China's Monthly CPI
大二下英语复习 课文翻译
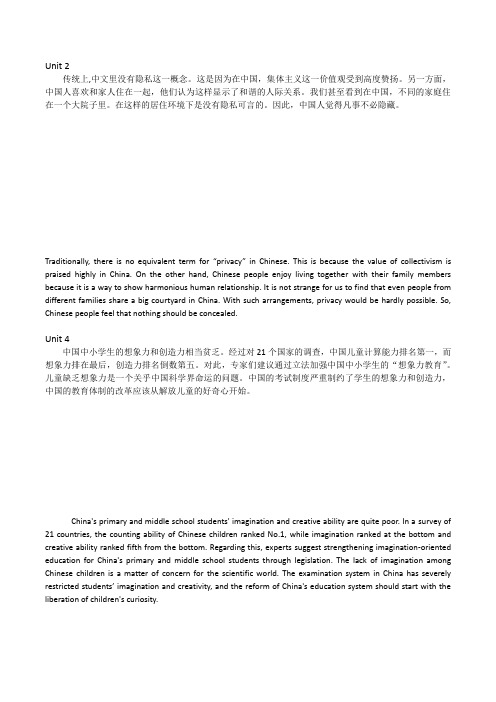
传统上,中文里没有隐私这一概念。
这是因为在中国,集体主义这一价值观受到高度赞扬。
另一方面,中国人喜欢和家人住在一起,他们认为这样显示了和谐的人际关系。
我们甚至看到在中国,不同的家庭住在一个大院子里。
在这样的居住环境下是没有隐私可言的。
因此,中国人觉得凡事不必隐藏。
Traditionally, there is no equivalent term for “privacy” in Chinese. This is because the value of collectivism is praised highly in China. On the other hand, Chinese people enjoy living together with their family members because it is a way to show harmonious human relationship. It is not strange for us to find that even people from different families share a big courtyard in China. With such arrangements, privacy would be hardly possible. So, Chinese people feel that nothing should be concealed.Unit 4中国中小学生的想象力和创造力相当贫乏。
经过对21个国家的调查,中国儿童计算能力排名第一,而想象力排在最后,创造力排名倒数第五。
对此,专家们建议通过立法加强中国中小学生的“想象力教育”。
儿童缺乏想象力是一个关乎中国科学界命运的问题。
中国的考试制度严重制约了学生的想象力和创造力,中国的教育体制的改革应该从解放儿童的好奇心开始。
China's primary and middle school students' imagination and creative ability are quite poor. In a survey of 21 countries, the counting ability of Chinese children ranked No.1, while imagination ranked at the bottom and creative ability ranked fifth from the bottom. Regarding this, experts suggest strengthening imagination-oriented education for China's primary and middle school students through legislation. The lack of imagination among Chinese children is a matter of concern for the scientific world. The examination system in China has severely restricted students’ imagination and creativity, and the reform of China's education system should start with the liberation of children's curiosity.自去年11月以来,中国的通货膨胀持续上扬。
中国GDP增长率,历年失业率,CPI增长率数据及图表
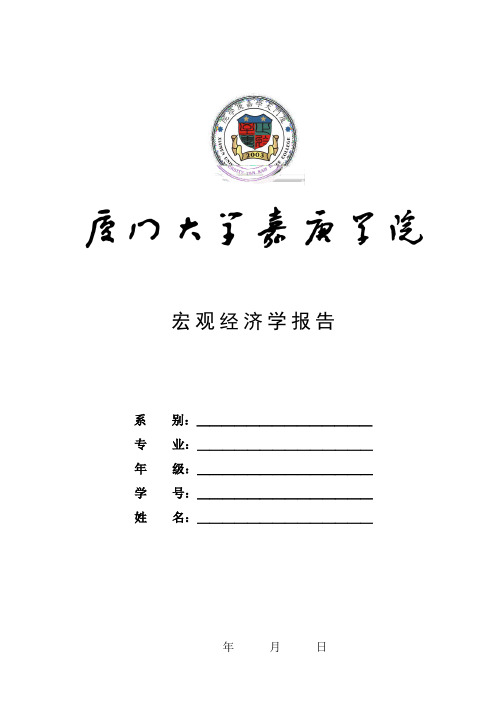
宏观经济学报告系别:______________专业:______________年级:______________学号:______________姓名:______________年月日一,GDP 数据从图表上可以看出,中国历年来的GDP 增长率在不断波动,特别是2008年经济危机,以至于由2007年的14.2%跌至2008年的9.6%,处于 不稳定状态,经济复苏困难重重。
支出法:由图投资所占GDP的比例历年都比较重,近年不断上升,投资带动经济增长。
由图消费的比例逐年有所下降,虽然国家有加强投入拉动内需消费的资金帮助但是由于中国人本来所固有的消费观念,从消费影响经济还有望提高。
由图政府购买的比例比较稳定,稳定的政府购买可以更好的平衡国民生产总值,对经济发展有一定的帮助。
贸易的比例变化比较大,可以看到近年来贸易比例不断上升,这说明中国已融入世界这个大家庭,但是如果对外贸易占国民生产总值的比例加大一旦发生金融危机那么国家的经济就会受到很大的冲击。
收入法:由图可知政府收入比例在历年有所下降,但是政府的实际财政收入增加了不少,由下表可以看出,政府越来越富有。
但是丹从比例上看,可以说明,社会主义下的市场经济越来越富有活力,政府干预减少了。
二,CPI 数据居民消费价格水平的变动率在一定程度上反映了通货膨胀(或紧缩)的程度,一般市场经济国家认为CPI 增长率在2%~3%属于可接受范围内,有时候CPI 的增长也代表着经济的增长。
由图表可以显示中国在大部分年份的增长是比较正常的。
三,失业率:机导致中国的失业率逐年上升,就业前景不容乐观。
按照近几年来国家人口资源和社会保障部公布的官方数据,即便在危机发生后的2008年和2009年,中国的城镇登记失业率也始终保持在5%以下。
但是,很多半官方和民间的研究机构根据自己调查的数据对中国失业率进行了估算,实际的调查结果远没有官方部门那么乐观。
例如,2008年12月16日中国社科院公布的《社会蓝皮书》称,中国城镇失业率约为9.4%,这一数字是保障部统计数字的两倍还要多。
2010年中国CPI指数
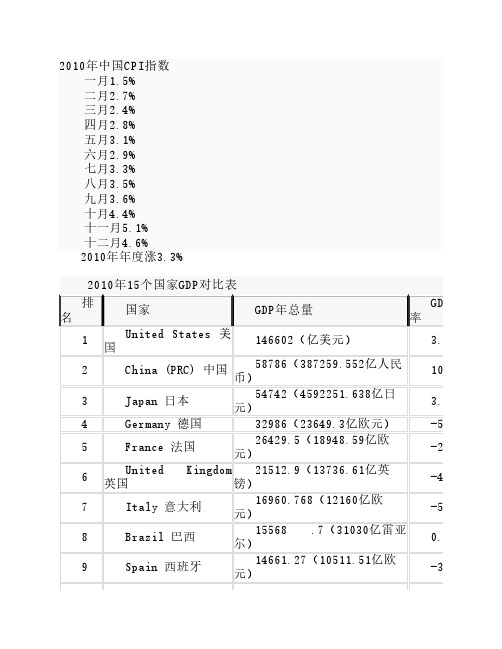
澳大利 9163.78(11664.58亿澳 元)
14
Mexico 墨西哥
8557 .5(115503.47亿比 索)
15 South Korea 韩国 8200 (1050万亿韩元)
-2.5%
-7.9% 7.2% -1.4% -3.7% 0.2%
2011年中国CPI指数 1月份,4.9% 1月份,居民消费价格总水平同比上涨4.9%。其中,城市上涨 4.8%,农村上涨5.2%;食品价格上涨10.3%,非食品价格上涨2.6%; 消费品价格上涨5.0%,服务项目价格上涨4.6%。居民消费价格总水平 环比上涨1.0%。其中,食品价格上涨2.8%,食品中鲜菜价格上涨 14.4%、鲜蛋价格上涨2.3%。 2月份,同比上涨4.9%涨幅与1月份持平 2月份,居民消费价格同比上涨4.9%,涨幅与1月份持平。其中, 城市上涨4.8%,农村上涨5.5%;食品价格上涨11.0%,非食品价格上 涨2.3%;消费品价格上涨5.4%,服务项目价格上涨3.8%。分类别看, 食品价格同比上涨11.0%,烟酒及用品类价格同比上涨1.9%,衣着类 价格同比上涨0.4%,家庭设备用品及维修服务价格同比上涨1.4%,医 疗保健及个人用品类价格同比上涨3.0%,娱乐教育文化用品及服务类 价格同比上涨0.3%,居住价格同比上涨6.1%,交通和通信类价格同比 下降0.3%。1-2月份,居民消费价格同比上涨4.9%。 3月份居民消费价格同比上涨5.4%,环比下降0.2%。一季度居民 消费价格同比上涨5.0%。其中,城市上涨4.9%,农村上涨5.5%。分类 别看,食品上涨11.0%,烟酒及用品上涨2.0%,衣着上涨0.3%,家庭 设备用品及维修服务上涨1.6%,医疗保健和个人用品上涨3.1%,交通 和通信下降0.1%,娱乐教育文化用品及服务上涨0.6%,居住上涨 6.5%。 4月份CPI统计数据,CPI同比上涨5.3%。通货膨胀压力依然很 大。
gpi的长短期影响因素:基于中国数据的检验
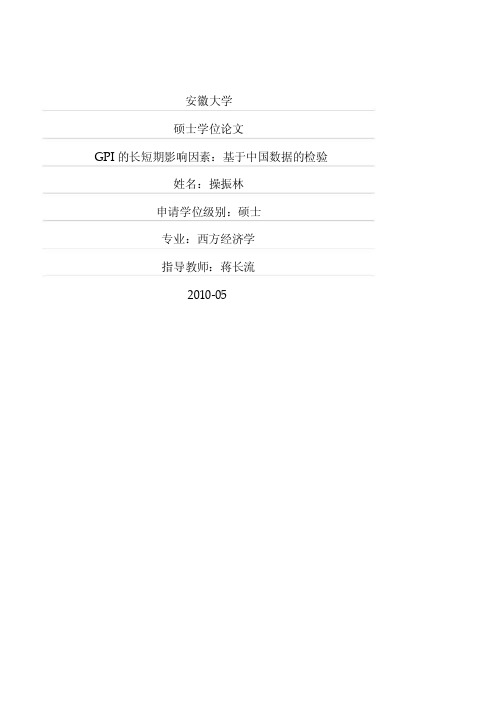
安徽大学硕士学位论文GPI的长短期影响因素:基于中国数据的检验姓名:操振林申请学位级别:硕士专业:西方经济学指导教师:***2010-05中文摘要CPI作为宏观经济的核心指标之一,是企业、政府等相关部门重点关注对象之一。
对于企业而言,它是企业增加或者减少投资的重要决策依据;对于政府而言,CPI则是掌握居民消费价格状况,研究和制定工资政策,货币政策的依据。
我国改革开放30年的时间内,CPI经历了高峰,也经历了低谷。
过高的CPI使得居民的消费成本提高,过低的CPI同时也表明整个国家的经济正处于萧条状态,正因如此,有必要研究CPI运行过程中的影响因素,从而为熨平CPI的波动提前做好相关工作,防止经济的大起大落。
本文以货币数量论和总需求-总供给模型为依托,选取货币供应量、城乡居民家庭人均可支配收入、社会消费品零售总额、贷款余额、财政支出、进口额、出口额、外汇储备、平均货币工资指数、农业生产资料价格指数、工业品出厂价格指数、原材料、燃料和动力购进价格指数这12 个因素作为分析CPI 的代表影响因素。
同时把这些因素分为长期因素和短期因素,长期因素选取货币供应量,其余的都作为短期影响因素。
文章利用Eviews 软件对影响CPI 的因素借助于货币数量论,AD-AS 模型进行理论说明。
接下来的实证分析中,用协整分析和误差修正模型分析我国的货币供给对CPI 影响,结果表明货币供应量和CPI 之间存在长期的均衡关系。
就CPI 的短期影响因素通过因子分析,提取了需求因子、成本因子和投资因子,并且就所提取的三个因子中载荷最高的因素进行深入分析,建立了向量自回归模型来考察它们对CPI 的影响。
实证分析表明,对我国CPI 影响最大的因素在于需求方而不是供给方,其次影响CPI 的上游价格会最终传导给CPI,但是中途会有时滞。
最后固定资产投资对我过CPI 的影响很大。
文章最后提出针对当前通胀预期不断加大的趋势,应该收缩信贷规模,可以适当提高利率或存款准备金率。
