Multivariate Analysis ch5
多元方差分析
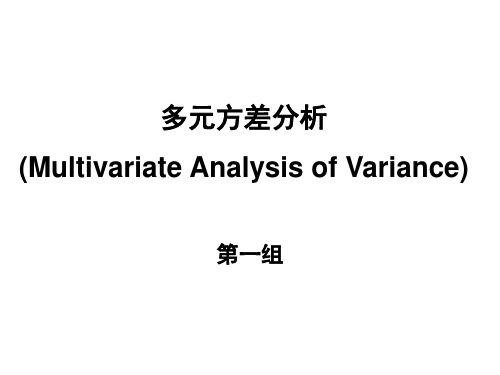
u22
H0: = ... = … = … =
up1
up2
பைடு நூலகம்
或H0:u1=u2=…=un
Ha: u1,u2,…,un不全相等
u1n u2n …
upn
MANOVA原理讲解
检验统计量的计算
单因子多元方差分析:
SSCPT= SH+SE 来源
df
自由度
SSCP ……
组间
k1
H
威尔克斯统 计量
组内
Nk
E
总和
Ab1
Aj1
Ag1
2
Ah2
Ab2
Aj2
Ag2
…
…
…
…
…
10
Ah10
Ab10
Aj10
Ag10
One-way MANOVA原始数据
N=n1+n2+…+ng p: 响应变量个数
One-way MANOVA举例
来自黑龙江、北京、江苏、广东4省的芦苇在光 合效率(A),叶片长度(B),开花时间(C)上有无显 著差异,每地各量测10株。
(Yij yi)2
i1 j1
SStreat
= 34
g
=
ni(yi
i1
y)2
= 160
MANOVA的SSCP计算示例
处理
观测值
高 Y1 5 3 Y2 4 6
中 Y1 1 1 Y2 3 1
低 Y1 6 5 Y2 5 9
样本 总均 均值 值向 向量 量
44 55
14 25
76 77
备选方法: 1 对各因变量分别进行单因素方差分析. 2 用Bonferroni修正的两两比较.
CHA_(2)DS_(2)-VASc评分及中性粒细胞淋巴细胞比值对介入术后对比剂肾病预测价值
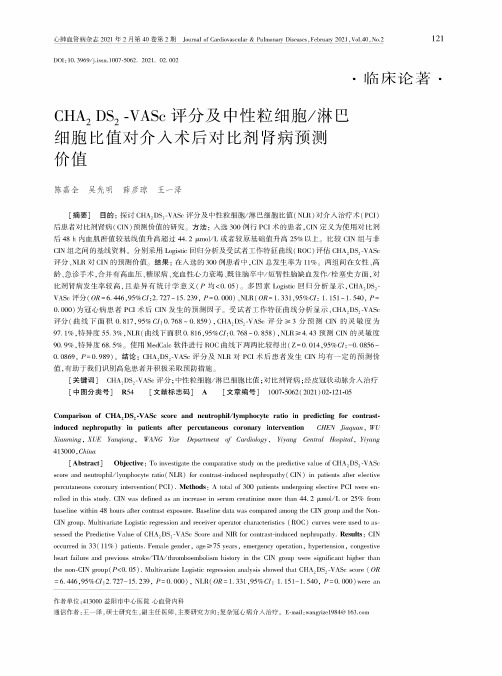
DOI:10.3969/j.issn.l007-5062.2021.02.002•临床论著•CHA2DS2-VASc评分及中性粒细胞/淋巴细胞比值对介入术后对比剂肾病预测价值陈嘉全吴先明薛彦琼王一泽[摘要]目的:探讨CHA2DS2-VASc评分及中性粒细胞/淋巴细胞比值(NLR)对介入治疗术(PCI)后患者对比剂肾病(CIN)预测价值的研究。
方法:入选300例行PCI术的患者,CIN定义为使用对比剂后48h内血肌酐值较基线值升高超过44.2zmoVL或者较原基础值升高25%以上。
比较CIN组与非CIN组之间的基线资料。
分别采用Logistic回归分析及受试者工作特征曲线(ROC)评估CHA2DS2-VASc评分、NLR对CIN的预测价值。
结果:在入选的300例患者中,CIN总发生率为11%。
两组间在女性、高龄、急诊手术,合并有高血压、糖尿病、充血性心力衰竭、既往脑卒中/短暂性脑缺血发作/栓塞史方面,对比剂肾病发生率较高,且差异有统计学意义(P均<0.05)。
多因素Logistic回归分析显示,CHA DS2-VASc评分(OR=6.446,95%C/:2.727~15.239,P=0.000)、NLR(OR=1.331,95%CI: 1.151~1.540,P=0.000)为冠心病患者PCI术后CIN发生的预测因子。
受试者工作特征曲线分析显示,CHA DS2-VASc评分(曲线下面积0.817,95%CI:0.768-0.859),CHA2DS2-VASc评分M3分预测CIN的灵敏度为97.1%,特异度55.3%,NLR(曲线下面积0.816,95%CI:0.768-0.858),NLRM4.43预测CIN的灵敏度90.9%,特异度68.5%。
使用MedCalc软件进行ROC曲线下两两比较得岀(Z=0.014,95%CI:-0.0856~0.0869,P=0.989)。
结论:CHA2DS2-VASc评分及NLR对PCI术后患者发生CIN均有一定的预测价值,有助于我们识别高危患者并积极采取预防措施。
壶腹周围癌胰十二指肠切除术后并发症的危险因素分析及其对预后的影响
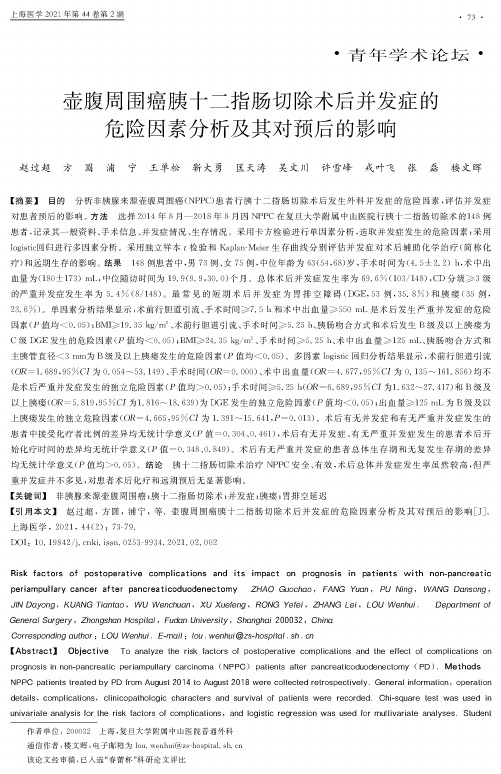
上海医学2021年第44卷第2期・73・・青年学术论坛・壶腹周围癌胰十二指肠切除术后并发症的危险因素分析及其对预后的影响赵过超方圆浦宁王单松靳大勇匡天涛吴文川许雪峰戎叶飞张磊楼文晖【摘要】目的分析非胰腺来源壶腹周围癌(NPPC)患者行胰十二指肠切除术后发生外科并发症的危险因素,评估并发症对患者预后的影响。
方法选择2014年8月—2018年8月因NPPC在复旦大学附属中山医院行胰十二指肠切除术的148例患者,记录其一般资料、手术信息、并发症情况、生存情况。
采用卡方检验进行单因素分析,选取并发症发生的危险因素;采用logistic回归进行多因素分析。
采用独立样本£检验和Kaplan-Meier生存曲线分别评估并发症对术后辅助化学治疗(简称化疗)和远期生存的影响。
结果4例患者中,男73例、女75例冲位年龄为63(54,68)岁,手术时间为简5+2.2)h,术中出血量为(180+173)mL冲位随访时间为19.9(9.9,30.0)个月。
总体术后并发症发生率为69.6%(103/148),CD分级$3级的严重并发症发生率为5.年简/14年。
最常见的短期术后并发症为胃排空障碍(DGEC3例评5.例)和胰痿简5例, 23.6%患单因素分析结果显示,术前行胆道引流、手术时间$7.5例和术中出血量$550mL是术后发生严重并发症的危险因素简值均VO.O5);BMI$9.53kg/rL、术前行胆道引流、手术时间$5.23h、胰肠吻合方式和术后发生B级及以上胰痿为C级DGE发生的危险因素简值均<0.05);BMI$4.35kg/rL、手术时间$5.25h、术中出血量$25mL、胰肠吻合方式和主胰管直径V3mmLB级及以上胰痿发生的危险因素简值均<0.05)。
