Networks of Spiking Neurons Using Unreliable Synapses
国开作业人工智能专题-专题二-测验57参考(含答案)剖析
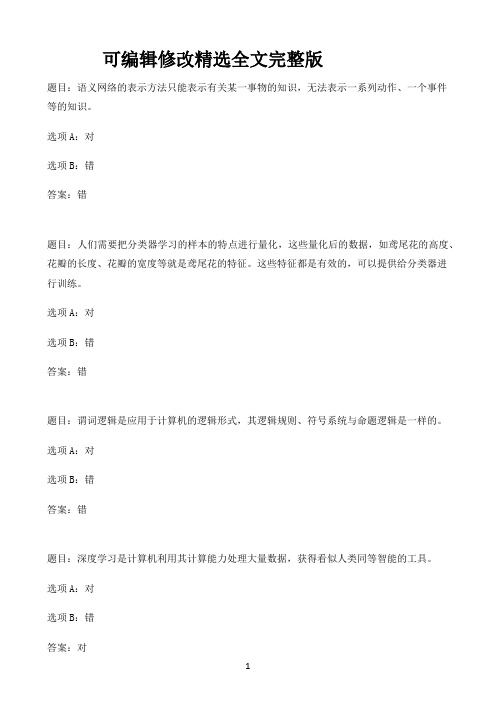
可编辑修改精选全文完整版题目:语义网络的表示方法只能表示有关某一事物的知识,无法表示一系列动作、一个事件等的知识。
选项A:对选项B:错答案:错题目:人们需要把分类器学习的样本的特点进行量化,这些量化后的数据,如鸢尾花的高度、花瓣的长度、花瓣的宽度等就是鸢尾花的特征。
这些特征都是有效的,可以提供给分类器进行训练。
选项A:对选项B:错答案:错题目:谓词逻辑是应用于计算机的逻辑形式,其逻辑规则、符号系统与命题逻辑是一样的。
选项A:对选项B:错答案:错题目:深度学习是计算机利用其计算能力处理大量数据,获得看似人类同等智能的工具。
选项A:对选项B:错答案:对题目:贝叶斯定理是为了解决频率概率问题提出来的。
选项A:对选项B:错答案:错题目:状态空间图是对一个问题的表示,通过问题表示,人们可以探索和分析通往解的可能的可替代路径。
特定问题的解将对应状态空间图中的一条路径。
选项A:对选项B:错答案:对题目:分层规划中包含基本动作和高层动作。
选项A:对选项B:错答案:对题目:启发式规划的两种方法是减少更多的边或者状态抽象。
选项A:对选项B:错答案:错题目:P(A∣B)代表事件A发生的条件下事件B发生的概率。
选项A:对选项B:错答案:错题目:现实世界中的规划问题需要先调度,后规划。
选项A:对选项B:错答案:错题目:当神经网络接收到工作任务时,就是用()来接收这些任务所对应的数据集,如图像每个像素点的特征数值——色彩、亮度等。
()的每个神经元都是任务的特征,即特征数值。
选项A:隐含层选项B:应用层选项C:输入层选项D:输出层答案:输入层题目:机器学习过程中,近似于人类的归纳推理式学习方式,被誉为“人工智能最有价值的地方”的学习方式是()。
选项A:机器学习选项B:无监督学习选项C:监督学习答案:无监督学习题目:算法模型看起来像一棵倒立的树,数据沿着树根输入,再从叶子节点输出,中间的分支要根据不同特征的信息进行判断,决定该向左走还是向右走,这种算法称为()。
人工智能导论考核试卷

3. CNN通过卷积和池化操作提取图像特征,降低参数数量,提高模型泛化能力,从而提高图像识别准确性。
4.伦理问题:隐私保护、算法偏见、责任归属。解决策略:制定伦理准则、透明度提升、多样化团队、责任追溯机制。
1.人工智能包括以下哪些技术领域?()
A.机器学习
B.语音识别
C.量子计算
D.数据挖掘
E.虚拟现实
2.以下哪些属于监督学习算法?()
A.支持向量机
B.决策树
C. K-均值聚类
D.线性回归
E.随机森林
3.深度学习中的卷积神经网络(CNN)主要用于哪些任务?()
A.图像分类
B.语音识别
C.自然语言处理
D.视频分析
人工智能导论考核试卷
考生姓名:__________答题日期:__________得分:__________判卷人:__________
一、单项选择题(本题共20小题,每小题1分,共20分,在每小题给出的四个选项中,只有一项是符合题目要求的)
1.以下哪个不是人工智能的研究领域?()
A.机器学习
B.深度学习
D.随机森林
E.支持向量回归
9.以下哪些是推荐系统中的冷启动问题?()
A.用户冷启动
B.项目冷启动
C.模型冷启动
D.数据冷启动
E.系统冷启动
10.以下哪些是迁移学习的主要挑战?()
A.数据分布差异
B.标签空间不匹配
C.模型泛化能力不足
D.源域数据不足
E.目标域数据过拟合
2023年深度学习师真题
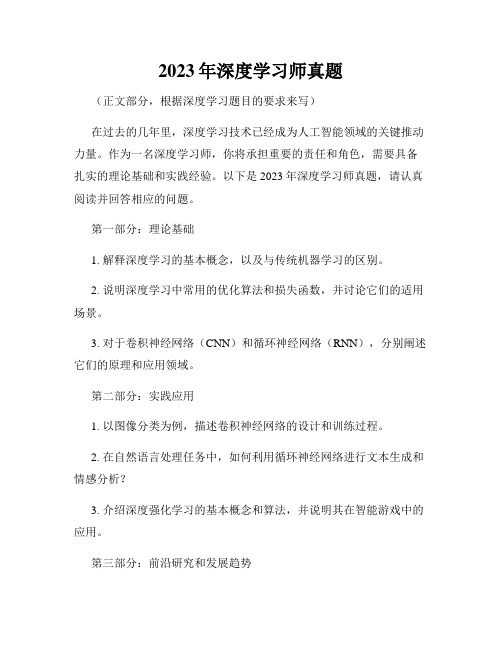
2023年深度学习师真题
(正文部分,根据深度学习题目的要求来写)
在过去的几年里,深度学习技术已经成为人工智能领域的关键推动力量。
作为一名深度学习师,你将承担重要的责任和角色,需要具备扎实的理论基础和实践经验。
以下是2023年深度学习师真题,请认真阅读并回答相应的问题。
第一部分:理论基础
1. 解释深度学习的基本概念,以及与传统机器学习的区别。
2. 说明深度学习中常用的优化算法和损失函数,并讨论它们的适用场景。
3. 对于卷积神经网络(CNN)和循环神经网络(RNN),分别阐述它们的原理和应用领域。
第二部分:实践应用
1. 以图像分类为例,描述卷积神经网络的设计和训练过程。
2. 在自然语言处理任务中,如何利用循环神经网络进行文本生成和情感分析?
3. 介绍深度强化学习的基本概念和算法,并说明其在智能游戏中的应用。
第三部分:前沿研究和发展趋势
1. 评述深度学习在计算机视觉领域的最新研究进展,例如目标检测、图像分割等。
2. 讨论深度学习在自然语言处理和语音识别领域的挑战和发展方向。
3. 分析深度学习在医疗诊断、智能交通等领域的潜在应用和社会影响。
以上是2023年深度学习师真题的部分内容,希望你能在备考之前
认真研究和准备,充分掌握深度学习的理论知识和实践经验。
祝你成
功取得优异的成绩,成为一名优秀的深度学习师!
(文章结束,无需再重复标题或其他内容)。
深度学习与神经网络硬件考核试卷

B.梯度下降
C.动量方法
D.二阶优化方法
5.神经网络中常见的正则化方法有哪些?()
A. L1正则化
B. L2正则化
C. Dropout
D.数据增强
6.以下哪些是神经网络加速器的优点?()
A.高能效比
B.低延迟
C.高度并行化
D.易于编程
7.以下哪些技术可以用于减少神经网络模型的过拟合现象?()
A.早期停止
B.数据增强
C.添加噪声
D.增加模型容量
8.哪些类型的神经网络层在卷积神经网络中常见?()
A.全连接层
B.卷积层
C.池化层
D.激活层
9.以下哪些硬件平台适合深度学习训练任务?()
A. CPU
B. GPU
C. TPU
D. FPGA
10.深度学习中,以下哪些因素影响模型的泛化能力?()
A.训练数据量
C.对比神经网络
D.生成对抗网络
5.以下哪种方法不适用于提高神经网络硬件性能?()
A.提高内存带宽
B.增加处理器核心数
C.降低处理器频率
D.采用新型内存技术
6.在神经网络中,下列哪个参数调整可能导致过拟合?()
A.学习率
B.批量大小
C.隐藏层神经元数
D.优化器
7.以下哪个芯片制造商推出了专为深度学习设计的GPU?()
16.在深度学习中,哪种方法可以减少模型参数?()
A.添加正则化项
B.增加隐藏层
C.减少训练数据
D.采用批量归一化
17.以下哪个概念与神经网络模型泛化能力无关?()
A.过拟合
B.模型容量
C.数据分布
D.损失函数
2023年人工智能现代科技知识考试题与答案
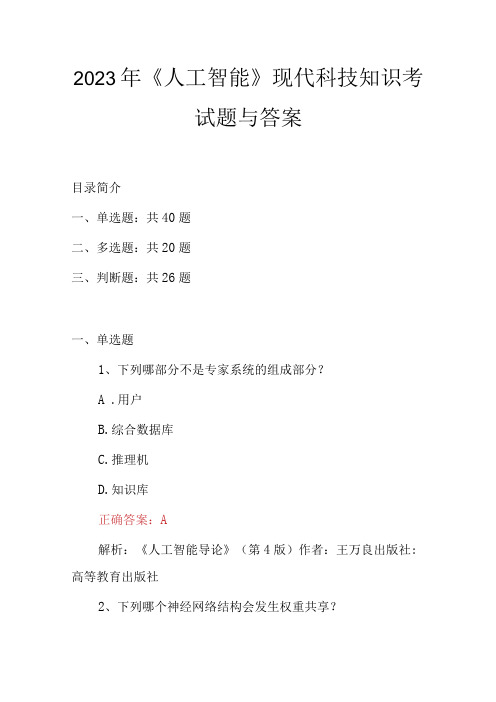
2023年《人工智能》现代科技知识考试题与答案目录简介一、单选题:共40题二、多选题:共20题三、判断题:共26题一、单选题1、下列哪部分不是专家系统的组成部分?A .用户B.综合数据库C.推理机D.知识库正确答案:A解析:《人工智能导论》(第4版)作者:王万良出版社: 高等教育出版社2、下列哪个神经网络结构会发生权重共享?A.卷积神经网络B.循环神经网络C.全连接神经网络D. A 和B正确答案:D解析:《深度学习、优化与识别》作者:焦李成出版社: 清华大学出版社3、下列哪个不属于常用的文本分类的特征选择算法?A.卡方检验值B.互信息C .信息增益D.主成分分析正确答案:D解析:《自然语言处理》作者:刘挺出版社:高等教育出版社4、下列哪个不是人工智能的技术应用领域?A.搜索技术B.数据挖掘C.智能控制D .编译原理解析:《走进人工智能》作者:周旺出版社:高等教育出版社5、Q(s,a)是指在给定状态s的情况下,采取行动a之后,后续的各个状态所能得到的回报()。
A.总和B.最大值C.最小值D.期望值正确答案:D解析:《深度学习、优化与识别》作者:焦李成出版社: 清华大学出版社6、数据科学家可能会同时使用多个算法(模型)进行预测,并且最后把这些算法的结果集成起来进行最后的预测(集成学习),以下对集成学习说法正确的是()。
A.单个模型之间有高相关性B.单个模型之间有低相关性C,在集成学习中使用“平均权重”而不是“投票”会比较好D.单个模型都是用的一个算法解析:《机器学习方法》作者:李航出版社:清华大学出版社7、以下哪种技术对于减少数据集的维度会更好?A.删除缺少值太多的列B.删除数据差异较大的列C.删除不同数据趋势的列D.都不是正确答案:A解析:《机器学习》作者:周志华出版社:清华大学出版社8、在强化学习过程中,学习率越大,表示采用新的尝试得到的结果比例越(),保持旧的结果的比例越()。
A .大,小B.大,大C.小,大D.小,小正确答案:A解析:《深度学习、优化与识别》作者:焦李成出版社: 清华大学出版社9、以下哪种方法不属于特征选择的标准方法?A.嵌入B.过滤C ,包装D.抽样正确答案:D解析:《深度学习、优化与识别》作者:焦李成出版社: 清华大学出版社10、要想让机器具有智能,必须让机器具有知识。
Spiking Neural models
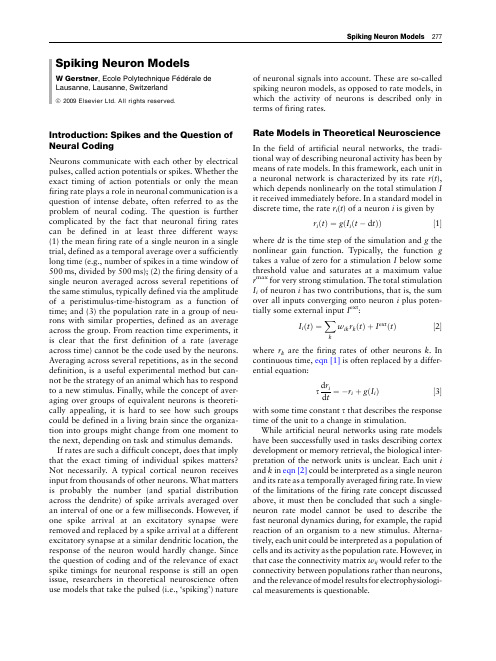
Spiking Neuron ModelsW Gerstner , Ecole Polytechnique Fe´ de´ rale deLausanne, Lausanne, Switzerlandã 2009 Elsevier Ltd. All rights reserved. Introduction: Spikes and the Question ofNeural CodingNeur o ns co m munica t e with each other by ele c trical pulse s, called action potential s or sp i kes. W h ether the exact timin g of actio n potent i als or only the mean firing rate plays a role in neuronal co m munica t ion is a questio n of intense debate , often referre d to as the proble m of neural coding. The question is further compl i cated by the fact that neuron a l fir i ng rates can be defi n ed in at least three differe n t ways: (1) the mean firing rate of a singl e neuron in a single trial, defined as a temporal averag e over a suff i ciently long time (e.g., num b er of spike s in a time win d ow of 500 ms, divided by 500 ms) ; (2) the firing density of a single neuron averag e d across several repetitions of the same stimul u s, typical l y defined via the ampl i tude of a peri s timulu s-time-hist o gram as a function of time; and (3) the populat i on rate in a group of neu-rons with simila r pr o perties , defi n ed as an averag e across the group . From reaction time experime n ts, it is clear that the first defin i tion of a rate (average across time) cann o t be the code used by the neuron s. Averaging across several repet i tions, as in the second definit i on, is a us e ful experime n tal met h od but can-not be the strategy of an an i mal which has to respond to a new stimulus. Final l y, while the concept of aver-aging over groups of equ i valent neurons is theore t i-cally app e aling, it is hard to see how such g r oups could be de f ined in a living br a in since the organiza-tion into groups might chan g e from one mom e nt to the next, dependi n g on task and stimul u s demands . If rates are su c h a diffic u lt concept , does that imply that the exa c t timi n g of individual spikes mat t ers? Not neces s arily. A typi c al cortical neuron rece i ves input from thousands of oth e r neuron s. What mat t ers is proba b ly the numb e r (and spatial dist r ibution across the dendri t e) of spike arrival s averag e d over an interval of one or a few mi l liseconds. How e ver, if one spike arriva l at an exci t atory syn a pse were remo v ed and repl a ced by a spike arri v al at a diff e rent excitat o ry synapse at a similar dendri t ic location, the respons e of the neuro n would hardly change. S i nce the questi o n of coding and of the rel e vance of exact spike timings for neuronal respon s e is sti l l an open issue, researche r s in theore t ical neurosc i ence ofte n use mod e ls that take the pulse d (i.e., ‘spik i ng’) nature of neuron a l signa l s into accoun t. These are so-ca l led spikin g neuron model s, as oppos e d to rate models, in which the activity of neuro n s is descr i bed only in terms of firing rate s.Rate Models in Theoretical Neuroscience In the field of artifici a l neu r al netwo r ks, the tradi-tiona l way of descr i bing neurona l activity ha s been by means of rate mod e ls. In this framew o rk, each unit in a neuronal network is character i zed by its rate r( t), which depends nonlin e arly on the total stimul a tion I it rece i ved im m ediate l y before . In a standard model in discret e time, the rate r i( t) of a neuron i is given byr i ðtÞ¼gðI i ðtÀ d t ÞÞ½1 where d t is the time step of the simula t ion and g the nonli n ear gain function. Typica l ly, the function g takes a value of zero for a stimul a tion I be l ow some threshold value and saturates at a maximum value r max for very strong stimulation.The total stimulation I i of neuron i has two contributions,that is,the sum over all inputs converging onto neuron i plus poten-tially some external input I ext:I iðtÞ¼Xkw ik r kðtÞþI extðtÞ½2where r k are the firing rates of other neurons k.In contin u ous time, eqn [1] is often replaced by a differ-ential equation:t d r id t¼Àr iþgðI iÞ½3with some time constant t that describes the response time of the unit to a change in stimulation.While artificial neural networks using rate models have been successfully used in tasks describing cortex development or memory retrieval,the biological inter-pretation of the network units is unclear.Each unit i and k in eqn [2] could be interpreted as a single neuron and its rate as a temporally averaged firing rate.In view of the limitations of the firing rate concept discussed above,it must then be concluded that such a single-neuron rate model cannot be used to describe the fast neuronal dynamics during,for example,the rapid reaction of an organism to a new stimulus.Alterna-tively,each unit could be interpreted as a population of cells and its activity as the population rate.However,in that case the connectivity matrix w ij would refer to the connectivity between populations rather than neurons, and the relevance of model results for electrophysiologi-cal measurements is questionable.Spiking Neuron Models277Detailed Models of a Spiking NeuronThe classi c al model of a spikin g ne u ron is the math-ematical description of Hodgkin and Huxley of action