A Time-Recursive Interpolation Filter for Motion Compensated Prediction Considering Aliasin
国际自动化与计算杂志.英文版.
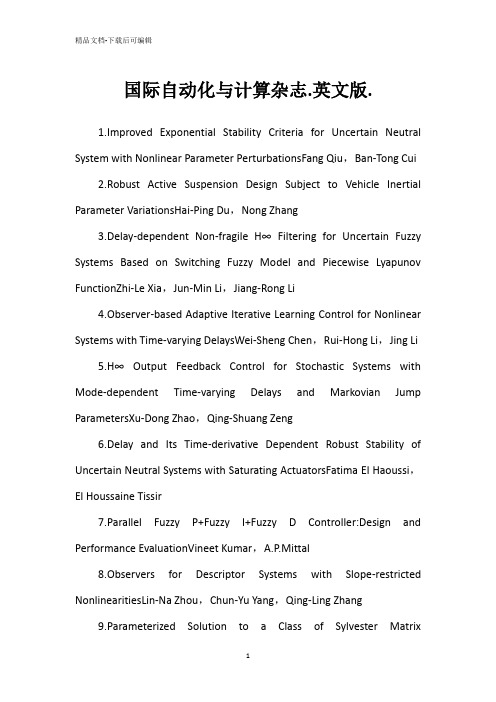
国际自动化与计算杂志.英文版.1.Improved Exponential Stability Criteria for Uncertain Neutral System with Nonlinear Parameter PerturbationsFang Qiu,Ban-Tong Cui2.Robust Active Suspension Design Subject to Vehicle Inertial Parameter VariationsHai-Ping Du,Nong Zhang3.Delay-dependent Non-fragile H∞ Filtering for Uncertain Fuzzy Systems Based on Switching Fuzzy Model and Piecewise Lyapunov FunctionZhi-Le Xia,Jun-Min Li,Jiang-Rong Li4.Observer-based Adaptive Iterative Learning Control for Nonlinear Systems with Time-varying DelaysWei-Sheng Chen,Rui-Hong Li,Jing Li5.H∞ Output Feedback Control for Stochastic Systems with Mode-dependent Time-varying Delays and Markovian Jump ParametersXu-Dong Zhao,Qing-Shuang Zeng6.Delay and Its Time-derivative Dependent Robust Stability of Uncertain Neutral Systems with Saturating ActuatorsFatima El Haoussi,El Houssaine Tissir7.Parallel Fuzzy P+Fuzzy I+Fuzzy D Controller:Design and Performance EvaluationVineet Kumar,A.P.Mittal8.Observers for Descriptor Systems with Slope-restricted NonlinearitiesLin-Na Zhou,Chun-Yu Yang,Qing-Ling Zhang9.Parameterized Solution to a Class of Sylvester MatrixEquationsYu-Peng Qiao,Hong-Sheng Qi,Dai-Zhan Cheng10.Indirect Adaptive Fuzzy and Impulsive Control of Nonlinear SystemsHai-Bo Jiang11.Robust Fuzzy Tracking Control for Nonlinear Networked Control Systems with Integral Quadratic ConstraintsZhi-Sheng Chen,Yong He,Min Wu12.A Power-and Coverage-aware Clustering Scheme for Wireless Sensor NetworksLiang Xue,Xin-Ping Guan,Zhi-Xin Liu,Qing-Chao Zheng13.Guaranteed Cost Active Fault-tolerant Control of Networked Control System with Packet Dropout and Transmission DelayXiao-Yuan Luo,Mei-Jie Shang,Cai-Lian Chen,Xin-Ping Guanparison of Two Novel MRAS Based Strategies for Identifying Parameters in Permanent Magnet Synchronous MotorsKan Liu,Qiao Zhang,Zi-Qiang Zhu,Jing Zhang,An-Wen Shen,Paul Stewart15.Modeling and Analysis of Scheduling for Distributed Real-time Embedded SystemsHai-Tao Zhang,Gui-Fang Wu16.Passive Steganalysis Based on Higher Order Image Statistics of Curvelet TransformS.Geetha,Siva S.Sivatha Sindhu,N.Kamaraj17.Movement Invariants-based Algorithm for Medical Image Tilt CorrectionMei-Sen Pan,Jing-Tian Tang,Xiao-Li Yang18.Target Tracking and Obstacle Avoidance for Multi-agent SystemsJing Yan,Xin-Ping Guan,Fu-Xiao Tan19.Automatic Generation of Optimally Rigid Formations Using Decentralized MethodsRui Ren,Yu-Yan Zhang,Xiao-Yuan Luo,Shao-Bao Li20.Semi-blind Adaptive Beamforming for High-throughput Quadrature Amplitude Modulation SystemsSheng Chen,Wang Yao,Lajos Hanzo21.Throughput Analysis of IEEE 802.11 Multirate WLANs with Collision Aware Rate Adaptation AlgorithmDhanasekaran Senthilkumar,A. Krishnan22.Innovative Product Design Based on Customer Requirement Weight Calculation ModelChen-Guang Guo,Yong-Xian Liu,Shou-Ming Hou,Wei Wang23.A Service Composition Approach Based on Sequence Mining for Migrating E-learning Legacy System to SOAZhuo Zhang,Dong-Dai Zhou,Hong-Ji Yang,Shao-Chun Zhong24.Modeling of Agile Intelligent Manufacturing-oriented Production Scheduling SystemZhong-Qi Sheng,Chang-Ping Tang,Ci-Xing Lv25.Estimation of Reliability and Cost Relationship for Architecture-based SoftwareHui Guan,Wei-Ru Chen,Ning Huang,Hong-Ji Yang1.A Computer-aided Design System for Framed-mould in Autoclave ProcessingTian-Guo Jin,Feng-Yang Bi2.Wear State Recognition of Drills Based on K-means Cluster and Radial Basis Function Neural NetworkXu Yang3.The Knee Joint Design and Control of Above-knee Intelligent Bionic Leg Based on Magneto-rheological DamperHua-Long Xie,Ze-Zhong Liang,Fei Li,Li-Xin Guo4.Modeling of Pneumatic Muscle with Shape Memory Alloy and Braided SleeveBin-Rui Wang,Ying-Lian Jin,Dong Wei5.Extended Object Model for Product Configuration DesignZhi-Wei Xu,Ze-Zhong Liang,Zhong-Qi Sheng6.Analysis of Sheet Metal Extrusion Process Using Finite Element MethodXin-Cun Zhuang,Hua Xiang,Zhen Zhao7.Implementation of Enterprises' Interoperation Based on OntologyXiao-Feng Di,Yu-Shun Fan8.Path Planning Approach in Unknown EnvironmentTing-Kai Wang,Quan Dang,Pei-Yuan Pan9.Sliding Mode Variable Structure Control for Visual Servoing SystemFei Li,Hua-Long Xie10.Correlation of Direct Piezoelectric Effect on EAPap under Ambient FactorsLi-Jie Zhao,Chang-Ping Tang,Peng Gong11.XML-based Data Processing in Network Supported Collaborative DesignQi Wang,Zhong-Wei Ren,Zhong-Feng Guo12.Production Management Modelling Based on MASLi He,Zheng-Hao Wang,Ke-Long Zhang13.Experimental Tests of Autonomous Ground Vehicles with PreviewCunjia Liu,Wen-Hua Chen,John Andrews14.Modelling and Remote Control of an ExcavatorYang Liu,Mohammad Shahidul Hasan,Hong-Nian Yu15.TOPSIS with Belief Structure for Group Belief Multiple Criteria Decision MakingJiang Jiang,Ying-Wu Chen,Da-Wei Tang,Yu-Wang Chen16.Video Analysis Based on Volumetric Event DetectionJing Wang,Zhi-Jie Xu17.Improving Decision Tree Performance by Exception HandlingAppavu Alias Balamurugan Subramanian,S.Pramala,B.Rajalakshmi,Ramasamy Rajaram18.Robustness Analysis of Discrete-time Indirect Model Reference Adaptive Control with Normalized Adaptive LawsQing-Zheng Gao,Xue-Jun Xie19.A Novel Lifecycle Model for Web-based Application Development in Small and Medium EnterprisesWei Huang,Ru Li,Carsten Maple,Hong-Ji Yang,David Foskett,Vince Cleaver20.Design of a Two-dimensional Recursive Filter Using the Bees AlgorithmD. T. Pham,Ebubekir Ko(c)21.Designing Genetic Regulatory Networks Using Fuzzy Petri Nets ApproachRaed I. Hamed,Syed I. Ahson,Rafat Parveen1.State of the Art and Emerging Trends in Operations and Maintenance of Offshore Oil and Gas Production Facilities: Some Experiences and ObservationsJayantha P.Liyanage2.Statistical Safety Analysis of Maintenance Management Process of Excavator UnitsLjubisa Papic,Milorad Pantelic,Joseph Aronov,Ajit Kumar Verma3.Improving Energy and Power Efficiency Using NComputing and Approaches for Predicting Reliability of Complex Computing SystemsHoang Pham,Hoang Pham Jr.4.Running Temperature and Mechanical Stability of Grease as Maintenance Parameters of Railway BearingsJan Lundberg,Aditya Parida,Peter S(o)derholm5.Subsea Maintenance Service Delivery: Mapping Factors Influencing Scheduled Service DurationEfosa Emmanuel Uyiomendo,Tore Markeset6.A Systemic Approach to Integrated E-maintenance of Large Engineering PlantsAjit Kumar Verma,A.Srividya,P.G.Ramesh7.Authentication and Access Control in RFID Based Logistics-customs Clearance Service PlatformHui-Fang Deng,Wen Deng,Han Li,Hong-Ji Yang8.Evolutionary Trajectory Planning for an Industrial RobotR.Saravanan,S.Ramabalan,C.Balamurugan,A.Subash9.Improved Exponential Stability Criteria for Recurrent Neural Networks with Time-varying Discrete and Distributed DelaysYuan-Yuan Wu,Tao Li,Yu-Qiang Wu10.An Improved Approach to Delay-dependent Robust Stabilization for Uncertain Singular Time-delay SystemsXin Sun,Qing-Ling Zhang,Chun-Yu Yang,Zhan Su,Yong-Yun Shao11.Robust Stability of Nonlinear Plants with a Non-symmetric Prandtl-Ishlinskii Hysteresis ModelChang-An Jiang,Ming-Cong Deng,Akira Inoue12.Stability Analysis of Discrete-time Systems with Additive Time-varying DelaysXian-Ming Tang,Jin-Shou Yu13.Delay-dependent Stability Analysis for Markovian Jump Systems with Interval Time-varying-delaysXu-Dong Zhao,Qing-Shuang Zeng14.H∞ Synchronization of Chaotic Systems via Delayed Feedback ControlLi Sheng,Hui-Zhong Yang15.Adaptive Fuzzy Observer Backstepping Control for a Class of Uncertain Nonlinear Systems with Unknown Time-delayShao-Cheng Tong,Ning Sheng16.Simulation-based Optimal Design of α-β-γ-δ FilterChun-Mu Wu,Paul P.Lin,Zhen-Yu Han,Shu-Rong Li17.Independent Cycle Time Assignment for Min-max SystemsWen-De Chen,Yue-Gang Tao,Hong-Nian Yu1.An Assessment Tool for Land Reuse with Artificial Intelligence MethodDieter D. Genske,Dongbin Huang,Ariane Ruff2.Interpolation of Images Using Discrete Wavelet Transform to Simulate Image Resizing as in Human VisionRohini S. Asamwar,Kishor M. Bhurchandi,Abhay S. Gandhi3.Watermarking of Digital Images in Frequency DomainSami E. I. Baba,Lala Z. Krikor,Thawar Arif,Zyad Shaaban4.An Effective Image Retrieval Mechanism Using Family-based Spatial Consistency Filtration with Object RegionJing Sun,Ying-Jie Xing5.Robust Object Tracking under Appearance Change ConditionsQi-Cong Wang,Yuan-Hao Gong,Chen-Hui Yang,Cui-Hua Li6.A Visual Attention Model for Robot Object TrackingJin-Kui Chu,Rong-Hua Li,Qing-Ying Li,Hong-Qing Wang7.SVM-based Identification and Un-calibrated Visual Servoing for Micro-manipulationXin-Han Huang,Xiang-Jin Zeng,Min Wang8.Action Control of Soccer Robots Based on Simulated Human IntelligenceTie-Jun Li,Gui-Qiang Chen,Gui-Fang Shao9.Emotional Gait Generation for a Humanoid RobotLun Xie,Zhi-Liang Wang,Wei Wang,Guo-Chen Yu10.Cultural