多因素1/ic回归分析结果显示,术前行胆道引流1=1.6%,评%犆为6.054—55.4年、手术时间1=0.000)、术中出血量1=4.677,评%犆为5.135—167.年例均不是术后严重并发症发生的独立危险因素简值均〉0.05)采术时间$5.25和犗46%,评%犆为1.632〜27.4年)和B级及以上胰发1=5.例,评%(为为4年〜1例3例为DGE发生的独立危险因素简值均<0.05);出血量$25mL为B级及以上胰发发生的独立危险因素14665,评%犆为1.3例〜26%术=45)。
permutation multivariate analysis of variance 解析说明

permutation multivariate analysis of variance 解析说明1. 引言1.1 概述在统计学领域,多元方差分析(Multivariate Analysis of Variance, MANOVA)是一种用于比较两个或多个组之间均值是否具有显著差异的统计方法。
传统的MANOVA假设数据满足正态性、方差齐性和协方差矩阵齐性等假设条件。
然而,当数据不满足这些假设时,传统的MANOVA会失效,因此需要使用其他替代方法。
本文将重点讨论一种替代方法——排列多元方差分析(Permutation Multivariate Analysis of Variance, PERMANOVA)。
PERMANOVA通过基于观察到的样本排列进行总体均值比较,并利用置换检验来评估组间差异是否显著。
相对于传统MANOVA,PERMANOVA在数据分析中更加灵活与适应性强。
1.2 文章结构本文将按照以下结构进行论述:- 第1部分为引言部分,对文章内容进行概述,并介绍排列多元方差分析的背景和意义。
- 第2部分为排列多元方差分析解析部分,主要涵盖其基本概念、方法和步骤以及数学原理的详细说明。
- 第3部分将探讨排列多元方差分析在不同领域中的应用,包括社会科学、医学研究和生态学研究等。
- 第4部分将对排列多元方差分析的优势与局限性进行深入分析,并探讨其结果解释的影响因素。
- 最后,第5部分总结全文,回顾研究内容,并展望排列多元方差分析在未来的发展趋势。
1.3 目的本文旨在全面解析排列多元方差分析(PERMANOVA),从介绍基本概念到详细说明方法与步骤,探讨其数学原理以及重要性。
同时,还将通过案例和实际应用领域来阐述PERMANOVA在社会科学、医学研究和生态学研究等领域中的具体应用。
此外,在总结优势与局限性时,将重点关注其解决传统MANOVA假设条件限制的优势,并分析结果解释受何种因素影响。
最后,展望未来针对PERMANOVA方法改进和发展的可能性。
市场研究中的多元统计分析方法Multivariate
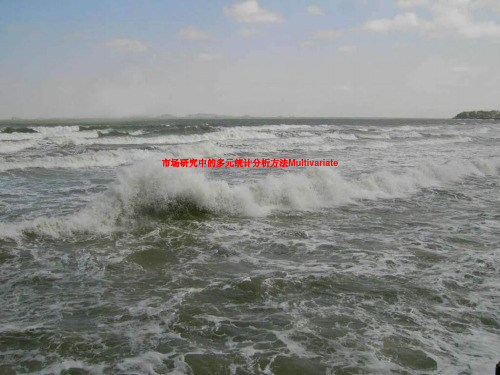
讨论议题
• 我们的研究工作是什么? • 什么是多元统计分析(MVA)? • 为什么我们需要它? • 通常的分析技术 • MVA详细介绍及例子:
– 相关分析(Correspondence analysis) – 回归/多元回归分析(Regression / Multiple
31
Copyright CAE
Hale Waihona Puke 概念MAP(Perceptual Mapping) 的基本方法
• 通过因子分析程式来运行一组数据
– 减少大量的变量(如产品属性)到小规模的基础变 量。这些变量是高度自相关的变量,例如,受访者 的回答模式都非常相似
– 通过因子提取来解释因子变量。高的得分意味着更 加重要的变量已经被因子所包含
努力来显示..
- 那些动物在颜色方面最相似,那些区别最大? - 那些颜色更倾向那类动物 - 那些动物和那些颜色有更强的相关性,那些相关性很弱
17
Copyright CAE
WHITE
MIXED
BLACK BROWN
19
Copyright CAE
WHITE
MIXED
BLACK BROWN
20
Copyright CAE
35
Copyright CAE
多元回归 象线性回归一样只不过有更多
的独立变量
Y = c + b1x1 + b2x2 + b3x3 + ... + e
36
Copyright CAE
多元回归在市场研究中的运用
37
Copyright CAE
关键的驱动因素 - 在上升的咖啡市场
统计分析入门与应用 SPSS 中文版 + SmartPLS 4 中文版说明书
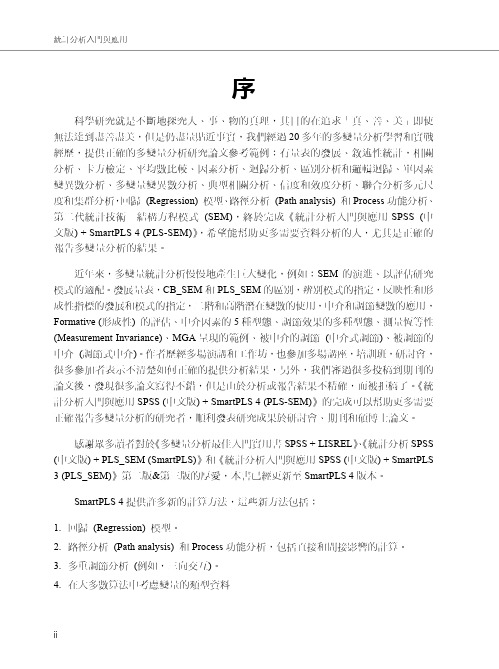
統計分析入門與應用序科學研究就是不斷地探究人、事、物的真理,其目的在追求「真、善、美」即使無法達到盡善盡美,但是仍盡量貼近事實,我們經過20多年的多變量分析學習和實戰經歷,提供正確的多變量分析研究論文參考範例:有量表的發展、敘述性統計,相關分析、卡方檢定、平均數比較、因素分析、迴歸分析、區別分析和邏輯迴歸、單因素變異數分析、多變量變異數分析、典型相關分析、信度和效度分析、聯合分析多元尺度和集群分析,回歸(Regression) 模型、路徑分析(Path analysis) 和Process功能分析、第二代統計技術–結構方程模式(SEM),終於完成《統計分析入門與應用SPSS (中文版) + SmartPLS 4 (PLS-SEM)》,希望能幫助更多需要資料分析的人,尤其是正確的報告多變量分析的結果。
近年來,多變量統計分析慢慢地產生巨大變化,例如:SEM的演進、以評估研究模式的適配。
發展量表,CB_SEM和PLS_SEM的區別,辨別模式的指定,反映性和形成性指標的發展和模式的指定,二階和高階潛在變數的使用,中介和調節變數的應用,Formative (形成性) 的評估、中介因素的5種型態、調節效果的多種型態、測量恆等性(Measurement Invariance)、MGA呈現的範例、被中介的調節(中介式調節)、被調節的中介(調節式中介)。
