Annotating multi-media multimodal resources with ELAN
多模态目标检测与跟踪算法研究

多模态目标检测与跟踪算法研究随着计算机视觉和机器学习的快速发展,多模态目标检测与跟踪成为了研究的热点。
多模态目标检测与跟踪是指利用多种传感器或数据源,如图像、视频、语音等,对目标进行同时检测和跟踪的技术。
这种技术在智能交通、智能安防等领域具有广泛的应用前景。
本文将对多模态目标检测与跟踪算法进行深入研究,并探讨其在实际应用中的挑战和发展方向。
首先,我们将介绍多模态目标检测算法的研究进展。
传统的单模态图像目标检测算法主要基于深度学习方法,如卷积神经网络(CNN)和循环神经网络(RNN)。
这些方法在单一数据源上取得了很好的效果。
然而,在实际应用中,我们往往需要同时利用图像、视频和语音等多种数据源来进行综合分析。
因此,研究者们提出了一系列基于传感器融合或特征融合的多模态目标检测算法。
这些算法将多种数据源的信息进行融合,从而提高了目标检测的准确性和鲁棒性。
同时,还有一些研究致力于解决多模态数据的异构性问题,通过学习跨模态特征映射来实现跨模态目标检测。
其次,我们将探讨多模态目标跟踪算法的研究进展。
传统的目标跟踪算法主要基于单一数据源,如图像序列或视频序列。
这些方法通过建立目标模型或运动模型来实现目标跟踪。
然而,在复杂场景下,单一数据源往往无法提供足够准确的信息进行精确跟踪。
因此,研究者们提出了基于多传感器或多特征融合的多模态目标跟踪算法。
这些算法通过同时利用图像、视频、语音等不同数据源来进行综合分析和建模,在复杂场景下取得了较好的效果。
然而,在实际应用中,多模态目标检测与跟踪还面临许多挑战。
首先是异构性问题。
不同传感器或数据源之间存在着差异,如分辨率、噪声、视角等。
如何有效地融合这些异构数据,提取有效的跨模态特征,是一个亟待解决的问题。
其次是数据关联问题。
在多模态目标跟踪中,如何准确地建立多个时间步之间的目标关联关系,是一个复杂而困难的问题。
当前的研究主要基于传统的图像处理和统计方法,还需要进一步探索更有效和准确的数据关联方法。
人工智能多模态算法模型-概述说明以及解释
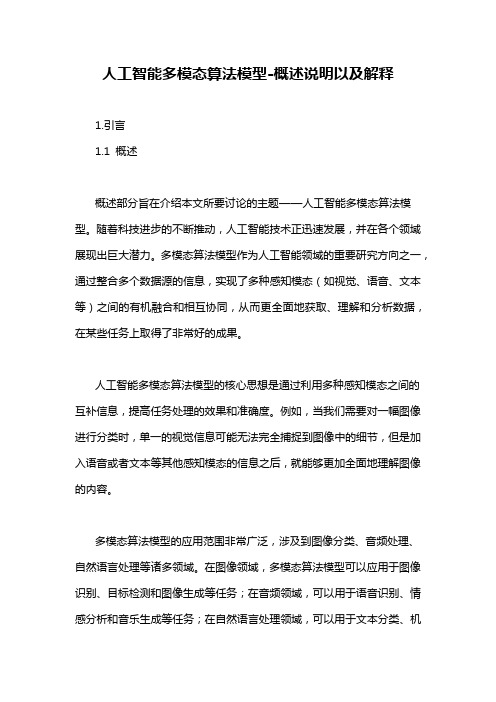
人工智能多模态算法模型-概述说明以及解释1.引言1.1 概述概述部分旨在介绍本文所要讨论的主题——人工智能多模态算法模型。
随着科技进步的不断推动,人工智能技术正迅速发展,并在各个领域展现出巨大潜力。
多模态算法模型作为人工智能领域的重要研究方向之一,通过整合多个数据源的信息,实现了多种感知模态(如视觉、语音、文本等)之间的有机融合和相互协同,从而更全面地获取、理解和分析数据,在某些任务上取得了非常好的成果。
人工智能多模态算法模型的核心思想是通过利用多种感知模态之间的互补信息,提高任务处理的效果和准确度。
例如,当我们需要对一幅图像进行分类时,单一的视觉信息可能无法完全捕捉到图像中的细节,但是加入语音或者文本等其他感知模态的信息之后,就能够更加全面地理解图像的内容。
多模态算法模型的应用范围非常广泛,涉及到图像分类、音频处理、自然语言处理等诸多领域。
在图像领域,多模态算法模型可以应用于图像识别、目标检测和图像生成等任务;在音频领域,可以用于语音识别、情感分析和音乐生成等任务;在自然语言处理领域,可以用于文本分类、机器翻译和情感分析等任务。
通过将多模态的信息进行融合和分析,多模态算法模型能够更好地解决现实生活中复杂多变的问题。
本文旨在深入探讨多模态算法模型的概念、应用领域、优势以及发展前景。
通过对多模态算法模型的研究和实践,将有助于推动人工智能技术在多个领域的应用,为实现智能化社会做出更大贡献。
文章结构部分的内容如下:1.2 文章结构本文主要探讨人工智能中的多模态算法模型。
文章分为引言、正文和结论三个部分。
在引言部分,我们将对多模态算法模型进行一个概述,介绍其基本概念和重要性。
同时,我们还会对本文的结构进行简要的说明,以便读者对全文有一个整体的了解。
最后,我们会明确本文的目的,即为读者提供关于多模态算法模型的全面理解。
在正文部分,我们将进一步探讨多模态算法模型的概念,并介绍其在各个领域的应用。
我们将重点介绍多模态算法模型在语音识别、图像处理和自然语言处理等领域的应用,并阐述其在这些领域中的优势和挑战。
医学多模态可解释模型-概述说明以及解释
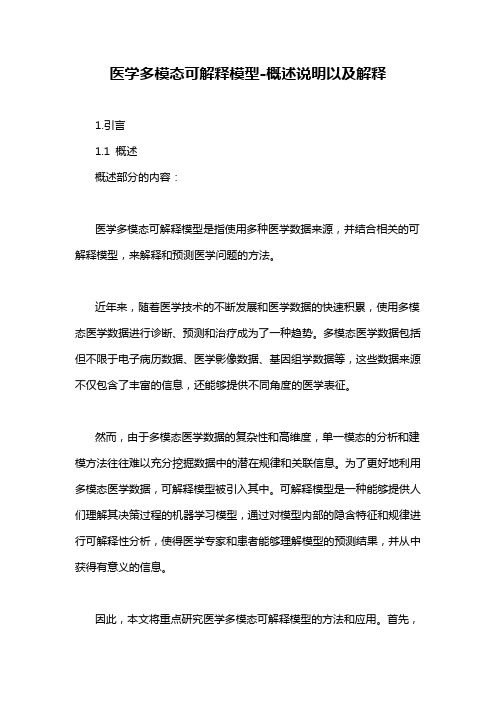
医学多模态可解释模型-概述说明以及解释1.引言1.1 概述概述部分的内容:医学多模态可解释模型是指使用多种医学数据来源,并结合相关的可解释模型,来解释和预测医学问题的方法。
近年来,随着医学技术的不断发展和医学数据的快速积累,使用多模态医学数据进行诊断、预测和治疗成为了一种趋势。
多模态医学数据包括但不限于电子病历数据、医学影像数据、基因组学数据等,这些数据来源不仅包含了丰富的信息,还能够提供不同角度的医学表征。
然而,由于多模态医学数据的复杂性和高维度,单一模态的分析和建模方法往往难以充分挖掘数据中的潜在规律和关联信息。
为了更好地利用多模态医学数据,可解释模型被引入其中。
可解释模型是一种能够提供人们理解其决策过程的机器学习模型,通过对模型内部的隐含特征和规律进行可解释性分析,使得医学专家和患者能够理解模型的预测结果,并从中获得有意义的信息。
因此,本文将重点研究医学多模态可解释模型的方法和应用。
首先,我们将介绍多模态医学数据的概念和特点,包括数据的来源、类型和处理方法。
然后,我们将详细介绍可解释模型的基本原理和常用算法,探讨其在医学领域中的应用场景和价值。
通过综合多模态医学数据和可解释模型的优势,我们将能够更准确地预测和诊断疾病,为医学研究和临床实践提供有力支持。
总之,医学多模态可解释模型的出现为医学研究和临床实践带来了新的机遇和挑战。
通过综合利用多模态医学数据和可解释模型的能力,我们可以更好地理解和解释医学问题,为患者提供更准确、个性化的诊疗方案。
本文将对该领域的研究进行深入探讨,希望能够为医学界的同仁和研究人员提供一定的参考和启发。
1.2 文章结构文章结构部分的内容主要是介绍和解释整篇文章的组织结构和各个部分的内容安排。
在本篇文章中,整体结构可以分为引言、正文和结论三个部分。
在引言部分,我们会首先给出对本篇文章主题的概述,简要介绍医学多模态可解释模型的背景、意义和应用领域。
接着,我们会详细介绍文章的结构,即下文将涉及的各个具体部分,以及它们在整个文章中的位置和作用。
融合多尺度通道注意力的开放词汇语义分割模型SAN
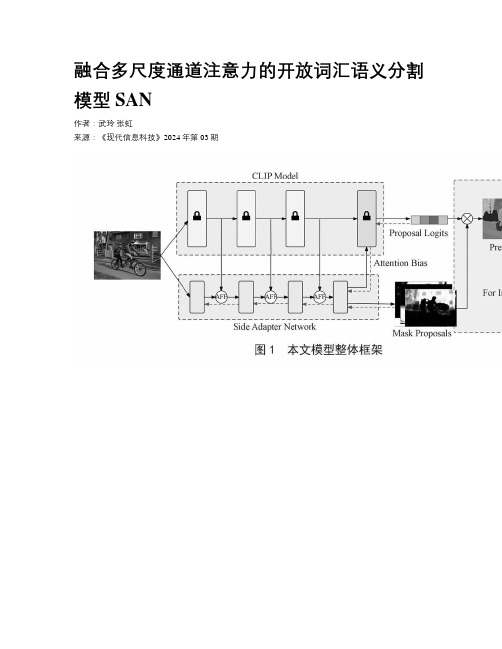
融合多尺度通道注意力的开放词汇语义分割模型SAN作者:武玲张虹来源:《现代信息科技》2024年第03期收稿日期:2023-11-29基金项目:太原师范学院研究生教育教学改革研究课题(SYYJSJG-2154)DOI:10.19850/ki.2096-4706.2024.03.035摘要:随着视觉语言模型的发展,开放词汇方法在识别带注释的标签空间之外的类别方面具有广泛应用。
相比于弱监督和零样本方法,开放词汇方法被证明更加通用和有效。
文章研究的目标是改进面向开放词汇分割的轻量化模型SAN,即引入基于多尺度通道注意力的特征融合机制AFF来改进该模型,并改进原始SAN结构中的双分支特征融合方法。
然后在多个语义分割基准上评估了该改进算法,结果显示在几乎不改变参数量的情况下,模型表现有所提升。
这一改进方案有助于简化未来开放词汇语义分割的研究。
关键词:开放词汇;语义分割;SAN;CLIP;多尺度通道注意力中图分类号:TP391.4;TP18 文献标识码:A 文章编号:2096-4706(2024)03-0164-06An Open Vocabulary Semantic Segmentation Model SAN Integrating Multi Scale Channel AttentionWU Ling, ZHANG Hong(Taiyuan Normal University, Jinzhong 030619, China)Abstract: With the development of visual language models, open vocabulary methods have been widely used in identifying categories outside the annotated label. Compared with the weakly supervised and zero sample method, the open vocabulary method is proved to be more versatile and effective. The goal of this study is to improve the lightweight model SAN for open vocabularysegmentation, which introduces a feature fusion mechanism AFF based on multi scale channel attention to improve the model, and improve the dual branch feature fusion method in the original SAN structure. Then, the improved algorithm is evaluated based on multiple semantic segmentation benchmarks, and the results show that the model performance has certain improvement with almost no change in the number of parameters. This improvement plan will help simplify future research on open vocabulary semantic segmentation.Keywords: open vocabulary; semantic segmentation; SAN; CLIP; multi scale channel attention 0 引言識别和分割任何类别的视觉元素是图像语义分割的追求。
多模态信号特征提取和识别算法研究
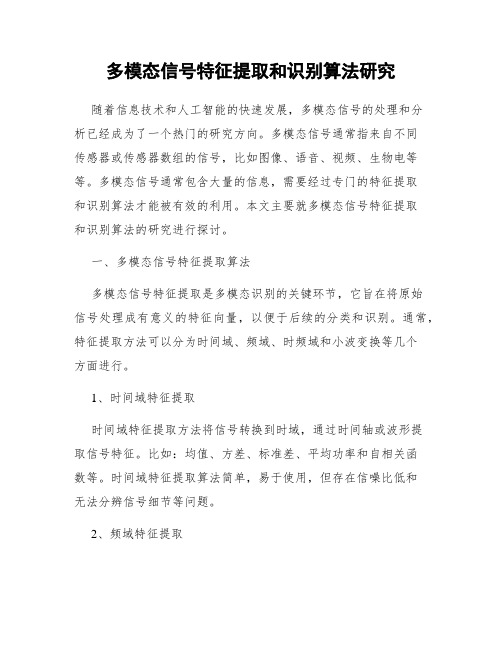
多模态信号特征提取和识别算法研究随着信息技术和人工智能的快速发展,多模态信号的处理和分析已经成为了一个热门的研究方向。
多模态信号通常指来自不同传感器或传感器数组的信号,比如图像、语音、视频、生物电等等。
多模态信号通常包含大量的信息,需要经过专门的特征提取和识别算法才能被有效的利用。
本文主要就多模态信号特征提取和识别算法的研究进行探讨。
一、多模态信号特征提取算法多模态信号特征提取是多模态识别的关键环节,它旨在将原始信号处理成有意义的特征向量,以便于后续的分类和识别。
通常,特征提取方法可以分为时间域、频域、时频域和小波变换等几个方面进行。
1、时间域特征提取时间域特征提取方法将信号转换到时域,通过时间轴或波形提取信号特征。
