一种基于概率统计特征的剪接位点识别方法
基于结合HM—SVM方法的HMM剪接位点识别研究
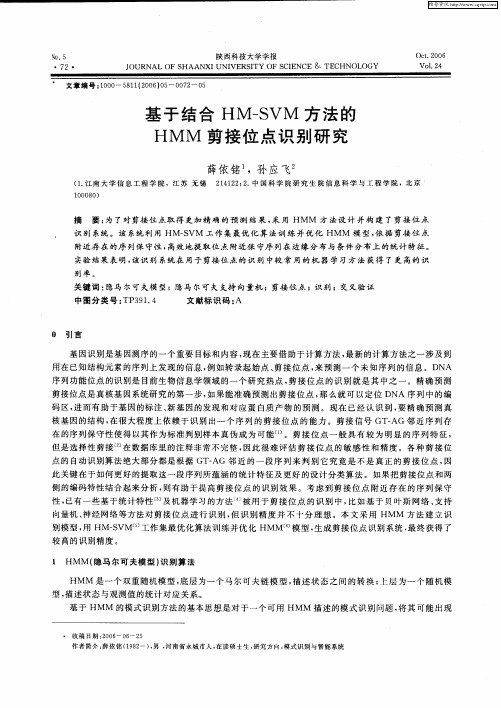
核 基 因的结 构 , 很 大程度 上依 赖 于识 别 出一 个 序 列 的 剪 接位 点 的 能 力 。剪 接 信 号 GT AG邻 近 序 列 存 在 — 在的序 列保 守性 使得 以其 作为 标准 判别 样本 真伪 成 为 可能n 。剪接 位 点 一 般具 有 较 为 明 显 的序 列 特 征 , 但 是选 择性 剪接 在 数据库 里 的注 释非 常不完 整 , 因此 很 难评 估 剪 接 位 点 的敏 感 性 和 精 度 。各 种 剪 接 位 点 的 自动识 别算 法绝 大部分 都 是根 据 GT AG 邻 近 的一 段 序 列来 判 别 它 究 竟是 不 是 真 正 的剪 接 位 点 , — 因 此 关键 在于 如何 更好 的提 取这 一段 序列 所蕴 涵 的统计 特 征及更 好 的设计 分 类算 法 。如果 把 剪接位 点 和两 侧 的编 码特 性结 合起 来分 析 , 有助 于提 高剪 接位 点 的识 别 效 果 。考 虑 到剪 接 位 点 附近 存 在 的 序列 保 守 则
别 模型 , HM— VM 工作 集最 优化 算法 训练 并优 化 HMM 模 型 , 成剪 接位 点识 别 系统 , 终 获得 了 用 S 生 最
较 高 的识别 精度 。 1 HMM( 隐马 尔可 夫模 型 ) 别算 法 识 HMM 是 一个 双重 随机模 型 , 底层 为一 个 马尔 可夫 链模 型 , 描述 状 态 之 间 的转 换 ; 上层 为一 个 随机 模 型, 描述 状态 与观 测值 的统 计对 应关 系 基 于 HMM 的模 式识 别方 法 的基本 思想 是对 于一 个 可用 HMM 描 述 的模 式识别 问题 , 其 可 能 出现 将
基于支持向量机基因剪接位点研究
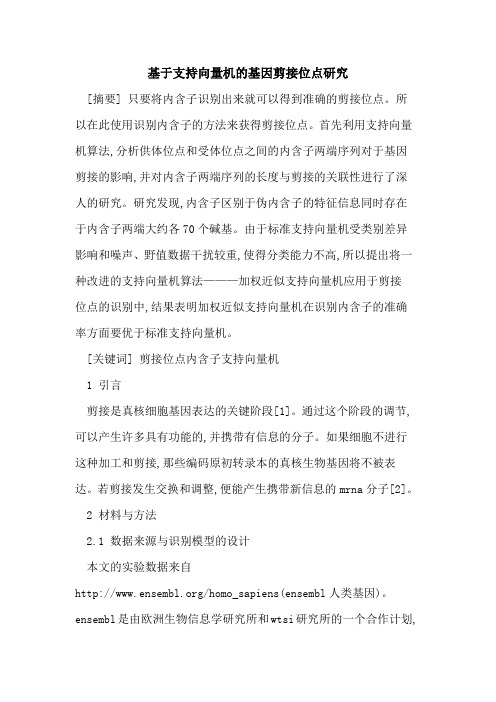
基于支持向量机的基因剪接位点研究[摘要] 只要将内含子识别出来就可以得到准确的剪接位点。
所以在此使用识别内含子的方法来获得剪接位点。
首先利用支持向量机算法,分析供体位点和受体位点之间的内含子两端序列对于基因剪接的影响,并对内含子两端序列的长度与剪接的关联性进行了深人的研究。
研究发现,内含子区别于伪内含子的特征信息同时存在于内含子两端大约各70个碱基。
由于标准支持向量机受类别差异影响和噪声、野值数据干扰较重,使得分类能力不高,所以提出将一种改进的支持向量机算法———加权近似支持向量机应用于剪接位点的识别中,结果表明加权近似支持向量机在识别内含子的准确率方面要优于标准支持向量机。
[关键词] 剪接位点内含子支持向量机1 引言剪接是真核细胞基因表达的关键阶段[1]。
通过这个阶段的调节,可以产生许多具有功能的,并携带有信息的分子。
如果细胞不进行这种加工和剪接,那些编码原初转录本的真核生物基因将不被表达。
若剪接发生交换和调整,便能产生携带新信息的mrna分子[2]。
2 材料与方法2.1 数据来源与识别模型的设计本文的实验数据来自/homo_sapiens(ensembl人类基因)。
ensembl是由欧洲生物信息学研究所和wtsi研究所的一个合作计划,它包括了9种生物的基因组。
ensembl计划维护了一个非编码rna 数据库,而且实时更新。
在剪接的过程中如果将内含子准确的识别出来,就可以得到剪接位点。
所以,在此就采用识别内含子的方法来得到剪接位点,识别模型如图1所示:图1 内含子识别模型2.2 训练集与测试集的组成从ensembl数据库中选取2000个满足以gt开始并以ag结束且序列长度大于144的真实内含子和4000个满足以gt开始并以ag 结束且序列长度大于144的虚假的内含子,然后按照一定长度截取组成相互独立的训练集和测试集。
如表1所示:表1 训练集与测试集的组成表1中训练集用于建立识别任务的数学模型,测试集用于检验所建模型的正确性。
《基于序列信息预测选择性剪接位点和盒式外显子》范文
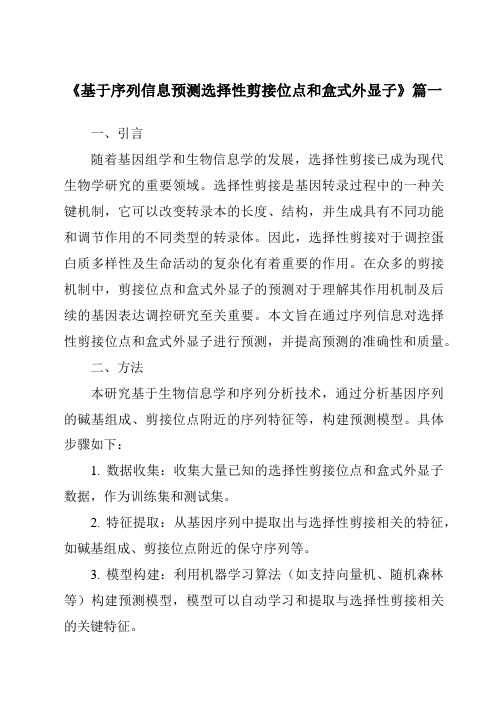
《基于序列信息预测选择性剪接位点和盒式外显子》篇一一、引言随着基因组学和生物信息学的发展,选择性剪接已成为现代生物学研究的重要领域。
选择性剪接是基因转录过程中的一种关键机制,它可以改变转录本的长度、结构,并生成具有不同功能和调节作用的不同类型的转录体。
因此,选择性剪接对于调控蛋白质多样性及生命活动的复杂化有着重要的作用。
在众多的剪接机制中,剪接位点和盒式外显子的预测对于理解其作用机制及后续的基因表达调控研究至关重要。
本文旨在通过序列信息对选择性剪接位点和盒式外显子进行预测,并提高预测的准确性和质量。
二、方法本研究基于生物信息学和序列分析技术,通过分析基因序列的碱基组成、剪接位点附近的序列特征等,构建预测模型。
具体步骤如下:1. 数据收集:收集大量已知的选择性剪接位点和盒式外显子数据,作为训练集和测试集。
2. 特征提取:从基因序列中提取出与选择性剪接相关的特征,如碱基组成、剪接位点附近的保守序列等。
3. 模型构建:利用机器学习算法(如支持向量机、随机森林等)构建预测模型,模型可以自动学习和提取与选择性剪接相关的关键特征。
4. 模型验证与优化:使用独立的测试集对模型进行验证和优化,不断调整模型的参数以提高预测的准确性和质量。
三、结果通过上述方法,我们成功构建了一个基于序列信息的选择性剪接位点和盒式外显子预测模型。
该模型在测试集上的预测准确率达到了较高的水平,为后续的基因表达调控研究提供了有力的支持。
具体结果如下:1. 剪接位点预测:我们的模型能够准确预测出选择性剪接位点的位置,并能够区分不同类型的剪接位点(如内含子保留型和外显子跳跃型)。
2. 盒式外显子预测:我们的模型能够准确识别出盒式外显子的序列特征,并预测其可能的功能和作用机制。
3. 模型性能评估:通过与已知的剪接位点和盒式外显子数据进行比较,我们发现我们的模型在预测准确率、灵敏度和特异性等方面均表现优异。
四、讨论本研究通过分析基因序列的碱基组成和剪接位点附近的序列特征,成功构建了一个基于序列信息的选择性剪接位点和盒式外显子预测模型。
《基于序列信息预测选择性剪接位点和盒式外显子》范文
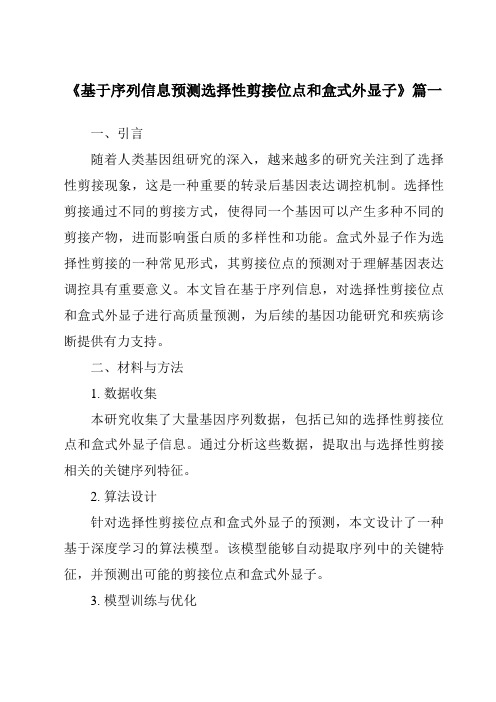
《基于序列信息预测选择性剪接位点和盒式外显子》篇一一、引言随着人类基因组研究的深入,越来越多的研究关注到了选择性剪接现象,这是一种重要的转录后基因表达调控机制。
选择性剪接通过不同的剪接方式,使得同一个基因可以产生多种不同的剪接产物,进而影响蛋白质的多样性和功能。
盒式外显子作为选择性剪接的一种常见形式,其剪接位点的预测对于理解基因表达调控具有重要意义。
本文旨在基于序列信息,对选择性剪接位点和盒式外显子进行高质量预测,为后续的基因功能研究和疾病诊断提供有力支持。
二、材料与方法1. 数据收集本研究收集了大量基因序列数据,包括已知的选择性剪接位点和盒式外显子信息。
通过分析这些数据,提取出与选择性剪接相关的关键序列特征。
2. 算法设计针对选择性剪接位点和盒式外显子的预测,本文设计了一种基于深度学习的算法模型。
该模型能够自动提取序列中的关键特征,并预测出可能的剪接位点和盒式外显子。
3. 模型训练与优化使用收集到的数据对模型进行训练和优化,通过调整模型参数和结构,提高预测的准确性和稳定性。
同时,采用交叉验证等方法对模型进行评估和验证。
三、结果与分析1. 预测结果通过模型预测,我们得到了大量的选择性剪接位点和盒式外显子信息。
与已知的数据库进行比对,发现我们的预测结果具有较高的准确性和可靠性。
2. 特征分析通过对预测结果进行特征分析,我们发现某些序列特征与选择性剪接位点和盒式外显子的存在具有显著相关性。
这些特征包括特定类型的碱基序列、剪接位点的保守性等。
这些发现为后续的基因功能研究和疾病诊断提供了重要线索。
3. 模型评估通过交叉验证等方法对模型进行评估,我们发现我们的模型在预测选择性剪接位点和盒式外显子方面具有较高的准确性和稳定性。
同时,我们还对模型的鲁棒性进行了测试,发现模型在不同类型的数据上均能保持良好的预测性能。
四、讨论与展望本研究基于序列信息对选择性剪接位点和盒式外显子进行了高质量预测,为后续的基因功能研究和疾病诊断提供了有力支持。
RNA剪接位点预测中的机器学习算法优化

RNA剪接位点预测中的机器学习算法优化第一章引言1.1 研究背景在生物学研究中,RNA剪接是一种常见的基因表达调控方式。
RNA剪接使得基因组内的同一段DNA可以产生多种不同的转录本,从而扩大了生物体内可译码蛋白质的种类和多样性。
而RNA剪接位点的准确预测,则对于理解基因功能、疾病诊断和治疗等方面具有重要意义。
1.2 问题陈述然而,由于剪接位点序列的复杂性和多样性,准确预测RNA剪接位点一直是一个具有挑战性的问题。
