Time Series Analysis
Time Series Analysis(英文版)(ppt 37页)

1999
2020/1/19
-12-
O:/Network/Path/Filename.ppt
Advantages of Time Series
• Advantages of time series: – Removes variation in monthly sales caused by calendar differences and seasonality – Help us to accurately estimate the direction and rate of sales growth/decline – They are an improvement over other methods such as year-over-year growth or moving averages because they show us what is happening sooner… an early warning of changing sales conditions
211
X 1.004 ÷ 1.210 =
175
Jul-03
221
X 0.958 ÷ 1.212 =
175
Aug-03
196
X 1.054 ÷ 1.190 =
174
Sep-03
160
X 1.004 ÷ 0.948 =
169
2020/1/19
-10-
O:/Network/Path/Filename.ppt
2020/1/19
-7-
O:/Network/Path/Filename.ppt
Selling Days
August 2003 SMTWT F S
第八章 时间序列分析
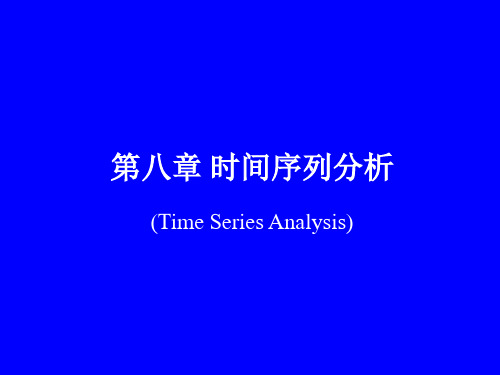
这类检验可用t检验进行,检验统计量为:
t
ˆ
S
(6)
ˆ 的标准误差。 其中,S 为参数估计值
这里的问题是,(6)式计算的t值不服从t分布,而 是服从一个非标准的甚至是非对称的分布。因而不 能使用t分布表,需要用另外的分布表。
DF检验
迪奇( Dickey ) 和福勒( Fuller )以蒙特卡罗 模拟为基础,编制了( 6 )中 tδ 统计量的临界值表, 表中所列已非传统的t统计值,他们称之为η 统计值。 后来该表由麦金农(Mackinnon)通过蒙特卡罗模拟 法加以扩充。 综上所述,DF检验法建立在下面方程的基础上:
为了放宽 ARCH 模型的约束条件, Bollerslov ( 1986 ) 提 出 了 GARCH 模 型 ( generalized autoregressive conditional heteroscedasticity model); 随后, Engle 等( 1987 )提出了 ARCH-M 模型( ARCHin-mean model ), GARCH-M 模型; Nelson(1991) 提出 了 EGARCH 模 型 ( exponential GARCH model ) ; Glosten 等 ( 1993 ) 提 出 了 TARCH 模 型 ( Threshold ARCH model)等,这些异方差模型是对经典的ARIMA模 型的很好补充,能够更为准确的刻画金融市场风险的 变化过程,特别适用于金融时间序列的分析。
根据平稳时间序列均值、方差为常数的特点, 可知平稳序列的时间序列图应该围绕其均值随机 波动,且波动的范围有界。如果所考察的时间序 列的时间序列图具有明显的趋势性或者周期性, 那么通常认为该序列是不平稳的。
时间序列分析课件

时间序列分析方法
时间序列分析的基本思想
同样,对于时间序列可以在时间域中进行分析,也可以在频 率域中进行分析,也可以进行时频分析(即在时间域和频率域中 同时进行分析),还可以对其进行统计分析。
无论采用何种分析方法,无论在哪个数据空间(域)对时间序列 进行分析,其目的都是把原来的序列分解成为一系列便于理解 和处理的成分,然后分别对这些成分进行某种处理后重新进行 叠加组合,最终实现预测和控制的目的。
时间序列可能是应用最普遍的数据表现形式和数据存储格式。 在生物医学领域也存在着大量的时间序列,
人体从清晨到深夜的体温记录 人体餐后血糖浓度的变化 不同睡眠阶段的脑电波形等都是时间序列 。。。。。。
绝大多数情况下时间序列被看作为随机序列。
时间序列
时间序列的表示
X {x(T ), x(2T ),, x(kT )} {x[n]}
时间序列的分类
Noisy
Trending
Meandering
Periodic/Cyclic
Quasiperiodic源自Integer-Valued
时间序列
时间序列的组合成分
(1)长期趋势(T) (2)指时间序列随时间的变化而逐渐增加或减少的长期变化趋势。
(2) 季节变动(S) 指时间序列在一年中或固定时间内,呈现出的固定规则的变 动。 (3) 循环变动(C) 指沿着趋势线如钟摆般地循环变动,即周期性成分。 (4) 不规则变动(I) 指在时间序列中由于随机因素影响所引起的变动。
……
时间序列分析方法
时间序列分析的基本思想
序列=确定性成分+随机性成分
对于这两种成分可以采用不同的分析方法进行提取和处理,
最终达到预测和控制的目的。
确定型时间序列分析方法
Chapter 4 Time Series Analysis
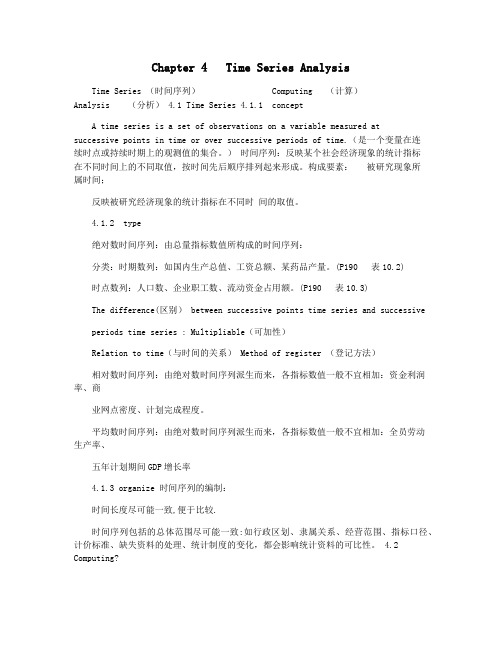
Chapter 4 Time Series AnalysisTime Series (时间序列) Computing (计算)Analysis (分析) 4.1 Time Series 4.1.1 conceptA time series is a set of observations on a variable measured atsuccessive points in time or over successive periods of time.(是一个变量在连续时点或持续时期上的观测值的集合。
)时间序列:反映某个社会经济现象的统计指标在不同时间上的不同取值,按时间先后顺序排列起来形成。
构成要素:被研究现象所属时间;反映被研究经济现象的统计指标在不同时间的取值。
4.1.2 type绝对数时间序列:由总量指标数值所构成的时间序列:分类:时期数列:如国内生产总值、工资总额、某药品产量。
(P190 表10.2)时点数列:人口数、企业职工数、流动资金占用额。