potent i al genera t ion in the giant axon of the sq u id. Generat i on of spikes in the model arises through the interpl a y of four nonl i near differe n tial equati o ns. The first equatio n summa r izes the curren t co n servati o n on a small piece of mem b rane. A current I ext inj e cted onto the membrane can either charge the capacity C of the membran e or pass through one of the ion chann e ls. With three different ion chan n el types (one for sodium, one for potass i um, an d one for all rem a ining ‘leak ’ compo n ents), the change of the voltag e is give n byC d ud t¼ I ext À g Na m3 hðuÀ E N a ÞÀ g K n4 ðuÀ E K ÞÀg L ðuÀ E L Þ½4where g Na and g K are the maxi m um cond u ctanc e of the sodium and potass i um ch a nnel, respe c tively; E Na and E L a r e their reversal potent i als; and m ,h, and n are ad d itional ‘gating’ vari a bles that describe the state of the chan n el as a funct i on of time. Each of the three variab l es m ,n, and h follow s an equati o n of the formd x d t ¼Àx À x0ðu Þt ðu Þ½5with empiri c al funct i on x0 ( u) and t (u) derived from the experime n ts (and differe n t for m ,n,and h). Com p uter simula t ions as well as math e matical analys i s of the system of four nonl i near differe n tial equati o ns sh o ws that actio n pot e ntials are genera t ed only if the total stimulati o n I ext reaches a critical value . To a first degree of app r oximatio n, one may therefore conclu d e that action potent i al g e neration is an all-or-none pro c ess. However, a clos e r examinat i on of the be h avior sho w s that there is neith e r a strict voltag e thresh o ld nor a strict curre n t thres h old. In particul a r, for time-depen d ent inputs (e.g., curre n t steps and ramps or ran d omly fluc t uating curre n t as input) , not only the momen t ary vo l tage or curre n t amplit u de matter s, but the stimulati o n history as well. Formal Spiking Neuron ModelsAlthough neither the Hodgki n–Huxl e y model nor real neurons ha v e a strict firing thres h old, in pract i ce the proces s of neuron a l action potent i al g e neration can often be well approxima t ed as a thresho l d pro-cess. The simplest model in the clas s of formal sp i king neuron model s is the leaky integrat e-and- f ire model. In this model, spike s are trigger e d whene v er the mem-brane potent i al u reaches a give n thres h old y. Below thresh o ld, the mem b rane poten t ial is descr i bed by its capaci t y C and input resistan c e R . Curr e nt con s erva-tion gives the lin e ar differenti a l equ a tion.Cd ud t¼ÀuRþ I ext ½6If u reache s the thres h old y, the spike time t f is noted and the mem b rane potent i al reset to a fixed value u reset , before integrat i on of eqn [6] is resum e d. The essen c e of the leaky integrate-a n d-fire model is a clear separa t ion betw e en a compl e tely linear (pass i ve) sub-thresh o ld regi m e and a strict firing threshold. The leaky inte g rate-and- f ire mod e l is today the standar d neuron model in many simula t ion studies concer n ing the dynami c s of large neuron a l netw o rks or the ques-tion of neural coding.Gene r alizations of the leaky inte g rate-and- f ire model have been propo s ed in sever a l dire c tions. First,since some neurons exhibit in the subthreshold regime a damped oscillatory response to changes in the input(as opposed to a simple exponential decay), the volta g e eqn [6] can be couple d with a second equation.As long as this second equation is linear, the subthreshold behavior of the neuron is still completely linear,that is,doubling the amplitude of an input generates a subthreshold response which is twice as large.Second,refractory effects(i.e.,reduced responsiveness of a neuron immediately after firing) have been included.The combination of refractory effects with a linear subthreshold behavior leads to the spike response model with a membrane potentialuðtÞ¼ ðtÀ^tÞþZkðtÀt^;sÞI extðtÀsÞd s½7where t^is the firing time of the last spike of the neuron, describes the form of the action potential and its spike after-potential,and k the linear response to an input pulse.The next spike occurs if the mem-brane potential u hits a threshold y from below,in which case t^is updated.Hence the main character-istics of the Spike Response Model are identical to those of the leaky integrate-and-fire model,that is,a linear subthreshold regime in combination with a strict firing threshold.However,the spike response model allows refractory effects to be included(since the functions and k depend on the time since the last firing)as well as subthreshold oscillations(expressed through an appropriate shape of and k).A third generalization of the standard leaky inte-grate-and-fire model concerns adaptation.By intro-duction of a second equation that summarizes processes on a slower timescale,it is possible to describe the slow adaptation of the neuron after a step in the stimulating current.278Spiking Neuron ModelsFour t h, the sp i ke trigger e d by a strict thres h old proces s at the soma can be combi n ed with one or severa l add i tional equ a tions descr i bing the (pass i ve) propert i es of the dendri t e. Final l y, the strict thres h old can be replaced by a smooth threshold process if eqn [6] is turned into a nonli n ear equatio n:C d ud t¼ÀfðuÞþI ext½8where f(u)describes the nonlinearities of the mem-brane in the vicinity of the firing threshold.Two standard choices of f(u)are the quadratic integrate-and-fire model f(u)¼a(uÀu1)(uÀu2)and the expo-nential integrate-and-fire model f(u)¼À(u/R)þb exp[(uÀy)/D].Both models have free parameters,that is,a,u1,and u2for the quadratic and b,y,D,and R for the exponential integrate-and-fire model,that can be used to adapt the model and put it into a desired firing regime.Limitations of Formal Spiking Neuron ModelsFormal spiking neuron models approximate the elec-trical properties of neurons by one or a few equations that summarize basic features of normal neuronal behavior with a small number of phenomenological parameters.Formal spiking neurons are therefore not suitable for predicting electrophysiological experi-ments under nonstandard stimulation conditions.In particular,since all electrical properties of a neuron are summarized in one or two phenomenological equations,these models cannot be used to predict changes of behavior caused by,for example,pharma-cological blockage of specific ion channels.Moreover, since the spatial structure of real neurons is not repre-sented in detail,all nonlinear dendritic effects cannot be incorporated into formal spiking neuron models (passive properties,however,can be).Similarly,since the model summarizes neuronal behavior under some reference condition,slow changes in neuronal behav-ior,caused by slow current ramps or accumulation of intracellular calcium or a simple fatigue of the neuron, for example,cannot be captured.However,under some stimulation conditions,for-mal spiking neurons perform surprisingly well.A direct comparison of the spike response model with the Hodgkin–Huxley model during stimulation with random current shows that up to90%of spike times of the Hodgkin–Huxley model are correctly predicted by the spike response model with a tempo-ral precision of2ms.Moreover,a spike response model with a second equation describing adaptation has also been used to predict spike times of layer-V pyramidal neurons in rat cortex under random cur-rent injection.The same time-dependent input I ext(t) was given to both a model neuron and a pyramidal neuron.If the fluctuating current had large ampli-tude,the neuron itself generated spikes reliably with the same timing across several repetitions of the experiments,but less so if the fluctuation amplitude was reduced.Similarly,the model neuron was able to correctly predict the spike times of the pyramidal neuron(up to70%)if the fluctuation amplitude of the current was high,but much less well if it was low. Thus,under random current injection,formal spiking neurons describe action potential generation in pyra-midal neurons to a high degree of accuracy. Spiking Neurons in Large NetworksA major advantage of formal spiking neurons such as the leaky integrate-and-fire model is their simplicity, which has two important consequences.First,it is possible to simulate neural networks with a large number of neurons at a reasonable numerical cost. Second,network properties such as the mean firing rate of neurons in a network of randomly connected integrate-and-fire units can be studied analytically with tools from mathematical probability theory, statistical physics,and bifurcation theory.One important insight that has arisen from studies with formal spiking neurons is the importance of the subthreshold regime for cortical activity.In order to have large networks of interacting spiking neurons working in a state in which individual neurons show irregular firing with broad interspike interval distribu-tions,it turns out to be necessary that the total drive from thousands of excitatory inputs is approximately balanced by inhibition.If this is