Wavelet Cross-Correlation Analysis of Turbulent Mixing from Large-Eddy-Simulations
质子交换膜燃料电池系统远程状态监控和故障诊断综述

FRONTIER DISCUSSION | 前沿探讨1 引言传统能源为全球带来了巨大便利的同时也带来了相应的负面影响,氢能作为一种清洁、高效的新型能源,受到了全世界关注。
PEMFC系统故障会引起系统性能衰减甚至缩短其寿命,为了检测和隔离故障,PEMFC系统的在线故障诊断成为了亟待解决的问题。
2 PEMFC系统及故障分析2.1 PEMFC系统构成PEMFC系统是一种电化学能量转换装置,可以将氢气和氧气的化学能转化为电能,反应产物为水[1]。
PEMFC系统一般由燃料电池模块、氢气供给系统、空气供给系统、热管理系统和控制系统等组成,系统结构框图如图1。
燃料电池模块中燃料电池堆由多节单体串联而成,是进行电化学反应的场所;氢气供给系统的功能是为燃料电池堆阳极提供氢气,并保证足够的流量和压力;空气供给系统的功能是为燃料电池堆的阴极提供空气,并保证足够的流量和压力以及适宜的湿度;热管理系统的功能主要是调节燃料电池堆的温度,使其维持在合适的范围内[2]。
质子交换膜燃料电池系统远程状态监控和故障诊断综述赵越 张国庆 苗盼盼潍柴巴拉德氢能科技有限公司 山东省潍坊市 261061摘 要:质子交换膜燃料电池(PEMFC)由于其零排放、可再生和高效率等优势,被认为是下一代能源的最佳选择,但该系统目前还存在可靠性差、寿命短等多种问题急需解决。
本文在分析PEMFC系统构成、故障特性、故障分类和故障机理的基础上,对PEMFC系统远程状态监控研究进展进行分析,分别介绍了两种远程监控架构。
又对PEMFC系统故障诊断研究进展进行综述,探讨基于当前研究进展更适合PEMFC在线故障诊断的方法为基于数据驱动诊断方法。
最后提出了PEMFC系统在远程状态监控和在线故障诊断的发展趋势及展望,期望为后续研究及商业化应用提供参考。
关键词:质子交换膜燃料电池 远程状态监控 故障诊断 数据驱动A Review on Remote Condition Monitoring and Fault Diagnosis for Proton Exchange Membrane Fuel Cell SystemsZhao Yue Zhang Guoqing Miao PanpanAbstract: P roton exchange membrane fuel cell (PEMFC) is considered as the best choice for the next generation of energy due to its advantages of zero emission, renewable energy and high effi ciency. but there ar e still bottlenecks such as poor reliability and short life. Based on analyzing the composition, fault characteristics, fault classifi cation and failure mechanism of PEMFC systems. this paper analyzes the research progress of remote condition monitoring of PEMFC systems, and introduces two architectures of remote condition monitoring .This paper summarizes the research progress of fault diagnosis in PEMFC systems, discusses the data-driven diagnosis method which is more suitable for online fault diagnosis of PEMFC systems. Finally, the development trend and prospect of PEMFC system in remote condition monitoring, online fault diagnosis are proposed, hoping to provide reference for the follow-up research and commercial application.Key words:Proton Exchange Membrane Fuel Cell; Remote Condition Monitoring; Fault Diagnosis; Data-driven图1 PEMFC系统构框图燃料电池散热系统氢气源高压供电空气源辅机冷却系统PEMFC系统边界氢气供给系统热管理系统含水泵、加热器等燃料电池模块支架、管路及线束系统内原件的集成支架、连接管路、供电和通讯线束控制系统水路气路电路低压供电排气系统负载含控制器、传感器含集成外壳、电堆、通风装置等氧气供给系统含空压机、中冷器、增湿器等含压力流量调节、安全泄压、氢气循环装置等22AUTO TIMEAUTO TIME23FRONTIER DISCUSSION | 前沿探讨时代汽车 2.2 PEMFC 故障分析PEMFC 系统是一个多输入多输出的非线性强耦合系统,其故障原因是多方面的,故障分析时除了考虑核心部件特性外,还需要考虑使用环境、人为、电气以及控制策略匹配程度等因素[3]。
分布式相参雷达多脉冲积累相参参数估计方法
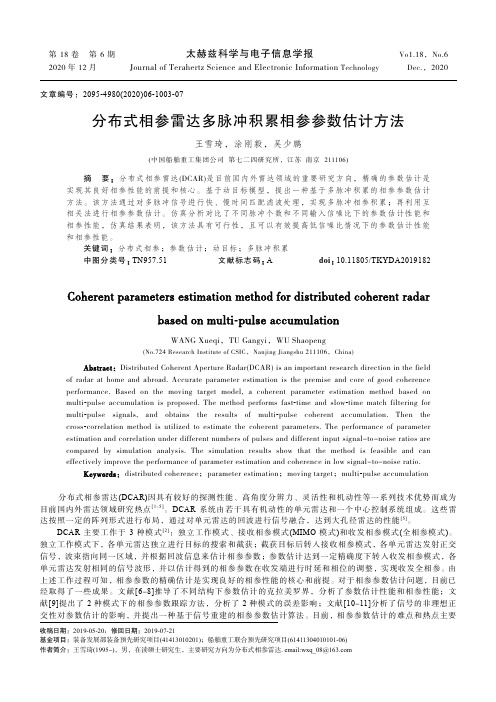
第18卷 第6期 太赫兹科学与电子信息学报Vo1.18,No.6 2020年12月 Journal of Terahertz Science and Electronic Information Technology Dec.,2020 文章编号:2095-4980(2020)06-1003-07分布式相参雷达多脉冲积累相参参数估计方法王雪琦,涂刚毅,吴少鹏(中国船舶重工集团公司第七二四研究所,江苏南京 211106)摘 要:分布式相参雷达(DCAR)是目前国内外雷达领域的重要研究方向,精确的参数估计是实现其良好相参性能的前提和核心。
基于动目标模型,提出一种基于多脉冲积累的相参参数估计方法。
该方法通过对多脉冲信号进行快、慢时间匹配滤波处理,实现多脉冲相参积累;再利用互相关法进行相参参数估计。
仿真分析对比了不同脉冲个数和不同输入信噪比下的参数估计性能和相参性能,仿真结果表明,该方法具有可行性,且可以有效提高低信噪比情况下的参数估计性能和相参性能。
关键词:分布式相参;参数估计;动目标;多脉冲积累中图分类号:TN957.51文献标志码:A doi:10.11805/TKYDA2019182Coherent parameters estimation method for distributed coherent radarbased on multi-pulse accumulationWANG Xueqi,TU Gangyi,WU Shaopeng(No.724 Research Institute of CSIC,Nanjing Jiangshu 211106,China)Abstract:Distributed Coherent Aperture Radar(DCAR) is an important research direction in the field of radar at home and abroad. Accurate parameter estimation is the premise and core of good coherenceperformance. Based on the moving target model, a coherent parameter estimation method based onmulti-pulse accumulation is proposed. The method performs fast-time and slow-time match filtering formulti-pulse signals, and obtains the results of multi-pulse coherent accumulation. Then thecross-correlation method is utilized to estimate the coherent parameters. The performance of parameterestimation and correlation under different numbers of pulses and different input signal-to-noise ratios arecompared by simulation analysis. The simulation results show that the method is feasible and caneffectively improve the performance of parameter estimation and coherence in low signal-to-noise ratio.Keywords:distributed coherence;parameter estimation;moving target;multi-pulse accumulation分布式相参雷达(DCAR)因具有较好的探测性能、高角度分辨力、灵活性和机动性等一系列技术优势而成为目前国内外雷达领域研究热点[1-5]。
地震勘探英语
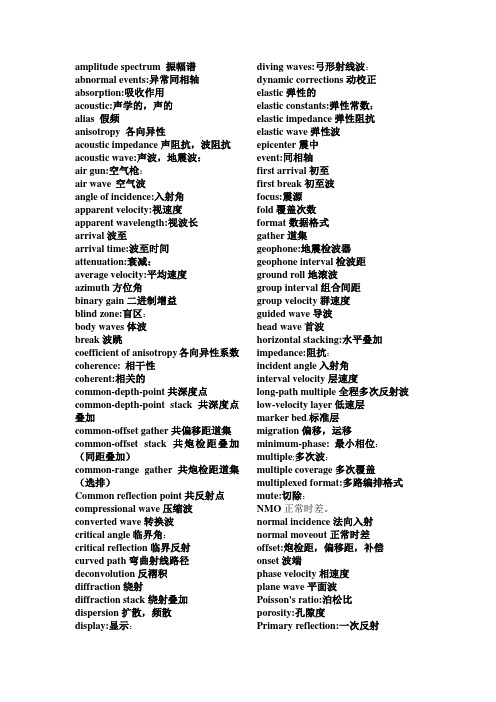
amplitude spectrum 振幅谱abnormal events:异常同相轴absorption:吸收作用acoustic:声学的,声的alias 假频anisotropy 各向异性acoustic impedance声阻抗,波阻抗acoustic wave:声波,地震波:air gun:空气枪:air wave空气波angle of incidence:入射角apparent velocity:视速度apparent wavelength:视波长arrival波至arrival time:波至时间attenuation:衰减:average velocity:平均速度azimuth方位角binary gain二进制增益blind zone:盲区:body waves体波break波跳coefficient of anisotropy各向异性系数coherence:相干性coherent:相关的common-depth-point共深度点common-depth-point stack共深度点叠加common-offset gather共偏移距道集common-offset stack共炮检距叠加(同距叠加)common-range gather共炮检距道集(选排)Common reflection point共反射点compressional wave压缩波converted wave转换波critical angle临界角:critical reflection临界反射curved path弯曲射线路径deconvolution反褶积diffraction绕射diffraction stack绕射叠加dispersion扩散,频散display:显示:diving waves:弓形射线波:dynamic corrections动校正elastic弹性的elastic constants:弹性常数:elastic impedance弹性阻抗elastic wave弹性波epicenter震中event:同相轴first arrival初至first break初至波focus:震源fold覆盖次数format数据格式gather道集geophone:地震检波器geophone interval检波距ground roll地滚波group interval组合间距group velocity群速度guided wave导波head wave首波horizontal stacking:水平叠加impedance:阻抗:incident angle入射角interval velocity层速度long-path multiple全程多次反射波low-velocity layer低速层marker bed.标准层migration偏移,运移minimum-phase:最小相位:multiple:多次波:multiple coverage多次覆盖multiplexed format:多路编排格式mute:切除:NMO正常时差。