(P190 表10.3)The difference(区别) between successive points time series and successiveperiods time series : Multipliable(可加性)Relation to time(与时间的关系) Method of register (登记方法)相对数时间序列:由绝对数时间序列派生而来,各指标数值一般不宜相加:资金利润率、商业网点密度、计划完成程度。
平均数时间序列:由绝对数时间序列派生而来,各指标数值一般不宜相加:全员劳动生产率、五年计划期间GDP增长率4.1.3 organize 时间序列的编制:时间长度尽可能一致,便于比较.时间序列包括的总体范围尽可能一致:如行政区划、隶属关系、经营范围、指标口径、计价标准、缺失资料的处理、统计制度的变化,都会影响统计资料的可比性。
《时间序列分析》课程教学大纲(本科)
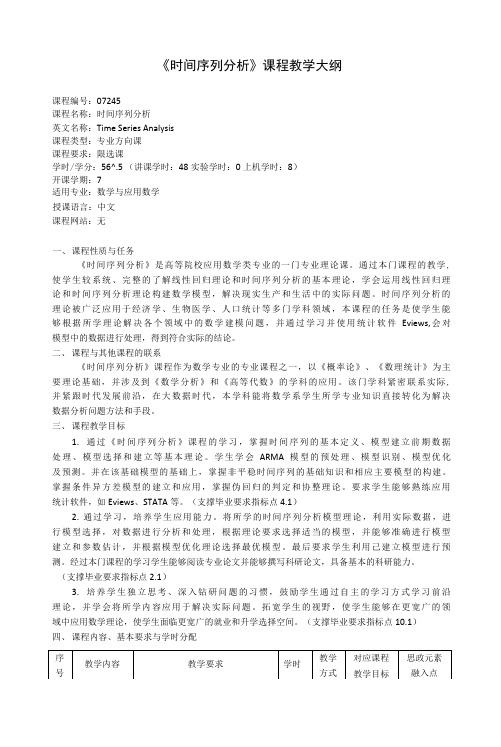
《时间序列分析》课程教学大纲课程编号:07245课程名称:时间序列分析英文名称:Time Series Analysis课程类型:专业方向课课程要求:限选课学时/学分:56^.5 (讲课学时:48实验学时:0上机学时:8)开课学期:7适用专业:数学与应用数学授课语言:中文课程网站:无一、课程性质与任务《时间序列分析》是高等院校应用数学类专业的一门专业理论课。
通过本门课程的教学, 使学生较系统、完整的了解线性回归理论和时间序列分析的基本理论,学会运用线性回归理论和时间序列分析理论构建数学模型,解决现实生产和生活中的实际问题。
时间序列分析的理论被广泛应用于经济学、生物医学、人口统计等多门学科领域,本课程的任务是使学生能够根据所学理论解决各个领域中的数学建模问题,并通过学习并使用统计软件Eviews,会对模型中的数据进行处理,得到符合实际的结论。
二、课程与其他课程的联系《时间序列分析》课程作为数学专业的专业课程之一,以《概率论》、《数理统计》为主要理论基础,并涉及到《数学分析》和《高等代数》的学科的应用。
该门学科紧密联系实际, 并紧跟时代发展前沿,在大数据时代,本学科能将数学系学生所学专业知识直接转化为解决数据分析问题方法和手段。
三、课程教学目标1.通过《时间序列分析》课程的学习,掌握时间序列的基本定义、模型建立前期数据处理、模型选择和建立等基本理论。
学生学会ARMA模型的预处理、模型识别、模型优化及预测。
并在该基础模型的基础上,掌握非平稳时间序列的基础知识和相应主要模型的构建。
掌握条件异方差模型的建立和应用,掌握伪回归的判定和协整理论。
要求学生能够熟练应用统计软件,如Eviews、STATA等。
(支撑毕业要求指标点4.1)2.通过学习,培养学生应用能力。
将所学的时间序列分析模型理论,利用实际数据,进行模型选择,对数据进行分析和处理,根据理论要求选择适当的模型,并能够准确进行模型建立和参数估计,并根据模型优化理论选择最优模型。
时间序列分析法概述

时间序列分析法概述时间序列分析(Time Series Analysis)是一种对时间序列数据进行统计分析和预测的方法。
时间序列数据是以时间顺序排列的、按一定时间间隔收集到的一系列数据观测值。
时间序列分析通过对过去的数据进行分析,揭示出数据内部的规律和变化趋势,从而对未来的数据进行预测和模拟。
时间序列分析方法广泛应用于经济学、金融学、工程学、气象学等领域,可以用于分析和预测股票价格、销售数据、气温变化等各种现象。
时间序列分析方法包括描述性统计分析、平稳性检验、自相关与偏相关分析、谱分析、移动平均模型和自回归模型等。
描述性统计分析是时间序列分析的起点,其目的是对时间序列数据的基本特征进行描述和总结。
描述性统计分析通常包括计算数据的均值、方差、极值等指标,以及绘制数据的线图、直方图等图形。
通过对描述性统计分析的结果进行观察和比较,可以初步了解数据的分布和趋势。
平稳性检验是时间序列分析的基础,其目的是判断时间序列数据是否具有平稳性。
平稳性是指时间序列数据的统计特性在不同时间段内是相似的,即均值和方差不随时间的变化而变化。
常用的平稳性检验方法有ADF检验和KPSS检验。
如果时间序列数据不具有平稳性,需要进行平稳化处理,以满足时间序列分析的前提条件。
自相关与偏相关分析是时间序列分析中的重要内容,其目的是研究时间序列数据之间的相关性和连接性。
自相关是指时间序列数据与其在不同时间点上的滞后值之间的相关性,反映了时间序列数据的时间间隔相关性。
偏相关是在控制其他变量的影响下,研究两个时间序列数据之间的相关性。
通过自相关与偏相关分析,可以揭示时间序列数据内部的规律和关系。
谱分析是时间序列分析的重要方法之一,其目的是研究时间序列数据的频率特征和功率谱密度。
谱分析基于傅里叶变换,将时间序列数据转换到频域分析。
谱分析可以揭示时间序列数据的周期性和趋势性,为进一步的数据分析和预测提供依据。
移动平均模型是一种常用的时间序列预测方法,它基于过去若干个时间点的数据,预测未来一个时间点的数据。
《时间序列分析》(双语)课程教学大纲
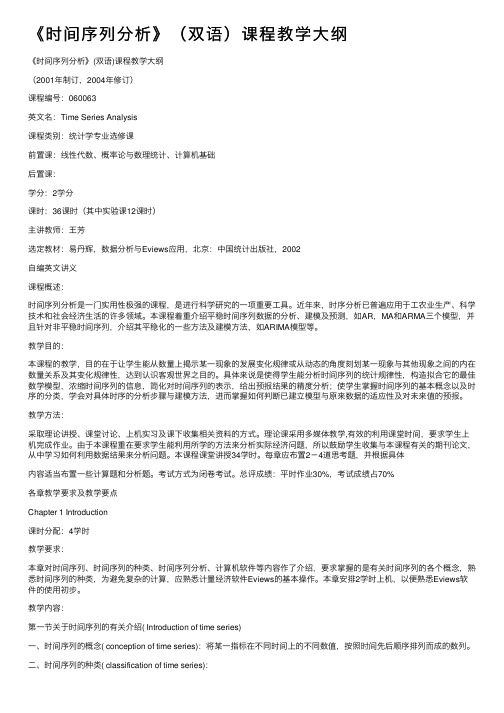
《时间序列分析》(双语)课程教学⼤纲《时间序列分析》(双语)课程教学⼤纲(2001年制订,2004年修订)课程编号:060063英⽂名:Time Series Analysis课程类别:统计学专业选修课前置课:线性代数、概率论与数理统计、计算机基础后置课:学分:2学分课时:36课时(其中实验课12课时)主讲教师:王芳选定教材:易丹辉,数据分析与Eviews应⽤,北京:中国统计出版社,2002⾃编英⽂讲义课程概述:时间序列分析是⼀门实⽤性极强的课程,是进⾏科学研究的⼀项重要⼯具。
近年来,时序分析已普遍应⽤于⼯农业⽣产、科学技术和社会经济⽣活的许多领域。
本课程着重介绍平稳时间序列数据的分析、建模及预测,如AR,MA和ARMA三个模型,并且针对⾮平稳时间序列,介绍其平稳化的⼀些⽅法及建模⽅法,如ARIMA模型等。
教学⽬的:本课程的教学,⽬的在于让学⽣能从数量上揭⽰某⼀现象的发展变化规律或从动态的⾓度刻划某⼀现象与其他现象之间的内在数量关系及其变化规律性,达到认识客观世界之⽬的。
具体来说是使得学⽣能分析时间序列的统计规律性,构造拟合它的最佳数学模型,浓缩时间序列的信息,简化对时间序列的表⽰,给出预报结果的精度分析;使学⽣掌握时间序列的基本概念以及时序的分类,学会对具体时序的分析步骤与建模⽅法,进⽽掌握如何判断已建⽴模型与原来数据的适应性及对未来值的预报。
教学⽅法:采取理论讲授、课堂讨论、上机实习及课下收集相关资料的⽅式。
理论课采⽤多媒体教学,有效的利⽤课堂时间,要求学⽣上机完成作业。
由于本课程重在要求学⽣能利⽤所学的⽅法来分析实际经济问题,所以⿎励学⽣收集与本课程有关的期刊论⽂,从中学习如何利⽤数据结果来分析问题。
