CONSIDERING TOPOLOGY IN THE CLUSTERING OF SELF-ORGANIZING MAPS 1
DCPC_基于能量保护的传感器网络分布式拓扑控制协议

护协议, 它加强了节点之间链接的可靠性。使用该协议, 一个 节点就能够判断它的 父节点 何时失效 , 进 而根据 拓扑信 息计 算出到达簇头节点的替代路径; 然后节点通知簇头, 簇头更新 成簇搜索树。如果该节 点找不 到替代 路径, 则开始 一个标 示 进程, 将该节点的子树的所有节点变为一个独立的簇。
尽管现有的许多协 议在减少能量消耗进而提高能源有效
性的道路上做出了自 己的贡 献, 然 而这些 协议并 没有有 效地 解决网络生命周期的问题。在网络中存在 着这么一些特定的
节点, 它们总是位于通讯 的链路 中。虽然动 态路由 通过将 数 据送往剩余能量较多的 节点能够 解决该! 热点∀ 问题, 但 是它 同时带来了极大的网络 延迟和路由回路问题。
从图论的角度出发, 拓 扑管理 中的节 能策略 本质是 通过 功率管理策略保持一个较 小的始 终处于 活动状态 的节点 集, 这些节点构 成 一个 连 通支 配 集 ( Co nnected Do mination Set. CDS) ( 网络中节点要么属于 CDS, 要么是 CDS 中某 个节点的 一跳邻居) , 它保 证了 网络 的一 般连 通性。 报文 在通 过 CDS 时没有延时开销, 其 它的节 点在 CDS 生 成后 进入睡 眠状 态, 以实现能量保护。因此拓扑管理策略的核心问题是如何生成 CDS。分布式的 CDS 生成 策略 的关 键环 节是 邻居 节点 之间 如何根据本地信息来决定自己是否成 为簇头。我们 的 DCPC 协议采用确定性准则选取 簇头, 基于剩 余能量 和节点 度数来 计算节点成为簇头的概率。
为了提高能源的有效性, 进而延长网络的生命周期, 簇内 通讯消耗被当作次要 的成簇 变量, 它可以 被看作 是邻居 密度 或者成簇密度的函数; 同时, 它被用作解决 节点多个从属关系 问题的判断条件。这里我们将节点的度数 作为簇头选择的次 要因素。一个节点的度数是指所有距离这 个节点一跳的邻居
复杂网络演化博弈理论研究综述
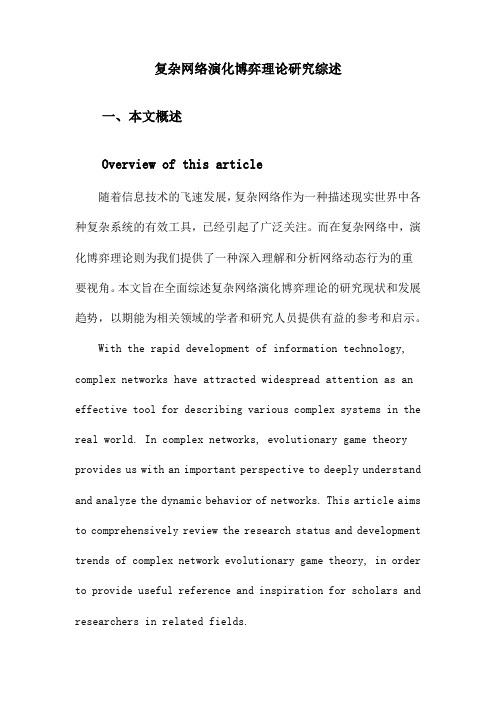
复杂网络演化博弈理论研究综述一、本文概述Overview of this article随着信息技术的飞速发展,复杂网络作为一种描述现实世界中各种复杂系统的有效工具,已经引起了广泛关注。
而在复杂网络中,演化博弈理论则为我们提供了一种深入理解和分析网络动态行为的重要视角。
本文旨在全面综述复杂网络演化博弈理论的研究现状和发展趋势,以期能为相关领域的学者和研究人员提供有益的参考和启示。
With the rapid development of information technology, complex networks have attracted widespread attention as an effective tool for describing various complex systems in the real world. In complex networks, evolutionary game theory provides us with an important perspective to deeply understand and analyze the dynamic behavior of networks. This article aims to comprehensively review the research status and development trends of complex network evolutionary game theory, in order to provide useful reference and inspiration for scholars and researchers in related fields.本文首先回顾了复杂网络和演化博弈理论的基本概念和研究背景,阐述了两者结合的必要性和重要性。
接着,文章从网络结构、博弈规则、动态演化等多个方面对复杂网络演化博弈理论进行了深入的分析和讨论。
信息工程专业术语(7)

chain 电路 chain code 链式码 chain command flag 命令链特栈 chain data 链式数据 chain data flag 数据链特栈 chain database 链式数据库 chain job 链式椎 chain printer 链式打印机 chained command 连接命令 chained file 链接⽂件 chained list 链表 chained program 连接程序 chained scheduling 链式等 chained search 连接检索 chaining 链接 chaining file 连接⽂件 chaining of commands 命令的链接 chaining of data 数据链接 chaining search 链接检索 chance machine 概率计算机 change 变更 change bit 更换位 change dump 改后转储 change file 变更⽂件 change mode key 改变⽅式键 change over contact 转换接点 change record 变更记录 change tape 变更带 changeable storage 可换存储器 changed data dump 变更数据转储 channel 通道 channel adapter 通道适配器通道转接器 channel address 通道地址 channel address word 通道地址字 channel bank 信道组合器 channel capacity 通道传输能⼒ channel check handler 通道检验处理程序 channel command 通道命令 channel command code 通道命令代码 channel command register 通道命令寄存器 channel command word 通道命令字 channel control check 通道控制检查 channel control unit 通道控制器 channel controller 通道控制器 channel coupled multiprocessor 通道耦合多处理机 channel data check 通道数据检验 channel director 通道管理机 channel distributor 通道分配器 channel encoder 信道编码器 channel end 通道传输结束 channel interface 通道接⼝ channel interrupt 通道中断 channel mask 通道屏蔽 channel multiplexer 通道多路转换器 channel number 信道号 channel processor 通道处理机 channel program 通道程序 channel program block 通道程序块 channel program translation 通道程序转换 channel retry 通道重试 channel scheduler 通道等程序 channel status word 通道状态字 channel switch 通道开关 channel switching 通道转换 channel to channel adapter 通道通道适配器通道通道选择器 channel type 通道类型 channel waiting queue 通道等待队列 channeling 沟道酌 channelizing 通道化 character 符号 character assembly 字符装配 character at a time printer 单字符打印机 character attribute 字符属性 character blanking 字符消隐 character boundary 字符界 character code 字符码 character code translation 字符代码转换 character constant 字符常数 character counter 字符计数器 character crowding 字符拥挤 character deletion 字符删除 character deletion character 字符删除字符 character density 字符密度 character design 字符设计 character disassembly 字符拆卸 character display 字数显⽰器 character edge 字符边缘 character emitter 字符发⽣器 character erase 擦除字符 character expression 字符表达式 character field 字符段 character fill 字符填充 character font 字体 character generator 字符发⽣器 character graphics 字符图形 character identification 字符识别 character image 字符映像 character literal 字符⽂字 character manipulation 字符外理 character mode 字符⽅式 character oriented communication 字符式通信 character oriented machine 字符式计算机 character oriented representation ⾯向字符的表⽰ character outline 字符外形 character pattern 字形 character printer 字符打印机 character rate 字符传输率 character reader 字符输⼊机 character recognition 字符识别 character recognition device 字符识别设备 character recognition system 字符识别系统 character relation 字符关系 character representation 字符表⽰ character row 字符⾏ character screen 字符屏⾯ character selection 字符选择 character sensing 字符读出 character set 字符集 character signal 字符信号 character skew 字符歪斜 character spacing 字符间距 character string 字符串 character string constant 字符串常数 character string data 字符串数据 character stroke 字符笔划 character subset 字符⼦集 character terminal 字符终端 character type 字符类型 character type file 字符⽂件 character value 字符值 characteristic 阶 characteristic admittance 特性导纳 characteristic distortion 特性失真 characteristic frequency 特盏率 characteristic impedance 特砧抗 characteristic of action 动棕性 characteristic overflow 阶码溢出 characteristic polynomial 特锗项式 characters per inch 字符/英⼨ characters per second 每秒字符数 charactron 字码管 charge 充电 charge carrier 电荷载劣 charge coupled cell 电荷耦合元件 charge coupled device 电荷耦合掐 charge transfer device 电荷传送掐 chargeable time indicator 时间计数器计时器 chart 图表 chassis 底板 check 检查 check bit 校验位 check bus 检验总线 check byte 检验字节 check character 检验字符 check code 检验码 check digit 校验数字 check digit check 检验位检验 check indicator 校验指⽰器 check lamp 监视灯 check number 检验数 check point 检验点 check position 检验位置 check problem 校验问题 check read 校验读 check register 校验寄存器检验寄存器 check reset 校验复位 check row 检验⾏ check solution 检验解 check symbol 检验符号 check total 检查和 check word 校验字 checked operation 检验操作 checker 测试程序 checking code time 代码检验时间 checking feature 检验特性 checking routine 检查程序 checking the calibration 刻度校准 checkout 检验 checkout compiler 检验编译程序 checkout routine 校检程序 checkpoint 检验点 checkpoint data set 检验点数据集 checkpoint entry 检验点⼊⼝ checkpoint label 检验点标号 checkpoint record 检验点记录 checkpoint restart 检查点再启动 checkpoint routine 检查点程序 checkpoint space 检查点空间 checkpointing 检验指⽰ checksum 检查和 chi square criterion 判定 chi square distribution 分布 chief programmer 痔序员 chief programmer team 痔序员组 child ⼦⼥ child node ⼦节点 child process ⼦⼥进程 chinese binary 中国式⼆进制数 chinese binary code 坚式⼆进制代码 chip 芯⽚ chip card 芯⽚卡 chip carrier 芯⽚外壳 chip carrier socket 芯⽚插座 chip diode 芯⽚⼆极管 chip enable 芯⽚启动 chip manufacturer 元件制造者 chip microprocessor 单⽚微处理器 chip select 芯⽚选择 chip set 芯⽚集 choice 迭择 choice structure 选择结构 chord keyboard 弦键盘 cim 计算机⼀体化制造 cims 计算机⼀体化制造系统 cipher 密码 cipher control technology 暗号控制技术 ciphony 密码电话学 circuit 电路 circuit analysis 电路分析 circuit analyzer 电路分析机 circuit breaker 断路器电路保护器 circuit description 电路说明 circuit grade 电路等级 circuit load 电路负载 circuit logic 电路逻辑 circuit noise level 电路噪声电平 circuit simulation 电路模拟 circuit switching 线路交换 circuit switching system 线路交换系统 circuit time 电路⼯妆间 circuitry 电路 circular buffer 环形缓冲器 circular list 循环表 circular reference 循环引⽤ circulating memory 循环存储器 circulating register 循环寄存器 circulating storage 循环存储器 circumvention 绕过 cisc 复杂指令集计算机 citation index 引证她 cket interleaving 包交替 cl 互补恒吝辑 clamp 钳位 clamp on 得等待 clamping circuit 钳位电路 clamping diode 钳位⼆极管 clamping roller 压轮 clanying roller 压轮 class condition 类别条件 class interruption 分级中断 class of accuracy 准确度等级 class test 分类测试 classified data 分类数据 classifier 分类机 clause ⼦句 clean up editing 最终编辑 clear 清除 clear area 空⽩区 clear band 清除区 clear data 未加密数据 clear disk 清除盘 clear instruction 清除指令 clear key 清除键 clear screen 清除屏⾯ clear statement 清除语句 clear text 媚 clear text dialog 秒通信对话 clearing 清除 clearing device 清除装置 clic 按 click 按 client 顾客 client of window 窗⽤户 clipboard 剪贴板 clipped corner 切⾓ clipping 裁剪 clock 计时器定时器 clock channel 时标信道 clock driver 时钟脉冲驱动器 clock edge 时钟脉冲边沿 clock frequency 时钟脉冲频率 clock generation 时钟脉冲振荡 clock generator 时钟脉冲发⽣器 clock input 时钟输⼊ clock interrupt 时钟中断 clock pulse 时钟脉冲 clock pulse generator 时钟脉冲发⽣器同步脉冲发⽣器 clock rate 时钟步率 clock signal 时钟信号 clock track 时钟脉冲道 clock unit 时钟部件 clocked flip flop 时标触发器定时触发器 clocking 同步 clone 兼容产品 close coupling 紧密耦合 close down 停机 closed circuit 闭合电路 closed circuit television 闭路电视 closed loop 闭环 closed loop circuit 闭环电路 closed loop control 闭环控制 closed loop control system 闭环控制系统 closed loop system 闭环系统 closed routine 闭型例⾏程序 closed shop 不开放式计算站 closed subroutine 闭型⼦程序 closed system 封闭系统 closed user group 封闭⽤户组 closely coupled interface 密耦合接⼝ closing 关闭 closing of a file 封闭⽂件 closure 闭包 cluster 群集 cluster analysis 群集分析 cluster control 群控 cluster controller 群控 cluster sampling 分组取样 clustered access 群集存取 clustering 群集 clusters topology 群集拓扑学 cml 电镣逻辑电路 cmos 互补⾦属氧化物半导体 cmos structure 互补⾦氧半导体结构 cmos technology cmos 技术 coaxial antenna 同轴天线 coaxial cable 同轴电缆 coaxial transmission line 同轴传输线 cobol ⾯向商业的语⾔ cobol character cobol字符 cobol library processor cobol 程序库处理程序 cobol word cobol 字 codasyl 数据系统语⾔协会 code 码 code and go 快速编译和运⾏ code audit 代码审计 code block 代码块 code book 电码本 code character 编码字符 code check 代码检验 code checking time 代码检验时间 code combination 代码组合 code compare 代码⽐较 code conversion 代码转换 code converter 代码转换器 code data 编码数据 code dependent system 代码相关系统 code dictionary 电码本 code distance 码距离 code division multiple access 分码多址访问 code element 代码单值 code extension character 代码扩充字符 code for code compatibility 代码兼容性 code generation 代码⽣成 code generator 代码⽣成程序 code hole 代码孔 code holes 代码孔 code independent system 代码⽆关系统 code insensitive system 代码⽆关系统 code inspection 代码检查 code length 码长 code line 代码⾏ code modulation 编码灯 code position 代码位置 code reader 代码阅读器 code register 代码寄存器 code removal 代码除去 code scanner 代码阅读器 code set 代码集 code sheet 程序纸 code signal 编码信号 code track 代码道 code transformation 代码变换 code translator 代码转换器译码器 code transparent transmission 代码透⽑输 code type 代码类型 code walkthrough 代码⾛查 code word 代码字 codec 编码译码器 coded data 编码数据 coded decimal 编码的⼗进制 coded decimal digit 编码的⼗进制数字 coded decimal notation 编码的⼗进制记数法 coded image 编码图象 coded program 编码程序 coder 编码器 coder decoder 编码译码器 coding 编码 coding convention 编码约定 coding form 编码形式 coding line 指令字 coding matrix 编码矩阵 coding scheme 编码⽅案 coding sheet 程序纸 coding system 编码系统 coding theory 编码理沦 coefficient 系数 coercion 强制转换 coherent radar 相⼲雷达 coherent signal 相⼲信号 cohesion 内聚性 coincidence 重合 coincidence detector 重合检测器 coincidence element 重合元件 coincidence error 重合误差 coincidence gate 与门 coincidence type adder 重合型加法器 coincident current selection 电霖合选取法 cold cathod gaseous laser 冷阴极⽓体激光器 cold restart 冷重启动 cold standby 冷备⽤ cold type system 冷排系统 collating sequence 整理顺序 collation 校对 collection 采集 collector 集电极 collector characteristic 集电极特性 collector current 集极电流 collision 冲突 collision detection 冲突检出 colon 冒号 colon equal symbol 赋值符号 color bar code 彩⾊条形码 color code 颜⾊代码 color display 彩⾊显⽰器 color gamut 颜⾊范围 color graphic mode 彩⾊图形模式 color graphics 彩⾊图形 color image 彩⾊图象 color mode 彩⾊模式 color monitor 彩⾊监视器 color plane 彩⾊⾯ color television 彩⾊电视 color tv 彩⾊电视 column 列 column binary card 竖式⼆进制卡⽚ column binary code 坚式⼆进制代码 column binary representation 坚式⼆进制代码 column diagram 直⽅图 column indicator 列指⽰器 column split 列分割 column splitting 列分割 combination 组合 combination automatic controller 组合⾃动控制器 combination circuit 组合电路 combination scale 组合刻度 combinational logic element 组合逻辑元件 combinational logic gate 组合逻辑门 combinatorial circuit 组合电路 combinatorial logic 组合逻辑 combined code 组合代码 combined error 总合误差 combined head 读写兼⽤头 combined station 复合站 comma 逗号 command 命令 command character 命令字符 command code 命令码 command control block 命令控制块 command control program 命令控制程序 command decoder 指令译码器 command driven interface 命令驱动接⼝ command environment 命令环境 command file 命令⽂件 command interpreter 命令解释程序 command interrupt 命令中断 command language 命令语⾔ command level 命令⽔平 command library 命令库 command line 命令⾏ command line parameter 命令⾏参数 command mode 命令⽅式 command name 命令名字 command procedure 命令过程 command processing 命令处理 command processor 命令处理程序 command pulse 指令脉冲 command qualifier 命令限定词 command register 命令寄存器 command scan 命令扫描 command scan program 命令扫描程序 command statement 命令语句 command system 命令系统 command word 命令字 comment field 注解栏 comment statement 注解语句。
Social games in a social network
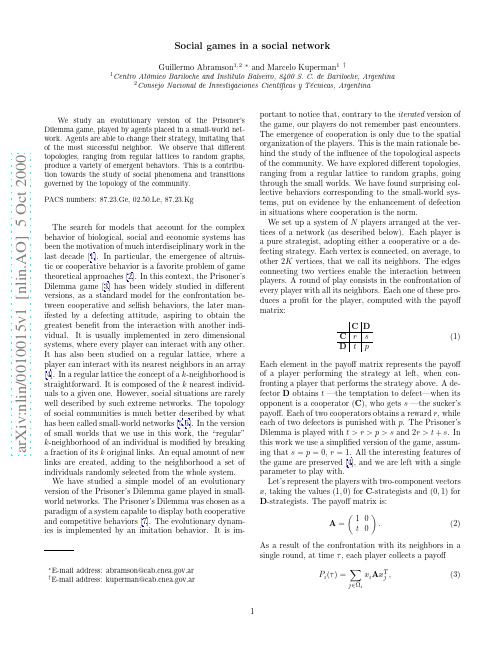
a r X i v :n l i n /0010015v 1 [n l i n .A O ] 5 O c t 2000Social games in a social networkGuillermo Abramson 1,2∗and Marcelo Kuperman 1†1Centro At´o mico Bariloche and Instituto Balseiro,8400S.C.de Bariloche,Argentina2Consejo Nacional de Investigaciones Cient´ıficas y T´e cnicas,ArgentinaWe study an evolutionary version of the Prisoner’sDilemma game,played by agents placed in a small-world net-work.Agents are able to change their strategy,imitating that of the most successful neighbor.We observe that different topologies,ranging from regular lattices to random graphs,produce a variety of emergent behaviors.This is a contribu-tion towards the study of social phenomena and transitions governed by the topology of the community.PACS numbers:87.23.Ge,02.50.Le,87.23.KgThe search for models that account for the complex behavior of biological,social and economic systems has been the motivation of much interdisciplinary work in the last decade [1].In particular,the emergence of altruis-tic or cooperative behavior is a favorite problem of game theoretical approaches [2].In this context,the Prisoner’s Dilemma game [3]has been widely studied in different versions,as a standard model for the confrontation be-tween cooperative and selfish behaviors,the later man-ifested by a defecting attitude,aspiring to obtain the greatest benefit from the interaction with another indi-vidual.It is usually implemented in zero dimensional systems,where every player can interact with any other.It has also been studied on a regular lattice,where a player can interact with its nearest neighbors in an array [4].In a regular lattice the concept of a k -neighborhood is straightforward.It is composed of the k nearest individ-uals to a given one.However,social situations are rarely well described by such extreme networks.The topology of social communities is much better described by what has been called small-world networks [5,6].In the version of small worlds that we use in this work,the “regular”k -neighborhood of an individual is modified by breaking a fraction of its k original links.An equal amount of new links are created,adding to the neighborhood a set of individuals randomly selected from the whole system.We have studied a simple model of an evolutionary version of the Prisoner’s Dilemma game played in small-world networks.The Prisoner’s Dilemma was chosen as a paradigm of a system capable to display both cooperative and competitive behaviors [7].The evolutionary dynam-ics is implemented by an imitation behavior.It is im-C Cstwhere Ωi is the set of neighbors of element i .P i is the profit earned by a player in a time step,and it is not accumulated from round to round.After this,the players are allowed to inspect the profit collected by its neighbors in that round,adopting the strategy of the wealthiest among them for the next round of play.If there is a draw between more than one neigh-bor,one of them is chosen at random to be imitated.If the element under consideration is itself one of the win-ners of the round,it keeps its own strategy.That is,explicitly writing the time dependence of the strategies:x i (τ+1)=x i (τ)if P i (τ)≥max(P ∈Ωi )x j (τ)if j ∈Ωi and P j (τ)=max(P ∈Ωi ).We have found that a small amount of noise is essen-tial to prevent the system from falling in a frozen state.After a round of play,we chose one element at random and flip its strategy.This is enough to keep the system out of equilibrium and allow transitions between different states.As a playground for our system,we have used a familyof small-world networks that depend on a parameter ǫ[6].We start from a regular,one-dimensional,periodic lattice of coordination number 2K .We then run sequen-tially through each of the sites,rewiring K of its links with probability ǫ.Running from 0to 1,this parame-ter changes the wiring properties of the network,ranging from a completely ordered lattice at ǫ=0,to a ran-dom network at ǫ=1.Intermediate values of ǫproduce a continuous spectrum of small-world networks.Double connections between sites,as well as the connection of a site with itself,are avoided in the construction of the network.Since we neither destroy nor create links,the resulting network has an average coordination number 2K ,equal to the initial one.This method,however,can produce disconnected graphs,that we have avoided in our analysis.Note that ǫis related to the fraction of modified regular links.Two magnitudes characterize the topological proper-ties of the small-world networks generated by the indi-cated procedure.One of them,L (ǫ),measures the typical separation between any pair of elements in the network.The other,C (ǫ),measures the clustering of an element’s neighborhood [6].Ordered lattices are highly clustered,and have large L .Random graphs have short character-istic length and small clusterization.In between,small worlds can be characterized by a high clusterization (like lattices)and short path lengths (like random networks).The opposing tendencies of cooperation and defection perform differently for different payofftables and differ-ent topologies,through the values of t and ǫ.Disregard-ing ǫ,one may qualitatively expect that,for sufficiently high values of t it would pay to defect while,for low val-ues of t ,it would be worth to cooperate.In either of these two extremes,the system would collapse to a stateformed only by defectors (in the first case)or only by co-operators (in the second case).For intermediate values of t the system would settle into a mixed state consisting in cooperators and defectors.Cooperators would thrive through the formation of clusters,that can resist the in-vasion by defectors.The dependency on the topology of the network appears on top of these three regimes.From the structure of the payoffmatrix one may conjecture that the high values of t referred to above will be around t =2(where a defector earns twice as much as a pair of cooperators).Correspondingly,the low values of t will be around t =1(where a single cooperator earns more than a defector).In the following we show the results of simulations per-formed in systems with 1000elements.The initial strate-gies are assigned at random with equal probability.Then several hundred rounds are played to allow for an asymp-totic regime to be achieved.All the results shown are averages over realizations where both the networks and the initial conditions are randomly chosen,excluding all disconnected graphs from our analysis.d e f e c t o r sthe tempta-probability to 10in-500rounds of t ex-a proper The number of cooperators and defectors are fluctu-ating variables,with bell shaped distributions.In figure 1we show the average fraction of defectors in systems with K =2,that is,systems with an average coordina-tion number of four.Four curves are shown as a function of the parameter t .Each curve corresponds to a network characterized by the parameter ǫshown in the legend.All the curves show a growth in the fraction of defectors for growing values of t ,as expected.We can see however,that the small world corresponding to ǫ=0.1displays an enhanced number of defectors at values of t around 1.2.For systems with a fixed K and a fixed t ,this meansthat the existence of a small world topology with ǫ∼0.1represents that nearly 40%of the population adopts the defecting strategy,against the 20%ofmoreregular or more random networks.(Note that,in fig.1,we have included values of t lower than 1,where the game is not a proper Prisoner’s Dilemma,since the reward for coop-eration is greater that the temptation to defect.We have done so because the state of the system at t =1,for all values of ǫ,still contains a small fraction of defectors.We wanted to stress that for low enough values of t the state is complete cooperation.)1the rewiring in the leg-realizations of 1000but with emphasize the changes in behavior as the structure of the network varies.The four curves correspond to different coordina-tion numbers.The game corresponds to the value t =1.2in the payoffmatrix,so that the curve with K =2is a slice of figure 1cut at t =1.2.Note that only this curve has a clear high peak of defectors centered near ǫ=0.1.Systems with K =3have a downward peak instead,in the region of small worlds,indicating a slight enhance-ment of the cooperative strategy.For K =4we can see again a small peak of defectors.Systems with K =5and greater (not shown)display a monotonous behavior in ǫ.Some conjecture on the origin of these features may be appropriate here.We think that the competition between the stability of clusters of cooperators and their exploita-tion by neighbor defectors at the borders contributes to the features observed here.When K =2,the coopera-tors survive in small compact groups.As ǫgrows,these groups can be formed by elements widely dispersed in the system,where they will have more defector neighbors to compete with.In this way,there will be less configura-tions to support them and,consequently,more defectorsin the system.For ǫeven greater,and more long range links,cooperators may start to reconnect and survive the competition with the defectors.When K >2,the coop-erators can only survive in larger groups,because de-fecting neighbors at the border of a group can penetrate deeper.When ǫgrows,cooperators belonging to faraway groups may become connected to form large clusters able to survive.The fact that K =3,at t =1.2shows a slight decrease in the fraction of defectors at intermediate val-ues of ǫremains,however,unexplained in this picture.At other values of t ,we observed that the system with K =3performs like that with K =2,namely with an enhancement of defector at intermediate values of ǫ.ac-in-that change their strategy.There is a Gaussian distribution of these unsatisfied elements,whose mean increases with ǫ,as shown in figure 3.This behavior is observed for all values of K and of t ,namely that regular lattices contain a smaller number of unsatisfied elements than random networks,with small worlds in between.Most of what is analytically known about small worlds refers to the distribution of shortest paths between pairs of elements (see for example [8–10]).It is known that reg-ular lattices stand apart from even infinitesimally rewired small worlds,that behave like random networks.The ex-istence of a phenomenon like the enhancement of defec-tors density at a finite value of ǫ,as shown in this work,points to the existence of an interesting phenomenology in small worlds.The broad spectrum of behaviors of a given system as a function of the topological features of the network is the main aspect that we want to empha-size.This suggests the possibility of modelling a certain system featuring well known interactions and analyzing the influence of the particular organization the commu-nity.Moreover,the possibility of a self organizing net-work with changing links opens the possibility of mod-elling more realistically social and economical situations [11].At this point we can state that the self organization of the network can lead to a nontrivial behavior of the whole system.Another interesting example of this state-ment would be a simple SIR model for the propagation of an epidemic.This is the subject of work under way [12].The authors thank Dami´a n H.Zanette for interesting discussions.。
跨学科视角下的高校集群化科研建筑空间组织设计研究——以重庆大学理科楼,斯坦福大学克拉克中心和麻省理工
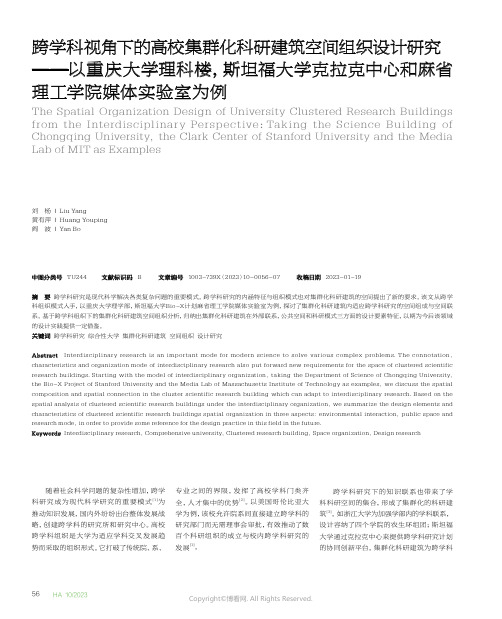
中图分类号 TU244 文献标识码 B 文章编号 1003-739X (2023)10-0056-07 收稿日期 2023-01-19摘 要 跨学科研究是现代科学解决各类复杂问题的重要模式。
跨学科研究的内涵特征与组织模式也对集群化科研建筑的空间提出了新的要求。
该文从跨学科组织模式入手,以重庆大学理学部,斯坦福大学Bio-X计划麻省理工学院媒体实验室为例,探讨了集群化科研建筑内适应跨学科研究的空间组成与空间联系。
基于跨学科组织下的集群化科研建筑空间组织分析,归纳出集群化科研建筑在外部联系,公共空间和科研模式三方面的设计要素特征,以期为今后该领域的设计实践提供一定借鉴。
