In Vitro Phototoxicity Testing Development and
中国体外诊断技术发展的历史进程

·633· E-mail:zgqkyx@·方案·指南·新进展·中国体外诊断技术发展的历史进程邢菁华【摘要】 中国体外诊断技术的历史变迁自新中国成立之日起,已经走过六十多个年头。
中国体外诊断技术的发展经历了从经典手工、作坊式设备,到标准化、智能化、自动化管理的全过程,在不同时代有其不同特征。
体外诊断在学科理念上也从“医学检验”到“检验医学”发生着重大转变,并为临床提供重要的病理、生理信息,是患者诊疗过程中的重要组成部分。
【关键词】 诊断技术和方法;中国;体外诊断【中图分类号】 R446.9 【文献标识码】 A DOI :10.3969/j.issn.1007-9572.2017.00.105邢菁华.中国体外诊断技术发展的历史进程[J ].中国全科医学,2018,21(6):633-637.[ ]XING J H.Historical development of in vitro diagnostics technology in China [J ].Chinese General Practice ,2018,21(6):633-637.Historical Development of In Vitro Diagnostics Technology in China XING Jing-hua Institute of Medical Humanities, Peking University, Beijing 100191,China【Abstract 】 Since the founding of new China, the development of in vitro diagnostics technology in China has been more than 60 years from the original manual method to the whole process of standardization, intelligent, automated management with different characteristics in different times. The discipline concept of in vitro diagnostics changes from medical examination to laboratory medicine providing clinically significant pathological and physiological information, which is an important part of thediagnosis and treatment of patients.【Key words 】 Diagnostic techniques and procedures; China; In vitro diagnostic100191北京市,北京大学医学人文研究院随着中国经济的快速增长,体外诊断技术也在不断地发展与创新。
3T3细胞中性红摄取光毒性试验方法研究进展
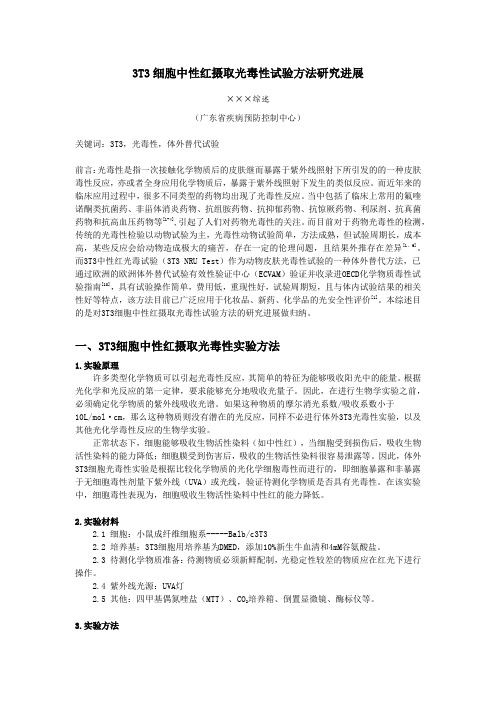
3T3细胞中性红摄取光毒性试验方法研究进展×××综述(广东省疾病预防控制中心)关键词:3T3,光毒性,体外替代试验前言:光毒性是指一次接触化学物质后的皮肤继而暴露于紫外线照射下所引发的的一种皮肤毒性反应,亦或者全身应用化学物质后,暴露于紫外线照射下发生的类似反应。
而近年来的临床应用过程中,很多不同类型的药物均出现了光毒性反应。
当中包括了临床上常用的氟喹诺酮类抗菌药、非甾体消炎药物、抗组胺药物、抗抑郁药物、抗惊厥药物、利尿剂、抗真菌药物和抗高血压药物等[1-4],引起了人们对药物光毒性的关注。
而目前对于药物光毒性的检测,传统的光毒性检验以动物试验为主,光毒性动物试验简单,方法成熟,但试验周期长,成本高,某些反应会给动物造成极大的痛苦,存在一定的伦理问题,且结果外推存在差异[1,9]。
而3T3中性红光毒试验(3T3 NRU Test)作为动物皮肤光毒性试验的一种体外替代方法,已通过欧洲的欧洲体外替代试验有效性验证中心(ECVAM)验证并收录进OECD化学物质毒性试验指南[10],具有试验操作简单,费用低,重现性好,试验周期短,且与体内试验结果的相关性好等特点,该方法目前已广泛应用于化妆品、新药、化学品的光安全性评价[1]。
本综述目的是对3T3细胞中性红摄取光毒性试验方法的研究进展做归纳。
一、3T3细胞中性红摄取光毒性实验方法1.实验原理许多类型化学物质可以引起光毒性反应,其简单的特征为能够吸收阳光中的能量。
根据光化学和光反应的第一定律,要求能够充分地吸收光量子。
因此,在进行生物学实验之前,必须确定化学物质的紫外线吸收光谱。
如果这种物质的摩尔消光系数/吸收系数小于10L/mol·cm,那么这种物质则没有潜在的光反应,同样不必进行体外3T3光毒性实验,以及其他光化学毒性反应的生物学实验。
正常状态下,细胞能够吸收生物活性染料(如中性红),当细胞受到损伤后,吸收生物活性染料的能力降低;细胞膜受到伤害后,吸收的生物活性染料很容易泄露等。
三维荧光光谱结合二阶校正法测定保健品中的褪黑素
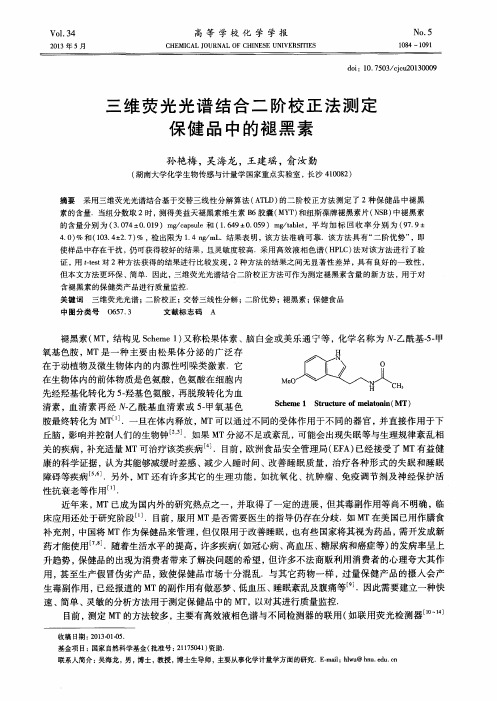
M
在生物体内的前体物质是色氨酸, 色氨酸在细胞 内 先经羟基化转化为 5 一 羟基色氨酸 , 再脱羧转化为血 清素 , 血清素再经 Ⅳ 一 乙酰基血清素或 5 - 甲氧基色
S c h e me 1 S t r u c t u r e o f me l a t o n i n ( M T)
褪 黑素 ( M T, 结 构见 S c h e m e 1 ) 又称松 果体 素 、 脑 白金 或美 乐 通 宁等 ,化学 名 称 为 Ⅳ 一 乙酰 基一 5 - 甲 氧基 色胺 , MT是一 种 主要 由松 果 体 分 泌 的 广 泛存 在 于 动植物 及微 生物 体 内的 内源性 吲 哚类 激素 .它
胺最终转化为 M T 1 j .一旦在体 内释放 , M T可以通过不同的受体作用于不 同的器官, 并直接作用于下 丘脑 , 影 响并 控制 人们 的生 物钟 I 3 J .如果 M T分 泌不足 或紊 乱 , 可能会 出现失 眠等与 生理 规律紊 乱 相
关的疾病 , 补充适量 M T可治疗该类疾病 J .目 前, 欧洲食品安全管理局( E F A ) 已经接受了 M T有益健 康的科学证据 , 认为其能够减缓时差感 、 减少入睡时间、 改善睡眠质量 , 治疗各种形式 的失眠和睡眠 障 碍等疾 病 J .另外 ,M T还有 许多 其它 的生 理 功 能 ,如 抗 氧化 、抗肿 瘤 、免疫 调 节剂 及 神 经保 护 活
性 抗衰 老等作 用 .