作者歷經多場演講和工作坊,也參加多場講座,培訓班,研討會,很多參加者表示不清楚如何正確的提供分析結果,另外,我們審過很多投稿到期刊的論文後,發現很多論文寫得不錯,但是由於分析或報告結果不精確,而被拒稿了。
《統計分析入門與應用SPSS (中文版) + SmartPLS 4 (PLS-SEM)》的完成可以幫助更多需要正確報告多變量分析的研究者,順利發表研究成果於研討會、期刊和碩博士論文。
感謝眾多讀者對於《多變量分析最佳入門實用書SPSS + LISREL》、《統計分析SPSS (中文版) + PLS_SEM (SmartPLS)》和《統計分析入門與應用SPSS (中文版) + SmartPLS 3 (PLS_SEM)》第二版&第三版的厚愛,本書已經更新至SmartPLS 4版本。
第17章 多元分析简介
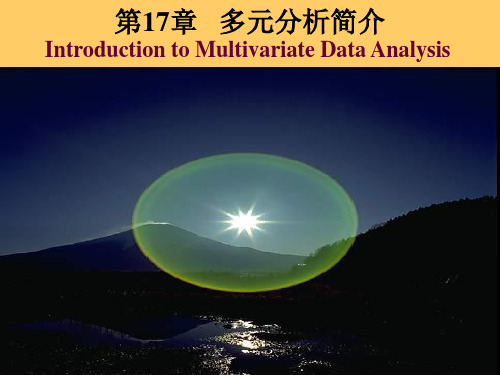
Cluster Analysis 聚类分析
• Cluster Analysis Defined
– Cluster analysis is a procedure for identifying subgroups of individuals or items that are homogeneous within subgroups and different from other subgroups.
Factor Analysis 因子分析
• Factor Analysis Defined
– Factor analysis permits the analyst to reduce a set of variables to a smaller set of factors or composite variables by identifying dimensions under the data.
Discriminant Analysis 判别分析
• Discriminant Analysis Defined 定义
– Multiple discriminant analysis enables the researcher to predict group membership on the basis of two or more independent variables.
Multiple Regression Analysis
• Basic Equation(方程)
Y = a + b1X1 + b2X2 + b3X3 + …+ BnXn
where
Y = dependent or criterion variable X = estimated constant b 1-n = coefficients associated with the predictor variables so that
Multivariate Analysis
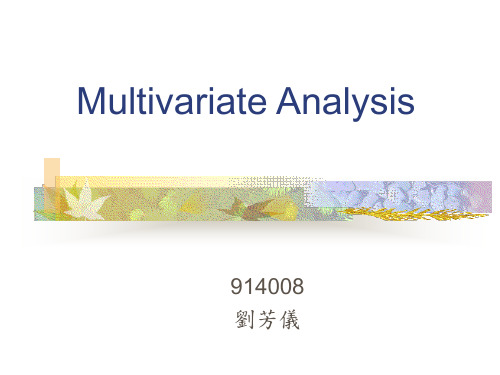
( X 1 1 )( X 2 2 ) ( X 2 2 )2 E( X 2 2 ) 2
( X 1 1 )( X p p ) ( X 2 2 )( X p p ) 2 (X p p ) E ( X 1 1 )( X p p ) E ( X 2 2 )( X p p ) E( X p p ) 2
Var ( Χ )
Postulation:X is linear dependent upon a few unobservable random variables F1,….,Fm, called common factors,and p additional source of variation,called errors.
3 Factor Analysis 因素分析
Introduction
The essential purpose of factor analysis is to describe,if possible, the covariance relationships among many variables in terms of a few underlying,but unobservable,random quantities called factors.
The Factor Analysis Model
X 1 1 11 F1 12 F2 ........ 1m Fm 1 X 2 2 21 F1 22 F2 ........ 2 m Fm 2 X p p p1 F1 p 2 F2 ........ pm Fm p or in matrixnotat ion
多元统计分析推断(英文)

_
60
M2;,
9 4
CONTENTS
பைடு நூலகம்vii
Tests for H0:a'(fi1 — fi2) = a '^o and H0j: tnj ~ Mj = 0, 94 3.6.5. Test for H0: C(/A, - fi2) = 0, 95 3.7. Robustness of the r 2 -test, 96 3.7.1. Robustness to 2 , + X2, 96 3.7.2. Robustness to Nonnormality, 96 3.8. Paired Observation Test, 97 3.9. Testing H0: Mi = M2 When 21 ¥= X2, 99 3.9.1. Univariate Case , 99 3.9.2. Multivariate Case, 100 3.10. Power and Sample Size, 104 