VAR中变量非平稳时,如何进行脉冲分析
2.3VAR--脉冲-方差分解-协整剖析.
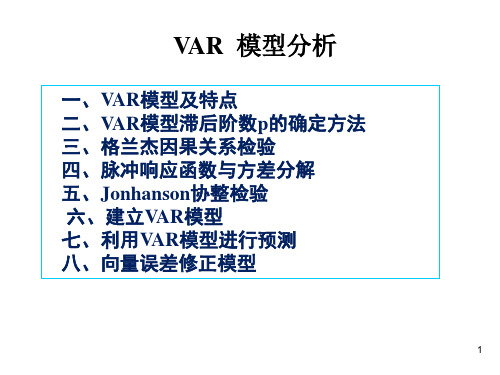
由此可知,经济理论指导下建立的结构性经典计量模
型存在不少问题。为解决这些问题而提出了一种用非结构 性方法建立各变量之间关系的模型。本章所要介绍的VAR模 型和VEC模型,就是非结构性的方程组模型。
VAR (Vector Autoregression)模型由西姆斯
(C.A.Sims,1980)提出,他推动了对经济系统动态分析的
yt xt
111 121
112 122
yt1 xt1
ቤተ መጻሕፍቲ ባይዱ
211 221
212 222
yt2 xt2
u1t u2 t
待估参数个数为2 × 2×2= PN2
用线性方程组表示VAR(2)模型:
yt 111 yt1 112 xt1 211yt2 212xt2 u1t
pYt p Ut
(1)
4
式中,i (i 1,2, ,p) 是第i个待估参数N×N阶矩阵; Ut (u1t u2t uNt )T 是N×1阶随机误差列向量;
是N×N阶方差协方差矩阵;
p 为模型最大滞后阶数。
由式(11.1)知,VAR(p)模型,是以N个第t期变量
y1t y2t
yNt 为应变量,以N个应变量y1t y2t
yNt
的最大p阶滞后变量为解释变量的方程组模型,方程组模 型中共有N个方程。显然,VAR模型是由单变量AR模型推广到 多变量组成的“向量”自回归模型。
对于两个变量(N=2),Yt ( yt xt )T 时,VAR(2)模型为
2
Yt iYti Ut 1Yt1 2Yt2 Ut i 1
5
用矩阵表示:
广泛应用,是当今世界上的主流模型之一。受到普遍重视,
得到广泛应用。
VAR模型主要用于预测和分析随机扰动对系统的动态冲
VAR模型的原理及应用
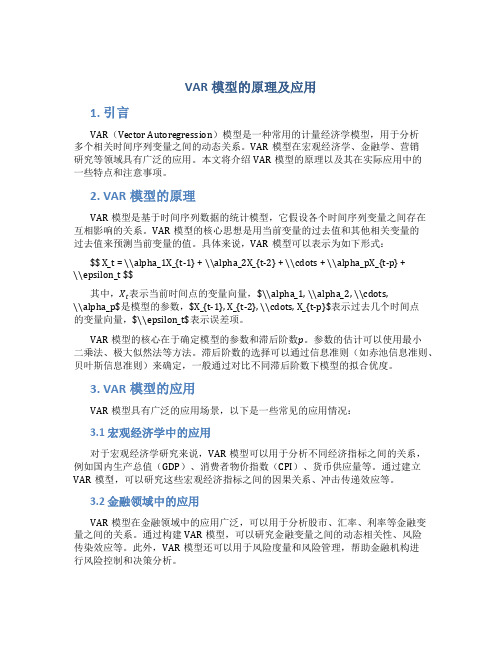
VAR模型的原理及应用1. 引言VAR(Vector Autoregression)模型是一种常用的计量经济学模型,用于分析多个相关时间序列变量之间的动态关系。
VAR模型在宏观经济学、金融学、营销研究等领域具有广泛的应用。
本文将介绍VAR模型的原理以及其在实际应用中的一些特点和注意事项。
2. VAR模型的原理VAR模型是基于时间序列数据的统计模型,它假设各个时间序列变量之间存在互相影响的关系。
VAR模型的核心思想是用当前变量的过去值和其他相关变量的过去值来预测当前变量的值。
具体来说,VAR模型可以表示为如下形式:$$ X_t = \\alpha_1X_{t-1} + \\alpha_2X_{t-2} + \\cdots + \\alpha_pX_{t-p} +\\epsilon_t $$其中,X t表示当前时间点的变量向量,$\\alpha_1, \\alpha_2, \\cdots,\\alpha_p$是模型的参数,$X_{t-1}, X_{t-2}, \\cdots, X_{t-p}$表示过去几个时间点的变量向量,$\\epsilon_t$表示误差项。
VAR模型的核心在于确定模型的参数和滞后阶数p。
参数的估计可以使用最小二乘法、极大似然法等方法。
滞后阶数的选择可以通过信息准则(如赤池信息准则、贝叶斯信息准则)来确定,一般通过对比不同滞后阶数下模型的拟合优度。
3. VAR模型的应用VAR模型具有广泛的应用场景,以下是一些常见的应用情况:3.1 宏观经济学中的应用对于宏观经济学研究来说,VAR模型可以用于分析不同经济指标之间的关系,例如国内生产总值(GDP)、消费者物价指数(CPI)、货币供应量等。
通过建立VAR模型,可以研究这些宏观经济指标之间的因果关系、冲击传递效应等。
3.2 金融领域中的应用VAR模型在金融领域中的应用广泛,可以用于分析股市、汇率、利率等金融变量之间的关系。
通过构建VAR模型,可以研究金融变量之间的动态相关性、风险传染效应等。
VAR模型
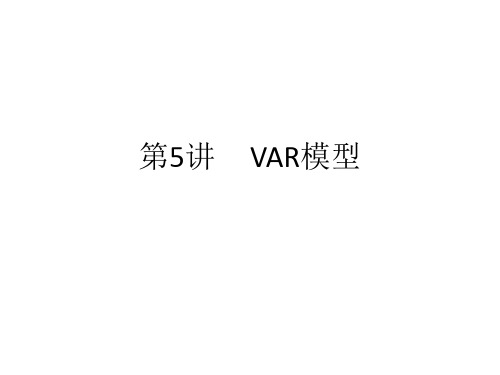
• 选择信息准则统计值最小时的滞后期数。
• 似然比检验法 简单地说,LR检验法就是比较不同滞 后期数对应的似然函数值。 具体地说,考虑VAR 与VAR ,并 且p2 p1。这样,分别估计对应的两个VAR ˆ ˆ 系统,获得相应的 1 和 2。LR检验统计量 定义为: ˆ ln ˆ ) (T )( ln 1 2
可以看出,模型(8.46)对应的正是利用 OLS方法, 对Y jt进行回归得到的系数估计 值。
• 由于在VAR模型的随机扰动项服从独立同分 布时MLE和OLS估计出来的参数具有一致性, VAR模型采用OLS进行估计。 • STATA应用实例:美国通货膨胀率与短期利 率的VAR分析
三、格兰杰因果关系检验
• 指导性的原则: • 如果要分析不同变量之间可能存在的长期 均衡关系,则可以直接选用非平稳序列; • 如果分析的是短期的互动关系,则选用平 稳序列,对于涉及到的非平稳序列,必须 先进行差分或去除趋势使其转化成对应的 平稳序列,然后包含在VAR模型中进行进一 步分析。
• 3. 滞后阶数的选择 • 信息准则法(AIC或者SIC)
LR 检验:
如果拒绝原假设,则称 y2t 是y1t的格兰杰 因果关系。 与此不同, y1t C1 1 y1,t 1 2 y1,t 2 L p y1,t p 1 y2,t 1 2 y2,t 2 L p y2,t - p 1t H 0 : 1 2 L p 0
• STATA应用实例:继续 使用美国通货膨胀与 利率数据
• STATA应用实例:上证 综合指数收益率与标 准普尔500股指收益率
Eviews中VAR模型的操作、脉冲响应分析和方差分解的实现
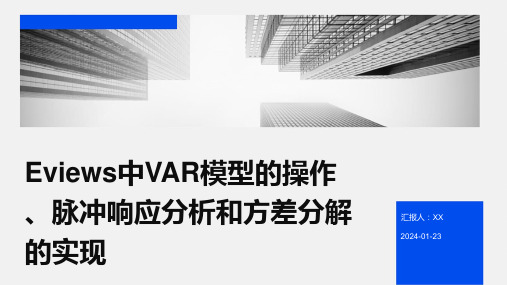
数据准备
在Eviews中导入需要分析的时间 序列数据,并进行必要的预处理 ,如缺失值处理、平稳性检验等 。
模型设定
根据研究目的和数据特征,选择 合适的VAR模型阶数(滞后阶数 ),并设定模型的约束条件(如 外生变量、季节性等)。
参数估计
运用最小二乘法(OLS)或极大 似然法(ML)等估计方法对VAR 模型进行参数估计,得到模型的 系数矩阵和截距项。
3. 在弹出的对话框中,设置 冲击的滞后期数(即观察冲 击影响的期数),并选择要 分析的变量。
4. 点击“OK”按钮, Eviews将生成脉冲响应结果 。
脉冲响应结果的解读
脉冲响应图
通常以图形形式展示脉冲响应结果,横轴表示滞后期数,纵轴表示内生变量对冲击的响应程度。通过脉冲响应图可以 直观地观察变量之间的动态影响关系。
Eviews中VAR模型的实现步骤
模型诊断
对估计得到的VAR模型进行诊断检验,包括残差自相关检验、异方差性检验等,以确保模 型的合理性。
脉冲响应分析
在Eviews中利用脉冲响应函数(Impulse Response Function,IRF)分析VAR模型中各 变量对冲击的反应程度和持续时间。通过设置冲击大小和滞后期数,可以得到不同变量之 间的动态影响关系图。
在Eviews中选择 "Quick"->"Estimate Equation",在弹出的 对话框中选择VAR模型 ,并设定滞后阶数。
变量选择
根据研究目的选择合适 的变量,并将其添加到 模型中。
模型估计
点击"OK"按钮,Eviews 将自动进行VAR模型的 估计,并显示估计结果 。
脉冲响应分析与方差分解
VAR模型、Johansen协整检验在eviews中的具体操作步骤及结果解释

19
利用Genr命令可算得用于检验原假设是否 成立的伴随概率 P:
p=1-@cchisq(42.4250,18) =0.000964
故 P=0.000964< =0.05,应拒绝原假设
,建立VAR(3)模型。
20
三、约翰森(Jonhamson)协整检验
Jonhamson(1995)协整检验是基于VAR模 型的一种检验方法,但也可直接用于多变量间的协 整检验。
1.Johanson协整似然比(LR)检验 H0:有 0个协整关系; H1:有M个协整关系。 检验迹统计量:
N
LRM n
log(1 i )
i M 1
图11-1和图11-2,由图11-2可以看出,三个对数序列的
变化趋势基本一致,可能存在协整关系。
13
160000
120000
80000
40000
0 55 60 65 70 75 80 85 90 95 00
GDP
CT
IT
图11-1 GDPt、 Ct和 It
的时序图
12
11
10
9
8
7
6
5 55 60 65 70 75 80 85 90 95 00
LR 2(Lnl(1) Lnl(3)) 2(108.7551 129.9676) 42.4250
其中,Lnl(1)和Lnl(3)分别为P=1和P=3时VAR(P) 模型的对数似然函数值。在零假设下,该统计量 服从渐进的 2 ( f ) 分布,其自由度f为从VAR(3) 到VAR(1)对模型参数施加的零约束个数。对本 例:
这种方程组模型主要用于分析联合内生变量 间的动态关系。联合是指研究N个变量 y1t y2t yNt 间的相互影响关系,动态是指p期滞后。故称VAR 模型是分析联合内生变量间的动态关系的动态模 型,而不带有任何约束条件,故又称为无约束 VAR模型。建VAR模型的目的:
VAR--脉冲-方差分解-协整

VAR(p+i)模型的对数似然函数值;f为自由度。 用对数似然比统计量LR确定P的方法用案例说
明。
12
格兰杰因果关系
1.格兰杰因果性定义
克莱夫.格兰杰(Clive.Granger,1969)和西姆 斯(C.A.Sims,1972)分别提出了含义相同的定义, 故除使用“格兰杰非因果性”的概念外,也使用“ 格兰杰因果性”的概念。其定义为:
,即分别建立VAR(1)、VAR(2)、VAR(3)、VAR(4)模型 ,比较AIC、SC,使它们同时取最小值的p值即为所求 。而对月度数据,一般比较到P=12。
当AIC与SC的最小值对应不同的p值时,只能用LR 检验法。
11
(2)用似然比统计量LR选择p值。LR定义为 :
LR 2ln l(p) ln l( p i) 2( f ) (11.2)
归式,因此,要求受检变量是平稳的,对非平稳变量 要求是协整的,以避免伪回归。故在进行格兰杰因果 关系检验之前,要进行单位根检验、对非平稳变量要 进行协整检验。
16
(2)格兰杰因果性,指的是双向因果关系, 即相关关系。单向因果关系是指因果关系,近年 有学者认为单向因果关系的变量也可作为内生变 量加入VAR模型;
注意: “VAR”需大写,以区别金融风险管理 中的VaR。
9
二、VAR模型中滞后阶数p的确 定方法
建立VAR模型只需做两件事 第一,哪些变量可作为应变量?VAR模型中应 纳入具有相关关系的变量作为应变量,而变量间 是否具有相关关系,要用格兰杰因果关系检验确 定。 第二,确定模型的最大滞后阶数p。首先介绍 确定VAR模型最大滞后阶数p的方法:在VAR模型 中解释变量的最大滞后阶数p太小,残差可能存在 自相关,并导致参数估计的非一致性。适当加大p 值(即增加滞后变量个数),可消除残差中存在
var模型原理与步骤
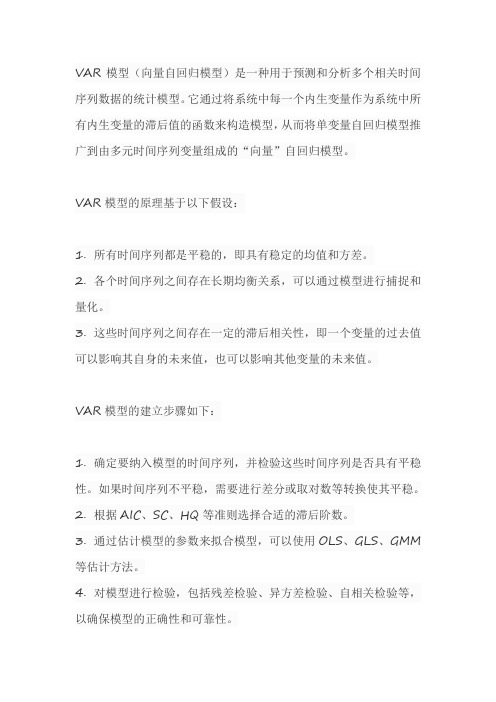
VAR模型(向量自回归模型)是一种用于预测和分析多个相关时间序列数据的统计模型。
它通过将系统中每一个内生变量作为系统中所有内生变量的滞后值的函数来构造模型,从而将单变量自回归模型推广到由多元时间序列变量组成的“向量”自回归模型。
VAR模型的原理基于以下假设:
1. 所有时间序列都是平稳的,即具有稳定的均值和方差。
2. 各个时间序列之间存在长期均衡关系,可以通过模型进行捕捉和量化。
3. 这些时间序列之间存在一定的滞后相关性,即一个变量的过去值可以影响其自身的未来值,也可以影响其他变量的未来值。
VAR模型的建立步骤如下:
1. 确定要纳入模型的时间序列,并检验这些时间序列是否具有平稳性。
如果时间序列不平稳,需要进行差分或取对数等转换使其平稳。
2. 根据AIC、SC、HQ等准则选择合适的滞后阶数。
3. 通过估计模型的参数来拟合模型,可以使用OLS、GLS、GMM 等估计方法。
4. 对模型进行检验,包括残差检验、异方差检验、自相关检验等,以确保模型的正确性和可靠性。
5. 利用拟合好的模型进行预测和分析。