消费物价指数CPI

消费物价指数(CPI)市场敏感度:非常高。
含义:十分流行的测算零售商品和服务价格通货膨胀的经济指标。
美国发布时间:美国东部时间上午8时30分;在报告当月的第二周或第三周发布。
频率:每月一次。
来源:美国劳工部劳动统计局。
修正:没有月度的修正。
年度的变化会在2月份,随1月GDP数据公布时做下介绍。
修正能上溯到5年前。
中国发布时间:月度一般在月后13号左右,季度、年度则延至月后20号左右。
频率:每月一次。
来源:中华人民共和国国家统计局CPI在我国叫做城乡居民消费价格指数。
由于我国城乡人口有13亿,这13亿消费者中有乡村居民有城市居民,有收入高的也有收入低的,有生活在东南沿海的居民也有生活在内地的居民,所以我们说CPI是13亿人平均水平的CPI。
消费物价指数英文缩写为CPI,是根据与居民生活有关的产品及劳务价格统计出来的物价变动指标,通常作为观察通货膨胀水平的重要指标。
讨论通货膨胀时,最常提及的物价指数之一。
消费者物价指数上升,有通货膨胀的压力,此时中央银行可能藉由调高利率来加以控制,对美元来说是利多,利空黄金。
反之煮熟下降,利空美元,利多黄金。
不过,由于与生活相关的产品多为最终产品,其价格只涨不跌,因此,消费者物价指数也未能完全反应价格变动的实情。
一般说来当CPI>3%的增幅时我们称为INFLATION,就是通货膨胀;而当CPI>5%的增幅时,我们把他称为SERIOUS INFLATION,就是严重的通货膨胀。
消费者物价指数(CPI , Consumer Price Index)是世界各国普遍编制的一种指数,它可以用于分析市场价格的基本动态,是政府制定物价政策和工资政策的重要依据。
指的是衡量所选定的一篮子消费品购买价格的指数。
计算指数时,每种商品的权数依据1982-1984年间该商品在城市消费者生活开支中所占的份额来确定。
它是反映与居民生活有关的产品及劳务价格统计出来的物价变动指标,通常作为观察通货膨胀水平的重要指标。
中国日报中英文对照解析1

China dismisses deflation concerns,expects further demand recoveryBEIJING -- Despite(prep. 尽管,虽然;不由自主)slower price growth in China since(prep. 自…以后;何曾conj. 自从;因为;既然adv. 后来)the start of this year, concerns over(对)deflations in the country are unnecessary as(conj. 当;因为)its economy is on a solid recovery track(在一个坚实的恢复轨道上)amid pro-growth policies(在促进增长的政策), officials and analysts said.北京——官员和分析人士表示,尽管自今年年初以来,中国的物价增长有所放缓,但对通货紧缩的担忧是不必要的,因为在支持增长的政策下,中国经济正处于坚实的复苏轨道上。
China's consumer price index (CPI), a main gauge(指标)of inflation, rose 0.7 percent year on year(年同比,与前一年同期比较)in March, compared with(与…比较,与…相比)the 2.1 percent and 1 percent gain seen in January and February, respectively(adv. 各自地;各个地;分别地).中国的消费者价格指数(CPI)是通货膨胀的主要指标,三月份同比上涨0.7%,而一月份和二月份的涨幅分别为2.1%和1%。
At a press conference(在新闻发布会上)this week, Fu Linghui, a spokesperson with the National Bureau of Statistics, said deflation is not evident(不明显)in China at present and will not appear in the next stage(在下一阶段).在本周的新闻发布会上,国家统计局发言人傅令辉表示,中国目前的通货紧缩并不明显,下一阶段也不会出现。
CPI定义及计算公式.doc
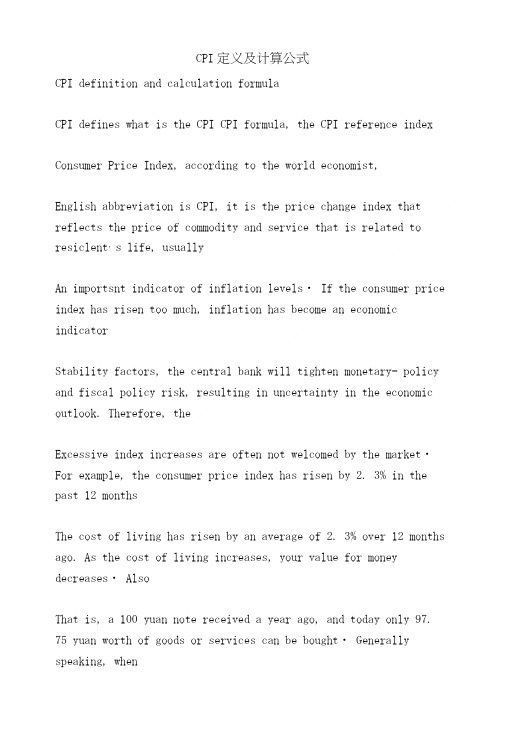
CPI定义及计算公式CPI definition and calculation formulaCPI defines what is the CPI CPI formula, the CPI reference indexConsumer Price Index, according to the world economist,English abbreviation is CPI, it is the price change index that reflects the price of commodity and service that is related to resiclent,s life, usuallyAn importsnt indicator of inflation levels・ If the consumer price index has risen too much, inflation has become an economic indicatorStability factors, the central bank will tighten monetary- policy and fiscal policy risk, resulting in uncertainty in the economic outlook. Therefore, theExcessive index increases are often not welcomed by the market・For example, the consumer price index has risen by 2. 3% in the past 12 monthsThe cost of living has risen by an average of 2. 3% over 12 months ago. As the cost of living increases, your value for money decreases・ AlsoThat is, a 100 yuan note received a year ago, and today only 97. 75 yuan worth of goods or services can be bought・ Generally speaking, whenThe increase in CPI>3%, which we call Inflation, is inflation;and when CPI>5% increases, we call it ,z inflation."Serious Inflation, that's serious inflation・The price change that consumers pay for goods and services is also Consumer price index, CPIConsumer Price Index (consumer price index), is a fixed consumer goods basket price measurement, mainly reflecteda tool for measuring inflation, varying in percentage termsIn the form of expression. In the United States, the main commodities that constitute the indicators are divided into seven categories, including: food, wine and beverage homes;Clothing, transportation, medicine, health, recreation, other goods and services・ In the United States, the consumer price index is determined by the Bureau of labor statistics every monthThere are two different consumer price indices published・ One is the consumer price index (CPI-W) for workers and staff members・Two is the cityConsumer price index (CPI), referred to as CPI~U・Referenee index(CPI) is a lagging data, but it is often an important reference indicator of market economic activity and government monetarypolic y.CPI stability, full employment and GDP growth are often the most important socio-economic goals. However, from the reality of China, CPIStability and its importance are not as authoritative as developed countries think, and market