3.11. Tests on a Subvector, 108 3.11.1. Two-Sample Case, 108 3.11.2. Step-Down Test, 110 3.11.3. Selectionof Variables, 111 3.11.4. One-Sample Case, 112 3.12. Nonnormal Approaches to Hypothesis Testing, 112 3.12.1. Elliptically Contoured Distributions, 112 3.12.2. Nonparametric Tests, 113 3.12.3. Robust Versions of T2, 114 3.13. Application of T2 In Multivariate Quality Control, 114 3.6.4. 4. Multivariate Analysis of Variance 4.1. One-Way Classification, 121 4.1.1. Model for One-Way Multivariate Analysis of Variance, 121 4.1.2. Wilks' Likelihood Ratio Test, 122 4.1.3. Roy's Union-Intersection Test, 127 4.1.4. The Pillai and Lawley-Hotelling Test Statistics, 130 4.1.5. Summary of the Four Test Statistics, 131 4.1.6. Effect of an Additional Variable on Wilks' A, 132 4.1.7. Tests on Individual Variables, 134 4.2. Power and Robustness Comparisons for the Four MANOVA Test Statistics, 135 4.3. Tests for Equality of Covariance Matrices, 138 4.4. Power and Sample Size for the Four MANOVA Tests, 140 4.5. Contrasts Among Mean Vectors, 142 4.5.1. Univariate Contrasts, 142 4.5.2. Multivariate Contrasts, 145 121
MultivariateAnalysis
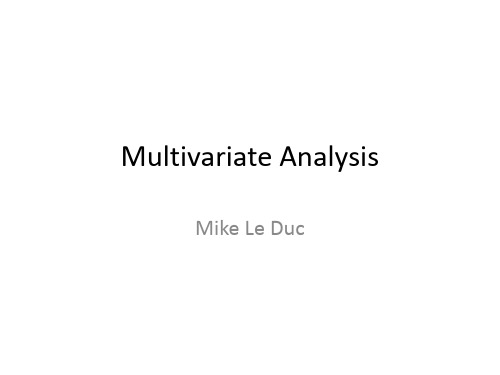
• The cover has been averaged over a number of quadrats to improve the precision of measurement • Our job is to model this response, using a number of fixed and /or random effects, in order to explain the variation found (using, e.g. lme)
Multivariate Analysis
Mike Le Duc
Univariate data
• Example of a univariate data set
> Chr2Paq Site Paqu Blak 0.001 Blck 0.000 Clnn 40.882 Dale 0.069 Lang 2.337 Lowt 0.001 NHl3 0.038 NHl4 0.000 NHRJ 0.917 Poss 20.288 Slte 0.001 Stag 0.013 Whit 0.000 Wnfl 0.039
c jk
n
(Yij Y j )(Y jk Yk )
i 1
n 1
Note, the ‘vector’ and ‘matrix’ shown above are, strictly, dataframes; they have row and column names
Eigenvalues
• They are analogous to covariance
… and Arcsine transformed
> Chr2sppt Acap Blak 0.056 Blck 0.001 Clnn 0.609 Dale 0.001 Lang 0.006 Lowt 0.558 NHl3 0.004 NHl4 0.007 NHRJ 0.001 Poss 0.036 Slte 0.048 Stag 0.008 Whit 0.006 Wnfl 0.000
微分方程的有限差分和谱方法-ch5Dissipation, dispersion, and group velocity

ut = ux : utt = uxx : ut = uxx : ut = iuxx :
!= !2 = 2 i.e., ! = i! = ; 2 ! = ; 2:
(5:1:3) (5:1:4) (5:1:5) (5:1:6)
These relations are plotted in Figure 5.1.1. Notice the double-valuedness of the dispersion relation for utt = uxx, and the dashed curve indicating complex values for ut = uxx. More general solutions to these partial di erential equations can be obtained by superimposing plane waves (5.1.1), so long as each component satis es the dispersion relation the mathematics behind such Fourier synthesis
