Signal and Image Processing for Remote Sensing
IEEE SENSORS JOURNAL
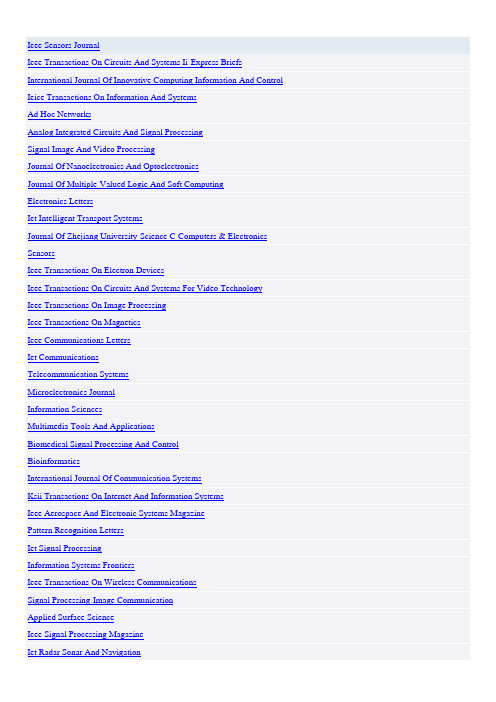
Ieee Sensors JournalIeee Transactions On Circuits And Systems Ii-Express Briefs International Journal Of Innovative Computing Information And Control Ieice Transactions On Information And SystemsAd Hoc NetworksAnalog Integrated Circuits And Signal ProcessingSignal Image And Video ProcessingJournal Of Nanoelectronics And OptoelectronicsJournal Of Multiple-Valued Logic And Soft ComputingElectronics LettersIet Intelligent Transport SystemsJournal Of Zhejiang University-Science C-Computers & Electronics SensorsIeee Transactions On Electron DevicesIeee Transactions On Circuits And Systems For Video Technology Ieee Transactions On Image ProcessingIeee Transactions On MagneticsIeee Communications LettersIet CommunicationsTelecommunication SystemsMicroelectronics JournalInformation SciencesMultimedia Tools And ApplicationsBiomedical Signal Processing And ControlBioinformaticsInternational Journal Of Communication SystemsKsii Transactions On Internet And Information SystemsIeee Aerospace And Electronic Systems MagazinePattern Recognition LettersIet Signal ProcessingInformation Systems FrontiersIeee Transactions On Wireless CommunicationsSignal Processing-Image CommunicationApplied Surface ScienceIeee Signal Processing MagazineIet Radar Sonar And NavigationIeee Journal Of Selected Topics In Signal ProcessingIeee Transactions On Audio Speech And Language Processing Eurasip Journal On Wireless Communications And Networking Materials LettersIeee Transactions On NanotechnologyIeee Transactions On Antennas And PropagationIeee Transactions On Microwave Theory And Techniques Ieice Transactions On CommunicationsScience China-Information SciencesIeee Microwave And Wireless Components LettersJournal Of Communications And NetworksChinese Journal Of ElectronicsJournal Of ElectrostaticsOptical EngineeringChina CommunicationsEurasip Journal On Advances In Signal ProcessingIeee Transactions On Vehicular TechnologySoft Computing偏重的研究方向信息科学(5) 自动化(3) 人工智能与知识工程(3) 数理科学(2) 数学(2) 计算机软件(1) 计算机科学(1) 计算数学与科学工程计算(1) 数理逻辑和与计算机相关的数(1) 信息理论与信息系统(1) 电子学与信息系统(1)投稿录用比例40%审稿速度平均4.28571个月的审稿周期RadioengineeringComputer Vision And Image UnderstandingProgress In Electromagnetics Research-PierIeee Transactions On Geoscience And Remote SensingAeu-International Journal Of Electronics And Communications Digital Signal ProcessingIet Image ProcessingSignal ProcessingIeee Transactions On Ultrasonics Ferroelectrics And Frequency Control 电子学与信息系统计算机科学(75)IEEE transactions on electron devicesJournal of guidance control and dynamicsJournal of grey systemInternational journal of communication systemsIEEE transactions on semiconductor manufacturingIEEE transactions on circuits and systems for video technologyIEEE communications lettersJournal of systems engineering and electronicsNano lettersPattern recognition lettersPhysical review lettersJournal of information scienceElectronics lettersImage and vision computingExpert systems with applicationsInformation sciencesIeee transactions on automatic controlIntelligent automation and soft computingJournal of electronic imagingBiomedical signal processing and controlOptical reviewOrganic electronicsBioinformaticsPhysical