例如,可以使用模型来预测多个时间序列的未来值,或者分析一个时间序列与其他时间序列之间的动态关系。
需要注意的是,VAR模型只适用于分析平稳时间序列数据,对于非平稳时间序列数据,需要进行差分、对数转换等处理使其平稳后再进行分析。
同时,VAR模型的假设和参数选择需要根据具体数据进行判断和选择,不同的模型适用于不同类型的数据和问题。
第七章非平稳时间序列分析解读
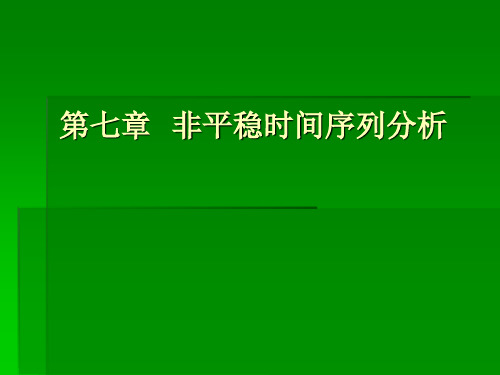
ˆ 1 t ˆ ˆ
W 1 1 W 1 W r dr ˆ 1 N L 0 1 2 2 12 2 1 1 ˆ (N 2 2 ˆ) W r dr [ W r dr]
二、单位根过程检验统计量分布基础
如前所述,对单位根过程这种非平稳序列 的分析,传统分析方法失效,需寻找新的 处理方法和技巧。这些新的分析方法都是 建立在维纳过程(布朗运动)和泛函中心 极限定理之上。
(一)维纳过程
设 W (t ) 是定义在闭区间[0, 1]上一连续变化的随机过程, 若 该过程满足: (a) W(0)=0; (b) 独立增量过程:对闭区间 [0 , 1] 上任意一组分割 0 t1 t 2 t k 1 , W (t ) 的 变 化 量 :
模拟一、 模拟二
二、非平稳序列的分类
(一) 随机趋势非平稳过程(stochastic trend process) 随机趋势非平稳过程又称为差分平稳过程 (difference stationary process)、有漂 移项的非平稳过程(non-stationary process with drift)。
H 0 : 1;
情形四:假设数据由(真实过程) (7.30)产生,在回归模型 yt yt 1 t t 中检 验假设:
H 0 : 1; 0
t统计量的极限分布依赖于回归模型形式的选
择(即是否包含常数项和趋势项)
(一) 情形一的DF检验法
y ˆ y
第七章 非平稳时间序列分析
脉冲响应函数

脉冲响应函数注意VAR模型过程中的格兰杰检验与变量间的格兰杰检验不是一回事啊!变量间的格兰杰因果是前提是同阶单整Var模型后的格兰杰前提是非同阶单整后差分平稳做VAR模型是非结构化的,且模型形式已被确定为线性形式,需要确定哪些变量间有相互作用及反应变量彼此之间相互影响的最大可能滞后阶数。
因为经济问题中长出现伪回归问题,即经济意义表明几乎没有联系的序列可能出项较大的相关系数。
因此格兰杰检验是做VAR模型必须的。
var的前提是系统稳定(并不一定是各个变量都是稳定的)例如对于3变量的var若有2个水平不平稳有1个水平平稳但是他们3个都是一阶平稳则需要做协整判断用水平的还是用一阶差分的变量进行var若水平的存在协整关系且做单位圆检验系统稳定则可以直接用水平变量做var但是若不存在协整或则系统不稳定则就得用一阶差分变量来做若3个变量都是水平的则直接var就好了用s-plus进行多元VAR-GARCH估计时,是用的MGARCH命令,比如var.bekk=mgarch(It.St.getreturns[,c("interestrate","stockindex")]~ar( 2),~bekk(1,1),armaType="full")。
这时var.bekk的类型是mgarch,即class(var.bekk)="mgarch"。
能不能将模型估计的var部分提取出来,形成一个var对象?这样就可以进行脉冲响应分析了。
请高人指点啊。
建议看一下Nakatani,T.and T.Terasvirta(2009)."Testing for volatilityinteractions in the Constant Conditional Correlation GARCH model."Econometrics Journal 12(1):147-163.Impulse Response Function for Conditional Volatility in GARCH Models Wen-Ling Lin Journal of Business&Economic Statistics,Vol.15,No.1(Jan.,1997),pp.15-25 VAR模型中方程的特征根的倒数要在单位圆内,否则VAR模型不稳定,不能做脉冲响应脉冲响应分析很多时候是根据既定的条件进行的,比如经济意义。
VAR与脉冲响应函数
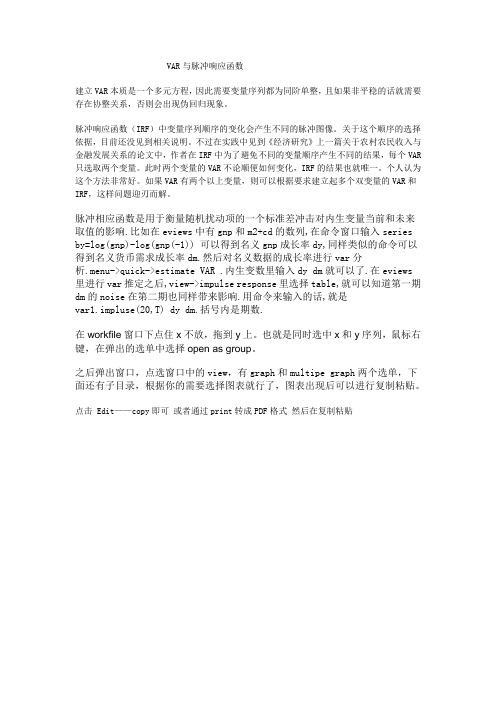
VAR与脉冲响应函数建立VAR本质是一个多元方程,因此需要变量序列都为同阶单整,且如果非平稳的话就需要存在协整关系,否则会出现伪回归现象。
脉冲响应函数(IRF)中变量序列顺序的变化会产生不同的脉冲图像。
关于这个顺序的选择依据,目前还没见到相关说明。
不过在实践中见到《经济研究》上一篇关于农村农民收入与金融发展关系的论文中,作者在IRF中为了避免不同的变量顺序产生不同的结果,每个VAR 只选取两个变量。
此时两个变量的VAR不论顺便如何变化,IRF的结果也就唯一。
个人认为这个方法非常好。
如果VAR有两个以上变量,则可以根据要求建立起多个双变量的VAR和IRF,这样问题迎刃而解。
脉冲相应函数是用于衡量随机扰动项的一个标准差冲击对内生变量当前和未来取值的影响.比如在eviews中有gnp和m2+cd的数列,在命令窗口输入series by=log(gnp)-log(gnp(-1)) 可以得到名义gnp成长率dy,同样类似的命令可以得到名义货币需求成长率dm.然后对名义数据的成长率进行var分析.menu->quick->estimate VAR .内生变数里输入dy dm就可以了.在eviews里进行var推定之后,view->impulse response里选择table,就可以知道第一期dm的noise在第二期也同样带来影响.用命令来输入的话,就是var1.impluse(20,T) dy dm.括号内是期数.在workfile窗口下点住x不放,拖到y上。
也就是同时选中x和y序列,鼠标右键,在弹出的选单中选择open as group。
之后弹出窗口,点选窗口中的view,有graph和multipe graph两个选单,下面还有子目录,根据你的需要选择图表就行了,图表出现后可以进行复制粘贴。
点击 Edit——copy即可或者通过print转成PDF格式然后在复制粘贴。
平稳性和非平稳时间序列分析
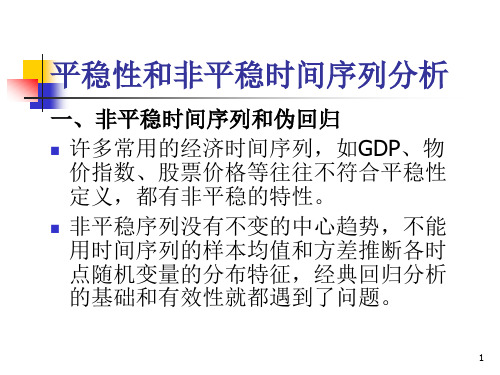
“单积”(Integrated)的,并记I为(d) 。
14
四、时间序列的协积性 (一)定义 如果一组时间序列都 X1, , X n 是同阶单积
的(I (d) ),并且存在向量 (1, , n )
使加权组合1X1 n X n 为平稳序列 (I (0)),则称这组时间序列为“协积的”
(Cointegrated),其中 (1, , n ) 称为
“协积向量”。
15
具有协积性的非平稳序列各自的非平稳 趋势和波动有相互抵消的作用,因此虽 然非平稳本身有导致回归分析失效的影 响,但如果模型中的几个非平稳时间序 列具有协积性,回归分析仍然可以是有 效的,不需要担心非平稳性会造成问题。
16
11
不少非平稳时间序列作差分变换得到的差分序 列都是平稳序列。对于这种非平稳时间序列的 差分序列,基于平稳数据的计量分析就是有效 的。
由于时间序列的差分序列与时间序列本身包含 许多一致的信息,差分与原变量之间常常可以 相互转换,因此利用差分数据进行计量分析也 是有意义的。
并不是所有非平稳时间序列的差分序列都是平 稳的。利用差分数据进行分析之前,必须对差 分序列进行平稳性检验。检验的方法是把单位 根检验用于时间序列的差分序列。
Yt Yt1 t ,其中 t 为白噪声过程。
(2)检验思路 首先 Yt 服从如下的自回归模型
Yt Yt 1 t
6
如果其中 1 ,或者变换成如下的回归 模型 Yt Yt 1 t
中的 0 ,那么时间序列{Yt }就是最基
本的单位根过程 Yt Yt1 t ,肯定是非平 稳的。 对上述差分模型中的显著性检验,就是 检验时间序列是否存在上述单位根问题。
var不正交的脉冲响应

var不正交的脉冲响应
不正交的脉冲响应通常指的是在信号处理中,两个不同的脉冲响应函数之间存在相互干扰或重叠的情况。
这可能导致信号的混叠或者损失精度。
在正交系统中,不同的脉冲响应函数是相互独立的,它们之间没有相互干扰的情况。
但是在不正交系统中,不同的脉冲响应函数之间可能存在相互干扰,这可能会导致信号的交叉干扰或者误差累积。
对于不正交的脉冲响应,我们可以采取一些方法来解决问题。
其中一种方法是通过改变脉冲响应函数的设计,使其与其他脉冲响应函数相互正交,从而减少干扰。
另一种方法是在信号处理过程中使用滤波器或者相关技术,来分离或者抵消不正交的脉冲响应。
总之,不正交的脉冲响应会引起信号处理中的干扰和误差。
通过合适的设计和信号处理技术,我们可以减少不正交脉冲响应带来的问题,提高信号处理的精度和可靠性。
中国经济持续性增长的理论与实证研究——基于VAR模型及脉冲响应函数的分析
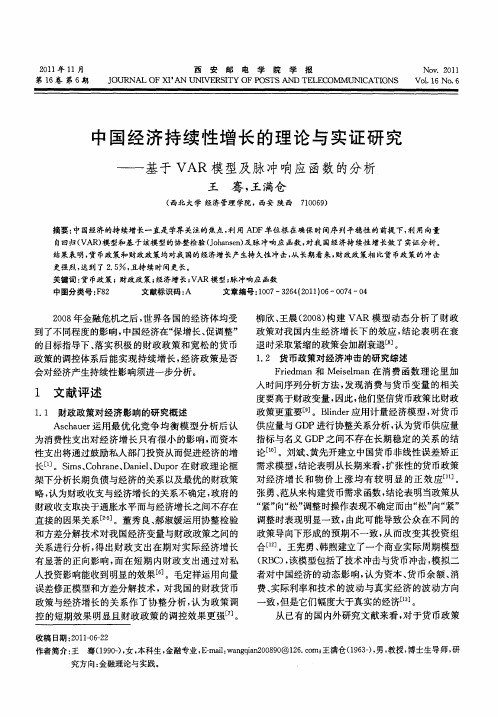
1 文 献 评 述
和方差分解技术对我国经济变量与财政政策之间的 关系进行分析, 出财政支 出在期对实际经济增长 得 有显著 的正向影响 , 而在短期 内财政支出通过对私 人投资影响能收到明显的效果[。毛定祥运用 向量 6 ] 误差修正模型和方差分解技术 , 对我 国的财政货币 政策与经济增长的关系作 了协整分析, 为政策调 认 控的短期效果 明显且财政政策的调控效果更强_ 。 _ 7 ]
20 08年金 融危 机之 后 , 界 各 国 的经 济体 均 受 世 到 了不 同程 度 的影 响 , 中国经济 在“ 增长 、 保 促调整 ”
的目标指导下、 落实积极的财政政策和宽松 的货币
政策 的调控 体 系后 能 实现 持 续 增 长 , 济政 策 是 否 经 会对 经济产 生持 续性 影 响须进 一步分 析 。
更强烈, 达到 了 2 5 , . 且持续 时间更长。 关键词 : 币政策 ;财政政 策 ; 货 经济增长 ; R模型 ; 冲响应 函数 VA 脉
中图分 类号 :8 F2
文献标识码 : A
文章编号 :0 7— 24 2 1 )6 0 7 —0 1 0 3 6 (0 10 — 0 4 4
柳欣 、 王晨(0 8 构建 VA 20 ) R模型动态分 析了财政 政 策对 我 国内生经 济 增 长下 的效应 , 论 表 明在 衰 结 退 时采 取 紧缩 的政 策会 加剧衰 退[ 。 8 ] 1 2 货币政 策对经 济 冲击的研 究综述 . F id n和 Mesl n在 消 费 函数 理论 里 加 r ma e i ma e
政策与货币政策对中国经济增长的持续性影响。
自于 R S E E S T金融研究数据库。所做实证检验使 用的软件是 E i s. 。 v w 31 e 3 2 实证检 验 . V R模型在本文表现为 : A Y 一 +8 1 Y一+ X 一 +J +. 3 H u t 其中 ~( , ) OQ G 一 +p 1 G一 +
非平稳时间序列的随机分析
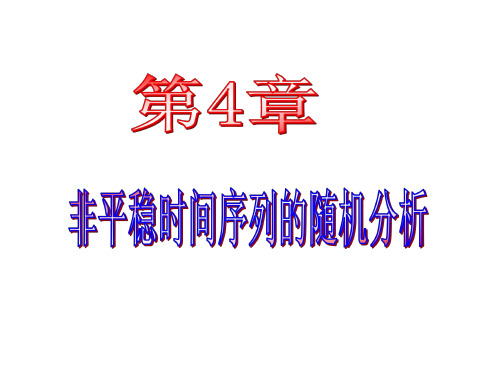
第二节 差分运算
对于随机非平稳序列来说,我们难以直接找 到其变化发展规律,主要是因为非平稳序列通常 都具有某种不稳定的趋势。所以,分析非平稳序 列的第一步是采取有效的手段提取其趋势使序列 变为平稳序列,然后利用平稳序列分析方法来处 理。提取序列趋势的工具主要是差分运算。
kt
t
例如,若
xt a bt t
则对序列 xt 做一阶差分
xt b t
就提取了序列中的确定性趋势信息。
若 xt a bt ct2 t ,则对 xt 做二阶差分
2 x 2c 2
t
t
即可提取序列中的确定性趋势信息。
yt 01yt q 2yt q1 vt
式中,vt 为残差序列。如果我们基于历史信息: ytq , ytq1, 预测 yt 的值,则 vt 可以理解为预测
误差,记 Var(v ) 2(q) ,显然有 2(q) Var( y ) ,
t
v
v
t
且滞后期 q 越大,意味着预测的步长越长,预测
的误差就越大,即2v(q) 越大。
