Efficient GSVD Based Multi-user MIMO Linear
基于GSVD的分布式MIMO雷达测向算法

基于GSVD的分布式MIMO雷达测向算法
张颢月;师俊朋;史姝赟;吴奇龙
【期刊名称】《信息对抗技术》
【年(卷),期】2024(3)1
【摘要】针对分布式多输入多输出(multi-input multi-output,MIMO)雷达测向中存在的数据信息提取不充分、运算量偏大等问题,开展了基于广义奇异值分解(generalized singular value decomposition,GSVD)的测向算法研究,以提高低信噪比条件下的角度估计性能。
首先,建立了分布式阵列MIMO雷达回波信号的统一化表征模型;其次,将分布式MIMO雷达系统接收阵列数据的多线程GSVD问题转换为一个联合优化问题,运用交替最小二乘(alternating least squares,ALS)技术实现阵列信号流行矩阵的拟合,并引入子空间类算法实现目标角度联合估计;最后,对优化问题增加l1范数约束,避免了每次迭代中进行的奇异值分解运算,降低了算法运算量。
仿真实验从角度联合估计、均方误差、运算时间等方面验证了所提算法的有效性。
【总页数】13页(P57-69)
【作者】张颢月;师俊朋;史姝赟;吴奇龙
【作者单位】国防科技大学电子对抗学院;安徽省军区
【正文语种】中文
【中图分类】TN958
【相关文献】
1.基于张量ESP RIT?SVD算法的双基地MIMO雷达测向方法
2.MIMO雷达对分布式目标测向研究
3.基于逐次剔除算法的多任务下分布式MIMO雷达有限阵元选取
4.基于广义互相关的分布式MIMO雷达信号合成算法
5.基于分布式MIMO雷达的检测前跟踪算法研究
因版权原因,仅展示原文概要,查看原文内容请购买。
基于石墨烯超表面的效率可调太赫兹聚焦透镜

DOI: 10.12086/oee.2021.200319基于石墨烯超表面的效率可调太赫兹聚焦透镜王俊瑶,樊俊鹏,舒 好,刘 畅,程用志*武汉科技大学信息科学与工程学院,湖北 武汉 430081摘要:本文提出了一种基于石墨烯超表面的效率可调太赫兹聚焦透镜。
该超表面单元结构由两层对称的圆形镂空石墨烯和中间介质层组成,其中镂空圆形中间由长方形石墨烯片连接。
该结构可实现偏振转换,入射到超表面的圆偏振波将以其正交的形式出射,如左旋圆到右旋圆偏振转换。
利用几何相位原理,通过旋转长方形条的方向,透射波会携带额外的附加相位并能满足2π范围内覆盖。
合适地排列石墨烯超表面的单元结构,以实现太赫兹聚焦透镜。
仿真结果表明:通过改变石墨烯的费米能级,可以对超表面圆偏振转换幅度进行调节,进而超透镜的聚焦效率也可以动态调节。
因此,这种基于石墨烯超表面的效率可调聚焦透镜不用改变单元结构的尺寸,只需通过改变费米能级便可实现,可以广泛地应用到能量收集、成像等太赫兹应用领域。
关键词:超表面;聚焦透镜;石墨烯;太赫兹中图分类号:TH74;TQ127.11 文献标志码:A王俊瑶,樊俊鹏,舒好,等. 基于石墨烯超表面的效率可调太赫兹聚焦透镜[J]. 光电工程,2021,48(4): 200319Wang J Y , Fan J P , Shu H, et al. Efficiency-tunable terahertz focusing lens based on graphene metasurface[J]. Opto-Electron Eng , 2021, 48(4): 200319Efficiency-tunable terahertz focusing lens based on graphene metasurfaceWang Junyao, Fan Junpeng, Shu Hao, Liu Chang, Cheng Yongzhi *School of Information Science and Engineering, Wuhan University of Science and Technology, Wuhan, Hubei 430081, China Abstract: This paper proposes an efficiency-tunable terahertz focusing lens based on the graphene metasurface. The unit cell is composed of two symmetrical circular graphene hollows and an intermediate dielectric layer, wherein the hollow circular middle is connected by a rectangular graphene sheet. This structure can realize polarization conversion, for example, when an incidence with left-hand circular polarization emitted on the metasurface the po-larization of the transmitted light is right-hand circular polarization. According to the principle of geometric phase, by rotating the direction of the rectangular bar, the transmitted wave will carry an additional phase and can cover the range of 2π. An THz focusing lens can be realized by properly arranging the unit structure of the graphene metasurface. The simulation results show that the conversion amplitude of circular polarized light can be adjusted by changing the Fermi level of graphene, and the focusing efficiency of the metalens can also be dynamically adjusted.LCPRCP(cross-polarization)xy zV g——————————————————收稿日期:2020-08-27; 收到修改稿日期:2020-10-26基金项目:湖北省教育厅科技研究计划重点项目(D2*******);武汉科技大学研究生创新基金项目(JCX201959);大学生创新基金项目资助课题(20ZA083)作者简介:王俊瑶(2000-),女,主要从事电子科学与技术专业。
超过硅极限的弹道二维晶体管
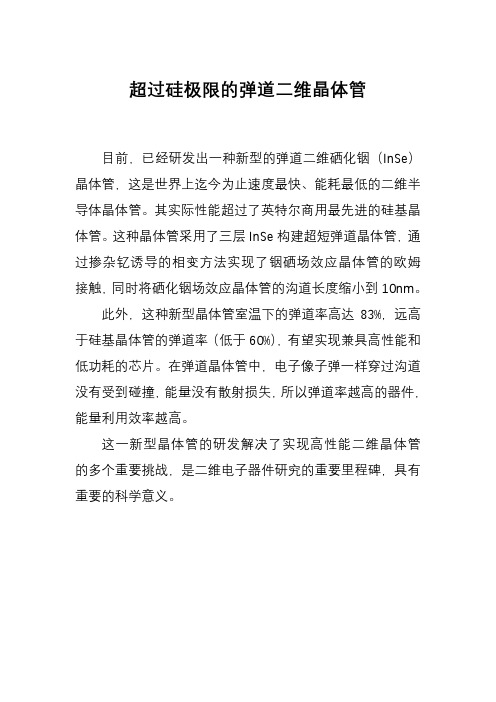
超过硅极限的弹道二维晶体管
目前,已经研发出一种新型的弹道二维硒化铟(InSe)晶体管,这是世界上迄今为止速度最快、能耗最低的二维半导体晶体管。
其实际性能超过了英特尔商用最先进的硅基晶体管。
这种晶体管采用了三层InSe构建超短弹道晶体管,通过掺杂钇诱导的相变方法实现了铟硒场效应晶体管的欧姆接触,同时将硒化铟场效应晶体管的沟道长度缩小到10nm。
此外,这种新型晶体管室温下的弹道率高达83%,远高于硅基晶体管的弹道率(低于60%),有望实现兼具高性能和低功耗的芯片。
在弹道晶体管中,电子像子弹一样穿过沟道没有受到碰撞,能量没有散射损失,所以弹道率越高的器件,能量利用效率越高。
这一新型晶体管的研发解决了实现高性能二维晶体管的多个重要挑战,是二维电子器件研究的重要里程碑,具有重要的科学意义。
基于卸载策略的物联网边缘计算任务调度优化
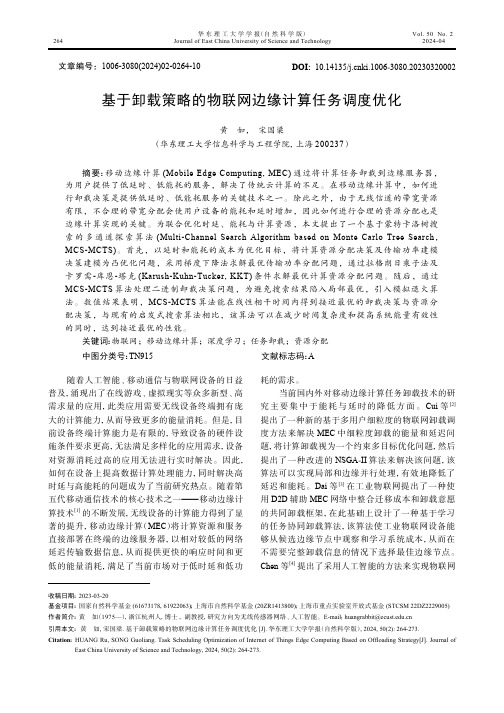
文章编号:1006-3080(2024)02-0264-10DOI: 10.14135/ki.1006-3080.20230320002基于卸载策略的物联网边缘计算任务调度优化黄 如, 宋国梁(华东理工大学信息科学与工程学院, 上海200237)摘要:移动边缘计算(Mobile Edge Computing, MEC)通过将计算任务卸载到边缘服务器,为用户提供了低延时、低能耗的服务,解决了传统云计算的不足。
在移动边缘计算中,如何进行卸载决策是提供低延时、低能耗服务的关键技术之一。
除此之外,由于无线信道的带宽资源有限,不合理的带宽分配会使用户设备的能耗和延时增加,因此如何进行合理的资源分配也是边缘计算实现的关键。
为联合优化时延、能耗与计算资源,本文提出了一个基于蒙特卡洛树搜索的多通道探索算法(Multi-Channel Search Algorithm based on Monte Carlo Tree Search ,MCS-MCTS)。
首先,以延时和能耗的成本为优化目标,将计算资源分配决策及传输功率建模决策建模为凸优化问题,采用梯度下降法求解最优传输功率分配问题,通过拉格朗日乘子法及卡罗需-库恩-塔克(Karush-Kuhn-Tucker, KKT)条件求解最优计算资源分配问题。
随后,通过MCS-MCTS 算法处理二进制卸载决策问题,为避免搜索结果陷入局部最优,引入模拟退火算法。
数值结果表明,MCS-MCTS 算法能在线性相干时间内得到接近最优的卸载决策与资源分配决策,与现有的启发式搜索算法相比,该算法可以在减少时间复杂度和提高系统能量有效性的同时,达到接近最优的性能。
关键词:物联网;移动边缘计算;深度学习;任务卸载;资源分配中图分类号:TN915文献标志码:A 随着人工智能、移动通信与物联网设备的日益普及,涌现出了在线游戏、虚拟现实等众多新型、高需求量的应用,此类应用需要无线设备终端拥有庞大的计算能力,从而导致更多的能量消耗。
智能反射面增强的多无人机辅助语义通信资源优化
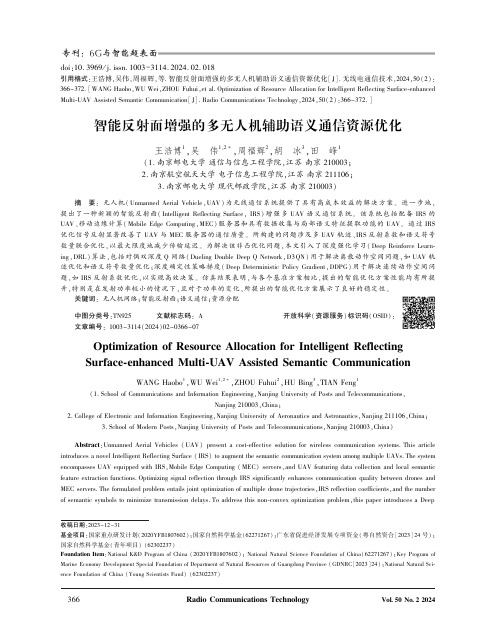
doi:10.3969/j.issn.1003-3114.2024.02.018引用格式:王浩博,吴伟,周福辉,等.智能反射面增强的多无人机辅助语义通信资源优化[J].无线电通信技术,2024,50(2): 366-372.[WANG Haobo,WU Wei,ZHOU Fuhui,et al.Optimization of Resource Allocation for Intelligent Reflecting Surface-enhanced Multi-UAV Assisted Semantic Communication[J].Radio Communications Technology,2024,50(2):366-372.]智能反射面增强的多无人机辅助语义通信资源优化王浩博1,吴㊀伟1,2∗,周福辉2,胡㊀冰3,田㊀峰1(1.南京邮电大学通信与信息工程学院,江苏南京210003;2.南京航空航天大学电子信息工程学院,江苏南京211106;3.