传统的方法在准确性和效率两个方面都存在一定的局限性。
因此,研究者们开始运用机器学习算法对RNA剪接位点进行预测,并取得了一定的成果。
1.3 研究目标本文旨在探讨机器学习算法在RNA剪接位点预测中的优化方法,进一步提高预测模型的准确性和效率。
第二章 RNA剪接位点的特征表示2.1 序列特征表示RNA剪接位点序列通常由核苷酸组成,因此可以将其转化为序列特征。
常用的序列特征包括短序列片段(k-mer)、核苷酸组合频率(nucleotide composition)等。
2.2 结构特征表示RNA剪接位点的结构特征可以通过RNA二级结构预测方法获得。
常用的结构特征包括碱基配对概率、互信息等。
2.3 组合特征表示将序列特征和结构特征进行组合,可以得到更加全面和准确的RNA剪接位点特征表示。
常用的组合特征表示方法包括特征拼接、特征加权等。
第三章机器学习算法在RNA剪接位点预测中的优化3.1 特征选择特征选择是指从原始特征集合中,选择出对于预测任务最重要的特征子集。
在RNA剪接位点预测中,特征选择可以帮助减少特征维度,提高模型训练的效率。
常用的特征选择方法包括相关性分析、互信息、卡方检验等。
3.2 机器学习算法选择不同的机器学习算法具有不同的特点和适应性。
在RNA剪接位点预测中,研究者们可以根据数据集的规模和特征情况选择合适的机器学习算法,如支持向量机(SVM)、随机森林(Random Forest)等。
条件随机场模型在基因序列分析中的应用(八)
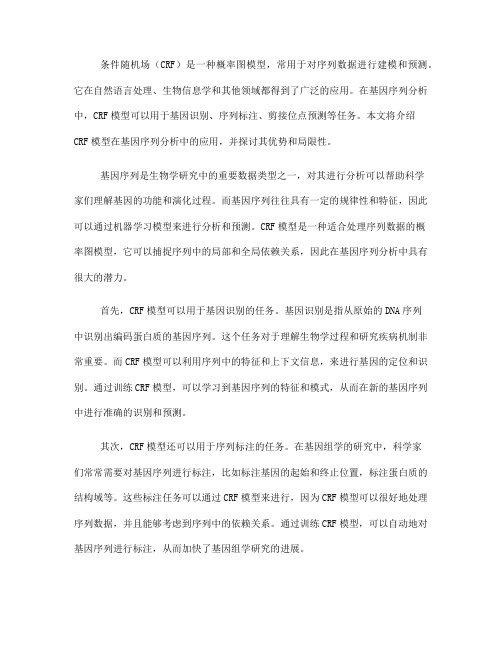
条件随机场(CRF)是一种概率图模型,常用于对序列数据进行建模和预测。
它在自然语言处理、生物信息学和其他领域都得到了广泛的应用。
在基因序列分析中,CRF模型可以用于基因识别、序列标注、剪接位点预测等任务。
本文将介绍CRF模型在基因序列分析中的应用,并探讨其优势和局限性。
基因序列是生物学研究中的重要数据类型之一,对其进行分析可以帮助科学家们理解基因的功能和演化过程。
而基因序列往往具有一定的规律性和特征,因此可以通过机器学习模型来进行分析和预测。
CRF模型是一种适合处理序列数据的概率图模型,它可以捕捉序列中的局部和全局依赖关系,因此在基因序列分析中具有很大的潜力。
首先,CRF模型可以用于基因识别的任务。
基因识别是指从原始的DNA序列中识别出编码蛋白质的基因序列。
这个任务对于理解生物学过程和研究疾病机制非常重要。
而CRF模型可以利用序列中的特征和上下文信息,来进行基因的定位和识别。
通过训练CRF模型,可以学习到基因序列的特征和模式,从而在新的基因序列中进行准确的识别和预测。
其次,CRF模型还可以用于序列标注的任务。
在基因组学的研究中,科学家们常常需要对基因序列进行标注,比如标注基因的起始和终止位置,标注蛋白质的结构域等。
这些标注任务可以通过CRF模型来进行,因为CRF模型可以很好地处理序列数据,并且能够考虑到序列中的依赖关系。
通过训练CRF模型,可以自动地对基因序列进行标注,从而加快了基因组学研究的进展。
此外,CRF模型还可以用于剪接位点预测的任务。
在基因的转录和翻译过程中,剪接位点的位置对于蛋白质的结构和功能具有重要的影响。
因此,预测剪接位点的准确性对于理解基因的功能和疾病机制非常重要。
CRF模型可以结合基因序列的特征和上下文信息,来进行剪接位点的预测。
通过训练CRF模型,可以得到预测准确度较高的剪接位点,从而帮助科学家们更好地理解基因的功能和调控机制。
然而,CRF模型在基因序列分析中也存在一些局限性。
剪接位点突变的表示方法
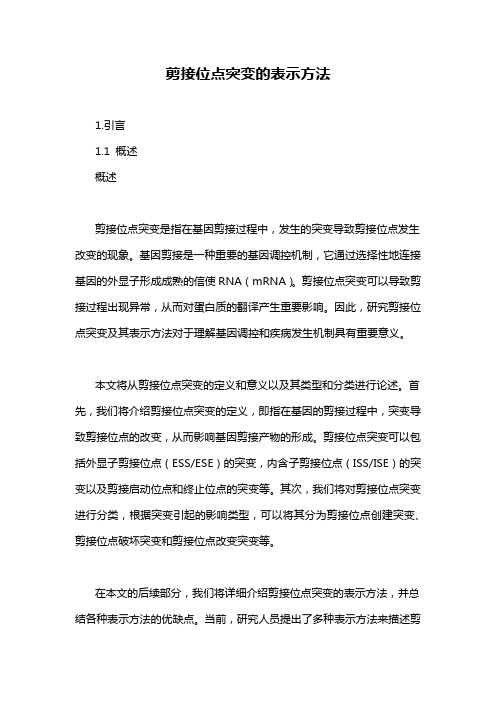
剪接位点突变的表示方法1.引言1.1 概述概述剪接位点突变是指在基因剪接过程中,发生的突变导致剪接位点发生改变的现象。
基因剪接是一种重要的基因调控机制,它通过选择性地连接基因的外显子形成成熟的信使RNA(mRNA)。
剪接位点突变可以导致剪接过程出现异常,从而对蛋白质的翻译产生重要影响。
因此,研究剪接位点突变及其表示方法对于理解基因调控和疾病发生机制具有重要意义。
本文将从剪接位点突变的定义和意义以及其类型和分类进行论述。
首先,我们将介绍剪接位点突变的定义,即指在基因的剪接过程中,突变导致剪接位点的改变,从而影响基因剪接产物的形成。
剪接位点突变可以包括外显子剪接位点(ESS/ESE)的突变,内含子剪接位点(ISS/ISE)的突变以及剪接启动位点和终止位点的突变等。
其次,我们将对剪接位点突变进行分类,根据突变引起的影响类型,可以将其分为剪接位点创建突变、剪接位点破坏突变和剪接位点改变突变等。
在本文的后续部分,我们将详细介绍剪接位点突变的表示方法,并总结各种表示方法的优缺点。
当前,研究人员提出了多种表示方法来描述剪接位点突变,包括序列比对方法、机器学习方法和结构建模方法等。
每种方法都有其适用的场景和局限性。
我们将评估各种表示方法在准确性、效率和易用性方面的表现,并讨论它们在不同研究领域的应用前景和研究方向。
综上所述,剪接位点突变的研究对于理解基因调控和疾病发生机制具有重要意义。
本文将对剪接位点突变的定义、分类和表示方法进行全面的介绍和总结,旨在为相关领域的研究人员提供参考,并推动该领域的进一步发展和深入探索。
1.2文章结构1.2 文章结构本文将按照以下几个部分来分析剪接位点突变的表示方法:1. 引言:在本部分中,我们将概述剪接位点突变的概念和研究意义,并介绍本篇文章的目的。
2. 正文:本部分将分为两个小节来讨论剪接位点突变的定义、意义以及类型和分类。
- 2.1 剪接位点突变的定义和意义:我们将详细解释什么是剪接位点突变,以及为什么对其进行研究具有重要意义。
可变剪接因子结合位点预测
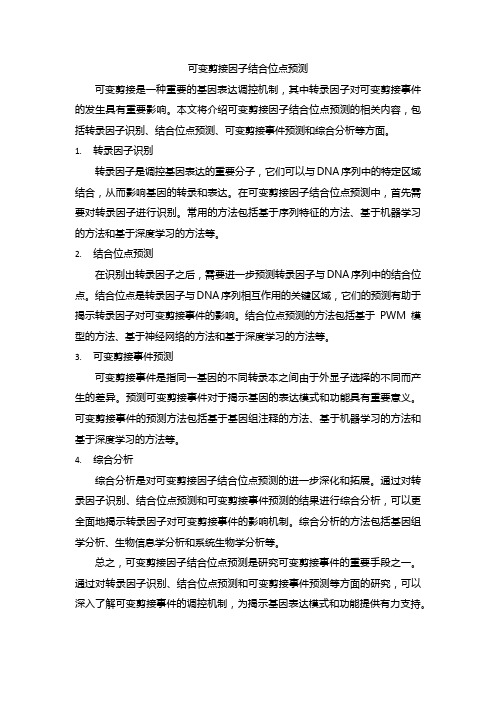
可变剪接因子结合位点预测可变剪接是一种重要的基因表达调控机制,其中转录因子对可变剪接事件的发生具有重要影响。
本文将介绍可变剪接因子结合位点预测的相关内容,包括转录因子识别、结合位点预测、可变剪接事件预测和综合分析等方面。
1.转录因子识别转录因子是调控基因表达的重要分子,它们可以与DNA序列中的特定区域结合,从而影响基因的转录和表达。
在可变剪接因子结合位点预测中,首先需要对转录因子进行识别。
常用的方法包括基于序列特征的方法、基于机器学习的方法和基于深度学习的方法等。
2.结合位点预测在识别出转录因子之后,需要进一步预测转录因子与DNA序列中的结合位点。
结合位点是转录因子与DNA序列相互作用的关键区域,它们的预测有助于揭示转录因子对可变剪接事件的影响。
结合位点预测的方法包括基于PWM模型的方法、基于神经网络的方法和基于深度学习的方法等。
3.可变剪接事件预测可变剪接事件是指同一基因的不同转录本之间由于外显子选择的不同而产生的差异。
预测可变剪接事件对于揭示基因的表达模式和功能具有重要意义。
可变剪接事件的预测方法包括基于基因组注释的方法、基于机器学习的方法和基于深度学习的方法等。
4.综合分析综合分析是对可变剪接因子结合位点预测的进一步深化和拓展。
通过对转录因子识别、结合位点预测和可变剪接事件预测的结果进行综合分析,可以更全面地揭示转录因子对可变剪接事件的影响机制。
综合分析的方法包括基因组学分析、生物信息学分析和系统生物学分析等。
总之,可变剪接因子结合位点预测是研究可变剪接事件的重要手段之一。
通过对转录因子识别、结合位点预测和可变剪接事件预测等方面的研究,可以深入了解可变剪接事件的调控机制,为揭示基因表达模式和功能提供有力支持。
同时,综合分析有助于将不同层次的研究结果进行整合,从而更全面地揭示转录因子对可变剪接事件的影响机制。
内含子的剪接位点具有的特征
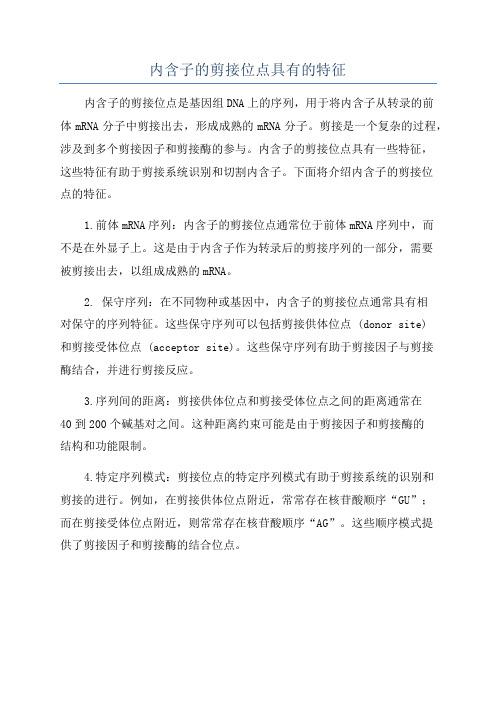
内含子的剪接位点具有的特征
内含子的剪接位点是基因组DNA上的序列,用于将内含子从转录的前
体mRNA分子中剪接出去,形成成熟的mRNA分子。
剪接是一个复杂的过程,涉及到多个剪接因子和剪接酶的参与。
内含子的剪接位点具有一些特征,
这些特征有助于剪接系统识别和切割内含子。
下面将介绍内含子的剪接位
点的特征。
1.前体mRNA序列:内含子的剪接位点通常位于前体mRNA序列中,而
不是在外显子上。
这是由于内含子作为转录后的剪接序列的一部分,需要
被剪接出去,以组成成熟的mRNA。
2. 保守序列:在不同物种或基因中,内含子的剪接位点通常具有相
对保守的序列特征。
这些保守序列可以包括剪接供体位点 (donor site)
和剪接受体位点 (acceptor site)。
这些保守序列有助于剪接因子与剪接
酶结合,并进行剪接反应。
3.序列间的距离:剪接供体位点和剪接受体位点之间的距离通常在
40到200个碱基对之间。
这种距离约束可能是由于剪接因子和剪接酶的
结构和功能限制。
4.特定序列模式:剪接位点的特定序列模式有助于剪接系统的识别和
