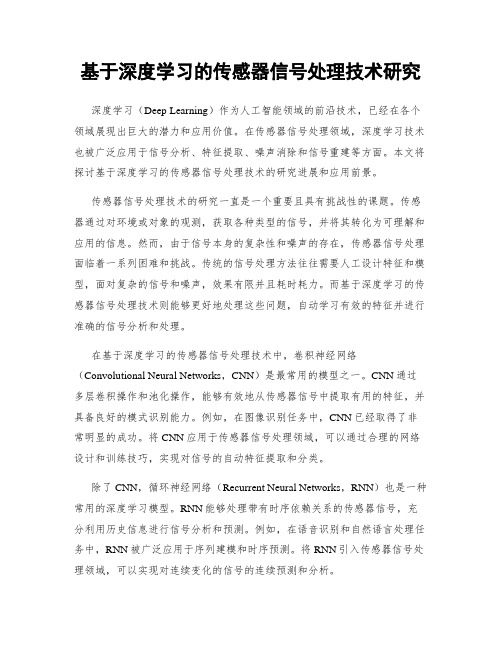
基于深度学习的传感器信号处理技术研究深度学习(Deep Learning)作为人工智能领域的前沿技术,已经在各个领域展现出巨大的潜力和应用价值。
在传感器信号处理领域,深度学习技术也被广泛应用于信号分析、特征提取、噪声消除和信号重建等方面。
本文将探讨基于深度学习的传感器信号处理技术的研究进展和应用前景。
传感器信号处理技术的研究一直是一个重要且具有挑战性的课题。
传感器通过对环境或对象的观测,获取各种类型的信号,并将其转化为可理解和应用的信息。
然而,由于信号本身的复杂性和噪声的存在,传感器信号处理面临着一系列困难和挑战。
传统的信号处理方法往往需要人工设计特征和模型,面对复杂的信号和噪声,效果有限并且耗时耗力。
而基于深度学习的传感器信号处理技术则能够更好地处理这些问题,自动学习有效的特征并进行准确的信号分析和处理。
在基于深度学习的传感器信号处理技术中,卷积神经网络(Convolutional Neural Networks,CNN)是最常用的模型之一。
CNN通过多层卷积操作和池化操作,能够有效地从传感器信号中提取有用的特征,并具备良好的模式识别能力。
例如,在图像识别任务中,CNN已经取得了非常明显的成功。
将CNN应用于传感器信号处理领域,可以通过合理的网络设计和训练技巧,实现对信号的自动特征提取和分类。
除了CNN,循环神经网络(Recurrent Neural Networks,RNN)也是一种常用的深度学习模型。
RNN能够处理带有时序依赖关系的传感器信号,充分利用历史信息进行信号分析和预测。
例如,在语音识别和自然语言处理任务中,RNN被广泛应用于序列建模和时序预测。
将RNN引入传感器信号处理领域,可以实现对连续变化的信号的连续预测和分析。
基于深度学习的传感器信号处理技术还可以应用于噪声消除和信号重建。
噪声是所有传感器信号处理中的一个主要问题,它严重影响了信号质量和信息提取的准确性。
传统的噪声消除方法对于复杂的噪声类型和高斯白噪声的消除效果有限,且需要依赖准确的模型假设。
压缩感知理论

压缩感知理论
压缩感知理论(Compressive Sensing Theory, CSP)是一种用来提高信号采集和处
理效率、使采集传输系统节省资源的研究方向。
它的基本思想是:若一个实际的信号可以
满足一定的限制条件,则其采样、处理和传输所需的资源会比完全采集处理和传输这个信
号所需资源少得多。
简言之,就是在一定的稀疏假设下,有效的采样、处理和传输数据不
仅具有可行性,而且这种方法能够加速传输效率,降低资源消耗。
压缩感知理论(CSP)把信号采集、传输单元称为“感知器(Sensor)”,它是一种
缺乏全部信息的单元,可以仅仅通过选择部分子采集到的信息来对整体信号进行局部估计。
压缩传感的实现的关键在于建立能够快速地准确地完成局部估计的估计方法。
即使是在相
对限制的采样数据和传输带宽的情况下,也可以采取最优或者次优的估计方法,实现高效
而精准的压缩传播。
压缩感知理论(CSP)已经在诸多领域中取得了很大成功。
例如,它可以用来提高影
像处理效率、优化无线通信采样和图像传输、进行脑磁共振图像分析和信号处理等。
同时,它也可以在多源数据合成、脑科学和科学的计算中发挥作用。
压缩感知理论(CSP)为科
学研究带来了各自领域的新途径,使采集、传输技术得以突破性发展,从而为实时信号采
集和处理带来了极大的方便。
压缩感知理论与应用
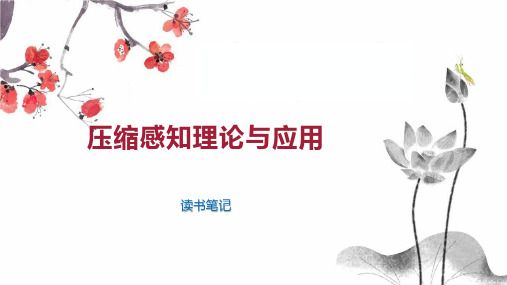
阅读感受
阅读感受
《压缩感知理论与应用》:理论与应用的新领域 在信息科学快速发展的今天,新的理论和技术不断涌现,为我们的生活和工 作带来前所未有的便利。《压缩感知理论与应用》这本书,以其独特的视角和深 度的分析,为我们在信息科学领域开辟了新的视野。
阅读感受
这本书由机械工业社于2019年3月,作者约琳娜·C.埃尔达(Yonina C. Eldar)和吉(G.)为我们提供了压缩感知这一主题的全面介绍。压缩感知是一 种新兴的理论和技术,它允许我们通过少量的测量来恢复信号或图像,从而在数 据采集和处理的效率上带来了革命性的改变。
精彩摘录
精彩摘录
在科技日新月异的今天,压缩感知理论与应用这本书为我们提供了一个全新 的视角,来理解和应用信号处理和数据分析中的一些复杂问题。这本书汇集了众 多领域专家的研究成果,深入浅出地介绍了压缩感知的基本理论、算法和应用。
精彩摘录
书中一个引人注目的观点是“稀疏表示”。在信号处理中,稀疏表示是一种 重要的思想,它认为大多数信号都可以在某种变换下表示为少数非零元素的集合。 这种思想在压缩感知中得到了充分的应用,使得我们可以在远低于奈奎斯特采样 率的条件下,实现对信号的准确重建。
作者简介
作者简介
这是《压缩感知理论与应用》的读书笔记,暂无该书作者的介绍。
谢谢观看
目录分析
目录分析
《压缩感知理论与应用》是一本全面介绍压缩感知理论及其在多个领域应用 的书籍。本书深入浅出地阐述了压缩感知的基本原理、算法和应用,为读者提供 了关于这一前沿领域的一个全面的视角。以下是对本书目录的分析。
目录分析
本书的引言部分为读者提供了关于压缩感知的基本概念和历史背景。作者约 琳娜·C.埃尔达(Yonina C. Eldar)和吉尔·吉(Gill Girilot)在引言中详 细介绍了压缩感知的起源、发展以及在信号处理、图像处理、医学成像等多个领 域的应用。
机器学习与人工智能领域中常用的英语词汇
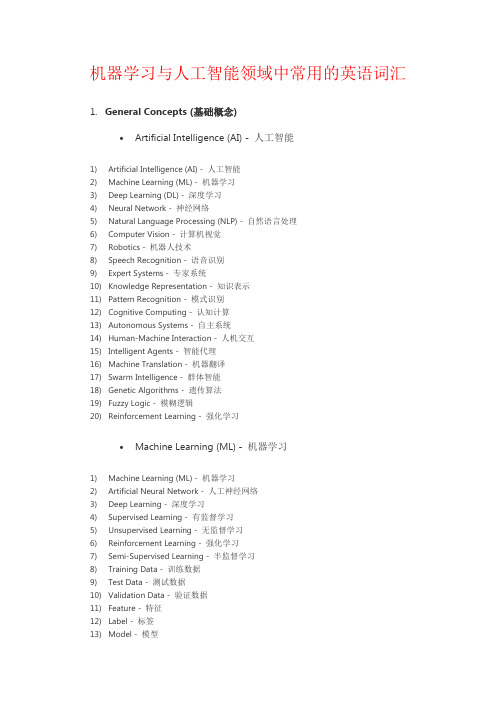
机器学习与人工智能领域中常用的英语词汇1.General Concepts (基础概念)•Artificial Intelligence (AI) - 人工智能1)Artificial Intelligence (AI) - 人工智能2)Machine Learning (ML) - 机器学习3)Deep Learning (DL) - 深度学习4)Neural Network - 神经网络5)Natural Language Processing (NLP) - 自然语言处理6)Computer Vision - 计算机视觉7)Robotics - 机器人技术8)Speech Recognition - 语音识别9)Expert Systems - 专家系统10)Knowledge Representation - 知识表示11)Pattern Recognition - 模式识别12)Cognitive Computing - 认知计算13)Autonomous Systems - 自主系统14)Human-Machine Interaction - 人机交互15)Intelligent Agents - 智能代理16)Machine Translation - 机器翻译17)Swarm Intelligence - 群体智能18)Genetic Algorithms - 遗传算法19)Fuzzy Logic - 模糊逻辑20)Reinforcement Learning - 强化学习•Machine Learning (ML) - 机器学习1)Machine Learning (ML) - 机器学习2)Artificial Neural Network - 人工神经网络3)Deep Learning - 深度学习4)Supervised Learning - 有监督学习5)Unsupervised Learning - 无监督学习6)Reinforcement Learning - 强化学习7)Semi-Supervised Learning - 半监督学习8)Training Data - 训练数据9)Test Data - 测试数据10)Validation Data - 验证数据11)Feature - 特征12)Label - 标签13)Model - 模型14)Algorithm - 算法15)Regression - 回归16)Classification - 分类17)Clustering - 聚类18)Dimensionality Reduction - 降维19)Overfitting - 过拟合20)Underfitting - 欠拟合•Deep Learning (DL) - 深度学习1)Deep Learning - 深度学习2)Neural Network - 神经网络3)Artificial Neural Network (ANN) - 人工神经网络4)Convolutional Neural Network (CNN) - 卷积神经网络5)Recurrent Neural Network (RNN) - 循环神经网络6)Long Short-Term Memory (LSTM) - 长短期记忆网络7)Gated Recurrent Unit (GRU) - 门控循环单元8)Autoencoder - 自编码器9)Generative Adversarial Network (GAN) - 生成对抗网络10)Transfer Learning - 迁移学习11)Pre-trained Model - 预训练模型12)Fine-tuning - 微调13)Feature Extraction - 特征提取14)Activation Function - 激活函数15)Loss Function - 损失函数16)Gradient Descent - 梯度下降17)Backpropagation - 反向传播18)Epoch - 训练周期19)Batch Size - 批量大小20)Dropout - 丢弃法•Neural Network - 神经网络1)Neural Network - 神经网络2)Artificial Neural Network (ANN) - 人工神经网络3)Deep Neural Network (DNN) - 深度神经网络4)Convolutional Neural Network (CNN) - 卷积神经网络5)Recurrent Neural Network (RNN) - 循环神经网络6)Long Short-Term Memory (LSTM) - 长短期记忆网络7)Gated Recurrent Unit (GRU) - 门控循环单元8)Feedforward Neural Network - 前馈神经网络9)Multi-layer Perceptron (MLP) - 多层感知器10)Radial Basis Function Network (RBFN) - 径向基函数网络11)Hopfield Network - 霍普菲尔德网络12)Boltzmann Machine - 玻尔兹曼机13)Autoencoder - 自编码器14)Spiking Neural Network (SNN) - 脉冲神经网络15)Self-organizing Map (SOM) - 自组织映射16)Restricted Boltzmann Machine (RBM) - 受限玻尔兹曼机17)Hebbian Learning - 海比安学习18)Competitive Learning - 竞争学习19)Neuroevolutionary - 神经进化20)Neuron - 神经元•Algorithm - 算法1)Algorithm - 算法2)Supervised Learning Algorithm - 有监督学习算法3)Unsupervised Learning Algorithm - 无监督学习算法4)Reinforcement Learning Algorithm - 强化学习算法5)Classification Algorithm - 分类算法6)Regression Algorithm - 回归算法7)Clustering Algorithm - 聚类算法8)Dimensionality Reduction Algorithm - 降维算法9)Decision Tree Algorithm - 决策树算法10)Random Forest Algorithm - 随机森林算法11)Support Vector Machine (SVM) Algorithm - 支持向量机算法12)K-Nearest Neighbors (KNN) Algorithm - K近邻算法13)Naive Bayes Algorithm - 朴素贝叶斯算法14)Gradient Descent Algorithm - 梯度下降算法15)Genetic Algorithm - 遗传算法16)Neural Network Algorithm - 神经网络算法17)Deep Learning Algorithm - 深度学习算法18)Ensemble Learning Algorithm - 集成学习算法19)Reinforcement Learning Algorithm - 强化学习算法20)Metaheuristic Algorithm - 元启发式算法•Model - 模型1)Model - 模型2)Machine Learning Model - 机器学习模型3)Artificial Intelligence Model - 人工智能模型4)Predictive Model - 预测模型5)Classification Model - 分类模型6)Regression Model - 回归模型7)Generative Model - 生成模型8)Discriminative Model - 判别模型9)Probabilistic Model - 概率模型10)Statistical Model - 统计模型11)Neural Network Model - 神经网络模型12)Deep Learning Model - 深度学习模型13)Ensemble Model - 集成模型14)Reinforcement Learning Model - 强化学习模型15)Support Vector Machine (SVM) Model - 支持向量机模型16)Decision Tree Model - 决策树模型17)Random Forest Model - 随机森林模型18)Naive Bayes Model - 朴素贝叶斯模型19)Autoencoder Model - 自编码器模型20)Convolutional Neural Network (CNN) Model - 卷积神经网络模型•Dataset - 数据集1)Dataset - 数据集2)Training Dataset - 训练数据集3)Test Dataset - 测试数据集4)Validation Dataset - 验证数据集5)Balanced Dataset - 平衡数据集6)Imbalanced Dataset - 不平衡数据集7)Synthetic Dataset - 合成数据集8)Benchmark Dataset - 基准数据集9)Open Dataset - 开放数据集10)Labeled Dataset - 标记数据集11)Unlabeled Dataset - 未标记数据集12)Semi-Supervised Dataset - 半监督数据集13)Multiclass Dataset - 多分类数据集14)Feature Set - 特征集15)Data Augmentation - 数据增强16)Data Preprocessing - 数据预处理17)Missing Data - 缺失数据18)Outlier Detection - 异常值检测19)Data Imputation - 数据插补20)Metadata - 元数据•Training - 训练1)Training - 训练2)Training Data - 训练数据3)Training Phase - 训练阶段4)Training Set - 训练集5)Training Examples - 训练样本6)Training Instance - 训练实例7)Training Algorithm - 训练算法8)Training Model - 训练模型9)Training Process - 训练过程10)Training Loss - 训练损失11)Training Epoch - 训练周期12)Training Batch - 训练批次13)Online Training - 在线训练14)Offline Training - 离线训练15)Continuous Training - 连续训练16)Transfer Learning - 迁移学习17)Fine-Tuning - 微调18)Curriculum Learning - 课程学习19)Self-Supervised