南京邮电大学现代邮政学院,江苏南京210003)摘㊀要:无人机(Unmanned Aerial Vehicle,UAV)为无线通信系统提供了具有高成本效益的解决方案㊂进一步地,提出了一种新颖的智能反射面(Intelligent Reflecting Surface,IRS)增强多UAV语义通信系统㊂该系统包括配备IRS的UAV㊁移动边缘计算(Mobile Edge Computing,MEC)服务器和具有数据收集与局部语义特征提取功能的UAV㊂通过IRS 优化信号反射显著改善了UAV与MEC服务器的通信质量㊂所构建的问题涉及多UAV轨迹㊁IRS反射系数和语义符号数量联合优化,以最大限度地减少传输延迟㊂为解决该非凸优化问题,本文引入了深度强化学习(Deep Reinforce Learn-ing,DRL)算法,包括对偶双深度Q网络(Dueling Double Deep Q Network,D3QN)用于解决离散动作空间问题,如UAV轨迹优化和语义符号数量优化;深度确定性策略梯度(Deep Deterministic Policy Gradient,DDPG)用于解决连续动作空间问题,如IRS反射系数优化,以实现高效决策㊂仿真结果表明,与各个基准方案相比,提出的智能优化方案性能均有所提升,特别是在发射功率较小的情况下,且对于功率的变化,所提出的智能优化方案展示了良好的稳定性㊂关键词:无人机网络;智能反射面;语义通信;资源分配中图分类号:TN925㊀㊀㊀文献标志码:A㊀㊀㊀开放科学(资源服务)标识码(OSID):文章编号:1003-3114(2024)02-0366-07Optimization of Resource Allocation for Intelligent ReflectingSurface-enhanced Multi-UAV Assisted Semantic CommunicationWANG Haobo1,WU Wei1,2∗,ZHOU Fuhui2,HU Bing3,TIAN Feng1(1.School of Communications and Information Engineering,Nanjing University of Posts and Telecommunications,Nanjing210003,China;2.College of Electronic and Information Engineering,Nanjing University of Aeronautics and Astronautics,Nanjing211106,China;3.School of Modern Posts,Nanjing University of Posts and Telecommunications,Nanjing210003,China)Abstract:Unmanned Aerial Vehicles(UAV)present a cost-effective solution for wireless communication systems.This article introduces a novel Intelligent Reflecting Surface(IRS)to augment the semantic communication system among multiple UAVs.The system encompasses UAV equipped with IRS,Mobile Edge Computing(MEC)servers,and UAV featuring data collection and local semantic feature extraction functions.Optimizing signal reflection through IRS significantly enhances communication quality between drones and MEC servers.The formulated problem entails joint optimization of multiple drone trajectories,IRS reflection coefficients,and the number of semantic symbols to minimize transmission delays.To address this non-convex optimization problem,this paper introduces a Deep收稿日期:2023-12-31基金项目:国家重点研发计划(2020YFB1807602);国家自然科学基金(62271267);广东省促进经济发展专项资金(粤自然资合[2023]24号);国家自然科学基金(青年项目)(62302237)Foundation Item:National K&D Program of China(2020YFB1807602);National Natural Science Foundation of China(62271267);Key Program of Marine Economy Development Special Foundation of Department of Natural Resources of Guangdong Province(GDNRC[2023]24);National Natural Sci-ence Foundation of China(Young Scientists Fund)(62302237)ReinforcementLearning(DRL)algorithm.Specifically,theDuelingDoubleDeepQNetwork(D3QN)isemployedtoaddressdiscreteactionspaceproblemssuchasdronetrajectoryandsemanticsymbolquantityoptimization.Additionally,DeepDeterministicPolicyGra dient(DDPG)algorithmisutilizedtosolvecontinuousactionspaceproblems,suchasIRSreflectioncoefficientoptimization,enablingefficientdecision making.Simulationresultsdemonstratethattheproposedintelligentoptimizationschemeoutperformsvariousbenchmarkschemes,particularlyinscenarioswithlowtransmissionpower.Furthermore,theintelligentoptimizationschemeproposedinthispaperexhibitsrobuststabilityinresponsetopowerchanges.Keywords:UAVnetwork;IRS;semanticcommunication;resourceallocation0 引言当前技术飞速发展的背景下,无人机(UnmannedAerialVehicle,UAV)已经成为无线通信系统中一种重要的技术[1]。
基于边缘计算的高效低时延通信传输技术研究

I G I T C W技术 研究Technology Study14DIGITCW2023.10随着物联网、5G 、AI 等技术的飞速发展,数据产生的速度和数量都在爆炸式增长[1],这大大增加了对高效、低时延的通信传输技术的需求。
边缘计算作为一种新型的计算范式,因其能够在靠近数据源的地方完成数据处理,从而大大减少了延迟,提高了数据处理的效率,得到了广泛的关注和研究[2]。
边缘计算不仅能够处理离散的、由边缘设备产生的大量数据,还能够快速响应服务请求,满足实时性的需求[3]。
尤其在一些对时延敏感的应用中,如自动驾驶、远程医疗、智能制造等,边缘计算展现出了无可比拟的优势。
然而,尽管边缘计算具有显著的优势,如何将其与通信技术相结合,实现高效、低时延的数据传输,仍然是一个重要而且具有挑战性的问题。
因此,本文将重点研究基于边缘计算的高效低时延通信传输技术,详细介绍边缘计算和通信技术的总框架,探讨结合方式,以及如何通过优化技术策略实现高效、低时延的数据传输。
希望本文的研究能为边缘计算和通信技术的进一步发展提供一些有价值的思考和参考。
1 基于边缘计算的传输架构基于边缘计算的传输架构由网络服务、核心网EPC 、移动中继节点、汇聚节点以及MEC 服务器(多接入边缘计算)组成。
如图1所示。
网络服务负责管理和控制边缘网络,包括边缘服务器、边缘操作系统、边缘应用程序、边缘云平台和传输协议栈[4]。
EPC 是边缘网络中的一个关键组成部分,它负责管理和配置边缘网络,并提供网络配置、性能监测、安全管理等功能。
移动中继节点负责在移动设备和汇聚节点之间传递数据,并支持多跳、协作传基于边缘计算的高效低时延通信传输技术研究郑 毅(北京华麒通信科技有限公司,北京 100080)摘要:近年来,边缘计算凭借其在灵活性、高效性和可靠性方面的优势,已经成为5G通信研究的热点之一。
边缘计算与5G通信相结合可以进一步提高通信传输的效率和质量。
文章提出了基于边缘计算的高效低时延通信传输技术,对基于边缘计算的高效低时延通信传输技术进行了深入研究,探讨边缘计算与5G通信相结合时的特点和优势,构架了总体传输框架,发现了该技术在实际应用中的问题和挑战,希望能够实现高效低时延的网络通信传输技术。
基于深度卷积-长短期记忆神经网络的整车道路载荷预测

拟路谱技术和基于机器学习的路谱识别技术。前者首 先通过激光扫描技术获取试验场路面不平度信号,然 后对包括轮胎、衬套悬置等弹性元件的整车模型进行 动力学仿真分析 ;后 [1-4] 者首先利用合适的机器学习模 型直接根据方便测量的整车参数预测道路载荷,然后 利用整车动力学仿真分析获取底盘结构件的动态响 应载荷 。 [5-8] 通过对比这 2 种方法,发现与虚拟路谱技术 相比,基于机器学习的路谱识别技术省去了操作复杂且 代价高昂的路面不平度测量工作,且不需要在整车动力 学模型中建立轮胎模型。
经 网 络(DCNN-LSTM)模 型 ,提 出 了 基 于 数 据 驱 动 的 整 车 轮 心 载 荷 预 测 方 法 。 对 比 试 验 结 果 表 明 ,该 方 法 预 测 的 整 车 轮 心
载荷与试验场采集数据非常接近,有利于逐步取消路谱采集试验并极大地提高整车耐久性分析的效率。
主题词:道路载荷 深度学习 数据库 疲劳耐久分析 深度卷积神经网络 长短期记忆
每小块求取统计值(如均值或最大值)即可得到池化层 的输出信息。在整车道路载荷预测中,需要处理的汽车
算和求和运算,然后通过非线性转换获得卷积层的输出 信息。在池化层,输入的数据被分为很多小块,通过对
运行参数属于一维时序数据,因此 DCNN 层选用如图 2 所示的一维卷积神经网络层。
x(1) x(2)
x(S - 1) x(S)
1 前言
在现有的汽车底盘结构疲劳耐久分析流程中,为了 获得整车的道路载荷谱,通常需要在项目开发早期开展 整车道路耐久试验,该试验需要特制的试制样车、测量 设备、试验场所以及数周的试验时间。随着控制成本和 缩减开发周期的要求日益严格,道路试验成本高、周期 长的问题更加突出,亟待解决。
新“半导体之王”
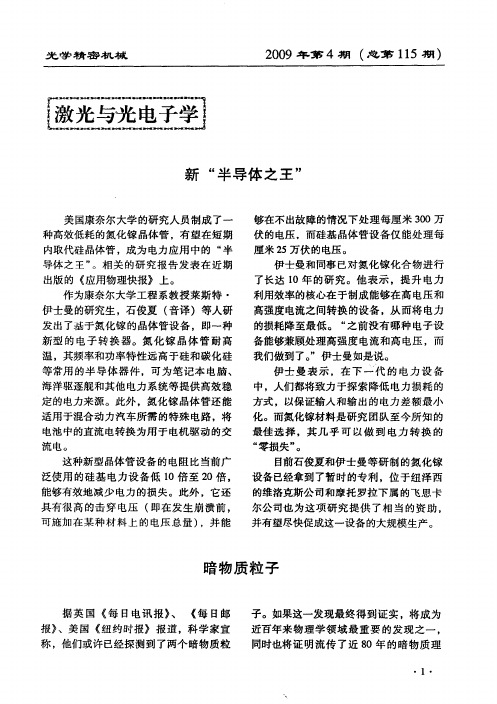
设备已经拿到了暂时 的专利 ,位于纽泽西
的维洛克 斯公 司和 摩 托罗 拉下 属 的飞 思卡 尔 公司也 为 这 项 研 究 提供 了 相 当 的资 助 , 并有 望尽快 促成这 一设 备 的大规模 生产 。
新 型 的 电子 转 换 器 。 氮 化 镓 晶 体 管 耐 高
利用 效率 的核心 在 于制 成 能够 在 高 电压 和 高强 度 电流 之 间转换 的设 备 ,从 而将 电力 的损耗 降 至最 低 。 “ 之前 没 有 哪 种 电子 设 备能 够兼 顾处 理 高 强度 电流 和高 电压 ,而 我 们做 到 了。 伊士 曼如是 说 。 ” 伊 士 曼 表 示 ,在 下 一 代 的 电 力 设 备
适用 于混 合动 力汽 车 所需 的特 殊 电路 ,将 电池 中的直流 电转 换 为用 于 电机 驱动 的交
流 电。
“ 零损 失” 。 目前 石俊 夏和 伊 士曼 等 研 制 的氮 化 镓
这 种新 型 晶体 管 设 备 的电 阻 比当前 广
泛使用 的硅基 电力设 备低 1 0倍至 2 O倍 , 能够有 效地 减少 电力 的损 失 。此外 ,它 还
光 学精 密 机械
20 0 9年 第 4期 ( 第 15期 ) 总 1
新 “ 半导体 之 王 ”
美 国康奈 尔 大学 的研 究 人员 制 成 了一 种 高效低 耗 的氮化 镓 晶 体管 ,有 望 在短 期 内取代硅 晶体 管 ,成 为 电力 应 用 中的 “ 半 导体 之 王 ” 。相 关 的研 究 报 告 发 表 在 近 期
暗物质 粒子
空间目标自适应光学图像椭圆部件检测
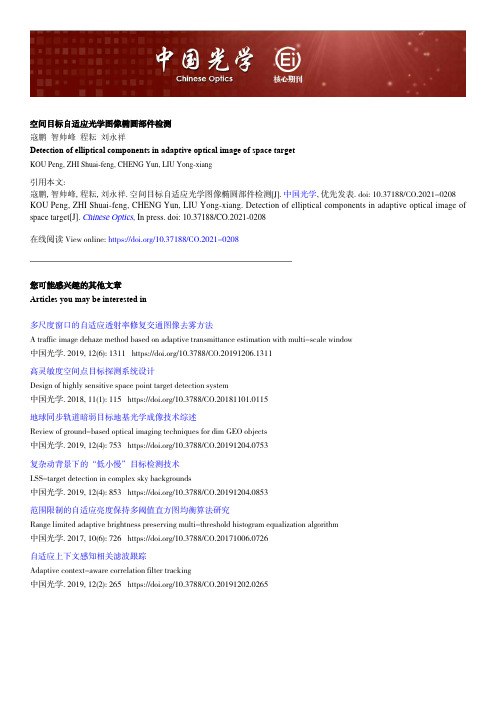
第 x 卷 第 x 期 xxxx 年 x 月
文章编号 2095-1531(xxxx)x-0001-10
中国光学 Chinese Optics
Vol. x No. x xxx. xxxx
空间目标自适应光学图像椭圆部件检测
寇 鹏1,2,智帅峰1,程 耘1,刘永祥1
(1. 国防科技大学 电子科学学院, 湖南 长沙 410073; 2. 西安卫星测控中心, 陕西 西安 710600)
近年来,基于边缘连接的检测方法大大提高 了椭圆检测性能。这类方法的主要问题是如何确 定 属 于 同 一 椭 圆 的 椭 圆 弧 。 ELSD(Ellipse and Line Segment Detector) 方法通过检测 LS(Line Segments) 和对 LS 分组,充分利用了椭圆的梯度和 几何特征,可以在不调整任何参数的情况下减少 对各种类型图像的错检率[9]。文献 [10] 结合了基 于 HT 和基于边缘链接的方法的优点来检测工业 图像中的椭圆,但它们不适用于一般的椭圆检 测。文献 [11] 提出了一种弧段基于弧邻接矩阵
