ZJ_SaliencyBasedAttention
【浙江省自然科学基金】_人脸识别_期刊发文热词逐年推荐_20140811

推荐指数 3 1 1 1 1 1 1 1 1 1 1 1 1 1 1
2011年 序号 1 2 3 4 5 6 7 8 9 10
2011年 科研热词 面诊 面色识别 辨证推演 表情识别 白化散度差矩阵 独立元分析 特征定位 最近邻准则 散度差矩阵 人脸检测 推荐指数 1 1 1 1 1 1 1 1 1 1
推荐指数 5 2 1 1 1 1 1 1 1 1 1 1 1 1 1 1 1 1 1 1 1 1
2009年 序号 1 2 3 4 5 6 7 8 9 10 11 12 13 14 15 16 17 18 19 20 21 22 23 24 25 26 27 28 29 30
科研热词 人脸识别 特征融合 鉴别共同矢量 重构 近邻保持嵌入 身份识别 识别 表情变化 线性近邻传递算法 神经网络 灰度特征 流形学习 核方法 最小平方误差方法 掌纹识别 局部二元模式 小波子图 小波增强 小波基选择 小波变换 小样本问题 双目视觉 判别融合 分解层数 光照归一化 光照变化 二值边缘特征 主元分析 两类核fisher鉴别分析 三维人脸
推荐指数 6 2 1 1 1 1 1 1 1 1 1 1 1 1 1 1 1 1 1 1 1 1 1 1 1 1 1 1 1 1
2010年 序号 1 2 3 4 5 6 7 8 9 10 11 12 13 14 15
科研热词 人脸识别 重构 身份识别 表情分析 细分法 曲波变换 掌纹识别 小波增强 基于稀疏表征的分类 双目视觉 单样本 人脸检测 人机交互 主成分分析 三维人脸
2008年 序号 1 2 3 4 5 6 7 8 9 10 11 12 13 பைடு நூலகம்4 15 16 17 18 19 20 21 22
科研热词 人脸识别 奇异值分解 随机游动 统计形状分析 统一特征空间 约柬线性描述分析 粒子群算法 白化变换 特征提取 正交子空间投影 模块2dpca 核直接fisher描述分析 最优鉴别矢量集 小波变换 实时人脸特征提取 各向异性扩散过程 单训练样本 单样本人脸识别 动态粒子群算法 判别公共向量 光流场 信息熵
高考英语《语法填空+阅读理解+应用文写作》真题含答案
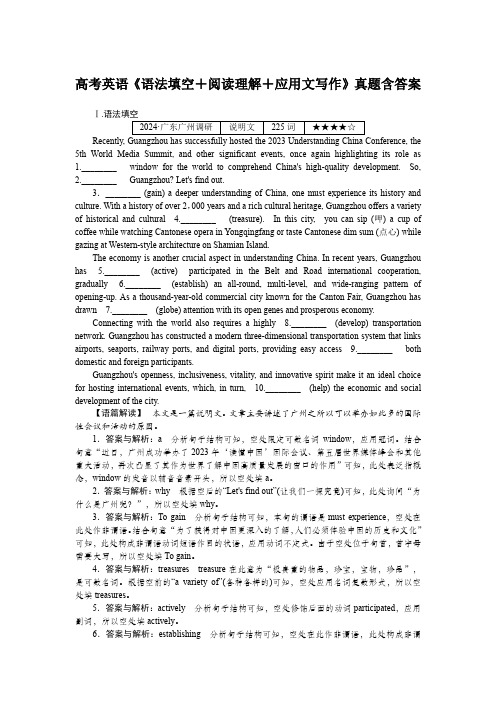
高考英语《语法填空+阅读理解+应用文写作》真题含答案Ⅰ.语法填空5th World Media Summit, and other significant events, once again highlighting its role as1.________ window for the world to comprehend China's highquality development. So,2.________ Guangzhou? Let's find out.3.________ (gain) a deeper understanding of China, one must experience its history and culture. With a history of over 2,000 years and a rich cultural heritage, Guangzhou offers a variety of historical and cultural 4.________ (treasure). In this city, you can sip (呷) a cup of coffee while watching Cantonese opera in Yongqingfang or taste Cantonese dim sum (点心) while gazing at Westernstyle architecture on Shamian Island.The economy is another crucial aspect in understanding China. In recent years, Guangzhou has 5.________ (active) participated in the Belt and Road international cooperation, gradually 6.________ (establish) an allround, multilevel, and wideranging pattern of openingup. As a thousandyearold commercial city known for the Canton Fair, Guangzhou has drawn 7.________ (globe) attention with its open genes and prosperous economy.Connecting with the world also requires a highly 8.________ (develop) transportation network. Guangzhou has constructed a modern threedimensional transportation system that links airports, seaports, railway ports, and digital ports, providing easy access 9.________ both domestic and foreign participants.Guangzhou's openness, inclusiveness, vitality, and innovative spirit make it an ideal choice for hosting international events, which, in turn, 10.________ (help) the economic and social development of the city.【语篇解读】本文是一篇说明文。
基于选择性神经网络集成的船舶避碰问题研究

pe 是粒子 i bt sd 在第 d维 的个体极值 的位 置;b t ge: s
收稿 日期 :0 1 1—1 2 1 —1 7
是群体在第 d 维的全局极值的位置 ; ∞为惯性权重; C 和 c 被称为学习因子 ; n ( 为介于( , ) rd) a 0 1 之间 的随机数 , 这几个参数决定 了 P O的开发能力和探 S
第2 9卷
第 2期
江苏船舶
JANGS HI I US P
Vo . 9 No 2 12 . Ap . r 201 2
21 0 2年 0 4月
基 于选 择 性 神 经 网络 集成 的船 舶 避 碰 问题 研究 王 鹏 , 孟 非
( 江苏科技大学 电子信息学院 , 苏 镇江 2 20 ) 江 10 3
测 能力 。
基金项 目: 江苏科技大学本科生创新项 目, :00 2 0 编号 1 32 04 作者简介 : 王鹏( 9 8 , 江苏科技 大学电子信息学 院测 控技术 18 一) 男, 与仪器专业学生 ; 孟非( 9 7一) 女 , 17 , 讲师 , 研究 方 向为智能技 术在
船舶工程 中的应用。
位置 :
・
要航 海 国家 十分关 注 的问题 。许 多 学者 近年来 研究 了优 化算 法 在 船 舶 避 碰 上 的应 用 , 文献 [ ] 1 和文 献 [ ] 别将 遗传算 法应 用 到 船舶 避 碰决 策 中的危 险 2分 度确 定 和避让 幅 度 的计 算 , 考 虑 了方 位 、 离 、 并 距 船
想 来 源于人 工生 命 和进 化 计 算 理论 , 早 是受 鸟群 最 觅食行 为 的启 发 提 出的 。在 PO算 法 中 , 化 问题 S 优 的所有 可 能解被 视 为是 搜 索 空 间 中 的一 个 位 置 , 称 之为 “ 子 ” 粒 。所 有 粒 子 都 有 一 个 由被 优 化 的 函数 决定 的适应 值 , 了现 在 的 位 置外 还 记 录 了到 目前 除 为止 该粒 子 曾达 到 过 最 好 适 应 值 的 位 置 (bs) p et 和 群 体 中 所 有 粒 子 曾 达 到 过 最 好 适 应 值 的 位 置 (bs 。对 每个 粒 子 还 需 确 定 一 个 速 度 矢 量 决 定 get ) 它们 飞翔 的方 向和位 移 。P O初 始化 为一 群 随机 粒 S 子 , 随机 的初 始解 , 后粒 子们 参照 最优 粒子 的位 即 然 置在 解 空间 中搜 索 , 通 过 迭代 找 到 最 优 解 。每 次 并 迭代 时 , 粒子 根据 如 下 的公 式 来 更 新 自己 的速 度 和
Attention-based Learning
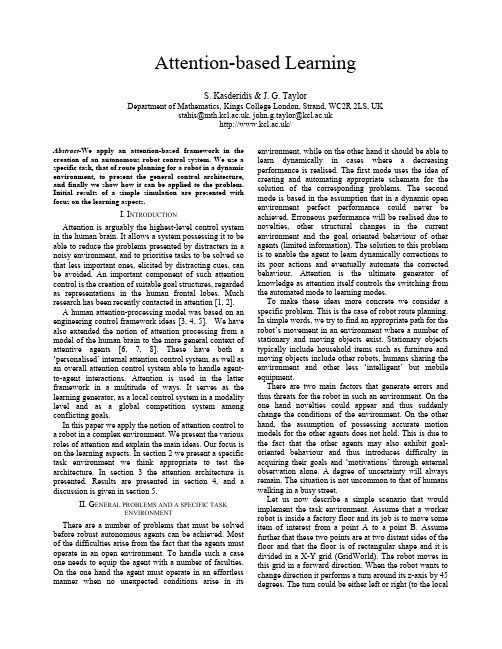
Attention-based LearningS. Kasderidis & J. G. TaylorDepartment of Mathematics, Kings College London, Strand, WC2R 2LS, UK stahis@, john.g.taylor@/Abstract-We apply an attention-based framework in the creation of an autonomous robot control system. We use a specific task, that of route planning for a robot in a dynamic environment, to present the general control architecture, and finally we show how it can be applied to the problem. Initial results of a simple simulation are presented with focus on the learning aspects.I. I NTRODUCTIONAttention is arguably the highest-level control system in the human brain. It allows a system possessing it to be able to reduce the problems presented by distracters in a noisy environment, and to prioritise tasks to be solved so that less important ones, elicited by distracting cues, can be avoided. An important component of such attention control is the creation of suitable goal structures, regarded as representations in the human frontal lobes. Much research has been recently contacted in attention [1, 2].A human attention-processing model was based on an engineering control framework ideas [3, 4, 5]. We have also extended the notion of attention processing from a model of the human brain to the more general context of attentive agents [6, 7, 8]. These have both a ‘personalised’ internal attention control system, as well as an overall attention control system able to handle agent-to-agent interactions. Attention is used in the latter framework in a multitude of ways. It serves as the learning generator, as a local control system in a modality level and as a global competition system among conflicting goals.In this paper we apply the notion of attention control to a robot in a complex environment. We present the various roles of attention and explain the main ideas. Our focus is on the learning aspects. In section 2 we present a specific task environment we think appropriate to test the architecture. In section 3 the attention architecture is presented. Results are presented in section 4, and a discussion is given in section 5.II. G ENERAL PROBLEMS AND A SPECIFIC TASKENVIRONMENTThere are a number of problems that must be solved before robust autonomous agents can be achieved. Most of the difficulties arise from the fact that the agents must operate in an open environment. To handle such a case one needs to equip the agent with a number of faculties. On the one hand the agent must operate in an effortless manner when no unexpected conditions arise in its environment, while on the other hand it should be able to learn dynamically in cases where a decreasing performance is realised. The first mode uses the idea of creating and automating appropriate schemata for the solution of the corresponding problems. The second mode is based in the assumption that in a dynamic open environment perfect performance could never be achieved. Erroneous performance will be realised due to novelties, other structural changes in the current environment and the goal oriented behaviour of other agents (limited information). The solution to this problem is to enable the agent to learn dynamically corrections to its poor actions and eventually automate the corrected behaviour. Attention is the ultimate generator of knowledge as attention itself controls the switching from the automated mode to learning modes.To make these ideas more concrete we consider a specific problem. This is the case of robot route planning. In simple words, we try to find an appropriate path for the robot’s movement in an environment where a number of stationary and moving objects exist. Stationary objects typically include household items such as furniture and moving objects include other robots, humans sharing the environment and other less ‘intelligent’ but mobile equipment.There are two main factors that generate errors and thus threats for the robot in such an environment. On the one hand novelties could appear and thus suddenly change the conditions of the environment. On the other hand, the assumption of possessing accurate motion models for the other agents does not hold. This is due to the fact that the other agents may also exhibit goal-oriented behaviour and thus introduces difficulty in acquiring their goals and ‘motivations’ through external observation alone. A degree of uncertainty will always remain. The situation is not uncommon to that of humans walking in a busy street.Let us now describe a simple scenario that would implement the task environment. Assume that a worker robot is inside a factory floor and its job is to move some item of interest from a point A to a point B. Assume further that these two points are at two distant sides of the floor and that the floor is of rectangular shape and it is divided in a X-Y grid (GridWorld). The robot moves in this grid in a forward direction. When the robot wants to change direction it performs a turn around its z-axis by 45 degrees. The turn could be either left or right (to the localsystem of coordinates). The robot carries a number of sensors. They are: a power sensor for detecting the power level, an overall proximity sensor (with a 360 degrees view of the environment), and one position/speed sensor for knowing its own coordinates and speed. In addition an actuator exists which controls the speed and the direction of the robot. The GridWorld is shown in figure 1.Fig. 1. Factory floor layout.Terminal C is the recharging station for the robot. Sobj x is the name of a stationary object. Mobj x is the name of a moving object. The figure shows a possible configuration of the GridWorld with three stationary objects and two moving ones. Realisations for the trajectories of the moving objects are also shown with dashed lines.The problem that we face is how to move from point A to point B and possibly C avoiding collisions both with static and moving objects.III. A RCHITECTUREThe architecture we use is based on that extracted from the human brain, in terms of a goal system with an attention control mechanism (the details appear in [7, 8]). An agent possesses a number of goals represented as a tree structure (with higher importance goals positioned nearer the root, less important ones further apart). Each goal is associated with a service procedure (a schema that satisfies the goal, given as a template with adaptive elements). The general schema template with local attention control is shown in figure 2 (more detail in [8]).The architecture has four processing levels: level one has sensors and actuators, level two has pre-processing facilities, level three has schemata (services) for atomic goals, and level four has schemata for composite goals. Figure 2 represents the service of an atomic goal, which tries to achieve a target state. A composite goal has a different structure than the one shown in figure 2, due to the fact that its service function manages and produces an overall result from a number of children goals. We will not extend our discussion at this level further, but focus on level 3 atomic goals.In figure 2 seven main modules appear: the State, Observer, Goals, Monitor, Attention Controller, Rules and Forward Models modules. The State module collects sensor signals and constructs representations, using three distinct representations, of which only one is used here, the Native one, which is the Cartesian product of the incoming signals. The Observer module is a state predictor for estimating the next sensory state and building an “expected state”, to be compared, in the Monitor module, with the currently observed state (coming from the State module) to