关键词 跨学科研究 综合性大学 集群化科研建筑 空间组织 设计研究Abstract Interdisciplinary research is an important mode for modern science to solve various complex problems. The connotation, characteristics and organization mode of interdisciplinary research also put forward new requirements for the space of clustered scientific research buildings. Starting with the model of interdisciplinary organization, taking the Department of Science of Chongqing University, the Bio-X Project of Stanford University and the Media Lab of Massachusetts Institute of Technology as examples, we discuss the spatial composition and spatial connection in the cluster scientific research building which can adapt to interdisciplinary research. Based on the spatial analysis of clustered scientific research buildings under the interdisciplinary organization, we summarize the design elements and characteristics of clustered scientific research buildings spatial organization in three aspects: environmental interaction, public space and research mode, in order to provide some reference for the design practice in this field in the future.Keywords Interdisciplinary research, Comprehensive university, Clustered research building, Space organization, Design research刘 杨 | Liu Yang黄有萍 | Huang Youping 阎 波 | Yan Bo跨学科视角下的高校集群化科研建筑空间组织设计研究——以重庆大学理科楼,斯坦福大学克拉克中心和麻省理工学院媒体实验室为例The Spatial Organization Design of University Clustered Research Buildings from the Interdisciplinary Perspective:Taking the Science Building of Chongqing University , the Clark Center of Stanford University and the Media Lab of MIT as Examples随着社会科学问题的复杂性增加,跨学科研究成为现代科学研究的重要模式[1]为推动知识发展,国内外纷纷出台整体发展战略,创建跨学科的研究所和研究中心。
知识图谱拓扑推理在软件定义网络故障检测中的应用
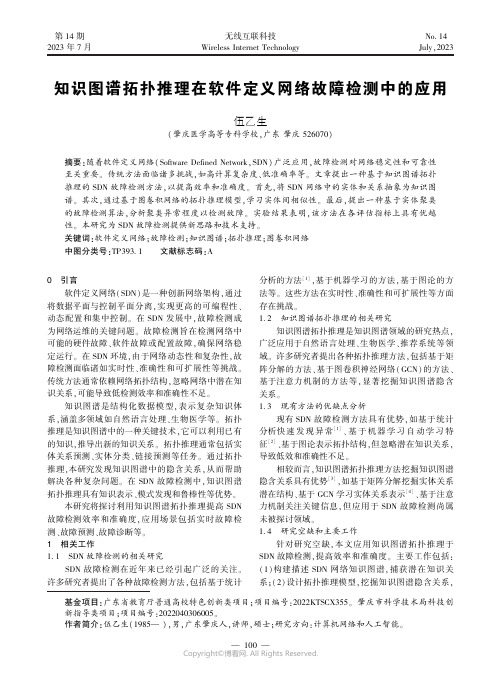
第14期2023年7月无线互联科技Wireless Internet TechnologyNo.14July,2023基金项目:广东省教育厅普通高校特色创新类项目;项目编号:2022KTSCX355㊂肇庆市科学技术局科技创新指导类项目;项目编号:2022040306005㊂作者简介:伍乙生(1985 ),男,广东肇庆人,讲师,硕士;研究方向:计算机网络和人工智能㊂知识图谱拓扑推理在软件定义网络故障检测中的应用伍乙生(肇庆医学高等专科学校,广东肇庆526070)摘要:随着软件定义网络(Software Defined Network ,SDN )广泛应用,故障检测对网络稳定性和可靠性至关重要㊂传统方法面临诸多挑战,如高计算复杂度㊁低准确率等㊂文章提出一种基于知识图谱拓扑推理的SDN 故障检测方法,以提高效率和准确度㊂首先,将SDN 网络中的实体和关系抽象为知识图谱㊂其次,通过基于图卷积网络的拓扑推理模型,学习实体间相似性㊂最后,提出一种基于实体聚类的故障检测算法,分析聚类异常程度以检测故障㊂实验结果表明,该方法在各评估指标上具有优越性㊂本研究为SDN 故障检测提供新思路和技术支持㊂关键词:软件定义网络;故障检测;知识图谱;拓扑推理;图卷积网络中图分类号:TP393.1㊀㊀文献标志码:A 0㊀引言㊀㊀软件定义网络(SDN)是一种创新网络架构,通过将数据平面与控制平面分离,实现更高的可编程性㊁动态配置和集中控制㊂在SDN 发展中,故障检测成为网络运维的关键问题㊂故障检测旨在检测网络中可能的硬件故障㊁软件故障或配置故障,确保网络稳定运行㊂在SDN 环境,由于网络动态性和复杂性,故障检测面临诸如实时性㊁准确性和可扩展性等挑战㊂传统方法通常依赖网络拓扑结构,忽略网络中潜在知识关系,可能导致低检测效率和准确性不足㊂知识图谱是结构化数据模型,表示复杂知识体系,涵盖多领域如自然语言处理㊁生物医学等㊂拓扑推理是知识图谱中的一种关键技术,它可以利用已有的知识,推导出新的知识关系㊂拓扑推理通常包括实体关系预测㊁实体分类㊁链接预测等任务㊂通过拓扑推理,本研究发现知识图谱中的隐含关系,从而帮助解决各种复杂问题㊂在SDN 故障检测中,知识图谱拓扑推理具有知识表示㊁模式发现和鲁棒性等优势㊂本研究将探讨利用知识图谱拓扑推理提高SDN 故障检测效率和准确度,应用场景包括实时故障检测㊁故障预测㊁故障诊断等㊂1㊀相关工作1.1㊀SDN 故障检测的相关研究㊀㊀SDN 故障检测在近年来已经引起广泛的关注㊂许多研究者提出了各种故障检测方法,包括基于统计分析的方法[1],基于机器学习的方法,基于图论的方法等㊂这些方法在实时性㊁准确性和可扩展性等方面存在挑战㊂1.2㊀知识图谱拓扑推理的相关研究㊀㊀知识图谱拓扑推理是知识图谱领域的研究热点,广泛应用于自然语言处理㊁生物医学㊁推荐系统等领域㊂许多研究者提出各种拓扑推理方法,包括基于矩阵分解的方法㊁基于图卷积神经网络(GCN)的方法㊁基于注意力机制的方法等,显著挖掘知识图谱隐含关系㊂1.3㊀现有方法的优缺点分析㊀㊀现有SDN 故障检测方法具有优势,如基于统计分析快速发现异常[1]㊁基于机器学习自动学习特征[2]㊁基于图论表示拓扑结构,但忽略潜在知识关系,导致低效和准确性不足㊂相较而言,知识图谱拓扑推理方法挖掘知识图谱隐含关系具有优势[3],如基于矩阵分解挖掘实体关系潜在结构㊁基于GCN 学习实体关系表示[4]㊁基于注意力机制关注关键信息,但应用于SDN 故障检测尚属未被探讨领域㊂1.4㊀研究空缺和主要工作㊀㊀针对研究空缺,本文应用知识图谱拓扑推理于SDN 故障检测,提高效率和准确度㊂主要工作包括:(1)构建描述SDN 网络知识图谱,捕获潜在知识关系;(2)设计拓扑推理模型,挖掘知识图谱隐含关系,应用于故障检测;(3)提出基于知识图谱拓扑推理的SDN故障检测算法,提高检测效率和准确度;(4)实验评估验证所提方法的有效性和优越性,为实际应用提供支持㊂2㊀方法2.1㊀基于知识图谱的SDN故障检测方法概述㊀㊀本文提出的基于知识图谱拓扑推理的SDN故障检测方法,包含知识图谱构建㊁拓扑推理模型㊁故障检测算法3个部分㊂首先,构建描述SDN网络的知识图谱;其次,设计拓扑推理模型挖掘隐含关系;最后,提出基于知识图谱拓扑推理的故障检测算法,提高检测效率和准确度㊂2.2㊀知识图谱构建㊀㊀为了描述SDN网络,本研究构建了一个知识图谱,其中包括以下实体和关系:(1)实体㊂交换机(Switch)㊁控制器(Controller)㊁主机(Host)㊁链路(Link)㊂(2)关系㊂连接(Connected)㊁控制(Controlled)㊂本研究从SDN网络的拓扑信息中提取实体和关系,从网络配置文件中提取交换机㊁控制器和主机的信息以及他们之间的连接和控制关系㊂2.3㊀拓扑推理模型㊀㊀本研究设计了一个拓扑推理模型,用于挖掘知识图谱中的隐含关系㊂具体来说,本研究采用了基于图卷积神经网络(GCN)的方法,自动地学习实体和关系的表示,给定一个知识图谱G=(V,E),其中V是实体集合,E是关系集合㊂首先,将实体和关系表示为低维向量;然后,利用GCN对知识图谱进行拓扑推理,以更新实体和关系的表示㊂具体地说,GCN的更新公式如下:h(l+1) i =σðjɪN(i)1c ij W(l)h(l)j()(1)其中,h l i表示第l层的实体i的表示,N(i)表示实体i的邻居集合,c ij表示实体i和实体j之间的归一化因子,W(l)表示第l层的权重矩阵,σ表示激活函数㊂2.4㊀故障检测算法㊀㊀基于知识图谱拓扑推理模型,本研究提出了一个故障检测算法㊂首先,使用拓扑推理模型学习实体和关系的表示㊂然后,计算实体之间的相似度,并根据相似度阈值将相似实体聚合到一起㊂具体来说,使用余弦相似度计算实体之间的相似度,即:sim(i,j)=h i㊃h jh i h j (2)其中,h i和h j分别表示实体i和实体j的表示向量, h i 和 h j 分别表示它们的模长,即向量的欧几里得范数,sim(i,j)表示实体i和实体j之间的相似度㊂根据相似度阈值θ将相似实体聚合到一起,形成一个实体聚类㊂对于每个实体聚类,计算其异常分数,以评估该聚类中的故障概率㊂具体来说,使用以下公式计算异常分数:S(c)=1|c|ðiɪcðjɪN(i)sim(i,j)-si-m(c)(3)其中,c表示一个实体聚类,|c|表示实体聚类中的实体数量,N(i)表示实体i的邻居集合,si-m(c)表示实体聚类c的平均相似度㊂根据异常分数阈值α判断实体聚类是否存在故障㊂如果一个实体聚类的异常分数超过阈值α,认为该聚类存在故障,并进一步定位故障实体㊂2.5㊀流程㊀㊀基于知识图谱拓扑推理的SDN故障检测方法的流程如下:(1)构建SDN网络的知识图谱㊂(2)使用拓扑推理模型学习实体和关系的表示㊂(3)计算实体之间的相似度,并根据相似度阈值将相似实体聚合到一起㊂(4)计算实体聚类的异常分数,并根据异常分数阈值判断实体聚类是否存在故障㊂(5)如果存在故障,进一步定位故障实体㊂3㊀实验和结果讨论3.1㊀实验环境和数据集㊀㊀实验在Python3.8下进行,用PyTorch㊁NetworkX 和NumPy等库㊂硬件配置:Intel Core i7-8700CPU㊁32GB RAM㊁NVIDIA GeForce GTX1080Ti GPU㊂数据集来自真实SDN网络,包含网络流量㊁设备信息㊁故障记录㊂预处理后划分为训练集㊁验证集㊁测试集,比例分别为70%㊁15%㊁15%㊂3.2㊀评估指标和对比方法㊀㊀评估指标:准确率(Accuracy)㊁召回率(Recall)㊁F1分数(F1-Score)以及ROC曲线下面积(AUC-ROC)㊂对比方法:基于传统机器学习(ML-based)㊁基于深度学习(DL-based)㊁基于图卷积网络(GCN-based)㊂3.3㊀实验结果展示和分析㊀㊀不同方法在各评估指标上的性能对比如图1所示㊂从结果可以看出,提出的基于知识图谱拓扑推理的SDN故障检测方法在所有评估指标上均优于其他对比方法㊂这表明本文的方法在检测SDN网络中的故障时具有更高的准确性和效率㊂图1㊀本文方法和传统机器学习㊁深度学习㊁图卷积网络方法性能对比综上,通过对比实验,本研究验证了基于知识图谱拓扑推理的SDN故障检测方法在准确率㊁召回率㊁F1分数㊁AUC-ROC等评估指标上的优势㊂这些实验结果表明,本研究的方法可以有效地检测和定位SDN 网络中的故障,提高网络的稳定性和可靠性㊂4㊀结语㊀㊀本论文提出了一种基于知识图谱拓扑推理的SDN故障检测方法,虽然实验证明了其在故障检测和㊀㊀定位方面的优越性,但仍存在局限:(1)在大规模SDN网络下,计算复杂度和内存消耗挑战㊂未来工作需探索高效算法和优化技术提高可扩展性㊂(2)采用静态知识图谱构建方法可能无法捕获网络动态变化㊂未来可考虑采用动态知识图谱技术实现准确建模㊂(3)现有故障检测关注局部拓扑异常,未充分利用全局信息㊂未来需探讨基于全局拓扑结构的方法,以提高准确性和鲁棒性㊂参考文献[1]纪洪泉,何潇,周东华.基于多元统计分析的故障检测方法[J].上海交通大学学报,2015(6):842-848,854.[2]刘冶,朱蔚恒,潘炎,等.基于低秩和稀疏矩阵分解的多源融合链接预测算法[J].计算机研究与发展, 2015(2):423-436.[3]彭成,张春霞,张鑫,等.基于实体多元编码的时序知识图谱推理[J].数据分析与知识发现,2023 (1):138-149.[4]赵晓娟,贾焰,李爱平,等.基于层级注意力机制的链接预测模型研究[J].通信学报,2021(3):36-44.(编辑㊀王永超)Application of knowledge graph topology reasoning in softwaredefined network fault detectionWu YishengZhaoqing Medical College Zhaoqing526070 ChinaAbstract As software-defined networking SDN gains popularity fault detection is vital for network stability and reliability.Traditional methods face challenges like high complexity and low accuracy.This paper introduces an SDN fault detection method based on knowledge graph topology reasoning enhancing efficiency and accuracy.We abstract SDN network entities and relationships into knowledge graphs learn inter-entity similarity and propose a fault detection algorithm based on entity clustering for analyzing abnormal clustering degrees.Experiment results show superiority in evaluation indices providing new ideas and technical support for SDN fault detection.Key words software-defined networking fault detection knowledge graph topology inference graph convolutional networks。
Statistical mechanics of complex networks,RevModPhys.74.47

Statistical mechanics of complex networksRe´ka Albert*and Albert-La´szlo´Baraba´siDepartment of Physics,University of Notre Dame,Notre Dame,Indiana46556(Published30January2002)Complex networks describe a wide range of systems in nature and society.Frequently cited examples include the cell,a network of chemicals linked by chemical reactions,and the Internet,a network of routers and computers connected by physical links.While traditionally these systems have been modeled as random graphs,it is increasingly recognized that the topology and evolution of real networks are governed by robust organizing principles.This article reviews the recent advances in the field of complex networks,focusing on the statistical mechanics of network topology and dynamics.After reviewing the empirical data that motivated the recent interest in networks,the authors discuss the main models and analytical tools,covering random graphs,small-world and scale-free networks, the emerging theory of evolving networks,and the interplay between topology and the network’s robustness against failures and attacks.CONTENTSI.Introduction48II.The Topology of Real Networks:Empirical Results49A.World Wide Web49B.Internet50C.Movie actor collaboration network52D.Science collaboration graph52E.The web of human sexual contacts52F.Cellular networks52G.Ecological networks53H.Phone call network53I.Citation networks53works in linguistics53 K.Power and neural networks54 L.Protein folding54 III.Random-Graph Theory54A.The Erdo˝s-Re´nyi model54B.Subgraphs55C.Graph evolution56D.Degree distribution57E.Connectedness and diameter58F.Clustering coefficient58G.Graph spectra59 IV.Percolation Theory59A.Quantities of interest in percolation theory60B.General results601.The subcritical phase(pϽp c)602.The supercritical phase(pϾp c)61C.Exact solutions:Percolation on a Cayley tree61D.Scaling in the critical region62E.Cluster structure62F.Infinite-dimensional percolation62G.Parallels between random-graph theory andpercolation63 V.Generalized Random Graphs63A.Thresholds in a scale-free random graph64B.Generating function formalism64ponent sizes and phase transitions652.Average path length65C.Random graphs with power-law degreedistribution66D.Bipartite graphs and the clustering coefficient66 VI.Small-World Networks67A.The Watts-Strogatz model67B.Properties of small-world networks681.Average path length682.Clustering coefficient693.Degree distribution704.Spectral properties70 VII.Scale-Free Networks71A.The Baraba´si-Albert model71B.Theoretical approaches71C.Limiting cases of the Baraba´si-Albert model73D.Properties of the Baraba´si-Albert model741.Average path length742.Node degree correlations753.Clustering coefficient754.Spectral properties75 VIII.The Theory of Evolving Networks76A.Preferential attachment⌸(k)761.Measuring⌸(k)for real networks762.Nonlinear preferential attachment773.Initial attractiveness77B.Growth781.Empirical results782.Analytical results78C.Local events791.Internal edges and rewiring792.Internal edges and edge removal79D.Growth constraints801.Aging and cost802.Gradual aging81petition in evolving networks811.Fitness model812.Edge inheritance82F.Alternative mechanisms for preferentialattachment821.Copying mechanism822.Edge redirection823.Walking on a network834.Attaching to edges83G.Connection to other problems in statisticalmechanics831.The Simon model832.Bose-Einstein condensation85*Present address:School of Mathematics,University of Min-nesota,Minneapolis,Minnesota55455.REVIEWS OF MODERN PHYSICS,VOLUME74,JANUARY20020034-6861/2002/74(1)/47(51)/$35.00©2002The American Physical Society47IX.Error and Attack Tolerance86A.Numerical results861.Random network,random node removal872.Scale-free network,random node removal873.Preferential node removal87B.Error tolerance:analytical results88C.Attack tolerance:Analytical results89D.The robustness of real networks90munication networks902.Cellular networks913.Ecological networks91X.Outlook91A.Dynamical processes on networks91B.Directed networks92C.Weighted networks,optimization,allometricscaling92D.Internet and World Wide Web93E.General questions93F.Conclusions94 Acknowledgments94 References94 I.INTRODUCTIONComplex weblike structures describe a wide variety of systems of high technological and intellectual impor-tance.For example,the cell is best described as a com-plex network of chemicals connected by chemical reac-tions;the Internet is a complex network of routers and computers linked by various physical or wireless links; fads and ideas spread on the social network,whose nodes are human beings and whose edges represent various social relationships;the World Wide Web is an enormous virtual network of Web pages connected by hyperlinks.These systems represent just a few of the many examples that have recently prompted the scien-tific community to investigate the mechanisms that de-termine the topology of complex networks.The desire to understand such interwoven systems has encountered significant challenges as well.Physics,a major benefi-ciary of reductionism,has developed an arsenal of suc-cessful tools for predicting the behavior of a system as a whole from the properties of its constituents.We now understand how magnetism emerges from the collective behavior of millions of spins,or how quantum particles lead to such spectacular phenomena as Bose-Einstein condensation or superfluidity.The success of these mod-eling efforts is based on the simplicity of the interactions between the elements:there is no ambiguity as to what interacts with what,and the interaction strength is uniquely determined by the physical distance.We are at a loss,however,to describe systems for which physical distance is irrelevant or for which there is ambiguity as to whether two components interact.While for many complex systems with nontrivial network topology such ambiguity is naturally present,in the past few years we have increasingly recognized that the tools of statistical mechanics offer an ideal framework for describing these interwoven systems as well.These developments have introduced new and challenging problems for statistical physics and unexpected links to major topics in condensed-matter physics,ranging from percolation to Bose-Einstein condensation.Traditionally the study of complex networks has been the territory of graph theory.While graph theory ini-tially focused on regular graphs,since the1950s large-scale networks with no apparent design principles have been described as random graphs,proposed as the sim-plest and most straightforward realization of a complex network.Random graphs werefirst studied by the Hun-garian mathematicians Paul Erdo˝s and Alfre´d Re´nyi. According to the Erdo˝s-Re´nyi model,we start with N nodes and connect every pair of nodes with probability p,creating a graph with approximately pN(NϪ1)/2 edges distributed randomly.This model has guided our thinking about complex networks for decades since its introduction.But the growing interest in complex sys-tems has prompted many scientists to reconsider this modeling paradigm and ask a simple question:are the real networks behind such diverse complex systems as the cell or the Internet fundamentally random?Our in-tuition clearly indicates that complex systems must dis-play some organizing principles,which should be at some level encoded in their topology.But if the topology of these networks indeed deviates from a random graph, we need to develop tools and measurements to capture in quantitative terms the underlying organizing prin-ciples.In the past few years we have witnessed dramatic ad-vances in this direction,prompted by several parallel de-velopments.First,the computerization of data acquisi-tion in allfields led to the emergence of large databases on the topology of various real networks.Second,the increased computing power allowed us to investigate networks containing millions of nodes,exploring ques-tions that could not be addressed before.Third,the slow but noticeable breakdown of boundaries between disci-plines offered researchers access to diverse databases, allowing them to uncover the generic properties of com-plex networks.Finally,there is an increasingly voiced need to move beyond reductionist approaches and try to understand the behavior of the system as a whole.Along this route,understanding the topology of the interac-tions between the components,i.e.,networks,is un-avoidable.Motivated by these converging developments and cir-cumstances,many new concepts and measures have been proposed and investigated in depth in the past few years.However,three concepts occupy a prominent place in contemporary thinking about complex net-works.Here we define and briefly discuss them,a discus-sion to be expanded in the coming sections.Small worlds:The small-world concept in simple terms describes the fact that despite their often large size,in most networks there is a relatively short path between any two nodes.The distance between two nodes is defined as the number of edges along the short-est path connecting them.The most popular manifesta-tion of small worlds is the‘‘six degrees of separation’’concept,uncovered by the social psychologist Stanley Milgram(1967),who concluded that there was a path of48R.Albert and A.-L.Baraba´si:Statistical mechanics of complex networks Rev.Mod.Phys.,Vol.74,No.1,January2002acquaintances with a typical length of about six betweenmost pairs of people in the United States(Kochen,1989).The small-world property appears to characterizemost complex networks:the actors in Hollywood are onaverage within three co-stars from each other,or thechemicals in a cell are typically separated by three reac-tions.The small-world concept,while intriguing,is notan indication of a particular organizing principle.In-deed,as Erdo˝s and Re´nyi have demonstrated,the typi-cal distance between any two nodes in a random graphscales as the logarithm of the number of nodes.Thusrandom graphs are small worlds as well.Clustering:A common property of social networks isthat cliques form,representing circles of friends or ac-quaintances in which every member knows every othermember.This inherent tendency to cluster is quantifiedby the clustering coefficient(Watts and Strogatz,1998),a concept that has its roots in sociology,appearing underthe name‘‘fraction of transitive triples’’(Wassermannand Faust,1994).Let us focusfirst on a selected node iin the network,having k i edges which connect it to k iother nodes.If the nearest neighbors of the originalnode were part of a clique,there would be k i(k iϪ1)/2 edges between them.The ratio between the number E iof edges that actually exist between these k i nodes andthe total number k i(k iϪ1)/2gives the value of the clus-tering coefficient of node i,C iϭ2E ik i͑k iϪ1͒.(1)The clustering coefficient of the whole network is the average of all individual C i’s.An alternative definition of C that is often used in the literature is discussed in Sec.VI.B.2(Barrat and Weigt,2000;Newman,Strogatz, and Watts,2000).In a random graph,since the edges are distributed randomly,the clustering coefficient is Cϭp(Sec.III.F). However,in most,if not all,real networks the clustering coefficient is typically much larger than it is in a compa-rable random network(i.e.,having the same number of nodes and edges as the real network).Degree distribution:Not all nodes in a network have the same number of edges(same node degree).The spread in the node degrees is characterized by a distri-bution function P(k),which gives the probability that a randomly selected node has exactly k edges.Since in a random graph the edges are placed randomly,the major-ity of nodes have approximately the same degree,close to the average degree͗k͘of the network.The degree distribution of a random graph is a Poisson distribution with a peak at P(͗k͘).One of the most interesting de-velopments in our understanding of complex networks was the discovery that for most large networks the de-gree distribution significantly deviates from a Poisson distribution.In particular,for a large number of net-works,including the World Wide Web(Albert,Jeong, and Baraba´si,1999),the Internet(Faloutsos et al.,1999), or metabolic networks(Jeong et al.,2000),the degree distribution has a power-law tail,P͑k͒ϳkϪ␥.(2) Such networks are called scale free(Baraba´si and Al-bert,1999).While some networks display an exponential tail,often the functional form of P(k)still deviates sig-nificantly from the Poisson distribution expected for a random graph.These discoveries have initiated a revival of network modeling in the past few years,resulting in the introduc-tion and study of three main classes of modeling para-digms.First,random graphs,which are variants of the Erdo˝s-Re´nyi model,are still widely used in manyfields and serve as a benchmark for many modeling and em-pirical studies.Second,motivated by clustering,a class of models,collectively called small-world models,has been proposed.These models interpolate between the highly clustered regular lattices and random graphs.Fi-nally,the discovery of the power-law degree distribution has led to the construction of various scale-free models that,by focusing on the network dynamics,aim to offer a universal theory of network evolution.The purpose of this article is to review each of these modeling efforts,focusing on the statistical mechanics of complex networks.Our main goal is to present the the-oretical developments in parallel with the empirical data that initiated and support the various models and theo-retical tools.To achieve this,we start with a brief de-scription of the real networks and databases that repre-sent the testing ground for most current modeling efforts.II.THE TOPOLOGY OF REAL NETWORKS:EMPIRICAL RESULTSThe study of most complex networks has been initi-ated by a desire to understand various real systems, ranging from communication networks to ecological webs.Thus the databases available for study span sev-eral disciplines.In this section we review briefly those that have been studied by researchers aiming to uncover the general features of complex networks.Beyond a de-scription of the databases,we shall focus on three robust measures of a network’s topology:average path length, clustering coefficient,and degree distribution.Other quantities,as discussed in the following sections,will again be tested on these databases.The properties of the investigated databases,as well as the obtained expo-nents,are summarized in Tables I and II.A.World Wide WebThe World Wide Web represents the largest network for which topological information is currently available. The nodes of the network are the documents(web pages)and the edges are the hyperlinks(URL’s)that point from one document to another(see Fig.1).The size of this network was close to one billion nodes at the end of1999(Lawrence and Giles,1998,1999).The in-terest in the World Wide Web as a network boomed after it was discovered that the degree distribution of the web pages follows a power law over several orders of magnitude(Albert,Jeong,and Baraba´si,1999;Kumar49R.Albert and A.-L.Baraba´si:Statistical mechanics of complex networks Rev.Mod.Phys.,Vol.74,No.1,January2002et al.,1999).Since the edges of the World Wide Web aredirected,the network is characterized by two degree dis-tributions:the distribution of outgoing edges,P out(k), signifies the probability that a document has k outgoinghyperlinks,and the distribution of incoming edges, P in(k),is the probability that k hyperlinks point to acertain document.Several studies have established that both P out(k)and P in(k)have power-law tails: P out͑k͒ϳkϪ␥out and P in͑k͒ϳkϪ␥in.(3) Albert,Jeong,and Baraba´si(1999)have studied asubset of the World Wide Web containing325729nodes and have found␥outϭ2.45and␥inϭ2.1.Kumar et al. (1999)used a40-million-document crawl by Alexa Inc., obtaining␥outϭ2.38and␥inϭ2.1(see also Kleinberg et al.,1999).A later survey of the World Wide Web to-pology by Broder et al.(2000)used two1999Altavistacrawls containing in total200million documents,obtain-ing␥outϭ2.72and␥inϭ2.1with scaling holding close to five orders of magnitude(Fig.2).Adamic and Huber-man(2000)used a somewhat different representation of the World Wide Web,with each node representing a separate domain name and two nodes being connected if any of the pages in one domain linked to any page in the other.While this method lumped together pages that were on the same domain,representing a nontrivial ag-gregation of the nodes,the distribution of incoming edges still followed a power law with␥in domϭ1.94.Note that␥in is the same for all measurements at the document level despite the two-years’time delay be-tween thefirst and last web crawl,during which the World Wide Web had grown at leastfive times larger. However,␥out has a tendency to increase with the sample size or time(see Table II).Despite the large number of nodes,the World Wide Web displays the small-world property.This wasfirst re-ported by Albert,Jeong,and Baraba´si(1999),who found that the average path length for a sample of 325729nodes was11.2and predicted,usingfinite size scaling,that for the full World Wide Web of800million nodes that would be a path length of around19.Subse-quent measurements by Broder et al.(2000)found that the average path length between nodes in a50-million-node sample of the World Wide Web is16,in agreement with thefinite size prediction for a sample of this size. Finally,the domain-level network displays an average path length of3.1(Adamic,1999).The directed nature of the World Wide Web does not allow us to measure the clustering coefficient using Eq.