the case,the neuronal membrane potential hovers normally in the subthresh-old regime but stays close to the threshold so that neur-ons are very responsive to small changes in the input. Furthermore,studies with networks of spiking neu-rons have been used repeatedly to elucidate the poten-tial of different neural codes.For example,the stability and reproducibility of spatiotemporal spike patterns in networks of integrate-and-fire neurons have been studied in the context of synfire chains,a potential coding mechanism relying on precise spike times.Sim-ilarly,it can be understood why and under what con-ditions a population of spiking neurons responds instantaneously to changes in the input,that is,much faster than the membrane time constant that charac-terizes the passive response of the membrane.Finally, formal spiking neurons have been used for studying the functional consequences of spike timing-dependent plasticity.Spiking Neuron Models279The exampl e of oscillator y activi t y allows one to see how form a l spiking neuron s can be used to predi c t by a purel y mat h ematic a l argum e nt the firing activi t y in large networks. For the sake of sim p licity, we suppose that the netw o rk consists of N neu r ons with identical propert i es an d that every neuron is co n nected to all other neurons by synapse s of the same strength. Since we are interes t ed in oscillator y activi t y, we assum e that all neurons in the network fire at the same time, except one that lags behind by a small amou n t. Will this neuron event u ally join the group of the others? To answ e r this que s tion, we calculate the total synaptic input to this specifi c neuron, genera t ed by the a c tion potent i als in the group of syn c hronous neuron s. Know i ng the inpu t, we can deri v e from eq n [6] or eqn [7] the time course of its membrane pot e ntial, and from the time course,we can predict its firing time,that is,the moment when the membrane poten-tial hits the threshold.An analogous calculation is repeated to predict the firing time of the group of synchronous neurons and hence the timing difference between the activity of the single neuron and that of the group.The one neuron lagging behind will even-tually join the group of synchronous neurons(i.e.,the oscillation is stable)if the timing difference is reduced from one firing cycle to the next.This is just a simple example,but similar mathematical arguments can be used to predict the population activity in large net-works and answer questions such as:What is the mean firing activity of neurons in the network?Do neurons fire asynchronously,or do the firing times tend to cluster?Does the network activity show spontaneous oscillations?Will the network switch to a different state on a new input?If yes,could this explain short-term memory in neural networks?To summarize,formal spiking neuron models are a highly simplified and compressed way of describing action potential generation in real neurons.While such an approach has obvious limitations and cannot be used to study detailed properties of isolated neu-rons(such as active dendrites or effects of pharmaco-logical blockage of specific channels),the models have been useful in the past to elucidate the essence of spike generation in single neurons as well as prin-ciples of neuronal coding in large neuronal networks, and they will probably remain important tools for modeling studies in the future.See also:Action Potential Initiation and Conduction in Axons;Hodgkin–Huxley Models;Population Codes: Theoretic Aspects;Spike-Timing Dependent Plasticity (STDP);Spike-Timing-Dependent Plasticity Models.Further ReadingAbeles M(1991)Corticonics.Cambridge,UK:Cambridge Univer-sity Press.Brette R and Gerstner W(2005)Adaptive exponential integrate-and-fire model as an effective description of neuronal activity.Journal of Neurophysiology94:3637–3642.Fourcaud-Trocme N,Hansel D,van Vreeswijk C,and Brunel N (2003)How spike generation mechanisms determine the neuro-nal response to fluctuating input.Journal of Neuroscience23: 11628–11640.Gerstner W and Kistler WK(2002)Spiking Neuron Models.Cambridge,UK:Cambridge University Press.Izhikevich EM(2004)Which model to use for cortical spiking neurons?IEEE Transactions on Neural Networks15: 1063–1070.Jolivet R,Rauch A,Lscher H-R,and Gerstner W(2006)Predicting spike timing of neocortical pyramidal neurons by simple thresh-old models.Journal of Computational Neuroscience21:35–49. Koch C and Segev I(2000)The role of single neurons in informa-tion processing.Nature Neuroscience3(supplement):1171–1177.Rieke F,Warland D,de Ruyter van Steveninck R,and Bialek W (1996)Spikes Exploring the Neural Code.Cambridge,MA: MIT Press.280Spiking Neuron Models。
《神经网络与深度学习综述DeepLearning15May2014
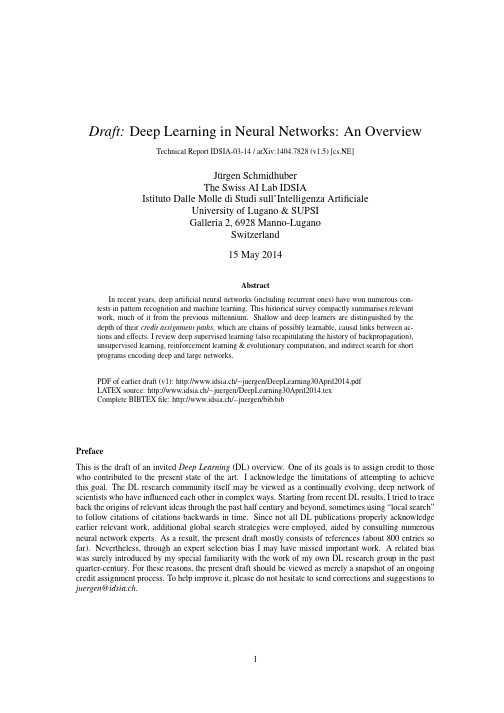
Draft:Deep Learning in Neural Networks:An OverviewTechnical Report IDSIA-03-14/arXiv:1404.7828(v1.5)[cs.NE]J¨u rgen SchmidhuberThe Swiss AI Lab IDSIAIstituto Dalle Molle di Studi sull’Intelligenza ArtificialeUniversity of Lugano&SUPSIGalleria2,6928Manno-LuganoSwitzerland15May2014AbstractIn recent years,deep artificial neural networks(including recurrent ones)have won numerous con-tests in pattern recognition and machine learning.This historical survey compactly summarises relevantwork,much of it from the previous millennium.Shallow and deep learners are distinguished by thedepth of their credit assignment paths,which are chains of possibly learnable,causal links between ac-tions and effects.I review deep supervised learning(also recapitulating the history of backpropagation),unsupervised learning,reinforcement learning&evolutionary computation,and indirect search for shortprograms encoding deep and large networks.PDF of earlier draft(v1):http://www.idsia.ch/∼juergen/DeepLearning30April2014.pdfLATEX source:http://www.idsia.ch/∼juergen/DeepLearning30April2014.texComplete BIBTEXfile:http://www.idsia.ch/∼juergen/bib.bibPrefaceThis is the draft of an invited Deep Learning(DL)overview.One of its goals is to assign credit to those who contributed to the present state of the art.I acknowledge the limitations of attempting to achieve this goal.The DL research community itself may be viewed as a continually evolving,deep network of scientists who have influenced each other in complex ways.Starting from recent DL results,I tried to trace back the origins of relevant ideas through the past half century and beyond,sometimes using“local search”to follow citations of citations backwards in time.Since not all DL publications properly acknowledge earlier relevant work,additional global search strategies were employed,aided by consulting numerous neural network experts.As a result,the present draft mostly consists of references(about800entries so far).Nevertheless,through an expert selection bias I may have missed important work.A related bias was surely introduced by my special familiarity with the work of my own DL research group in the past quarter-century.For these reasons,the present draft should be viewed as merely a snapshot of an ongoing credit assignment process.To help improve it,please do not hesitate to send corrections and suggestions to juergen@idsia.ch.Contents1Introduction to Deep Learning(DL)in Neural Networks(NNs)3 2Event-Oriented Notation for Activation Spreading in FNNs/RNNs3 3Depth of Credit Assignment Paths(CAPs)and of Problems4 4Recurring Themes of Deep Learning54.1Dynamic Programming(DP)for DL (5)4.2Unsupervised Learning(UL)Facilitating Supervised Learning(SL)and RL (6)4.3Occam’s Razor:Compression and Minimum Description Length(MDL) (6)4.4Learning Hierarchical Representations Through Deep SL,UL,RL (6)4.5Fast Graphics Processing Units(GPUs)for DL in NNs (6)5Supervised NNs,Some Helped by Unsupervised NNs75.11940s and Earlier (7)5.2Around1960:More Neurobiological Inspiration for DL (7)5.31965:Deep Networks Based on the Group Method of Data Handling(GMDH) (8)5.41979:Convolution+Weight Replication+Winner-Take-All(WTA) (8)5.51960-1981and Beyond:Development of Backpropagation(BP)for NNs (8)5.5.1BP for Weight-Sharing Feedforward NNs(FNNs)and Recurrent NNs(RNNs)..95.6Late1980s-2000:Numerous Improvements of NNs (9)5.6.1Ideas for Dealing with Long Time Lags and Deep CAPs (10)5.6.2Better BP Through Advanced Gradient Descent (10)5.6.3Discovering Low-Complexity,Problem-Solving NNs (11)5.6.4Potential Benefits of UL for SL (11)5.71987:UL Through Autoencoder(AE)Hierarchies (12)5.81989:BP for Convolutional NNs(CNNs) (13)5.91991:Fundamental Deep Learning Problem of Gradient Descent (13)5.101991:UL-Based History Compression Through a Deep Hierarchy of RNNs (14)5.111992:Max-Pooling(MP):Towards MPCNNs (14)5.121994:Contest-Winning Not So Deep NNs (15)5.131995:Supervised Recurrent Very Deep Learner(LSTM RNN) (15)5.142003:More Contest-Winning/Record-Setting,Often Not So Deep NNs (16)5.152006/7:Deep Belief Networks(DBNs)&AE Stacks Fine-Tuned by BP (17)5.162006/7:Improved CNNs/GPU-CNNs/BP-Trained MPCNNs (17)5.172009:First Official Competitions Won by RNNs,and with MPCNNs (18)5.182010:Plain Backprop(+Distortions)on GPU Yields