本课程课堂讲授34学时。
每章应布置2-4道思考题,并根据具体内容适当布置⼀些计算题和分析题。
考试⽅式为闭卷考试。
总评成绩:平时作业30%,考试成绩占70%各章教学要求及教学要点Chapter 1 Introduction课时分配:4学时教学要求:本章对时间序列、时间序列的种类、时间序列分析、计算机软件等内容作了介绍,要求掌握的是有关时间序列的各个概念,熟悉时间序列的种类,为避免复杂的计算,应熟悉计量经济软件Eviews的基本操作。
时间序列分析
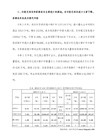
进行时间序列分析中的各种统计分析,以代替对ω(t)的分析。在理论上也已证明,在适当的条件下,这样的替代具有满意的渐近性质。由于ω(t)的真值不能直接量测,这些理论结果显然有重要的实际意义。这方面的研究仍在不断发展。 时间序列分析中的最优预测、控制与滤波等方面的内容见平稳过程条。近年来多维时间序列分析的研究有所进展,并应用到工业生产自动化及经济分析中。此外非线性模型统计分析及非参数统计分析等方面也逐渐引起人们的注意。
中文名称:时间序列分析 英文名称:time series analysis 定义:对沿一个方向演化形成的数据序列特征的统计分析。 所属学科:地理学(一级学科);数量地理学(二级学科)
时间序列分析(Time series analysis)是一种动态数据处理的统计方法。该方法基于随机过程理论和数理统计学方法,研究随机数据序列所遵从的统计规律,以用于解决实际问题。
的。特别是关于p,q值的估计及其渐近理论,出现得更晚些。除ARMA模型之外,还有其他的模型分析的研究,其中以线性模型的研究较为成熟,而且都与ARMA模型分析有密切关系。 公式
公式
回归分析 如果时间序列x(t)可表示为确定性分量φ(t)与随机性分量ω(t)之和,根据样本值x(1),x(2),…,x(T)来估计φ(t)及分析ω(t)的统计规律,属于时间序列分析中的回归分析问题。它与经典回归分析不同的地方是,ω(t)一般不 公式
于满足ARMA模型的平稳序列,其线性最优预测与控制等问题都有较简捷的解决方法,尤其是自回归模型,使用更为方便。G.U.尤尔在1925~1930年间就提出了平稳自回归 公式
的概念。1943年,Η.Β.曼和Α.瓦尔德发表了关于这种模型的统计方法及其渐近性质的一些理论结果。一般ARMA模型的统计分析研究,则是20世纪60年代后才发展起来 公式
时间序列分析(Time Serials Analysis)2

k y= − bx 1 + ae
(a、b、k均>0)
逻辑(皮尔)曲线
其一般形式为: 其一般形式为:
L Yt = − bt 1 + ae
其中,L为变量Yt的极限值, a,b为常数,t为时间变量
龚珀兹曲线
龚珀兹曲线可以描述一种新产品从试制期到
饱和期产量的增长趋势。 饱和期产量的增长趋势。
Yt = ka (0 < a < 1, 0 < b < 1)
将时间序列数据均分为三个部分, 将时间序列数据均分为三个部分,分别 求和。 求和。 根据S1,S 2,S3的三等式,求参数:
S3 − S 2 b = S 2 − S1
n
S 2 − S1 1 k = ( S1 − n ) n b −1
b −1 a = ( S 2 − S1 ) n 2 (b − 1)
生长曲线拟合
计算不一致系数u
yi 估计值 yi 估计值) ( yi -yi 估计值 ) 3218.1 739428.01 16630084 4404.5 331200.25 24800400 5590.9 63453.61 28504921 6777.3 901170.49 33965584 7963.7 713518.09 50680161 9150.1 883788.01 67404100 10336.5 2.25 106874244 11522.9 2399710.81 170877184 499736678 6032271.52 U=sqrt(∑ (yi-yi估计值)2)/ ∑yi 2 ) 估计值) 估计值
逐步修匀法
移动平均法(一次、二次、 移动平均法(一次、二次、三次移动平 均) 指数平滑法(一次指数平滑、 指数平滑法(一次指数平滑、二次指数 平滑和三次指数平滑) 平滑和三次指数平滑)
Time Series Analysis and Forecasting
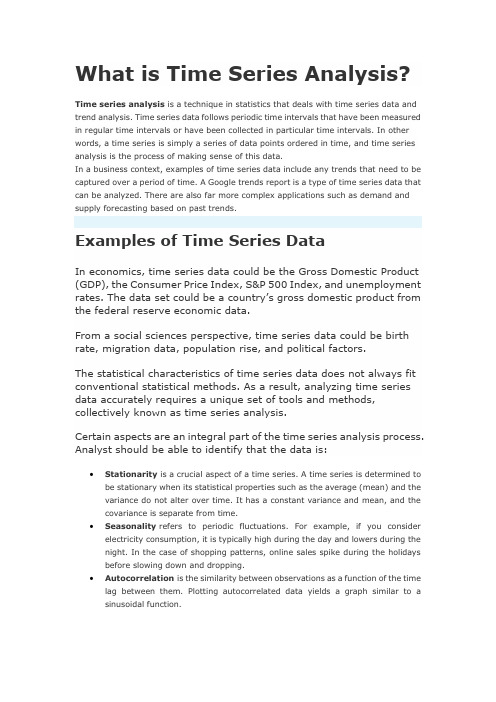
What is Time Series Analysis?Time series analysis is a technique in statistics that deals with time series data and trend analysis. Time series data follows periodic time intervals that have been measured in regular time intervals or have been collected in particular time intervals. In other words, a time series is simply a series of data points ordered in time, and time series analysis is the process of making sense of this data.In a business context, examples of time series data include any trends that need to be captured over a period of time. A Google trends report is a type of time series data that can be analyzed. There are also far more complex applications such as demand and supply forecasting based on past trends.Examples of Time Series DataIn economics, time series data could be the Gross Domestic Product (GDP), the Consumer Price Index, S&P 500 Index, and unemployment rates. The data set could