剪接的进行。
例如,在剪接供体位点附近,常常存在核苷酸顺序“GU”;
而在剪接受体位点附近,则常常存在核苷酸顺序“AG”。
这些顺序模式提
供了剪接因子和剪接酶的结合位点。
《2024年基于序列信息预测选择性剪接位点和盒式外显子》范文
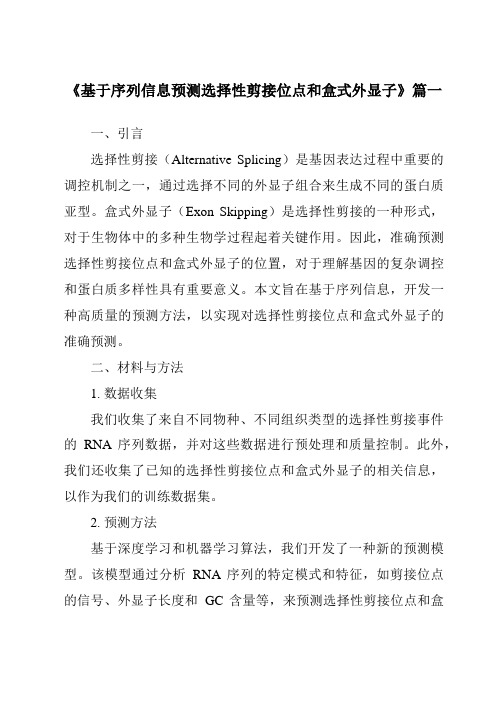
《基于序列信息预测选择性剪接位点和盒式外显子》篇一一、引言选择性剪接(Alternative Splicing)是基因表达过程中重要的调控机制之一,通过选择不同的外显子组合来生成不同的蛋白质亚型。
盒式外显子(Exon Skipping)是选择性剪接的一种形式,对于生物体中的多种生物学过程起着关键作用。
因此,准确预测选择性剪接位点和盒式外显子的位置,对于理解基因的复杂调控和蛋白质多样性具有重要意义。
本文旨在基于序列信息,开发一种高质量的预测方法,以实现对选择性剪接位点和盒式外显子的准确预测。
二、材料与方法1. 数据收集我们收集了来自不同物种、不同组织类型的选择性剪接事件的RNA序列数据,并对这些数据进行预处理和质量控制。
此外,我们还收集了已知的选择性剪接位点和盒式外显子的相关信息,以作为我们的训练数据集。
2. 预测方法基于深度学习和机器学习算法,我们开发了一种新的预测模型。
该模型通过分析RNA序列的特定模式和特征,如剪接位点的信号、外显子长度和GC含量等,来预测选择性剪接位点和盒式外显子的位置。
我们使用大量的正负样本进行训练和优化,以提高模型的准确性和泛化能力。
3. 模型评估我们使用交叉验证和独立测试集来评估模型的性能。
通过计算敏感性、特异性、精确度和F1值等指标,我们评估了模型在预测选择性剪接位点和盒式外显子方面的准确性。
三、结果与讨论1. 预测结果我们的模型在测试集上取得了较高的预测准确率,能够有效地识别出选择性剪接位点和盒式外显子的位置。
与传统的预测方法相比,我们的模型在敏感性和特异性方面均表现出较好的性能。
2. 结果分析通过对预测结果的分析,我们发现RNA序列的特定模式和特征与选择性剪接位点和盒式外显子的位置密切相关。
我们的模型能够有效地捕捉这些特征,从而提高预测的准确性。
此外,我们还发现不同物种和不同组织类型的RNA序列在选择性剪接方面存在差异,这为进一步研究基因表达调控和蛋白质多样性提供了新的思路。
剪切位点预测方法
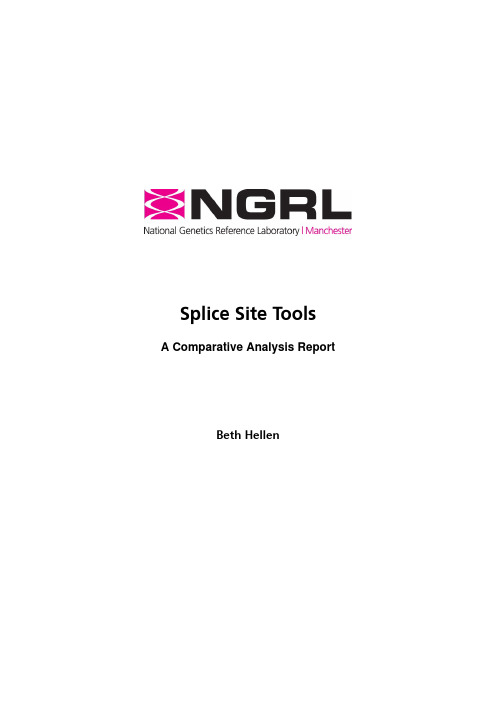
Splice Site Tools A Comparative Analysis ReportBeth HellenContentsIntroduction 3 Methods 4 Results 5 Conclusions 9 References 10 Appendix 1 Variants found in literature 11IntroductionSplicing is a process which modifies mRNA after transcription. It allows for introns to beremoved and exons joined together to form mature mRNA, ready for translation into protein.The splice site junction, found where an intron meets an exon, contains multiple sequence motifs. These motifs provide signals to allow for correct splicing to occur. The best characterisedof these are the acceptor and donor splice site signals. These signals consist of invariant dinucleotides at positions +1, +2, -1 and -2 of the intron and less well conserved nucleotides both within the immediate adjoining exonic sequence and deeper into the intron from the +3 and -3 positions (Seif et al., 1979). The specific splicing of a gene can be easily affected by mutations in the sequence surrounding the splice site junction. This can lead to alternate splicing and thus adversely affect the translated protein (Novoyatleva et al., 2006; Tazi et al., 2009).In-silico splice site prediction tools can be used to predict the effect of a genetic variant on splicing. A large number of prediction tools are currently available, either as standalone programs or as part of the Alamut (http://www.interactive-/alamut/doc/1.5/index.html) or Human Splicing Finder (Desmet, 2009) interfaces. Some small analyses of these algorithms have been carried out, but no large scale analyses (Hartmann et al., 2008; Holler et al., 2009; Houdayer et al., 2008). Although the UV guidelines (Bell et al., 2007) provided by the CMGS (/) suggest several splice site prediction algorithms, the performance of these algorithms have not been formally assessed and may give divergent results. This analysis aims to provide an assessment of the performance of these algorithms in the prediction of splicing-related variant pathogenicity. It will also assess the scope of the splice-site prediction tools to ensure that they can be used in the most appropriate way. The analysis will allow scientists to use splice site prediction tools in the prediction of pathogenesis with more confidence.In this analysis, six of the most common donor and acceptor prediction algorithms have been assessed for their ability to predict the pathogenicity of splice site variants. The algorithms chosen were those suggested by the UV guidelines, plus MaxEntScan, which are used as part of the Alamut and HSF splicing interfaces. The six algorithms were: GeneSplicer (Pertea et al., 2001), Human Splicing Finder (HSF) (Desmet et al., 2009), MaxEntScan (Yeo & Burge, 2004), NetGene2 (Brunak et al., 1991), NNSplice (Reese et al., 1997) and