Excellent Results (18)5.192011:MPCNNs on GPU Achieve Superhuman Vision Performance (18)5.202011:Hessian-Free Optimization for RNNs (19)5.212012:First Contests Won on ImageNet&Object Detection&Segmentation (19)5.222013-:More Contests and Benchmark Records (20)5.22.1Currently Successful Supervised Techniques:LSTM RNNs/GPU-MPCNNs (21)5.23Recent Tricks for Improving SL Deep NNs(Compare Sec.5.6.2,5.6.3) (21)5.24Consequences for Neuroscience (22)5.25DL with Spiking Neurons? (22)6DL in FNNs and RNNs for Reinforcement Learning(RL)236.1RL Through NN World Models Yields RNNs With Deep CAPs (23)6.2Deep FNNs for Traditional RL and Markov Decision Processes(MDPs) (24)6.3Deep RL RNNs for Partially Observable MDPs(POMDPs) (24)6.4RL Facilitated by Deep UL in FNNs and RNNs (25)6.5Deep Hierarchical RL(HRL)and Subgoal Learning with FNNs and RNNs (25)6.6Deep RL by Direct NN Search/Policy Gradients/Evolution (25)6.7Deep RL by Indirect Policy Search/Compressed NN Search (26)6.8Universal RL (27)7Conclusion271Introduction to Deep Learning(DL)in Neural Networks(NNs) Which modifiable components of a learning system are responsible for its success or failure?What changes to them improve performance?This has been called the fundamental credit assignment problem(Minsky, 1963).There are general credit assignment methods for universal problem solvers that are time-optimal in various theoretical senses(Sec.6.8).The present survey,however,will focus on the narrower,but now commercially important,subfield of Deep Learning(DL)in Artificial Neural Networks(NNs).We are interested in accurate credit assignment across possibly many,often nonlinear,computational stages of NNs.Shallow NN-like models have been around for many decades if not centuries(Sec.5.1).Models with several successive nonlinear layers of neurons date back at least to the1960s(Sec.5.3)and1970s(Sec.5.5). An efficient gradient descent method for teacher-based Supervised Learning(SL)in discrete,differentiable networks of arbitrary depth called backpropagation(BP)was developed in the1960s and1970s,and ap-plied to NNs in1981(Sec.5.5).BP-based training of deep NNs with many layers,however,had been found to be difficult in practice by the late1980s(Sec.5.6),and had become an explicit research subject by the early1990s(Sec.5.9).DL became practically feasible to some extent through the help of Unsupervised Learning(UL)(e.g.,Sec.5.10,5.15).The1990s and2000s also saw many improvements of purely super-vised DL(Sec.5).In the new millennium,deep NNs havefinally attracted wide-spread attention,mainly by outperforming alternative machine learning methods such as kernel machines(Vapnik,1995;Sch¨o lkopf et al.,1998)in numerous important applications.In fact,supervised deep NNs have won numerous of-ficial international pattern recognition competitions(e.g.,Sec.5.17,5.19,5.21,5.22),achieving thefirst superhuman visual pattern recognition results in limited domains(Sec.5.19).Deep NNs also have become relevant for the more generalfield of Reinforcement Learning(RL)where there is no supervising teacher (Sec.6).Both feedforward(acyclic)NNs(FNNs)and recurrent(cyclic)NNs(RNNs)have won contests(Sec.5.12,5.14,5.17,5.19,5.21,5.22).In a sense,RNNs are the deepest of all NNs(Sec.3)—they are general computers more powerful than FNNs,and can in principle create and process memories of ar-bitrary sequences of input patterns(e.g.,Siegelmann and Sontag,1991;Schmidhuber,1990a).Unlike traditional methods for automatic sequential program synthesis(e.g.,Waldinger and Lee,1969;Balzer, 1985;Soloway,1986;Deville and Lau,1994),RNNs can learn programs that mix sequential and parallel information processing in a natural and efficient way,exploiting the massive parallelism viewed as crucial for sustaining the rapid decline of computation cost observed over the past75years.The rest of this paper is structured as follows.Sec.2introduces a compact,event-oriented notation that is simple yet general enough to accommodate both FNNs and RNNs.Sec.3introduces the concept of Credit Assignment Paths(CAPs)to measure whether learning in a given NN application is of the deep or shallow type.Sec.4lists recurring themes of DL in SL,UL,and RL.Sec.5focuses on SL and UL,and on how UL can facilitate SL,although pure SL has become dominant in recent competitions(Sec.5.17-5.22). Sec.5is arranged in a historical timeline format with subsections on important inspirations and technical contributions.Sec.6on deep RL discusses traditional Dynamic Programming(DP)-based RL combined with gradient-based search techniques for SL or UL in deep NNs,as well as general methods for direct and indirect search in the weight space of deep FNNs and RNNs,including successful policy gradient and evolutionary methods.2Event-Oriented Notation for Activation Spreading in FNNs/RNNs Throughout this paper,let i,j,k,t,p,q,r denote positive integer variables assuming ranges implicit in the given contexts.Let n,m,T denote positive integer constants.An NN’s topology may change over time(e.g.,Fahlman,1991;Ring,1991;Weng et al.,1992;Fritzke, 1994).At any given moment,it can be described as afinite subset of units(or nodes or neurons)N= {u1,u2,...,}and afinite set H⊆N×N of directed edges or connections between nodes.FNNs are acyclic graphs,RNNs cyclic.Thefirst(input)layer is the set of input units,a subset of N.In FNNs,the k-th layer(k>1)is the set of all nodes u∈N such that there is an edge path of length k−1(but no longer path)between some input unit and u.There may be shortcut connections between distant layers.The NN’s behavior or program is determined by a set of real-valued,possibly modifiable,parameters or weights w i(i=1,...,n).We now focus on a singlefinite episode or epoch of information processing and activation spreading,without learning through weight changes.The following slightly unconventional notation is designed to compactly describe what is happening during the runtime of the system.During an episode,there is a partially causal sequence x t(t=1,...,T)of real values that I call events.Each x t is either an input set by the environment,or the activation of a unit that may directly depend on other x k(k<t)through a current NN topology-dependent set in t of indices k representing incoming causal connections or links.Let the function v encode topology information and map such event index pairs(k,t)to weight indices.For example,in the non-input case we may have x t=f t(net t)with real-valued net t= k∈in t x k w v(k,t)(additive case)or net t= k∈in t x k w v(k,t)(multiplicative case), where f t is a typically nonlinear real-valued activation function such as tanh.In many recent competition-winning NNs(Sec.5.19,5.21,5.22)there also are events of the type x t=max k∈int (x k);some networktypes may also use complex polynomial activation functions(Sec.5.3).x t may directly affect certain x k(k>t)through outgoing connections or links represented through a current set out t of indices k with t∈in k.Some non-input events are called output events.Note that many of the x t may refer to different,time-varying activations of the same unit in sequence-processing RNNs(e.g.,Williams,1989,“unfolding in time”),or also in FNNs sequentially exposed to time-varying input patterns of a large training set encoded as input events.During an episode,the same weight may get reused over and over again in topology-dependent ways,e.g.,in RNNs,or in convolutional NNs(Sec.5.4,5.8).I call this weight sharing across space and/or time.Weight sharing may greatly reduce the NN’s descriptive complexity,which is the number of bits of information required to describe the NN (Sec.4.3).In Supervised Learning(SL),certain NN output events x t may be associated with teacher-given,real-valued labels or targets d t yielding errors e t,e.g.,e t=1/2(x t−d t)2.A typical goal of supervised NN training is tofind weights that yield episodes with small total error E,the sum of all such e t.The hope is that the NN will generalize well in later episodes,causing only small errors on previously unseen sequences of input events.Many alternative error functions for SL and UL are possible.SL assumes that input events are independent of earlier output events(which may affect the environ-ment through actions causing subsequent perceptions).This assumption does not hold in the broaderfields of Sequential Decision Making and Reinforcement Learning(RL)(Kaelbling et al.,1996;Sutton and Barto, 1998;Hutter,2005)(Sec.6).In RL,some of the input events may encode real-valued reward signals given by the environment,and a typical goal is tofind weights that yield episodes with a high sum of reward signals,through sequences of appropriate output actions.Sec.5.5will use the notation above to compactly describe a central algorithm of DL,namely,back-propagation(BP)for supervised weight-sharing FNNs and RNNs.