economic activities will be based on CPIChange to adjust〃・In recent years, the growth of GDP in Europe and America has fluctuated around 2%, and CPI has changed from 0%to 3% in the same way,The situation in China is quite different・ The first is the rapid growth of the domestic economy. In the past two years, GDP growth has exceeded 9%, while CPI has notHow much volatility, seemingly, this can be said to be "the government,s economic regulation and control freely, the market behavior reflects very rationa1.The two isWithin a year, CPI is volatile, with a difference of several percentage points; in general, unless there are major emergenciesin economic lifeCPI, such as the Asian financial crisis in 1997, was unlikely torise and fall, so China,s CPI fluctuated sharply in 2004Often. Three, with the volatility of the CPI, the domestic economy has a high rate of inflation at one time, the people,s savingsby a fixed set of goods negative interest rates are serious, a time residentSavings again bid farewell to negative returns and shadow ofdeflation recur ・ Such an economic environment is worrying, so how do you understand the CPI index?Become a very important question.Calculation formulaThe calculating formula of CPI is CPI 二(a fixed set of goods is calculated according to the current price (value) divided according to the base periodThe value of the price) multiplied by 100. What CPI tells people is that for the expenditure of the average family, buy arepresentative oneGroup goods cost more today than at some time in the past, for example, in 1995, an average family buys a group each monthThe cost of goods was $800, while the cost of buying this group in 2000 was $1000, then the country's consumer price index for 2000 The number of CPI 二(base year 1995) 1000/800*100二 125, is rising by 25%・ In our daily life, we are more concerned aboutIt is inflation rate, which is defined as the percentage change in price level from one period to another ・ The formula is T 二 (Pl - PO) /PO,The formula T is 1 times the rate of inflation, Pl and PO are respectively 1 times and 0 times the price level. If you use the above informationThe price index is a measure of the price level, and inflation is the percentage of changes in the consumer price index at different times・ If oneThe consumer price index of an economy rose from 100 last year to 112 this year, and the rate of inflation during this period wasT= (112 一100) /100* 100%= 12%, that is to say, inflation rate of 12%, representing a 12% rise in prices・。
告诉你30年来中国的实际年通货膨胀率
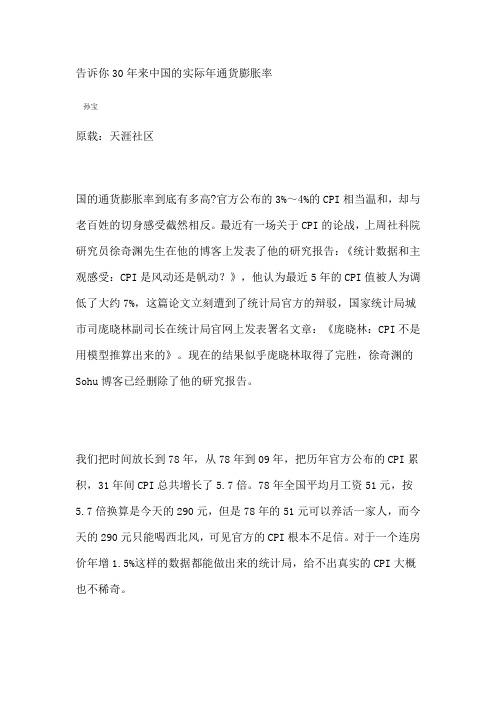
告诉你30年来中国的实际年通货膨胀率孙宝原载:天涯社区国的通货膨胀率到底有多高?官方公布的3%~4%的CPI相当温和,却与老百姓的切身感受截然相反。
最近有一场关于CPI的论战,上周社科院研究员徐奇渊先生在他的博客上发表了他的研究报告:《统计数据和主观感受:CPI是风动还是帆动?》,他认为最近5年的CPI值被人为调低了大约7%,这篇论文立刻遭到了统计局官方的辩驳,国家统计局城市司庞晓林副司长在统计局官网上发表署名文章:《庞晓林:CPI不是用模型推算出来的》。
现在的结果似乎庞晓林取得了完胜,徐奇渊的Sohu博客已经删除了他的研究报告。
我们把时间放长到78年,从78年到09年,把历年官方公布的CPI累积,31年间CPI总共增长了5.7倍。
78年全国平均月工资51元,按5.7倍换算是今天的290元,但是78年的51元可以养活一家人,而今天的290元只能喝西北风,可见官方的CPI根本不足信。
对于一个连房价年增1.5%这样的数据都能做出来的统计局,给不出真实的CPI大概也不稀奇。
我们从货币购买力的角度来估计一下实际的CPI。
1、按基本物价计算,78年至今货币贬值了58倍,年CPI高达14%网上有一篇徐以升先生的博文《系统性质疑CPI》,介绍了南方基金高级研究员万晓西先生做过的统计,他从《北京至&#·物价志》、《上海价格志》、《广州市志&#·物价志》等资料上查询了1978年的单品价格,包括食品、医疗、衣服、交通、烟酒和居住等6大类,然后和今天的价格相比,估算出78年的51元的购买力,大概与今天北京3000元相当。
31年贬值了58倍,换算成通货膨胀率的话高达每年14%!CPI指数14%,比GDP增长率还高,这个结论太耸人听闻了。
这种算法当然是有缺陷的,原因就在于电子、汽车等工业制造品在78年的时候无从买起,物价的比较只考虑了78年能买到的商品。
工业制造品跌价很快,如果将工业制造品的价格也列入比较的话,算出的CPI不会有14%那么离谱。
chinadaily经济类新闻 中英对照版 口译必备
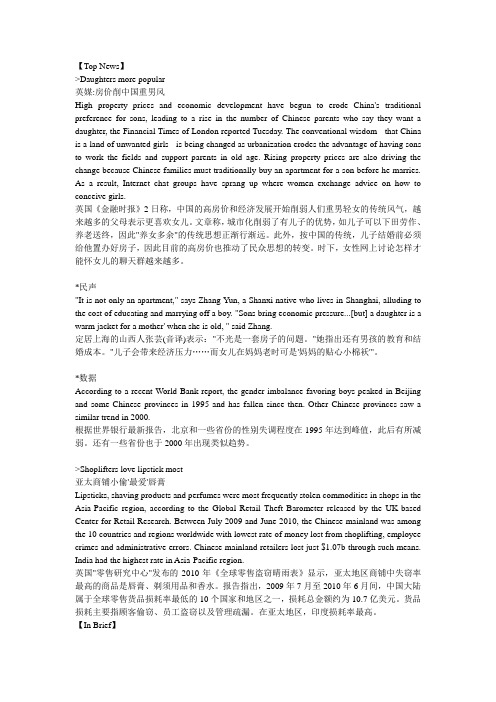
【Top News】>Daughters more popular英媒:房价削中国重男风High property prices and economic development have begun to erode China's traditional preference for sons, leading to a rise in the number of Chinese parents who say they want a daughter, the Financial Times of London reported Tuesday. The conventional wisdom - that China is a land of unwanted girls - is being changed as urbanization erodes the advantage of having sons to work the fields and support parents in old age. Rising property prices are also driving the change because Chinese families must traditionally buy an apartment for a son before he marries. As a result, Internet chat groups have sprang up where women exchange advice on how to conceive girls.英国《金融时报》2日称,中国的高房价和经济发展开始削弱人们重男轻女的传统风气,越来越多的父母表示更喜欢女儿。
文章称,城市化削弱了有儿子的优势,如儿子可以下田劳作、养老送终,因此"养女多余"的传统思想正渐行渐远。
中英文双语外文文献翻译:一种基于...