Algorithm for Minimization of Binary Decision Diagram and Its Application in Crosstalk Fault DetectionZhong-Liang Pan,Ling Chen,Guang-Zhao Zhang11.A Novel Fuzzy Direct Torque Control System for Three-level Inverter-fed Induction MachineShu-Xi Liu,Ming-Yu Wang,Yu-Guang Chen,Shan Li12.Statistic Learning-based Defect Detection for Twill FabricsLi-Wei Han,De Xu13.Nonsaturation Throughput Enhancement of IEEE 802.11b Distributed Coordination Function for Heterogeneous Traffic under Noisy EnvironmentDhanasekaran Senthilkumar,A. Krishnan14.Structure and Dynamics of Artificial Regulatory Networks Evolved by Segmental Duplication and Divergence ModelXiang-Hong Lin,Tian-Wen Zhang15.Random Fuzzy Chance-constrained Programming Based on Adaptive Chaos Quantum Honey Bee Algorithm and Robustness AnalysisHan Xue,Xun Li,Hong-Xu Ma16.A Bit-level Text Compression Scheme Based on the ACW AlgorithmHussein A1-Bahadili,Shakir M. Hussain17.A Note on an Economic Lot-sizing Problem with Perishable Inventory and Economies of Scale Costs:Approximation Solutions and Worst Case AnalysisQing-Guo Bai,Yu-Zhong Zhang,Guang-Long Dong1.Virtual Reality: A State-of-the-Art SurveyNing-Ning Zhou,Yu-Long Deng2.Real-time Virtual Environment Signal Extraction and DenoisingUsing Programmable Graphics HardwareYang Su,Zhi-Jie Xu,Xiang-Qian Jiang3.Effective Virtual Reality Based Building Navigation Using Dynamic Loading and Path OptimizationQing-Jin Peng,Xiu-Mei Kang,Ting-Ting Zhao4.The Skin Deformation of a 3D Virtual HumanXiao-Jing Zhou,Zheng-Xu Zhao5.Technology for Simulating Crowd Evacuation BehaviorsWen-Hu Qin,Guo-Hui Su,Xiao-Na Li6.Research on Modelling Digital Paper-cut PreservationXiao-Fen Wang,Ying-Rui Liu,Wen-Sheng Zhang7.On Problems of Multicomponent System Maintenance ModellingTomasz Nowakowski,Sylwia Werbinka8.Soft Sensing Modelling Based on Optimal Selection of Secondary Variables and Its ApplicationQi Li,Cheng Shao9.Adaptive Fuzzy Dynamic Surface Control for Uncertain Nonlinear SystemsXiao-Yuan Luo,Zhi-Hao Zhu,Xin-Ping Guan10.Output Feedback for Stochastic Nonlinear Systems with Unmeasurable Inverse DynamicsXin Yu,Na Duan11.Kalman Filtering with Partial Markovian Packet LossesBao-Feng Wang,Ge Guo12.A Modified Projection Method for Linear FeasibilityProblemsYi-Ju Wang,Hong-Yu Zhang13.A Neuro-genetic Based Short-term Forecasting Framework for Network Intrusion Prediction SystemSiva S. Sivatha Sindhu,S. Geetha,M. Marikannan,A. Kannan14.New Delay-dependent Global Asymptotic Stability Condition for Hopfield Neural Networks with Time-varying DelaysGuang-Deng Zong,Jia Liu hHTTp://15.Crosscumulants Based Approaches for the Structure Identification of Volterra ModelsHouda Mathlouthi,Kamel Abederrahim,Faouzi Msahli,Gerard Favier1.Coalition Formation in Weighted Simple-majority Games under Proportional Payoff Allocation RulesZhi-Gang Cao,Xiao-Guang Yang2.Stability Analysis for Recurrent Neural Networks with Time-varying DelayYuan-Yuan Wu,Yu-Qiang Wu3.A New Type of Solution Method for the Generalized Linear Complementarity Problem over a Polyhedral ConeHong-Chun Sun,Yan-Liang Dong4.An Improved Control Algorithm for High-order Nonlinear Systems with Unmodelled DynamicsNa Duan,Fu-Nian Hu,Xin Yu5.Controller Design of High Order Nonholonomic System with Nonlinear DriftsXiu-Yun Zheng,Yu-Qiang Wu6.Directional Filter for SAR Images Based on NonsubsampledContourlet Transform and Immune Clonal SelectionXiao-Hui Yang,Li-Cheng Jiao,Deng-Feng Li7.Text Extraction and Enhancement of Binary Images Using Cellular AutomataG. Sahoo,Tapas Kumar,B.L. Rains,C.M. Bhatia8.GH2 Control for Uncertain Discrete-time-delay Fuzzy Systems Based on a Switching Fuzzy Model and Piecewise Lyapunov FunctionZhi-Le Xia,Jun-Min Li9.A New Energy Optimal Control Scheme for a Separately Excited DC Motor Based Incremental Motion DriveMilan A.Sheta,Vivek Agarwal,Paluri S.V.Nataraj10.Nonlinear Backstepping Ship Course ControllerAnna Witkowska,Roman Smierzchalski11.A New Method of Embedded Fourth Order with Four Stages to Study Raster CNN SimulationR. Ponalagusamy,S. Senthilkumar12.A Minimum-energy Path-preserving Topology Control Algorithm for Wireless Sensor NetworksJin-Zhao Lin,Xian Zhou,Yun Li13.Synchronization and Exponential Estimates of Complex Networks with Mixed Time-varying Coupling DelaysYang Dai,YunZe Cai,Xiao-Ming Xu14.Step-coordination Algorithm of Traffic Control Based on Multi-agent SystemHai-Tao Zhang,Fang Yu,Wen Li15.A Research of the Employment Problem on Common Job-seekersand GraduatesBai-Da Qu。
经典kalman滤波PPT经典实用
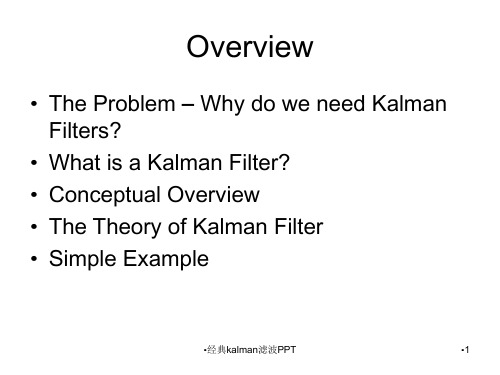
•经典kalman滤波PPT
•7
Conceptual Overview
prediction ŷ-(t2)
0.16 0.14 0.12
corrected optimal estimate ŷ(t2)
0.1
0.08
measurement
z(t2)
0.06
0.04
0.02
0 0 10 20 30 40 50 60 70 80 90 100
•11
Conceptual Overview
0.16
0.14
Corrected optimal estimate ŷ(t3)
0.120.1来自Measurement z(t3)
0.08
0.06
Prediction ŷ-(t3)
0.04
0.02
0 0 10 20 30 40 50 60 70 80 90 100
Blending Factor
• If we are sure about measurements:
– Measurement error covariance (R) decreases to zero – K decreases and weights residual more heavily than prediction
Measurement Error Sources
• System state cannot be measured directly
• Need to estimate “optimally” from measurements
•经典kalman滤波PPT
•2
What is a Kalman Filter?
基于bem的快时变信道估计算法研究
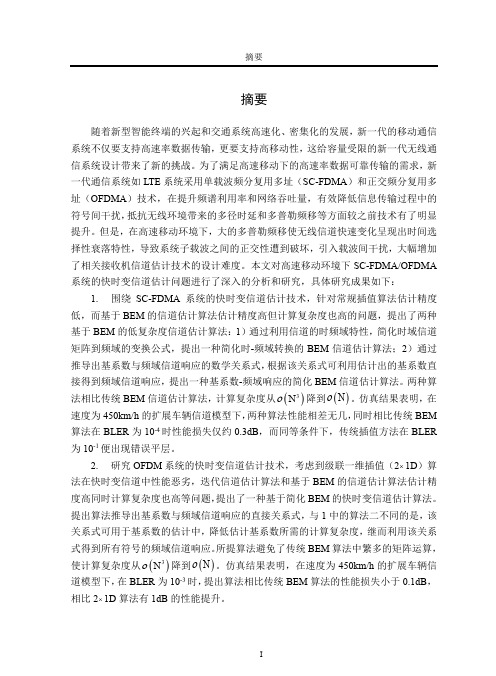
摘要摘要随着新型智能终端的兴起和交通系统高速化、密集化的发展,新一代的移动通信系统不仅要支持高速率数据传输,更要支持高移动性,这给容量受限的新一代无线通信系统设计带来了新的挑战。
为了满足高速移动下的高速率数据可靠传输的需求,新一代通信系统如LTE 系统采用单载波频分复用多址(SC-FDMA )和正交频分复用多址(OFDMA )技术,在提升频谱利用率和网络吞吐量,有效降低信息传输过程中的符号间干扰,抵抗无线环境带来的多径时延和多普勒频移等方面较之前技术有了明显提升。
但是,在高速移动环境下,大的多普勒频移使无线信道快速变化呈现出时间选择性衰落特性,导致系统子载波之间的正交性遭到破坏,引入载波间干扰,大幅增加了相关接收机信道估计技术的设计难度。
本文对高速移动环境下SC-FDMA/OFDMA 系统的快时变信道估计问题进行了深入的分析和研究,具体研究成果如下:1. 围绕SC-FDMA 系统的快时变信道估计技术,针对常规插值算法估计精度低,而基于BEM 的信道估计算法估计精度高但计算复杂度也高的问题,提出了两种基于BEM 的低复杂度信道估计算法:1)通过利用信道的时频域特性,简化时域信道矩阵到频域的变换公式,提出一种简化时-频域转换的BEM 信道估计算法;2)通过推导出基系数与频域信道响应的数学关系式,根据该关系式可利用估计出的基系数直接得到频域信道响应,提出一种基系数-频域响应的简化BEM 信道估计算法。
两种算法相比传统BEM 信道估计算法,计算复杂度从()3N ο降到()N ο。
仿真结果表明,在速度为450km/h 的扩展车辆信道模型下,两种算法性能相差无几,同时相比传统BEM 算法在BLER 为10-4时性能损失仅约0.3dB ,而同等条件下,传统插值方法在BLER 为10-1便出现错误平层。
2. 研究OFDM 系统的快时变信道估计技术,考虑到级联一维插值(2⨯1D )算法在快时变信道中性能恶劣,迭代信道估计算法和基于BEM 的信道估计算法估计精度高同时计算复杂度也高等问题,提出了一种基于简化BEM 的快时变信道估计算法。
CIC滤波器学习笔记
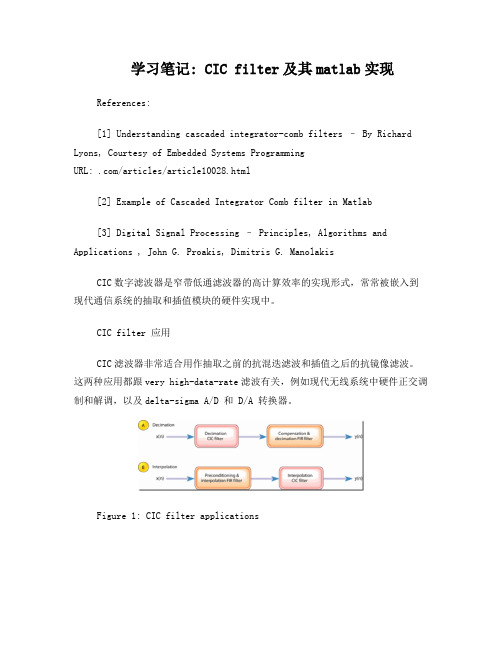
学习笔记: CIC filter及其matlab实现References:[1] Understanding cascaded integrator-comb filters – By Richard Lyons, Courtesy of Embedded Systems ProgrammingURL: .com/articles/article10028.html[2] Example of Cascaded Integrator Comb filter in Matlab[3] Digital Signal Processing – Principles, Algorithms and Applications , John G. Proakis, Dimitris G. ManolakisCIC数字滤波器是窄带低通滤波器的高计算效率的实现形式,常常被嵌入到现代通信系统的抽取和插值模块的硬件实现中。
CIC filter 应用CIC滤波器非常适合用作抽取之前的抗混迭滤波和插值之后的抗镜像滤波。
这两种应用都跟very high-data-rate滤波有关,例如现代无线系统中硬件正交调制和解调,以及delta-sigma A/D 和 D/A 转换器。
Figure 1: CIC filter applications因为CIC滤波器的幅频响应包络象sin(x)/x,通常在CIC滤波器之前或者之后都有一个high-performance linear-phase lowpass tapped-delay-line FIR filters, 用于补偿CIC滤波器不够平坦的通带。
CIC滤波器不需要乘法运算,易于硬件实现。
抽取CIC滤波器只不过是滑动平均滤波器的一个非常高效的迭代实现,有NR taps, 其输出再进行 R 抽取 . 同样,插值CIC滤波器在每两个输入采样之间插入R -1个0,然后通过一个NR -tap的工作在输出采样率?s ,out 的滑动平均滤波器。
连续微段样条曲线重构加工算法

连续微段样条曲线重构加工算法陶佳安;陈胜;黄宇亮;施群【摘要】针对复杂曲面在采用连续微段模式加工的过程中合成速度波动大导致加工效率降低的问题,提出了适用于微段加工的样条曲线重构新算法,该方法包含建立一种具有快速递推性质的样条曲线,及基于该曲线的速度规划和快速递推插补加工的方法。
实验表明,算法在保证加速度连续的条件下,通过样条重构及速度规划减少了频繁加减速,提高了加工效率;快速递推则提高了插补计算的速度,插补点精确通过微段节点,保证了加工精度,提升了数控系统的性能。
%In complex contour machining process with continuous micro-lines mode, machining efficiency was reduced by big fluctuation of composite velocity. To solve this problem, a curve reconstruction micro-line machining algo- rithm was presented. This algorithm included a new spline which had rapid recursive formula, and a method of ve- locity planning and recursive interpolation machining algorithm based on this spline. The experiment result showed the algorithm could reduce the situation of frequentacceleration/deceleration and improve the machining efficiency through spline reconstruction and velocity planning on the conditions that ensure continuity of acceleration in machi- ning process. Meantime, computational speed of interpolation was improved by fast recursion, and interpolation point could pass knot of micro line precisely, so machining accuracy was ensured and performance of CNC was promoted.【期刊名称】《计算机集成制造系统》【年(卷),期】2012(018)006【总页数】5页(P1195-1199)【关键词】样条曲线;微段;加减速;合成速度;插补;算法【作者】陶佳安;陈胜;黄宇亮;施群【作者单位】上海大学通信与信息工程学院,上海200072;上海大学机电工程与自动化学院,上海200072;上海大学机电工程与自动化学院,上海200072;上海大学机电工程与自动化学院,上海200072【正文语种】中文【中图分类】TG6590 引言复杂型面数控加工的计算机辅助制造(Computer Aided Manuf act uring,CA M)系统中用直线逼近曲线,生成大量微小直线段G代码,再经过系统解释执行控制轴运动。
WRF 3DVAR同化系统介绍
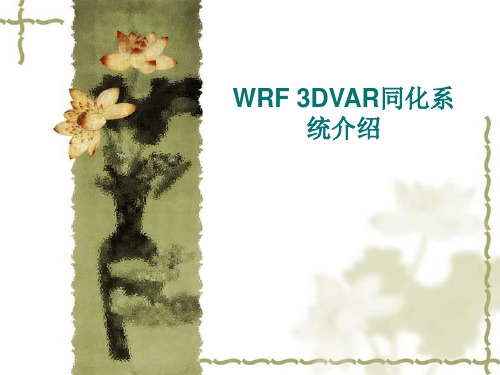
3DVAR同化系统主要部分 WRF 3DVAR同化系统主要部分
WRF 3DVAR :利用更多的描述大气真实状况的观测 资料信息,获得与预报模式更匹配的模式初值。 WRF同化模式:一个完全可压的非静力模式,网格采 同化模式: 用Arakawa C格点形式。模式的动力框架有不同的 方案可供选择。WRF模式中物理过程及参数化方案 也有多种选择。 模式后处理检验:将模式预报结果插值到标准等压面, 把WRF模式的NetCDF格式的结果转换成图变分同化系统
解压缩后,进入目录,./configure,选择configure var,然后compile var,生成wrfvar.exe.安装成 功. 运行很简单,在可执行脚本DA Run wrfVar.csh中修改然后运行即可.