(1).One way to avoid this difficulty is to make the net-work undirected,making each edge bidirectional.This was the path followed by Adamic(1999),who studied the World Wide Web at the domain level using a1997 Alexa crawl of50million web pages distributed among 259794sites.Adamic removed the nodes that had have only one edge,focusing on a network of153127sites. While these modifications are expected to increase the clustering coefficient somewhat,she found Cϭ0.1078, orders of magnitude higher than C randϭ0.00023corre-sponding to a random graph of the same size and aver-age degree.B.InternetThe Internet is a network of physical links between computers and other telecommunication devices(Fig.TABLE I.The general characteristics of several real networks.For each network we have indicated the number of nodes,the average degree͗k͘,the average path length l,and the clustering coefficient C.For a comparison we have included the average path length l rand and clustering coefficient C rand of a random graph of the same size and average degree.The numbers in the last column are keyed to the symbols in Figs.8and9.Network Size͗k͘l l rand C C rand Reference Nr. WWW,site level,undir.15312735.21 3.1 3.350.10780.00023Adamic,19991 Internet,domain level3015–6209 3.52–4.11 3.7–3.76 6.36–6.180.18–0.30.001Yook et al.,2001a,Pastor-Satorras et al.,20012 Movie actors22522661 3.65 2.990.790.00027Watts and Strogatz,19983 LANL co-authorship529099.7 5.9 4.790.43 1.8ϫ10Ϫ4Newman,2001a,2001b,2001c4 MEDLINE co-authorship152025118.1 4.6 4.910.066 1.1ϫ10Ϫ5Newman,2001a,2001b,2001c5 SPIRES co-authorship56627173 4.0 2.120.7260.003Newman,2001a,2001b,2001c6 NCSTRL co-authorship11994 3.599.77.340.4963ϫ10Ϫ4Newman,2001a,2001b,2001c7 Math.co-authorship70975 3.99.58.20.59 5.4ϫ10Ϫ5Baraba´si et al.,20018 Neurosci.co-authorship20929311.56 5.010.76 5.5ϫ10Ϫ5Baraba´si et al.,20019 E.coli,substrate graph2827.35 2.9 3.040.320.026Wagner and Fell,200010 E.coli,reaction graph31528.3 2.62 1.980.590.09Wagner and Fell,200011 Ythan estuary food web1348.7 2.43 2.260.220.06Montoya and Sole´,200012 Silwood Park food web154 4.75 3.40 3.230.150.03Montoya and Sole´,200013 Words,co-occurrence460.90270.13 2.67 3.030.4370.0001Ferrer i Cancho and Sole´,200114 Words,synonyms2231113.48 4.5 3.840.70.0006Yook et al.,2001b15 Power grid4941 2.6718.712.40.080.005Watts and Strogatz,199816C.Elegans28214 2.65 2.250.280.05Watts and Strogatz,199817 50R.Albert and A.-L.Baraba´si:Statistical mechanics of complex networksRev.Mod.Phys.,Vol.74,No.1,January20021).The topology of the Internet is studied at two differ-ent levels.At the router level,the nodes are the routers,and edges are the physical connections between them.At the interdomain (or autonomous system)level,eachwork structure of the World Wide Web and the Internet.Upper panel:the nodes of the World Wide Web are web documents,connected with directed hyperlinks (URL’s).Lower panel:on the Internet the nodes are the routers and computers,and the edges are the wires and cables that physi-cally connect them.Figure courtesy of Istva´n Albert.TABLE II.The scaling exponents characterizing the degree distribution of several scale-free networks,for which P (k )follows apower law (2).We indicate the size of the network,its average degree ͗k ͘,and the cutoff for the power-law scaling.For directed networks we list separately the indegree (␥in )and outdegree (␥out )exponents,while for the undirected networks,marked with an asterisk (*),these values are identical.The columns l real ,l rand ,and l pow compare the average path lengths of real networks with power-law degree distribution and the predictions of random-graph theory (17)and of Newman,Strogatz,and Watts (2001)[also see Eq.(63)above],as discussed in Sec.V .The numbers in the last column are keyed to the symbols in Figs.8and workSize͗k ͘␥out ␥in lreallrandlpowReference Nr.WWW 325729 4.51900 2.45 2.111.28.32 4.77Albert,Jeong,and Baraba´si 19991WWW 4ϫ1077 2.38 2.1Kumar et al.,19992WWW 2ϫ1087.54000 2.72 2.1168.857.61Broder et al.,20003WWW,site 260000 1.94Huberman and Adamic,20004Internet,domain *3015–4389 3.42–3.7630–40 2.1–2.2 2.1–2.246.3 5.2Faloutsos,19995Internet,router *3888 2.5730 2.48 2.4812.158.757.67Faloutsos,19996Internet,router *150000 2.6660 2.4 2.41112.87.47Govindan,20007Movie actors *21225028.78900 2.3 2.3 4.54 3.65 4.01Baraba´si and Albert,19998Co-authors,SPIRES *566271731100 1.2 1.24 2.12 1.95Newman,2001b9Co-authors,neuro.*20929311.54400 2.1 2.16 5.01 3.86Baraba´si et al.,200110Co-authors,math.*70975 3.9120 2.5 2.59.58.2 6.53Baraba´si et al.,200111Sexual contacts *2810 3.4 3.4Liljeros et al.,200112Metabolic,E.coli 7787.4110 2.2 2.2 3.2 3.32 2.89Jeong et al.,200013Protein,S.cerev.*1870 2.39 2.4 2.4Jeong,Mason,et al.,200114Ythan estuary *1348.735 1.05 1.052.43 2.26 1.71Montoya and Sole´,200014Silwood Park *154 4.7527 1.13 1.13 3.43.232Montoya and Sole´,200016Citation 7833398.573Redner,199817Phone call 53ϫ1063.16 2.1 2.1Aiello et al.,200018Words,co-occurrence *46090270.13 2.7 2.7Ferrer i Cancho and Sole´,200119Words,synonyms *2231113.48 2.8 2.8Yook et al.,2001b20FIG.2.Degree distribution of the World Wide Web from twodifferent measurements:ᮀ,the 325729-node sample of Albert et al.(1999);᭺,the measurements of over 200million pages by Broder et al.(2000);(a)degree distribution of the outgoing edges;(b)degree distribution of the incoming edges.The data have been binned logarithmically to reduce noise.Courtesy of Altavista and Andrew Tomkins.The authors wish to thank Luis Amaral for correcting a mistake in a previous version of this figure (see Mossa et al.,2001).51R.Albert and A.-L.Baraba´si:Statistical mechanics of complex networks Rev.Mod.Phys.,Vol.74,No.1,January 2002domain,composed of hundreds of routers and comput-ers,is represented by a single node,and an edge is drawn between two domains if there is at least one route that connects them.Faloutsos et al.(1999)have studied the Internet at both levels,concluding that in each case the degree distribution follows a power law.The inter-domain topology of the Internet,captured at three dif-ferent dates between 1997and the end of 1998,resultedin degree exponents between ␥I as ϭ2.15and ␥I asϭ2.2.The 1995survey of Internet topology at the router level,containing 3888nodes,found ␥I rϭ2.48(Faloutsos et al.,1999).Recently Govindan and Tangmunarunkit (2000)mapped the connectivity of nearly 150000router inter-faces and nearly 200000router adjacencies,confirmingthe power-law scaling with ␥I rӍ2.3[see Fig.3(a)].The Internet as a network does display clustering and small path length as well.Yook et al.(2001a)and Pastor-Satorras et al.(2001),studying the Internet at the do-main level between 1997and 1999,found that its clus-tering coefficient ranged between 0.18and 0.3,to be compared with C rand Ӎ0.001for random networks with similar parameters.The average path length of the In-ternet at the domain level ranged between 3.70and 3.77(Pastor-Satorras et al.,2001;Yook et al.2001a)and at the router level it was around 9(Yook et al.,2001a),indicating its small-world character.C.Movie actor collaboration networkA much-studied database is the movie actor collabo-ration network,based on the Internet Movie Database,which contains all movies and their casts since the 1890s.In this network the nodes are the actors,and two nodes have a common edge if the corresponding actors have acted in a movie together.This is a continuously expand-ing network,with 225226nodes in 1998(Watts and Stro-gatz,1998),which grew to 449913nodes by May 2000(Newman,Strogatz,and Watts,2000).The average path length of the actor network is close to that of a random graph with the same size and average degree,3.65com-pared with 2.9,but its clustering coefficient is more than 100times higher than a random graph (Watts and Stro-gatz,1998).The degree distribution of the movie actor network has a power-law tail for large k [see Fig.3(b)],following P (k )ϳk Ϫ␥actor ,where ␥actor ϭ2.3Ϯ0.1(Bara-ba´si and Albert,1999;Albert and Baraba ´si,2000;Ama-ral et al.,2000).D.Science collaboration graphA collaboration network similar to that of the movie actors can be constructed for scientists,where the nodes are the scientists and two nodes are connected if the two scientists have written an article together.To uncover the topology of this complex graph,Newman (2001a,2001b,2001c)studied four databases spanning physics,biomedical research,high-energy physics,and computer science over a five-year window (1995–1999).All these networks show a small average path length but a high clustering coefficient,as summarized in Table I.The de-gree distribution of the collaboration network of high-energy physicists is an almost perfect power law with an exponent of 1.2[Fig.3(c)],while the other databases display power laws with a larger exponent in the tail.Baraba´si et al.(2001)investigated the collaboration graph of mathematicians and neuroscientists publishing between 1991and 1998.The average path length of these networks is around l math ϭ9.5and l nsci ϭ6,their clustering coefficient being C math ϭ0.59and C nsci ϭ0.76.The degree distributions of these collaboration networks are consistent with power laws with degree ex-ponents 2.1and 2.5,respectively [see Fig.3(d)].E.The web of human sexual contactsMany sexually transmitted diseases,including AIDS,spread on a network of sexual relationships.Liljeros et al.(2001)have studied the web constructed from the sexual relations of 2810individuals,based on an exten-sive survey conducted in Sweden in 1996.Since the edges in this network are relatively short lived,they ana-lyzed the distribution of partners over a single year,ob-taining for both females and males a power-law degree distribution with an exponent ␥f ϭ3.5Ϯ0.2and ␥m ϭ3.3Ϯ0.2,respectively.F .Cellular networksJeong et al.(2000)studied the metabolism of 43or-ganisms representing all three domains of life,recon-structing them in networks in which the nodes aretheFIG.3.The degree distribution of several real networks:(a)Internet at the router level.Data courtesy of Ramesh Govin-dan;(b)movie actor collaboration network.After Baraba´si and Albert 1999.Note that if TV series are included as well,which aggregate a large number of actors,an exponential cut-off emerges for large k (Amaral et al.,2000);(c)co-authorship network of high-energy physicists.After Newman (2001a,2001b);(d)co-authorship network of neuroscientists.AfterBaraba´si et al.(2001).52R.Albert and A.-L.Baraba´si:Statistical mechanics of complex networks Rev.Mod.Phys.,Vol.74,No.1,January 2002。
思维进化算法在BP神经网络拟合非线性函数中的应用研究

思维进化算法在BP神经网络拟合非线性函数中的应用研究刘俊【摘要】直接使用BP神经网络拟合非线性函数,具有预测精度差、收敛速度慢等缺点。
该文提出利用极强全局搜索能力的思维进化算法来优化BP神经网络。
首先根据BP神经网络拓扑结构构建思维进化算法模型,然后用思维进化算法得到的最优解作为BP神经网络的初始权值和阈值,最后利用MATLAB软件对多个非线性函数进行拟合仿真实验,比较思维进化算法优化BP神经网络和单纯使用BP神经网络的预测结果。
数据表明,优化后的BP神经网络具有更高的拟合精度和更短的网络训练时间。
%Owing to the poor accuracy,slow convergence speed and other shortcomings after the direct appli-cation of BP neural network in the fitting of nonlinear functions,this paper proposed that BP neural network can be optimized by mind evolutionary algorithm,which enjoys strong global search ability. Firstly,the mind evolu-tionary algorithm model is constructed based on neural network topology;then,it is used to get the optimal solu-tions,which is served as initial weights and the threshold value of BP neural network;lastly,the MATLAB soft-ware is used to simulate multiple nonlinear function fitting,comparing the different results between optimized BP neural network and simply application of the BP neural network. Statistics indicate that the optimized BP neural network enjoys higher accuracy and shorter training time.【期刊名称】《绵阳师范学院学报》【年(卷),期】2015(000)002【总页数】5页(P79-83)【关键词】思维进化算法;BP神经网络;函数拟合【作者】刘俊【作者单位】商洛学院电子信息与电气工程学院,陕西商洛 726000【正文语种】中文【中图分类】TP183在工程应用领域中,经常需要对大量采集的历史数据进行函数拟合.然而这些数据常常是复杂、多元的非线性关系,传统的最小二乘、多项式回归等拟合方法无法满足拟合精度要求.人工神经网络具有良好的非线性并行处理能力,强大的学习和泛化能力,为非线性函数拟合提供了有效途径.张宝堃等[1]利用非线性映射能力较强的BP 神经网络拟合了一组单输入单输出非线性函数,但该方法存在局部最优问题等缺点[2,3].徐富强等[4]采用遗传算法优化BP 神经网络的初始权值和阈值,并用于非线性函数拟合,该方法全局搜索能力较强,但误差依然较大.沈学利等[5]将粒子群优化算法应用于BP神经网络的参数训练,对非线性函数拟合有一定的效果,但存在精度较差,容易陷入局部最优等[6]缺点.本文提出了思维进化算法与BP 神经网络结合的算法,利用思维进化算法优化BP 神经网络的初始权值和阈值,通过多个非线性函数拟合实验验证了该算法的强拟合能力和有效性.1 思维进化算法1.1 思维进化算法概述思维进化算法(Mind Evolutionary Algorithm,简称MEA)由孙承意等[7]研究者于1998年提出,该算法是针对遗传算法的缺陷[8]而提出的一种新型进化算法,其思想来源于模仿人类思维进化过程.思维进化算法继承了遗传算法的“群体”和“进化”的思想,提出了新的操作算子——“趋同”和“异化”,这两种操作相互协调,其中任一操作改进都可以提高算法的整体搜索效率.由于思维进化算法具有良好的扩充性、移植性和极强的全局优化能力,已经成功应用于图像处理、自动控制、经济预测等领域[9-13]1.2 思维进化算法基本思想思维进化算法是一种通过趋同、异化等操作,不断迭代进行优化学习的方法,基本的进化过程如下:(1)群体生成.在解空间中随机生成P 个个体,所有个体组成一个群体.根据适应度函数计算出每个个体的得分.(2)子群体生成.得分最高的前M 个个体作为优胜个体,前第M+1 到第M+N 共N 个个体作为临时个体.以所选优胜个体和临时个体为中心,生成M 个优胜子群体和N 个临时子群体,每个子群体的个体数目为P/(N+M).(3)趋同操作.各子群体内部个体为成为胜者而进行局部竞争,此过程为趋同过程.若一个子群体不在产生新的胜者(即子群体成熟),则竞争结束,该子群体的得分就是子群体中最优个体的得分,并把得分张贴在全局公告板上.直到所有子群体全部成熟,趋同过程结束.(4)异化操作.成熟后的子群体之间为成为胜者而进行全局竞争,不断探索新的解空间,此过程为异化操作.从全局公告板上,比较优胜子群体和临时子群体的得分高低,完成子群体间的替换、废弃、个体释放的过程,最后得到全局最优个体及其得分.(5)迭代操作.异化结束后,被释放的个体重新被新的临时子群体补充,重复(3)-(4)过程,直到最优个体的得分不再提高或迭代结束,则认为运算收敛,输出最优个体.思维进化算法框图如图1 所示:图1 思维进化算法框图Fig.1 Block diagram of mind evolutionary algorithm 2 BP 神经网络BP(Back Propagation)神经网络一种误差反向传播的多层前馈神经网络,由Rumelhart 和McCelland 等学者在1986年提出.BP 神经网络由三层网络--输入层、隐含层和输出层组成,隐含层可以有一层或多层.输入信号经输入层逐层传输到各隐含层,最后传向输出层.隐含层和输出层根据相应神经元的权值和阈值完成数据计算工作.若输出结果不满足期望值,误差信号反向逐层传到各隐含层和输入层,利用梯度最速下降法,调整各神经元的权值和阈值.输入正向传播和误差反向传播反复迭代,直到输出误差最小或输出达到期望值,计算结束.利用BP 神经网络拟合非线性函数的一般过程如下:(1)构建BP 神经网络.根据需要拟合的非线性函数特征,确定隐含层层数,选择各层网络节点数目和隐含层、输出层传输函数等.(2)训练BP 神经网络.初始化连接权值和阈值,设定学习速率和训练目标;依次计算隐含层输出、输出层输出和输出误差;根据误差信号,依次更新各层神经元间的权值和阈值;反复迭代,直到输出误差最小或满足期望值,训练结束.(3)BP 神经网络预测.用训练好的BP 网络预测非线性函数输出,然后分析预测结果. BP 神经网络具有较强的非线性映射能力,拟合函数具有一定的效果,但拟合精度较低,且容易陷入局部极值点.为了提高精度,实现全局优化,本文采用思维进化算法来优化BP 神经网络,实现非线性函数的高精度拟合.3 MEA-BP 神经网络拟合非线性函数的算法实现思维进化算法在BP 神经网络拟合非线性函数的算法实现,首先根据拟合函数的输入输出参数确定BP 神经网络拓扑结构,进而得到思维进化算法个体的编码长度,并构建优化算法模型.然后,用思维进化算法对BP 神经网络的初始权值和阈值进行优化,选取训练数据的均方误差的倒数作为各个种群和个体的得分函数,经过不断趋同、异化、迭代,输出最优个体.最后解析最优个体,得到BP 神经网络的初始权值和阈值,再利用训练数据样本训练BP 神经网络,利用测试数据样本预测网络性能.优化算法实现流程图如图2 所示.图2 算法流程图Fig.2 Flow chart of mind evolutionary algorithm4 仿真实验为了验证思维进化算法优化BP 神经网络后的预测精度,选择非线性函数式(1)进行拟合.采用MATLAB 软件编程实现BP 神经网络拟合算法和思维进化算法优化BP 神经网络拟合算法,并对这两种算法的预测精度进行比较.拟合的非线性函数如下:4.1 参数设置拟合函数为两个输入,一个输出,设定BP 网络拓扑结构为2-10-1,即输入层、隐含层和输出层的节点数分别为2个、10 个和1 个.网络学习速率为0.1,训练次数1000 次,训练目标10-6.隐含层和输出层的传输函数都选择S 型正切函数‘tansig’;网络训练函数选择L-M 算法函数‘trainlm’;权值学习函数选择梯度下降动量学习函数‘learngdm’.思维进化算法种群大小设定为400,优胜子种群和临时子种群个数全部为5,子种群大小为40,个体编码长度为21,迭代次数20,适应度函数为均方误差的倒数.根据式(1)随机产生2000 组数据,任取1950 组用于训练网络,其余50 组用于预测网络.4.2 结果分析为了清晰观察网络优化后的预测结果,首先利用1950 组数据分别训练BP 神经网络和思维进化算法优化后的BP 神经网络,然后利用训练后的两个网络预测其余50 组数据,最后分析比较这两种算法的预测误差和误差百分比参数.预测误差及误差百分比如图3 和图4 所示.图3 BP 神经网络与MEA-BP 神经网络预测误差比较Fig.3 Prediction errors between BP neural network and MEA-BP neural network图4 BP 神经网络与MEA-BP 神经网络预测误差百分比Fig.4 Percentage of prediction errors between BP neural network and MEA-BP neural network 从图3 和图4 可以清楚看到,对于非线性函数,BP 神经网络具有一定的拟合能力,但预测精度仍然较差,但是,经过思维进化算法优化后的BP 神经网络预测误差明显减小,且误差相对稳定.其他预测参数比较如表1 所示.表1 BP 神经网络与MEA-BP 神经网络预测结果比较Tab.1 Prediction results of BP neural network and MEA-BP neural network从表1 可以看到,MEA-BP 神经网络预测误差远小于BP 神经网络的预测误差,且网络训练时间也较短,可见MEA-BP 神经网络具有更高的拟合性能.4.3 其他函数的拟合按照同样的参数设定和拟合方法,对其他非线性函数进行拟合实验,实验函数如式(2)、(3)和(4).通过网络训练和预测,得到两种算法的实验结果,如表2 所示.表2 实验结果比较Tab.2 Comparison of experimental consequences从表2 中可以看出,对非线性函数拟合,经过思维进化算法优化后的BP 神经网络的预测精度高于直接使用BP 神经网络,且网络训练时间相对较短,也可以看出优化后的BP 神经网络泛化性能得到进一步提高.5 结语本文采用全局搜索能力极强的思维进化算法对BP 神经网络进行优化.该算法的基本思想是在训练BP 神经网络前,利用思维进化算法对BP 神经网络的初始权值和阈值进行优化,以提高网络的准确性.从多个非线性函数拟合实验结果分析比较得到,思维进化算法优化BP 神经网络的性能明显优于BP神经网络,非线性函数拟合精度更高.本文研究方法在工程和实验的数据拟合方面具有重要意义,也为研究和改进神经网络提供了一种新的思路,为解决其他实际问题提供了新的手段.参考文献:[1]张宝堃,张宝一.基于BP 神经网络的非线性函数拟合[J].电脑知识与技术,2012,8(27):6579-6583.[2]Li Song,Liu Lijun,Huo Man.Prediction for short-term traffic flow based on modified PSO optimized BP neural network[J].Systems Engineering-Theory & Practice,2012,32(9):2045-2049.[3]Xu Yishan,Zeng Bi,Yin Xiuwen,et al.BP neural network and its applications based on improved PSO[J].Computer Engineering and Applications,2009,45(35):233-235.[4]徐富强,钱云,刘相国.GA-BP 神经网络的非线性函数拟合[J].微计算机信息,2012,28(7):148-150.[5]沈学利,张红岩,张纪锁.改进粒子群算法对BP 神经网络的优化[J].计算机系统应用,2012,19(2):57-61.[6]乔冰琴,常晓明.改进粒子群算法在BP 神经网络拟合非线性函数方面的应用[J].太原理工大学学报,2012,43(5):558-559.[7]Chengyi Sun.Mind-Evolution-Based Machine Learning:Frameworkand the Implementation of Optimization[A].In:Proceedings of IEEE International Conference on Intelligent Engineering Systems[C].1998,355-359.[8]谢刚.免疫思维进化算法及其工程应用[D].太原:太原理工大学,2006,27-28.[9]Sun Yan,Sun Yu,Sun Chengyi.Clustering and Reconstruction of Color images Using MEBML[A].In:Proceedings of International Conference on Neural Networks & Brain[C].Beijing,China,1998,361-365.[10]Cheng Mingqi.Gray image segmentation on MEBML frame [J].Intelligent Control and Automation,2000,1:135-137.[11]Chengyi Sun,Yan Sun,Yu Sun.Economic prediction system using double models[J].Systems,Man,and Cyberntics,2000,3:1978-1983. [12]韩晓霞,谢克明.基于思维进化算法的模糊自寻优控制[J].太原理工大学学报,2004,35(5):523-525.[13]Keming Xie,Changhua Mou,Gang Xie.The multi-parameter combination mind-evolutionary-based machine learning and its application[J].Systems,Man,and Cybernetics,2000,1:183-187.。
Survey of clustering data mining techniques
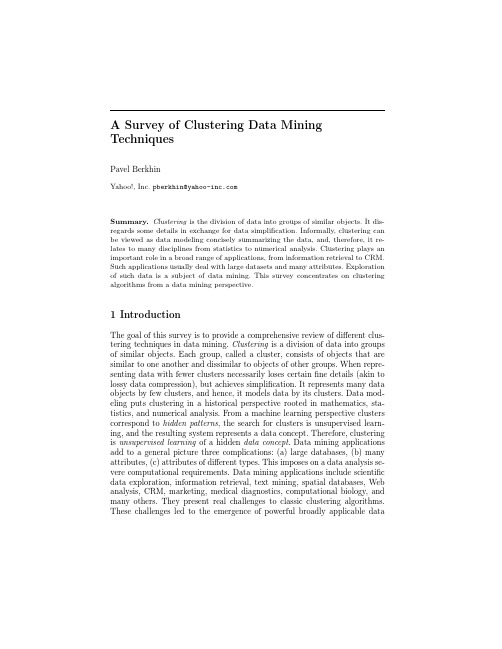
A Survey of Clustering Data Mining TechniquesPavel BerkhinYahoo!,Inc.pberkhin@Summary.Clustering is the division of data into groups of similar objects.It dis-regards some details in exchange for data simplifirmally,clustering can be viewed as data modeling concisely summarizing the data,and,therefore,it re-lates to many disciplines from statistics to numerical analysis.Clustering plays an important role in a broad range of applications,from information retrieval to CRM. Such applications usually deal with large datasets and many attributes.Exploration of such data is a subject of data mining.This survey concentrates on clustering algorithms from a data mining perspective.1IntroductionThe goal of this survey is to provide a comprehensive review of different clus-tering techniques in data mining.Clustering is a division of data into groups of similar objects.Each group,called a cluster,consists of objects that are similar to one another and dissimilar to objects of other groups.When repre-senting data with fewer clusters necessarily loses certainfine details(akin to lossy data compression),but achieves simplification.It represents many data objects by few clusters,and hence,it models data by its clusters.Data mod-eling puts clustering in a historical perspective rooted in mathematics,sta-tistics,and numerical analysis.From a machine learning perspective clusters correspond to hidden patterns,the search for clusters is unsupervised learn-ing,and the resulting system represents a data concept.Therefore,clustering is unsupervised learning of a hidden data concept.Data mining applications add to a general picture three complications:(a)large databases,(b)many attributes,(c)attributes of different types.This imposes on a data analysis se-vere computational requirements.Data mining applications include scientific data exploration,information retrieval,text mining,spatial databases,Web analysis,CRM,marketing,medical diagnostics,computational biology,and many others.They present real challenges to classic clustering algorithms. These challenges led to the emergence of powerful broadly applicable data2Pavel Berkhinmining clustering methods developed on the foundation of classic techniques.They are subject of this survey.1.1NotationsTo fix the context and clarify terminology,consider a dataset X consisting of data points (i.e.,objects ,instances ,cases ,patterns ,tuples ,transactions )x i =(x i 1,···,x id ),i =1:N ,in attribute space A ,where each component x il ∈A l ,l =1:d ,is a numerical or nominal categorical attribute (i.e.,feature ,variable ,dimension ,component ,field ).For a discussion of attribute data types see [106].Such point-by-attribute data format conceptually corresponds to a N ×d matrix and is used by a majority of algorithms reviewed below.However,data of other formats,such as variable length sequences and heterogeneous data,are not uncommon.The simplest subset in an attribute space is a direct Cartesian product of sub-ranges C = C l ⊂A ,C l ⊂A l ,called a segment (i.e.,cube ,cell ,region ).A unit is an elementary segment whose sub-ranges consist of a single category value,or of a small numerical bin.Describing the numbers of data points per every unit represents an extreme case of clustering,a histogram .This is a very expensive representation,and not a very revealing er driven segmentation is another commonly used practice in data exploration that utilizes expert knowledge regarding the importance of certain sub-domains.Unlike segmentation,clustering is assumed to be automatic,and so it is a machine learning technique.The ultimate goal of clustering is to assign points to a finite system of k subsets (clusters).Usually (but not always)subsets do not intersect,and their union is equal to a full dataset with the possible exception of outliersX =C 1 ··· C k C outliers ,C i C j =0,i =j.1.2Clustering Bibliography at GlanceGeneral references regarding clustering include [110],[205],[116],[131],[63],[72],[165],[119],[75],[141],[107],[91].A very good introduction to contem-porary data mining clustering techniques can be found in the textbook [106].There is a close relationship between clustering and many other fields.Clustering has always been used in statistics [10]and science [158].The clas-sic introduction into pattern recognition framework is given in [64].Typical applications include speech and character recognition.Machine learning clus-tering algorithms were applied to image segmentation and computer vision[117].For statistical approaches to pattern recognition see [56]and [85].Clus-tering can be viewed as a density estimation problem.This is the subject of traditional multivariate statistical estimation [197].Clustering is also widelyA Survey of Clustering Data Mining Techniques3 used for data compression in image processing,which is also known as vec-tor quantization[89].Datafitting in numerical analysis provides still another venue in data modeling[53].This survey’s emphasis is on clustering in data mining.Such clustering is characterized by large datasets with many attributes of different types. Though we do not even try to review particular applications,many important ideas are related to the specificfields.Clustering in data mining was brought to life by intense developments in information retrieval and text mining[52], [206],[58],spatial database applications,for example,GIS or astronomical data,[223],[189],[68],sequence and heterogeneous data analysis[43],Web applications[48],[111],[81],DNA analysis in computational biology[23],and many others.They resulted in a large amount of application-specific devel-opments,but also in some general techniques.These techniques and classic clustering algorithms that relate to them are surveyed below.1.3Plan of Further PresentationClassification of clustering algorithms is neither straightforward,nor canoni-cal.In reality,different classes of algorithms overlap.Traditionally clustering techniques are broadly divided in hierarchical and partitioning.Hierarchical clustering is further subdivided into agglomerative and divisive.The basics of hierarchical clustering include Lance-Williams formula,idea of conceptual clustering,now classic algorithms SLINK,COBWEB,as well as newer algo-rithms CURE and CHAMELEON.We survey these algorithms in the section Hierarchical Clustering.While hierarchical algorithms gradually(dis)assemble points into clusters (as crystals grow),partitioning algorithms learn clusters directly.In doing so they try to discover clusters either by iteratively relocating points between subsets,or by identifying areas heavily populated with data.Algorithms of thefirst kind are called Partitioning Relocation Clustering. They are further classified into probabilistic clustering(EM framework,al-gorithms SNOB,AUTOCLASS,MCLUST),k-medoids methods(algorithms PAM,CLARA,CLARANS,and its extension),and k-means methods(differ-ent schemes,initialization,optimization,harmonic means,extensions).Such methods concentrate on how well pointsfit into their clusters and tend to build clusters of proper convex shapes.Partitioning algorithms of the second type are surveyed in the section Density-Based Partitioning.They attempt to discover dense connected com-ponents of data,which areflexible in terms of their shape.Density-based connectivity is used in the algorithms DBSCAN,OPTICS,DBCLASD,while the algorithm DENCLUE exploits space density functions.These algorithms are less sensitive to outliers and can discover clusters of irregular shape.They usually work with low-dimensional numerical data,known as spatial data. Spatial objects could include not only points,but also geometrically extended objects(algorithm GDBSCAN).4Pavel BerkhinSome algorithms work with data indirectly by constructing summaries of data over the