The COST 273 MIMO Channel Model Three Kinds of Clusters
FD-MIMO
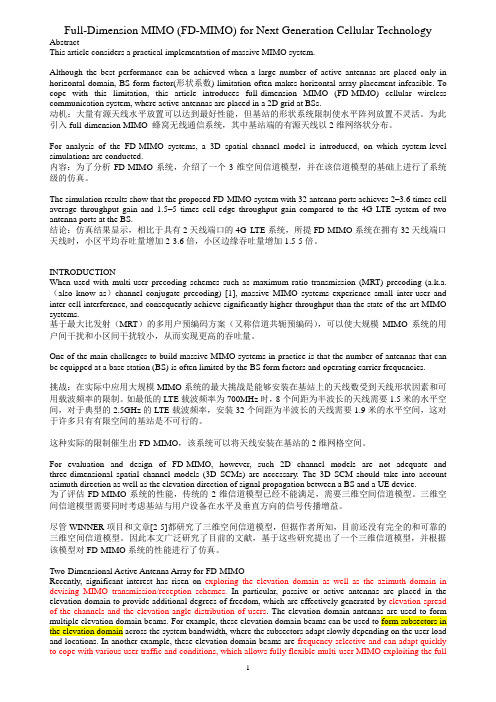
Full-Dimension MIMO (FD-MIMO) for Next Generation Cellular Technology AbstractThis article considers a practical implementation of massive MIMO system.Although the best performance can be achieved when a large number of active antennas are placed only in horizontal domain, BS form factor(形状系数) limitation often makes horizontal array placement infeasible. To cope with this limitation, this article introduces full-dimension MIMO (FD-MIMO) cellular wireless communication system, where active antennas are placed in a 2D grid at BSs.动机:大量有源天线水平放置可以达到最好性能,但基站的形状系统限制使水平阵列放置不灵活。
为此引入full-dimension MIMO 蜂窝无线通信系统,其中基站端的有源天线以2维网络状分布。
For analysis of the FD-MIMO systems, a 3D spatial channel model is introduced, on which system-level simulations are conducted.内容:为了分析FD-MIMO系统,介绍了一个3维空间信道模型,并在该信道模型的基础上进行了系统级的仿真。
The simulation results show that the proposed FD-MIMO system with 32 antenna ports achieves 2–3.6 times cell average throughput gain and 1.5–5 times cell edge throughput gain compared to the 4G LTE system of two antenna ports at the BS.结论:仿真结果显示,相比于具有2天线端口的4G LTE系统,所提FD-MIMO系统在拥有32天线端口天线时,小区平均吞吐量增加2-3.6倍,小区边缘吞吐量增加1.5-5倍。
基于相关矩阵的MIMO建模与仿真
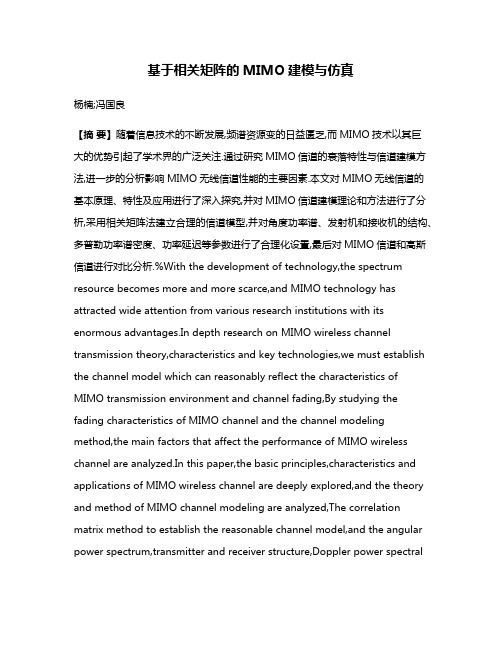
基于相关矩阵的MIMO建模与仿真杨楠;冯国良【摘要】随着信息技术的不断发展,频谱资源变的日益匮乏,而MIMO技术以其巨大的优势引起了学术界的广泛关注.通过研究MIMO信道的衰落特性与信道建模方法,进一步的分析影响MIMO无线信道性能的主要因素.本文对MIMO无线信道的基本原理、特性及应用进行了深入探究,并对MIMO信道建模理论和方法进行了分析,采用相关矩阵法建立合理的信道模型,并对角度功率谱、发射机和接收机的结构、多普勒功率谱密度、功率延迟等参数进行了合理化设置,最后对MIMO信道和高斯信道进行对比分析.%With the development of technology,the spectrum resource becomes more and more scarce,and MIMO technology has attracted wide attention from various research institutions with its enormous advantages.In depth research on MIMO wireless channel transmission theory,characteristics and key technologies,we must establish the channel model which can reasonably reflect the characteristics of MIMO transmission environment and channel fading,By studying the fading characteristics of MIMO channel and the channel modeling method,the main factors that affect the performance of MIMO wireless channel are analyzed.In this paper,the basic principles,characteristics and applications of MIMO wireless channel are deeply explored,and the theory and method of MIMO channel modeling are analyzed,The correlation matrix method to establish the reasonable channel model,and the angular power spectrum,transmitter and receiver structure,Doppler power spectraldensity,power delay and other parameters are set,finally carries on the contrast analysis to the MIMO channel and the Gaussian channel.【期刊名称】《电子设计工程》【年(卷),期】2017(025)024【总页数】4页(P74-77)【关键词】MIMO;相关矩阵;信道模型;传输环境【作者】杨楠;冯国良【作者单位】西安铁路职业技术学院陕西西安710014;西安铁路职业技术学院陕西西安710014【正文语种】中文【中图分类】TN915.9LTE系统采用多输入多输出(MIMO)技术用以应对系统对高容量、高速率和高移动性的需求。
毕业论文毕业设计任务书 (136)
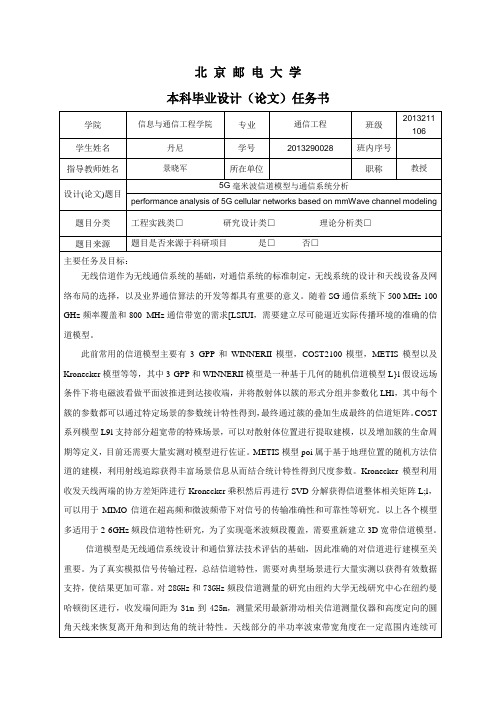
工程实践类□研究设计类□ 理论分析类□
题目来源
题目是否来源于科研项目是□否□
主要任务及目标:
无线信道作为无线通信系统的基础,对通信系统的标准制定,无线系统的设计和天线设备及网络布局的选择,以及业界通信算法的开发等都具有重要的意义。随着SG通信系统下500 MHz-100 GHz频率覆盖和800 MHz通信带宽的需求[LSIUI,需要建立尽可能逼近实际传播环境的准确的信道模型。
[8] 龚勇,景小宁,陈云翔,吕振中,张林. 基于多信号流图的飞控系统实时故障诊断[J]. 电光与控制. 2006(06)
[9] 肖勇,邵世煌. 分布式测控网络重构及其应用[J]. 北京邮电大学学报. 2009(06)
[10] 高鑫宇,刘冠军,邱静,李天梅,吕克洪. 基于模糊概率多信号流图的故障传播模型研究[J]. 测试技术学报. 2009(04)
[13] K. Yu, M. Bengtsson, B. Ottersten, D. McNamara, P. Karls-son, and M. Beach, "A wideband statistical model for NLOS indoor MIMO channels," in Proc. IEEE 55th Veh. TechnolConf. (VTC-Spring), vol. 1. May 2002, pp. 370-374.
[11]"Spatial Channel Model for Multiple Input Multiple Output(MIMO) Simulations," Tech. Rep. 3GPP 25.996 V12.0.0,Sept. 2014.
[12] P. Kyosti et al., "WINNER II channel models," European Commission, IST-WINNER, Tech. Rep. D1.1.2, Sept. 2007.
MIMO技术的简介
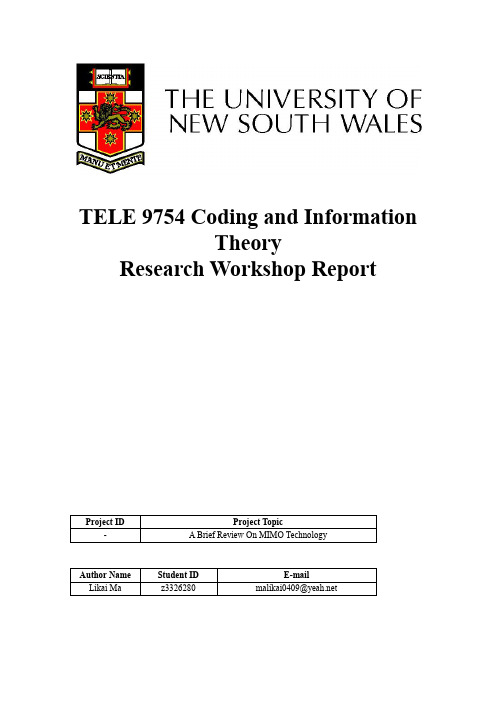
TELE 9754 Coding and InformationTheoryResearch Workshop ReportAbstract—Mobile wireless communication has become one of the most important aspects of our daily life. The continuously increasing usage has imposed great pressure upon telecommunication system where the availability of channel capacity and spectral resources are limited. Multiple Input Multiple Output (MIMO) is considered as one of the possible solutions to the above problem and has attracted considerable attention among researchers and engineers in the field of mobile communication due to the great advantages it exhibits. In recent years, MIMO technology has been developed into more sophisticated forms and utilized in some common communication devices around us. This report is intended to provide readers with a brief review of the historical and technological developments of MIMO, and its applications.I. INTRODUCTIONOur wireless communication systems have undergone remarkable developments and progresses in the past 20 years, from 1G to 4G and the upcoming 5G. Such systems have provided our life with significant conveniences which were otherwise impossible and unachievable before the 1980s. However, under the condition of limited bandwidth resources and channel capacity, the developing communication scheme is unable to meet the fast growing demand from users of mobile devices. In other words, our communication system has somewhat attained its bottleneck and needs some new technology to enhance its performance. On the other hand, MIMO equipped with modern efficient signal processing techniques and processing hardware demonstrates prominent characteristics that could be taken to mitigate the above problems. MIMO can be defined, in simple terms, as a system which consists of multiple antennas at both the transmitter and receiver sides [6]. A systematic diagram of MIMO is illustrated by Figure 1.Figure 1. Systematic diagram of a MIMO systemThe underlying fact which enables MIMO to attract intense attention is that it could exploit the advantages of beamforming gain, spatial diversity and spatial multiplexing to enhance the performance of a communication system without extra consumption of spectral resources.The content of this report is organized in six separate sections. Section II offers readers a set of abbreviations used throughout the report. Section III illustrates the historical developments and milestones of MIMO from theory to implementations. Section IV introduces, in general sense, how MIMO functions and achieves the aforementioned advantages. Section V categorizes MIMO into various classes based on the properties it composes and some comparisons among them would be made. Section VI provides some examples of application of MIMO in modern communication scheme. Finally, a brief conclusion will be drawn in Section VI. Additional information can be found by referring to the Appendix section.II. TABLE OF ABBREVIATIONSThe following table (Table 1) lists a set of commonlyA BRIEF REVIEW ON MIMO TECHNOLOGY AND ITS APPLICATIONSLikai Ma z3326280used abbreviations to which will be referred in the following sections of this report. Table 1. Table of abbreviations III. HISTORICAL DEVELOPMENT OF MIMO [1] The history of MIMO can be dated back several decades ago. Although the idea of MIMO was not proposed until the 1970s, antenna arrays, also known as smart antennas (illustrated in Figure 2) had been developed to take the advantage of diversity and enhance wireless transmission and reception in analogue communications. CLASSIFICATION OF MIMO Figure 2. An example of antenna array. The idea of MIMO was first conceived in the 1970s in Bell Laboratory, which was inspired by the desire to overcome the problem of bandwidth limitation and interference in transmission cables. Such idea was too difficult to be realized and had remained in the form of theory for a long period of time, due to the limitation that the processing hardware and signal processing algorithms available at that stage was unable to support MIMO signal processing. Nevertheless, the theory of MIMO had continued to be enriched by some of the early researchers ’, including A.R Kaye, D.A George, Branderburg, Wyner and W. Van. Etten. In the late 1980s, MIMO theory had further been developed by Jack Salz and Jack Winters whose work centralizedaround the idea of beamforming.The concept of SM was proposed in 1993 by Arogyaswami Paulraj and Thomas. In 1996, Greg Raleigh and Gerard J. Foschini further developed the approaches towards MIMO using co-located antennas at the transmitter. Significant breakthrough in practical application of MIMO did not take place until the late 1990s. In 1998, SM was first demonstrated in the formFigure 2.Timeline of development of MIMO.of prototype in Bell Lab. Since then, the development of MIMO had been accelerated and some products with such technology integrated started to be available commercially. In 2002, Iospan Wireless Inc. launch the first commercial product with MIMO embedded, which was a milestone in the real application of this technology. Later, in 2005, the first standard of WLAN (IEEE 802.11n), also commonly known as Wi-Fi, with MIMO-OFDM was produced by Airgo Networks and has become more and more popular since then. The more detailed historical development of MIMO is depicted as a timeline and can be found in Figure 2.IV. HOW DOES MIMO WORKThe underlying principle of MIMO is that signals transmitted and received at both the transmitter and receiver sides combine together so that either parallel data sub-streams are formed or SNR is improved [3]. The benefits that MIMO exploits are known as beamforming, spatial diversity and spatial multiplexing.Figure 3.Smith chart showing the technique of beamformingBeamforming is achieved by focusing energy in some desired angular direction through appropriate choice of antenna parameters [1, 2]. The Smith chart in Figure 3 illustrates the idea of beamforming where the main lob is pointing at a particular angular direction while the side lobes are significantly suppressed. When the channel between the transmitter and receiver are located within the range of LOS, MIMO can be configured to exploit the advantages of beamforming so that the antenna gains combine constructively and thereby an enhanced receiving power and SNR are attained in the link.When multiple copies of a signal are transmitted from the transmitter, they may subject to non-idealities in the communication channel, for example fading, reflection and refraction, to different extents. Multiple replicas of the signal incoming from different directions can be analyzed by employing some sophisticated DSP algorithms to recover the original transmitted signal if those signals are highly uncorrelated. Such technique is referred as spatial diversity [2]. In general, the more the extent of uncorrelation, the better the effect of spatial diversity. MIMO could also take the advantages of spatial diversity to improve the quality of the received signal (ie, increased SNR) and hence to provide a more reliable communication link.Figure 4. The MIMO channel capacity increases almost linearly with the number of transmitting or receiving antennas [5]In a fading channel, particularly Rayleigh fading with CSI known to the receiver, MIMO could form a number of parallel and independent sub-channels through which a code word can be divided into a number of pieces and transmitted separately [4, 5]. In other words, a higher transmission rate (channel capacity) could be achieved. In theoretical sense, the channel capacity increases approximately with the number of transmitting or receiving antennas, as depicted in Figure 4. This discovery has a tremendous implicationuponcommunication system, that higher information exchange rate can be achieved without consuming extra bandwidth, by introducing additional antennas at the transmitter and receiver sides. The benefits exploited by MIMO are summarized in the following table (Table 2).Table 2. Summary table of MIMO techniquesIn general, beamforming, spatial diversity and spatial multiplexing are