(FNNs may be viewed as RNNs with certainfixed zero weights.)Sec.6will address the more general RL case.3Depth of Credit Assignment Paths(CAPs)and of ProblemsTo measure whether credit assignment in a given NN application is of the deep or shallow type,I introduce the concept of Credit Assignment Paths or CAPs,which are chains of possibly causal links between events.Let usfirst focus on SL.Consider two events x p and x q(1≤p<q≤T).Depending on the appli-cation,they may have a Potential Direct Causal Connection(PDCC)expressed by the Boolean predicate pdcc(p,q),which is true if and only if p∈in q.Then the2-element list(p,q)is defined to be a CAP from p to q(a minimal one).A learning algorithm may be allowed to change w v(p,q)to improve performance in future episodes.More general,possibly indirect,Potential Causal Connections(PCC)are expressed by the recursively defined Boolean predicate pcc(p,q),which in the SL case is true only if pdcc(p,q),or if pcc(p,k)for some k and pdcc(k,q).In the latter case,appending q to any CAP from p to k yields a CAP from p to q(this is a recursive definition,too).The set of such CAPs may be large but isfinite.Note that the same weight may affect many different PDCCs between successive events listed by a given CAP,e.g.,in the case of RNNs, or weight-sharing FNNs.Suppose a CAP has the form(...,k,t,...,q),where k and t(possibly t=q)are thefirst successive elements with modifiable w v(k,t).Then the length of the suffix list(t,...,q)is called the CAP’s depth (which is0if there are no modifiable links at all).This depth limits how far backwards credit assignment can move down the causal chain tofind a modifiable weight.1Suppose an episode and its event sequence x1,...,x T satisfy a computable criterion used to decide whether a given problem has been solved(e.g.,total error E below some threshold).Then the set of used weights is called a solution to the problem,and the depth of the deepest CAP within the sequence is called the solution’s depth.There may be other solutions(yielding different event sequences)with different depths.Given somefixed NN topology,the smallest depth of any solution is called the problem’s depth.Sometimes we also speak of the depth of an architecture:SL FNNs withfixed topology imply a problem-independent maximal problem depth bounded by the number of non-input layers.Certain SL RNNs withfixed weights for all connections except those to output units(Jaeger,2001;Maass et al.,2002; Jaeger,2004;Schrauwen et al.,2007)have a maximal problem depth of1,because only thefinal links in the corresponding CAPs are modifiable.In general,however,RNNs may learn to solve problems of potentially unlimited depth.Note that the definitions above are solely based on the depths of causal chains,and agnostic of the temporal distance between events.For example,shallow FNNs perceiving large“time windows”of in-put events may correctly classify long input sequences through appropriate output events,and thus solve shallow problems involving long time lags between relevant events.At which problem depth does Shallow Learning end,and Deep Learning begin?Discussions with DL experts have not yet yielded a conclusive response to this question.Instead of committing myself to a precise answer,let me just define for the purposes of this overview:problems of depth>10require Very Deep Learning.The difficulty of a problem may have little to do with its depth.Some NNs can quickly learn to solve certain deep problems,e.g.,through random weight guessing(Sec.5.9)or other types of direct search (Sec.6.6)or indirect search(Sec.6.7)in weight space,or through training an NNfirst on shallow problems whose solutions may then generalize to deep problems,or through collapsing sequences of(non)linear operations into a single(non)linear operation—but see an analysis of non-trivial aspects of deep linear networks(Baldi and Hornik,1994,Section B).In general,however,finding an NN that precisely models a given training set is an NP-complete problem(Judd,1990;Blum and Rivest,1992),also in the case of deep NNs(S´ıma,1994;de Souto et al.,1999;Windisch,2005);compare a survey of negative results(S´ıma, 2002,Section1).Above we have focused on SL.In the more general case of RL in unknown environments,pcc(p,q) is also true if x p is an output event and x q any later input event—any action may affect the environment and thus any later perception.(In the real world,the environment may even influence non-input events computed on a physical hardware entangled with the entire universe,but this is ignored here.)It is possible to model and replace such unmodifiable environmental PCCs through a part of the NN that has already learned to predict(through some of its units)input events(including reward signals)from former input events and actions(Sec.6.1).Its weights are frozen,but can help to assign credit to other,still modifiable weights used to compute actions(Sec.6.1).This approach may lead to very deep CAPs though.Some DL research is about automatically rephrasing problems such that their depth is reduced(Sec.4). In particular,sometimes UL is used to make SL problems less deep,e.g.,Sec.5.10.Often Dynamic Programming(Sec.4.1)is used to facilitate certain traditional RL problems,e.g.,Sec.6.2.Sec.5focuses on CAPs for SL,Sec.6on the more complex case of RL.4Recurring Themes of Deep Learning4.1Dynamic Programming(DP)for DLOne recurring theme of DL is Dynamic Programming(DP)(Bellman,1957),which can help to facili-tate credit assignment under certain assumptions.For example,in SL NNs,backpropagation itself can 1An alternative would be to count only modifiable links when measuring depth.In many typical NN applications this would not make a difference,but in some it would,e.g.,Sec.6.1.be viewed as a DP-derived method(Sec.5.5).In traditional RL based on strong Markovian assumptions, DP-derived methods can help to greatly reduce problem depth(Sec.6.2).DP algorithms are also essen-tial for systems that combine concepts of NNs and graphical models,such as Hidden Markov Models (HMMs)(Stratonovich,1960;Baum and Petrie,1966)and Expectation Maximization(EM)(Dempster et al.,1977),e.g.,(Bottou,1991;Bengio,1991;Bourlard and Morgan,1994;Baldi and Chauvin,1996; Jordan and Sejnowski,2001;Bishop,2006;Poon and Domingos,2011;Dahl et al.,2012;Hinton et al., 2012a).4.2Unsupervised Learning(UL)Facilitating Supervised Learning(SL)and RL Another recurring theme is how UL can facilitate both SL(Sec.5)and RL(Sec.6).UL(Sec.5.6.4) is normally used to encode raw incoming data such as video or speech streams in a form that is more convenient for subsequent goal-directed learning.In particular,codes that describe the original data in a less redundant or more compact way can be fed into SL(Sec.5.10,5.15)or RL machines(Sec.6.4),whose search spaces may thus become smaller(and whose CAPs shallower)than those necessary for dealing with the raw data.UL is closely connected to the topics of regularization and compression(Sec.4.3,5.6.3). 4.3Occam’s Razor:Compression and Minimum Description Length(MDL) Occam’s razor favors simple solutions over complex ones.Given some programming language,the prin-ciple of Minimum Description Length(MDL)can be used to measure the complexity of a solution candi-date by the length of the shortest program that computes it(e.g.,Solomonoff,1964;Kolmogorov,1965b; Chaitin,1966;Wallace and Boulton,1968;Levin,1973a;Rissanen,1986;Blumer et al.,1987;Li and Vit´a nyi,1997;Gr¨u nwald et al.,2005).Some methods explicitly take into account program runtime(Al-lender,1992;Watanabe,1992;Schmidhuber,2002,1995);many consider only programs with constant runtime,written in non-universal programming languages(e.g.,Rissanen,1986;Hinton and van Camp, 1993).In the NN case,the MDL principle suggests that low NN weight complexity corresponds to high NN probability in the Bayesian view(e.g.,MacKay,1992;Buntine and Weigend,1991;De Freitas,2003), and to high generalization performance(e.g.,Baum and Haussler,1989),without overfitting the training data.Many methods have been proposed for regularizing NNs,that is,searching for solution-computing, low-complexity SL NNs(Sec.5.6.3)and RL NNs(Sec.6.7).This is closely related to certain UL methods (Sec.4.2,5.6.4).4.4Learning Hierarchical Representations Through Deep SL,UL,RLMany methods of Good Old-Fashioned Artificial Intelligence(GOFAI)(Nilsson,1980)as well as more recent approaches to AI(Russell et al.,1995)and Machine Learning(Mitchell,1997)learn hierarchies of more and more abstract data representations.For example,certain methods of syntactic pattern recog-nition(Fu,1977)such as grammar induction discover hierarchies of formal rules to model observations. The partially(un)supervised Automated Mathematician/EURISKO(Lenat,1983;Lenat and Brown,1984) continually learns concepts by combining previously learnt concepts.Such hierarchical representation learning(Ring,1994;Bengio et al.,2013;Deng and Yu,2014)is also a recurring theme of DL NNs for SL (Sec.5),UL-aided SL(Sec.5.7,5.10,5.15),and hierarchical RL(Sec.6.5).Often,abstract hierarchical representations are natural by-products of data compression(Sec.4.3),e.g.,Sec.5.10.4.5Fast Graphics Processing Units(GPUs)for DL in NNsWhile the previous millennium saw several attempts at creating fast NN-specific hardware(e.g.,Jackel et