WRFVAR_BC后处理
升级边界条件与新的初始场相协调,进入目录 后,make编译即可以生成update_wrf_bc.exe 把旧边界条件场文件wrfbdy.d01和初始场文 件放入同一目录,执行new_bc.csh即可.
背景场形成
采用WRF输入形式(netCDF格式) a) 来源于其它模式经过SI 标准初始化处理,形 成模式输入的netCDF形式(cold start) b) WRF模式本身输出(warm start)。
COLD START
WARM START
T213 预报场 WRF 预报场 SI 标准初始化 转换接口
WRF 3DVAR参数配置说明文件 WRF 3DVAR 的一些参数配置是通过 namelist.input和namelist.3dvar文件给入的。 这里包括定义分析的时间,格点维数的大小、 水平与垂直分辨率,观测资料类型的选取与 使用,背景误差系数的调整,变量尺度因子 的控制,极小化迭代次数的控制,控制变量 的选择等等。
数字信号处理英语词汇
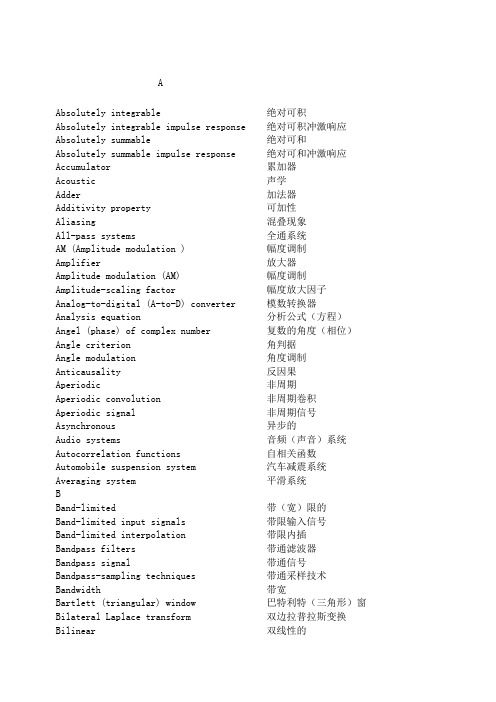
AAbsolutely integrable绝对可积Absolutely integrable impulse response绝对可积冲激响应Absolutely summable绝对可和Absolutely summable impulse response绝对可和冲激响应Accumulator累加器Acoustic 声学Adder加法器Additivity property可加性Aliasing混叠现象All-pass systems全通系统AM (Amplitude modulation )幅度调制Amplifier放大器Amplitude modulation (AM)幅度调制Amplitude-scaling factor幅度放大因子Analog-to-digital (A-to-D) converter模数转换器Analysis equation分析公式(方程)Angel (phase) of complex number复数的角度(相位)Angle criterion角判据Angle modulation角度调制Anticausality反因果Aperiodic非周期Aperiodic convolution非周期卷积Aperiodic signal非周期信号Asynchronous异步的Audio systems音频(声音)系统Autocorrelation functions自相关函数Automobile suspension system汽车减震系统Averaging system平滑系统BBand-limited带(宽)限的Band-limited input signals带限输入信号Band-limited interpolation带限内插Bandpass filters带通滤波器Bandpass signal带通信号Bandpass-sampling techniques带通采样技术Bandwidth带宽Bartlett (triangular) window巴特利特(三角形)窗Bilateral Laplace transform双边拉普拉斯变换Bilinear双线性的Bilinear transformation双线性变换Bit(二进制)位,比特Block diagrams方框图Bode plots波特图Bounded有界限的Break frequency折转频率Butterworth filters巴特沃斯滤波器C“Chirp” transform algorithm“鸟声”变换算法Capacitor电容器Carrier载波Carrier frequency载波频率Carrier signal载波信号Cartesian (rectangular) form 直角坐标形式Cascade (series) interconnection串联,级联Cascade-form串联形式Causal LTI system因果的线性时不变系统Channel信道,频道Channel equalization信道均衡Chopper amplifier斩波器放大器Closed-loop闭环Closed-loop poles闭环极点Closed-loop system闭环系统Closed-loop system function闭环系统函数Coefficient multiplier系数乘法器Coefficients系数Communications systems通信系统Commutative property交换性(交换律)Compensation for nonideal elements非理想元件的补偿Complex conjugate复数共轭Complex exponential carrier复指数载波Complex exponential signals复指数信号Complex exponential(s)复指数Complex numbers 复数Conditionally stable systems条件稳定系统Conjugate symmetry共轭对称Conjugation property共轭性质Continuous-time delay连续时间延迟Continuous-time filter连续时间滤波器Continuous-time Fourier series连续时间傅立叶级数Continuous-time Fourier transform连续时间傅立叶变换Continuous-time signals连续时间信号Continuous-time systems连续时间系统Continuous-to-discrete-time conversion连续时间到离散时间转换Convergence 收敛Convolution卷积Convolution integral卷积积分Convolution property卷积性质Convolution sum卷积和Correlation function相关函数Critically damped systems临界阻尼系统Crosss-correlation functions互相关函数Cutoff frequencies截至频率DDamped sinusoids阻尼正弦振荡Damping ratio阻尼系数Dc offset直流偏移Dc sequence直流序列Deadbeat feedback systems临界阻尼反馈系统Decibels (dB) 分贝Decimation抽取Decimation and interpolation抽取和内插Degenerative (negative) feedback负反馈Delay延迟Delay time延迟时间Demodulation解调Difference equations差分方程Differencing property差分性质Differential equations微分方程Differentiating filters微分滤波器Differentiation property微分性质Differentiator微分器Digital-to-analog (D-to-A) converter数模转换器Direct Form I realization直接I型实现Direct form II realization直接II型实现Direct-form直接型Dirichlet conditions狄里赫利条件Dirichlet, P.L.狄里赫利Discontinuities间断点,不连续Discrete-time filters 离散时间滤波器Discrete-time Fourier series离散时间傅立叶级数Discrete-time Fourier series pair离散时间傅立叶级数对Discrete-time Fourier transform (DFT)离散时间傅立叶变换Discrete-time LTI filters离散时间线性时不变滤波器Discrete-time modulation离散时间调制Discrete-time nonrecursive filters离散时间非递归滤波器Discrete-time signals离散时间信号Discrete-time systems离散时间系统Discrete-time to continuous-time conversion离散时间到连续时间转换Dispersion弥撒(现象)Distortion扭曲,失真Distribution theory(property)分配律Dominant time constant主时间常数Double-sideband modulation (DSB)双边带调制Downsampling减采样Duality对偶性EEcho回波Eigenfunctions特征函数Eigenvalue特征值Elliptic filters椭圆滤波器Encirclement property围线性质End points终点Energy of signals信号的能量Energy-density spectrum能量密度谱Envelope detector包络检波器Envelope function包络函数Equalization均衡化Equalizer circuits均衡器电路Equation for closed-loop poles闭环极点方程Euler, L.欧拉Euler’s relation欧拉关系(公式)Even signals偶信号Exponential signals指数信号Exponentials指数FFast Fourier transform (FFT)快速傅立叶变换Feedback反馈Feedback interconnection反馈联结Feedback path反馈路径Filter(s)滤波器Final-value theorem终值定理Finite impulse response (FIR)有限长脉冲响应Finite impulse response (FIR) filters有限长脉冲响应滤波器Finite sum formula有限项和公式Finite-duration signals有限长信号First difference一阶差分First harmonic components基波分量(一次谐波分量)First-order continuous-time systems一阶连续时间系统First-order discrete-time systems一阶离散时间系统First-order recursive discrete-time filters一阶递归离散时间滤波器First-order systems一阶系统Forced response受迫响应Forward path正向通路Fourier series傅立叶级数Fourier transform傅立叶变换Fourier transform pairs傅立叶变换对Fourier, Jean Baptiste Joseph傅立叶(法国数学家,物理学家)Frequency response频率响应Frequency response of LTI systems线性时不变系统的频率响应Frequency scaling of continuous-time Fourier transform 连续时间傅立叶变化的频率尺度(变换性质)Frequency shift keying (FSK)频移键控Frequency shifting property频移性质Frequency-division multiplexing (FDM)频分多路复用Frequency-domain characterization频域特征Frequency-selective filter频率选择滤波器Frequency-shaping filters频率成型滤波器Fundamental components基波分量Fundamental frequency基波频率Fundamental period基波周期GGain增益Gain and phase margin增益和相位裕度General complex exponentials一般复指数信号Generalized functions广义函数Gibbs phenomenon吉伯斯现象Group delay群延迟HHalf-sample delay半采样间隔时延Hanning window汉宁窗Harmonic analyzer谐波分析议Harmonic components谐波分量Harmonically related谐波关系Heat propagation and diffusion热传播和扩散现象Higher order holds高阶保持Highpass filter高通滤波器Highpass-to-lowpass transformations高通到低通变换Hilbert transform希尔波特滤波器Homogeneity (scaling) property齐次性(比例性)IIdeal理想的Ideal bandstop characteristic理想带阻特征Ideal frequency-selective filter理想频率选择滤波器Idealization理想化Identity system恒等系统Imaginary part虚部Impulse response 冲激响应Impulse train冲激串Incrementally linear systems增量线性系统Independent variable独立变量Infinite impulse response (IIR)无限长脉冲响应Infinite impulse response (IIR) filters无限长脉冲响应滤波器Infinite sum formula无限项和公式Infinite taylor series无限项泰勒级数Initial-value theorem初值定理Inpulse-train sampling冲激串采样Instantaneous瞬时的Instantaneous frequency瞬时频率Integration in time-domain时域积分Integration property积分性质Integrator积分器Interconnection互联Intermediate-frequency (IF) stage中频级Intersymbol interference (ISI)码间干扰Inverse Fourier transform傅立叶反变换Inverse Laplace transform拉普拉斯反变换Inverse LTI system逆线性时不变系统Inverse system design逆系统设计Inverse z-transform z反变换Inverted pendulum倒立摆Invertibility of LTI systems线性时不变系统的可逆性Invertible systems逆系统LLag network滞后网络Lagrange, J.L.拉格朗日(法国数学家,力学家)Laplace transform拉普拉斯变换Laplace, P.S. de拉普拉斯(法国天文学家,数学家)lead network超前网络left-half plane左半平面left-sided signal左边信号Linear线性Linear constant-coefficient difference线性常系数差分方程equationsLinear constant-coefficient differential线性常系数微分方程equationsLinear feedback systems线性反馈系统Linear interpolation线性插值Linearity线性性Log magnitude-phase diagram对数幅-相图Log-magnitude plots对数模图Lossless coding无损失码Lowpass filters低通滤波器Lowpass-to-highpass transformation低通到高通的转换LTI system response线性时不变系统响应LTI systems analysis线性时不变系统分析MMagnitude and phase幅度和相位Matched filter匹配滤波器Measuring devices测量仪器Memory记忆Memoryless systems无记忆系统Modulating signal调制信号Modulation调制Modulation index调制指数Modulation property调制性质Moving-average filters移动平均滤波器Multiplexing多路技术Multiplication property相乘性质Multiplicities多样性NNarrowband窄带Narrowband frequency modulation窄带频率调制Natural frequency自然响应频率Natural response自然响应Negative (degenerative) feedback负反馈Nonanticipatibe system不超前系统Noncausal averaging system非因果平滑系统Nonideal非理想的Nonideal filters非理想滤波器Nonmalized functions归一化函数Nonrecursive非递归Nonrecursive filters非递归滤波器Nonrecursive linear constant-coefficient非递归线性常系数差分方程difference equationsNyquist frequency奈奎斯特频率Nyquist rate奈奎斯特率Nyquist stability criterion奈奎斯特稳定性判据OOdd harmonic 奇次谐波Odd signal奇信号Open-loop开环Open-loop frequency response开环频率响应Open-loop system开环系统Operational amplifier运算放大器Orthogonal functions正交函数Orthogonal signals正交信号Oscilloscope示波器Overdamped system过阻尼系统Oversampling过采样Overshoot超量PParallel interconnection并联Parallel-form block diagrams并联型框图Parity check奇偶校验检查Parseval’s relation帕斯伐尔关系(定理)Partial-fraction expansion部分分式展开Particular and homogeneous solution特解和齐次解Passband通频带Passband edge通带边缘Passband frequency通带频率Passband ripple通带起伏(或波纹)Pendulum钟摆Percent modulation调制百分数Periodic周期的Periodic complex exponentials周期复指数Periodic convolution周期卷积Periodic signals周期信号Periodic square wave周期方波Periodic square-wave modulating signal周期方波调制信号Periodic train of impulses周期冲激串Phase (angle) of complex number复数相位(角度)Phase lag相位滞后Phase lead相位超前Phase margin相位裕度Phase shift相移Phase-reversal相位倒置Phase modulation相位调制Plant工厂Polar form极坐标形式Poles极点Pole-zero plot(s)零极点图Polynomials 多项式Positive (regenerative) feedback正(再生)反馈Power of signals信号功率Power-series expansion method幂级数展开的方法Principal-phase function主值相位函数Proportional (P) control比例控制Proportional feedback system比例反馈系统Proportional-plus-derivative比例加积分Proportional-plus-derivative