比如:均值、方差、标准差、平均功率和自相关函数等。
时间域特征提取算法简单,易于使用,但存在信噪比低和无法分辨信号细节等问题。
2、频域特征提取频域特征提取方法将信号转换到频域,通过频域分析提取信号特征。
比如:傅里叶变换、短时傅里叶变换和快速傅里叶变换等。
频域分析可以分析信号的频率和相位信息,但过多的频域维数负面影响分类性能和计算效率。
3、时频域特征提取时频域特征提取方法将信号转换到时频域,通过时频分析提取信号特征。
比如:连续小波变换和离散小波变换等。
时频分析能同时提取信号的时间和频率信息,能有效防止时域和频域方法的局限性,但计算量较大。
4、小波变换特征提取小波变换特征提取方法将信号通过小波基函数分解为多个分量的线性组合,提取每个分量的特征向量。
小波变换是一种非局部、信号分辨率分层和多分辨率分析的信号处理方法,能提取信号的时-频特征,在处理信号时可有效的抑制噪声干扰。
二、多模态信号识别算法多模态信号识别算法是通过对多模态信号特征进行聚类、分类等方法进行识别。
识别算法有许多,例如支持向量机(SVM)、人工神经网络(ANN)、朴素贝叶斯分类(Naive Bayes)、隐马尔可夫模型(HMM)和决策树分类等。
多模态融合
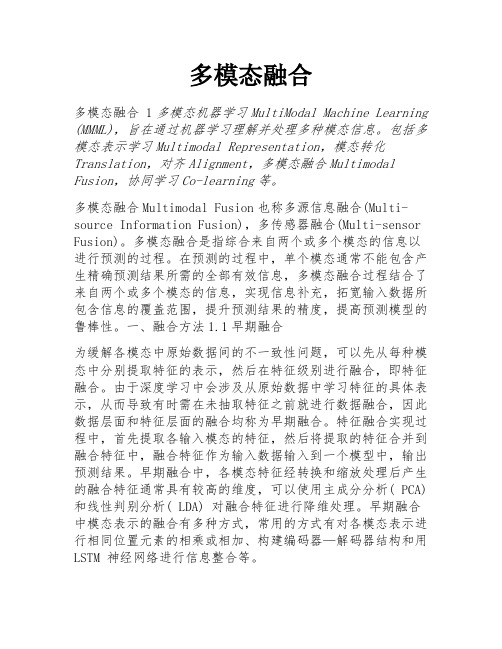
多模态融合多模态融合 1多模态机器学习MultiModal Machine Learning (MMML),旨在通过机器学习理解并处理多种模态信息。
包括多模态表示学习Multimodal Representation,模态转化Translation,对齐Alignment,多模态融合Multimodal Fusion,协同学习Co-learning等。
多模态融合Multimodal Fusion也称多源信息融合(Multi-source Information Fusion),多传感器融合(Multi-sensor Fusion)。
多模态融合是指综合来自两个或多个模态的信息以进行预测的过程。
在预测的过程中,单个模态通常不能包含产生精确预测结果所需的全部有效信息,多模态融合过程结合了来自两个或多个模态的信息,实现信息补充,拓宽输入数据所包含信息的覆盖范围,提升预测结果的精度,提高预测模型的鲁棒性。
一、融合方法1.1早期融合为缓解各模态中原始数据间的不一致性问题,可以先从每种模态中分别提取特征的表示,然后在特征级别进行融合,即特征融合。
由于深度学习中会涉及从原始数据中学习特征的具体表示,从而导致有时需在未抽取特征之前就进行数据融合,因此数据层面和特征层面的融合均称为早期融合。
特征融合实现过程中,首先提取各输入模态的特征,然后将提取的特征合并到融合特征中,融合特征作为输入数据输入到一个模型中,输出预测结果。
早期融合中,各模态特征经转换和缩放处理后产生的融合特征通常具有较高的维度,可以使用主成分分析( PCA) 和线性判别分析( LDA) 对融合特征进行降维处理。
早期融合中模态表示的融合有多种方式,常用的方式有对各模态表示进行相同位置元素的相乘或相加、构建编码器—解码器结构和用LSTM 神经网络进行信息整合等。
1.2后期融合后期融合法又称决策级融合法,先用不同的模型训练不同的模式,然后融合多个模型的输出结果。
数据挖掘中的多模态数据分析方法
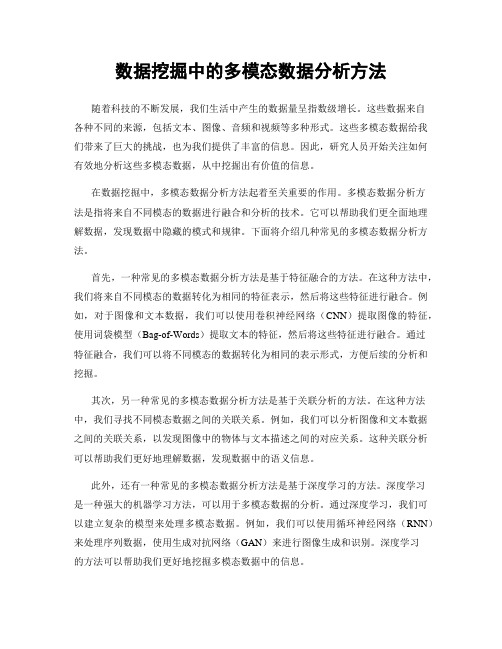
数据挖掘中的多模态数据分析方法随着科技的不断发展,我们生活中产生的数据量呈指数级增长。
这些数据来自各种不同的来源,包括文本、图像、音频和视频等多种形式。
这些多模态数据给我们带来了巨大的挑战,也为我们提供了丰富的信息。
因此,研究人员开始关注如何有效地分析这些多模态数据,从中挖掘出有价值的信息。
在数据挖掘中,多模态数据分析方法起着至关重要的作用。
多模态数据分析方法是指将来自不同模态的数据进行融合和分析的技术。
它可以帮助我们更全面地理解数据,发现数据中隐藏的模式和规律。
下面将介绍几种常见的多模态数据分析方法。
首先,一种常见的多模态数据分析方法是基于特征融合的方法。
在这种方法中,我们将来自不同模态的数据转化为相同的特征表示,然后将这些特征进行融合。
例如,对于图像和文本数据,我们可以使用卷积神经网络(CNN)提取图像的特征,使用词袋模型(Bag-of-Words)提取文本的特征,然后将这些特征进行融合。
通过特征融合,我们可以将不同模态的数据转化为相同的表示形式,方便后续的分析和挖掘。
其次,另一种常见的多模态数据分析方法是基于关联分析的方法。
在这种方法中,我们寻找不同模态数据之间的关联关系。
例如,我们可以分析图像和文本数据之间的关联关系,以发现图像中的物体与文本描述之间的对应关系。
这种关联分析可以帮助我们更好地理解数据,发现数据中的语义信息。
此外,还有一种常见的多模态数据分析方法是基于深度学习的方法。
深度学习是一种强大的机器学习方法,可以用于多模态数据的分析。
通过深度学习,我们可以建立复杂的模型来处理多模态数据。
例如,我们可以使用循环神经网络(RNN)来处理序列数据,使用生成对抗网络(GAN)来进行图像生成和识别。
深度学习的方法可以帮助我们更好地挖掘多模态数据中的信息。
除了以上介绍的方法,还有许多其他的多模态数据分析方法,如基于图模型的方法、基于聚类的方法等。
这些方法都在不同的场景中发挥着重要的作用,帮助我们更好地理解和利用多模态数据。
蚁群优化算法优化支持向量机的视频分类

蚁群优化算法优化支持向量机的视频分类蚁群优化算法(Ant Colony Optimization, ACO)是一种模拟蚁群觅食行为的启发式算法,它由意大利学者Dorigo等人于1992年提出。
蚁群优化算法是一种基于群体智能的算法,通过模拟蚂蚁在寻找食物时的行为方式,来解决组合优化问题。
蚁群优化算法的核心思想是通过蚂蚁在寻找食物时释放信息素的方式来引导其他蚂蚁找到最优解。
支持向量机(Support Vector Machine, SVM)是一种广泛应用于模式识别、数据挖掘和机器学习领域的监督学习算法。
SVM以最大化分类间隔为目标,将数据映射到高维空间中,通过构建一个最优超平面来实现数据的分类。
视频分类是指将视频数据按照一定的规则或者特征进行分类,以便于后续的检索、管理和分析。
视频分类在视频内容检索、视频监控、视频编辑和视频推荐等方面有着广泛的应用。
本文将介绍蚁群优化算法在优化支持向量机用于视频分类中的应用。
将介绍视频分类领域的研究现状,然后介绍蚁群优化算法和支持向量机算法的原理,最后具体分析蚁群优化算法优化支持向量机用于视频分类的应用。
一、视频分类研究现状随着互联网技术的不断发展,视频数据呈现出爆炸式增长的趋势,视频分类作为对视频数据进行处理和管理的重要手段,受到了广泛关注。
目前,视频分类主要分为基于内容的视频分类和基于行为的视频分类两大类。
基于内容的视频分类主要是根据视频的内容特征对视频进行分类,如颜色、纹理、运动特征等。
这种方法通常需要使用图像处理技术和机器学习算法来提取视频的特征,并进行分类。
目前,视频分类技术面临的主要问题包括特征提取的难度、特征表达的复杂性和分类准确度的提升。
为了解决这些问题,研究人员将一些优化算法引入到视频分类中,以提高分类准确度和效率。
二、蚁群优化算法的原理与应用蚁群优化算法是一种基于蚂蚁在寻找食物时释放信息素的方式来引导其他蚂蚁找到最优解的启发式算法。
蚂蚁在寻找食物时会释放信息素,并且路径上的信息素浓度会影响其他蚂蚁的选择。
多模态大模型构建方法
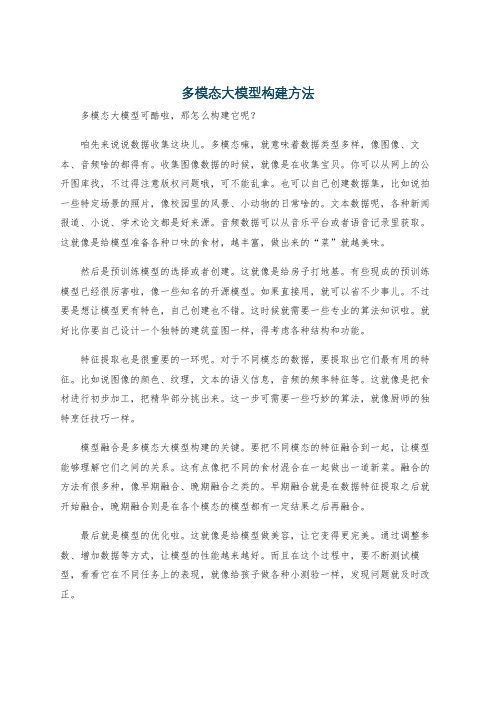
多模态大模型构建方法多模态大模型可酷啦,那怎么构建它呢?咱先来说说数据收集这块儿。
多模态嘛,就意味着数据类型多样,像图像、文本、音频啥的都得有。
收集图像数据的时候,就像是在收集宝贝。
你可以从网上的公开图库找,不过得注意版权问题哦,可不能乱拿。
也可以自己创建数据集,比如说拍一些特定场景的照片,像校园里的风景、小动物的日常啥的。
文本数据呢,各种新闻报道、小说、学术论文都是好来源。
音频数据可以从音乐平台或者语音记录里获取。
这就像是给模型准备各种口味的食材,越丰富,做出来的“菜”就越美味。
然后是预训练模型的选择或者创建。
这就像是给房子打地基。
有些现成的预训练模型已经很厉害啦,像一些知名的开源模型。
如果直接用,就可以省不少事儿。
不过要是想让模型更有特色,自己创建也不错。
这时候就需要一些专业的算法知识啦。
就好比你要自己设计一个独特的建筑蓝图一样,得考虑各种结构和功能。
特征提取也是很重要的一环呢。
对于不同模态的数据,要提取出它们最有用的特征。
比如说图像的颜色、纹理,文本的语义信息,音频的频率特征等。
这就像是把食材进行初步加工,把精华部分挑出来。
这一步可需要一些巧妙的算法,就像厨师的独特烹饪技巧一样。
模型融合是多模态大模型构建的关键。
要把不同模态的特征融合到一起,让模型能够理解它们之间的关系。
这有点像把不同的食材混合在一起做出一道新菜。
融合的方法有很多种,像早期融合、晚期融合之类的。
早期融合就是在数据特征提取之后就开始融合,晚期融合则是在各个模态的模型都有一定结果之后再融合。
最后就是模型的优化啦。
这就像是给模型做美容,让它变得更完美。
通过调整参数、增加数据等方式,让模型的性能越来越好。
而且在这个过程中,要不断测试模型,看看它在不同任务上的表现,就像给孩子做各种小测验一样,发现问题就及时改正。
多模态大模型的构建就像是一场奇妙的冒险,每一步都充满了挑战和乐趣。
只要用心去做,就能构建出一个超棒的多模态大模型啦。
多模态目标检测研究综述
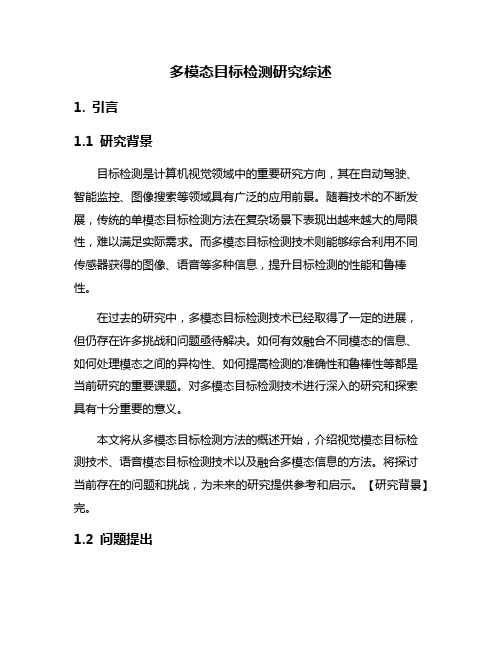
多模态目标检测研究综述1. 引言1.1 研究背景目标检测是计算机视觉领域中的重要研究方向,其在自动驾驶、智能监控、图像搜索等领域具有广泛的应用前景。
随着技术的不断发展,传统的单模态目标检测方法在复杂场景下表现出越来越大的局限性,难以满足实际需求。
而多模态目标检测技术则能够综合利用不同传感器获得的图像、语音等多种信息,提升目标检测的性能和鲁棒性。
在过去的研究中,多模态目标检测技术已经取得了一定的进展,但仍存在许多挑战和问题亟待解决。
如何有效融合不同模态的信息、如何处理模态之间的异构性、如何提高检测的准确性和鲁棒性等都是当前研究的重要课题。
对多模态目标检测技术进行深入的研究和探索具有十分重要的意义。
本文将从多模态目标检测方法的概述开始,介绍视觉模态目标检测技术、语音模态目标检测技术以及融合多模态信息的方法。
将探讨当前存在的问题和挑战,为未来的研究提供参考和启示。
【研究背景】完。
1.2 问题提出在现实生活和工程应用中,多模态目标检测技术具有重要的应用价值和研究意义。
传统的单模态目标检测方法存在着一些问题和局限性,如在检测复杂场景下的性能不稳定性、对特定模态数据的过度依赖、难以实现跨模态信息的融合等。
如何有效地融合多模态信息,提高目标检测的准确性和稳定性,成为当前研究中亟待解决的问题之一。
针对多模态目标检测中存在的问题和挑战,研究人员需要不断探索和创新,以提出更加有效和高效的多模态目标检测方法,从而推动该领域的发展和进步。
1.3 研究意义多模态目标检测是目标检测领域的一个重要研究方向,其在实际应用中具有重要意义。
多模态目标检测可以将不同模态的信息进行融合,提高目标检测的准确性和鲁棒性。
通过结合视觉和语音等多种信息,可以更加全面地理解目标,从而实现更加精准的检测和识别。