be a country’s gross domestic product from the federal reserve economic data.From a social sciences perspective, time series data could be birth rate, migration data, population rise, and political factors.The statistical characteristics of time series data does not always fit conventional statistical methods. As a result, analyzing time series data accurately requires a unique set of tools and methods, collectively known as time series analysis.Certain aspects are an integral part of the time series analysis process. Analyst should be able to identify that the data is:•Stationarity is a crucial aspect of a time series. A time series is determined to be stationary when its statistical properties such as the average (mean) and the variance do not alter over time. It has a constant variance and mean, and the covariance is separate from time.•Seasonality refers to periodic fluctuations. For example, if you consider electricity consumption, it is typically high during the day and lowers during the night. In the case of shopping patterns, online sales spike during the holidays before slowing down and dropping.•Autocorrelation is the similarity between observations as a function of the time lag between them. Plotting autocorrelated data yields a graph similar to a sinusoidal function.Data: Types, Terms, and ConceptsData, in general, is considered to be one of these three types:1.Time series data: A set of observations on the values that a variable takes onat different points of time.2.Cross-sectional data: Data of one or more variables, collected at the samepoint in time.3.Pooled data: A combination of time series data and cross-sectional data. These are some of the terms and concepts associated with time series data analysis:•Dependence: Dependence refers to the association of two observations with the same variable at prior time points.•Stationarity: This parameter measures the mean or average value of the series.If a value remains constant over the given time period, if there are spikes throughout the data, or if these values tend toward infinity, then it is not stationarity.•Differencing: Differencing is a technique to make the time series stationary and to control the correlations that arise automatically. That said, not all time series analyses need differencing and doing so can produce inaccurate estimates.•Curve fitting: Curve fitting as a regression method is useful for data not in a linear relationship. In such cases, the mathematical equation for curve fitting ensures that data that falls too much on the fringes to have any real impact is “regressed” onto a curve with a distinct formula that systems can use and interpret.Identifying Cross Sectional Data vs Time Series DataThe opposite of time series data is cross-sectional data. This is when various entities such as individuals and organizations