近年来 , M T已成为国内外的研究热点之一 , 并取得了一定的进展 , 但其毒副作用等 尚不 明确 , 临
床 应 用还处 于研 究 阶段 … .目前 , 服用 M T是否 需要 医生 的指导 仍存 在分 歧.如 M T在 美 国已用作膳 食
A Unique Approach to Development of Near-Infrared Fluorescent Sensors for in Vivo Imaging
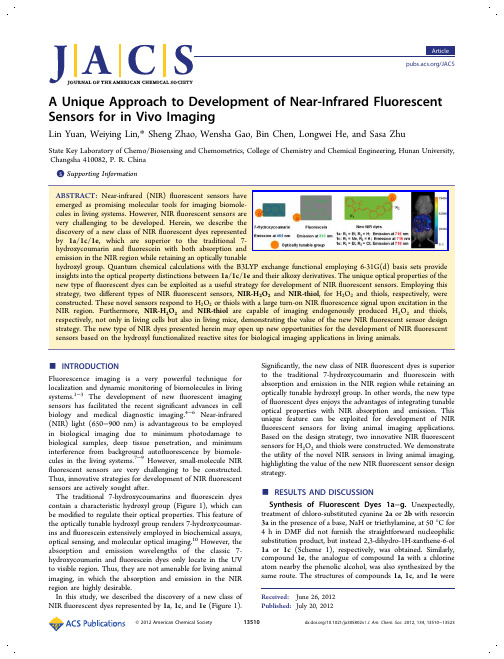
Article
Figure 1. The chemical structures of the classic and new dyes bear an optically tunable hydroxyl group. The chemical structures and absorption/ emission wavelengths of the classic dyes, such as 7-hydroxycoumarin and fluorescein, and the new NIR dyes reported herein (represented by 1a, 1c, and 1e) with an optically tunable hydroxyl group.
S Supporting Information *
ABSTRACT: Near-infrared (NIR) fluorescent sensors have emerged as promising molecular tools for imaging biomolecules in living systems. However, NIR fluorescent sensors are very challenging to be developed. Herein, we describe the discovery of a new class of NIR fluorescent dyes represented by 1a/1c/1e, which are superior to the traditional 7hydroxycoumarin and fluorescein with both absorption and emission in the NIR region while retaining an optically tunable hydroxyl group. Quantum chemical calculations with the B3LYP exchange functional employing 6-31G(d) basis sets provide insights into the optical property distinctions between 1a/1c/1e and their alkoxy derivatives. The unique optical properties of the new type of fluorescent dyes can be exploited as a useful strategy for development of NIR fluorescent sensors. Employing this strategy, two different types of NIR fluorescent sensors, NIR-H2O2 and NIR-thiol, for H2O2 and thiols, respectively, were constructed. These novel sensors respond to H2O2 or thiols with a large turn-on NIR fluorescence signal upon excitation in the NIR region. Furthermore, NIR-H2O2 and NIR-thiol are capable of imaging endogenously produced H2O2 and thiols, respectively, not only in living cells but also in living mice, demonstrating the value of the new NIR fluorescent sensor design strategy. The new type of NIR dyes presented herein may open up new opportunities for the development of NIR fluorescent sensors based on the hydroxyl functionalized reactive sites for biological imaging applications in living animals.
皮肤光变态反应试验SkinPhotoallergyTest-中国食品药品检定研究院
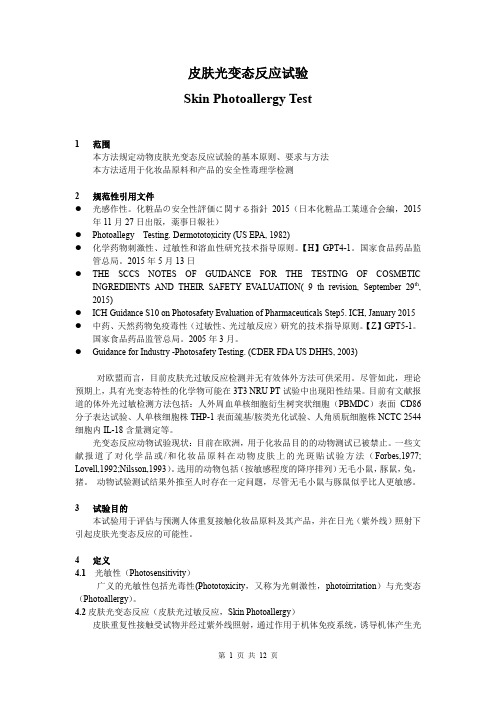
皮肤光变态反应试验Skin Photoallergy Test1范围本方法规定动物皮肤光变态反应试验的基本原则、要求与方法本方法适用于化妆品原料和产品的安全性毒理学检测2规范性引用文件●光感作性。
化粧品の安全性評価に関する指針2015(日本化粧品工業連合会編,2015年11月27日出版,薬事日報社)●Photoallegy Testing. Dermototoxicity (US EPA, 1982)●化学药物刺激性、过敏性和溶血性研究技术指导原则。
【H】GPT4-1。
国家食品药品监管总局。
2015年5月13日●THE SCCS NOTES OF GUIDANCE FOR THE TESTING OF COSMETICINGREDIENTS AND THEIR SAFETY EV ALUATION( 9 th revision, September 29th, 2015)●ICH Guidance S10 on Photosafety Evaluation of Pharmaceuticals Step5. ICH, January 2015 ●中药、天然药物免疫毒性(过敏性、光过敏反应)研究的技术指导原则。
【Z】GPT5-1。
国家食品药品监管总局。
2005年3月。
●Guidance for Industry -Photosafety Testing. (CDER FDA US DHHS, 2003)对欧盟而言,目前皮肤光过敏反应检测并无有效体外方法可供采用。
尽管如此,理论预期上,具有光变态特性的化学物可能在3T3 NRU PT试验中出现阳性结果。
目前有文献报道的体外光过敏检测方法包括:人外周血单核细胞衍生树突状细胞(PBMDC)表面CD86分子表达试验、人单核细胞株THP-1表面巯基/胺类光化试验、人角质朊细胞株NCTC 2544 细胞内IL-18含量测定等。
光变态反应动物试验现状:目前在欧洲,用于化妆品目的的动物测试已被禁止。
化妆品安全评价指南说明书

1. Introduction
Safety assurances for quasi-drugs and cosmetics pertain not only to the safety of the individual ingredients that the products consist of but also to such aspects as the use of the products and the sites on the body where they are to be applied. In this chapter, we look first at the basic thinking behind ensuring the safety of existing ingredients and new ingredients (hereinafter "functional ingredients") whose efficacy has been evaluated in terms of their anti-aging, whitening, and sunscreen functions by means of guidelines; and second, we discuss additional guidelines for checking the safety of preparations containing functional ingredients.