SSFL, an algorithm based on Alex Dong Li’s Splice Site Finder (no longer available). In each algorithm the splice signal given by the wild type sequence is compared to the splice site signal given by a mutated sequence supplied by the user.MethodsSix algorithms were assessed for their ability to predict disruption to normal splicing patterns, caused by genetic variants. SSFL, MaxEntScan, NNSplice and GeneSplicer were accessed through the Alamut interface. HSF and a second implementation of MaxEntScan were accessed through the HSF interface. Netgene2 was implemented using a stand alone web interface. The majority of these methods were chosen because they had been recommended by the UV guidelines; MaxEntScan was included because it is used in both the HSF and Alamut splicing interfaces. A set of 265 pathogenic variants and 15 non-pathogenic variants from a total of 180 genes (see figure 1 and appendix 1) were retrieved from the literature. These variants were used to assess the splice site prediction algorithms using their default settings and recommended lengths of sequence. Sensitivity (equation 1), specificity (equation 2) and accuracy (equation 3) werecalculated, as were the standard errors for each of the statistics. For the purposes of this analysis a true positive was defined as a pathogenic variant correctly classified as pathogenic and a true negative was a non-pathogenic variant correctly classified as non-pathogenic. A change in splice site signal of ≥10% was considered to predict a pathogenic effect.(1)(2)(3)A second set of sensitivity, specificity and accuracy calculations were made for those variants which did not fall into the invariant di-nucleotide positions at -1, -2, +1, +2. The datasetconsisted of 110 pathogenic variants and 15 non-pathogenic variants. The variants occurred in 83 different genes. This analysis will allow the algorithms to be assessed on their performance with the more difficult splice site variants.The UV guidelines for splice site analysis recommend the use of three prediction algorithms to give a consensus prediction. Combinations of three high performing algorithms were compared to determine whether the accuracy was improved. The criteria required to categorise a variant as pathogenic or non-pathogenic was that at least two of the algorithms must agree on the prediction. The accuracy scores were calculated and compared to those given by the single algorithms.To test the range of predictions made by the algorithms at each intronic position near the splice site junction, an in-silico analysis was performed. Thirteen acceptor and donor splice sitejunctions from BRCA1 and BRCA2 were analysed. Only junctions where the wild type splice site signal was found by all four of the highest performing algorithms were used. The wild type base at each position from +1 to +10 or -1 to -10 was artificially mutated in-silico to each of theremaining 3 nucleotides and the proportional change in splice site signal given by each algorithm was recorded. The mean change in splice site prediction (equation 4) at each position was plotted for each algorithm. The mean change in splice site signal strength is described inequation 4, where SS M is the mutated splice site signal, SS W is the wild type splice site signal and N is the number of examples analysed.(4)ResultsPathogenic and non-pathogenic splice site related variants retrieved from the literature were found at a range of positions relative to the splice site junction (Figure 1). The majority of splice site related pathogenic mutations used in this analysis were found within intronic positionsbetween 1 and 10 nucleotides from the splice site junction. However, >40 of the variants were found in positions within the exon, and pathogenic mutations were also found at >100bp from the splice site junction. Only 15 non-pathogenic variants were found and they mainly occurred at positions further from the splice site junction. The small number of non-pathogenic variants arises from the problem of non-reporting of negative results. This is likely to increase the error associated with the specificity scores.