Learning - 自监督学习20)Active Learning - 主动学习•Testing - 测试1)Testing - 测试2)Test Data - 测试数据3)Test Set - 测试集4)Test Examples - 测试样本5)Test Instance - 测试实例6)Test Phase - 测试阶段7)Test Accuracy - 测试准确率8)Test Loss - 测试损失9)Test Error - 测试错误10)Test Metrics - 测试指标11)Test Suite - 测试套件12)Test Case - 测试用例13)Test Coverage - 测试覆盖率14)Cross-Validation - 交叉验证15)Holdout Validation - 留出验证16)K-Fold Cross-Validation - K折交叉验证17)Stratified Cross-Validation - 分层交叉验证18)Test Driven Development (TDD) - 测试驱动开发19)A/B Testing - A/B 测试20)Model Evaluation - 模型评估•Validation - 验证1)Validation - 验证2)Validation Data - 验证数据3)Validation Set - 验证集4)Validation Examples - 验证样本5)Validation Instance - 验证实例6)Validation Phase - 验证阶段7)Validation Accuracy - 验证准确率8)Validation Loss - 验证损失9)Validation Error - 验证错误10)Validation Metrics - 验证指标11)Cross-Validation - 交叉验证12)Holdout Validation - 留出验证13)K-Fold Cross-Validation - K折交叉验证14)Stratified Cross-Validation - 分层交叉验证15)Leave-One-Out Cross-Validation - 留一法交叉验证16)Validation Curve - 验证曲线17)Hyperparameter Validation - 超参数验证18)Model Validation - 模型验证19)Early Stopping - 提前停止20)Validation Strategy - 验证策略•Supervised Learning - 有监督学习1)Supervised Learning - 有监督学习2)Label - 标签3)Feature - 特征4)Target - 目标5)Training Labels - 训练标签6)Training Features - 训练特征7)Training Targets - 训练目标8)Training Examples - 训练样本9)Training Instance - 训练实例10)Regression - 回归11)Classification - 分类12)Predictor - 预测器13)Regression Model - 回归模型14)Classifier - 分类器15)Decision Tree - 决策树16)Support Vector Machine (SVM) - 支持向量机17)Neural Network - 神经网络18)Feature Engineering - 特征工程19)Model Evaluation - 模型评估20)Overfitting - 过拟合21)Underfitting - 欠拟合22)Bias-Variance Tradeoff - 偏差-方差权衡•Unsupervised Learning - 无监督学习1)Unsupervised Learning - 无监督学习2)Clustering - 聚类3)Dimensionality Reduction - 降维4)Anomaly Detection - 异常检测5)Association Rule Learning - 关联规则学习6)Feature Extraction - 特征提取7)Feature Selection - 特征选择8)K-Means - K均值9)Hierarchical Clustering - 层次聚类10)Density-Based Clustering - 基于密度的聚类11)Principal Component Analysis (PCA) - 主成分分析12)Independent Component Analysis (ICA) - 独立成分分析13)T-distributed Stochastic Neighbor Embedding (t-SNE) - t分布随机邻居嵌入14)Gaussian Mixture Model (GMM) - 高斯混合模型15)Self-Organizing Maps (SOM) - 自组织映射16)Autoencoder - 自动编码器17)Latent Variable - 潜变量18)Data Preprocessing - 数据预处理19)Outlier Detection - 异常值检测20)Clustering Algorithm - 聚类算法•Reinforcement Learning - 强化学习1)Reinforcement Learning - 强化学习2)Agent - 代理3)Environment - 环境4)State - 状态5)Action - 动作6)Reward - 奖励7)Policy - 策略8)Value Function - 值函数9)Q-Learning - Q学习10)Deep Q-Network (DQN) - 深度Q网络11)Policy Gradient - 策略梯度12)Actor-Critic - 演员-评论家13)Exploration - 探索14)Exploitation - 开发15)Temporal Difference (TD) - 时间差分16)Markov Decision Process (MDP) - 马尔可夫决策过程17)State-Action-Reward-State-Action (SARSA) - 状态-动作-奖励-状态-动作18)Policy Iteration - 策略迭代19)Value Iteration - 值迭代20)Monte Carlo Methods - 蒙特卡洛方法•Semi-Supervised Learning - 半监督学习1)Semi-Supervised Learning - 半监督学习2)Labeled Data - 有标签数据3)Unlabeled Data - 无标签数据4)Label Propagation - 标签传播5)Self-Training - 自训练6)Co-Training - 协同训练7)Transudative Learning - 传导学习8)Inductive Learning - 归纳学习9)Manifold Regularization - 流形正则化10)Graph-based Methods - 基于图的方法11)Cluster Assumption - 聚类假设12)Low-Density Separation - 低密度分离13)Semi-Supervised Support Vector Machines (S3VM) - 半监督支持向量机14)Expectation-Maximization (EM) - 期望最大化15)Co-EM - 协同期望最大化16)Entropy-Regularized EM - 熵正则化EM17)Mean Teacher - 平均教师18)Virtual Adversarial Training - 虚拟对抗训练19)Tri-training - 三重训练20)Mix Match - 混合匹配•Feature - 特征1)Feature - 特征2)Feature Engineering - 特征工程3)Feature Extraction - 特征提取4)Feature Selection - 特征选择5)Input Features - 输入特征6)Output Features - 输出特征7)Feature Vector - 特征向量8)Feature Space - 特征空间9)Feature Representation - 特征表示10)Feature Transformation - 特征转换11)Feature Importance - 特征重要性12)Feature Scaling - 特征缩放13)Feature Normalization - 特征归一化14)Feature Encoding - 特征编码15)Feature Fusion - 特征融合16)Feature Dimensionality Reduction - 特征维度减少17)Continuous Feature - 连续特征18)Categorical Feature - 分类特征19)Nominal Feature - 名义特征20)Ordinal Feature - 有序特征•Label - 标签1)Label - 标签2)Labeling - 标注3)Ground Truth - 地面真值4)Class Label - 类别标签5)Target Variable - 目标变量6)Labeling Scheme - 标注方案7)Multi-class Labeling - 多类别标注8)Binary Labeling - 二分类标注9)Label Noise - 标签噪声10)Labeling Error - 标注错误11)Label Propagation - 标签传播12)Unlabeled Data - 无标签数据13)Labeled Data - 有标签数据14)Semi-supervised Learning - 半监督学习15)Active Learning - 主动学习16)Weakly Supervised Learning - 弱监督学习17)Noisy Label Learning - 噪声标签学习18)Self-training - 自训练19)Crowdsourcing Labeling - 众包标注20)Label Smoothing - 标签平滑化•Prediction - 预测1)Prediction - 预测2)Forecasting - 预测3)Regression - 回归4)Classification - 分类5)Time Series Prediction - 时间序列预测6)Forecast Accuracy - 预测准确性7)Predictive Modeling - 预测建模8)Predictive Analytics - 预测分析9)Forecasting Method - 预测方法10)Predictive Performance - 预测性能11)Predictive Power - 预测能力12)Prediction Error - 预测误差13)Prediction Interval - 预测区间14)Prediction Model - 预测模型15)Predictive Uncertainty - 预测不确定性16)Forecast Horizon - 预测时间跨度17)Predictive Maintenance - 预测性维护18)Predictive Policing - 预测式警务19)Predictive Healthcare - 预测性医疗20)Predictive Maintenance - 预测性维护•Classification - 分类1)Classification - 分类2)Classifier - 分类器3)Class - 类别4)Classify - 对数据进行分类5)Class Label - 类别标签6)Binary Classification - 二元分类7)Multiclass Classification - 多类分类8)Class Probability - 类别概率9)Decision Boundary - 决策边界10)Decision Tree - 决策树11)Support Vector Machine (SVM) - 支持向量机12)K-Nearest Neighbors (KNN) - K最近邻算法13)Naive Bayes - 朴素贝叶斯14)Logistic Regression - 逻辑回归15)Random Forest - 随机森林16)Neural Network - 神经网络17)SoftMax Function - SoftMax函数18)One-vs-All (One-vs-Rest) - 一对多(一对剩余)19)Ensemble Learning - 集成学习20)Confusion Matrix - 混淆矩阵•Regression - 回归1)Regression Analysis - 回归分析2)Linear Regression - 线性回归3)Multiple Regression - 多元回归4)Polynomial Regression - 多项式回归5)Logistic Regression - 逻辑回归6)Ridge Regression - 岭回归7)Lasso Regression - Lasso回归8)Elastic Net Regression - 弹性网络回归9)Regression Coefficients - 回归系数10)Residuals - 残差11)Ordinary Least Squares (OLS) - 普通最小二乘法12)Ridge Regression Coefficient - 岭回归系数13)Lasso Regression Coefficient - Lasso回归系数14)Elastic Net Regression Coefficient - 弹性网络回归系数15)Regression Line - 回归线16)Prediction Error - 预测误差17)Regression Model - 回归模型18)Nonlinear Regression - 非线性回归19)Generalized Linear Models (GLM) - 广义线性模型20)Coefficient of Determination (R-squared) - 决定系数21)F-test - F检验22)Homoscedasticity - 同方差性23)Heteroscedasticity - 异方差性24)Autocorrelation - 自相关25)Multicollinearity - 多重共线性26)Outliers - 异常值27)Cross-validation - 交叉验证28)Feature Selection - 特征选择29)Feature Engineering - 特征工程30)Regularization - 正则化2.Neural Networks and Deep Learning (神经网络与深度学习)•Convolutional Neural Network (CNN) - 卷积神经网络1)Convolutional Neural Network (CNN) - 卷积神经网络2)Convolution Layer - 卷积层3)Feature Map - 特征图4)Convolution Operation - 卷积操作5)Stride - 步幅6)Padding - 填充7)Pooling Layer - 池化层8)Max Pooling - 最大池化9)Average Pooling - 平均池化10)Fully Connected Layer - 全连接层11)Activation Function - 激活函数12)Rectified Linear Unit (ReLU) - 线性修正单元13)Dropout - 随机失活14)Batch Normalization - 批量归一化15)Transfer Learning - 迁移学习16)Fine-Tuning - 微调17)Image Classification - 图像分类18)Object Detection - 物体检测19)Semantic Segmentation - 语义分割20)Instance Segmentation - 实例分割21)Generative Adversarial Network (GAN) - 生成对抗网络22)Image