feedback比例加积分反馈Proportional-plus-integral-plus-different比例-积分-微分控制ial (PID) controlPulse-amplitude modulation脉冲幅度调制Pulse-code modulation脉冲编码调制Pulse-train carrier冲激串载波QQuadrature distortion正交失真Quadrature multiplexing正交多路复用Quality of circuit电路品质(因数)RRaised consine frequency response升余弦频率响应Rational frequency responses有理型频率响应Rational transform有理变换RC highpass filter RC 高阶滤波器RC lowpass filter RC 低阶滤波器Real实数Real exponential signals实指数信号Real part实部Rectangular (Cartesian) form 直角(卡笛儿)坐标形式Rectangular pulse矩形脉冲Rectangular pulse signal矩形脉冲信号Rectangular window矩形窗口Recursive (infinite impulse response)递归(无时限脉冲响应)滤波器filtersRecursive linear constant-coefficient 递归的线性常系数差分方程difference equationsRegenerative (positive) feedback再生(正)反馈Region of comvergence收敛域right-sided signal右边信号Rise time上升时间Root-locus analysis根轨迹分析(方法)Running sum动求和SS domain S域Sampled-data feedback systems采样数据反馈系统Sampled-data systems采样数据系统Sampling采样Sampling frequency采样频率Sampling function采样函数Sampling oscilloscope采样示波器Sampling period采样周期Sampling theorem采样定理Scaling (homogeneity) property比例性(齐次性)性质Scaling in z domain z域尺度变换Scrambler扰频器Second harmonic components二次谐波分量Second-order二阶Second-order continuous-time system二阶连续时间系统Second-order discrete-time system二阶离散时间系统Second-order systems二阶系统sequence序列Series (cascade) interconnection级联(串联)Sifting property筛选性质Sinc functions sinc函数Single-sideband单边带Single-sideband sinusoidal amplitude单边带正弦幅度调制modulationSingularity functions奇异函数Sinusoidal正弦(信号)Sinusoidal amplitude modulation正弦幅度调制Sinusoidal carrier正弦载波Sinusoidal frequency modulation正弦频率调制Sliding滑动Spectral coefficient频谱系数Spectrum频谱Speech scrambler语音加密器S-plane S平面Square wave方波Stability稳定性Stabilization of unstable systems不稳定系统的稳定性(度)Step response阶跃响应Step-invariant transformation阶跃响应不定的变换Stopband阻带Stopband edge阻带边缘Stopband frequency阻带频率Stopband ripple 阻带起伏(或波纹)Stroboscopic effect频闪响应Summer加法器Superposition integral叠加积分Superposition property叠加性质Superposition sum叠加和Suspension system减震系统Symmetric periodic 周期对称Symmetry对称性Synchronous同步的Synthesis equation综合方程System function(s)系统方程TTable of properties 性质列表Taylor series泰勒级数Time时间,时域Time advance property of unilateral单边z变换的时间超前性质z-transformTime constants时间常数Time delay property of unilateral单边z变换的时间延迟性质z-transformTime expansion property时间扩展性质Time invariance时间变量Time reversal property时间反转(反褶)性Time scaling property时间尺度变换性Time shifting property时移性质Time window时间窗口Time-division multiplexing (TDM)时分复用Time-domain时域Time-domain properties时域性质Tracking system (s)跟踪系统Transfer function转移函数transform pairs变换对Transformation变换(变形)Transition band过渡带Transmodulation (transmultiplexing) 交叉调制Triangular (Barlett) window三角型(巴特利特)窗口Trigonometric series三角级数Two-sided signal双边信号Type l feedback system l 型反馈系统UUint impulse response单位冲激响应Uint ramp function单位斜坡函数Undamped natural frequency无阻尼自然相应Undamped system无阻尼系统Underdamped systems欠阻尼系统Undersampling欠采样Unilateral单边的Unilateral Laplace transform单边拉普拉斯变换Unilateral z-transform单边z变换Unit circle单位圆Unit delay单位延迟Unit doublets单位冲激偶Unit impulse单位冲激Unit step functions单位阶跃函数Unit step response 单位阶跃响应Unstable systems不稳定系统Unwrapped phase展开的相位特性Upsampling增采样VVariable变量WWalsh functions沃尔什函数Wave波形Wavelengths波长Weighted average加权平均Wideband宽带Wideband frequency modulation宽带频率调制Windowing加窗zZ domain z域Zero force equalizer置零均衡器Zero-Input response零输入响应Zero-Order hold零阶保持Zeros of Laplace transform拉普拉斯变换的零点Zero-state response零状态响应z-transform z变换z-transform pairs z变换对。
GENERALIZATION

GENERALIZATIONAnalysisinTheoryandApplications22:4,2006,301—318 GENERALIZTIONOFTHEINTERACTION BETWEENHAARAPPROXIMIONANDPOINOMIALOPERORSTOHIGHERORDERMETHODSFrancoisChaplais(~coleNationaleSupdrieuredesMinesdePn,France)ReceivedJune1,2004Abstract/napplicationsitisusefultocomputethelocalaverageofafunctionI(4)ofaninput,r0m empiricalstatisticson.AverysimplerelationexistswhenthelocalaveragesaregivenbyaHaar approximation.Thequestionistoknow寸itholdsforhigherorderapproximationmethods.TodoSOl itisnecessarytouseapproximateproductoperatorsdnedoverlinearapproximationspaces.T heseproductsarecharacterizedbyaStrangandFixlikecondition.Anexplicitconstructionofthese productoperatorsisexhibitedlorpiecewisepolynomialnctions,usingHermiteinterpolation.Theave raging relationwhichholdsfortheHaarapproximationisthenrecoveredwhentheproductisdefined byatwopointHermiteinterpolationKeywordsStrangandFixconditions,productapproximation,Hermiteinterpolation,wavele tsAMS(2000)subjectclassification41A05,41A35,42C401IntroductionThetheoryofaveraging[】approximatesthesolutionsofadifferentialsystemsdx/dt=/(x,t,t/E),whereEisasmallparameter,bythesolutionofan"averaged"systemdx/dt=,(z,t). Inpractice,fisnotgivenasafunctionoftwotimescalestandt/E,butratherasafunction/(x,u)whereuisaninputwhichisafunctionoftime.Thequestionistogiveapracticalsense302.AnalysisinTheoryandApplications22:4,2006tothe"average"of/(x,u)asafunctionoftheinputu. Theanswerisrelativelysimplewhentheaveragingof,(andu)isobtainedbycomputingits averagesonasequenceofintervals【k6,(k+1)Inthispaper,weassumethat,isapolynomial function.IfEdenotestheaveragingoperator,itcanbeeasilyshownthatthefollowingidentity holds:00E())=∑k--0(1)wheree=E(u)andw=u—e.Wecanseethat,isexpandedaroundtheaverageofu,and thepowersofwarereplacedintheexpansionbytheiraverages.Ifoneinterpretstheaveraging operatorEasanempiricalexpectationjthen(1)showsthattheexpectationof/(u)iscomputed byusingthederivativesof,attheexpectationeofandthemomentsofthe"noise"w.While itiseasytoderive,equation(1)appearstobeneworatleastnotaclassic. Thispreviousmethodofaveragingisalsoanapproximationprocedurewhichisrelatedto theHaarmultiresolutionanalysis.Thereareothermethodsofapproximationwhicharemore efficientandyetgiveasenseoflocalaverage.Wavelets[2-4]providesuchmethods.Theorder of approximationarethengivenbytheclassicStrangandFixconditions[5】whichstatesthatthe orderofapproximationofsmoothfunctionsischaracterizedbytheabilityoftheapproximatio nmethodtoreproducepolynomialsuptoacertainorder.Thisconditionisrelatedtothenumber ofvanishingmomentsofthewavelet.Theapproximationofafunctionisobtainedbyprojectio nonaresolutionspace.whichisitselfgeneratedbythetranslatesofasocalledscalingfunction.Thepreviousaveragingisaparticularcaseofapproximationonaresolutionspacewherethe scalingfunctionisthecharacteristicfunctionofaninterva1.Thecorrespondingmultiresoluti onanalysisiscalledtheHaarsystem.Amongallwaveletmethods,ityieldsthelowestorderof approximation,sinceitsscalingfunctionreproducesonlypolynomialsofdegreezero. Thefollowingquestionthenarises:isapproximationmethods(waveletsornot)?itpossibletohavetherelation(1)forhigherorder Thiswillbethemainsubjectofthisarticle. Itturnsoutthatprojectorswhichsatisfyequation(1)canbesimplycharacterizedbytwo conditionsontheirimagespaceandtheirkernel:Proposition1.LetVbetheimagespaceoftheprojectorEandWtheimageofId—E Equation(1)isverifiedifandonlyif(P1)theproductoftwoelementsofVbelongstoV(P2)theproductofanelementofVwithanelementofWisanelementofW.—F.Chaplais:Gen—eralizationoftheInteraetiontoHigherOrderMethod;.303 Thesufficientpartisprovedbyexpanding1aroundeandapplyingE.Thenecessary Conditionisderivedfrom(1)using/(x)=.with=e+band=e—b,e∈V and successivelyb∈V andb∈W. Findingapproximationprojectorswhichsatisfyequation(1)isthusequivalenttosatisfying properties(P1)and(P2).Asweshallseeinthenextsection,property(P1)impliesthatthe functiox~whosetranslatesgeneratesVisthecharacteristicfunctionofaninterva1.Itappears thenthathighorderapproximationmethodscannotsatisfyproperty(P1). Thislastlimitationisduetotheuseoftheclassicalproductonfunctions.SinCeweare dealingwithapproximationsoffunctions,wecanreplacetheclassicalproductbyanapproxi mateproductwithoutdegradingtheorderofapproximationoftheresult. Ageneralcharacterizationofsuchapproximateproductsisgiveninsection2.2.2.LiketheStrangandFixconditions.itis basedonthereproductionofpolynomials. Thereremainstoconstructsuchapproximateproducts.Thedifficultyistoverifytheassociativityoftheproductoperator.Inthecasewherethefunctionsarepiecewisepolynomial ,wegiveinsection3.2aconstructivecharacterizationofapproximateproductsinthisfunction alspace.TheseproductsaredefinedbyHermiteinterpolation.Inthesimplecaseofatwo.point interpolation,weexhibitinsection3.4anapproximationprojectorwhichsatisfiesproperties( P1)and(P2),andthusequation(1).2ProductInvarianceandApproximation2.1TwoResultsOnProductInvarianceTheproperty(P1)statesthatthespaceVshouldbeproductinvariant.Weconsiderhere thecasewhereV=V6cL(R)hasaRieszbasis()∈zwith(t)=(£/一k)lv~6),and iscompactlysupported.Inthewaveletcontext,Visaresolutionspaceandisascaling function.Givenafunctione∈V,Ck(e)willdenoteitscoordinateonthevector.The followinggenerallemmacharacterizestheproductoperatorsforwhich(P1)holds: Lemma1.Let}bea(nonzero)productoperatorsuchthat(,+,幸)isacommutativering.Itisassumedthatcommuteswiththeshoflength,andthattheproductoftwo compactlysupported如nctionsiszerobeyondsome缸eddistance.ThenthereexistaconstantA≠0suchthat,foranyands『ln,andanyn∈,(Y)=A()Cn(s『)304Analysis讥Theoryand—Applic—ations22:4,2006I|istheusualproductonfunctionsIthentheintersectionoythesupportso|tandjisol. measurezero矿i≠J.ThislemmaisprovedinappendixA.LetUSapplyittothecasewhereanapproximation