determine if major deviations exist. If a deviation threshold is crossed, an attention event is created and sent to the Attention Controller; it carries an Attention Index, a monotonically increasing function of the observed deviation in the Monitor module, bounded in the interval [0, 1]. The Attention Controller module is conceptually a stack/queue that determines the priority with which the dispatched attention events will be handled. In the simplest case the identity function can be used to determine the priority. The Rules module is the action generator component of the schema, using typically a symbolic model, i.e. rules, to produce appropriate responses, with input the current state classification and any attention event present. The Forward Models module produces predictions of the next state of the agent, given the realization of the action proposed by the Rules module. The Goals module serves as a database where a number of adaptive models necessary in the scope of the schema are necessary. It also includes the required target state that must be achieved.We assume that the robot carries three sensors, and an actuator. In figure 2, the robot uses a number of sensors: the power sensor, the proximity sensor and the position/speed sensor. For simplicity we assume that the proximity sensor provides information for both the current position and speed of the other objects. In addition a single actuator exists which controls the speed and the direction of the robot. Inside the robot only one user defined goal is executed: the Move Item goal. The purpose of this goal is to have the robot move an item from a point A to point B. It has two children goals: the Transport and Collision Avoidance (CA) goals. The transport goal is decomposed in four sub-goals: Goto A, Get Item, Goto B, Leave Item. The interesting goal to discuss here is the Goto x. It is further decomposed into the Route Planning (RP) and Move goals.The Move goal accepts a newly calculated position from the Route Planner and acts on the actuator. The State module collects information about the current position and speeds of the other agents and itself. It evaluates the current trajectories of the other moving objects through the Observer module. The module uses a motion model for each of the other moving objects,realised as a suitable neural network. If the observed positions/speeds of other agents differ enough from the expected ones,Fig. 2. Level 3 schema architecture.then an attention event is created. The attention index is calculated as:(where vectors X obs and X exp represent the observed and expected position of a given moving object respectively). T is a suitable threshold, which measures the proximity of the two locations; Step(•) is the step function; it outputs 1 if the argument is positive, else it is zero. the vector X uses the x and y coordinates of a GridWorld cell. Given the information about the current positions of the various agents, the Rules module suggests a new position closer to the target position, which is then checked against the prediction of the Forward Models so as to make sure that in the next step the other objects and the robot will not collide or that it is not is otherwise occupied. If no problem is detected then the suggested new position is accepted and it is forwarded for execution to the Move goal.There are two sources of problems. On the one hand, mechanical problems may not allow the proper actuator commands to be performed or alternatively the executed commands may not result in bringing the agent in the desired state that the planner system intended. In both cases we have failures of the Move goal. This goal uses another type of attention, which is called motor attention (in contrast with the sensory attention described above in (1)). It also observes and compares the expected and the occurred (internal) states of the agent: it makes sure that the agent is brought to the required state so as to conform to the overall environmental state (which includes the other agents) according to the plan of the RP.During the execution of the planned motor action (i.e. moving to the next cell) we may find that the other agents did not really move according to prediction of the motion model. The Collision Avoidance goal will capture this deviation and create an appropriate attention event, which will result in taking corrective action: simply moving away in the opposite direction to an approaching object. We assume the existence of a safety zone around the agent, with radius R. If the boundary of the safety zone is crossed then an attention event is created with the following attention index:The idea behind (2) is that the event is more severe as we enter further into the safety zone. X obs is the observed position of another agent, whereas X agent is the agent’s own position. The action that is created as a response to an attention event is twofold: First, it leads to the update of the current motion model for the corresponding other agent. Second, it takes averting action so as to avoid a collision. The structure of the Collision Avoidance goal follows the template of figure 2. The Observer is used for ‘following’ in runtime the path of the other objects.It should be made clear at this point that the CA-Observer, RP-Observer and RP-Forward Models modules all use the same underlying motion model for a given observed (other) agent. This is a neural network model that has as inputs the current position, current speed and previous positions (up to some lag time K) of the other agent. The model is updated dynamically as a result of Collision Avoidance’s attention control. This is the role of attention as learning initiator. At this point the second role of attention is highlighted: that of a global competition for conflict resolution. We have explained previously that if a collision is detected, corrective action is taken. This is achieved because the CA goal effectively suppresses the sub-tree starting at the Transport goal, which belongs to the same level as itself. The mechanism with which this result is achieved is based on Action Indices (a generalisation of the Attention Index concept). The details are given in [8]. We just provide the relevant formula here:Where S-AI and M-AI are the sensory and motor attention indices and W is the goal’s intrinsic weight (available through learning of a value system). A child is considering as contributing if any kind of attention event is created in its scope (at the given time) or if it posses itself a contributing child. Formula (3) introduces a mechanism, which can be used for comparing ‘importance’ of execution among conflicting goals. WithS-AI-RP=Step(||X obs-X exp ||-T)*(2/[1+exp(-(||X obs-X exp||-T)]-1) (1)S-AI-CA=exp(-||X obs-X agent ||), if||X obs-X agent || <R,S-AI-CA=0, if ||X obs-X agent || ≥ R(2)Action Index=(W+S-AI+M-AI + ∑jδ(j, contribut.))/ (3+# Contributing Children)(3)appropriate weighting the Collision Avoidance goal can be made to take precedence over the Transport goal. Thus suppression of the Transport sub-tree is achieved and its execution is stopped for the given processing step. The only action that will be generated is this originating from the CA goal. In normal circumstances the Collision Avoidance is suppressed from the Transport goal. Local attention control in figure 2 is achieved by changing sampling rates or otherwise of appropriate sensors. Control is needed in this level in order to minimise the power requirements of enhanced resolution information (in time and space) against the need for higher service times of the robot.Finally we must observe that the attention indices, (1) and (2), can be used as modulators of the learning rates present in the motion models. In particular a suitable formula is given by (4):Where ε is a permanent small rate, say 10-4, AI is a suitable attention index and r is the learning rate used in the neural network motion model. Learning is assumed to take place in the background continually, using in (4) as attention index formula (2).IV. S IMULATION & RESULTSWe use the GridWorld of figure 1 to perform a number of simulations. The size of the grid is 50x50. The stationary objects are located as in the figure. A number of moving objects were used. The motion model was implemented using a neural network approach to the target cell. The network used as inputs the positions from current time up to K steps in the past and the current speed. K was varied from 0 to 5. We assumed also infinite sensing range. The speed of the robot is either set to one or zero. The target of the simulations is to study the effectiveness of the proposed architecture in detecting a danger and learning effective motion models.The simulation was setup as follows: We have used a varying number of moving objects for checking the agent’s effectiveness on avoiding a collision. We used 5, 10, 15, 20 and 25 moving objects. All the other agents were given fixed but unknown trajectories to follow. Randomness in the trajectories was introduced in two ways. On the one hand, the trajectory was formed by selecting random start and end service points for a given moving object. This had the effect of covering the whole floor with intersecting trajectories. On the other hand, small random movements around the main calculated trajectory were introduced at random points. The trajectory was calculated using a linear approach to the target cell and taking corrective steps when approaching a stationary object. When another moving object was approaching, no special action was taken, so collisions were possible. In this way we had a population of simple moving agents, which collided with each other and with our own attention-based agent. The statistic of interest was the number of collisions taking place per step. This was calculated as follows: We assumed a fixed simulation duration of 350 steps; we used 10 different trajectory configurations so as to average the randomness in the experiment’s initial configuration and we studied three cases. A baseline case (dummy agent), a predictive agent and an attention-based predictive agent. All of them were using the same basic route-planning algorithm. The difference was introduced in the way that the three types of agents were using the suggestions of the route planning system. The dummy agent simply accepted the suggestions. The predictive agent was using its motion model for forecasting future collisions. If a collision was predicted, then another call to the route planner was made so as to calculate a new position given the new information. The same approach was used by the attention-based agent. The predictive and attentive agents were also updating their motion models (in the next step) if an unsuccessful prediction was made. For these experiments we used a back-propagation method. The resulting network had as inputs the current x and y coordinates of the GridWorld cell, the current heading vector, expressed as a value out of a fixed set of directions and possibly x and y coordinates of previous positions. We used up to K=5 lag vectors of x/y coordinates in the motion models. Typically, the higher the number of the lag steps the better the prediction accuracy of the model. However, all models suffered, naturally, when discontinuities in the path were realised due to a sudden turn due to random variations or due to route planner’s calculated trajectory at that point.The