equations. The dashed curve in (c) is a reminder that ! is complex.
5.1. DISPERSION RELATIONS
TREFETHEN 1994
195
was described in Chapter 2, and examples were given in x3.1. For a PDE of rst order in t, the result is 1 Z 1 ei( x+!( )t)u ( ) d : u(x t) = 2 ^0 (5:1:7) ;1 Since most partial di erential equations of practical importance have variable coe cients, nonlinearity, or boundary conditions, it is rare that this integral representation is exactly applicable, but it may still provide insight into local behavior. Discrete approximations to di erential equations also admit plane wave solutions (5.1.1), at least if the grid is uniform, and so they too have dispersion relations. To begin with, let us discretize in x only so as to obtain a semidiscrete formula. Here are the dispersion relations for the standard centered semidiscretizations of (5.1.3){(5.1.6): 1 ut = 0u : ! = h sin h (5:1:8) 4 utt = u : !2 = h2 sin2 2h (5:1:9) 4 ut = u : i! = ; h2 sin2 2h (5:1:10) 4 (5:1:11) ut = i u : ! = ; h2 sin2 2h : These formulas are obtained by substituting (5.1.1) into the nite di erence formulas with x = xj . In keeping with the results of x2.2, each dispersion relation is 2 =h-periodic in , and it is natural to take 2 ; =h =h] as a fundamental domain. The dispersion relations are plotted in Figure 5.1.2, superimposed upon dotted curves from Figure 5.1.1 for comparison. Stop for a moment to compare the continuous and semidiscrete curves in Figure 5.1.2. In each case the semidiscrete dispersion relation is an accurate approximation when is small, which corresponds to many grid points per wavelength. (The number of points per spatial wavelength for the wave (5.1.1) is 2 = h.) In general, the dispersion relation for a partial di erential equation is a polynomial relation between and !, while a discrete model amounts to a trigonometric approximation. Although other design principles are possible, the standard discrete approximations are chosen so that the trigonometric function matches the polynomial to as high a degree as possible at the origin = ! = 0. To illustrate this idea, Figure 5.1.3 plots dispersion relations for the standard semidiscrete nite di erence approximations to ut = ux and ut = iuxx of orders 2, 4, and 6. The formulas were given in x3.3.