多模态目标检测也可以应用于智能监控、智能交通等领域,帮助提升系统的自主性和智能性。
深入研究多模态目标检测技术对于推动人工智能技术的发展具有重要的意义。
英语作文阅读方式的改变

英语作文阅读方式的改变English Response:The way we read in English has undergone a profound transformation in recent years. From traditional paper books to the advent of digital devices, the methods we employ to consume written content have evolved significantly. This shift has had a multifaceted impact on our reading habits, shaping how we interact with text, acquire information, and engage with literature.Technological Advancements:The most notable change has been the proliferation of digital technologies. E-books, tablets, and smartphones have replaced physical books for many readers, offering a portable and convenient way to access a vast repository of texts. The ease of highlighting, annotating, and searching within digital documents has enhanced the reading experience, making it more interactive and efficient.Multimodal Reading:The digital realm has also introduced the concept of multimodal reading. Modern texts often incorporate multimedia elements such as images, videos, and interactive graphics. This blended approach enhances comprehension and engages readers' multiple senses. By presenting information in different formats, multimodal texts cater to diverse learning styles and make reading more accessible.Social Media and Collaborative Reading:The rise of social media has fostered a culture of collaborative reading. Platforms like Goodreads and BookTok allow readers to connect with others who share similar interests, discuss books, and participate in online book clubs. This social aspect fosters a sense of community and can motivate readers to engage more deeply with texts.Shorter Attention Spans:While technology has provided numerous benefits, it has also posed some challenges. The constant stream of information and the immediacy of digital communication have shortened our attention spans. This can make it difficult for readers to focus on longer, more complex texts.Adaptive Reading:To address this issue, adaptive reading tools have emerged. These technologies adjust the difficulty level of texts based on the reader's comprehension and preferences. This personalized approach helps improve reading fluency and comprehension, particularly for struggling readers.Implications for Education:The changing landscape of English reading has significant implications for education. Educators must adapt their teaching methods to cater to the evolving reading habits of students. This includes incorporating multimodal texts, leveraging technology, and promoting collaborative reading experiences.Conclusion:The way we read in English has undergone a profound transformation, driven by technological advancements and the rise of digital media. These changes have both enhanced and challenged our reading practices, requiring us to navigate a diverse and ever-evolving landscape of written content. As we continue to explore the possibilities of digital reading, we must remain mindful of the challenges it presents and adapt our educational approaches accordingly.中文回答:英语阅读方式的改变。
多模态数据处理方法在人工智能中的应用
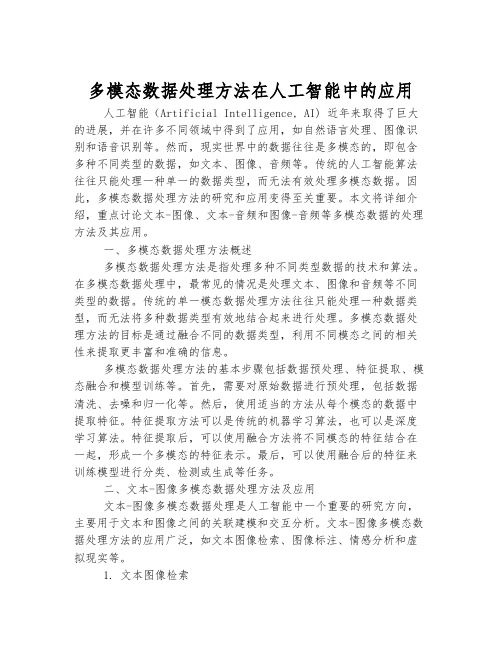
多模态数据处理方法在人工智能中的应用人工智能(Artificial Intelligence, AI) 近年来取得了巨大的进展,并在许多不同领域中得到了应用,如自然语言处理、图像识别和语音识别等。
然而,现实世界中的数据往往是多模态的,即包含多种不同类型的数据,如文本、图像、音频等。
传统的人工智能算法往往只能处理一种单一的数据类型,而无法有效处理多模态数据。
因此,多模态数据处理方法的研究和应用变得至关重要。
本文将详细介绍,重点讨论文本-图像、文本-音频和图像-音频等多模态数据的处理方法及其应用。
一、多模态数据处理方法概述多模态数据处理方法是指处理多种不同类型数据的技术和算法。
在多模态数据处理中,最常见的情况是处理文本、图像和音频等不同类型的数据。
传统的单一模态数据处理方法往往只能处理一种数据类型,而无法将多种数据类型有效地结合起来进行处理。
多模态数据处理方法的目标是通过融合不同的数据类型,利用不同模态之间的相关性来提取更丰富和准确的信息。
多模态数据处理方法的基本步骤包括数据预处理、特征提取、模态融合和模型训练等。
首先,需要对原始数据进行预处理,包括数据清洗、去噪和归一化等。
然后,使用适当的方法从每个模态的数据中提取特征。
特征提取方法可以是传统的机器学习算法,也可以是深度学习算法。
特征提取后,可以使用融合方法将不同模态的特征结合在一起,形成一个多模态的特征表示。
最后,可以使用融合后的特征来训练模型进行分类、检测或生成等任务。
二、文本-图像多模态数据处理方法及应用文本-图像多模态数据处理是人工智能中一个重要的研究方向,主要用于文本和图像之间的关联建模和交互分析。
文本-图像多模态数据处理方法的应用广泛,如文本图像检索、图像标注、情感分析和虚拟现实等。
1. 文本图像检索文本图像检索是通过输入文本查询来检索相关的图像。
传统的基于文本的图像检索方法往往只考虑文本的语义信息,而忽略了图像的特征。
多模态文本-图像检索方法结合文本和图像的特征,能够提供更准确和丰富的检索结果。
机器学习技术中的多模态数据处理方法

机器学习技术中的多模态数据处理方法随着技术的不断发展,我们现在可以轻松地从各种来源获取到多模态数据,这些数据包括文本、图像、声音、视频等不同形式和类型的信息。
然而,这种多模态数据的复杂性也给机器学习任务带来了挑战。
为了更好地应对这些挑战,研究人员们提出了一系列多模态数据处理方法,以实现更准确和全面的数据分析和学习。
一种常见的多模态数据处理方法是融合。
融合可以将不同模态的数据合并起来,形成更具信息丰富性的数据表示。
对于文本和图像的多模态数据,常见的融合方法是将文本特征和图像特征连接在一起,形成一个更大的特征向量。
这种融合方法可以在同一模型中同时处理文本和图像信息,使得模型能够更好地理解数据。
另一种常见的多模态数据处理方法是对齐。
对齐方法旨在将不同模态数据的表示空间映射到同一空间中,使得它们之间具有可比性。
例如,对于图像和声音的多模态数据,可以使用神经网络将它们分别映射到一个共享的隐空间中,使得它们之间的相似性能够得到衡量和比较。
通过对齐,可以更好地利用不同模态数据的互补性,提高机器学习任务的性能。
此外,还有一些特定领域的多模态数据处理方法。
例如,在医学图像诊断中,常常需要结合图像和医疗知识,利用多模态数据提高疾病诊断的准确性。
这种方法通常包括先利用图像数据进行特征提取,然后将提取的特征与医学知识进行融合。
这种多模态数据处理方法在医学领域具有很高的应用价值,可以帮助医生进行更准确的诊断和治疗。
在使用多模态数据处理方法时,还需要考虑数据集的标注和对齐。
数据集的标注可以提供更准确的监督信号,进而提高机器学习任务的性能。
而数据的对齐可以保证不同模态数据之间的一致性,使得模型能够更好地从中学习到有用的特征。
总之,在机器学习技术中,多模态数据处理方法可以帮助我们更好地利用多种类型的信息,提高数据分析和学习的准确性和全面性。
无论是通过融合、对齐还是特定领域的方法,多模态数据处理方法都具有广泛的应用前景,并在许多领域取得了令人瞩目的成果。
