Learning classes of probabilistic automata
《大学英语》课件
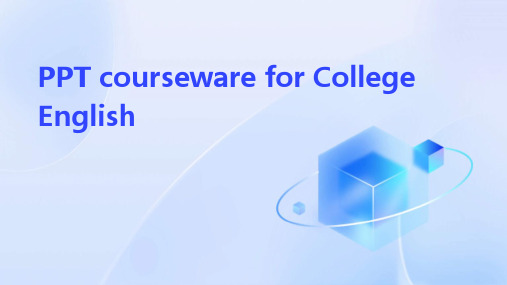
Basic reading comprehension
要点一
要点二
Comparative Analysis
Enhance students to compare and contrast their own culture with those reported in the texts they are studying to enhance their cultural awareness
Advanced listening comprehension
further improve students' ability to understand English at a high level, including complex senses, advanced vocabulary, and suggestive expressions
Translation Techniques
Teach students translation techniques such as literal translation, free translation, and adaptation
Context Understanding
Help students understand the cultural and historical backgrounds of the source texts they are translating to enhance their translation accuracy
PromotingLearnerAutonomyforCollegeEnglishMajorsthr
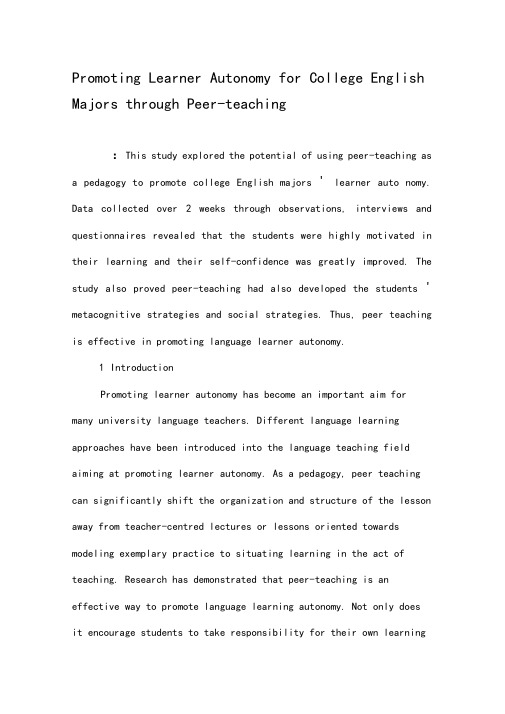
Promoting Learner Autonomy for College English Majors through Peer-teaching:This study explored the potential of using peer-teaching as a pedagogy to promote college English majors ' learner auto nomy. Data collected over 2 weeks through observations, interviews and questionnaires revealed that the students were highly motivated in their learning and their self-confidence was greatly improved. The study also proved peer-teaching had also developed the students ' metacognitive strategies and social strategies. Thus, peer teaching is effective in promoting language learner autonomy.1IntroductionPromoting learner autonomy has become an important aim for many university language teachers. Different language learning approaches have been introduced into the language teaching field aiming at promoting learner autonomy. As a pedagogy, peer teaching can significantly shift the organization and structure of the lesson away from teacher-centred lectures or lessons oriented towards modeling exemplary practice to situating learning in the act of teaching. Research has demonstrated that peer-teaching is aneffective way to promote language learning autonomy. Not only doesit encourage students to take responsibility for their own learningbut it also helps them to improve their motivation, develop their learning strategies. This paper reports on a collaborative self-study aimed at exploring the potential of using peer-teaching as a pedagogy to promote college English majors ' learner autonomy.2Background of the Study2.1Learner AutonomyThe last three decades have witnessed the growing influence of learner autonomy in education. Thus there has been a shift in emphasis in language teaching from a teacher-directed approach to a learner-centred one, along with the perceived need to promote learners' efforts at developing autonomy. Encouraging language learners to become more autonomous in managing their own learning is an appealing notion for several reasons. Onereason is that "learning is more effective when learners are active in the learning process, assuming responsibility for their learning and participating in the decisions which affectit." (Sheerin 1997:56). Particularly for mixed ability groups of students, the promotion of learner independencein language study can provide a meansto meet the differing needs, expectations, and proficiency levels of individual learners. Finally, the need for developing greater autonomy in language learning can be seen as one facet of lifelong learning, in whicheach individual effectively makes decisions about which learning path to take.2.2Brief overview of peer-teachingPeer-teaching refers to the concept of learners teaching other learners. Peer-teaching as a model of instruction became redundant in the nineteenth century when teaching developed as an organized profession but there was a resurgence during the 1960s. A growing interest in improving the standards of achievement in American schools le d to a desire to focus on “individualized instruction ” which was lacking in the teacher -centred style (Topping, 1988: 16; Goodlad, 1998: 2). During the 1970s,particularly in Britain, there was an increase in peer-assisted learning. Many research projects were set up to ascertain the effectiveness of peer-teaching in assisting. The findings of these early research projectshave been very positive. There was a reported gain in cognitive development as well as improvement in self-concepts, social skills and communication skills for both the peer-learner and the peer-helper (Goodlad, 1998: 5; Hill & Topping, 1995: 142-145 ).Research has demonstrated that peer-teaching is aneffective way to promote language learning (Assinder, W, 1991; Catrine Carpenter, 1996;). Many researchers found that learners weremore interactive and exhibited greater variety in their language use in peer-group settings. Assinder(1991) concluded on her experience of implementing peer-teaching with students of English as a foreign Ianguage: “The giving -over of control to the learners […]not only increased motivation, sense of purpose, responsibility and commitment, but allowed for different abilities and learning styles”. Catrine Carpenter 'sstudy (1996) on the value of peer teaching on an advanced level university language course revealed that peer-teaching promoted learner motivation, developed students ' learning strategies and addressed different learning needs. Thus, learners should be given many more chances to ‘peer -work' such as pair work or group work as possible.3Research DesignBased on the assumption that peer-teaching has a positiveeffect on language learning, the aim of this case study is to find out whether peer-teaching can promote English majors ' learner aut onomy, so as to bring about the effective language learning achievements for students.3.1SubjectsThe participants of the present study are 63 sophomore fromtwo classes at Jining university. They are all English majors whoseaverage age is 21.8.3.2InstrumentThe main instrument in this study is a questionnaire which is designed on the basis of the questionnaire of CatrineCarpenter(1996). The questionnaire consists of 3 parts: students ' perception of peer teaching, Students ' perception of being taught by their peer teachers and Students ' perception of being peer -teachers. Responses to the items ranged from 5 to 1 on a 5-point Likert scale: as follows: 5=strongly agree, 4=agree, 3=neutral,2=disagree, 1=strongly disagree. In addition to the questionnaire, data were also collected through interview and classroom observations.3.3ProceduresAt the beginning of the term, students received a detailed description of the peer-teaching task. Then two weeks before the peer-teaching activity, the students were divided into 6 groups consisting of 5-6 students. Meanwhile, the text was also dividedinto several parts and each group was assigned a part to make preparation. After that each group worked cooperatively to study the text, discuss the difficult and key parts, search the internet for relevant materials, prepare the lesson plan and design activities. Then on the teaching day, the peer-teachers from each group gave apresentation, explaining the s and key ideas, interacting with students, providing background information, organizing and managing class activities. After the peer-teaching activity had been carried out for 2 weeks, a questionnaire was administered to the students, aiming at investigating their perception of the activity. The subjects ' responses to each item were summedand mean scores were computed afterwards. After the administration and the initial analysis of the questionnaire data, a face-to-face structured interview was conducted.4Findings and Analysis4.1Studen ts ' perception of peer teachingTable 1.Table 1 shows most students were positive about peer-teaching. Firstly they thought this style of learning interesting and motivating and it could create a lively learning environment. Secondly, they felt peer-teaching gave them incentives to make greater efforts to learn English. In the interview, the interviewees agreed that they felt peer-teaching motivating and useful it also fostered team spirit to perform better, as other teams are assessing their team.4.2Students ' perception of being taught by their peer teachersTable 2The data in table 2 indicate the students had a great passion for peer teaching and they enjoyed the presentation of their peers. They would like to have more of such activities later on. Meanwhile many students admitted they found their deficiency in study in the process of participating in peer-teaching. In the interview the teacher asked the students why they applauded thepeer-teachers. They said that they wanted to show their appreciation and give the peer-teachers encouragement This proved that the students would like to participate in the activity and to be cooperative when peer-teachers were teaching4.3Students ' perception of being peer -teachers Table 3The results below are the average responses from the peer-teachers on questions relating to their feelings about being responsible for teaching. The evaluation of peer- teaching was even more positive than that of being in a peer-taught class. Over 80% of the peer-teachers agreed that they had madefull preparation, spent more time in learning. They found the experience motivating , challenging and useful. What 's more, they felt that theactivity had improved their confidence, developed their organizational skills and oral English. In addition, the teacher observed that learners who were sometimes passive in class teacher-led sessions changed behavior when involved in peer-teaching. It seemed their commitment and competence were triggered by the responsibility invested in them by their peers and the class teacher. In theinterview, some interviewees said they felt more involvedin their study because they had to plan the lesson, make decisions on selecting useful and important lexis from texts and they appreciated being able to take control of their learning. They also agreed peer-teaching gave them a sense of achievement and encouraged them to be more responsible for their own learning.The data collected and analyzed in this case study indicate that peer-teaching can yield many benefits to students who engage in it. Firstly, it promotes students ' language learning autonomy. By engaging in peer-teaching, the students take responsibility fortheir own language learning. They select materials and prepare the linguistic input for the teaching session. In so doing, they can experiment with various learning activities, depending on their interests and their assessment of their own and their fellow students learning needs.Secondly, it helps the students to develop their metacognitive strategies The students used metacognitive strategies extensively during the peer-teaching experience. They were aware ofaims and objectives when planning the lesson and evaluated it afterwards.Peer-teaching can also offer students an opportunity to developtheir social skills, with peer-teaching activity the students have to assume responsibility not only for their own learning but alsofor that of their peers. They need to show sensitivity and empathy towards individual lear ners while promoting their peers ' learning experience. Learners respect and support each other and are tolerant of less able peer-teachers.5ConclusionThe data obtained from this study indicate by implementingpeer-teaching in language learning, the students are highly motivated in their learning and their self-confidence is greatly improved. The study also proves peer- teaching has also developed the students 'metacognitive strategies and social strategies. In conclusion, peer-teaching can promote students language learning autonomy. Although this experience of peer-teaching was positive, a number of problematic issues did arise. For example, some peer-teachers were not able to offer a high level of instruction. Some lacked theability to organize teaching and handling the classroom situation. And peer-teaching could also be time-consuming since some peer-teachers were not be able to finish their presentation within the time given to them. Educators need to consider these problems when implementing peer-teaching. Although this study contributes some useful information about peer teaching, there exist somelimitations. First, the sample size was small.Second theparticipants were only students of English majors. These restrict the generalizability of findings. Therefore it would be premature to draw a qualitative conclusion. More experiments and further studies need to be done so as to find out the advantages and disadvantagesof this learning style.Reference :[1]Assinder, W. Peer Teaching, peer Learning:One[J].Model. ELT Journal, 1991. 45/3: 218-29[2]Dickinson L. Autonomy and motivation: a literary view[J]. In System ,1995. 23/2:163-174[3]Goodlad, S. Mentoring and tutoring by students[M]. London: Kogan Page ,1998.[4]Hill, S. & Topping, K. Cognitive and transferable gains for student tutors[J]. In S. Goodlad (ed.), Students as teachers and mentors. London: Kogan ,1995. Page, pp.135-154.[5]Topping, K. The peer tutoring handbook: promoting co-operative learning[M]. Cambridge: Brookline Books. 1988.。
机器学习与人工智能领域中常用的英语词汇
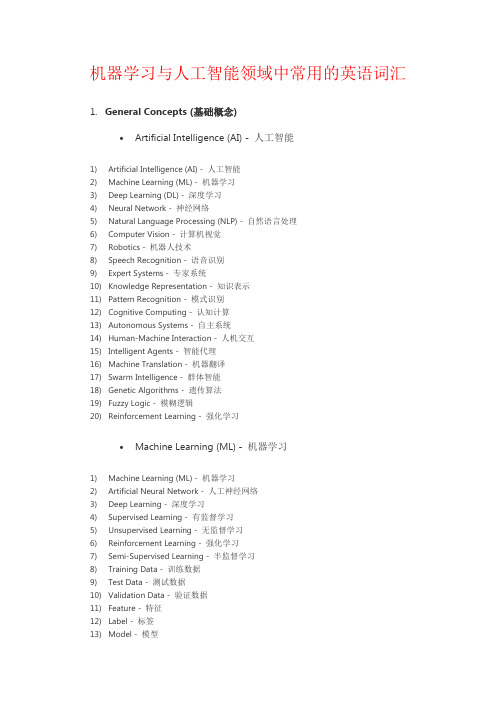