Generation - 图像生成23)Style Transfer - 风格迁移24)Convolutional Autoencoder - 卷积自编码器25)Recurrent Neural Network (RNN) - 循环神经网络•Recurrent Neural Network (RNN) - 循环神经网络1)Recurrent Neural Network (RNN) - 循环神经网络2)Long Short-Term Memory (LSTM) - 长短期记忆网络3)Gated Recurrent Unit (GRU) - 门控循环单元4)Sequence Modeling - 序列建模5)Time Series Prediction - 时间序列预测6)Natural Language Processing (NLP) - 自然语言处理7)Text Generation - 文本生成8)Sentiment Analysis - 情感分析9)Named Entity Recognition (NER) - 命名实体识别10)Part-of-Speech Tagging (POS Tagging) - 词性标注11)Sequence-to-Sequence (Seq2Seq) - 序列到序列12)Attention Mechanism - 注意力机制13)Encoder-Decoder Architecture - 编码器-解码器架构14)Bidirectional RNN - 双向循环神经网络15)Teacher Forcing - 强制教师法16)Backpropagation Through Time (BPTT) - 通过时间的反向传播17)Vanishing Gradient Problem - 梯度消失问题18)Exploding Gradient Problem - 梯度爆炸问题19)Language Modeling - 语言建模20)Speech Recognition - 语音识别•Long Short-Term Memory (LSTM) - 长短期记忆网络1)Long Short-Term Memory (LSTM) - 长短期记忆网络2)Cell State - 细胞状态3)Hidden State - 隐藏状态4)Forget Gate - 遗忘门5)Input Gate - 输入门6)Output Gate - 输出门7)Peephole Connections - 窥视孔连接8)Gated Recurrent Unit (GRU) - 门控循环单元9)Vanishing Gradient Problem - 梯度消失问题10)Exploding Gradient Problem - 梯度爆炸问题11)Sequence Modeling - 序列建模12)Time Series Prediction - 时间序列预测13)Natural Language Processing (NLP) - 自然语言处理14)Text Generation - 文本生成15)Sentiment Analysis - 情感分析16)Named Entity Recognition (NER) - 命名实体识别17)Part-of-Speech Tagging (POS Tagging) - 词性标注18)Attention Mechanism - 注意力机制19)Encoder-Decoder Architecture - 编码器-解码器架构20)Bidirectional LSTM - 双向长短期记忆网络•Attention Mechanism - 注意力机制1)Attention Mechanism - 注意力机制2)Self-Attention - 自注意力3)Multi-Head Attention - 多头注意力4)Transformer - 变换器5)Query - 查询6)Key - 键7)Value - 值8)Query-Value Attention - 查询-值注意力9)Dot-Product Attention - 点积注意力10)Scaled Dot-Product Attention - 缩放点积注意力11)Additive Attention - 加性注意力12)Context Vector - 上下文向量13)Attention Score - 注意力分数14)SoftMax Function - SoftMax函数15)Attention Weight - 注意力权重16)Global Attention - 全局注意力17)Local Attention - 局部注意力18)Positional Encoding - 位置编码19)Encoder-Decoder Attention - 编码器-解码器注意力20)Cross-Modal Attention - 跨模态注意力•Generative Adversarial Network (GAN) - 生成对抗网络1)Generative Adversarial Network (GAN) - 生成对抗网络2)Generator - 生成器3)Discriminator - 判别器4)Adversarial Training - 对抗训练5)Minimax Game - 极小极大博弈6)Nash Equilibrium - 纳什均衡7)Mode Collapse - 模式崩溃8)Training Stability - 训练稳定性9)Loss Function - 损失函数10)Discriminative Loss - 判别损失11)Generative Loss - 生成损失12)Wasserstein GAN (WGAN) - Wasserstein GAN(WGAN)13)Deep Convolutional GAN (DCGAN) - 深度卷积生成对抗网络(DCGAN)14)Conditional GAN (c GAN) - 条件生成对抗网络(c GAN)15)Style GAN - 风格生成对抗网络16)Cycle GAN - 循环生成对抗网络17)Progressive Growing GAN (PGGAN) - 渐进式增长生成对抗网络(PGGAN)18)Self-Attention GAN (SAGAN) - 自注意力生成对抗网络(SAGAN)19)Big GAN - 大规模生成对抗网络20)Adversarial Examples - 对抗样本•Encoder-Decoder - 编码器-解码器1)Encoder-Decoder Architecture - 编码器-解码器架构2)Encoder - 编码器3)Decoder - 解码器4)Sequence-to-Sequence Model (Seq2Seq) - 序列到序列模型5)State Vector - 状态向量6)Context Vector - 上下文向量7)Hidden State - 隐藏状态8)Attention Mechanism - 注意力机制9)Teacher Forcing - 强制教师法10)Beam Search - 束搜索11)Recurrent Neural Network (RNN) - 循环神经网络12)Long Short-Term Memory (LSTM) - 长短期记忆网络13)Gated Recurrent Unit (GRU) - 门控循环单元14)Bidirectional Encoder - 双向编码器15)Greedy Decoding - 贪婪解码16)Masking - 遮盖17)Dropout - 随机失活18)Embedding Layer - 嵌入层19)Cross-Entropy Loss - 交叉熵损失20)Tokenization - 令牌化•Transfer Learning - 迁移学习1)Transfer Learning - 迁移学习2)Source Domain - 源领域3)Target Domain - 目标领域4)Fine-Tuning - 微调5)Domain Adaptation - 领域自适应6)Pre-Trained Model - 预训练模型7)Feature Extraction - 特征提取8)Knowledge Transfer - 知识迁移9)Unsupervised Domain Adaptation - 无监督领域自适应10)Semi-Supervised Domain Adaptation - 半监督领域自适应11)Multi-Task Learning - 多任务学习12)Data Augmentation - 数据增强13)Task Transfer - 任务迁移14)Model Agnostic Meta-Learning (MAML) - 与模型无关的元学习(MAML)15)One-Shot Learning - 单样本学习16)Zero-Shot Learning - 零样本学习17)Few-Shot Learning - 少样本学习18)Knowledge Distillation - 知识蒸馏19)Representation Learning - 表征学习20)Adversarial Transfer Learning - 对抗迁移学习•Pre-trained Models - 预训练模型1)Pre-trained Model - 预训练模型2)Transfer Learning - 迁移学习3)Fine-Tuning - 微调4)Knowledge Transfer - 知识迁移5)Domain Adaptation - 领域自适应6)Feature Extraction - 特征提取7)Representation Learning - 表征学习8)Language Model - 语言模型9)Bidirectional Encoder Representations from Transformers (BERT) - 双向编码器结构转换器10)Generative Pre-trained Transformer (GPT) - 生成式预训练转换器11)Transformer-based Models - 基于转换器的模型12)Masked Language Model (MLM) - 掩蔽语言模型13)Cloze Task - 填空任务14)Tokenization - 令牌化15)Word Embeddings - 词嵌入16)Sentence Embeddings - 句子嵌入17)Contextual Embeddings - 上下文嵌入18)Self-Supervised Learning - 自监督学习19)Large-Scale Pre-trained Models - 大规模预训练模型•Loss Function - 损失函数1)Loss Function - 损失函数2)Mean Squared Error (MSE) - 均方误差3)Mean Absolute Error (MAE) - 平均绝对误差4)Cross-Entropy Loss - 交叉熵损失5)Binary Cross-Entropy Loss - 二元交叉熵损失6)Categorical Cross-Entropy Loss - 分类交叉熵损失7)Hinge Loss - 合页损失8)Huber Loss - Huber损失9)Wasserstein Distance - Wasserstein距离10)Triplet Loss - 三元组损失11)Contrastive Loss - 对比损失12)Dice Loss - Dice损失13)Focal Loss - 焦点损失14)GAN Loss - GAN损失15)Adversarial Loss - 对抗损失16)L1 Loss - L1损失17)L2 Loss - L2损失18)Huber Loss - Huber损失19)Quantile Loss - 分位数损失•Activation Function - 激活函数1)Activation Function - 激活函数2)Sigmoid Function - Sigmoid函数3)Hyperbolic Tangent Function (Tanh) - 双曲正切函数4)Rectified Linear Unit (Re LU) - 矩形线性单元5)Parametric Re LU (P Re LU) - 参数化Re LU6)Exponential Linear Unit (ELU) - 指数线性单元7)Swish Function - Swish函数8)Softplus Function - Soft plus函数9)Softmax Function - SoftMax函数10)Hard Tanh Function - 硬双曲正切函数11)Softsign Function - Softsign函数12)GELU (Gaussian Error Linear Unit) - GELU(高斯误差线性单元)13)Mish Function - Mish函数14)CELU (Continuous Exponential Linear Unit) - CELU(连续指数线性单元)15)Bent Identity Function - 弯曲恒等函数16)Gaussian Error Linear Units (GELUs) - 高斯误差线性单元17)Adaptive Piecewise Linear (APL) - 自适应分段线性函数18)Radial Basis Function (RBF) - 径向基函数•Backpropagation - 反向传播1)Backpropagation - 反向传播2)Gradient Descent - 梯度下降3)Partial Derivative - 偏导数4)Chain Rule - 链式法则5)Forward Pass - 前向传播6)Backward Pass - 反向传播7)Computational Graph - 计算图8)Neural Network - 神经网络9)Loss Function - 损失函数10)Gradient Calculation - 梯度计算11)Weight Update - 权重更新12)Activation Function - 激活函数13)Optimizer - 优化器14)Learning Rate - 学习率15)Mini-Batch Gradient Descent - 小批量梯度下降16)Stochastic Gradient Descent (SGD) - 随机梯度下降17)Batch Gradient Descent - 批量梯度下降18)Momentum - 动量19)Adam Optimizer - Adam优化器20)Learning Rate Decay - 学习率衰减•Gradient Descent - 梯度下降1)Gradient Descent - 梯度下降2)Stochastic Gradient Descent (SGD) - 随机梯度下降3)Mini-Batch Gradient Descent - 小批量梯度下降4)Batch Gradient Descent - 批量梯度下降5)Learning Rate - 学习率6)Momentum - 动量7)Adaptive Moment Estimation (Adam) - 自适应矩估计8)RMSprop - 均方根传播9)Learning Rate Schedule - 学习率调度10)Convergence - 收敛11)Divergence - 发散12)Adagrad - 自适应学习速率方法13)Adadelta - 自适应增量学习率方法14)Adamax - 自适应矩估计的扩展版本15)Nadam - Nesterov Accelerated Adaptive Moment Estimation16)Learning Rate Decay - 学习率衰减17)Step Size - 步长18)Conjugate Gradient Descent - 共轭梯度下降19)Line Search - 线搜索20)Newton's Method - 牛顿法•Learning Rate - 学习率1)Learning Rate - 学习率2)Adaptive Learning Rate - 自适应学习率3)Learning Rate Decay - 学习率衰减4)Initial Learning Rate - 初始学习率5)Step Size - 步长6)Momentum - 动量7)Exponential Decay - 指数衰减8)Annealing - 退火9)Cyclical