《2024年应用语言学研究的多模态分析方法》范文

《应用语言学研究的多模态分析方法》篇一一、引言随着科技的发展和信息社会的进步,语言的使用形式和交流方式也在不断变化。
在这样的大背景下,应用语言学研究愈发重要,其旨在探讨语言在不同社会、文化、科技背景下的应用与变化。
多模态分析方法作为一种新兴的研究手段,为应用语言学研究提供了新的视角和方法。
本文将详细介绍应用语言学研究中的多模态分析方法,并探讨其在实际研究中的应用。
二、多模态分析方法的定义及特点多模态分析方法是一种综合运用语言学、心理学、计算机科学等多个学科理论,以多媒体资源为研究对象的分析方法。
该方法注重从文字、图像、声音、视频等多种信息模式出发,分析语言在多模态环境中的使用方式和特征。
其特点在于综合性强、信息量大、分析维度丰富。
三、多模态分析方法在应用语言学研究中的应用1. 语言教学研究:多模态分析方法可以用于研究语言教学中的教学方法、教学资源以及学生的学习方式。
例如,通过分析多媒体教学资源(如视频、图片等)在语言教学中的作用,探讨其对学生语言学习效果的影响。
2. 跨文化交际研究:多模态分析方法可以用于研究不同文化背景下语言的交际方式和特征。
通过分析不同文化背景下的语言使用方式、语言交际行为以及交际过程中使用的多种符号资源,揭示不同文化背景下的语言交际差异和特点。
3. 媒体语言研究:在媒体语言研究中,多模态分析方法可用于分析媒体语言的多模态性、传播效果及媒体话语的社会影响等。
通过综合运用文字、图像、声音等资源,分析媒体文本在不同媒介平台上的传播方式和效果,揭示媒体话语的内在逻辑和价值取向。
4. 语言与认知研究:多模态分析方法还可以用于研究语言与认知的关系。
通过分析人们在多模态环境中的认知过程和认知方式,探讨语言在认知过程中的作用和影响,揭示人类认知的多样性和复杂性。
四、多模态分析方法的应用步骤1. 确定研究问题:明确研究目标和研究问题,确定研究范围和研究对象。
2. 收集数据:收集相关多媒体资源,包括文字、图像、声音、视频等。
面向人工智能的多模态数据处理与分析
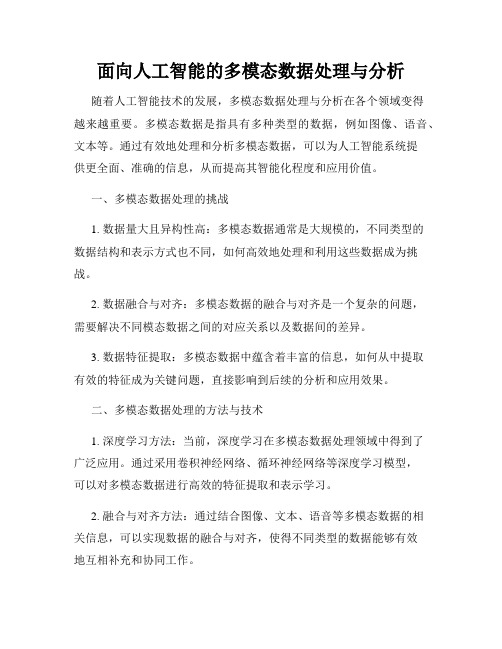
面向人工智能的多模态数据处理与分析随着人工智能技术的发展,多模态数据处理与分析在各个领域变得越来越重要。
多模态数据是指具有多种类型的数据,例如图像、语音、文本等。
通过有效地处理和分析多模态数据,可以为人工智能系统提供更全面、准确的信息,从而提高其智能化程度和应用价值。
一、多模态数据处理的挑战1. 数据量大且异构性高:多模态数据通常是大规模的,不同类型的数据结构和表示方式也不同,如何高效地处理和利用这些数据成为挑战。
2. 数据融合与对齐:多模态数据的融合与对齐是一个复杂的问题,需要解决不同模态数据之间的对应关系以及数据间的差异。
3. 数据特征提取:多模态数据中蕴含着丰富的信息,如何从中提取有效的特征成为关键问题,直接影响到后续的分析和应用效果。
二、多模态数据处理的方法与技术1. 深度学习方法:当前,深度学习在多模态数据处理领域中得到了广泛应用。
通过采用卷积神经网络、循环神经网络等深度学习模型,可以对多模态数据进行高效的特征提取和表示学习。
2. 融合与对齐方法:通过结合图像、文本、语音等多模态数据的相关信息,可以实现数据的融合与对齐,使得不同类型的数据能够有效地互相补充和协同工作。
3. 增强学习方法:基于增强学习的方法可以在多模态数据处理中完成智能化的决策和控制。
通过建立适应性的决策模型,可以使多模态数据处理系统具备自主学习和优化能力。
三、多模态数据分析的应用领域1. 计算机视觉:多模态数据处理可以应用于图像识别、目标检测、图像分割等计算机视觉任务中,提高图像处理的准确性和效率。
2. 自然语言处理:通过将文本与图像、语音等多模态数据进行结合,可以实现更准确、全面的自然语言理解和生成,例如智能问答系统、机器翻译等。
3. 语音与音频处理:多模态数据处理可以应用于语音识别、情感分析、音乐生成等领域,为语音与音频信号的处理和分析提供更丰富的信息。
4. 医疗与健康:多模态数据处理可以应用于医学图像分析、器官自动识别、疾病预测等任务,为医疗领域提供更准确、可靠的辅助决策。
数据科学中的多模态数据集成与分析研究
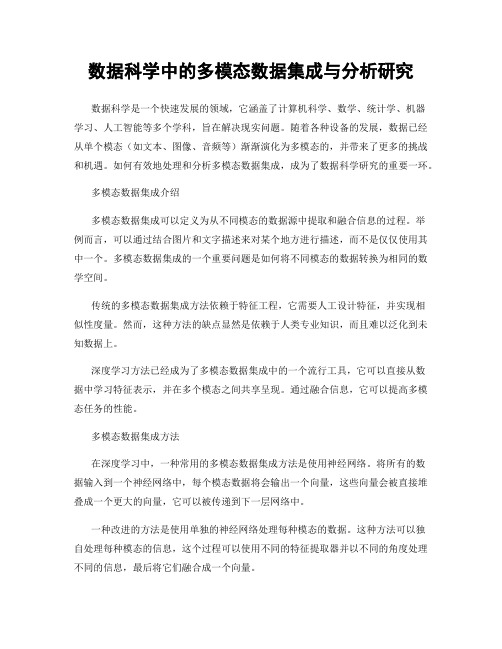
数据科学中的多模态数据集成与分析研究数据科学是一个快速发展的领域,它涵盖了计算机科学、数学、统计学、机器学习、人工智能等多个学科,旨在解决现实问题。
随着各种设备的发展,数据已经从单个模态(如文本、图像、音频等)渐渐演化为多模态的,并带来了更多的挑战和机遇。
如何有效地处理和分析多模态数据集成,成为了数据科学研究的重要一环。
多模态数据集成介绍多模态数据集成可以定义为从不同模态的数据源中提取和融合信息的过程。
举例而言,可以通过结合图片和文字描述来对某个地方进行描述,而不是仅仅使用其中一个。
多模态数据集成的一个重要问题是如何将不同模态的数据转换为相同的数学空间。
传统的多模态数据集成方法依赖于特征工程,它需要人工设计特征,并实现相似性度量。
然而,这种方法的缺点显然是依赖于人类专业知识,而且难以泛化到未知数据上。
深度学习方法已经成为了多模态数据集成中的一个流行工具,它可以直接从数据中学习特征表示,并在多个模态之间共享呈现。
通过融合信息,它可以提高多模态任务的性能。
多模态数据集成方法在深度学习中,一种常用的多模态数据集成方法是使用神经网络。
将所有的数据输入到一个神经网络中,每个模态数据将会输出一个向量,这些向量会被直接堆叠成一个更大的向量,它可以被传递到下一层网络中。
一种改进的方法是使用单独的神经网络处理每种模态的数据。
这种方法可以独自处理每种模态的信息,这个过程可以使用不同的特征提取器并以不同的角度处理不同的信息,最后将它们融合成一个向量。
另一种改进的方法是使用门控神经网络,利用门控机制来控制具有不同重要性的模态。
通过门控单元,它可以将某些模态数据忽略或增强。
这种方法能够处理没有特定映射的模态数据,从而避免了特征工程的紧缺问题。
多模态数据集成应用多模态数据集成应用广泛,其中一大类是语音识别和语音相关任务。
语音信号通常由音频和文本组成,现有的技术可以将音频信号转换成文本,以便于人们进行搜索和理解。
此外,这项技术也可以用于语音情感识别、语音转换、语音合成等多个领域。
AI变现策略中的多模态数据处理与分析方法
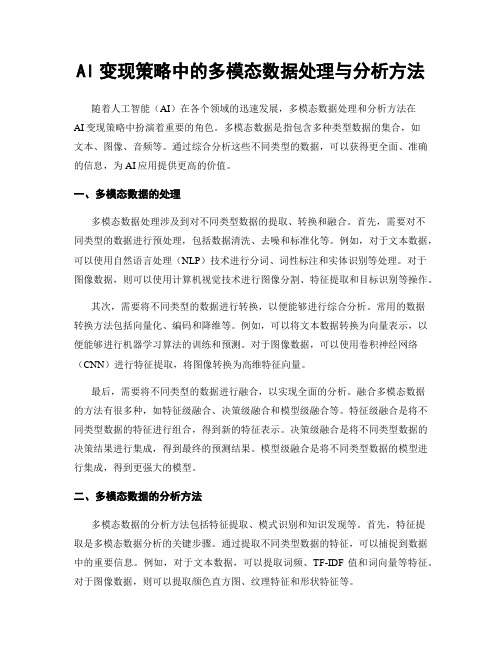
AI变现策略中的多模态数据处理与分析方法随着人工智能(AI)在各个领域的迅速发展,多模态数据处理和分析方法在AI变现策略中扮演着重要的角色。
多模态数据是指包含多种类型数据的集合,如文本、图像、音频等。
通过综合分析这些不同类型的数据,可以获得更全面、准确的信息,为AI应用提供更高的价值。
一、多模态数据的处理多模态数据处理涉及到对不同类型数据的提取、转换和融合。
首先,需要对不同类型的数据进行预处理,包括数据清洗、去噪和标准化等。
例如,对于文本数据,可以使用自然语言处理(NLP)技术进行分词、词性标注和实体识别等处理。
对于图像数据,则可以使用计算机视觉技术进行图像分割、特征提取和目标识别等操作。
其次,需要将不同类型的数据进行转换,以便能够进行综合分析。
常用的数据转换方法包括向量化、编码和降维等。
例如,可以将文本数据转换为向量表示,以便能够进行机器学习算法的训练和预测。
对于图像数据,可以使用卷积神经网络(CNN)进行特征提取,将图像转换为高维特征向量。
最后,需要将不同类型的数据进行融合,以实现全面的分析。
融合多模态数据的方法有很多种,如特征级融合、决策级融合和模型级融合等。
特征级融合是将不同类型数据的特征进行组合,得到新的特征表示。
决策级融合是将不同类型数据的决策结果进行集成,得到最终的预测结果。
模型级融合是将不同类型数据的模型进行集成,得到更强大的模型。
二、多模态数据的分析方法多模态数据的分析方法包括特征提取、模式识别和知识发现等。
首先,特征提取是多模态数据分析的关键步骤。
通过提取不同类型数据的特征,可以捕捉到数据中的重要信息。
例如,对于文本数据,可以提取词频、TF-IDF值和词向量等特征。
对于图像数据,则可以提取颜色直方图、纹理特征和形状特征等。
其次,模式识别是多模态数据分析的核心任务。
模式识别是指从多模态数据中发现隐藏的模式和规律。
常用的模式识别方法包括聚类、分类和回归等。
聚类是将数据分成不同的组别,使得组内的数据相似度最大化,组间的数据相似度最小化。
多模态多目标智能优化算法及其应用研究
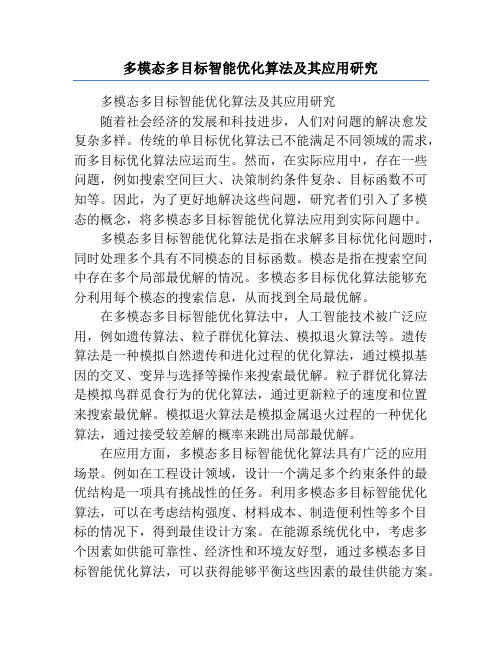
多模态多目标智能优化算法及其应用研究多模态多目标智能优化算法及其应用研究随着社会经济的发展和科技进步,人们对问题的解决愈发复杂多样。
传统的单目标优化算法已不能满足不同领域的需求,而多目标优化算法应运而生。
然而,在实际应用中,存在一些问题,例如搜索空间巨大、决策制约条件复杂、目标函数不可知等。
因此,为了更好地解决这些问题,研究者们引入了多模态的概念,将多模态多目标智能优化算法应用到实际问题中。
多模态多目标智能优化算法是指在求解多目标优化问题时,同时处理多个具有不同模态的目标函数。
模态是指在搜索空间中存在多个局部最优解的情况。
多模态多目标优化算法能够充分利用每个模态的搜索信息,从而找到全局最优解。
在多模态多目标智能优化算法中,人工智能技术被广泛应用,例如遗传算法、粒子群优化算法、模拟退火算法等。
遗传算法是一种模拟自然遗传和进化过程的优化算法,通过模拟基因的交叉、变异与选择等操作来搜索最优解。
粒子群优化算法是模拟鸟群觅食行为的优化算法,通过更新粒子的速度和位置来搜索最优解。
模拟退火算法是模拟金属退火过程的一种优化算法,通过接受较差解的概率来跳出局部最优解。
在应用方面,多模态多目标智能优化算法具有广泛的应用场景。
例如在工程设计领域,设计一个满足多个约束条件的最优结构是一项具有挑战性的任务。
利用多模态多目标智能优化算法,可以在考虑结构强度、材料成本、制造便利性等多个目标的情况下,得到最佳设计方案。
在能源系统优化中,考虑多个因素如供能可靠性、经济性和环境友好型,通过多模态多目标智能优化算法,可以获得能够平衡这些因素的最佳供能方案。
在金融投资领域,多模态多目标智能优化算法可以帮助投资者找到符合风险偏好和收益预期的最佳投资组合。
尽管多模态多目标智能优化算法在实际应用中取得了显著的成效,但仍存在一些挑战。
首先,针对具体问题选择适合的多模态多目标智能优化算法是一个难题,需要根据问题特点进行针对性选择。
其次,参数调节也是一个关键问题,不同参数设置可能导致算法性能的差异。
AI技术如何处理多模态数据

AI技术如何处理多模态数据随着科技的不断发展,人工智能(AI)技术在各个领域的应用越来越广泛。
其中,处理多模态数据是一个备受关注的领域。
多模态数据指的是包含不同类型信息的数据,比如图像、文本、语音等。
如何高效地处理这些数据,成为了AI技术发展的重要课题。
首先,AI技术需要能够识别和提取多模态数据中的不同信息。
对于图像数据,计算机视觉技术可以帮助识别物体、场景等。
通过深度学习算法,计算机可以学习到图像的特征,并进行分类、检测等任务。
对于文本数据,自然语言处理技术可以帮助计算机理解和分析文本内容。
通过文本挖掘、情感分析等技术,计算机可以从文本中提取有用的信息。
对于语音数据,语音识别技术可以将语音转换为文本或命令。