attribute space subsets.They perform space segmentation and then aggregate appropriate segments.We discuss them in the section Grid-Based Methods.They frequently use hierarchical agglomeration as one phase of processing.Algorithms BANG,STING,WaveCluster,and FC are discussed in this section.Grid-based methods are fast and handle outliers well.Grid-based methodology is also used as an intermediate step in many other algorithms (for example,CLIQUE,MAFIA).Categorical data is intimately connected with transactional databases.The concept of a similarity alone is not sufficient for clustering such data.The idea of categorical data co-occurrence comes to the rescue.The algorithms ROCK,SNN,and CACTUS are surveyed in the section Co-Occurrence of Categorical Data.The situation gets even more aggravated with the growth of the number of items involved.To help with this problem the effort is shifted from data clustering to pre-clustering of items or categorical attribute values. Development based on hyper-graph partitioning and the algorithm STIRR exemplify this approach.Many other clustering techniques are developed,primarily in machine learning,that either have theoretical significance,are used traditionally out-side the data mining community,or do notfit in previously outlined categories. The boundary is blurred.In the section Other Developments we discuss the emerging direction of constraint-based clustering,the important researchfield of graph partitioning,and the relationship of clustering to supervised learning, gradient descent,artificial neural networks,and evolutionary methods.Data Mining primarily works with large databases.Clustering large datasets presents scalability problems reviewed in the section Scalability and VLDB Extensions.Here we talk about algorithms like DIGNET,about BIRCH and other data squashing techniques,and about Hoffding or Chernoffbounds.Another trait of real-life data is high dimensionality.Corresponding de-velopments are surveyed in the section Clustering High Dimensional Data. The trouble comes from a decrease in metric separation when the dimension grows.One approach to dimensionality reduction uses attributes transforma-tions(DFT,PCA,wavelets).Another way to address the problem is through subspace clustering(algorithms CLIQUE,MAFIA,ENCLUS,OPTIGRID, PROCLUS,ORCLUS).Still another approach clusters attributes in groups and uses their derived proxies to cluster objects.This double clustering is known as co-clustering.Issues common to different clustering methods are overviewed in the sec-tion General Algorithmic Issues.We talk about assessment of results,de-termination of appropriate number of clusters to build,data preprocessing, proximity measures,and handling of outliers.For reader’s convenience we provide a classification of clustering algorithms closely followed by this survey:•Hierarchical MethodsA Survey of Clustering Data Mining Techniques5Agglomerative AlgorithmsDivisive Algorithms•Partitioning Relocation MethodsProbabilistic ClusteringK-medoids MethodsK-means Methods•Density-Based Partitioning MethodsDensity-Based Connectivity ClusteringDensity Functions Clustering•Grid-Based Methods•Methods Based on Co-Occurrence of Categorical Data•Other Clustering TechniquesConstraint-Based ClusteringGraph PartitioningClustering Algorithms and Supervised LearningClustering Algorithms in Machine Learning•Scalable Clustering Algorithms•Algorithms For High Dimensional DataSubspace ClusteringCo-Clustering Techniques1.4Important IssuesThe properties of clustering algorithms we are primarily concerned with in data mining include:•Type of attributes algorithm can handle•Scalability to large datasets•Ability to work with high dimensional data•Ability tofind clusters of irregular shape•Handling outliers•Time complexity(we frequently simply use the term complexity)•Data order dependency•Labeling or assignment(hard or strict vs.soft or fuzzy)•Reliance on a priori knowledge and user defined parameters •Interpretability of resultsRealistically,with every algorithm we discuss only some of these properties. The list is in no way exhaustive.For example,as appropriate,we also discuss algorithms ability to work in pre-defined memory buffer,to restart,and to provide an intermediate solution.6Pavel Berkhin2Hierarchical ClusteringHierarchical clustering builds a cluster hierarchy or a tree of clusters,also known as a dendrogram.Every cluster node contains child clusters;sibling clusters partition the points covered by their common parent.Such an ap-proach allows exploring data on different levels of granularity.Hierarchical clustering methods are categorized into agglomerative(bottom-up)and divi-sive(top-down)[116],[131].An agglomerative clustering starts with one-point (singleton)clusters and recursively merges two or more of the most similar clusters.A divisive clustering starts with a single cluster containing all data points and recursively splits the most appropriate cluster.The process contin-ues until a stopping criterion(frequently,the requested number k of clusters) is achieved.Advantages of hierarchical clustering include:•Flexibility regarding the level of granularity•Ease of handling any form of similarity or distance•Applicability to any attribute typesDisadvantages of hierarchical clustering are related to:•Vagueness of termination criteria•Most hierarchical algorithms do not revisit(intermediate)clusters once constructed.The classic approaches to hierarchical clustering are presented in the sub-section Linkage Metrics.Hierarchical clustering based on linkage metrics re-sults in clusters of proper(convex)shapes.Active contemporary efforts to build cluster systems that incorporate our intuitive concept of clusters as con-nected components of arbitrary shape,including the algorithms CURE and CHAMELEON,are surveyed in the subsection Hierarchical Clusters of Arbi-trary Shapes.Divisive techniques based on binary taxonomies are presented in the subsection Binary Divisive Partitioning.The subsection Other Devel-opments contains information related to incremental learning,model-based clustering,and cluster refinement.In hierarchical clustering our regular point-by-attribute data representa-tion frequently is of secondary importance.Instead,hierarchical clustering frequently deals with the N×N matrix of distances(dissimilarities)or sim-ilarities between training points sometimes called a connectivity matrix.So-called linkage metrics are constructed from elements of this matrix.The re-quirement of keeping a connectivity matrix in memory is unrealistic.To relax this limitation different techniques are used to sparsify(introduce zeros into) the connectivity matrix.This can be done by omitting entries smaller than a certain threshold,by using only a certain subset of data representatives,or by keeping with each point only a certain number of its nearest neighbors(for nearest neighbor chains see[177]).Notice that the way we process the original (dis)similarity matrix and construct a linkage metric reflects our a priori ideas about the data model.A Survey of Clustering Data Mining Techniques7With the(sparsified)connectivity matrix we can associate the weighted connectivity graph G(X,E)whose vertices X are data points,and edges E and their weights are defined by the connectivity matrix.This establishes a connection between hierarchical clustering and graph partitioning.One of the most striking developments in hierarchical clustering is the algorithm BIRCH.It is discussed in the section Scalable VLDB Extensions.Hierarchical clustering initializes a cluster system as a set of singleton clusters(agglomerative case)or a single cluster of all points(divisive case) and proceeds iteratively merging or splitting the most appropriate cluster(s) until the stopping criterion is achieved.The appropriateness of a cluster(s) for merging or splitting depends on the(dis)similarity of cluster(s)elements. This reflects a general presumption that clusters consist of similar points.An important example of dissimilarity between two points is the distance between them.To merge or split subsets of points rather than individual points,the dis-tance between individual points has to be generalized to the distance between subsets.Such a derived proximity measure is called a linkage metric.The type of a linkage metric significantly affects hierarchical algorithms,because it re-flects a particular concept of closeness and connectivity.Major inter-cluster linkage metrics[171],[177]include single link,average link,and complete link. The underlying dissimilarity measure(usually,distance)is computed for every pair of nodes with one node in thefirst set and another node in the second set.A specific operation such as minimum(single link),average(average link),or maximum(complete link)is applied to pair-wise dissimilarity measures:d(C1,C2)=Op{d(x,y),x∈C1,y∈C2}Early examples include the algorithm SLINK[199],which implements single link(Op=min),Voorhees’method[215],which implements average link (Op=Avr),and the algorithm CLINK[55],which implements complete link (Op=max).It is related to the problem offinding the Euclidean minimal spanning tree[224]and has O(N2)complexity.The methods using inter-cluster distances defined in terms of pairs of nodes(one in each respective cluster)are called graph methods.They do not use any cluster representation other than a set of points.This name naturally relates to the connectivity graph G(X,E)introduced above,because every data partition corresponds to a graph partition.Such methods can be augmented by so-called geometric methods in which a cluster is represented by its central point.Under the assumption of numerical attributes,the center point is defined as a centroid or an average of two cluster centroids subject to agglomeration.It results in centroid,median,and minimum variance linkage metrics.All of the above linkage metrics can be derived from the Lance-Williams updating formula[145],d(C iC j,C k)=a(i)d(C i,C k)+a(j)d(C j,C k)+b·d(C i,C j)+c|d(C i,C k)−d(C j,C k)|.8Pavel BerkhinHere a,b,c are coefficients corresponding to a particular linkage.This formula expresses a linkage metric between a union of the two clusters and the third cluster in terms of underlying nodes.The Lance-Williams formula is crucial to making the dis(similarity)computations feasible.Surveys of linkage metrics can be found in [170][54].When distance is used as a base measure,linkage metrics capture inter-cluster proximity.However,a similarity-based view that results in intra-cluster connectivity considerations is also used,for example,in the original average link agglomeration (Group-Average Method)[116].Under reasonable assumptions,such as reducibility condition (graph meth-ods satisfy this condition),linkage metrics methods suffer from O N 2 time complexity [177].Despite the unfavorable time complexity,these algorithms are widely used.As an example,the algorithm AGNES (AGlomerative NESt-ing)[131]is used in S-Plus.When the connectivity N ×N matrix is sparsified,graph methods directly dealing with the connectivity graph G can be used.In particular,hierarchical divisive MST (Minimum Spanning Tree)algorithm is based on graph parti-tioning [116].2.1Hierarchical Clusters of Arbitrary ShapesFor spatial data,linkage metrics based on Euclidean distance naturally gener-ate clusters of convex shapes.Meanwhile,visual inspection of spatial images frequently discovers clusters with curvy appearance.Guha et al.[99]introduced the hierarchical agglomerative clustering algo-rithm CURE (Clustering Using REpresentatives).This algorithm has a num-ber of novel features of general importance.It takes special steps to handle outliers and to provide labeling in assignment stage.It also uses two techniques to achieve scalability:data sampling (section 8),and data partitioning.CURE creates p partitions,so that fine granularity clusters are constructed in parti-tions first.A major feature of CURE is that it represents a cluster by a fixed number,c ,of points scattered around it.The distance between two clusters used in the agglomerative process is the minimum of distances between two scattered representatives.Therefore,CURE takes a middle approach between the graph (all-points)methods and the geometric (one centroid)methods.Single and average link closeness are replaced by representatives’aggregate closeness.Selecting representatives scattered around a cluster makes it pos-sible to cover non-spherical shapes.As before,agglomeration continues until the requested number k of clusters is achieved.CURE employs one additional trick:originally selected scattered points are shrunk to the geometric centroid of the cluster by a user-specified factor α.Shrinkage suppresses the affect of outliers;outliers happen to be located further from the cluster centroid than the other scattered representatives.CURE is capable of finding clusters of different shapes and sizes,and it is insensitive to outliers.Because CURE uses sampling,estimation of its complexity is not straightforward.For low-dimensional data authors provide a complexity estimate of O (N 2sample )definedA Survey of Clustering Data Mining Techniques9 in terms of a sample size.More exact bounds depend on input parameters: shrink factorα,number of representative points c,number of partitions p,and a sample size.Figure1(a)illustrates agglomeration in CURE.Three clusters, each with three representatives,are shown before and after the merge and shrinkage.Two closest representatives are connected.While the algorithm CURE works with numerical attributes(particularly low dimensional spatial data),the algorithm ROCK developed by the same researchers[100]targets hierarchical agglomerative clustering for categorical attributes.It is reviewed in the section Co-Occurrence of Categorical Data.The hierarchical agglomerative algorithm CHAMELEON[127]uses the connectivity graph G corresponding to the K-nearest neighbor model spar-sification of the connectivity matrix:the edges of K most similar points to any given point are preserved,the rest are pruned.CHAMELEON has two stages.In thefirst stage small tight clusters are built to ignite the second stage.This involves a graph partitioning[129].In the second stage agglomer-ative process is performed.It utilizes measures of relative inter-connectivity RI(C i,C j)and relative closeness RC(C i,C j);both are locally normalized by internal interconnectivity and closeness of clusters C i and C j.In this sense the modeling is dynamic:it depends on data locally.Normalization involves certain non-obvious graph operations[129].CHAMELEON relies heavily on graph partitioning implemented in the library HMETIS(see the section6). Agglomerative process depends on user provided thresholds.A decision to merge is made based on the combinationRI(C i,C j)·RC(C i,C j)αof local measures.The algorithm does not depend on assumptions about the data model.It has been proven tofind clusters of different shapes,densities, and sizes in2D(two-dimensional)space.It has a complexity of O(Nm+ Nlog(N)+m2log(m),where m is the number of sub-clusters built during the first initialization phase.Figure1(b)(analogous to the one in[127])clarifies the difference with CURE.It presents a choice of four clusters(a)-(d)for a merge.While CURE would merge clusters(a)and(b),CHAMELEON makes intuitively better choice of merging(c)and(d).2.2Binary Divisive PartitioningIn linguistics,information retrieval,and document clustering applications bi-nary taxonomies are very useful.Linear algebra methods,based on singular value decomposition(SVD)are used for this purpose in collaborativefilter-ing and information retrieval[26].Application of SVD to hierarchical divisive clustering of document collections resulted in the PDDP(Principal Direction Divisive Partitioning)algorithm[31].In our notations,object x is a docu-ment,l th attribute corresponds to a word(index term),and a matrix X entry x il is a measure(e.g.TF-IDF)of l-term frequency in a document x.PDDP constructs SVD decomposition of the matrix10Pavel Berkhin(a)Algorithm CURE (b)Algorithm CHAMELEONFig.1.Agglomeration in Clusters of Arbitrary Shapes(X −e ¯x ),¯x =1Ni =1:N x i ,e =(1,...,1)T .This algorithm bisects data in Euclidean space by a hyperplane that passes through data centroid orthogonal to the eigenvector with the largest singular value.A k -way split is also possible if the k largest singular values are consid-ered.Bisecting is a good way to categorize documents and it yields a binary tree.When k -means (2-means)is used for bisecting,the dividing hyperplane is orthogonal to the line connecting the two centroids.The comparative study of SVD vs.k -means approaches [191]can be used for further references.Hier-archical divisive bisecting k -means was proven [206]to be preferable to PDDP for document clustering.While PDDP or 2-means are concerned with how to split a cluster,the problem of which cluster to split is also important.Simple strategies are:(1)split each node at a given level,(2)split the cluster with highest cardinality,and,(3)split the cluster with the largest intra-cluster variance.All three strategies have problems.For a more detailed analysis of this subject and better strategies,see [192].2.3Other DevelopmentsOne of early agglomerative clustering algorithms,Ward’s method [222],is based not on linkage metric,but on an objective function used in k -means.The merger decision is viewed in terms of its effect on the objective function.The popular hierarchical clustering algorithm for categorical data COB-WEB [77]has two very important qualities.First,it utilizes incremental learn-ing.Instead of following divisive or agglomerative approaches,it dynamically builds a dendrogram by processing one data point at a time.Second,COB-WEB is an example of conceptual or model-based learning.This means that each cluster is considered as a model that can be described intrinsically,rather than as a collection of points assigned to it.COBWEB’s dendrogram is calleda classification tree.Each tree node(cluster)C is associated with the condi-tional probabilities for categorical attribute-values pairs,P r(x l=νlp|C),l=1:d,p=1:|A l|.This easily can be recognized as a C-specific Na¨ıve Bayes classifier.During the classification tree construction,every new point is descended along the tree and the tree is potentially updated(by an insert/split/merge/create op-eration).Decisions are based on the category utility[49]CU{C1,...,C k}=1j=1:kCU(C j)CU(C j)=l,p(P r(x l=νlp|C j)2−(P r(x l=νlp)2.Category utility is similar to the GINI index.It rewards clusters C j for in-creases in predictability of the categorical attribute valuesνlp.Being incre-mental,COBWEB is fast with a complexity of O(tN),though it depends non-linearly on tree characteristics packed into a constant t.There is a similar incremental hierarchical algorithm for all numerical attributes called CLAS-SIT[88].CLASSIT associates normal distributions with cluster nodes.Both algorithms can result in highly unbalanced trees.Chiu et al.[47]proposed another conceptual or model-based approach to hierarchical clustering.This development contains several different use-ful features,such as the extension of scalability preprocessing to categori-cal attributes,outliers handling,and a two-step strategy for monitoring the number of clusters including BIC(defined below).A model associated with a cluster covers both numerical and categorical attributes and constitutes a blend of Gaussian and multinomial models.Denote corresponding multivari-ate parameters byθ.With every cluster C we associate a logarithm of its (classification)likelihoodl C=x i∈Clog(p(x i|θ))The algorithm uses maximum likelihood estimates for parameterθ.The dis-tance between two clusters is defined(instead of linkage metric)as a decrease in log-likelihoodd(C1,C2)=l C1+l C2−l C1∪C2caused by merging of the two clusters under consideration.The agglomerative process continues until the stopping criterion is satisfied.As such,determina-tion of the best k is automatic.This algorithm has the commercial implemen-tation(in SPSS Clementine).The complexity of the algorithm is linear in N for the summarization phase.Traditional hierarchical clustering does not change points membership in once assigned clusters due to its greedy approach:after a merge or a split is selected it is not refined.Though COBWEB does reconsider its decisions,its。
中兴ZXR10 5960系列交换机数据表说明书
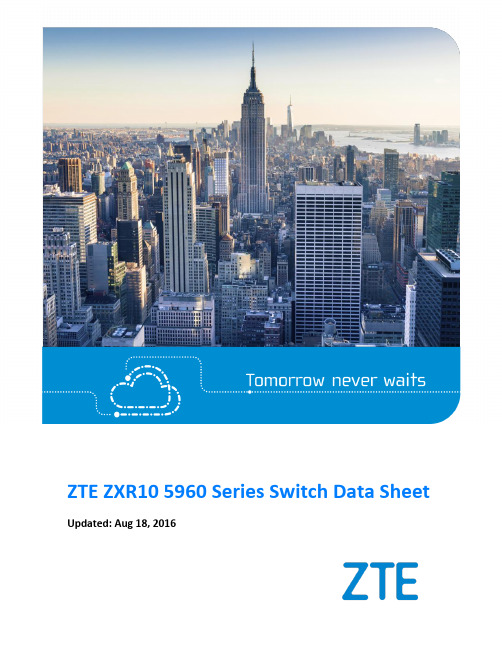
ZTE ZXR10 5960 Series Switch Data Sheet Updated: Aug 18, 2016Product OverviewThe ZXR10 5960 Series switch is next-generation switch with high switching capacity and high port density for data center TOR and carrier access and aggregation scenario. It provides high density 10GE/40GE interfaces, carrier-class reliability and superior scalability. The ZXR10 5960 Series switch supports extensive data center service features such as VSC2.0 (Virtual Switch Cluster)/ TRILL (Transparent Interconnection of Lots of Links)/ Front-to-back Airflow and Ethernet ring protection for L2 Ethernet service. The ZXR10 5960 Series switch can work with the ZXR10 9900 Series switch to build an elastic, virtualized, high-quality switching network that meets the requirements of cloud-computing data centers.The ZXR10 5960 Series switch offers the following switch products:ZXR10 5960-32DL ZXR10 5960-64DL5960-32DL: 24 10GE SFP+ optical ports and 2 40GE QSFP+ optical ports, 2 fan module, 2 AC/DC/HVDC power supply modules, front-to-back airflow.5960-64DL: 48 10GE SFP+ optical ports and 4 40GE QSFP+ optical ports, 2 fan module, 2 AC/DC/HVDC power supply modules, front-to-back airflow.ZXR10 5960-64NL ZXR10 5960-28TM5960-64NL:48 10GE RJ45 electrical ports and 4 40GE QSFP+ optical ports, 2 fan modules, 2 AC/DC/HVDC power supply modules, front-to-back airflow.5960-28TM:24 Ethernet 10/100/1000M RJ45 electrical ports and one expansion slot, 2 fan modules, 2 AC/DC/HVDC power supply modules, front-to-back airflow.ZXR10 5960-52TM5960-52TM:48 Ethernet 10/100/1000M RJ45 electrical ports and one expansion slot, 2 fan modules, 2 AC/DC/HVDC power supply modules, front-to-back airflow.The expansion card slot can be equipped with the following expansion cards:● 4 * 10GE SFP+ optical ports card● 4 * GE SFP optical ports card● 4 * GE RJ45 electrical ports cardProduct Features•Up to 64×10GE Ports, 1RU Switch with 40GE Interface-The ZXR10 5960 Series switch supports up to 1.28Tbps wire-speed switching capacity with high density10GE/40GE interfaces. It delivers huge bandwidth capability to fulfill the growing service requirement in data center.-1RU switch supports up to 64×10GE ports when expand 40GE port into 4 10GE ports, in order to access large scale 10GE servers.-The 10 GE ports support working as GE ports, 40GE port can be expanded into 4 10 GE ports, flexible interface combination delivers easy deployment for TOR switch and savecost for customer.•Deliver Better Data Center Service Experience.-Support TRILL (Transparent Interconnection of Lots of Links) L2 multi-path technology and meet large L2 networking needs without STP. The load is shared between links andbandwidth resources usage is nearly 100%. The ZXR10 5960 Series switch can be used to build large L2 network with over 500 nodes, which can meet the requirement of VM(Virtual Machine) migration.-Support DCB (Data Center Bridging) protocol family and fully guarantee network reliability and no loss in full range. The ZXR10 5960 Series switch supports PFC(Priority-based Flow Control), QCN (Quantized Congestion Notification), ETS (EnhancedTransmission Selection), DCBX (Data Center Bridging Exchange), which ensure lowlatency and zero packet loss for high-speed computing services.-Support multiple EVB (Ethernet Virtual Bridging) patterns including VM, VEB and VEPA (Virtual Ethernet Port aggregator), and these different patterns can coexist, so it can fulfilldifferent networking requirements. With full support for related protocols like VDP (VSIDiscovery Protocol), EDCP (Edge Devices Communication Protocol) and CDCP(S-Channel Discovery and Configuration Protocol), EVB can run smoothly.-Provide strict front and rear air ducts in line with the requirements of data center construction.Innovative VSC2.0 (Virtual Switch Cluster) Technology-Support VSC2.0 (Virtual Switch Cluster), which enhances cluster system capacity and port density, simplifies network topology and management.