介绍dbf测角方法及ti的实现方法
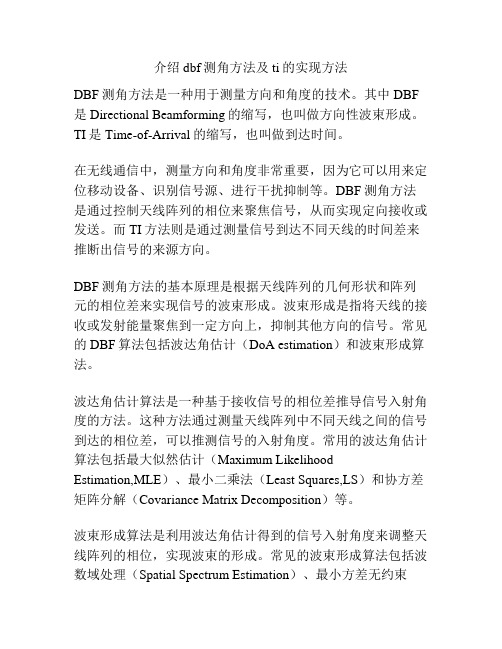
介绍dbf测角方法及ti的实现方法DBF测角方法是一种用于测量方向和角度的技术。
其中DBF 是Directional Beamforming的缩写,也叫做方向性波束形成。
TI是Time-of-Arrival的缩写,也叫做到达时间。
在无线通信中,测量方向和角度非常重要,因为它可以用来定位移动设备、识别信号源、进行干扰抑制等。
DBF测角方法是通过控制天线阵列的相位来聚焦信号,从而实现定向接收或发送。
而TI方法则是通过测量信号到达不同天线的时间差来推断出信号的来源方向。
DBF测角方法的基本原理是根据天线阵列的几何形状和阵列元的相位差来实现信号的波束形成。
波束形成是指将天线的接收或发射能量聚焦到一定方向上,抑制其他方向的信号。
常见的DBF算法包括波达角估计(DoA estimation)和波束形成算法。
波达角估计算法是一种基于接收信号的相位差推导信号入射角度的方法。
这种方法通过测量天线阵列中不同天线之间的信号到达的相位差,可以推测信号的入射角度。
常用的波达角估计算法包括最大似然估计(Maximum LikelihoodEstimation,MLE)、最小二乘法(Least Squares,LS)和协方差矩阵分解(Covariance Matrix Decomposition)等。
波束形成算法是利用波达角估计得到的信号入射角度来调整天线阵列的相位,实现波束的形成。
常见的波束形成算法包括波数域处理(Spatial Spectrum Estimation)、最小方差无约束(Minimum Variance Unconstraint,MVU)和线性约束最小方差(Linear Constrained Minimum Variance,LCMV)等。
TI方法是另一种测角方法,它通过测量信号到达不同天线的时间差来推断出信号的来源方向。
计算时间差的方法包括互相关(Cross-Correlation)、互谱密度(Cross-Spectral Density)和希尔伯特变换(Hilbert Transform)等。
电信专业英语2,4,9三个单元的单词

wagon四轮马车
spoke轮辐
spurious假的,伪造的
arbitrarily任意地
uncertainty不确定性
quantum量子,量化
demltiplexer解复用器
telemetry遥测
generalized通用化
functionality功能
polysilicon多层硅
breadboard面包板
propagation delay传播延迟
base die基底
inductance电感
layout布局,布线
hardware description language (HDL)硬件描述语言
logarithmic对数的
redundancy冗余,多余
fidelity保真度,逼真
faithfully如实地,正确地
intermediary中间的,媒介的
第九单元
sub-field分领域,子领域
sonar声呐
sensor array传感器阵列
biomedical生物特征的
algorithm算法
abbreviate缩写,缩略
interval间隔
discretization离散化
quantization量化Leabharlann partition分割,分区
finite set有限的集
theorem定理
scenario情节,方案
carrier载波
ingredient成分,因素
demodulation解调
enhancement增强
filtering滤波
routing路由,联线
reconfigurable可重新设置的
全球SPEI数据的应用及其与环流因子的多尺度分析
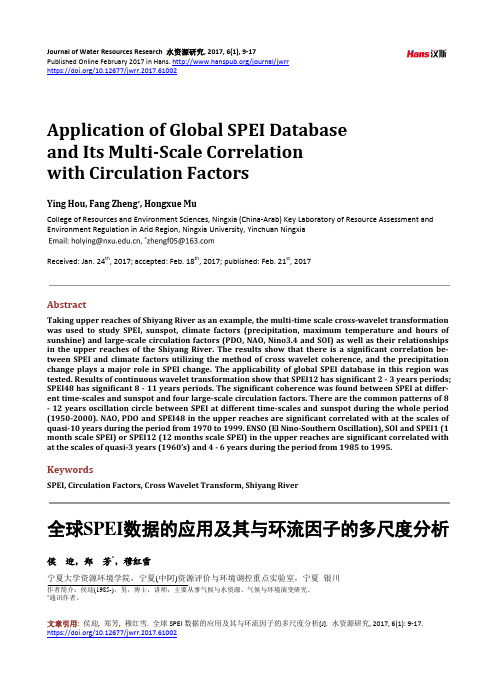
Journal of Water Resources Research 水资源研究, 2017, 6(1), 9-17 Published Online February 2017 in Hans. /journal/jwrr https:///10.12677/jwrr.2017.61002文章引用: 侯迎, 郑芳, 穆红雪. 全球SPEI 数据的应用及其与环流因子的多尺度分析[J]. 水资源研究, 2017, 6(1): 9-17.Application of Global SPEI Database and Its Multi-Scale Correlation with Circulation FactorsYing Hou, Fang Zheng *, Hongxue MuCollege of Resources and Environment Sciences, Ningxia (China-Arab) Key Laboratory of Resource Assessment and Environment Regulation in Arid Region, Ningxia University, Yinchuan NingxiaReceived: Jan. 24th , 2017; accepted: Feb. 18th , 2017; published: Feb. 21st, 2017Abstract Taking upper reaches of Shiyang River as an example, the multi-time scale cross-wavelet transformation was used to study SPEI, sunspot, climate factors (precipitation, maximum temperature and hours of sunshine) and large-scale circulation factors (PDO, NAO, Nino3.4 and SOI) as well as their relationships in the upper reaches of the Shiyang River. The results show that there is a significant correlation be-tween SPEI and climate factors utilizing the method of cross wavelet coherence, and the precipitation change plays a major role in SPEI change. The applicability of global SPEI database in this region was tested. Results of continuous wavelet transformation show that SPEI12 has significant 2 - 3 years periods; SPEI48 has significant 8 - 11 years periods. The significant coherence was found between SPEI at differ-ent time-scales and sunspot and four large-scale circulation factors. There are the common patterns of 8 - 12 years oscillation circle between SPEI at different time-scales and sunspot during the whole period (1950-2000). NAO, PDO and SPEI48 in the upper reaches are significant correlated with at the scales of quasi-10 years during the period from 1970 to 1999. ENSO (El Nino-Southern Oscillation), SOI and SPEI1 (1 month scale SPEI) or SPEI12 (12 months scale SPEI) in the upper reaches are significant correlated with at the scales of quasi-3 years (1960’s) and 4 - 6 years during the period from 1985 to 1995. KeywordsSPEI, Circulation Factors, Cross Wavelet Transform, Shiyang River全球SPEI 数据的应用及其与环流因子的多尺度分析 侯 迎,郑 芳*,穆红雪宁夏大学资源环境学院,宁夏(中阿)资源评价与环境调控重点实验室,宁夏 银川作者简介:侯迎(1985-),男,博士,讲师,主要从事气候与水资源、气候与环境演变研究。
地震勘探常用词汇

各向异性
各向同性介质 各向异性非均匀介质 脉冲 尖脉冲 振幅 频率
isotropic medium
anisotropic non-homogeneous medium impulse spike amplitude frequency wave number
波数
克劳德子波 零相位子波 最小相位子波 波峰 波谷
Ricker wavelet
Klauder wavelet zero phase wavelet minimum phase wavelet wave peak, peak wave trough, trough
(复数) 介质 各向同性
medium, media
弯曲测线法 crooked line method
宽线调查 wide line survey
垂直地震剖面 地震地层学
VSP vertical seismic profile seismic stratigraphy
反射地震学
地震记录仪 96道记录仪 多道记录仪 检波器 陆用检波器 沼泽检波器
reflection seismology
速度
密度 波阻抗
velocity
density acoustic impedance
反射系数
反射系数;反射率 入射角 入射角 反射角 法线 临界角
reflection coefficient
reflectivity incident angle angle of incidence angle of reflection normal line critical angle
seismic recording instrument 96-channel recorder , 96-channel recording instrument multi-channel recorder geophone land case geophone marsh case geophone
纹理物体缺陷的视觉检测算法研究--优秀毕业论文
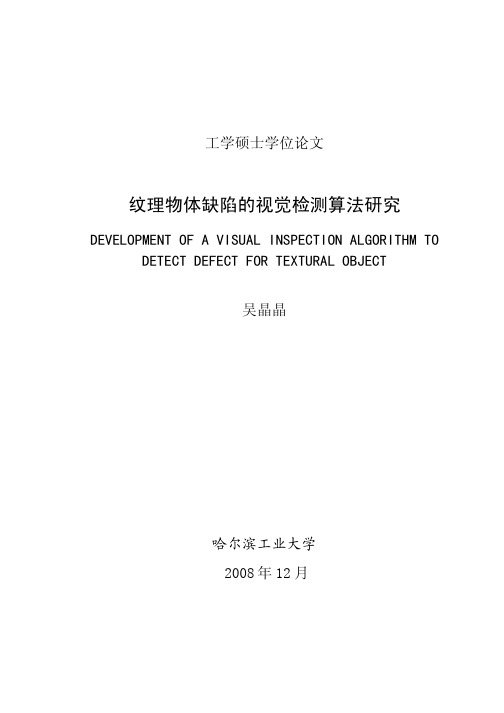
摘 要