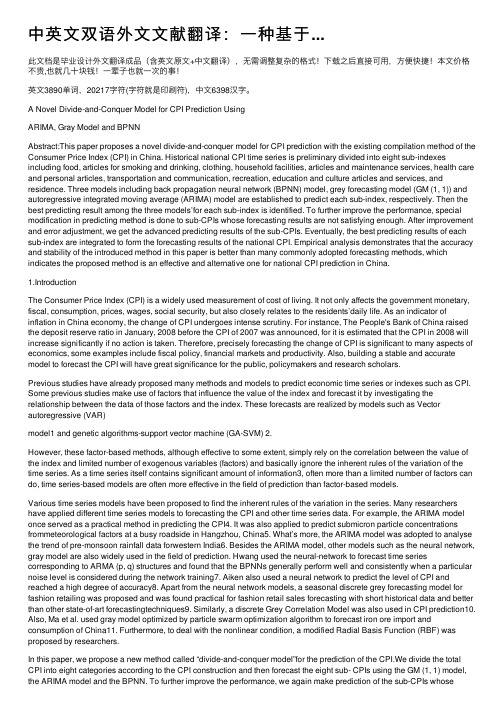
中英⽂双语外⽂⽂献翻译:⼀种基于...此⽂档是毕业设计外⽂翻译成品(含英⽂原⽂+中⽂翻译),⽆需调整复杂的格式!下载之后直接可⽤,⽅便快捷!本⽂价格不贵,也就⼏⼗块钱!⼀辈⼦也就⼀次的事!英⽂3890单词,20217字符(字符就是印刷符),中⽂6398汉字。
A Novel Divide-and-Conquer Model for CPI Prediction UsingARIMA, Gray Model and BPNNAbstract:This paper proposes a novel divide-and-conquer model for CPI prediction with the existing compilation method of the Consumer Price Index (CPI) in China. Historical national CPI time series is preliminary divided into eight sub-indexes including food, articles for smoking and drinking, clothing, household facilities, articles and maintenance services, health care and personal articles, transportation and communication, recreation, education and culture articles and services, and residence. Three models including back propagation neural network (BPNN) model, grey forecasting model (GM (1, 1)) and autoregressive integrated moving average (ARIMA) model are established to predict each sub-index, respectively. Then the best predicting result among the three models’for each sub-index is identified. To further improve the performance, special modification in predicting method is done to sub-CPIs whose forecasting results are not satisfying enough. After improvement and error adjustment, we get the advanced predicting results of the sub-CPIs. Eventually, the best predicting results of each sub-index are integrated to form the forecasting results of the national CPI. Empirical analysis demonstrates that the accuracy and stability of the introduced method in this paper is better than many commonly adopted forecasting methods, which indicates the proposed method is an effective and alternative one for national CPI prediction in China.1.IntroductionThe Consumer Price Index (CPI) is a widely used measurement of cost of living. It not only affects the government monetary, fiscal, consumption, prices, wages, social security, but also closely relates to the residents’daily life. As an indicator of inflation in China economy, the change of CPI undergoes intense scrutiny. For instance, The People's Bank of China raised the deposit reserve ratio in January, 2008 before the CPI of 2007 was announced, for it is estimated that the CPI in 2008 will increase significantly if no action is taken. Therefore, precisely forecasting the change of CPI is significant to many aspects of economics, some examples include fiscal policy, financial markets and productivity. Also, building a stable and accurate model to forecast the CPI will have great significance for the public, policymakers and research scholars.Previous studies have already proposed many methods and models to predict economic time series or indexes such as CPI. Some previous studies make use of factors that influence the value of the index and forecast it by investigating the relationship between the data of those factors and the index. These forecasts are realized by models such as Vector autoregressive (VAR)model1 and genetic algorithms-support vector machine (GA-SVM) 2.However, these factor-based methods, although effective to some extent, simply rely on the correlation between the value of the index and limited number of exogenous variables (factors) and basically ignore the inherent rules of the variation of the time series. As a time series itself contains significant amount of information3, often more than a limited number of factors can do, time series-based models are often more effective in the field of prediction than factor-based models.Various time series models have been proposed to find the inherent rules of the variation in the series. Many researchers have applied different time series models to forecasting the CPI and other time series data. For example, the ARIMA model once served as a practical method in predicting the CPI4. It was also applied to predict submicron particle concentrations frommeteorological factors at a busy roadside in Hangzhou, China5. What’s more, the ARIMA model was adopted to analyse the trend of pre-monsoon rainfall data forwestern India6. Besides the ARIMA model, other models such as the neural network, gray model are also widely used in the field of prediction. Hwang used the neural-network to forecast time series corresponding to ARMA (p, q) structures and found that the BPNNs generally perform well and consistently when a particular noise level is considered during the network training7. Aiken also used a neural network to predict the level of CPI and reached a high degree of accuracy8. Apart from the neural network models, a seasonal discrete grey forecasting model for fashion retailing was proposed and was found practical for fashion retail sales forecasting with short historical data and better than other state-of-art forecastingtechniques9. Similarly, a discrete Grey Correlation Model was also used in CPI prediction10. Also, Ma et al. used gray model optimized by particle swarm optimization algorithm to forecast iron ore import and consumption of China11. Furthermore, to deal with the nonlinear condition, a modified Radial Basis Function (RBF) was proposed by researchers.In this paper, we propose a new method called “divide-and-conquer model”for the prediction of the CPI.We divide the total CPI into eight categories according to the CPI construction and then forecast the eight sub- CPIs using the GM (1, 1) model, the ARIMA model and the BPNN. To further improve the performance, we again make prediction of the sub-CPIs whoseforecasting results are not satisfying enough by adopting new forecasting methods. After improvement and error adjustment, we get the advanced predicting results of the sub-CPIs. Finally we get the total CPI prediction by integrating the best forecasting results of each sub-CPI.The rest of this paper is organized as follows. In section 2, we give a brief introduction of the three models mentioned above. And then the proposed model will be demonstrated in the section 3. In section 4 we provide the forecasting results of our model and in section 5 we make special improvement by adjusting the forecasting methods of sub-CPIs whose predicting results are not satisfying enough. And in section 6 we give elaborate discussion and evaluation of the proposed model. Finally, the conclusion is summarized in section 7.2.Introduction to GM(1,1), ARIMA & BPNNIntroduction to GM(1,1)The grey system theory is first presented by Deng in 1980s. In the grey forecasting model, the time series can be predicted accurately even with a small sample by directly estimating the interrelation of data. The GM(1,1) model is one type of the grey forecasting which is widely adopted. It is a differential equation model of which the order is 1 and the number of variable is 1, too. The differential equation is:Introduction to ARIMAAutoregressive Integrated Moving Average (ARIMA) model was first put forward by Box and Jenkins in 1970. The model has been very successful by taking full advantage of time series data in the past and present. ARIMA model is usually described as ARIMA (p, d, q), p refers to the order of the autoregressive variable, while d and q refer to integrated, and moving average parts of the model respectively. When one of the three parameters is zero, the model is changed to model “AR”, “MR”or “ARMR”. When none of the three parameters is zero, the model is given by:where L is the lag number,?t is the error term.Introduction to BPNNArtificial Neural Network (ANN) is a mathematical and computational model which imitates the operation of neural networks of human brain. ANN consists of several layers of neurons. Neurons of contiguous layers are connected with each other. The values of connections between neurons are called “weight”. Back Propagation Neural Network (BPNN) is one of the most widely employed neural network among various types of ANN. BPNN was put forward by Rumelhart and McClelland in 1985. It is a common supervised learning network well suited for prediction. BPNN consists of three parts including one input layer, several hidden layers and one output layer, as is demonstrated in Fig 1. The learning process of BPNN is modifying the weights of connections between neurons based on the deviation between the actual output and the target output until the overall error is in the acceptable range.Fig. 1. Back-propagation Neural Network3.The Proposed MethodThe framework of the dividing-integration modelThe process of forecasting national CPI using the dividing-integration model is demonstrated in Fig 2.Fig. 2.The framework of the dividing-integration modelAs can be seen from Fig. 2, the process of the proposed method can be divided into the following steps: Step1: Data collection. The monthly CPI data including total CPI and eight sub-CPIs are collected from the official website of China’s State Statistics Bureau (/doc/d62de4b46d175f0e7cd184254b35eefdc9d31514.html /).Step2: Dividing the total CPI into eight sub-CPIs. In this step, the respective weight coefficient of eight sub- CPIs in forming the total CPI is decided by consulting authoritative source .