predictive type of agent had a fixed learning rate of r=0.05, whereas the attentive agent used formula (4) for learning rate modulation.In figure 3 we show a number of series. The labels of Dummy, Smart and Smart-Att correspond to the dummy, predictive and attentive agents respectively. The first sub-graph describes the severity of the collisions while the second one describes the number of collisions suffered. Both figures describe average numbers per step of the trajectory. The results were also averaged over 10 different floor configurations per case of agent, i.e. dummy, predictive and attentive. The calculation of collision was made as follows: We assumed the existence of a safety zone around the agent of interest. The radius of the zone was variable. In figure 3 we used a radius of 6 cells. If during its movement from one cell to another the agent experienced the crossing of its safety zone from another agent, this was counted as a collision, i.e. the route planning system failed to provide a safe enough movement direction. Clearly, the entrance in the safety zone introduces a varying degree of danger, with the highest being an impact by moving on the same grid cell. This could be thought as a hazard function (severity inr t+1=r t*AI+ε(4)the figure). We used a scheme where we assigned 10 ‘danger’ units per cell crossed in the safety zone. For example, assume a radius of 6 cells in every direction and another moving object having entered two cells inside the boundary, i.e. four cells away from our agent, then a value of 20 units was assigned.Fig. 3. Simulation results.The results that are presented in figure 3 show that the predictive agent performed better than the dummy one, and the attentive one better than the predictive one. This observation was confirmed for varying sizes of the safety zone, training time for online model fitting, learning rates (in the case of the predictive agent) and number of training epochs. We used a fixed number (per experiment) of training epochs, typically 150, for updating the motion models. This procedure is equivalent to early stopping so as to avoid over fitting problems. No cross validation or similar approaches were used, as this simulation was intended to study expected “online” performance where not all necessary computational resources are assumed to be available. We used a batch version of the standard gradient descent back-propagation algorithm for this “online” training.V. C ONCLUSIONS & DISCUSSIONThe simulation results seem to indicate the validity of our assumption that attentive control should speed up the learning of corrections in the motion models. This coupled with the fact that the attentive agent has also used a pure reflexive response when attention levels were high, thus discarding the normal route planning process, indicate that the attention-based framework is a natural way to integrate online learning with normal (i.e. automated) processing when no events are present.Clearly one can argue that a more sophisticated learning algorithm for the motion model, possibly with modulation of the learning rate parameter, can be applied so as to further increase the performance of the predictive agent. The point that is made here is that the goal-oriented attention-control framework is a natural way to integrate processing and learning aspects in run-time. The attentive type of agent is already a superset of the predictive agent and thus any performance increase in the latter will also benefit the former. In our framework, we do not make any explicit assumptions regarding the nature of the underlying prediction (motion) models. They can be implemented by using many approaches. The Kalman filter approach is a useful tool in many applications, but it is not applicable in systems that do not assume a linear observation function or linear evolution of the underlying dynamical system. In our example, one can express the equations of motion in a Kalman filter formulation, but what remains unclear is the fact if this is a suitable framework in order to model the goals and “intentions” of the other agents, which eventually lead to the lack of information so as to establish a mean path. In other words, it is not immediately clear if the underlying generating mechanism of goals / sub-goal structures follows a linear generation scheme / evolution.Inclusion of reinforcement type of learning is also possible. This would allow us to further increase the usefulness of the attentive agent by using multiple learning paradigms in an integrated way. These aspects and verification of the current results by using additional configurations and different experimental setups are the next steps to be followed. Variations of the modulating scheme of formula (4) will also be considered.There is much further work to be done to extend the system beyond the level of simulation described here. We have already mentioned the manner in which training should be extended, as well as consideration needed of the Goals module. The further extensions, already under consideration in the ORESTEIA attention agent project [6, 7, 8], are to develop more general control with greater flexibility still. This could be by using a multi-modal system, so that sensor information in a given modality can be properly assessed at a low level (level 3 in our above discussion) but then combined with other modalities at level 4. This requires careful discussion and development of fused state representatives as well as thehigher-level goal, forward and observed models as well as developing overall rules for the system. Even greater flexibility can be inserted by enabling the system to create its own goals and response rules, most efficiently by reward learning (the rewards arising form the environment), as considered earlier. We hope to develop, and report on, these aspects elsewhere.Closing this discussion we note the fact that it is possible to use this framework in order to decompose a task in a set of competing goal families; our global attention mode. Local attention control is present in the form of sensor and actuator control. What is not currently present is the implementation of a third mode of attention, which is related with the signal enhancement process, i.e. amplifying suitable features and discarding distracting ones. This can be considered essentially as a feature selection process and it will be integrated in the existing framework in a future stage.VI. A CKNOWLEDGMENTSThe work presented here was originated and developed during the EU IST ORESTEIA project (IST-2000-26091). We kindly acknowledge their support to our work.R EFERENCES[1] Miall R. and Walpert D. M. (1996). Forward models forphysiological motor control. Neural Networks 9, 1265-1279.[2] Rushworth M. F. S. et al (1997). The left parietal cortex and motorattention. Neuropsychologia 33, 1261-1273.[3] Taylor J. G. (2000). Attentional movement: the control basis forConsciousness. Soc. Neuroscience Abstracts 26, 2231, #893.3[4] Taylor J. G. (2001). Attention as a neural control system. Proc. Int.Joint Conf. Neural Networks, pp 262-276, IJCNN ’01.[5] Taylor J. G. (2003). Paying attention to Consciousness. Progress inNeurobiology 71:305-335[6] Kasderidis S. & Taylor J.G. (2003). Drawing attention to thedangerous. ICANN 2003 Conference, Istanbul, 27-29 June 2003, Springer.[7] Kasderidis S. & Taylor J.G. (2003). Attention-based Robot Control.KES 2003 Conference, Oxford, 10-12 September 2003, Springer. [8] Kasderidis S. & Taylor J.G. (2003). Attentional Agents and RobotControl. KES Journal, IOS Press, 2003. (submitted).。
基于自转一阶非连续式微球双平盘研磨的运动学分析与实验研究
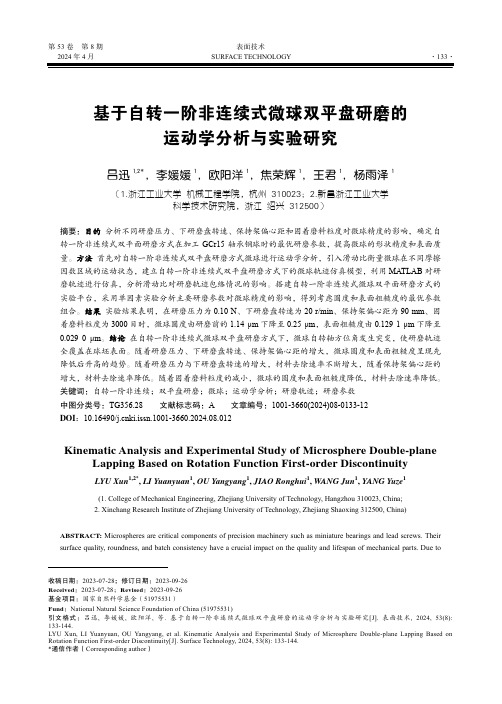
第53卷第8期表面技术2024年4月SURFACE TECHNOLOGY·133·基于自转一阶非连续式微球双平盘研磨的运动学分析与实验研究吕迅1,2*,李媛媛1,欧阳洋1,焦荣辉1,王君1,杨雨泽1(1.浙江工业大学 机械工程学院,杭州 310023;2.新昌浙江工业大学科学技术研究院,浙江 绍兴 312500)摘要:目的分析不同研磨压力、下研磨盘转速、保持架偏心距和固着磨料粒度对微球精度的影响,确定自转一阶非连续式双平面研磨方式在加工GCr15轴承钢球时的最优研磨参数,提高微球的形状精度和表面质量。
方法首先对自转一阶非连续式双平盘研磨方式微球进行运动学分析,引入滑动比衡量微球在不同摩擦因数区域的运动状态,建立自转一阶非连续式双平盘研磨方式下的微球轨迹仿真模型,利用MATLAB对研磨轨迹进行仿真,分析滑动比对研磨轨迹包络情况的影响。
搭建自转一阶非连续式微球双平面研磨方式的实验平台,采用单因素实验分析主要研磨参数对微球精度的影响,得到考虑圆度和表面粗糙度的最优参数组合。
结果实验结果表明,在研磨压力为0.10 N、下研磨盘转速为20 r/min、保持架偏心距为90 mm、固着磨料粒度为3000目时,微球圆度由研磨前的1.14 μm下降至0.25 μm,表面粗糙度由0.129 1 μm下降至0.029 0 μm。
结论在自转一阶非连续式微球双平盘研磨方式下,微球自转轴方位角发生突变,使研磨轨迹全覆盖在球坯表面。
随着研磨压力、下研磨盘转速、保持架偏心距的增大,微球圆度和表面粗糙度呈现先降低后升高的趋势。
随着研磨压力与下研磨盘转速的增大,材料去除速率不断增大,随着保持架偏心距的增大,材料去除速率降低。
随着固着磨料粒度的减小,微球的圆度和表面粗糙度降低,材料去除速率降低。
关键词:自转一阶非连续;双平盘研磨;微球;运动学分析;研磨轨迹;研磨参数中图分类号:TG356.28 文献标志码:A 文章编号:1001-3660(2024)08-0133-12DOI:10.16490/ki.issn.1001-3660.2024.08.012Kinematic Analysis and Experimental Study of Microsphere Double-plane Lapping Based on Rotation Function First-order DiscontinuityLYU Xun1,2*, LI Yuanyuan1, OU Yangyang1, JIAO Ronghui1, WANG Jun1, YANG Yuze1(1. College of Mechanical Engineering, Zhejiang University of Technology, Hangzhou 310023, China;2. Xinchang Research Institute of Zhejiang University of Technology, Zhejiang Shaoxing 312500, China)ABSTRACT: Microspheres are critical components of precision machinery such as miniature bearings and lead screws. Their surface quality, roundness, and batch consistency have a crucial impact on the quality and lifespan of mechanical parts. Due to收稿日期:2023-07-28;修订日期:2023-09-26Received:2023-07-28;Revised:2023-09-26基金项目:国家自然科学基金(51975531)Fund:National Natural Science Foundation of China (51975531)引文格式:吕迅, 李媛媛, 欧阳洋, 等. 基于自转一阶非连续式微球双平盘研磨的运动学分析与实验研究[J]. 表面技术, 2024, 53(8): 133-144.LYU Xun, LI Yuanyuan, OU Yangyang, et al. Kinematic Analysis and Experimental Study of Microsphere Double-plane Lapping Based on Rotation Function First-order Discontinuity[J]. Surface Technology, 2024, 53(8): 133-144.*通信作者(Corresponding author)·134·表面技术 2024年4月their small size and light weight, existing ball processing methods are used to achieve high-precision machining of microspheres. Traditional concentric spherical lapping methods, with three sets of circular ring trajectories, result in poor lapping accuracy. To achieve efficient and high-precision processing of microspheres, the work aims to propose a method based on the first-order discontinuity of rotation for double-plane lapping of microspheres. Firstly, the principle of the first-order discontinuity of rotation for double-plane lapping of microspheres was analyzed, and it was found that the movement of the microsphere changed when it was in different regions of the upper variable friction plate, resulting in a sudden change in the microsphere's rotational axis azimuth and expanding the lapping trajectory. Next, the movement of the microsphere in the first-order discontinuity of rotation for double-plane lapping method was analyzed, and the sliding ratio was introduced to measure the motion state of the microsphere in different friction coefficient regions. It was observed that the sliding ratio of the microsphere varied in different friction coefficient regions. As a result, when the microsphere passed through the transition area between the large and small friction regions of the upper variable friction plate, the sliding ratio changed, causing a sudden change in the microsphere's rotational axis azimuth and expanding the lapping trajectory. The lapping trajectory under different sliding ratios was simulated by MATLAB, and the results showed that with the increase in simulation time, the first-order discontinuity of rotation for double-plane lapping method could achieve full coverage of the microsphere's lapping