KOU Peng1,2,ZHI Shuai-feng1,CHENG Yun1,LIU Yong-xiang1 (1. School of Electronic Science, University of Defense Science and technology, Changsha 410073, China;
摘要:为了识别空间目标的椭圆部件,提出了一种基于自适应光学图像的椭圆检测方法。首先,利用
RL(Richardson–Lucy) 方法对自应光学图像进行复原,在此基础上,采用弧支撑线段 (ASLS, Arc-support Line Seg-
复旦研发出基于金属硒化物的太阳电池高效电极
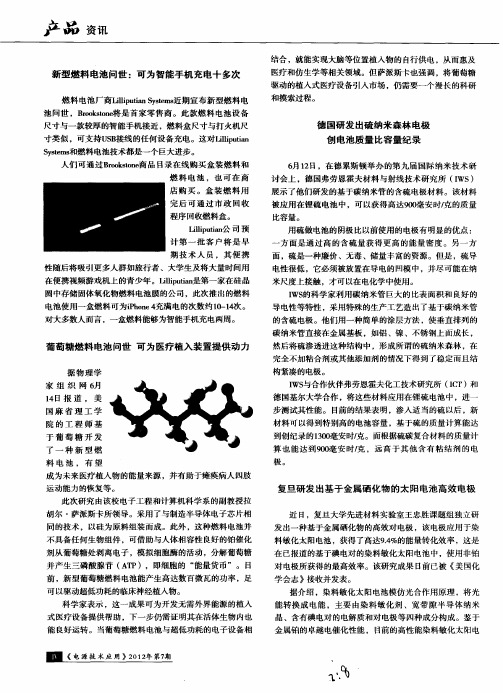
式 医疗设 备提供帮助 ,下一步仍需证明其在活体 生物 内也 能 良好运转 。当葡萄糖燃 料电池与超低功耗 的电子设 备相
《电 源 技 术 应 用 》2 2 - 期 01 # 第7
金属铂的卓越 电催化性 能 ,目前的高性能染 料敏 化太 阳电
米尺度上接触 ,才可以在 电化学 中使用 。
I 的科学家利 用碳纳米管 巨大 的比表面积和 良好 的 WS
导 电性等特性 ,采用特殊 的生产工艺造 出了基 于碳 纳米管 的含硫 电极 。他 们用一种简单 的涂层方法 ,使垂直排列 的
碳纳米管直 接在金属基板 ,如铝 、镍 、不 锈钢上面成长 ,
’
镀有金属 铂的导 电玻璃 作为对 电极 。但 由于铂 的储量低 、价格高 ,极 大地限制 了染料敏化太 阳电池 的大 规模 生产和应用 。因此 ,研发低成 本 、高效 的非铂对 电极
一
集 成 电路 、太 阳能 电池 、场发 射 源 、能量 耗散 纤维 等领
被应用 在锂硫 电池中 ,可 以获得 高达9 o 0 毫安时/ 的质量 克 比容量 。 用硫做 电池的阴极 比以前使用 的电极有明显的优点 :
一
寸类似 ,可支持 U B S 接线 的任何设 备充 电。这对 Llp t n iiui l a
S s m 和燃料电池技术都是一个巨大进 步。 yt s e
尺寸与一款较厚 的智能手机接 近 ,燃料盒尺寸与 打火 机尺
医疗和仿生 学等相关 领域 。但 萨派斯卡 也强调 ,将葡 萄糖 驱动 的植入式 医疗设 备引入市场 ,仍需 要一个漫 长的科研
和摸索过程 。
德 国研 发 出硫 纳 米森 林 电极 创 电池 质量 比容量 纪录
中科院携手浙大研制成功硅基导模量子集成光学芯片
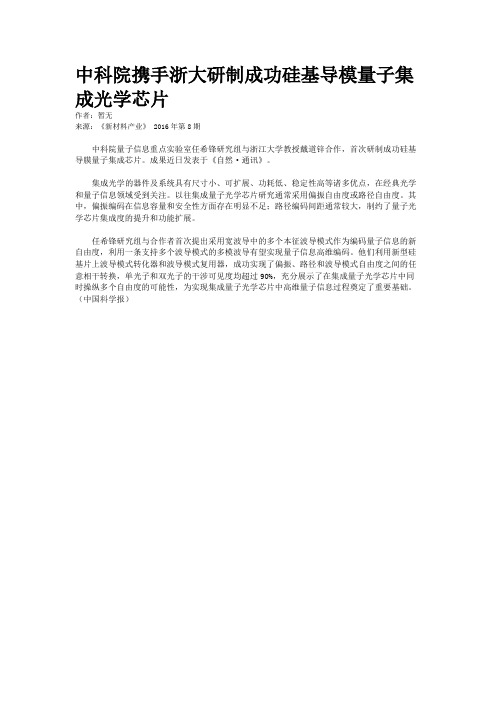
中科院携手浙大研制成功硅基导模量子集成光学芯片
作者:暂无
来源:《新材料产业》 2016年第8期
中科院量子信息重点实验室任希锋研究组与浙江大学教授戴道锌合作,首次研制成功硅基导膜量子集成芯片。
成果近日发表于《自然·通讯》。
集成光学的器件及系统具有尺寸小、可扩展、功耗低、稳定性高等诸多优点,在经典光学和量子信息领域受到关注。
以往集成量子光学芯片研究通常采用偏振自由度或路径自由度。
其中,偏振编码在信息容量和安全性方面存在明显不足;路径编码间距通常较大,制约了量子光学芯片集成度的提升和功能扩展。
任希锋研究组与合作者首次提出采用宽波导中的多个本征波导模式作为编码量子信息的新自由度,利用一条支持多个波导模式的多模波导有望实现量子信息高维编码。
他们利用新型硅基片上波导模式转化器和波导模式复用器,成功实现了偏振、路径和波导模式自由度之间的任意相干转换,单光子和双光子的干涉可见度均超过90%,充分展示了在集成量子光学芯片中同时操纵多个自由度的可能性,为实现集成量子光学芯片中高维量子信息过程奠定了重要基础。
(中国科学报)。
深度学习的多视角三维重建技术综述

深度学习的多视角三维重建技术综述目录一、内容概览 (2)1.1 背景与意义 (2)1.2 国内外研究现状 (3)1.3 研究内容与方法 (5)二、基于单目图像的三维重建技术 (6)2.1 基于特征匹配的三维重建 (7)2.1.1 SIFT与SURF算法 (8)2.1.2 PCA与LDA算法 (10)2.2 基于多视图立体视觉的三维重建 (11)2.3 基于深度学习的三维重建 (12)2.3.1 立体卷积网络 (14)2.3.2 多视图几何网络 (15)三、基于双目图像的三维重建技术 (17)3.1 双目立体视觉原理 (19)3.2 基于特征匹配的双目三维重建 (20)3.3 基于深度学习的双目三维重建 (21)3.3.1 双目卷积网络 (22)3.3.2 GANbased双目三维重建 (23)四、基于多视角图像的三维重建技术 (25)4.1 多视角几何关系 (26)4.2 基于特征匹配的多视角三维重建 (27)4.2.1 ORB特征在多视角场景中的应用 (28)4.2.2 ALOHA算法在多视角场景中的应用 (29)4.3 基于深度学习的多视角三维重建 (30)4.3.1 三维卷积网络(3DCNN)在多视角场景中的应用 (32)4.3.2 注意力机制在多视角场景中的应用 (33)五、三维重建技术在深度学习中的应用 (35)5.1 三维形状描述与识别 (36)5.2 三维物体检测与跟踪 (37)5.3 三维场景理解与渲染 (39)六、结论与展望 (40)6.1 研究成果总结 (41)6.2 现有方法的局限性 (42)6.3 未来发展方向与挑战 (44)一、内容概览多视角数据采集与处理:分析多视角三维重建的关键技术,如相机标定、图像配准、点云配准等,以及如何利用深度学习方法提高数据采集和处理的效率。
深度学习模型与算法:详细介绍深度学习在多视角三维重建中的应用,包括卷积神经网络(CNN)、循环神经网络(RNN)、生成对抗网络(GAN)等,以及这些模型在多视角三维重建任务中的优势和局限性。
二硫化钼光响应

二硫化钼光响应
二硫化钼是一种具有高开关比、合适带隙、较强稳定性的过渡金属氧化物,被认为是下一代纳米光电子器件的有利竞争者。
关于二硫化钼光响应的研究有:
-武汉大学物理科学与技术学院肖湘衡教授课题组利用离子注入技术在二氧化硅衬底上构筑杂质元素埋层,随后通过化学气相沉积(CVD)工艺成功实现了薄层二维材料的大面积无损精确掺杂,并有效提高了其光响应速率。
-西安交通大学电子科学与工程学院电子陶瓷与器件教育部重点实验室任巍教授和牛刚教授团队利用机械剥离获得少层二硫化钼材料制备具有背栅结构和纳米级沟道长度的光电晶体管,实现了较高探测率(>10^13 Jones)和响应率(>10^3 A/W)。
探索记忆电阻 创新人工智能——记北京大学信息科学技术学院微纳电子学研究院助理研究员黄鹏
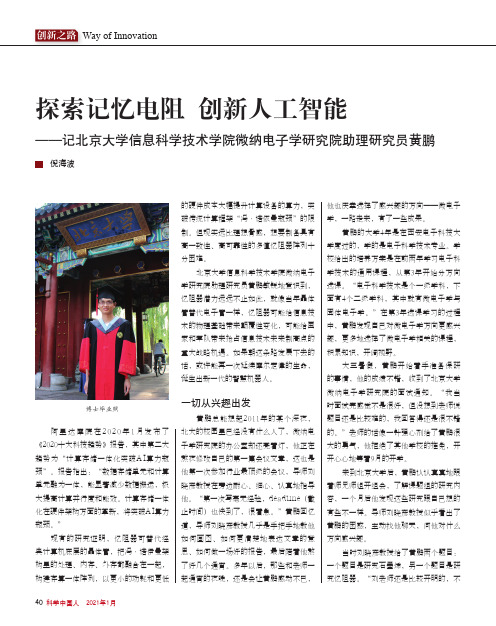
40 科学中国人 2021年1月创新之路Way of Innovation探索记忆电阻 创新人工智能——记北京大学信息科学技术学院微纳电子学研究院助理研究员黄鹏 倪海波阿里达摩院在2020年1月发布了《2020十大科技趋势》报告,其中第二大趋势为“计算存储一体化突破A I算力瓶颈”。
报告指出:“数据存储单元和计算单元融为一体,能显著减少数据搬运,极大提高计算并行度和能效。
计算存储一体化在硬件架构方面的革新,将突破A I算力瓶颈。
”现有的研究证明,忆阻器可替代经典计算机底层的晶体管,把冯·诺伊曼架构里的处理、内存、外存都融合在一起,构建存算一体阵列,以更小的功耗和更低的硬件成本大幅提升计算设备的算力,突破传统计算框架“冯·诺依曼瓶颈”的限制。
但现实远比理想骨感,想要制备具有高一致性、高可靠性的多值忆阻器阵列十分困难。
北京大学信息科学技术学院微纳电子学研究院助理研究员黄鹏敏锐地意识到,忆阻器潜力远远不止如此,就像当年晶体管替代电子管一样,忆阻器可能给信息技术的物理基础带来颠覆性变化,可能给国家和军队带来抢占信息技术未来制高点的重大战略机遇。
如果朝这条路发展下去的话,或许能再一次延续摩尔定律的生命,诞生出新一代的智慧机器人。
一切从兴趣出发黄鹏总能想起2011年的某个深夜,北大的校园里已经没有什么人了,微纳电子学研究院的办公室却还亮着灯,他正在熬夜修改自己的第一篇会议文章,这也是他第一次参加行业最顶级的会议,导师刘晓彦教授在旁边耐心、细心、认真地指导他。
“第一次写毫无经验,deadline(截止时间)也快到了,很着急。
”黄鹏回忆道,导师刘晓彦教授几乎是手把手地教他如何画图、如何更清楚地表达文章的意思、如何做一场好的报告,最后陪着他熬了好几个通宵。
多年以后,那些和老师一起通宵的夜晚,还是会让黄鹏感动不已,他也庆幸选择了感兴趣的方向——微电子学,一路走来,有了一些成果。
黄鹏的大学4年是在西安电子科技大学度过的,学的是电子科学技术专业。
基于智能脉冲喷涂的高效叠层太阳电池制造关键技术开发
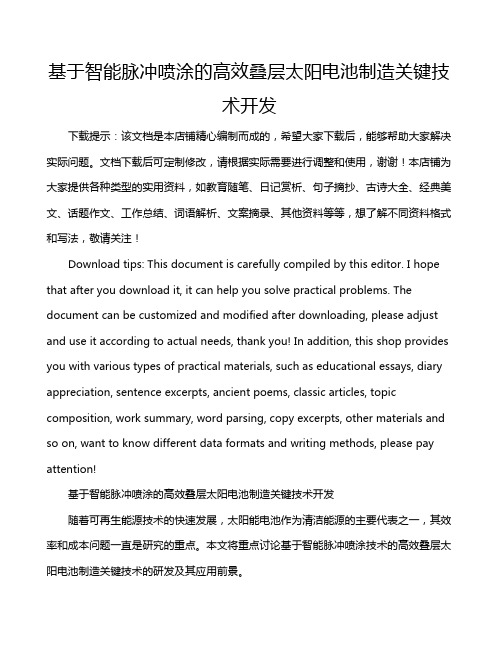
基于智能脉冲喷涂的高效叠层太阳电池制造关键技术开发下载提示:该文档是本店铺精心编制而成的,希望大家下载后,能够帮助大家解决实际问题。
文档下载后可定制修改,请根据实际需要进行调整和使用,谢谢!本店铺为大家提供各种类型的实用资料,如教育随笔、日记赏析、句子摘抄、古诗大全、经典美文、话题作文、工作总结、词语解析、文案摘录、其他资料等等,想了解不同资料格式和写法,敬请关注!Download tips: This document is carefully compiled by this editor. I hope that after you download it, it can help you solve practical problems. The document can be customized and modified after downloading, please adjust and use it according to actual needs, thank you! In addition, this shop provides you with various types of practical materials, such as educational essays, diary appreciation, sentence excerpts, ancient poems, classic articles, topic composition, work summary, word parsing, copy excerpts, other materials and so on, want to know different data formats and writing methods, please pay attention!基于智能脉冲喷涂的高效叠层太阳电池制造关键技术开发随着可再生能源技术的快速发展,太阳能电池作为清洁能源的主要代表之一,其效率和成本问题一直是研究的重点。
一种柔性方硅阵列探测器及其制备方法[发明专利]
![一种柔性方硅阵列探测器及其制备方法[发明专利]](https://img.taocdn.com/s3/m/4a3224f181eb6294dd88d0d233d4b14e85243e13.png)
专利名称:一种柔性方硅阵列探测器及其制备方法专利类型:发明专利
发明人:李宇航,樊宣青,陈嘉昀,崔馨博
申请号:CN202111592542.9
申请日:20211223
公开号:CN114420680A
公开日:
20220429
专利内容由知识产权出版社提供
摘要:本发明涉及一种柔性方硅阵列探测器及其制备方法,柔性方硅阵列探测器包括集成电路板和柔性方硅阵列,集成电路板上设置有高能粒子感应元件,高能粒子感应元件位于集成电路板和柔性方硅阵列之间,高能粒子感应元件与柔性方硅阵列中的方硅芯片的位置重合,探测器中的柔性方硅阵列采用“岛‑桥”结构,使探测器能紧密包覆在电子器件上,真正实现对电子器件高能粒子辐射的精确探测。
申请人:北京航空航天大学宁波创新研究院
地址:315832 浙江省宁波市北仑区梅山街道康达路三创基地一期7号组团
国籍:CN
代理机构:北京中创云知识产权代理事务所(普通合伙)
代理人:肖佳
更多信息请下载全文后查看。
清华大学段海新团队获ACM计算机与通信安全会议杰出论文奖

清华大学段海新团队获ACM计算机与通信安全会议杰出论文
奖
佚名
【期刊名称】《信息网络安全》
【年(卷),期】2024()1
【摘要】2023年11月26日至30日,“第三十届ACM计算机与通信安全会议(ACM Conference on Computer and Communications Security,CCS)”在丹麦哥本哈根举行。