are observed at a single point in time to draw inferences. Both forms of data analysis have their own value, and sometimes businesses use both forms of analysis to draw better conclusions.Time series data can be found in nearly every area of business and organizational application affected by the past. This ranges from economics, social sciences, and anthropology to climate change, business, finance, operations, and even epidemiology. In a timeseries, time is often the independent variable, and the goal is to make a forecast for the future.The most prominent advantage of time series analysis is that—because data points in a time series are collected in a linear manner at adjacent time periods—it can potentially make correlations between observations. This feature sets time series data apart from cross-sectional data.Time Series Analysis TechniquesAs we have seen above, time series analysis can be an ambitious goal for organizations. In order to gain accurate results from model-fitting, one of several mathematical models may be used in time series analysis such as:•Box-Jenkins autoregressive integrated moving average (ARIMA) models•Box-Jenkins multivariate models•Holt-Winters exponential smoothingWhile the exact mathematical models are beyond the scope of this article, these are some specific applications of these models that are worth discussing here.The Box-Jenkins models of both the ARIMA and multivariate varieties use the past behavior of a variable to decide which model is best to analyze it. The assumption is that any time series data for analysis can be characterized by a linear function of its past values, past errors, or both. When the model was first developed, the data used was from a gas furnace and its variable behavior over time.In contrast, the Holt-Winters exponential smoothing model is best suited to analyzing time series data that exhibits a defining trend and varies by seasons.Such mathematical models are a combination of several methods of measurement; the Holt-Winters method uses weighted averages which can seem simple enough, but these values are layered on the equations for exponential smoothing.Applications of Time Series AnalysisTime series analysis models yield two outcomes:•Obtain an understanding of the underlying forces and structure that produced the observed data patterns. Complex, real-world scenarios very rarely fall into set patterns, and time series analysis allows for their study—along with all of their variables as observed over time. This application is usually meant to understand processes that happen gradually and over a period of time such as the impact of climate change on the rise of infection rates.