实际上,时间序列中的差分运算类似于函数的 求导运算,如果一个时间序列的确定性趋势是时间 的 d 次多项式,则 d 阶差分后的序列的确定性趋势 就一定是常数,将不会再蕴含时间趋势,从而实现 序列的平稳化。
d
d
tj
j k,
( k 为常数)
j0
而由Cramer分解定理知,方差齐性非平稳序 列都可以分解为如下形式:
y
)t
,说明序列发展的
随机性强,历史信息对现值估计效果差,这时称
序列 yt是随机序列。
例如,对于平稳的ARMA(p,q) 模型:
VAR模型的适用范围:用于时间序列的情况
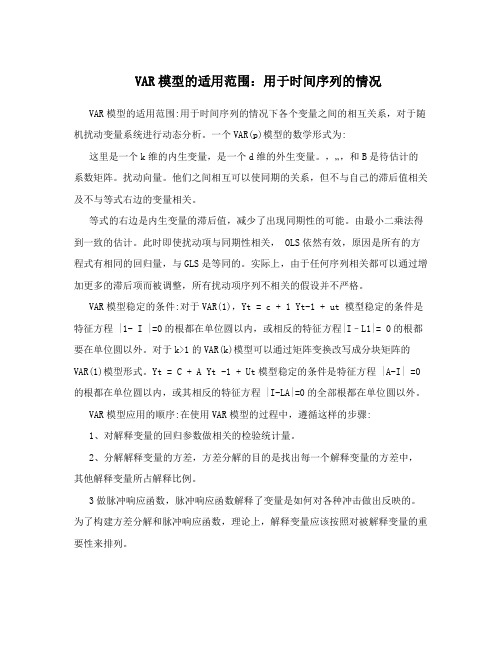
VAR模型的适用范围:用于时间序列的情况VAR模型的适用范围:用于时间序列的情况下各个变量之间的相互关系,对于随机扰动变量系统进行动态分析。
一个VAR(p)模型的数学形式为: 这里是一个k维的内生变量,是一个d维的外生变量。
,…,和B是待估计的系数矩阵。
扰动向量。
他们之间相互可以使同期的关系,但不与自己的滞后值相关及不与等式右边的变量相关。
等式的右边是内生变量的滞后值,减少了出现同期性的可能。
由最小二乘法得到一致的估计。
此时即使扰动项与同期性相关, OLS依然有效,原因是所有的方程式有相同的回归量,与GLS是等同的。
实际上,由于任何序列相关都可以通过增加更多的滞后项而被调整,所有扰动项序列不相关的假设并不严格。
VAR模型稳定的条件:对于VAR(1),Yt = c + 1 Yt-1 + ut 模型稳定的条件是特征方程 |1- I |=0的根都在单位圆以内,或相反的特征方程|I–L1|= 0的根都要在单位圆以外。
对于k>1的VAR(k)模型可以通过矩阵变换改写成分块矩阵的VAR(1)模型形式。
Yt = C + A Yt -1 + Ut模型稳定的条件是特征方程 |A-I| =0的根都在单位圆以内,或其相反的特征方程 |I-LA|=0的全部根都在单位圆以外。
VAR模型应用的顺序:在使用VAR模型的过程中,遵循这样的步骤:1、对解释变量的回归参数做相关的检验统计量。
2、分解解释变量的方差,方差分解的目的是找出每一个解释变量的方差中,其他解释变量所占解释比例。
3做脉冲响应函数,脉冲响应函数解释了变量是如何对各种冲击做出反映的。
为了构建方差分解和脉冲响应函数,理论上,解释变量应该按照对被解释变量的重要性来排列。
文中采用了双变量滞后k期的VAR模型,来研究FDI和经济增长各个效应之间的动态关系,形式如下:方程变量的解释:是2×1阶列向量;表示d×1阶确定项向量(d表示确定性变量个数);用来描述常数项Ц;时间趋势项t;季节虚拟变量(如果需要和其他一些有必要设置的虚拟变量;, …均为2×2阶参数矩阵;Ф是确定性变量;的2×d阶系数矩阵;[ ]是2×1阶随机误差列向量;在模型中,每一个元素都是非自相关的,但是不同的方程对应的随机变量之间可能存在相关性。
非平稳时间序列分析
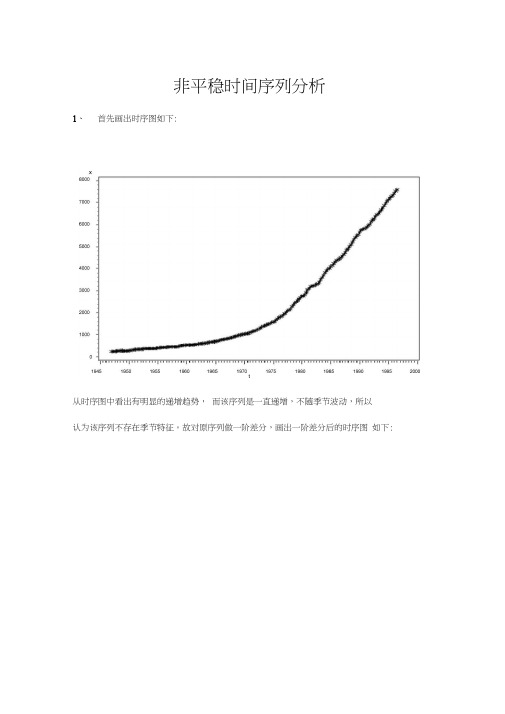
非平稳时间序列分析1、首先画出时序图如下:t从时序图中看出有明显的递增趋势,而该序列是一直递增,不随季节波动,所以认为该序列不存在季节特征。
故对原序列做一阶差分,画出一阶差分后的时序图如下:difx140 130 120 110 100 90 80 70 60 50 40 30 20 10 0 -10从中可以看到一阶差分后序列仍然带有明显的增长趋势,再做二阶差分:dif2x90 80 70 60 50 40 30 20 10 0 -10 -20 -30 -40 -50 -60 -70 -80 -90 -100 -110做完二阶差分可以看到,数据的趋势已经消除,接下来对二阶差分后的序列进行194519501945 19551960196519701975198019851990199520001950 1955 1960 1965 1970 1975 1980 1985 1990 1995 2000检验:AutocorrelationsLag Covariance Correlation -1 9 8 7 6 5 4 3 2 1 0 1 2 3 4 5 6 7 8 9 1 Std Error0 577.333 1.00000 | |********************| 01 -209.345 -.36261 | *******| . | 0.0712472 -52.915660 -.09166 | .**| . | 0.0800693 9.139195 0.01583 | . | . | 0.0806004 15.375892 0.02663 . |* . | 0.0806155 -59.441547 -.10296 .**| . | 0.0806606 -23.834489 -.04128 | . *| . | 0.0813247 100.285 0.17370 | . |*** | 0.0814318 -146.329 -.25346 | *****| . | 0.0832909 52.228658 0.09047 | . |**. | 0.08711810 21.008575 0.03639 | . |* . | 0.08759311 134.018 0.23213 | . |***** | 0.08767012 -181.531 -.31443 | ******| . | 0.09073613 23.268470 0.04030 | . |* . | 0.09610814 71.112195 0.12317 | . |** . | 0.09619415 -105.621 -.18295 | ****| . | 0.09699116 37.591996 0.06511 . |* . | 0.09872717 23.031506 0.03989 | . |* . | 0.09894518 45.654745 0.07908 | . |** . | 0.09902719 -101.320 -.17550 | ****| . | 0.09934720 127.607 0.22103 | . |**** | 0.10090821 -61.519663 -.10656 | . **| . | 0.10333722 35.825317 0.06205 | . |* . | 0.10389323 -93.627333 -.16217 | .***| . | 0.10408124 55.451208 0.09605 | . |** . |从其自相关图中可以看出二阶差分后的序列自相关系数很快衰减为零,且都在两倍标准差范围之内,所以认为平稳,白噪声检验结果:Autocorrelation Check for White NoiseTo Chi- Pr >Lag Square DF ChiSq------------------- Autocorrelations -------------------6 30.70 6 <.0001 -0.363 -0.092 0.016 0.027 -0.103 -0.04112 84.54 12 <.0001 0.174 -0.253 0.090 0.036 0.232 -0.31418 97.98 18 <.0001 0.040 0.123 -0.183 0.065 0.040 0.07924 126.99 24 <.0001 -0.175 0.221 -0.107 0.062 -0.162 0.096P 值都小于 0.05 ,认为不是白噪声。
Eviews中VAR模型的操作、脉冲响应分析和方差分解的实现
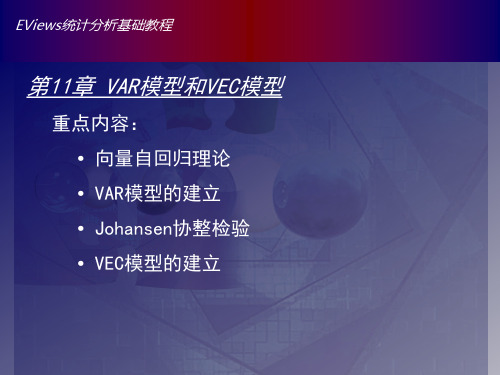
EViews统计分析基础教程
四、Johansen协整检验
1、Johansen协整理论
其中,Δyt和Δyt-j(j=1,2,…,p)都是由I(0)变量构成 的向量,如果 yt-1是I(0)的向量,即y1t-1,y2t-1,…, ykt-1之间具有协整关系,则Δyt是平稳的。
EViews统计分析基础教程
EViews统计分析基础教程
四、Johansen协整检验
1、Johansen协整理论
在VAR(p)模型中,设变量y1t, y2t,…,ykt均是非平 稳的一阶单整序列,即yt~I(1)。xt是d维外生向量,代 表趋势项、常数项等, yt=A1 yt-1 +A2 yt-2 +…+ Ap yt-p+B xt + μt 变量y1t, y2t,…,ykt的一阶单整过程I(1)经过差分后 变为零阶单整过程I(0)
其中, r是特征根迹统计量。
EViews统计分析基础教程
四、Johansen协整检验
2、Johansen协整检验
(1)特征根迹(Trace)检验 当 0< 临界值时,接受H00,没有协整向量; 当 1> 临界值时,接受H10,至少有一个协整向量; 当 1< 临界值时,接受H10,只有一个协整向量; 当 1> 临界值时,拒绝H10,至少有两个协整向量; … 当 r< 临界值时,接受Hr0,只有r个协整向量。
EViews统计分析基础教程
四、Johansen协整检验
2、Johansen协整检验 (1)特征根迹(Trace)检验
(2)最大特征值检验
EViews统计分析基础教程
四、Johansen协整检验
2、Johansen协整检验
stata向量自回归 ,变量不稳定差分命令

在统计学中,Stata是一个功能强大的统计分析软件,其向量自回归(VAR)模型是一种经济计量学中常用的模型之一,用于分析多个变量之间的动态关系。
在Stata中,可以使用VAR模型进行时间序列数据的分析和预测,从而更好地理解不同变量之间的相互作用和影响。
变量的不稳定差分命令也是VAR模型中一个重要且值得关注的内容,它可以帮助我们更好地理解并处理变量不稳定的情况。
让我们来了解一下Stata中向量自回归模型的概念和基本原理。
向量自回归模型是一种多元时间序列模型,它可以用来描述多个变量之间的相互动态关系。
在Stata中,我们可以使用var命令来拟合向量自回归模型,并进行相关分析。
通过对向量自回归模型的估计和检验,我们可以得到不同变量之间动态相关性的信息,这对于宏观经济预测和政策制定具有重要意义。
在进行向量自回归模型分析时,一个重要的问题就是变量的不稳定性。
在实际数据中,很多情况下变量并不是平稳的,这就需要我们对变量进行差分以消除非平稳性。
在Stata中,我们可以使用差分命令来进行变量的差分处理,从而得到平稳的时间序列数据。
具体来说,可以使用差分命令(diff)来对变量进行一阶差分处理,从而消除非平稳性。
在实际应用中,我们可能会遇到一些变量不平稳的情况,特别是在经济领域的数据分析中。
这时,我们可以利用Stata中的差分命令来处理这些不稳定的变量。
差分处理可以将非平稳的时间序列数据转化为平稳的时间序列数据,从而更好地满足模型估计和检验的要求。
除了前面提到的内容,我个人认为在使用向量自回归模型进行分析时,除了要考虑变量之间的相关性和动态关系,还应该充分考虑到变量的不稳定性对模型估计和检验的影响。
合理地运用Stata中的变量不稳定差分命令是非常重要的,它可以帮助我们更好地理解和处理实际数据中的非平稳性问题,从而提高模型分析的准确性和有效性。
Stata中的向量自回归模型和变量不稳定差分命令在实际数据分析中具有重要的应用意义。
脉冲响应函数分析请高手解答
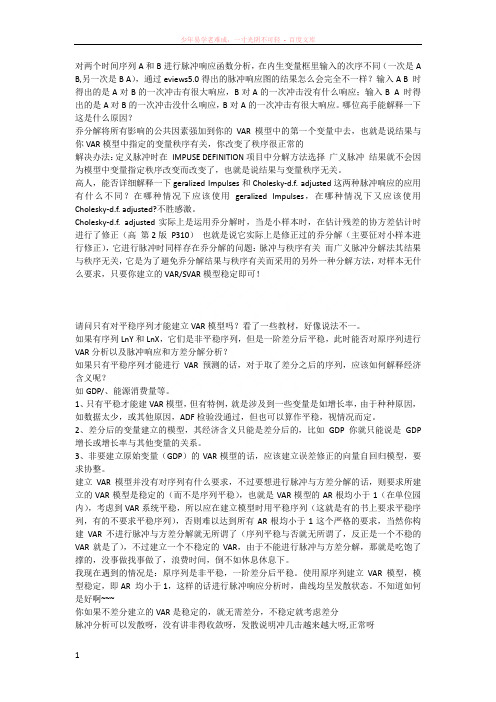
对两个时间序列A和B进行脉冲响应函数分析,在内生变量框里输入的次序不同(一次是A B,另一次是B A),通过eviews5.0得出的脉冲响应图的结果怎么会完全不一样?输入A B 时得出的是A对B的一次冲击有很大响应,B对A的一次冲击没有什么响应;输入B A 时得出的是A对B的一次冲击没什么响应,B对A的一次冲击有很大响应。
哪位高手能解释一下这是什么原因?乔分解将所有影响的公共因素强加到你的VAR模型中的第一个变量中去,也就是说结果与你VAR模型中指定的变量秩序有关,你改变了秩序很正常的解决办法:定义脉冲时在IMPUSE DEFINITION项目中分解方法选择广义脉冲结果就不会因为模型中变量指定秩序改变而改变了,也就是说结果与变量秩序无关。