Learning Rate - 循环学习率10)Learning Rate Schedule - 学习率调度11)Warm-up - 预热12)Learning Rate Policy - 学习率策略13)Learning Rate Annealing - 学习率退火14)Cosine Annealing - 余弦退火15)Gradient Clipping - 梯度裁剪16)Adapting Learning Rate - 适应学习率17)Learning Rate Multiplier - 学习率倍增器18)Learning Rate Reduction - 学习率降低19)Learning Rate Update - 学习率更新20)Scheduled Learning Rate - 定期学习率•Batch Size - 批量大小1)Batch Size - 批量大小2)Mini-Batch - 小批量3)Batch Gradient Descent - 批量梯度下降4)Stochastic Gradient Descent (SGD) - 随机梯度下降5)Mini-Batch Gradient Descent - 小批量梯度下降6)Online Learning - 在线学习7)Full-Batch - 全批量8)Data Batch - 数据批次9)Training Batch - 训练批次10)Batch Normalization - 批量归一化11)Batch-wise Optimization - 批量优化12)Batch Processing - 批量处理13)Batch Sampling - 批量采样14)Adaptive Batch Size - 自适应批量大小15)Batch Splitting - 批量分割16)Dynamic Batch Size - 动态批量大小17)Fixed Batch Size - 固定批量大小18)Batch-wise Inference - 批量推理19)Batch-wise Training - 批量训练20)Batch Shuffling - 批量洗牌•Epoch - 训练周期1)Training Epoch - 训练周期2)Epoch Size - 周期大小3)Early Stopping - 提前停止4)Validation Set - 验证集5)Training Set - 训练集6)Test Set - 测试集7)Overfitting - 过拟合8)Underfitting - 欠拟合9)Model Evaluation - 模型评估10)Model Selection - 模型选择11)Hyperparameter Tuning - 超参数调优12)Cross-Validation - 交叉验证13)K-fold Cross-Validation - K折交叉验证14)Stratified Cross-Validation - 分层交叉验证15)Leave-One-Out Cross-Validation (LOOCV) - 留一法交叉验证16)Grid Search - 网格搜索17)Random Search - 随机搜索18)Model Complexity - 模型复杂度19)Learning Curve - 学习曲线20)Convergence - 收敛3.Machine Learning Techniques and Algorithms (机器学习技术与算法)•Decision Tree - 决策树1)Decision Tree - 决策树2)Node - 节点3)Root Node - 根节点4)Leaf Node - 叶节点5)Internal Node - 内部节点6)Splitting Criterion - 分裂准则7)Gini Impurity - 基尼不纯度8)Entropy - 熵9)Information Gain - 信息增益10)Gain Ratio - 增益率11)Pruning - 剪枝12)Recursive Partitioning - 递归分割13)CART (Classification and Regression Trees) - 分类回归树14)ID3 (Iterative Dichotomiser 3) - 迭代二叉树315)C4.5 (successor of ID3) - C4.5(ID3的后继者)16)C5.0 (successor of C4.5) - C5.0(C4.5的后继者)17)Split Point - 分裂点18)Decision Boundary - 决策边界19)Pruned Tree - 剪枝后的树20)Decision Tree Ensemble - 决策树集成•Random Forest - 随机森林1)Random Forest - 随机森林2)Ensemble Learning - 集成学习3)Bootstrap Sampling - 自助采样4)Bagging (Bootstrap Aggregating) - 装袋法5)Out-of-Bag (OOB) Error - 袋外误差6)Feature Subset - 特征子集7)Decision Tree - 决策树8)Base Estimator - 基础估计器9)Tree Depth - 树深度10)Randomization - 随机化11)Majority Voting - 多数投票12)Feature Importance - 特征重要性13)OOB Score - 袋外得分14)Forest Size - 森林大小15)Max Features - 最大特征数16)Min Samples Split - 最小分裂样本数17)Min Samples Leaf - 最小叶节点样本数18)Gini Impurity - 基尼不纯度19)Entropy - 熵20)Variable Importance - 变量重要性•Support Vector Machine (SVM) - 支持向量机1)Support Vector Machine (SVM) - 支持向量机2)Hyperplane - 超平面3)Kernel Trick - 核技巧4)Kernel Function - 核函数5)Margin - 间隔6)Support Vectors - 支持向量7)Decision Boundary - 决策边界8)Maximum Margin Classifier - 最大间隔分类器9)Soft Margin Classifier - 软间隔分类器10) C Parameter - C参数11)Radial Basis Function (RBF) Kernel - 径向基函数核12)Polynomial Kernel - 多项式核13)Linear Kernel - 线性核14)Quadratic Kernel - 二次核15)Gaussian Kernel - 高斯核16)Regularization - 正则化17)Dual Problem - 对偶问题18)Primal Problem - 原始问题19)Kernelized SVM - 核化支持向量机20)Multiclass SVM - 多类支持向量机•K-Nearest Neighbors (KNN) - K-最近邻1)K-Nearest Neighbors (KNN) - K-最近邻2)Nearest Neighbor - 最近邻3)Distance Metric - 距离度量4)Euclidean Distance - 欧氏距离5)Manhattan Distance - 曼哈顿距离6)Minkowski Distance - 闵可夫斯基距离7)Cosine Similarity - 余弦相似度8)K Value - K值9)Majority Voting - 多数投票10)Weighted KNN - 加权KNN11)Radius Neighbors - 半径邻居12)Ball Tree - 球树13)KD Tree - KD树14)Locality-Sensitive Hashing (LSH) - 局部敏感哈希15)Curse of Dimensionality - 维度灾难16)Class Label - 类标签17)Training Set - 训练集18)Test Set - 测试集19)Validation Set - 验证集20)Cross-Validation - 交叉验证•Naive Bayes - 朴素贝叶斯1)Naive Bayes - 朴素贝叶斯2)Bayes' Theorem - 贝叶斯定理3)Prior Probability - 先验概率4)Posterior Probability - 后验概率5)Likelihood - 似然6)Class Conditional Probability - 类条件概率7)Feature Independence Assumption - 特征独立假设8)Multinomial Naive Bayes - 多项式朴素贝叶斯9)Gaussian Naive Bayes - 高斯朴素贝叶斯10)Bernoulli Naive Bayes - 伯努利朴素贝叶斯11)Laplace Smoothing - 拉普拉斯平滑12)Add-One Smoothing - 加一平滑13)Maximum A Posteriori (MAP) - 最大后验概率14)Maximum Likelihood Estimation (MLE) - 最大似然估计15)Classification - 分类16)Feature Vectors - 特征向量17)Training Set - 训练集18)Test Set - 测试集19)Class Label - 类标签20)Confusion Matrix - 混淆矩阵•Clustering - 聚类1)Clustering - 聚类2)Centroid - 质心3)Cluster Analysis - 聚类分析4)Partitioning Clustering - 划分式聚类5)Hierarchical Clustering - 层次聚类6)Density-Based Clustering - 基于密度的聚类7)K-Means Clustering - K均值聚类8)K-Medoids Clustering - K中心点聚类9)DBSCAN (Density-Based Spatial Clustering of Applications with Noise) - 基于密度的空间聚类算法10)Agglomerative Clustering - 聚合式聚类11)Dendrogram - 系统树图12)Silhouette Score - 轮廓系数13)Elbow Method - 肘部法则14)Clustering Validation - 聚类验证15)Intra-cluster Distance - 类内距离16)Inter-cluster Distance - 类间距离17)Cluster Cohesion - 类内连贯性18)Cluster Separation - 类间分离度19)Cluster Assignment - 聚类分配20)Cluster Label - 聚类标签•K-Means - K-均值1)K-Means - K-均值2)Centroid - 质心3)Cluster - 聚类4)Cluster Center - 聚类中心5)Cluster Assignment - 聚类分配6)Cluster Analysis - 聚类分析7)K Value - K值8)Elbow Method - 肘部法则9)Inertia - 惯性10)Silhouette Score - 轮廓系数11)Convergence - 收敛12)Initialization - 初始化13)Euclidean Distance - 欧氏距离14)Manhattan Distance - 曼哈顿距离15)Distance Metric - 距离度量16)Cluster Radius - 聚类半径17)Within-Cluster Variation - 类内变异18)Cluster Quality - 聚类质量19)Clustering Algorithm - 聚类算法20)Clustering Validation - 聚类验证•Dimensionality Reduction - 降维1)Dimensionality Reduction - 降维2)Feature Extraction - 特征提取3)Feature Selection - 特征选择4)Principal Component Analysis (PCA) - 主成分分析5)Singular Value Decomposition (SVD) - 奇异值分解6)Linear Discriminant Analysis (LDA) - 线性判别分析7)t-Distributed Stochastic Neighbor Embedding (t-SNE) - t-分布随机邻域嵌入8)Autoencoder - 自编码器9)Manifold Learning - 流形学习10)Locally Linear Embedding (LLE) - 局部线性嵌入11)Isomap - 等度量映射12)Uniform Manifold Approximation and Projection (UMAP) - 均匀流形逼近与投影13)Kernel PCA - 核主成分分析14)Non-negative Matrix Factorization (NMF) - 非负矩阵分解15)Independent Component Analysis (ICA) - 独立成分分析16)Variational Autoencoder (VAE) - 变分自编码器17)Sparse Coding - 稀疏编码18)Random Projection - 随机投影19)Neighborhood Preserving Embedding (NPE) - 保持邻域结构的嵌入20)Curvilinear Component Analysis (CCA) - 曲线成分分析•Principal Component Analysis (PCA) - 主成分分析1)Principal Component Analysis (PCA) - 主成分分析2)Eigenvector - 特征向量3)Eigenvalue - 特征值4)Covariance Matrix - 协方差矩阵。
高光谱图像距离度量与无监督特征学习研究

高光谱图像距离度量与无监督特征学习研究随着遥感技术的不断发展,高光谱图像在土地覆盖分类、环境监测、农业资源管理等领域展示出了巨大的潜力。
然而,高光谱图像的特征维度高、信息冗余度大等问题给图像处理和分析带来了巨大的挑战。
为了应对这些问题,研究人员提出了一种无监督特征学习的方法,以降低高光谱图像的维度并挖掘其潜在信息。
同时,距离度量也成为了高光谱图像处理中一个重要的研究方向,用于度量高维特征空间中样本之间的相似性。
无监督特征学习是一种在没有标签信息的情况下进行特征学习的方法。
在高光谱图像处理中,无监督特征学习可以通过自动学习和发现数据中存在的特征,从而明显降低了数据的维度,并提供了对数据潜在结构的理解。
其中,主成分分析(Principal Component Analysis,PCA)是无监督降维的主要方法之一。
它通过线性变换将高维数据映射到低维空间,其中低维空间保留了大部分的原始数据方差,从而达到降维目的。
此外,独立成分分析(Independent Component Analysis,ICA)也是一种经典的无监督特征学习方法,它通过解决数据的盲源分离问题,得到独立的高维特征表示。
这些无监督特征学习方法为高光谱图像处理提供了有力的工具,可以有效地降低维度、减少冗余信息,并提高后续任务的性能。
距离度量是用于衡量样本之间相似性或距离的方法。
在高光谱图像处理中,距离度量可以用于聚类、分类和检索等任务中。
传统的欧几里得距离在高光谱图像处理中常被用来度量样本之间的相似性,但是欧几里得距离只考虑了样本之间的空间距离,并忽略了样本在高维特征空间中的分布信息。
因此,传统的距离度量方法往往无法准确地刻画高光谱图像中样本之间的差异。
为了解决这个问题,研究人员提出了许多新的距离度量方法。
例如,流形学习方法可以将高维数据映射到低维流形空间,从而有效地保留了数据的局部结构信息。
核函数方法通过在高维特征空间中引入核函数,将样本映射到特征空间中的非线性空间,从而更好地刻画了样本之间的相似性。
人工智能算法工程师:深度学习与神经网络算法培训ppt