review eJournal of modern opticsInternational journal of advanced manufacturing technology International journal of software engineering and knowledge engineering Ieee electron device lettersElectromagneticsMicroelectronics journalJournal of computer science and technologyIet communicationsVisual computerJournal of network and computer applicationsPattern recognitionComputer journalWorld wide web-internet and web information systemsInformation processing lettersActa astronauticaInternational journal of wavelets multiresolution and information processing Ieee aerospace and electronic systems magazineMultimedia systemsIet signal processingIeee transactions on aerospace and electronic systemsJournal of physics a-mathematical and theoreticalDigital signal processingJournal of micromechanics and microengineeringJournal of applied mechanics-transactions of the asmeInformation systems frontiersIndustrial & engineering chemistry research信息科学(207)IEEE journal of selected topics in signal processing International journal on artificial intelligence toolsIEEE transactions on wireless communicationsIet control theory and applicationsEurasip journal on wireless communications and networking Telecommunication systemsOptics communicationsKsii transactions on internet and information systems Engineering applications of artificial intelligenceIEEE transactions on control systems technologyIEEE signal processing magazineIet radar sonar and navigationIEEE transactions on audio speech and language processing Neural processing lettersScience china-information sciencesAcm transactions on the webThin solid filmsIntegration-the vlsi journalAcm transactions on graphicsIEEE transactions on nanotechnologyFuzzy sets and systemsJournal of zhejiang university-science c-computers & electronics SensorsJournal of communications and networksInternational journal of controlIEEE transactions on visualization and computer graphics Journal of physics-condensed matterAerospace science and technologyFundamenta informaticaeIEEE transactions on information forensics and securityIEEE transactions on antennas and propagationIEEE transactions on microwave theory and techniques MeasurementMultimedia tools and applicationsIEICE transactions on communicationsApplied opticsKnowledge and information systemsChaosComputing and informaticsJournal of electrostaticsIeee transactions on mobile computingComputers & security信息科学(1) 计算机科学(1) 信息安全(1) ;平均3个月的审稿周期;/wps/find ... ription#descriptionIeee-acm transactions on computational biology and bioinformaticsACTA physico-chimica sinicaJournal of systems and software国外计算机、控制与信息技术重要期刊/journal-of-systems-and-software/Soft matterAd hoc & sensor wireless networksIEEE-asme transactions on mechatronicsIEEE microwave and wireless components lettersWireless communications & mobile computingArtificial intelligenceJournal of management information systemsComputer communicationsMaterials lettersSoft computing信息科学(5) 自动化(3) 人工智能与知识工程(3) 数理科学(2) 数学(2) 计算机软件(1) 计算机科学(1) 计算数学与科学工程计算(1) 数理逻辑和与计算机相关的数(1) 信息理论与信息系统(1) 电子学与信息系统(1) 投稿录用比例40%审稿速度平均4.28571个月的审稿周期China communicationsSensors and materialsIeee transactions on vehicular technologyApplied surface scienceJournal of vacuum science & technology bJournal of testing and evaluationJapanese journal of applied physicsIeee transactions on magneticsOptical engineering月刊,最快的投稿到见刊就两个月,当然也有慢的。