高人,能否详细解释一下geralized Impulses和Cholesky-d.f. adjusted这两种脉冲响应的应用有什么不同?在哪种情况下应该使用geralized Impulses,在哪种情况下又应该使用Cholesky-d.f. adjusted?不胜感激。
Cholesky-d.f. adjusted实际上是运用乔分解时,当是小样本时,在估计残差的协方差估计时进行了修正(高第2版P310)也就是说它实际上是修正过的乔分解(主要征对小样本进行修正),它进行脉冲时同样存在乔分解的问题:脉冲与秩序有关而广义脉冲分解法其结果与秩序无关,它是为了避免乔分解结果与秩序有关而采用的另外一种分解方法,对样本无什么要求,只要你建立的VAR/SVAR模型稳定即可!请问只有对平稳序列才能建立VAR模型吗?看了一些教材,好像说法不一。
如果有序列LnY和LnX,它们是非平稳序列,但是一阶差分后平稳,此时能否对原序列进行VAR分析以及脉冲响应和方差分解分析?如果只有平稳序列才能进行VAR预测的话,对于取了差分之后的序列,应该如何解释经济含义呢?如GDP/、能源消费量等。
1、只有平稳才能建VAR模型,但有特例,就是涉及到一些变量是如增长率,由于种种原因,如数据太少,或其他原因,ADF检验没通过,但也可以算作平稳,视情况而定。
VAR的有一些问题
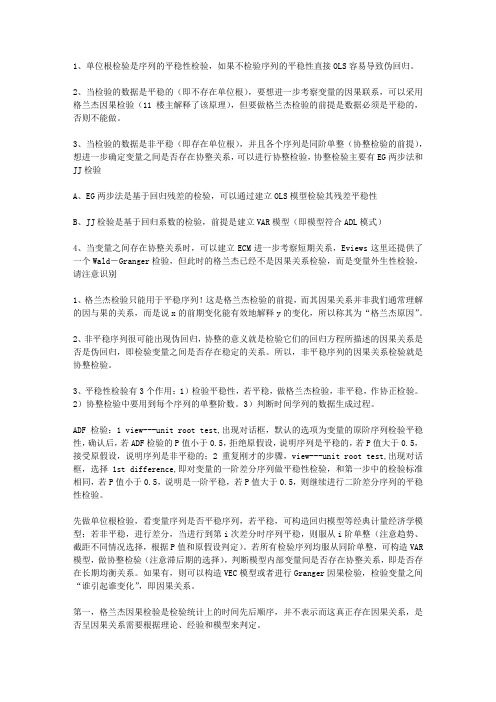
1、单位根检验是序列的平稳性检验,如果不检验序列的平稳性直接OLS容易导致伪回归。
2、当检验的数据是平稳的(即不存在单位根),要想进一步考察变量的因果联系,可以采用格兰杰因果检验(11楼主解释了该原理),但要做格兰杰检验的前提是数据必须是平稳的,否则不能做。
3、当检验的数据是非平稳(即存在单位根),并且各个序列是同阶单整(协整检验的前提),想进一步确定变量之间是否存在协整关系,可以进行协整检验,协整检验主要有EG两步法和JJ检验A、EG两步法是基于回归残差的检验,可以通过建立OLS模型检验其残差平稳性B、JJ检验是基于回归系数的检验,前提是建立VAR模型(即模型符合ADL模式)4、当变量之间存在协整关系时,可以建立ECM进一步考察短期关系,Eviews这里还提供了一个Wald-Granger检验,但此时的格兰杰已经不是因果关系检验,而是变量外生性检验,请注意识别1、格兰杰检验只能用于平稳序列!这是格兰杰检验的前提,而其因果关系并非我们通常理解的因与果的关系,而是说x的前期变化能有效地解释y的变化,所以称其为“格兰杰原因”。
2、非平稳序列很可能出现伪回归,协整的意义就是检验它们的回归方程所描述的因果关系是否是伪回归,即检验变量之间是否存在稳定的关系。
所以,非平稳序列的因果关系检验就是协整检验。
3、平稳性检验有3个作用:1)检验平稳性,若平稳,做格兰杰检验,非平稳,作协正检验。
2)协整检验中要用到每个序列的单整阶数。
3)判断时间学列的数据生成过程。
ADF检验:1 view---unit root test,出现对话框,默认的选项为变量的原阶序列检验平稳性,确认后,若ADF检验的P值小于0.5,拒绝原假设,说明序列是平稳的,若P值大于0.5,接受原假设,说明序列是非平稳的;2 重复刚才的步骤,view---unit root test,出现对话框,选择1st difference,即对变量的一阶差分序列做平稳性检验,和第一步中的检验标准相同,若P值小于0.5,说明是一阶平稳,若P值大于0.5,则继续进行二阶差分序列的平稳性检验。
- 1、下载文档前请自行甄别文档内容的完整性,平台不提供额外的编辑、内容补充、找答案等附加服务。
- 2、"仅部分预览"的文档,不可在线预览部分如存在完整性等问题,可反馈申请退款(可完整预览的文档不适用该条件!)。
- 3、如文档侵犯您的权益,请联系客服反馈,我们会尽快为您处理(人工客服工作时间:9:00-18:30)。
OIL PRICE SHOCKS AND EMERGING STOCK MARKETS:A GENERALIZED VAR APPROACHMAGHYEREH, Aktham* AbstractThis study examines the dynamic linkages between crude oil price shocks and stock market returns in 22 emerging economies. The vector autoregression (VAR) analysis is carried on daily data for the period spanned from January 1, 1998 to April 31, 2004. This study utilized the generalized approach to forecast error variance decomposition and impulse response analysis in favor of the more traditional orthogonal ized approach. Inconsistent with prior research on developed economies, the findings imply that oil shocks have no significant impact on stock index returns in emerging economies. The results also suggest that stock market returns in these economies do not rationally signal shocks in the crude oil market.JEL Classification: G10, G12.Keywords: Oil Prices, Emerging stock markets, VAR model.1. IntroductionThe oil price shock of 1973 and the subsequent recession give rise to a plethora of studies analyzing the interrelation between economic variables and oil price changes. The early studies include Pierce and Enzler (1974), Rasche and Tatom (1977), and Draby (1982), all of which documented and explained the inverse relationship between oil price increases and aggregate economic activity. Later empirical studies-such as, Hickman et al. (1987), Jones and Leiby (1996), Hooker (1999), Hammes and Wills (2003) and Leigh et al. (2003)-* Aktham Maghyereh is Assistant Dean at the Faculty of Economics and Business Administration, the Hashemite University, Jordan. E-mail: maghyreh@.joconfirm the inverse relationship between oil prices and aggregateeconomic activity.Although the bulk of the empirical studies focus on the relationbetween economic activity and oil price changes, it is surprising thatfew studies have been conducted on the relationship between financial markets and oil price shocks- and those mainly for a fewindustrialized countries such as the United States, United Kingdom,Japan, and Canada. For example, Jones and Kaul (1992) examine theeffect of oil prices on stock prices in the U.S. They find an effect ofoil prices on aggregate real stock returns, including a lagged effect,in the period 1947 to 1991. In more recent study, Jones and Kaul(1996) test whether the reaction of international stock markets to oil shocks can be justified by current and future changes in real cash flows and/or changes in expected returns.They find that in the postwar period, the reaction of United States and Canadian stock prices to oil shocks can be completely accounted for by the impact of these shocks on real cash flows. In contrast, the results for both the United Kingdom and Japan are not as strong. In an important study, Haung et al. (1996) examine the link between daily oil future returns and daily U.S. stock returns. The evidence suggests that oil futures returns do lead some individual oil company stock returns but oil future returns do not have much impact on general market indices. Gjerde and Saettem (1999) demonstrate that stock returns have a positive and delayed response to changes in industrial production and that the stock market responds rationally to oil price changes in the Norwegian market. Sadorsky (1999) finds that oil prices play an important role in affecting real stock returns.Although all of these studies recognize the importance of causal relationships between oil prices and stock market returns in some industrial countries, the results from such studies cannot be generalized to other countries. Consequently, this paper extends the understanding on the dynamic relationship between oil