血液透析患者原发性动静脉瘘功能障碍的危险因素分析
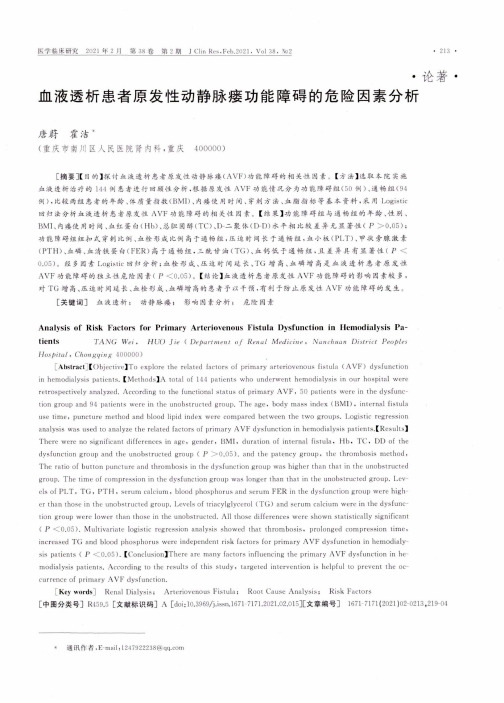
•论著•血液透析患者原发性动静脉瘘功能障碍的危险因素分析唐蔚霍洁*(重庆市南川区人民医院肾内科,重庆400000)[摘要]【目的】探讨血液透析患者原发性动静脉瘘(A V F)功能障碍的相关性因素。
【方法】选取本院实施血液透析治疗的144例患者进行回顾性分析,根据原发性A V F功能情况分为功能障碍组(50例)、通畅组(94例),比较两组患者的年龄、体质量指数(B M I)、内瘘使用时间、穿剌方法、血脂指标等基本资料,采用Logistic回归法分析血液透析患者原发性A V F功能障碍的相关性因素。
【结果】功能障碍组与通畅组的年龄、性别、B M I、内瘘使用时间、血红蛋白(H b)、总胆固醇(T C)、D■二聚体(I>D)水平相比较差异无显著性(P >0.05);功能障碍组纽扣式穿刺比例、血栓形成比例高于通畅组,压迫时间长于通畅组,血小板(P L T)、甲状旁腺激素(P T H)、血磷、血清铁蛋白(F E R)高于通畅组,三酰甘油(T G)、血钙低于通畅组,且差异具有显著性(P <0.05)。
经多因素Logistic回归分析;血栓形成、压迫时间延长、T G增高、血磷增高是血液透析患者原发性A V F功能障碍的独立性危险因素(P <0.05)。
【结论】血液透析患者原发性A V F功能障碍的影响因素较多,对T G增高、压迫时间延长、血栓形成、血磷增高的患者予以干预,有利于防止原发性A V F功能障碍的发生。
[关键词]血液透析;动静脉癌;影响因素分析;危险因素Analysis of Risk Factors for Primary Arteriovenous Fistula Dysfunction in Hemodialysis Patients T A N G W e i»HUO Jie(D epartm ent o f R enal M edicine •,Nanchuan D istrict PeoplesH ospital-, C hongqing 400000)LAbstract]【()bjective】T o explore the related factors of primary arteriovenous fistula (A V F)dysfunctionin hemodialysis patients.【Meth od s】A total of 144 patients w h o underwent hemodialysis in our hospital wereretrospectively analyzed. According to the functional status of primary A V F. 50 patients were in the dysfunction group and 94 patients were in the unobstructed group. T h e age, body m a v s s index (BM1),internal fistulause time, puncture m ethod and blood lipid index were compared between the two groups. Logistic regressionanalysis was used to analyze the related factors of primary AVP' dysfunction in hemodialysis patients.[Results!There were no significant differences in age,gender•B M I.duration of internal fistula,H b,T C,D D of thedysfunction group and the unobstructed group ( P >0.05). and the patency group, the thrombosis method,T h e ratio of button puncture and thrombosis in the dysfunction group was higher than that in the unobstructedgroup. Th e time of compression in the dysfunction group was longer than that in the unobstructed group. L e vels of P L T. T G, P T H. serum calcium, blood phosphorus and serum F E R in the dysfunction group were higher than those in the unobstructed group. Levels of triacylglycerol (T G) and serum calcium were in the dysfunc-tion group were lower than those in the unobstructed. All those differences were s h o w n statistically significant(P <C0.05). Multivariate logistic regression analysis showed that thrombosis, prolonged compression time,increased T G and blood phosphorus were independent risk factors for primary A V F dysfunction in hemodialy-sis patients ( P <0.05).【Conclusion】There are m a n y factors influencing the primary A V F dysfunction in he-modialysis patients. According to the results of this study, targeted intervention i s helpful to prevent the occurrence of primary A VP' dysfunction.[Key words] Renal Dialysis;Arteriovenous Fistula; Root Cause Analysis;Risk Factors[中图分类号]R459.5 [文献标识码]A [doi:10.3969/j.issn_1671-7171.2021.02.015][文章编号]1671-7171(2021)02-0213,219»04通讯作者,E-mail:*****************随着医疗技术的不断发展,血液透析技术也得到了一定的发展。
CH5 多分辨率分析

1 2 k
k
(2) G() ()
^
^
G( )
1
2
k
gk eik
( x) {gk } G( )
目录 上页 下页 返回 结束
Harr小波函数
Harr小波函数
(x) (2x) (2x 1)
Wj
的规范正交基为
j Z
j ,k
| j, k Z , 其中 j ,k ( x ) 2
j
2
(2 j x k )
由 L2 W j 知, L2 的规范正交基为: j ,k | j, k Z 即
f L2 ( R ) f f , j ,k j ,k ( x )
Wj
V
j
V j 1 V j W j 1 V j W j 2 V j 尺度空间 小波空间
j 1
V
W
j 1 J
V W
j2 J 1
W
j2
W
j 1
j 1
.... V J W ...