al.,1990;Faggin,1992;Ramacher et al.,1993;Widrow et al.,1994;Heemskerk,1995;Korkin et al., 1997;Urlbe,1999),and at exploiting standard hardware(e.g.,Anguita et al.,1994;Muller et al.,1995; Anguita and Gomes,1996),the new millennium brought a DL breakthrough in form of cheap,multi-processor graphics cards or GPUs.GPUs are widely used for video games,a huge and competitive market that has driven down hardware prices.GPUs excel at fast matrix and vector multiplications required not only for convincing virtual realities but also for NN training,where they can speed up learning by a factorof50and more.Some of the GPU-based FNN implementations(Sec.5.16-5.19)have greatly contributed to recent successes in contests for pattern recognition(Sec.5.19-5.22),image segmentation(Sec.5.21), and object detection(Sec.5.21-5.22).5Supervised NNs,Some Helped by Unsupervised NNsThe main focus of current practical applications is on Supervised Learning(SL),which has dominated re-cent pattern recognition contests(Sec.5.17-5.22).Several methods,however,use additional Unsupervised Learning(UL)to facilitate SL(Sec.5.7,5.10,5.15).It does make sense to treat SL and UL in the same section:often gradient-based methods,such as BP(Sec.5.5.1),are used to optimize objective functions of both UL and SL,and the boundary between SL and UL may blur,for example,when it comes to time series prediction and sequence classification,e.g.,Sec.5.10,5.12.A historical timeline format will help to arrange subsections on important inspirations and techni-cal contributions(although such a subsection may span a time interval of many years).Sec.5.1briefly mentions early,shallow NN models since the1940s,Sec.5.2additional early neurobiological inspiration relevant for modern Deep Learning(DL).Sec.5.3is about GMDH networks(since1965),perhaps thefirst (feedforward)DL systems.Sec.5.4is about the relatively deep Neocognitron NN(1979)which is similar to certain modern deep FNN architectures,as it combines convolutional NNs(CNNs),weight pattern repli-cation,and winner-take-all(WTA)mechanisms.Sec.5.5uses the notation of Sec.2to compactly describe a central algorithm of DL,namely,backpropagation(BP)for supervised weight-sharing FNNs and RNNs. It also summarizes the history of BP1960-1981and beyond.Sec.5.6describes problems encountered in the late1980s with BP for deep NNs,and mentions several ideas from the previous millennium to overcome them.Sec.5.7discusses afirst hierarchical stack of coupled UL-based Autoencoders(AEs)—this concept resurfaced in the new millennium(Sec.5.15).Sec.5.8is about applying BP to CNNs,which is important for today’s DL applications.Sec.5.9explains BP’s Fundamental DL Problem(of vanishing/exploding gradients)discovered in1991.Sec.5.10explains how a deep RNN stack of1991(the History Compressor) pre-trained by UL helped to solve previously unlearnable DL benchmarks requiring Credit Assignment Paths(CAPs,Sec.3)of depth1000and more.Sec.5.11discusses a particular WTA method called Max-Pooling(MP)important in today’s DL FNNs.Sec.5.12mentions afirst important contest won by SL NNs in1994.Sec.5.13describes a purely supervised DL RNN(Long Short-Term Memory,LSTM)for problems of depth1000and more.Sec.5.14mentions an early contest of2003won by an ensemble of shallow NNs, as well as good pattern recognition results with CNNs and LSTM RNNs(2003).Sec.5.15is mostly about Deep Belief Networks(DBNs,2006)and related stacks of Autoencoders(AEs,Sec.5.7)pre-trained by UL to facilitate BP-based SL.Sec.5.16mentions thefirst BP-trained MPCNNs(2007)and GPU-CNNs(2006). Sec.5.17-5.22focus on official competitions with secret test sets won by(mostly purely supervised)DL NNs since2009,in sequence recognition,image classification,image segmentation,and object detection. Many RNN results depended on LSTM(Sec.5.13);many FNN results depended on GPU-based FNN code developed since2004(Sec.5.16,5.17,5.18,5.19),in particular,GPU-MPCNNs(Sec.5.19).5.11940s and EarlierNN research started in the1940s(e.g.,McCulloch and Pitts,1943;Hebb,1949);compare also later work on learning NNs(Rosenblatt,1958,1962;Widrow and Hoff,1962;Grossberg,1969;Kohonen,1972; von der Malsburg,1973;Narendra and Thathatchar,1974;Willshaw and von der Malsburg,1976;Palm, 1980;Hopfield,1982).In a sense NNs have been around even longer,since early supervised NNs were essentially variants of linear regression methods going back at least to the early1800s(e.g.,Legendre, 1805;Gauss,1809,1821).Early NNs had a maximal CAP depth of1(Sec.3).5.2Around1960:More Neurobiological Inspiration for DLSimple cells and complex cells were found in the cat’s visual cortex(e.g.,Hubel and Wiesel,1962;Wiesel and Hubel,1959).These cellsfire in response to certain properties of visual sensory inputs,such as theorientation of plex cells exhibit more spatial invariance than simple cells.This inspired later deep NN architectures(Sec.5.4)used in certain modern award-winning Deep Learners(Sec.5.19-5.22).5.31965:Deep Networks Based on the Group Method of Data Handling(GMDH) Networks trained by the Group Method of Data Handling(GMDH)(Ivakhnenko and Lapa,1965; Ivakhnenko et al.,1967;Ivakhnenko,1968,1971)were perhaps thefirst DL systems of the Feedforward Multilayer Perceptron type.The units of GMDH nets may have polynomial activation functions imple-menting Kolmogorov-Gabor polynomials(more general than traditional NN activation functions).Given a training set,layers are incrementally grown and trained by regression analysis,then pruned with the help of a separate validation set(using today’s terminology),where Decision Regularisation is used to weed out superfluous units.The numbers of layers and units per layer can be learned in problem-dependent fashion. This is a good example of hierarchical representation learning(Sec.4.4).There have been numerous ap-plications of GMDH-style networks,e.g.(Ikeda et al.,1976;Farlow,1984;Madala and Ivakhnenko,1994; Ivakhnenko,1995;Kondo,1998;Kord´ık et al.,2003;Witczak et al.,2006;Kondo and Ueno,2008).5.41979:Convolution+Weight Replication+Winner-Take-All(WTA)Apart from deep GMDH networks(Sec.5.3),the Neocognitron(Fukushima,1979,1980,2013a)was per-haps thefirst artificial NN that deserved the attribute deep,and thefirst to incorporate the neurophysiolog-ical insights of Sec.5.2.It introduced convolutional NNs(today often called CNNs or convnets),where the(typically rectangular)receptivefield of a convolutional unit with given weight vector is shifted step by step across a2-dimensional array of input values,such as the pixels of an image.The resulting2D array of subsequent activation events of this unit can then provide inputs to higher-level units,and so on.Due to massive weight replication(Sec.2),relatively few parameters may be necessary to describe the behavior of such a convolutional layer.Competition layers have WTA subsets whose maximally active units are the only ones to adopt non-zero activation values.They essentially“down-sample”the competition layer’s input.This helps to create units whose responses are insensitive to small image shifts(compare Sec.5.2).The Neocognitron is very similar to the architecture of modern,contest-winning,purely super-vised,feedforward,gradient-based Deep Learners with alternating convolutional and competition lay-ers(e.g.,Sec.5.19-5.22).Fukushima,however,did not set the weights by supervised backpropagation (Sec.5.5,5.8),but by local un supervised learning rules(e.g.,Fukushima,2013b),or by pre-wiring.In that sense he did not care for the DL problem(Sec.5.9),although his architecture was comparatively deep indeed.He also used Spatial Averaging(Fukushima,1980,2011)instead of Max-Pooling(MP,Sec.5.11), currently a particularly convenient and popular WTA mechanism.Today’s CNN-based DL machines profita lot from later CNN work(e.g.,LeCun et al.,1989;Ranzato et al.,2007)(Sec.5.8,5.16,5.19).5.51960-1981and Beyond:Development of Backpropagation(BP)for NNsThe minimisation of errors through gradient descent(Hadamard,1908)in the parameter space of com-plex,nonlinear,differentiable,multi-stage,NN-related systems has been discussed at least since the early 1960s(e.g.,Kelley,1960;Bryson,1961;Bryson and Denham,1961;Pontryagin et al.,1961;Dreyfus,1962; Wilkinson,1965;Amari,1967;Bryson and Ho,1969;Director and Rohrer,1969;Griewank,2012),ini-tially within the framework of Euler-LaGrange equations in the Calculus of Variations(e.g.,Euler,1744). Steepest descent in such systems can be performed(Bryson,1961;Kelley,1960;Bryson and Ho,1969)by iterating the ancient chain rule(Leibniz,1676;L’Hˆo pital,1696)in Dynamic Programming(DP)style(Bell-man,1957).A simplified derivation of the method uses the chain rule only(Dreyfus,1962).The methods of the1960s were already efficient in the DP sense.However,they backpropagated derivative information through standard Jacobian matrix calculations from one“layer”to the previous one, explicitly addressing neither direct links across several layers nor potential additional efficiency gains due to network sparsity(but perhaps such enhancements seemed obvious to the authors).。
Spiking神经网络学习算法及其应用研究