trajectory, making it more suitable for precision machining of microspheres. Finally, based on the above research, an experimental platform for the first-order discontinuity of rotation for double-plane lapping of microsphere was constructed. With 1 mm diameter bearing steel balls as the processing object, single-factor experiments were conducted to study the effects of lapping pressure, lower plate speed, eccentricity of the holding frame, and grit size of fixed abrasives on microsphere roundness, surface roughness, and material removal rate. The experimental results showed that under the first-order discontinuity of rotation for double-plane lapping, the microsphere's rotational axis azimuth underwent a sudden change, leading to full coverage of the lapping trajectory on the microsphere's surface. Under the lapping pressure of 0.10 N, the lower plate speed of 20 r/min, the eccentricity of the holder of 90 mm, and the grit size of fixed abrasives of 3000 meshes, the roundness of the microsphere decreased from 1.14 μm before lapping to 0.25 μm, and the surface roughness decreased from 0.129 1 μm to 0.029 0 μm. As the lapping pressure and lower plate speed increased, the microsphere roundness and surface roughness were firstly improved and then deteriorated, while the material removal rate continuously increased. As the eccentricity of the holding frame increased, the roundness was firstly improved and then deteriorated, while the material removal rate decreased. As the grit size of fixed abrasives decreased, the microsphere's roundness and surface roughness were improved, and the material removal rate decreased. Through the experiments, the optimal parameter combination considering roundness and surface roughness is obtained: lapping pressure of 0.10 N/ball, lower plate speed of 20 r/min, eccentricity of the holder of 90 mm, and grit size of fixed abrasives of 3000 meshes.KEY WORDS: rotation function first-order discontinuity; double-plane lapping; microsphere; kinematic analysis; lapping trajectory; lapping parameters随着机械产品朝着轻量化、微型化的方向发展,微型电机、仪器仪表等多种工业产品对微型轴承的需求大量增加。
2024年下半年教师资格考试初中英语学科知识与教学能力自测试卷与参考答案
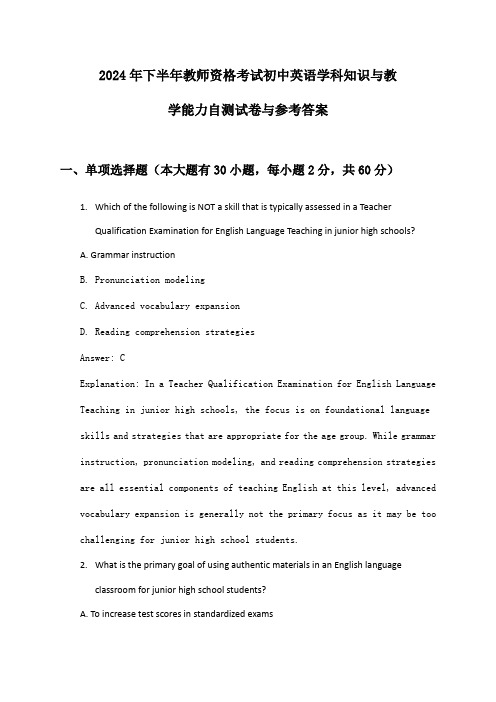
2024年下半年教师资格考试初中英语学科知识与教学能力自测试卷与参考答案一、单项选择题(本大题有30小题,每小题2分,共60分)1.Which of the following is NOT a skill that is typically assessed in a TeacherQualification Examination for English Language Teaching in junior high schools?A. Grammar instructionB. Pronunciation modelingC. Advanced vocabulary expansionD. Reading comprehension strategiesAnswer: CExplanation: In a Teacher Qualification Examination for English Language Teaching in junior high schools, the focus is on foundational language skills and strategies that are appropriate for the age group. While grammar instruction, pronunciation modeling, and reading comprehension strategies are all essential components of teaching English at this level, advanced vocabulary expansion is generally not the primary focus as it may be too challenging for junior high school students.2.What is the primary goal of using authentic materials in an English languageclassroom for junior high school students?A. To increase test scores in standardized examsB. To expose students to real-life language use and cultural contextsC. To improve students’ ability to memorize grammar rulesD. To reduce the need for explicit grammar instructionAnswer: BExplanation: Using authentic materials, such as newspaper articles, songs, videos, and podcasts, in an English language classroom exposes students to real-life language use and cultural contexts. This helps students understand how English is used in different situations and by diverse speakers, enhancing their language proficiency and cultural awareness. While these materials may indirectly contribute to test scores and grammar understanding, their primary goal is to provide authentic language experiences.3.Which teaching method emphasizes student-centered learning and encouragesstudents to actively participate in the learning process through group discussions, debates, and projects?A. Grammar-Translation MethodB. Audio-Lingual MethodC. Communicative Language Teaching (CLT)D. Direct MethodAnswer: CExplanation: Communicative Language Teaching (CLT) is a teaching method that emphasizes student-centered learning and encourages students to actively participate in the learning process. In CLT classrooms, studentsare encouraged to communicate in English for meaningful purposes, using language to express ideas, opinions, and feelings. This approach often involves group discussions, debates, and projects that require students to use English in a variety of contexts and situations. The Grammar-Translation Method, Audio-Lingual Method, and Direct Method, on the other hand, have different focuses and do not necessarily prioritize student-centered learning and active participation to the same extent as CLT.4、Which of the following is NOT a strategy for effective vocabulary teaching in junior high school English?A)Using context clues to infer meaningB)Memorizing word lists without contextC)Encouraging students to use new words in sentencesD)Integrating vocabulary into daily conversationsAnswer: BExplanation: Effective vocabulary teaching in junior high school English emphasizes using context to understand and remember words, as well as encouraging students to actively use them. Memorizing word lists without context is generally considered less effective, as it does not provide the necessary context for students to truly grasp the meaning and usage of the words.5、In the area of English grammar teaching, which of the following principles is most important for fostering students’ understanding and ac curate use of grammar structures?A)Repetition and drillingB)Meaningful contextC)Grammar-translation methodD)Memorization of grammar rulesAnswer: BExplanation: In English grammar teaching, providing meaningful context is crucial for students to understand and accurately use grammar structures. This approach allows students to see how grammar is used in real-life situations, rather than just memorizing rules in isolation. Repetition and drilling can be helpful, but should be done within a meaningful context. Grammar-translation method and memorization of grammar rules are less effective in fostering true understanding and usage.6、Which teaching method best promotes student engagement and active learning in a junior high school English class, particularly when teaching reading comprehension?A)Direct instruction with teacher-led explanationsB)Cooperative learning groups to discuss and analyze textsC)Independent silent reading with comprehension questionsD)Whole-class discussion without prior reading or preparationAnswer: BExplanation: Cooperative learning groups promote student engagement and active learning by encouraging students to work together to discuss and analyze texts. This approach allows students to share their thoughts, askquestions, and build on each ot her’s ideas, leading to deeper understanding of the material. Direct instruction with teacher-led explanations can be helpful, but may not always promote active learning. Independent silent reading with comprehension questions can be effective, but may not foster the same level of engagement and discussion. Whole-class discussion without prior reading or preparation may lead to confusion and lack of focus. 7、Which of the following teaching strategies is most effective in enhancing students’ listening compre hension in junior high school English classes?A) Focusing solely on grammar explanationsB) Providing a combination of authentic listening materials and targeted exercisesC) Relying solely on textbooks for listening practiceD) Using only teacher-made recordings and discussionsAnswer: BExplanation: To improve students’ listening comprehension in junior high school English, a combination of authentic listening materials that reflect real-life situations and targeted exercises that focus on specific listening skills is most effective. This approach exposes students to a wide range of accents and language styles, while also providing opportunities to practice and apply listening strategies. Solely focusing on grammar explanations (A), relying solely on textbooks (C), or using only teacher-made recordings and discussions (D) may limit the variety andauthenticity of listening materials, thereby reducing the effectiveness of the teaching strategy.8、What is the primary goal of the reading comprehension section in junior high school English exams?A) To assess students’ memorization of vocabulary wordsB) To evaluate students’ ability to analyze and interpret textsC) To test students’ knowledge of grammatical structuresD) To measure students’ speed of readingAnswer: BExplanation: The primary goal of the reading comprehension section in junior high school English exams is to evaluate students’ ability to analyze and interpret texts. This includes understanding the main idea, identifying details, inferring meaning, and making connections between the text and real-life situations. While memorization of vocabulary words (A), knowledge of grammatical structures (C), and reading speed (D) are all important aspects of reading comprehension, they are not the primary focus of this section of the exam.9、Which teaching method is particularly effective in helping junior high school students improve their speaking skills in English?A) Role-play activitiesB) Extensive grammar lecturesC) Silent reading practiceD) Extensive vocabulary memorizationAnswer: AExplanation: Role-play activities (A) are particularly effective in helping junior high school students improve their speaking skills in English. They provide students with opportunities to practice using language in real-life situations, engage in meaningful conversations, and develop their communication skills. Extensive grammar lectures (B), silent reading practice (C), and extensive vocabulary memorization (D) are all valuable teaching methods, but they do not directly address the development of speaking skills in the same way as role-play activities.10、Which of the following is NOT a characteristic of effective teaching in junior high school English?A) Engaging students in interactive activitiesB) Focusing solely on grammar rules and vocabulary memorizationC) Encouraging critical thinking and creativityD) Providing opportunities for authentic language useAnswer: BExplanation: Effective teaching in junior high school English involves engaging students in various learning activities that promote language acquisition and use. Focusing solely on grammar rules and vocabulary memorization, without integrating them into meaningful contexts, is not an effective approach as it lacks the interactive and authentic language usethat is crucial for language learning.11、Which teaching method emphasizes student-centered learning and encourages students to discover knowledge through exploration and experimentation?A) Direct InstructionB) Inquiry-Based LearningC) Lecture-Style TeachingD) Behaviorist ApproachAnswer: BExplanation: Inquiry-Based Learning is a student-centered approach that encourages students to actively engage in the learning process by asking questions, conducting research, and drawing conclusions based on their findings. It fosters critical thinking, creativity, and problem-solving skills.12、Which of the following is a key component of assessing students’ language proficiency in junior high school