prices and stock market return by using data from 22 emerging stock markets, which helps to fill in the gap. Specifically, this paper investigates thedynamic interactions between crude oil prices and stock prices in alarge sample of emerging economies.If oil plays a prominent role in an economy, one would expectchanges in oil prices to be correlated with changes in stock prices.Specifically, it can be argued that if oil affects real economic activity, it will affect earnings of companies through which oil is adirect or indirect cost of operation. Thus, an increase in oil priceswill cause expected earnings to decline, and this would bring aboutan immediate decrease in stock prices if the stock market effectivelycapitalizes the cash flow implications of the oil price increases. If thestock market is inefficient, stock returns might be slowly.Given the evidence of stronger linkages between crude oil pricesand stock markets in developed economies, this study considers thisissue in the emerging economies. The study examines the dynamiclinkages between crude oil prices and stock market returns in manyemerging economies and essentially asks two questions. To whatextent are price changes or returns in crude oil market lead stockreturns in emerging markets? How efficiently are innovations/shocksin crude oil market transmitted to the stock markets in the emerging economies? In answering these questions it is hoped that some light may shed on the importance of the crude oil on economic output in the emerging economies. The vector autoregression (VAR) technique that is employed in this study is well suited to answering these questions.The study utilized the generalized approach to forecast error variance decomposition and impulse response analysis in favor of the more traditional orthogonalized approach. The problem with the orthogonalized approach to variance decomposition and impulse response analysis is that the order of the variables in the VAR determines the outcome of the results. The generalized approach is invariant to the ordering of the variables in the VAR and produces one unique result.The paper proceeds as follows. Section 2 describes the data used in the paper. Section 3 provides an overview of the methodological issues. Section 4 presents the empirical evidence. Section 5 provides some concluding remarks.2. DataThe stock market data in this paper are obtained from Morgan Stanley Capital International (MSCI). The sample is daily encompasses the period from 1 January 1998 to 31 April 2004 and contains U.S. dollar dominated value-weighted stock market indices for the following 22 emerging countries: Argentina, Brazil, Chile, China, Czech Republic, Egypt, Greece, India, Indonesia, Jordan, Korea, Malaysia, Mexico, Morocco, Hungary, Pakistan, Philippines, Poland, South Africa, Taiwan, Thailand, and Turkey.We choose to use the MSCI indices rather than other local stock price indices for several reasons. First, these indices are constructed on a consistent basis by the MSCI, making cross-country comparison more meaningful. Second, these indices are value-weighted reflects a substantial percentage of total market capitalization which could minimize the problem of autocorrelation in returns result from nonsynchronous trading. Third, MSCI indices are widely employed in the literature on the basis of the degree of comparability and avoidance of dual listing.The crude oil market is the largest commodity market in the world. Total world consumption equals around 80 million barrels a day in 2003. Prices of three types of oil- Brent, West Texas Intermediate and Dubai-serve as a benchmark for other types of crude oil. Processing costs and therefore prices of oil depend on two important characteristics: sulphur content and density. Oil that has a low sulphur content ("sweet") and a low density ("light") is cheaper than process than oil that has a high sulphur content ("sour") and high density ("heavy"). For instance the price of West Texas Intermediate is generally higher than Brent oil as it is sweeter and lighter than Brent oil. Of total world oil consumption of about 80 million barrelsa day in 2003, Brent oil serves as a benchmark for about 50 millionbarrels a day, West Texas Intermediate for about 15 million barrels aday and Dubai for about 15 million barrels a day.Even though price differences do exist, crude oil prices tend tomove very closely together. Since Brent oil serves as a benchmark in the crude oil market, daily closing prices of crude oil Brent are usedas our primary proxy for the world price of crude oil1. The dailyclosing prices for crude oil Brent for the period from 1 January 1995to 31 April 2004 are obtained from the U.S. Energy InformationAdministration. Finally, consistent with convention, all data used inthis study has been transformed by taking the natural logarithm ofthe raw data.3.