清华大学网络研究院段海新教授带领的网络和系统安全研究团队发表的论文“Silence is not Golden:Disrupting the Load Balancing of Authoritative DNS Servers”获得杰出论文奖。
论文第一作者为清华大学网络研究院2020级博士生张丰露。
这是段海新教授团队关于域名服务系统安全研究成果在CCS会议上获得的第二个杰出论文奖。
【总页数】1页(P35-35)
【正文语种】中文
【中图分类】TP3
【相关文献】
1.关注中国的网络安全--访清华大学中国教育和科研计算机网络紧急响应组段海新博士
2.清华陈建军等关于CDN攻击的研究获杰出论文奖NDSS'16会议在美国举行
3.清华大学信息学院刘云浩、李振华团队获ACM SIGCOMM 2021最佳学生论文奖
4.清华大学杨家海团队获第三十届网络与分布式系统安全研讨会(NDSS)杰出论文奖
5.清华大学团队荣获USENIX Security杰出论文奖
因版权原因,仅展示原文概要,查看原文内容请购买。
- 1、下载文档前请自行甄别文档内容的完整性,平台不提供额外的编辑、内容补充、找答案等附加服务。
- 2、"仅部分预览"的文档,不可在线预览部分如存在完整性等问题,可反馈申请退款(可完整预览的文档不适用该条件!)。
- 3、如文档侵犯您的权益,请联系客服反馈,我们会尽快为您处理(人工客服工作时间:9:00-18:30)。
Efficient GSVD Based Multi-user MIMO Linear Precoding and Antenna Selection SchemeJaehyun Park∗,Joohwan Chun∗,and Haesun Park†∗Division of Electrical Engineering,School of Electrical Engineering and Computer ScienceKorea Advanced Institute of Science and Technology,Daejeon,KoreaEmail:{jhpark,chun}@sclab.kaist.ac.kr†Division of Computational Science and Engineering,College of ComputingGeorgia Institute of Technology,Atlanta,Georgia30332Email:hpark@Abstract—We present a multi-user multiple-input multiple-output(MU-MIMO)precoding scheme utilizing generalized sin-gular value decomposition(GSVD).Our work is motivated by the precoding scheme developed by Sadek,Tarighat,and Sayed that maximizes the signal to leakage and noise ratio(SLNR),in which the precoding weight is obtained by the generalized eigenvalue decomposition(GEVD).However,the covariance matrix utilized in GEVD becomes close to be singular as the signal to noise ratio goes high.To improve the numerical accuracy,a GSVD based algorithm is exploited in this paper and a novel method is derived to compute the precoding weights for multiple users by removing redundant computational loads rather than repeating the GSVD algorithm for each user.In addition,we propose a technique to speed up the GSVD based precoding algorithm by preprocessing using the QR decomposition.Finally,to improve the system performance,the antenna selection scheme using GSVD is also proposed which does not require the exhaustive search to choose the active antennas.I.I NTRODUCTIONRecently,multiple-input multiple-output(MIMO)broad-casting scenario with the multiple antennas at the transmit side and the multi-user(MU)at the receive side has been studied extensively since the higher performance can be achieved by simply increasing the number of antennas at the transmit side [1].Previously,due to the simplicity in implementation and good performance,several linear precoding schemes have been proposed to suppress the cochannel interference[2]–[4].In [4],an iterative method is developed to compute precoding weights.In[2,3],a closed-form solution is proposed by maximizing the signal to leakage and noise ratio(SLNR)[2,3] (or the signal to interference and noise ratio at the transmitter (Tx-SINR)in[5]).SLNR maximizing precoding weight can be computed by the generalized eigenvalue decomposition (GEVD)of two covariance matrices of each user’s channel and interferences’channel plus noise.However computation of GEVD may become numerically unstable because the covariance matrix of interference and noise becomes closer to be singular as the signal to noise ratio(SNR)goes high. In this paper,we propose a GSVD based precoding algo-rithm using generalized singular value decomposition(GSVD) for MU-MIMO system,which produces numerically better and computationally fast solution.The GSVD algorithm developed in[6,7]resolves the singularity problem in the linear discrim-inant analysis(LDA)for high dimensional(or undersampled) data successfully.By modifying the LDA/GSVD algorithm for MU-MIMO,we show how the singularity in covariance matrix can be handled properly.In addition,we show how the GSVD algorithm can be utilized to compute the precoding weights for all users rather than repeating the GSVD for each user.Finally,we also propose the method to speed up our precoding algorithm when the number of transmit antennas is greater than the sum of all users’receive antennas,in which the dimension of precoding weight is reduced from preprocessing by the QR decomposition(QRD)[8].In[3],when applying the SLNR criterion to design the linear MU-MIMO precoding, the probability density function(PDF)of SLNR and its mean are derived for the various numbers of receive antennas,which shows that the SLNR decreases when the number of receive antennas increases.Similarly,the performance of the proposed scheme may be degraded when the number of receive antennas increases since the increase in the number of the receive antennas causes the interferences to other users.Accordingly, in this paper,the antenna selection scheme using GSVD is developed to reduce the number of the active antennas for each user.The rest of this paper is organized as follows.In Section II the system model considered in this paper is described and in Section III SLNR maximizing precoding scheme is reviewed briefly.In Section IV we review the GSVD due to Paige and Saunders[9].We then propose MU-MIMO precoding scheme based on GSVD for single user and modify it for multiple users.Furthermore,the fast GSVD based precoding algorithms using QRD is developed.In Section V the antenna selection scheme using GSVD is proposed.Several simulation results, showing the bit error rate(BER)curves for various parameters, are given in Section VI.Concluding remarks are made in Section VII.The superscripts in A T,A H,and A†denote,respectively, the