-Real time hot-standby information synchronization between master and standby master to ensure seamless switch over against network failures, enhancing network reliability.-Stacking bandwidth between the VSC switches can be up to 320Gbps, which can solve the bandwidth bottleneck of VSC and deliver customers a real-time non-blocking VSCsystem.-The stacking distance can be up to 80km, it helps customers get rid of distance restriction while designing a reliable VSC system.-Master and slave in VSC works in 1+N redundancy mode, MAD (Multi-active Detect) technology is used to detect and avoid dual master in VSC system when failure happens.Together with real-time hot-standby and seamless switchover it brings customer a moreflexible VSC network.-No need special stacking sub-card, the normal ports can be used for VSC2.0 connecting, which helps customers save investments.•Powerful Service Bearing Capability-By supporting rich L2 switching and L3 routing functions and low latency forwarding, The ZXR10 5960 Series switch can bear lots of service including WLAN, Internet, Voice,Video, Enterprise private network and other data services.-Support Voice VLAN (which means the automatic assignment of dedicated VLAN and QoS strategy to voice equipment), thus enabling the voice traffic to enjoy high priority.-Support L2/L3 multicast, including IGMP snooping, Filtering, Proxy and Fast leave, MVR (Multicast VLAN Registration) and PIM to facilitate the services deployments such asMulti-terminal HD video surveillance and video conferencing. •Comprehensive IPv6 Solution-The ZXR10 5960 Series switch has passed the IPv6 Ready Phase 2 Gold Medal Certification issued by IPv6 Forum.-Support IPv6 unicast routing protocols: IPv6 static routing, RIPng, OSPFv3, IS-ISv6, and BGP4+ and multicast features: MLD v1/v2, MLD snooping, PIMv6.-Support IPv4-to-IPv6 tunnel technologies: IPv6 manual tunnels, 6-to-4 tunnel, ISATAP tunnel and IPv4-compatible automatic tunnel, etc.•Carrier-Grade Reliability and Multi-Dimensional Security-Support dual redundant modular fan and dual modular power supply.-Support Ethernet OAM, including IEEE 802.3ah, 802.1ag, help monitor network real-time operating status and fulfill fast fault detection, fault location.-Support various authentication methods such as 802.1x, Radius, TACACS+. Support CPU overload protection, anti-DDOS, deliver customer a security network.•Easy Maintenance, Saving OPEX-The innovative M-Button delivers instant trouble-shooting by reading indicators on front panel without login via terminal. It helps to solve some common problems immediately.-Zero-touch provisioning, download software and configuration to the switch automatically from the server, Reduce provision process and man power requirement.-Support the SQA (Service Quality Analyzer), detecting the network quality periodically or in real time. In order to provide better quality of service for more valuable services.-Support ALS (automatic laser shutdown), protect people against laser injury when plug out the optical module.Green for More-Front-to-back shoot-through airflow and more air hole design in the front panel improve heat dissipation to reduce power consumption.-Fan speed can automatically adjust by 5 levels in accordance with the temperature inside the switch. It not only saves the power consumption, but also reduces noise andextends life cycle of the fans.-Complying with ROHS, WEEE and ISO14001 certification, No plumbum (Pb) in not only product materials but also the whole processing technic. Meanwhile, use re-cyclesdegradable packing materials, practice green for more.System SpecificationFunction SpecificationApplication Scenarios•TOR in Typical Data Center NetworkThe ZXR10 5960 Series switch works as TOR switch on a typical data center network. The ZXR10 5960 Series switch has high-density 10GE/40GE interfaces to interconnect the 10GE servers and aggregates to the ZXR10 9900 Series switch through 40GE/100GE interfaces. Deploy EBGP and VSC technology to build a non-blocking Layer 3 network, which allows large-scale VM migrations and flexible service deployments.•Aggregation in Carrier NetworkThe ZXR10 5960 Series switch works as high density 10GE aggregation switch which provide40GE as uplink. Through L2/L3 features and ZESR ring protection, the ZXR10 5960 Series switch aggregates the traffic from the access and forward it to the core switch. VSC2.0 technology can realize fast switchover when failure happens.10GE Access for Business CustomerAs the bandwidth increasing fast, more and more business customers need 10GE interface access. The ZXR10 5960 Series switch works as high density 10GE interface switch which provide 40GE as uplink. Through L2/L3 features and ZESR ring protection, the ZXR10 5960 Series switch access the traffic from the business customer and forward it to the aggregation switch.Order Information MainframePower ModuleFan ModuleNO. 55, Hi-tech Road South,ShenZhen,P. R. China Postcode: 518057Web: Tel: +86-755-26770000Fax: +86-755-26771999。
Entropy changes in the clustering of galaxies in a

Vol.3, No.1, 65-68 (2011)doi:10.4236/ns.2011.31009Natural ScienceEntropy changes in the clustering of galaxies in an expanding universeNaseer Iqbal1,2*, Mohammad Shafi Khan1, Tabasum Masood11Department of Physics, University of Kashmir, Srinagar, India; *Corresponding Author:2Interuniversity Centre for Astronomy and Astrophysics, Pune, India.Received 19 October 2010; revised 23 November 2010; accepted 26 November 2010.ABSTRACTIn the present work the approach-thermody- namics and statistical mechanics of gravitating systems is applied to study the entropy change in gravitational clustering of galaxies in an ex-panding universe. We derive analytically the expressions for gravitational entropy in terms of temperature T and average density n of the par-ticles (galaxies) in the given phase space cell. It is found that during the initial stage of cluster-ing of galaxies, the entropy decreases and fi-nally seems to be increasing when the system attains virial equilibrium. The entropy changes are studied for different range of measuring correlation parameter b. We attempt to provide a clearer account of this phenomena. The entropy results for a system consisting of extended mass (non-point mass) particles show a similar behaviour with that of point mass particles clustering gravitationally in an expanding uni-verse.Keywords:Gravitational Clustering; Thermodynamics; Entropy; Cosmology1. INTRODUCTIONGalaxy groups and clusters are the largest known gravitationally bound objects to have arisen thus far in the process of cosmic structure formation [1]. They form the densest part of the large scale structure of the uni-verse. In models for the gravitational formation of struc-ture with cold dark matter, the smallest structures col-lapse first and eventually build the largest structures; clusters of galaxies are then formed relatively. The clus-ters themselves are often associated with larger groups called super-clusters. Clusters of galaxies are the most recent and most massive objects to have arisen in the hiearchical structure formation of the universe and the study of clusters tells one about the way galaxies form and evolve. The average density n and the temperature T of a gravitating system discuss some thermal history of cluster formation. For a better larger understanding of this thermal history it is important to study the entropy change resulting during the clustering phenomena be-cause the entropy is the quantity most directly changed by increasing or decreasing thermal energy of intraclus-ter gas. The purpose of the present paper is to show how entropy of the universe changes with time in a system of galaxies clustering under the influence of gravitational interaction.Entropy is a measure of how disorganised a system is. It forms an important part of second law of thermody-namics [2,3]. The concept of entropy is generally not well understood. For erupting stars, colloiding galaxies, collapsing black holes - the cosmos is a surprisingly or-derly place. Supermassive black holes, dark matter and stars are some of the contributors to the overall entropy of the universe. The microscopic explanation of entropy has been challenged both from the experimental and theoretical point of view [11,12]. Entropy is a mathe-matical formula. Standard calculations have shown that the entropy of our universe is dominated by black holes, whose entropy is of the order of their area in planck units [13]. An analysis by Chas Egan of the Australian National University in Canberra indicates that the col-lective entropy of all the supermassive black holes at the centers of galaxies is about 100 times higher than previ-ously calculated. Statistical entropy is logrithmic of the number of microstates consistent with the observed macroscopic properties of a system hence a measure of uncertainty about its precise state. Statistical mechanics explains entropy as the amount of uncertainty which remains about a system after its observable macroscopic properties have been taken into account. For a given set of macroscopic quantities like temperature and volume, the entropy is a function of the probability that the sys-tem is in various quantumn states. The more states avail-able to the system with higher probability, the greater theAll Rights Reserved.N. Iqbal et al. / Natural Science 3 (2011) 65-6866 disorder and thus greater the entropy [2]. In real experi-ments, it is quite difficult to measure the entropy of a system. The technique for doing so is based on the thermodynamic definition of entropy. We discuss the applicability of statistical mechanics and thermodynam-ics for gravitating systems and explain in what sense the entropy change S – S 0 shows a changing behaviour with respect to the measuring correlation parameter b = 0 – 1.2. THERMODYNAMIC DESCRIPTION OF GALAXY CLUSTERSA system of many point particles which interacts by Newtonian gravity is always unstable. The basic insta-bilities which may occur involve the overall contraction (or expansion) of the system, and the formation of clus-ters within the system. The rates and forms of these in-stabilities are governed by the distribution of kinetic and potential energy and the momentum among the particles. For example, a finite spherical system which approxi-mately satisfies the viral theorem, contracts slowlycompared to the crossing time ~ ()12G ρ- due to the evaporation of high energy particles [3] and the lack of equipartition among particles of different masses [4]. We consider here a thermodynamic description for the sys-tem (universe). The universe is considered to be an infi-nite gas in which each gas molecule is treated to be agalaxy. The gravitational force is a binary interaction and as a result a number of particles cluster together. We use the same approximation of binary interaction for our universe (system) consisting of large number of galaxies clustering together under the influence of gravitational force. It is important to mention here that the characteri-zation of this clustering is a problem of current interest. The physical validity of the application of thermody-namics in the clustering of galaxies and galaxy clusters has been discussed on the basis of N-body computer simulation results [5]. Equations of state for internal energy U and pressure P are of the form [6]:(3122NTU =-)b (1) (1NTP V=-)b (2) b defines the measuring correlation parameter and is dimensionless, given by [8]()202,23W nb Gm n T r K Tτξ∞=-=⎰,rdr (3)W is the potential energy and K the kinetic energy ofthe particles in a system. n N V = is the average num-ber density of the system of particles each of mass m, T is the temperature, V the volume, G is the universalgravitational constant. (),,n T r ξ is the two particle correlation function and r is the inter-particle distance. An overall study of (),n T r ξ has already been dis-cussed by [7]. For an ideal gas behaviour b = 0 and for non-ideal gas system b varies between 0 and 1. Previ-ously some workers [7,8] have derived b in the form of:331nT b nT ββ--=+ (4) Eq.4 indicates that b has a specific dependence on the combination 3nT -.3. ENTROPY CALCULATIONSThermodynamics and statistical mechanics have been found to be equal tools in describing entropy of a system. Thermodynamic entropy is a non-conserved state func-tion that is of great importance in science. Historically the concept of entropy evolved in order to explain why some processes are spontaneous and others are not; sys-tems tend to progress in the direction of increasing en-tropy [9]. Following statistical mechanics and the work carried out by [10], the grand canonical partition func-tion is given by()3213212,1!N N N N mkT Z T V V nT N πβ--⎛⎫⎡=+ ⎪⎣Λ⎝⎭⎤⎦(5)where N! is due to the distinguishability of particles. Λrepresents the volume of a phase space cell. N is the number of paricles (galaxies) with point mass approxi-mation. The Helmholtz free energy is given by:ln N A T Z =- (6)Thermodynamic description of entropy can be calcu-lated as:,N VA S T ∂⎛⎫=- ⎪∂⎝⎭ (7)The use of Eq.5 and Eq.6 in Eq.7 gives()3120ln ln 13S S n T b b -⎛⎫-=-- ⎪ ⎪⎝⎭- (8) where S 0 is an arbitary constant. From Eq.4 we write()31bn b T β-=- (9)Using Eq.9, Eq.8 becomes as3203ln S S b bT ⎡⎤-=-+⎢⎣⎦⎥ (10)Again from Eq.4All Rights Reserved.N. Iqbal et al. / Natural Science 3 (2011) 65-68 6767()13221n b T b β-⎡⎤=⎢⎣⎦⎥ (11)with the help of Eq.11, Eq.10 becomes as()011ln ln 1322S S n b b b ⎡-=-+-+⎡⎤⎣⎦⎢⎥⎣⎦⎤ (12) This is the expression for entropy of a system consist-ing of point mass particles, but actually galaxies have extended structures, therefore the point mass concept is only an approximation. For extended mass structures we make use of softening parameter ε whose value is taken between 0.01 and 0.05 (in the units of total radius). Following the same procedure, Eq.8 becomes as()320ln ln 13N S S N T N b Nb V εε⎡⎤-=---⎢⎥⎣⎦(13)For extended structures of galaxies, Eq.4 gets modi-fied to()()331nT R b nT R εβαεβαε--=+ (14)where α is a constant, R is the radius of a cell in a phase space in which number of particles (galaxies) is N and volume is V . The relation between b and b ε is given by: ()11b b b εαα=+- (15) b ε represents the correlation energy for extended mass particles clustering gravitationally in an expanding uni-verse. The above Eq.10 and Eq.12 take the form respec-tively as;()()3203ln 111bT b S S b b ααα⎡⎤⎢⎥-=-+⎢⎥+-+-⎢⎥⎣⎦1 (16) ()()()120113ln ln 2111b b b S S n b b ααα⎡⎤-⎡⎤⎢⎥⎣⎦-=-++⎢⎥+-+-⎢⎥⎣⎦1 (17)where2R R εεεα⎛⎫⎛⎫=⎪ ⎪⎝⎭⎝⎭(18)If ε = 0, α = 1 the entropy equations for extended mass galaxies are exactly same with that of a system of point mass galaxies approximation. Eq.10, Eq.12, Eq.16and Eq.17 are used here to study the entropy changes inthe cosmological many body problem. Various entropy change results S – S 0 for both the point mass approxima-tion and of extended mass approximation of particles (galaxies) are shown in (Figures 1and2). The resultshave been calculated analytically for different values ofFigure 1. (Color online) Comparison of isothermal entropy changes for non-point and point mass particles (galaxies) for an infinite gravitating system as a function of average relative temperature T and the parameter b . For non-point mass ε = 0.03 and R = 0.06 (left panel), ε = 0.04 and R = 0.04 (right panel).All Rights Reserved.N. Iqbal et al. / Natural Science 3 (2011) 65-68 68Figure 2. (Color online) Comparison of equi-density entropy changes for non-point and point mass particles (galaxies) for an infinite gravitating system as a function of average relative density n and the parameter b. For non-point mass ε= 0.03 and R = 0.04.R (cell size) corresponding to different values of soften-ing parameter ε. We study the variations of entropy changes S – S0with the changing parameter b for differ-ent values of n and T. Some graphical variations for S – S0with b for different values of n = 0, 1, 100 and aver-age temperature T = 1, 10 and 100 and by fixing value of cell size R = 0.04 and 0.06 are shown. The graphical analysis can be repeated for different values of R and by fixing values of εfor different sets like 0.04 and 0.05. From both the figures shown in 1 and 2, the dashed line represents variation for point mass particles and the solid line represents variation for extended (non-point mass) particles (galaxies) clustering together. It has been ob-served that the nature of the variation remains more or less same except with some minor difference.4. RESULTSThe formula for entropy calculated in this paper has provided a convenient way to study the entropy changes in gravitational galaxy clusters in an expanding universe. Gravity changes things that we have witnessed in this research. Clustering of galaxies in an expanding universe, which is like that of a self gravitating gas increases the gases volume which increases the entropy, but it also increases the potential energy and thus decreases the kinetic energy as particles must work against the attrac-tive gravitational field. So we expect expanding gases to cool down, and therefore there is a probability that the entropy has to decrease which gets confirmed from our theoretical calculations as shown in Figures 1 and 2. Entropy has remained an important contributor to our understanding in cosmology. Everything from gravita-tional clustering to supernova are contributors to entropy budget of the universe. A new calculation and study of entropy results given by Eqs.10, 12, 16 and 17 shows that the entropy of the universe decreases first with the clustering rate of the particles and then gradually in-creases as the system attains viral equilibrium. The gravitational entropy in this paper furthermore suggests that the universe is different than scientists had thought.5. ACKNOWLEDGEMENTSWe are thankful to Interuniversity centre for Astronomy and Astro-physics Pune India for providing a warm hospitality and facilities during the course of this work.REFERENCES[1]Voit, G.M. (2005) Tracing cosmic evolution with clus-ters of galaxies. Reviews of Modern Physics, 77, 207- 248.[2]Rief, F. (1965)Fundamentals of statistical and thermalphysics. McGraw-Hill, Tokyo.[3]Spitzer, L. and Saslaw, W.C. (1966) On the evolution ofgalactic nuclei. Astrophysical Journal, 143, 400-420.doi:10.1086/148523[4]Saslaw, W.C. and De Youngs, D.S. (1971) On the equi-partition in galactic nuclei and gravitating systems. As-trophysical Journal, 170, 423-429.doi:10.1086/151229[5]Itoh, M., Inagaki, S. and Saslaw, W.C. (1993) Gravita-tional clustering of galaxies. Astrophysical Journal, 403,476-496.doi:10.1086/172219[6]Hill, T.L. (1956) Statistical mechanics: Principles andstatistical applications. McGraw-Hill, New York.[7]Iqbal, N., Ahmad, F. and Khan, M.S. (2006) Gravita-tional clustering of galaxies in an expanding universe.Journal of Astronomy and Astrophysics, 27, 373-379.doi:10.1007/BF02709363[8]Saslaw, W.C. and Hamilton, A.J.S. (1984) Thermody-namics and galaxy clustering. Astrophysical Journal, 276, 13-25.doi:10.1086/161589[9]Mcquarrie, D.A. and Simon, J.D. (1997) Physical chem-istry: A molecular approach. University Science Books,Sausalito.[10]Ahmad, F, Saslaw, W.C. and Bhat, N.I. (2002) Statisticalmechanics of cosmological many body problem. Astro-physical Journal, 571, 576-584.doi:10.1086/340095[11]Freud, P.G. (1970) Physics: A Contemporary Perspective.Taylor and Francis Group.[12]Khinchin, A.I. (1949) Mathamatical Foundation of statis-tical mechanics. Dover Publications, New York.[13]Frampton, P., Stephen, D.H., Kephar, T.W. and Reeb, D.(2009) Classical Quantum Gravity. 26, 145005.doi:10.1088/0264-9381/26/14/145005All Rights Reserved.。
NetworkStructures:网络结构
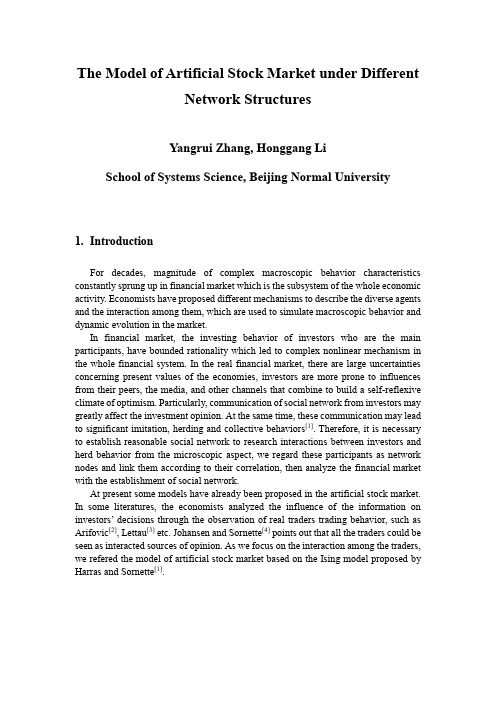
The Model of Artificial Stock Market under DifferentNetwork StructuresYangrui Zhang, Honggang LiSchool of Systems Science, Beijing Normal University1.IntroductionFor decades, magnitude of complex macroscopic behavior characteristics constantly sprung up in financial market which is the subsystem of the whole economic activity. Economists have proposed different mechanisms to describe the diverse agents and the interaction among them, which are used to simulate macroscopic behavior and dynamic evolution in the market.In financial market, the investing behavior of investors who are the main participants, have bounded rationality which led to complex nonlinear mechanism in the whole financial system. In the real financial market, there are large uncertainties concerning present values of the economies, investors are more prone to influences from their peers, the media, and other channels that combine to build a self-reflexive climate of optimism. Particularly, communication of social network from investors may greatly affect the investment opinion. At the same time, these communication may lead to significant imitation, herding and collective behaviors[1]. Therefore, it is necessary to establish reasonable social network to research interactions between investors and herd behavior from the microscopic aspect, we regard these participants as network nodes and link them according to their correlation, then analyze the financial market with the establishment of social network.At present some models have already been proposed in the artificial stock market. In some literatures, the economists analyzed the influence of the information on investors’ decisions through the o bservation of real traders trading behavior, such as Arifovic[2], Lettau[3] etc. Johansen and Sornette[4] points out that all the traders could be seen as interacted sources of opinion. As we focus on the interaction among the traders, we refered the model of artificial stock market based on the Ising model proposed by Harras and Sornette[1].Based on complex network theory and behavioral finance theory, we also take the rules of random network, scale-free network and small world network into consideration, building an evolution model according to the characteristics of the investors’ investing behavior under the network system, and studying the effect of herd behavior on the rate of return and price volatility under different network structures from a kind of macroscopic aspect.2. ModelWe consider a fixed environment composed of N agents who are trading a single asset. At each time step, agents have the possibility to either trade (buy or sell) or to remain passive. The trading decision s i (t ) of agent i is based on his opinion on the future price development. The opinion of agent i at time t , w i (t), consists of three different sources: idiosyncratic opinion, global news and their network of acquaintances.1231()(1)[()](1)()()Ji i ij i j i i i j w t c k t E s t c u t n t c t ε==-+-+∑ (1)where εi (t) represents the private information of agent i , n(t) is the public information, J is the number of neighbors that agent i polls for their opinion and E i [s j (t)] is the action of the neighbor j at time t −1, (c 1i ,c 2i ,c 3i ) is the form of the weights the agent attributes to each of the three pieces of information.Assuming that each agent is characterized by a fixed threshold w i to control the triggering s i (t ) of an investment action. An agent i decides to buy a stock if his conviction w i (t) is sufficiently positive so as to reach the threshold: w i (t)≥w i . Reversely, she decides to sell if w i (t)≤w i . Once all the agents have decided on their orders, the new price of the asset is determined by the following equations:11()()()Nii i r t s t v t N λ==⋅⋅∑ (2) log[()]log[(1)]()p t p t r t =-+ (3)here r(t) is the return and v i (t) is the volume at time t , λ represents the relative impact of the excess demand upon the price, i.e. the market depth.The agents adapt their belief concerning the credibility of the news n (t ) and their trust in the advice E i [s j (t)] of their social contacts, according to time-dependent weights u(t) and k ij (t), which take into account their recent past performance. And here, α refers to the memory discount factor.()()(1)(1)(1)r r t u t u t n t αασ=-+-- (4)()()(1)(1)[(1)]ij ij i j r r t k t k t E s t αασ=-+-- (5)3.Finding and DiscussionWe establish a kind of relation among agents by the rules of random network, scale-free network and small world network, the upcoming research mainly includes the following aspects:1.Analyze and compare the evolution of the log-price log [p(t)], the one-time stepreturn r(t), the prediction performance of the news, u(t)and the ensemble average of the prediction performance of the neighbors, k ij(t), with the change of time, under the three different network structures.paring the market volatility with the existence of herd behavior or not in themarket under different network structures. Predictably, the higher transmission sensitivity investors hold, the greater volatility price will be. Furthermore, we adjust the network scale to observe whether the former volatility have a change.3.Analyzing how different parameters of network topology impact on macro marketbehavior, and we focus on the time series characteristics of r(t)to observe if they are consistent with empirical observation and whether volatility clustering, bubbles and crashes these phenomena emerge in the market model. Finally, exploring possible economic mechanism according to the above results.References[1]Harras, G., Sornette, D. (2011) How to grow a bubble: A model of myopic adapting agents,Journal of Economic Behavior & Organization.[2]The Behavior of the Exchange Rate in the Genetic Algorithm and Experimental Economies Jasmina Arifovic Journal of Political Economy V ol. 104, No. 3 (Jun., 1996) , pp. 510-541.[3]Lettau M.Explaining the facts with adaptive agents: the case of mutual fund flows. Journal of Econometrics . 1997.[4]Zhou, W.-X., Sornette, D., 2007. Self-fulfilling ising model of financial markets. European Physical Journal B 55, 175–181.。