机器学习与人工智能领域中常用的英语词汇1.General Concepts (基础概念)•Artificial Intelligence (AI) - 人工智能1)Artificial Intelligence (AI) - 人工智能2)Machine Learning (ML) - 机器学习3)Deep Learning (DL) - 深度学习4)Neural Network - 神经网络5)Natural Language Processing (NLP) - 自然语言处理6)Computer Vision - 计算机视觉7)Robotics - 机器人技术8)Speech Recognition - 语音识别9)Expert Systems - 专家系统10)Knowledge Representation - 知识表示11)Pattern Recognition - 模式识别12)Cognitive Computing - 认知计算13)Autonomous Systems - 自主系统14)Human-Machine Interaction - 人机交互15)Intelligent Agents - 智能代理16)Machine Translation - 机器翻译17)Swarm Intelligence - 群体智能18)Genetic Algorithms - 遗传算法19)Fuzzy Logic - 模糊逻辑20)Reinforcement Learning - 强化学习•Machine Learning (ML) - 机器学习1)Machine Learning (ML) - 机器学习2)Artificial Neural Network - 人工神经网络3)Deep Learning - 深度学习4)Supervised Learning - 有监督学习5)Unsupervised Learning - 无监督学习6)Reinforcement Learning - 强化学习7)Semi-Supervised Learning - 半监督学习8)Training Data - 训练数据9)Test Data - 测试数据10)Validation Data - 验证数据11)Feature - 特征12)Label - 标签13)Model - 模型14)Algorithm - 算法15)Regression - 回归16)Classification - 分类17)Clustering - 聚类18)Dimensionality Reduction - 降维19)Overfitting - 过拟合20)Underfitting - 欠拟合•Deep Learning (DL) - 深度学习1)Deep Learning - 深度学习2)Neural Network - 神经网络3)Artificial Neural Network (ANN) - 人工神经网络4)Convolutional Neural Network (CNN) - 卷积神经网络5)Recurrent Neural Network (RNN) - 循环神经网络6)Long Short-Term Memory (LSTM) - 长短期记忆网络7)Gated Recurrent Unit (GRU) - 门控循环单元8)Autoencoder - 自编码器9)Generative Adversarial Network (GAN) - 生成对抗网络10)Transfer Learning - 迁移学习11)Pre-trained Model - 预训练模型12)Fine-tuning - 微调13)Feature Extraction - 特征提取14)Activation Function - 激活函数15)Loss Function - 损失函数16)Gradient Descent - 梯度下降17)Backpropagation - 反向传播18)Epoch - 训练周期19)Batch Size - 批量大小20)Dropout - 丢弃法•Neural Network - 神经网络1)Neural Network - 神经网络2)Artificial Neural Network (ANN) - 人工神经网络3)Deep Neural Network (DNN) - 深度神经网络4)Convolutional Neural Network (CNN) - 卷积神经网络5)Recurrent Neural Network (RNN) - 循环神经网络6)Long Short-Term Memory (LSTM) - 长短期记忆网络7)Gated Recurrent Unit (GRU) - 门控循环单元8)Feedforward Neural Network - 前馈神经网络9)Multi-layer Perceptron (MLP) - 多层感知器10)Radial Basis Function Network (RBFN) - 径向基函数网络11)Hopfield Network - 霍普菲尔德网络12)Boltzmann Machine - 玻尔兹曼机13)Autoencoder - 自编码器14)Spiking Neural Network (SNN) - 脉冲神经网络15)Self-organizing Map (SOM) - 自组织映射16)Restricted Boltzmann Machine (RBM) - 受限玻尔兹曼机17)Hebbian Learning - 海比安学习18)Competitive Learning - 竞争学习19)Neuroevolutionary - 神经进化20)Neuron - 神经元•Algorithm - 算法1)Algorithm - 算法2)Supervised Learning Algorithm - 有监督学习算法3)Unsupervised Learning Algorithm - 无监督学习算法4)Reinforcement Learning Algorithm - 强化学习算法5)Classification Algorithm - 分类算法6)Regression Algorithm - 回归算法7)Clustering Algorithm - 聚类算法8)Dimensionality Reduction Algorithm - 降维算法9)Decision Tree Algorithm - 决策树算法10)Random Forest Algorithm - 随机森林算法11)Support Vector Machine (SVM) Algorithm - 支持向量机算法12)K-Nearest Neighbors (KNN) Algorithm - K近邻算法13)Naive Bayes Algorithm - 朴素贝叶斯算法14)Gradient Descent Algorithm - 梯度下降算法15)Genetic Algorithm - 遗传算法16)Neural Network Algorithm - 神经网络算法17)Deep Learning Algorithm - 深度学习算法18)Ensemble Learning Algorithm - 集成学习算法19)Reinforcement Learning Algorithm - 强化学习算法20)Metaheuristic Algorithm - 元启发式算法•Model - 模型1)Model - 模型2)Machine Learning Model - 机器学习模型3)Artificial Intelligence Model - 人工智能模型4)Predictive Model - 预测模型5)Classification Model - 分类模型6)Regression Model - 回归模型7)Generative Model - 生成模型8)Discriminative Model - 判别模型9)Probabilistic Model - 概率模型10)Statistical Model - 统计模型11)Neural Network Model - 神经网络模型12)Deep Learning Model - 深度学习模型13)Ensemble Model - 集成模型14)Reinforcement Learning Model - 强化学习模型15)Support Vector Machine (SVM) Model - 支持向量机模型16)Decision Tree Model - 决策树模型17)Random Forest Model - 随机森林模型18)Naive Bayes Model - 朴素贝叶斯模型19)Autoencoder Model - 自编码器模型20)Convolutional Neural Network (CNN) Model - 卷积神经网络模型•Dataset - 数据集1)Dataset - 数据集2)Training Dataset - 训练数据集3)Test Dataset - 测试数据集4)Validation Dataset - 验证数据集5)Balanced Dataset - 平衡数据集6)Imbalanced Dataset - 不平衡数据集7)Synthetic Dataset - 合成数据集8)Benchmark Dataset - 基准数据集9)Open Dataset - 开放数据集10)Labeled Dataset - 标记数据集11)Unlabeled Dataset - 未标记数据集12)Semi-Supervised Dataset - 半监督数据集13)Multiclass Dataset - 多分类数据集14)Feature Set - 特征集15)Data Augmentation - 数据增强16)Data Preprocessing - 数据预处理17)Missing Data - 缺失数据18)Outlier Detection - 异常值检测19)Data Imputation - 数据插补20)Metadata - 元数据•Training - 训练1)Training - 训练2)Training Data - 训练数据3)Training Phase - 训练阶段4)Training Set - 训练集5)Training Examples - 训练样本6)Training Instance - 训练实例7)Training Algorithm - 训练算法8)Training Model - 训练模型9)Training Process - 训练过程10)Training Loss - 训练损失11)Training Epoch - 训练周期12)Training Batch - 训练批次13)Online Training - 在线训练14)Offline Training - 离线训练15)Continuous Training - 连续训练16)Transfer Learning - 迁移学习17)Fine-Tuning - 微调18)Curriculum Learning - 课程学习19)Self-Supervised Learning - 自监督学习20)Active Learning - 主动学习•Testing - 测试1)Testing - 测试2)Test Data - 测试数据3)Test Set - 测试集4)Test Examples - 测试样本5)Test Instance - 测试实例6)Test Phase - 测试阶段7)Test Accuracy - 测试准确率8)Test Loss - 测试损失9)Test Error - 测试错误10)Test Metrics - 测试指标11)Test Suite - 测试套件12)Test Case - 测试用例13)Test Coverage - 测试覆盖率14)Cross-Validation - 交叉验证15)Holdout Validation - 留出验证16)K-Fold Cross-Validation - K折交叉验证17)Stratified Cross-Validation - 分层交叉验证18)Test Driven Development (TDD) - 测试驱动开发19)A/B Testing - A/B 测试20)Model Evaluation - 模型评估•Validation - 验证1)Validation - 验证2)Validation Data - 验证数据3)Validation Set - 验证集4)Validation Examples - 验证样本5)Validation Instance - 验证实例6)Validation Phase - 验证阶段7)Validation Accuracy - 验证准确率8)Validation Loss - 验证损失9)Validation Error - 验证错误10)Validation Metrics - 验证指标11)Cross-Validation - 交叉验证12)Holdout Validation - 留出验证13)K-Fold Cross-Validation - K折交叉验证14)Stratified Cross-Validation - 分层交叉验证15)Leave-One-Out Cross-Validation - 留一法交叉验证16)Validation Curve - 验证曲线17)Hyperparameter Validation - 超参数验证18)Model Validation - 模型验证19)Early Stopping - 提前停止20)Validation Strategy - 验证策略•Supervised Learning - 有监督学习1)Supervised Learning - 有监督学习2)Label - 标签3)Feature - 特征4)Target - 目标5)Training Labels - 训练标签6)Training Features - 训练特征7)Training Targets - 训练目标8)Training Examples - 训练样本9)Training Instance - 训练实例10)Regression - 回归11)Classification - 分类12)Predictor - 预测器13)Regression Model - 回归模型14)Classifier - 分类器15)Decision Tree - 决策树16)Support Vector Machine (SVM) - 支持向量机17)Neural Network - 神经网络18)Feature Engineering - 特征工程19)Model Evaluation - 模型评估20)Overfitting - 过拟合21)Underfitting - 欠拟合22)Bias-Variance Tradeoff - 偏差-方差权衡•Unsupervised Learning - 无监督学习1)Unsupervised Learning - 无监督学习2)Clustering - 聚类3)Dimensionality Reduction - 降维4)Anomaly Detection - 异常检测5)Association Rule Learning - 关联规则学习6)Feature Extraction - 特征提取7)Feature Selection - 特征选择8)K-Means - K均值9)Hierarchical Clustering - 层次聚类10)Density-Based Clustering - 基于密度的聚类11)Principal Component Analysis (PCA) - 主成分分析12)Independent Component Analysis (ICA) - 独立成分分析13)T-distributed Stochastic Neighbor Embedding (t-SNE) - t分布随机邻居嵌入14)Gaussian Mixture Model (GMM) - 高斯混合模型15)Self-Organizing Maps (SOM) - 自组织映射16)Autoencoder - 自动编码器17)Latent Variable - 潜变量18)Data Preprocessing - 数据预处理19)Outlier Detection - 异常值检测20)Clustering Algorithm - 聚类算法•Reinforcement Learning - 强化学习1)Reinforcement Learning - 强化学习2)Agent - 代理3)Environment - 环境4)State - 状态5)Action - 动作6)Reward - 奖励7)Policy - 策略8)Value Function - 值函数9)Q-Learning - Q学习10)Deep Q-Network (DQN) - 深度Q网络11)Policy Gradient - 策略梯度12)Actor-Critic - 演员-评论家13)Exploration - 探索14)Exploitation - 开发15)Temporal Difference (TD) - 时间差分16)Markov Decision Process (MDP) - 马尔可夫决策过程17)State-Action-Reward-State-Action (SARSA) - 状态-动作-奖励-状态-动作18)Policy Iteration - 策略迭代19)Value Iteration - 值迭代20)Monte Carlo Methods - 蒙特卡洛方法•Semi-Supervised Learning - 半监督学习1)Semi-Supervised Learning - 半监督学习2)Labeled Data - 有标签数据3)Unlabeled Data - 无标签数据4)Label Propagation - 标签传播5)Self-Training - 自训练6)Co-Training - 协同训练7)Transudative Learning - 传导学习8)Inductive Learning - 归纳学习9)Manifold Regularization - 流形正则化10)Graph-based Methods - 基于图的方法11)Cluster Assumption - 聚类假设12)Low-Density Separation - 低密度分离13)Semi-Supervised Support Vector Machines (S3VM) - 半监督支持向量机14)Expectation-Maximization (EM) - 期望最大化15)Co-EM - 协同期望最大化16)Entropy-Regularized EM - 熵正则化EM17)Mean Teacher - 平均教师18)Virtual Adversarial Training - 虚拟对抗训练19)Tri-training - 三重训练20)Mix Match - 混合匹配•Feature - 特征1)Feature - 特征2)Feature Engineering - 特征工程3)Feature Extraction - 特征提取4)Feature Selection - 特征选择5)Input Features - 输入特征6)Output Features - 输出特征7)Feature Vector - 特征向量8)Feature Space - 特征空间9)Feature Representation - 特征表示10)Feature Transformation - 特征转换11)Feature Importance - 特征重要性12)Feature Scaling - 特征缩放13)Feature Normalization - 特征归一化14)Feature Encoding - 特征编码15)Feature Fusion - 特征融合16)Feature Dimensionality Reduction - 特征维度减少17)Continuous Feature - 连续特征18)Categorical Feature - 分类特征19)Nominal Feature - 名义特征20)Ordinal Feature - 有序特征•Label - 标签1)Label - 标签2)Labeling - 标注3)Ground Truth - 地面真值4)Class Label - 类别标签5)Target Variable - 目标变量6)Labeling Scheme - 标注方案7)Multi-class Labeling - 多类别标注8)Binary Labeling - 二分类标注9)Label Noise - 标签噪声10)Labeling Error - 标注错误11)Label Propagation - 标签传播12)Unlabeled Data - 无标签数据13)Labeled Data - 有标签数据14)Semi-supervised Learning - 半监督学习15)Active Learning - 主动学习16)Weakly Supervised Learning - 弱监督学习17)Noisy Label Learning - 噪声标签学习18)Self-training - 自训练19)Crowdsourcing Labeling - 众包标注20)Label Smoothing - 标签平滑化•Prediction - 预测1)Prediction - 预测2)Forecasting - 预测3)Regression - 回归4)Classification - 分类5)Time Series Prediction - 时间序列预测6)Forecast Accuracy - 预测准确性7)Predictive Modeling - 预测建模8)Predictive Analytics - 预测分析9)Forecasting Method - 预测方法10)Predictive Performance - 预测性能11)Predictive Power - 预测能力12)Prediction Error - 预测误差13)Prediction Interval - 预测区间14)Prediction Model - 预测模型15)Predictive Uncertainty - 预测不确定性16)Forecast Horizon - 预测时间跨度17)Predictive Maintenance - 预测性维护18)Predictive Policing - 预测式警务19)Predictive Healthcare - 预测性医疗20)Predictive Maintenance - 预测性维护•Classification - 分类1)Classification - 分类2)Classifier - 分类器3)Class - 类别4)Classify - 对数据进行分类5)Class Label - 类别标签6)Binary Classification - 二元分类7)Multiclass Classification - 多类分类8)Class Probability - 类别概率9)Decision Boundary - 决策边界10)Decision Tree - 决策树11)Support Vector Machine (SVM) - 支持向量机12)K-Nearest Neighbors (KNN) - K最近邻算法13)Naive Bayes - 朴素贝叶斯14)Logistic Regression - 逻辑回归15)Random Forest - 随机森林16)Neural Network - 神经网络17)SoftMax Function - SoftMax函数18)One-vs-All (One-vs-Rest) - 一对多(一对剩余)19)Ensemble Learning - 集成学习20)Confusion Matrix - 混淆矩阵•Regression - 回归1)Regression Analysis - 回归分析2)Linear Regression - 线性回归3)Multiple Regression - 多元回归4)Polynomial Regression - 多项式回归5)Logistic Regression - 逻辑回归6)Ridge Regression - 岭回归7)Lasso Regression - Lasso回归8)Elastic Net Regression - 弹性网络回归9)Regression Coefficients - 回归系数10)Residuals - 残差11)Ordinary Least Squares (OLS) - 普通最小二乘法12)Ridge Regression Coefficient - 岭回归系数13)Lasso Regression Coefficient - Lasso回归系数14)Elastic Net Regression Coefficient - 弹性网络回归系数15)Regression Line - 回归线16)Prediction Error - 预测误差17)Regression Model - 回归模型18)Nonlinear Regression - 非线性回归19)Generalized Linear Models (GLM) - 广义线性模型20)Coefficient of Determination (R-squared) - 决定系数21)F-test - F检验22)Homoscedasticity - 同方差性23)Heteroscedasticity - 异方差性24)Autocorrelation - 自相关25)Multicollinearity - 多重共线性26)Outliers - 异常值27)Cross-validation - 交叉验证28)Feature Selection - 特征选择29)Feature Engineering - 特征工程30)Regularization - 正则化2.Neural Networks and Deep Learning (神经网络与深度学习)•Convolutional Neural Network (CNN) - 卷积神经网络1)Convolutional Neural Network (CNN) - 卷积神经网络2)Convolution Layer - 卷积层3)Feature Map - 特征图4)Convolution Operation - 卷积操作5)Stride - 步幅6)Padding - 填充7)Pooling Layer - 池化层8)Max Pooling - 最大池化9)Average Pooling - 平均池化10)Fully Connected Layer - 全连接层11)Activation Function - 激活函数12)Rectified Linear Unit (ReLU) - 线性修正单元13)Dropout - 随机失活14)Batch Normalization - 批量归一化15)Transfer Learning - 迁移学习16)Fine-Tuning - 微调17)Image Classification - 图像分类18)Object Detection - 物体检测19)Semantic Segmentation - 语义分割20)Instance Segmentation - 实例分割21)Generative Adversarial Network (GAN) - 生成对抗网络22)Image Generation - 图像生成23)Style Transfer - 风格迁移24)Convolutional Autoencoder - 卷积自编码器25)Recurrent Neural Network (RNN) - 循环神经网络•Recurrent Neural Network (RNN) - 循环神经网络1)Recurrent Neural Network (RNN) - 循环神经网络2)Long Short-Term Memory (LSTM) - 长短期记忆网络3)Gated Recurrent Unit (GRU) - 门控循环单元4)Sequence Modeling - 序列建模5)Time Series Prediction - 时间序列预测6)Natural Language Processing (NLP) - 自然语言处理7)Text Generation - 文本生成8)Sentiment Analysis - 情感分析9)Named Entity Recognition (NER) - 命名实体识别10)Part-of-Speech Tagging (POS Tagging) - 词性标注11)Sequence-to-Sequence (Seq2Seq) - 序列到序列12)Attention Mechanism - 注意力机制13)Encoder-Decoder Architecture - 编码器-解码器架构14)Bidirectional RNN - 双向循环神经网络15)Teacher Forcing - 强制教师法16)Backpropagation Through Time (BPTT) - 通过时间的反向传播17)Vanishing Gradient Problem - 梯度消失问题18)Exploding Gradient Problem - 梯度爆炸问题19)Language Modeling - 语言建模20)Speech Recognition - 语音识别•Long Short-Term Memory (LSTM) - 长短期记忆网络1)Long Short-Term Memory (LSTM) - 长短期记忆网络2)Cell State - 细胞状态3)Hidden State - 隐藏状态4)Forget Gate - 遗忘门5)Input Gate - 输入门6)Output Gate - 输出门7)Peephole Connections - 窥视孔连接8)Gated Recurrent Unit (GRU) - 门控循环单元9)Vanishing Gradient Problem - 梯度消失问题10)Exploding