three rivaling techniques that engineers should make appropriate decisions on what could be sacrificed in order to gain more advantages from the others. The inter-relations among these techniques are depicted in Figure 5 [2].Figure 5. Inter-relations among three MIMO techniquesAlthough they are rivaling factors, they are not necessarily mutually exclusive, meaning that by making appropriate decisions on to what extent those are used, one can design a communication scheme which employs a combination of those techniques such that certain degrees of advantages of them can be involved. Such decision should be based solely upon the specific engineering problem to be solved. V. V ARIOUS TYPES OF MIMOA MIMO system can be divided into different classes according to some specific criterion. A MIMO system is commonly classified according to the criterions that whether multiple users are able to be served simultaneously. The classifications is shown as in Figure 6.Figure 6. Classification of MIMOIn the case of multiple users, a MIMO system is referred as SU-MIMO if only a single user among them is served at a time. In contrary, the term MU-MIMO is defined for the case where multiple users can be served in parallel. The following figure (Figure 7) depicts a comparison between SU-MIMO and MU-MIMO.Figure 7. Comparison between SU-MIMO and MU-MIMO [7](A) SU-MIMO SYSTEM [7, 8]In SU-MIMO, the time-frequency resources are allocated entirely to a single user in a given communication session. If SM is employed, multiple sub-streams can then be created to scale up the channel capacity by the order dictated by the minimum of transmitting or receiving antennas. Different users can be served through the use of TDMA or FDMA.One can see that since the order of increases in channel capacity in SU-MIMO is limited by the transmitter or receiver side which consists of the smallest number of antennas, the improvements in channel throughput may be very limited, particularly for cellular communication networks. In other words, the user end would likely be the constraint on the enhancements of channel capacity. The number of antennas that can be integrated to the users’ mobile devices, such as mobile phones, is very limited, mainly due to limitations like portability and space availability.(B)MU-MIMO SYSTEM [7, 8]MU-MIMO can be considered as an extension to the theory of SU-MIMO. In a MU-MIMO system, multiple users can be served in parallel with the same time-frequency resources available. By exploiting the advantages of SM, the channel throughput for MU-MIMO can then be enhanced by the number of transmit antennas with sufficient number of users, namely a similar scaling principle carried by the case of SU-MIMO.As oppose to SU-MIMO, MU-MIMO better exploits the multiplexing gain provided by SM, which is achieved by allocating different users to different sub-channels. Different users can not only be served by employing TDMA or FDMA (in SU-MIMO), but also by means of SDMA. Therefore, MU-MIMO has more advantages over SU-MIMO in terms of time, frequency and spatial allocations.VI. APPLICATION OF MIMO IN MODERNCOMMUNICATION SCHEME [3]As the developments in both powerful signal processing hardware and more sophisticated MIMO models have become available in recent years, the application of MIMO in our modern communication systems have been made possible as oppose to the past, mainly by the ITU and 3GPP.Some of the common communication systems, including the 3G/4G network, Wi-Fi (IEEE 802.11n) and WiMAX have already integrated some MIMO technologies to a certain extent where various forms of MIMO have been deployed and different advantages are exploited. The use of MIMO technology in modern communication systems can be depicted by the following figure (Figure 8).Figure 8.Application of MIMO in modern communication systems.The current CDMA2000 standard, one of the 3G standards (WCDMA, CDMA2000 and TD-SCDMA) has adopted transmit diversity, while the WCDMA-based UMTS has also enabled implementation of transmit diversity and beamforming at base stations. Furthermore, the 3GPP LTE employs SU-MIMO with SM and STC. The more advanced version, so called 3GPP LTE-Advanced further extends from what has been designed in LTE and has involved MU-MIMO and multi-cell MIMO.In IEEE802.16 standard (also commonly known as WiMAX), MIMO-OFDMA, a technique that utilizes OFDM modulation scheme in combination with multiple antennas, has been deployed.IEEE802.11n or Wi-Fi is another commonly used communication standard and has implemented several MIMO technologies to enhance its data through put, channel capacity and overall performance. The techniques employed by Wi-Fi are mainly antenna selection, STC and beam forming. The following table (Table 3) provides a summary for the different MIMO technologies used in those communication schemes and their performance (data rate).Table 3. Summary of MIMO technologies in modern communication systems and their overall performances.VII. CONCLUSIONIn conclusion, the historical developments, classification and current applications associated with MIMO technologies have been outlined and reviewed in this report. It can be seen that MIMO has a great deal of advantages over other traditional communication technologies. MIMO can also be used in conjunction with other existing techniques including digital modulation (OFDM in particular), coding (STC, DPC and etc) and multiple access (TDMA and FDMA) in order to derive more powerful and efficient communication schemes and provide users with better communication quality. Although there still exits some compelling problems regarding the wide application of MIMO, one can see that such technology will be more extensively integrated in our future generation wireless communication systems.REFERENCE[1] Raut, Pravin W., and S. L. Badjate. "MIMO-Future Wireless Communication."[2] Sibille, Alain, Claude Oestges, and Alberto Zanella. MIMO: from theory to implementation. Academic Press, 2010.[3] Clerckx, Bruno, and Claude Oestges. MIMO Wireless Networks: Channels, Techniques and Standards for Multi-antenna, Multi-user and Multi-cell Systems. Academic Press, 2013.[4] Holter, Bengt. "On the capacity of the MIMO channel: A tutorial introduction."Proc. IEEE Norwegian Symposium on Signal Processing. 2001.[5] Liang, Yang Wen. "Ergodic and Outage Capacity of Narrowband MIMO Gaussian Channels." Dept. of Electrical and computer Engg. University of British Columbia, V ancouver, British Columbia (2005).[6] Telatar, Emre. "Capacity of Multi‐antenna Gaussian Channels." European transactions on telecommunications 10.6 (1999): 585-595.[7] Bauch, Gerhard, and Guido Dietl. "Multi-user MIMO for achieving IMT-Advanced requirements." Telecommunications, 2008. ICT 2008. International Conference on. IEEE, 2008.[8] Li, Qinghua, et al. "MIMO techniques in WiMAX andLTE: a feature overview."Communications Magazine, IEEE 48.5 (2010): 86-92.。
MIMO资料整理-2014

个平坦的窄带子信道,每个子信道上的信号带宽小于信道的相关带宽, 所以每个子信道上的频率选择性衰落可以看作是平坦性衰落。
• 而MIMO多天线技术能在不增加带宽的情况下,在每一个窄带平坦子信
道上获得更大的信道容量,可以成倍地提高通信系统的容量和频谱效率, 是一种利用空间资源换取频谱资源的技术。
Taking LTE MIMO from Standards to Starbucks Moray Rumney 30th April 2009
通过声音来理解预编码(Precoding)
• 为了使接收机侧的不同流更加隔离,
可以采用预编码码技术。
• 声音的例子中,Precoding可以看成对
立体声进行”balance”
MIMO+OFDM系统,通过在OFDM传输系统中采用天线阵列来实现空 间分集,以提高信号质量,是MIMO与OFDM相结合而产生的一种新 技术。它采用了时间、频率结合空间三种分集方法,使无线系统 对噪声、干扰、多径的容限大大增加。
LTE发送端信号流程
(0) (0) a0 , a1 ,..., a(0) A1
LTE 下行MIMO简介
2014-10
常见问题
1. 什么是MIMO? 2. LTE中为什么使用MIMO? 3. MIMO的如何理解和解释?
4. MIMO如何作用?
5. MIMO模式如何区分? 6. MIMO中的基本概念和作用过程? 7. MIMO各模式的特点如何? 8. MIMO对性能的影响如何?
Taking LTE MIMO from Standards to Starbucks Moray Rumney 30th April 2009
面向汽车的MIMO OTA测试技术

专题:移动通信(5G )测试面向汽车的MIMO OTA 测试技术李雷1,魏贵明1,姜国凯2,冯家煦2,吴翔1(1.中国信息通信研究院,北京 100191;2.中国汽车技术研究中心有限公司,天津 300300)摘 要:汽车智能网联是当前交通产业发展的主要方向之一。
通过汽车与周围车辆、行人、交通设施、蜂窝网络进行信息交互,提升交通网决策管理智能化水平,改善道路安全与效率。
如何定量精确地评估整车通信性能是业界关注的热点问题,然而目前尚缺少成熟的解决方案,特别是面向整车产品。
主要研究了基于多探头吸波暗室(multi-probe anechoic chamber ,MPAC )的汽车空口测量系统的搭建方法,并针对整车空口测试提出了一种低成本解决方案,可以在信道模拟器数字通路受限的情况下,通过数字变换,成倍拓展测试区域。
数字仿真结果表明,基于此方案所构造的测试区域,其空间相关性、时间相关性均满足相关标准要求。
关键词:汽车空口测试;多探头吸波暗室;空间相关性;时间相关性 中图分类号:TN929.5 文献标识码:Adoi : 10.11959/j.issn.1000−0801.2021040MIMO OTA performance testing of vehiclesLI Lei 1, WEI Guiming 1, JIANG Guokai 2, FENG Jiaxu 2, WU Xiang 11. China Academy of Information and Communications Technology, Beijing 100191, China2. China Automotive Technology and Research Center Co., Ltd., Tianjin 300300, ChinaAbstract: Intelligent traffic system is one of promising technologies for traffic industry. Based on communication among cars, pedestrian, traffic infrastructure and cellular networks, it’s able to significantly promote on road safety and efficiency by making proper traffic instructions. How to precisely evaluate the communication performance of a whole vehicle become a hot-topic in the field. Unfortunately, there is still no solution about the setup and procedures for the OTA testing. A low-cost solution was proposed by reconstructing channel models and optimizing the existing testing system framework that referred to as multi-probe anechoic chamber. The proposed method was able to mul-tiple the test area with limited channel emulator RF channels by introducing a low-cost digital converter. Numerical simulations indicate that the proposed method has the ability to reproduce spatial and temporal correlation features of the target channel model, which is coherent with related standards’ requirements.Key words: OTA testing for vehicles, multi-probe anechoic chamber, spatial correlation, temporal correlation1 引言多输入多输出(multi-input multi-output ,MIMO )通过在收发两端配置多路天线,基于合适的空时编码技术,可以在相同的时频资源上传输多流数据,从而成倍地提升系统的频谱效率,收稿日期:2020−07−29;修回日期:2021−02−18 通信作者:魏贵明,*******************.cn·33·电信科学 2021年第2期是长期演进(long term evolution,LTE)和新空口(new radio,NR)接入网络的核心物理层技术之一。
透镜天线
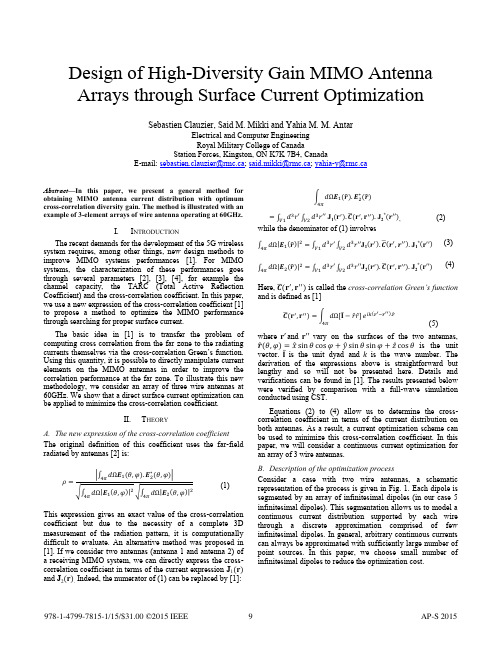
Design of High-Diversity Gain MIMO Antenna Arrays through Surface Current OptimizationSebastien Clauzier, Said M. Mikki and Yahia M. M. AntarElectrical and Computer EngineeringRoyal Military College of CanadaStation Forces, Kingston, ON K7K 7B4, CanadaE-mail: sebastien.clauzier@rmc.ca; said.mikki@rmc.ca; yahia-y@rmc.caAbstract—In this paper, we present a general method forobtaining MIMO antenna current distribution with optimumcross-correlation diversity gain. The method is illustrated with anexample of 3-element arrays of wire antenna operating at 60GHz.I.I NTRODUCTIONThe recent demands for the development of the 5G wirelesssystem requires, among other things, new design methods toimprove MIMO systems performances [1]. For MIMOsystems, the characterization of these performances goesthrough several parameters [2], [3], [4], for example thechannel capacity, the TARC (Total Active ReflectionCoefficient) and the cross-correlation coefficient. In this paper,we use a new expression of the cross-correlation coefficient [1]to propose a method to optimize the MIMO performancethrough searching for proper surface current.The basic idea in [1] is to transfer the problem ofcomputing cross correlation from the far zone to the radiatingcurrents themselves via the cross-correlation Green’s function.Using this quantity, it is possible to directly manipulate currentelements on the MIMO antennas in order to improve thecorrelation performance at the far zone. To illustrate this newmethodology, we consider an array of three wire antennas at60GHz. We show that a direct surface current optimization canbe applied to minimize the cross-correlation coefficient.II.T HEORYA.The new expression of the cross-correlation coefficientThe original definition of this coefficient uses the far-fieldradiated by antennas [2] is:ρ=|∫dΩE1(θ,φ).E∗(θ,φ)4π|√∫dΩ|E1(θ,φ)|24π√∫dΩ|E2(θ,φ)|24π(1)This expression gives an exact value of the cross-correlation coefficient but due to the necessity of a complete 3D measurement of the radiation