operatorisassociatedtothespaceV6:Theorem1.LetE6aprojectorondefinedbyarescaledkernel:岛(£)=1/nfg(t/,s/)(s)ds.WenssumethatE6isanapproximationoperator~e.g.,forany,∈L(R),E6f—filL(R)_+0when_+0isproductinvariantfortheusualproduct,thenisproportionaltothecharacteristic functionoyanintervaloylengthj.ProoLThankstotheprojector岛,thelinearformisextendedtoL2().Undermild assumptions,itcanberepresentedbyanL(R)function;onecaneasilyseethat(t)=(t/一七)/,//)with}compactlysupportedandthatK(t,s)(t)(s).TheStrangand Fixconditions[5】implythatE(1)isdefinedandisequalto1.Sincethe'Shavedi~oint supportandhavethesameshape,theymustbeproportionaltothecharacteristicfunctionofan intervaloflength1.Theorem1impliesthat,ifE6yieldsanorderofapproximationstrictlygreaterthanthe minimumprovidedbytheHaarbasis,thenV6cannotsatisfy(P1).Indeed,cannotbea piecewiseconstantfunctionanymore,andtheonlyassumptionthatmaybeinvalidinLemma 1withtheusualproductistheproductinvarianceofV.2.2CharacterizationofApproximateProducts(ageneralizationoftheStrang andFixConditions)2.2.1IntroductionWhile(2)doesnotholdforhigherorderapproximations,itisnotfarfrombeingverified; ifissmall,1/(t)isclosetoaDirac,andthesampleofaproductistheproductofthe samFles..Ingeneral,thisproductonlyyieldsafirstorderapproximation.Coiflets[2,61provided scalingfunctionswithahighorderapproximationwhicharegoodapproximationsofDiracs. Thissuggeststhatusingapproximateproductoperatorsmayrecovertheproductinvarianc eof V.Suchapproximateproductoperatorsarecharacterizedinthenextsection..F...........C.....h....a...p.....1..a....i..s......:....—G—————e———n—————e———r———a———l——i———z——a—t——ionoftheInteractiontoHigherOrderMethods.3052.2?2ThePolynomialConditionforProductApproximation AssumptionsLetbeaproductoperatoronVlsuchthat(Vl,+,)isacommutativering?ItisassumedthatthatcommuteswiththeshiftoflengthL,L∈Randthatiscontinuous andlocalizeda8follows:thereexists(K,)suchthatfgl(t)Ksupl,(s)1suplg(8)ls-tl<__t~Is一≠ITheproductoperatorisdefinedonV6byrescaling:f(t/5)9(t/)=(,9)(£/).Itis assumedthatsatisfiestheStrangandFixconditions【5.atorderN,e.g.E6(ti)=tiifiN. ThenTheorem2.Thefollowingtwoconditionsareequivalent ThereexistsKsuchthatforany,andgoyclassCⅣ+andany1,fg(£)一[((驯(£)1<__K~N+If)∈,Ⅳ1supl(s)l1whereINdenotesthesetoIintegers(k,f)∈N×Nsuchthat0kN+1,0fN+1andk+f≥N+1.t=ti+Jifi+歹N(5)TheproofofthistheoremisinappendixB.ObserveitssimilaritywiththeclassicalStrang andFixconditionsforlinearoperators[引.NotethatBeylkin[Jhasdesignedarecursivealgorithmtocomputethewalveletcoefficients ontheproductoftwofunctions.Itconvergesfastifthefunctionsareregular.Thecomputationo ftheexactproductinvolvesaninfinitenumberofcomputations;allapproximationiscomputed bystoppingtherecursionbeforetheinfinity.Theresultpresentedhereisdifferentinthesensethat itcharacterizesapproximateproductoperatorswhicharecompatiblewiththescalingoperati on.Insection3weeffectivelybuildsuchoperatorsforpiecewisepolynomialfunctions.3WorkingwithPiecewisePolynomialFunctions3.1IntroductionSincethemappingz—}a×zislinear.buildingaproductoperatorappearsatafirst glanceastheconstructionofacollectionoflinearoperators.Theextrarequirementthatthe operatorisassociativeshowsthatthingsaremorecomplicate;indeed,checkingthenszociativ ityisanonlinearproblem.ThiseliminatesFouriermethodsforfindingapproximateproducts.AnalysisinTheoryandApplications22:4.2006 Acasewhereapproximateproductsareeasytofindiswhenthecoordinateoftheapprox- imationofafunctionisasample(interpolatingscalingfunctions[S,91)oranapproximationof asample(Coiflets).Theapproximateproductisdefinedbyhavingthecoordinateoftheproduct tobetheproductofthecoordinates,asinLemma1.Thefunctionalspaceonwhichthegeneralproductisdefinedisaresolutionspaceatafinescale.Thereremainstodefineanapproximation subspacewhichisproductinvariant.Sincewearedealingwithmultiresolutionanalysis,then at—uralideaistoconsideracoarserresolutionspace.Ingeneral,itisnotproductinvariantunder theactionoftheproductdefinedonthefinescale.TakeforinstancetheSchauderbasis,where approximationsaredefinedbylinearapproximationsbetweensamples.Thescalingfunction isthehatfunction,whichistheautocorrelationoftheHaarfunction1[0.1).Thenthecoarserresolutionspacesarenotproductinvariant.Indeed,letusconsideracoarsescalingfunction, e.g.ascaledhatfunction;iftheresolutionspacewasproductinvariant,thesquareofthescaling functionwouldbealinearinterpolationoverthecoarsegrid.Thisisnotthecase,becausethe approximatesquareiscomputedbyperforminganinterpolationofthesquareoverthefinegrid ,andtheresultisalinearinterpolationoversamplesofaparabola.Itisnotalinearinterpolation overpointsofthecoarsegrid.So,eveninthissimplecase,thematteroffindingproductinvarian tapproximationsubspacesisnotobvious. Thenatureofapproximatingproductinvariantsubspacesislinkedtothechoiceofthe approximateproductoperator.Tobetterunderstandthenatureofthislink,wehavechosento restrictourattentiontothespecialcasewherefunctionsarepiecewisepolynomialones.Thisw ill allowustocharacterizetherelationshipbetweenthechoiceoftheoperatorandtheapproximat ionsubalgebras. Observethatwaveletscandetectsingularitiesinpiecewiseregularfunctions(providedthe singularitiesarenottooclose)andanalyzethem[10,2].Thenthesefunctionpiecescanbeappr ox—imatedbypiecewisepolynomialfunctions.Wecanthereforeassumetohaveanapproximatio nofpiecewiseregularfunctionsaspiecewisepolynomials. Wenowassumethatthefunctionhasbeenpreprocessedtoberepresentedasanequally spacedpiecewisepolynomialfunctionofdegreesmallerthansomeN∈N.Thestudyofconditions(P1)and(P2)willnotbearongeneralfunctionsbutonpiecewisepolynomialones. Thissectionisorganizedasfollows.Section3.2characterizestheapproximateproducton piecewisepolynomialfunctionsbyshowingthattheymustbedefinedbyaHermiteinterpolati on.Torecoverproperty(P1),section3.3studiestheapproximationsubspacesofpiecewisepolyn omial:!rnlizationoy舭InteractiontoHigherOrderMethods.301 functionswhichareproductinvariant.Inparticular,allofthesesubspacesincludeaminimal onewhichconsistsinSOcalledregularfunctions.Finally,section3.4studiesthecasewhereth e approximateproductisdefinedbyaHermiteinterpolationbetweentwopointsandexhibitsan approximationprojectorwhichsatisfiesproperties(P1)and(P2),andhenceequation(1). 3.2CharacterizationofApproximateProductsforPiecewisePolynomial Approximations3.2?1TheAlgebraicStrangandFixConditionsforPiecewisePolynomial ApproximationsDenotebySN,6(t)thespaceofpiecewisepolynomialfunctionsoversuccessiveintervalsof lengthandwithadegreesmallerorequaltoN.Tomaintainconsistencywiththelinear andnonlinearapproximationconditionspreviouslymentioned,weassumethereexistsaline arapproximationoperatorP6whichtransformsfunctionsintoelementsofSN,6(£).P6isdefin edbyscalingakernelKwhichisassumedtohavethestructure(t,s)=∑∑(t一)l[k,k+1)(t)(s一)(6)kEZl=0 andtosatisfytheassumptionsoftheresultofStrangandFixwiththeorderN(twosuch operatorsarepresentedin[11]).Theorem2istransposedtoSN.6(t)andrelatesittoanalgebraic condition:Proposition2.DenotebyR|Ⅳ[叫thespaceofrealorequaltoN,andbyF6theoperator[F6p](t)=∑知∈ZpolynomialsequencesinRⅣ[tofunctionsinSN,(t).istransposedtoanoperator牛onfunctionsinSN,6(t) valuedpolynomialswithdegreesmallerPk(t/a—k)l[k,+1)(t/)whichidentifiesIf×isaproductoperatoroverR|Ⅳitasfollows:(,牛6g)(t)F6((F×(Fg))Then牛6approximatestheproductontheimageoflikeincondition(4)ofTheorem2ifand onlyif×satisfiest×tj=ti+J∈R|ⅣIt]ifi+J≤N.Thisresultisprovedin[11]Inparticular,itisdefinedbyashiftinvariantscaledkernel(8)308?AnalysisTheoryandApplica—tio—ns22:4,2006Comment.ThedegreeoftheproductisrestrictedtobesmallerorequaltoNinorder tohaveSⅣ,(t)productinvaxiant.This,inturns,makessurethatthecomplexityofthefunction representationdoesnotincreasewitheveryproductoperation.ItalsomakessensenumericallsincetheelementsofSN,6(£)areatbestapproximationsofregularfunctionsattheorderN, thereisnoneedforagreaterprecisiononthecomputationoftheproduct.3.2.2DescriptionofaUApproximateProductsProposition2reducesthesearchforapproximateproductsoverSN,6(t)toalgebraicproducts onⅣ[司whichsatisfy(8).Thesearecharacterizedinthefollowinglemma:Lemma2.(Hermiteinterpolationproduct)Let×beanassociative,commutative productoverRⅣ【£]whichsatisfies(8),anddefinelⅣ+l∈酞Ⅳ【£]byTN+I=tⅣ×t.Then,foranyPandqinⅣ【f】,P×qistheHer'miteinterpolationofpqatthe(possiblymultiple)zeros oftN+I—TN+l(t)inC.Conversely,anysuchproductisassociativeJcommutative,andsatisfies (8)inⅣ[ThislemmaisprovedinappendixC.Interpretation.Itisconvenienttounderstandtheproductoperators×ofLemma2as follows:ateveryinterpolationpointzoforder0,aTaylorexpansionatorder0ofthepolynomials andiscomputed;ateveryinterpolationpointoforder0,theproductoftheexpansionsiscomputedand thecoefficientsofdegreegreaterthan0axesettozero;denotebyP=theresult; theproductz×YisthentheHermiteinterpolationofthevariousPz. Thisleadstothefollowingconstructivecharacteriz?。
去隔行算法解析
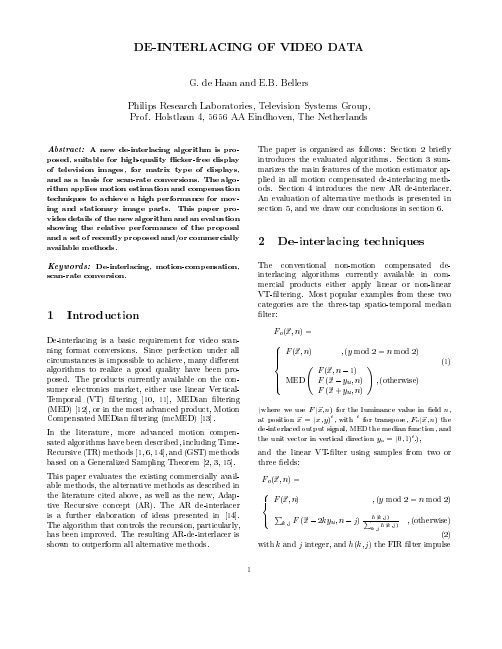
2