Digital Signal Processing
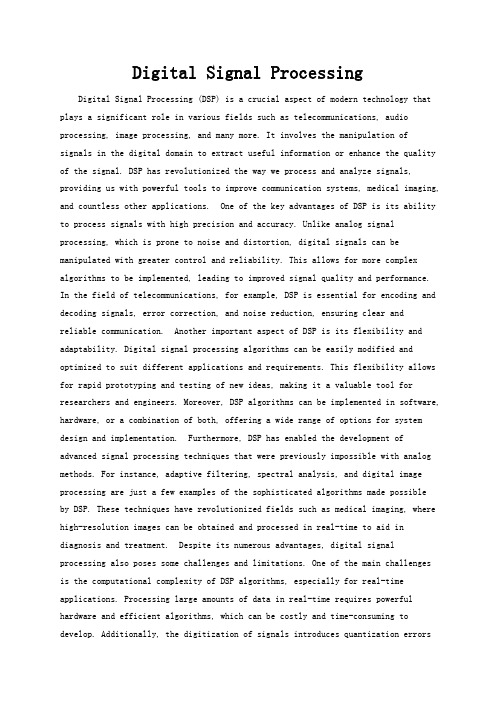
Digital Signal Processing Digital Signal Processing (DSP) is a crucial aspect of modern technology that plays a significant role in various fields such as telecommunications, audio processing, image processing, and many more. It involves the manipulation of signals in the digital domain to extract useful information or enhance the quality of the signal. DSP has revolutionized the way we process and analyze signals, providing us with powerful tools to improve communication systems, medical imaging, and countless other applications. One of the key advantages of DSP is its ability to process signals with high precision and accuracy. Unlike analog signal processing, which is prone to noise and distortion, digital signals can be manipulated with greater control and reliability. This allows for more complex algorithms to be implemented, leading to improved signal quality and performance. In the field of telecommunications, for example, DSP is essential for encoding and decoding signals, error correction, and noise reduction, ensuring clear andreliable communication. Another important aspect of DSP is its flexibility and adaptability. Digital signal processing algorithms can be easily modified and optimized to suit different applications and requirements. This flexibility allows for rapid prototyping and testing of new ideas, making it a valuable tool for researchers and engineers. Moreover, DSP algorithms can be implemented in software, hardware, or a combination of both, offering a wide range of options for system design and implementation. Furthermore, DSP has enabled the development of advanced signal processing techniques that were previously impossible with analog methods. For instance, adaptive filtering, spectral analysis, and digital image processing are just a few examples of the sophisticated algorithms made possibleby DSP. These techniques have revolutionized fields such as medical imaging, where high-resolution images can be obtained and processed in real-time to aid in diagnosis and treatment. Despite its numerous advantages, digital signal processing also poses some challenges and limitations. One of the main challengesis the computational complexity of DSP algorithms, especially for real-time applications. Processing large amounts of data in real-time requires powerful hardware and efficient algorithms, which can be costly and time-consuming to develop. Additionally, the digitization of signals introduces quantization errorsand round-off noise, which can degrade the signal quality if not properly managed. In conclusion, digital signal processing is a powerful and versatile technologythat has transformed the way we process and analyze signals in various fields. Its precision, flexibility, and advanced capabilities have made it an indispensabletool for modern technology. While there are challenges and limitations associated with DSP, its benefits far outweigh the drawbacks, making it an essential component of today's digital world. As technology continues to advance, the roleof digital signal processing will only become more prominent, driving innovation and progress in countless applications.。