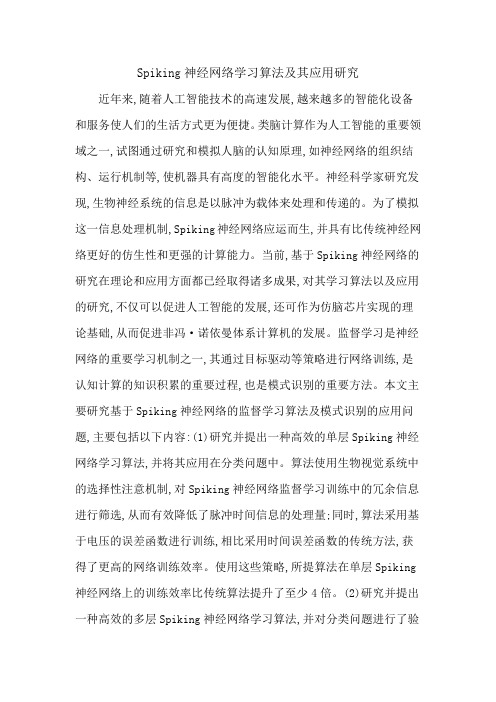
Spiking神经网络学习算法及其应用研究近年来,随着人工智能技术的高速发展,越来越多的智能化设备和服务使人们的生活方式更为便捷。
类脑计算作为人工智能的重要领域之一,试图通过研究和模拟人脑的认知原理,如神经网络的组织结构、运行机制等,使机器具有高度的智能化水平。
神经科学家研究发现,生物神经系统的信息是以脉冲为载体来处理和传递的。
为了模拟这一信息处理机制,Spiking神经网络应运而生,并具有比传统神经网络更好的仿生性和更强的计算能力。
当前,基于Spiking神经网络的研究在理论和应用方面都已经取得诸多成果,对其学习算法以及应用的研究,不仅可以促进人工智能的发展,还可作为仿脑芯片实现的理论基础,从而促进非冯·诺依曼体系计算机的发展。
监督学习是神经网络的重要学习机制之一,其通过目标驱动等策略进行网络训练,是认知计算的知识积累的重要过程,也是模式识别的重要方法。
本文主要研究基于Spiking神经网络的监督学习算法及模式识别的应用问题,主要包括以下内容:(1)研究并提出一种高效的单层Spiking神经网络学习算法,并将其应用在分类问题中。
算法使用生物视觉系统中的选择性注意机制,对Spiking神经网络监督学习训练中的冗余信息进行筛选,从而有效降低了脉冲时间信息的处理量;同时,算法采用基于电压的误差函数进行训练,相比采用时间误差函数的传统方法,获得了更高的网络训练效率。
使用这些策略,所提算法在单层Spiking 神经网络上的训练效率比传统算法提升了至少4倍。
(2)研究并提出一种高效的多层Spiking神经网络学习算法,并对分类问题进行了验证。
针对传统学习算法中电压函数不连续导致的误差反传效率低下问题,算法创新使用前突触脉冲的时间移动策略对训练误差进行反传;同时,算法采用归一化的误差参数以及选择性注意机制对传统训练过程中的冗余信息进行有效筛选;这些策略大幅度提升了Spiking神经网络的多层训练效率。
一种新的部分神经进化网络的股票预测(英文)
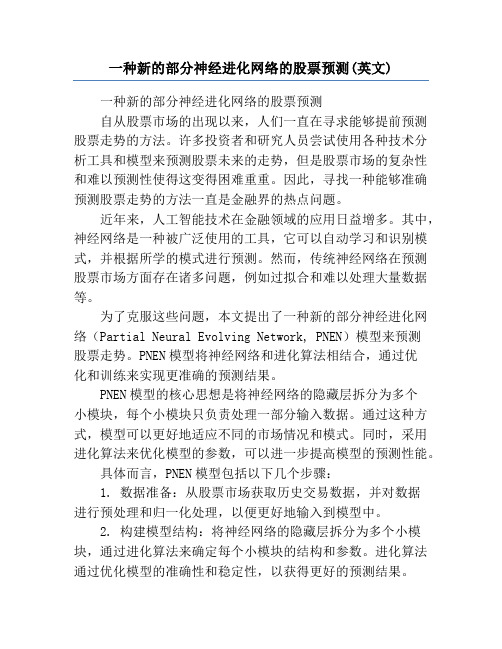
一种新的部分神经进化网络的股票预测(英文)一种新的部分神经进化网络的股票预测自从股票市场的出现以来,人们一直在寻求能够提前预测股票走势的方法。
许多投资者和研究人员尝试使用各种技术分析工具和模型来预测股票未来的走势,但是股票市场的复杂性和难以预测性使得这变得困难重重。
因此,寻找一种能够准确预测股票走势的方法一直是金融界的热点问题。
近年来,人工智能技术在金融领域的应用日益增多。
其中,神经网络是一种被广泛使用的工具,它可以自动学习和识别模式,并根据所学的模式进行预测。
然而,传统神经网络在预测股票市场方面存在诸多问题,例如过拟合和难以处理大量数据等。
为了克服这些问题,本文提出了一种新的部分神经进化网络(Partial Neural Evolving Network, PNEN)模型来预测股票走势。
PNEN模型将神经网络和进化算法相结合,通过优化和训练来实现更准确的预测结果。
PNEN模型的核心思想是将神经网络的隐藏层拆分为多个小模块,每个小模块只负责处理一部分输入数据。
通过这种方式,模型可以更好地适应不同的市场情况和模式。
同时,采用进化算法来优化模型的参数,可以进一步提高模型的预测性能。
具体而言,PNEN模型包括以下几个步骤:1. 数据准备:从股票市场获取历史交易数据,并对数据进行预处理和归一化处理,以便更好地输入到模型中。
2. 构建模型结构:将神经网络的隐藏层拆分为多个小模块,通过进化算法来确定每个小模块的结构和参数。
进化算法通过优化模型的准确性和稳定性,以获得更好的预测结果。
3. 训练模型:使用历史数据集对模型进行训练,并通过反向传播算法来更新模型的权重和偏置。
同时,通过与进化算法的交互,不断调整模型结构和参数。
4. 预测结果:使用训练好的模型对未来的股票走势进行预测。
通过模型对市场的分析和判断,可以为投资者提供决策参考。
为了验证PNEN模型的效果,我们在实际的股票市场数据上进行了实验。
结果表明,与传统神经网络模型相比,PNEN 模型在预测股票走势方面具有更好的准确性和稳定性。
人工智能岗位招聘笔试题与参考答案(某大型集团公司)
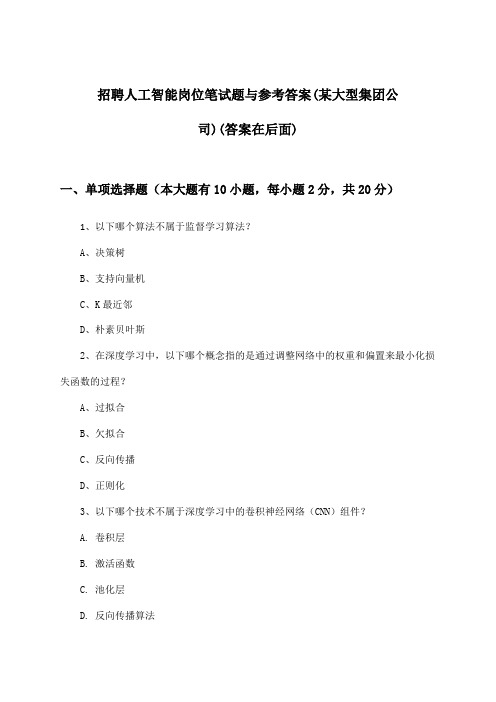
招聘人工智能岗位笔试题与参考答案(某大型集团公司)(答案在后面)一、单项选择题(本大题有10小题,每小题2分,共20分)1、以下哪个算法不属于监督学习算法?A、决策树B、支持向量机C、K最近邻D、朴素贝叶斯2、在深度学习中,以下哪个概念指的是通过调整网络中的权重和偏置来最小化损失函数的过程?A、过拟合B、欠拟合C、反向传播D、正则化3、以下哪个技术不属于深度学习中的卷积神经网络(CNN)组件?A. 卷积层B. 激活函数C. 池化层D. 反向传播算法4、在自然语言处理(NLP)中,以下哪种模型通常用于文本分类任务?A. 决策树B. 朴素贝叶斯C. 支持向量机D. 长短期记忆网络(LSTM)5、题干:以下哪项不属于人工智能的核心技术?A. 机器学习B. 深度学习C. 数据挖掘D. 计算机视觉6、题干:以下哪个算法在处理大规模数据集时,通常比其他算法更具有效率?A. K最近邻(K-Nearest Neighbors, KNN)B. 支持向量机(Support Vector Machines, SVM)C. 决策树(Decision Tree)D. 随机森林(Random Forest)7、以下哪个技术不属于深度学习领域?A. 卷积神经网络(CNN)B. 支持向量机(SVM)C. 递归神经网络(RNN)D. 随机梯度下降(SGD)8、以下哪个算法不是用于无监督学习的?A. K-均值聚类(K-means)B. 决策树(Decision Tree)C. 主成分分析(PCA)D. 聚类层次法(Hierarchical Clustering)9、以下哪个技术不属于深度学习中的神经网络层?A. 卷积层(Convolutional Layer)B. 循环层(Recurrent Layer)C. 线性层(Linear Layer)D. 随机梯度下降法(Stochastic Gradient Descent)二、多项选择题(本大题有10小题,每小题4分,共40分)1、以下哪些技术或方法通常用于提升机器学习模型的性能?()A、特征工程B、数据增强C、集成学习D、正则化E、迁移学习2、以下关于深度学习的描述,哪些是正确的?()A、深度学习是一种特殊的机器学习方法,它通过多层神经网络来提取特征。
Neural Networks Mimicking the Mind
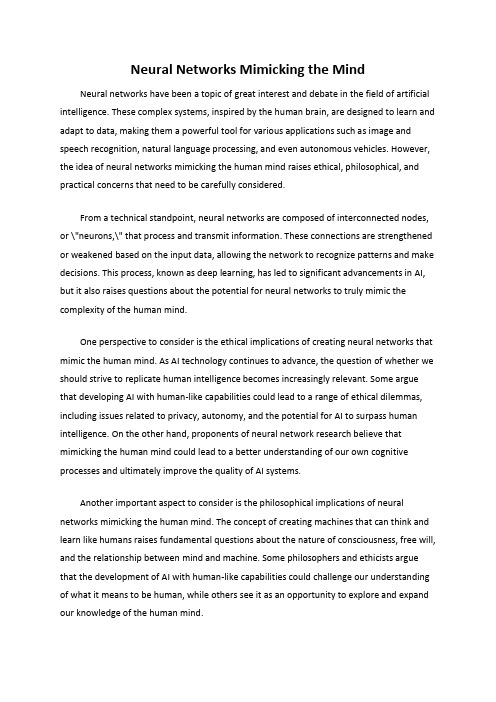
Neural Networks Mimicking the Mind Neural networks have been a topic of great interest and debate in the field of artificial intelligence. These complex systems, inspired by the human brain, are designed to learn and adapt to data, making them a powerful tool for various applications such as image and speech recognition, natural language processing, and even autonomous vehicles. However, the idea of neural networks mimicking the human mind raises ethical, philosophical, and practical concerns that need to be carefully considered.From a technical standpoint, neural networks are composed of interconnected nodes, or \"neurons,\" that process and transmit information. These connections are strengthened or weakened based on the input data, allowing the network to recognize patterns and make decisions. This process, known as deep learning, has led to significant advancements in AI, but it also raises questions about the potential for neural networks to truly mimic the complexity of the human mind.One perspective to consider is the ethical implications of creating neural networks that mimic the human mind. As AI technology continues to advance, the question of whether we should strive to replicate human intelligence becomes increasingly relevant. Some argue that developing AI with human-like capabilities could lead to a range of ethical dilemmas, including issues related to privacy, autonomy, and the potential for AI to surpass human intelligence. On the other hand, proponents of neural network research believe that mimicking the human mind could lead to a better understanding of our own cognitive processes and ultimately improve the quality of AI systems.Another important aspect to consider is the philosophical implications of neural networks mimicking the human mind. The concept of creating machines that can think and learn like humans raises fundamental questions about the nature of consciousness, free will, and the relationship between mind and machine. Some philosophers and ethicists argue that the development of AI with human-like capabilities could challenge our understanding of what it means to be human, while others see it as an opportunity to explore and expand our knowledge of the human mind.From a practical standpoint, the potential applications of neural networks that mimic the human mind are vast and varied. For example, in the field of healthcare, AI systems with human-like cognitive abilities could revolutionize medical diagnosis and treatment planning. Similarly, in the realm of customer service, AI chatbots that can understand and respond to human emotions and intentions could greatly improve the user experience. However, these advancements also raise concerns about the potential for AI to replace human jobs and the need for regulations to ensure the responsible use of this technology.In conclusion, the idea of neural networks mimicking the human mind is a complex and multifaceted issue that requires careful consideration from technical, ethical, philosophical, and practical perspectives. While the potential benefits of developing AI with human-like capabilities are significant, it is essential to approach this technology with a thoughtful and cautious mindset. As we continue to explore the potential of neural networks, it is crucial to engage in open and honest discussions about the implications and limitations of creating machines that can think and learn like humans. Only by doing so can we ensure that AI technology is developed and used in a way that aligns with our values and benefits society as a whole.。
SPIKING NEURAL NETWORK
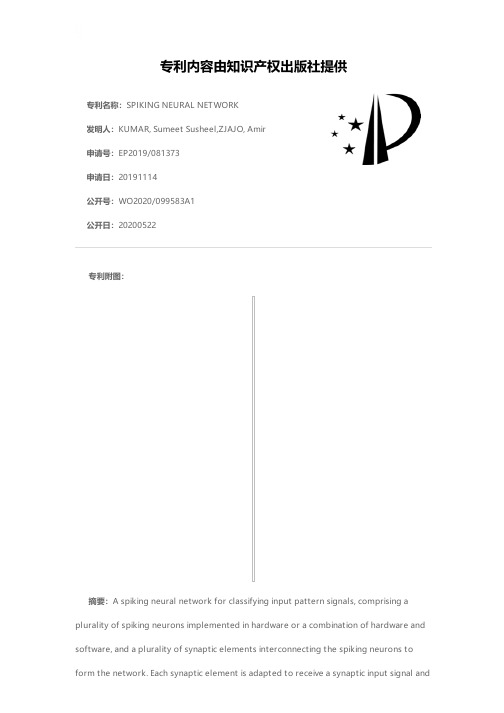
专利名称:SPIKING NEURAL NETWORK发明人:KUMAR, Sumeet Susheel,ZJAJO, Amir 申请号:EP2019/081373申请日:20191114公开号:WO2020/099583A1公开日:20200522专利内容由知识产权出版社提供专利附图:摘要:A spiking neural network for classifying input pattern signals, comprising a plurality of spiking neurons implemented in hardware or a combination of hardware and software, and a plurality of synaptic elements interconnecting the spiking neurons to form the network. Each synaptic element is adapted to receive a synaptic input signal andapply a weight to the synaptic input signal to generate a synaptic output signal, the synaptic elements being configurable to adjust the weight applied by each synaptic element, and each of the spiking neurons is adapted to receive one or more of the synaptic output signals from one or more of the synaptic elements, and generate a spatio-temporal spike train output signal in response to the received one or more synaptic output signals.申请人:INNATERA NANOSYSTEMS B.V.地址:Mekelweg 4 (TUD-EWI-CAS) 2628CD DELFT NL国籍:NL代理人:HOYNG ROKH MONEGIER LLP更多信息请下载全文后查看。
Spiking神经网络及其在图像分割中的应用
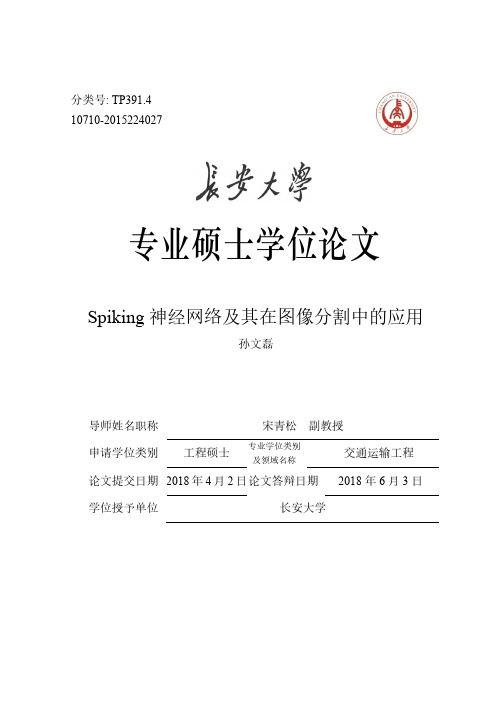
长安大学
SPIKING NEURAL NETWORKS AND ITS APPLICATION IN IMAGE SEGMENTATION
A Thesis Submitted for the Degree of Master
Candidate:Sun Wenlei Supervisor:A/Prof.Song Qingsong Chang’an University, Xi’an, China
ii
calculated the superpixel and constructed the Spiking-SOM neural network, besides, the network weight matrix was initialized and the Hebbian rules training network is adopted. Last, realized this method simulation and made the comparative evaluation with other methods.
Spiking机器学习算法

汇报人: 日期:
目录
• spiking神经元模型 • 机器学习算法概述 • spiking机器学习算法的原理 • spiking机器学习算法的实现方法 • spiking机器学习算法的优化策略 • spiking机器学习算法的前景与挑战
01
spiking神经元模型
神经元模型的基本概念
01
02
03
神经元
神经元是构成神经系统基 本单元的结构,它能够接 收、传递并处理来自外界 的信息。
突触
突触是神经元之间的连接 ,它能够传递电信号或者 化学信号。
膜电位
膜电位是神经元细胞膜内 外两侧的电位差,它能够 影响神经元的兴奋性。
spiking神经元模型的特点
脉冲输出
Spiking神经元模型以脉冲的 形式输出信号,这种输出方式 更接近于生物神经元的实际工
根据输入信息的时序依赖关系,决定神经元的发放状态。
04
spiking机器学习算法 的实现方法
基于模拟的spiking机器学习算法实现方法
1 2
模拟神经元
模拟神经元是spiking机器学习算法的基础,可 以通过模拟神经元的电位变化和脉冲发放来实现 学习算法。
基于脉冲发放的学习算法
利用神经元的脉冲发放进行学习,通过调整神经 元的阈值和脉冲发放频率来达到学习目的。
利用数值计算方法实现神经元的电位变化和脉冲发放
利用数值计算方法如Euler法、Runge-Kutta法等实现对神经元电位变化的数值模拟,并利用模拟结果控制神经 元的脉冲发放。
基于数值优化方法的spiking机器学习算法
利用数值优化方法如梯度下降法、牛顿法等实现对神经元参数的优化,从而实现对输入信号的分类和识别。
Spiking神经网络学习算法及其应用
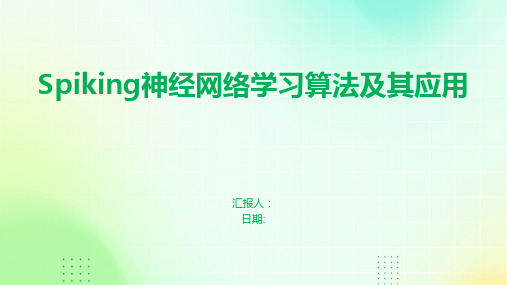
脑机接口
利用Spiking神经网络模拟生物 大脑的信息处理机制,实现更高 效的脑机接口技术,为医疗康复 、娱乐产业等领域提供创新解决
方案。
硬件实现与部署
专用芯片设计
针对Spiking神经网络的计算特点,设计专用的硬件芯片 ,提高计算效率,降低成本,推动Spiking神经网络的广 泛应用。
神经拟态计算硬件
能源效率
相较于传统神经网络,Spiking神经 网络在处理任务时具有更高的能源效 率,符合低功耗计算的需求。