English?A) Only focusing on standardized test scoresB) Evaluating students’ ability to communicate effectively in EnglishC) Ignoring students’ individual strengths and weaknessesD) Relying solely on teacher observationsAnswer: BExplanation: Assessing students’ language proficiency in junior highschool English involves evaluating their ability to communicate effectively in English, which includes listening, speaking, reading, and writing skills. While standardized test scores and teacher observations can be part of the assessment process, they should not be the sole criteria. It’s important to consider students’ individual strengths and weaknesses and provide them with opportunities to demonstrate their proficiency in various contexts.13、Which of the following teaching methods best encourages student interaction and communication in a junior high school English class?A) Grammar-Translation MethodB) Direct MethodC) Communicative Language Teaching (CLT)D) Audio-Lingual MethodAnswer: CExplanation: The Communicative Language Teaching (CLT) method emphasizes the use of authentic language for meaningful communication and encourages student-centered interaction and collaboration. It aligns well with promoting English language proficiency in a junior high school setting.14、When planning a lesson on past simple tense for junior high students, which of the following activities would be most appropriate to engage students and reinforce understanding?A) Asking students to recite grammar rules from a textbookB) Having students complete a gap-fill exercise with past simple verb formsC) Showing a video of a historical event and discussing it in the present tenseD) Encouraging students to write a short story in the future tense Answer: BExplanation: A gap-fill exercise that requires students to use past simple verb forms directly addresses the target grammar point in a practical and engaging way. It promotes understanding and application of the tense. Option A is too rote and memorization-based, option C misuses the tense, and optionD focuses on a different tense entirely.15、What is the primary goal of a lesson on reading comprehension in a junior high school English class?A) To teach students all the vocabulary words in the reading passageB) To ensure students can recite the reading passage word for wordC) To help students understand the main idea and details of the textD) To introduce students to as many grammatical structures as possible Answer: CExplanation: The primary goal of a reading comprehension lesson in a junior high school English class is to develop students’ ability to understand the main idea and key details of a text. This involves comprehension skills such as identifying the topic, understanding supporting details, and inferring meaning. Vocabulary expansion and grammatical analysis are important, but they are secondary to comprehending the overall content.16、Which of the following sentences demonstrates correct usage of the present continuous tense?A)She writes a letter every day.B)She is writing a letter right now.C)She wrote a letter yesterday.D)She has written a letter before.Answer: BExplanation: The present continuous tense is used to describe an action that is happening at the moment of speaking or to talk about an action that is planned or arranged for the near future. Option B, “She is writing a letter right now,” is the only one that fits this description. Option A is in the simple present tense, C is in the simple past tense, and D is in the present perfect tense.17、What grammatical term refers to the change in the form of a word to show the relationship between members of a sentence, such as subject and verb?A)ConcordB)ConcordanceC)ConcordancyD)ConjugationAnswer: AExplanation: Concord, also known as agreement, refers to the harmonious relationship between words in a sentence, especially with regard to number, gender, person, and tense. In this context, it refers to the way in which the form of a word (such as a verb) changes to agree with the subject of the sentence. Option B, “Concordance,” is typically used in text analysis to refer to the frequency of word occurrence; C, “Concordancy,” isnot a standard grammatical term; and D, “Conjugation,” refers to the changing of a verb’s form to show person, number, tense, mood, or voice.18、Which sentence best illustrates the use of the present perfect continuous tense?A)She has lived in New York for five years.B)She lived in New York for five years.C)She will have lived in New York for five years by next year.D)She has been living in New York for five years.Answer: DExplanation: The present perfect continuous tense is used to show that an action started in the past and has continued up until now, with no clear end point. It is formed using “has/have been” + the present participle (ing form) of the verb. Option D, “She has been living in New York for five years,” is the correct use of this tense. Option A uses the present perfect tense without the continuous aspect; B is in the simple past tense; and C is in the future perfect tense.19、Which of the following teaching methods emphasizes student-centered learning and encourages students to actively participate in the learning process?A) Direct InstructionB) Cooperative LearningC) Lecture MethodD) Discovery LearningAnswer: B) Cooperative LearningExplanation: Cooperative Learning is a teaching method that emphasizes student-centered learning and promotes active participation, collaboration, and communication among students. In this method, students work in small groups to achieve common learning goals.20、Which of the following is NOT a key component of Bloom’s Taxonomy of Educational Objectives?A) RememberingB) UnderstandingC) BelievingD) EvaluatingAnswer: C) BelievingExplanation: Bloom’s Taxonomy of Educational Objectives comprises six cognitive levels: Remembering, Understanding, Applying, Analyzing, Evaluating, and Creating. “Believing” is not a key component of this taxonomy.21、What is the primary purpose of the “Warm-up” stage in a lesson plan for English language teaching?A) To introduce new vocabularyB) To activate prior knowledge and interestC) To explain grammar rulesD) To assess students’ comprehensionAnswer: B) To activate prior knowledge and interestExplanation: The “Warm-up” stage in a lesson plan is typically used to engage students, activate their prior knowledge and interest related to the upcoming topic, and set the context for learning. It prepares students mentally and emotionally for the main activities of the lesson.22、Which of the following is NOT a grammar focus in junior high school English?A. TensesB. PronounsC. PhoneticsD. VoiceAnswer: CExplanation: Phonetics, which refers to the study of the sounds of language, is generally not a specific grammar focus in junior high school English curricula. Rather, grammar focuses in junior high school tend to include aspects such as tenses (A), pronouns (B), and voice (D), which are crucial for developing students’ understanding and use of English grammar.23、In the context of teaching reading comprehension, which strategy would be most effective for helping students understand difficult vocabulary?A. Ignoring unknown words and focusing on the main ideaB. Skipping over the passage and only answering comprehension questionsC. Using context clues to infer the meaning of wordsD. Immediately looking up every unknown word in a dictionaryAnswer: CExplanation: When teaching reading comprehension, encouraging students to use context clues to infer the meaning of unknown words is a highly effective strategy. This not only helps students understand the passage better but also enhances their vocabulary development. Ignoring unknown words (A) or skipping over the passage (B) can lead to a lack of comprehension. Immediately looking up every unknown word in a dictionary (D) can disrupt the flow of reading and limit students’ ability to develop their own inferencing skills.24、Which teaching method is most suitable for practicing listening comprehension in a junior high school English class?A. Dictation exercisesB. Reading aloud in groupsC. Grammar drillsD. Role-play activitiesAnswer: AExplanation: Dictation exercises, where the teacher reads a passage and students write down what they hear, are an excellent method for practicing listening comprehension in a junior high school English class. This activity requires students to listen attentively and process the information they hear, which helps develop their listening skills. Reading aloud in groups (B) is more focused on pronunciation and fluency, while grammar drills (C)and role-play activities (D) are more suitable for practicing speaking and grammar.25、Which of the following is NOT a grammatical function of English prepositions?A. Indicating the relationship between two nounsB. Showing the time or place of an actionC. Conjugating verbs with subjectsD. Linking adjectives to nounsAnswer: CExplanation: English prepositions serve multiple grammatical functions, including indicating the relationship between two nouns (e.g., “the book on the table”), showing the time or place of an action (e.g., “in the morning,” “at the store”), and linking adjectives to nouns (e.g., “good at math”). However, prepositions do not conjugate verbs with subjects, which is the function of verb forms and tenses. Conjugation refers to the changes in verb form to agree with the subject in number, person, and tense.26、Which of the following is an example of a compound noun in English?A. WatermelonB. UnhappinessC. RunningD. QuicklyAnswer: AExplanation: A compound noun is a noun that is composed of two or more words. In the case of “watermelon,” it is a single noun formed from the combination of “water” and “melon.” “Unhappiness” is an adjective (un-) modifying a noun (happiness), making it a compound adjective rather than a noun. “Running” is a verb form (present participle) and “quickly” is an adverb, neither of which are nouns.27、Which pronoun is used to refer to a non-specific person in English when making a general statement?A. HeB. SheC. TheyD. ItAnswer: CExplanation: When making a general statement about a non-specific person or people, English speakers often use the plural pronoun “they” as a gender-neutral alternative to avoid specifying a particular gender. For example, “If someone leaves their phone at home, they’ll need to come back for it.” Here, “they” is used as a singular, gender-neutral pronoun to refer to the non-specific “someone.” “He” and “she” would imply a specific gender, and “it” is typically used for non-human objects. 