剪切散斑干涉条纹图像处理技术研究进展
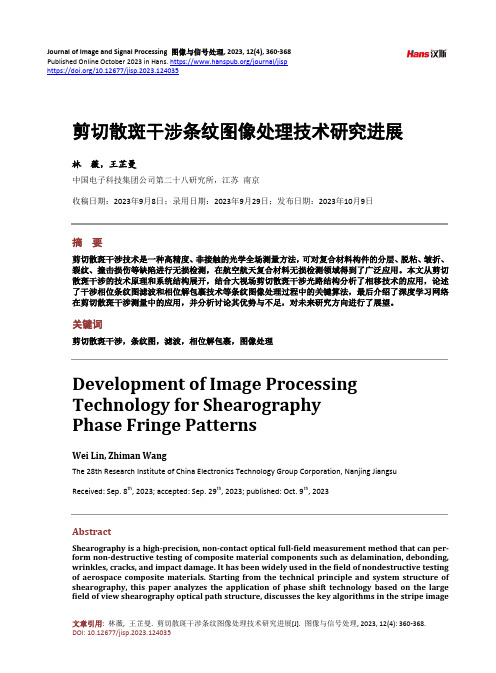
Journal of Image and Signal Processing 图像与信号处理, 2023, 12(4), 360-368Published Online October 2023 in Hans. https:///journal/jisphttps:///10.12677/jisp.2023.124035剪切散斑干涉条纹图像处理技术研究进展林薇,王芷曼中国电子科技集团公司第二十八研究所,江苏南京收稿日期:2023年9月8日;录用日期:2023年9月29日;发布日期:2023年10月9日摘要剪切散斑干涉技术是一种高精度、非接触的光学全场测量方法,可对复合材料构件的分层、脱粘、皱折、裂纹、撞击损伤等缺陷进行无损检测,在航空航天复合材料无损检测领域得到了广泛应用。
本文从剪切散斑干涉的技术原理和系统结构展开,结合大视场剪切散斑干涉光路结构分析了相移技术的应用,论述了干涉相位条纹图滤波和相位解包裹技术等条纹图像处理过程中的关键算法,最后介绍了深度学习网络在剪切散斑干涉测量中的应用,并分析讨论其优势与不足,对未来研究方向进行了展望。
关键词剪切散斑干涉,条纹图,滤波,相位解包裹,图像处理Development of Image ProcessingTechnology for ShearographyPhase Fringe PatternsWei Lin, Zhiman WangThe 28th Research Institute of China Electronics Technology Group Corporation, Nanjing JiangsuReceived: Sep. 8th, 2023; accepted: Sep. 29th, 2023; published: Oct. 9th, 2023AbstractShearography is a high-precision, non-contact optical full-field measurement method that can per-form non-destructive testing of composite material components such as delamination, debonding, wrinkles, cracks, and impact damage. It has been widely used in the field of nondestructive testing of aerospace composite materials. Starting from the technical principle and system structure of shearography, this paper analyzes the application of phase shift technology based on the large field of view shearography optical path structure, discusses the key algorithms in the stripe image林薇,王芷曼processing process such as interference phase fringe map filtering and phase dewrapping tech-nology, and finally introduces the application of deep learning network in shearography, analyzes and discusses its advantages and disadvantages, and looks forward to the future research direc-tion.KeywordsShearography, Phase Fringe Patterns, Filtering, Phase Unwrapping, Image ProcessingThis work is licensed under the Creative Commons Attribution International License (CC BY 4.0)./licenses/by/4.0/1. 引言剪切散斑干涉技术是结合应用光学、计算机技术和数字图像处理等现代技术发展起来的无损检测技术,是一种高精度、非接触的光学全场测量方法[1]。
图像处理领域的SCI期刊

图像处理领域的SCI期刊International JournalsACM Transactions on Applied PerceptionACM Transactions on GraphicsACM SIGGRAPH Computer GraphicsACM Transactions on Information SystemsJournal of VisionJournal of Visual Communication and Image RepresentationJournal of Mathematical Imaging and VisionJournal of Electronic ImagingJournal of Pattern Recognition ResearchJournal of Soft ComputingIEEE Computer Graphics and ApplicationsIEEE Signal Processing LettersIEEE Signal Processing MagazineIEEE Transactions on Circuits and Systems for Video TechnologyIEEE Transactions on Information Forensics and SecurityIEEE Transactions on Image ProcessingIEEE Transactions on Pattern Analysis and Machine IntelligenceIEEE Transactions on Visualization and Computer GraphicsInternational Journal of Image and GraphicsInternational Journal of Remote SensingInternational Journal of Computer VisionInternational Journal of Imaging Systems and TechnologyInternational Journal of Wavelets, Multiresolution and Information Processing International Journal of Pattern Recognition and Artificial Intelligence Computer Vision and Image UnderstandingComputer Graphics ForumElectronic Letters on Computer Vision and Image