OECD Guidelines
*conduct in case concern exists in metaboli1; safety
2. Evaluating the Safety of Functional Ingredients
银杏肽锌螯合物的制备、体外消化及抗氧化活性分析
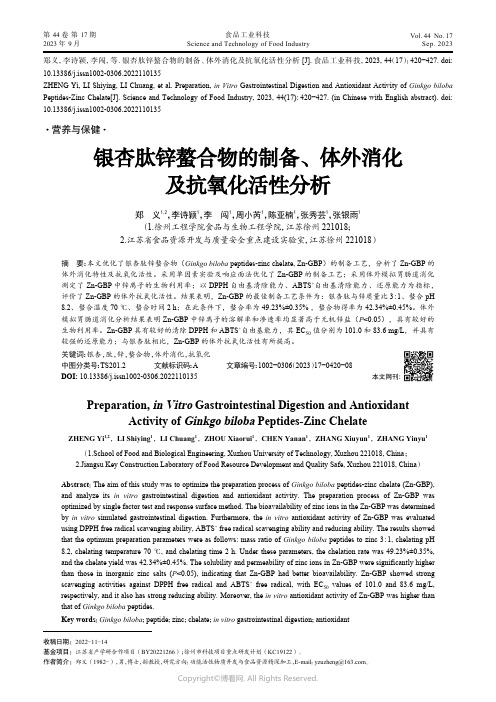
郑义,李诗颖,李闯,等. 银杏肽锌螯合物的制备、体外消化及抗氧化活性分析[J]. 食品工业科技,2023,44(17):420−427. doi:10.13386/j.issn1002-0306.2022110135ZHENG Yi, LI Shiying, LI Chuang, et al. Preparation, in Vitro Gastrointestinal Digestion and Antioxidant Activity of Ginkgo biloba Peptides-Zinc Chelate[J]. Science and Technology of Food Industry, 2023, 44(17): 420−427. (in Chinese with English abstract). doi:10.13386/j.issn1002-0306.2022110135· 营养与保健 ·银杏肽锌螯合物的制备、体外消化及抗氧化活性分析郑 义1,2,李诗颖1,李 闯1,周小芮1,陈亚楠1,张秀芸1,张银雨1(1.徐州工程学院食品与生物工程学院,江苏徐州 221018;2.江苏省食品资源开发与质量安全重点建设实验室,江苏徐州 221018)摘 要:本文优化了银杏肽锌螯合物(Ginkgo biloba peptides-zinc chelate, Zn-GBP )的制备工艺,分析了Zn-GBP 的体外消化特性及抗氧化活性。
采用单因素实验及响应面法优化了Zn-GBP 的制备工艺;采用体外模拟胃肠道消化测定了Zn-GBP 中锌离子的生物利用率;以DPPH 自由基清除能力、ABTS +自由基清除能力、还原能力为指标,评价了Zn-GBP 的体外抗氧化活性。
结果表明,Zn-GBP 的最佳制备工艺条件为:银杏肽与锌质量比3:1、螯合pH 8.2、螯合温度70 ℃、螯合时间2 h ;在此条件下,螯合率为49.23%±0.35% ,螯合物得率为42.34%±0.45%。
活体成像在药物研发研究领域的应用

PerkinElmer 小动物活体光学成像技术已在生命科学基础研究、临床前医学研究及药物研发等领域得到广泛应用。
药物研发是科研机构和医药公司的重点研究领域。
在活体光学成像实验中,常用于药物研发的方法包括:1.使用构建好的生物发光转基因疾病动物模型,应用小动物活体光学成像技术观测给药后疾病信号的改变,从而评价药物对疾病的治疗效果。
2.通过注射功能性荧光探针,观测疾病发展过程中分子事件,从而反映药物对疾病的治疗效果。
下面结合一些具体实例阐述应用小动物活体光学成像技术进行药物研发的七个方面:1.抗肿瘤癌症药物研发。
2. 关节炎治疗药物研发。
3. 感染性疾病的药物研发。
4. 抗炎症的药物研发。
5. 抗病毒药物的研发。
6.神经系统疾病的药物治疗。
7.构建新型老鼠模型。
一.抗肿瘤癌症药物研发肝细胞性肝癌是肝癌的主要形式,而且每年死亡多达598,000人以上。
索拉非尼(Sorafenib )是一种合成的多酪氨酸激酶抑制剂,而且用于治疗30%的肝细胞性肝癌患者和肝硬化,因此研究人员开发其他类型的药物用于治疗肝癌患者。
表观遗传变换能够引发肝癌和促进肿瘤的发展,表观遗传变换而且是药物可逆的,因此转录后调控可用于肝癌的治疗。
DNA 异常甲基化是早期和晚期肿瘤癌症的重要事件,因此研究异常甲基化对于癌症风险性评估,治疗和化学预防都有积极的作用。
抑制DNA 甲基转移酶1(DNMT1,DNA methyltransferase 1) 能够再激活表观遗传沉默的肿瘤抑制基因,抑制肿瘤细胞生长,而且促进细胞分化,凋亡和提高免疫监督。
研究者在免疫缺陷的小鼠(NOD/SCID )脾内移植萤火虫荧光素酶标记的敏感人肝癌肿瘤细胞株(Huh7-luc ),从而建立人异种移植肝细胞性肝癌肿瘤模型。
使用PerkinElmer 的IVIS 系统成像显示小鼠服用低毒性的Zebularine (第二代稳定的亲水性DNA 甲基转移酶1抑制剂)后,生物发光强度明显降低(下图)。
体外3T3细胞光毒性中性红摄取法评价化妆品的光毒性作用
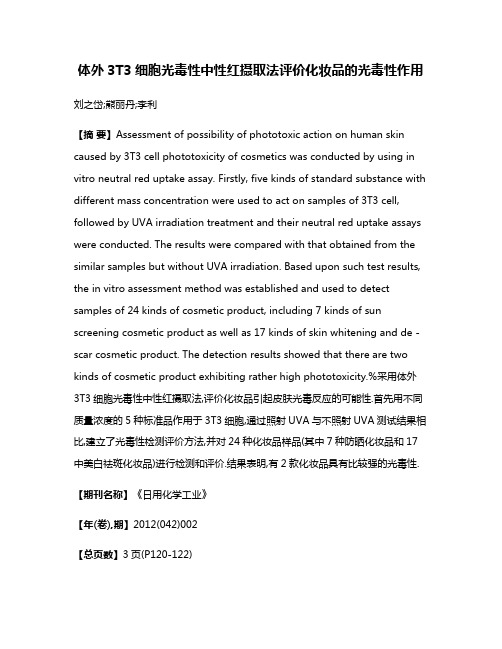
体外3T3细胞光毒性中性红摄取法评价化妆品的光毒性作用刘之岱;熊丽丹;李利【摘要】Assessment of possibility of phototoxic action on human skin caused by 3T3 cell phototoxicity of cosmetics was conducted by using in vitro neutral red uptake assay. Firstly, five kinds of standard substance with different mass concentration were used to act on samples of 3T3 cell, followed by UVA irradiation treatment and their neutral red uptake assays were conducted. The results were compared with that obtained from the similar samples but without UVA irradiation. Based upon such test results, the in vitro assessment method was established and used to detect samples of 24 kinds of cosmetic product, including 7 kinds of sun screening cosmetic product as well as 17 kinds of skin whitening and de - scar cosmetic product. The detection results showed that there are two kinds of cosmetic product exhibiting rather high phototoxicity.%采用体外3T3细胞光毒性中性红摄取法,评价化妆品引起皮肤光毒反应的可能性.首先用不同质量浓度的5种标准品作用于3T3细胞,通过照射UVA与不照射UVA测试结果相比,建立了光毒性检测评价方法,并对24种化妆品样品(其中7种防晒化妆品和17中美白祛斑化妆品)进行检测和评价.结果表明,有2款化妆品具有比较强的光毒性.【期刊名称】《日用化学工业》【年(卷),期】2012(042)002【总页数】3页(P120-122)【关键词】化妆品;光毒性;体外替代法;3T3细胞;中性红【作者】刘之岱;熊丽丹;李利【作者单位】四川化妆品工程技术研究中心,四川成都 610041;四川华肤生物科技有限责任公司,四川成都610041;四川化妆品工程技术研究中心,四川成都 610041;四川华肤生物科技有限责任公司,四川成都610041;四川化妆品工程技术研究中心,四川成都 610041;四川大学华西医院皮肤科,四川成都 610041【正文语种】中文【中图分类】TQ658光毒性是指皮肤接触化学物质继而暴露于紫外线照射下所引发的一种皮肤毒性反应,或者全身使用化学物质后,暴露于紫外线照射下发生的类似反应[1]。
体外诊断领域的英文综述

体外诊断领域的英文综述The field of in vitro diagnostics, commonly referred to as IVD, encompasses a wide range of medical tests and devices that are performed outside of the body to aid in the diagnosis, monitoring, and management of various diseases and conditions. In vitro diagnostics include tests conducted on samples such as blood, urine, saliva, and tissue, and are essential for providing accurate and timely information to healthcare professionals.In recent years, there have been significant advancements in the field of in vitro diagnostics, with the development of new technologies and the introduction of innovative testing methods. These advancements have led to improvements in the accuracy, speed, and reliability of diagnostic tests, ultimately enhancing patient care and outcomes.One key area of focus in the field of in vitro diagnostics is the development of point-of-care testing,which allows for rapid and convenient testing at the bedside or in other clinical settings. This has the