pattern, it is computationally difficult to evaluate. An alternative method was proposed in[1]. If we consider two antennas (antenna 1 and antenna 2) ofa receiving MIMO system, we can directly express the cross-correlation coefficient in terms of the current expression J1(r) and J2(r). Indeed, the numerator of (1) can be replaced by [1]:∫dΩE1(r̂).E2∗(r̂)4π=∫d3r′∫d3r′′V2V1J1(r′).C̅(r′,r′′). J2*(r′′),(2) while the denominator of (1) involves∫dΩ|E1(r̂)|24π=∫d3r′∫d3r′′V2V1J1(r′).C̅(r′,r′′). J1*(r′′) (3)∫dΩ|E2(r̂)|24π=∫d3r′∫d3r′′V2V1J2(r′).C̅(r′,r′′). J2*(r′′)(4)Here, C̅(r′,r′′) is called the cross-correlation Green’s function and is defined as [1]C̅(r′,r′′)=∫dΩ[I−r̂r]4πe ik(r′−r′′).r̂(5)where r′and r′′ vary on the surfaces of the two antennas, r̂(θ,φ)=x̂sinθcosφ+ŷsinθsinφ+ẑcosθ is the unit vector. I is the unit dyad and k is the wave number. The derivation of the expressions above is straightforward but lengthy and so will not be presented here. Details and verifications can be found in [1]. The results presented below were verified by comparison with a full-wave simulation conducted using CST.Equations (2) to (4) allow us to determine the cross-correlation coefficient in terms of the current distribution on both antennas. As a result, a current optimization scheme can be used to minimize this cross-correlation coefficient. In this paper, we will consider a continuous current optimization for an array of 3 wire antennas.B.Description of the optimization processConsider a case with two wire antennas, a schematic representation of the process is given in Fig. 1. Each dipole is segmented by an array of infinitesimal dipoles (in our case 5 infinitesimal dipoles). This segmentation allows us to model a continuous current distribution supported by each wire through a discrete approximation comprised of few infinitesimal dipoles. In general, arbitrary continuous currents can always be approximated with sufficiently large number of point sources. In this paper, we choose small number of infinitesimal dipoles to reduce the optimization cost.9978-1-4799-7815-1/15/$31.00 ©2015 IEEE AP-S 2015Fig. 1. Schematic representation of the segmentation of thewire antennas by an array of infinitesimal dipoles. Each infinitesimal dipole will be associated with a complex moment corresponding to the amplitude and the phase of the continuous current on the wire. Then, an optimization program (genetic algorithm) will update these two parameters on each dipole in order to minimize the cross-correlation coefficient calculated with the formula belowρIJ =∑∑ρInJm Nn=1M m=1(6)where M and N are, respectively, the number of infinitesimal dipoles considered for the dipole I and the dipole J (Here chosen both to be 5). ρInJm is the cross-correlation between the n tℎ infinitesimal dipole of the wire I and the m tℎ infinitesimal dipole of the wire J.III. E XAMPLE OF AN ARRAY OF 3 WIRE ANTENNAS AT60GH Z We apply the design process described above to an array of three half-wave dipole antennas at 60GHz (Figure 2). The genetic algorithm is used to optimize the amplitude and the phase of each infinitesimal dipole (segment on the three wire antennas).Fig. 3 shows the variation of the cross-correlation during the optimization process. We notice that after 100 iterations, we have reached a cross-correlation coefficient equal to 0.35. Fig. 4 shows the optimized amplitude and phase of the current on each dipole. If we compare the diversity gains, calculated through the formulation exposed in [1], before and after the optimization, we obtain with a non-optimized current a diversity gain of 0.41, while after optimization with the current represented in Fig. 4, a diversity gain of 0.8 is achieved. Therefore, proper choice of the current allows us to multiply by almost 2 the diversity gain.The numerical examples tried by the authors suggest that phase variations on radiating currents are more important for diversity gain performance than the amplitude distribution. Therefore, linear wires with special currents can significantly boast up the performance of MIMO systems. Realizations of these currents can be attained by engineering the surface impedance boundary condition on specially coated wires or by direct implementation of arrays of electrically-small antennas to mimic the continuous current obtained by our method.Fig. 2. Schematic representation of an array of three wireantennas.Fig. 3. Schematic representation of an array of three wireantennas.(a) (b)Fig. 4. Optimized amplitude (a) and phase (b) distribution onthe 3 half-wave dipoles.IV. C ONCLUSIONIn this paper, it was shown that by careful manipulation of the amplitude and phase of the radiating current, it is possible to minimize the cross-correlation coefficient (and therefore maximizing the diversity gain) in arbitrary MIMO system antenna arrays. The method was outlined and applied successfully to three half-wavelength dipole antennas.R EFERENCES[1] S. Mikki and Y. Antar, ‘On cross correlation in antenna arrays withapplications to spatial diversity and MIMO systems,’ submitted to IEEE Transactions on Antennas and Propagation,, 2014.[2] M.S. Sharawi, ‘Printed MIMO antenna system: Performance Metrics,Implementations and Challenges,’ Forum for Electromagnetic Research Methods and Application Technologies (FERMAT), 2014.[3] W. C. Jakes et al, Microwave Mobile Communications , John Wiley &Sons, 1974.[4] Nelson Costa and Simon Haykin, Multiple-Input Multiple OutputChannel Models , John Wiley & Sons, 2010.10。
LTE系统的MIMO信道建模与仿真
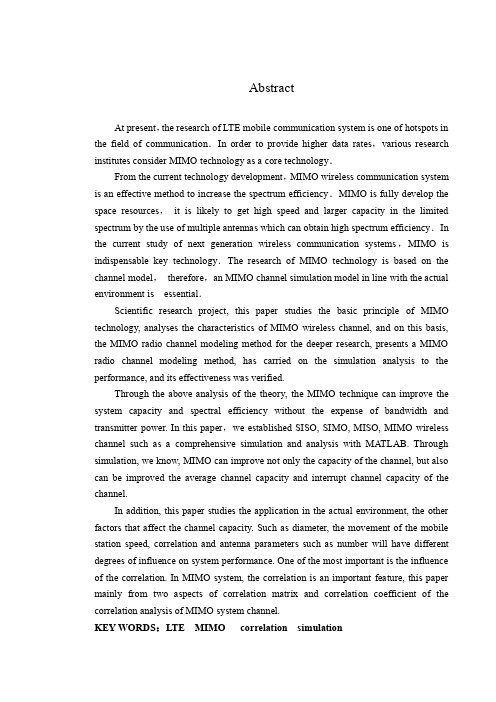
indispensable key technology.The research of MIMO technology is based on the
mainly from two aspects of correlation matrix and correlation coefficient of the
correlation analysis of MIMO system channel.
KEY WORDS:LTE
MIMO
correlation simulation
radio channel modeling method, has carried on the simulation analysis to the
performance, and its effectiveness was verified.
Through the above analysis of the theory, the MIMO technique can improve the
3.3 信道衰落 ....................................................................................................... 14
3.3.1 小尺度衰落特性 ............................................................................... 14
can be improved the average channel capacity and interrupt channel capacity of the
MIMO信道的三维互相关模型及其相关特性分析
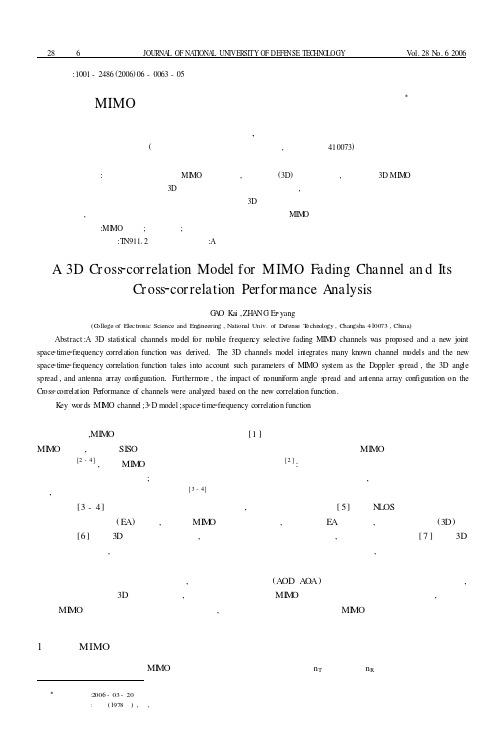
文章编号:1001-2486(2006)06-0063-05MIMO 信道的三维互相关模型及其相关特性分析Ξ高 凯,张尔扬(国防科技大学电子科学与工程学院,湖南长沙 410073)摘 要:针对频率选择性衰落MI MO 移动信道,建立三维(3D )纯随机模型,并推导出3D MIM O 信道模型的联合空时频相关函数。
新的3D 模型统一了现有的多种信道模型,新的联合空时相关函数综合考虑了信道收发两端的多普勒扩展、信号到达方向与离开方向的3D 非均匀扩展与两端天线阵的配置。
最后基于新的相关函数,分析了非均匀角度扩展参数以及阵元配置参数的变化对MIM O 信道相关特性的影响。
关键词:MI MO 信道;三维模型;空时频相关函数中图分类号:T N91112 文献标识码:AA 3D Cr oss 2cor relation Model for MIMO Fading Channel an d ItsCr oss 2cor relation Perfor mance A nalysisG A O K ai ,ZH A NG Er 2yang(C ollege of Electronic Science and Engineering ,National Univ.of De fens e T echnology ,C hangsha 410073,China)Abstract :A 3D statis tical channels m odel for m ob ile frequen cy selective fading MIM O channels w as prop osed and a new joint space 2time 2frequency correlation function w as derived.T he 3D channels model in tegrates many kn own channel m odels and the new space 2time 2frequency correlation function tak es into account such parameters o f MIMO sys tem as the D oppler s pread ,the 3D ang le spread ,and antenna array con figuration.Furth erm ore ,the impact of n onuni form angle s pread and an tenna array con figuration on the Cross 2correlation Performance o f channels w ere analy zed bas ed on the new correlation function.K ey w or ds :MI MO channel ;32D model ;space 2time 2frequency correlation function近年来,MI MO 系统得到了广泛的关注。
无线通信工程中的MIMO系统应用与性能分析
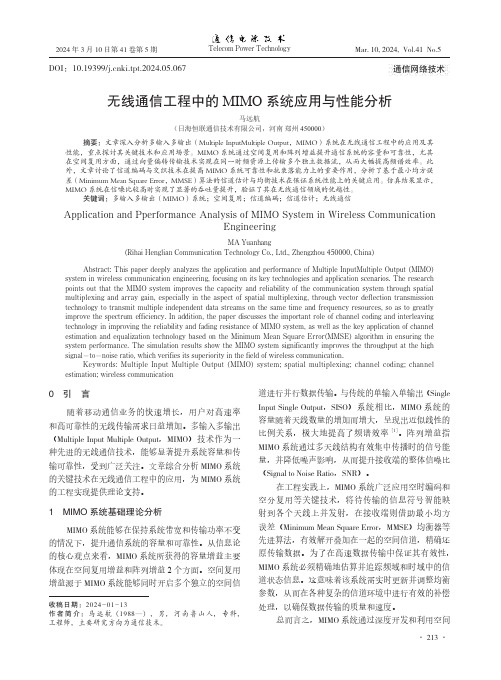
通信网络技术无线通信工程中的MIMO系统应用与性能分析马远航(日海恒联通信技术有限公司,河南郑州文章深入分析多输入多输出(Multiple InputMultiple Output,MIMO)系统在无线通信工程中的应用及其性能,重点探讨其关键技术和应用场景。
MIMO系统通过空间复用和阵列增益提升通信系统的容量和可靠性,尤其在空间复用方面,通过向量偏转传输技术实现在同一时频资源上传输多个独立数据流,从而大幅提高频谱效率。
此MIMO系统可靠性和抗衰落能力上的重要作用,分析了基于最小均方误差(Minimum Mean Square Error,MMSE)算法的信道估计与均衡技术在保证系统性能上的关键应用。
仿真结果显示,系统在信噪比较高时实现了显著的吞吐量提升,验证了其在无线通信领域的优越性。
多输入多输出(MIMO)系统;空间复用;信道编码;信道估计;无线通信Application and Pperformance Analysis of MIMO System in Wireless CommunicationEngineeringMA Yuanhang(Rihai Henglian Communication Technology Co., Ltd., Zhengzhou维度资源,扩大了通信系统容量,提升了通信系统可靠性,成为现代无线通信技术进步的重要支撑力之一。
系统关键技术分析实验室提出的向量偏转传输技术,系统的空间复用,从而获得多径增益[2]。
个天线看作一个发射向量空间,个天线看作一个接收向量空间,通过个正交基矢量,并根据信的奇异值进行分解,得到发射端和接。
经过预编码矩阵V变换个正交的个不同的数据流且不发生的严格要求。
2.3 信道估计与均衡为跟踪间的快速时变信道,需要进行准确可靠的信道估计。
本设计采用基于训练序列的据传输之前,发送已知的训练序列,接收端获得经信道冲激响应的序列。
接收序列为式中:N为提高估计准确性,训练序列之间采用循环移位设计,接收端收集多个传输块的训练序列进行联合信道估计。
suppercell

Coordinated Multipoint Transmission Systems with the Clustered Super-cell Structure ConfigurationXIAO Shang-hui †, ‡, ZHANG Zhong-pei †† National Key Lab of Commun., University of Electronic Science and Technology of China, Chengdu, 610054, P.R. of China ‡ Department of Physics & Electronic Engineering, Yibin University, Sichuan, 644007, P.R. of ChinaE-mail: xiaosh@; zhangzp@Abstract—Coordinated multipoint transmission and reception technologies are considered as good candidates for improving system capacity and cell edge experience to meet the requirements set by LTE-Advanced. This paper explicitly discusses the clustered super-cell multipoint cooperation transmission structures with the optimal precoding design. Some analysis results show that a clustered super-cell is a reasonable choice for clustered coordination with the given transmit power.Keywords-Network multiple input-multiple output (MIMO); Coordinated multipoint transmission(Co-MP); Clustered; SupercellI.I NTRODUCTIONRecently, there has been extension work on wireless systems with multiple transmit and receive antennas (known as MIMO) combined with advancing coding and signal processing. For the scattering fading channels, it has been demonstrated [1]-[5] that MIMO systems yield considerable gain in power (range) and/or data rate (bit/sec/Hz) in a given bandwidth, when compared to wireless systems with a single transmit and a single receive antenna.However, achieving the predicted enormous capacity gain in realistic cellular multi-user MIMO networks could be problematic. For realistic cellular systems, the sharing of common system resources by multiple users and the frequency reuse among adjacent cells will bring in co-channel interference (CCI), which may greatly diminish the advantages of MIMO systems [6]. In the conventional cellular systems, CCI is treated as background noise, and reduced by careful radio resource management techniques such as power control, frequency reuse, and spreading code assignments [7]. However, these techniques are all implemented at single cell without inter-cell cooperation on the physical layer, and limit the achievable spectral efficiency gain and/or lead to insufficiency suppression to CCI.This paper mainly discusses the clustered super-cell multipoint cooperation transmission system with the optimal precoding design, where multiple cells will perform joint signal processing for transmission to multiple users, and accordingly the systems are able to efficiently mitigate inter-cell CCI interference, and significantly achieve spectral efficiently gain. II.C OORDINATED MULTIPOINT TRANSMISSION SYSTEMS Already in current cellular networks, geographically dispersed multiple antennas connected to a central baseband processing unit are used as a cost-efficient way of building networks [8], which can provide improved resistance to shadowing and extended range. Such structures open up new transmission strategies. Recently, In [9], it has been shown that coordinated multipoint (Co-MP) transmission and reception technology, in which multiple different base stations (BSs) or radio access points (RAPs) together transmit signal for different mobile user equipments (UEs) as shown in figure 1, accordingly