TensorFlow框架特点及使用方法
特点
TensorFlow是一个开源的深度学习框架,具有高度的灵活性 和可扩展性。它支持分布式训练,能够在多个GPU和CPU上 加速训练过程。TensorFlow还提供了丰富的API和工具,方 便用户进行模型开发和调试。
使用方法
使用TensorFlow进行深度学习需要先安装TensorFlow库,然 后通过编写Python代码来定义模型、加载数据、进行训练和 评估等操作。TensorFlow提供了高级的API,如Keras,可以 简化模型开发和训练过程。
PyTorch框架特点及使用方法
特点
PyTorch是一个轻量级的深度学习框架,具有简单易用的特点。它支持动态计算 图,使得模型开发和调试更加灵活。PyTorch还提供了GPU加速和分布式训练功 能,能够提高训练速度。
使用方法
使用PyTorch进行深度学习需要先安装PyTorch库,然后通过编写Python代码来 定义模型、加载数据、进行训练和评估等操作。PyTorch提供了高级的API,如 torch.nn和torch.optim,可以简化模型开发和训练过程。
模型可解释性不足
深度学习模型的可解释性一直是研究 难点。未来需要加强模型可解释性的 研究,以更好地理解模型的工作原理 。
THANKS。
将有更多创新方法被提出。
面临的挑战与解决方案探讨
数据隐私与安全
计算资源需求大
随着深度学习应用的广泛使用,数据 隐私和安全问题日益突出。需要采取 数据脱敏、加密等技术手段来保护用 户隐私。
深度学习模型的训练和推理需要大量 的计算资源,如高性能计算机、GPU 等。需要进一步优化算法和模型结构 ,以降低计算资源需求。
人工智能算法工程师:深度学习 与神经网络算法培训
基于深度学习的图像去雾技术研究
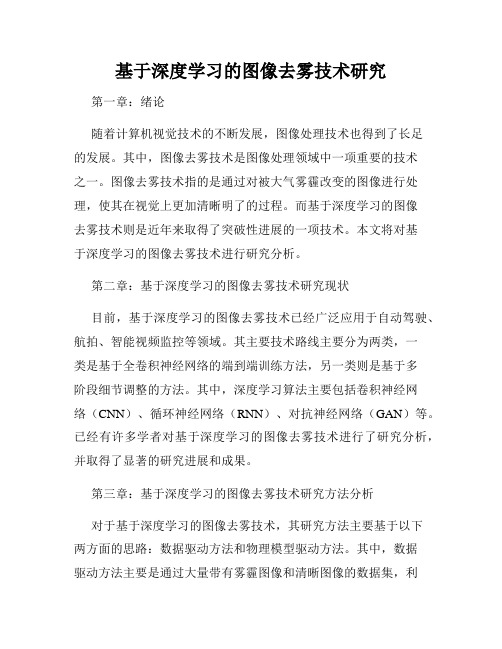
基于深度学习的图像去雾技术研究第一章:绪论随着计算机视觉技术的不断发展,图像处理技术也得到了长足的发展。
其中,图像去雾技术是图像处理领域中一项重要的技术之一。
图像去雾技术指的是通过对被大气雾霾改变的图像进行处理,使其在视觉上更加清晰明了的过程。
而基于深度学习的图像去雾技术则是近年来取得了突破性进展的一项技术。
本文将对基于深度学习的图像去雾技术进行研究分析。
第二章:基于深度学习的图像去雾技术研究现状目前,基于深度学习的图像去雾技术已经广泛应用于自动驾驶、航拍、智能视频监控等领域。
其主要技术路线主要分为两类,一类是基于全卷积神经网络的端到端训练方法,另一类则是基于多阶段细节调整的方法。
其中,深度学习算法主要包括卷积神经网络(CNN)、循环神经网络(RNN)、对抗神经网络(GAN)等。
已经有许多学者对基于深度学习的图像去雾技术进行了研究分析,并取得了显著的研究进展和成果。
第三章:基于深度学习的图像去雾技术研究方法分析对于基于深度学习的图像去雾技术,其研究方法主要基于以下两方面的思路:数据驱动方法和物理模型驱动方法。
其中,数据驱动方法主要是通过大量带有雾霾图像和清晰图像的数据集,利用深度学习算法进行训练,从而生成更高质量的去雾图像。
物理模型驱动方法则是针对不同环境下的雾霾现象,建立相应的物理模型,并应用深度学习算法进行计算求解,生成更加真实的去雾结果。
第四章:基于深度学习的图像去雾技术研究应用案例分析目前,基于深度学习的图像去雾技术已经广泛应用于多个领域。
例如,基于深度学习算法的图像去雾技术可应用于自动驾驶领域,实现更加清晰的行车视角;在航拍领域,通过基于深度学习的图像去雾技术,可以实现更加真实的航拍效果;在智能视频监控领域,应用基于深度学习的图像去雾技术可以提升监控图像清晰度,提高监控效果。
第五章:基于深度学习的图像去雾技术研究存在的问题与展望尽管基于深度学习的图像去雾技术已经取得了较大的进展和成果,但是在实际应用中仍然存在一些问题,例如,算法效率不高、处理时间长等。
基于深度学习的无人机目标检测与跟踪技术研究

基于深度学习的无人机目标检测与跟踪技术研究无人机目标检测与跟踪是无人机领域的一个重要研究方向,它对无人机在各种应用场景中的自主飞行和任务执行能力具有关键性的作用。
随着深度学习技术的逐渐成熟,基于深度学习的无人机目标检测与跟踪技术正在快速发展,并取得了令人瞩目的成果。
无人机目标检测与跟踪技术的研究主要涉及到目标检测和目标跟踪两个方面。
目标检测是指在无人机拍摄的图像或视频中,通过算法自动识别和定位感兴趣的目标物体。
而目标跟踪则是指在目标检测的基础上,通过连续追踪目标物体的位置、大小和形状等特征,实现对目标的持续追踪。
在基于深度学习的无人机目标检测与跟踪技术中,卷积神经网络(Convolutional Neural Network, CNN)是一种常用的深度学习架构。
它具有强大的图像特征提取和分类能力,可以自动学习并提取图像中的高级特征。
对于无人机目标检测任务,研究者们通常采用两阶段检测方法。
首先,利用卷积神经网络提取图像的特征,然后使用目标检测算法进行目标定位和分类。
其中,YOLO(You Only Look Once)和Faster R-CNN(Region-Based Convolutional Neural Networks)等方法被广泛应用于无人机目标检测任务中。
这些方法通过对图像进行密集的特征提取和分类,可以实现快速准确的目标检测。
除了目标检测,无人机目标跟踪也是一个非常重要的研究方向。
针对无人机目标跟踪任务,研究者们提出了许多基于深度学习的跟踪算法。
这些算法通常采用离线训练和在线跟踪两个阶段。
在离线训练阶段,通过大量的有标注数据对模型进行训练,在线跟踪阶段则通过实时获取的图像数据,使用训练好的模型对目标进行跟踪。
其中,Siamese网络和深度学习相关滤波器(DCF)等方法被广泛应用于无人机目标跟踪任务,它们通过对目标和背景之间的关系进行学习,实现对目标的准确跟踪。
基于深度学习的无人机目标检测与跟踪技术在无人机领域具有广阔的应用前景。
基于深度学习的压缩感知技术
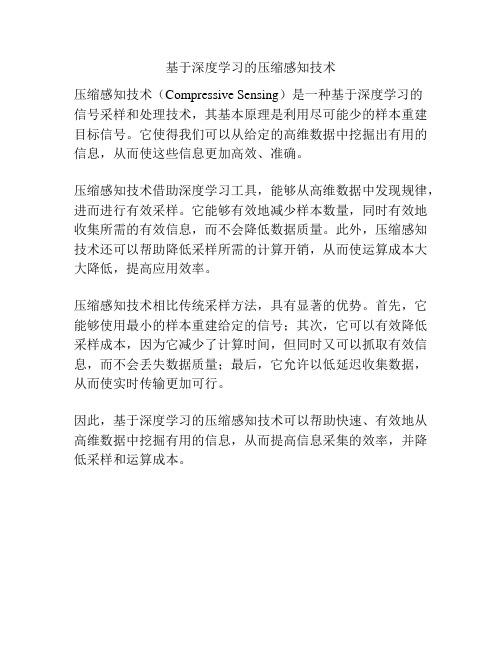
基于深度学习的压缩感知技术
压缩感知技术(Compressive Sensing)是一种基于深度学习的
信号采样和处理技术,其基本原理是利用尽可能少的样本重建目标信号。
它使得我们可以从给定的高维数据中挖掘出有用的信息,从而使这些信息更加高效、准确。
压缩感知技术借助深度学习工具,能够从高维数据中发现规律,进而进行有效采样。
它能够有效地减少样本数量,同时有效地收集所需的有效信息,而不会降低数据质量。
此外,压缩感知技术还可以帮助降低采样所需的计算开销,从而使运算成本大大降低,提高应用效率。
压缩感知技术相比传统采样方法,具有显著的优势。
首先,它能够使用最小的样本重建给定的信号;其次,它可以有效降低采样成本,因为它减少了计算时间,但同时又可以抓取有效信息,而不会丢失数据质量;最后,它允许以低延迟收集数据,从而使实时传输更加可行。
因此,基于深度学习的压缩感知技术可以帮助快速、有效地从高维数据中挖掘有用的信息,从而提高信息采集的效率,并降低采样和运算成本。
人工智能技术在油藏工程领域评价方法

人工智能技术在油藏工程领域评价方法Artificial intelligence (AI) technology has been increasingly used inthe field of reservoir engineering for the evaluation of oil and gas reservoirs. It has provided great potential in improving the efficiency and accuracy of reservoir evaluation, leading to significant advancements in the industry.人工智能(AI)技术在油藏工程领域的应用日益增多,用于油气储层的评价。
它在提高储层评价的效率和准确性方面具有巨大潜力,促进了该行业的显著进步。
One major aspect of AI in reservoir engineering is its capability to analyze vast amounts of data. AI algorithms can process and interpret geological, geophysical, and engineering data, allowing for a more comprehensive understanding of reservoir characteristics and behavior.人工智能在油藏工程中的一个主要方面是其分析海量数据的能力。
AI算法可以处理和解释地质、地球物理和工程数据,使人们更全面地了解储层的特征和行为。
Additionally, AI can be utilized for predictive modeling of reservoir performance. By using machine learning and data-driven techniques, AI can forecast production rates, reservoir pressure, and other key parameters with improved accuracy, aiding in the optimization of production strategies and reservoir management.此外,人工智能还可以用于油藏性能的预测建模。
基于深度学习的图像去雾算法研究与实现
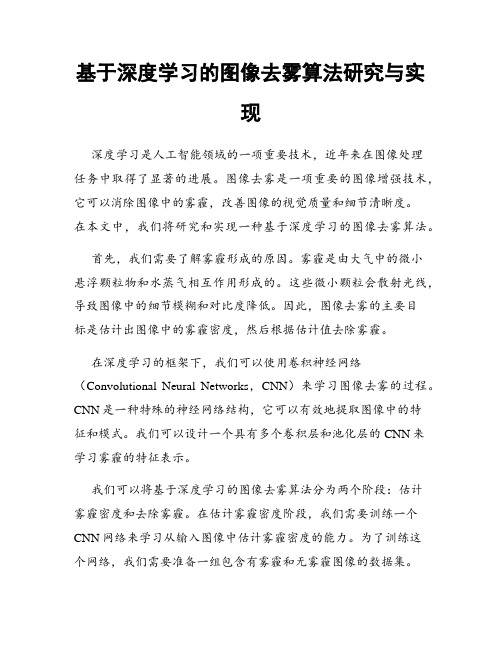
基于深度学习的图像去雾算法研究与实现深度学习是人工智能领域的一项重要技术,近年来在图像处理任务中取得了显著的进展。
图像去雾是一项重要的图像增强技术,它可以消除图像中的雾霾,改善图像的视觉质量和细节清晰度。
在本文中,我们将研究和实现一种基于深度学习的图像去雾算法。
首先,我们需要了解雾霾形成的原因。
雾霾是由大气中的微小悬浮颗粒物和水蒸气相互作用形成的。
这些微小颗粒会散射光线,导致图像中的细节模糊和对比度降低。
因此,图像去雾的主要目标是估计出图像中的雾霾密度,然后根据估计值去除雾霾。
在深度学习的框架下,我们可以使用卷积神经网络(Convolutional Neural Networks,CNN)来学习图像去雾的过程。
CNN是一种特殊的神经网络结构,它可以有效地提取图像中的特征和模式。
我们可以设计一个具有多个卷积层和池化层的CNN来学习雾霾的特征表示。
我们可以将基于深度学习的图像去雾算法分为两个阶段:估计雾霾密度和去除雾霾。
在估计雾霾密度阶段,我们需要训练一个CNN网络来学习从输入图像中估计雾霾密度的能力。
为了训练这个网络,我们需要准备一组包含有雾霾和无雾霾图像的数据集。
我们可以通过在有雾霾的环境中拍摄照片并与无雾霾照片进行配对来构建这个数据集。
然后,我们可以使用这个数据集来训练CNN网络,以学习从输入图像中估计雾霾密度的能力。
在去除雾霾阶段,我们可以使用估计的雾霾密度来去除图像中的雾霾。
我们可以设计一个去雾网络,它接收输入图像和估计的雾霾密度,并输出去除雾霾后的图像。
这个网络可以由多个卷积层和反卷积层组成,以学习如何从输入图像中恢复原始图像的能力。
在实际的算法实现中,我们可以使用深度学习框架如TensorFlow或PyTorch来构建和训练这些CNN网络。
我们可以使用预训练的模型来加速训练过程,并且可以使用GPU来加快计算速度。
此外,我们还可以对数据集进行数据增强来提高模型的鲁棒性,例如随机旋转、缩放和裁剪图像。
基于深度学习的目标检测技术研究(英文中文双语版优质文档)
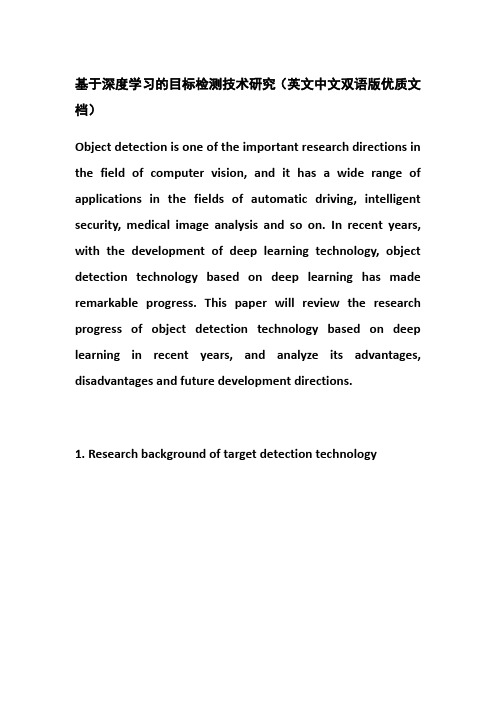
基于深度学习的目标检测技术研究(英文中文双语版优质文档)Object detection is one of the important research directions in the field of computer vision, and it has a wide range of applications in the fields of automatic driving, intelligent security, medical image analysis and so on. In recent years, with the development of deep learning technology, object detection technology based on deep learning has made remarkable progress. This paper will review the research progress of object detection technology based on deep learning in recent years, and analyze its advantages, disadvantages and future development directions.1. Research background of target detection technologyObject detection is one of the important research directions in the field of computer vision. Its main task is to detect the location, size and category of objects in images or videos. Object detection technology is widely used in autonomous driving, intelligent security, medical image analysis and other fields.In traditional target detection techniques, commonly used methods include methods based on feature extraction and traditional machine learning algorithms, such as Haar features and HOG features, and methods based on background modeling, such as ViBe and MoG. However, there are many problems in these traditional methods, such as the feature is not learnable, the robustness is poor, and it is limited by the background model.With the development of deep learning technology, object detection technology based on deep learning has become a research hotspot. These techniques perform end-to-end training on images by using deep neural networks, without the need to manually design features, and thus have better learnability and robustness.2. Overview of target detection technology based on deep learningAt present, object detection techniques based on deep learning are mainly divided into two categories: two-stage detection and one-stage detection. Among them, the two-stage detection method first generates a series of candidate boxes through a Region Proposal Network (RPN), and then classifies and regresses these candidate boxes to obtain the final target