transposition,conjugate transposition,and pseudo-inverse of a matrix A. A F and tr(A)denote Frobenious norm and trace of A,respectively.I K and0K,M denote a K×K identity matrix and a zero K×M matrix,respectively. diag{a1,...,a N}denotes a diagonal matrix with diagonal978-1-4244-3435-0/09/$25.00 ©2009 IEEEelements{a1,...,a N}.Finally A(i:j,k:l)denotes a submatrix of A with elements from the i th row to the j th row and from the k th column to the l th column.II.S YSTEM MODELWe consider N transmit antennas at base station and K users with M receive antennas per each user.The channel is assumed to be frequencyflat fading and constant over a transmission frame duration.The received signal for the k th user can then be represented asy k=H k x+n k,H k∈C M ×N,x∈C N×1(1)where y k=y(k)1,...,y(k)MT.The precoded transmit signalfor all users is denoted as x and n k is the complex whiteGaussian noise vector having a varianceσ2.Here,entries of H k are assumed to be complex Gaussian variables with zero-mean and unit-variance.Concatenating the received signals ofall users,we can rewrite(1)as:y=Hx+n,(2)where y=⎡⎢⎣y1...y K⎤⎥⎦,n=⎡⎢⎣n1...n K⎤⎥⎦,H=⎡⎢⎣H1...H K⎤⎥⎦,(3)and M=KM .Since we only consider the linear precoding scheme,the precoded signal x can be written as:x=Kk=1W k s k,W k∈C N×m k,s k∈C m k×1(4)where s k and W k are,respectively,the data stream vector and the precoding matrix for the k th user with m k≤M .Here we assume that s k and W k are normalized as follows:E[s k s H k]=I m k,tr(W k W H k)=1,for k=1,...,K.(5) III.SLNR MAXIMIZING P RECODING SCHEMEIn this section,we briefly review the precoding scheme to maximize SLNR[2],which gives the closed form solution. The SLNR of each user is defined as the ratio between the desired signal power summation for each user and the interference introduced to the other users and noise power summation as follows[2]:J(W k)=H k W k 2FKi=1,i=kH i W k 2F+M σ2=trW H k H H k H k W ktrW H kM σ2I N+˜H H k˜H kW k,(6)with the constraint that tr(W H k W k)=1.Here,˜H k is defined as[H1;...;H k−1;H k+1;...;H K](∈C(M−M )×N),which is the sub-channel of all users except the k th user.Since M σ2I N+˜H H k˜H k is symmetric positive definite, there exists a nonsingular matrix X k∈C N×N such that[10] X H k H H k H k X k=Λk=Diag{λ1,k,λ2,k,...,λN,k}X H kM σ2I N+˜H H k˜H kX k=I N,(7) withλ1,k≥λ2,k≥...≥λN,k≥0.Therefore,the equation(6)can be rewritten asJ(W k)=trW H k X−H kΛk X−1k W ktrW H k X−H k X−1k W k.(8)We then set˜W k=X−1k W k and substitute its thin SVD(= U kΣk V H k,where U k∈C N×m k andΣk,V k∈C m k×m k) into(8)asJ(W k)=trV kΣH k U H kΛk U kΣk V H ktrV kΣH k U H k U kΣk V H k=trΣH k U H kΛk U kΣkmki=1d2i,k,(9)where d i,k is the i th diagonal element ofΣk.Note that J(W k)is independent of V k.By using the approach in[2], we can obtain U k maximizing J(W k)asU k=I mk0N−mk,m k,(10)and{d1,k,...,d mk,k}={1,...,1},so that W k is given by the first m k rows of X H k multiplied by the scaling factor to satisfy tr(W k W H k)=m k,i.e.,[2]W k=αk G k,G k∈C N×m k,(11) whereαk is proper scaling factor for the k th user and the columns of G k are the eigenvectors corresponding to the m k maximum generalized eigenvalues of the matrix pairH H k H k,M σ2I N+˜H H k˜H k.The solution of the above method tends to be numerically less accurate especially whenM σ2I N+˜H H k˜H kbecomes close to be singular at high SNR.In the next section we introduce the GSVD developed by Paige and Saunders[9],which is applied to circumvent the nonsingularity problem in LDA[6,7]and propose a precoding scheme based on GSVD that offers better numerical stability. The idea is based on the observation that(11)can be obtained by the GSVD of the matrix pair(H k,[˜H k;√M σI N]),sinceM σ2I N+˜H H k˜H k=˜H H k√M σI N˜H k√M σI N.(12) IV.G ENERALIZED S INGULAR V ALUE D ECOMPOSITION AND MU-MIMO PRECODING SCHEME BASED ON GSVD A.Generalized Singular Value DecompositionAlthough there are several kinds of GSVD formulations[10, 11],we review the GSVD algorithm due to Paige and Saunders [9],which has a more complicated but a less restrictive form. Theorem1:[Paige and Saunders’s GSVD]Suppose any two matrices K b∈C m×n and K w∈C p×n,with the same number of columns,are given.Then there exist unitarymatrices U ∈C m ×n ,V ∈C p ×p ,and a nonsingular matrix X ∈C n ×n such thatU H K b X =(Σb ,0)and V H K w X =(Σw ,0),(13)where t =rank K bK wandΣb m ×t =⎛⎝I r D b 0b ⎞⎠,Σw p ×t=⎛⎝0wD w I t −r −s ⎞⎠,where r =rank K bK w −rank (K w ),s =rank (K b )+rank (K w )−rankK bK w,and the matrices 0b ∈R (m −r −s )×(t −r −s )and 0w ∈R (p −t +r )×r are zero ma-trices with possibly no rows or no columns.The ma-trices,D b =diag (αr +1,...,αr +s )∈R s ×s and D w =diag (βr +1,...,βr +s )∈R s ×s satisfy 1>αr +1≥...≥αr +s >0,0<βr +1≤...≤βr +s <1,and α2i+β2i =1for i =r +1,...,r +s . According to the constructive proof of Theorem 1by Paige and Saunders,the computation of the GSVD starts with the complete orthogonal decomposition [10]of K ≡ K bK w,i.e.,P HK KQ K = R K 000,(14)where P K ∈C (m +p )×(m +p )and Q K ∈C n ×n are unitary and R K ∈C t ×t is nonsingular.It then proceeds with the SVDs of submatrices of P K ,partitioned asP K = P 11P 12P 21P 22 ,P 11∈C m ×t ,P 21∈C p ×t .