-40-2002040010203040506070Intronic_positionF r e q u e n cyFigure 1 Chart showing the position of variants retrieved from the literature. Variants in exonic positions are shown at 0, variants >50bp from the splice site junction are binned and represented as asingle frequency at 50bp from the splice site. Black lines represent the frequency of pathogenic variants and red lines represent the frequency of non-pathogenic variants.The sensitivity, specificity and accuracy scores showed that the four highest performing algorithms were NNSplice, MaxEntScan, GeneSplicer and SSFL (Figure 2). These algorithmsachieved between 80 and 92% accuracy and sensitivity. The specificity scores (between 73 and 93%) were less reliable due to the smaller number of variants tested. These four algorithms are those implemented through the Alamut interface. It is possible that the ease of interpretation of the results, when using the Alamut interface, has influenced this result. With the HSF interface it was more difficult to determine the predicted difference in splice site signal.Figure 2Accuracy, Sensitivity and Specificity values for each of the splice site prediction algorithms tested. Sensitivity measures the ability to predict pathogenic variants (TP) and specificity measures the ability to predict non-pathogenic variants (TN).The removal of variants occurring at +1, +2, -1 and -2 positions reduced the performance of the algorithms, as was expected (Figure 3). However, two algorithms (MaxEntScan & NNSplice) still achieved an accuracy score of >80%. Therefore it can be seen that these algorithms perform reasonably well, even with variants where it is more difficult to predict the splicing effect.Figure 3Accuracy, Sensitivity and Specificity values for each of the splice site prediction algorithms tested. Only variants which did not occur at one of the +1, +2,-1 or -2 positions were analysed.The accuracy given by the consensus prediction of splice site signals was found to be between 86% and 92% for all combinations (Figure 4). The highest accuracy obtained through a consensus method was comparable to that given by MaxEntScan when implemented through Alamut. None of the consensus methods achieved an accuracy that was significantly higher than the individual algorithms.SSFL MES NNSPlice GenesplicerGroup 1 X X XGroup 2 X X XGroup 3 X X XGroup 4 X X XFigure 4 The chart shows the accuracy obtained by combining results from three algorithms and using the consensus to predict pathogenicity of variants. The accompanying table describes the combinations of programs used in each consensus group.Genetic variants which occur in the invariant dinucleotides at -1, -2, +1 and +2 were predicted to always disrupt splice site signalling (Figure 5). This would be assumed by most users and so no further information is gained by using the splice site prediction tools at these positions. The algorithms were shown to be the most useful for the prediction of both pathogenic and non-pathogenic splice site variants when applied to positions between +3 and +7 and -3 to at least -10 (Figure 5). At positions further from the splice site junction, no disruption in splice site signal was seen. The scope of these tools can therefore be defined as the prediction of the disruption of splice sites within these regions. The effect of variants on splice sites further than this cannot be predicted by any of the algorithms. The tools are, however, able