- MethodologyThe unrestricted vector autoregression (VAR) approach used inthis study was developed by Sims (1980). The VAR was developedto account for problems with intervention and transfer functionanalysis. This model provides a multivariate framework wherechanges in a particular variable are related to changes in its own lags and to changes in other variables. The VAR treats all variables as jointly endogeneous and imposes no a priori restrictions on the structural relationships, if any, between variables being analyzed. Because the VAR expresses the dependent variables in terms of only predetermined lagged variables, the VAR model is a reduced form model.An argument that naturally arises in the context of a VAR iswhether one should use levels or first differences in the VAR.Clearly if the variable are I(0) processes this is not an issue. Thedifficultly arises, however, when the variables need to be differencedto get a stationary process, as they almost invariably do when dealingstock index data. Because of the information that is lost in 1We also estimated the results using daily for Arab light, Arab Medium, Dubai and West Texas as alternatives for the world price of oil and found these measures did not substantively affect our results.differencing, Sims (1980) and Doan (1992) have argued against it. The majority view, highlighted by Granger and Newbold (1974) and Phillips (1986) is that stationary data should be used since non-stationary data can lead to spurious regression results. Further, Toda and Yamamoto (1995) noted that conventional asymptotic theory is, in general, not applicable to hypothesis testing in levels VARs if the variables are integrated, say I(1). Thus, as the first step, the order of integration of the variables is tested. Tests for the presence of a unit root based on the work of Dickey and Fuller (1979, 1981), Perron (1988), Phillips (1987), Phillips and Perron (1988)2, and Kwiatkowski et al . (1992)3 are used to investigate the degree of integration of the variables used in the empirical analysis. If a I(1) process does exist, the second step involves estimation of the VAR model with first differences 4, otherwise VAR is estimated in levels.To determine the appropriate number of lag length of the VAR2 makes a semi-parametric correlation for autocorrelation and is more robust in the case of weakly autocorrelated and heteroskedastic regression residuals.3 The KPSS procedure assumes the univariate series can be decomposed into the sum of a deterministic trend, random walk, and stationary I(0) disturbance and is based on a Lagrange Multiplier score testing principle. This test reverses the null and alternative hypothesis. A finding favorable to a unit root in this case requires strong evidence against the hypothesis of stationarity.4 Earlier studies of stock returns have shown that stock returns exhibit a number of important seasonalities (e.g. January and week-end effects). These sesonalities are accounted for in our analysis by introducing dummy variables in the VAR model. Furthermore, important events in oil and equity markets during the period under investigation are the September 11th attacks and its subsequent and the U.S. invitation of Iraq on March 19. Oil and equity markets fluctuated dramatically as a consequence of these events, therefore these events are also accounted for in the analysis by introducing dummy variables.Next, the generalized variance decomposition and generalizedimpulse response functions are employed to analysis the short-rundynamics of the variables. The purpose of the investigation is to findhow each of emerging markets responds to shocks by the crude oilmarket. The forecast-error of generalized variance decompositionanalysis reveals information about the proportion of the movements in market returns due to its “own” shocks versus shocks to the oilcrude market. The dynamic responses of stock market to innovationsin the c rude oil market can also be traced out using the generalizedimpulse response analysis. Plotting the generalized impulse responsefunctions is a particular way to explore the response of a stockmarket to a shock immediately or with various lags. Unlike t h eorthogonalized variance decomposition and impulse response functions obtained using the Choleskey factorization, the generalized variance decomposition and impulse response functions are unique solution and invariant to the ordering of the variables in the VAR (Koop et al. 1996; and Pesaran and Shin, 1998).Another argument that arises in the context of an unrestricted VAR is whether this model should be used where the variables in the VAR are cointegrated. There is a body of literature that supports the use of a vector error correction model (VECM), or cointegrating VAR if variables are integrated, I(1). Because the cointegrating vectors bind the long run behavior of the variables, the VECM is expected to produce results in the impulse response analysis and variance decomposition that more accurately reflect the relationship between the variables than the standard unrestricted VAR.It has been argued, however, that in the short run unrestricted VARs perform better than a cointegrating VAR (see for example, Naka and Tufte, 1997). Furthermore, Engle and Yoo (1987), Clements and Hendry (1995), and Hoffman and Rasche (1996) have shown that an unrestricted VAR is superior (in terms of forecast variance) to a restricted VECM at short horizons when the restriction is true. Naka and Tufte (1997) also studied the performance of VECMs and unrestricted VARs for impulse response analysis over the short-run and