•Fit a mathematical model as accurately as possible so the process can move into forecasting, monitoring, or even certain feedback loops. This is a use-case for businesses that look to operate at scale and need all the input they can get to succeed.While the data is numerical and the analysis process seems mathematical, time series analysis can seem almost abstract. However, any organization can realize a number of present-day applications of such methods. For example, it is interesting to imagine that large, global supply chains such as those of Amazon are only kept afloat due to the interpretation of such complex data across various time periods. Even during the COVID-19 pandemic where supply chains suffered maximum damage, the fact that they have been able to bounce back faster is thanks to the numbers, and the comprehension of these numbers, that continues to happen throughout each day and week.Time series analysis is used to determine the best model that can be used to forecast business metrics. For instance, stock market price fluctuations, sales, turnover, and any other process that can use time series data to make predictions about the future. It enables management to understand time-dependent patterns in data and analyze trends in business metrics.From a practical standpoint, time series analysis in organizations are mostly used for:•Economic forecasting•Sales forecasting•Utility studies•Budgetary analysis•Stock market analysis•Yield projections•Census analysis•Process and quality control•Inventory studies•Workload projectionsAdvantages of Time Series AnalysisData analysts have much to gain from time series analysis. From cleaning raw data, making sense of it, and uncovering patterns to help with projections much can be accomplished through the application of various time series models.Here are a few advantages of time series analysis:It Cleans Data and Removes Confounding FactorsData cleansing filters out noise, removes outliers, or applies various averages to gain a better overall perspective of data. It means zoning in on the signal by filtering out the noise. The process of time series analysis removes all the noise and allows businesses to truly get a clearer picture of what is happening day-to-day.Provides Understanding of DataThe models used in time series analysis do help to interpret the true meaning of the data in a data set, making life easier for data analysts. Autocorrelation patterns and seasonality measures can be applied to predict when a certain data point can be expected. Furthermore, stationarity measures can gain an estimate of the value of said data point.This means that businesses can look at data and see patterns across time and space, rather than a mass of figu res and numbers that aren’t meaningful to the core function of the organization.Forecasting DataTime series analysis can be the basis to forecast data. Time series analysis is inherently equipped to uncover patterns in data which form the base to predict future data