k j 1
... W
Wk
问题: 找到 W j 的标准正交基?
hk eik
^
^
H ( )
1
2
k
( x) {hk } H ( )
目录 上页 下页 返回 结束
二尺度方程(2)
由 ( x) W0 V1 知, ( x) V1 j 则 ( x) hk1,k ( x) k, j (x) 2 2(2 j x k)
目录
上页
下页
Multi Vari 多变量分析
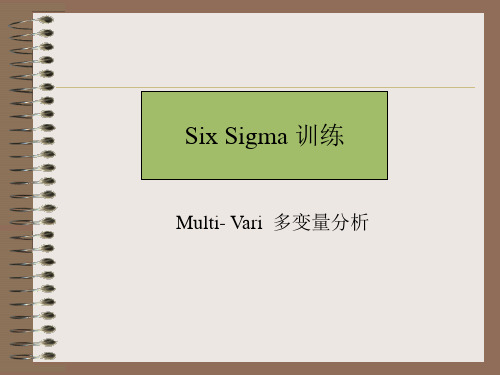
子群分组的黄金定律 (1)包含每一子群组内的变异,所有变异来源对此分析而言是一 般起因或正态流程变异 (2)选取连续的子群组时,特殊起因的变异来源将会发生在子群 组间
组内最小化、组间最大化
Special 及Common Cause 变异
范例:库存盘点的正确性
重要的X变量可能包含: 哪个仓库? 在架子的高层或低层? 何时记录月库存? 谁负责最料; 不论流程产出好的或坏的结果我们都要收集资料
抽样设计(Sampling Design)
• 你可使用一种或多种下列抽样设计 • 抽样设计有助于确保取得流程的代表性样本(representative sample, 并可避免采集大量资料
– Nilson Rating 基于1500个观众 – 这些观众代表全国的收视者
• 抽样设计:
– – – – – Simple Random Sample (简易随机抽样) Stratified Sampling (分层抽样) Cluster Sampling (聚类抽样) Systematic Sampling(系统抽样) Subgroup Sampling(子群组抽样)
您有15分钟完成此练习
选择资料收集方法
• 项目小组应在何时、何处收集资料?
• 我们应收集多少资料?
错误的方法会误导整个项目
为何要抽样?
分析100%流程或母体的资料点是不实际也不可能的
抽样策略通常被两个与变量相关的问题所主导
• 什么变异来源对此分析而言可视为正态、一般或无关? • 什么变异来源对特殊起因而言可视为特殊的或可指定的因素?
Y’s
输出资料
流程基线—我们流程目前状况如何
X’s
输入资料
确认哪个输入变量影响流程输出
多元方差分析
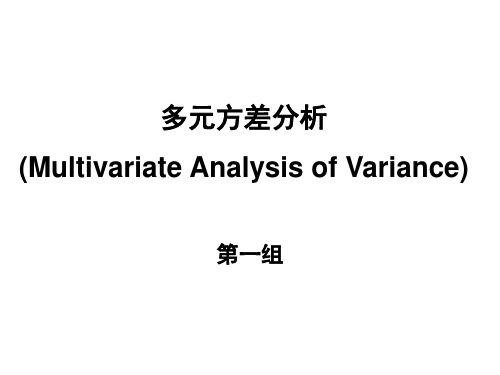
表3.1 身体指标化数据
比较三个组(k=3)的4项指标(p=4)间是否有差异,就 是检验多样本均值向量是否相等。
SPSS中的实现方式有2种: 1)通过菜单:GLM过程 2)通过编程:MANOVA过程
区别:对分类变量进行参数估计时应用的矩阵不同。
• GLM过程以某一水平为参照水平,其他水平与参 照水平进行比较,即Indicator对比(Indicator Contrast) 或Simple对比(Simple Contrast)。
(重复) 用ni表示各处理的重复数 N=n1+n2+…+ng
One-way ANOVA举例
芦苇(Phragmites australis)是广布种。欲检验产 于黑龙江、北京、江苏、广东4省的芦苇在光合效 率(A)上有无显著差异,每地各量测10株。
黑龙江(h) 北京(b) 江苏(j) 广东(g)
1
Ah1
called covariance matrix)
ANOVA的SS计算示例
例:3个营养梯度下一枝黄花(Solidago spp.)的生物量是否有显著差异,每个营 养梯度下有5棵植株
营养
生物量(g)
均值
高5
4
8
6
7
6
中1
3
1
3
2
2
低 10 13 7
9 11 10
total
6
SSerror =
g ni
(Yij yi)2
i1 j1
SStreat
= 34
g
=
ni(yi
i1
y)2
= 160
MANOVA的SSCP计算示例
处理
观测值
- 1、下载文档前请自行甄别文档内容的完整性,平台不提供额外的编辑、内容补充、找答案等附加服务。
- 2、"仅部分预览"的文档,不可在线预览部分如存在完整性等问题,可反馈申请退款(可完整预览的文档不适用该条件!)。
- 3、如文档侵犯您的权益,请联系客服反馈,我们会尽快为您处理(人工客服工作时间:9:00-18:30)。
?—Reach valid conclusions concerning a population on the basis of information from a sample. We shall concentrate on inferences about a mean vector and its componentfor a normal population meandenote a random sample from a normal population, the appropriate test statistic is1μ−2 1() 1njjX X=−∑1 at significance level ,2), where (2) denotesth percentile of th n t αα−10e -distribution|| is large is equivalent () (5-1) in (5-1) is the square X t t μ−−0 of the to the test value .μConsider the problem of determining whether a givenis a plausible value for the mean of a multivariate normal distribution.A natural generalization of the squared0distance in)) (5-2)μand ,)')(−j X X X Hotelling’s T2inhonor of Harold Hotelling. Here (1/n )S is the estimated covariance matrix of .X1, be a random sample from an1) population. Then with and ) (5-3)e nj j p n n F =−⎞⎟=⎠∑X X () is the upper distribution.p α001,Statement (5-3) leads immediately to a test of the : . At the in favor of if the 1))p n p F n H H p α−≠−μμ() (5-4)n p α−Perspiration from 20 healthy females was analyzed. Three components, X 1=sweat rate, =potassium content, were measured, and the results,sweat data , are presented in '=[4, 50, 10]against [4, 50, 10] at significance α=.10.We assume the sweat data are multivariate normal. This can be tested by the methods ofof level 10% at the see we ,18.8)10(.74.9 observed the 3.628 5.6402T p ==⎥⎥⎦will be rejected if one or