28、Which of the following is NOT a characteristic of effective teaching in English language arts for junior high school students?A)Encouraging critical thinking and analysisB)Focusing solely on grammar and vocabulary drillsC)Utilizing a variety of teaching methodsD)Engaging students in authentic communication activitiesAnswer: BExplanation: Effective teaching in English language arts for junior high school students often emphasizes critical thinking, analysis, and authentic communication activities. Focusing solely on grammar and vocabulary drills is a narrow approach that may not be as engaging or comprehensive as incorporating a variety of teaching methods that cater to different learning styles and needs.29、Which instructional strategy is particularly useful for helping junior high school students develop their reading comprehension skills?A)Providing students with pre-reading questions that guide their understandingB)Allowing students to choose their own reading materials without any guidanceC)Focusing solely on vocabulary development during reading lessonsD)Skipping over difficult passages and moving on to easier onesAnswer: AExplanation: Providing students with pre-reading questions can help guide their understanding and engage them more actively in the reading process. This strategy supports the development of reading comprehension skills by encouraging students to anticipate, predict, and reflect on the text. Allowing students to choose their own materials without guidance, focusingsolely on vocabulary, or skipping difficult passages are less effective strategies for improving reading comprehension.30、When designing a lesson plan for a junior high school English class,which of the following should be a primary consideration?A)The teacher’s personal preferences for teaching methodsB)The students’ interests and prior knowledgeC)The textbook’s curriculum without modificationD)The latest educational trends and fadsAnswer: BExplanation: When designing a lesson plan, it is essential to consider the students’ interests and prior kno wledge. This ensures that the lesson is relevant, engaging, and builds upon what students already know. While the teacher’s preferences, the textbook’s curriculum, and educational trends are all factors to consider, they should not overshadow the primary focus of tailoring instruction to meet the needs and interests of the students.二、简答题(20分)Question:Explain the importance of incorporating authentic materials in teaching English as a Foreign Language (EFL) for junior high school students, and provide at least two examples of such materials that can be used in a classroom setting.Answer:The incorporation of authentic materials in teaching English as a Foreign Language (EFL) to junior high school students is paramount for several reasons. Firstly, authentic materials, such as news articles, songs, videos, and podcasts, expose students to real-world language usage, thereby enhancing their comprehension and appreciation of English beyond the confines of traditional textbooks. This helps bridge the gap between classroom learning and everyday communication, fostering a deeper understanding of the language’s cultural and social contexts. Secondly, authentic materials engage students more effectively than often repetitive or contrived textbook content. They provide a variety of genres, styles, and topics that cater to different interests and learning styles, increasing motivation and retention. When students encounter language used in a natural, unedited manner, they become more adept at recognizing and processing it in a wide range of situations.Furthermore, authentic materials encourage critical thinking andproblem-solving skills as students analyze and interpret the information presented. They are often accompanied by visual and auditory cues that support comprehension, making the learning process more multisensory and memorable.Examples:1.News Articles: Choosing articles from reputable online news sources ornewspapers tailored to junior high school reading levels can enrichstudents’ vocabulary, expose them to current events, and develop their reading comprehension skills. For instance, a simplified article about environmental conservation in English can be used to discuss eco-friendly practices while teaching vocabulary related to nature and sustainability.2.Songs with Lyrics: Incorporating English songs into the curriculum notonly enhances listening skills but also provides opportunities forpronunciation practice and cultural insights. Teachers can select songs with lyrics that align with the class’s learning objectives, such as a pop song with simple vocabulary for beginners or a song with more complex grammar structures for intermediate learners. After listening, students can discuss the song’s meaning, identify new vocabulary, or even try to sing along, improving their pronunciation and fluency.In conclusion, the integration of authentic materials in EFL teaching for junior highschool students is crucial for fostering a more holistic, engaging, and culturally sensitive learning experience.三、教学情境分析题(30分)Question: Teaching Context Analysis (for Junior High School English Subject Knowledge and Teaching Ability)Scenario:Imagine you are preparing a lesson for Grade 8 students on the topic of“Future Plans and Dreams.” The students have already covered basic tense structures, including the simple present, present continuous, and past simple. This lesson aims to introduce and practice the use of the future tense (will/going to) in the context of discussing their personal aspirations and future plans.Task:1.Design a teaching activity that would effectively engage students inpracticing the use of the future tense to express their future plans and dreams. The activity should incorporate at least one technology tool or resource (e.g., interactive whiteboard, educational apps, online videos, etc.).2.Explain how this activity aligns with the following teaching objectives: •Students will be able to accurately use the future tense (will/going to) to describe their future plans and dreams.•Students will be able to listen attentively to their peers’ plans and provide supportive feedback.•Students will develop critical thinking skills through analyzing and comparing different future plans.Answer:1.Teaching Activity Design:Activity Name: “Dream Board Creation”Materials:•Interactive whiteboard or computer with a projection system•Access to an educational app or website that provides a digital canvas for creating visual presentations (e.g., Padlet, Google Slides, or a similar tool)•Pre-made slides or templates showcasing examples of future plans (careers, travel destinations, personal goals)Procedure:a.Introduction (5 minutes): Begin by using the interactive whiteboard todisplay a series of inspiring quotes and images related to future plans and dreams. Engage students in a brief discussion about their ownaspirations, encouraging them to share briefly in pairs.b.Tense Review (5 minutes): Quickly review the future tense (will/going to)structures, using the whiteboard to illustrate sentence structures and differences between the two forms.c.Modeling (10 minutes): Demonstrate how to create a “Dream Board” o n thechosen digital platform, using the pre-made slides as examples. Explain how they can incorporate text, images, and even audio/video clips to showcase their future plans.d.Individual/Pair Work (20 minutes): Have students work individually or inpairs to create their own Dream Boards. Encourage them to use the future tense to caption their images and describe their plans. Circulate around the room to provide individual support and guidance.e.Sharing and Feedback (15 minutes): Students take turns presenting their。
itti-A Model of Saliency-Based Visual Attention
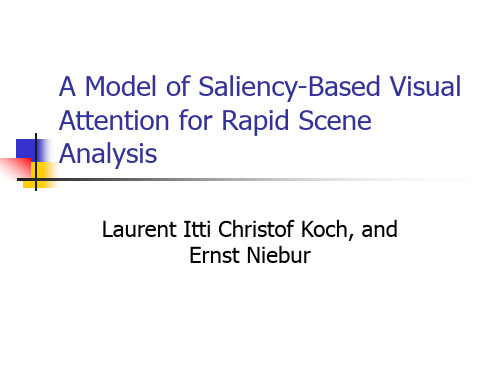
3.2 显著性图
,
,
特征图被结合成3个醒目度图,I表示灰度,C表示颜色,O表示方 向,位于显著性图的中间尺度-尺度4(sigma=4)。 通过跨尺度相加得到,将每个图压缩到尺度4,然后进行点对点 相加。 创建3个独立通道和进行独立的归一化的动机基于这样的假设: 相似的特征为了获得显著性而强烈竞争,而不同模态独立地对显 著性图做贡献。 3个醒目性图进行归一化后,求和,作为显著性图的最终输入S。
2 背景知识-其他
高斯金字塔 Gabor金字塔 WTA网络 弹出效应(pop-out) BU(Bottom-Up)和TD(Top-Down)
Fig. 3. Feature maps produced by the proposed model using a benchmark image. Saliency with respect to each feature is represented by brightness of grey shade. (a) Input. (b) Color contrast. (c) Eccentricity. (d) Orientation. (e) Symmetry. (f) Size. (g) Master map.
1.FOA转移到获胜的神经元位置; 2.激发了WTA的全局禁止机制,对所有WTA神经元禁止或重 置; 3.对SM中FOA新位置和一定大小的区域的局部禁止被暂时激 活,一方面可以使得下一个显著性变为胜者使得FOA转移,另 一方面也可以阻止FOA转移到前一个注意位置。
3.2 显著性图
为了保证向后续的与当前注意位置邻近的空间 显著性位置的跳跃模型有一定偏好,在FOA附 近的区域,一个小的刺激在SM中瞬时激活。 由于没有使用任何TD注意成分,FOA只是一个 圆区,其半径固定为输入图像宽度或高度中较 小者的1/6。 时间常数,电导和模拟神经元的激发阈值依据 神经物理学的结果进行选择:从一个显著位置 到另一个的FOA跳转大约为30-70ms,对已被 注意的位置的禁止时间大约为500-900ms。
基于注意力机制的轻量级水下图像风格迁移方法
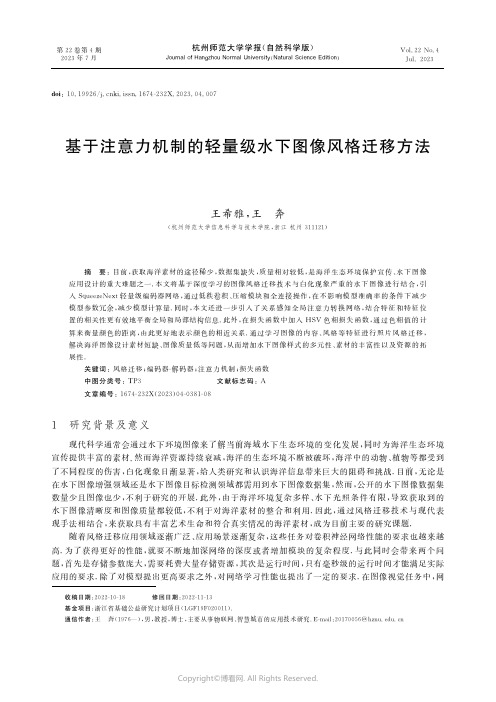
第22卷第4期2023年7月杭州师范大学学报(自然科学版)JournalofHangzhouNormalUniversity(NaturalScienceEdition)Vol.22No.4Jul.2023收稿日期:2022 10 18 修回日期:2022 11 13基金项目:浙江省基础公益研究计划项目(LGF19F020011).通信作者:王 奔(1976—),男,教授,博士,主要从事物联网、智慧城市的应用技术研究.E mail:20170056@hznu.edu.cn犱狅犻:10.19926/j.cnki.issn.1674 232X.2023.04.007基于注意力机制的轻量级水下图像风格迁移方法王希雅,王 奔(杭州师范大学信息科学与技术学院,浙江杭州311121)摘 要:目前,获取海洋素材的途径稀少,数据集缺失,质量相对较低,是海洋生态环境保护宣传、水下图像应用设计的重大难题之一.本文将基于深度学习的图像风格迁移技术与白化现象严重的水下图像进行结合,引入SqueezeNext轻量级编码器网络,通过低秩卷积、压缩模块和全连接操作,在不影响模型准确率的条件下减少模型参数冗余,减少模型计算量.同时,本文还进一步引入了关系感知全局注意力转换网络,结合特征和特征位置的相关性更有效地平衡全局和局部结构信息.此外,在损失函数中加入HSV色相损失函数,通过色相值的计算来衡量颜色的距离,由此更好地表示颜色的相近关系.通过学习图像的内容、风格等特征进行照片风格迁移,解决海洋图像设计素材短缺、图像质量低等问题,从而增加水下图像样式的多元性、素材的丰富性以及资源的拓展性.关键词:风格迁移;编码器 解码器;注意力机制;损失函数中图分类号:TP3 文献标志码:A文章编号:1674 232X(2023)04 0381 081 研究背景及意义现代科学通常会通过水下环境图像来了解当前海域水下生态环境的变化发展,同时为海洋生态环境宣传提供丰富的素材.然而海洋资源持续衰减,海洋的生态环境不断被破坏,海洋中的动物、植物等都受到了不同程度的伤害,白化现象日渐显著,给人类研究和认识海洋信息带来巨大的阻碍和挑战.目前,无论是在水下图像增强领域还是水下图像目标检测领域都需用到水下图像数据集,然而,公开的水下图像数据集数量少且图像也少,不利于研究的开展.此外,由于海洋环境复杂多样、水下光照条件有限,导致获取到的水下图像清晰度和图像质量都较低,不利于对海洋素材的整合和利用.因此,通过风格迁移技术与现代表现手法相结合,来获取具有丰富艺术生命和符合真实情况的海洋素材,成为目前主要的研究课题.随着风格迁移应用领域逐渐广泛、应用场景逐渐复杂,这些任务对卷积神经网络性能的要求也越来越高.为了获得更好的性能,就要不断地加深网络的深度或者增加模块的复杂程度.与此同时会带来两个问题,首先是存储参数庞大,需要耗费大量存储资源,其次是运行时间,只有毫秒级的运行时间才能满足实际应用的要求.除了对模型提出更高要求之外,对网络学习性能也提出了一定的要求.在图像视觉任务中,网Copyright ©博看网. All Rights Reserved.络模型往往需要通过快速扫描全局图像来获得需要关注的目标区域,而这过程中如何高效分配有限的注意力资源也成为一大难题.在此基础上,照片风格迁移更加重视图像色彩分布和色彩平衡,因此,网络模型除了要提升模型运行效率,保留内容主体结构之外,更要结合风格图像的色彩风格,生成既真实又美观的迁移图像.为解决上述问题,本文尝试将基于深度学习的图像风格迁移技术与白化现象严重的水下图像进行结合,提出一种基于关系感知全局注意力的轻量级水下图像照片风格迁移方法.通过引入SqueezeNext轻量级编码器网络,在不影响模型准确率的条件下减少模型参数冗余,提高模型计算的效率.同时,引入了关系感知全局注意力转换网络,结合特征和特征位置的相关性更有效地平衡全局和局部结构信息.此外,在损失函数中加入HSV色相损失函数,通过色相值的计算来衡量颜色的距离,由此更好地表示颜色相近的关系.通过学习图像特征结合注意力机制等方法进行真实感的水下图像风格迁移,以解决水下图像设计素材短缺、数据集缺少和图像质量差等问题,从而增加水下图像样式的多元性、素材的丰富性以及资源的拓展性.2 基于关系感知全局注意力的轻量级水下图像照片风格迁移算法2.1 模型构建由于编码器 解码器架构拥有“图到图”转换的优势,本章基于编码器解码器构建了如图1所示的风格迁移模型,该模型主要由SqueezeNext[1]轻量级网络、关系感知全局注意力、DIN动态实例归一化[2]和损失函数模块组成.其中SqueezeNext用于内容编码,减少网络参数量和计算成本;关系感知全局注意力用于增强特征的表示能力;DIN模块参数根据不同的风格自适应地改变,能更精确地对齐复杂风格的特征统计信息;损失函数用于对风格迁移网络进行迭代优化.图1 网络结构犉犻犵.1 犖犲狋狑狅狉犽狊狋狉狌犮狋狌狉犲2.2 轻量级编码器网络图2 犛狇狌犲犲狕犲犖犲狓狋网络结构犉犻犵.2 犛狇狌犲犲狕犲犖犲狓狋狀犲狋狑狅狉犽狊狋狉狌犮狋狌狉犲 本文选择SqueezeNext网络结构如图2所示,其主要操作有如下几个步骤:1)低秩卷积核.低秩分解设计的核心就是用小矩阵近似代替大矩阵,这样极大地减少了网络的参数数量,同时增加了网络的深度;2)模块压缩.深度卷积虽然确实能够减少计算量,但是实际在终端设备上的运行效率并不高,通过模块压缩有效地减少参数量,提高模型运算效率;3)全连接层.SqueezeNext相比于AlexNet中全连接层占比极高的参数量,通过瓶颈层压缩全连接层的输入维度减少了网络的参数量.由图2橙色部分可以看出,SqueezeNext网络将犓×犓卷积分解为两个大小分别为1×犓和犓×1的可分离卷积,有效地将参数的数量从犓2减少到2犓,并且在减少参数量的同时增加了网络的深度.283杭州师范大学学报(自然科学版)2023年 Copyright ©博看网. All Rights Reserved.2.3 关系感知全局注意力本章引入的“关系感知全局注意力”(relation awareglobalattention,RGA)[3]模块,如图3所示.在全局的结构信息上,能够进行有效的获取和高效的利用.RGA模块利用浅层卷积来学习注意力,核心是通过特征计算连接,将成对相关性/亲和力与其叠堆起来,来获取更全面和丰富的全局结构信息和局部信息,从而学习到更容易判别的高质量显著特征.图3 关系感知全局注意力模块结构犉犻犵.3 犜犺犲狊狋狉狌犮狋狌狉犲狅犳狉犲犾犪狋犻狅狀狊犺犻狆犪狑犪狉犲犵犾狅犫犪犾犪狋狋犲狀狋犻狅狀犿狅犱狌犾犲2.4 损失函数2.4.1 HSV色相损失函数HSV颜色模型[4]适合用于表示颜色相近的关系并衡量颜色的距离,但是在计算机中的图片主要使用RGB模进行图片的存储和表示,所以需要将图片的RGB表示模式转化为HSV模式,转换的公式为犎=0,ifmax=min;60×犌-犅max-min,ifmax=犚and犅≤犌;60×犌-犅max-min+360,ifmax=犚and犌<犅;60×犌-犅max-min+120,ifmax=犌;60×犌-犅max-min+240,ifmax=犅烅烄烆.(1) 其中犚、犌、犅分别为该图像点的RGB颜色量的分值,max和min为犚、犌、犅3个值中的最大值和最小值.为了使生成的迁移图像保持内容图像原有的色相特征,所以考虑只使用HSV颜色模型中的色相(H)维度,来平衡颜色相似度.颜色损失函数的定义为犾color=1狀H×狀W∑狀H犻=1∑狀W犼=1(犺犻,犼-^犺犻,犼)2.(2) 其中犺犻,犼和^犺犻,犼分别表示风格图像和生成图像在像素点处的色相值.2.4.2 总损失1)内容损失假设第犾层VGG 16网络犆犾有个大小的特征图.犎犾和犠犾分别表示犾层特征图的高和宽.犾层的响应可用矩阵表示为犉犾∈犚(犎犾×犠犾)×犆犾.(3) 内容损失通过计算内容图像在VGG 16网络第犾层的特征图和合成图像之间的欧氏距离的平方获得,即383 第4期王希雅,等:基于注意力机制的轻量级水下图像风格迁移方法Copyright ©博看网. All Rights Reserved.犾content=1(犎犾×犠犾)×犆犾‖犉犾(^狔)-犉犾(狔犮)‖22.(4) 2)风格损失通过计算风格图像和转换图像在VGG 16网络中特征图的Gram矩阵的犉范数的平方得到风格损失,其计算过程如下:犾style=‖犌犾(^狔)-犌犾(狔犛)‖2犉.