在竞争激烈的工业自动化生产过程中,机器视觉对产品质量的把关起着举足 轻重的作用,机器视觉在缺陷检测技术方面的应用也逐渐普遍起来。与常规的检 测技术相比,自动化的视觉检测系统更加经济、快捷、高效与 安全。纹理物体在 工业生产中广泛存在,像用于半导体装配和封装底板和发光二极管,现代 化电子 系统中的印制电路板,以及纺织行业中的布匹和织物等都可认为是含有纹理特征 的物体。本论文主要致力于纹理物体的缺陷检测技术研究,为纹理物体的自动化 检测提供高效而可靠的检测算法。 纹理是描述图像内容的重要特征,纹理分析也已经被成功的应用与纹理分割 和纹理分类当中。本研究提出了一种基于纹理分析技术和参考比较方式的缺陷检 测算法。这种算法能容忍物体变形引起的图像配准误差,对纹理的影响也具有鲁 棒性。本算法旨在为检测出的缺陷区域提供丰富而重要的物理意义,如缺陷区域 的大小、形状、亮度对比度及空间分布等。同时,在参考图像可行的情况下,本 算法可用于同质纹理物体和非同质纹理物体的检测,对非纹理物体 的检测也可取 得不错的效果。 在整个检测过程中,我们采用了可调控金字塔的纹理分析和重构技术。与传 统的小波纹理分析技术不同,我们在小波域中加入处理物体变形和纹理影响的容 忍度控制算法,来实现容忍物体变形和对纹理影响鲁棒的目的。最后可调控金字 塔的重构保证了缺陷区域物理意义恢复的准确性。实验阶段,我们检测了一系列 具有实际应用价值的图像。实验结果表明 本文提出的纹理物体缺陷检测算法具有 高效性和易于实现性。 关键字: 缺陷检测;纹理;物体变形;可调控金字塔;重构
Keywords: defect detection, texture, object distortion, steerable pyramid, reconstruction
II
2016年厦门大学现代统计学研讨会会议议程

2016年厦门⼤学现代统计学研讨会会议议程地点:经济楼C20808:40am-10:40am特邀报告I地点:经济楼N303主持⼈:杨灿,厦门⼤学08:40am-09:20am【1】中国政府统计重点领域改⾰许宪春,国家统计局09:20am-10:00am【2】⼤数据、供给侧结构性改⾰与统计⽣产⼒肖红叶,天津财经⼤学统计学系10:00am-10:40am【3】经济测度中性悖论及其意义邱东,北京师范⼤学统计学院10:40am-11:10am全体合影和茶歇合影地点:经济楼A座门前茶歇地点:经济楼N座5楼三味咖啡厅11:10am-12:10pm分组报告会I分组报告会I-1地点:经济楼N303主持⼈:李迎星,厦门⼤学11:10am-11:30am 【1】 A Lack-of-fit Test with Screening in Sufficient Dimension Reduction for Ultrahigh Dimensional Data 朱利平,中国⼈民⼤学11:30am-11:50am【2】 Lack-of-fit Tests for Quantile Regression Models冯兴东,上海财经⼤学11:50am-12:10pm【3】 Multiple Changepoints Detection via Deep Learning薛原,对外经济贸易⼤学分组报告会I-2地点:经济楼N401主持⼈:冯峥晖,厦门⼤学11:10am-11:30am【1】 Kriging Over Space and Time Based on a Latent Low-Dimensional Structure 张荣茂,浙江⼤学11:30am-11:50am 【2】 Promoting Sign Concordance of Group Penalized Integrative Analysis of Multiple Datasets张庆昭,厦门⼤学11:50am-12:10pm 【3】 Feature Selection for Generalized Varying Coefficient Mixed-Effect Models and Application to Functional GWAS on Obesity刘婧媛,厦门⼤学2016年厦门⼤学现代统计学研讨会会议议程中国·厦门2016年12⽉24⽇(周六)2016年12⽉23⽇(周五)分组报告会I-3地点:经济楼N301主持⼈:许杏柏,厦门⼤学11:10am-11:30am【1】 Covariance Regression Analysis兰伟,西南财经⼤学11:30am-11:50am【2】Robust Inference for Predictive Quantile Regressions with Persistent Predictors 廖⼩赛,厦门⼤学11:50am-12:10pm【3】 Two New Tests for Equality of Several Covariance Functions郭佳,新加坡国⽴⼤学分组报告会I-4地点:经济楼N308主持⼈:许永洪,厦门⼤学11:10am-11:30am【1】产业结构优化⼀定能降低能源消费吗?——来⾃中国地级市卫星灯光数据研究赵楠,北京师范⼤学11:30am-11:50am【2】投⼊产出模型的合并偏差问题研究郑正喜,上海财经⼤学11:50am-12:10pm【3】全球价值链背景下我国⾼技术服务业发展现状分析薛洁,杭州电⼦科技⼤学12:15pm-02:00pm午餐02:00pm-03:20pm特邀报告II地点:经济楼 N303主持⼈:张虎,中南财经政法⼤学统计与数学学院02:00pm-02:40pm【1】拥抱⼤数据发展统计学李⾦昌,浙江财经⼤学02:40pm-03:20pm【2】不完全回答数据下基尼系数的估计房祥忠,北京⼤学数学科学学院03:20pm-03:50pm茶歇地点:经济楼N座5楼三味咖啡厅03:50pm-05:30pm分组报告会II分组报告会II-1地点:经济楼N303主持⼈:林明,厦门⼤学03:50pm-04:10pm【1】 Robust Closed-form Estimators for the integer-valued GARCH(1, 1) Model 朱复康,吉林⼤学04:10pm-04:30pm 【2】 Bridging Compound Poisson Processes and Brownian Motions with Applications to Threshold Models李东,清华⼤学04:30pm-04:50pm【3】 Optimal Model Averaging Estimation for Generalized Linear Models 喻达磊,云南财经⼤学04:50pm-05:10pm【4】Detecting Non-stationary Volatility in Time Series Models吴吉林,⼭东⼤学05:10pm-05:30pm 【5】Inferences for Partially Varying-Coefficient Panel Data Models with Cross-sectional Dependence徐秋华,西南财经⼤学分组报告会II-2地点:经济楼N401主持⼈:钟威,厦门⼤学03:50pm-04:10pm【1】 Lasso Adjustments of Treatment Effect Estimates in Randomized Experiments 刘汉中,清华⼤学04:10pm-04:30pm【2】 Group Sparse Estimation via l0(l2) Regularization Theory and Algorithm 焦⾬领,中南财经政法⼤学04:30pm-04:50pm 【3】 A New Stability Measure for Stationary Functional Processes with Applications to High Dimensional Functional Autoregressive Models郭绍俊,中国⼈民⼤学04:50pm-05:10pm【4】Sufficient Dimension Reduction for Multivariate Response Data张耀武,上海财经⼤学05:10pm-05:30pm【5】Promoting Structural Effects of Covariates in the Cure Rate Model⽅匡南,厦门⼤学分组报告会II-3地点:经济楼N301主持⼈:王学新,厦门⼤学03:50pm-04:10pm【1】基于SVM-Logistic的互联⽹⾦融信⽤评价混合模型探析钱争鸣,厦门⼤学04:10pm-04:30pm【2】 Joint and Marginal Significance Testing for Nonparametric Regression 宋晓军,北京⼤学04:30pm-04:50pm 【3】 Simultaneous equation models with discrete outcomes: Coherence and completeness using panel dataAndrew Pua,厦门⼤学04:50pm-05:10pm【4】Landsat-based Assessment of Wetlands Loss in the Trans-boundary Wusuli River Basin路春燕,福建农林⼤学05:10pm-05:30pm【5】Semi-parametric Estimation of a Partially Varying Coefficient Expectile Model ⽥丁⽯,厦门⼤学分组报告会II-4地点:经济楼N308主持⼈:周⼭,厦门⼤学03:50pm-04:10pm【1】收⼊分配橄榄型程度的测度⽅法洪兴建,浙江⼯商⼤学04:10pm-04:30pm【2】基于福利占优的我国城乡居民消费的税费调整戴平⽣,厦门⼤学04:30pm-04:50pm【3】中国基尼系数的城乡和地区双因素分解艾⼩青,北京⼯业⼤学04:50pm-05:10pm【4】中国代际收⼊弹性的再估计汪燕敏,安徽财经⼤学05:10pm-05:30pm 【5】混合分布模型下的基尼系数计算⽅法研究——基于“中国家庭追踪调查(CFPS)”数据的基尼系数区间估计祁磊,北京师范⼤学05:40pm-08:00pm晚餐09:00am-10:20am特邀报告III地点:经济楼N303主持⼈:蔡宗武,美国堪萨斯⼤学、厦门⼤学2016年12⽉25⽇(周⽇)09:00am-09:40am【1】 Empirical Likelihood for Right Censored Data何书元,⾸都师范⼤学数学科学学院09:40am-10:20am【2】 Nonnested Model Selection蒋建成,北卡罗来纳⼤学夏洛特分校数学与统计系10:20am-10:50am茶歇地点:经济楼N座5楼三味咖啡厅10:50am-12:10pm分组报告会III分组报告会III-1地点:经济楼N303主持⼈:柳冠男,厦门⼤学10:50am-11:10am 【1】 Randomizing the Simplex: Scalable and Accurate Inference for Clustered Categorical Data 林伟,北京⼤学11:10am-11:30am【2】 Testing for Vector White Noise Using Maximum Cross Correlations 常晋源,西南财经⼤学11:30am-11:50am 【3】 A Joint Test for Parametric Specification and Independence in Nonlinear Regression Models 李硕,天津财经⼤学分组报告会III-2地点:经济楼N401主持⼈:杨亚星,厦门⼤学10:50am-11:10am【1】 A Wavelet-based Jump Information Criterion for High-frequency Financial Series Data张伟平,中国科学技术⼤学11:10am-11:30am 【2】 The Impact of Shanghai-Hong Kong Stock Connect Policy on A-H Share Price Premium范青亮,厦门⼤学11:30am-11:50am 【3】 Demographic Associations and Death-Adjusted Probability Estimations of Self-rated Health Statuses among the Elderly in China赵博娟,天津财经⼤学11:50am-12:10pm 【4】Adaptive Estimation of Functional-coefficient Cointegration Models with Nonstationary Volatility 王莹,北京⼤学分组报告会III-3地点:经济楼N406主持⼈:郑挺国,厦门⼤学10:50am-11:10am【1】指数加总能够解释中国通货膨胀的⾼持久性吗?来⾃CPI分类数据的经验证据吕光明,北京师范⼤学11:10am-11:30am【2】⼤数据驱动下中国CPI编制⽅法创新趋向及其挑战陈⽴双,闽南师范⼤学商学院11:30am-11:50am【3】流动⼈⼝城镇社会融⼊信⼼指数编制及应⽤杨江澜,河北农业⼤学12:15pm-02:00pm午餐。