to predict new splice sites at other positions. This could occur if the variant caused the sequence surrounding the new splice site to become a closer match to the statistical models used by the tools.Figure 5 Graphs showing the proportional signal strength change on known splice sites when a mutation was introduced at positions in the intron between -1 and -10 or between +1 and +10. A score of 1 indicates that no disruption in the splice site signal was observed, a score of 0 indicates that the signal was completely destroyed. Lines between points have been added to ease interpretation although the data is discrete.ConclusionsThe four algorithms used in Alamut were shown to have a high degree of accuracy and users can be confident in the safe interpretation of these results as part of the assessment of a variant. It should still be noted that the algorithms alone are not sufficient evidence for a clinical decision.These algorithms, with the exception of SSFL, can be used as standalone web tools as well as via the Alamut interface. However, the results obtained through alternative implementations may differ, as shown by the MaxEntScan results obtained through Alamut and HSF.The range of splice site signal strength predictions given by the algorithms is determined by the position of the variant. At +1, +2, -1 or -2 the algorithms always predict a large change in splice site signal, as would be predicted by experts. Variations in the wild type sequence further than +7 or -10 from the splice site junction do not cause any reduction in the wild type splice site signal predicted by the algorithms. Variants found between these two regions show a range of splice site reduction predicted by the algorithms and it is in this range that the algorithms are likely to be the most useful. This mirrors the reduction in occurrence of pathogenic variants found in the literature at these positions. The algorithms are still useful for prediction of splice site signals related to variants further into the intron, however it is only new splice sites which can be detected, not the reduction in wild type splice sites.Although the use of three different algorithms is suggested in the UV guidelines, the accuracy was not improved by using a consensus method, therefore there does not seem to be a need for this step. However, as the Alamut interface performs all four analyses simultaneously, it is easy to compare predictions without a formal consensus method. The Alamut interface also contains methods to predict splicing enhancer or silencer motifs (ESE, ESS etc.) and branch point motifs. These methods have not been assessed and as the mechanisms by which these motifs regulate splicing are less clearly understood, the methods should be only be used with caution.ReferencesBell, J., Bodmer, D., Sistermans, E., Ramsden, S. (2007) Practice guidelines for the interpretation and reporting of unclassified variants in clinical molecular genetics. Available:/BPGs/pdfs current bpgs/UV GUIDELINES ratified.pdfBrunak, S., Engelbrecht, J., Knudsen, S. (1991) Prediction of human mRNA donor and acceptor sites from the DNA sequence. J. Mol. Biol., 220:49-65.Desmet, F.O., Hamroun, D., Lalande, M., Collod-Béroud, G., Claustres, M., Béroud, C. (2009) Human Splicing Finder: an online bioinformatics tool to predict splicing signals. Nucleic Acids Res., 37(9):e67.Hartmann, L., Theiss, S., Niederacher, D., Schaal, H. (2008) Diagnosis of pathogenic splicing mutations: does bioinformatics cover all bases? Front Biosci., 13:3252-72.Holla, Ø. L., Nakken, S., Mattingsdal, M., Ranheim, T., Berge, K.E., Defesche, J.C., Leren, T.P. (2009) Effects of intronic mutations in the LDLR gene on pre-mRNA splicing: Comparison of wet-lab and bioinformatics analyses. Mol. Genet. Metab., 