De-interlacing techniques
De-interlacing, motion-compensation, scan-rate conversion.
Keywords:
1
Introduction
The conventional non-motion compensated deinterlacing algorithms currently available in commercial products either apply linear or non-linear VT- ltering. Most popular examples from these two categories are the three-tap spatio-temporal median lter:
Ph(k;j )
k;j
(2) with k and j integer, and h(k; j ) the FIR lter impulse
h(k;j )
response, for which we used (two- elds)1 : 8 ; (k = 3; 1; 1; 3) ^ (j = 0) > 1; 8; 8; 1 > > > < 5; 10; 5 ; (k = 2; 0; 2) ^ (j = 1) h(k; j ) = > > > > : 0 ; (otherwise) (3) Motion compensation can be straighforwardly added, e.g. for the median lter [4, 13], using the displacement ~ ~ x vector D short for: D(~ ; n) = (Dx (~ ; n); Dy (~ ; n))t : x x
explanatory sequential mixed method

explanatory sequential mixed method Explanatory Sequential Mixed MethodsIntroduction:Research methodology plays a crucial role in informing decision-making and understanding complex phenomena. Mixed methods research designs have gained popularity in recent years due to their ability to provide a comprehensive and holistic understanding of a research problem. One such design is the explanatory sequential mixed methods approach, which incorporates both quantitative and qualitative components in a sequential manner. This article aims to explain the explanatory sequential mixed methods design, its components, advantages, and limitations, with examples from various research studies.Components of the Explanatory Sequential Mixed Methods Design:The explanatory sequential mixed methods design consists of two distinct phases, namely the quantitative phase and the qualitative phase. In this design, the quantitative component is conducted first and is followed by the qualitative component. The purpose of the quantitative phase is to explore relationships and identify patterns in the data, while the qualitative phase aims to provide a deeper understanding of these relationships and patterns.Quantitative Phase:During the quantitative phase, researchers collect and analyze quantitative data using structured surveys, experiments, orsecondary data sources. The goal is to generate numerical data that can be analyzed using statistical techniques to test hypotheses or patterns. The findings from the quantitative analysis inform the selection of participants and the focus of the qualitative phase.Qualitative Phase:The qualitative phase involves collecting and analyzing qualitative data, such as interviews, observations, or document analysis. The purpose of this phase is to understand the underlying reasons and processes behind the quantitative findings. Qualitative data collection methods allow researchers to gather rich and detailed information that provides context and meaning to the statistical results. The qualitative analysis involves coding, categorizing, and interpreting the data to identify themes and patterns.Integration of Findings:The integration of findings is a crucial step in the explanatory sequential mixed methods design. During this stage, researchers compare and contrast the findings from both the quantitative and qualitative phases to develop a comprehensive understanding of the research problem. This integration can occur in different ways, such as comparing the results side by side, using statistical findings to interpret qualitative data, or using qualitative findings to explain statistical patterns. The aim is to provide a richer and more nuanced understanding of the research topic than could be achieved through a single method or phase.Advantages of the Explanatory Sequential Mixed Methods Design:The explanatory sequential mixed methods design offers several advantages over traditional quantitative or qualitative approaches. Firstly, it provides a more comprehensive understanding of the research problem by combining quantitative and qualitative data. The sequential nature of the design allows researchers to build on the findings from the quantitative phase and explore them in more depth during the qualitative phase. This approach strengthens the validity and reliability of the research findings.Secondly, the design allows researchers to address research questions that require both numerical data and contextual information. Some phenomena cannot be fully understood or explained by numbers alone, and qualitative data can provide valuable insights into the underlying reasons and processes.Thirdly, the design enhances triangulation, which refers to the use of multiple data sources or methods to validate findings. By combining quantitative and qualitative data, researchers can compare and contrast the different perspectives and identify converging or conflicting evidence. This strengthens the overall validity and trustworthiness of the research.Limitations of the Explanatory Sequential Mixed Methods Design:Despite its advantages, the explanatory sequential mixed methods design also has some limitations. Firstly, it requires time and resources to implement both quantitative and qualitative components. Researchers need to consider the feasibility of conducting both phases and ensure that they have the necessaryskills and expertise in both quantitative and qualitative methods.Secondly, the design may face challenges in terms of data integration and interpretation. Combining quantitative and qualitative findings can be complex and may require expertise in both types of data analysis. Researchers need to carefully consider how to integrate the findings in a meaningful and coherent manner.Example Studies:To illustrate the application of the explanatory sequential mixed methods design, three example studies are presented below:1. A study on the effectiveness of a health intervention program uses a quantitative survey to measure participants' health outcomes and satisfaction levels. The qualitative phase involves in-depth interviews with a sub-sample of participants to explore their experiences and perceptions of the program.2. A study on the impact of a teacher training program uses a quantitative pre-test and post-test design to measure changes in students' academic performance. The qualitative phase involves focus group discussions with teachers to understand their perspectives on the program's effectiveness and challenges.3. A study on the factors influencing employee satisfaction and retention uses a quantitative survey to measure employee satisfaction levels. The qualitative phase involves semi-structured interviews with a subset of employees to explore the underlying reasons for their satisfaction or dissatisfaction.Conclusion:The explanatory sequential mixed methods design offers a powerful approach to research that combines the strengths of quantitative and qualitative methods. By integrating numerical data with contextual information, this design provides a comprehensive and holistic understanding of research problems. Despite its limitations, the design has gained popularity due to its ability to address complex research questions and enhance the validity and reliability of findings. Researchers should consider the feasibility and appropriateness of this design for their specific research objectives and resources.。
信息论与编码理论中的英文单词和短语
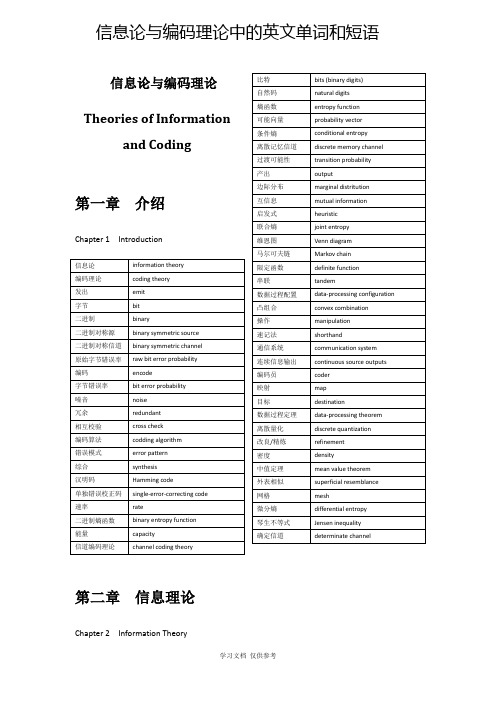
信息论与编码理论Theories of Information
and Coding
第一章介绍
Chapter 1 Introduction
第二章信息理论Chapter 2 Information Theory
信息论与编码理论中的英文单词和短语
第三章 离散无记忆信
道和容量成本方程
Chapter 3 Discrete Memory less Channels and their Capacity -Cost Equations
第四章 离散无记忆信
源和扭曲率方程
Chapter 4 Discrete Memoryless Sources and their Rate -Distortion Equations
第五章 高斯信道和信
源
Chapter 5 Gaussian Channel and Source
第六章 信源-信道编码
理论
Chapter 6 Source -Channel Coding Theory
第七章 第一部分访问
先进标题
Chapter 7 Survey of Advanced Topics for Part One
第八章线性码Chapter 8 Linear codes
第九章循环码Chapter 9 Cyclic Codes
第十章 香农码和相关
的码
Chapter 10 Shannon Codes and Related Codes
第十一章 卷积码
Chapter 11 Convolution Codes
第十二章变量长度源编
码
Chapter 12 Variable-length Source Coding。
HEVC

Bi-linear interpolation filter for chroma samples (1/8-sample) Advanced motion vector prediction Context adaptive binary arithmetic entropy coding Internal bit-depth increase (4 bits) X Low complexity entropy coding phase 2 X Transform precision extension (4 bits) Deblocking filter Adaptive loop filter X
• 从上图中可以看到一种 新的不对称运动分割预 测(Asymmetric Motion Partition,AMP),这也是 HEVC与H.264在分块预 测技术中最为不同之处。 所谓AMP即将编码单元 分割成两个大小不一致 的预测块其中一个PU单 元的宽/长为CU单元的 3/4,这种预测考虑了大 尺寸可能的纹理分布可 以有效提高大尺寸块的 预测效率
1、Picture Partitioning
1.1. Treeblock(TB) Partitioning • Pictures are divided into slices and slices are divided into a sequence of treeblocks. • A treeblock is an NxN block of luma samples together with the two corresponding blocks of chroma samples for a picture that has three sample arrays. • The treeblock concept is broadly analogous to that of the macroblock in previous standards such as H.264/AVC [6].