子波提取

子波提取褶积模型是所有反演的基础:地震道=地震子波*反射系数+噪声频率域内, 褶积则为乘积的关系.反演相当于地震道除以地震子波, 得到反射系数:反射系数=地震道/地震子波频域内窄频段的子波限制了信息的获取范围.The narrow band wavelet restricts the available range of information in the frequency domain.地震子波完全由它的振幅谱和相位谱来定义:The Wavelet is defined completely by its amplitudespectrum and its phase spectrum:在有限频率范围内, 相位谱通常可近似为一条直线. 直线的截距是子波的常数相位旋转, 它是子波的最佳表征. 直线的斜率标示着子波的时移.The intercept of the line is the constant phase rotation which best characterizes this wavelet.The slope of the line measures the time-shift of the wavelet.极性的约定:极性约定是一个特殊的子波相位问题. 默认的约定便是: 声阻抗的增加在零相位的地震数据上代表一个波峰.A special wavelet phase issue is the Polarity convention.The default convention is that an increase in acoustic impedance is represented as a peak on zero-phase seismic data:另一个默认的约定便是: 声阻抗的增加在零相位的地震数据上代表一个波谷.The alternate convention is that an increase in acoustic impedance is represented as a trough on zero-phase seismic data:使用ì极性约定菜单î可以设置极性约定:The polarity convention is set using the SyntheticPolarity Convention menu:地震子波在时间和空间上都存在着变化, 即具有时变性和空变性, 这是基于以下几个原因:Wavelets in the earth vary both laterally (spatially) and temporally for a variety of reasons:近地表效应(空变)Near surface effects (space variant)频率吸收(时变和空变)Frequency-dependent absorption (space and time variant)层间多次波(时变和空变)Inter-bed multiples (space and time variant)NMO 拉伸处理过程中的人为因素Processing artifactsSTRATA 假定子波是常数, 不随时间和空间变化: 时间不变性: 这意味着反演就是在有限的时窗内求最优化的波阻抗Time invariant: This means that the inversion is optimized for a limited time window.空间不变性: 这意味着去除子波的空变后被最优化处理. 通常, 许多方法有可以用来提取子波. STRATA中用了以下几种:In general, a variety of methods can be used for wavelet extraction. Some are available in STRATA. (1) 仅用地震数据估计地震子波的振幅谱. 假设相位谱已经从别的渠道得知.子相关autocorrelation最大熵谱分析maximum entropy spectral analysis交互谱分析cross spectral analysisSTRATA中统计子波的提取用自相关: Statistical wavelet extraction uses the autocorrelation(2) 单独使用地震数据估算振幅谱和相位谱Estimate both amplitude and phase spectra from the seismic data alone.最小熵子波估计高阶力矩法higher order momentsSTRATA 不用这种方法, 因为STRATA认为该方法不可靠.(3) 使用给定的测量数据估计振幅谱和相位谱Estimate both amplitude and phase spectra using deterministic measurements.海洋信号marine signaturesVSP 分析STRATA中, 以ASCII文件形式读入外部子波(4) 用地震和测井资料估算振幅谱和相位谱Estimate both amplitude and phase spectra using both seismic and well log measurements.STRATA中用测井资料提取全子波.(5) 用地震资料和测井资料估算振幅谱和常数相位谱STRATA中用测井资料提取常数相位子波.STRATA中提取子波的方法:第一步, 是否用测井资料来估算子波的相位. 关键是看测井资料与地震资料的相关性是否好. 通常情况下, 必须首先进行手动校正测井曲线. The critical issue for this decision is how well the logs tie the seismic data. Usually, manual correlation must be done to align the logs first.1 提取统计子波(不用井资料):这个过程只是通对地震道进行自相关计算子波的振幅谱, 并假设已知子波的相位.主要参数:ï道范围(通常设置为较大值以增加统计所用的道数) Trace range (usually set this large to increase statistics)ï时窗(至少应该为子波长度的两倍)ï子波长度(取决于层厚和分辨率, 层厚一般取200ms, 薄层取50~100ms).2 用测井资料提取子波:用测井资料提取子波:此方法用测井资料估算子波的振幅谱和相位谱. 效果取决于测井曲线和地震道的相关程度.主要参数:选择要用的井(只用标定效果好的井)道范围(距井的距离)时窗子波长度3 用测井资料计算单一常数相位值该方法使用地震道的自相关计算子波的振幅谱, 与统计子波提取方法中一样, 用测井资料计算子波的相位谱, 并且相位谱被近似为一个单一的常数谱.This procedure calculates the amplitude spectrum of the wavelet using the autocorrelation of the seismic traces,exactly as in the statistical procedure.The phase spectrum is approximated as a single constant value, using the well logs.这种方法比较稳定, 特别是测井资料与地震数据的相关性较差时.This procedure is more robust than the full phase spectrum calculation, especially when the tie between logs and seismic is poor.计算相位的步骤:(1) 用统计子波提取方法计算子波(不用井资料).(2) 对所提取的子波进行一系列的常相位旋转(3) 用每一次旋转后的子波计算合成道, 并且与地震道进行相关.(4) 选出与地震道产生最大相关值的相位旋转子波提取中的问题:用井提取子波时, 必须首先求出测井曲线之间的最优化相关To extract a wavelet using logs, an optimum correlation must be done first.正确地相关必须以子波已知为前提To perform correlation properly, the wavelet must already be known.实际子波提取的流程:(1) 用统计子波提取来确定一个初步的子波, 假设子波的近似相位已知.(2) 拉伸或压缩测井曲线来标定地震道.(3) 使用新的测井曲线来提取新的子波.(4) 重复第(2)、(3)步,直到提取的子波达到要求为止.。
本科生、分析研究生均可使用《化学》中常见词汇的中英对照
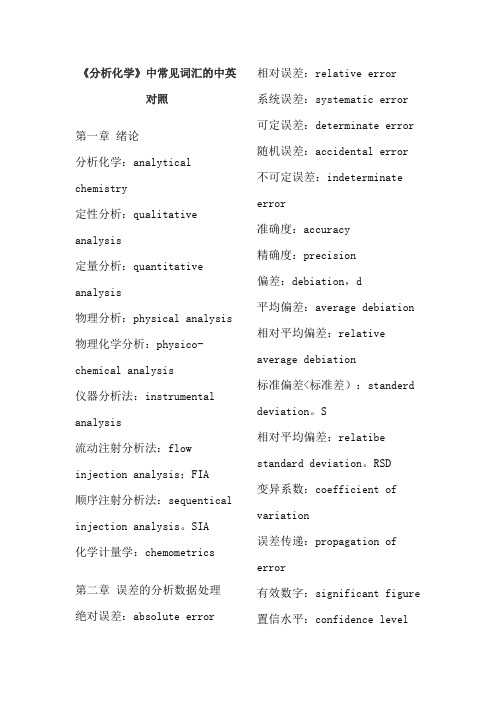
《分析化学》中常见词汇的中英对照第一章绪论分析化学:analytical chemistry定性分析:qualitative analysis定量分析:quantitative analysis物理分析:physical analysis 物理化学分析:physico-chemical analysis仪器分析法:instrumental analysis流动注射分析法:flow injection analysis;FIA顺序注射分析法:sequentical injection analysis。
SIA化学计量学:chemometrics第二章误差的分析数据处理绝对误差:absolute error 相对误差:relative error系统误差:systematic error 可定误差:determinate error 随机误差:accidental error 不可定误差:indeterminate error准确度:accuracy精确度:precision偏差:debiation,d平均偏差:average debiation 相对平均偏差:relative average debiation标准偏差<标准差):standerd deviation。
S相对平均偏差:relatibe standard deviation。
RSD变异系数:coefficient of variation误差传递:propagation of error有效数字:significant figure 置信水平:confidence level显著性水平:level of significance合并标准偏差<组合标准差):pooled standard debiation 舍弃商:rejectionquotient 。