Gradient Problem - 梯度爆炸问题11)Sequence Modeling - 序列建模12)Time Series Prediction - 时间序列预测13)Natural Language Processing (NLP) - 自然语言处理14)Text Generation - 文本生成15)Sentiment Analysis - 情感分析16)Named Entity Recognition (NER) - 命名实体识别17)Part-of-Speech Tagging (POS Tagging) - 词性标注18)Attention Mechanism - 注意力机制19)Encoder-Decoder Architecture - 编码器-解码器架构20)Bidirectional LSTM - 双向长短期记忆网络•Attention Mechanism - 注意力机制1)Attention Mechanism - 注意力机制2)Self-Attention - 自注意力3)Multi-Head Attention - 多头注意力4)Transformer - 变换器5)Query - 查询6)Key - 键7)Value - 值8)Query-Value Attention - 查询-值注意力9)Dot-Product Attention - 点积注意力10)Scaled Dot-Product Attention - 缩放点积注意力11)Additive Attention - 加性注意力12)Context Vector - 上下文向量13)Attention Score - 注意力分数14)SoftMax Function - SoftMax函数15)Attention Weight - 注意力权重16)Global Attention - 全局注意力17)Local Attention - 局部注意力18)Positional Encoding - 位置编码19)Encoder-Decoder Attention - 编码器-解码器注意力20)Cross-Modal Attention - 跨模态注意力•Generative Adversarial Network (GAN) - 生成对抗网络1)Generative Adversarial Network (GAN) - 生成对抗网络2)Generator - 生成器3)Discriminator - 判别器4)Adversarial Training - 对抗训练5)Minimax Game - 极小极大博弈6)Nash Equilibrium - 纳什均衡7)Mode Collapse - 模式崩溃8)Training Stability - 训练稳定性9)Loss Function - 损失函数10)Discriminative Loss - 判别损失11)Generative Loss - 生成损失12)Wasserstein GAN (WGAN) - Wasserstein GAN(WGAN)13)Deep Convolutional GAN (DCGAN) - 深度卷积生成对抗网络(DCGAN)14)Conditional GAN (c GAN) - 条件生成对抗网络(c GAN)15)Style GAN - 风格生成对抗网络16)Cycle GAN - 循环生成对抗网络17)Progressive Growing GAN (PGGAN) - 渐进式增长生成对抗网络(PGGAN)18)Self-Attention GAN (SAGAN) - 自注意力生成对抗网络(SAGAN)19)Big GAN - 大规模生成对抗网络20)Adversarial Examples - 对抗样本•Encoder-Decoder - 编码器-解码器1)Encoder-Decoder Architecture - 编码器-解码器架构2)Encoder - 编码器3)Decoder - 解码器4)Sequence-to-Sequence Model (Seq2Seq) - 序列到序列模型5)State Vector - 状态向量6)Context Vector - 上下文向量7)Hidden State - 隐藏状态8)Attention Mechanism - 注意力机制9)Teacher Forcing - 强制教师法10)Beam Search - 束搜索11)Recurrent Neural Network (RNN) - 循环神经网络12)Long Short-Term Memory (LSTM) - 长短期记忆网络13)Gated Recurrent Unit (GRU) - 门控循环单元14)Bidirectional Encoder - 双向编码器15)Greedy Decoding - 贪婪解码16)Masking - 遮盖17)Dropout - 随机失活18)Embedding Layer - 嵌入层19)Cross-Entropy Loss - 交叉熵损失20)Tokenization - 令牌化•Transfer Learning - 迁移学习1)Transfer Learning - 迁移学习2)Source Domain - 源领域3)Target Domain - 目标领域4)Fine-Tuning - 微调5)Domain Adaptation - 领域自适应6)Pre-Trained Model - 预训练模型7)Feature Extraction - 特征提取8)Knowledge Transfer - 知识迁移9)Unsupervised Domain Adaptation - 无监督领域自适应10)Semi-Supervised Domain Adaptation - 半监督领域自适应11)Multi-Task Learning - 多任务学习12)Data Augmentation - 数据增强13)Task Transfer - 任务迁移14)Model Agnostic Meta-Learning (MAML) - 与模型无关的元学习(MAML)15)One-Shot Learning - 单样本学习16)Zero-Shot Learning - 零样本学习17)Few-Shot Learning - 少样本学习18)Knowledge Distillation - 知识蒸馏19)Representation Learning - 表征学习20)Adversarial Transfer Learning - 对抗迁移学习•Pre-trained Models - 预训练模型1)Pre-trained Model - 预训练模型2)Transfer Learning - 迁移学习3)Fine-Tuning - 微调4)Knowledge Transfer - 知识迁移5)Domain Adaptation - 领域自适应6)Feature Extraction - 特征提取7)Representation Learning - 表征学习8)Language Model - 语言模型9)Bidirectional Encoder Representations from Transformers (BERT) - 双向编码器结构转换器10)Generative Pre-trained Transformer (GPT) - 生成式预训练转换器11)Transformer-based Models - 基于转换器的模型12)Masked Language Model (MLM) - 掩蔽语言模型13)Cloze Task - 填空任务14)Tokenization - 令牌化15)Word Embeddings - 词嵌入16)Sentence Embeddings - 句子嵌入17)Contextual Embeddings - 上下文嵌入18)Self-Supervised Learning - 自监督学习19)Large-Scale Pre-trained Models - 大规模预训练模型•Loss Function - 损失函数1)Loss Function - 损失函数2)Mean Squared Error (MSE) - 均方误差3)Mean Absolute Error (MAE) - 平均绝对误差4)Cross-Entropy Loss - 交叉熵损失5)Binary Cross-Entropy Loss - 二元交叉熵损失6)Categorical Cross-Entropy Loss - 分类交叉熵损失7)Hinge Loss - 合页损失8)Huber Loss - Huber损失9)Wasserstein Distance - Wasserstein距离10)Triplet Loss - 三元组损失11)Contrastive Loss - 对比损失12)Dice Loss - Dice损失13)Focal Loss - 焦点损失14)GAN Loss - GAN损失15)Adversarial Loss - 对抗损失16)L1 Loss - L1损失17)L2 Loss - L2损失18)Huber Loss - Huber损失19)Quantile Loss - 分位数损失•Activation Function - 激活函数1)Activation Function - 激活函数2)Sigmoid Function - Sigmoid函数3)Hyperbolic Tangent Function (Tanh) - 双曲正切函数4)Rectified Linear Unit (Re LU) - 矩形线性单元5)Parametric Re LU (P Re LU) - 参数化Re LU6)Exponential Linear Unit (ELU) - 指数线性单元7)Swish Function - Swish函数8)Softplus Function - Soft plus函数9)Softmax Function - SoftMax函数10)Hard Tanh Function - 硬双曲正切函数11)Softsign Function - Softsign函数12)GELU (Gaussian Error Linear Unit) - GELU(高斯误差线性单元)13)Mish Function - Mish函数14)CELU (Continuous Exponential Linear Unit) - CELU(连续指数线性单元)15)Bent Identity Function - 弯曲恒等函数16)Gaussian Error Linear Units (GELUs) - 高斯误差线性单元17)Adaptive Piecewise Linear (APL) - 自适应分段线性函数18)Radial Basis Function (RBF) - 径向基函数•Backpropagation - 反向传播1)Backpropagation - 反向传播2)Gradient Descent - 梯度下降3)Partial Derivative - 偏导数4)Chain Rule - 链式法则5)Forward Pass - 前向传播6)Backward Pass - 反向传播7)Computational Graph - 计算图8)Neural Network - 神经网络9)Loss Function - 损失函数10)Gradient Calculation - 梯度计算11)Weight Update - 权重更新12)Activation Function - 激活函数13)Optimizer - 优化器14)Learning Rate - 学习率15)Mini-Batch Gradient Descent - 小批量梯度下降16)Stochastic Gradient Descent (SGD) - 随机梯度下降17)Batch Gradient Descent - 批量梯度下降18)Momentum - 动量19)Adam Optimizer - Adam优化器20)Learning Rate Decay - 学习率衰减•Gradient Descent - 梯度下降1)Gradient Descent - 梯度下降2)Stochastic Gradient Descent (SGD) - 随机梯度下降3)Mini-Batch Gradient Descent - 小批量梯度下降4)Batch Gradient Descent - 批量梯度下降5)Learning Rate - 学习率6)Momentum - 动量7)Adaptive Moment Estimation (Adam) - 自适应矩估计8)RMSprop - 均方根传播9)Learning Rate Schedule - 学习率调度10)Convergence - 收敛11)Divergence - 发散12)Adagrad - 自适应学习速率方法13)Adadelta - 自适应增量学习率方法14)Adamax - 自适应矩估计的扩展版本15)Nadam - Nesterov Accelerated Adaptive Moment Estimation16)Learning Rate Decay - 学习率衰减17)Step Size - 步长18)Conjugate Gradient Descent - 共轭梯度下降19)Line Search - 线搜索20)Newton's Method - 牛顿法•Learning Rate - 学习率1)Learning Rate - 学习率2)Adaptive Learning Rate - 自适应学习率3)Learning Rate Decay - 学习率衰减4)Initial Learning Rate - 初始学习率5)Step Size - 步长6)Momentum - 动量7)Exponential Decay - 指数衰减8)Annealing - 退火9)Cyclical Learning Rate - 循环学习率10)Learning Rate Schedule - 学习率调度11)Warm-up - 预热12)Learning Rate Policy - 学习率策略13)Learning Rate Annealing - 学习率退火14)Cosine Annealing - 余弦退火15)Gradient Clipping - 梯度裁剪16)Adapting Learning Rate - 适应学习率17)Learning Rate Multiplier - 学习率倍增器18)Learning Rate Reduction - 学习率降低19)Learning Rate Update - 学习率更新20)Scheduled Learning Rate - 定期学习率•Batch Size - 批量大小1)Batch Size - 批量大小2)Mini-Batch - 小批量3)Batch Gradient Descent - 批量梯度下降4)Stochastic Gradient Descent (SGD) - 随机梯度下降5)Mini-Batch Gradient Descent - 小批量梯度下降6)Online Learning - 在线学习7)Full-Batch - 全批量8)Data Batch - 数据批次9)Training Batch - 训练批次10)Batch Normalization - 批量归一化11)Batch-wise Optimization - 批量优化12)Batch Processing - 批量处理13)Batch Sampling - 批量采样14)Adaptive Batch Size - 自适应批量大小15)Batch Splitting - 批量分割16)Dynamic Batch Size - 动态批量大小17)Fixed Batch Size - 固定批量大小18)Batch-wise Inference - 批量推理19)Batch-wise Training - 批量训练20)Batch Shuffling - 批量洗牌•Epoch - 训练周期1)Training Epoch - 训练周期2)Epoch Size - 周期大小3)Early Stopping - 提前停止4)Validation Set - 验证集5)Training Set - 训练集6)Test Set - 测试集7)Overfitting - 过拟合8)Underfitting - 欠拟合9)Model Evaluation - 模型评估10)Model Selection - 模型选择11)Hyperparameter Tuning - 超参数调优12)Cross-Validation - 交叉验证13)K-fold Cross-Validation - K折交叉验证14)Stratified Cross-Validation - 分层交叉验证15)Leave-One-Out Cross-Validation (LOOCV) - 留一法交叉验证16)Grid Search - 网格搜索17)Random Search - 随机搜索18)Model Complexity - 模型复杂度19)Learning Curve - 学习曲线20)Convergence - 收敛3.Machine Learning Techniques and Algorithms (机器学习技术与算法)•Decision Tree - 决策树1)Decision Tree - 决策树2)Node - 节点3)Root Node - 根节点4)Leaf Node - 叶节点5)Internal Node - 内部节点6)Splitting Criterion - 分裂准则7)Gini Impurity - 基尼不纯度8)Entropy - 熵9)Information Gain - 信息增益10)Gain Ratio - 增益率11)Pruning - 剪枝12)Recursive Partitioning - 递归分割13)CART (Classification and Regression Trees) - 分类回归树14)ID3 (Iterative Dichotomiser 3) - 迭代二叉树315)C4.5 (successor of ID3) - C4.5(ID3的后继者)16)C5.0 (successor of C4.5) - C5.0(C4.5的后继者)17)Split Point - 分裂点18)Decision Boundary - 决策边界19)Pruned Tree - 剪枝后的树20)Decision Tree Ensemble - 决策树集成•Random Forest - 随机森林1)Random Forest - 随机森林2)Ensemble Learning - 集成学习3)Bootstrap Sampling - 自助采样4)Bagging (Bootstrap Aggregating) - 装袋法5)Out-of-Bag (OOB) Error - 袋外误差6)Feature Subset - 特征子集7)Decision Tree - 决策树8)Base Estimator - 基础估计器9)Tree Depth - 树深度10)Randomization - 随机化11)Majority Voting - 多数投票12)Feature Importance - 特征重要性13)OOB Score - 袋外得分14)Forest Size - 森林大小15)Max Features - 最大特征数16)Min Samples Split - 最小分裂样本数17)Min Samples Leaf - 最小叶节点样本数18)Gini Impurity - 基尼不纯度19)Entropy - 熵20)Variable Importance - 变量重要性•Support Vector Machine (SVM) - 支持向量机1)Support Vector Machine (SVM) - 支持向量机2)Hyperplane - 超平面3)Kernel Trick - 核技巧4)Kernel Function - 核函数5)Margin - 间隔6)Support Vectors - 支持向量7)Decision Boundary - 决策边界8)Maximum Margin Classifier - 最大间隔分类器9)Soft Margin Classifier - 软间隔分类器10) C Parameter - C参数11)Radial Basis Function (RBF) Kernel - 径向基函数核12)Polynomial Kernel - 多项式核13)Linear Kernel - 线性核14)Quadratic Kernel - 二次核15)Gaussian Kernel - 高斯核16)Regularization - 正则化17)Dual Problem - 对偶问题18)Primal Problem - 原始问题19)Kernelized SVM - 核化支持向量机20)Multiclass SVM - 多类支持向量机•K-Nearest Neighbors (KNN) - K-最近邻1)K-Nearest Neighbors (KNN) - K-最近邻2)Nearest Neighbor - 最近邻3)Distance Metric - 距离度量4)Euclidean Distance - 欧氏距离5)Manhattan Distance - 曼哈顿距离6)Minkowski Distance - 闵可夫斯基距离7)Cosine Similarity - 余弦相似度8)K Value - K值9)Majority Voting - 多数投票10)Weighted KNN - 加权KNN11)Radius Neighbors - 半径邻居12)Ball Tree - 球树13)KD Tree - KD树14)Locality-Sensitive Hashing (LSH) - 局部敏感哈希15)Curse of Dimensionality - 维度灾难16)Class Label - 类标签17)Training Set - 训练集18)Test Set - 测试集19)Validation Set - 验证集20)Cross-Validation - 交叉验证•Naive Bayes - 朴素贝叶斯1)Naive Bayes - 朴素贝叶斯2)Bayes' Theorem - 贝叶斯定理3)Prior Probability - 先验概率4)Posterior Probability - 后验概率5)Likelihood - 似然6)Class Conditional Probability - 类条件概率7)Feature Independence Assumption - 特征独立假设8)Multinomial Naive Bayes - 多项式朴素贝叶斯9)Gaussian Naive Bayes - 高斯朴素贝叶斯10)Bernoulli Naive Bayes - 伯努利朴素贝叶斯11)Laplace Smoothing - 拉普拉斯平滑12)Add-One Smoothing - 加一平滑13)Maximum A Posteriori (MAP) - 最大后验概率14)Maximum Likelihood Estimation (MLE) - 最大似然估计15)Classification - 分类16)Feature Vectors - 特征向量17)Training Set - 训练集18)Test Set - 测试集19)Class Label - 类标签20)Confusion Matrix - 混淆矩阵•Clustering - 聚类1)Clustering - 聚类2)Centroid - 质心3)Cluster Analysis - 聚类分析4)Partitioning Clustering - 划分式聚类5)Hierarchical Clustering - 层次聚类6)Density-Based Clustering - 基于密度的聚类7)K-Means Clustering - K均值聚类8)K-Medoids Clustering - K中心点聚类9)DBSCAN (Density-Based Spatial Clustering of Applications with Noise) - 基于密度的空间聚类算法10)Agglomerative Clustering - 聚合式聚类11)Dendrogram - 系统树图12)Silhouette Score - 轮廓系数13)Elbow Method - 肘部法则14)Clustering Validation - 聚类验证15)Intra-cluster Distance - 类内距离16)Inter-cluster Distance - 类间距离17)Cluster Cohesion - 类内连贯性18)Cluster Separation - 类间分离度19)Cluster Assignment - 聚类分配20)Cluster Label - 聚类标签•K-Means - K-均值1)K-Means - K-均值2)Centroid - 质心3)Cluster - 聚类4)Cluster Center - 聚类中心5)Cluster Assignment - 聚类分配6)Cluster Analysis - 聚类分析7)K Value - K值8)Elbow Method - 肘部法则9)Inertia - 惯性10)Silhouette Score - 轮廓系数11)Convergence - 收敛12)Initialization - 初始化13)Euclidean Distance - 欧氏距离14)Manhattan Distance - 曼哈顿距离15)Distance Metric - 距离度量16)Cluster Radius - 聚类半径17)Within-Cluster Variation - 类内变异18)Cluster Quality - 聚类质量19)Clustering Algorithm - 聚类算法20)Clustering Validation - 聚类验证•Dimensionality Reduction - 降维1)Dimensionality Reduction - 降维2)Feature Extraction - 特征提取3)Feature Selection - 特征选择4)Principal Component Analysis (PCA) - 主成分分析5)Singular Value Decomposition (SVD) - 奇异值分解6)Linear Discriminant Analysis (LDA) - 线性判别分析7)t-Distributed Stochastic Neighbor Embedding (t-SNE) - t-分布随机邻域嵌入8)Autoencoder - 自编码器9)Manifold Learning - 流形学习10)Locally Linear Embedding (LLE) - 局部线性嵌入11)Isomap - 等度量映射12)Uniform Manifold Approximation and Projection (UMAP) - 均匀流形逼近与投影13)Kernel PCA - 核主成分分析14)Non-negative Matrix Factorization (NMF) - 非负矩阵分解15)Independent Component Analysis (ICA) - 独立成分分析16)Variational Autoencoder (VAE) - 变分自编码器17)Sparse Coding - 稀疏编码18)Random Projection - 随机投影19)Neighborhood Preserving Embedding (NPE) - 保持邻域结构的嵌入20)Curvilinear Component Analysis (CCA) - 曲线成分分析•Principal Component Analysis (PCA) - 主成分分析1)Principal Component Analysis (PCA) - 主成分分析2)Eigenvector - 特征向量3)Eigenvalue - 特征值4)Covariance Matrix - 协方差矩阵。
人工智能原理_北京大学中国大学mooc课后章节答案期末考试题库2023年
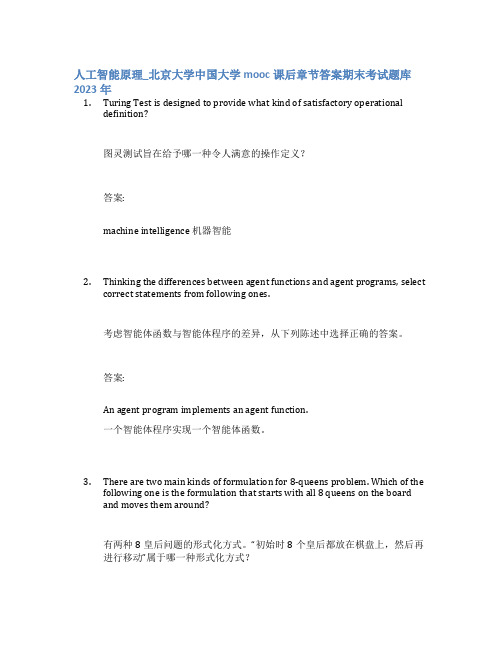
人工智能原理_北京大学中国大学mooc课后章节答案期末考试题库2023年1.Turing Test is designed to provide what kind of satisfactory operationaldefinition?图灵测试旨在给予哪一种令人满意的操作定义?答案:machine intelligence 机器智能2.Thinking the differences between agent functions and agent programs, selectcorrect statements from following ones.考虑智能体函数与智能体程序的差异,从下列陈述中选择正确的答案。
答案:An agent program implements an agent function.一个智能体程序实现一个智能体函数。
3.There are two main kinds of formulation for 8-queens problem. Which of thefollowing one is the formulation that starts with all 8 queens on the boardand moves them around?有两种8皇后问题的形式化方式。