(5) 其中,^狔为转换图像;狔犛为风格目标图像;犌犾为Gram矩阵,大小为犌犾×犌犾.3)总损失本文模型的总损失犾total将内容损失、风格损失、HSV色相损失和全变差正则化损失合并为一个线性函数,即犾total=α犾content(^狔,狔犮)+β犾style(^狔,狔狊)+γ犾color(^狔,狔狊)+δ犾TV.(6) 其中^狔,狔犮和狔狊分别为转换图像、内容图像和风格图像;α,β和γ分别为内容损失、风格损失和自适应加权损失的惩罚系数.全变差正则化犾TV用来增加合成图像的平滑性.3 实验结果分析3.1 实验环境及模型训练3.1.1 实验环境本文实验环境如表1所示.表1 实验运行环境犜犪犫.1 犈狓狆犲狉犻犿犲狀狋犪犾狅狆犲狉犪狋犻狅狀犲狀狏犻狉狅狀犿犲狀狋3.1.2 数据集选取内容图像数据集来自于UIEB[5]水下图像数据集,并于网络爬虫所得1200余张水下图片,共同组成本实验的数据集.水下图像数据集部分图像如图4所示.图4 水下图像数据集部分图像示例犉犻犵.4 犘犪狉狋犻犪犾犻犿犪犵犲犲狓犪犿狆犾犲狊狅犳狌狀犱犲狉狑犪狋犲狉犻犿犪犵犲犱犪狋犪狊犲狋483杭州师范大学学报(自然科学版)2023年 Copyright ©博看网. All Rights Reserved.3.1.3 模型训练由于使用SqueezeNext轻量级内容编码器代替了主流的VGGNet,因此分别从模型参数、模型执行一次所占内存、浮点运算数(FLOPs)、模型大小和模型运行时间对模型进行评价,并与VGGNet进行对比,结果如表2所示.表2 模型对比犜犪犫.2 犕狅犱犲犾犮狅犿狆犪狉犻狊狅狀模型模型参数内存/MBFLOPs/G模型大小/MB运行时间/sVGGNet1389235216.0822.166.430.65SqueezeNext428546125.837.742.800.34 从表2中数据可以看出,SqueezeNext在模型参数、模型执行一次所占内存、浮点运算数、模型大小方面较VGGNet小50%以上,证明本算法使用的轻量化网络在减少模型参数量,提升模型运算效率等方面的有效性.因此本模型对硬件设备的要求更低,更容易部署在移动设备上,实现轻量化的图像风格迁移.3.1.4 模型超参数配置本章提出的基于关系感知全局注意力的轻量级水下图像照片风格迁移方法超参数配置如表3所示.表3 基于关系感知全局注意力的轻量级照片风格迁移方法训练参数犜犪犫.3 犜狉犪犻狀犻狀犵狆犪狉犪犿犲狋犲狉狊狅犳犾犻犵犺狋狑犲犻犵犺狋狆犺狅狋狅狊狋狔犾犲狋狉犪狀狊犳犲狉犿犲狋犺狅犱犫犪狊犲犱狅狀狉犲犾犪狋犻狅狀狆犲狉犮犲狆狋犻狅狀犪狀犱犵犾狅犫犪犾犪狋狋犲狀狋犻狅狀照片信息训练参数EPOCH500BatchSize8优化算法Adam初始学习率0.001图像尺寸256×256损失函数权重(α,β,γ,δ)1,3×10-2,1×10-2和1×10-33.2 可视化对比实验本实验的对比算法主要选取了Avatar net[6]算法、SANet[7]算法、WCT2[8]和ArtFlow[9].Avatar net通过特征装饰进行多尺度零样本风格迁移;SANet通过平衡全局和局部风格模式进行风格迁移;WCT2将编码器和解码器中的下采样和上采样都换成了小波采样;ArtFlow基于内容泄漏问题提出无偏样式风格迁移.3.2.1 实验图像可视化对比照片风格迁移是在保留内容图像结构和细节的基础上,将图像的色调风格迁移成风格图像色调,因此对于生成图像来说,既要重视图像色调的迁移,保证图像的美观度,也要重视图像内容主体结构,保证图像的真实性.如图5所示,从内容角度来看,Avatar net存在明显的失真,部分内容语义信息出现明显的风格错误;SANet内容结构与原图较为一致,但在图像纹理上缺乏一定的美观度;WCT2结构主体保留得相对较好,只在部分内容细节处出现了一点伪影;ArtFlow内容结构较为突出明显,较好地区分了语义信息.从色彩角度来看,Avatar net生成图像的色彩明暗关系和色彩饱和度都与风格图像存在较大差异;SANet视觉质量较差,C2和C5图像颜色与风格图像存在一定的差异;WCT2色调迁移的效果较好,但在色彩细节处仍有明显的失真,如C3海水的高亮区颜色偏黄;ArtFlow色调美观度较差,视觉感受不平衡.本文方法在内容结构上,通过引入关系感知全局注意力,增强全局和局部的结构特征,更好保证了内容轮廓和内容细节的完整性;在色彩层次上,通过引入HSV色相损失函数平衡生成图像和风格图像的颜色相似度,在实现图像整体色调迁移的同时更好把握细节处的色彩.583 第4期王希雅,等:基于注意力机制的轻量级水下图像风格迁移方法Copyright ©博看网. All Rights Reserved.图5 可视化对比实验犉犻犵.5 犞犻狊狌犪犾犮狅狀狋狉犪狊狋犲狓狆犲狉犻犿犲狀狋3.2.2 消融实验1)关系感知全局注意力消融实验将本节算法与消除关系感知全局注意力机制后的生成图像进行对比,结果如图6所示.在去掉关系感知全局注意力之后,大鱼的主体轮廓边缘出现纹理扭曲的现象,且海水区域也存在纹理色块斑驳,其图像清晰度和图像视觉感受都远不如加入注意力机制后生成的图像.而通过增加关系感知全局注意力模块,结合了全局和局部的信息后,生成图像即能展示出更好的特征表示能力,避免细节的不清晰和纹理的斑驳.图6 关系感知全局注意力消融实验犉犻犵.6 犚犲犾犪狋犻狅狀狊犺犻狆狆犲狉犮犲狆狋犻狅狀犵犾狅犫犪犾犪狋狋犲狀狋犻狅狀犪犫犾犪狋犻狅狀犲狓狆犲狉犻犿犲狀狋图7 犎犛犞色相损失消融实验犉犻犵.7 犎犛犞犺狌犲犾狅狊狊犪犫犾犪狋犻狅狀犲狓狆犲狉犻犿犲狀狋683杭州师范大学学报(自然科学版)2023年 Copyright©博看网. All Rights Reserved. 2)色相损失消融实验本节算法与消除HSV色相损失函数后的生成图像对比结果如图7所示.在去掉HSV色相损失之后,生成图像不能很好地迁移风格图像的色调且图像色彩饱和度较低.加入HSV色相损失函数后,更好地平衡图像的颜色相似度,从而极大地提升了风格迁移的色彩美观度.3)消融实验定量指标对比本文使用峰值信噪比(PSNR)和结构相似性(SSIM)这2个指标进行图像的消融实验定量指标对比,PSNR指标作为评价图像的客观标准,SSIM指标用于评价图像的结构相似性,两者值越大表示图像的质量越高.注意力消融实验和色相损失消融实验图像的PSNR和SSIM指标数值对比如表4所示,其实验数据证明了本章加入的关系感知全局注意力和色相损失函数在风格迁移上的有效性.表4 消融实验犘犛犖犚和犛犛犐犕定量指标对比犜犪犫.4 犆狅犿狆犪狉犻狊狅狀狅犳狇狌犪狀狋犻狋犪狋犻狏犲犻狀犱犲狓犲狊狅犳犘犛犖犚犪狀犱犛犛犐犕犻狀犪犫犾犪狋犻狅狀犲狓狆犲狉犻犿犲狀狋3.2.3 定量指标对比实验PSNR定量指标数值对比如表5所示,SSIM定量指标数值对比如表6所示.从可视化图像中可以看出Avatar net图像清晰度和迁移效果较差,因此PSNR和SSIM值也较低;SANet和ArtFlow色彩层次不鲜明,局部语义失真,PSNR和SSIM值较为平均;WCT2需要对图像进行语义分割,PSNR和SSIM值相对较高,但局部内容细节风格溢出.而本文方法更好地关联全局和局部结构特征,更高地保留内容语义信息,色彩层次丰富,结构轮廓清晰,图像质量更高,因此PSNR和SSIM值也更高.表5 犘犛犖犚定量指标对比犜犪犫.5 犆狅犿狆犪狉犻狊狅狀狅犳犘犛犖犚狇狌犪狀狋犻狋犪狋犻狏犲犻狀犱犻犮犪狋狅狉狊图像Avatar netSANetWCT2ArtFlowOursC127.39527.84727.72427.45927.882C228.01328.20428.04927.68628.218C327.91428.34928.82628.65828.424C427.92828.20727.99628.00028.420C528.10227.89828.17528.20728.267表6 犛犛犐犕定量指标对比犜犪犫.6 犆狅犿狆犪狉犻狊狅狀狅犳犛犛犐犕狇狌犪狀狋犻狋犪狋犻狏犲犻狀犱犻犮犪狋狅狉狊图像Avatar netSANetWCT2ArtFlowOursC10.6710.3140.5790.4800.695C20.5800.4180.5450.6090.588C30.5120.4690.7280.6110.792C40.3120.3840.6240.5580.658C50.5230.4190.7090.3970.7924 总结本文将基于深度学习的图像风格迁移技术与白化现象严重的水下图像进行结合,提出基于关系感知全局注意力的轻量级水下图像照片风格迁移方法,通过引入SqueezeNext轻量级编码器网络、关系感知全局注意力转换网络以及在损失函数中加入HSV色相损失函数进行风格迁移.实验证明,本文方法不仅更好地保留了内容的主体结构,并且有效地丰富了图像的色彩层次,提升了水下图像样式的多元性以及素材的丰富性.783 第4期王希雅,等:基于注意力机制的轻量级水下图像风格迁移方法Copyright ©博看网. All Rights Reserved.参考文献:[1]GHOLAMIA,KWONK,WUB,etal.SqueezeNext:hardware awareneuralnetworkdesign[C]//ProceedingsoftheIEEEConferenceonComputerVisionandPatternRecognition(CVPR)Workshops.SaltLakeCity:IEEE,2018:1638 1647.[2]JINGYC,LIUXA,DINGYK,etal.Dynamicinstancenormalizationforarbitrarystyletransfer[J].ProcAAAIConfArtifIntell,2020,34(4):4369 4376.[3]ZHANGZZ,LANCL,ZENGWJ,etal.Relation awareglobalattentionforpersonre identification[C]//2020IEEE/CVFConferenceonComputerVisionandPatternRecognition(CVPR).Seattle:IEEE,2020:3186 3195.[4]王飞,金玉苹,祖培福.基于HSV颜色模型的图像风格迁移算法[J].电子元器件与信息技术,2022,6(6):217 220.[5]LICY,GUOCL,RENWQ,etal.Anunderwaterimageenhancementbenchmarkdatasetandbeyond[J].IEEETransImageProcess,2020,29:4376 4389.[6]LIYJ,FANGC,YANGJM,etal.Universalstyletransferviafeaturetransforms[C]//Proceedingsofthe31stInternationalConferenceonNeuralInformationProcessingSystems.LongBeach:ACM,2017:385 395.[7]PARKDY,LEEKH.Arbitrarystyletransferwithstyle attentionalnetworks[C]//2019IEEE/CVFConferenceonComputerVisionandPatternRecognition(CVPR).LongBeach:IEEE,2019:5880 5888.[8]YOOJ,UHY,CHUNS,etal.Photorealisticstyletransferviawavelettransforms[C]//2019IEEE/CVFInternationalConferenceonComputerVision(ICCV).Seoul:IEEE,2019:9036 9045.[9]ANJ,HUANGSY,SONGYB,etal.ArtFlow:unbiasedimagestyletransferviareversibleneuralflows[C]//2021IEEE/CVFConferenceonComputerVisionandPatternRecognition(CVPR).Nashville:IEEE,2021:862 871.犚犲狊犲犪狉犮犺狅狀犔犻犵犺狋狑犲犻犵犺狋犝狀犱犲狉狑犪狋犲狉犐犿犪犵犲犛狋狔犾犲犜狉犪狀狊犳犲狉犕犲狋犺狅犱犅犪狊犲犱狅狀犚犲犾犪狋犻狅狀狊犺犻狆犪狑犪狉犲犃狋狋犲狀狋犻狅狀犕犲犮犺犪狀犻狊犿WANGXiya,WANGBen(SchoolofInformationScienceandTechnology,HangzhouNormalUniversity,Hangzhou311121,China)犃犫狊狋狉犪犮狋:Atpresent,therearefewwaystoobtainmarinematerialswiththemissingdatasetsandrelativelylowquality,whichisoneofthemajorproblemsinmarineecologicalenvironmentprotectionpublicityandunderwaterimageapplicationdesign.Thispapercombinedimagestyletransfertechnologybasedondeeplearningwiththeunderwaterimagewithseriouswhiteningphenomenon,andintroducedtheSqueezeNextlightweightencodernetwork.Throughlowrankconvolution,compressionmoduleandfullconnectionoperation,theredundancyofmodelparametersandtheamountofmodelcalculationwerereducedwithoutaffectingtheaccuracyofthemodel.Furthermore,thispaperalsointroducedrelationship awareglobalattentionnetworktobalancetheglobalandlocalstructuralinformationeffectivelybycombiningthecorrelationbetweenfeaturesandfeaturepositions.Inaddition,HSVhuelossfunctionwasaddedtothelossfunctiontomeasurethedistanceofcolorsthroughthecalculationofhuevalues,whichcouldbetterrepresentthecloserelationshipofcolors.Byphotostyletransferringinfeaturesoflearningimagescontentsandstyle,theproblemssuchastheshortageofmaterialsandlowimagequalityinmarineimagedesigncouldbesolved.Consequently,thediversityofunderwaterimagestyles,therichnessofmaterialsandtheexpansionofresourceswereenhanced.犓犲狔狑狅狉犱狊:styletransfer;encoder decoder;attentionmechanism;lossfunction883杭州师范大学学报(自然科学版)2023年 Copyright ©博看网. All Rights Reserved.。
国外认知症友好社区研究现状与启示

作者: 傅琦[1]
作者机构: [1]浙江树人大学树兰国际医学院
出版物刊名: 社会与公益
页码: 35-40页
年卷期: 2021年 第5期
主题词: 认知症友好社区;阿尔茨海默病;CiteSpace
摘要:认知症是常见的老年病之一,也是全球关注的重大公共卫生问题.如何从国外的认知症友好社区研究中汲取经验,拓展我国的研究与实践,是本文力图解决的问题.本文以科学网数据库(Web of Science)核心合集2009—2020年发表的219篇文献为数据样本,利用CiteSpace软件绘制知识图谱,对国外认知症友好社区研究的发文量趋势、国别(地区)差异、热点词汇与前沿主题、关键节点文献进行介绍,并从研究的主题、对象及方法三个角度总结拓展国内研究的路径及启示.。
面板排序模型结构渐变的识别及应用
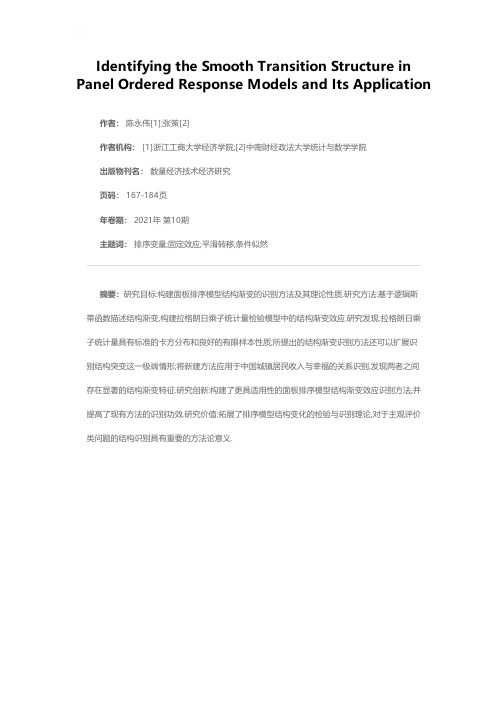
Identifying the Smooth Transition Structure in Panel Ordered Response Models and Its Application 作者: 陈永伟[1];张策[2]
作者机构: [1]浙江工商大学经济学院;[2]中南财经政法大学统计与数学学院
出版物刊名: 数量经济技术经济研究
页码: 167-184页
年卷期: 2021年 第10期
主题词: 排序变量;固定效应;平滑转移;条件似然
摘要:研究目标:构建面板排序模型结构渐变的识别方法及其理论性质.研究方法:基于逻辑斯
蒂函数描述结构渐变,构建拉格朗日乘子统计量检验模型中的结构渐变效应.研究发现:拉格朗日乘子统计量具有标准的卡方分布和良好的有限样本性质;所提出的结构渐变识别方法还可以扩展识
别结构突变这一极端情形;将新建方法应用于中国城镇居民收入与幸福的关系识别,发现两者之间存在显著的结构渐变特征.研究创新:构建了更具适用性的面板排序模型结构渐变效应识别方法,并提高了现有方法的识别功效.研究价值:拓展了排序模型结构变化的检验与识别理论,对于主观评价类问题的结构识别具有重要的方法论意义.。
一种针对海面SAR图像的视觉注意模型设计

一种针对海面SAR图像的视觉注意模型设计熊伟;徐永力【摘要】On the basis of studying the theories of classical ITTI visual attention models, the defects of traditional visual models applied to sea-surface SAR images are summarized according to the characteristics of the background and the target of sea-surface SAR images. A visual attention model design algorithm for sea-surface SAR images is proposed. Firstly, the model uses the basic framework of the classical ITTI model, selects and extracts the texture and shape features that can describe the SAR image well. Then the corresponding saliency map of features is obtained. Secondly, the new integration mechanism of the saliency map of features is adopted to replace the linear-adding mechanism of the classical model for fusing the saliency maps and obtaining the overall saliency map. Finally, the gray features of the attention focus of all the saliency maps are integrated to select the optimal significance characterization. By using the multi-scale competitive strategy, the filtering and threshold segmentation are completed to realize the accurate screening of significant areas. Therefore, the detection of the significant areas of SAR images is completed. Experiments were carried out by using Terra-SAR-X and other satellite data, and their results verified the good significance-detection effects of the model. The model can better meet the demands of the detection of high-resolution image targets. By carrying out further comparative analysis with the classical visual model, it is discovered thatthe proposed algorithm can not only reduce the impact of the false alarm caused by speckle noise and uneven sea-clutter background on the detection result, but also greatly improve the detection speed by 25% to 45%.%在研究了经典ITTI等视觉注意模型的理论基础上,结合海面SAR图像背景及目标特点,对传统视觉模型应用于海面SAR图像的缺陷进行分析总结,提出一种适用于海面SAR图像视觉注意模型设计算法.首先,模型借鉴经典IT-TI模型的基本框架,选择并提取了能够较好描述SAR图像的纹理和形状特征,求取相应的特征显著图;其次,采用新的特征显著图整合机制替代经典模型的线性相加机制进行显著图融合得到总显著图;最后,综合各特征显著图下注意焦点的灰度特征,选择最佳的显著性表征,完成通过多尺度竞争策略对显著图的滤波及阈值分割实现显著区域的精确筛选,从而完成SAR图像的显著区域检测.实验采用TerraSAR-X等多幅卫星数据进行仿真实验,结果验证了模型良好的显著性检测效果,更符合实际高分辨率图像目标检测的应用需求.通过进一步与经典视觉模型对比分析,模型在改善了由斑点噪声和不均匀的海杂波背景对检测结果产生的虚警影响的同时,检测速度也较之提高了25%~45%.【期刊名称】《电光与控制》【年(卷),期】2018(025)005【总页数】7页(P73-78,91)【关键词】合成孔径雷达图像;视觉注意模型;特征显著图;融合策略;注意焦点【作者】熊伟;徐永力【作者单位】海军航空大学信息融合研究所,山东烟台 264001;海军航空大学信息融合研究所,山东烟台 264001【正文语种】中文【中图分类】TP7530 引言合成孔径雷达(SAR)具有全天时、全天候、大范围、多参数等特点,是海洋监测与监视应用的重要组成部分。
salience theory代码 -回复

salience theory代码-回复什么是salience theory?Salience theory是一种心理学理论,主要用于解释人们如何从信息中选择关注的内容。
它认为,当人们被大量信息所包围时,他们往往会选择关注那些对自己有意义、与自身经验有关,以及与目标和需求一致的信息。
这就意味着,需求、经验和个人诉求会对人们的注意力产生显著影响,并决定信息的重要性和吸引力。
在salience theory中,salience是指信息的重要性或吸引力。
这与人们的注意力定向有关,人们倾向于关注那些对他们当前的需求和目标最具有意义的信息。
这也是为什么人们会在购物网站上看到自己感兴趣的商品推荐,或者在社交媒体上看到与自己兴趣相关的内容推送。
这些平台通过分析用户的需求和喜好,将最相关和有吸引力的信息发送给用户,以引起他们的注意。
人们的经验和知识也会影响信息的salience。
当人们对某个主题有更多的了解和经验时,他们会更容易注意和理解与该主题相关的信息。
例如,一个喜欢足球的人可能更容易注意和关注与足球相关的新闻;而一个对动漫了解较深的人可能更容易留意与动漫有关的话题。
除了需求和经验之外,个人诉求也是决定信息salience的重要因素。
人们常常会被那些与自己的目标、欲望和价值观一致的信息所吸引。
例如,对于一个环保主义者来说,关注环保问题的报道更具有吸引力,而对于一个汽车爱好者来说,与汽车相关的信息将更容易引起他的关注。
总的来说,salience theory提供了对信息选择和注意力分配的一个解释框架。
人们倾向于关注那些与自己需求和经验相关,以及与自身目标和价值观一致的信息。
此理论具有深远的影响,不仅适用于个人的日常生活,还适用于广告宣传、市场营销等领域。
通过了解salience theory,我们能够更好地理解人们的注意力偏好,并为信息的有效传递提供指导。
知识自动获取的实现
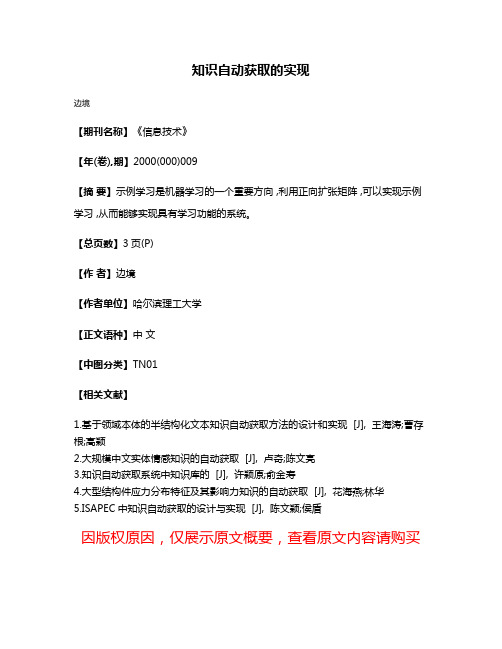
知识自动获取的实现
边境
【期刊名称】《信息技术》
【年(卷),期】2000(000)009
【摘要】示例学习是机器学习的一个重要方向 ,利用正向扩张矩阵 ,可以实现示例学习 ,从而能够实现具有学习功能的系统。
【总页数】3页(P)
【作者】边境
【作者单位】哈尔滨理工大学
【正文语种】中文
【中图分类】TN01
【相关文献】
1.基于领域本体的半结构化文本知识自动获取方法的设计和实现 [J], 王海涛;曹存根;高颖
2.大规模中文实体情感知识的自动获取 [J], 卢奇;陈文亮
3.知识自动获取系统中知识库的 [J], 许颖原;俞金寿
4.大型结构件应力分布特征及其影响力知识的自动获取 [J], 花海燕;林华
5.ISAPEC中知识自动获取的设计与实现 [J], 陈文颖;侯盾
因版权原因,仅展示原文概要,查看原文内容请购买。
图像数据挖掘之研究

图像数据挖掘之研究
刘钊;蒋良孝
【期刊名称】《计算机工程与应用》
【年(卷),期】2003(039)033
【摘要】从介绍一个图像数据挖掘可以采用的系统原型出发,对图像数据挖掘的常用方法和基本过程进行了详细的阐述.