Q化学定量分析第三章滴定分析概论滴定分析法:titrametric analysis滴定:titration容量分析法:volumetric analysis化学计量点:stoichiometric point等当点:equivalent point电荷平衡:charge balance电荷平衡式:charge balance equation质量平衡:mass balance物料平衡:material balance 质量平衡式:mass balance equation第四章酸碱滴定法酸碱滴定法:acid-base titrations质子自递反应:autoprotolysis reaction质子自递常数:autoprotolysis constant质子条件式:proton balance equation酸碱指示剂:acid-base indicator指示剂常数:indicator constant变色范围:colour change interval混合指示剂:mixed indicator 双指示剂滴定法:double indicator titration第五章非水滴定法非水滴定法:nonaqueous titrations质子溶剂:protonic solvent 酸性溶剂:acid solvent碱性溶剂:basic solvent两性溶剂:amphototeric solvent无质子溶剂:aprotic solvent 均化效应:differentiating effect区分性溶剂:differentiating solvent离子化:ionization离解:dissociation结晶紫:crystal violet萘酚苯甲醇: α-naphthalphenol benzyl alcohol奎哪啶红:quinadinered百里酚蓝:thymol blue 偶氮紫:azo violet溴酚蓝:bromophenol blue第六章配位滴定法配位滴定法:compleximetry乙二胺四乙酸:ethylenediamine tetraacetic acid,EDTA螯合物:chelate compound金属指示剂:metal lochrome indcator第七章氧化还原滴定法氧化还原滴定法:oxidation-reduction titration碘量法:iodimetry溴量法:bromimetry ]溴量法:bromine method铈量法:cerimetry高锰酸钾法:potassium permanganate method条件电位:conditional potential溴酸钾法:potassium bromate method硫酸铈法:cerium sulphate method偏高碘酸:metaperiodic acid 高碘酸盐:periodate亚硝酸钠法:sodium nitrite method重氮化反应:diazotization reaction重氮化滴定法:diazotization titration亚硝基化反应:nitrozation reaction亚硝基化滴定法:nitrozation titration外指示剂:external indicator 外指示剂:outside indicator 重铬酸钾法:potassium dichromate method第八章沉淀滴定法沉淀滴定法:precipitation titration容量滴定法:volumetric precipitation method银量法:argentometric method 第九章重量分析法重量分析法:gravimetric analysis挥发法:volatilization method引湿水<湿存水):water of hydroscopicity包埋(藏>水:occluded water 吸入水:water of imbibition 结晶水:water of crystallization组成水:water of composition 液-液萃取法:liquid-liquid extration溶剂萃取法:solvent extration反萃取:counter extraction 分配系数:partitioncoefficient分配比:distribution ratio 离子对<离子缔合物):ion pair沉淀形式:precipitation forms称量形式:weighing forms 《分析化学》下册仪器分析概述物理分析:physical analysis 物理化学分析:physicochemical analysis仪器分析:instrumental analysis第十章电位法及永停滴定法电化学分析:electrochemical analysis电解法:electrolytic analysis method电重量法:electtogravimetry 库仑法:coulometry 库仑滴定法:coulometric titration电导法:conductometry电导分析法:conductometric analysis电导滴定法:conductometric titration电位法:potentiometry直接电位法:dirext potentiometry电位滴定法:potentiometric titration伏安法:voltammetry极谱法:polarography溶出法:stripping method电流滴定法:amperometric titration化学双电层:chemical double layer相界电位:phase boundary potential金属电极电位:electrodepotential化学电池:chemical cell液接界面:liquid junction boundary原电池:galvanic cell电解池:electrolytic cell 负极:cathrode正极:anode电池电动势:eletromotive force指示电极:indicator electrode参比电极:reference electroade标准氢电极:standard hydrogen electrode一级参比电极:primary reference electrode饱和甘汞电极:standard calomel electrode银-氯化银电极:silver silver-chloride electrode 液接界面:liquid junction boundary不对称电位:asymmetry potential表观PH值:apparent PH复合PH电极:combination PH electrode离子选择电极:ion selective electrode敏感器:sensor晶体电极:crystalline electrodes均相膜电极:homogeneous membrance electrodes非均相膜电极:heterog eneous membrance electrodes非晶体电极:non- crystalline electrodes刚性基质电极:rigid matrix electrode流流体载动电极:electrode with a mobile carrier气敏电极:gas sensing electrodes酶电极:enzyme electrodes金属氧化物半导体场效应晶体管:MOSFET离子选择场效应管:ISFET总离子强度调节缓冲剂:total ion strength adjustment buffer,TISAB永停滴定法:dead-stop titration双电流滴定法<双安培滴定法):double amperometric titration第十一章光谱分析法概论普朗克常数:Plank constant 电磁波谱:electromagnetic spectrum光谱:spectrum光谱分析法:spectroscopic analysis原子发射光谱法:atomic emission spectroscopy质量谱:mass spectrum质谱法:mass spectroscopy,MS 第十二章紫外-可见分光光度法紫外-可见分光光度法:ultraviolet and visible spectrophotometry。
基于小波变换与灰色系统理论的图像边缘检测
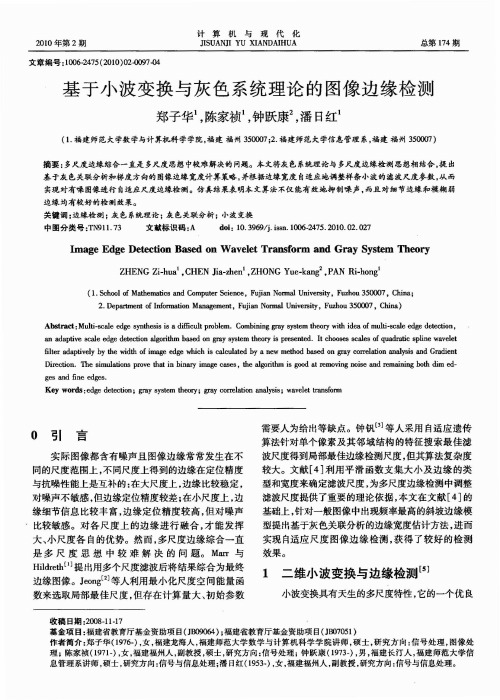
关键词:边缘检测:灰色系统理论:灰色关联分析;小泼交换
中图分类号 : TN9 11. 73 文 献标识ω6-2475.2010.02 .027
Image Edge Detection
B描cd
on Wavelet Transform and Gray System Th eory
o
51
言
需要人为给出等缺点。钟饥[3 ]等人采用自适应遗传
算法针对单个像素及其邻域结构的特征搜索最佳滤 波尺度得到局部最佳边缘检测尺度,但其算法复杂度
较大。文献 [ 4 ]利用平滑函数支集大小及边缘的类
实际图像都含有噪声且图像边缘常常发生在不
同的尺度范围上,不同尺度上得到的边缘在定位精度
与抗噪性能上是互补的:在大尺度上,边缘比较稳定, 对噪声不敏感,但边缘定位精度较差;在小尺度上,边
缘细节信息比较丰富,边缘定位精度较高 ,但对噪声
.比较敏感 。对各尺度上的边缘进行融合,才能发挥
型和宽度来确定滤波尺度,为多尺度边缘检测中调整
滤波尺度提供了重要的理论依据,本文在文献 [ 4 ]的
基础上,针对一般图像中出现频率最高的斜坡边缘模 型提出基于灰色关联分析的边缘宽度估计方法,进而 实现自适应尺度图像边缘检测,获得了较好的检测
摘要:多尺度边缘综合一直是多尺度思想中较难解决的问题。本文将灰色系统理论与多尺度边缘检测思想相结合,提出
基于灰色关联分析和梯度方向的图像边缘宽度计算革略 边缘均有较好的检测效果 。
,并根据边缘宽度自适应地调整样条小泼的泼 、波尺度参数,从而
实现对有噪困像进行自适应尺度边缘检测。仿真结果表明本文算法不仅能有效地抑制噪声,而且对细节边缘和模糊弱
大、小尺度各自的优势。然而,多尺度边缘综合一直
08-信息科学与电子工程专业英语(第2版)-吴雅婷-清华大学出版社
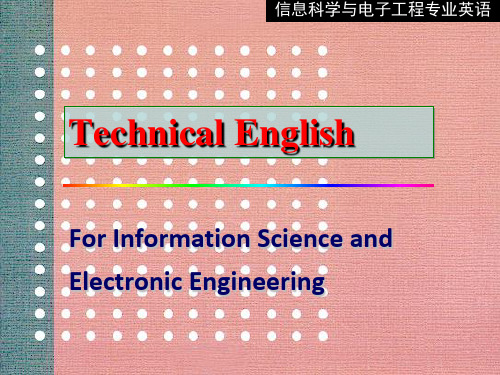
hifi (high fidelity) 高保真(音乐) reinforcement 加强
animation 动画
loudspeakec 算术
magnetic resonanceimaging (MRI) fixed-point arithmetic
IIR (infinite impulse response filter) 无限冲击响应滤波器
field-programmablegate array (FPGA) 现场可编程门阵列
computer aidedtomography (CAT) 计算机断层扫描
seismology 地震学
equalization 均衡
数字信号处理的目标通常是 测量连续的真实世界的模拟 信号或对其滤波
7
3
The algorithms required for DSP are sometimes performed using specialized computers, which make use of specialized microprocessors called digital signal processors (also abbreviated DSP). These process signals in real time, and are generally purpose-designed application-specific integrated circuits (ASICs).1
Part I
Digital Signal Processing
New Words
sub-field 分领域,子领域 sensor array 传感器阵列 algorithm 算法 purpose-designed 针对目的设计的 integrated circuits 集成电路 wavelet 小波 informed 有知识的,有见闻的 cross-correlation 互相关 discretization 离散化 partition 分割,分区
211174835_Halcyon2.0医用直线加速器胸部调强放射治疗计划射野复杂度指标与剂量验证的
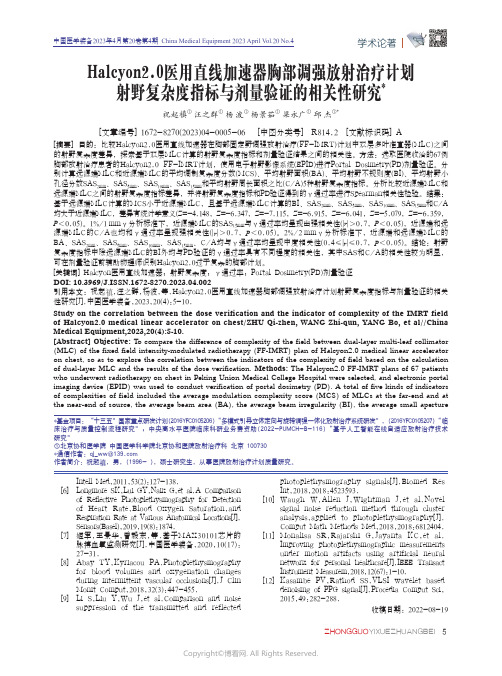