found that the performance of the two methods isnearly identical. This suggests that abandoning vector autoregressions for short horizon work is premature, especially when one considers their low computational burden. Although Johansen multivariate cointegration analysis is carried out in this study and cointegrating relationships founds, unrestricted VARs are used impulse response analysis.4. Empirical Resultsstationary variables, these results necessitated the use of first differenced data to carry out the VAR analysis.As we mentioned above, the interpretation of the VAR model can brought to light through the generalized variance decomposition analysis and the estimation of the generalized impulse response functions. The results of variance decomposition are presented in Table 2. The reported numbers indicate the percentage of the forecast error in each stock market that can be attributed to innovations in the crude oil market at four different time horizons: one day, 5, 10 and 15-day ahead. The results of generalized variance decomposition analysis and generalized impulse response function provide the same conclusions regardless of order of decomposition since their estimation is independent of the ordering.The generalized decomposition tends to suggests that the crude oil price shocks have no significant impact on any of emerging stock market under investigation. Specifically, in all cases the crude oil shocks explain less than 2% of the forecast errors variances and in 16 of the 22 emerging markets this ratio falls to less than 1%.Table 1. Unit Root TestsLevel First Difference Variable ADF PP KPSS ADF PP KPSS Argentina -1.0 -1.1 2.9* -16.1* -36.4* 0.3 Brazil -1.3 -1.3 1.4* -16.1* -30.5* 0.2 China -1.1 -1.1 1.4* -13.5* -28.1* 0.3 Czech Rep. -1.5 -1.4 3.1* -15.5* -32.5* 0.3 Egypt -1.4 -1.2 3.5* -15.1* -30.5* 0.4 Greece 0.1 -0.1 2.4* -16.8* -33.5* 0.2 India -1.0 -0.9 3.1* -14.6* -34.4* 0.6 Indonesia -1.1 -1.0 1.3* -15.1* -31.5* 0.3 Jordan -1.4 -1.3 1.8* -15.8* -31.2* 0.3 Korea 2.1 1.9 2.5* -16.2* -34.8* 1.2 Malaysia -1.6 -1.6 0.8* -16.4* -34.6* 0.1 Mexico -2.2 -2.3 0.1 -14.7* -30.8* 0.4 Morocco -2.5 -2.5 0.2 -16.8* -31.5* 0.1 Hungary -1.7 -1.6 2.9* -14.6* -30.7* 0.6 Pakistan -1.0 -0.9 2.7* -15.2* -32.8* 0.8 Philippines -0.4 -0.5 0.9* -14.7* -34.3* 0.5 Poland -0.4 -0.5 1.7* -14.7* -34.3* 0.3-1.1 -1.2 3.2* -14.4* -30.1* 0.2 SouthAfricaTaiwan -2.5 -2.4 1.6* -16.1* -30.8* 0.1 Thailand -1.7 -1.7 2.5* -15.3* -32.8* 0.2 Turkey -1.4 -1.4 0.9* -15.1* -34.1* 0.3 Oil Price -0.9 -1.7 0.3** -25.1* -83.2* 0.4-3.4 -3.4 0.7 -3.4 -3.4 0.7 CriticalValue 1%Critical-2.9 -2.9 0.5 -2.5 -2.9 0.5 Value 5%Notes: *and ** indicate statistical significant at the 1% and 5% level, respectively. The Augmented Dickey-Fuller (ADF) and the Phillips-Perron (PP) tested the null hypothesis of that the relevant series contains a unit root I(1) against the alternative that it does not, while the Kwiatowski-Pillips-Schmidt-Shin (KPSS) tested the null hypothesis that the series are I(0). The critical values for the ADF and PP are obtained from Dickey-Fuller (1981) while the KPSS critical values are obtained from Kwiatkowski et al. (1992).Table 2. Generalized decomposition of forecast error in emergingstock markets in response to shocks in the crude oil market (%)5 days 10 days 15 daysArgentina 0.622064 0.634352 0.641797Brazil 0.181891 0.237485 0.255282China 0.076167 0.053178 0.045921Czech Republic 0.169356 0.245609 0.270753Egypt 0.065641 0.086715 0.093538Greece 1.146167 1.393299 1.546380India 0.212965 0.731871 1.074984Indonesia 0.014805 0.036561 0.049455Jordan 0.172647 0.586863 0.855067Korea 1.703050 2.020706 2.028246Malaysia 1.375227 2.005126 2.384872Mexico 0.042720 0.178622 0.261737Morocco 0.022182 0.015533 0.012700Hungary 0.117332 0.076421 0.059594Pakistan 0.110283 0.272700 0.370517Philippines 0.041558 0.036562 0.034073Poland 0.041558 0.036562 0.034073South Africa 1.130735 1.734103 2.051688Taiwan 1.115818 1.353554 1.501680Thailand 0.157792 0.530656 0.817114Turkey 2.101294 2.381148 2.568307Furthermore, the results show some interesting differences acrosscountries in response to the oil market shocks, depending on theenergy intensity of consumption and production. Specifically, the impact of oil shocks on stock m arket is highest in the largest Asianand Emerging Europe economies, as they have higher energyintensity consumption than most other emerging economies. Forexample, at the 15-day horizons, the percentage of error variance of astock market explained by innovations in the crude oil market is 2.57for Turkey followed by 2.38 for Malaysia. The Poland marketappears to be the least influence by the oil market.Turning to the question of how effectively innovations may transmit from the oil market to the emerging stock markets, Figure 1 plots the responses of each of the twenty two emerging stock markets to a one standard error shock in the oil market. The plots in Figure 1 show that innovations in the oil market are slowly transmitted in all of the emerging stock markets with markets responding to the oil shock two day after the shock.The speed with which the responses taper off to zero after the initial shock is felt for the most markets on day 4. Only six markets namely: Argentina, Brazil, China, Czech Republic Egypt and Greece, responses continuous until day 7. These results may indicate that the emerging markets are inefficient in transmitting innovations/shocks in the oil market. The inefficiency in responses to a shock in the oil market is also reflected in the inaccuracy of the initial response, to the shock. However, the small size of the responses (between 0.00051 to 0.00126, on day 2) reflecting that the oil market is very weak in influencing stock markets in emerging economies.5.