96(4):245-252.Houdayer, C., Dehainault, C., Mattler, C., Michaux, D., Caux-Moncoutier, V., Pagès-Berhouet, S., d’Enghien, C.D., Laugé, A., Castera, L., Cauthier-Villars, M., Stoppa-Lyonnet, D. (2008) Evalutation of in silico splice tools for decision-making in molecular diagnosis. Hum. Mutat.,29(7): 975-82.Novoyatleva, T., Tang, Y., Rafalska, I., Stamm, S. (2006) Pre-mRNA missplicing as a cause of human disease. Prog. Mol. Subcell. Biol., 44:27-46.Pertea, M., Lin, X., Salzberg, S.L. (2001) GeneSplicer: a new computational method for splice site prediction. Nucleic Acids Res., 29(5):1185-90.Reese, M.G., Eeckman, F.H., Kulp, D., Haussler, D. (1997) Improved splice site detection in Genie. J. Comp. Biol., 4(3):311-23.Seif, I., Khoury, G., Dhar, R. (1979) BKV splice sequences based on analysis of preferred donor and acceptor sites. Nucleic Acids Res., 6(10):3387-98.Tazi, J., Bakkour, N., Stamm, S. (2009) Alternative splicing and disease. Biochim. Biophys. Acta., 1792(1):14-26.Yeo, G. and Burge, C.B. (2004) Maximum entropy modelling of short sequence motifs with applications to RNA splicing signals. J. Comput. Biol., 11(2-3):377-394.Appendix 1 Variants found in literatureTable 1The number of pathogenic and non-pathogenic variants found for each gene in the literature search.Gene # PathogenicVariants# Non-PathogenicVariantsGene # PathogenicVariants# Non-PathogenicVariantsAAA S 1 KLK8 1A BCA 1 1 KRIT1 1A BCA 4 2 KRT1 1A CA DVL 1 L1CAM 0 2A CA T1 1 LDLR 4 2A COX1 1 LHB 1A IP 1 LMNA 2A IRE 1 LPIN2 1A LDOB 1 MANBA 1A LS2 2 MAPT 1A PC 1 MCOLN1 1A POA 5 1 MECP2 1A POB 2 MEN1 1A RSA 1 MERTK 1A RSB 2 MFSD8 2A TM 3 MIP 1A TP2C1 3 MPV17 1A TP7B 2 MPZ 1BRCA 1 11 3 MSH2 1BRCA 2 17 MSX1 1BTK 5 MTM1 1CA SR 1 MYBPC3 1CDH23 1 MYO15A 2CERKL 1 MYO7A 1CETP 1 NF1 1CHM 1 NPC1 1CHRNA 1 1 NR2E3 1COG1 1 OTC 1COG7 1 PAH 1COL1A 1 2 PAK3 1COL4A 3 1 PCCA 3COL7A 1 1 PCCB 2COL8A 2 0 2 PDHA1 1CRYBA 1 1 PHEX 3CTSK 1 PHYH 1CYBA 1 PITX2 2CYBB 5 PKHD1 1CYP11A 1 1 PMM2 3DDC 1 PMS2 1DFNA 5 1 POMGNT1 1DGUOK 1 POU1F1 1DMD 2 PPOX 1DOK7 1 PROP1 1DSPP 11 PRPF31 2EDA 1 PTEN 1EFNB1 1 PYGM 6ERCC3 1 RAPSN 1ERCC8 1 RB1 3 1F11 2 REEP1 1F13A 1 1 RHO 1F5 2 RS1 3FA S 1 RSPO1 1FBN1 1 SBDS 1FECH 2 SETX 1FGB 1 SLC12A3 1FGFR1 2 SLC25A20 2Table 1 Continued...Gene # PathogenicVariants# Non-PathogenicVariantsGene# PathogenicVariants# Non-PathogenicVariantsFTSJ1 1 SLC26A4 5GA MT 1 SLC40A1 1GBA 2 SLC4A11 2GBE1 1 SMARCB1 1GDA P1 1 SPAST 1GHR 1 SPG11 1GHRHR 1 SPINK1 1GLB1 21 SPR 1GLRX5 1 STK11 1 1GNPTA B 1 TCIRG1 1GNPTG 1 TFR2 1GNS 1 TG 8GRN 22 TGM1 1HBB 1 TMC1 1HEXB 3 TMEM67 1HMGCL 2 TNFRSF1A 2IDS 11 TRAPPC2 1IGHMBP2 1 TREM2 1IKBKA P 1 UPB1 2ITPA 2 VCAN 1IVD 1 VPS33B 1KCNH2 2 WT1 2KCNQ1 1 XK 1KIF5A 1 ZMPSTE24 1。
生物博士论文内含子保留型可变剪切的识别方法和相关特征研究
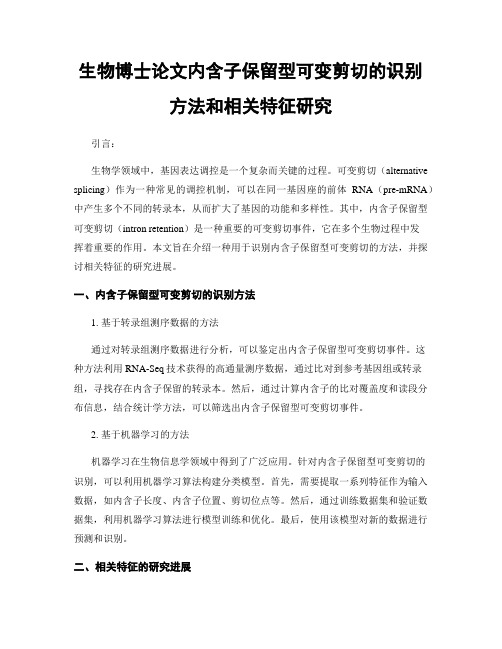
生物博士论文内含子保留型可变剪切的识别方法和相关特征研究引言:生物学领域中,基因表达调控是一个复杂而关键的过程。
可变剪切(alternative splicing)作为一种常见的调控机制,可以在同一基因座的前体RNA(pre-mRNA)中产生多个不同的转录本,从而扩大了基因的功能和多样性。
其中,内含子保留型可变剪切(intron retention)是一种重要的可变剪切事件,它在多个生物过程中发挥着重要的作用。
本文旨在介绍一种用于识别内含子保留型可变剪切的方法,并探讨相关特征的研究进展。
一、内含子保留型可变剪切的识别方法1. 基于转录组测序数据的方法通过对转录组测序数据进行分析,可以鉴定出内含子保留型可变剪切事件。
这种方法利用RNA-Seq技术获得的高通量测序数据,通过比对到参考基因组或转录组,寻找存在内含子保留的转录本。
然后,通过计算内含子的比对覆盖度和读段分布信息,结合统计学方法,可以筛选出内含子保留型可变剪切事件。
2. 基于机器学习的方法机器学习在生物信息学领域中得到了广泛应用。
针对内含子保留型可变剪切的识别,可以利用机器学习算法构建分类模型。
首先,需要提取一系列特征作为输入数据,如内含子长度、内含子位置、剪切位点等。
然后,通过训练数据集和验证数据集,利用机器学习算法进行模型训练和优化。
最后,使用该模型对新的数据进行预测和识别。
二、相关特征的研究进展1. 内含子长度内含子长度是影响内含子保留型可变剪切的重要特征之一。
研究发现,相对于其他可变剪切事件,内含子保留型可变剪切的内含子长度通常更长。
这可能与内含子保留型可变剪切需要保留较长的内含子序列有关。
2. 内含子位置内含子位置也是一个重要的特征。
研究表明,在内含子保留型可变剪切事件中,内含子通常位于转录本的5'端或3'端。
这可能与内含子保留型可变剪切在调控基因表达和蛋白质功能方面的重要作用有关。
3. 剪切位点剪切位点是内含子保留型可变剪切的关键特征之一。
剪切因子识别序列
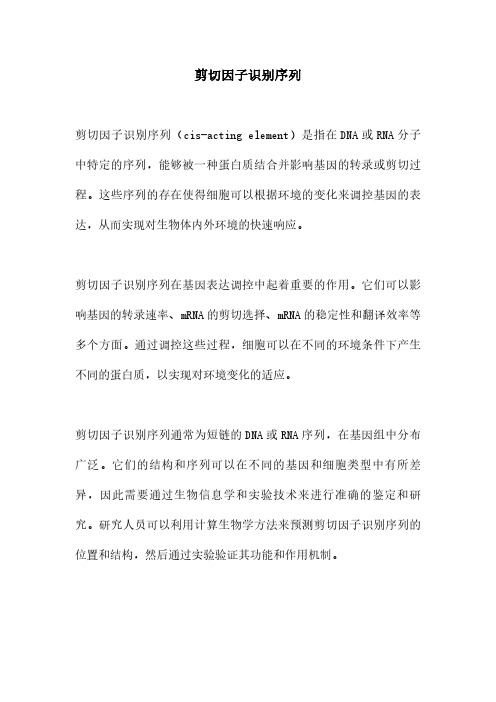
剪切因子识别序列
剪切因子识别序列(cis-acting element)是指在DNA或RNA分子中特定的序列,能够被一种蛋白质结合并影响基因的转录或剪切过程。
这些序列的存在使得细胞可以根据环境的变化来调控基因的表达,从而实现对生物体内外环境的快速响应。
剪切因子识别序列在基因表达调控中起着重要的作用。