通过声学模型和语言模型的结合,计算机可以准确地识别语音内容。
其次,AI技术需要能够将不同类型的数据进行融合和关联。
多模态数据的处理不仅仅是单独处理每种类型的数据,更重要的是将不同类型的数据进行融合和关联,以获取更全面和准确的信息。
例如,在图像和文本数据的处理中,可以将图像的特征与文本的语义进行融合,以提高图像分类、图像生成等任务的性能。
在语音和文本数据的处理中,可以将语音转换为文本后,再与文本数据进行关联分析,以实现更复杂的语义理解和交互。
此外,AI技术还需要能够处理多模态数据之间的时序关系。
多模态数据往往包含了时间上的变化和顺序信息。
例如,视频数据是由一系列图像帧组成的,音频数据是由一系列声音片段组成的。
AI技术需要能够对这些时序数据进行建模和分析,以实现动作识别、语音合成等任务。
通过时序建模,计算机可以捕捉到不同模态数据之间的时序关系,并进行合理的推理和预测。
最后,AI技术还需要能够处理多模态数据的不确定性和噪声。
多模态数据往往包含各种噪声和不确定性,如图像中的遮挡、文本中的歧义、语音中的背景噪声等。
AI技术需要具备对不确定性进行建模和处理的能力,以提高系统的鲁棒性和可靠性。
通过概率模型和统计方法,计算机可以对多模态数据的不确定性进行建模,并进行合理的推断和决策。
- 1、下载文档前请自行甄别文档内容的完整性,平台不提供额外的编辑、内容补充、找答案等附加服务。
- 2、"仅部分预览"的文档,不可在线预览部分如存在完整性等问题,可反馈申请退款(可完整预览的文档不适用该条件!)。
- 3、如文档侵犯您的权益,请联系客服反馈,我们会尽快为您处理(人工客服工作时间:9:00-18:30)。
Annotating Multi-media / Multi-modal resources with ELANHennie Brugman, Albert RusselMax-Planck-Institute for PsycholinguisticsWundtlaan 1, 6525 XD Nijmegen{Hennie.Brugman, Albert.Russel}@mpi.nlAbstractThis paper shows the actual state of development of the manual annotation tool ELAN. It presents usage requirements from three different groups of users and how one annotation model and a number of generic design principles guided the choices made during the development process of ELAN.IntroductionAt the Max-Planck-Institute for Psycholinguistics1 (MPI) software development on annotation tools for the manual annotation of multimedia data has been going on since the early 90’s. Over this decade there have been large changes in enabling technology and insights in the nature of linguistic annotation. Media frameworks for the handling of digital audio and especially digital video files have matured, as has media streaming technology. XML has come to existence and has become highly relevant in a short time. Rendering and input of Unicode characters is now commonplace.Simultaneously, users made experiences with the first generation of video annotation tools and became aware of and got used to these new technologies. From this a new set of requirements arose.Finally, annotation tool builders are better aware of each other’s approaches, annotation models and annotation document formats. Clearly convergence is going on, leading to easier exchange of data between annotation tools. An important role in this process was played by the paper by (Bird & Liberman, 2001) that introduced Annotation Graphs. We are closely watching and trying to participate in standards initiatives, as for example ISO TC37/SC4.The first video annotation tool developed at the MPI was MediaTagger, a QuickTime based application that runs only on pre-OS X Macintoshes. It started as a first attempt to exploit the QuickTime Movie data structure, and especially it’s text tracks, as an informal model for linguistic annotation. Since then several new formal models where made, each one building on the experiences of the previous ones and considering new user requirements. The formal modeling languages that were used are Entity-Relationship diagrams and UML. A detailed presentation and evaluation of these models can be found in (Brugman & Wittenburg, 2001).The next chapters will discuss the requirements of several different groups of users and describe the latest state of ELAN functionality. We will then present our model for annotation in some detail and show how we can cover the needs of very different user groups with one relatively simple model. In the discussions plans for future development will presented.1 http://www.mpi.nlUser requirementsELAN is developed with a number of different user groups in mind. These users are situated both within the MPI and, in an increasing number of cases, outside the MPI. Often they are participating in externally funded projects (DoBeS2, ECHO3). We will discuss the main requirements per group, although there is of course a substantial overlap between each group’s needs.Linguistic researchFor many linguists one of the first steps in their research is the creation of an orthographic or phonetic transcription of some recorded event or experiment. In an iterative process they add more and more analytic layers of annotation to this transcription. These additional layers typically do not annotate the primary (speech) signal anymore, but refer to previously added annotations.Layers that are added later are typically connected to already existing layers in increasingly complex referential structures. Orthography or phonetic transcription is linked directly to media time intervals in the primary signal utterance-by-utterance or phrase-by-phrase. Words are either ordered decompositions of these utterances, or are linked to media time themselves. Morphemes are ordered sequences of annotations that are symbolically linked to words. Part-of-speech annotations can refer to either words or morphemes. Structurally more complex annotation layers are recursive trees (syntax), non-contiguous annotations (co-reference), or annotations that refer to other annotations across several layers (general comments). All of these structural requirements are covered by a relatively simple and elegant annotation model called Abstract Corpus Model (ACM). ACM will be discussed in more detail in a next chapter.An additional requirement from linguists is support for import and export of legacy annotation formats, the most important ones being the Childes format CHAT (MacWhinney) and Shoebox4.With respect to searching, linguists are typically interested in locating patterns on specific tiers, with the possibility to relate different patterns by means of a distance specified in milliseconds or in number of annotations on some tier. Results can be visualized in the context of their containing documents or in concordance-like representations, or they2 http://www.mpi.nl/DOBES3 http://www.mpi.nl/ECHO4 can be the input for modules that calculate specific statistical or linguistic measures.Documentation of Endangered LanguagesOne of the main application areas for ELAN is the documentation of endangered languages, both by MPI researchers and by field teams participating in the DoBeS project (Dokumentation Bedrohter Sprachen – Documentation of Endangered Languages), funded by the Volkswagen Stiftung5.Since one of the main components of language documentation is the result of linguistic research, all linguistic requirements hold here as well. With respect to complex annotation structures this is illustrated very clearly by the Advanced Glossing proposal (Drude & Lieb, 2002) that was made in the context of the DoBeS program. More than in the case of general linguistics, the support for entry and rendering of Unicode characters is required.With respect to legacy formats interlinear text6 in a rangeof document formats and with a number of proprietary and often undocumented conventions is widely used. Conversion of such texts to archival formats is required. For a good description of requirements for archive formats, see (Bird & Simons, 2003).For a complete language documentation of some linguistic event or text it is also necessary to document used terminology (such as for example used tag sets) in an archival format.Other important products of language documentation are lexica. The existence of lexica imposes additional requirements on ELAN. First, users want to add lexicon entries from the context of an annotation document, second, they want to add annotations on basis of consultation of a lexicon, third, they want to jump to instances of lexicon entries in an annotated corpus, fourth, they want to jump to a lexicon entry from an annotation, fifth, they want to use a lexicon for the formulation or execution of queries on annotated corpora.Finally, next to linguistic work, there is a large cultural and ethnological component to documentation of especially endangered languages. Good examples are the widely felt need for annotations of music, dance, rituals, etc in such a way that they can be inspected and analyzedin relation to linguistic annotations.Gesture and sign language researchAt MPI and the University of Nijmegen (UN) we are faced with the following studies that push the requirements for an efficient framework for manually creating multimodal annotations (only some will be mentioned here):- Gesture studies where gestures in various contexts and from various cultural backgroundsare compared.- Multimodal interaction studies where the precise timing between the speech and gesture modalitiesare analyzed to distinguish production models.5 http://www.volkswagen-stiftung.de6 Widely used in field linguistics. Typically blocks of text with parallel lines, where association of tokens across lines is represented by vertical text alignment- Studies where the different types of gestures used in minority languages are analyzed (Enfield,2002).- Studies where the differences between several European sign languages are analyzed (Crasborn,2003).- Studies where the differences between sign languages world wide are analyzed (Zeshan,2004).In these types of studies often many different annotation layers are needed, for example to annotate different articulators. We have seen cases of up to 50 layers. These layers are either completely independent with respect to their time alignment, or they can be explicitly dependent. Since there can be so many layers, often associated with controlled vocabularies, it is required that complete specifications for such tier setups can be made available in repositories for re-use.Because gesture research and sign language annotation is mainly based on video recordings and because it is concerned with details on a very short time scale, there are high demands on video handling. Synchronized playback of multiple video recordings of the same event is necessary, MPEG2 support and video zooming