more of the component means, or some combination of means, differs too much from the hypothesized values [4, 50, 10]. At this point, we have no idea which of these hypothesized values may not be supported byratio testsThere is a general principle for constructing test likelihood ratio method , -statistics can be derived as the μ= μ0. Likelihood ratio tests have several optimal properties for reasonably large samples, and they are particularly convenient for hypotheses formulated in terms of multivariate normalover their varied is the of maximum the ,estimates.1ˆ }2/exp{bygiven is values possible 1Σx x μ==−∑=n np n j jis a plausible value of , the ) is compared with the unrestricted μ/200). The resulting ratio is called the ,)ˆ||ˆ(,)||n ⎛⎞=⎜⎟⎜⎟⎝⎠μΣΣμΣΣ/20)th perce )'|nti le of th )'|e n j j c α⎞−⎟⎟<⎟−⎟⎠x x x μ. Because of the following relation , we need not the distribution of the00 be a random sample from an ) population. Then the test in (5-4) based on is equivalent to the likehood ratio test of : H =μμbe a vector of unknown populationbe the set of all possibleconfidence region is a regionvalues. It is a generalization of theconfidence interval. This region is determined by the data, we shall=[X1, X2,…, Xn]'is) is said to be a 100(1-α)%if, before the sample is(X) will cover the true θ]= 1-αThis probability is calculated under the true,12 sample observations from an ) population. Then a 100(1)% 2))(/j confidence x αα−−⎤⎥⎦1, and (/2)2)th percentile of distribution with n t t α−, sample observations from an ) population. Then a 100(1)% is the ellipsoid determined by all p n confidence F α−2,,), and ())th percentile of distributio () (5-n.5)j p n p n p p p F F αα−−−−xat of favor in reject for vectors all of consists that the see we ,analogous is this Since region.,)(])/()1 the If ).(]))()'(1000,,010ααH H F p n n F p p n p p n p μμμμx S μx ≠−−−−−−−62.6)05(.402(41)603.564..0146 .0117 values all of consists .population ),(.0146 .0117 40,2211=⎥⎦⎤⎢⎣⎡−−⎥⎦⎤⎥⎦⎤−F μμμΣμ5.1. Figure in plotted .05 at the .589]' be not would .589]' ,562 region. in the is 62.61.30 589.603.562.564..0146.01171=≤=⎥⎦⎤⎢⎣⎡−−⎥⎦⎤−αabout a population mean vector When the sample size is large, tests of hypotheses and confidence regions for μcan be constructed without the assumption of a normal population. In fact,serious departures from a normal population can be overcome by large sample sizes. Both tests of hypotheses and confidence regions will then possess (approximately) their nominal levels.The advantages associated with large samples may be partially offset by a loss in sample information caused byx Susing only the summary statistics and .On the othersummary fornormal populations, the closer the underlying population is to multivariate normal, the more efficiently the sample information will be utilized in making inferences.2All large-sample inferences about are based on - be a random sample from and positive definite covariance is large,by the r χμ)1 (5-6esu )lts in Chapter 4,)th percentile of the αα⎤≈−⎦μis the ellipsoid determined by all such thate the sample observations.is rejected in favor of, at a level of significance approximately ,αsample observations.Chap. 5 Exercises 5.1, 5.3。