(15)Note that P 11 2≤1and hence the singular values of P 11do not exceed one.We can then write the SVD of P 11as U H P 11W =Σb ,where U ∈C m ×m and W ∈C t ×t are unitary and Σb has the form shown in (13).Then,P 21W is decomposed as P 21W =VL ,where V ∈C p ×p is unitary and L ∈C p ×t is lower triangular which can be obtainedin the similar way as QRD.Since P 11P 21 has orthonormalcolumns,the columns ofΣbLshould be also orthonormal,which implies L =Σw .Using these results,we can get the following equation:K bK w Q K =P 11R K 0P 21R K 0 = UΣb W H R K 0VΣw W H R K 0.(16)Here,the first t columns of X are given by Q K R −1K W.Defining αi =1,βi =0for i =1,...,r and αi =0,βi =1for i =r +s +1,...,t ,we can easily obtainβ2iK H b K b x i =α2i K H w K w x i ,(17)Algorithm 1MU-MIMO precoding based on GSVD for single user Given a channel H =[H 1;...;H K ]for K users as in (3),this algorithmcomputes the precoding matrix W k ∈C N ×m k for the k th user.1)Set K k = H k ;˜Hk ;√M σI N ∈C (M +N )×N .2)Compute the reduced QRD of K k ,i.e.,P H k K k =R k ,where P k ∈C(M +N )×N has orthonormal columns and R k ∈C N ×N is upper triangular.3)Compute ˇVk from the SVD of P k (1:M ,1:N ),i.e.,ˇU H kP k (1:M ,1:N )ˇV k =ˇΣk .4)Solve the triangular system R k W k =ˇVk (:,1:m k )for W k ,which is the precoding matrix for the k th user.for 1≤i ≤t ,where x i represents the i th column of X .Here,the columns of X are the generalized right singular vectors for the matrix pair (K b ,K w ).B.MU-MIMO precoding scheme based on GSVDPrecoding scheme for single user:Since x i in (17)is a gen-eralized eigenvector for the matrix pair (K H b K b ,K Hw K w ),we can find the precoding matrix by applying the GSVD algorithm to the matrix pair (H k ,[˜H k ;√M σI N ]).The GSVD algorithmfor the precoding matrix is summarized in Algorithm 1.Since rank (K k )is always full due to the term √M σI N in K k ,the reduced QRD in Step 2of Algorithm 1is utilized as the rank revealing decomposition in (14),which is similar to the regularized LDA/GSVD [8]and makes the algorithm much simpler as in [8].Algorithm 1can be applied without any restriction on M or N .Algorithm 1requires computation of one QRD (Step 2),one SVD of M ×N matrix (Step 3),and m k backward substitutions (Step 4)and overall takes much less computa-tional complexities than the GEVD of an N ×N matrix pair.By utilizing the GSVD based precoding scheme described above,we can deal with better-conditioned matrices 1and avoid numerical errors during the matrix multiplications.Precoding scheme for multiple users:For total K users in the system we need to repeat Algorithm 1K times to compute the precoding matrices.To reduce its computational loads,the follwoing property is useful:Property 1:Let A ∈C M ×N ,M >N ,have a QRD as:A =Q 1R ,Q 1∈C M ×N ,R ∈C N ×N(18)where Q H 1Q 1=I N and R is upper triangular.The QRD ofUA ,where U ∈C M ×M is unitary,is then given byUA=Q R ,(19)where Q =UQ 1D and R =DR ,and D =diag {±1,...,±1}∈R N ×N .Even though the QRD of UA is not unique,by setting D =I N ,it can be acheived that R =R and Q =UQ 1,which is a key factor in the following derivation.Suppose that the precoding matrix W 1for the first user is computed by Algorithm 1.The matrix K 1in Step 2is then1Generally,the condition number of A H A is the square of that of A .given byK 1=⎡⎣H 1˜H 1√M σI N⎤⎦= H √M σI N=P 1R 1,(20)where P 1∈C (M +N )×N has orthonormal columns and R 1∈C N ×N is upper triangular.For the second user,K 2=⎡⎣H 2˜H 2√M σI N ⎤⎦=S 2,1K 1=P 2R 2,(21)where P 2∈C (M +N )×N has orthonormal columns and R 2∈C N ×N is upper triangular,andS 2,1=⎡⎣0M ×MI M I M0M ×M I M +N −2M ⎤⎦.(22)It can then be easily derived that:R 2=R 1,(23)P 2(1:M,1:N )=P 1(M+1:2M,1:N ).(24)Therefore,given the QRD of K 1,it is unnecessary to computethe QRD for the second user in Step 2of Algorithm 1.Similarly,for the k th user,R k=R 1,P k (1:M ,1:N )=P 1((k −1)M +1:k M ,1:N ).Based on these observations,an efficient precoding algorithm for multiple users can be developed,which is summarized in Algorithm 2.Note that Algorithm 2requires only one QRD in Step 2for K users,thus eliminating the redundant computational complexities when computing the precoding matrices of K users by employing Algorithm 1repeatedly.The interesting thing is that,if the channel plus noise matrix K in Step 2of Algorithm 2is not transformed into the unitary matrix P by R −1,Algorithm 2gives the equivalent precoding weight to the optimal solution for the single user MIMO precoding.C.MU-MIMO precoding scheme based on GSVD with QRD preprocessingThe computational complexity of the precoding scheme proposed in Section IV increases as the number of transmit antenna (N )increases,which is undesirable when M ≤N .2Accordingly we propose a fast MU-MIMO precoding scheme based on GSVD using QRD preprocessing,where QRD reduces the dimension of the precoding weight during the computing process.Since Q H 1HH=R by using reduced QRD,where Q 1∈CN ×M,M ≤N ,the N -dimensional channel vectors H H for users can be represented in an M -dimensional space as R by the dimension reducing transformation Q H 1.2Itcould often arise in general MU-MIMO problem.In addition,the case of M >N can be sometimes forced to M ≤N by scheduling and random beamforming schemes [12,13].Algorithm 2MU-MIMO precoding algorithm based on GSVD for multiple usersGiven a channel H =[H 1;...;H K ]for K users as in (3),this algorithmcomputes the precoding matrix W k ∈C N ×m k for all users.1)Set K = H ;√M σI N ∈C (M +N )×N .2)Compute the reduced QRD of K ,i.e.,P H K =R ,where P ∈C (M +N )×N has orthonormal columns and R ∈C N ×N is upper triangular.3)For k =1:K3.1)Compute ˇVk from the SVD of P ((k −1)M +1:kM ,1:N ),i.e.,ˇU