(/doc/d62de4b46d175f0e7cd184254b35eefdc9d31514.html /). The eight sub-CPIs are as follows: 1. Food CPI; 2. Articles for Smoking and Drinking CPI; 3. Clothing CPI; 4. Household Facilities, Articles and Maintenance Services CPI; 5. Health Care and Personal Articles CPI; 6. Transportation and Communication CPI;7. Recreation, Education and Culture Articles and Services CPI; 8. Residence CPI. The weight coefficient of each sub-CPI is shown in Table 8.Table 1. 8 sub-CPIs weight coefficient in the total indexNote: The index number stands for the corresponding type of sub-CPI mentioned before. Other indexes appearing in this paper in such form have the same meaning as this one.So the decomposition formula is presented as follows:where TI is the total index; Ii (i 1,2, ,8) are eight sub-CPIs. To verify the formula, we substitute historical numeric CPI and sub-CPI values obtained in Step1 into the formula and find the formula is accurate.Step3: The construction of the GM (1, 1) model, the ARIMA (p, d, q) model and the BPNN model. The three models are established to predict the eight sub-CPIs respectively.Step4: Forecasting the eight sub-CPIs using the three models mentioned in Step3 and choosing the best forecasting result for each sub-CPI based on the errors of the data obtained from the three models.Step5: Making special improvement by adjusting the forecasting methods of sub-CPIs whose predicting results are not satisfying enough and get advanced predicting results of total CPI. Step6: Integrating the best forecasting results of 8 sub-CPIs to form the prediction of total CPI with the decomposition formula in Step2.In this way, the whole process of the prediction by the dividing-integration model is accomplished.3.2. The construction of the GM(1,1) modelThe process of GM (1, 1) model is represented in the following steps:Step1: The original sequence:Step2: Estimate the parameters a and u using the ordinary least square (OLS). Step3: Solve equation as follows.Step4: Test the model using the variance ratio and small error possibility.The construction of the ARIMA modelFirstly, ADF unit root test is used to test the stationarity of the time series. If the initial time series is not stationary, a differencing transformation of the data is necessary to make it stationary. Then the values of p and q are determined by observing the autocorrelation graph, partial correlation graph and the R-squared value.After the model is built, additional judge should be done to guarantee that the residual error is white noise through hypothesis testing. Finally the model is used to forecast the future trend ofthe variable.The construction of the BPNN modelThe first thing is to decide the basic structure of BP neural network. After experiments, we consider 3 input nodes and 1 output nodes to be the best for the BPNN model. This means we use the CPI data of time , ,toforecast the CPI of time .The hidden layer level and the number of hidden neurons should also be defined. Since the single-hidden- layer BPNN are very good at non-liner mapping, the model is adopted in this paper. Based on the Kolmogorov theorem and testing results, we define 5 to be the best number of hidden neurons. Thus the 3-5-1 BPNN structure is determined.As for transferring function and training algorithm, we select ‘tansig’as the transferring function for middle layer, ‘logsig’for input layer and ‘traingd’as training algorithm. The selection is based on the actual performance of these functions, as there are no existing standards to decide which ones are definitely better than others.Eventually, we decide the training times to be 35000 and the goal or the acceptable error to be 0.01.4.Empirical AnalysisCPI data from Jan. 2012 to Mar. 2013 are used to build the three models and the data from Apr. 2013 to Sept. 2013 are used to test the accuracy and stability of these models. What’s more, the MAPE is adopted to evaluate the performance of models. The MAPE is calculated by the equation:Data sourceAn appropriate empirical analysis based on the above discussion can be performed using suitably disaggregated data. We collect the monthly data of sub-CPIs from the website of National Bureau of Statistics of China(/doc/d62de4b46d175f0e7cd184254b35eefdc9d31514.html /).Particularly, sub-CPI data from Jan. 2012 to Mar. 2013 are used to build the three models and the data from Apr. 2013 to Sept. 2013 are used to test the accuracy and stability of these models.Experimental resultsWe use MATLAB to build the GM (1,1) model and the BPNN model, and Eviews 6.0 to build the ARIMA model. The relative predicting errors of sub-CPIs are shown in Table 2.Table 2.Error of Sub-CPIs of the 3 ModelsFrom the table above, we find that the performance of different models varies a lot, because the characteristic of the sub-CPIs are different. Some sub-CPIs like the Food CPI changes drastically with time while some do not have much fluctuation, like the Clothing CPI. We use different models to predict the sub- CPIs and combine them by equation 7.Where Y refers to the predicted rate of the total CPI, is the weight of the sub-CPI which has already been shown in Table1and is the predicted value of the sub-CPI which has the minimum error among the three models mentioned above. The model chosen will be demonstrated in Table 3:Table 3.The model used to forecastAfter calculating, the error of the total CPI forecasting by the dividing-integration model is 0.0034.5.Model Improvement & Error AdjustmentAs we can see from Table 3, the prediction errors of sub-CPIs are mostly below 0.004 except for two sub- CPIs: Food CPI whose error reaches 0.0059 and Transportation & Communication CPI 0.0047.In order to further improve our forecasting results, we modify the prediction errors of the two aforementioned sub-CPIs by adopting other forecasting methods or models to predict them. The specific methods are as follows.Error adjustment of food CPIIn previous prediction, we predict the Food CPI using the BPNN model directly. However, the BPNN model is not sensitive enough to investigate the variation in the values of the data. For instance, although the Food CPI varies a lot from month to month, the forecasting values of it are nearly all around 103.5, which fails to make meaningful prediction.We ascribe this problem to the feature of the training data. As we can see from the original sub-CPI data on the website of National Bureau of Statistics of China, nearly all values of sub-CPIs are around 100. As for Food CPI, although it does have more absolute variations than others, its changes are still very small relative to the large magnitude of the data (100). Thus it will be more difficult for the BPNN model to detect the rules of