“初始时8个皇后都放在棋盘上,然后再进行移动”属于哪一种形式化方式?答案:Complete-state formulation 全态形式化4.What kind of knowledge will be used to describe how a problem is solved?哪种知识可用于描述如何求解问题?答案:Procedural knowledge 过程性知识5.Which of the following is used to discover general facts from trainingexamples?下列中哪个用于训练样本中发现一般的事实?答案:Inductive learning 归纳学习6.Which statement best describes the task of “classification” in machinelearning?哪一个是机器学习中“分类”任务的正确描述?答案:To assign a category to each item. 为每个项目分配一个类别。
Modeling probabilistic actions for practical decision-theoretic planning
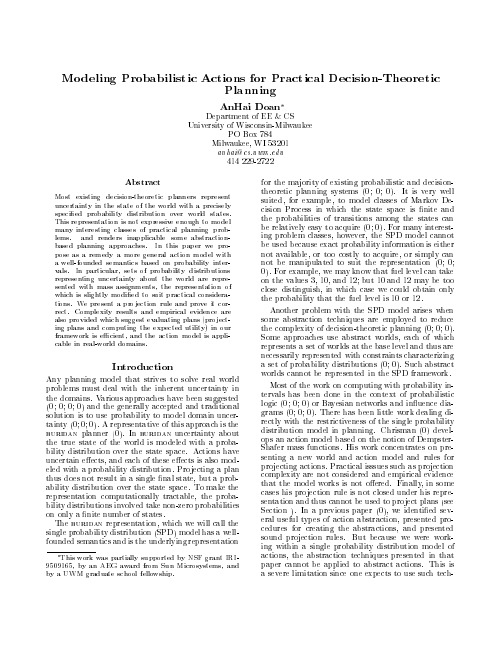
Any planning model that strives to solve real world problems must deal with the inherent uncertainty in the domains. Various approaches have been suggested (0; 0; 0; 0) and the generally accepted and traditional solution is to use probability to model domain uncertainty (0; 0; 0). A representative of this approach is the buridan planner (0). In buridan uncertainty about the true state of the world is modeled with a probability distribution over the state space. Actions have uncertain e ects, and each of these e ects is also modeled with a probability distribution. Projecting a plan thus does not result in a single nal state, but a probability distribution over the state space. To make the representation computationally tractable, the probability distributions involved take non-zero probabilities on only a nite number of states. The buridan representation, which we will call the single probability distribution (SPD) model has a wellfounded semantics and is the underlying representation
汽车制造领域“岗课赛证”综合育人的高职人才培养模式探索

1 引言2021年4月,全国职业教育大会传达了习近平重要指示和李克强批示,中共中央政治局委员、国务院副总理孙春兰指出,要一体化设计中职、高职、本科职业教育培养体系,深化“三教”改革,“岗课赛证”综合育人,提升教育质量。
教育部印发《关于学习宣传贯彻习近平总书记重要指示和全国职业教育大会精神的通知》,要求加快完善人才培养体系,探索“岗课赛证”相互融合,建好国家“学分银行”。
持续探索校企‘双元’‘岗课赛证’融通育人模式,激励更多学生走上技能报国之路,为国家输送更多高质量技能型人才,是高职院校的重要使命[1]。
2 岗课赛证综合育人的必要性2.1 社会适应性与职业岗位的频繁变换的需求岗课赛证融通协同育人符合教育的社会适应性规律。
从我国社会发展趋势来看,职业教育的社会适应性需要加强学生综合素养的全面发展,强化新时代大学生的价值塑造。
培养学生与社会发展对应的综合技能是岗课赛证融通的目的,利于培养高素质技能人才[2]。
另外,随着社会的不断进步,各行各业趋于智能化网络化发展,职业岗位也不断更新变换,“岗课赛证”综合育人是学生的能力提升和新时代职业岗位频繁变换的需求。
2.2 教育学角度,人的全面发展需要在培养技能型人才的过程中除了培养学生具备扎实的专业知识、过硬的实践技能、良好的道德品格、优秀的职业素养等外,还需要培育正确的人生观、世界观、价值观。
重要培养方式之一就是“岗课赛证”综合育人。
职业院校的学生在理论与实践相结合的学习过程中形成良好的思想观念和道德品质。
此外,在真实的工作情境中还能够锻炼学生的综合职业素养,如职业精神、劳动观念等。
2.3 知识经济时代,社会对人才的要求随着时代的发展,企业对人才有了更高的要求。
在学校死读书的学生会被社会淘汰,不会沟通埋头苦干的员工常常得不到重视,汽车制造领域“岗课赛证”综合育人的高职人才培养模式探索卜素婷益阳职业技术学院 湖南省益阳市 413055摘 要:岗课证赛四者看似相互分离,实则联系紧密,本质都与学生的职业能力相关。
工程勘察英语
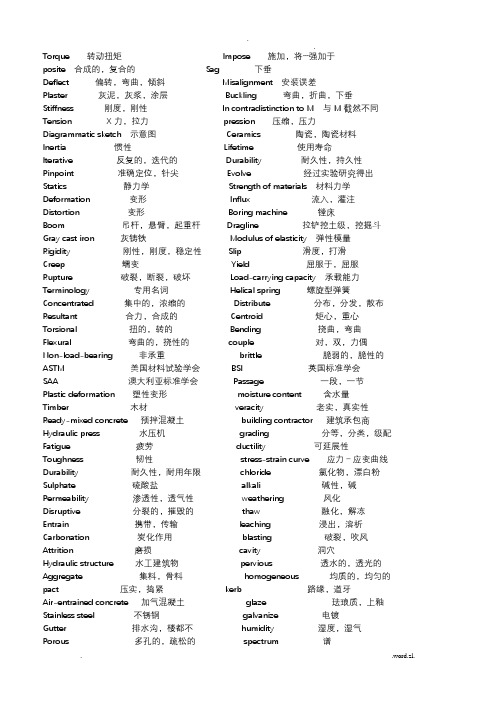
..Torque 转动扭矩Impose 施加,将…强加于posite 合成的,复合的Sag 下垂Deflect 偏转,弯曲,倾斜Misalignment 安装误差Plaster 灰泥,灰浆,涂层Buckling 弯曲,折曲,下垂Stiffness 刚度,刚性In contradistinction to M 与M截然不同Tension X力,拉力pression 压缩,压力Diagrammatic sketch 示意图Ceramics 陶瓷,陶瓷材料Inertia 惯性Lifetime 使用寿命Iterative 反复的,迭代的Durability 耐久性,持久性Pinpoint 准确定位,针尖Evolve 经过实验研究得出Statics 静力学Strength of materials 材料力学Deformation 变形Influx 流入,灌注Distortion 变形Boring machine 镗床Boom 吊杆,悬臂,起重杆Dragline 拉铲挖土级,挖掘斗Gray cast iron 灰铸铁Modulus of elasticity 弹性模量Rigidity 刚性,刚度,稳定性Slip 滑度,打滑Creep 蠕变Yield 屈服于,屈服Rupture 破裂,断裂,破坏Load-carrying capacity 承载能力Terminology 专用名词Helical spring 螺旋型弹簧Concentrated 集中的,浓缩的Distribute 分布,分发,散布Resultant 合力,合成的Centroid 矩心,重心Torsional 扭的,转的Bending 挠曲,弯曲Flexural 弯曲的,挠性的couple 对,双,力偶Non-load-bearing 非承重brittle 脆弱的,脆性的ASTM 美国材料试验学会BSI 英国标准学会SAA 澳大利亚标准学会Passage 一段,一节Plastic deformation 塑性变形moisture content 含水量Timber 木材veracity 老实,真实性Ready-mixed concrete 预拌混凝土building contractor 建筑承包商Hydraulic press 水压机grading 分等,分类,级配Fatigue 疲劳ductility 可延展性Toughness 韧性stress-strain curve 应力-应变曲线Durability 耐久性,耐用年限chloride 氯化物,漂白粉Sulphate 硫酸盐alkali 碱性,碱Permeability 渗透性,透气性weathering 风化Disruptive 分裂的,摧毁的thaw 融化,解冻Entrain 携带,传输leaching 浸出,溶析Carbonation 炭化作用blasting 破裂,吹风Attrition 磨损cavity 洞穴Hydraulic structure 水工建筑物pervious 透水的,透光的Aggregate 集料,骨料homogeneous 均质的,均匀的pact 压实,捣紧kerb 路缘,道牙Air-entrained concrete 加气混凝土glaze 珐琅质,上釉Stainless steel 不锈钢galvanize 电镀Gutter 排水沟,楼都不humidity 湿度,湿气Porous 多孔的,疏松的spectrum 谱. .word.zl...Infrared 红外的,产生红外辐射的ultraviolet 紫外的Gravel 砾石,卵石anodized 受过阳极化处理的Hydrate 水合物reinforcement 加强,加固Spall 剥落,散裂erosion 侵蚀,腐蚀Abrasion 磨损,磨耗quarry 方形砖Eddy 涡流,漩涡运动reinforcing steel 钢筋Reinforced concrete 钢筋混凝土reinforcing bar 钢筋Longitudinal 长度的,纵向的,轴向的dispose 处置Incline 倾斜,弄斜moment 力矩Bond 结合,结合力,粘合力interlock 连动,连接Forestall 阻止,预防embed 放入,埋入Prestressing steel 预应力钢筋rebar 钢筋Splice 接头congestion 充满Form 模坂ACI 美国混凝土协会Code 法规,规程galvanize 电镀Undue 过度的,过分的BS 英国标准Blending machine 弯筋机mandrel 芯棒Jig 夹具dowel 夹缝丁,暗销Wire clip 钢丝剪reference number 参考号数Mill scale 热轧钢外表氧化皮spacer 隔离物Clip 夹子,剪刀slab 板,块,楼板Cradle 吊架,托架,支架chair 托架,支板Mild steel 低碳钢tack 图钉,点焊焊缝Hydraulic 水力的,液力的additive 添加剂Admixture 外加剂binder 胶结剂Aggregate 聚集,结合,骨料,填料aerate 充气Aerate concrete 加气混凝土cellular 蜂窝状的Portland 波特兰水泥pulverize 粉化,磨碎Granulate 成粒状,轧碎blastfurnace 高炉,鼓风炉Slag 矿渣,炉渣pigment 颜料,色料Retarder 延迟剂,缓凝剂accelerator 加速剂,促凝剂Curing (混凝土)养护paction 压实,捣实Ambient 周围的,外界的shrinkage 收缩,下沉,压缩Autoclave 蒸压器,热压处理frost 粗糙的,无光泽的Efflorescence 风化,粉化,粉化物sodium 钠Calcium 钙potassium 钾Carbonate 炭化pozzolana 火山灰Sulphate 硫酸盐autoclaved aerated concrete 高压蒸养加气混凝土Hard-burnt 高温焙烧的,炼制的veneer 镶片,砌面,表层Mallet 木锤,大锤chisel 凿子Mold 模子,模具mass production 大量生产Update 使现代化trade journal 行业杂志Periodical 定期的,定期刊行的editorial board 编辑委员会Beholder 旁观者ordinance 规格,条例,法令Suspended structure 悬吊构造joist 梁,垳条Through bridge 下承桥chord 弦杆. .word.zl...Bracing 拉条,支撑web-girder 腹板大梁Box-girder 箱梁plan view 平面图Outline 外形,剖面drawing 附图Bills of materials 材料清单radiator 散热器General arrangement 总体布置detail drawing 详图Cladding 包层,包壳panel 面板,仪表盘GA drawing 总体平面图zoom 变焦距In situ 原地,在原地cumbersome 笨重的,麻烦的Parameterized 参数化specification 详细说明,标准Verify 校验,验证,说明homogeneous 均匀的,对等的Buckling 弯曲,翘曲,弯折deterministic 确定的,决定的Probabilistic 概率的,随机的deviation 偏差,偏移,参数Psychological 心理的,精神的cross-section 横断面,横截面Amortization 阻尼proof-load 检验荷载Field 场地,工地survey 调查,测量,勘察Intrinsic 内在的,固有的stochastic 随机的,不确定的Seismic 地震的,与地震有关的single load 集中荷载Foundation 根底,地基gravity 重力Lateral 横向的,侧面的transient 瞬时的,不稳定的Basement 底座,根底,地下室erratic 反复无常的,无规律的Footing 根底,基脚,底座,垫层overdesign 保险设计Underdesign 欠平安设计appurtenance 附属物,附属设备Firewall 防火墙parapet 栏杆,护墙,女儿墙Remold 改造,改型,重铸ductwork 管道系统,管网Tenant 租用latitude 纬度,宽度,范围Tributary 支流,附庸,辅助的cantilever 悬臂,悬臂梁,支架Provision 预防,规定,条款overload 超载,超重Reference 参考书uncertainty 不确定性Slighting 轻蔑的,不尊重的likelihood 像有,相似Pef = pounds per cubic foot 磅/平方英尺reliability 可靠性,平安性Methodology 方法论shield 屏蔽Shield 屏蔽,遮护without recourse to 不依赖Reason 推理,论证weir 堰,拦河堰Spillway 溢洪道,泻水道clause 条款工程Deploy 适用,配置filler wall 填充墙,柱间墙Shear wall 剪力墙ignorance 外行Up-to-date 现代的,最新likewise 同样Bucket 吊斗,挖斗wheelarrow 手推车,独轮小车Buggy 手推车,小斗车segregation 分凝,离析Chute 斜槽place 场所,浇筑Lift 混凝土浇筑层,,升降机displacement 偏移pact 夯实honeyb 蜂窝构造Tamping 夯实,捣固,捣实spinkling 喷洒Calcium chloride 氯化钙settlement 沉陷Grout 水浆,灌浆cohesive 粘聚的,粘结的Workable 和易性好的,塑性的slump 坍塌度. .word.zl...Workability 可塑性air-entraining 加气的Deposit 存储,浇注consolidation 加固,压实Bleeding 渗漏subsidence 沉降,下降Laitance 水泥浮浆float 镘刀,抹子Capillary 毛细管的,毛细现象flash set 急凝Aluminate 铝酸盐burlap 麻袋,粗麻布Membrane 膜,隔板emulsion 乳胶体,乳液Polyethylene 聚乙烯waterproof 防水Harden 硬化,凝固gravel 砾石,卵石Immersion 浸入,插入headroom 净空高度,头上空间Nominal 铭牌的,名义的power-driven 动力驱动的,电动的External vibrator 外表振捣器architect 建筑师Nonhomogeneous 不均匀的,多相的creep 蠕变Shrink 缩小structure code 构造标准Ultimate strength 极限强度load factor 荷载系数From M onwards 从m算起来prestressed concrete 预应力混凝土Faulty 有缺点的,报废的,不合格的embed 放入,埋入Abutment 桥台,岸墩thrust 推,轴向力Jack 千斤顶,支柱motorway 汽车道,快车道Tendon 钢筋束,筋post-tensioned prestressing 后X法预应力Pre-tensioned prestressing 先X法预应力manoeuvrable 机动的,容易驾驶的Plain concrete 无筋混凝土prepression 预加压力,预先压缩Leak 漏,渗漏camber 弯度,曲度,凸形Proportioning 使成比例,确定几何尺寸analogous 类似的,类比的,模拟的With reference to 参考,参照,关于centroid 矩心,面积矩心,质心Refinement 精制,改善,改良bond 结合,粘合,粘合强度Transmit 传递,寄,传送interlocking 可联动的,联锁Plain bar 光面钢筋,无结钢筋formed bar 变形钢筋,竹结钢筋Hoop 环,箍,铁箍tie 拉杆,系杆Stirrup 箍筋,钢筋箍crushing 压碎,轧碎Bearing 承载,承受,支撑点bearing pressure 支承压力Rib 肋,棱,perimeter 周边,周长,周界Blanketing 覆盖,包上,掩盖mortar 砂浆,灰浆,灰泥Bentonite 膨润土,膨土岩trench 沟槽,沟道,沟管Tremie 混凝土导管〔水下浇筑用〕full prestressing 全预应力Partial prestressing 局部预应力reservoir 水库,蓄水池Eccentric 偏心的,呈偏心运动的,偏心器counteract 中和,平衡Intermitterntly 间隙的,断续的,周期的infrequent 不常见的,稀少的Objectionable 不能采用的,不适宜的reverse 颠倒的,改变方向的,交换的Cement paste 水泥浆sack 袋,包,一包,一袋Water-cement ratio 水灰比trial-batch 〔混凝土〕小量试拌Slump test 坍塌度试验truncate 缩短,截去Air-entraining agent 加气剂plasticizer 增塑剂,塑化剂revolving-drum mixer 转筒式搅拌机ready-mixed 运送时搅拌的transit0mixed 运送时拌的batch 配料,分批配料congested 拥挤的,充塞的haul 搬运,运输. .word.zl...discharge 卸货,卸载hopper 漏斗,装料车batching plant 拌和厂batching 配料,定配合比ecclesiastical 基督教会的,教士的landmark 陆标,里程碑,界标mobility 可动性,机动性,流动性topographical 地形的,地形测量的subservient 辅助性的tax 使受压力,负担shear wall core 剪力墙筒体prefabrication 预制的,预制品slip-formwork 滑模crane 起重机,用起重机吊codify 编成法典,编纂elevator 升降机escalator 升降梯,自动扶梯drift 漂移,位移prescribe 规定,命令,指示I-beam 工字梁,工字钢masonry 砖石工程,砌筑体staircase 楼梯lofty 高耸的,极高的condominium 各住户认购各自一套公寓楼brace 支撑,支柱bundle 捆,扎,粘合soaring 高耸的,高涨的tributary 支流,附属的prototype 原型,样机web 腹板,梁板torsion 扭转,转矩orthogonal 互相垂直的,正交的,直角的stiffen 加强,加固urbanization 都市化habitation 住宅,住所deterrent 制止物,威慑因素premium 奖金,质量改良的clutter 混乱,干扰,弄乱multi-discipline 多学科pedestrian 行人,步行者plaza 广场,大空地sway 摇,摆动overturn 倾倒,翻转clear span 净高clear spacing 净距zoning 分区,区域化excavation 挖掘,挖方overlook 检查,监视interim 间歇底,暂时的empiricism 经历主义elastoplastic 弹塑性的subset 子系统partmentalize 间隔化,隔开,分段mantle 罩,外壳,地幔erosion 侵蚀,冲刷,磨损waterborne 水生的,水力运输的boulder 漂砾,大块石cobble 圆石,鹅软石pebble 小软石,小砾石degradation 剥蚀,退化avalanche 山崩,坍方,崩塌stratum 地层,岩层meandering 曲折的,弯曲的,曲流的stratified 有层次的,分层的water table 地下水位detritus 瓦砾,腐质percolation 渗透,渗滤soil profile 土壤剖面,土层剖面residual soil 残积土transported soil 运积土ground water 地下水superstructure 上层构造earth fill 土堤substructure 下部构造,根底工事demarcation 分界限sanitary 环境卫生的sanitary fill 垃圾堆积场reclamation 废料回收,改造,垦殖disposal 处理,清理,处置boring 钻探,打眼mat 席,垫,钢筋网caisson 沉箱retaining-wall 挡土墙uneven settlement 不均匀沉降overburden 超载,过载strap beam 搭扣形梁unsound 不巩固的,不结实的pile 桩,打桩hardpan 硬土层,巩固根底raft 筏,木排bined footing 联合根底cantilever footing 悬臂根底. .word.zl...raft of floating foundation 筏式根底或浮筏根底soil mass 土体pile shaft 桩身stratum 地层,矿层end-bearing pile 端承桩uplift 举起,抬起water table 地下水位scour 冲刷,洗涤deposit 沉积,浇注,矿床soil-exploration 土质勘察footing 根底,基脚,底座strip 带,条,狭长片raft 筏,垫板capital 柱头fixity 固定,不变eccentric 偏心的,不同圆心的nonuniformity 不均匀性,不均质性scheduling 编制时间表drain 消耗,耗尽premium 奖金,佣金hold up 阻碍procurement 取得,获得,采购vector 矢量,向量prerequisite 先决条件,前提concurrent 同时进展的,并行的lead time 产品设计至实际投产时间litigation 诉讼,打官司chronological 按时间顺序的hoist 卷扬机,起重机utility 实用的,公用事业utility line 公用事业管线accrue 产生,出现dismantle 撤除,拆卸remuneration 酬劳,报酬mensurate 同量的,相应的degenerate into 简化成,变质成in-house 机构内部的critical path 关键路径lay down 规定,制定方案wearing surface 磨耗面base course 基层asphalt 柏油landscaping 风景设计,风景布置bulldozer 推土机,压路机vibrating roller 振动压路机bitumen 沥青grill 格栅,铁格网mesh 网,网格,筛concrete train 混凝土铺路机组slipform paver 滑模铺路机hold in place 把…固定在引诱的位置runway 跑道,通道Tokaido line 新干线catalyst 触媒,催化剂Side-effect 副作用decentralization 分散,疏散Unity 整体,单元outstrip 超过Crude 天然的,原油piecemeal 片断,点Ferment 酵素,发酵,蓬勃开展underlying 根底的,根本的Geographer 地理学家operations research 运筹学Cable-stayed 斜拉的,X拉的girder 梁,垳,梁杆Cast-in-place 现场浇筑的AASHT美国洲际公路及运输工作者协会AREA 美国铁路工程师协会crossing 穿插,十字路口Pier 桥墩elevated 高架的,高的,高架铁路Clearance 间隙,净空,间距segment 局部,段Dominance 支配,控制,优势one-way slab 单向配筋板Versus 与…的关系曲线statically determinate structure 静定构造Skew 斜的,扭的,弯曲的on-site 现场的,就地的,工地Shear key 受剪键bituminous concrete 沥青混凝土Dissipate 去除,消除posite structure 混合构造Acronym 缩写词,缩语chore 零星工作,零活In the foreseeable future 在可遇见的未来一段时间内impetus 动力,刺激Draughtsman 绘图员,起草者repetitious 重复的,反复的Mundane 世间的,世俗的jargon 行话,术语,难懂的话. .word.zl...Level 水平,水位,水平仪theodolite 精细经纬仪Envision 想象,遇见,展望contour map 等高线,地形图Plat 地段,地区图,地段图grading 校准,定标,土工修正Stake 标桩,定位木桩picture 图画,设想,想象Cut 挖土,挖方fill 填筑,填方,填土,路堤Borrow 取土,采料,采料场indeterminate structure 超静定构造Myriad 万,无数,无数的photogrammetry 摄影测绘sGeotechnical engineering 岩土工程mass transit 公共交通Transmission line 输电线infiltrate 渗入,渗透Estimator 估价者,评价者rival 对手,竞争,比得上Off-the-shelf 现成的,成品的,畅销的afterthought 回想,反省,懊悔,马后炮Timeliness 及时,时间性,好时机inception 开场,起头,开端Culminate 到达顶点,完毕beneficiary 受益人,受惠人Outset 开场,最初working drawing 施工图Prescribe 规定,命令delineation 描绘,描述Co-ordinate 一样,同等的事物prerogative 特权,特性Board of directors 董事会estimating 估价Margin 边缘,余量,余地tender 投标,承包,标件Incisive 锋利的,深刻的,透彻的realm 范围,部门,类Forethought 预先考虑好的modus 方法,程式Modus operandi 做法,方法,工作方式nullify 废弃,取消Indispensable 不可缺少的,必需的corollary 推论,结果Doom 消灭,灭亡,判决obverse 较显著面Tactical 战术的,策略的deployment 部署,调度,配置Ensemble 整体,总效果pictorial 绘图的,插图的Dispassionate 冷静的,不带偏见的prognosis 预测,预报Ubiquitous 普遍存在的,随遇的denominator 分母,标准Handmaiden 起陪衬作用的liken 比较Jobbing 做临时工trade journal 行业期刊Cost plus 附加费premise 前提,房产Speculation 投机,投机买卖sub-contractor 转包商,分包商Order 订单on-cost 杂费,间接本钱Microfiche 缩微胶片,平片. .word.zl.。
传说中编程界的龙书、虎书、鲸书、魔法书……指的都是哪些?
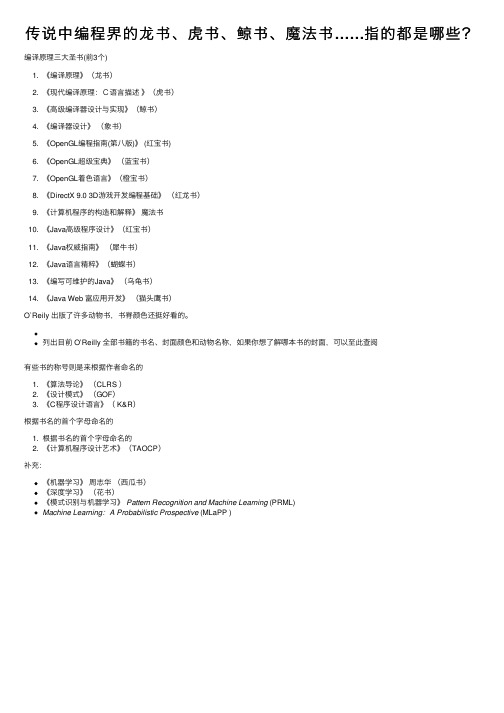
传说中编程界的龙书、虎书、鲸书、魔法书……指的都是哪些?编译原理三⼤圣书(前3个)
1. 《编译原理》(龙书)
2. 《现代编译原理:C语⾔描述》(虎书)
3. 《⾼级编译器设计与实现》(鲸书)
4. 《编译器设计》(象书)
5. 《OpenGL编程指南(第⼋版)》 (红宝书)
6. 《OpenGL超级宝典》(蓝宝书)
7. 《OpenGL着⾊语⾔》(橙宝书)
8. 《DirectX 9.0 3D游戏开发编程基础》(红龙书)
9. 《计算机程序的构造和解释》魔法书
10. 《Java⾼级程序设计》(红宝书)
11. 《Java权威指南》(犀⽜书)
12. 《Java语⾔精粹》(蝴蝶书)
13. 《编写可维护的Java》(乌龟书)
14. 《Java Web 富应⽤开发》(猫头鹰书)
O`Reily 出版了许多动物书,书脊颜⾊还挺好看的。
列出⽬前 O’Reilly 全部书籍的书名、封⾯颜⾊和动物名称,如果你想了解哪本书的封⾯,可以⾄此查阅
有些书的称号则是来根据作者命名的
1. 《算法导论》(CLRS )
2. 《设计模式》(GOF)
3. 《C程序设计语⾔》( K&R)
根据书名的⾸个字母命名的
1. 根据书名的⾸个字母命名的
2. 《计算机程序设计艺术》(TAOCP)
补充:
《机器学习》周志华(西⽠书)
《深度学习》(花书)
《模式识别与机器学习》Pattern Recognition and Machine Learning (PRML)
Machine Learning:A Probabilistic Prospective (MLaPP )。
An introduction to machine learning and graphical
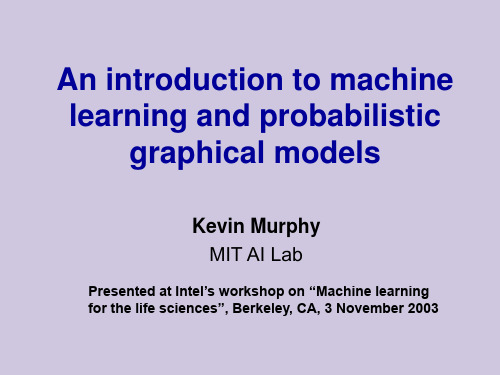
4
Key issue: generalization
yes
no
?