- ConclusionThis study examines the dynamic linkages between oil price shocks and stock market returns in 22 emerging economies. Vector autoregression (VAR) analysis is carried on daily data for the period, January 1, 1998 to April 31, 2004. This study utilized the generalized approach to forecast error variance decomposition and impulse response analysis in favor of the more traditional orthogonalized approach. Inconsistent with the pervious empirical studies in developed economies, the results from the variance decomposition analysis provided very weak evidence that there is a relationship between the crude oil price shocks and stock market returns in the emerging economies. Furthermore, the results from impulse analysis reveal that innovations in the oil market are slowly transmitted in the emerging stock markets. These results suggest that stock markets in the emerging economies are inefficient in transmission of new information of the oil market.These results may also indicate that the importance of oil price for the aggregate economy, especially inemerging economies, is greatly over-estimated. These results may also suggest that the stock market returns in the emerging economies do not rationally signal changes in the crude oil prices.R EFERENCESClements, M.P. and D.F. Hendry, 1995, "Forecasting in Cointegrated System," Journal of Applied Econometrics, 10, 127-146.Darby, M. R., 1982, "The Price of Oil and World Inflation and Recession," American Economic Review, 72, 738-751.Dickey, D., W.A. Fuller, 1979, "Distribution of the Estimates for Autoregressive Time Series with a Unit Root," Journal of the American Statistical Association, 74, 427-0431.Dickey, D., W.A. Fuller, 1981, "Likelihood Ratio Statistics for Autoregressive Time Series with a Unit Root," Econometrica, 49, 1057-1072.Doan, T. 1992, RATS User's Manual, Evanston III: Estima.Engle, R.F. and B.S. Yoo, 1987, "Forecasting and Testing in Cointegrated Systems," Journal of Econometrics, 35, 143-159. Granger, C.W.J and P. Newbold, 1994, "Spurious Regressions in Econometrics," Journal of Econometrics, 2, 111-120.Hammes, D. and D. Wills, 2003, “Black Gold: The End of Bretton Woods and the Oil Price Shocks of the 1970s,” Working Paper, University of Hawaii Hilo.Hickman, B., H. Huntington, and J. Sweeney, 1987, Macroeconomic Impacts of Energy Shocks, Amsterdam: north-Holland. Hoffman, D.L. and R.H. Rasche, 1996, "Assessing Forecast Performance in a Cointegrated System," Journal of Applied Econometrics, 11, 495-517.Hooker, M. 1999, "Are O il Shocks Inflationary? Asymmetric and Nonlinear Specifications versus Changes in Regime," Working Paper, Federal Reserve Board of Governors.Huang, R. D., R. W. Masulis, and H. R. Stoll, 1996, "Energy Shocks and Financial Markets," 27. The Journal of Future Markets, 16, 1-25.Jones, C. M. and G. Kaul, 1992, "Oil and Stock Markets," Journal of Finance, 51, 463-491.Jones, C. M. and G. Kaul, 1992, "Oil and Stock Markets," Working Paper, University of Michigan.Jones, D. W. and P. Leiby, 1996, "The Macroeconomic Impacts of Oil Price Shocks: A review of the Literature and Issues," Working Paper, Oak Ridge National Laboratory.Kwiatkowski, D., P.C.B. Phillips, P. Schmidt, and Y. Shim, 1992, "Testing the Null Hypothesis of Stationarity Against the Alternative of a Unit Root," Journal of Econometrics, 54, 159-178.Leigh, A., J. Wolfers, and E. Zitzewitz, 2003, "What do financial Markets Think about the War of Iraq?" Working Paper, Stanford Graduate School of Business.Naka, A. and D. Tufte, 1997, "Examining Impulse Response Functions in Cointegrated Systems," Applied Economics, 29, 1593-1603.Perron, P., 1988, "Trends and Random Walks in Macroeconomic Time: Series Further Evidence from a New Approach," Journal of Economic Dynamic and Control, 12, 297-332.Phillips, P.C.B. and P. Perron, 1988, "Testing for a Unit Root in Time Series regression," Biometrika, 75, 335-346.Phillips, P.C.B., 1986, "Understanding Spurious Regressions in Econometrics," Journal of Econometrics, 33, 311-340.Phillips, P.C.B., 1987, "Time Series Regression with a Unit Root," Econometrica, 55, 277-347.Pierce J. L., and J. E. Jared, 1974, "the Effects of External Inflationary Shocks," Brooking Papers on Economic Activity, 1, 13-61.Rasche, R. H., and J. A. Tatom, 1977, "The effect of the New energy Regime on Economic Capacity, Production and Prices," Economic Review, 59, 2-12.Sadorsky, P., 1999, "Oil Price Shocks and Stock Market Activity," Energy Economics, 21, 449-469.Sims, C. A., 1980, "Macroeconomics and reality," Econometrica, 48, 1-48.Toda, B.H. and T. Yamanoto, 1995, "Statistical Inference in Vector Autoregressions with Possibly Int.____________________Journal IJAEQS published by AEEADE: c.es/economet/eaa.htm。