the inter-cell CCI interference mitigation becomes easy to implement, can significantly improve the system performance, especially for the spectral efficiency of cell-edge user over the cell. Several radio access points (if possible, including some remote radio units/sites, i.e. RRU/sites) are linked to one enhanced NodeB (eNodeB) by means of radio over wires/fibers, whereas each access point may contain oneor multiple antenna elements.Fig.1 System setup of coordinated multipoint transmission and reception For the uplink (UL), it supports for joint processing of signals received at multiple geographically separated points; otherwise, in the downlink (DL), it supports dynamic coordination in the scheduling/transmission, including joint transmission, from multiple geographically separated points. Co-MP is a cooperative transmission and reception technology, which can be easily deployed in a semi-distributed communication system with distributed antennas but centralized control functionally. Multiple UEs can be served by978-1-4244-3693-4/09/$25.00 ©2009 IEEEone or multiple access points of the same or different eNodeBs simultaneously. The coordinated central controllers retrieve information from distributed access points and allocate resources to satisfy the QoS requirements of the UEs while maximizing the network performance.III.M ATHEMATICAL ANALYSIS MODEL OF THE CLUSTERED MULTIPOINT COOPERATION SYSTEMSA.Clustered Super-cell System StructureFor a large Co-MP network with great number of cells, it is impractical to do coordination across over all the RAPs. We may divide the Co-MP network into a number of the clustered super cells, where each super-cell contains a group of adjacent cells. The antennas of all the cells in the super-cell participating in cooperation can be considered as a virtual antenna array within a “virtual cell”. With coordination among the RAPs with the same clustered supercell, we may effectively increase the number of spatial degree of freedom, which will be used to suppress interference, including intra-supercell and inter-supercell interference, and provide sum rate gain. Suppose in general that there are M mobile users arbitrarily distributed in the downlink coordinated multipoint transmission super-cell system, with N t the number of transmit antennas at each RAP, and N r the number of receiver antennas at each UE, respectively. Suppose that N p is the total number of adjacent RAPs in the super-cell system, so (N t, N r, N p, M) can be used to represent the overall systems. As illustrated in Fig. 2, the three cooperative RAPs together transmit L j data streams to UE j.Fig. 2 System analytical model for multi-cell in intra-eNodeB coordination The different links are independent and undergo frequency-flat Rayleigh fading. Therefore, H pj, the baseband matrix representation of the channel from RAP p to UE j, has complex Gaussian elements. τpj denotes the propagation delay from RAP p to UE j. For any UE, the multiple RAPs cooperate and jointly transmit the signals intended for it. The transmit vector for UE j from RAP p is linearly precoded by the N t×L j matrix T pj as X pj (m)= T pj S j(m), where S j (m) denotes the zero-mean data vector, of size L j×1 at time m, meant for UE j.B.System Received Signal ModelAssume that the timing-advance mechanisms can ensure that the desired signals for an UE that are transmitted from multiple RAPs reach the UE at exactly the same time. When the asynchronous nature of interference is neglected, the N r×1 received signal vector model at the j th user in the super-cell c can be represented as follows()()()()()()()()(()()()()()()__,_,_1_,_,_11__,_,_111_pppNc j c jc p j c p jpN Mc kjc p j c p kp kk jNC Mc lj c jc p j c p lc p lc cc jm mmm mm m m m===≠===+++=+++∑∑∑∑∑∑r H T XH T iH T i nD P Q n""(1)where D(m) is desired signal vector, P(m) is the intra-supercell interference signal vector, Q(m) is the inter-supercell interference signal vector. X c_j is the L j×1 transmitted vector for UE j in the clustered super-cell c (C denotes total number of the clustered super-cell). H(c,p)_j is the N r×N t channel matrix from RAP p in the clustered super-cell c to UE j. T(c,p)_j is the N t×L j precoding matrix for UE j at the p th RAP in the clustered super-cell c. n c_j(m) is the additive white Gaussian noise at UE j in the clustered super-cell c, with zero mean and variance()()*2__rc j c j Nm mσ⎡⎤Ε=⎣⎦n n I (2) where (·)* denotes the conjugate transpose of a matrix and I n denotes the n×n identity matrix.As clearly seen from (1), the following constraint holds in general()min,j r p tL N N N≤ (3) Consequently, if N r<N p N t (which is more likely in practice), the excess dimensions simply serve to offer selection diversity gain. In addition, coordinated network MIMO cannot increase the system peak data rate of any of the cells in the super-cell unless N r>min (N t).Because all the RAPs within the same cluster structure coordinate to work as a super-cell, the signal model can be written as()()()() ____1____11Mc j c j c k c kjkC Mc j c l c lj c jc lc cm mm m===≠=++∑∑∑r H T XH T X n(4)where()()()_,1_,2_,_,,,pc j c j c j c N j⎡⎤=⎢⎥⎣⎦H H H H" is the N r×N p N t aggregate channel transfer matrix from the super–cell c to UE j. and()()()****_,1_,2_,_,,,pc j c j c j c N j⎡⎤=⎢⎥⎣⎦T T T T"is the aggregate transmit precoder for UE j over all N p RAPs. Unlike the traditional downlink with co-located MIMO channels, the channel gains from any two antennas at thedifferent RAPs are guaranteed to be independent.Denote ()()()_____11C Mc jc jc l c ljc jc l c cm m m ==+∑∑z H T X nasthe sum of the noise and interference from other clustered super-cell, the covariance matrix of which is2***_______112**_____11r r CMc j N c j c l c l c l c l c jc l c c CM N c j c l c l c l c jc l c cE σσ==≠=⎡⎤=+⎣⎦=+∑∑∑∑R I H T X X T H I H T Q T H (5)where *___c l c l c l E ⎡⎤=⎣⎦Q X X is the covariance matrix of X c _l . This covariance matrix can be estimated at mobile UEs by various methods, including the usage of silent period of the desired signal [10], the usage of pilot signal [11] and blind estimation [12] according to multiple access strategies. After such estimation, each UE user will feed back it to RAPs, which will be used to design precoding matrix. Accordingly, we can assume that the covariance matrix of the interference plus noise is perfectly known at the UEs and RAPs in the same eNodeB. C. Clustered System Cooperation StrategiesDepending on the area of multipoint coordination, the coordinated multipoint transmission can be classified into the followings: Coordination everywhere in the cell and Coordination only at the cell edge. If coordinated multipoint transmission happens for all UEs in the cell regardless of the UE position, it may provide a better performance at the expense of UE feedback and backhaul overhead compared with the coordination only at the cell edge [13]. On the other hand, the single-cell transmission is used for UEs in the cell-center while multipoint coordination transmission only applies to UEs at the cell-edge. This approach may provide a good compromise between performance and reduction in the UE feedback and backhaul overhead. Note that the single-cell transmission for cell-center UEs can be achieved by designating only the home cell as the active cell.A group antenna units to serve a Co-MP configured UE is defined as e.g. the clustered super-cell. It is more easily implemented for a super cell to be composed of antenna units only under the same eNodeB than of antenna units belonging to different eNodeBs. The super-cell can be constructed in a UE-specific manner or can have one universal layout applied to all UEs. In the UE-specific super-cell construction, the coordination set of cells for a certain UE depends on the UE position. As the UE moves around cells, a set of cells for coordination is dynamically chosen from among cells near the UE, which can be done through the UE measurement reports. The UE-specific dynamic super-cell creation results in overlapping of supercells of different UEs, hence resulting in an eNodeB belonging to more than one supercell. In the universal creation the layout of super-cells is the same for all UEs regardless of the UE position. For a given UE belonging to a super-cell, it may be beneficial to designate a set of cellswhich are participating in the actual transmission for the UE. The cells in actual transmission to a UE are called active cells for the UE. The active cells can be defined from the UE perspective based on signal strengths from the cells (normally cells with strong signal strength are chosen among cells within the super-cell). The designation of active cells can reduce the UE feedback overhead and the backhaul load due to unnecessary coordination among cells.IV. S YSTEM PRECODING MATRIX DESIGNFor the codebook-based precoding method, two different design approaches exist, i.e. per-cell separation design and joint design. In the per-cell codebook design approach, the codebook consists of precoding matrices of constant size regardless of the number of coordinating cells. Assuming that each cell has N t transmit antennas, precoding for the clustered multi-cell MIMO with γ cells in coordination can be described by an (γN t ×γN t ) matrix, which is comprised of γ sub-matrices with size (N t ×γN t ) each describing the precoding for thecorresponding cell, as shown in Fig. 3(a).Fig. 3 Precoding matrix for (a) per-cell and (b) joint precoding in clusteredsuper-cell systemsThe set of sub-precoding matrices for a cell, i.e., the codebook can be designed to be identical between different cells and the codebook for single-cell transmission can be reused for simplicity. The UE may estimate preferred precoding for transmission from individual cells. The same CQI/PMI/RI report format for single-cell transmission can be reused (or with a small modification) for multi-cell coordinated multipoints transmissions (e.g., report over multiple instances to take into account transmission from multiple cells). In the joint-codebook design approach, precoding matrices are designed by considering jointly all the available transmit antennas in the coordination cells set. Assuming that each cell has N t transmit antennas, precoding matrices are of size (γN t ×γN t ) as shown in Fig. 3(b). In this case, the single “super-codebook” is designed separately for all possible cases with a different number of transmit antennas. In view of frequency reuse, the coordinated multi-cell transmission incurs “loss” of frequency resources available per cell since resources for multiple cells are now all shared as aco-channel between multiple cells. To overcome the spectrum loss incurred by the multi-cell coordinated transmission, we may need to fully utilize additional spatial-domain degree of freedom made available by multiple points transmission/reception and accommodate multiple UEs in the same frequency band simultaneously.V.N UMERICAL SIMULATION ANALYSISIf the clustered super-cell size is small, there will be too many super-cell edge users which will consume lots of degreeof freedom and lower the effective sum rate. However, the requirement of full CSI and synchronization will prohibit a very large super-cell size, and due to path loss the users benefit little from those RAPs far away. Therefore, to select a suitable super-cell size is important for practical systems. Figure 4 shows the effective sum rates per cell for differentsuper-cell size.Fig.4 Effective sum rate per-cell for different super-cell sizeAs illustrated in Fig. 4, we can see that the 3-cell cluster hasa much higher sum rate than the 1-cell cluster, and a 7-cell cluster has a rate gain about 2.5 bit/sec/Hz over a 3-cell cluster, while from 7-cell to 19-cell cluster the sum rate increases about 1bit/sec/Hz. The lower sum rate for 1-cell is due to its relative