variations in training data and the forecastingresults are marred.Therefore, we use the first-order difference series of Food CPI instead of the original series to magnify the relative variation of the series forecasted by the BPNN. The training data and testing data are the same as that in previous prediction. The parameters and functions of BPNN are automatically decided by the software, SPSS.We make 100 tests and find the average forecasting error of Food CPI by this method is 0.0028. The part of the forecasting errors in our tests is shown as follows in Table 4:Table 4.The forecasting errors in BPNN testError adjustment of transportation &communication CPIWe use the Moving Average (MA) model to make new prediction of the Transportation and Communication CPI because the curve of the series is quite smooth with only a few fluctuations. We have the following equation(s):where X1, X2…Xn is the time series of the Transportation and Communication CPI, is the value of moving average at time t, is a free parameter which should be decided through experiment.To get the optimal model, we range the value of from 0 to 1. Finally we find that when the value of a is 0.95, the forecasting error is the smallest, which is 0.0039.The predicting outcomes are shown as follows in Table5:Table 5.The Predicting Outcomes of MA modelAdvanced results after adjustment to the modelsAfter making some adjustment to our previous model, we obtain the advanced results as follows in Table 6: Table 6.The model used to forecast and the Relative ErrorAfter calculating, the error of the total CPI forecasting by the dividing-integration model is 0.2359.6.Further DiscussionTo validate the dividing-integration model proposed in this paper, we compare the results of our model with the forecasting results of models that do not adopt the dividing-integration method. For instance, we use the ARIMA model, the GM (1, 1) model, the SARIMA model, the BRF neural network (BRFNN) model, the Verhulst model and the Vector Autoregression (VAR) model respectively to forecast the total CPI directly without the process of decomposition and integration. The forecasting results are shown as follows in Table7.From Table 7, we come to the conclusion that the introduction of dividing-integration method enhances the accuracy of prediction to a great extent. The results of model comparison indicate that the proposed method is not only novel but also valid and effective.The strengths of the proposed forecasting model are obvious. Every sub-CPI time series have different fluctuation characteristics. Some are relatively volatile and have sharp fluctuations such as the Food CPI while others are relatively gentle and quiet such as the Clothing CPI. As a result, by dividing the total CPI into several sub-CPIs, we are able to make use of the characteristics of each sub-CPI series and choose the best forecasting model among several models for every sub-CPI’s prediction. Moreover, the overall prediction error is provided in the following formula:where TE refers to the overall prediction error of the total CPI, is the weight of the sub-CPI shown in table 1 and is the forecasting error of corresponding sub-CPI.In conclusion, the dividing-integration model aims at minimizing the overall prediction errors by minimizing the forecasting errors of sub-CPIs.7.Conclusions and future workThis paper creatively transforms the forecasting of national CPI into the forecasting of 8 sub-CPIs. In the prediction of 8 sub-CPIs, we adopt three widely used models: the GM (1, 1) model, the ARIMA model and the BPNN model. Thus we can obtain the best forecasting results for each sub-CPI. Furthermore, we make special improvement by adjusting the forecasting methods of sub-CPIs whose predicting results are not satisfying enough and get the advanced predicting results of them. Finally, the advanced predicting results of the 8 sub- CPIs are integrated to formthe forecasting results of the total CPI.Furthermore, the proposed method also has several weaknesses and needs improving. Firstly, The proposed model only uses the information of the CPI time series itself. If the model can make use of other information such as the information provided by factors which make great impact on the fluctuation of sub-CPIs, we have every reason to believe that the accuracy and stability of the model can be enhanced. For instance, the price of pork is a major factor in shaping the Food CPI. If this factor is taken into consideration in the prediction of Food CPI, the forecasting results will probably be improved to a great extent. Second, since these models forecast the future by looking at the past, they are not able to sense the sudden or recent change of the environment. So if the model can take web news or quick public reactions with account, it will react much faster to sudden incidence and affairs. Finally, the performance of sub-CPIs prediction can be higher. In this paper we use GM (1, 1), ARIMA and BPNN to forecast sub-CPIs. Some new method for prediction can be used. For instance, besides BPNN, there are other neural networks like genetic algorithm neural network (GANN) and wavelet neural network (WNN), which might have better performance in prediction of sub-CPIs. Other methods such as the VAR model and the SARIMA model should also be taken into consideration so as to enhance the accuracy of prediction.References1.Wang W, Wang T, and Shi Y. Factor analysis on consumer price index rising in China from 2005 to 2008. Management and service science 2009; p. 1-4.2.Qin F, Ma T, and Wang J. The CPI forecast based on GA-SVM. Information networking and automation 2010; p. 142-147.3.George EPB, Gwilym MJ, and Gregory CR. Time series analysis: forecasting and control. 4th ed. Canada: Wiley; 20084.Weng D. The consumer price index forecast based on ARIMA model. WASE International conferenceon information engineering 2010;p. 307-310.5.Jian L, Zhao Y, Zhu YP, Zhang MB, Bertolatti D. An application of ARIMA model to predict submicron particle concentrations from meteorological factors at a busy roadside in Hangzhou, China. Science of total enviroment2012;426:336-345.6.Priya N, Ashoke B, Sumana S, Kamna S. Trend analysis and ARIMA modelling of pre-monsoon rainfall data forwestern India. Comptesrendus geoscience 2013;345:22-27.7.Hwang HB. Insights into neural-network forecasting of time seriescorresponding to ARMA(p; q) structures. Omega2001;29:273-289./doc/d62de4b46d175f0e7cd184254b35eefdc9d31514.html am A. Using a neural network to forecast inflation. Industrial management & data systems 1999;7:296-301.9.Min X, Wong WK. A seasonal discrete grey forecasting model for fashion retailing. Knowledge based systems 2014;57:119-126.11. Weimin M, Xiaoxi Z, Miaomiao W. Forecasting iron ore import and consumption of China using grey model optimized by particleswarm optimization algorithm. Resources policy 2013;38:613-620.12. Zhen D, and Feng S. A novel DGM (1, 1) model for consumer price index forecasting. Greysystems and intelligent services (GSIS)2009; p. 303-307.13. Yu W, and Xu D. Prediction and analysis of Chinese CPI based on RBF neural network. Information technology and applications2009;3:530-533.14. Zhang GP. Time series forecasting using a hybrid ARIMA and neural network model. Neurocomputing 2003;50:159-175.15. Pai PF, Lin CS. A hybrid ARIMA and support vector machines model in stock price forecasting. Omega 2005;33(6):497-505.16. Tseng FM, Yu HC, Tzeng GH. Combining neural network model with seasonal time series ARIMA model. Technological forecastingand social change 2002;69(1):71-87.17.Cho MY, Hwang JC, Chen CS. Customer short term load forecasting by using ARIMA transfer function model. Energy management and power delivery, proceedings of EMPD'95. 1995 international conference on IEEE, 1995;1:317-322.译⽂:⼀种基于ARIMA、灰⾊模型和BPNN对CPI(消费物价指数)进⾏预测的新型分治模型摘要:在本⽂中,利⽤我国现有的消费者价格指数(CPI)的计算⽅法,提出了⼀种新的CPI预测分治模型。