?
Can’t just memorize the training set (overfitting)
5
Hypothesis spaces
Decision trees Neural networks K-nearest neighbors Naïve Bayes classifier Support vector machines (SVMs) Boosted decision stumps …
2
Supervised learning
yes
no
Color
Shape
Size
Output
Blue
Torus
Big
Y
Blue
Square
Small
Y
Blue
Star
Small
Y
Red
Arrow
Small
N
Learn to approximate function F(x1, x2, x3) -> t
Boosting maximizes the margin
13
Supervised learning success stories
Face detection Steering an autonomous car across the US Detecting credit card fraud Medical diagnosis …
6
Perceptron (neural net with no hidden layers)
Linearly separable data
L M S A l g o r i t h m 最 小 均 方 算 法 ( 2 0 2 0 )
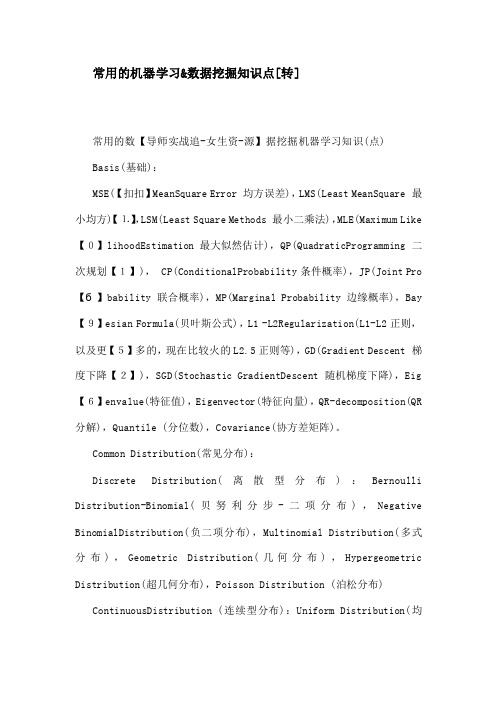
常用的机器学习&数据挖掘知识点[转]常用的数【导师实战追-女生资-源】据挖掘机器学习知识(点)Basis(基础):MSE(【扣扣】MeanSquare Error 均方误差),LMS(Least MeanSquare 最小均方)【⒈】,LSM(Least Square Methods 最小二乘法),MLE(Maximum Like 【0】lihoodEstimation最大似然估计),QP(QuadraticProgramming 二次规划【1】), CP(ConditionalProbability条件概率),JP(Joint Pro 【б】bability 联合概率),MP(Marginal Probability边缘概率),Bay 【9】esian Formula(贝叶斯公式),L1 -L2Regularization(L1-L2正则,以及更【5】多的,现在比较火的L2.5正则等),GD(Gradient Descent 梯度下降【2】),SGD(Stochastic GradientDescent 随机梯度下降),Eig 【6】envalue(特征值),Eigenvector(特征向量),QR-decomposition(QR 分解),Quantile (分位数),Covariance(协方差矩阵)。
Common Distribution(常见分布):Discrete Distribution(离散型分布):Bernoulli Distribution-Binomial(贝努利分步-二项分布),Negative BinomialDistribution(负二项分布),Multinomial Distribution(多式分布),Geometric Distribution(几何分布),Hypergeometric Distribution(超几何分布),Poisson Distribution (泊松分布) ContinuousDistribution (连续型分布):Uniform Distribution(均匀分布),Normal Distribution-GaussianDistribution(正态分布-高斯分布),Exponential Distribution(指数分布),Lognormal Distribution(对数正态分布),Gamma Distribution(Gamma分布),Beta Distribution(Beta分布),Dirichlet Distribution(狄利克雷分布),Rayleigh Distribution(瑞利分布),Cauchy Distribution(柯西分布),Weibull Distribution (韦伯分布)Three Sampling Distribution(三大抽样分布):Chi-square Distribution(卡方分布),t-distribution(t-distribution),F-distribution(F-分布)Data Pre-processing(数据预处理):MissingValue Imputation(缺失值填充),Discretization(离散化),Mapping(映射),Normalization(归一化-标准化)。
Learning spatiotemporal models from training examples
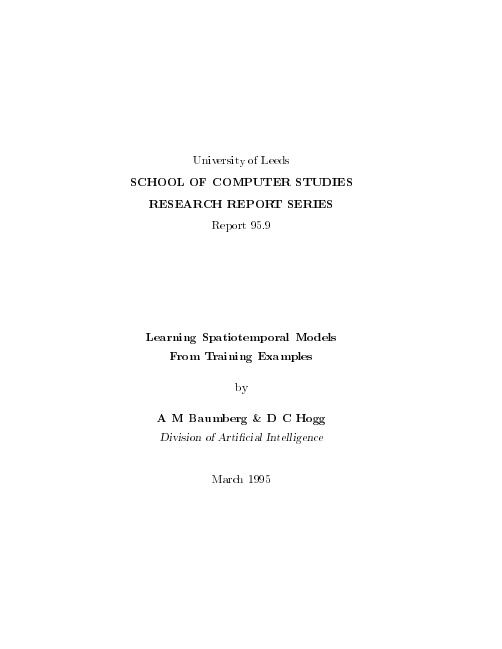
~ C =b I+b
0
1
2
Hence the linear system of equations is decoupled into kn independent 2nd order di erential equations. 2
2.1 State space metric
The object to be modelled is assumed to have a constant (uniform) density , and the mass matrix is calculated in the usual way by :M = R H (u)H (u)du = H
University of Leeds
SCHOOL OF COMPUTER STUDIES RESEARCH REPORT SERIES
Report 95.9
Learning Spatiotemporal Models From Training Examples
by
A M Baumberg & D C Hogg
1 Introduction
The application of physically based constraints allows di cult problems in computer vision to be solved by ensuring the system is overconstrained. These constraints are not necessarily based on real physical properties but merely motivated by the assumed physical nature of the problem. We are interested in accurately tracking a non-rigid deforming object. Pentland and Horowitz 1] describe a method for recovering non-rigid motion and structure by deriving physically based \free vibration" modes using the Finite Element Method (FEM). The method relies on making physical assumptions about the object, such as uniform distribution of mass and constant elasticity. The vibration modes are derived from the governing equation of the FEM nodal parametrisation. The mass and sti ness matrices in the governing equation are either known or derived from the physical assumptions. Physically based \modal analysis" has been used in a wide range of applications (e.g. Nastar and Ayache 2], 3]). The use of training information has been shown to be a powerful tool in computer vision and pattern recognition (e.g. to train neural networks). In the Point Distribution Model (PDM), Cootes and Taylor 4] utilise a set of static training shapes to derive a set of orthogonal \modes of variation". The training shapes can be accurately represented by a basis consisting of a subset of these vectors. The PDM has proven useful in model-based image interpretation (e.g. Cootes et al 5], Hill et al 6]) and in image sequence analysis (e.g. real-time contour tracking 7], robust tracking of deformable models 8]). However, one drawback of this approach is that there is no temporal aspect to the model. Hence it is not possible to extrapolate forward in time to get good estimates of the expected shape of the object. 1
Traffic Classification Using Clustering Algorithms

Traffic Classification Using Clustering AlgorithmsJeffrey Erman,Martin Arlitt,Anirban MahantiUniversity of Calgary,2500University Drive NW,Calgary,AB,Canada{erman,arlitt,mahanti}@cpsc.ucalgary.caABSTRACTClassification of network traffic using port-based or payload-based analysis is becoming increasingly difficult with many peer-to-peer (P2P)applications using dynamic port numbers,masquerading tech-niques,and encryption to avoid detection.An alternative approach is to classify traffic by exploiting the distinctive characteristics of applications when they communicate on a network.We pursue this latter approach and demonstrate how cluster analysis can be used to effectively identify groups of traffic that are similar using only transport layer statistics.Our work considers two unsupervised clustering algorithms,namely K-Means and DBSCAN,that have previously not been used for network traffic classification.We eval-uate these two algorithms and compare them to the previously used AutoClass algorithm,using empirical Internet traces.The experi-mental results show that both K-Means and DBSCAN work very well and much more quickly then AutoClass.Our results indicate that although DBSCAN has lower accuracy compared to K-Means and AutoClass,DBSCAN produces better clusters.Categories and Subject DescriptorsI.5.4[Computing Methodologies]:Pattern Recognition—Appli-cationsGeneral TermsAlgorithms,classificationKeywordsmachine learning,unsupervised clustering1.INTRODUCTIONAccurate identification and categorization of network traffic ac-cording to application type is an important element of many net-work management tasks such asflow prioritization,traffic shap-ing/policing,and diagnostic monitoring.For example,a network operator may want to identify and throttle(or block)traffic from peer-to-peer(P2P)file sharing applications to manage its band-width budget and to ensure good performance of business criti-Permission to make digital or hard copies of all or part of this work for personal or classroom use is granted without fee provided that copies are not made or distributed for profit or commercial advantage and that copies bear this notice and the full citation on thefirst page.To copy otherwise,to republish,to post on servers or to redistribute to lists,requires prior specific permission and/or a fee.SIGCOMM’06Workshops September11-15,2006,Pisa,Italy. Copyright2006ACM1-59593-417-0/06/0009...$5.00.cal applications.Similar to network management tasks,many net-work engineering problems such as workload characterization and modelling,capacity planning,and route provisioning also benefit from accurate identification of network traffic.In this paper,we present preliminary results from our experience with using a ma-chine learning approach called clustering for the network traffic identification problem.In the remainder of this section,we moti-vate why clustering is useful,discuss the specific contributions of this paper,and outline our ongoing work.The classical approach to traffic classification relies on mapping applications to well-known port numbers and has been very suc-cessful in the past.To avoid detection by this method,P2P appli-cations began using dynamic port numbers,and also started dis-guising themselves by using port numbers for commonly used pro-tocols such as HTTP and FTP.Many recent studies confirm that port-based identification of network traffic is ineffective[8,15]. To address the aforementioned drawbacks of port-based classi-fication,several payload-based analysis techniques have been pro-posed[3,6,9,11,15].In this approach,packet payloads are ana-lyzed to determine whether they contain characteristic signatures of known applications.Studies show that these approaches work very well for the current Internet traffic including P2P traffic.In fact, some commercial packet shaping tools have started using these techniques.However,P2P applications such as BitTorrent are be-ginning to elude this technique by using obfuscation methods such as plain-text ciphers,variable-length padding,and/or encryption. In addition,there are some other disadvantages.First,these tech-niques only identify traffic for which signatures are available and are unable to classify any other traffic.Second,these techniques typically require increased processing and storage capacity.The limitations of port-based and payload-based analysis have motivated use of transport layer statistics for traffic classification[8, 10,12,14,17].These classification techniques rely on the fact that different applications typically have distinct behaviour patterns when communicating on a network.For instance,a largefile trans-fer using FTP would have a longer connection duration and larger average packet size than an instant messaging client sending short occasional messages to other clients.Similarly,some P2P appli-cations such as BitTorrent1can be distinguished from FTP data transfers because these P2P connections typically are persistent and send data bidirectionally;FTP data transfer connections are non-persistent and send data only unidirectionally.Transport layer statistics such as the total number of packets sent,the ratio of the bytes sent in each direction,the duration of the connection,and the average size of the packets characterize these behaviours.In this paper,we explore the use of a machine learning approach called clustering for classifying traffic using only transport layerstatistics.Cluster analysis is one of the most prominent methods for identifying classes amongst a group of objects,and has been used as a tool in manyfields such as biology,finance,and com-puter science.Recent work by McGregor et al.[10]and Zander et al.[17]show that cluster analysis has the ability to group Inter-net traffic using only transport layer characteristics.In this paper, we confirm their observations by evaluating two clustering algo-rithms,namely K-Means[7]and DBSCAN[5],that to the best of our knowledge have not been previously applied to this problem. In addition,as a baseline,we present results from the previously considered AutoClass[1]algorithm[10,17].The algorithms evaluated in this paper use an unsupervised learn-ing mechanism,wherein unlabelled training data is grouped based on similarity.This ability to group unlabelled training data is ad-vantageous and offers some practical benefits over learning ap-proaches that require labelled training data(discussed in Section 2).Although the selected algorithms use an unsupervised learning mechanism,each of these algorithms,however,is based on differ-ent clustering principles.The K-Means clustering algorithm is a partition-based algorithm[7],the DBSCAN algorithm is a density-based algorithm[5],and the AutoClass algorithm is a probabilistic model-based algorithm[1].One reason in particular why K-Means and DBSCAN algorithms were chosen is that they are much faster at clustering data than the previously used AutoClass algorithm. We evaluate the algorithms using two empirical traces:a well-known publicly available Internet traffic trace from the University of Auckland,and a recent trace we collected from the University of Calgary’s Internet connection.The algorithms are compared based on their ability to generate clusters that have a high predictive power of a single application.We show that clustering works for a variety of different applications,including Web,P2Pfile-sharing, andfile transfer with the AutoClass and K-Means algorithm’s ac-curacy exceeding85%in our results and DBSCAN achieving an accuracy of75%.Furthermore,we analyze the number of clusters and the number of objects in each of the clusters produced by the different algorithms.In general,the ability of an algorithm to group objects into a few“good”clusters is particularly useful in reducing the amount of processing required to label the clusters.We show that while DBSCAN has a lower overall accuracy the clusters it forms are the most accurate.Additionally,wefind that by looking at only a few of DBSCAN’s clusters one could identify a significant portion of the connections.Ours is a work-in-progress.Preliminary results indicate that clustering is indeed a useful technique for traffic identification.Our goal is to build an efficient and accurate classification tool using clustering techniques as the building block.Such a clustering tool would consist of two stages:a model building stage and a classifi-cation stage.In thefirst stage,an unsupervised clustering algorithm clusters training data.This produces a set of clusters that are then labelled to become our classification model.In the second stage, this model is used to develop a classifier that has the ability to label both online and offline network traffic.We note that offline classifi-cation is relatively easier compared to online classification,asflow statistics needed by the clustering algorithm may be easily obtained in the former case;the latter requires use of estimation techniques forflow statistics.We should also note that this approach is not a “panacea”for the traffic classification problem.While the model building phase does automatically generate clusters,we still need to use other techniques to label the clusters(e.g.,payload anal-ysis,manual classification,port-based analysis,or a combination thereof).This task is manageable because the model would typi-cally be built using small data sets.We believe that in order to build an accurate classifier,a good classification model must be used.In this paper,we focused on the model building step.Specifically,we investigate which clustering algorithm generates the best model.We are currently investigating building efficient classifiers for K-Means and DBSCAN and testing the classification accuracy of the algorithms.We are also investi-gating how often the models should be retrained(e.g.,on a daily, weekly,or monthly basis).The remainder of this paper is arranged as follows.The different Internet traffic classification methods including those using cluster analysis are reviewed in Section2.Section3outlines the theory and methods employed by the clustering algorithms studied in this paper.Section4and Section5present our methodology and out-line our experimental results,respectively.Section6discusses the experimental results.Section7presents our conclusions.2.BACKGROUNDSeveral techniques use transport layer information to address the problems associated with payload-based analysis and the diminish-ing effectiveness of port-based identification.McGregor et al.hy-pothesize the ability of using cluster analysis to groupflows using transport layer attributes[10].The authors,however,do not evalu-ate the accuracy of the classification as well as whichflow attributes produce the best results.Zander et al.extend this work by using another Expectation Maximization(EM)algorithm[2]called Au-toClass[1]and analyze the best set of attributes to use[17].Both [10]and[17]only test Bayesian clustering techniques implemented by an EM algorithm.The EM algorithm has a slow learning time. This paper evaluates clustering algorithms that are different and faster than the EM algorithm used in previous work.Some non-clustering techniques also use transport layer statis-tics to classify traffic[8,9,12,14].Roughan et e nearest neighbor and linear discriminate analysis[14].The connection du-rations and average packet size are used for classifying traffic into four distinct classes.This approach has some limitations in that the analysis from these two statistics may not be enough to classify all applications classes.Karagiannis et al.propose a technique that uses the unique be-haviors of P2P applications when they are transferring data or mak-ing connections to identify this traffic[8].Their results show that this approach is comparable with that of payload-based identifica-tion in terms of accuracy.More recently,Karagiannis et al.devel-oped another method that uses the social,functional,and applica-tion behaviors to identify all types of traffic[9].These approaches focus on higher level behaviours such as the number of concurrent connections to an IP address and does not use the transport layer characteristics of single connection that we utilize in this paper. In[12],Moore et e a supervised machine learning algo-rithm called Na¨ıve Bayes as a classifier.Moore et al.show that the Na¨ıve Bayes approach has a high accuracy classifying traffic.Su-pervised learning requires the training data to be labelled before the model is built.We believe that an unsupervised clustering approach offers some advantages over supervised learning approaches.One of the main benefits is that new applications can be identified by examining the connections that are grouped to form a new clus-ter.The supervised approach can not discover new applications and can only classify traffic for which it has labelled training data. Another advantage occurs when the connections are being labelled. Due to the high accuracy of our clusters,only a few of the connec-tions need to be identified in order to label the cluster with a high degree of confidence.Also consider the case where the data set be-ing clustered contains encrypted P2P connections or other types of encrypted traffic.These connections would not be labelled using payload-based classification.These connections would,therefore,be excluded from the supervised learning approach which can only use labelled training data as input.This could reduce the super-vised approach’s accuracy.However,the unsupervised clustering approach does not have this limitation.It might place the encrypted P2P traffic into a cluster with other unencrypted P2P traffic.By looking at the connections in the cluster,an analyst may be able to see similarities between unencrypted P2P traffic and the encrypted traffic and conclude that it may be P2P traffic.3.CLUSTERING ALGORITHMSThis section reviews the clustering algorithms,namely K-Means,DBSCAN,and AutoClass,considered in this work.The K-Means algorithm produces clusters that are spherical in shape whereas the DBSCAN algorithm has the ability to produce clusters that are non-spherical.The different cluster shapes that DBSCAN is capable of finding may allow for a better set of clusters to be found that minimize the amount of analysis required.The AutoClass algo-rithm uses a Bayesian approach and can automatically determine the