detection result. The one-stage detection method directly classifies and regresses the entire image to obtain the target detection result.1. Two-stage detection method(1) Faster R-CNNFaster R-CNN is a typical two-stage object detection method, which proposes an RPN to generate candidate boxes, and classifies and regresses the candidate boxes through the R-CNN network. RPN network is a sliding window framework based on convolutional neural network, which can extract multiple regions that may contain targets in the image, and classify and regress these regions to generate candidate boxes. Then, these candidate boxes are input into the R-CNN network, and they are classified and regressed to obtain the final target detection result.Compared with traditional target detection methods, Faster R-CNN has greatly improved its accuracy and speed. However, there are two problems: one is that the candidate frame generated by RPN requires a lot of calculation, resulting in a slow calculation speed; the other is that the network needs to perform two forward propagations, resulting in a large amount of calculation.(2) Mask R-CNNMask R-CNN is an extension of Faster R-CNN. It adds a segmentation branch to Faster R-CNN, which can simultaneously complete target detection and pixel-level semantic segmentation. Based on Faster R-CNN, Mask R-CNN adds a fully convolutional network to generate target masks to achieve pixel-level semantic segmentation. Mask R-CNN has achieved excellent results on multiple datasets, proving its effectiveness on object detection and semantic segmentation tasks.(3) Cascade R-CNNCascade R-CNN is improved on the basis of Faster R-CNN, and its idea is to perform cascaded classification and regression on candidate frames. Cascade R-CNN improves the detection accuracy by cascading multiple R-CNN networks, and each R-CNN network performs stricter screening of samples that were misclassified by the previous network. Cascade R-CNN achieves state-of-the-art performance on multiple datasets, proving its effectiveness in the field of object detection.2. One-stage detection method(1) YOLO seriesYOLO (You Only Look Once) is a typical one-stage target detection method. YOLO obtains target detection results by classifying and regressing the entire image. YOLO is characterized by being fast and simple, and can be used in real-time scenarios. The YOLO series has now been developed to the fourth edition, and its detection speed and accuracy have been greatly improved. However, there are also some problems in the YOLO series, such as poor detection of small targets.(2) SSD seriesSSD (Single Shot MultiBox Detector) is another typical one-stage target detection method. Unlike YOLO, SSD uses multi-scale feature maps to detect targets, thereby improving the detection effect on small targets. The SSD series has also experienced multiple versions of development, and its detection speed and accuracy have been greatly improved. However, compared with YOLO, the detection speed of SSD is relatively slow, and there are also problems such as poor detection effect on objects with high aspect ratio.(3) RetinaNetRetinaNet is a one-stage target detection method based on Focal Loss. RetinaNet improves the detection effect of small targets by improving the loss function to pay more attention to positive and negative samples that are difficult to distinguish. RetinaNet has achieved excellent results on multiple datasets, proving its effectiveness in the field of object detection.(4) EfficientDetEfficientDet is a one-stage object detection method based on EfficientNet. EfficientDet builds a series of efficient network structures by using different expansion coefficients and depth and width scaling factors, thus achieving a good balance between detection speed and accuracy. EfficientDet achieves state-of-the-art performance on multiple datasets, proving its effectiveness in the field of object detection.In general, the one-stage object detection method has faster detection speed than the two-stage method, but the detection effect on small objects and high aspect ratio objects is relatively poor. Different methods have their own advantages and disadvantages, and the appropriate method needs to be selected according to the specific application scenario.目标检测是计算机视觉领域的重要研究方向之一,其在自动驾驶、智能安防、医学图像分析等领域都有广泛的应用。
基于深度学习的目标检测技术研究
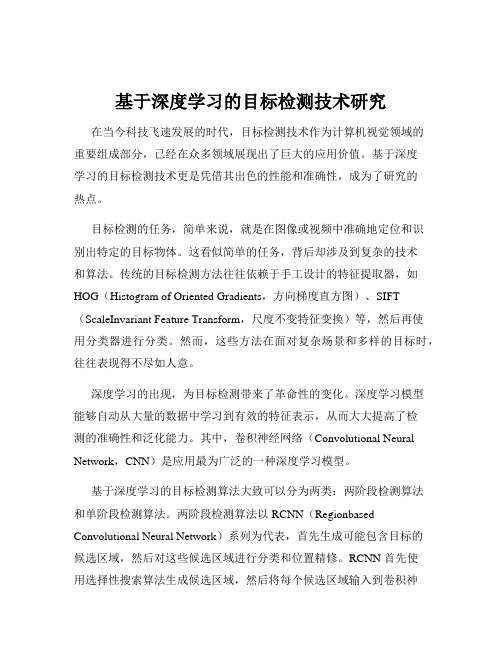
基于深度学习的目标检测技术研究在当今科技飞速发展的时代,目标检测技术作为计算机视觉领域的重要组成部分,已经在众多领域展现出了巨大的应用价值。
基于深度学习的目标检测技术更是凭借其出色的性能和准确性,成为了研究的热点。
目标检测的任务,简单来说,就是在图像或视频中准确地定位和识别出特定的目标物体。
这看似简单的任务,背后却涉及到复杂的技术和算法。
传统的目标检测方法往往依赖于手工设计的特征提取器,如HOG(Histogram of Oriented Gradients,方向梯度直方图)、SIFT (ScaleInvariant Feature Transform,尺度不变特征变换)等,然后再使用分类器进行分类。
然而,这些方法在面对复杂场景和多样的目标时,往往表现得不尽如人意。
深度学习的出现,为目标检测带来了革命性的变化。
深度学习模型能够自动从大量的数据中学习到有效的特征表示,从而大大提高了检测的准确性和泛化能力。
其中,卷积神经网络(Convolutional Neural Network,CNN)是应用最为广泛的一种深度学习模型。
基于深度学习的目标检测算法大致可以分为两类:两阶段检测算法和单阶段检测算法。
两阶段检测算法以 RCNN(Regionbased Convolutional Neural Network)系列为代表,首先生成可能包含目标的候选区域,然后对这些候选区域进行分类和位置精修。
RCNN 首先使用选择性搜索算法生成候选区域,然后将每个候选区域输入到卷积神经网络中提取特征,最后使用支持向量机进行分类。
虽然 RCNN 取得了比传统方法更好的效果,但其计算量非常大,检测速度很慢。
为了解决 RCNN 速度慢的问题,Fast RCNN 应运而生。
Fast RCNN不再对每个候选区域单独提取特征,而是将整个图像输入到卷积神经网络中,得到共享的特征图,然后通过感兴趣区域池化(Region of Interest Pooling,RoI Pooling)从特征图中提取每个候选区域的特征。
基于深度学习的无人机目标检测研究
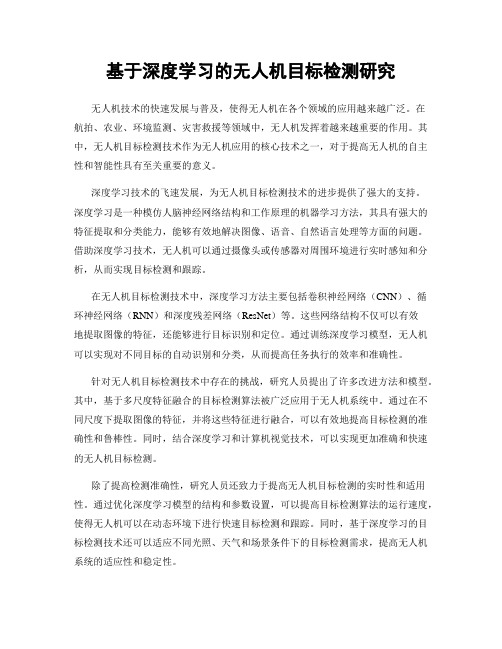
基于深度学习的无人机目标检测研究无人机技术的快速发展与普及,使得无人机在各个领域的应用越来越广泛。
在航拍、农业、环境监测、灾害救援等领域中,无人机发挥着越来越重要的作用。
其中,无人机目标检测技术作为无人机应用的核心技术之一,对于提高无人机的自主性和智能性具有至关重要的意义。
深度学习技术的飞速发展,为无人机目标检测技术的进步提供了强大的支持。
深度学习是一种模仿人脑神经网络结构和工作原理的机器学习方法,其具有强大的特征提取和分类能力,能够有效地解决图像、语音、自然语言处理等方面的问题。
借助深度学习技术,无人机可以通过摄像头或传感器对周围环境进行实时感知和分析,从而实现目标检测和跟踪。
在无人机目标检测技术中,深度学习方法主要包括卷积神经网络(CNN)、循环神经网络(RNN)和深度残差网络(ResNet)等。
这些网络结构不仅可以有效地提取图像的特征,还能够进行目标识别和定位。
通过训练深度学习模型,无人机可以实现对不同目标的自动识别和分类,从而提高任务执行的效率和准确性。
针对无人机目标检测技术中存在的挑战,研究人员提出了许多改进方法和模型。
其中,基于多尺度特征融合的目标检测算法被广泛应用于无人机系统中。
通过在不同尺度下提取图像的特征,并将这些特征进行融合,可以有效地提高目标检测的准确性和鲁棒性。
同时,结合深度学习和计算机视觉技术,可以实现更加准确和快速的无人机目标检测。
除了提高检测准确性,研究人员还致力于提高无人机目标检测的实时性和适用性。
通过优化深度学习模型的结构和参数设置,可以提高目标检测算法的运行速度,使得无人机可以在动态环境下进行快速目标检测和跟踪。
同时,基于深度学习的目标检测技术还可以适应不同光照、天气和场景条件下的目标检测需求,提高无人机系统的适应性和稳定性。
在无人机目标检测技术的研究中,还有许多待解决的问题和挑战。
例如,如何克服目标遮挡、变形和多目标重叠等复杂场景下的目标检测问题;如何提高目标检测的鲁棒性和抗干扰能力;如何实现多无人机之间的协同目标检测等。
基于深度学习的无人机目标识别与跟踪系统设计
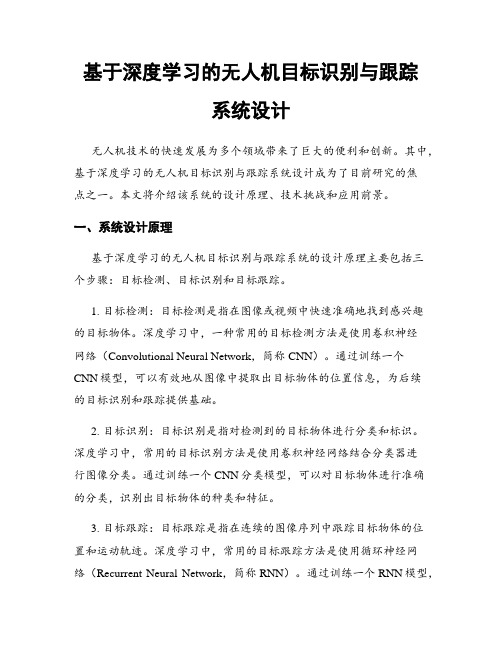
基于深度学习的无人机目标识别与跟踪系统设计无人机技术的快速发展为多个领域带来了巨大的便利和创新。
其中,基于深度学习的无人机目标识别与跟踪系统设计成为了目前研究的焦点之一。
本文将介绍该系统的设计原理、技术挑战和应用前景。
一、系统设计原理基于深度学习的无人机目标识别与跟踪系统的设计原理主要包括三个步骤:目标检测、目标识别和目标跟踪。
1. 目标检测:目标检测是指在图像或视频中快速准确地找到感兴趣的目标物体。
深度学习中,一种常用的目标检测方法是使用卷积神经网络(Convolutional Neural Network,简称CNN)。
通过训练一个CNN模型,可以有效地从图像中提取出目标物体的位置信息,为后续的目标识别和跟踪提供基础。
2. 目标识别:目标识别是指对检测到的目标物体进行分类和标识。
深度学习中,常用的目标识别方法是使用卷积神经网络结合分类器进行图像分类。
通过训练一个CNN分类模型,可以对目标物体进行准确的分类,识别出目标物体的种类和特征。
3. 目标跟踪:目标跟踪是指在连续的图像序列中跟踪目标物体的位置和运动轨迹。
深度学习中,常用的目标跟踪方法是使用循环神经网络(Recurrent Neural Network,简称RNN)。
通过训练一个RNN模型,可以根据目标物体的当前位置和历史运动信息,预测目标物体在下一帧图像中的位置,实现对目标物体的稳定跟踪。
二、技术挑战设计基于深度学习的无人机目标识别与跟踪系统面临着一些技术挑战。
以下是几个重要的挑战:1. 实时性要求:基于深度学习的目标识别与跟踪系统需要在实时视频流中进行目标检测、识别和跟踪处理。
而深度学习算法通常需要较长的处理时间,因此如何在保证准确性的同时提高算法的运行速度,是一个重要的技术挑战。
2. 复杂环境下的鲁棒性:无人机进行目标识别和跟踪时,常常面临复杂的环境条件,如天气变化、光照变化、目标物体遮挡等。
如何提高系统的鲁棒性,实现对目标物体的稳定识别与跟踪,是一个具有挑战性的问题。
基于深度强化学习的自主无人机导航系统设计
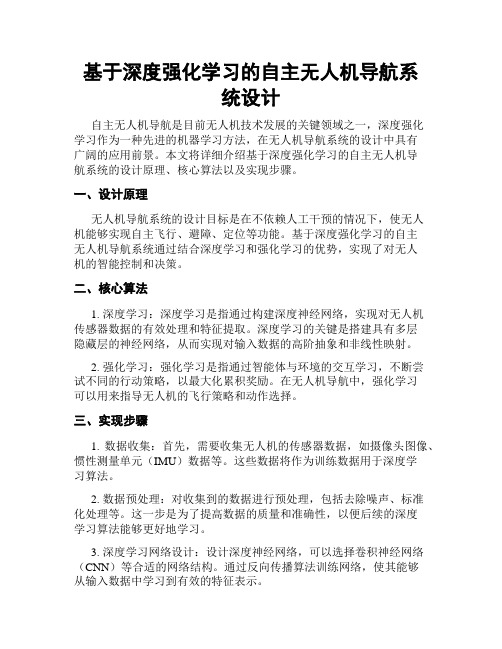
基于深度强化学习的自主无人机导航系统设计自主无人机导航是目前无人机技术发展的关键领域之一,深度强化学习作为一种先进的机器学习方法,在无人机导航系统的设计中具有广阔的应用前景。