它们可以影响基因的转录速率、mRNA的剪切选择、mRNA的稳定性和翻译效率等多个方面。
通过调控这些过程,细胞可以在不同的环境条件下产生不同的蛋白质,以实现对环境变化的适应。
剪切因子识别序列通常为短链的DNA或RNA序列,在基因组中分布广泛。
它们的结构和序列可以在不同的基因和细胞类型中有所差异,因此需要通过生物信息学和实验技术来进行准确的鉴定和研究。
研究人员可以利用计算生物学方法来预测剪切因子识别序列的位置和结构,然后通过实验验证其功能和作用机制。
剪切因子识别序列的研究对于理解基因表达调控的机制具有重要的意义。
它不仅有助于揭示细胞在不同环境条件下如何调整基因表达,还可以为疾病的发生和治疗提供重要的参考。
许多疾病如癌症、免疫疾病和神经系统疾病都与基因表达调控的异常有关,因此对剪切因子识别序列的研究也有助于揭示这些疾病的发病机制和寻找新的治疗方法。
总之,剪切因子识别序列是基因表达调控中的重要因素,它们可以影响基因的转录和剪切过程,从而调控蛋白质的表达和功能。
通过对剪切因子识别序列的研究,我们可以更深入地了解基因表达调控的机制,为疾病的治疗提供新的思路和方法。
人类基因组中选择性剪接位点的预测及序列特征分析的开题报告

人类基因组中选择性剪接位点的预测及序列特征分
析的开题报告
一、选题背景
随着基因组学技术的发展和生物信息学的应用,越来越多的基因组
序列被测序和注释。
选择性剪接是一种常见的RNA后转录修饰过程,对
于多种生物体细胞的生长发育以及疾病的发生等方面起着重要的调控作用。
因此,选择性剪接位点的预测和序列特征分析是基因组学和生物信
息学的热点之一。
二、研究目的
本研究旨在开发一种基于机器学习算法的选择性剪接位点预测模型,同时对不同类型的选择性剪接位点的序列特征进行分析,深入了解其生
物学功能和调控机制。
三、研究内容
(1)收集和整合不同物种的RNA测序数据及其相应的剪接注释信息。
(2)采用多种特征筛选和选择性剪接事件的分类算法,建立选择性剪接位点预测模型,并通过交叉验证和测试数据集对模型进行验证和优化。
(3)在预测模型的基础上,对不同类型的选择性剪接位点的序列特征进行分析,包括剪接区域的保守性、二级结构、启动子元件和转录因
子结合位点等。
(4)对模型预测出的结果进行生物学功能验证,例如通过RT-PCR、Western Blot等方法验证预测的选择性剪接事件是否存在,以及对选择性剪接变异所涉及的基因和代谢通路等进行深入探讨。
四、研究意义
选择性剪接位点的预测和序列特征分析对于深入理解基因调控机制、揭示相关疾病的发生发展和预测基因功能具有重要的意义。
本研究将为
预测选择性剪接位点提供一种可靠的预测模型,并深入探究选择性剪接
事件背后的生物学机理,为基因组学和生物信息学的发展提供新的思路
和方法。
- 1、下载文档前请自行甄别文档内容的完整性,平台不提供额外的编辑、内容补充、找答案等附加服务。
- 2、"仅部分预览"的文档,不可在线预览部分如存在完整性等问题,可反馈申请退款(可完整预览的文档不适用该条件!)。
- 3、如文档侵犯您的权益,请联系客服反馈,我们会尽快为您处理(人工客服工作时间:9:00-18:30)。
t a po ait, d e ro dlHMM)a d u p r V co c ie ( V i l rb bl Hidn Mak v Mo e ( c i y n S p o etr Mahn s S M) ec c rig o h cr lt n b- t t. odn t e or a o e Ac t e i
Ke r s s l e st e o nt n ma hn e r ig s t t a e trs y wo d : pi i rc g io ; c ie lan ;t i i lfaue c e i n a sc
摘
要: 依据 剪接 位 点附近存 在 的序 列保 守性 出现 了多种机 器学 习识 别方 法 , 如基 于统计 概率 的方 法 、 于 隐马 尔可 夫模 型 基
( i e ro dl MM) Hd nMa vMo e H d k , 的方法和基 于支持向量机(upr V c rMah e,V 的方法等, Spo et ci sS M) t o n 这些方法识别精度较高, 但算法过程复杂。基于剪接位点附近碱基之间的相关性和统计特征, 构造 了一种固定位点上碱基 间的网络结构 图, 并在此网络
to fma hn e rig wi ih rp e iin,u e p o e s o loi m s mo e c mp e sc sm eh d b sd o tt — in o c ie lann t hg e rcso b tt rc s f ag rt h h h i r o lx,u h a to a e n sai s
t e p ie sts a d sait a faue ,hs p p r p ee t tt tc lfau e m eh d whc s b s d o tu tr lda n we n s l i n ttsi l e trs t i a e r sns a sai ia e tr to ih i a e n srcu a rwig c e c s n t r ewe n te b ss tss a d e au ts te me o efr n c s g N2 9 a b s.h x ei na eut h w a ewok b t e ae ,et h n v lae t d p roma e u i 6 d t a eT e e p r h h n a me tlrsls s o t t h
S h o f Co ue ce c & T c n lg S o h w iest S z o Ja g u 2 0 6, ia c o lo mp tr S in e e h oo y, o c o Un v ri y, u h u,in s 0 Chn 1 5
LI S a y n, NG e.d n fc to f s l e sts a e o r b b l y sa s c l fau e . mpue gn e i g a d h o a DE W i e t ain o p i i b s d n p o a i t tt t a e t r sCo I i i c e i ii t r En ie rn n
teme o rp sd hsbtrpe r ac r ii eut wt esp r t sad ̄g e peio a te lo tms h t d po oe a e e r o n ei pe co rsl i ls aa e h t fm n d tn s h me r n h r r s n tn o ragrh . ci h h i
结构 图的基础 上提 出了基 于概 率统计特征 的剪接 位点识别计 算公 式 , 利用 N 6 数据库 对识别方 法和其他传统方 法的性能进行 29
了比较。实验结果表明, 基于概率统计特征的方法预测人类的剪接位点, 有较好的预测效果, 与其他的一些算法相比, 表现出参
数少 , 精度 高等优 点。
C m u r ni ei d p laos o p t gn r g n A pi t n计算机工程与应用 eE e n a ci
一
种基于概率统计特征的剪接位点识别方法
伟
李绍燕 , 邓
L S a y n, NG e I h o a DE W i
苏州大学 计算机科学与技术学院, 江苏 苏州 250 106
关键词: 剪接位点识别; 机器学习; 概率统计特征 - D I1. 78 s . 0.3 1 0 1 1 5 文章编号 :0283 (0 13-120 文献标识码 : 中图分类号 :P 9. O :0 78 .s 1 2 3. 1. . 2 3 in 0 8 2 3 0 10.3 1 1)1 8. 2 0 3 A T 31 4
1 引言 随着人类基因组计划的完成, 人类进入了后基因组时代 , 有关基因组结构分析 , 基因识别及其功能预测的研究在生物 信息学界也越来越重要。R A剪接是真核生物基因表达过程 N 中的一个重要步骤。精确预测剪接位点是真核基因系统研究 的第一步 , 如果能准确预测到剪接位点就可以定位D A序列 N 中的编码区, 有助于基因的标注、 新基因的发现和对应蛋白质
Ap l a o s2 1 ,7 3 )1 21 4 p ct n ,0 1 4 ( 1 :8 ・8 . i i
Ab ta t Ac odn o te sait a e trs o o sn u sq e c s ao n p ie sts tee e it ait f ie t e - sr c : c r ig t h tt i l fau e fc n e s s e u n e r u d s l i ,h r x ss a v re o d ni a sc c e y l f