学术论著收稿日期:2022-08-19[6][7][8][9]Intell Med,2011,53(2):127-138.Longmore SK,Lui GY,Naik G,et al.A Comparison of Reflective Photoplethysmography for Detection of Heart Rate,Blood Oxygen Saturation,and Respiration Rate at Various Anatomical Locations[J].Sensors(Basel),2019,19(8):1874.姬军,王景华,曾毅东,等.基于MAX30101芯片的脉搏血氧监测研究[J].中国医学装备,2020,10(17):27-31.Abay TY,Kyriacou PA.Photoplethysmography for blood volumes and oxygenation changes during intermittent vascular occlusions[J].J Clin Monit Comput,2018,32(3):447-455.Li S,Liu Y,Wu J,et parison and noise suppression of the transmitted and reflected[10][11][12]photoplethysmography signals[J].Biomed Res Int,2018,2018:4523593.Waugh W,Allen J,Wightman J,et al.Novelsignal noise reduction method through cluster analysis,applied to photoplethysmography[J].Comput Math Methods Med,2018,2018:6812404.Monalisa SR,Rajarshi G,Jayanta KC,et al.Improving photoplethysmographic measurements under motion artifacts using artificial neural network for personal healthcare[J].IEEE Transact Instrument Measurem,2018,12(67):1-10.Kasambe PV,Rathod SS.VLSI wavelet baseddenoising of PPG signal[J].Procedia Comput Sci,2015,49:282-288.*基金项目:“十三五”国家重点研发计划(2016YFC0105206)“多模式引导立体定向与旋转调强一体化放射治疗系统研发”,(2016YFC0105207)“临床治疗与质量控制流程研究”;中央高水平医院临床科研业务费资助(2022-PUMCH-B-116)“基于人工智能在线自适应放射治疗技术研究”①北京协和医学院 中国医学科学院北京协和医院放射治疗科 北京 100730*通信作者:*************作者简介:祝起禛,男,(1996- ),硕士研究生,从事医院放射治疗计划质量研究。
cc(Correlation coefficient)英文帮助
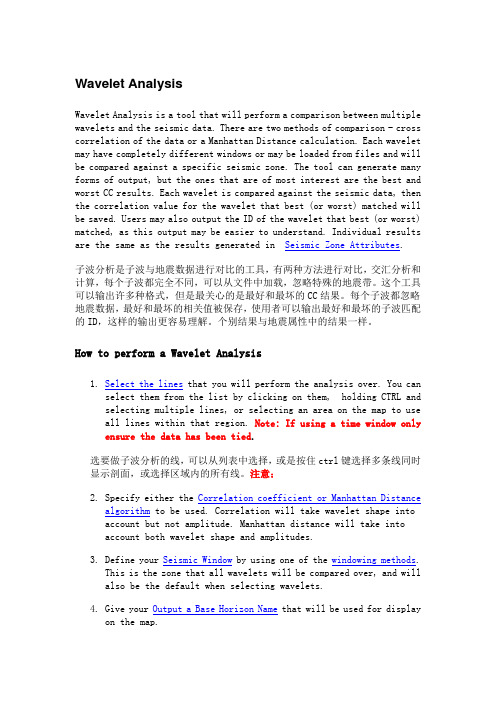
Wavelet AnalysisWavelet Analysis is a tool that will perform a comparison between multiple wavelets and the seismic data. There are two methods of comparison - cross correlation of the data or a Manhattan Distance calculation. Each wavelet may have completely different windows or may be loaded from files and will be compared against a specific seismic zone. The tool can generate many forms of output, but the ones that are of most interest are the best and worst CC results. Each wavelet is compared against the seismic data, then the correlation value for the wavelet that best (or worst) matched will be saved. Users may also output the ID of the wavelet that best (or worst) matched, as this output may be easier to understand. Individual results are the same as the results generated in Seismic Zone Attributes.子波分析是子波与地震数据进行对比的工具,有两种方法进行对比,交汇分析和计算,每个子波都完全不同,可以从文件中加载,忽略特殊的地震带。
信号处理常用的函数
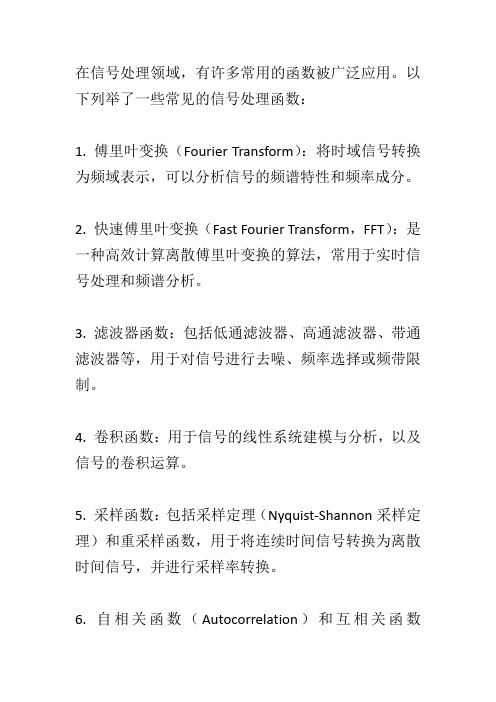
在信号处理领域,有许多常用的函数被广泛应用。
以下列举了一些常见的信号处理函数:
1. 傅里叶变换(Fourier Transform):将时域信号转换为频域表示,可以分析信号的频谱特性和频率成分。
2. 快速傅里叶变换(Fast Fourier Transform,FFT):是一种高效计算离散傅里叶变换的算法,常用于实时信号处理和频谱分析。
3. 滤波器函数:包括低通滤波器、高通滤波器、带通滤波器等,用于对信号进行去噪、频率选择或频带限制。
4. 卷积函数:用于信号的线性系统建模与分析,以及信号的卷积运算。
5. 采样函数:包括采样定理(Nyquist-Shannon采样定理)和重采样函数,用于将连续时间信号转换为离散时间信号,并进行采样率转换。
6. 自相关函数(Autocorrelation)和互相关函数
(Cross-correlation):用于分析信号的自相似性和信号之间的相似度。
7. 小波变换(Wavelet Transform):用于对非平稳信号进行局部频率分析,具有时频域分辨率可调的特点。
8. 包络检测函数:用于提取信号的包络轨迹,常用于振动信号分析和故障诊断。
9. 平滑函数:如移动平均、指数平滑等,用于对信号进行平滑处理,降低噪声或波动。
10. 调制函数:如调幅(AM)、调频(FM)、调相(PM)等,用于在调制与解调过程中改变信号的特性。
当然,这只是信号处理领域中的一小部分常用函数。
实际应用中,根据具体问题和需求,可能还会涉及其他更多的函数和算法。
基于VMD的广义二次互相关时延估计方法

第39卷第3期 吉林大学学报(信息科学版)V d.39 No.3 2021 年 5 月Journal of Jilin University(Information Science Edition)May2021文章编号:1671 -5896 (2021) 03 -0260>07基于V M D的广义二次互相关时延估计方法李宏3,田雷%路敬祎b’%刘庆强a(东北石油大学a.电气信息工程学院;b.人工智能能源研究院;c.黑龙江省网络化重点实验室,黑龙江大庆163318)摘要:针对广义互相关(GCC:Generalized Cross-Correlalion)时延估计方法在低信噪比的情况下会产生较大误差的问题,提出一种基于变分模态分解(VMD:Variational Mode Decomposition)结合广义二次互相关(GSCC: Generalized Second Cross-Correlation)进行时延估计的方法。
该方法首先对两路信号分别进行变分模态分解,分离有效模态和噪声模态,使用豪斯多夫距离(HD:HaiisdorffDislance)优选模态并重构信号,然后运用广义二次互相关对处理后的信号进行时延估计。
理论分析和仿真实验结果表明,与广义二次互相关方法、小波去噪结合广义二次互相关(WT-GSCC:Wavelet-GSCC)方法比较,该方法能有效提升估计精度,具有良好的抗噪性能。
关键词:变分模态分解;广义二次互相关;豪斯多夫距离;时延估计中图分类号:TN911.72 文献标识码:ATime Delay Estimation Method of Generalized SecondCross Correlation Based on VMDL I Hong a,TIAN L e i a,LU J i n g y i b c,UU Q i n g q i a n g8(a. School of Electrical Engineering and Information;b. Artificial Intelligence Energy Research Institute;c. Heilongjiang Provincial Key Laboratory of Networking and Intelligent Control, Northeast Petroleum University, Daqing 163318, China)A b s t r a c t:A i m i n g a t t h e p r o b l e m t h a t t h e GCC(G e n e r a l i z e d C r o s s-C o r r e l a t i o n)t i m e d e l a y e s t i m a t i o n m e t h o d w i l l p r o d u c e l a r g e e r r o r s u n d e r t h e c o n d i t i o n o f l o w s i g n a l-t o-n o i s e r a t i o,a m e t h o d o f t i m e d e l a y e s t i m a t i o n b a s e d o n V M D(V a r i a t i o n a l Mo de D e c o m p o s i t i o n)c o m b i n e d w i t h GSCC(G e n e r a l i z e d S e c o n d C r o s s-C o r r e l a t i o n)i s p r o p o s e d.T h i s m e t h o d f i r s t p e r f o r m s v a r i a t i o n a l m o d a l d e c o m p o s i t i o n o f t h e t w o s i g n a l s s e p a r a t e l y,s e p a r a t e s t h e e f f e c t i v e m o d e a n d t h e n o i s e mode,u s e s t h e HD (H a u s d o r f f D i s t a n c e)t o o p t i m i z e t h e m o d e a n d r e c o n s t r u c t s t h e s i g n a l.T h e n u s e s t h e g e n e r a l i z e d s e c o n d c r o s s-c o r r e l a t i o n t o a n a l y z e t h e p r o c e s s e d s i g n a l a n d p e r f o r m d e l a y e s t i m a t i o n.T h e o r e t i c a l a n a l y s i s a n d s i m u l a t i o n e x p e r i m e n t r e s u l t s s h o w t h a t c o m p a r e d w i t h t h e g e n e r a l i z e d s e c o n d c r o s s-c o r r e l a t i o n m e t h o d,w a v e l e t d e n o i s i n g c o m b i n e d w i t h t h e g e n e r a l i z e d s e c o n d c r o s s-c o r r e l a t i o n (WT-GSCC:W a v e l e t-G S C C)m e t h o d,t h i s m e t h o d c a n e f f e c t i v e l y i m p r o v e t h e e s t i m a t i o n a c c u r a c y a n d h a s g o o d a n t i-n o i s e p e r f o r m a n c e.Key words:v a r i a t i o n a l m o d a l d e c o m p o s i t i o n;g e n e r a l i z e d s e c o n d c r o s s-c o r r e l a t i o n;h a u s d o r f f d i s t a n c e;t i m ed e l a y e s t i m a t i o n0引言时间延迟作为信号特征的一个重要参数,随着信号处理技术的飞速发展,时延估计算法逐渐成为众 多学者的研究热点m。