【总页数】3页(P202-204)
【作者】刘钊;蒋良孝
【作者单位】中国地质大学信息工程学院,武汉,430074;中国地质大学信息工程学院,武汉,430074
【正文语种】中文
【中图分类】TP311.13
【相关文献】
1.基于图像映射的关联规则数据挖掘方法研究 [J], 王飞
2.基于决策树的海量医学图像数据挖掘方法研究 [J], 任仪
3.支持差分隐私的图像数据挖掘方法研究 [J], 杨云鹿;周亚建;宁华
4.基于关联规则的压缩图像数据挖掘方法研究 [J], 赵燕萍
5.基于改进Faster R-CNN的变压器巡检图像数据挖掘研究 [J], 贺月;常永娟因版权原因,仅展示原文概要,查看原文内容请购买。
结合句法增强与图注意力网络的方面级情感分类
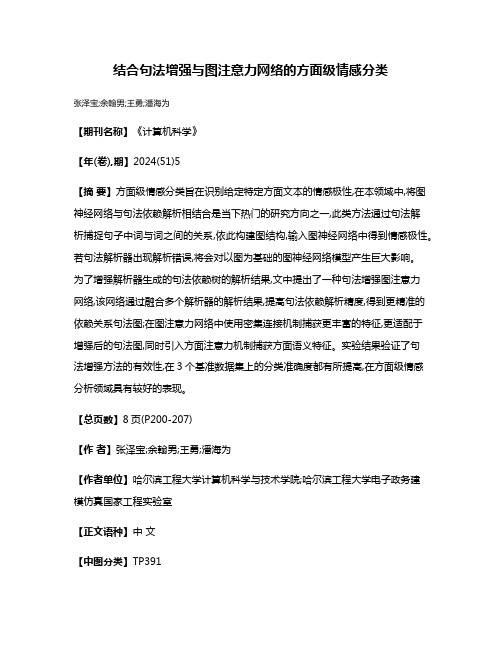
结合句法增强与图注意力网络的方面级情感分类
张泽宝;余翰男;王勇;潘海为
【期刊名称】《计算机科学》
【年(卷),期】2024(51)5
【摘要】方面级情感分类旨在识别给定特定方面文本的情感极性,在本领域中,将图神经网络与句法依赖解析相结合是当下热门的研究方向之一,此类方法通过句法解
析捕捉句子中词与词之间的关系,依此构建图结构,输入图神经网络中得到情感极性。
若句法解析器出现解析错误,将会对以图为基础的图神经网络模型产生巨大影响。
为了增强解析器生成的句法依赖树的解析结果,文中提出了一种句法增强图注意力
网络,该网络通过融合多个解析器的解析结果,提高句法依赖解析精度,得到更精准的依赖关系句法图;在图注意力网络中使用密集连接机制捕获更丰富的特征,更适配于
增强后的句法图,同时引入方面注意力机制捕获方面语义特征。
实验结果验证了句
法增强方法的有效性,在3个基准数据集上的分类准确度都有所提高,在方面级情感分析领域具有较好的表现。
【总页数】8页(P200-207)
【作者】张泽宝;余翰男;王勇;潘海为
【作者单位】哈尔滨工程大学计算机科学与技术学院;哈尔滨工程大学电子政务建
模仿真国家工程实验室
【正文语种】中文
【中图分类】TP391
【相关文献】
1.面向上下文注意力联合学习网络的方面级情感分类模型
2.结合显式句法依赖与分层注意力进行方面级情感分析
3.基于句法结构树和混合注意力网络的方面级情感分类
4.使用方面引导图注意网络的方面级情感分类模型
5.基于注意力融合网络的方面级多模态情感分类
因版权原因,仅展示原文概要,查看原文内容请购买。
一种基于注意力与反注意力机制的视频超分辨率重建模型研究
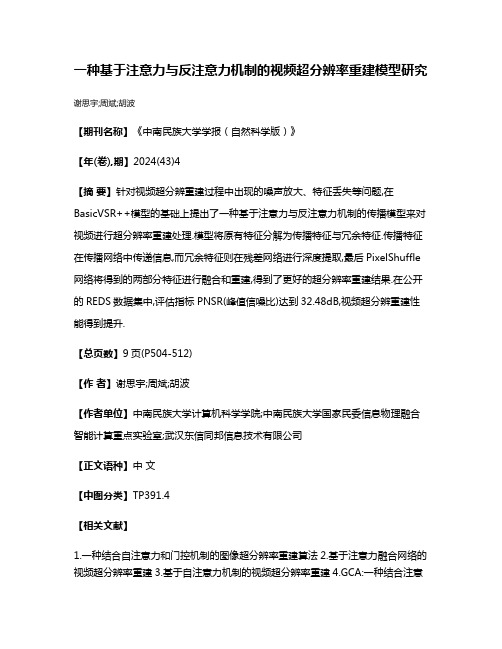
一种基于注意力与反注意力机制的视频超分辨率重建模型研究谢思宇;周斌;胡波
【期刊名称】《中南民族大学学报(自然科学版)》
【年(卷),期】2024(43)4
【摘要】针对视频超分辨重建过程中出现的噪声放大、特征丢失等问题,在BasicVSR++模型的基础上提出了一种基于注意力与反注意力机制的传播模型来对视频进行超分辨率重建处理.模型将原有特征分解为传播特征与冗余特征.传播特征在传播网络中传递信息,而冗余特征则在残差网络进行深度提取,最后PixelShuffle 网络将得到的两部分特征进行融合和重建,得到了更好的超分辨率重建结果.在公开的REDS数据集中,评估指标PNSR(峰值信噪比)达到32.48dB,视频超分辨重建性能得到提升.
【总页数】9页(P504-512)
【作者】谢思宇;周斌;胡波
【作者单位】中南民族大学计算机科学学院;中南民族大学国家民委信息物理融合智能计算重点实验室;武汉东信同邦信息技术有限公司
【正文语种】中文
【中图分类】TP391.4
【相关文献】
1.一种结合自注意力和门控机制的图像超分辨率重建算法
2.基于注意力融合网络的视频超分辨率重建
3.基于自注意力机制的视频超分辨率重建
4.GCA:一种结合注意
力机制的图像超分辨率重建模型5.一种基于通道注意力机制的交通监控视频超分辨率算法
因版权原因,仅展示原文概要,查看原文内容请购买。
- 1、下载文档前请自行甄别文档内容的完整性,平台不提供额外的编辑、内容补充、找答案等附加服务。
- 2、"仅部分预览"的文档,不可在线预览部分如存在完整性等问题,可反馈申请退款(可完整预览的文档不适用该条件!)。
- 3、如文档侵犯您的权益,请联系客服反馈,我们会尽快为您处理(人工客服工作时间:9:00-18:30)。
17
Results
Image
Saliency Map
High saliency Locations (yellow circles)
Michigan State University
18
Shifting Attention
l
l
Using 2D “winnertake-all” neural network at scale 4 FOA shifts every 30-70 ms
11
Normalization Operator
l l
Promotes maps with few strong peaks Surpresses maps with many comparable peaks
1. 2. 3. 4.
Normalization of map to range [0…M] Compute average m of all local maxima Find the global maximum M Multiply the map by (M – m)2
Michigan State University
3
Architecture
Michigan State University
4
Gaussian Pyramids
80x60
Scaling by a factor 2x2
l
Repeated low-pass filtering
I (σ + 1) = Subsampled [ I (σ ) ∗ G5×5 ]
l
l l
The key idea of saliency map is to extract local spatial discontinuities in the modalities of color, intensity and orientation. Use two layers of neurons to model shifting attention. Model appears to work accurately and robustly (but difficult to evaluate)
RG(c, s) = | (R(c) - G(c)) Θ (G(s) - R(s)) | BY(c, s) = | (B(c) - Y(c)) Θ (Y(s) - B(s)) | Same c and s as with intensity
10
Michigan State University
Orientation Feature Maps
l
Create Gabor pyramids for θ = {0º, 45º, 90º, 135º}
O(c, s,θ ) = O(c,θ ) − O( s,θ )
l
c and s again similar to intensity
Michigan State University
c = 2 s =c +3
O= ∑ º,135º} N c⊕2 s =⊕+3 N (O(c, s,θ )) = c θ ∈{0 º , 45 º , 90
4
Michigan State University 14
c+4
Saliency Map
l
Average all conspicuity maps
“Saliency-Based Visual Attention”
“Computational Modeling of Visual Attention”, Itti, Koch, (Nature Reviews – Neuroscience 2001 ) “A Model of Saliency-Based Visual Attention for Rapid Scene Analysis” , Itti, Koch and Niebur’s (IEEE PAMI 1998)
20
Michigan State University
Discussion
l
l l l
l
No top-down attention modeling, e.g., top-down spacial control, obejct-based attention. Biologically plausible? Neuromorphic architecture? In which way the top-down and bottom-up processes are related? In which way the attention and recognition are integrated and interacted with each other?
9
Michigan State University
Colour Feature Maps
Similar to double-opponent cells (Prim. V. C) Red-Green and Yellow-Blue
+R-G +G-R +G-R +R-G +B-Y +Y-B +Y-B +B-Y
Itti, Koch: “Computational Modeling of Visual Attention”
Nature Reviews – Neuroscience Vol. 2 (2001)
Michigan State University
22
l l l
Focus of attention Results Conclusion
Michigan State University
2
Related Work
l l l
“Feature Integration Theory,” Treisman & Gelade, 1980. Computational model of bottom-up attention, Koch and Ullman, 1985 Saliency map is believed to be located in the posterior parietal cortex (Gotlieb, et al., 1998) and the pulvinar nuclei of the thalamus (Roinson & Peterson, 1992)
Michigan State University
19
Summary
l
Saliecy map can be broken down into main steps
l l l
Create pyramids for 5 channels of original image Determine feature maps then conspicuity maps Combine into saliency map (after normalizing)
Michigan State University
6
Preprocessing
Intensity
R
G
B
Y
Michigan State University
7
Center-surround Difference
l
Achieve center-surround difference through across-scale difference
5
Preprocessing
l l l
Original image with red, green, blue channels Intensity as I = (r + g + b)/3 Broadly tuned color channels
R = r - (g + b)/2 G = g - (r + b)/2 B = b - (r + g)/2 Y = (r + g)/2 - |r – g|/2 - b
21
Michigan State University
References
Itti, Koch, and Niebur: “A Model of Saliency-Based Visual Attention for Rapid Scene Analysis”
IEEE PAMI Vol. 20, No. 11, November (1998)
N ( I ) + N (C ) + N (O) S= 3
Michigan State University
15
Neural Layers
Stimulus
l
l
l
Saliency Map (SM) modeled as layer of leaky integrate-and-fire neurons SM feeds into winner-take-all (WTA) neural network Inhibition of Return as transient inhibition of SM at FOA
Michigan State University
12
Normalization Operator
Michigan State University
13
Conspicuity Maps
I = ⊕ ⊕ N ( I (c, s ))
c = 2 s =c +3
4 c+4
4
c+ 4
C = ⊕ ⊕ [N ( RG(c, s)) + N ( BY (c, s))]