large edge area. Therefore, a 7-cell clustered super-cell can already achieve a significant part of the performance gain of the clustered super-cell coordination.Also, the CSI requirement for clustered super-cell coordination is on a super-cell scale, which is greatly reduced compared to global coordination. With C clustered super-cells and N p cells each cluster, totally there are CN p RAPs in the MIMO network. For global coordination, the effective channel matrix for each user is N r×CN p N t, while for clustered coordination it is N r×N p N t. For 7-cell cluster system, the amount of CSI feedback is only 7/19 of that for a 19-cell cluster system, while the performance of the 7-cell cluster system does not degrade much as shown in Fig. 4, so a clustered super-cell size of 7 is a reasonable choice for clustered coordination with the given transmit power.VI.C ONCLUSIONIn this contribution, we discussed some approaches forcooperation of multiple points using the clustered supercell techniques, namely the antennas of all the cells in the super-cell participating in cooperation as a virtual antenna array within a “virtual cell”. Different clustering schemes for defining cooperating cells were suggested to limit the complexity of scheduling and changes to the network architecture. For the case when the cooperating cells are co-located cells, distributed antenna systems, or remote radio units/sites (RRUs/sites), belonging to the same eNodeB, i.e., Intra-eNodeB cases, we believe that joint processing techniques are practically feasible. The requirements for Inter-eNodeB techniques are more stringent. Backhaul requirements in terms of capacity and latency, network architecture changes and scheduler complexity are some of the issues that should be addressed for enabling Inter-eNodeB techniques.A CKNOWLEDGMENTThis work is supported by National High Technology Research and Development Plans (the “863” Projects) of China (No. 2006AA01Z269, 2007AA01Z299).R EFERENCES[1]H. Jafarkhani, Space-Time Coding: Theory and Practice. NewYork:Cambridge, 2005.[2]G. J. Foschini and M. Gans, On limits of wireless communications in afading environment when using multiple antennas, Wireless Personal Communications, vol. 6, pp. 311–335, Mar. 1998.[3]V. Tarokh, N. Seshadri, and A. R. Calderbank, Space-time codes forhigh data rate wireless communication: Performance criterion and code construction, IEEE Trans. Inform. Theory, pp. 744–765, Mar. 1998[4]S. M. Alamouti, A simple transmitter diversity scheme for wirelesscommunications, IEEE J. Select. Areas Commun., pp. 1451–1458, Oct.1998.[5]I. E. Telatar, Capacity of multi-antenna Gaussian channels, Eur. Trans.Telecom., vol. 10, pp. 585–595, Nov. 1999.[6]S. Catreux, P. E. Driessen and L. J. Greenstein, Simulation results for aninterference-limited multiple-input multiple-output cellular system, IEEE Commun. Lett., vol. 4, no.11, pp.334-336, Nov. 2000.[7] A. F. Molisch, Wireless Communications. New York: Wiley-IEEEPress, 2005..[8]Haralabos C. Papadopoulos, Carl-Erik W. Sundberg, Space-Time Codesfor MIMO Systems with Non-Collocated Transmit Antennas , IEEE J.Select. Areas Commun., vol. 26, no.6 pp. 927-937, Aug. 2008[9]REV-080030, LTE-Advanced technology components, Ericsson,Shenzhen, China, April 7-8, 2008.[10]M. L. Honig, U. Madhow, and S. Verdu, Blind adaptive multiuserdetection, IEEE Trans. Inform. Theory, vol. 41, pp. 944–960, Jul. 1995 [11]R1-082501, Collaborative MIMO for LTE-Advanced downlink, AlcatelShanghai Bell, Alcatel Lucent, Warsaw, Poland, June 30-July 4, 2008. [12] D. A. Pados and S. N. Batalama, Joint space time auxiliary-vectorfiltering for DS/CDMA systems with antenna arrays, IEEE Trans.Commun., vol. 47, pp. 1406–1415, Sept. 1999.[13] A. Kansal, S. N. Batalama, and D. A. Pados, Adaptive maximum SINRRAKE filtering for DS-CDMA multipath fading channels, IEEE J.Select. Areas Commun., vol. 16, pp. 1765–1773, Dec. 1998.。
多输入多输出通信系统的信道模型及容量

……………………………………………………………精品资料推荐…………………………………………………多输入多输出通信系统的信道模型及容量专业:通信工程摘要随着无线通信事业的迅速发展,用户对无线通信的速率和服务质量提出了越来越高的要求。
然而频谱资源的匮乏限制了无线通信的进一步发展;另一方面,无线信道的多径传播特性和时变特性会对其中传输的信号带来非常大的损害。
近年来多输入天线多输出天线(MIMO)技术因为能大幅度增加无线通信系统的谱效率和提高传输可靠性而得到了越来越多的关注。
MIMO多天线系统所提供的空间复用增益和空间分集增益可以极大地提高无线链路的容量和质量。
现有的研究成果己经表明,MIMO所能达到的极高的频谱效率是目前任何一种无线技术所不及的,因此它被认为是未来新一代移动通信系统的备选关键技术之一。
根据信道的输入输出情况,使用多天线技术的通信系统可以分为单输入多输出SIMO(Single-Input Multiple-Output)系统、多输入单输出MISO(Multiple-Input Single-Output)系统,以及多输入多输出MIMO(Multiple-Input Multiple-Output)系统三种类型。
本文首先介绍了MIMO系统的基本概念和信道模型,然后我们从信息论的角度,根据传统SISO信道的香农容量,推导出MIMO信道容量公式,并且依次推导出SIMO信道和MISO信道的容量。
本文对MIMO信道模型和信道容量的研究表明,MIMO技术对于未来新一代无线通信具有极其的重要意义。
关键词:MIMO,SIMO,MISO,多天线系统,信道模型,信道容量。
I……………………………………………………………精品资料推荐…………………………………………………Channel Model and Capacity of the MIMOCommunication SystemsAbstractAs the wireless communication makes rapid progress, the demand for higher data rates and higher quality in wireless communication systems has recently seen unprecedented growth. However, one of the most limiting factors in growth of wireless communications is the scarcity of spectrum. In addition, the multi-path propagation and time variance characteristics of wireless channel bring some impairment to the signals transmitted over it. In recent years, multiple input multiple output (MIMO) antennas technique has received more and more attention, as it can dramatically increase the spectral efficiency and improve the transmission reliability of wireless communication systems. The MIMO channel gain of MIMO systems that include spatial multiplexing (SM) gain and the spatial diversity (SD) gain can increase greatly the capacity and the quality of the wireless link, and the research results show spectral efficiency of MIMO technique is higher than any other existing wireless techniques. So MIMO technique is considered as one of candidacy techniques that can be used in the next new generation of mobile cellular communication systems.Any given communication system that utilizes the multiple antenna technique can be classified into three categories: single input multiple output (SIMO), multiple input single output (MISO) and multiple input multiple output (MIMO) system respectively. This paper firstly introduces the basic concept and model of MIMO channel. Secondly, in the view of information theory, according to traditional SISO channel Shannon capacity, we derived the capacity of MIMO channel, MISO channel and SIMO channel.In this paper, the studies on MIMO channel model and channel capacity show that MIMO technique is vital to the new generation of wireless communications. Keywords: MIMO, SIMO, MISO, Multiple Antenna System, Channel Model, Channel Capacity.II……………………………………………………………精品资料推荐…………………………………………………目录摘要 (I)Abstract (II)第一章绪论 (1)1.1 引言 (1)1.2 无线移动通信的发展概述 (1)1.3 天线阵列在移动通信系统中的引入 (2)1.4 MIMO技术综述 (3)第二章多输入多输出通信系统信道模型 (5)2.1 通信系统模型 (5)2.2 模拟通信系统模型 (5)2.3 数字通信系统模型 (6)2.4 无线信道传播环境 (6)2.5 几种常用的无线通信信道模型 (7)2.5.1 高斯信道 (7)2.5.2 瑞利信道 (7)2.5.3 莱斯信道 (7)2.6 多输入多输出通信系统信道模型 (7)2.6.1 SISO信道 (7)2.6.2 SIMO信道 (8)2.6.3 MISO信道 (9)2.6.4 MIMO信道 (10)第三章多输入多输出通信系统信道容量 (12)3.1 MIMO系统模型的分解 (12)3.2 多输入多输出系统信道容量分析 (13)3.2.1 SISO系统信道容量 (13)3.2.2 MIMO系统信道容量 (14)3.2.3 SIMO系统信道容量 (16)3.2.4 MISO系统信道容量 (17)结论 (19)参考文献(References) (20)谢辞 (21)附录 (22)III……………………………………………………………精品资料推荐…………………………………………………第一章绪论1.1 引言自Marconi首次无线通信取得成功以来,人们对无线通信的研究就一直在不懈地努力着,但直到20世纪60年代,随着蜂窝概念的引入和70年代超大规模集成电路技术的进步,移动通信才开始得到真正的应用。
The COST 2100 MIMO channel model
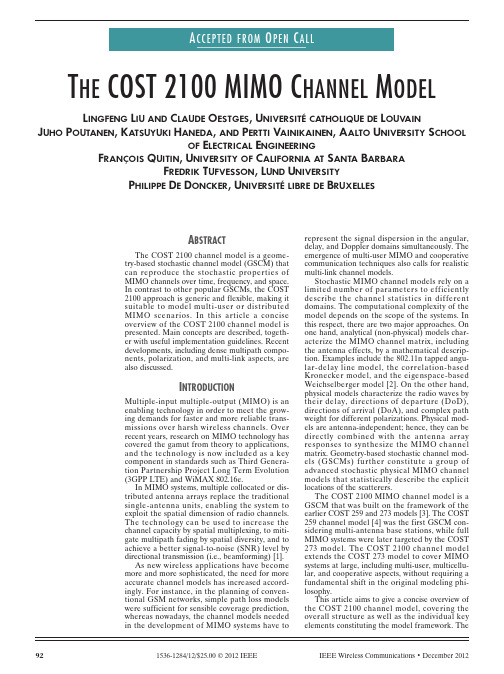
I NTRODUCTIONMultiple-input multiple-output (MIMO) is an enabling technology in order to meet the grow-ing demands for faster and more reliable trans-missions over harsh wireless channels. Overrecent years, research on MIMO technology has covered the gamut from theory to applications, and the technology is now included as a key component in standards such as Third Genera-tion Partnership Project Long Term Evolution (3GPP LTE) and WiMAX 802.16e.In MIMO systems, multiple collocated or dis-tributed antenna arrays replace the traditional single-antenna units, enabling the system to exploit the spatial dimension of radio channels.The technology can be used to increase the channel capacity by spatial multiplexing, to miti-gate multipath fading by spatial diversity, and to achieve a better signal-to-noise (SNR) level by directional transmission (i.e., beamforming) [1].As new wireless applications have become more and more sophisticated, the need for more accurate channel models has increased accord-ingly. For instance, in the planning of conven-tional GSM networks, simple path loss models were sufficient for sensible coverage prediction, whereas nowadays, the channel models needed in the development of MIMO systems have to represent the signal dispersion in the angular, delay, and Doppler domains simultaneously. The emergence of multi-user MIMO and cooperative communication techniques also calls for realistic multi-link channel models.Stochastic MIMO channel models rely on a limited number of parameters to efficiently describe the channel statistics in different domains. The computational complexity of the model depends on the scope of the systems. In this respect, there are two major approaches. On one hand, analytical (non-physical) models char-acterize the MIMO channel matrix, including the antenna effects, by a mathematical descrip-tion. Examples include the 802.11n tapped angu-lar-delay line model, the correlation-based Kronecker model, and the eigenspace-based Weichselberger model [2]. On the other hand, physical models characterize the radio waves by their delay, directions of departure (DoD), directions of arrival (DoA), and complex path weight for different polarizations. Physical mod-els are antenna-independent; hence, they can be directly combined with the antenna array responses to synthesize the MIMO channel matrix. Geometry-based stochastic channel mod-els (GSCMs) further constitute a group of advanced stochastic physical MIMO channel models that statistically describe the explicit locations of the scatterers.The COST 2100 MIMO channel model is a GSCM that was built on the framework of the earlier COST 259 and 273 models [3]. The COST 259 channel model [4] was the first GSCM con-sidering multi-antenna base stations, while full MIMO systems were later targeted by the COST 273 model. The COST 2100 channel model extends the COST 273 model to cover MIMO systems at large, including multi-user, multicellu-lar, and cooperative aspects, without requiring a fundamental shift in the original modeling phi-losophy.This article aims to give a concise overview of the COST 2100 channel model, covering the overall structure as well as the individual key elements constituting the model framework. TheL INGFENG L IU AND C LAUDE O ESTGES, U NIVERSITÉCA THOLIQUE DE L OUV AINJ UHO P OUTANEN, K A TSUYUKI H ANEDA, AND P ERTTI V AINIKAINEN, A ALTO U NIVERSITY S CHOOLOF E LECTRICAL E NGINEERINGF RANÇOIS Q UITIN, U NIVERSITY OF C ALIFORNIA A T S ANTA B ARBARAF REDRIK T UFVESSON, L UND U NIVERSITYP HILIPPE D E D ONCKER, U NIVERSITÉLIBRE DE B RUXELLESA BSTRACTThe COST 2100 channel model is a geome-try-based stochastic channel model (GSCM) thatcan reproduce the stochastic properties ofMIMO channels over time, frequency, and space.In contrast to other popular GSCMs, the COST2100 approach is generic and flexible, making itsuitable to model multi-user or distributedMIMO scenarios. In this article a conciseoverview of the COST 2100 channel model ispresented. Main concepts are described, togeth-er with useful implementation guidelines. Recentdevelopments, including dense multipath compo-nents, polarization, and multi-link aspects, arealso discussed.T HE COST 2100 MIMO C HANNEL M ODELmost recent achievements, including multi-link aspects, are presented, while considerations about parameterization, implementation, and validation are discussed later.R EVIEW OF G EOMETRY-B ASEDS TOCHASTIC C