number of clusters.Additionally,it performs soft clustering wherein objects are assigned to multiple clusters fractionally.The Cluster 3.0[4]software suite is used to obtain the results for K-Means clustering.The DBSCAN results are obtained the WEKA software suite [16].The AutoClass results are obtained using an implementation provided by [1].In order for the clustering of the connections to occur,a similar-ity (or distance)measurement must be established first.While vari-ous similarity measurements exist,Euclidean distance is one of the most commonly used metrics for clustering problems [7,16].With Euclidean distance,a small distance between two objects implies a strong similarity whereas a large distance implies a low similarity.In an n-dimensional space of features,Euclidean distance can be calculated between objects x and y as follows:dist (x,y )=v u ut4.METHODOLOGY4.1Empirical TracesTo analyze the algorithms,we used data from two empirical packet traces.One is a publicly available packet trace called Auck-land IV2,the other is a full packet trace that we collected ourselves at the University of Calgary.Auckland IV:The Auckland IV trace contains only TCP/IP head-ers of the traffic going through the University of Auckland’s link to the Internet.We used a subset of the Auckland IV trace from March16,2001at06:00:00to March19,2001at05:59:59.This subset provided sufficient connection samples to build our model (see Section4.4).Calgary:This trace was collected from a traffic monitor attached to the University of Calgary’s Internet link.We collected this trace on March10,2006from1to2pm.This trace is a full packet trace with the entire payloads of all the packets captured.Due to the amount of data generated when capturing full payloads,the disk capacity(60GB)of our traffic monitor wasfilled after one hour of collection,thus,limiting the duration of the trace.4.2Connection IdentificationTo collect the statisticalflow information necessary for the clus-tering evaluations,theflows must be identified within the traces. Theseflows,also known as connections,are a bidirectional ex-change of packets between two nodes.In the traces,the data is not exclusively from connection-based transport layer protocols such as TCP.While this study focused solely on the TCP-based applications it should be noted that statis-ticalflow information could be calculated for UDP traffic also.We identified the start of a connection using TCP’s3-way handshake and terminated a connection when FIN/RST packets were received. In addition,we assumed that aflow is terminated if the connection was idle for over90seconds.The statisticalflow characteristics considered include:total num-ber of packets,mean packet size,mean payload size excluding headers,number of bytes transfered(in each direction and com-bined),and mean inter-arrival time of packets.Our decision to use these characteristics was based primarily on the previous work done by Zander et al.[17].Due the heavy-tail distribution of many of the characteristics and our use of Euclidean distance as our similarity metric,we found that the logarithms of the characteristics gives much better results for all the clustering algorithms[13,16].4.3Classification of the Data SetsThe publicly available Auckland IV traces include no payload information.Thus,to determine the connections“true”classifica-tions port numbers are used.For this trace,we believe that a port-based classification will be largely accurate,as this archived trace predates the widespread use of dynamic port numbers.The classes considered for the Auckland IV datasets are DNS,FTP(control), FTP(data),HTTP,IRC,LIMEWIRE,NNTP,POP3,and SOCKS. LimeWire is a P2P application that uses the Gnutella protocol.In the Calgary trace,we were able to capture the full payloads of the packets,and therefore,were able to use an automated payload-based classification to determine the“true”classes.The payload-based classification algorithm and signatures we used is very sim-ilar to those described by Karagiannis et al.[9].We augmented their signatures to classify some newer P2P applications and instant messaging programs.The traffic classes considered for the Calgary trace are HTTP,P2P,SMTP,and POP3.The application breakdownConnections%Bytes1,132,92047.3% P2P17,578,995,93446,882 6.0% IMAP228,156,0603,6740.1% MSSQL23,824,93641,239 1.3%354,7989.6%of the Calgary trace is presented in Table1.The breakdown of the Auckland IV trace has been omitted due to space limitations.How-ever,HTTP is also the most dominant application accounting for over76%of the bytes and connections.4.4Testing MethodologyThe majority of the connections in both traces carry HTTP traf-fic.This unequal distribution does not allow for equal testing of the different classes.To address this problem,the Auckland data sets used for the clustering consist of1000random samples of each traf-fic class,and the Calgary data sets use2000random sample of each traffic category.This allows the test results to fairly judge the abil-ity on all traffic and not just HTTP.The size of the data sets were limited to8000connections because this was the upper bound that the AutoClass algorithm could cluster within a reasonable amount of time(4-10hours).In addition,to achieve a greater confidence in the results we generated10different data sets for each trace.Each of these data sets was then,in turn,used to evaluate the cluster-ing algorithms.We report the minimum,maximum,and average results from the data sets of each trace.In the future,we plan on examining the practical issue of what is the best way to pick the connections used as samples to build the model.Some ways that we think this could be accomplished is by random selection or a weighted selection using different criteria such as bytes transfered or duration.Also,in order to get a reason-able representative model of the traffic,one would need to select a fairly large yet manageable number of samples.We found that K-Means and DBSCAN algorithms are able to cluster much larger data sets(greater than100,000)within4-10hours.5.EXPERIMENTAL RESULTSIn this section,the overall effectiveness of each clustering algo-rithm is evaluatedfirst.Next,the number of objects in each cluster produced by the algorithms are analyzed.5.1Algorithm EffectivenessThe overall effectiveness of the clustering algorithms is calcu-lated using overall accuracy.This overall accuracy measurement determines how well the clustering algorithm is able to create clus-ters that contain only a single traffic category.The traffic class that makes up the majority of the connections in a cluster is used to label the cluster.The number of correctly classified connections in a cluster is referred to as the True Pos-itives(TP).Any connections that are not correctly classified are considered False Positives(FP).Any connection that has not been assigned to a cluster is labelled as noise.The overall accuracy is thus calculated as follows:overall accuracy=P T P for all clusters0 0.1 0.2 0.3 0.4 0.5 0.6 0.7 0.8 0.9 1 020406080 100 120 140 160O v e r a l l A c c u r a c yNumber of ClustersCalgary AucklandIVFigure 1:Accuracy using K-Means 0 0.1 0.2 0.3 0.4 0.5 0.6 0.7 0.8 0.9 1 00.010.020.030.04O v e r a l l A c c u r a c yEpsilon DistanceAuckland IV (3 minPts)Calgary (3 minPts)Figure 2:Accuracy using DBSCAN0 0.1 0.2 0.3 0.4 0.5 0.6 0.7 0.8 0.9 1 00.010.020.03 0.04O v e r a l l A c c u r a c yEpsilon Distance3 minPts 6 minPts 12 minPts 24 minPtsFigure 3:Parametrization of DBSCANTable 2:Accuracy using AutoClass Data Set Minimum Auckland IV 91.5%88.7%90.0%5.1.1K-Means ClusteringThe K-Means algorithm has an input parameter of K.This inputparameter as mentioned in Section 3.1,is the number of disjoint partitions used by K-Means.In our data sets,we would expect there would be at least one cluster for each traffic class.In ad-dition,due to the diversity of the traffic in some classes such as HTTP (e.g.,browsing,bulk download,streaming)we would ex-pect even more clusters to be formed.Therefore,based on this,the K-Means algorithm was evaluated with K initially being 10and K being incremented by 10for each subsequent clustering.The min-imum,maximum,and average results for the K-Means clustering algorithm are shown in Figure 1.Initially,when the number of clusters is small the overall ac-curacy of K-Means is approximately 49%for the Auckland IV data sets and 67%for the Calgary data sets.The overall accuracy steadily improves as the number of clusters increases.This contin-ues until K is around 100with the overall accuracy being 79%and 84%on average,for the Auckland IV and Calgary data sets,respec-tively.At this point,the improvement is much more gradual with the overall accuracy only improving by an additional 1.0%when K is 150in both data sets.When K is greater than 150,the improve-ment is further diminished with the overall accuracy improving to the high 80%range when K is 500.However,large values of K increase the likelihood of over-fitting.5.1.2DBSCAN ClusteringThe accuracy results for the DBSCAN algorithm are presented in Figure 2.Recall that DBSCAN has two input parameters (minPts,eps).We varied these parameters,and in Figure 2report results for the combination that produce the best clustering results.The values used for minPts were tested between 3and 24.The eps dis-tance was tested from 0.005to 0.040.Figure 3presents results for different combinations of (minPts,eps)values for the Calgary data sets.As may be expected,when the minPts was 3better results were produced than when the minPts was 24because smaller clus-ters are formed.The additional clusters found using three minPts were typically small clusters containing only 3to 5connections.When using minPts equal to 3while varying the eps distance between 0.005and 0.020(see Figure 2),the DBSCAN algorithm improved its overall accuracy from 59.5%to 75.6%for the Auck-land IV data sets.For the Calgary data sets,the DBSCAN algo-rithm improved its overall accuracy from 32.0%to 72.0%as the eps distance was varied with these same values.The overall ac-curacy for eps distances greater than 0.020decreased significantly0.1 0.2 0.3 0.4 0.5 0.6 0.7 0.8 0.9 1 0 0.1 0.2 0.3 0.4 0.5 0.6 0.7 0.8 0.9 1% C o n n e c t i o n s% ClustersDBSCAN K-Means AutoClassFigure 4:CDF of cluster weightsas the distance increased.Our analysis indicates that this large de-crease occurs because the clusters of different traffic classes merge into a single large cluster.We found that this larger cluster was for connections with few packets,few bytes transfered,and short dura-tions.This cluster contained typically equal amounts of P2P,POP3,and SMTP connections.Many of the SMTP connections were for emails with rejected recipient addresses and connections immedi-ately closed after connecting to the SMTP server.For POP3,many of the connections contained instances where no email was in the users mailbox.Gnutella clients attempting to connect to a remote node and having its “GNUTELLA CONNECT”packets rejected accounted for most of the P2P connections.5.1.3AutoClass ClusteringThe results for the AutoClass algorithm are shown in Table 2.For this algorithm,the number of clusters and the cluster param-eters are automatically determined.Overall,the AutoClass algo-rithm has the highest accuracy.On average,AutoClass is 92.4%and 88.7%accurate in the Auckland IV and Calgary data sets,re-spectively.AutoClass produces an average of 167clusters for the Auckland IV data sets,and 247clusters for the Calgary data sets.5.2Cluster WeightsFor the traffic classification problem,the number of clusters pro-duced by a clustering algorithm is an important consideration.The reason being that once the clustering is complete,each of the clus-ters must be labelled.Minimizing the number of clusters is also cost effective during the classification stage.One way of reducing the number of clusters to label is by evalu-ating the clusters with many connections in them.For example,if a clustering algorithm with high accuracy places the majority of the connections in a small subset of the clusters,then by analyzing only this subset a majority of the connections can be classified.Figure 4shows the percentage of connections represented as the percentage of clusters increases,using the Auckland IV data sets.In this eval-uation,the K-Means algorithm had 100for K.For the DBSCAN and AutoClass algorithms,the number of clusters can not be set.0.50.6 0.7 0.8 0.9 1P r e c i s i o nFigure 5:Precision using DBSCAN,K-Means,and AutoClass DBSCAN uses 0.03for eps,3for minPts,and has,on average,190clusters.We selected this point because it gave the best overall accuracy for DBSCAN.AutoClass has,on average,167clusters.As seen in Figure 4,both K-Means and AutoClass have more evenly distributed clusters than DBSCAN.The 15largest clusters produced by K-Means only contain 50%of the connections.In contrast,for the DBSCAN algorithm the five largest clusters con-tain over 50%of the connections in the data sets.These five clus-ters identified 75.4%of the NNTP,POP3,SOCKS,DNS,and IRC connections with a 97.6%overall accuracy.These results are un-expected when considering that by only looking at five of the 190clusters,one can identify a significant portion of traffic.Qualita-tively similar results were obtained for the Calgary data sets.6.DISCUSSIONThe DBSCAN algorithm is the only algorithm considered in this paper that can label connections as noise.The K-Means and Au-toClass algorithms place every connection into a cluster.The con-nections that are labelled as noise reduce the overall accuracy of the DBSCAN algorithm because they are regarded as misclassified.We have found some interesting results by excluding the connec-tions labelled as noise and just examining the clusters produced by DBSCAN.Figure 5shows the precision values for the DBSCAN (eps=0.02,minPts=3),the K-Means (K=190),and the AutoClass algorithms using the Calgary data sets.Precision is the ratio of TP to FP for a traffic class.Precision measures the accuracy of the clusters to classify a particular category of traffic.Figure 5shows that for the Calgary data sets,the DBSCAN algo-rithm has the highest precision values for three of the four classes of traffic.While not shown for the Auckland IV data sets,seven of the nine traffic classes have average precision values over 95%.This shows that while DBSCAN’s overall accuracy is lower than K-Means and AutoClass it produces highly accurate clusters.Another noteworthy difference among the clustering algorithms is the time required to build the models.On average to build the models,the K-Means algorithm took 1minute,the DBSCAN algo-rithm took 3minutes,and the AutoClass algorithm took 4.5hours.Clearly,the model building phase of AutoClass is time consum-ing.We believe this may deter systems developers from using this algorithm even if the frequency of retraining the model is low.7.CONCLUSIONSIn this paper,we evaluated three different clustering algorithms,namely K-Means,DBSCAN,and AutoClass,for the network traffic classification problem.Our analysis is based on each algorithm’s ability to produce clusters that have a high predictive power of a single traffic class,and each algorithm’s ability to generate a min-imal number of clusters that contain the majority of the connec-tions.The results showed that the AutoClass algorithm produces the best overall accuracy.However,the DBSCAN algorithm hasgreat potential because it places the majority of the connections in a small subset of the clusters.This is very useful because these clusters have a high predictive power of a single category of traffic.The overall accuracy of the K-Means algorithm is only marginally lower than that of the AutoClass algorithm,but is more suitable for this problem due to its much faster model building time.Ours in a work-in-progress and we continue to investigate these and other clustering algorithms for use as an efficient classification tool.8.ACKNOWLEDGMENTSThis work was supported by the Natural Sciences and Engineer-ing Research Council (NSERC)of Canada and Informatics Circle of Research Excellence (iCORE)of the province of Alberta.We thank Carey Williamson for his comments and suggestions which helped improve this paper.9.REFERENCES[1]P.Cheeseman and J.Strutz.Bayesian Classification (AutoClass):Theory and Results.In Advances in Knowledge Discovery and Data Mining,AAI/MIT Press,USA ,1996.[2]A.P.Dempster,N.M.Paird,and D.B.Rubin.Maximum likelihoodfrom incomeplete data via the EM algorithm.Journal of the Royal Statistical Society ,39(1):1–38,1977.[3]C.Dews,A.Wichmann,and A.Feldmann.An analysis of internetchat systems.In IMC’03,Miami Beach,USA,Oct 27-29,2003.[4]M.B.Eisen,P.T.Spellman,P.O.Brown,and D.Botstein.ClusterAnalysis and Display of Genome-wide Expression Patterns.Genetics ,95(1):14863–15868,1998.[5]M.Ester,H.Kriegel,J.Sander,and X.Xu.A Density-basedAlgorithm for Discovering Clusters in Large Spatial Databases with Noise.In 2nd Int.Conf.on Knowledge Discovery and Data Mining (KDD 96),Portland,USA,1996.[6]P.Haffner,S.Sen,O.Spatscheck,and D.Wang.ACAS:AutomatedConstruction of Application Signatures.In SIGCOMM’05MineNet Workshop ,Philadelphia,USA,August 22-26,2005.[7]A.K.Jain and R.C.Dubes.Algorithms for Clustering Data .PrenticeHall,Englewood Cliffs,USA,1988.[8]T.Karagiannis,A.Broido,M.Faloutsos,and K.claffy.TransportLayer Identification of P2P Traffic.In IMC’04,Taormina,Italy,October 25-27,2004.[9]T.Karagiannis,K.Papagiannaki,and M.Faloutsos.BLINK:Multilevel Traffic Classification in the Dark.In SIGCOMM’05,Philadelphia,USA,August 21-26,2005.[10]A.McGregor,M.Hall,P.Lorier,and J.Brunskill.Flow ClusteringUsing Machine Learning Techniques.In PAM 2004,Antibes Juan-les-Pins,France,April 19-20,2004.[11]A.W.Moore and K.Papagiannaki.Toward the AccurateIdentification of Network Applications.In PAM 2005,Boston,USA,March 31-April 1,2005.[12]A.W.Moore and D.Zuev.Internet Traffic Classification UsingBayesian Analysis Techniques.In SIGMETRIC’05,Banff,Canada,June 6-10,2005.[13]V .Paxson.Empirically-Derived Analytic Models of Wide-Area TCPConnections.IEEE/ACM Transactions on Networking ,2(4):316–336,August 1998.[14]M.Roughan,S.Sen,O.Spatscheck,and N.Duffield.Class-of-Service Mapping for QoS:A Statistical Signature-based Approach to IP Traffic Classification.In IMC’04,Taormina,Italy,October 25-27,2004.[15]S.Sen,O.Spatscheck,and D.Wang.Accurate,Scalable In-NetworkIdentification of P2P Traffic Using Application Signatures.In WWW2005,New York,USA,May 17-22,2004.[16]I.H.Witten and E.Frank.(2005)Data Mining:Pratical MachineLearning Tools and Techniques .Morgan Kaufmann,San Francisco,2nd edition,2005.[17]S.Zander,T.Nguyen,and G.Armitage.Automated TrafficClassification and Application Identification using Machine Learning.In LCN’05,Sydney,Australia,Nov 15-17,2005.。
贝叶斯决策理论(英文)--非常经典!
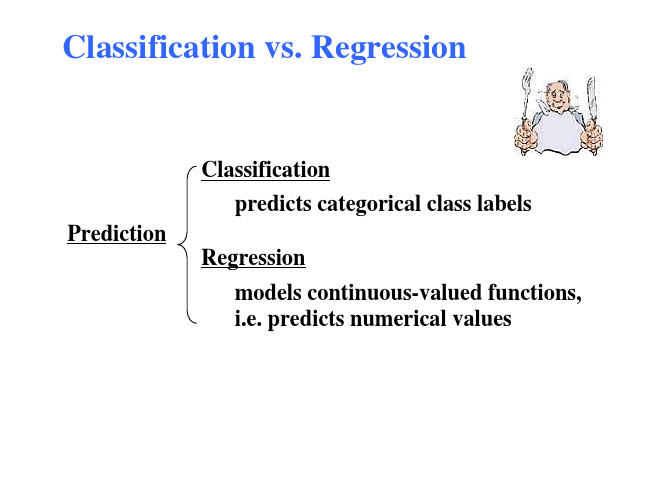
What is Bayesian classification?