H kP ((k −1)M +1:kM ,1:N )ˇV k =ˇΣk .4)Solve the triangular system R W k =ˇVk (:,1:m k )for W k ,k =1,...,K .Suppose we find the weight matrix ˆWk for the dimension reduced channel matrix R .The cost function can then be written as:J ˆWk =tr ˆW H k R k R H kˆW ktr ˆW H k M σ2I M +˜R k ˜R H k ˆW k,(25)where R k =Q H 1H H k and ˜R k =Q H 1˜H H k ,which are given bythe corresponding columns of R .SinceM σ2I M +˜R k ˜R H k =Q H 1 M σ2I N +˜H H k ˜H kQ 1,(25)can be rewritten asJ ˆW k =tr ˆW H k Q H 1H H k H k Q 1ˆW ktr ˆW H k Q H 1 M σ2I N +˜H H k ˜H k Q 1ˆW k ,(26)which means that W k =Q 1ˆWk provides the solution for (6).The above derivation shows that the reduced QRD preprocessing to H H enables us to manipulate the matrices of smaller size M ×M rather than M ×N in computing the GSVD based precoding weights.In addition,the above prerpocessing can work together with the efficient algorithm for multiple users discussed in Secction IV-B.Based on these results,the new algorithm is summarized in Algorithm 3.Note that the SVDs of M ×N matrices for k =1,...,K in Step 3of Algorithm 1or Algorithm 2,the dominant part of the computational complexities,are replaced with those of much smaller M ×M matrices.To detect symbol s k from (1),we use the simplematched filter W H kH H k as in [2]because W H k H H k H k W k =diag {λ1,k ,...,λm k ,k }.V.A NTENNA SELECTION SCHEME BASED ON GSVD Since the increase in the number of the receive antennas causes the interferences to other users,the performance of the proposed scheme could be affected by the number of receive antennas.In [3],the PDF of SLNR is characterized for the number of receive antennas which concludes that the increase in the number of the active antennas reduces the SLNR.Based on this observation,we propose the antenna selection algorithm to reduce the number of the active antennas.Since M ≥m k ,the minimum required number of the receive antenna for k th user is m k .Therefore,the proposed antennaAlgorithm 3MU-MIMO precoding algorithm based on GSVD withQRD preprocessing for multiple users Given a channel H =[H 1;...;H K ]for K users as in (3)and M ≤N ,this algorithm computes the precoding matrix W k ∈C N ×m k for all users.1)Compute the reduced QRD of H H ,i.e,H H =Q 1R ,where Q 1has orthonormal columns and R is upper triangular.2)Set ˆK= R H ;√M σI M ∈C 2M ×M .3)Compute the reduced QRD of ˆK,i.e.,ˆP T ˆK =ˆR ,where ˆP has orthonormal columns and ˆRis upper triangular.4)For k =1:K4.1)Compute ˇV k from the SVD of ˆP ((k −1)M +1:kM ,1:M ),i.e.,ˇU T k ˆP ((k −1)M +1:kM ,1:M )ˇV k =ˇΣ k.5)Solve the triangular system ˆR ˆW k =ˇV k(:,1:m k )for ˆW k .6)W k =Q 1ˆWk for k =1,...,K .selection algorithm chooses m k active antennas for each user.For simplicity,we assume that m k =m for all k .Let ˇΣk in Step 3of Algorithm 2as ˇΣk =diag {σ(1)k ,...,σ(M )k },where σ(1)k ≥...≥σ(M )k .From Theorem 1,the generalized eigenvalues of the matrix pair H H k H k , M σ2I N +˜H H k˜H k are then given as:λ(i )k=(σ(i )k )21−(σ(i )k )2,(27)which implies that,for a large σ(i )k ,λ(i )k also becomes large.Therefore,it is natural that the data streams of the k th user are precoded by the linear combination of the first m k columns of ˇVk in Step 4of Algorithm 2,since the SLNR of the i th stream of k th user is λ(i )k .However,the data streams leak throughthe transformed channel corresponding to σ(m +1)k ,...,σ(M )k causing the interferences,which can be represented as:E k =σ(m +1)kˇUk (:,m +1)ˇV k (:,m +1)H +...+σ(M )kˇU k (:,M )ˇVk (:,M )H .(28)Therefore,the antennas corresponding to the rows of P ((k −1)M +1:kM ,1:N )geometrically close to E k may yield more interferences and need to be deactivated.That is,let C (k )i = P E k P ((k −1)M +i,1:N )H 2for i =1,...,M ,where P E k is the projection matrix given by P E k =E †k E k ,and define C (k )(1),C (k )(2),...,C (k )(M )be the ascending ordering of C (k )1,C (k )2,...,C (k )M .The active antenna index set is then given as:I (k )Active ={j |C (k )j=C (k )(i ),i =1,...,m ,m ≤M }.(29)Note that the proposed selection method requires mainly K additional SVD computations.On the other hand,the exhaus-tive search needs (M C m )K GSVD precoding computations which results in a prohibitive complexity.VI.S IMULATION R ESULTSWe assess performance of the proposed MU-MIMO precod-ing scheme based on GSVD through Monte-Carlo simulationfor different conditions.Each entry of MIMO channel H is generated according to complex white Gaussian distribution with zero-mean and unit-variance.In all simulations,each user’s data are modulated by quadrature phase shift keying (QPSK)signaling and for the matrix decompositions such as QRD,GEVD,and SVD,MATLAB built-in functions are used.Fig.1shows the BER performance of the proposed scheme for N =10,K =4,M =2,and m i =2for i =1,2,3,4.For the reference of comparison we also evaluate the BER performances of the iterative nullspace-directed SVD (iterative null SVD)scheme in [4]and the SLNR maximizing precoding scheme in [2].The SLNR maximizing scheme outperforms the iterative null SVD scheme especially at low SNR since the SLNR maximizing scheme considers the noise variance.At low SNR,SLNR maximizing precoding scheme and GSVD based scheme have similar BERs,but,when SNR >0dB,the latter scheme has lower BERs and the difference becomes larger as SNR increases,i.e.,the GSVD based scheme has a steeper BER curve due to the fact that the GEVD in (11)could be computed inaccurately