Modularity and community structure in networks
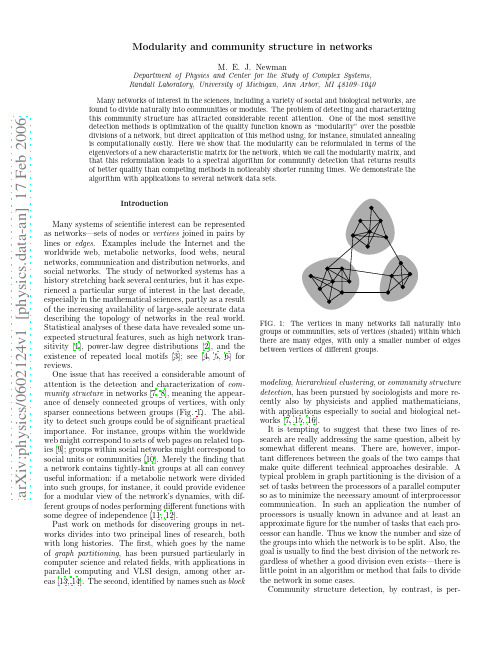
a r X i v :p h y s i c s /0602124v 1 [p h y s i c s .d a t a -a n ] 17 F eb 2006Modularity and community structure in networksM. E.J.NewmanDepartment of Physics and Center for the Study of Complex Systems,Randall Laboratory,University of Michigan,Ann Arbor,MI 48109–1040Many networks of interest in the sciences,including a variety of social and biological networks,are found to divide naturally into communities or modules.The problem of detecting and characterizing this community structure has attracted considerable recent attention.One of the most sensitive detection methods is optimization of the quality function known as “modularity”over the possible divisions of a network,but direct application of this method using,for instance,simulated annealing is computationally costly.Here we show that the modularity can be reformulated in terms of the eigenvectors of a new characteristic matrix for the network,which we call the modularity matrix,and that this reformulation leads to a spectral algorithm for community detection that returns results of better quality than competing methods in noticeably shorter running times.We demonstrate the algorithm with applications to several network data sets.IntroductionMany systems of scientific interest can be represented as networks—sets of nodes or vertices joined in pairs by lines or edges .Examples include the Internet and the worldwide web,metabolic networks,food webs,neural networks,communication and distribution networks,and social networks.The study of networked systems has a history stretching back several centuries,but it has expe-rienced a particular surge of interest in the last decade,especially in the mathematical sciences,partly as a result of the increasing availability of large-scale accurate data describing the topology of networks in the real world.Statistical analyses of these data have revealed some un-expected structural features,such as high network tran-sitivity [1],power-law degree distributions [2],and the existence of repeated local motifs [3];see [4,5,6]for reviews.One issue that has received a considerable amount of attention is the detection and characterization of com-munity structure in networks [7,8],meaning the appear-ance of densely connected groups of vertices,with only sparser connections between groups (Fig.1).The abil-ity to detect such groups could be of significant practical importance.For instance,groups within the worldwide web might correspond to sets of web pages on related top-ics [9];groups within social networks might correspond to social units or communities [10].Merely the finding that a network contains tightly-knit groups at all can convey useful information:if a metabolic network were divided into such groups,for instance,it could provide evidence for a modular view of the network’s dynamics,with dif-ferent groups of nodes performing different functions with some degree of independence [11,12].Past work on methods for discovering groups in net-works divides into two principal lines of research,both with long histories.The first,which goes by the name of graph partitioning ,has been pursued particularly in computer science and related fields,with applications in parallel computing and VLSI design,among other ar-eas [13,14].The second,identified by names such as blockFIG.1:The vertices in many networks fall naturally into groups or communities,sets of vertices (shaded)within which there are many edges,with only a smaller number of edges between vertices of different groups.modeling ,hierarchical clustering ,or community structure detection ,has been pursued by sociologists and more re-cently also by physicists and applied mathematicians,with applications especially to social and biological net-works [7,15,16].It is tempting to suggest that these two lines of re-search are really addressing the same question,albeit by somewhat different means.There are,however,impor-tant differences between the goals of the two camps that make quite different technical approaches desirable.A typical problem in graph partitioning is the division of a set of tasks between the processors of a parallel computer so as to minimize the necessary amount of interprocessor communication.In such an application the number of processors is usually known in advance and at least an approximate figure for the number of tasks that each pro-cessor can handle.Thus we know the number and size of the groups into which the network is to be split.Also,the goal is usually to find the best division of the network re-gardless of whether a good division even exists—there is little point in an algorithm or method that fails to divide the network in some cases.Community structure detection,by contrast,is per-2haps best thought of as a data analysis technique used to shed light on the structure of large-scale network datasets,such as social networks,Internet and web data, or biochemical munity structure meth-ods normally assume that the network of interest divides naturally into subgroups and the experimenter’s job is to find those groups.The number and size of the groups is thus determined by the network itself and not by the experimenter.Moreover,community structure methods may explicitly admit the possibility that no good division of the network exists,an outcome that is itself considered to be of interest for the light it sheds on the topology of the network.In this paper our focus is on community structure de-tection in network datasets representing real-world sys-tems of interest.However,both the similarities and differences between community structure methods and graph partitioning will motivate many of the develop-ments that follow.The method of optimal modularity Suppose then that we are given,or discover,the struc-ture of some network and that we wish to determine whether there exists any natural division of its vertices into nonoverlapping groups or communities,where these communities may be of any size.Let us approach this question in stages and focus ini-tially on the problem of whether any good division of the network exists into just two communities.Perhaps the most obvious way to tackle this problem is to look for divisions of the vertices into two groups so as to mini-mize the number of edges running between the groups. This“minimum cut”approach is the approach adopted, virtually without exception,in the algorithms studied in the graph partitioning literature.However,as discussed above,the community structure problem differs crucially from graph partitioning in that the sizes of the commu-nities are not normally known in advance.If community sizes are unconstrained then we are,for instance,at lib-erty to select the trivial division of the network that puts all the vertices in one of our two groups and none in the other,which guarantees we will have zero intergroup edges.This division is,in a sense,optimal,but clearly it does not tell us anything of any worth.We can,if we wish,artificially forbid this solution,but then a division that puts just one vertex in one group and the rest in the other will often be optimal,and so forth.The problem is that simply counting edges is not a good way to quantify the intuitive concept of commu-nity structure.A good division of a network into com-munities is not merely one in which there are few edges between communities;it is one in which there are fewer than expected edges between communities.If the num-ber of edges between two groups is only what one would expect on the basis of random chance,then few thought-ful observers would claim this constitutes evidence of meaningful community structure.On the other hand,if the number of edges between groups is significantly less than we expect by chance—or equivalently if the number within groups is significantly more—then it is reasonable to conclude that something interesting is going on. This idea,that true community structure in a network corresponds to a statistically surprising arrangement of edges,can be quantified using the measure known as modularity[17].The modularity is,up to a multiplicative constant,the number of edges falling within groups mi-nus the expected number in an equivalent network with edges placed at random.(A precise mathematical formu-lation is given below.)The modularity can be either positive or negative,with positive values indicating the possible presence of com-munity structure.Thus,one can search for community structure precisely by looking for the divisions of a net-work that have positive,and preferably large,values of the modularity[18].The evidence so far suggests that this is a highly effective way to tackle the problem.For instance, Guimer`a and Amaral[12]and later Danon et al.[8]op-timized modularity over possible partitions of computer-generated test networks using simulated annealing.In di-rect comparisons using standard measures,Danon et al. found that this method outperformed all other methods for community detection of which they were aware,in most cases by an impressive margin.On the basis of con-siderations such as these we consider maximization of the modularity to be perhaps the definitive current method of community detection,being at the same time based on sensible statistical principles and highly effective in practice.Unfortunately,optimization by simulated annealing is not a workable approach for the large network problems facing today’s scientists,because it demands too much computational effort.A number of alternative heuris-tic methods have been investigated,such as greedy algo-rithms[18]and extremal optimization[19].Here we take a different approach based on a reformulation of the mod-ularity in terms of the spectral properties of the network of interest.Suppose our network contains n vertices.For a par-ticular division of the network into two groups let s i=1 if vertex i belongs to group1and s i=−1if it belongs to group2.And let the number of edges between ver-tices i and j be A ij,which will normally be0or1,al-though larger values are possible in networks where mul-tiple edges are allowed.(The quantities A ij are the el-ements of the so-called adjacency matrix.)At the same time,the expected number of edges between vertices i and j if edges are placed at random is k i k j/2m,where k i and k j are the degrees of the vertices and m=14m ijA ij−k i k j4m s T Bs,(1)where s is the vector whose elements are the s i.The leading factor of1/4m is merely conventional:it is in-cluded for compatibility with the previous definition of modularity[17].We have here defined a new real symmetric matrix B with elementsk i k jB ij=A ij−FIG.2:Application of our eigenvector-based method to the “karate club”network of Ref.[23].Shapes of vertices indi-cate the membership of the corresponding individuals in the two known factions of the network while the dotted line indi-cates the split found by the algorithm,which matches the fac-tions exactly.The shades of the vertices indicate the strength of their membership,as measured by the value of the corre-sponding element of the eigenvector.groups,but to place them on a continuous scale of“how much”they belong to one group or the other.As an example of this algorithm we show in Fig.2the result of its application to a famous network from the so-cial science literature,which has become something of a standard test for community detection algorithms.The network is the“karate club”network of Zachary[23], which shows the pattern of friendships between the mem-bers of a karate club at a US university in the1970s. This example is of particular interest because,shortly after the observation and construction of the network, the club in question split in two as a result of an inter-nal dispute.Applying our eigenvector-based algorithm to the network,wefind the division indicated by the dotted line in thefigure,which coincides exactly with the known division of the club in real life.The vertices in Fig.2are shaded according to the val-ues of the elements in the leading eigenvector of the mod-ularity matrix,and these values seem also to accord well with known social structure within the club.In partic-ular,the three vertices with the heaviest weights,either positive or negative(black and white vertices in thefig-ure),correspond to the known ringleaders of the two fac-tions.Dividing networks into more than two communities In the preceding section we have given a simple matrix-based method forfinding a good division of a network into two parts.Many networks,however,contain more than two communities,so we would like to extend our method tofind good divisions of networks into larger numbers of parts.The standard approach to this prob-lem,and the one adopted here,is repeated division into two:we use the algorithm of the previous sectionfirst to divide the network into two parts,then divide those parts,and so forth.In doing this it is crucial to note that it is not correct, afterfirst dividing a network in two,to simply delete the edges falling between the two parts and then apply the algorithm again to each subgraph.This is because the degrees appearing in the definition,Eq.(1),of the mod-ularity will change if edges are deleted,and any subse-quent maximization of modularity would thus maximize the wrong quantity.Instead,the correct approach is to define for each subgraph g a new n g×n g modularity matrix B(g),where n g is the number of vertices in the subgraph.The correct definition of the element of this matrix for vertices i,j isB(g)ij=A ij−k i k j2m ,(4)where k(g)i is the degree of vertex i within subgraph g and d g is the sum of the(total)degrees k i of the vertices in the subgraph.Then the subgraph modularity Q g=s T B(g)s correctly gives the additional contribution to the total modularity made by the division of this subgraph.In particular,note that if the subgraph is undivided,Q g is correctly zero.Note also that for a complete network Eq.(4)reduces to the previous definition for the modu-larity matrix,Eq.(2),since k(g)i→k i and d g→2m in that case.In repeatedly subdividing our network,an important question we need to address is at what point to halt the subdivision process.A nice feature of our method is that it provides a clear answer to this question:if there exists no division of a subgraph that will increase the modular-ity of the network,or equivalently that gives a positive value for Q g,then there is nothing to be gained by divid-ing the subgraph and it should be left alone;it is indi-visible in the sense of the previous section.This happens when there are no positive eigenvalues to the matrix B(g), and thus our leading eigenvalue provides a simple check for the termination of the subdivision process:if the lead-ing eigenvalue is zero,which is the smallest value it can take,then the subgraph is indivisible.Note,however,that while the absence of positive eigen-values is a sufficient condition for indivisibility,it is not a necessary one.In particular,if there are only small positive eigenvalues and large negative ones,the terms in Eq.(3)for negativeβi may outweigh those for positive.It is straightforward to guard against this possibility,how-ever:we simply calculate the modularity contribution for each proposed split directly and confirm that it is greater than zero.Thus our algorithm is as follows.We construct the modularity matrix for our network andfind its leading (most positive)eigenvalue and eigenvector.We divide the network into two parts according to the signs of the elements of this vector,and then repeat for each of the parts.If at any stage wefind that the proposed split makes a zero or negative contribution to the total mod-5ularity,we leave the corresponding subgraph undivided. When the entire network has been decomposed into in-divisible subgraphs in this way,the algorithm ends. One immediate corollary of this approach is that all “communities”in the network are,by definition,indi-visible subgraphs.A number of authors have in the past proposed formal definitions of what a community is[9,16,24].The present method provides an alter-native,first-principles definition of a community as an indivisible subgraph.Further techniques for modularity maximization In this section we describe briefly another method we have investigated for dividing networks in two by mod-ularity optimization,which is entirely different from our spectral method.Although not of especial interest on its own,this second method is,as we will shortly show,very effective when combined with the spectral method.Let us start with some initial division of our vertices into two groups:the most obvious choice is simply to place all vertices in one of the groups and no vertices in the other.Then we proceed as follows.Wefind among the vertices the one that,when moved to the other group, will give the biggest increase in the modularity of the complete network,or the smallest decrease if no increase is possible.We make such moves repeatedly,with the constraint that each vertex is moved only once.When all n vertices have been moved,we search the set of in-termediate states occupied by the network during the operation of the algorithm tofind the state that has the greatest modularity.Starting again from this state,we repeat the entire process iteratively until no further im-provement in the modularity results.Those familiar with the literature on graph partitioning mayfind this algo-rithm reminiscent of the Kernighan–Lin algorithm[25], and indeed the Kernighan–Lin algorithm provided the inspiration for our method.Despite its simplicity,wefind that this method works moderately well.It is not competitive with the best pre-vious methods,but it gives respectable modularity val-ues in the trial applications we have made.However, the method really comes into its own when it is used in combination with the spectral method introduced ear-lier.It is a common approach in standard graph par-titioning problems to use spectral partitioning based on the graph Laplacian to give an initial broad division of a network into two parts,and then refine that division us-ing the Kernighan–Lin algorithm.For community struc-ture problems wefind that the equivalent joint strategy works very well.Our spectral approach based on the leading eigenvector of the modularity matrix gives an ex-cellent guide to the general form that the communities should take and this general form can then befine-tuned by our vertex moving method,to reach the best possible modularity value.The whole procedure is repeated to subdivide the network until every remaining subgraph is indivisible,and no further improvement in the modular-ity is possible.Typically,thefine-tuning stages of the algorithm add only a few percent to thefinal value of the modularity, but those few percent are enough to make the difference between a method that is merely good and one that is, as we will see,exceptional.Example applicationsIn practice,the algorithm developed here gives excel-lent results.For a quantitative comparison between our algorithm and others we follow Duch and Arenas[19] and compare values of the modularity for a variety of networks drawn from the literature.Results are shown in Table I for six different networks—the exact same six as used by Duch and Arenas.We compare mod-ularityfigures against three previously published algo-rithms:the betweenness-based algorithm of Girvan and Newman[10],which is widely used and has been incor-porated into some of the more popular network analysis programs(denoted GN in the table);the fast algorithm of Clauset et al.[26](CNM),which optimizes modularity using a greedy algorithm;and the extremal optimization algorithm of Duch and Arenas[19](DA),which is ar-guably the best previously existing method,by standard measures,if one discounts methods impractical for large networks,such as exhaustive enumeration of all parti-tions or simulated annealing.The table reveals some interesting patterns.Our al-gorithm clearly outperforms the methods of Girvan and Newman and of Clauset et al.for all the networks in the task of optimizing the modularity.The extremal opti-mization method on the other hand is more competitive. For the smaller networks,up to around a thousand ver-tices,there is essentially no difference in performance be-tween our method and extremal optimization;the mod-ularity values for the divisions found by the two algo-rithms differ by no more than a few parts in a thousand for any given network.For larger networks,however,our algorithm does better than extremal optimization,and furthermore the gap widens as network size increases, to a maximum modularity difference of about a6%for the largest network studied.For the very large networks that have been of particular interest in the last few years, therefore,it appears that our method for detecting com-munity structure may be the most effective of the meth-ods considered here.The modularity values given in Table I provide a use-ful quantitative measure of the success of our algorithm when applied to real-world problems.It is worthwhile, however,also to confirm that it returns sensible divisions of networks in practice.We have given one example demonstrating such a division in Fig.2.We have also checked our method against many of the example net-works used in previous studies[10,17].Here we give two more examples,both involving network representationsmodularity Q network GN CNM DA this paper3419845311331068027519maximal value of the quantity known as modularity over possible divisions of a network.We have shown that this problem can be rewritten in terms of the eigenval-ues and eigenvectors of a matrix we call the modularity matrix,and by exploiting this transformation we have created a new computer algorithm for community de-tection that demonstrably outperforms the best previ-ous general-purpose algorithms in terms of both quality of results and speed of execution.We have applied our algorithm to a variety of real-world network data sets, including social and biological examples,showing it to give both intuitively reasonable divisions of networks and quantitatively better results as measured by the modu-larity.AcknowledgmentsThe author would like to thank Lada Adamic,Alex Arenas,and Valdis Krebs for providing network data and for useful comments and suggestions.This work was funded in part by the National Science Foundation un-der grant number DMS–0234188and by the James S. McDonnell Foundation.[1]D.J.Watts and S.H.Strogatz,Collective dynamics of‘small-world’networks.Nature393,440–442(1998). [2]A.-L.Barab´a si and R.Albert,Emergence of scaling inrandom networks.Science286,509–512(1999).[3]o,S.Shen-Orr,S.Itzkovitz,N.Kashtan,D.Chklovskii,and U.Alon,Network motifs:Simplebuilding blocks of complex networks.Science298,824–827(2002).[4]R.Albert and A.-L.Barab´a si,Statistical mechanics ofcomplex networks.Rev.Mod.Phys.74,47–97(2002).[5]S.N.Dorogovtsev and J.F.F.Mendes,Evolution ofnetworks.Advances in Physics51,1079–1187(2002). [6]M.E.J.Newman,The structure and function of complexnetworks.SIAM Review45,167–256(2003).[7]M.E.J.Newman,Detecting community structure in net-works.Eur.Phys.J.B38,321–330(2004).[8]L.Danon,J.Duch, A.Diaz-Guilera,and A.Arenas,Comparing community structure identification.J.Stat.Mech.p.P09008(2005).[9]G.W.Flake,wrence,C.L.Giles,and F.M.Co-etzee,Self-organization and identification of Web com-munities.IEEE Computer35,66–71(2002).[10]M.Girvan and M.E.J.Newman,Community structurein social and biological networks.Proc.Natl.Acad.Sci.USA99,7821–7826(2002).[11]P.Holme,M.Huss,and H.Jeong,Subnetwork hierar-chies of biochemical pathways.Bioinformatics19,532–538(2003).[12]R.Guimer`a and L.A.N.Amaral,Functional cartogra-phy of complex metabolic networks.Nature433,895–900 (2005).[13]U.Elsner,Graph partitioning—a survey.Technical Re-port97-27,Technische Universit¨a t Chemnitz(1997). [14]P.-O.Fj¨a llstr¨o m,Algorithms for graph partitioning:Asurvey.Link¨o ping Electronic Articles in Computer and Information Science3(10)(1998).[15]H.C.White,S.A.Boorman,and R.L.Breiger,Socialstructure from multiple networks:I.Blockmodels of roles and positions.Am.J.Sociol.81,730–779(1976). [16]S.Wasserman and K.Faust,Social Network Analysis.Cambridge University Press,Cambridge(1994).[17]M.E.J.Newman and M.Girvan,Finding and evaluat-ing community structure in networks.Phys.Rev.E69, 026113(2004).[18]M.E.J.Newman,Fast algorithm for detecting com-munity structure in networks.Phys.Rev.E69,066133 (2004).[19]J.Duch and A.Arenas,Community detection in complexnetworks using extremal optimization.Phys.Rev.E72, 027104(2005).[20]F.R.K.Chung,Spectral Graph Theory.Number92in CBMS Regional Conference Series in Mathematics, American Mathematical Society,Providence,RI(1997).[21]M.Fiedler,Algebraic connectivity of graphs.Czech.Math.J.23,298–305(1973).[22]A.Pothen,H.Simon,and K.-P.Liou,Partitioning sparsematrices with eigenvectors of graphs.SIAM J.Matrix Anal.Appl.11,430–452(1990).[23]W.W.Zachary,An informationflow model for conflictandfission in small groups.Journal of Anthropological Research33,452–473(1977).[24]F.Radicchi,C.Castellano,F.Cecconi,V.Loreto,andD.Parisi,Defining and identifying communities in net-A101,2658–2663 (2004).[25]B.W.Kernighan and S.Lin,An efficient heuristic proce-dure for partitioning graphs.Bell System Technical Jour-nal49,291–307(1970).[26]A.Clauset,M.E.J.Newman,and C.Moore,Findingcommunity structure in very large networks.Phys.Rev.E70,066111(2004).[27]P.Gleiser and L.Danon,Community structure in jazz.Advances in Complex Systems6,565–573(2003). [28]H.Jeong,B.Tombor,R.Albert,Z.N.Oltvai,and A.-L.Barab´a si,The large-scale organization of metabolic networks.Nature407,651–654(2000).[29]H.Ebel,L.-I.Mielsch,and S.Bornholdt,Scale-free topol-ogy of e-mail networks.Phys.Rev.E66,035103(2002).[30]X.Guardiola,R.Guimer`a,A.Arenas,A.Diaz-Guilera,D.Streib,and L. A.N.Amaral,Macro-and micro-structure of trust networks.Preprint cond-mat/0206240 (2002).[31]M.E.J.Newman,The structure of scientific collabora-tion A98,404–409 (2001).[32]L.A.Adamic and N.Glance,The political blogosphereand the2004us election.In Proceedings of the WWW-2005Workshop on the Weblogging Ecosystem(2005).。