points. It is this forecasting aspect of time series analysis that makes it extremely popular in the business area. Where most data analytics use past data to retroactively gain insights, time series analysis helps predict the future. It is this very edge that helps management make better business decisions. Disadvantages of Time Series AnalysisTime series analysis is not perfect. It can suffer from generalization from a single study where more data points and models were warranted. Human error could misidentify the correct data model, which can have a snowballing effect on the output.It could also be difficult to obtain the appropriate data points. A major point of difference between time-series analysis and most other statistical problems is that in a time series, observations are not always independent.For example, a single chance event may affect all later data points, and it is up to every data scientist to accurately gauge which of these events may have an impact on the analysis in question. Are there similarities in predictions that can make historical data useful?。
probabilistic transformer for time series analysis
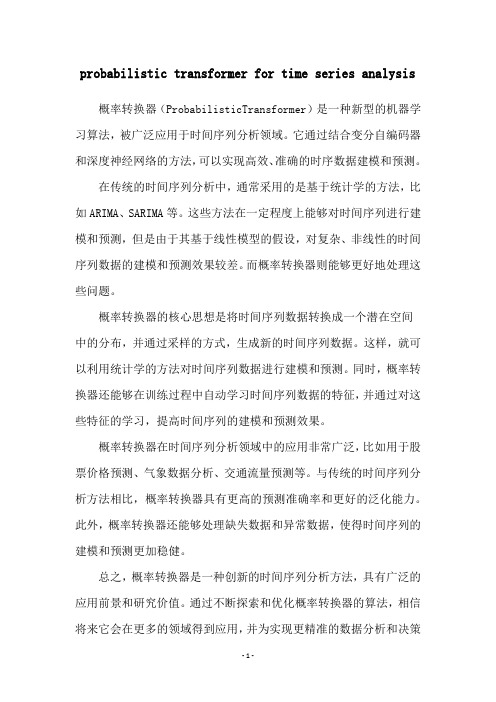
probabilistic transformer for time series analysis 概率转换器(ProbabilisticTransformer)是一种新型的机器学习算法,被广泛应用于时间序列分析领域。
它通过结合变分自编码器和深度神经网络的方法,可以实现高效、准确的时序数据建模和预测。
在传统的时间序列分析中,通常采用的是基于统计学的方法,比如ARIMA、SARIMA等。
这些方法在一定程度上能够对时间序列进行建模和预测,但是由于其基于线性模型的假设,对复杂、非线性的时间序列数据的建模和预测效果较差。
而概率转换器则能够更好地处理这些问题。
概率转换器的核心思想是将时间序列数据转换成一个潜在空间
中的分布,并通过采样的方式,生成新的时间序列数据。
这样,就可以利用统计学的方法对时间序列数据进行建模和预测。
同时,概率转换器还能够在训练过程中自动学习时间序列数据的特征,并通过对这些特征的学习,提高时间序列的建模和预测效果。
概率转换器在时间序列分析领域中的应用非常广泛,比如用于股票价格预测、气象数据分析、交通流量预测等。
与传统的时间序列分析方法相比,概率转换器具有更高的预测准确率和更好的泛化能力。
此外,概率转换器还能够处理缺失数据和异常数据,使得时间序列的建模和预测更加稳健。
总之,概率转换器是一种创新的时间序列分析方法,具有广泛的应用前景和研究价值。
通过不断探索和优化概率转换器的算法,相信将来它会在更多的领域得到应用,并为实现更精准的数据分析和决策
提供更好的技术支持。
多元统计分析04 时间序列分析

幂 指数 S曲线 Logistic 增长
常用曲线拟合模型
方程
线性化
Y=α+βt
Y=α+βlnt Y=α+β/t
Y=α+β×(1/t)
Y=α+β1t+β2t2 Y=α+β1t+β2t2+β3t3 Y=αtβ
lnY=lnα+βlnt
Y=αβt Y=e(α+β/t) Y=1/((1/M)+αβt)
lnY=lnα+tlnβ lnY=α+β×(1/t) ln(Y-M)=lnα+tlnβ
则销量y11的区间预测为:
y11
t
2
s p(11)
y 11
y11
t
2
s
(11)
p
2949 2.380 y 2949 2.380 11
(5)检验预测有效性
预测有效性及模型可靠性的检验,要 求比较预测值 yT k 与支持区间 外的预测区间[T+1,T+k]中的实际值。
Y t
按β 值不同,模型有不同的形状
当β >1时,递增上升 当0<β <1时,递减下降
Y R 1600
t
(
2 0.971)
幂函数 与指数函数一样灵活,但包含三个未
知参数:
Y t u
当γ >1,递增上升;0<γ <1,递减上 升;γ =0.5,为平方根模型
模型 估计 R2
F
函数
11期 11期预 预测值 测误差
线性 平方根 对数 指数 幂
- 1、下载文档前请自行甄别文档内容的完整性,平台不提供额外的编辑、内容补充、找答案等附加服务。
- 2、"仅部分预览"的文档,不可在线预览部分如存在完整性等问题,可反馈申请退款(可完整预览的文档不适用该条件!)。
- 3、如文档侵犯您的权益,请联系客服反馈,我们会尽快为您处理(人工客服工作时间:9:00-18:30)。
Time Series Analysis
ACCA P3考试:Time Series Analysis
1. Uses
The use of time series models is twofold:
To obtain an understanding of the underlying forces and structure that produced the observed data point;
To fit a model and proceed to forecasting, monitoring or even feedback and feed-forward control.