are desirable, video frame accurate annotation is a necessity.A highly desirable feature is the support for the annotation of spatial regions of the video signal during some time interval, for example to mark relevant locations or areas, or trajectories over time.For gesture and sign language studies it is sometimes required that other types of media than video and audio can be visualized and used as the basis for annotation. Examples are eye tracking or data glove time series. It is necessary that each of those signals can be visualized using a time axis that is shared with audio and annotation data.Collaborative annotationA problem that all user groups share is that they want to collaborate on annotation projects from different geographical locations. ELAN is therefore in the process of being extended to support peer-to-peer cooperation. A group of users can share an annotation document, potentially including streamed video and audio data, during a working session. Users can chat, they can point at elements, times and locations in the document viewers and they can propose and commit changes to the document. All of this is propagated instantly to all participants using peer-to-peer technology7.This is more thoroughly discussed in (Brugman, Crasborn, Russel, 2004)ELAN’s main functionsFor the design of ELAN a number of guiding principles are used:- As is common practice in software engineering, representations of annotation structures on thescreen or on print are decoupled fromrepresentations used for persistence, documentexchange or searching.7 Implementation is done using JXTA, - Several alternative viewers on the same underlying annotation data are supported. Eachviewer is optimized to support certain tasks.- All viewers are synchronized with respect to media time, selected time interval and activeannotation. Modifications can be made in eachviewer and show up in all other viewersinstantaneously.Figure 1: Screenshot of a document opened in ELAN 2.0- We try to impose as few restrictions on user’s annotation projects as possible. Numbers andtypes of annotation layers are thereforeunrestricted and user definable. Time alignmenton different tiers can be completely independent,or made dependent at the user’s choice.- As much information as possible is represented explicitly. We try to avoid implicit representationof annotation data such as codes that areembedded within annotation values, structurethat is encoded by text alignment on a page or bythe hierarchical structure of XML documents.- We adhere to principles of stand-off annotation in the sense that different layers of annotation arekept separate (but not necessarily in differentfiles).- As much as possible, we adhere to standards, as for example Unicode.Figure 1 shows a multi-layer annotation document opened in ELAN. ELAN, the displayed document and ELAN sources can be downloaded from the MPI tools website8.8 http://www.mpi.nl/tools ELAN’s document window shows several different panels or viewers, most of which are optional and can be detached as a separate window from the main window.The upper left viewer shows the video signal making use of either the Java Media Framework (JMF) on Windows or QuickTime on Macintosh. When the video viewer is detached it can be scaled, for example to show the full resolution of MPEG-2. Two video viewers can be used toshow two video signals that arerecorded in sync. To the right of thevideo viewer a number of alternativeannotation viewers can be madevisible using tab panes. The Gridviewer shows a clickable list ofannotations on a chosen tier withtheir begin and end times anddurations, the Text viewer shows allannotation values on a chosen tier asrunning text. It is also clickable,editable, selectable and showscurrent media time. The Subtitlepanel shows up to four selectabletiers as video subtitles that playalong with media time. The controltab contains sliders of play back rateand audio volume.The button panel shows groups ofbuttons for play/pause and steppingthrough time with several step sizes,for operating on the time selectionand for jumping from annotation toannotation.An annotation density viewer showswhere annotations exist between thebeginning and end of the document’smedia files. A wave form panelshows sample data for mono orstereo speech.In the bottom panel two alternative annotation viewers can be shown: the Timeline viewer and the Interlinear viewer. The Timeline viewer shows annotations for each tier as a time segment with a text label. Black segments represent annotations that are or can be aligned with media time. Yellow segments represent annotations that refer to other annotations. Their begin and end times are derived from their parent annotation’s begin and end times. Annotation tiers can be made visible or invisible, or can be reordered with a simple drag-and-drop operation. The