potential to improve patient management by enabling quick decision-making and timely initiation of treatment.Furthermore, the integration of molecular diagnostics into in vitro testing has revolutionized the way certain diseases are diagnosed and managed. Techniques such as polymerase chain reaction (PCR) and next-generation sequencing have enabled the detection of genetic and molecular markers, leading to more personalized and targeted treatment approaches.In addition, the field of in vitro diagnostics plays a crucial role in infectious disease testing, cancer screening, and monitoring of chronic conditions such as diabetes and cardiovascular disease. The continuous evolution of diagnostic technologies and the increasing availability of biomarkers and novel testing platforms are driving the expansion and diversification of in vitro diagnostics.Overall, the field of in vitro diagnostics continues to be a dynamic and rapidly evolving area of healthcare, with ongoing research and development efforts aimed at further enhancing the performance and utility of diagnostic tests for the benefit of patients and healthcare providers.。
小鼠光学相干断层扫描(oct)过程

小鼠光学相干断层扫描(oct)过程全文共四篇示例,供读者参考第一篇示例:小鼠光学相干断层扫描(OCT)是一种非侵入性的成像技术,可以用来观察和诊断小鼠眼部结构。
这种技术利用光学描记扫描的原理,通过测量光学延迟和幅度来获取组织的结构和信息。
OCT成像具有高分辨率、高速度和高灵敏度的优点,能够实时监测小鼠视网膜和表面结构的变化,为研究小鼠眼部疾病提供重要的数据支持。
小鼠光学相干断层扫描的过程首先需要将小鼠固定在扫描台上,然后用眼科专用的透明凝胶或者透明夹板固定小鼠的眼睛,确保眼睛处于最佳成像位置。
接下来,利用OCT设备的扫描头对小鼠眼睛进行扫描,获取眼部结构的高分辨率图像。
在OCT扫描过程中,光源会产生一束光束,通过分束器将光束分为参考光束和探测光束。
参考光束会直接反射到参考镜上,而探测光束则会被聚焦到样本组织上,与组织相互作用后反射回来。
通过测量参考光束和探测光束的干涉信号,可以获取组织的反射率和位置信息,从而重建成像。
在小鼠OCT扫描中,需要对眼睛进行水平和垂直方向的扫描,以获取不同位置的眼部结构图像。
一般来说,OCT扫描会在几秒钟内完成,而且可以连续扫描多个位置,实时监测眼部结构的变化。
通过OCT扫描,可以观察到小鼠视网膜的各个层次结构,包括视网膜神经纤维层、视网膜色素上皮层、视网膜感光细胞层等。
这些结构的变化和异常往往与眼部疾病的发生和发展密切相关,因此OCT成像可以为小鼠眼部疾病的研究提供重要的帮助。
除了眼部结构的观察外,OCT技术还可用于观察小鼠眼部功能的变化。
可以利用OCT功能成像技术观察小鼠视网膜的血流速度、血管密度和血流动力学变化,为疾病的诊断和治疗提供参考依据。
第二篇示例:小鼠光学相干断层扫描(OCT)是一种非侵入性的图像技术,常用于研究小鼠眼部结构和病变。
OCT能够提供高分辨率的眼部断层图像,帮助研究人员观察和诊断眼部疾病。
本文将介绍小鼠光学相干断层扫描的原理、应用和操作过程。
利用体外3T3细胞中性红摄取试验检测化学物质光毒性作用
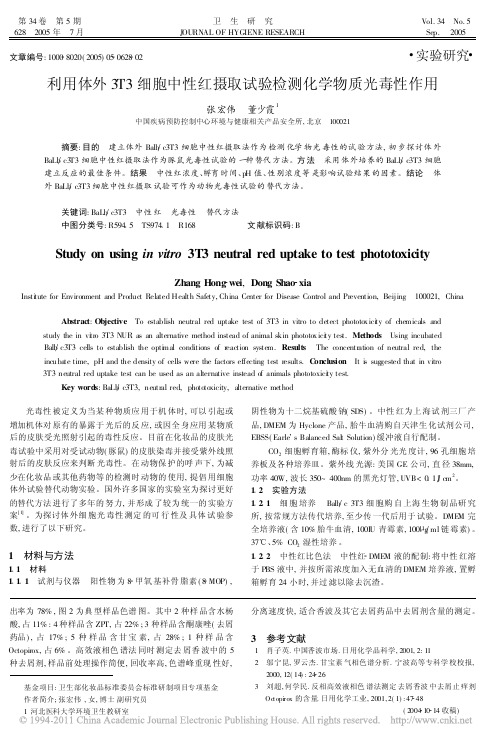
BaLb c3T3 细胞中性红摄取法作为豚鼠光毒性试验的一种替代 方法 。方 法 采用体外培养的 BaLb c3T3 细胞 建立反应的最佳条件 。 结果 中性红浓度 、 孵育时间 、 pH 值 、 性别浓度等是影响试验结果 的因素 。 结论 体 外 BaLb c3T3 细胞中性红摄取 试验可作为动物光毒性试验的替代方法 。 关键词 : BaLb c3T3 中性 红 光毒性 中图分类号 : R594 5 TS974 1 R168 替代方法 文 献标识码 : B
卫 生 研 究 JOURNAL OF HYGIENE RESEARCH
Vol. 34 No. 5 Sep. 2005
实验研究
利用体外 3T3 细胞中性红摄取试验检测化学物质光毒性作用
张宏伟 董少霞
1
中国疾病预防控制中心环境与健康相关产品安全所 , 北京
100021
摘要 : 目的
建立体外 Balb c3T3 细胞中性红摄取法作为 检测化 学物光 毒性的试 验方法 , 初 步探讨体 外
2 2
中性红最佳浓度的测定
由表 2 可见 , 在浓度超过 100 g ml 时 , 吸光度值增加 相对 较慢 , 并有较大的 A 值 , 故中性红的浓度可取 100 g ml。
表 2 不同浓度中性红与相应吸光度值 Table 2 Relationship of NRU oncentration and absorbance 浓度 ( g ml ) 吸光度 ( 平均值 ) 2 0 4 10 25 50 100 150 200 1 331
11218不同照射时间不同辐射量细胞活力的测定取常规培养的细胞14皿分为7组置于紫外线光源照射箱内分别照射102030506090120分钟用台盼兰染色计数法计数活细胞数并根据空白对照未照射的活细胞数来计算细胞活力
生物医学成像技术的最新进展
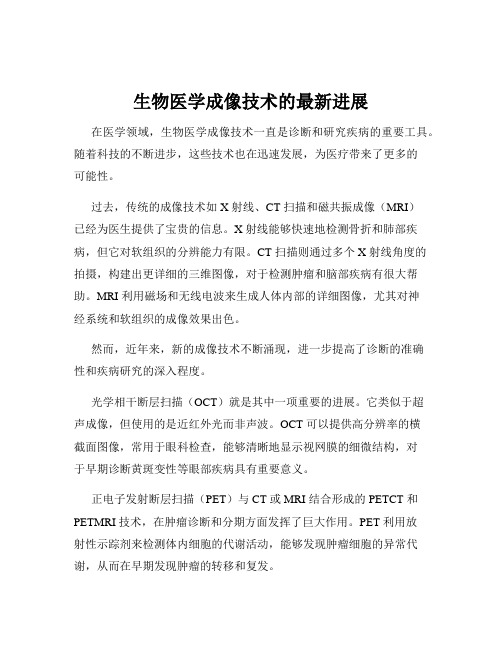
生物医学成像技术的最新进展在医学领域,生物医学成像技术一直是诊断和研究疾病的重要工具。
随着科技的不断进步,这些技术也在迅速发展,为医疗带来了更多的可能性。
过去,传统的成像技术如 X 射线、CT 扫描和磁共振成像(MRI)已经为医生提供了宝贵的信息。
X 射线能够快速地检测骨折和肺部疾病,但它对软组织的分辨能力有限。
CT 扫描则通过多个 X 射线角度的拍摄,构建出更详细的三维图像,对于检测肿瘤和脑部疾病有很大帮助。
MRI 利用磁场和无线电波来生成人体内部的详细图像,尤其对神经系统和软组织的成像效果出色。
然而,近年来,新的成像技术不断涌现,进一步提高了诊断的准确性和疾病研究的深入程度。
光学相干断层扫描(OCT)就是其中一项重要的进展。
它类似于超声成像,但使用的是近红外光而非声波。
OCT 可以提供高分辨率的横截面图像,常用于眼科检查,能够清晰地显示视网膜的细微结构,对于早期诊断黄斑变性等眼部疾病具有重要意义。
正电子发射断层扫描(PET)与 CT 或 MRI 结合形成的 PETCT 和PETMRI 技术,在肿瘤诊断和分期方面发挥了巨大作用。
PET 利用放射性示踪剂来检测体内细胞的代谢活动,能够发现肿瘤细胞的异常代谢,从而在早期发现肿瘤的转移和复发。
多光子显微镜技术是另一个令人瞩目的发展。
它能够在细胞和分子水平上进行活体成像,观察细胞的动态过程,如细胞内的信号传导和蛋白质相互作用。
这对于研究疾病的发病机制和药物的作用机制提供了直接的观察手段。
此外,超分辨率成像技术突破了传统光学显微镜的分辨率极限,使得我们能够看到更小的细胞结构和分子。
例如,受激发射损耗(STED)显微镜和单分子定位显微镜(SMLM)等技术,让科学家能够更深入地了解细胞内的生物过程。
在心血管疾病的诊断中,心脏磁共振成像(CMR)的技术不断改进。
新的序列和成像方法能够更准确地评估心脏的结构和功能,包括心肌的灌注和纤维化情况,对于心肌病和冠心病的诊断和治疗监测具有重要价值。
COVAFRESH IV 水性薄荷
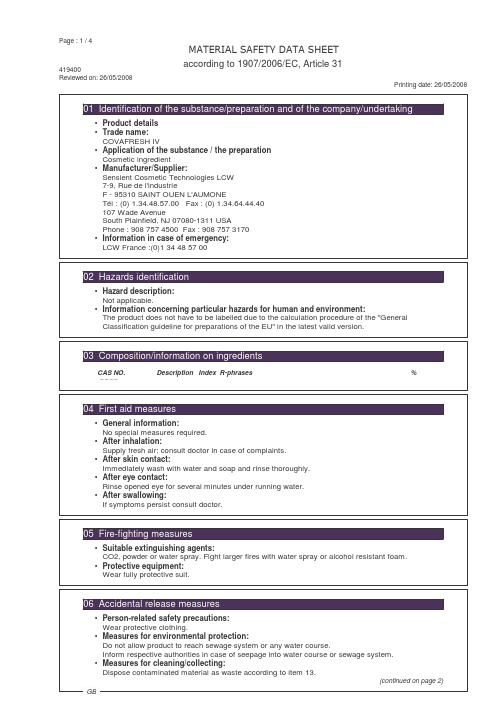
MATERIAL SAFETY DATA SHEET
419400 Reviewed on: 26/05/2008
according to 1907/2006/EC, Article 31
Printing date: 26/05/2008
01 Identification of the substance/preparation and of the company/undertaking
06 Accidental release measures
• Person-related safety precautions: Wear protective clothing. • Measures for environmental protection: Do not allow product to reach sewage system or any water course. Inform respective authorities in case of seepage into water course or sewage system. • Measures for cleaning/collecting: Dispose contaminated material as waste according to item 13.