本文将详细介绍基于深度强化学习的自主无人机导航系统的设计原理、核心算法以及实现步骤。
一、设计原理无人机导航系统的设计目标是在不依赖人工干预的情况下,使无人机能够实现自主飞行、避障、定位等功能。
基于深度强化学习的自主无人机导航系统通过结合深度学习和强化学习的优势,实现了对无人机的智能控制和决策。
二、核心算法1. 深度学习:深度学习是指通过构建深度神经网络,实现对无人机传感器数据的有效处理和特征提取。
深度学习的关键是搭建具有多层隐藏层的神经网络,从而实现对输入数据的高阶抽象和非线性映射。
2. 强化学习:强化学习是指通过智能体与环境的交互学习,不断尝试不同的行动策略,以最大化累积奖励。
在无人机导航中,强化学习可以用来指导无人机的飞行策略和动作选择。
三、实现步骤1. 数据收集:首先,需要收集无人机的传感器数据,如摄像头图像、惯性测量单元(IMU)数据等。
这些数据将作为训练数据用于深度学习算法。
2. 数据预处理:对收集到的数据进行预处理,包括去除噪声、标准化处理等。
这一步是为了提高数据的质量和准确性,以便后续的深度学习算法能够更好地学习。
3. 深度学习网络设计:设计深度神经网络,可以选择卷积神经网络(CNN)等合适的网络结构。
通过反向传播算法训练网络,使其能够从输入数据中学习到有效的特征表示。
4. 强化学习算法:将深度学习网络与强化学习算法相结合,构建强化学习平台。
在每个时间步骤上,根据当前状态选择动作,同时根据奖励信号更新网络的参数,以提高飞行策略和性能。
5. 离线训练:使用收集到的数据进行离线训练,优化网络的参数和策略。
通过多次迭代训练,使得网络能够快速学习到合适的导航策略。
6. 在线测试:将训练好的无人机导航系统进行在线测试,验证其在实际环境中的性能。
基于深度学习的无人机视觉感知与控制技术研究

基于深度学习的无人机视觉感知与控制技术研究随着无人机技术的飞速发展,无人机已经成为多个领域重要的工具。
然而,由于无人机的自主控制能力较弱,局限了其在一些复杂环境下的应用。
因此,深度学习技术成为了无人机视觉感知和控制技术研究的热点之一。
一、深度学习技术的应用深度学习是一种基于神经网络的人工智能技术。
它通过层层处理,将数据转换为高层次的抽象特征,并以此实现对模式识别、目标检测、语音识别等领域的优异表现。
近年来,深度学习技术已经被广泛应用于计算机视觉、语音识别、自然语言处理等领域。
对于无人机来说,深度学习技术可以用于视觉感知和控制中。
达到了增强无人机自主控制能力的目的。
二、无人机视觉感知在无人机的视觉感知中,深度学习技术主要用于目标检测、目标识别和场景理解。
目标检测是指在图像中检测出目标物体的存在,并在图像中标注出目标的位置和边界框。
常见的目标检测算法有RCNN、YOLO和SSD等。
这些算法通过深度卷积神经网络对图像进行分析和处理,能够有效地检测出目标物体,提高了目标检测的准确率和速度。
利用目标检测技术和无人机结合,可以实现无人机在空中对物体的自动巡航、照相等操作。
目标识别是指在检测出目标物体后,进一步对该物体进行识别和分类。
目标识别的常见算法有Fast R-CNN、Faster R-CNN和YOLOv2。
它们都是在目标检测的基础上,通过深度学习算法实现对目标物体的识别和分类。
例如,可以通过识别监测区域内的有毒植物,让无人机针对性地喷洒相应的药剂,实现农业生产的增产和优质。
场景理解是指在图像分析的过程中,理解图像中物体的空间分布关系和关键特征,从而对图像进行分类和识别。
近年来,语义分割和实例分割等场景理解技术受到了重视,它们可以将图像中的每一个像素都进行分类,以达到更加精细的图像分析和识别。
这些技术也可以被应用在无人机的航拍和地质勘探等领域中。
三、无人机控制无人机控制分为几个环节,包括目标跟踪、轨迹规划和避障等。
基于物理先验和对比学习的图像去雾算法
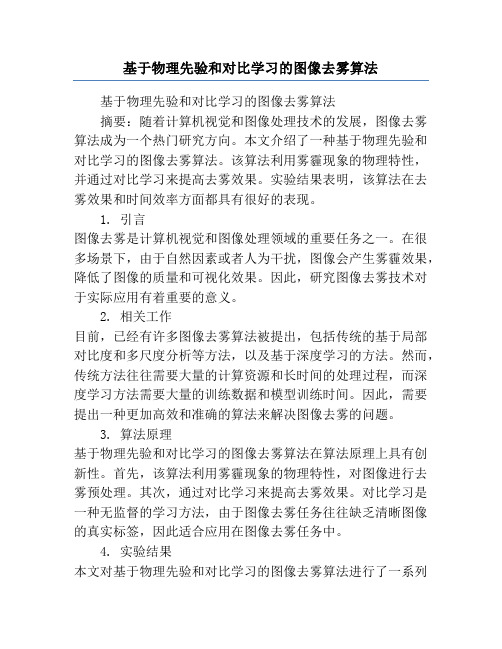
基于物理先验和对比学习的图像去雾算法基于物理先验和对比学习的图像去雾算法摘要:随着计算机视觉和图像处理技术的发展,图像去雾算法成为一个热门研究方向。
本文介绍了一种基于物理先验和对比学习的图像去雾算法。
该算法利用雾霾现象的物理特性,并通过对比学习来提高去雾效果。
实验结果表明,该算法在去雾效果和时间效率方面都具有很好的表现。
1. 引言图像去雾是计算机视觉和图像处理领域的重要任务之一。
在很多场景下,由于自然因素或者人为干扰,图像会产生雾霾效果,降低了图像的质量和可视化效果。
因此,研究图像去雾技术对于实际应用有着重要的意义。
2. 相关工作目前,已经有许多图像去雾算法被提出,包括传统的基于局部对比度和多尺度分析等方法,以及基于深度学习的方法。
然而,传统方法往往需要大量的计算资源和长时间的处理过程,而深度学习方法需要大量的训练数据和模型训练时间。
因此,需要提出一种更加高效和准确的算法来解决图像去雾的问题。
3. 算法原理基于物理先验和对比学习的图像去雾算法在算法原理上具有创新性。
首先,该算法利用雾霾现象的物理特性,对图像进行去雾预处理。
其次,通过对比学习来提高去雾效果。
对比学习是一种无监督的学习方法,由于图像去雾任务往往缺乏清晰图像的真实标签,因此适合应用在图像去雾任务中。
4. 实验结果本文对基于物理先验和对比学习的图像去雾算法进行了一系列实验。
实验使用了多个数据集,比较了该算法与传统方法和深度学习方法的去雾效果和时间效率。
实验结果表明,基于物理先验和对比学习的图像去雾算法在去雾效果和时间效率方面都具有很好的表现。
5. 结论和展望本文提出了一种基于物理先验和对比学习的图像去雾算法,并进行了实验验证。
实验结果表明,该算法在去雾效果和时间效率方面具有很好的表现。
未来,可以进一步研究算法的优化,提高去雾效果和时间效率。
同时,可以将该算法应用于实际场景,例如无人驾驶、监控等领域,来验证其实用性和性能。
总之,基于物理先验和对比学习的图像去雾算法在图像处理领域具有重要的应用价值。
思维导图英语范文

思维导图英语范文1Oh my goodness! Have you ever heard of mind maps? Well, let me tell you, a mind map is an amazing tool that can truly transform the way we learn and think! It's like a magical map that helps us organize and connect our thoughts.A mind map is basically a visual representation of information. It shows the relationships between different ideas and concepts. So, what are its uses? For starters, when it comes to learning English vocabulary, we can create a mind map to group words by topics like animals, food, or emotions. This makes it so much easier to remember and recall! And when dealing with grammar, a mind map can clearly show the rules and examples, helping us understand better.Imagine preparing for an English speech or writing an essay. A mind map can be our best friend! We can start with the main idea in the center and branch out with supporting points and details. How wonderful is that? It helps us organize our thoughts logically and ensures we don't miss anything important.In conclusion, mind maps are incredibly important! They make our learning more efficient and our thinking more clear. So, why not give them a try and see the amazing results for yourself?Oh my goodness! Let me tell you about the amazing effects of mind maps in English learning. It's truly a revolutionary tool! I used to struggle with grammar and vocabulary, constantly feeling lost and confused. But then, I discovered the power of mind maps. I started organizing all the grammar rules and vocabulary words systematically on a mind map. It was like a light shining in the darkness! I could see the connections and patterns clearly.For example, my classmate Tom was also having a hard time with English composition. But after he began to use mind maps to plan his ideas and structure his essays, his writing skills improved dramatically. He went from getting average grades to being one of the top students in our class! Isn't that incredible?Another time, before an important English competition, I used a mind map to review all the key points. I included grammar, vocabulary, reading comprehension strategies, and even common mistakes to avoid. And guess what? I performed exceptionally well and won a prize. How wonderful it was!So, if you haven't tried using mind maps for English learning, you should definitely give it a go. It might just change your English learning journey forever!Oh my goodness! Let me tell you about how to create an effective mind map for English learning. Firstly, you must determine the theme. For instance, if you choose a certain English unit as the theme, like 'My School Life', then you can start to branch it out. The branches could be vocabulary, sentence patterns, grammar and so on. V ocabulary could include words like 'classroom', 'teacher', 'student'. Sentence patterns might be 'There is a blackboard in the classroom.' or 'The teacher is very kind.' And grammar, well, it could be about tenses or noun plurals.Also, don't forget to use different colors and graphics to enhance the appeal and memory effect of your mind map! Imagine using bright colors for important points and interesting graphics to represent different parts. For example, a big red star for key grammar rules or a cute apple for vocabulary related to fruits.This way, your mind map will not only be a useful tool for learning but also a beautiful and attractive creation that makes you want to look at it again and again. Isn't that wonderful? So, go ahead and create your amazing mind map for English learning!4Oh my goodness! When it comes to learning English, the differences between mind mapping and traditional learning methods are trulyremarkable. Let's take memorizing words as an example. With traditional methods, we often repeat the words over and over again, but it's so boring and ineffective! How many times have we forgotten them soon after? However, with mind mapping, we can link the words with vivid images, related phrases, and even personal experiences. It's like building a colorful and interesting world in our minds! Isn't that amazing?When it comes to understanding articles, the contrast is also striking. Traditional methods might have us mechanically analyzing sentence structures and grammar rules. But with mind mapping, we can visually represent the main ideas, supporting details, and logical connections. It helps us see the big picture clearly and understand the context better. How wonderful is that?For instance, we can combine mind mapping with flashcards. When we create a mind map of a topic, we can attach related flashcards for quick review. This combination boosts our