Bayesian classification is based on Bayes theorem
Bayesian classifiers have exhibited high accuracy and fast speed when applied to large databases
贝叶斯决策理论英文非常经典
Classification vs. Regression
Classification predicts categorical class labels Prediction Regression models continuous-valued functions, i.e. predicts numerical values
Two step process of prediction (I)
Step 1: Construct a model to describe a training set
• the set of tuples used for model construction is called training set • the set of tuples can be called as a sample (a tuple can also be called as a sample) • a tuple is usually called an example (usually with the label) or an instance (usually without the label) • the attribute to be predicted is called label Training algorithm
LearnerAutonomy
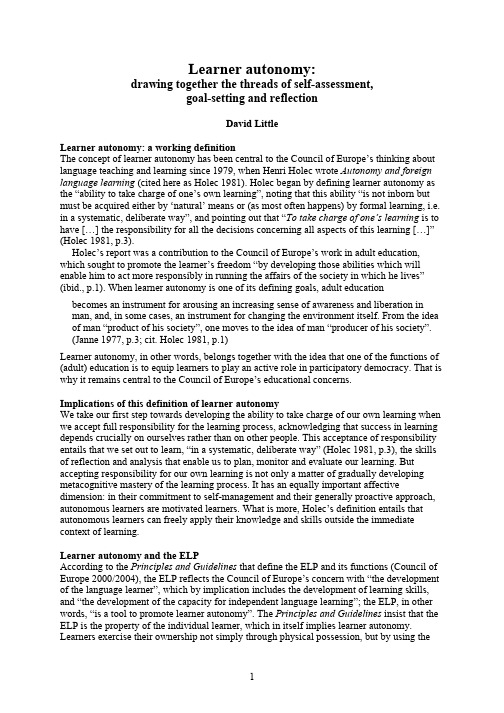
Learner autonomy:drawing together the threads of self-assessment,goal-setting and reflectionDavid LittleLearner autonomy: a working definitionThe concept of learner autonomy has been central to the Council of Europe’s thinking about language teaching and learning since 1979, when Henri Holec wrote Autonomy and foreign language learning (cited here as Holec 1981). Holec began by defining learner autonomy as the “ability to take charge of one’s own learning”, noting that this ability “is not inborn but must be acquired either by ‘natural’ means or (as most often happens) by formal learning, i.e. in a systematic, deliberate way”, and pointing out that “To take charge of one’s learning is to have […] the responsibility for all the decisions concerning all aspects of this learning […]” (Holec 1981, p.3).Holec’s report was a contribution to the Council of Europe’s work in adult education, which sought to promote the learner’s freedom “by developing those abilities which will enable him to act more responsibly in running the affairs of the society in which he lives” (ibid., p.1). When learner autonomy is one of its defining goals, adult education becomes an instrument for arousing an increasing sense of awareness and liberation in man, and, in some cases, an instrument for changing the environment itself. From the idea of man “product of his society”, one moves to the idea of man “producer of his society”.(Janne 1977, p.3; cit. Holec 1981, p.1)Learner autonomy, in other words, belongs together with the idea that one of the functions of (adult) education is to equip learners to play an active role in participatory democracy. That is why it remains central to the Council of Europe’s educational concerns.Implications of this definition of learner autonomyWe take our first step towards developing the ability to take charge of our own learning when we accept full responsibility for the learning process, acknowledging that success in learning depends crucially on ourselves rather than on other people. This acceptance of responsibility entails that we set out to learn, “in a systematic, deliberate way” (Holec 1981, p.3), the skills of reflection and analysis that enable us to plan, monitor and evaluate our learning. But accepting responsibility for our own learning is not only a matter of gradually developing metacognitive mastery of the learning process. It has an equally important affective dimension: in their commitment to self-management and their generally proactive approach, autonomous learners are motivated learners. What is more, Holec’s definition entails that autonomous learners can freely apply their knowledge and skills outside the immediate context of learning.Learner autonomy and the ELPAccording to the Principles and Guidelines that define the ELP and its functions (Council of Europe 2000/2004), the ELP reflects the Council of Europe’s concern with “the development of the language learner”, which by implication includes the development of learning skills, and “the development of the capacity for independent language learning”; the ELP, in other words, “is a tool to promote learner autonomy”. The Principles and Guidelines insist that the ELP is the property of the individual learner, which in itself implies learner autonomy. Learners exercise their ownership not simply through physical possession, but by using theELP to plan, monitor and evaluate their learning. In this, self-assessment plays a central role: the ongoing, formative self-assessment that is supported by the “can do” checklists attached to the language biography, and the periodic, summative self-assessment of the language passport, which is related to the so-called self-assessment grid in the CEF (Council of Europe 2001, pp.26–27).Learner autonomy and the CEFThe CEF does not concern itself with learner autonomy as such. However, learner autonomy is implied by the concept of savoir-apprendre (“ability to learn”), which the CEF defines as “the ability to observe and participate in new experience and to incorporate new knowledge into existing knowledge, modifying the latter where necessary” (Council of Europe 2001,p.106). When the CEF tells us that “ability to learn has several components, such as language and communication awareness; general phonetic skills; study skills; and heuristic skills” (CEF, pp.107), we may be prompted to recall the ways in which the ELP can support the development of reflective learning skills.Why is learner autonomy important?According to a large body of empirical research in social psychology, autonomy – “feeling free and volitional in one’s actions” (Deci 1995, p.2) – is a basic human need. It is nourished by, and in turn nourishes, our intrinsic motivation, our proactive interest in the world around us. This explains how learner autonomy solves the problem of learner motivation: autonomous learners draw on their intrinsic motivation when they accept responsibility for their own learning and commit themselves to develop the skills of reflective self-management in learning; and success in learning strengthens their intrinsic motivation. Precisely because autonomous learners are motivated and reflective learners, their learning is efficient and effective (conversely, all learning is likely to succeed to the extent that the learner is autonomous). And the efficiency and effectiveness of the autonomous learner means that the knowledge and skills acquired in the classroom can be applied to situations that arise outside the classroomAutonomy in formal language learningIn formal educational contexts, learner autonomy entails reflective involvement in planning, implementing, monitoring and evaluating learning. But note that language learning depends crucially on language use: we can learn to speak only by speaking, to read only by reading, and so on. Thus in formal language learning, the scope of learner autonomy is always constrained by what the learner can do in the target language; in other words, the scope of our autonomy as language learners is partly a function of the scope of our autonomy as target language users.The development of autonomy in language learning is governed by three basic pedagogical principles:•learner involvement – engaging learners to share responsibility for the learning process (the affective and the metacognitive dimensions);•learner reflection – helping learners to think critically when they plan, monitor and evaluate their learning (the metacognitive dimensions);•appropriate target language use – using the target language as the principal medium of language learning (the communicative and the metacognitive dimensions).What does the teacher do?According to these three principles the teacher should•use the target language as the preferred medium of classroom communication and require the same of her learners;•involve her learners in a non-stop quest for good learning activities, which are shared, discussed, analysed and evaluated with the whole class – in the target language, to begin with in very simple terms;•help her learners to set their own learning targets and choose their own learning activities, subjecting them to discussion, analysis and evaluation – again, in the target language; •require her learners to identify individual goals but pursue them through collaborative work in small groups;•require her learners to keep a written record of their learning – plans of lessons and projects, lists of useful vocabulary, whatever texts they themselves produce;•engage her learners in regular evaluation of their progress as individual learners and as a class – in the target language.ReferencesCouncil of Europe, 2000/2004: European Language Portfolio (ELP): Principles and Guidelines. With added explanatory notes. Strasbourg: Council of Europe.(DGIV/EDU/LANG (2000) 33 rev.1)Council of Europe, 2001: Common European Framework of Reference for Languages: Learning, teaching, assessment. Cambridge: Cambridge University Press.Deci, E. (with R. Flaste), 1995: Why we do what we do: understanding self-motivation. New York: Penguin.Holec, H., 1981: Autonomy and foreign language learning. Oxford: Pergamon. (First published 1979, Strasbourg: Council of Europe)。
Autonomous-learning
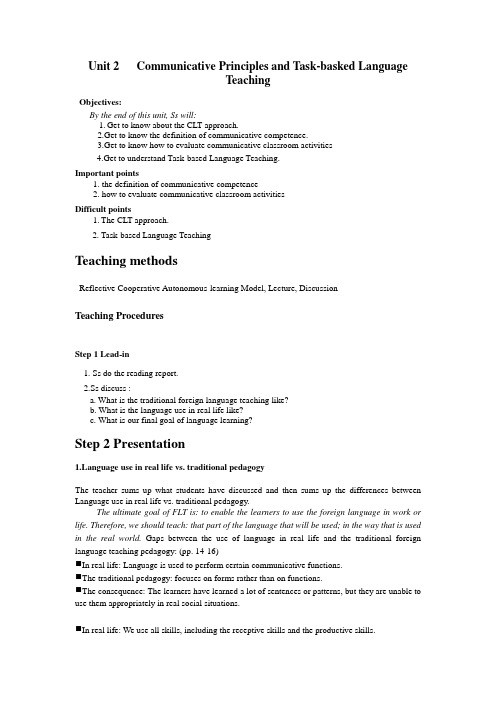
Unit 2 Communicative Principles and Task-basked LanguageTeachingObjectives:By the end of this unit, Ss will:1.Get to know about the CLT approach.2.Get to know the definition of communicative competence.3.Get to know how to evaluate communicative classroom activities4.Get to understand Task-based Language Teaching.Important points1. the definition of communicative competence2. how to evaluate communicative classroom activitiesDifficult points1.The CLT approach.2. Task-based Language TeachingTeaching methodsReflective Cooperative Autonomous-learning Model, Lecture, DiscussionTeaching ProceduresStep 1 Lead-in1. Ss do the reading report.2.Ss discuss :a. What is the traditional foreign language teaching like?b. What is the language use in real life like?c. What is our final goal of language learning?Step 2 Presentationnguage use in real life vs. traditional pedagogyThe teacher sums up what students have discussed and then sums up the differences between Language use in real life vs. traditional pedagogy.The ultimate goal of FLT is: to enable the learners to use the foreign language in work or life. Therefore, we should teach: that part of the language that will be used; in the way that is used in the real world. Gaps between the use of language in real life and the traditional foreign language teaching pedagogy: (pp. 14-16)In real life: Language is used to perform certain communicative functions.The traditional pedagogy: focuses on forms rather than on functions.The consequence: The learners have learned a lot of sentences or patterns, but they are unable to use them appropriately in real social situations.In real life: We use all skills, including the receptive skills and the productive skills.The traditional pedagogy tends to focus on one or two language skills and ignore the others.The consequence: The learners cannot use the language in an integrated way.In real life: Language is always used in a certain context.The traditional pedagogy tends to isolate language from its context. e.g. the passiveThe consequence:The students are puzzled about how to use the language in a particular context.2.What is communicative competence?2.1Defintion of communicative competenceHedge (2000: 46-55) discusses five main components of communicative competence: linguistic competence, pragmatic competence, discourse competence, strategic competence, and fluency. (PP28-19)1)linguistic competence语言能力是指理解语言本身,语言形式及其意义的能力。
211178229_基于DCGAN的点云滤波方法
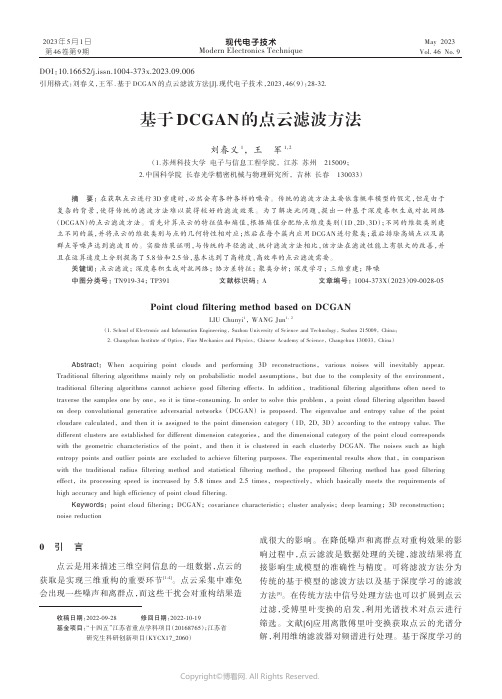
现代电子技术Modern Electronics Technique2023年5月1日第46卷第9期May 2023Vol.46No.90引言点云是用来描述三维空间信息的一组数据,点云的获取是实现三维重构的重要环节[1⁃4]。
点云采集中难免会出现一些噪声和离群点,而这些干扰会对重构结果造成很大的影响。
在降低噪声和离群点对重构效果的影响过程中,点云滤波是数据处理的关键,滤波结果将直接影响生成模型的准确性与精度。
可将滤波方法分为传统的基于模型的滤波方法以及基于深度学习的滤波方法[5]。
在传统方法中信号处理方法也可以扩展到点云过滤,受傅里叶变换的启发,利用光谱技术对点云进行筛选。
文献[6]应用离散傅里叶变换获取点云的光谱分解,利用维纳滤波器对频谱进行处理。
基于深度学习的基于DCGAN 的点云滤波方法刘春义1,王军1,2(1.苏州科技大学电子与信息工程学院,江苏苏州215009;2.中国科学院长春光学精密机械与物理研究所,吉林长春130033)摘要:在获取点云进行3D 重建时,必然会有各种各样的噪音。
传统的滤波方法主要依靠概率模型的假定,但是由于复杂的背景,使得传统的滤波方法难以获得较好的滤波效果。
为了解决此问题,提出一种基于深度卷积生成对抗网络(DCGAN )的点云滤波方法。
首先计算点云的特征值和熵值,根据熵值分配给点维度类别(1D 、2D 、3D );不同的维数类别建立不同的簇,并将点云的维数类别与点的几何特性相对应;然后在每个簇内应用DCGAN 进行聚类;最后排除高熵点以及离群点等噪声达到滤波目的。
实验结果证明,与传统的半径滤波、统计滤波方法相比,该方法在滤波性能上有很大的改善,并且在运算速度上分别提高了5.8倍和2.5倍,基本达到了高精度、高效率的点云滤波需要。
关键词:点云滤波;深度卷积生成对抗网络;协方差特征;聚类分析;深度学习;三维重建;降噪中图分类号:TN919⁃34;TP391文献标识码:A文章编号:1004⁃373X (2023)09⁃0028⁃05Point cloud filtering method based on DCGANLIU Chunyi 1,WANG Jun 1,2(1.School of Electronic and Information Engineering ,Suzhou University of Science and Technology ,Suzhou 215009,China ;2.Changchun Institute of Optics ,Fine Mechanics and Physics ,Chinese Academy of Science ,Changchun 130033,China )Abstract :When acquiring point clouds and performing 3D reconstructions ,various noises will inevitably appear.Traditional filtering algorithms mainly rely on probabilistic model assumptions ,but due to the complexity of the environment ,traditional filtering algorithms cannot achieve good filtering effects.In addition ,traditional filtering algorithms often need to traverse the samples one by one ,so it is time⁃consuming.In order to solve this problem ,a point cloud filtering algorithm basedon deep convolutional generative adversarial networks (DCGAN )is proposed.The eigenvalue and entropy value of the point cloudare calculated ,and then it is assigned to the point dimension category (1D,2D,3D )according to the entropy value.Thedifferent clusters are established for different dimension categories ,and the dimensional category of the point cloud corresponds with the geometric characteristics of the point ,and then it is clustered in each clusterby DCGAN.The noises such as highentropy points and outlier points are excluded to achieve filtering purposes.The experimental results show that ,in comparison with the traditional radius filtering method and statistical filtering method ,the proposed filtering method has good filtering effect ,its processing speed is increased by 5.8times and 2.5times ,respectively ,which basically meets the requirements ofhigh accuracy and high efficiency of point cloud filtering.Keywords :point cloud filtering ;DCGAN ;covariance characteristic ;cluster analysis ;deep learning ;3D reconstruction ;noise reductionDOI :10.16652/j.issn.1004⁃373x.2023.09.006引用格式:刘春义,王军.基于DCGAN 的点云滤波方法[J].现代电子技术,2023,46(9):28⁃32.收稿日期:2022⁃09⁃28修回日期:2022⁃10⁃19基金项目:“十四五”江苏省重点学科项目(20168765);江苏省研究生科研创新项目(KYCX17_2060)28第9期点云滤波中常用的基础网络模型包括自编码器(Autoencoder,AE)、卷积神经网络(Convolutional Neural Network,CNN)和对抗生成网络(Generative Adversarial Network,GAN)等[7]。
三种自动勾画软件应用于中上腹部危及器官勾画的准确性研究

RESEARCH WORK66中国医疗设备 2021年第36卷 03期 V OL.36 No.03引言随着科学技术的发展,调强放疗技术被越来越广泛地应用在临床肿瘤的治疗中,其高度的靶区适形度和陡峭的剂量梯度大大地提高了肿瘤治疗的增益比[1-2]。
因此在放疗计划设计阶段就要求临床医师精确定义和勾画靶区与危及器官(Organs at Risk ,OAR )。
传统的手工勾画OAR 不仅费时费力,而且重复性差,此外患者在经过一段时间放疗后,身体的解剖结构会发生变化,肿瘤缩小,则需要重新定位并制订放疗计划[3-5],而繁琐重复的OAR 勾画无疑大幅降低放疗计划制定的效率,也给临床医师造成负担。
目前国内外有基于图谱库和形变配准融合自动勾画软件应用于头颈部肿瘤[6-9]、胸部肿瘤[10-14]和下腹部肿瘤[15-20]的OAR 自动勾画临床可行性研究,较少检索到关于中上腹部的危及三种自动勾画软件应用于中上腹部危及器官勾画的准确性研究李桢,洪文松,胡丽彩广东省第二人民医院 放疗科,广东 广州 510317[摘 要] 目的 比较三种自动勾画软件(Pinnacle 9.10、LinkingMed 和Manteia )勾画上腹部危及器官(OAR )的准确性。
方法 选取了26例上腹部肿瘤患者,由一名资深的临床医师手动勾画OAR (肝脏、脊髓、双肾、胰腺和胃),并采用三种软件对其进行自动勾画。
以手动勾画为金标准,计算并比较三种自动勾画结果的质心偏差(Center of Mass Deviation ,DC )、Dice 相似性系数(Dice Similarity Coefficient ,DSC )、Hausdorff 距离(Hausdorff Dista nce ,HD )、包容性指数(Inclusive Index ,IncI )和敏感性指数(Sensitivity Inde x ,SI )。
采用单因素方差分析评价各项指标的统计学差异,同时比较了三种软件勾画结果的准确性。
Autonomous Learning

Autonomous LearningAutonomous learning,or learner autonomy,I think it's a very important capability,which is insufficient for Chinese students. Compared with other countries' students,Chinese students are accustomed to accept the standard answers without questions,but we all know,a little learning is a dangerous thing. So I approve of autonomous learning in Chinese education.According to the definition of autonomous learning,which is a school of education which sees learners as individuals who can and should be autonomous,in another word,be responsible for their own learning climate. We can see,individuality,I mean the learners are the main part in the autonomous learning,not teachers,not parents. Autonomous education helps students develop their self-consciousness, vision, practicality and freedom of discussion. These attributes serve to aid the student in his or her independent learning.we live in a learning society, That's far more from enough for us to learning knowledge only from the books and teachers. When we were kids,we had been told must be respect teachers and could not do anything bad. We should honor the teacher and respect his teaching. We should be diligent at our lessons. And we should on questions. The teachers flog learning into us,and we have to accept eventually. Burying ourselves in books,that trains many excellent students,who is good at math,who is good at literature,who is good at geography,but what stops us to forward to the Nobel Prize, to independent innovation? Because most of us students,lost the basic capability of autonomous learning. People don't learn anything today,I think it's a great shame the way educational standards are declining today. Education is about something much more important. It's about teaching people how to live, how to get on with one another, how to form relationships. It's about understanding things, not just knowing them. Yes,of course,seven sevens are forty-nine. But what does that mean? Our teachers never told us, It's not just a formula, maybe teachers would say so.but we need to know, and we need to understand. Autonomous learning is very popular with those who home educate their children. The child usually gets to decide what projects they wish to tackle or what interests to pursue. In home education this can be instead of or in addition to regular subjects like doing math or English. But some teachers and parents still approve of school education. So autonomous learning is a good choice or not,that depends on your ideas. But in one respect at least, the definition of autonomous learning is uncontroversial: it is the exercise of the capacity to think for oneself. Just as there is little contention over the minimal definition of what autonomous learning is, there is little dispute over how it is recognised. It is generally accepted that the capacity for autonomous learning is recognised by its expression in a number of different forms, such as the ability to understand an argument and set it in context; to search for, read, and understand relevant primary and secondary material; to explain and articulate an issue in oral and written form to others; and to demonstrate an awareness of the consequences of what has been learned. So I think developing responsible and autonomous learners is the key to motivating students for real wise teachers.Maybe you don't understand the academic definition of autonomous learning,neitherdo I.However, the minimal definition of autonomous learning can support two different views about the issue. One view is that autonomous learning simply and solely constitutes learning that students do for themselves. For those that hold such a view, an autonomous learner is someone who, given minimal information, would, for example, go away to the library, find sources for themselves and work by themselves. In the discipline of philosophy such work would amount to the student sitting down with a text and trying to come to an understanding of it on their own. Another view, however, and one that we believe significantly contradicts the first, has it that autonomous learning involves showing the student how to do something in such a way that they are then capable of undertaking a comparable activity by themselves. From this perspective, autonomous learning becomes the habitual exercise of skills, developed and perfected through continuous practice, which come to be second nature.In Chinese education,most teachers are frustrated by their unmotivated students. What they may not know is how important the connection is between student motivation and self-determination. Research has shown that motivation is related to whether or not students have opportunities to be autonomous and to make important academic choices. Having choices allows children to feel that they have control or ownership over their own learning. This, in turn, helps them develop a sense of responsibility and self-motivation. When students feel a sense of ownership, they want to engage in academic tasks and persist in learning. That's the first step for students to learn autonomously. Passion of the study makes students feel happy and energetic.There is no doubt that autonomous learners,who make many teachers fear that giving students more choice will lead to their losing control over classroom management. Research tells us that in fact the opposite happens. When students understand their role as agent over their feeling, thinking, and learning behaviors, they are more likely to take responsibility for their learning. To be autonomous learners, however, students need to have some choice and control. And teachers need to learn how to help students develop the ability to make appropriate choices and take control over their own learning. So teachers need not to worry about the uncontrollableness of the students.Autonomous learning is a lean approach to learning. At least, autonomous learning can help us to improve the independent creative ability,we need to know thing for a fact and know the ways and wherefores of it. Maybe we have many immature ideas. So what? Just learn it,Just do yourself. Before the coming of knowledge explosion,many experts in education field put forward that the mainly mission is not the knowledge any more, but the study of the study method. So we need to understand,not rote learning. The motivation for learning is our thirst for knowledge,our passions. learning can take place only when there is motivation. That's autonomous learning!。