计算机网络原理习题讲解
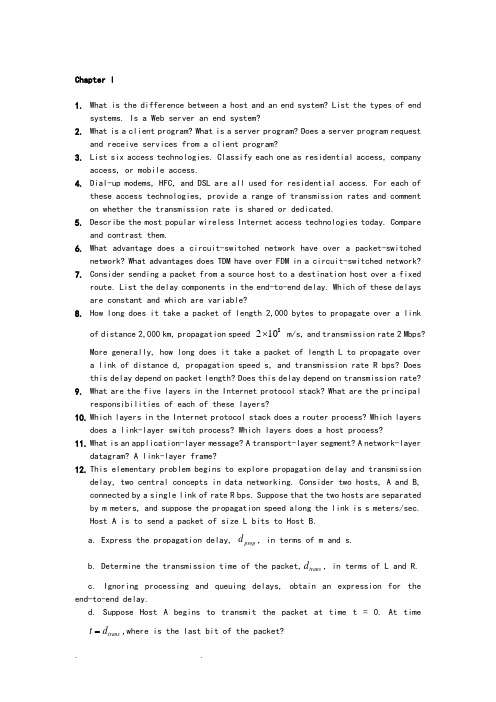
Chapter I1. What is the difference between a host and an end system? List the types of endsystems. Is a Web server an end system?2. What is a client program? What is a server program? Does a server program requestand receive services from a client program?3. List six access technologies. Classify each one as residential access, companyaccess, or mobile access.4. Dial-up modems, HFC, and DSL are all used for residential access. For each ofthese access technologies, provide a range of transmission rates and comment on whether the transmission rate is shared or dedicated.5. Describe the most popular wireless Internet access technologies today. Compareand contrast them.6. What advantage does a circuit-switched network have over a packet-switchednetwork? What advantages does TDM have over FDM in a circuit-switched network?7. Consider sending a packet from a source host to a destination host over a fixedroute. List the delay components in the end-to-end delay. Which of these delays are constant and which are variable?8. How long does it take a packet of length 2,000 bytes to propagate over a linkof distance 2,000 km, propagation speed 8102⨯ m/s, and transmission rate 2 Mbps? More generally, how long does it take a packet of length L to propagate over a link of distance d, propagation speed s, and transmission rate R bps? Does this delay depend on packet length? Does this delay depend on transmission rate?9. What are the five layers in the Internet protocol stack? What are the principalresponsibilities of each of these layers?10. Which layers in the Internet protocol stack does a router process? Which layersdoes a link-layer switch process? Which layers does a host process?11. What is an application-layer message? A transport-layer segment? A network-layerdatagram? A link-layer frame?12. This elementary problem begins to explore propagation delay and transmissiondelay, two central concepts in data networking. Consider two hosts, A and B, connected by a single link of rate R bps. Suppose that the two hosts are separated by m meters, and suppose the propagation speed along the link is s meters/sec. Host A is to send a packet of size L bits to Host B.a. Express the propagation delay, prop d , in terms of m and s.b. Determine the transmission time of the packet,trans d , in terms of L and R.c. Ignoring processing and queuing delays, obtain an expression for the end-to-end delay.d. Suppose Host A begins to transmit the packet at time t = 0. At time trans d t =,where is the last bit of the packet?e. Suppose prop d is greater than trans d . At time t = trans d ,where is the first bit of the packet?f. Suppose prop d is less than trans d . At time t = trans d , where is the first bit of the packet?g. Suppose 8105.2⨯=s , L = 100bits, and R = 28 kbps. Find the distance m so that prop d equals trans d .13. In modern packet-switched networks, the source host segments long,application-layer messages (for example, an image or a music file) into smaller packets and sends the packets into the network. The receiver then reassembles the packets back into the original message. We refer to this process as message segmentation. Figure 1.24 illustrates the end-to-end transport of a message with and without message segmentation. Consider a message that is 6108⨯ bits long that is to be sent from source to destination in Figure 1.24. Suppose each link in the figure is 2 Mbps. Ignore propagation, queuing, and processing delays.a. Consider sending the message from source to destination without message segmentation. How long does it take to move the message from the source host to the first packet switch? Keeping in mind that each switch uses store-and-forward packet switching, what is the total time to move the message from source host to destination host?b. Now suppose that the message is segmented into 4,000 packets, with each packet being 2,000 bits long. How long does it take to move the first packet from source host to the first switch? When the first packet is being sent from the first switch to the second switch, the second packet is being sent from the source host to the first switch. At what time will the second packet be fully received at the first switch?c. How long does it take to move the file from source host to destination hostwhen message segmentation is used? Compare this result with your answer in part(a) and comment.d. Discuss the drawbacks of message segmentation.14.下列说法中,正确的是( )。
中国历史人物英语作文

When delving into the annals of Chinese history,one is bound to encounter a plethora of influential figures who have shaped the course of the nations past.Writing an English essay on Chinese historical figures provides an opportunity to explore their lives, achievements,and the impact they have had on Chinese civilization.Here are some key elements to consider when crafting such an essay:1.Introduction to the Historical Context:Begin your essay by setting the stage for the period in which your chosen figure lived.This provides a backdrop that helps readers understand the circumstances that influenced the persons actions and decisions.2.Biographical Information:Provide a brief overview of the individuals life,including their birth,early years,and the environment in which they were raised.This can help to humanize the figure and provide context for their later actions.3.Major Achievements and Contributions:Discuss the key accomplishments of the historical figure.This could include military victories,political reforms,scientific discoveries,or cultural contributions.Be sure to explain why these achievements were significant and how they affected China and the world.4.Personality and Character Traits:Analyze the personality of the figure.Were they known for their wisdom,bravery,or perhaps their ruthlessness?How did their character traits contribute to their success or failure?5.Challenges and Obstacles:Every historical figure faced challenges.Describe the obstacles they overcame and how they dealt with adversity.This can provide insight into their resilience and determination.6.Legacy and Impact:Reflect on the lasting impact of the figures life and work.How have their contributions influenced subsequent generations?What lessons can be learned from their life story?7.Controversies and Criticisms:If applicable,address any controversies or criticisms associated with the figure.This can provide a more balanced view and encourage critical thinking about the persons actions and decisions.8.Conclusion:Summarize the key points of your essay and reiterate the significance of the historical figure.You might also consider ending with a reflection on the relevance of their story to the present day.9.Citations and References:Ensure that you cite your sources properly to lend credibilityto your essay.This is especially important when discussing historical figures,as there can be varying interpretations of their lives and actions.nguage and Style:Use clear,concise language and maintain a formal tone throughout your essay.Avoid using overly colloquial expressions or slang,and ensure that your grammar and punctuation are correct.By incorporating these elements into your essay,you can create a compelling and informative piece that provides readers with a deeper understanding of a Chinese historical figure and their place in history.。
基于复杂网络理论的地铁网络鲁棒性研究

基于复杂网络理论的地铁网络鲁棒性研究作者:时柏营程远丁东玥杨宇雷崔博伟来源:《物流科技》2024年第14期摘要:地鐵网络作为现代城市交通的重要组成部分,其运行的可靠性和稳定性对于城市的正常运转至关重要。
然而,地铁网络可能面临各种干扰和故障,如设备故障、自然灾害、人为破坏等,可能导致线路中断、列车延误和乘客服务中断。
因此,研究地铁网络的鲁棒性,即系统在面对这些干扰时的恢复能力,对于提高地铁网络的可靠性和抗干扰性具有重要意义。
文章基于复杂网络理论,综合考虑地铁网络的拓扑结构、节点重要性和客流分布等因素,对地铁网络的鲁棒性进行定量分析。
研究采用Space-L方法对杭州市地铁网络拓扑结构特性进行分析,并分析了网络的度、介数、聚类系数和最短路径长度等网络特性指标。
针对鲁棒性分析,文章采用了随机攻击和蓄意攻击的9种不同攻击策略,并对杭州市地铁网络进行实例分析。
研究结果表明,关键指标的变化对地铁网络的鲁棒性产生显著影响。
通过分析不同攻击策略下的网络性能指标,可以揭示系统中的脆弱节点和脆弱路径。
这些分析结果对于提高杭州市地铁网络的鲁棒性,增强其对干扰和攻击的抵抗能力具有重要意义。
关键词:Space-L方法;复杂网络;鲁棒性;聚类系数;介数中图分类号:F532;U231 文献标志码:A DOI:10.13714/ki.1002-3100.2024.14.012文章编号:1002-3100(2024)14-0059-05Robustness Analysis of Subway Network Based on Complex Network TheorySHI Baiying,CHENG Yuan,DING Dongyue,YANG Yulei,CUI Bowei(Department of Transportation Engineering, Shandong Jianzhu University, Jinan 250101,China)Abstract: As an important part of modern urban transportation, the reliability and stability of the metro network is crucial for the normal functioning of the city. However, metro networks may face a variety of disturbances and failures, such as equipment failures, natural disasters, and human damages, which may lead to line interruptions, train delays, and disruptions in passenger services. Therefore, it is important to study the robustness of metro networks, i.e. the ability of the system to recover in the face of these disturbances, to improve the reliability and anti-interference of metro networks. The paper quantitatively analyzes the robustness of the subway network based on complex network theory, taking into account the topology of the subway network, the importance of the nodes, and the distribution of passenger flow. This study uses the Space-L method to construct a passenger flow-weighted North Hangzhou metro network model, and analyzes the network characteristic indexes such as the degree, median, clustering coefficient, and shortest path length of the network. For robustness analysis, the article adopts nine different attack strategies of random attack and deliberate attack, and takes a case study of Hangzhou metro network . The results of the study show that the changes of the key indicators have a significant impact on therobustness of the subway network. By analyzing the network performance metrics under different attack strategies, vulnerable nodes and vulnerable paths in the system can be revealed. These analysis results are important for improving the robustness of Hangzhou metro network and enhancing its resistance to interference and attacks.Key words: Space-L method; complex networks; robustness; clustering coefficient; median0 引言地铁网络作为城市交通系统的核心组成部分,其可靠性和稳定性对于城市居民的出行和城市的正常运转至关重要。
bioviamaterialsstudio
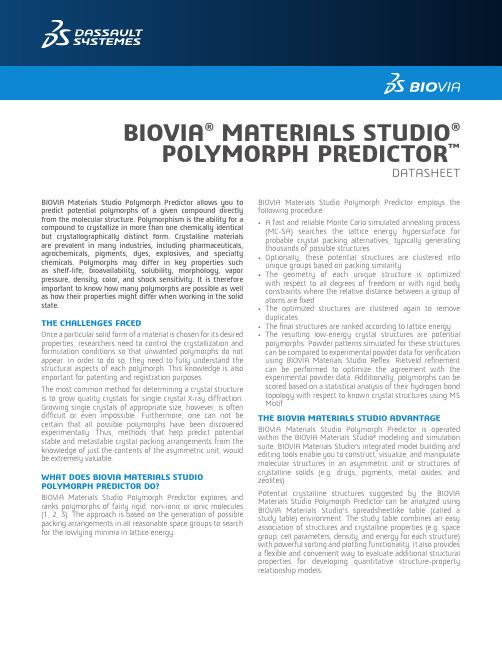
BIOVIA Materials Studio Po lymo rph Predicto r allo ws yo u to predict po tential po lymo rphs o f a given co mpo und directly from the molecular structure. Polymorphism is the ability for a compound to crystallize in more than one chemically identical but crystallo graphically distinct fo rm. Crystalline materials are prevalent in many industries, including pharmaceuticals, agro chemicals, pigments, dyes, explo sives, and specialty chemicals. Po lymo rphs may differ in key pro perties such as shelf-life, bio availability, so lubility, mo rpho lo gy, vapo r pressure, density, co lo r, and sho ck sensitivity. It is therefo re important to know how many polymorphs are possible as well as how their properties might differ when working in the solid state.THE CHALLENGES FACEDOnce a particular solid form of a material is chosen for its desired properties, researchers need to control the crystallization and formulation conditions so that unwanted polymorphs do not appear. In order to do so, they need to fully understand the structural aspects of each polymorph. This knowledge is also important for patenting and registration purposes.The most common method for determining a crystal structure is to grow quality crystals for single crystal X-ray diffraction. Growing single crystals of appropriate size, however, is often difficult or even impossible. Furthermore, one can not be certain that all possible polymorphs have been discovered experimentally. Thus, methods that help predict potential stable and metastable crystal packing arrangements from the knowledge of just the contents of the asymmetric unit, would be extremely valuable.WHAT DOES BIOVIA MATERIALS STUDIO POLYMORPH PREDICTOR DO?BIO VIA Materials Studio Polymorph Predictor explores and ranks polymorphs of fairly rigid, non-ionic or ionic molecules (1, 2, 3). The approach is based on the generation of possible packing arrangements in all reasonable space groups to search for the lowlying minima in lattice energy.BIO VIA Materials Studio Polymorph Predictor employs the following procedure:• A fast and reliable Monte Carlo simulated annealing process (MC-SA) searches the lattice energy hypersurface for probable crystal packing alternatives, typically generating thousands of possible structures• O ptionally, these potential structures are clustered into unique groups based on packing similarity• The geometry of each unique structure is optimized with respect to all degrees of freedom or with rigid body constraints where the relative distance between a group of atoms are fixed• The optimized structures are clustered again to remove duplicates• The final structures are ranked according to lattice energy • The resulting low-energy crystal structures are potential polymorphs. Powder patterns simulated for these structures can be compared to experimental powder data for verification using BIO VIA Materials Studio Reflex. Rietveld refinement can be performed to optimize the agreement with the experimental powder data. Additionally, polymorphs can be scored based on a statistical analysis of their hydrogen bond topology with respect to known crystal structures using MS Motif.THE BIOVIA MATERIALS STUDIO ADVANTAGEBIO VIA Materials Studio Polymorph Predictor is operated within the BIOVIA Materials Studio® modeling and simulation suite. BIOVIA Materials Studio’s integrated model building and editing tools enable you to construct, visualize, and manipulate molecular structures in an asymmetric unit or structures of crystalline solids (e.g. drugs, pigments, metal oxides, and zeolites).Potential crystalline structures suggested by the BIO VIA Materials Studio Polymorph Predictor can be analyzed using BIO VIA Materials Studio’s spreadsheetlike table (called a study table) environment. The study table combines an easy association of structures and crystalline properties (e.g. space group, cell parameters, density, and energy for each structure) with powerful sorting and plotting functionality. It also provides a flexible and convenient way to evaluate additional structural properties for developing quantitative structure-property relationship models.BIOVIA® MATERIALS STUDIO®POLYMORPH PREDICTOR™DATASHEETThe potential crystalline structures can further be optimized using molecular mechanics tools (BIO VIA Materials Studio Forcite and BIO VIA Materials Studio Compass), or quantum mechanics tools (BIO VIA Materials Studio DMol3 or BIO VIA Materials Studio CASTEP).HOW DOES BIOVIA MATERIALS STUDIO POLYMORPH PREDICTOR WORK?The goal of BIO VIA Materials Studio Polymorph Predictor is to search for lowlying minima of a high-dimensional potential energy surfaces representing all possible packing arrangements of molecules in a crystalline environment as a function of space groups, lattice parameters, and contents of an asymmetric unit.HOW DOES BIOVIA MATERIALS STUDIO POLYMORPH PREDICTOR BENEFIT YOU? Polymorphism has been recognized as a phenomenon that many industries are increasingly trying to control and exploit. The appearance of an undesirable polymorph late in product development can lead to costly delays. In order to control polymorphism, researchers need to understand how the crystal structures of each polymorph differ. Knowledge of polymorphic forms is also important for patenting and registration purposes. BIO VIA Materials Studio Polymorph Predictor searches for all possible packing arrangements of crystalline materials from their molecular structures. O nce the different crystal structures are known, additional analyses can be performed to help explain other characteristics unique to each polymorph, including analysis of surface chemistry for different facets. Researchers can define parameters for each step with an easy-touse graphical user interface. The required input is the molecular structures of the contents of an asymmetric unit. The starting conformations of these molecules can be imported from an existing crystal structure or created using 3D Sketcher tools in Materials Visualizer and conformational analysis.BIO VIA Materials Studio Polymorph Predictor can be utilized in two ways:1. When experimental powder data is available, it may be used to aid the identification of the correct crystal structure from the list of generated trial structures using the Powder Comparison feature and to refine the structural parameters using the Rietveld method in BIOVIA Materials Studio Reflex.2. Ab initio prediction of polymorphs when experimental powder data is not available.BIOVIA Materials Studio Polymorph Predictor can be used alone or as the first step in a sophisticated structural analysis with other modules in BIO VIA Materials Studio. BIO VIA Materials Studio Visualizer can be used to examine the packing accelrys. com/materials-studio arrangement and hydrogen bonding for each structure. In order to control crystal shape and growth, the surface chemistry can also be analyzed. Furthermore, if medium- to high quality experimental powder data is available, BIO VIA Materials Studio Reflex Plus, better suited to analyze salts, solvates, and more flexible compounds, can be used to determine the structure directly from the powder data.KEY FEATURES• Crystals with more than one molecule in the asymmetric unit can be considered.• Geometry Optimizations can be run in parallel• Simulation results for each space group are stored in trajectory files for further analysis.• Analysis is carried in study tables, displaying various properties such as space group, energy and cell parameters.• The Powder Comparison analysis feature allows for the automated quantitative comparison of experimental powder data to simulated powder patterns for each generated structure.• The Crystal Similarity Measure analysis feature allows for the automated quantitative comparison of experimentally determined crystal structure to each generated structure.• The Polymorph Clustering analysis feature allows for automated quantitative comparison of each generated structure to all the other structures generated in the same or different space group, and same or different simulation runs.• A variety of property calculations, including• Powder Comparison and Crystal Similarity measures, can be carried out on all or a subset of the crystal structures in a study table.• You can access Polymorph functionality through the MaterialsScript API. Scripting allows you to automaterepetitive task and customize workflows. BIOVIA Materials Studio Polymorph Predictor workflowRUNNING JOBS• All BIOVIA Materials Studio Polymorph Predictor jobs are run in the background freeing up the BIO VIA Materials Studio client for other research.• All BIOVIA Materials Studio Polymorph Predictor jobs can be submitted to remote computer servers.RESULTSSimulation results for each space group are stored in trajectory files for further analysis.ANALYSIS• Analysis is carried out with the help of spreadsheet-like tables, called study tables.• Multiple trajectories files (e.g. corresponding to different space groups) can be imported into a study table to be analyzed simultaneously.• When each trajectory file is loaded, various properties such as space group, energy and cell parameters, are automatically entered into the study table as well.• Each crystal structure is embedded in the study table, which can be viewed independently and displayed along with various properties.• The Powder Comparison analysis feature allows for the automated quantitative comparison of experimental powder data to simulated powder patterns for each generated structure.• The Crystal Similarity Measure analysis feature allows for the automated quantitative comparison of experimentally determined crystal structure to each generated structure.• The Polymorph Clustering analysis feature allows for automated quantitative comparison of each generated structure to all the other structures generated in the same or different space group, and same or different simulation runs.• A variety of property calculations, including Powder Comparison and Crystal Similarity measures, can be carried out on all or a subset of the crystal structures in a study table.To learn more about BIOVIA Materials Studio, go to/materials-studioOur 3D EXPERIENCE Platform powers our brand applications, serving 12 industries, and provides a rich portfolio of industry solution experiences.Dassault Systèmes, the 3D EXPERIENCE Company, provides business and people with virtual universes to imagine sustainable innovations. Its world-leading solutions transform the way products are designed, produced, and supported. Dassault Systèmes’ collaborative solutions foster social innovation, expanding possibilities for the virtual world to improve the real world. The group brings value to over 170,000 customers of all sizes in all industries in more than 140 countries. For more information, visit .Dassault Systèmes Corporate Dassault Systèmes 175 Wyman StreetWaltham, Massachusetts 02451-1223USA BIOVIA Corporate Europe BIOVIA334 Cambridge Science Park,Cambridge CB4 0WN EnglandBIOVIA Corporate Americas BIOVIA5005 Wateridge Vista Drive,San Diego, CA 92121USA©2014 D a s s a u l t S y s t èm e s . A l l r i g h t s r e s e r v e d . 3D E X P E R I E N C E , t h e C o m p a s s i c o n a n d t h e 3D S l o g o , C A T I A , S O L I D W O R K S , E N O V I A , D E L M I A , S I M U L I A , G E O V I A , E X A L E A D , 3D V I A , B I O V I A a n d N E T V I B E S a r e c o m m e r c i a l t r a d e m a r k s o r r e g i s t e r e d t r a d e m a r k s o f D a s s a u l t S y s t èm e s o r i t s s u b s i d i a r i e s i n t h e U .S . a n d /o r o t h e r c o u n t r i e s . A l l o t h e r t r a d e m a r k s a r e o w n e d b y t h e i r r e s p e c t i v e o w n e r s . U s e o f a n y D a s s a u l t S y s t èm e s o r i t s s u b s i d i a r i e s t r a d e m a r k s i s s u b j e c t t o t h e i r e x p r e s s w r i t t e n a p p r o v a l .DS-8058-1114。