时间序列期末考试英文

时间序列期末考试英文Title: Characterizing Long-Term Temporal Patterns in Stock Market DataAbstract:This study presents an analysis of long-term temporal patterns in stock market data, aiming to identify trends, cycles, and potential predictability. We employed advanced time series techniques to extract meaningful patterns from historical stock price data. Our findings suggest the presence of distinct long-term cycles, which may offer valuable insights for investors and traders.Keywords: Stock Market, Time Series Analysis, Long-Term Trends, CyclesIntroduction:The stock market is a complex system, characterized by high volatility and unpredictability. However, understanding its long-term temporal patterns can provide valuable insights for investors. In this study, we aim to identify long-term trends and cycles in stock market data, utilizing advanced time series analysis techniques.Methodology:We collected historical daily closing prices for a broad market index (e.g., S&P 500) spanning over a period of 20 years. We employed various time series analysis techniques, including autocorrelation, cross-correlation, Fourier analysis, and wavelet transforms, to extract meaningful patterns from the data.Results:Our analysis revealed several long-term temporal patterns in the stock market data. We identified significant autocorrelation in the data, suggesting the presence of persistent trends. Fourier analysis revealed distinct long-term cycles with periods ranging from 5 to 10 years. Wavelet transforms further confirmed these cycles and provided insights into their evolution over time.Conclusion:Our findings suggest that the stock market exhibits distinct long-term temporal patterns characterized by persistent trends and cycles. These patterns may offer valuable insights for investors and traders, enabling them to make more informed decisions. Future research should focus on exploring the underlying mechanisms responsible for these patterns and their implications for portfolio management and investment strategies.References:[Please provide the references here]Appendix:[Please provide the appendix here]。
地震资料相位差异分析方法与应用
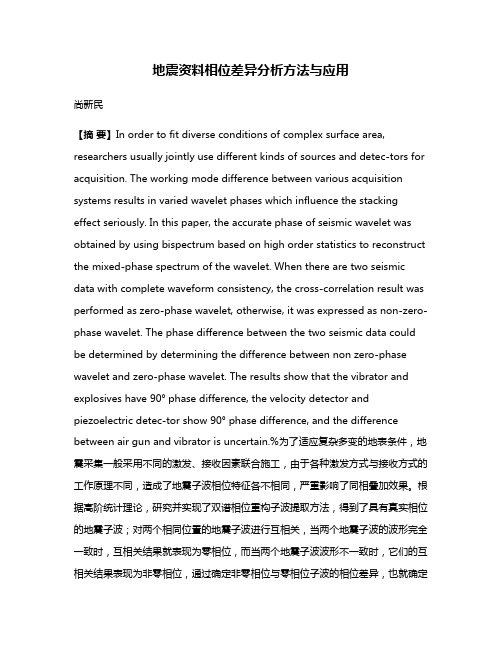
地震资料相位差异分析方法与应用尚新民【摘要】In order to fit diverse conditions of complex surface area, researchers usually jointly use different kinds of sources and detec-tors for acquisition. The working mode difference between various acquisition systems results in varied wavelet phases which influence the stacking effect seriously. In this paper, the accurate phase of seismic wavelet was obtained by using bispectrum based on high order statistics to reconstruct the mixed-phase spectrum of the wavelet. When there are two seismic data with complete waveform consistency, the cross-correlation result was performed as zero-phase wavelet, otherwise, it was expressed as non-zero-phase wavelet. The phase difference between the two seismic data could be determined by determining the difference between non zero-phase wavelet and zero-phase wavelet. The results show that the vibrator and explosives have 90° phase difference, the velocity detector and piezoelectric detec-tor show 90° phase difference, and the difference between air gun and vibrator is uncertain.%为了适应复杂多变的地表条件,地震采集一般采用不同的激发、接收因素联合施工,由于各种激发方式与接收方式的工作原理不同,造成了地震子波相位特征各不相同,严重影响了同相叠加效果。
- 1、下载文档前请自行甄别文档内容的完整性,平台不提供额外的编辑、内容补充、找答案等附加服务。
- 2、"仅部分预览"的文档,不可在线预览部分如存在完整性等问题,可反馈申请退款(可完整预览的文档不适用该条件!)。
- 3、如文档侵犯您的权益,请联系客服反馈,我们会尽快为您处理(人工客服工作时间:9:00-18:30)。
a rX iv:physics /0329v1[physics.flu-dyn]14Mar200Wavelet Cross-Correlation Analysis of Turbulent Mixing from Large-Eddy-Simulations S.Sello and J.Bellazzini Enel Research A.Pisano 120,Pisa 56122,ITALY Contact e-mail:sello@pte.enel.it 1Introduction The complex interactions existing between turbulence and mixing in a bluff-body stabilised flame configuration is investigated by means of a wavelet cross-correlation analysis on Large Eddy Simulations.The combined approach allows to better point out typical features of unsteady turbulent flows with mixing through the characterisation of the processes involved both in time and scales.The wavelet cross-correlation analysis of the time signals of velocity and mixture fraction fluctuations can be an an effective tool to study the processes involved in turbulent mixing flows which are of great interest in combustion problems.2Generalities on wavelet cross-correlation The continuous wavelet transform of a function f (t )is defined as the convolution between f and a dilated function ψcalled wavelet mother:W f (a,τ)=1a +∞−∞f (t )ψ∗(t −τ2where the symbol∗indicates the complex conjugate.When the wavelet mother is complex,the wavelet cross-scalogram W fg(a,τ)is also complex and can be written in terms of its real and imaginary parts:W fg(a,τ)=CoW fg(a,τ)−iQuadW fg(a,τ).