• Handling: • Information for safe handling: Store in cool, dry place in tightly closed receptacles. Open and handle receptacle with care. Wear suitable respiratory protective device when decanting larger quantities without extractor facilities. Ensure good ventilation/exhaustion at the workplace. • Storage: Durée de vie / Shelf life : T° :<25° C 3 years Tout conditionnement ouvert doit être testé chaque année / After opening, product must be rechecked every year • Requirements to be met by storerooms and receptacles: Store only in unopened original receptacles. • Information about storage in one common storage facility: Not required. • Further information about storage conditions: Protect from exposure to the light. Caution when reopening receptacles with broken seal. Open receptacle only under localized extractor facilities. Store receptacle in a well ventilated area.
Biosensors and Bioelectronics

Biosensors and Bioelectronics Biosensors and bioelectronics have revolutionized the field of healthcare and biotechnology, offering innovative solutions for disease diagnosis, environmental monitoring, and drug development. These technologies have the potential to improve the quality of life for millions of people around the world by providing accurate and timely information about their health and the environment. However, there are also challenges and concerns associated with the widespread use of biosensors and bioelectronics, including ethical considerations, regulatory issues, and potential risks to privacy and security. From a healthcare perspective, biosensors and bioelectronics offer tremendous promise in terms of early disease detection and personalized medicine. These technologies can detect biomarkers and otherindicators of disease with high sensitivity and specificity, allowing for earlier intervention and more targeted treatment. For example, biosensors can be used to monitor glucose levels in diabetic patients, detect cancer biomarkers, andidentify infectious diseases. This can lead to improved patient outcomes and reduced healthcare costs, making biosensors and bioelectronics an invaluable tool for healthcare providers and patients alike. In addition to healthcare applications, biosensors and bioelectronics also have significant potential for environmental monitoring and protection. These technologies can detect pollutants, toxins, and other environmental hazards with high precision, providing valuable data for environmental scientists and policymakers. For example, biosensors can be used to monitor water quality, air pollution, and soil contamination, helping to identify sources of pollution and develop strategies for remediation. By providing real-time, actionable information, biosensors and bioelectronics can contribute to the preservation of natural resources and the protection of public health.Despite their numerous benefits, biosensors and bioelectronics also raise ethical and regulatory concerns that must be carefully considered. For example, the use of biosensors for genetic testing and personalized medicine raises questions about privacy, consent, and the potential for discrimination based on genetic information. Additionally, the development and commercialization of biosensors and bioelectronics require robust regulatory oversight to ensure their safety, effectiveness, and ethical use. This includes considerations such as data security,informed consent, and the responsible use of personal health information. Furthermore, the widespread adoption of biosensors and bioelectronics also raises concerns about data security and the potential for misuse of sensitive information. As these technologies generate large amounts of data about individuals' health and the environment, there is a risk of unauthorized access, data breaches, and misuse of personal information. This highlights the importance of implementing robustdata security measures, ethical guidelines, and regulatory frameworks to protect the privacy and security of individuals and communities. In conclusion,biosensors and bioelectronics offer tremendous potential for improving healthcare, environmental monitoring, and biotechnological research. These technologies have the capacity to revolutionize the way we diagnose and treat diseases, monitor the environment, and develop new drugs and therapies. However, it is crucial to address the ethical, regulatory, and security challenges associated with their widespread use. By considering these concerns and implementing appropriate safeguards, we can harness the full potential of biosensors and bioelectronics while ensuring the protection of individuals' privacy, security, and ethical rights.。
3T3细胞中性红摄取光毒性试验方法研究进展
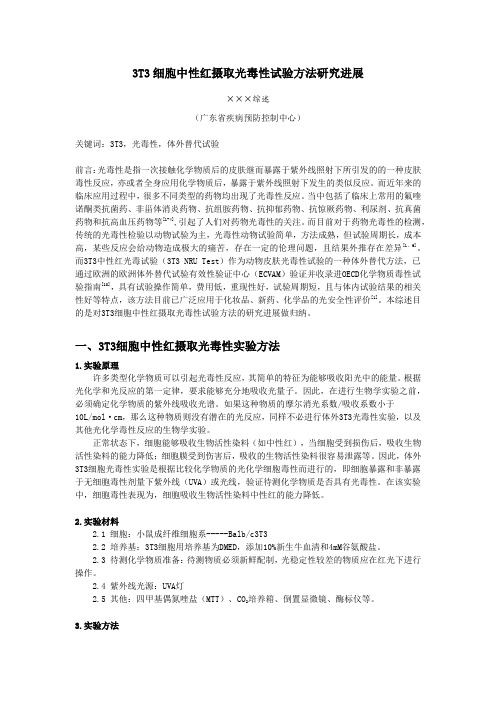
3T3细胞中性红摄取光毒性试验方法研究进展×××综述(广东省疾病预防控制中心)关键词:3T3,光毒性,体外替代试验前言:光毒性是指一次接触化学物质后的皮肤继而暴露于紫外线照射下所引发的的一种皮肤毒性反应,亦或者全身应用化学物质后,暴露于紫外线照射下发生的类似反应。
而近年来的临床应用过程中,很多不同类型的药物均出现了光毒性反应。
当中包括了临床上常用的氟喹诺酮类抗菌药、非甾体消炎药物、抗组胺药物、抗抑郁药物、抗惊厥药物、利尿剂、抗真菌药物和抗高血压药物等[1-4],引起了人们对药物光毒性的关注。
而目前对于药物光毒性的检测,传统的光毒性检验以动物试验为主,光毒性动物试验简单,方法成熟,但试验周期长,成本高,某些反应会给动物造成极大的痛苦,存在一定的伦理问题,且结果外推存在差异[1,9]。
而3T3中性红光毒试验(3T3 NRU Test)作为动物皮肤光毒性试验的一种体外替代方法,已通过欧洲的欧洲体外替代试验有效性验证中心(ECVAM)验证并收录进OECD化学物质毒性试验指南[10],具有试验操作简单,费用低,重现性好,试验周期短,且与体内试验结果的相关性好等特点,该方法目前已广泛应用于化妆品、新药、化学品的光安全性评价[1]。
本综述目的是对3T3细胞中性红摄取光毒性试验方法的研究进展做归纳。
一、3T3细胞中性红摄取光毒性实验方法1.实验原理许多类型化学物质可以引起光毒性反应,其简单的特征为能够吸收阳光中的能量。
根据光化学和光反应的第一定律,要求能够充分地吸收光量子。
因此,在进行生物学实验之前,必须确定化学物质的紫外线吸收光谱。
如果这种物质的摩尔消光系数/吸收系数小于10L/mol·cm,那么这种物质则没有潜在的光反应,同样不必进行体外3T3光毒性实验,以及其他光化学毒性反应的生物学实验。
正常状态下,细胞能够吸收生物活性染料(如中性红),当细胞受到损伤后,吸收生物活性染料的能力降低;细胞膜受到伤害后,吸收的生物活性染料很容易泄露等。
FDA-GLP规范介绍

Common GLP Terms and Definitions Test systems: any animal, plant
microorganism (or subpart) to which a test or control article is administered Sponsor: the person or facility who initiates and supports, either financially or otherwise, a nonclinical laboratory study
Responsibilities under the GLPS
Management Study director Quality Assurance Unit Personnel
6
Management responsibility
Assure the facility has a Quality Assurance Unit (QAU)
FDA GLP REGULATIONS
Keerti and Navin
Topics to be Discussed
Applications of GLP Regulations in industry History of the FDA GLP Regulations Common GLP terms and definitions Responsibilities under the GLPs SOPs Protocols Computer validation FDA inspection Disqualification and requalification of facilities
- 1、下载文档前请自行甄别文档内容的完整性,平台不提供额外的编辑、内容补充、找答案等附加服务。
- 2、"仅部分预览"的文档,不可在线预览部分如存在完整性等问题,可反馈申请退款(可完整预览的文档不适用该条件!)。
- 3、如文档侵犯您的权益,请联系客服反馈,我们会尽快为您处理(人工客服工作时间:9:00-18:30)。
Introduction: the Historical Background The dermal phototoxicity of a chemical is defined as a toxic response that is elicited after exposure of skin to the chemical or systemic administration of the chemical, and subsequent exposure to light. As demonstrated in several validation studies (1–3), the phototoxic potential of chemicals can be effec-tively assessed by in vitro methods. In 1996, an OECD workshop recommended an in vitro tier-test-ing approach for phototoxicity assessment (4). In 2000, the Commission of the European Com-munities put into force Directive 2000/33/EC, which introduces the in vitro 3T3 neutral red uptake (NRU) phototoxicity test as a validated replacement for testing methods involving the use of laboratory animals. The essence of the in vitro 3T3 NRU pho-totoxicity test is to compare the cytotoxicity of a chemical when tested in the presence and absence of exposure to a non-cytotoxic dose of UVA/visible light. Cytotoxicity is expressed as the concentra-tion-dependent reduction of the uptake of the vital dye neutral red (5), 24 hours after treatment with the chemical.Two prediction models have been proposed, which differ in the definitions of the measure used to quantify the difference between the concentra-tion–response curves recorded in the presence (+UV) and absence (–UV) of light.Prediction model 1The photo-irritancy factor (PIF) relates the half-effective concentration value EC50(–UV)1 of the curve for darkness, to the half-effective concentra-tion value EC50(+UV) of the curve in the presence of light, by means of the following formula:EC50(–UV)PIF = (Equation 1) EC50(+UV)Depending on whether the PIF value is larger or smaller than a properly chosen cut-off value (PIF c), the chemical is classified as phototoxic or non-pho-totoxic. A shortcoming of the measure in Equation 1 is that additional ad hoc definitions are requiredIn Vitro Phototoxicity Testing: Development and Validation of a New Concentration Response Analysis Software and Biostatistical Analyses Related to the Use of Various