Time series analysis is used for many applications, such as:
Economic forecasting;Stock market analysis;
Sales forecasting;Process and quality control;
Budgetary analysis;Inventory studies and workload projections.
2. Components
Most time series patterns can be described in terms of two basic components:trend and seasonal variation (“seasonality”).
Trend describes a direction of change in the data that tends to occur at a similar rate over the short run. Within a long-term trend, data may change at varying rates or even reverse direction for short periods before continuing at the trend rate. After introducing a new product, for example, a firm may see sales grow slowly, followed by exponential growth as the new product catches on.
Seasonality describes calendar-related effects such as sales preceding certain holidays, air miles flown during vacation seasons, etc.
Cyclicality is another component. It arises when data plots in a repeating pattern around the trend line over a period lasting more than one year (e.g. economic expansion and contraction). Business cycles are notoriously difficult to forecast, so they are often combined with trend effects into “trend-cycle”analysis.
Trend and seasonality components may coexist in real-life data. For example, sales of a company can grow over years but still follow consistent seasonal patterns (e.g. 25%of sales each year are made in December and only 4%in August). In many cases it is necessary to establish a trend for a series and then adjust each new data point for seasonality. This may be done monthly, quarterly or semi-annually (depending on the review period over which management will compare actual results to forecast). Various methods exist for removing seasonal and cyclical noise from data and to make forecasts:
Random walk:Next period's prediction is based on the latest actual. Because the data move up and down due to non-trend factors, however, this method may place too much emphasis on the latest actual result.
Simple moving average:Next period's prediction is based on the latest moving average of n values for the series.
Weighted moving average:* Weights are assigned to observations, such that more recent results may be given more weight than older results.
This still suffers from other problems with the simple moving average method, but can be another improvement over the random walk.
It may be better to select equal weights for highly variable series.
Exponential smoothing:Weights are assigned to last period's actual result using the “smoothing constant”and to last period's forecast amount (1 minus the smoothing constant). Because the forecast value for the current period is the weighted actual value plus weighted forecast value of the prior period, this method implicitly gives weight to all actual values in determining the next period forecast.。