(3) It can be shown that the following equation holds if f(t),g(t)∈L2(ℜ)+∞f(t)g(t)dt=1/cψ +∞0 +∞−∞CoW fg(a,τ)dτda,(4)−∞where1/cψis a constant depending on the choice of the wavelet mother.3Cross wavelet coherence functionsThe highly redundant information from a multiscale wavelet analysis of time series must be reduced by means of suitable selective procedures and quantities, in order to extract the main features correlated to an essentially intermittent dynamics.In this study,we analysed and compared the properties of two com-plementary wavelet local correlation coefficents which are able to well evidence peculiar and anomalous local events associated to the vortex dynamics.More precisely,given two signals f(t)and g(t),we refer to the so-called Wavelet Local Correlation Coefficent(Buresti et.al[1]),defined as:CoW fg(a,τ)W LCC(a,τ)=|W f(a,τ)|4+|W g(a,τ)|4,(6) which is essentially a measure of the intensity coherence of the ing the polar coordinates we can write the wavelet transforms of W f(a,τ),W g(a,τ) and W fg(a,τ)as:W f(a,τ)=ρf eıθf W g(a,τ)=ρg eıθg(7)W fg(a,τ)=ρfρg eı(θg−θf),(8) and the Cross Wavelet Coherence Function can be written also as:2ρ2fρ2gCW CF(a,τ)=3 4Numerical simulationWe considered a laboratory-scale axisymmetricflame of methane-air in a non confined bluff-body configuration.More precisely,the burner consists of a5.4 mm diameter methane jet located in the center of a50mm diameter cylinder. Air is supplied through a100mm outer diameter coaxial jet around the50mm diameter bluff-body.The Reynolds number of the central jet is7000(methane velocity=21m/s)whereas the Reynolds number of the coaxial jet is80000(air velocity=25m/s).This is a challenging test case for all the turbulence models, as well documented in the ERCOFTAC report(Chatou,1994)[2].Moreover,due to the highly intermittent,unsteady dynamics involved and the high turbulence level,especially for the reactive case,the Large Eddy Simulation(LES)appears as the most adequate numerical approach(Sello et.al[3]).5Results and discussionIn this analysis we are mainly interested to relations existing between evolution of turbulence and mixing,for the reactive case.Previous DNS simulations on coaxial jets at different Reynolds numbers,show the ability of the wavelet cross-correlation analysis to better investigate the relations between mixing process and the dynamics of vorticity(Salvetti et.al[4]).Thus,the signals analysed here are velocityfluctuations(for Reynolds stress contributions)and mixture fraction fluctuations(for mixing evolution)from LES.As an example,Figure1shows the wavelet co-spectrum maps for a significant time interval in the pseudo-stationary regime of motion.The main contributions to the Reynolds stress are evidenced by high intensity correlations(red)and anti-correlations(blue)regions,which evolve intermittently.The dominant frequencies involved are located around 130Hz.For the mechanisms responsable of the evolution of mixing,we note that the same regions of high Reynolds stress correspond to high correlation, or cooperation,between velocity and mixture fractionfluctuations,suggesting that,at the selected location,the same events of stretching and tilting of the vorticity layer,drive both Reynolds stress and mixing evolutions.Note that the large high value region located at low frequencies in the right map is statistically not significant if we assume a proper red noise background spectrum.To better investigate the role of the high correlation regions,we performed a cross section in the wavelet map at the frequency160Hz.Figure2(left)shows the time behaviour of the coherence functions WLCC,eq.(5),and CWCF,eq.(6).Here the phase and intensity coherence of signals are almost equivalent,but we can clearly point out an important anomalous event occurred at around t=0.19s, corresponding to a loss of both intensity and phase coherence,followed by a change of the correlation sign.The link between this event and the dynamics of vorticity is evidenced by Figure2(right),which displays the wavelet map of the related vorticity signal.The higher frequency significant regions(≈730Hz) result strongly intermittent,with a bifurcation to lower and higher values than4average,followed by a drop of activity,in phase with the anomalous event. Figure1:Cross-Wavelet co-spectrum maps for axial and radial velocityfluctu-ations(left)and for axial velocity and mixture fractionfluctuations(right)at a given spatial point near the edge of the central jet.Figure2:Coherence functions for axial velocity and mixture fractionfluctuations (left)and wavelet map of vorticity time series(right).These few examples support the usefulness of the cross-wavelet analysis ap-proach to better investigate turbulent mixing processes in real systems. References[1]G.Buresti and G.Lombardi.Application of continuous wavelet transformsto the analysis of experimental turbulent velocity signals.Proc.of the1st Int.Symp.on Turb.Shear Flow Phen.,S.Barbara USA,Sept.1999.[2]EDF Direction des Etudes et Researches.1st A.S.C.F.Workshop Final Re-sults,Chatou,France,October1994.[3]S.Sello and rge eddy simulation of a bluffbody stabilisedflame.Proc.of the4th ETMM Int.Symp.,Ajaccio France,May1999. [4]M.V.Salvetti,G.Lombardi and F.Beux.Application of a wavelet cross-correlation technique to the analysis of mixing.AIAA Jour.,37:1007–1009, 1999.This figure "fig1a_sel.gif" is available in "gif" format from: /ps/physics/0003029v1This figure "fig1b_sel.gif" is available in "gif" format from: /ps/physics/0003029v1This figure "fig2a_sel.gif" is available in "gif" format from: /ps/physics/0003029v1This figure "fig2b_sel.gif" is available in "gif" format from: /ps/physics/0003029v1。