as the SNR goes high.Fig.2shows BER curves for N =12,K =3,M =3,and m i =3for i =1,2,3.Since N >M ,we additionally evaluate the BER for the GSVD based precoding scheme with QRD preprocessing.The figure exhibits that the preprocessing does not affect the BER performance of the GSVD based scheme,having lower BERs than the SLNR maximizing precoding scheme.It is interesting to note that the performance gap between the proposed schemes and the SLNR maximizing scheme is more apparent than the result shown in Fig.1.Therefore,as N ,M ,and m k increase,it tends to be more unstable to compute the precoding weights in (11)resulting the more performance gain of the proposed scheme compared with the SLNR maximizing precoding scheme.Fig.3shows BER curves for N =9,K =3,M =4,and m i =3for i =1,2,3.We can also find that the GSVD based precoding has lower BERs than the SLNR maximizing scheme.However,since M >N causing more interferences to other users,both schemes can not maintain their slopes of BER curves at high SNR.On the other hand,the iterative null SVD has the lowest BERs.Note that,as pointed in [2],the iterative null SVD scheme is orders of magnitude more complex than the SLNR based precoding or GSVD based precoding schemes which provide closed form solutions.From the figure,the proposed antenna selection scheme improves the BERs of the GSVD based precoding scheme close to those of the iterative null SVD scheme.VII.C ONCLUDING REMARKSIn this paper,we have proposed the MU-MIMO precoding scheme based on GSVD to detect multiple users’symbols which has the improved numerical properties and computation-ally efficiency.The precoding weight of our proposed scheme transforms the MU-MIMO channels to maximize the separa-bility of the effective channels among the users in sending the multiple data streams to the multiple users.Moreover,we have shown how the GSVD based precoding scheme forsingle user can be extended to compute the precoding weights for multiple users efficiently rather than repeating the GSVD algorithm for each user.The simulation results demonstrate that the MU-MIMO precoding scheme based on GSVD has good performance gain especially at high SNR where the covariance matrix of interference and noise gets closer to be singular,and that the QRD preprocessing scheme has no performance degradation compared with the GSVD based precoding scheme even while reducing the computational complexities.As a further works,we need to analyze the computational complexity of our proposed schemes comparing with the conventional SLNR maximizing precoding scheme in more detail.A CKNOWLEDGMENTSThis work was supported in part by Brain Korea21,and by the Basic Research Program of Korea Science and Engineering Foundation(KOSEF),under grant No.R01-2007-000-20949-0.The work of the third author was supported in part by the National Science Foundation grants CCF-0728812and CCF-0732318.Any opinions,findings and conclusions or recommendations expressed in this material are those of the authors and do not necessarily reflect the views of the National Science Foundation.R EFERENCES[1] A.Goldsmith,S.A.Jafar,N.Jindal,and S.Vishwanath,“Capacity limitsof MIMO channels,”IEEE Journals on Selected Areas in Communica-tions,vol.21,no.5,pp.684–702,June2003.[2]M.Sadek,A.Tarighat,and A.H.Sayed,“A leakage-based precodingscheme for downlink multi-user MIMO channels,”IEEE transactions on Wireless Communications,vol.6,no.5,pp.1711–1721,May2007.[3]——,“Active antenna selection in multiuser MIMO communications,”IEEE transactions on Signal Processing,vol.55,no.4,pp.1498–1510, Apr.2007.[4]Z.Pan,K.-K.Wong,and T.-S.Ng,“Generalized multiuser orthogonalspace-division multiplexing,”IEEE transaction on Wireless Communi-cations,vol.3,no.6,pp.1969–1973,Nov.2004.[5] D.Gerlach and A.Paulraj,“Adaptive transmitting antenna methods formultipath environments,”in Proc.IEEE GLOBECOM,1994,vol.1,Nov.1994,pp.425–429.[6]P.Howland,M.Jeon,and H.Park,“Structure preserving dimensionreduction for clustered text data based on the generalized singular value decomposition,”SIAM Journal on Matrix Analysis and Applications, vol.25,no.1,pp.165–179,2003.[7]P.Howland and H.Park,“Generalizing discriminant analysis using thegeneralized singular value decomposition,”IEEE transactions on pattern analysis and machine intelligence,vol.26,no.8,pp.995–1006,Aug.2004.[8]H.Park,B.L.Drake,S.Lee,and C.Park,“Fast linear discriminantanalysis using QR decomposition and regularization,”Georgia Institute of Technology,GA,Tech.Rep.GT-CSE-07-21,Mar.2007.[9] C.C.Paige and M.A.Saunders,“Towards a generalized singular valuedecomposition,”SIAM Journal on Numerical Analysis,vol.18,no.3, pp.398–405,June1981.[10]G.H.Golub and C.F.V.Loan,Matrix Computations,3rd ed.Baltimore:Johns Hopkins Univ.Press,1996.[11] C.F.V.Loan,“Generalizing the singular value decomposition,”SIAMJournal on Numerical Analysis,vol.13,no.1,pp.76–83,1976. [12] E.Kim and J.Chun,“Random beamforming in MIMO systems exploit-ing efficient multiuser diversity,”in Proc.IEEE Vehicular Technology Conference,2005,vol.1,May2005,pp.202–205.[13]Z.G.Pan and L.Chen,“Random beamforming based user schedulingfor downlink of multiple antennas system,”in Proc.IEE International Conference on3G and Beyond,2005,Nov.2005,pp.495–499.Fig.1.BER-vs-SNR curves for N=10,K=4,M =2,and m i=2 for i=1,2,3,4.Fig.2.BER-vs-SNR curves for N=12,K=3,M =3,and m i=3 for i=1,2,3.Fig.3.BER-vs-SNR curves for N=9,K=3,M =4,and m i=3for i=1,2,3.。