乘势而上中国经济迈向新发展阶段
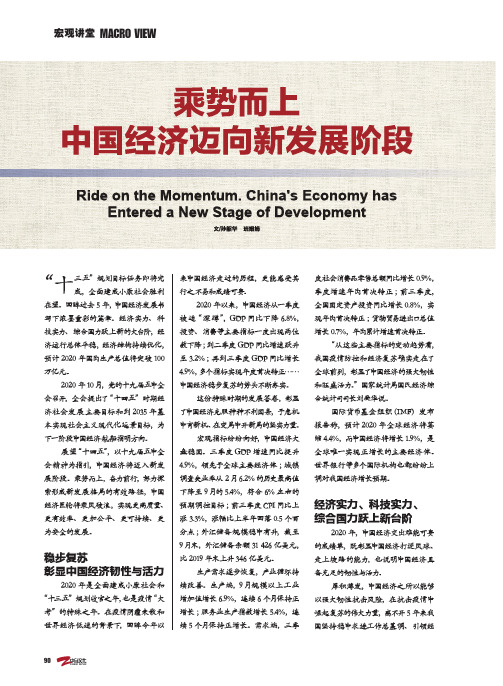
眼”落成启用,北斗导航系统正式开通,
“随着外贸红利、人口红利和房
5G 网络加速成型……创新型国家建设
成果丰硕,研发经费投入总量居世界第
二,通过《专利合作条约》(PCT)提
交国际专利申请量跃居世界第一,载人
航天、探月工程、超级计算、量子通
信等领域取得一大批重大科技成果。
5 年间,聚焦高质量发展的战略
目标,现代化经济体系加快布局,全
生产需求逐步恢复,产业循环持 续改善。生产端,9 月规 模以上工业 增加值增长 6.9%,连续 6 个月保持正 增长 ;服务业生产指数增长 5.4%,连 续 5 个月保持正增长。需求端,三季
度社会消费品零售总额同比增长 0.9%, 季度增速年内首次转正 ;前三季度, 全国固定资产投资同比增长 0.8%,实 现年内首次转正 ;货物贸易进出口总值 增长 0.7%,年内累计增速首次转正。
经济实力、科技实力、 综合国力跃上新台阶
2020 年,中国经济交出难能可贵 的成绩单,既彰显中国经济打逆风球、 走上坡路的能力,也说明中国经济具 备充足的韧性与活力。
厚积薄发,中国经济之所以能够 以强大韧性抗击风险,在抗击疫情中 崛起复苏的伟大力量,离不开 5 年来我 国坚持稳中求进工作总基调、引领经
构建新发展格局 高质量发展迈向新阶段
100 万亿元。人均国内生产总值突破 1
党的十九届五中全会强调,确保
万美元,标志着我国向高收入国家水 如期打赢脱贫攻坚战,确保如期全面
平又迈出坚实一步。
建成小康社会、实现第一个百年奋斗
产业结构优化升级不断提速,制 目标,为开启全面建设社会主义现代
造业增加值占全球份额近 30%,连续 化国家新征程奠定坚实基础。
这份特殊时期的发展答卷,彰显 了中国经济克服种种不利因素,于危机 中育新机、在变局中开新局的坚实力量。
中国CPI指数的时间序列分析
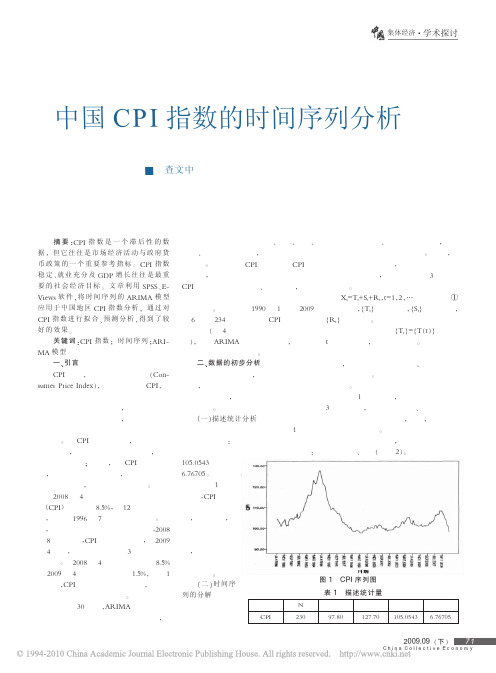
Xt=Tt+St+Rt,t=1 ,2 ,… ① 其 中 , {Tt} 是 趋 势 项 , {St} 是 季 节 项 , {Rt} 是随机项 。
通 常 认 为 趋 势 项 {Tt}={T (t )} 是 时 间
CPI 指 数 进 行 拟 合 、 预 测 分 析 , 得 到 了 较
好的效果。 关 键 词 :CPI 指 数 ; 时 间 序 列 ;ARI-
自相关 。 自 相关程 度 由 自 相 关 系 数 γk 度 量 , 表示时间序列中相隔 k 期 的观测值 之
xt, 在给 定 xt-1,xt-2, …,xt-k+1 的 条 件 下 ,xt 与 xt-k 之间的条件相关关系 。 其相关程度 用偏自相关系数 覬kk 度量 , 有 -1≤覬kk≤1 。
内外的学者将其运用于经济 、 旅游 、 能源 、 医 学 、环 境 等 许 多 领 域 ,出 现 了 一 批 较 好 的 成 果 。 以 每 月 的 CPI 指 数 构 成 CPI 指 数序列, 运用时间序列的建模方法对该
都 表 现 出 趋 势 性 、季 节 性 和 随 机 性 ,或 者 只表现出三者中的其二或 其一 。 这样 , 可 以认为每个时间序列 , 或经过适当 的函数 变换的时间序列 , 都可以分解 成 3 个部分 的叠加 。
CPI N 230
2008 年 4 月 份 中 国 居 民 消 费 价 格 指 数 (CPI)同比上涨 8.5% , 为 12 年以来的新 高 ,超过了 1996 年 7 月份创下的高点 。 然 而 ,由于受全球性的金融危机的影响 ,2008 年 8 月份开始 ,CPI 指数一路下滑 ,到 2009 年 4 月份 , 更是出现了连续 3 个月的同比 负增长 。 从 2008 年 4 月份的同比上涨 8.5% 到 2009 年 4 月份的同比下降 1.5%, 短短 1 年时间 ,CPI 指数如此大幅的波动 , 引发了
经济部统计英文
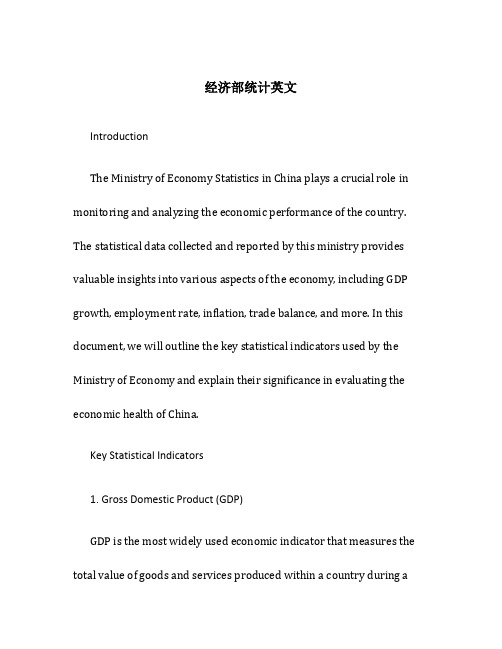
经济部统计英文IntroductionThe Ministry of Economy Statistics in China plays a crucial role in monitoring and analyzing the economic performance of the country. The statistical data collected and reported by this ministry provides valuable insights into various aspects of the economy, including GDP growth, employment rate, inflation, trade balance, and more. In this document, we will outline the key statistical indicators used by the Ministry of Economy and explain their significance in evaluating the economic health of China.Key Statistical Indicators1. Gross Domestic Product (GDP)GDP is the most widely used economic indicator that measures the total value of goods and services produced within a country during aspecific period, usually a year. It is considered a barometer of economic health and reflects the overall economic performance of a nation. The Ministry of Economy tracks and reports China’s GDP growth rate, which indicates the pace of economic development.2. Consumer Price Index (CPI)The CPI measures the average price change of a basket of goods and services commonly consumed by households. It is used to monitor inflationary trends and assess changes in purchasing power. The Minist ry of Economy calculates and publishes China’s CPI on a monthly basis, enabling policymakers and economists to make informed decisions to stabilize prices and control inflation.3. Industrial Production Index (IPI)The IPI reflects the output and performance of the industrial sector, indicating the overall level of productive activities. It measures the volume of manufactured goods, mining output, and electricitygeneration. The Ministry of Economy compiles and releases China’s IPI, providing valuable insights into the performance and growth of the manufacturing and mining sectors.4. Employment RateThe employment rate measures the percentage of the working-age population that is actively employed. It is a crucial indicator of economic health, reflecting the level of job creation and labor market conditions. The Ministry of Economy tracks the employment rate in China, helping policymakers to assess the effectiveness of employment policies and determine job market trends.5. Trade BalanceThe trade balance re flects the difference between a country’s exports and imports. A positive trade balance indicates an excess of exports, while a negative trade balance indicates an excess of imports. It is an important indicator of a country’s competitiveness in the globalmarket. The Ministry of Economy collects and publishes data on China’s trade balance, allowing policymakers to monitor international trade and formulate appropriate trade policies.ConclusionThe statistical indicators provided by the Ministry of Economy play a critical role in evaluating and monitoring China’s economic performance. These indicators, including GDP growth rate, CPI, IPI, employment rate, and trade balance, collectively provide a comprehensive understanding of the economy’s health. Policymak ers, economists, and analysts rely on these indicators to make informed decisions and formulate appropriate policies to ensure sustainable economic growth and stability.。
CPI编制中涉及的几个指数方法的比较
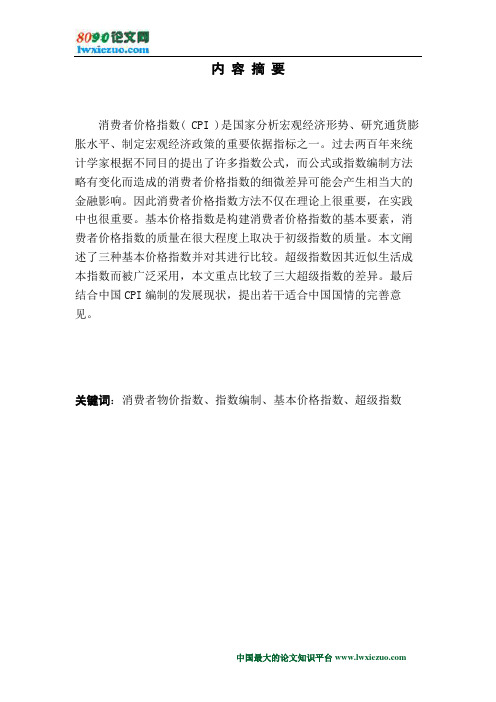
内容摘要消费者价格指数( CPI )是国家分析宏观经济形势、研究通货膨胀水平、制定宏观经济政策的重要依据指标之一。
过去两百年来统计学家根据不同目的提出了许多指数公式,而公式或指数编制方法略有变化而造成的消费者价格指数的细微差异可能会产生相当大的金融影响。
因此消费者价格指数方法不仅在理论上很重要,在实践中也很重要。
基本价格指数是构建消费者价格指数的基本要素,消费者价格指数的质量在很大程度上取决于初级指数的质量。
本文阐述了三种基本价格指数并对其进行比较。
超级指数因其近似生活成本指数而被广泛采用,本文重点比较了三大超级指数的差异。
最后结合中国CPI编制的发展现状,提出若干适合中国国情的完善意见。
关键词:消费者物价指数、指数编制、基本价格指数、超级指数ABSTRACTConsumer price index (CPI) is an important basis for nation to analysis the macroeconomic situation , to investigate the level of inflation, and to the formulate macroeconomic policy. It's also significant for macroeconomic analysis and forecasting to use the scientific and rational way for the CPI compilation. Statisticians over the past two hundred years put forward a number of index for different purposes A slight change of the formula or methodology which causes the nuances of the consumer price index may have a significant financial impact. Therefore, the consumer price index method is very important not only in theory, but also in practice. Elementary price index is the basic elements of the consumer price index, the quality of consumer price index depends largely on the quality of the primary index. This paper describes three basic price index and compare the differences between them. Superlative index is widely used because it approximates the cost of living index, this article compares the key differences between the three Superlative index. Finally, combined with the current situation of China's CPI compilation, a few suggestions to improve it is proposed.KEYWORDS:CPI, Price index, Elementary price index,Superlative index number正文目录第一章引言 (1)第二章 CPI的概念和测定目的 (2)第一节 CPI的概念和统计意义 (2)第二节居民消费价格指数的测定目的 (2)第三章消费者价格指数的分类 (3)第一节一般情形下的指数类型 (3)第二节在季节性产品问题上所涉及到的相关指数 (6)第四章几类基本价格指数的比较 (8)第一节几大角度比较基本价格指数 (8)第二节基本价格指数比较小结 (9)第五章三种超级指数的比较 (11)第一节超级指数的定义 (11)第二节三大超级指数的比较 (11)第六章关于我国CPI编制指数方法的若干思考 (16)第一节我国CPI编制发展现状 (16)第二节对在中国CPI编制中应用的各项指数的几点思考 (17)小结 (20)参考文献 (21)致谢................................. 错误!未定义书签。
英文报纸