Prediction ModelsBjörn Peters and Hermann-Georg HolzhütterHumboldt-Universität Berlin, Medizinische Fakultät (Charité), Institut für Biochemie, Monbijoustrasse 2, 10117 Berlin, GermanySummary— As demonstrated in several validation studies, the dermal phototoxic potential of chemicalsin humans can be effectively assessed by in vitro methods. The core of these methods is to monitordose–response curves of a chemical in the absence and presence of light, to quantify the difference betweenthese two curves by appropriate measures (either the photo-irritancy factor [PIF], or the mean photoeffect [MPE]), and to use these measures as predictors of in vivo phototoxicity. We present new concen-tration–response analysis software for in vitro phototoxicity testing, which runs on current personal com-puters, and takes into account all the limitations identified when using a former program. We alsodemonstrate the validity and robustness of this new software by applying it retrospectively to all dataavailable from two phases of the EU/COLIPA validation trial for the 3T3 neutral red update in vitro pho-totoxicity test. Some frequently raised questions pertaining to the use of prediction models in phototoxic-ity testing are addressed, including: the necessity of using prediction models based on a cut-off; whetherit is justifiable to use sharp prediction cut-off values; whether there is a biostatistical justification for thehighest concentration of the test chemical; and whether repeated testing of a chemical is required.Key words:bootstrapping, computer program, dose–response curve, phototoxicity, statistics.1In practical applications, no distinction is normally made between the half-effective concentration value EC50, representing the bio-available concentration of the chemical actually sensed by the biological target, and the half-inhibition concentration IC50, which is the added concentration of the chemical at which the response amounts to 50% of the original value.to cope with situations where no half-effective con-centration values can be derived from the corre-sponding concentration–response curve: a) if no EC50 value can be derived from one of the two curves, the corresponding EC50 value in Equation 1is replaced by the highest concentration tested, and the chemical is classified as phototoxic if this modi-fied PIF value is larger than unity; b) if no EC50value exists for both curves, the chemical is consid-ered non-phototoxic.Prediction model 2A second measure of the difference between the dark curve and light curve, the so-called mean photo effect (MPE), was proposed by Holzhütter (6). It aims to overcome the obvious limitations in the application of the PIF, by comparing the two curves at arbitrary doses. The MPE is defined as a weighted average across a set of individual photo-effect values.nΣw i PE C ii=1MPE = (Equation 2)n Σw ii=1In Equation 2, the photo effect (PE C ) at an arbitrary concentration C is defined as the product of theresponse effect (RE C ) and the dose effect (DE C ), i.e.PE C = RE C × DE C . The definition is illustrated in Figure 1.The response effect is the difference between the responses observed in the absence and presence o f light, i.e. RE C = R C (–UV) – R C (+UV). The dose effect is given by the formula:C/C* – 1DE C =|C/C* + 1|where C* represents the equivalence concentration to C, i.e. the concentration at which the +UV response equals the –UV response at concentration C. If C* cannot be determined because the response values of the +UV curve are systematically higher or lower than RE C , the dose effect is set to 1. The weighting factors w i are given by the highest response value, i.e. w i = Max{R i (–UV), R i (+UV)}.The discrete concentration values C i , used for the calculation of MPE according to Equation 2, are dis-tributed such that the same number of data points fall into the concentration intervals defined by the concentration values used in the experiment. The calculation of the MPE is restricted to the maxi-mum concentration value at which at least one of the two curves still exhibits a response value of at least 10%. If this maximum concentration is higher than the highest concentration used in the +UVFigure 1:Illustration for the photo effect calculationCalculation of the photo effect at the concentration 0.4. Applying the equations given in the text gives: response effect RE 0.4= (66% – 11%)/100% = 0.55, dose effect DE 0.4=(0.4/0.16 – 1)/(0.4/0.16 + 1) = 0.43, and photo effect PE 0.4= 0.24. The mean photo effect is obtained by averaging over the values for the photo effect at various concentrations.dose effect (DE c )photo effect (PE c )concentration (arbitrary units)p e r c e n t a g e r e s p o n s eexperiment, the residual part of the +UV curve is set to the response value 0. The MPE-based predic-tion model of in vivo phototoxicity classifies a chem-ical as “phototoxic” if the MPE value is larger than a cut-off value, otherwise the chemical is classified as “non-phototoxic”.Both measures of curve difference, PIF and MPE, represent statistical estimates that have to be derived from the observed concentration–response relations. To facilitate this work, and to harmonise the process of data analysis among various labora-tories, in 1996 we developed a computer program, NRU-PIT2. It calculates the statistical distribution of PIF and MPE values for given pairs of +UV and –UV concentration–response data. Based on these distributions, and at given cut-off values, the pro-gram calculates the probability (p-value) that a test chemical is phototoxic. The NRU-PIT2software is a hybrid program, composed of a 16-bit Visual Basic™dialogue shell, and a fitting module written in Turbo Pascal™, which we adopted from our pro-gram SIMFIT(7). Its use is restricted to the now-obsolete Windows 3™group of operating systems. Moreover, intensive use of this program during sev-eral international validation studies, as well as in in-house applications, has revealed some weak spots, both in the handling of the program and in the implementation of the MPE-based prediction model. Therefore, we have developed a new com-puter program, PHOTOTOX, which was written in C++, and runs on Windows 95™or higher operat-ing systems. The major functions of the program are described below, the substantial changes made in comparison with NRU-PIT2 are discussed, and the overall performance of the new program is assessed. To this end, we have applied the new soft-ware to all data compiled for the 3T3 NRU assay during two international studies (2, 3), as well as for the keratinocyte NRU assay (8), which was ini-tially used to determine the cut-off value for the MPE-based prediction model (6). The main objec-tive of this re-analysis was to make sure that the modifications made in the new software do not com-promise the results obtained with the old program, NRU-PIT2.We have also addressed some problems that specifically arose from comments on OECD draft Test Guideline ENV/JM/TG(2001)7: Status Report on Proposals for Test Guidelines on the In Vitro Skin Corrosion Test, and the In Vitro3T3 NRU Phototoxicity Test (private communication). The following questions were asked: a) is the use of cut-off based prediction models needed, or is it suffi-cient to check for a statistically significant difference between the two dose–response curves by using a simple t-test; b) given that we cannot renounce cut-off based prediction models, what are the “optimal” cut-off values for the PIF and the MPE in the light of all available data; c) are there biostatistical arguments for limiting the highest concentration used in the 3T3 NRU assay; and d) what is the benefit of performing at least two inde-pendent repeat experiments?Materials and Methods: Software DesignThe main functions of PHOTOTOX are depicted in Figure 2. The major changes made in comparison with the old program NRU-PIT2are shown in grey boxes.Data inputThe program accepts three modes of data input: a) import of absorbance values from a 96-well plate for arbitrary plate layouts (i.e. arrangement of blanks, controls and wells containing the test chemical), and transformation of these values into dose–response data; b) direct entry of dose–response data as a 2-column data sheet; and c) import of *.ddd-files generated by the old software NRU-PIT2.BootstrappingThis program module performs a bootstrap resam-pling of the original concentration–response data (9, 10), which results in a set of new computer-gen-erated concentration–response data, which can be considered as equally probable