QLogic 12200-BS21、12800-040 和 12800-180 InfiniBand
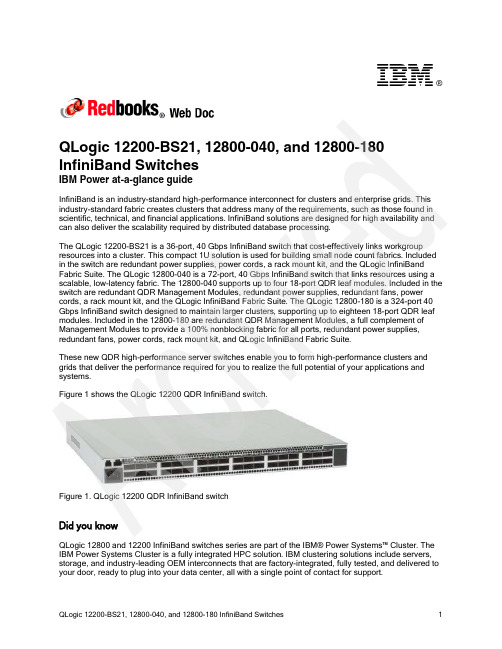
®QLogic 12200-BS21, 12800-040, and 12800-180 InfiniBand SwitchesIBM Power at-a-glance guideInfiniBand is an industry-standard high-performance interconnect for clusters and enterprise grids. This industry-standard fabric creates clusters that address many of the requirements, such as those found in scientific, technical, and financial applications. InfiniBand solutions are designed for high availability and can also deliver the scalability required by distributed database processing.The QLogic 12200-BS21 is a 36-port, 40 Gbps InfiniBand switch that cost-effectively links workgroup resources into a cluster. This compact 1U solution is used for building small node count fabrics. Included in the switch are redundant power supplies, power cords, a rack mount kit, and the QLogic InfiniBand Fabric Suite. The QLogic 12800-040 is a 72-port, 40 Gbps InfiniBand switch that links resources using a scalable, low-latency fabric. The 12800-040 supports up to four 18-port QDR leaf modules. Included in the switch are redundant QDR Management Modules, redundant power supplies, redundant fans, power cords, a rack mount kit, and the QLogic InfiniBand Fabric Suite. The QLogic 12800-180 is a 324-port 40 Gbps InfiniBand switch designed to maintain larger clusters, supporting up to eighteen 18-port QDR leaf modules. Included in the 12800-180 are redundant QDR Management Modules, a full complement of Management Modules to provide a 100% nonblocking fabric for all ports, redundant power supplies, redundant fans, power cords, rack mount kit, and QLogic InfiniBand Fabric Suite.These new QDR high-performance server switches enable you to form high-performance clusters and grids that deliver the performance required for you to realize the full potential of your applications and systems.Figure 1 shows the QLogic 12200 QDR InfiniBand switch.Figure 1. QLogic 12200 QDR InfiniBand switchDid you knowQLogic 12800 and 12200 InfiniBand switches series are part of the IBM® Power Systems™ Cluster. The IBM Power Systems Cluster is a fully integrated HPC solution. IBM clustering solutions include servers, storage, and industry-leading OEM interconnects that are factory-integrated, fully tested, and delivered to your door, ready to plug into your data center, all with a single point of contact for support.Table 1 shows the part numbers to order these modules and additional options for them. Table 1. IBM part numbers for orderingFigure 2 shows the 12800-180 switch module.Figure 2. QLogic 12800-180 InfiniBand switchThe QLogic 12800-180, 12800-040, and 12200-BS21 QDR InfiniBand switches, based on QLogic TrueScale ASIC technology, deliver the next evolution in switch fabric performance for High Performance Computing (HPC) environments.These switches deliver high port density and low power per port:The 12800-180 provides 324 QDR ports using 18-port Ultra-High Performance (UHP) leaf modules in za 14U chassis.The 12800-040 provides 72 QDR ports using 18-port UHP leaf modules in a 5U chassis.zThe 12200-BS21 provides 36 QDR ports a 1U chassis.zThe high-availability 12800 design includes hot swappable InfiniBand spine and leaf modules, fully redundant power and cooling, and redundant management processors supporting chassis management (CLI and GUI), as well as embedded InfiniBand Subnet Managers (SMs). The 12800 also supports advanced QLogic fabric features including adaptive routing, Virtual Fabrics (vFabric), Quality of Service (QoS), and management wizards for automated installation, configuration, and monitoring to maximize operational efficiency.The QLogic 12800 and 12200 InfiniBand switches include redundant power supplies, power cords, a rack mount kit, and the QLogic InfiniBand Fabric Suite.BenefitsThe QLogic 12800 and 12200 InfiniBand switches offer the following benefits:Low latency: The QLogic 12800 and 12200 provide scalable, predictable low latency, even at 90%ztraffic use. Predictable latency means that HPC applications can be scaled easily without worrying about diminished cluster performance or costly system-tuning efforts.Flexible partitioning: The QLogic 12800 and 12200 advanced design are based on an architecturezthat provides comprehensive virtual fabric partitioning capabilities that enable the InfiniBand fabric to support the evolving requirements of an organization. The TrueScale architecture, together with IFS, allows the fabric to be shared by mission-critical applications while delivering maximum bandwidth utilization.Modular design: InfiniBand port, power, cooling, and management modules are common in the series, zgiving customers the flexibility to deploy and grow HPC environments in a cost-effective fashion.Investment protection: The QLogic 12800 and 12200 adheres to the InfiniBand Trade AssociationzVersion 1.2 specification, ensuring the ability to interoperate with all other IBTA-compliant devices.Highly reliable: This system is designed for high availability with features that include port-to-port and zmodule-to-module failover, non-disruptive firmware upgrades, component-level diagnostics andalarming, and both in-band and out-of-band management.Easy to manage: The 12800 and 12200 use QLogic’s advanced IFS software for quicker installation zand configuration. IFS has advanced tools to verify fabric configuration, topology, and performance.Faults are automatically isolated to the component level and reported.Simple installation and configuration: Using the installation and configuration wizards contained in the zIFS package allows users to bring up fabrics in days instead of weeks.Power optimized: Maximum performance is delivered with minimal power and cooling requirements zas part of QLogic’s Star Power commitment to developing green solutions for the data center. Features and specificationsThe QLogic 12800 InfiniBand switches include the following features and functions:Between 36 and 324 ports of InfiniBand QDR (40 Gbps) performance with support for DDR and SDR z40/20/10 Gbps auto-negotiation linkszSupports Quad Small Form Factor Pluggable (QSFP) optical cable specifications zTrueScale architecture, with scalable, predictable low latencyzScales to 25.92 Tbps aggregate bandwidthzSwitching latency: 140 to 420 nszMultiple virtual lanes (VLs) per physical portzVirtual lanes: Eight plus one managementzMaximum MTU size: 4096 byteszMaximum multicast table size: 1024 entrieszSupports virtual fabric partitioningzFully redundant system designzOption to use UHD leafs for maximum connectivity and performance (only on QLogic 12800 switches) zUHP module: 18 QDR portszRedundant QDR managed spine modules (only on QLogic 12800-180 switch) -- a full complement of zspine modules to provide a 100% nonblocking fabric for all portsIntegrated chassis management capabilities for installation, configuration, and ongoing monitoringzOptional InfiniBand Fabric Suite (IFS) management solution that provides expanded fabric views and zfabric toolsComplies with InfiniBand Trade Association (IBTA) Version 1.2 standardzThe QLogic 12200 InfiniBand switch includes the following features and functions:Thirty-six ports of InfiniBand QDR (40 Gbps) performance with support for DDR and SDRz40/20/10-Gbps auto-negotiation linkszSupports Quad Small Form Factor Pluggable (QSFP) optical cable specifications zTrueScale architecture, with scalable, predictable low latencyz2.88 Tbps aggregate bandwidthzSwitching latency: < 140 nszMultiple Virtual Lanes (VLs) per physical portzVirtual lanes: Eight plus one managementzMaximum MTU size: 4096 byteszMaximum multicast table size: 1024 entrieszSupports virtual fabric partitioningzRedundant power (12200 models 0449-028 and 0449-029)zExternal chassis management via optional InfiniBand Fabric Suite (IFS) management solution, which zprovides an expanded set of fabric views and fabric tools.Complies with InfiniBand Trade Association (IBTA) Version 1.2 standardzQLogic 12800-180 and 12800-040The QLogic 12800-180 has the following specifications:Eighteen to 324 portsz25.92 Tbps switching capacityzSupports up to 18 leaf moduleszThe QLogic 12800-040 has the following specifications:Eighteen to 96 portsz5.76 Tbps switching capacityzSupports up to 4 leaf moduleszThe QLogic 12800 InfiniBand switch family supports the following management methods: Command-line interfacezOptional external server-based InfiniBand-compliant subnet managerzOptional embedded fabric managementzIBTA-compliant SMA, PMA, and BMAzDDR InfiniBand (20 Gbps) solution with BladeCenter servers and QLogic 12800 and QLogic 12200 series switchesTable 3 shows core components to create a full-speed DDR (20 Gbps) InfiniBand solution using QLogic 12800 InfiniBand switches and Power Systems servers./systems/clusters/QLogic 12200 series product pagez/Products/Switches/Pages/InfiniBandSwitches.aspxNoticesThis information was developed for products and services offered in the U.S.A.IBM may not offer the products, services, or features discussed in this document in other countries. Consult your local IBM representative for information on the products and services currently available in your area. Any reference to an IBM product, program, or service is not intended to state or imply that only that IBM product, program, or service may be used. Any functionally equivalent product, program, or service that does not infringe any IBM intellectual property right may be used instead. However, it is the user's responsibility to evaluate and verify the operation of any non-IBM product, program, or service. IBM may have patents or pending patent applications covering subject matter described in this document. The furnishing of this document does not give you any license to these patents. You can send license inquiries, in writing, to:IBM Director of Licensing, IBM Corporation, North Castle Drive, Armonk, NY 10504-1785 U.S.A.The following paragraph does not apply to the United Kingdom or any other country where such provisions are inconsistent with local law: INTERNATIONAL BUSINESS MACHINES CORPORATION PROVIDES THIS PUBLICATION "AS IS" WITHOUT WARRANTY OF ANY KIND, EITHER EXPRESS OR IMPLIED, INCLUDING, BUT NOT LIMITED TO, THE IMPLIED WARRANTIES OF NON-INFRINGEMENT, MERCHANTABILITY OR FITNESS FOR A PARTICULAR PURPOSE. Some states do not allow disclaimer of express or implied warranties in certain transactions, therefore, this statement may not apply to you. This information could include technical inaccuracies or typographical errors. Changes are periodically made to the information herein; these changes will be incorporated in new editions of the publication. IBM may make improvements and/or changes in the product(s) and/or the program(s) described in this publication at any time without notice.Any references in this information to non-IBM Web sites are provided for convenience only and do not in any manner serve as an endorsement of those Web sites. The materials at those Web sites are not part of the materials for this IBM product and use of those Web sites is at your own risk.IBM may use or distribute any of the information you supply in any way it believes appropriate without incurring any obligation to you. Information concerning non-IBM products was obtained from the suppliers of those products, their published announcements or other publicly available sources. IBM has not tested those products and cannot confirm the accuracy of performance, compatibility or any other claims related to non-IBM products. Questions on the capabilities of non-IBM products should be addressed to the suppliers of those products. This information contains examples of data and reports used in daily business operations. To illustrate them as completely as possible, the examples include the names of individuals, companies, brands, and products. All of these names are fictitious and any similarity to the names and addresses used by an actual business enterprise is entirely coincidental.Any performance data contained herein was determined in a controlled environment. Therefore, the results obtained in other operating environments may vary significantly. Some measurements may have been made on development-level systems and there is no guarantee that these measurements will be the same on generally available systems. Furthermore, some measurement may have been estimated through extrapolation. Actual results may vary. Users of this document should verify the applicable data for their specific environment.COPYRIGHT LICENSE:This information contains sample application programs in source language, which illustrate programming techniques on various operating platforms. You may copy, modify, and distribute these sample programs in any form without payment to IBM, for the purposes of developing, using, marketing or distributing application programs conforming to the application programming interface for the operating platform for which the sample programs are written. These examples have not been thoroughly tested under all conditions. IBM, therefore, cannot guarantee or imply reliability, serviceability, or function of these programs.© Copyright International Business Machines Corporation 2011. All rights reserved.Note to U.S. Government Users Restricted Rights -- Use, duplication or disclosure restricted byGSA ADP Schedule Contract with IBM Corp.This document was created or updated on October 14, 2011.Send us your comments in one of the following ways:Use the online Contact us review form found at:z/redbooksSend your comments in an e-mail to:z**************.comMail your comments to:zIBM Corporation, International Technical Support OrganizationDept. HYTD Mail Station P0992455 South RoadPoughkeepsie, NY 12601-5400 U.S.A.This document is available online at /redbooks/abstracts/tips0821.html . TrademarksIBM, the IBM logo, and are trademarks or registered trademarks of International Business Machines Corporation in the United States, other countries, or both. These and other IBM trademarked terms are marked on their first occurrence in this information with the appropriate symbol (® or ™), indicating US registered or common law trademarks owned by IBM at the time this information was published. Such trademarks may also be registered or common law trademarks in other countries. A current list of IBM trademarks is available on the Web at /legal/copytrade.shtmlThe following terms are trademarks of the International Business Machines Corporation in the United States, other countries, or both:BladeCenter®IBM®Power Systems™Redpaper™Redbooks (logo)®Other company, product, or service names may be trademarks or service marks of others.QLogic 12200-BS21, 12800-040, and 12800-180 InfiniBand Switches11。
一种通用的生命线工程网络事件空间聚类分析算法
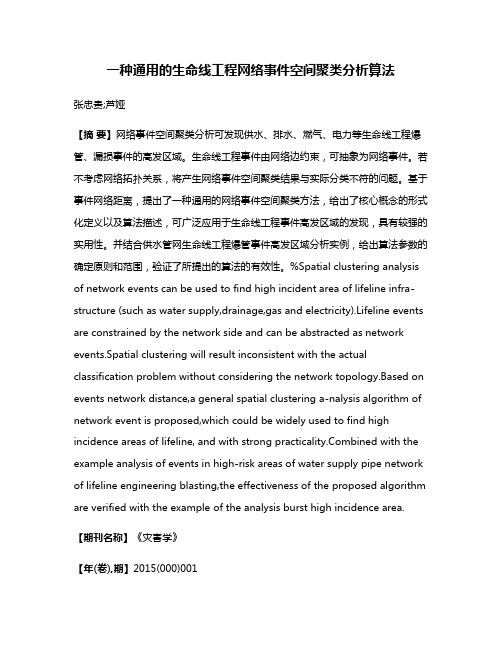
一种通用的生命线工程网络事件空间聚类分析算法张忠贵;芦娅【摘要】网络事件空间聚类分析可发现供水、排水、燃气、电力等生命线工程爆管、漏损事件的高发区域。
生命线工程事件由网络边约束,可抽象为网络事件。
若不考虑网络拓扑关系,将产生网络事件空间聚类结果与实际分类不符的问题。
基于事件网络距离,提出了一种通用的网络事件空间聚类方法,给出了核心概念的形式化定义以及算法描述,可广泛应用于生命线工程事件高发区域的发现,具有较强的实用性。
并结合供水管网生命线工程爆管事件高发区域分析实例,给出算法参数的确定原则和范围,验证了所提出的算法的有效性。
%Spatial clustering analysis of network events can be used to find high incident area of lifeline infra-structure (such as water supply,drainage,gas and electricity).Lifeline events are constrained by the network side and can be abstracted as network events.Spatial clustering will result inconsistent with the actual classification problem without considering the network topology.Based on events network distance,a general spatial clustering a-nalysis algorithm of network event is proposed,which could be widely used to find high incidence areas of lifeline, and with strong bined with the example analysis of events in high-risk areas of water supply pipe network of lifeline engineering blasting,the effectiveness of the proposed algorithm are verified with the example of the analysis burst high incidence area.【期刊名称】《灾害学》【年(卷),期】2015(000)001【总页数】5页(P29-33)【关键词】生命线工程;网络;事件;空间聚类;网络距离【作者】张忠贵;芦娅【作者单位】中国地质大学武汉信息工程学院,湖北武汉430074; 武汉中地数码科技有限公司,湖北武汉430074;湖北省地震局,湖北武汉430071【正文语种】中文【中图分类】P208;X4生命线工程设施是维系现代城市与区域经济功能的基础性工程设施[1],包括供水、排水、燃气、电力等基础设施。
RAxML建立极大似然进化树简明指南
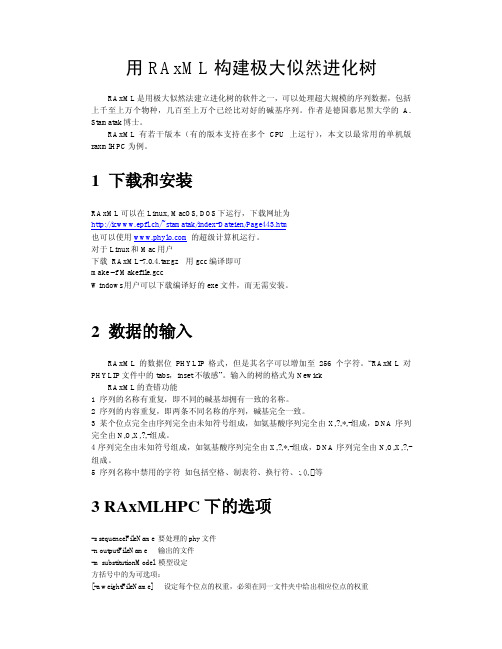
用RAxML构建极大似然进化树RAxML是用极大似然法建立进化树的软件之一,可以处理超大规模的序列数据,包括上千至上万个物种,几百至上万个已经比对好的碱基序列。
作者是德国慕尼黑大学的 A. Stamatak博士。
RAxML有若干版本(有的版本支持在多个CPU上运行),本文以最常用的单机版raxmlHPC为例。
1 下载和安装RAxML可以在Linux, MacOS, DOS下运行,下载网址为http://icwww.epfl.ch/~stamatak/index-Dateien/Page443.htm也可以使用的超级计算机运行。
对于Linux和Mac用户下载RAxML-7.0.4.tar.gz 用gcc编译即可make –f Makefile.gccWindows用户可以下载编译好的exe文件,而无需安装。
2 数据的输入RAxML的数据位PHYLIP格式,但是其名字可以增加至256个字符。
“RAxML对PHYLIP文件中的tabs,inset不敏感”。
输入的树的格式为NewickRAxML的查错功能1 序列的名称有重复,即不同的碱基却拥有一致的名称。
2 序列的内容重复,即两条不同名称的序列,碱基完全一致。
3 某个位点完全由序列完全由未知符号组成,如氨基酸序列完全由X,?,*,-组成,DNA序列完全由N,O,X,?,-组成。
4序列完全由未知符号组成,如氨基酸序列完全由X,?,*,-组成,DNA序列完全由N,O,X,?,-组成。
5 序列名称中禁用的字符如包括空格、制表符、换行符、:,(),[]等3 RAxMLHPC下的选项-s sequenceFileName 要处理的phy文件-n outputFileName 输出的文件-m substitutionModel 模型设定方括号中的为可选项:[-a weightFileName] 设定每个位点的权重,必须在同一文件夹中给出相应位点的权重[-b bootstrapRandomNumberSeed] 设定bootstrap起始随机数[-c numberOfCategories] 设定位点变化率的等级[-d] -d 完全随机的搜索进化树,而不是从maximum parsimony tree开始。
- 1、下载文档前请自行甄别文档内容的完整性,平台不提供额外的编辑、内容补充、找答案等附加服务。
- 2、"仅部分预览"的文档,不可在线预览部分如存在完整性等问题,可反馈申请退款(可完整预览的文档不适用该条件!)。
- 3、如文档侵犯您的权益,请联系客服反馈,我们会尽快为您处理(人工客服工作时间:9:00-18:30)。
2 Clustering of the SOM
Clustering data with an SOM consists of two steps. First the SOM is trained with any of several available learning rules [1] so that the weights of the Processing Elements (PEs, neural units), become optimally placed prototype vectors of the data space. After training, groups of similar weights are identified and data points assigned to the cluster of their prototype vector. This can be done in a number of ways including the methods referred to in the Introduction. Clustering the SOM weights is of particular interest because – if high quality – its potentials to both find detailed structure and provide a high level of automation are great. We propose a hierarchical agglomerative scheme, similar in its main steps to that in [13]. However, we introduce substantial differences, which we discuss at the respective points below. Some of these differences are adapted from [15], which we regard as the precursor of this work. We refer to [13] for a discussion of the relative merits of partitive and hierarchical approaches, and only recall here that the latter can handle arbitrary cluster shapes with appropriate metrics and it is of relatively low complexity due to the use of local information. It suits high-dimensional real data, which often are hard to describe with parametric models. We aim at detailed cluster identification of voluminous high-dimensional data by including two components of the SOM knowledge that were underutilized in previous methods: topology and local data density. We show, in particular, that this facilitates the capture of rare clusters. 2.1 Our Proposed Algorithm Defining characteristics of all clustering methods are the between- and within-cluster metrics and the cluster validity criterion used. Table 1 lists the metrics used in this paper. Our cluster validity criterion is the same as in [15]: A point in a cluster is closer to some point in the same cluster than to any point in any other cluster. This is in contrast to [13], where the cluster validity measure is the Davies-Bouldin index or a gap criterion. We follow [15] because it facilitates identification of finer cluster structure. The between- and within-cluster distances are single linkage and maximum nearest neighbour, respectively, in [15] as well as here. [13] uses centroid linkage and centroid distance; or single linkage and nearest neighbour respectively, for the same. We cluster the SOM in four Phases, following the general logic of [13]. Phase I. Building a dendrogram: Eaton cluster at first. Pairwise single linkage distances are computed between all clusters and the two clusters closest to each other are merged. This process is repeated until only one cluster is left. PEs with empty receptive fields are interpolating units in the SOM. For complex or noisy data, non-empty PEs can still be interpolating units, characterized by small receptive fields. Omitting these from the
OF
Abstract – The Self-Organizing Map (SOM) [1] is an effective tool for clustering and data mining. One way to extract cluster structure from a trained SOM is by clustering its weights, which has great potential for automation. This potential is not fully realized by existing algorithms, and leaves large, high-dimensional, complex data to semi-manual treatment. Our main contribution is the exploitation of the data topology in clustering the SOM. Combined with appropriate distance and cluster validity measures, this results in a high degree of precision and automation of cluster extraction, including the discovery of rare clusters. It may work with prototypes of other quantization methods since direct use of SOM locations can be avoided. Key words – SOM, clustering, boundary extraction, data mining
C ONSIDERI NG T OPO LOG Y I N T HE C LUS T ERING S ELF -O RG ANIZ ING M APS 1
Kadim Taşdemir, Erzsébet Merényi Rice University Houston, Texas, USA {tasdemir,erzsebet}@
This work was partially supported by grant NNG05GA94G from the Applied Information Systems Research Program, NASA, Science Mission Directorate. Figures are in color, request color copy by email.
1 Introduction
The Self-Organizing Map (SOM) [1] is a widely and successfully used neural paradigm for clustering. Automated and precise capture of cluster boundaries from a learned SOM has been a long-standing challenge that to date has only partial solutions. The problem is especially important for high-dimensional and large data sets with many meaningful clusters such as in remote sensing or medical imagery, which often also have interesting rare clusters to be discovered. The U-matrix approach [2] and its variants work well when relatively large SOM grid is applied to small data sets with a low number of clusters (e.g., [3], [4], [5]) but they tend to obscure finer structure for more complex data due to averaging of weight distances over neighbours or thresholding. Imaginative approaches such as [6] and gravitational methods (e.g., Adaptive Coordinates [4]) visualize distances between receptive field centres in informative ways that greatly guide the human operator. However, they do not extract clusters explicitly. Experiments with automated colour assignments are admittedly meant for qualitative exploration of the approximate cluster structure [7], [8], [9]. More overview and references are offered in [4]. [10] presents a growing SOM that handles high data dimension gracefully, but it appears less robust than the Kohonen SOM (KSOM) because of the larger number of parameters to adjust, and it is unclear how it would work for large data volumes. An elegant proposal is the use of higher order neurons [11] whereby clusters of highly complex shape can be represented by single tensorial weights. This is theoretically very attractive but has several problems, one being the computational complexity; another is the need for tight determination of the number of SOM neurons because of the one-to-one correspondence