Interlinear viewer (not shown) shows groups of hierarchically connected annotations as interlinear text.New documents are created by selecting one or more media files and, optionally, defining their time origins. The next step is to define Linguistic Types for annotation tiers. Such a definition specifies the semantics of annotation values, whether annotations are time alignable or refer to other annotations, and which constraints hold on annotation values or on structural connections with other annotations (see chapter on ACM ).Then Tiers can be defined and associated with Linguistic Types. Tiers can be independent or be connected to a parent tier. Annotations can now be created on each tier by simple user operations, taking constraints into account. ELAN’s user interface can be localized on the fly byselecting a language from a menu.Abstract Corpus ModelFigure 2: class diagram of the core part of the AbstractCorpus ModelFigure 2 shows the core part of the ACM. Tiers are containers for annotations. Annotations associated with these tiers have one of two reference types: (1) Annotations can be aligned with media time and then have a begin and end represented by a TimeSlot, or (2) they refer to one or several other annotations. TimeSlots can be explicitly aligned with media time but do not have to be. However, all TimeSlots are explicitly ordered within the AnnotationDocument by means of a TimeOrder.Finally, Tiers are associated with LinguisticTypes that can in turn be associated with Constraints. By implementing stereotypic sets of constraints in program code annotations can be connected in complex patterns. A number of these stereotypes are already implemented:- Time subdivision: annotations on a dependenttier are all within the time interval of an annotation on the parent tier, and between annotations with the same parent tiers no time gaps are allowed (example: gestures can be decomposed into separate gesture phases).- Symbolic subdivision: annotations on adependent tier refer to annotations on a parent tier. Annotations that point to the same parent annotation are explicitly ordered (example: words can be decomposed into morphemes).- Symbolic association: there is a 1-1 relationbetween a dependent annotation and it’s parent annotation (example: all cases where annotations can have some attribute value, like part-of-speech on a word or morpheme)A few other stereotypes are planned to be implemented:- Annotations on a dependent tier can refer to oneor more annotations on a specific parent tier. These parent annotations do not have to be consecutive. This stereotype can be used to model for example co-reference chains.- Dependent annotations can refer to one or moreannotations on the same tier and on a specific parent tier. This makes recursive trees, like syntax trees, representable.Using these basic elements and stereotypes it is possible torepresent very complex annotation documents. In the area of Endangered Languages it would for example be possible to combine time aligned phonetic transcriptions for several speakers with interlinear text analysis, and with gesture and musical annotation. Comments could be attached to combinations of annotations on any of these tiers.ConclusionAlthough the growth of ELAN’s user base confronted us with diverging requirements, careful modeling and using a set of proven design principles for annotation tools helped us cope with that. ELAN development is now in a state that allows straightforward expansion to cover new user needs.Moreover, the latest developments and insights on annotation tools, formats and standards seem to converge. Work on ELAN is consistent with this convergence, and we hope that it is actually contributing to it.ReferencesS. Bird and M. Liberman. 2001. A formal framework for linguistic annotation. Speech Communication, 33(1,2):23-60.S. Bird and G. Simons. 2003. Seven dimensions of portability for language documentation and description. In Bojan Petek (ed.), Portability issues in human language technologies: LREC 2002H. Brugman and P. Wittenburg. 2001. The application of annotation models for the construction of databases and tools. IRCS Workshop on Linguistic Databases, University of Pennsylvania. Philadelphia.H. Brugman, O. Crasborn and A. Russel. 2004. Collaborative annotation of sign language data with peer-to-peer technology. To be published. LREC 2004.O. Crasborn. 2003. Internal ECHO report (to be published). Nijmegen.S. Drude and H. Lieb. 2002. Advanced Glossing – a language documentation format and its implementation with Shoebox. International Workshop in Field Linguistics at LREC 2002. Las Palmas.N. Enfield. 2002. Hand pointing in Laos: form and function in a locality description task. MPI Annual Report 2002. Nijmegen.B. MacWhinney. CHILDES. U. Zeshan. 2004. Sign Language Typology Project (to be published). Nijmegen.。