HANNEL M ODELSThe principle of GSCMs is to model the stochas-tic properties of wireless channels, with respect to the delay and double-directional domains, by analyzing the geometric distribution of the inter-acting objects (or scatterers) in the environment that contribute scattering to the radio channels. In GSCMs, a radio channel results from the superposition of different propagation paths, known as the multipath components (MPCs). Those MPCs are caused by the interaction between the radio waves and the objects in the environment, where each scattered contribution is characterized in both delay and direction domains. These scattering mechanisms may con-sist of a single interaction, that is, with one object (single-bounce), or of a number of con-secutive interactions with multiple objects (mul-tiple-bounce). The MPCs generated by either ofthese scattering mechanisms are representedthrough a geometric description of their proper-ties in three parameter space domains: delay,DoD, and DoA.Experimentally, it was observed that MPCstend to appear in packets in these domains.Intuitively, this makes sense: if a building acts asan interacting object, it is likely that the buildingwill create several reflected paths, caused bywindows, balconies, and so on, with similardelays and directions, given the finite size of thebuilding. In addition to being experiment-based,grouping MPCs with similar delays and direc-tions into packets (or clusters, as they are usuallyknown) enables the number of modeling param-eters to be significantly reduced. This explainswhy clusters constitute the basis of all recentGSCMs, from COST 259 to WINNER II.Whereas clusters are the parameterized quan-tities, it is important that overall models reflectreality. In particular, the channel large-scaleparameters (LSPs), such as the global delay andangular spreads, as synthesized from GSCMs,should be statistically reliable and consistent withexperimental observations. This means that clus-ters should be parameterized individually, yet insuch way that the global accuracy is guaranteed.This is where two main approaches can be used:a system-level approach, such as the widelyknown 3GPP Spatial Channel Model (SCM) [5]and the recent WINNER II model [6], or a clus-ter-level approach, such as the COST family ofchannel models. Both approaches rely on essen-tially different simulation processes. In system-level GSCMs (taking WINNER II as anexample), the modeling process is specified foreach instance of the channel between a base sta-tion (BS) and a mobile station (MS) by:•Defining the LSPs by their stochastic distribu-tion for each channel instance•Generating the clusters and MPCs accordingto these LSPs for any given locations of bothBS and MSBy contrast, in the cluster-level COST 2100model, the modeling process is specified oncefor the entire environment by:•Defining a large quantity of clusters with con-sistent stochastic parameters throughout thesimulation environment based on the BS loca-tion (yet not all clusters are visible at any timeinstant)•Defining the MS location and determining thescattering from the so-called visible clusters ateach channel instance•Synthesizing the LSPs based on the clusterscatteringThe advantage of such a system-level channelmodel is that the LSP statistics in a specific sce-nario are always guaranteed in each series ofchannel instances. However, forcing the statisti-cal consistency of the LSPs brings two criticallimitations:•The rigid structure of the approach does notspontaneously support continuous channeldescriptions over intervals larger than theauto-correlation distance, hence hamperingthe simulation of larger MS motions.•As the propagation environment is describedfrom the original LSPs only, adding new LSPs(e.g., the inter-link correlation) requires thatwe redefine the entire initialization of theenvironment, thereby hindering a straightfor-ward extension of the model.By contrast, the cluster-level COST 2100model is not constrained by the LSPs, and theenvironment is described independent of the MSlocation. This allows for smoother modeling oftime-variant channels. Basically, it is the MSmotion through the environment that will causethe channel statistics to vary, even over periodslarger than the auto-correlation distance. Fur-thermore, accounting for new LSPs, such as thecorrelation properties of multi-link channels, canbe carried out in a flexible fashion (i.e., withoutmodifying the model structure) by exploringadvanced properties of the clusters. Naturally, inany single realization of the channel, a cluster-level GSCM is expected to exhibit larger devia-tions of the LSP statistics than system-levelGSCMs, as these statistics are not expresslyforced by the model. Nevertheless, the averageLSP statistics remain typically consistent withmeasurements, as illustrated later.Regarding complexity, both system- and clus-ter-level GSCMs are similar as far as simulationsare concerned, as they both rely on adding con-tributions from a number of MPCs. Arguably,the cluster-level COST 2100 model probablyrelies on a more complex representation of theclusters, making their identification, estimation,and parameterization from measurements a criti-cal task.G ENERAL S TRUCTURE OF THECOST 2100 C HANNEL M ODELThe COST 2100 channel model was originallyproposed for simulating the radio channelbetween a static multiple-antenna BS and a mul-tiple-antenna MS. In most cases, the MPCs aremapped to the corresponding scatterers, and arecharacterized by their delay, azimuth of depar-Arguably, thecluster-level COST2100 modelprobably relies on amore complexrepresentation of theclusters, makingtheir identification,estimation, andparameterizationfrom measurementsa critical task.K ATSUYUKI H ANEDA(katsuyuki.haneda@aalto.fi) received his Doctor of Engineering from the Tokyo Institute of Technol-ogy, Japan, in 2007. He has been a post-doctoral researcher at the SMARAD Centre of Excellence in the Aalto University (former Helsinki University of Technology) School of Electri-cal Engineering, Espoo, Finland, since 2007. He was the recipient of the Student Paper Award presented at the 7th International Symposium on Wireless Personal Multimedia Communications. He has been serving as a co-chair of the Topical Working Group Indoor of the Euro-COST Action IC1004 “Cooperative Radio Communications for Green Smart Environments”. His research expertise includes radio wave propagation measurements and modeling, ultrawide-band radio, and multiple-input multiple-output radio com-munications, radio sensors and antennas, and applied electromagnetics for medical scenarios. Since 2012, he has been serving as an Associate Editor of IEEE Transactions on Antennas and Propagation.P HILIPPE D E D ONCKER(pdedonck@ulb.ac.be) received his engineering and Ph.D. degrees from ULB in 1996 and 2001, respectively. He is currently a professor with ULB. His research interests focus on wireless communications andelectromagnetics.F REDRIK T UFVESSON(fredrik.tufvesson@eit.lth.se) received hisM.S. degree in electrical engineering in 1994, his Licentiatedegree, in 1998 and his Ph.D. degree in 2000, all fromLund University, Sweden. After almost two years at a start-up company, Fiberless Society, he is now an associate pro-fessor at the Department of Electrical and InformationTechnology. His main research interests are channel mea-surements and modeling for wireless communication,including channels for both MIMO and UWB systems.Besides this, he also works with research projects on radio-based positioning as well as on his company on wirelesssearch and rescue equipment.P ERTTI V AINIKAINEN(pertti.vainikainen@aalto.fi) received hisM.S. in technology, Licentiate of Science in technology,and Doctor of Science in technology from Helsinki Universi-ty of Technology (TKK) in 1982, 1989, and 1991, respec-tively. Since 1998 he has been a professor in radioengineering in the Radio Laboratory (since 2008 theDepartment of Radio Science and Engineering) of TKK(since 2010, Aalto University School of Science and Tech-nology). His main fields of interest are antennas and prop-agation in radio communications and industrialmeasurement applications of radio waves. He is the authoror coauthor of six books or book chapters and about 380refereed international journal or conference publications,and the holder of 11 patents.C LAUDE O ESTGES(claude.oestges@uclouvain.be) receivedM.S. and Ph.D. degrees in electrical engineering from UCLin 1996 and 2000. In January 2001, he joined as a post-doctoral scholar the Smart Antennas Research Group(Information Systems Laboratory), Stanford University, Cali-fornia. He is presently a research associate of the BelgianFonds de la Recherche Scientifique and assistant professorwith the Electrical Engineering Department, Institute forInformation and Communication Technologies, Electronicsand Applied Mathematics, UCL. He also currently serves asan Associate Editor for IEEE Transactions on Antennas andPropagation and IEEE Transactions on Vehicular Technolo-gy. He is the author or co-author of two books and morethan 170 journal papers and conference communications,and was the recipient of the 1999-2000 IET Marconi Premi-um Award and the 2004 IEEE Vehicular Technology SocietyNeal Shepherd Award.。
无线信道建模与仿真
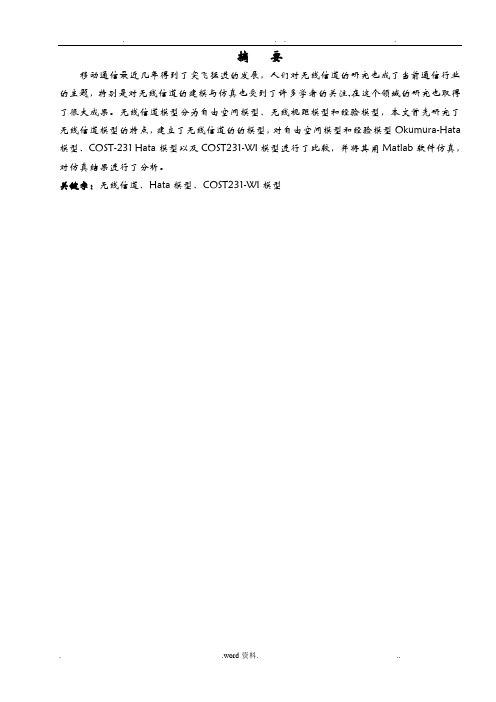
摘要移动通信最近几年得到了突飞猛进的发展,人们对无线信道的研究也成了当前通信行业的主题,特别是对无线信道的建模与仿真也受到了许多学者的关注,在这个领域的研究也取得了很大成果。
无线信道模型分为自由空间模型、无线视距模型和经验模型,本文首先研究了无线信道模型的特点,建立了无线信道的的模型,对自由空间模型和经验模型Okumura-Hata 模型、COST-231 Hata模型以及COST231-WI模型进行了比较,并将其用Matlab软件仿真,对仿真结果进行了分析。
关键字:无线信道、Hata模型、COST231-WI模型AbstractMobile communication several years obtained the development recently which progresses by leaps and bounds, The people have also become the current correspondence profession subject to the wireless channel research. Specially has also received many scholars' attention to the wireless channel modeling and simulation, Has also yielded the very big result in this domain research. Wireless channel model is divided into free space model, the wireless line of sight and empirical model, this paper studied the characteristics of wireless channel model is established radio channel model, on the free space model and empirical model Okumura-Hata model, COST-231 Hata model and COST231-WI model were compared, using Matlab software to simulate, the simulation results are analyzed. Keywords: Wireless channel, Hata model, COST231-WI model目录第一章绪论 (4)1.1 无线通信的发展和建模仿真的发展状况 (4)1.1.1 无线通信的发展 (4)1.1.2 信道建模仿真技术的发展概况 (4)1.2 本文研究的容 (5)第二章无线信道的概念和无线信道的模型 (6)2.1 无线信道的概念 (6)2.1.1 无线信道的定义 (6)2.1.2 无线信道的特点 (6)2.2 无线信道的模型 (15)2.2.1 自由空间传播模型 (15)2.2.2 无线视距传播模型 (17)2.2.3 无线信道经验模型 (19)第三章无线信道建模仿真及结果分析 (30)3.1 Matlab软件介绍 (30)3.2 路径损耗模型仿真及结果分析 (30)3.2.1 自由空间模型仿真及结果分析 (30)3.3 经验模型仿真及结果分析 (32)3.3.1 Okumura-Hata模型仿真及结果分析 (32)3.3.3 COST-231 Hata模型仿真及结果分析 (34)3.3.5 COST231-WI模型仿真及结果分析 (36)结论 (39)参考文献 (41)附录 (42)中英文翻译 (42)Matlab程序 (49)致 (54)第一章 绪论1.1 无线通信的发展和建模仿真的发展状况1.1.1 无线通信的发展无线通信的开端可以追溯到公元1901年,当年的12月12日,意大利科学家列莫·马可尼实现了人类历史上首次无线电通信。
Radio Propagation and Channel Modeling
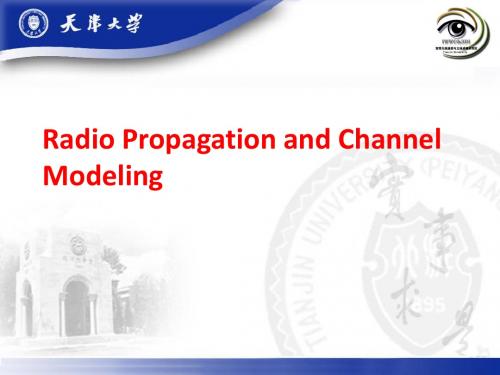
Softwares:WiSE, SitePlanner, Planet EV, et.al.
Two Path Model
Path loss for one LOS path and 1 ground (or reflected) bounce Ground bounce approximately cancels LOS path above critical distance Power falls off
Channel modeling methods MIMO Channel Models
Standardized channel models An Example - WiNNER II Channel Model
Conclusions
Lecture 1 Review
Course Information Wireless Vision Technical Challenges Multimedia Requirements Current Wireless Systems Spectrum Regulation and Standards
Free Space (LOS) Model
d=vt
PL dB 10 log10
4 d
Gl l 2
2
Path loss for unobstructed LOS path Power falls off :
More references:
Proportional to d2 Proportional to l2 (inversely proportional to f2) Herry L. Bertoni, Radio Propagation for Modern Wireless Systems, Publishing House of Electronics Industry.