realisations of the “true” concentration–response data hidden in the experimental observations.Curve fittingThe central module of the software is a conjugated-gradient minimiser that performs fitting of a con-tinuous model function to the original or bootstrap-resampled sets of discrete concentra-tion–response data. The mathematical concentra-tion–response model used belongs to the large class of polynomials. Nevertheless it has a semi-empirical background, in that it refers to a multi-state com-partment system, which upon addition of the test chemical is thought to be driven from its native state (with a response value of 100%) through a series of non-native states with altered response values (11). If the number of compartments is cho-sen correctly, the model is flexible enough to cope with complicated curve shapes, exhibiting, for example, plateaus or extreme points. A typical shortcoming of polynomial models, however, is their general tendency to exhibit artificial oscilla-tions. Therefore, model fitting to the data is com-bined with a damping procedure (constraint minimisation). To avoid damping out of realextreme points, curve fitting is preceded by an analysis of extreme points.In NRU-PIT2, an extreme point was definedby a set of three consecutive response valuesR i–1, R i, R i+1obeying the condition that themiddle value is significantly larger (smaller)than the two neighbouring values. In mathe-matical terms R i is a maximum if R i–1< R iand R i+1< Ri, and Ri is a minimum if R i–1>R i and R i+1> R i. When this definition wasused, the program failed to detect smoothextreme points in flat curves. Therefore, wenow define an extreme point by a set of con-secutive response values R i, R i+1, … , R i+k(k≥0), which meet the following two condi-tions: a) the differences between consecutivepoints are statistically not significant, i.e. R i≈R i+1 ≈… ≈R i+k; and b) the first and the lastresponse value of this set, R i and R i+k, areboth significantly smaller (maximum) orlarger (minimum) than their left and rightneighbours, i.e.:R i ›/‹ R i–1and R i+k ›/‹ R i+k+1Based on the fitted model, the software offers the possibility of calculating the mean value and variance of the effective dose ECx at which the response reaches x% (0 < x < 100) of the initial value. The default value of the residual response is x = 50% yielding the common EC50 value used in the calculation of a PIF. This fea-ture makes the program a valuable tool for dose–response analysis beyond phototoxicity testing.Figure 2: Data processing with PHOTOTOXMPE = mean photo effect; PIF = photo-irritancy factor.ECx mean values and variances of all dose–response curves contained in the project aretabulated in an extra report sheet. They areautomatically updated if the user changes theresidual response level x. The default value isx = 50%.PIF/MPE calculationCurve fits to the original and bootstrapped data sets provide a bundle of concentration-response curves for a single (+UV or –UV) experiment (Figure 3a). By pairing each curve of the –UV experiment with each curve of the +UV experiment, one arrives at an ensemble of different values for the PIF and the MPE. The statistical distribution of these values (Figure 3b) serves as a basis for the calculation of p-values (see below).AThe choice of the concentration grid used inthe calculation of the MPE according toEquation 2 was changed in the new program.In NRU-PIT2, 20 different concentration val-ues were distributed equidistantly along thewhole dose interval defined through the high-est concentration value common to both datasets under comparison. This choice of theconcentration grid has two drawbacks: a) itgives a higher weight to the high-concentra-tion parts of the two curves, if the test con-centrations are increased in a geometricseries (as in most experiments); and b) itrestricts the calculation of the MPE to theconcentration interval shared by both experi-ments. In the new program, the calculation ofthe MPE is performed across a concentrationinterval that is defined through the highestconcentration value (C max) up to which atleast one curve exhibits a response value of10% or higher. If C max is not reached in one ofthe two paired experiments (usually in the+UV experiment, because the response val-ues have already dropped to zero at smallerconcentrations), the missing responses up toC max are considered insignificant, and aretherefore set to 0.BThe concentration grid is now chosen so thatthe same numbers of points fall into eachconcentration interval (defined by the con-centration values used in the experiment).In PHOTOTOX, the total number of gridpoints is 50. For example, in Figure 3, theconcentration values applied were [0,1.5625, 3.125, 6.25, 12.5, 25, 50, 100, 200]for the +UV experiment, and [0, 7.8125,15.625, 31.25, 62.5, 125, 250, 500, 1000] forthe –UV experiment. For both experiments,a 500-point dose grid is constructed, con-taining the same number of equidistantpoints between each pair of doses used in theexperiment. For the +UV experiment, thisis: [0, 0.0252, 0.0504, …, 1.5373, 1.5625,1.5877, …, 3.010, 3.125, 3.175, …, 196.8,198.4, 200]. The grids for both experimentsare merged to a give a 1000-point grid. Fromthe merged grid, 50 points are selected,ranging from 0 to C max. The resulting evalu-ation grid with C max= 173 is [0.000, 0.328,0.680, …, 26.5, 29.0, 31.8, …, 143, 158, 173].CIn NRU-PIT2, the weighting factors w i inEquation 2 were taken as the sum of theresponse values of the two curves, w i=R i(+UV) + R i(–UV), to reduce the influenceof data points with low responses. Because ofthe changes in the choice of the concentrationgrid (see B), the weighting factors w i are nowdefined through the highest response value,w i= MAX{R i(+UV), R i(–UV)}. This enablesa sharper separation of MPE values betweenphototoxic and non-phototoxic chemicals, ashigher weighting is given to that part of theconcentration grid where one curve hasalready dropped while the other still com-prises high response values.PIF/MPE-based classification of phototoxicityThe probability (p-value) of a chemical exhibiting a phototoxic potential in a single ±UV experiment is defined by:n>– n<p tox= (Equation 3) n>+ n0+ n<where n>, n<and n0denote, respectively, the num-ber of PIF/MPE values within the bootstrap ensem-ble which are either larger than the cut-off value indicative for phototoxicity (i.e. the toxicity of the chemical is significantly increased in the presence of light), smaller than the cut-off value indicative for a photo-protective effect (i.e. the toxicity of the chemical is significantly decreased in the presence of light), or do not meet the previous two condi-tions.ANote that, in the definition of the phototoxic-ity probability used in NRU-PIT2, the num-Figure 3: The main functions of PHOTOTOXa)Experimental and theoretical concentration-response data. Five bootstrap concentration-response curves wereconstructed for both the –UV (continuous lines) and +UV (dotted lines) experiments, by fitting the concentration–response model to novel “synthetic” data sets derived from the original data (solid points) by randomly selectingresponse values with replacement from the original group of six replicates at each concentration value. The calculation of mean photo effect (MPE) was restricted to the maximal concentration C max = 173 concentration units (shaded area)at which the –UV curve falls below 10% of the maximal response. To include the control values (concentration = 0) on an otherwise logarithmic axis, the concentration axis consists of two parts. The scaling between 0 value and the first concentration value (here, 1.56) is linear, whereas the rest of the axis is scaled logarithmically. The length of the linear part of the axis is chosen to be equal to the distance between the first and the second concentration value in thelogarithmic part. Thus, a geometric concentration series, including a control concentration of 0, will be displayed as equidistant. The shaded box indicates the range considered for curve comparison.b) Statistical distribution of photo-irritancy factor (PIF) and MPE values. Values of the PIF and the MPE werecalculated for all 25 possible pairs of the five –UV and five +UV bootstrap curves shown in Figure 3a. Calculation of p-values according to Equation 3 gives p MPE = 8/25 = 0.32. and p PIF = 2/25 = 0.80, i.e. the MPE classifies the chemical as non-phototoxic (p < 0.5), whereas the PIF classifies it as phototoxic (p > 0.5).a)concentration (mg/l)p e r c e n t a g e r e s p o n s e1.8–1.92.0–2.12.2–2.3PIF