生物可解释性
Spiking神经网络由于更接近生物大 脑的结构和工作机制,因此具有更好 的生物可解释性。
02
Spiking神经网络学习算法
监督学习算法
SpikeProp算法
这是一种基于误差反向传播的监督学习算法,它通过调整突触权重来最小化目 标输出与实际输出之间的差异。SpikeProp利用了脉冲神经元的离散性和稀疏 性,使得网络能够更加高效地进行学习。
噪和增强。通过调整网络的参数和连接权重,可以实现图像的降噪、锐
化和边缘增强等效果。
语音识别与自然语言处理
语音识别
Spiking神经网络可以处理连续时间的语音信号,并将其转换为离散的脉冲序列 进行学习和识别。通过适当的特征提取和网络设计,可以实现准确的语音识别和 语音命令控制。
自然语言处理
Spiking神经网络可以应用于自然语言处理任务,如文本分类、情感分析等。利 用网络的时序编码能力和脉冲传递机制,可以处理文本序列并提取语义信息,实 现有效的自然语言处理。
04
Spiking神经网络的未来发展
算法优化与改进
1 2 3
脉冲发放机制优化
通过深入研究生物神经元的发放机制,进一步优 化Spiking神经网络的脉冲发放算法,提高网络 的计算效率和准确性。
基于spiking神经网络的白细胞图像边缘检测
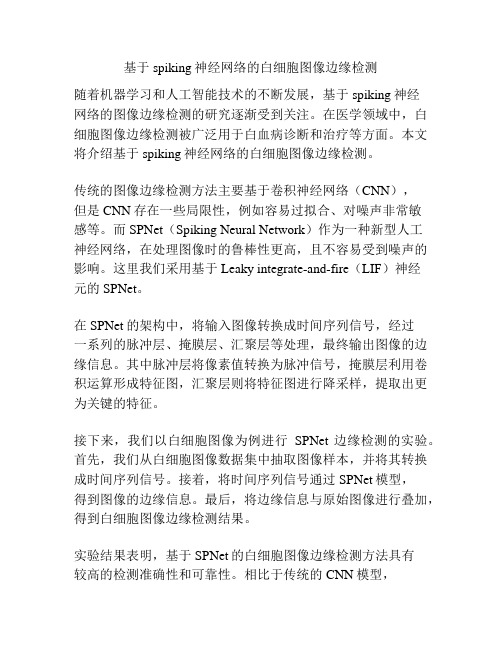
基于spiking神经网络的白细胞图像边缘检测随着机器学习和人工智能技术的不断发展,基于spiking神经网络的图像边缘检测的研究逐渐受到关注。
在医学领域中,白细胞图像边缘检测被广泛用于白血病诊断和治疗等方面。
本文将介绍基于spiking神经网络的白细胞图像边缘检测。
传统的图像边缘检测方法主要基于卷积神经网络(CNN),但是CNN存在一些局限性,例如容易过拟合、对噪声非常敏感等。
而SPNet(Spiking Neural Network)作为一种新型人工神经网络,在处理图像时的鲁棒性更高,且不容易受到噪声的影响。
这里我们采用基于Leaky integrate-and-fire(LIF)神经元的SPNet。
在SPNet的架构中,将输入图像转换成时间序列信号,经过一系列的脉冲层、掩膜层、汇聚层等处理,最终输出图像的边缘信息。
其中脉冲层将像素值转换为脉冲信号,掩膜层利用卷积运算形成特征图,汇聚层则将特征图进行降采样,提取出更为关键的特征。
接下来,我们以白细胞图像为例进行SPNet边缘检测的实验。
首先,我们从白细胞图像数据集中抽取图像样本,并将其转换成时间序列信号。
接着,将时间序列信号通过SPNet模型,得到图像的边缘信息。
最后,将边缘信息与原始图像进行叠加,得到白细胞图像边缘检测结果。
实验结果表明,基于SPNet的白细胞图像边缘检测方法具有较高的检测准确性和可靠性。
相比于传统的CNN模型,SPNet模型在处理噪声和非线性变换方面更为优越,可以有效解决白细胞图像边缘受到复杂噪声干扰的问题。
综上所述,基于spiking神经网络的白细胞图像边缘检测是一种高效、鲁棒的检测方法,可以在医学领域中得到广泛应用。
未来,我们可以进一步研究探索SPNet模型的性能和稳定性,以更好地实现白细胞图像边缘检测的应用。
《低资源神经机器翻译中关键问题的研究》范文
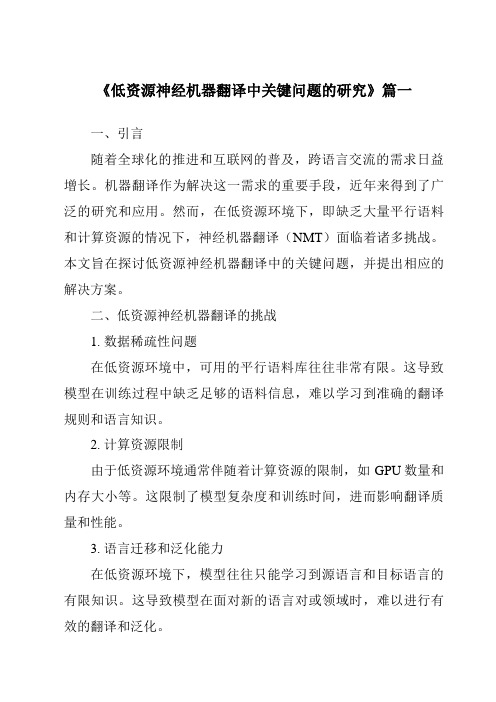
《低资源神经机器翻译中关键问题的研究》篇一一、引言随着全球化的推进和互联网的普及,跨语言交流的需求日益增长。
机器翻译作为解决这一需求的重要手段,近年来得到了广泛的研究和应用。
然而,在低资源环境下,即缺乏大量平行语料和计算资源的情况下,神经机器翻译(NMT)面临着诸多挑战。
本文旨在探讨低资源神经机器翻译中的关键问题,并提出相应的解决方案。
二、低资源神经机器翻译的挑战1. 数据稀疏性问题在低资源环境中,可用的平行语料库往往非常有限。
这导致模型在训练过程中缺乏足够的语料信息,难以学习到准确的翻译规则和语言知识。
2. 计算资源限制由于低资源环境通常伴随着计算资源的限制,如GPU数量和内存大小等。
这限制了模型复杂度和训练时间,进而影响翻译质量和性能。
3. 语言迁移和泛化能力在低资源环境下,模型往往只能学习到源语言和目标语言的有限知识。
这导致模型在面对新的语言对或领域时,难以进行有效的翻译和泛化。
三、关键问题研究1. 数据稀疏性问题的解决策略(1)数据增强技术:通过数据增强技术(如回译、单语语言模型等)扩大训练语料库的规模,提高模型的泛化能力。
(2)迁移学习:利用其他语言对或领域的预训练模型,将知识迁移到低资源语言对上,提高模型的翻译性能。
(3)共享参数和多任务学习:通过共享参数和多任务学习,使模型在学习不同语言对时共享知识,提高数据利用效率。
2. 计算资源限制的应对策略(1)模型压缩与剪枝:通过模型压缩和剪枝技术降低模型的复杂度,减少计算资源和内存需求。
(2)优化算法与并行计算:利用优化算法(如梯度下降优化算法)和并行计算技术(如分布式训练),提高训练速度和性能。
3. 语言迁移和泛化能力的提升方法(1)多源语言模型:引入多源语言信息,使模型能够从多个源语言中学习翻译知识,提高泛化能力。
(2)领域自适应:通过领域自适应技术,使模型能够在特定领域内学习到更准确的翻译规则和知识。
四、实验与分析本文通过实验验证了上述关键问题的解决方案。
spiking机器学习算法
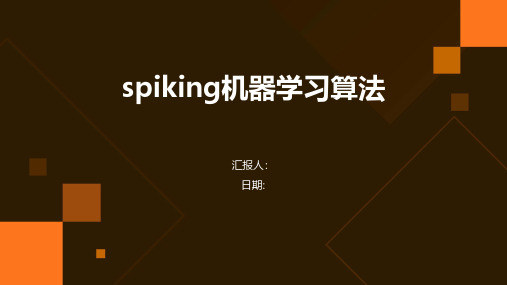
•Spiking神经网络概述•Spiking机器学习算法介绍•Spiking机器学习算法的应用目录•Spiking机器学习算法的挑战与未来发展01生物启发性模型脉冲传递信息Spiking神经网络定义脉冲编码神经元之间通过突触连接,突触的权重决定了脉冲传递的强度。
突触连接学习规则Spiking神经网络的基本原理信息编码方式动态行为生物可解释性能量效率Spiking神经网络与传统神经网络的差异02缺点需要精确的时间标签,计算复杂度较高。
优点能够学习复杂的时空模式,对噪声和输入变异具有一定的鲁棒性。
核心思想通过计算输出脉冲与实际标签之间的误差,调整权重以最小化误差。
类型目标调整权重,使得脉冲神经元的输出与实际标签一致SpikeProp算法目标类型核心思想缺点优点Tempotron算法0102类型目标核心思想优点缺点030405ReSuMe算法03图像识别与处理目标识别01图像分类02图像去噪和增强03语音识别自然语言处理语音识别和自然语言处理运动控制Spiking机器学习算法可以应用于神经机器人的运动控制。
通过模拟生物神经系统的工作机制,构建基于Spiking神经网络的控制器,可以实现机器人的稳定行走、姿态控制等运动任务。
感知与决策利用Spiking机器学习算法,可以构建感知与决策模型,实现机器人对环境的感知和理解。
这样的模型能够使机器人根据环境信息做出决策,如避障、目标追踪等。
神经机器人控制04计算资源消耗由于Spiking神经网络的动态性质,训练过程中需要消耗大量的计算资源,对计算机的运算速度和内存要求较高。
长时间训练Spiking机器学习算法的训练时间通常较长,需要大量的迭代次数来达到满意的精度,这限制了其在大型数据集上的应用。
学习率调整在训练过程中,学习率的设置对训练效率和效果具有重要影响,如何选择合适的学习率是一个待解决的问题。
算法的训练时间和效率问题硬件要求算法优化神经元模型选择硬件实现与算法优化的挑战算法改进硬件创新类脑计算融合多模态学习未来发展方向和可能的改进方案THANKS。
- 1、下载文档前请自行甄别文档内容的完整性,平台不提供额外的编辑、内容补充、找答案等附加服务。
- 2、"仅部分预览"的文档,不可在线预览部分如存在完整性等问题,可反馈申请退款(可完整预览的文档不适用该条件!)。
- 3、如文档侵犯您的权益,请联系客服反馈,我们会尽快为您处理(人工客服工作时间:9:00-18:30)。
Abstract. We investigate through theoretical analysis and computer
1 Introduction
It has been demonstrated in 10 that biological neural systems involving 10 or more synaptic stages are able to carry out complex computations within 100 to 150 ms. Since the ring rates in these neural systems are typically well below 100 Hz and interspike intervals are highly variable 7 this cannot be be explained by models based on the encoding of analog variables through ring rates of spiking neurons. A possible explanation is a model where analog values are encoded in small temporal di erences between the ring times of presynaptic neurons 10, 6 . However, these models do not provide satisfactory explanations for fast analog computation in neural systems where synaptic transmission is unreliable, as appears to be the case in cortical systems of most vertebrates, with failure probabilities ranging up to 0.9, see 2 . A more common type of coding encountered in vertebrate cortex is a population coding where information is encoded by fractions of neurons in various pools that re within some short time interval say, of length between 5 and 10 ms 3 . Although there exists substantial empirical evidence that many cortical systems encode relevant an it has remained unclear how networks of spiking neurons can compute in terms of such a code: If all neurons in a pool V have the same ring threshold and there are reliable synaptic connections from all neurons in pool U to all neurons in V with approximately equal weights, then all neurons v 2 V receive about the same input from U .
Hence, a ring of a fraction x of neurons in U will typically trigger almost none or almost all neurons in V to re. Several mechanisms have already been suggested to achieve a smooth graded response in terms of a space-rate code in V instead of a binary all or none" ring, e.g. strongly varying ring thresholds or a di erent number of synaptic connections from U for di erent neurons v 2 V 11 . Both option are not completely satisfactory, since ring thresholds of biological neurons appear to be rather homogeneous and a regulation of the response in V through the connectivity pattern seems to make it very di cult to implement changes due to some learning process. Furthermore, both options fail to spread activity over all neurons in V homogeneously, and hence would make the computation less robust against failures of individual neurons. In section 2 we investigate the question which functions hx1 ; : : : ; xn i ! y can be computed by a network of spiking neurons if space-rate code is used to encode the analog variables xi 2 0; 1 and y 2 0; 1 .1 The main result is that the output y encoded inP space-rate code in pool V of our model approximates a a sigmoidal function i wi xi for a proper sigmoidal function and proper weights wi 2 R. In section 3 we investigate what computations on times series can be performed by our model. We show there that our model can be described as a linear lter with in nite impulse response IIR.
Research for this article was partially supported by the ESPRIT Working Group NeuroCOLT, No. 8556, and the Fonds zur Forderung der wissenschaftlichen Forschung FWF, Austria, project P12153. We also would like to thank Peter Auer for helpful discussions.
ESANN'1999 proceedings - European Symposium on Artificial Neural Networks Bruges (Belgium), 21-23 April 1999, D-Facto public., ISBN 2-600049-9-X, pp. 417-422
Fast Analog Computation in Networks of Spiking Neurons Using Unreliable Synapses
Thomas Natschlager and Wolfgang Maass
Inst. for Theoretical Comp. Science, Technische Universitat Graz Klosterwiesgasse 32 2, A-8010 Graz, Austria email: tnatschl, maass@igi.tu-graz.ac.at simulations the consequences of unreliable synapses for fast analog computations in networks of spiking neurons, with analog variables encoded by the ring activities of pools of spiking neurons. Our results suggest that the known unreliability of synaptic transmission may be viewed as a useful tool for analog computing, rather than as a bug" in neuronal hardware. We also investigate computations on analog time series encoded by the ring activities of pools of spiking neurons.
2 A Model for Fast Analog Computation
We assume that n pools U1 ; : : : ; Un consisting of N neurons each are given, and that all neurons in these pools have synaptic connections to all neurons in another pool V of N neurons.2 The pools Ui encode the analog input variables xi 2 0; 1 in a space rate code whereas pool V encodes the output y of the network in a space-rate code, i.e. during a short time interval say of length 5 ms a total of Nxi Ny neurons re in pool Ui V where each neuron res only once 3 . In accordance with recent results from neurophysiology we assume that a spike from a neuron u 2 Ui triggers with a certain probability rvu release probability" the release of some vesicles lled with neurotransmitter at one or several release sites of a connection between neurons u 2 Ui and v 2 V . Data from 9, 2 strongly suggest that in the case of a release the amplitude of the resulting EPSP in neuron v is a stochastic quantity. Consequently, we model the amplitude of the EPSP or IPSP in the case of a release by a random variable r.v. avu with probability density function vu . Our model also allows multiple release sites per synapse, as reported for example in 9 . Figure 1B shows an example of vu for a synapse with 5 release sites. Figure 1A illustrates the basic input output behavior of our model. Shown are results of computer simulations of our model for n = 6 presynaptic pools P Ui and a poolsize of N = 200 black dots and a plot of i xi wi for a proper sigmoidal function solid line.3 We call wi the e ective weight"