MIMO和SCM信道模型解读课件
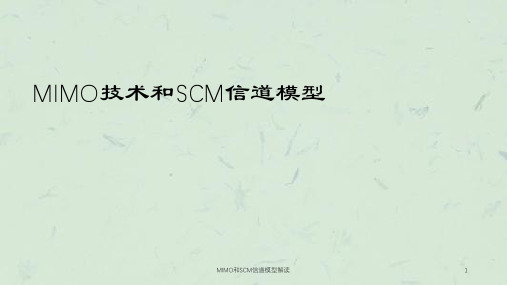
MIMO和SCM信道模型解读
33
SCM信道模型使用
4.关于 hu,s,n (t)
信道冲击响应的增益系 数
N
h(t,)hn(t)(n) n0
N
r(t) h (t,)s(t)d
h n(t)s(tn)
n 0
MIMO和SCM信道模型解读
基站
大型建筑
建筑
反射 视距
建筑
移动单元
散射
MIMO和SCM信道模型解读
12
MIMO信道模型
2.无线信道衰落模型
³ 大尺度衰落模型
® 长距离范围接收信号强度缓慢变化的规律;
³ 中尺度衰落模型
® 阴影衰落,它是重叠在大尺度传播特性中值电平上的平均功率 变化;
³ 小尺度衰落模型
® 短距离或短时间内接收信号强度的快速变化。
y(t) [y1 (t)y ,2(t),y .N .R(.t),T ]
式中 y为i (t)基站的第根天线端口的信号。
MIMO和SCM信道模型解读
16
MIMO信道模型
5.信道建模方法
³ MIMO信道模型主要分3类:
® 统计性模型(经验模型)
® 确定性模型
® 半确定性模型
MIMO和SCM信道模型解读
17
MIMO信道模型
统计性模型
统计性模型是基于信道各种统计特性建立的信道模型。在实际传播环 境中,存在着大量具有相同或相似传播特性的小区,对这些小区进行 实际测量,归纳出信道各种重要的统计特性(如时延扩展、角度扩展 等)及信道参数的概率密度分布,利用这些统计信息建立适用范围较 广的空间信道模型。典型方法如基于试验测量的冲激响应法,这是一 种完全随机的方法。 统计性模型的优点在于模型的复杂度较低,具有一定的通用性;缺点 是和实际的信道有较大偏差,这是因为模型的各种参数是用各自统计 特性随机生成,随机生成的参数和实际测量的参数可能会有比较大的 差别。 统计模型主要有李氏模型、离散均匀分布模型、高斯广义稳态非相关 散射模型等。
四元数Wishart矩阵分布及其在通信中的应用
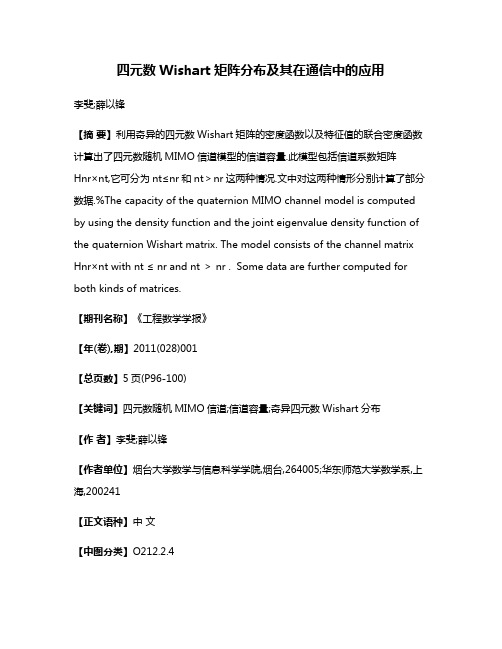
四元数Wishart矩阵分布及其在通信中的应用李斐;薛以锋【摘要】利用奇异的四元数Wishart矩阵的密度函数以及特征值的联合密度函数计算出了四元数随机MIMO信道模型的信道容量.此模型包括信道系数矩阵Hnr×nt,它可分为nt≤nr和nt>nr这两种情况.文中对这两种情形分别计算了部分数据.%The capacity of the quaternion MIMO channel model is computed by using the density function and the joint eigenvalue density function of the quaternion Wishart matrix. The model consists of the channel matrix Hnr×nt with nt ≤ nr and nt > nr . Some data are further computed for both kinds of matrices.【期刊名称】《工程数学学报》【年(卷),期】2011(028)001【总页数】5页(P96-100)【关键词】四元数随机MIMO信道;信道容量;奇异四元数Wishart分布【作者】李斐;薛以锋【作者单位】烟台大学数学与信息科学学院,烟台,264005;华东师范大学数学系,上海,200241【正文语种】中文【中图分类】O212.2.41 引言关于实数随机矩阵的多元统计在文献[1]中已有详细而系统的研究.在对复数随机矩阵的研究中,Ratnarajah[2],Vaillancourt[3]对复Wishart随机矩阵(奇异和非奇异)、复正态随机矩阵的密度函数和复Wishart随机矩阵特征值的联合分布密度函数做了一定的研究,并在文献[2]中给出了复随机矩阵在信息理论中的重要应用:MIMO Rayleigh衰减信道的容量计算.文献[4-8]在四元数随机矩阵的研究基础上,建立了四元数的随机MIMO信道模型,并求出了该信道模型的容量.以下是要用到的文献[5,7]中关于四元数随机矩阵结论.设四元数随机矩阵(即Y服从四元数的正态分布),其密度函数为四元数随机矩阵即W满足四元数的中心非奇异Wishart分布,则它的密度函数为其中Retr(·)表示取迹的实部,另外表示四元数矩阵的Γ函数.文献[7]还给出了W的特征值的联合分布如下其中是四元数超几何函数,是W 的特征值.当Σ =时,W的特征值的联合分布如下定理1 若X是m×n阶的四元数矩阵,秩为m(m 6 n),且f(X)是关于X的函数,仅通过XXH与X有关,则证明由W=XXH=V∆WVH(四元数矩阵的谱分解),其中∆W=diag(w1,···,wm),w1,···,wm是W的特征值,V是广义酉矩[9],则由文献[8]中的推论1,有及文献[6]中的定理6,有进而,我们得到2 非奇异四元数Wishart矩阵在MIMO中的应用一个MIMO信道系统可以用一个nr×nt的四元数随机矩阵H来表示,其中nt,nr 分别是通信系统的输入、输出数.该通信系统如图1所示.图1:四元数随机MIMO通信系统第j个输出接受到的四元数信号可以写作其中hij是第i个输入,第j个输出之间的四元数信道系数,xi是第i个输入的四元数信号,vj是服从四元数正态分布的干扰.输出端接收到的信号向量可以写作或者用向量表示如下在这一部分中,我们将通过线性模型(2),求得四元数MIMO信道模型的信道容量。
MIMO信道仿真模型比较及其验证
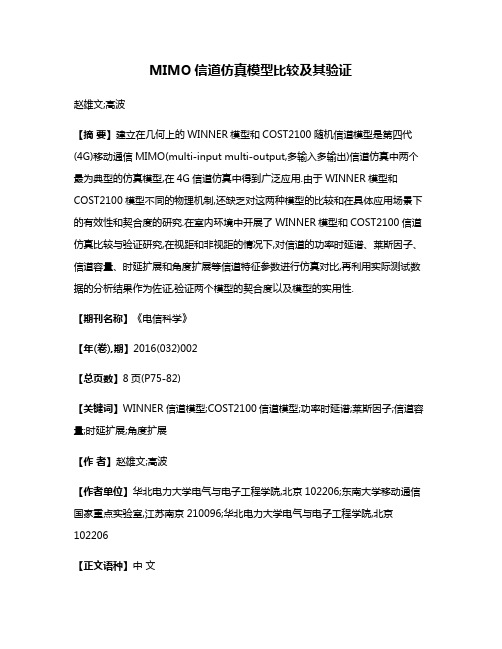
MIMO信道仿真模型比较及其验证赵雄文;高波【摘要】建立在几何上的WINNER模型和COST2100随机信道模型是第四代(4G)移动通信MIMO(multi-input multi-output,多输入多输出)信道仿真中两个最为典型的仿真模型,在4G信道仿真中得到广泛应用.由于WINNER模型和COST2100模型不同的物理机制,还缺乏对这两种模型的比较和在具体应用场景下的有效性和契合度的研究.在室内环境中开展了WINNER模型和COST2100信道仿真比较与验证研究,在视距和非视距的情况下,对信道的功率时延谱、莱斯因子、信道容量、时延扩展和角度扩展等信道特征参数进行仿真对比,再利用实际测试数据的分析结果作为佐证,验证两个模型的契合度以及模型的实用性.【期刊名称】《电信科学》【年(卷),期】2016(032)002【总页数】8页(P75-82)【关键词】WINNER信道模型;COST2100信道模型;功率时延谱;莱斯因子;信道容量;时延扩展;角度扩展【作者】赵雄文;高波【作者单位】华北电力大学电气与电子工程学院,北京102206;东南大学移动通信国家重点实验室,江苏南京210096;华北电力大学电气与电子工程学院,北京102206【正文语种】中文【中图分类】TN011由于第四代(fourth generation,4G)移动通信链路和系统仿真的需要,基于几何的随机信道模型的研究近年来一直是信道建模领域的研究热点[1]。
如3GPP标准提出了MIMO空间信道模型(spatial channel model,SCM)[2],欧洲4G WINNER[3]项目将SCM进行扩展,得到SCME(SCM extension)。
SCME由原来支持的5 MHz信道带宽扩展到100 MHz,载频由2 GHz扩展到6 GHz。
随着WINNER模型的升级和完善,其仿真模型已成为ITU-R[4]和3GPP 标准化的重要组成部分之一。
窄带MIMO信道最大似然估计的误差
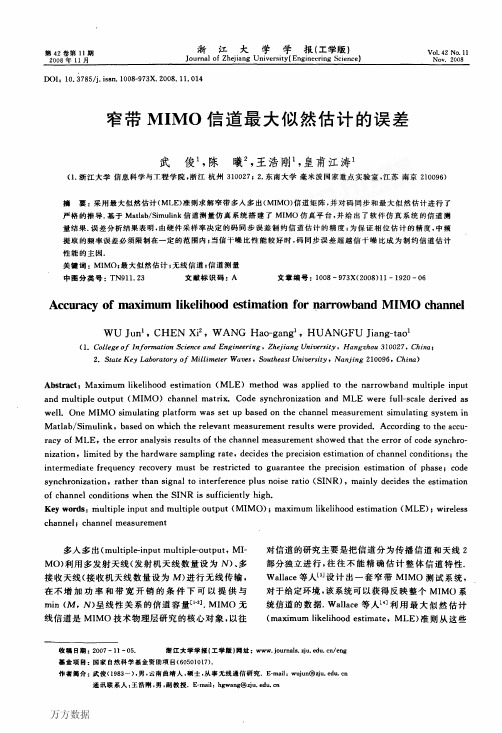
130I:10.3785/j.issn.1008‘973X.2008.11.014窄带MIMO信道最大似然估计的误差武俊1,陈曦2,王浩刚1,皇甫江涛(1.浙江大学信息科学与丁程学院,浙江杭州310027;2.东南大学毫米波国家重点实验室,江苏南京210096)摘要:采用最大似然估计(MI。
E)准则求解窄带多人多出(MIMO)信道矩阵,并对码同步和最大似然估计进行了严格的推导.基于Matlab/Simulink信道测量仿真系统搭建了MIMO仿真平台,并给出了软件仿真系统的信道测量结果.误差分析结果表明,由硬件采样率决定的码同步误差制约信道估计的精度;为保证相位估计的精度。
中频提取的频率误差必须限制在一定的范围内;当信干噪比性能较好时,码同步误差超越信十噪比成为制约信道估计性能的主因.关键词:MIMO;最大似然估计;无线信道;信道测量中图分类号:TN911.23文献标识码:A文章编号:1008—973X(2008)11—1920—06imationfornarrowbandMIMOchannelWUJunl,CHENXi2,WANGHao—gan91,HUANGFUJiang-ta01(1.CollegeofInformationScienceandEngineering,ZhejiangUniversity,Hangzhou310027,China;2.StateKeyLaboratoryofMillimeterWaves,SoutheastUniversity,Nanjing210096,China)Abstract:Maximumlikelihoodestimation(MLE)methodwasappliedtothenarrowbandmultipleinputandmultipleoutput(MIM0)channelmatrix.CodesynchronizationandMLEwerefull—scalederivedaswell.OneMIMosimulatingplatformwassetupbasedonthechannelmeasurementsimulatingsysteminMatlab/Simulink,basedonwhichtherelevantmeasurementresultswereprovided.Accordingtotheaccu-racyofMI。
- 1、下载文档前请自行甄别文档内容的完整性,平台不提供额外的编辑、内容补充、找答案等附加服务。
- 2、"仅部分预览"的文档,不可在线预览部分如存在完整性等问题,可反馈申请退款(可完整预览的文档不适用该条件!)。
- 3、如文档侵犯您的权益,请联系客服反馈,我们会尽快为您处理(人工客服工作时间:9:00-18:30)。
The COST273MIMO Channel Model: Three Kinds of ClustersNicolai Czink1,Claude Oestges21Forschungszentrum Telekommunikation Wien(ftw.),Vienna,Austria 2Microwave Laboratory,Universit´e catholique de Louvain(UCL),Belgiumczink@ftw.atAbstract—The novel COST273MIMO channel model is a good candidate for link-level and system-level simulations of multi-antenna communication systems.This geometry-based stochastic channel model is based on the concepts of multipath clusters,allowing for an implementation with low computational effort.The model is suitable to accurately reflect the frequency-selective,time-variant,fully-polarimetric nature of various prop-agation environments.Having only few external parameters,it is particularly interesting for signal processing engineers wanting to test their algorithms against realistic channels.A current shortcoming of the COST273MIMO channel model is its missing parametrisation for a number of scenarios. Particularly,the parametrisation of the cluster parameters is challenging,even more,since three different kinds of clusters are used to model the channel.This paper outlines an approach to consistently parametrise the clusters used in the COST273MIMO channel model from representative measurement data.Keywords—MIMO channel;geometry-based stochastic channel models;COST273MIMO channel modelI.I NTRODUCTIONThe new COST273MIMO channel model[1]aims to model a large number of different scenarios.Its generic structure uses clusters,i.e.groups of multipath components, to model the wideband,time-variant,double-directional,fully-polarimetric radio channel.Its ultimate goal is to provide time series of the multiple-input multiple-output(MIMO) channel for link-and system-level simulations,yielding values of essential characteristics of the MIMO channel(space-time correlations,mutual information,etc.),as a function of environmental and antenna array parameters.In contrast to analytical models,such as the Kronecker or the eigenbeam(a.k.a.Weichselberger[2])representations ,it must be understood that the COST273MIMO channel model does not allow for the explicit design of space-time coding techniques.While analytical models provide a tractable mathematical framework for algorithm design,they neglect important properties of the radio channel.Thus it is necessary to test MIMO algorithms against a realistic channel model, such as the COST273MIMO channel model,to quantify its applicability in real-world environments.The COST273MIMO channel model is not yet completely parametrised for all of the envisioned scenarios.Particularly, the cluster parameters are still an open issue.Tofind con-sistent model parameters,automatic methods identifying the model parameters from measurements,are required.To do so, multipath clusters need to be identified in measurement data. Identifying clusters from measurements was automated in the last years[3].However,the main difficulty in parametrising the COST273MIMO channel model is the fact that it uses three different kinds of clusters to accurately model the radio channel.In view of the above discussion,this paper provides an overview of the three different kinds of clusters in order to provide an impetus for future research on the automatic parametrisation of the COST273MIMO channel model.II.O VERVIEW OF THE COST273MIMO CHANNEL MODELThe COST273MIMO channel model is a geometry-based stochastic channel model,analogous the other recently developed models[4],[5],and aimed at link-and/or system-level simulations.A main difference with the above mentioned models lies however in the fact that the base station and mobile terminal(s)are specified by absolute positions in a two-dimensional space,rather then just relatively with respect to one another.The model itself has a generic structure,i.e.the core of the model is the same for all kinds of environments. Hence,the distinction between the environments is solely done by the model parameters.The following Section will provide a brief overview of the different kinds of environments.Subsequently,we will describe the external model parameters which are to be set by the user of the model.Finally,we will describe the internals of the COST273MIMO channel model.A.EnvironmentsThe COST273community envisioned4different groups of environments:macrocells,microcells,picocells,and ad-hoc networks.Each of these groups is further split up into significantly different propagation scenarios,leading to a total number of22different environments.Seven of these scenarios are indicated as mandatory scenarios,which are foreseen to be used for system testing:(i)small macrocells in city centre, (ii)large urban macrocells,(iii)outdoor-to-indoor urban,(iv) city centre microcells,(v)picocell in a hall,(vi)office line-of-sight,(vii)office non-line-of-sight.Unfortunately,the internal model parameters for a number of these scenarios are yet to be identified.er parametersDespite the large number of internal parameters and the plenitude of scenarios,the usage of the COST273MIMO channel model is quite simple.Only a few parameters need to be specified.These user parameters(“external parameters”)are the car-rier frequency and bandwidthfilter,base-station(BS)absolute position and mobile-station(MS)trajectory in a2-D space, antenna array specifications and the rotation of the array in the2-D coordinate system,and,optionally,a particular path-loss model.For the straight-forward usage of the COST273MIMO channel model,it is even foreseen to provide exemplary user parameter sets.C.Generic model structureThe internal structure of the COST273MIMO channel model is quite complex.This paper will not discuss the inter-nals in detail,but rather provide a comprehensible overview. As already mentioned,the environments are modeled ge-ometrically.Scattering obstacles are represented by clusters, each cluster consisting of a group of geometrically co-located scatterers,represented by paths from/to the BS and MS.In this way,specular reflection,diffraction,and also scattering, can be modeled with low complexity.The generation of the radio environment is as follows:1)Place a local cluster around the MS,and around the BS1.2)Place“single-interaction clusters”according to statisti-cal distributions,given the MS trajectory.3)Place“multiple-interaction clusters”according to statis-tical distributions,given the MS trajectory(applies only in selected environments).4)Depending on the scenario,the line-of-sight componentis considered.The three different kinds of clusters are described in more detail in Section III.After this step,the propagation environment is specified by a number of discrete propagation paths.By letting the MS move through the propagation environment along its trajectory,the parameters of the propagation paths,i.e.delay,direction of arrival,and direction of departure,change ing a system model including the antenna patterns,the MIMO channel matrix is calculated[6,Sec.6.7].Note that by the motion of the mobile station,the resulting modeled channels are smoothly time-variant and intrinsically correlated.III.T HREE KINDS OF CLUSTERSWhen initialising the propagation environment,three differ-ent kinds of clusters were used:(i)local clusters,(ii)single-interaction clusters,and(iii)multiple-interaction clusters.Fig-ure1provides a schematic representation of these clusters. Their properties are reviewed in the following paragraphs.1A local BS cluster is only placed in picocell and ad-hoc environments A.Local clusterThe local cluster is modeled by a ring and is usually found around the mobile station,and,in pico-cell or ad-hoc scenarios,around the base station.Local clusters are always taken as single-bounced and are always active,thus they always contribute to the channel impulse response.Their size is given by their delay spread,specified in the model. These clusters introduce an omnidirectional azimuth spread around the respective station at low delay,which is frequently observed in measurements.B.Single-interaction clustersSimilar to the local clusters,single-interaction clusters pro-vide a single-bounce link between the BS and the MS.In macrocells,this kind of clusters is the dominant mechanism, representing reflections off large buildings,hills,etc.In mi-crocells and picocells,single-bounce scattering also occurs, though less frequently.While the MS is moving,it observes contributions from multiple single-interaction clusters that have a smooth transi-tion from inactive to active state,and vice versa.Whether a cluster is active(i.e.accounted for in the channel response)or not is determined by the concept of visibility regions[7]. 