English snackShould the goal of college education be to ensure that the students find agood job or should it aim at cultivating the students’ overall abilities? What should be the purpose of college education? No doubt, different people, or even different universities, may see it quite differently. As to my teammates, they also have different opinions.China's consumer price index (CPI), a main gauge of inflation, grew 2 percent year on year in November, the National Bureau of Statistics announced Sunday.The inflation rate rose from a 33-month low of 1.7 percent in October as food prices increased. On a month-on-month basis, November's CPI rose 0.1 percent from the previous month, according to a statement posted on the website of the NBS. "The November growth rate was largely attributable to a surge in food costs," said Wang Jun, an expert with the China Center for International Economic Exchanges. Food prices, which account for nearly one-third of the weighting in the calculation of China's CPI, rose 3 percent in November from one year earlier, pushing the index up 0.95 percentage points. Vegetable prices jumped 11.3 percent year on year in November as cold weather disrupted supplies, pushing the CPI up 0.27 percentage points. Prices for aquatic products gained 4.9 percent from one year earlier, according to the statement. Excluding food prices, China's CPI edged up 1.6 percent. Wang forecast that China's economy will expand by 7.8 percent in 2012 and that the CPI will reach around 2.8 percent for the entire year, well below the government's target of keeping inflation under 4 percent.College educationForth issue – Dec. 2012-----Zhao Fangfang There are two wonderful inventions which were created by accident. In essencial, those ideas occurred to them when they were in search of way to solve or perfect them. That is to say, scientists created new stuff so as to make life more convenient. It’s more practical and meaningful for us that scientific researches are for using. They shorten our journey; they also widen the extent which we can hardly get before; they also make our mind broader. Furthermore, we benefit from improving speed, shorten time and improving efficiency,. we can turn our dream into reality with those scientific researches of using.However, there is one thing to say, there are several scientists who do science experiments just for wonder, they are not afraid of threat, misunderstanding, even death. I honor this spirit which spur them on doing science work for wonder. They are so insistent so as to forget about themselves.In conclusion, I think it is the two approaches to scientific research that make science work booming.School Culture----Ma Jiao School culture is a kind of group culture, which is based on the students, developing in school, and popularizing the essence of school.The free time activity of the students as the main content of school culture, are appear as the form of group, such as poem club, chess club, reading club, music club and so on. The school culture also includes the desire of school building and beautifies the environmentof school. On the other hand, it also pays attention to spirit of students.A healthy school culture can help the students to developing their emotion and some other skills.Learn from movie-----Li JingDo you like watching movies? Have you ever thought about a question when you watch movies, what a movie want to tell us?Maybe someone would ask me, what can a movie tell? But I want to tell you something I learnt from a movie, Black Swan. It talks about an examination of obsession. Obsession in striving for beauty, acceptance, and most of all perfection. It explores the dichotomy involved in both loving and hating yourself. I want to say, there are many people serious about success. Everyone wants to be perfect, but we should achieve it in a more realistic way.In my opinion, movies are the stories we have no chance to experience. So, let’s learn to watch movies, not only by eyes, but also by hearts.What is Mean to Human?----Ma Jiao The most important to be a human is being honest. Nowadays, people are troubled with mass of dishonest behaviors in society. Honest is the pillar of a society, people will benefit from being honest in the long run.And the next, is to be a person with the quality. In a family, to be the quality is a goal to train up children, and it will become more and more important.The third one is to be akind-hearted person, most people are wanting in loving heart, to be a kind-hearted person, you can help many people, and you will get more help from others.Do You Fear the WindHamilton GarlandDo you fear the force of the wind?The slash of the rain?Go face them and fight them,Be savage again.Go hungry and cold like the wolf,Go wade like the crane:The palms of your hands will thicken, The skin of your cheeks will tan,You’ll grow ragged and weary and swarthy,But you’ll walk like a man!There are somebook reports and let us share them!Sons and Lovers----Liang Xiuxiu Gertrude Morel is a woman of high principles, of character and refinement. Her husband is a miner who can barely read and write. Marrying him for passion,she is bitterly disappointed by his rough mamers, his drinking, his drinking, what she can only see as his withdrawn from Morel is beingredirected toward toward the first of her children. Such is the world into which Paul Morel is born.The Cloud of Hometown----Wang Hailing Xinjiang is a good place in which there are not only friendly people but also delicious food. And there is the most graceful scenery. Have you ever seen limpid sky? If you can, welcome to my hometown Xinjiang, and you could enjoy the most clean cloud. No matter how your mood is like, even though you are so depressed, if you raise your head, you will find that there is so calm. The white cloud looks warm like mom's hug. The light cloud fluttering in the sky make you feel peaceful. I love this ground deeply, especially the cloud of hometown from I was born. The cloud of hometown has been my favorite landscape.Is It necessary?----Li JingI ever feel confused about learning some subjects have no business with our major deeply.And now, I’m still confused. Why do I spend my time learning it? I don’t think we will not be human being without those kinds of education training. If we can pay enough attention to our daily life, we will learn something by ourselves. But it doesn’t mean we needn’t learn from the past, we should respect the past. In my opinion, we needn’t learn it deeply. The competition of employment requires us to specialize in our major well. So, we need more time and more energy in our major to see that at least we can support ourselves well.In a word, I think we should learn something as common sense, but I do think we needn’t spent so much time learning it as we do now.The First Snow in2012----Zhang Junru When we opened eyes, we were all surprised-----the whole world turned to white. It was the first snow in 2012.We can use heavy to describe the snow, many trees were bent because of the snow, they just like fireworks. The houses, trees cars and roads all wore whitecoats. Many people especiallygirlsshowedsurprised about the snow. We gradually smelt the winter’s smell.The first snow in 2012 was a gift for us, we all enjoyed it.Japan, Please Respect the Truth ----Zhang JunruAs we all know, we have a fierce dispute with Japan about DiaoYu Island. I want to say, no matter what country you are, you must respect the truth.As a big population country, China always respect other countries. We are eager to share resources with Japan, but Japan has wild heart, he wants to posses DiaoYu Island, which shows it doesn’t respect the truth.We all learn history, we know that China is the Japan’s teacher from Tang, but what Japan gives us? It is a question deserved to think.That DiaoYu Island belongs to us is the truth, so Japan should respect it instead of doing such ridiculous things.【That's the end 】。
统计表英语缩写
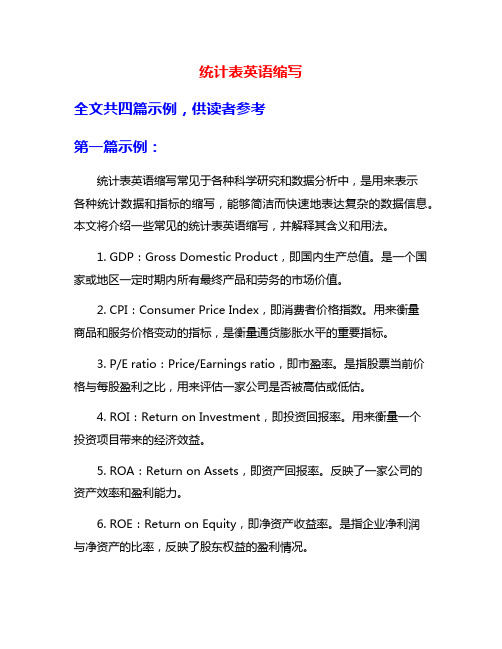
统计表英语缩写全文共四篇示例,供读者参考第一篇示例:统计表英语缩写常见于各种科学研究和数据分析中,是用来表示各种统计数据和指标的缩写,能够简洁而快速地表达复杂的数据信息。
本文将介绍一些常见的统计表英语缩写,并解释其含义和用法。
1. GDP:Gross Domestic Product,即国内生产总值。
是一个国家或地区一定时期内所有最终产品和劳务的市场价值。
2. CPI:Consumer Price Index,即消费者价格指数。
用来衡量商品和服务价格变动的指标,是衡量通货膨胀水平的重要指标。
3. P/E ratio:Price/Earnings ratio,即市盈率。
是指股票当前价格与每股盈利之比,用来评估一家公司是否被高估或低估。
4. ROI:Return on Investment,即投资回报率。
用来衡量一个投资项目带来的经济效益。
5. ROA:Return on Assets,即资产回报率。
反映了一家公司的资产效率和盈利能力。
6. ROE:Return on Equity,即净资产收益率。
是指企业净利润与净资产的比率,反映了股东权益的盈利情况。
7. NPV:Net Present Value,即净现值。
用来评估一个投资项目在折现率下的净现值,是决定是否进行投资的重要指标。
9. MRR:Monthly Recurring Revenue,即月度重复收入。
是一项企业的可预测性收入,通常用于评估企业的稳定性。
10. CAC:Customer Acquisition Cost,即客户获取成本。
用来衡量一个企业获取新客户所需的成本。
以上是一些常见的统计表英语缩写,可以帮助我们更方便地理解和分析各种数据信息。
在实际工作和研究中,了解这些缩写的含义和用法将更有利于我们完善数据分析和决策过程。
希望以上内容对您有所帮助!第二篇示例:统计表在日常生活和工作中经常用到,它是一种用数字和图表展示数据和信息的形式。