learning efficiency and makes the learning process more enjoyable. So, why not give mind mapping a try and revolutionize our English learning journey?5Oh, dear friends! Let's think about the future of mind mapping in English education. It's truly an exciting topic!In the era of rapid technological progress, mind mapping could potentially merge with artificial intelligence. Imagine this! AI-poweredmind mapping could analyze each student's learning patterns and preferences, and then offer highly personalized English learning plans. For example, it might tailor exercises based on a student's weaknesses in grammar or vocabulary. How amazing would that be?In the context of globalization, mind mapping plays a crucial role too. Different countries have diverse cultures and educational systems, but mind mapping provides a universal tool. It helps students organize their thoughts and knowledge systematically. For instance, a student from a non-English speaking country can use mind mapping to compare and contrast the grammar structures of their native language and English. Doesn't this make the learning process more clear and effective?So, it's evident that mind mapping has a bright future in English education. It will surely continue to evolve and bring more innovative and effective ways for students to master this global language. Won't it?。
深度学习在遥感图像变化检测中的革新之路
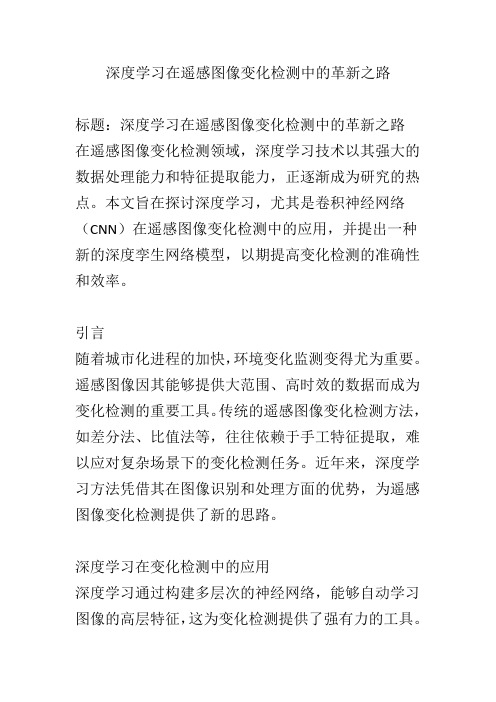
深度学习在遥感图像变化检测中的革新之路标题:深度学习在遥感图像变化检测中的革新之路在遥感图像变化检测领域,深度学习技术以其强大的数据处理能力和特征提取能力,正逐渐成为研究的热点。
本文旨在探讨深度学习,尤其是卷积神经网络(CNN)在遥感图像变化检测中的应用,并提出一种新的深度孪生网络模型,以期提高变化检测的准确性和效率。
引言随着城市化进程的加快,环境变化监测变得尤为重要。
遥感图像因其能够提供大范围、高时效的数据而成为变化检测的重要工具。
传统的遥感图像变化检测方法,如差分法、比值法等,往往依赖于手工特征提取,难以应对复杂场景下的变化检测任务。
近年来,深度学习方法凭借其在图像识别和处理方面的优势,为遥感图像变化检测提供了新的思路。
深度学习在变化检测中的应用深度学习通过构建多层次的神经网络,能够自动学习图像的高层特征,这为变化检测提供了强有力的工具。
特别是卷积神经网络(CNN),它通过卷积层、池化层和全连接层的堆叠,能够提取出图像的丰富特征,从而有效地识别出变化区域。
孪生网络模型的提出针对遥感图像变化检测的特殊性,我们提出了一种基于深度孪生网络的变化检测模型。
该模型通过构建两个并行的子网络,分别处理同一地区不同时间点的图像,并通过比较这两个子网络的输出来识别变化区域。
这种结构不仅能够提取图像的深层特征,还能够利用图像之间的时间关联性,从而提高变化检测的准确性。
条件随机场的融合为了进一步提升模型的性能,我们将条件随机场(CRF)模型融入到深度孪生网络中。
CRF模型能够考虑像素之间的空间关系,通过概率图模型来优化变化检测的结果,使得变化区域的边界更加清晰,减少了噪声的干扰。
实验验证通过在多个数据集上的实验验证,我们的模型在变化检测的准确性上取得了显著的提升。
与现有的变化检测方法相比,我们的模型不仅在检测速度上有优势,而且在检测精度上也有较大的提高。
讨论尽管深度学习方法在遥感图像变化检测中展现出了巨大的潜力,但仍存在一些挑战。
- 1、下载文档前请自行甄别文档内容的完整性,平台不提供额外的编辑、内容补充、找答案等附加服务。
- 2、"仅部分预览"的文档,不可在线预览部分如存在完整性等问题,可反馈申请退款(可完整预览的文档不适用该条件!)。
- 3、如文档侵犯您的权益,请联系客服反馈,我们会尽快为您处理(人工客服工作时间:9:00-18:30)。
This paper presents a set of methods by which a learning agent can learn a sequence of increasingly abstract and powerful interfaces to control a robot whose sensorimotor apparatus and environment are initially unknown. The result of the learning is a rich hierarchical model of the robot's world (its sensorimotor apparatus and environment). The learning methods rely on generic properties of the robot's world such as almosteverywhere smooth e ects of motor control signals on sensory features. At the lowest level of the hierarchy, the learning agent analyzes the e ects of its motor control signals in order to de ne a new set of control signals, one for each of the robot's degrees of freedom. It uses a generate-and-test approach to de ne sensory features that capture important aspects of the environment. It uses linear regression to learn models that characterize context-dependent e ects of the control signals on the learned features. It uses these models to de ne high-level control laws for nding and following paths de ned using constraints on the learned features. The agent abstracts these control laws, which interact with the continuous environment, to a nite set of actions that implement discrete state transitions. At this point, the agent has abstracted the robot's continuous world to a nite-state world and can use existing methods to learn its structure. The learning agent's methods are evaluated on several simulated robots with di erent sensorimotor systems and environments.
1
Journal Paper Draft
February 25, 1996
2
1 duction
Suppose a creature emerges into an unknown environment, with no knowledge of what its sensors are sensing or what its e ectors are e ecting. How can such a creature learn enough about its sensors and e ectors to learn about the nature of its environment? What primitive capabilities are su cient to support such a learning process? This problem is idealized to clarify the goals and results of our research. A real robot embodies knowledge designed and programmed in by engineers who select sensors and effectors appropriate to the environment, and implement control laws appropriate to the goals of the robot. A real biological organism embodies knowledge, acquired through evolution, that matches the sensorimotor capabilities of the organism to the demands of the environment. We idealize both of these to the problem faced by an individual learning agent with very little domain-speci c knowledge, but with the ability to apply a number of sophisticated, domain-independent learning methods. In addition to its scienti c value, this idealized learning agent would be of considerable practical value in allowing a newly-designed robot to learn the properties of its own sensorimotor system. We report here on one learning agent that solves a speci c instance of this problem, along with several variations that begin to explore the range of possible solutions to the general problem. Henceforth, we make a distinction between the learning agent and the robot. The robot is a machine (physical or simulated) that the learning agent must learn how to use. The robot's sensorimotor apparatus is comprised of a set of sensors and e ectors. The sensorimotor apparatus is uninterpreted , meaning that the agent that is learning how to use the robot has no a priori knowledge of the meaning of the sensors, of the structure of the sensory system, or of the e ects of the motor's control signals. From the learning agent's perspective, the sensorimotor apparatus is represented as a raw sense vector s and a raw motor control vector u. The former is a vector of real numbers giving the current values of all of the sensors. The latter is a vector of real numbers, called control signals, produced by the learning agent and sent to the robot's motor apparatus. The learning agent's situation is illustrated in Figure 1. This paper solves the learning problem by presenting a set of methods that the learning agent can use to learn (1) a model of the robot's set of sensors, (2) a model of the robot's motor apparatus, and (3) a set of behaviors that allow the learning agent to abstract the robot's continuous world to a discrete world of places and paths. These methods have been demonstrated on a simulated mobile robot with a ring of distance sensors. These learning methods comprise a body of knowledge that is given to the learning agent a priori . They incorporate a knowledge of basic mathematics, multivariate analysis, and control theory. The learning methods are domain independent in that they are not based on a particular set of sensors or e ectors and do not make assumptions about the structure or even the dimensionality of the robot's environment. In the rest of this paper, we describe a number of learning methods and show how they