自动wEKA 2.0:自动模型选择和超参数优化在wEKA说明书
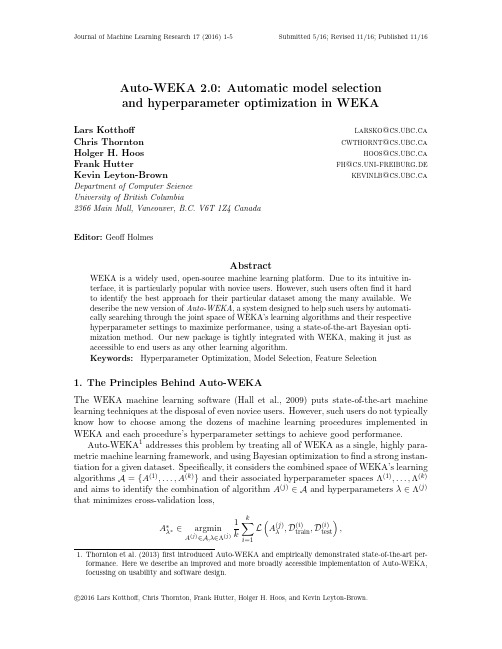
Journal of Machine Learning Research17(2016)1-5Submitted5/16;Revised11/16;Published11/16 Auto-WEKA2.0:Automatic model selectionand hyperparameter optimization in WEKALars Kotthoff*************.ca Chris Thornton***************.ca Holger H.Hoos***********.ca Frank Hutter******************.de Kevin Leyton-Brown**************.ca Department of Computer ScienceUniversity of British Columbia2366Main Mall,Vancouver,B.C.V6T1Z4CanadaEditor:GeoffHolmesAbstractWEKA is a widely used,open-source machine learning platform.Due to its intuitive in-terface,it is particularly popular with novice users.However,such users oftenfind it hard to identify the best approach for their particular dataset among the many available.We describe the new version of Auto-WEKA,a system designed to help such users by automati-cally searching through the joint space of WEKA’s learning algorithms and their respective hyperparameter settings to maximize performance,using a state-of-the-art Bayesian opti-mization method.Our new package is tightly integrated with WEKA,making it just as accessible to end users as any other learning algorithm.Keywords:Hyperparameter Optimization,Model Selection,Feature Selection1.The Principles Behind Auto-WEKAThe WEKA machine learning software(Hall et al.,2009)puts state-of-the-art machine learning techniques at the disposal of even novice users.However,such users do not typically know how to choose among the dozens of machine learning procedures implemented in WEKA and each procedure’s hyperparameter settings to achieve good performance.Auto-WEKA1addresses this problem by treating all of WEKA as a single,highly para-metric machine learning framework,and using Bayesian optimization tofind a strong instan-tiation for a given dataset.Specifically,it considers the combined space of WEKA’s learning algorithms A={A(1),...,A(k)}and their associated hyperparameter spacesΛ(1),...,Λ(k) and aims to identify the combination of algorithm A(j)∈A and hyperparametersλ∈Λ(j)that minimizes cross-validation loss,A∗λ∗∈argminA(j)∈A,λ∈Λ(j)1kk∑i=1L(A(j)λ,D(i)train,D(i)test),1.Thornton et al.(2013)first introduced Auto-WEKA and empirically demonstrated state-of-the-art per-formance.Here we describe an improved and more broadly accessible implementation of Auto-WEKA, focussing on usability and software design.Kotthoff,Thornton,Hutter,Hoos,Leyton-Brownwhere L (A λ,D (i )train ,D (i )test )denotes the loss achieved by algorithm A with hyperparameters λwhen trained on D (i )train and evaluated on D (i )test .We call this the combined algorithm selectionand hyperparameter optimization (CASH)problem.CASH can be seen as a blackbox func-tion optimization problem:determining argmin θ∈Θf (θ),where each configuration θ∈Θcomprises the choice of algorithm A (j )∈A and its hyperparameter settings λ∈Λ(j ).In this formulation,the hyperparameters of algorithm A (j )are conditional on A (j )being selected.For a given θrepresenting algorithm A (j )∈A and hyperparameter settings λ∈Λ(j ),f (θ)is then defined as the cross-validation loss 1k ∑k i =1L (A (j )λ,D (i )train ,D (i )test ).2Bayesian optimization (see,e.g.,Brochu et al.,2010),also known as sequential model-based optimization,is an iterative method for solving such blackbox optimization problems.In its n -th iteration,it fits a probabilistic model based on the first n −1function evaluations ⟨θi ,f (θi )⟩n −1i =1,uses this model to select the next θn to evaluate (trading offexploration of new parts of the space vs exploitation of regions known to be good)and evaluates f (θn ).While Bayesian optimization based on Gaussian process models is known to perform well for low-dimensional problems with numerical hyperparameters (see,e.g.,Snoek et al.,2012),tree-based models have been shown to be more effective for high-dimensional,structured,and partly discrete problems (Eggensperger et al.,2013),such as the highly conditional space of WEKA’s learning algorithms and their corresponding hyperparameters we face here.3Thornton et al.(2013)showed that tree-based Bayesian optimization methods yielded the best performance in Auto-WEKA,with the random-forest-based SMAC (Hutter et al.,2011)performing better than the tree-structured Parzen estimator,TPE (Bergstra et al.,2011).Auto-WEKA uses SMAC to determine the classifier with the best performance on the given data.2.Auto-WEKA 2.0Since the initial release of a usable research prototype in 2013,we have made substantial improvements to the Auto-WEKA package described by Thornton et al.(2013).At a prosaic level,we have fixed bugs,improved tests and documentation,and updated the software to work with the latest versions of WEKA and Java.We have also added four major features.First,we now support regression algorithms,expanding Auto-WEKA beyond its pre-vious focus on classification (starred entries in Fig.1).Second,we now support the op-timization of all performance metrics WEKA supports.Third,we now natively support parallel runs (on a single machine)to find good configurations faster and save the N best configurations of each run instead of just the single best.Fourth,Auto-WEKA 2.0is now fully integrated with WEKA.This is important,because the crux of Auto-WEKA lies in its simplicity:providing a push-button interface that requires no knowledge about the avail-able learning algorithms or their hyperparameters,asking the user to provide,in addition to the dataset to be processed,only a memory bound (1GB by default)and the overall time2.In fact,on top of machine learning algorithms and their respective hyperparameters,we also include attribute selection methods and their respective hyperparameters in the configurations θ,thereby jointly optimizing over their choice and the choice of algorithms.3.Conditional dependencies can also be accommodated in the Gaussian process framework (Hutter and Osborne,2013;Swersky et al.,2013),but currently,tree-based methods achieve better performance.Auto-WEKA2.0:Automatic model and hyperparameter selection in WEKA LearnersBayesNet2 DecisionStump*0 DecisionTable*4 GaussianProcesses*10 IBk*5 J489 JRip4 KStar*3 LinearRegression*3 LMT9Logistic1M5P4M5Rules4MultilayerPerceptron*8NaiveBayes2NaiveBayesMultinomial0OneR1PART4RandomForest7RandomTree*11REPTree*6SGD*5SimpleLinearRegression*0SimpleLogistic5SMO11SMOreg*13VotedPerceptron3ZeroR*0Ensemble MethodsStacking2Vote2 Meta-MethodsLWL5 AdaBoostM16 AdditiveRegression4AttributeSelectedClassifier2Bagging4RandomCommittee2RandomSubSpace3Attribute Selection MethodsBestFirst2GreedyStepwise4Figure1:Learners and methods supported by Auto-WEKA2.0,along with number of hyperparameters|Λ|.Every learner supports classification;starred learners also support regression.budget available for the entire learning process.4The overall budget is set to15minutes by default to accommodate impatient users;longer runs allow the Bayesian optimizer to search the space more thoroughly;we recommend at least several hours for production runs.The usability of the earlier research prototype was hampered by the fact that users had to download Auto-WEKA manually and run it separately from WEKA.In contrast, Auto-WEKA2.0is now available through WEKA’s package ers do not need to install software separately;everything is included in the package and installed automatically upon request.After installation,Auto-WEKA2.0can be used in two different ways:1.As a meta-classifier:Auto-WEKA can be run like any other machine learning algo-rithm in WEKA:via the GUI,the command-line interface,or the public API.Figure2 shows how to run it from the command line.2.Through the Auto-WEKA tab:This provides a customized interface that hides someof the complexity.Figure3shows the output of an example run.Source code for Auto-WEKA is hosted on GitHub(https:///automl/autoweka) and is available under the GPL license(version3).Releases are published to the WEKA package repository and available both through the WEKA package manager and from the Auto-WEKA project website(/autoweka).A manual describes how to use the WEKA package and gives a high-level overview for developers;we also provide lower-level Javadoc documentation.An issue tracker on GitHub,JUnit tests and the con-tinuous integration system Travis facilitate bug tracking and correctness of the code.Since its release on March1,2016,Auto-WEKA2.0has been downloaded more than15000times, with an average of about400downloads per week.4.Internally,to avoid using all its budget for executing a single slow learner,Auto-WEKA limits individualruns of any learner to1/12of the overall budget;it further limits feature search to1/60of the budget.Kotthoff,Thornton,Hutter,Hoos,Leyton-Brownjava-cp autoweka.jar weka.classifiers.meta.AutoWEKAClassifier -timeLimit5-t iris.arff-no-cvFigure2:Command-line call for running Auto-WEKA with a time limit of5minutes on training dataset iris.arff.Auto-WEKA performs cross-validation internally,so we disable WEKA’s cross-validation(-no-cv).Running with-h lists the available options.Figure3:Example Auto-WEKA run on the iris dataset.The resulting best classifier along with its parameter settings is printedfirst,followed by its performance.While Auto-WEKA runs,it logs to the status bar how many configurations it has evaluated so far.3.Related ImplementationsAuto-WEKA was thefirst method to use Bayesian optimization to automatically instantiate a highly parametric machine learning framework at the push of a button.This automated machine learning(AutoML)approach has recently also been applied to Python and scikit-learn(Pedregosa et al.,2011)in Auto-WEKA’s sister package,Auto-sklearn(Feurer et al., 2015).Auto-sklearn uses the same Bayesian optimizer as Auto-WEKA,but comprises a smaller space of models and hyperparameters,since scikit-learn does not implement as many different machine learning techniques as WEKA;however,Auto-sklearn includes additional meta-learning techniques.It is also possible to optimize hyperparameters using WEKA’s own grid search and MultiSearch packages.However,these packages only permit tuning one learner and one filtering method at a time.Grid search handles only one hyperparameter.Furthermore, hyperparameter names and possible values have to be specified by the user.Auto-WEKA2.0:Automatic model and hyperparameter selection in WEKAReferencesJ.Bergstra,R.Bardenet,Y.Bengio,and B.K´e gl.Algorithms for hyper-parameter opti-mization.In Advances in Neural Information Processing Systems24(NIPS’11),pages 2546–2554,2011.E.Brochu,V.Cora,and N.de Freitas.A tutorial on Bayesian optimization of expensive cost functions,with application to active user modeling and hierarchical reinforcement puting Research Repository(arXiv),abs/1012.2599,2010.K.Eggensperger,M.Feurer,F.Hutter,J.Bergstra,J.Snoek,H.Hoos,and K.Leyton-Brown.Towards an empirical foundation for assessing Bayesian optimization of hyper-parameters.In NIPS Workshop on Bayesian Optimization(BayesOpt’13),2013.M.Feurer,A.Klein,K.Eggensperger,J.Springenberg,M.Blum,and F.Hutter.Efficient and Robust Automated Machine Learning.In Advances in Neural Information Processing Systems28(NIPS’15),pages2944–2952,2015.M.Hall,E.Frank,G.Holmes,B.Pfahringer,P.Reutemann,and I.H.Witten.The WEKA Data Mining Software:An Update.SIGKDD Explor.Newsl.,11(1):10–18,Nov.2009. ISSN1931-0145.F.Hutter and M.Osborne.A Kernel for Hierarchical Parameter puting Re-search Repository(arXiv),abs/1310.5738,Oct.2013.F.Hutter,H.H.Hoos,and K.Leyton-Brown.Sequential Model-Based Optimization for General Algorithm Configuration.In Learning and Intelligent OptimizatioN Conference (LION5),pages507–523,2011.F.Pedregosa,G.Varoquaux,A.Gramfort,V.Michel,B.Thirion,O.Grisel,M.Blon-del,P.Prettenhofer,R.Weiss,V.Dubourg,J.Vanderplas,A.Passos,D.Cournapeau, M.Brucher,M.Perrot,and E.Duchesnay.Scikit-learn:Machine learning in Python. Journal of Machine Learning Research,12:2825–2830,2011.J.Snoek,rochelle,and R.P.Adams.Practical Bayesian optimization of machine learn-ing algorithms.In Advances in Neural Information Processing Systems25(NIPS’12), pages2951–2959,2012.K.Swersky, D.Duvenaud,J.Snoek, F.Hutter,and M.Osborne.Raiders of the lost architecture:Kernels for Bayesian optimization in conditional parameter spaces.In NIPS Workshop on Bayesian Optimization(BayesOpt’13),2013.C.Thornton, F.Hutter,H.H.Hoos,and K.Leyton-Brown.Auto-WEKA:Combined selection and hyperparameter optimization of classification algorithms.In19th ACM SIGKDD Conference on Knowledge Discovery and Data Mining(KDD’13),2013.。
- 1、下载文档前请自行甄别文档内容的完整性,平台不提供额外的编辑、内容补充、找答案等附加服务。
- 2、"仅部分预览"的文档,不可在线预览部分如存在完整性等问题,可反馈申请退款(可完整预览的文档不适用该条件!)。
- 3、如文档侵犯您的权益,请联系客服反馈,我们会尽快为您处理(人工客服工作时间:9:00-18:30)。
LIF-CMI, 39, rue F. Joliot Curie 13453 Marseille Cedex 13 FRANCE, fdenis,esposito@cmi.univ-mrs.fr
1
Introduction
Probabilistic automata (PFA) are formal objects which model stochastic languages, i.e. probability distributions over words [1]. They are composed of a structure which is a finite automaton (NFA) and of parameters associated with states and transitions which represent the probability for a state to be initial, terminal or the probability for a transition to be chosen. Given the structure of a probabilistic automaton A and a sequence of words u1 , . . . , un independently distributed according to a probability distribution P , computing parameters for A which maximize the likelihood of the observation is NP-hard [2]. However in practical cases, algorithms based on the EM (Expectation-Maximization ) method [3] can be used to compute approximate values. On the other hand, inferring a probabilistic automaton (structure and parameters) from a sequence of words is a widely open field of research. In some applications, prior knowledge may help to choose a structure (for example, the standard model for biological sequence analysis [4]). Without prior knowledge, a complete graph structure can be chosen. But it is likely that in general, inferring both the appropriate structure and parameters from data would provide better results (see for example [5]). Several learning frameworks can be considered to study inference of PFA. They often consist in adaptations to the stochastic case of classical learning models. We consider a variant of the identification in the limit model of Gold [6], adapted to the stochastic case in [7]. Given a PFA A and a sequence of words u1 , . . . , un , . . . independently drawn according to the associated distribution PA , an inference algorithm must compute a PFA An from each subsequence u1 , . . . , un such that with probability one, the support of An is stationary from
Abstract. Probabilistic finite automata (PFA) model stochastic languages, i.e. probability distributions over strings. Inferring PFA from stochastic data is an open field of research. We show that PFA are identifiable in the limit with probability one. Multiplicity automata (MA) is another device to represent stochastic languages. We show that a MA may generate a stochastic language that cannot be generated by a PFA, but we show also that it is undecidable whether a MA generates a stochastic language. Finally, we propose a learning algorithm for a subclass of PFA, called PRFA.
some index n and PAn converges to PA ; moreover, when parameters of the target are rational numbers, it can be requested that An itself is stationary from some index. The set of probabilistic automata whose structure is deterministic (PDFA) is identifiable in the limit with probability one [8,9,10], the identification being exact when the parameters of the target are rational numbers. However, PDFA are far less expressive than PFA, i.e. the set of probability distributions associated with PDFA is stricly included in the set of distributions generated from general PFA. We show that PFA are identifiable in the limit, with exact identification when the parameters of the target are rational numbers (Section 3). Multiplicity automata (MA) are devices which model functions from Σ ∗ to IR. It has been shown that functions that can be computed by MA are very efficiently learnable in a variant of the exact learning model of Angluin, where the learner can ask equivalence and extended membership queries [11,12,13]. As PFA are particular MA, they are learnable in this model. However, the learning is improper in the sense that the output function is not a PFA but a multiplicity automaton. We show that a MA is maybe not a very convenient representation scheme to represent a PFA if the goal is to learn it from stochastic data. This representation is not robust, i.e. there are MA which do not compute a stochastic language and which are arbitrarily close to a given PFA. Moreover, we show that it is undecidable whether a MA generates a stochastic language. That is, given a MA computed from stochastic data: it is possible that it does not compute a stochastic language and there may be no way to detect it! We also show that MA can compute stochastic languages that cannot be computable by PFA. These two results are proved in Section 4: they solve problems that were left open in [1]. Our identification in the limit algorithm of PFA is far from being efficient while algorithms that identifies PDFA in the limit can also be used in practical learning situations (ALERGIA [8], RLIPS [9], MDI [14]). Note also that we do not have a model that describes algorithms “that can be used in practical cases”: identification in the limit model is clearly too weak, exact learning via queries is irrealistic, PAC-model is maybe too strong (PDFA are not PAC-learnable [15]). So, it is important to define subclasses of PFA, as rich as possible, while keeping good empirical learnability properties. We have introduced in [16,17] a new class of PFA based on the notion of residual languages : a residual language of a stochastic language P is the language u−1 P defined by u−1 P (v ) = P (uv )/P (uΣ ∗ ). It can be shown that a stochastic language can be generated by a PDFA iff it has a finite number of residual languages. We consider the class of Probabilistic Residual Finite Automata (PRFA): a PFA A is a PRFA iff each of its states generates a residual language of PA . It can be shown that a stochastic language can be generated by a PRFA iff PA has a finite number 1 −1 of prime residual languages u− 1 P, . . . , un P sufficient to express all the resid1 −1 ual languages as a convex linear combination of u− 1 P, . . . , un P , i.e. for every 1 word v , there exist non negative real numbers αi such that v −1 P = αi u− i P ([17,16]). Clearly, the class of PRFA is much more expressive than PDFA. We introduce a first learning algorithm for PRFA, which identifies this class in the limit with probability one, and can be used in practical cases (Section 5).