rangen u m b e r i n r a n g e0.06–0.080.10–0.120.14–0.16MPE range n u m b e r i n r a n g eeffect (n<) has not been considered in the numerator of expression (Equation 3), i.e. the p-value was computed as the relative number of bootstrap pairs yielding a PIF or MPE value larger than a given cut-off value. Such a classification scheme results in a systematic bias toward higher probability values, because all curve pairs indicating the pres-ence of a phototoxic potential enlarge the p-value, whereas all curve pairs indicating the presence of a photo-protective effect, do not reduce the p-value. At the extreme, positive p-values could be produced by chance, even if the mean dose–response data for both exper-iments were identical, but large errors in the measured response data gave rise to single values of PE or PIF (derived from single curve pairs) exceeding the cut-off value.BBecause of the low calculation speed of NRU-PIT2, each bootstrap curve of the +UV experiment was paired with only a single (arbitrarily chosen) curve of the –UV experi-ment. In contrast, the new program provides more-reliable statistical estimates, by includ-ing all possible pairs of bootstrap curves. For example, if ten bootstrap curves are gener-ated for each experiment, 100 curve pairs are included in the computation of classification probabilities (see below).The cut-off values for photo-protection are chosen as 1/PIF c and –MPE c, where PIF c and MPE c are the respective cut-off values indicative of phototoxicity. Averaging across the p-values obtained in several independent experiments (runs) provides a mean p-value for both prediction models, which can be employed for decision making.Concentration-dependent photo effectThe value of the MPE represents a weighted aver-age across individual photo effect values calculated at various concentrations of the test chemical. Both MPE and PIF measure the difference between the +UV and –UV curves without making explicit ref-erence to the concentration range over which the light-induced difference between the curves is rele-vant. To visualise the concentration range for which significant photo effects can be expected, the program provides a concentration-dependent plot of the photo effect, as well as of the two effects (response effect and dose effect) contributing to it (Figure 3c).Results: Software ValidationDatabaseAs outlined above, the new program PHOTOTOX comprises a number of modifications of the calcula-tion procedure for MPE and of the curve-fitting to the data, in comparison with the old program NRU-PIT2. To investigate the consequences of these modifications for numerical PIF and MPE values and the related in vivo classifications of phototoxic-ity, we have re-analysed the experimental data gathered for the 3T3 NRU phototoxicity test during two international studies (2, 3) and for the ker-atinocyte NRU phototoxicity test (8; see Table 1). The latter database was used to define the cut-off value for the MPE-based prediction model in our previous work (6). Compiling the original measure-ments gathered in the first international study (2), we encountered technical difficulties in extracting data from compressed files from one laboratory. Therefore, these data could not be used in the re-analysis. Moreover, we did not include the data for one chemical tested in two studies (2) and (8), for which no in vivo toxicity was reported. The whole database used in the software validation comprised 635 different pairs of dose–response curves. Generally, each laboratory recorded two independ-ent pairs of +UV/–UV curves per chemical (repli-cates), but sometimes only one, while sometimes, three pairs were recorded. By averaging the results for the replicates, 322 classification results are obtained. If no other database is explicitly men-100101102103104PIF NRU-PIT2a)P I F P H O T O T O X1010101010MPE (NRU-PIT2)b)M P E P H O T O T O XFigure 4: Comparison of PIF and MPE values obtained by application of the two computerprograms NRU-PIT2and PHOTOTOXin vivo phototoxic chemical; = in vivo non-phototoxic chemicala) Photo-irritancy factor (PIF). Only those PIF values obtained from curve pairs with two existing EC50 values areplotted. Measure of determination: r 2=0.992. To understand how the striking differences between some PIF values (for example. PIF NRU-PIT2≈1, PIF PHOTOTOX ≈1000) could be generated, we examined the original data. These do not support the PIF values published for NRU-PIT2, so we have to conclude that errors were made when manually entering the concentration values for those curves.b) Mean photo effect (MPE).Measure of determination: r 2=0.831.tioned below, the results will refer to the database shown in Table 1.Comparing the old and the new softwareWe have checked whether the changes in the soft-ware give rise to significantly different in vivo clas-sifications of phototoxicity compared with those obtained with the old software NRU-PIT2. As can be seen in Table 2, this is not the case. For 302 out of 322 MPE-based classifications, and for 313 out of 322 PIF-based classifications, the two versions of the software provided identical results. The total misclassification rates obtained by means of the new software were 30/322 = 9.3% for PIF and 26/322 = 8.1% for MPE: these are marginally smaller than the total misclassification rates of 31/322 = 9.6% (PIF) and 28/322 = 8.7% (MPE) obtained when using the old software. Instead of comparing classification results, a more-sensitive detection of differences between the performance of the two software versions is achieved by comparing directly “old” and “new” PIF and MPE values (Figure 5). The concordance between old and new PIF values is higher (r2= 0.992) than for the MPE values (r2= 0.831), because the estimate of EC50 values, and hence the estimate of the PIF value, is less sensitive to slight changes in the shapes of the fitted dose–response curves. The few striking dif-ferences between old and new PIF values turned out to be caused by incorrect handling of the corre-sponding raw data in the previous analysis made with NRU-PIT2.Results: Biostatistical Issues Related to the Use of Prediction ModelsThe following results were obtained in attempts to respond to typical questions raised by users of the program, and in helping them to minimise the num-ber of misclassifications in future applications of the software. Moreover, we have responded to some critical remarks and suggestions made by interna-tional experts in their comments on the OECD draft Test Guideline ENV/JM/TG(2001)7 Status Report on Proposals for Test Guidelines on the In Vitro Skin Corrosion Test, and the In Vitro3T3 NRU Phototoxicity Test (private communication).Optimal cut-off values for PIF and MPEFigure 5 shows the percentage of total misclassifi-cations, false-negative classifications and false-posi-tive classifications at varying cut-off values of PIF and MPE. Putting together the classifications obtained for the three databases, a minimum of misclassifications is achieved when choosing PIF cut-off= 2 and MPE cut-off= 0.12. Hitherto, for the PIF, a cut-off value of 5 was stipulated in several inter-national studies, and was also recommended in the Standard Operating Procedures (SOPs) of the 3T3 NRU phototoxicity test. This cut-off value was pro-Table 1: Database used for the validation of the new software and for the computations related to statistical issues with prediction modelsEU/COLIPA: EU/COLIPA: Study on UV filterphase I (2)phase II (8)chemicals (3) Number of test chemicals292920Number of laboratories814Number of chemicals/laboratory29, 29, 28, 29, 16, 29, 26, 292920, 19, 20, 19 for which at least one curvepair was availableEU/COLIPA = international EU/COLIPA in vitro phototoxicity validation study.Figure 5: Misclassification rates at varying cut-off values for photo-irritancy factor (PIF) andmean photo effect (MPE)a) PIF; b) MPE.The rate of false-positive classifications is given as the percentage of misclassifications within the group of in vivo non-phototoxic chemicals. The rate of false-negative classifications is given as the percentage of misclassifications within the group of in vivo phototoxic chemicals.average probability of a non phototoxic chemical to be classified as phototoxic;average probability of a phototoxic chemical to be classified as phototoxic;average misclassification probability.cut-off valueb)p e r c e n t a g e m i s c l a s s i f i c a t i o n r a t e scut-off valuea)p e r c e n t a g e m i s c l a s s i f i c a t i o n r a t e s。