1)Visibility regions:Every single-interaction cluster is associated with one particular visibility region.A visibility region is a circular region of the2-D space characterised by two radii(the radius of the visibility region and the radius of a “transition region”),and itsfixed position in space.The radii are tabulated in the internal parameters of the chosen scenario. These visibility regions are placed randomly in space along the route of the MS in the model initialization.The number of visibility regions is linked to the expected number of clusters in the channel(which is also tabulated in the internal parameters)[7].2)Cluster position:The actual position of the cluster associated with a particular visibility region is set by the following geometric approach.First,we draw a line from the BS to the centre of the visibility region.The cluster position will be determined relative to this line.The radial distance from the BS is determined by an exponential distribution.The angle of the cluster centre relative to the line is drawn from a Gaussian distribution.The parameters for the distribution are again determined in the internal parameters.The size of the cluster is also drawn from respective distributions.Note that to accurately represent the propagation environment,the COST 273MIMO channel model defines autocorrelations and cross correlations between certain cluster parameters.C.Multiple-interaction clustersEspecially in pico cells,but also in micro-cell scenarios, contributions from multiple bounces are dominant.To ac-count for these distributions,a special type of cluster,the multiple-interaction cluster was invented.The frequency of occurrence of the multiple-interaction clusters with respect to single-interaction clusters is inversely proportional to theFig.1.Different kinds of clusters in the COST273MIMO channel model:local clusters(green colour),single-interaction clusters(blue colour),multiple-interaction clusters(red colour)selection factor,K sel,which indicates the ratio between single-interaction clusters and multiple-interaction clusters.In macro-and micro-and pico-cell scenarios,this factor is set to1,0.5 and0respectively.Similar to the case of single-interaction clusters,visibility regions are placed along the trajectory of the MS.However,the position of the multiple-interaction clusters is set differently. First,the angular position of the cluster seen from the BS is evaluated by randomly drawing from a pre-specified distri-bution given in the internal parameters,whereas the angular position seen from the MS is determined by drawing from another distribution.In this way,the direction of departure of the cluster(at the BS)is decoupled from its direction of arrival (at the MS).Secondly,the cluster mean delay,as well as the cluster delay spread,specifying the cluster extent in space,are drawn. Thirdly,both cluster azimuth spreads(seen from BS and MS) are drawn.Finally,the cluster is split up into its transmitter and receiver part.The distance to the BS is determined by the cluster delay spread and cluster azimuth spread at the BS,while the distance to the MS is determined in the same way by the cluster delay spread and the cluster azimuth spread at the MS.These two parts are connected by a cluster-link delay.We want to stress that the cluster spatial extent is only related to its delay-spread, whereas the azimuth spread is further related to the distance of the cluster to the MS/BS.Note that the paths within this“twin cluster”[8]are only specified by their amplitude,delay,azimuth of arrival,and azimuth of departure.The beauty of this approach is that the cluster has two representations in space(it is split up in Tx and Rx),but the path parameters are as few as for a single cluster.It must be mentioned that even though every individual cluster shows a Kronecker structure(because all angles of arrival and departure are independent within each cluster), the COST273MIMO channel model does not create a macroscopic Kronecker structure of the channel[9],i.e.in all generality,the obtained channel correlation matrix will not be separable.Yet,it does not mean that the COST273model cannot be approximated with more or less accuracy in the analytical world by a Kronecker representation.IV.A N APPROACH TO PARAMETRISING THEDIFFERENT CLUSTER TYPESThe major problem of the COST2100MIMO channel model is its missing parametrisation for the majority of the scenarios.Another latent issue is the quality of parametrisation —some internal parameters were obtained from educated guesses.To overcome these problems,a coherent parametrisation scheme is inevitable.This section presents an approach to estimate the internal parameters of the different cluster types from measurements.A.PrerequisitesFor proper function this parametrisation approach needs the following prerequisites:•MIMO channel measurements in multiple representative environments.The data must have been collected by a wide-band radio channel sounder with antenna arrays designed for spatial estimation of propagation paths.Preferably,the antenna arrays should support probing in the full azimuth and elevation domain.Accurate location information of the BS and trajectory of the MS in the measured environments are essential.•A high-resolution estimation algorithm estimating the complex amplitude,delay,direction of departure and direction of arrival of the propagation paths in a measured impulse response.Two good candidates for these are the Kalman-filter-extended RIMAX algorithm[10],or the SAGE-based ISIS algorithm[11].For these algorithms it is vital to provide the calibrated antenna patterns from the channel measurements.Using either of the algorithms,the propagation paths have to be estimated from all snapshots of the representative measurements.Note that the computation time of these algorithms are quite high.•A clustering-and-tracking algorithm that identifies clus-ters in measurements.A suitable algorithm was recently presented in[3],which is based on an extension of the K-means clustering algorithm,including an initial guess and tracking of the clusters.All path estimates from the measurements need to be post processed.The identified clusters of an individual snapshot constitute the result set of this snapshot.The result sets of all time instants are the input data to the parametrisation algorithm.B.Identification frameworkTo identify the cluster parameters of the different cluster types from measurements,we propose the following frame-work applied to the estimated clusters of every individual measured snapshot.1)Given the location of BS and MS,identify the LOS com-ponent,if any.Subsequently subtract the LOS cluster from the result set.2)Given the location of BS and MS in the measurements,identify the parameters of the local cluster and subtract the contributions of the local cluster from the result set.3)For each remaining cluster,given their delay spread,identify whether a cluster is single-bounced or multiple-bounced.Subsequently,identify the cluster parameters. In the following we describe this approach in detail.1)LOS component:In LOS scenarios,the location of the LOS component(which is also identified as cluster in the measurements)can easily be identified from the measurements using the delay,azimuth of departure and azimuth of arrival. Naturally,it should have stronger power than the surround-ing clusters.After identifying its parameters,this cluster is removed from the result set.2)Local cluster:Clustering algorithms that include power to identify clusters more accurately will naturally split up the local cluster into multiple smaller ones.However,using the knowledge of the BS and MS position,clusters contributing to the local cluster can easily be identified.The local cluster around the MS is found at a distinct angle seen from the BS,which can be obtained from the location information of MS and BS.Seen from the MS,this cluster is wide-spread.The same identification method is used for the local cluster around the BS.After having identified all clusters in the result set that contribute to the local cluster(s),they are removed from the result set,such that only single-interaction clusters and multiple-interaction clusters remain.3)Single-interaction and multiple-interaction clusters: First,we distinguish whether a cluster stems from single-bounce or multiple-bounce mechanisms using the following method(see Figure2):•Determine the diameter of the cluster in space from its delay spread as d C=3στ·c0,where c0denotes the speed of light.•Determine the distance of the cluster from the BS and from the MS by d BS/MS=d C2tan(3σφBS/MS).The associated delays are defined asτBS/MS=d BS/MS/c0.The total delay of the cluster is then described byτC=τC,BS+τC,MS+τC,link.The last term describes the link delay of the cluster.•The link delay decides whether the cluster stems from a single-bounce or double-bounce reflection byτC,link≤ ···single-interaction clusterτC,link> ···multiple-interaction cluster, where is set close to zero.Estimation errors and errors in identifying clusters can be accounted for by a larger value of .Note that it might sometimes happen that the link delay gets smaller than zero,which also evolves from the estimation variances of the path estimator or from the cluster identification.After having categorised the clusters,their parameters can be directly used to determine the internal parameters of the COST273MIMO channel model.V.S UMMARYThe COST273MIMO channel model is well suited for link-level and system-level simulations of MIMO algorithms. Its major shortcoming is the current lack of parameters for the different propagation environments.We presented an approach to parametrise the three different kinds of clusters used in the COST273MIMO channel model: (i)the local clusters,(ii)single-interaction clusters,and(iii) multiple-interaction clusters.Using this approach,it is possible to consistently parametrise the model from representative MIMO channel measurements in the different propagation environments.VI.A CKNOWLEDGEMENTSThis paper has been written in the framework of COST 2100“Pervasive Pervasive Mobile&Ambient Wireless Com-munications”.The work of Nicolai Czink was funded by the Wiener Wissenschafts-Forschungs-und Technologiefonds (WWTF)in the ftw.project“Cooperative Communications for Traffic Telematics(COCOMINT)”and by the Austrian COMET program.The work of Claude Oestges is funded by the Fonds de la Recherche Scientifique(FRS-FNRS).MSFig.2.Distinguishing between single-interaction clusters and multiple-interaction clusters:for single-interaction clusters,τlink is close to zero.R EFERENCES[1]L.Correia,Ed.,Mobile Broadband Multimedia Networks.AcademicPress,2006.[2]W.Weichselberger,M.Herdin,H.¨Ozcelik,and E.Bonek,“A stochasticMIMO channel model with joint correlation of both link ends,”IEEE Transactions on Wireless Communications,vol.5,no.1,pp.90–100, January2006.[3]N.Czink,R.Tian,S.Wyne,F.Tufvesson,J.-P.Nuutinen,J.Ylitalo,E.Bonek,and A.F.Molisch,“Tracking time-variant cluster parametersin MIMO channel measurements,”in ChinaCom2007,Shanghai,China, August2007.[4]“Spatial channel model for Multiple Input Multiple Output(MIMO)simulations(3GPP TR25.996),v6.1.0,”Sep.2003.[Online].Available: [5]“WINNER II interim channel models(D 1.1.1,V1.1),”WirelessWorld Initiative New Radio(WINNER II),November2006.[Online].Available:https://[6] A.F.Molisch,Wireless Communications.Wiley,2005.[7]H.Asplund, A. A.Glazunov, A. F.Molisch,K.I.Pedersen,andM.Steinbauer,“The COST259directional channel model—Part II.macrocells,”IEEE Transactions on Wireless Communications,vol.5,pp.3434–3450,2006.[8]H.Hofstetter,A.F.Molisch,and N.Czink,“A twin-cluster MIMOchannel model,”in European Conference on Antennas and Propagation (EuCAP)2006,Nice,France,2006.[9] C.Oestges, B.Clerckx, D.Vanhoenacker-Janvier,and A.Paulraj,“Impact of fading correlations on MIMO communication systems in geometrical scattering channel models,”IEEE Trans.Wireless Commun., vol.4,no.3,pp.1112–1120,May2005.[10]J.Salmi,A.Richter,and V.Koivunen,“Enhanced tracking of radio prop-agation path parameters using state-space modeling,”in14th European Signal Processing Conference(EUSIPCO),Florence,Italy,September 2006.[11] B.H.Fleury,M.Tschudin,R.Heddergott,D.Dahlhaus,and K.I.Pedersen,“Channel parameter estimation in mobile radio environments using the SAGE algorithm,”IEEE Journal on Selected Areas in Com-munications,no.3,pp.434–450,171999.。