信号与系统后面的索引(专业词汇中英对照表)
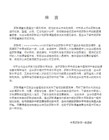
特解 particular solution
特征方程 characteristic equation
特征根 characteristic root
特征矩阵 characteristic matrix
起始值 original value
海诺塔 tower of hanoi
快速傅里叶变换(FFT) fast fourier transform
均匀性(齐次性) homogeneity
围线积分法 contour integral method
极点 pole
初值定理 initial value theorem
系统函数 system function
系统的仿真 simulation of system
倒立摆 inverted pendulum
根轨迹 root-locus
积分器 integrator
流图转置 transpose of flow graph
圆周移位 circular shifting
圆卷积 circular convolution
部分分式展开法 partial fraction expansion method
叠加性 supperposition property
零点 zero
频率响应 frequency response
频率有限信号 frequency finite signal
频率变换 frequency transform
解卷积(反卷积) deconvolution
数字滤波器 digital filter
蝶形计算 butterfly computation
revisiting time series outlier detection 参考文献

Revisiting Time Series Outlier DetectionIntroductionIn the field of time series analysis, outlier detection plays a crucial role in identifying abnormal patterns or data points that deviate significantly from the expected behavior. Detecting outliers in time series data is essential for various applications, such as anomaly detection, fault diagnosis, and fraud detection. This article revisits the topic of time series outlier detection, discussing its importance, challenges, and recent advancements.Challenges in Time Series Outlier DetectionDetecting outliers in time series data presents several challenges due to the sequential nature of the data. Some of the key challenges include:1.Temporal Dependencies: Time series data often exhibits temporaldependencies, where the current value depends on previous values.Detecting outliers requires considering both the current datapoint and its relationship with past observations.2.Seasonality and Trends: Time series data often contain seasonalpatterns or long-term trends. These underlying patterns can affect the detection of outliers, as they may be misinterpreted asabnormal behavior.3.Varying Magnitudes: Outliers in time series data can have varyingmagnitudes. Some outliers might be moderate deviations from theexpected behavior, while others can be extreme anomalies. A robust outlier detection technique should be able to capture outliers of different magnitudes.4.Data Imbalance: Time series data is typically imbalanced, with alarge number of normal instances and only a few outliers. Thismakes it challenging to build accurate models that can effectively identify outliers.Classical Approaches to Time Series Outlier DetectionSeveral classical approaches have been proposed for time series outlier detection. These approaches can be broadly classified into three categories:Statistical MethodsStatistical methods are widely used for detecting outliers in time series data. These methods rely on statistical properties, such as mean, standard deviation, or confidence intervals, to identify data pointsthat deviate significantly from the expected behavior. Some commonly used statistical techniques include:•Z-Score: This method measures the number of standard deviations an observation is away from the mean. Data points with a high z-score are considered outliers.•Grubbs’ Test:Grubbs’ Test is based on the hypothesis that the maximum or minimum value in a univariate dataset is an outlier.The test calculates a test statistic and determines whether it is significant enough to reject the null hypothesis.Distance-Based MethodsDistance-based methods treat time series data as high-dimensional vectors and measure the similarity or dissimilarity between data points. Outliers are identified based on their distance from the remaining data points. Some commonly used distance-based techniques include:•k-Nearest Neighbors: This method calculates the average distance to the k nearest neighbors. Observations with a significantlyhigher average distance are considered outliers.•Local Outlier Factor (LOF): LOF measures the density deviation ofa data point compared to its neighbors. Outliers have asignificantly lower density compared to their neighbors.Model-Based MethodsModel-based methods assume that the time series data follows a certain model, and outliers are defined as data points that do not conform tothe model. Some commonly used model-based techniques include:•ARIMA Models: Autoregressive Integrated Moving Average (ARIMA) models are widely used for time series forecasting. Outliers aredetected based on the residuals obtained from fitting an ARIMAmodel to the data.•Hidden Markov Models: Hidden Markov Models (HMMs) areprobabilistic models that assume the underlying system hasunobserved states. Outliers are identified based on the likelihood of the observed sequence given the HMM.Recent Advancements in Time Series Outlier DetectionRecent advancements in time series outlier detection have focused on improving the accuracy and robustness of existing methods. Some notable advancements include:1.Deep Learning-Based Approaches: Deep learning models, such asrecurrent neural networks (RNNs) and convolutional neural networks (CNNs), have shown promising results in time series outlierdetection. These models can capture temporal dependencies andlearn complex patterns in the data, improving the detection ofoutliers.2.Ensemble Methods: Ensemble methods combine multiple outlierdetection techniques to improve performance. By aggregating theoutputs of different methods, ensemble approaches can provide more reliable outlier detection results.3.Unsupervised Learning Techniques: Unsupervised learningtechniques, such as clustering algorithms and self-organizing maps, have been applied to time series outlier detection. These methodsleverage the inherent clustering structure in the data to identify outliers.4.Domain-Specific Approaches: Some recent advancements cater tospecific domains, such as financial data or sensor data. Thesedomain-specific approaches incorporate domain knowledge to improve outlier detection accuracy.Evaluation Metrics for Time Series Outlier DetectionTo evaluate the performance of time series outlier detection techniques, several metrics are commonly used. These include:1.Precision: Precision measures the proportion of true outliersamong the detected outliers. A higher precision indicates fewerfalse positives.2.Recall: Recall measures the proportion of true outliers that arecorrectly detected. A higher recall indicates fewer falsenegatives.3.F1 Score: The F1 score is the harmonic mean of precision andrecall. It provides a balance between precision and recall, withhigher values indicating better performance.4.Area Under the Receiver Operating Characteristic (ROC) Curve: TheROC curve plots the true positive rate against the false positive rate for different threshold values. The area under the ROC curve (AUC) provides an overall measure of a model’s performance, with larger values indicating better performance.ConclusionTime series outlier detection remains an important research area with significant practical implications. Classical approaches based on statistical, distance-based, and model-based methods have been widely used but have their limitations. Recent advancements in deep learning, ensemble methods, unsupervised learning, and domain-specific approaches have improved the accuracy and robustness of outlier detection techniques. Selecting appropriate evaluation metrics is crucial to assess the performance of these methods accurately. As time series data continues to grow in complexity and volume, further research is needed to develop more advanced techniques for detecting outliers effectively.。
rife算法的matlab -回复

rife算法的matlab -回复如何使用Matlab实现Rife算法1. 引言(100-200字)Rife算法是一种用于信号处理和图像处理的重要算法之一。
它可以用于音频信号的降噪、语音增强和图像超分辨率重建等领域。
本文将逐步介绍如何使用Matlab实现Rife算法,并探讨在实际应用中的一些注意事项。
2. 算法原理介绍(400-600字)Rife算法的全称为Recursive Inverse Filter Estimation(递归反滤波估计)算法,其基本原理是通过递归地计算逆滤波器来恢复原始信号。
具体而言,算法通过先前的估计结果来改进当前的估计结果,直到达到预设的终止条件。
Rife算法的核心是估计逆滤波器,该逆滤波器可以将包含噪声的信号转换为干净的信号。
算法首先将输入信号分解成一系列的子带,然后通过估计每个子带的逆滤波器来进行降噪处理。
估计逆滤波器的过程可以通过求解一个最小二乘问题来实现。
3. Matlab实现步骤(400-600字)要在Matlab中实现Rife算法,可以按照以下步骤进行:步骤1:加载信号首先,需要将待处理的信号加载到Matlab中。
可以使用Matlab提供的"audioread"函数来读取音频文件,并将其存储为一个向量。
步骤2:信号分解Rife算法需要将信号分解成多个子带,以便于对每个子带进行逆滤波处理。
可以使用Matlab中的小波变换函数(如"dwt")来将信号分解成多个尺度的子带。
步骤3:逆滤波器估计对于每个子带,需要估计一个逆滤波器来恢复干净的信号。
可以使用Matlab的信号处理工具箱中提供的函数(如"lsqminnorm")来求解一个最小二乘问题,以估计逆滤波器。
步骤4:逆滤波使用估计的逆滤波器来对每个子带进行逆滤波操作,以恢复干净的信号。
可以使用Matlab中的线性滤波函数(如"filter")来实现逆滤波。
人工智能机器学习特征选择之:Wrapper递归特征消除(FRE)
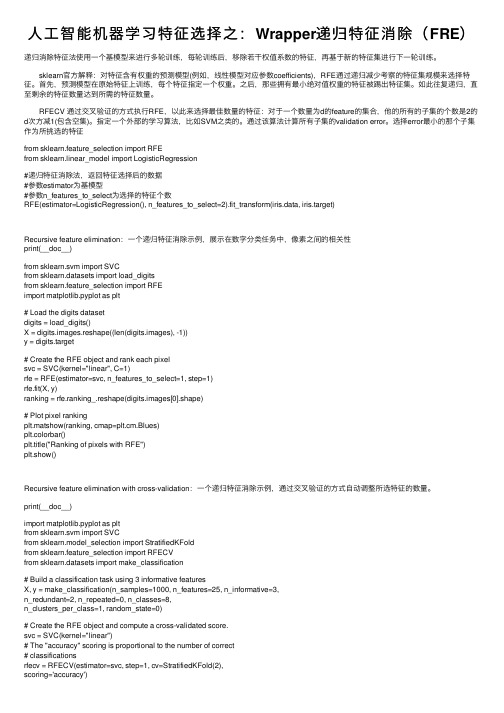
⼈⼯智能机器学习特征选择之:Wrapper递归特征消除(FRE)递归消除特征法使⽤⼀个基模型来进⾏多轮训练,每轮训练后,移除若⼲权值系数的特征,再基于新的特征集进⾏下⼀轮训练。
sklearn官⽅解释:对特征含有权重的预测模型(例如,线性模型对应参数coefficients),RFE通过递归减少考察的特征集规模来选择特征。
⾸先,预测模型在原始特征上训练,每个特征指定⼀个权重。
之后,那些拥有最⼩绝对值权重的特征被踢出特征集。
如此往复递归,直⾄剩余的特征数量达到所需的特征数量。
RFECV 通过交叉验证的⽅式执⾏RFE,以此来选择最佳数量的特征:对于⼀个数量为d的feature的集合,他的所有的⼦集的个数是2的d次⽅减1(包含空集)。
指定⼀个外部的学习算法,⽐如SVM之类的。
通过该算法计算所有⼦集的validation error。
选择error最⼩的那个⼦集作为所挑选的特征from sklearn.feature_selection import RFEfrom sklearn.linear_model import LogisticRegression#递归特征消除法,返回特征选择后的数据#参数estimator为基模型#参数n_features_to_select为选择的特征个数RFE(estimator=LogisticRegression(), n_features_to_select=2).fit_transform(iris.data, iris.target)Recursive feature elimination:⼀个递归特征消除⽰例,展⽰在数字分类任务中,像素之间的相关性print(__doc__)from sklearn.svm import SVCfrom sklearn.datasets import load_digitsfrom sklearn.feature_selection import RFEimport matplotlib.pyplot as plt# Load the digits datasetdigits = load_digits()X = digits.images.reshape((len(digits.images), -1))y = digits.target# Create the RFE object and rank each pixelsvc = SVC(kernel="linear", C=1)rfe = RFE(estimator=svc, n_features_to_select=1, step=1)rfe.fit(X, y)ranking = rfe.ranking_.reshape(digits.images[0].shape)# Plot pixel rankingplt.matshow(ranking, cmap=plt.cm.Blues)plt.colorbar()plt.title("Ranking of pixels with RFE")plt.show()Recursive feature elimination with cross-validation:⼀个递归特征消除⽰例,通过交叉验证的⽅式⾃动调整所选特征的数量。
CIC补偿滤波器设计

(3)
∑ H(z)
=
H IN ( z ) H cN ( z R )
=
⎛ ⎜ ⎜
R
M
–
1
z–k⎟⎟⎞
N
⎝ k=0 ⎠
In this equation, N is the number of integrator-comb filter pairs, and R is the rate change factor. Equation 3 implies that the equivalent time domain impulse response of a CIC filter can be viewed as a cascade of N rectangular pulses. Each rectangular pulse has RM taps.
(4) H(f) = -s--i--n---(---π---M------f--) N
sin
⎛ ⎝
π-R---f⎠⎞
Figure 3 shows an example of a CIC filter magnitude response:
Figure 3. Magnitude Response of a CIC Filter with N = 9, R = 8, and M = 1
字母列表词汇

Vocabulary 2Aabsolutely integrable绝对可积absolutely summable 绝对可和accumulation 累加,累加器adder 加法器additivity 可加性advantageou 有利的aliasing混叠amplitude modulation 幅度调制Analog-to-Digital(A/D) 模数转换analysis equation 分析公式angle criterion 角判椐angle 角,角度angular角度的anti-causal反因果aperiodic非周期approximated 近似associative Property结合律性质assume 假定,假设attenuate 衰减average power 平均功率Bbandpass filter 带通滤波器bank account 银行存款bilateral双边的block diagram方框图bounded input bounded output有界输入-有界输出Butterworth Filters 巴特沃兹滤波器Ccanonical 规范的,正则的causality 因果性characterized 表征classification of Signals 信号分类clockwise 顺时针closed-loop poles 闭环极点combinations 组合commutative property交换律性质compensation for nonideal elements 非理想元件的补偿complex amplitude factor复振幅因子complex exponential 复指数conjugate symmetry 共轭对称性conjugation 共轭constant-coefficient常系数construct 构成contribution 贡献controllable可控的convenient 方便的convergence收敛convolution Integral 卷积积分convolution property 卷积性质convolution Sum卷积和convolution 卷积counterclockwise逆时针ctate transition 状态变换cutoff frequency 截止频率Ddecomposition 分解demonstrate 示范, 证明, 论证(用实验说明,图解, 演示)destabilization 不稳定difference equations 差分方程differential equations 微分方程differentiating filters 微分滤波器differentiation frequency 频域微分Digital -to- Analog(D/A) 数模转换Dirichlet 狄里赫利discrete-timeFourier transform (DTFT) 离散傅立叶变换distributive Property分配律性质doublets 冲激偶duality 对偶性Eedges 边缘eigenfunction 特征函数eigenvalue 特征值eliminate 除去encirclement 围线energy-density spectrum 能量密度谱/能谱密度enhance 增强、增晰entirely 完全地envelope 包络equalizing filter 均/平衡滤波器essentially 基本上evaluate 求...的值explicit 显式exponential 指数Ffactors 因子feedback connection 反馈联接filtering 滤波filter滤波器61final-value theorems 终值定理Finite Impulse Response (FIR) system 有限脉冲响应系统(FIR)first difference 一次(介)差分first harmonic components 一次谐波分量forced response 受迫响应Fourier integral傅立叶积分Fourier series coefficients 傅立叶系数Fourier transform pair 傅立叶变换对Fourier transform傅立叶变换Fourier 傅立叶frequency response 频率响应frequency-selective filters 频率选择性滤波器frequency-shaping filters 频率成形滤波器full solution 完全解full-wave全波fundamental components 基波分量fundamental period 基波周期Ggain 增益geometric几何Gibbs phenomenon吉伯斯现象Hharmonically 谐波highpass filter 高通滤波器historical perspective 历史回顾hnit step单位阶跃homogeneity 齐次性homogeneous solution 齐次解Iideal frequency-selective filters 理想频率选择性滤波器idealized short pulsev理想化短脉冲identity system 恒等系统if and only if 当且仅当implicit 隐含impulse train 冲激串incrementally linear systems 增量线性系统independent variable 独立变量initial condition 初始条件initial rest 初始松弛initial-value theorems 初值定理instantaneous 瞬间integer multiples 整数倍integrator 积分器intermediate 中间的interpolation 内插interval 区间inverse Fourier transform 傅立叶反变换inverse system design 逆系统设计inverse systems 可逆系统inverse Z-transform Z反变换,Z逆变换invertibility 可逆性LLagrange 拉格朗日Laplace Transform拉普拉斯变换Laplace拉普拉斯inear feedback systems 线性反馈系统linearity 线性lowpass filter 低通滤波器Mmagnitude值,模值Mason 梅森modulation property 调制性质multilateral多边的multiplication 相乘multiplication by a coefficient 标量乘法器Nnatural response 自然响应nonrecursive equation 非递归方程nonrecursive 非递归Nyquist奈奎斯特Oobservable可观测的orthogonal 直角,正交oscilloscope 示波器Pparallel connection并联parallel 平行Parseval’s Relation帕斯瓦尔定理particular solution 特解passband 通带periodic convolution 周期卷积Phase Margins 相位裕度pole 极点Pole-Zero Plot 零极点图property 性质prove 证明, 证实, 检验Rradian弧度radius 半径rational 有理的reconstruct 重建rectified已整流的recursive equation 递归方程recursive 递归Region of Convergence(ROC)收敛域region区域relative amplitudes 相对振幅representation 表示restric限制Root-Locus 根轨迹Routh 罗斯62Ssample values 样本值sample 样本sampling theorem 采样定理sampling 采样scaling 比例性s-Domain s域second harmonic components 二次谐波分量series connection 串联,级联sifting property 筛选性质Signal Flow Graphs 信号流图significantly 显著地singularity functions 奇异函数sinusoidal 正弦spectral coefficients 频谱系数spectrum 频谱stability 稳定性stabilization of unstable systems 不稳定系统的稳定State-Space 状态空间stock market 股票市场stopband 阻带strip带状successive 逐次的summation累加器superposition principle 叠加原理symbolic function 符号函数symmetric 对称synthesis equation 综合公式system function 系统函数Ttime expansion 时域扩展time interval 时间间隔time invariance 时不变性time reversal 时间反转time scaling, time-scaled 时间尺度变换time shifting,time-shifted 时移time-varying system 时变系统tracking systems 跟踪系统triangular 三角Uundersampling欠采样undistorted 无失真unilateral 单边的uniquely 唯一地unit cricle单位圆unit delay 单位延时器unit impulse单位冲激, 单位脉冲unit step response 单位阶跃响应Vvariation 起伏变化vector矢量,向量verify 证实; 查证, 鉴定, 验证,证明,检验,核实Zz-domain z域zero 零点zero-input response 零输入响应zero-order hold 零阶保持zero-state response. 零状态响应Z-Transform Z变换63。
- 1、下载文档前请自行甄别文档内容的完整性,平台不提供额外的编辑、内容补充、找答案等附加服务。
- 2、"仅部分预览"的文档,不可在线预览部分如存在完整性等问题,可反馈申请退款(可完整预览的文档不适用该条件!)。
- 3、如文档侵犯您的权益,请联系客服反馈,我们会尽快为您处理(人工客服工作时间:9:00-18:30)。
ABSTRACT
s(tk )
?
+
e(tk ) intraframe encoder s(tk ) ^
intraframe decoder
s0 (tk -1) s0 (tk ) z ?1 ~ d(tk )
+
motion compensation motion estimation
Figure 1: Block diagram of a hybrid video encoder based on motion compensated prediction. of the sampling rate is denoted as upsampling. In case 1 of L -pel resolution of the displacement vectors, s (tk -1) has to be sampled up by the factor of L. The result of such an upsampling is denoted by su (tk -1). In the following the samples of s (tk -1) are called fullpel samples and the samples to be reconstructed, between the fullpel samples are called subpel samples. Due to non ideal LP- lters in the image acquisition process, there is aliasing in the sampled image signal s(tk ). Thus, the aliasing in the image to be coded s(tk ) should also be predicted by a motion compensated prediction in order to reduce the prediction error. Conventional motion compensated prediction uses bilinear interpolation to generate the upsampled image su (tk -1) and thus do not take into account aliasing 8]. Recent approaches use Wiener Filters in order to reduce the aliasing e ect 6, 7]. But, since the aliasing signal is unknown, Wiener lters can not compensate the aliasing perfectly 1, 2, 6]. Therefore the accuracy of
A TIME-RECURSIVE INTERPOLATION FILTER FOR MOTION COMPENSATED PREDICTION CONSIDERING ALIASING
Thomas Wedi
Institut fuer Theoretische Nachrichtentechnik und Informationsverarbeitung Universitaet Hannover, Appelstr. 9A, 30167 Hannover, Germany Email: wedi@tnt.uni-hannover.de
In this paper a new interpolation lter for motion compensated prediction in a hybrid video codec is presented. It allows to interpolate an aliasing a ected image signal more accurate than conventional interpolation lters like bilinear or Wiener lter. In contrast to conventional lters, the new time-recursive lter uses not only the image signal of the current frame, but also the image signal of previous frames in order to interpolate the current frame. The lter has been tested with synthetic and real sequences. The experimental results for the synthetic sequence show, that with conventional lters it is not possible to predict an aliasing a ected image perfectly, even if the estimated displacements perfectly t to the sequence. Furthermore it is shown, that with the new lter it is possible to predict an aliasing a ected image perfectly. For the used real sequence a coding gain of 0.8 dB is achieved compared to an MPEG-4 codec.
2. THE TIME-RECURSIVE INTERPOLATION FILTER
The idea of the new interpolation lter presented in this paper is the following: If an unmoved, analog image signal is sampled at the same positions at di erent times, the two sampled signals are identical even if the sampling rate doesn't t to the Nyquist frequency. Therefore the sampled images also have the same aliasing signal. If the image signal is displaced by one sample, the corresponding, displaced samples are identical and have the same aliasing. This shows, that at full-pel displacements the aliasing does not a ect the prediction. Furthermore, it induces a new interpolation approach for increasing the sampling rate. If it is known that a signal is shifted by a fractional-pel displacement, we may insert the former samples from the fullpel positions at the corresponding subpel positions in the current upsampled image, knowing that they contain the correct sampled image signal. Even the aliasing is predicted correctly. Based on this idea a new lter is presented in order to generate the upsampled image su (tk -1), which takes fullpel samples of previous frames to reconstruct the subpel samples of the frame to be interpolated. The
0 0 0 0
1. INTRODUCTION
Standardized hybrid video codecs like H.263, MPEG-2, MPEG-4 etc. are based on motion compensated prediction 3, 4, 8]. Figure 1 shows the block diagram of such a hybrid video encoder. In the motion estima~ tion module the displacement vector eld d(tk ) between consecutive images is estimated. The displacement vectors and the previous reconstructed image s (tk -1) are used in the motion compensation in order to create the prediction image s(tk ). The remaining prediction-error ^ e(tk ) and the displacement vector eld are encoded and transmitted. The standardized coding techniques use displacement vectors with fractional-pel resolution 4, 5, 8]. In order to estimate and compensate fractional-pel displacements, the image signal between the sampled positions of image s (tk -1) has to be reconstructed. This reconstruction is equivalent to an increase of the sampling rate of s (tk -1) 1, 2]. In the following the-recursive interpolation lter and is therefore called TRI-Filter. In Figure 2 the block diagram 1 of the TRI-Filter is shown for an L -pel resolution of the displacement vectors. The lter consists of three major components. The rst component is the expander "L, the second is the recursive structure with the merging and the motion compensation module and the third component is the spatial interpolation. These three components are described in the following. In the rst component, the module "L expands the sampling rate of image s (tk -1) by the factor of L, resulting in image se (tk -1).