AnalysisEURASIP Journal on Applied SignalGraphics Interface Graphics InterfaceImage and Vision ComputingICGST International Journal on Graphics, Vision and Image ProcessingIET Signal ProcessingIET Information SecurityIET Image ProcessingIET Computer VisionIETE Technical ReviewIETE Journal of ResearchNeurophysical Journals in Computer VisionNumerical Functional Analysis and OptimizationMGV: Machine GRAPHICS & VISIONPattern RecognitionPattern Recognition LettersReal-Time ImagingSIAM Journal on Imaging SciencesSignal, Image and Video ProcessingSignal Processing : Image CommunicationVision ResearchInternational ConferencesIEEE International Conference on Computer Vision and Pattern RecognitionIEEE International Conference on Computer VisionEuropean Conference on Computer VisionAsian Conference on Computer VisionInternational Conference on Image ProcessingInternational Conference on Pattern RecognitionACM SIGGRAPH International Conference and Exhibition on Computer Graphics and Interactive techniquesInternational Joint Conference on Artificial Intelligence。
遥感期刊 国外
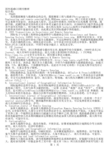
出版频率: Quarterly
出版社: PHOTOGRAMMETRIC SOC, UNIV COLL LONDON, DEPT GEOMATIC ENGINEERING,GOWER ST, LONDON, ENGLAND, WC1E 6BT
出版社网址: /
国际摄影测量与遥感协会ISPRS的会刊 ,有介绍,Elsevier数据库上有全文。该杂志 现在多分主题出版,介绍当今摄影测量与遥感最新动态,如建立DEM,TIN,激光测高,干涉测量等技术,还是有不少好文章的)不过南大图书室没有订阅该 刊,大家去看电子版吧。
7. 期刊名称:ISPRS JOURNAL OF PHOTOGRAMMETRY AND REMOTE SENSING
ISSN: 0924-2716
出版频率: Quarterly
出版社: ELSEVIER SCIENCE BV, PO BOX 211, AMSTERDAM, NETHERLANDS, 1000 AE
变更情况: 2005New
2. 期刊名称:SURVEY REVIEW
ISSN: 0039-6265
出版频率: Quarterly
出版社: COMMONWEALTH ASSOC SURVEYING LAND ECONOMY, C A S L E, UNIV WEST ENGLAND,C/O FACULTY BUILT ENVIRONMENT,FRENCHAY CAMPUS, COLDHARBOUR LBRISTOL, ENGLAND, BS16 1QY
9. Cartographica
加拿大地图学杂志,我没有细看,不便多说。好像重视地图设计地图综合等方面的研究。南大有改刊,近20年的都有。
2013年信号处理、图像处理国际会议 International Conferences on Signal Processing, Image Processing

International Journal of Advancements in Computing Technology, Jan. 30, 2013
2013 The 4th International Conference on Intelligent Control and Information Processing (ICICIP2013)
Website: /cvpr13/home.html
Venue/Country: Portland, Oregon / USA
Submission Deadline:Nov. 15, 2012
Conference Dates: Jun. 23-28, 2013
Website:
Venue/Country: Kingston / Canada
Submission Deadline: Jan. 6, 2013
Conference Dates: May 6-8 2013
2013 International Conference on Image Processing (ICIP2013)
2013 China-Ireland International Conference on Information and Communications Technologies (CIICT2013)
Website:
Venue/Country: Beijing / China
Website: /icicip2013/
Venue/Country: Beijing / China
Submission Deadline: Feb. 1, 2013
imagenoise

Simulation Example Of Frame Averaging
Low-pass Smoothing
Low-pass smoothing
Reduces highfrequency noise Smooths image Set filter cutoff at about SNR = 1
Photonelectronic noise
Photon noise Photon arrival statistics Low-light levels (nighttime imaging, astronomy) ( ρ T ) p e − ρT • Poisson density function P ( p | ρ , T ) = p! ρ T (signal-dependent) • Standard deviation = High-light levels (daytime imaging) • Poisson distribution > Gaussian distribution • Standard deviation = square root mean Thermal noise Electronic White (flat power spectrum), Gaussian distributed, zero-mean (signal-independent)
sigma filter near edges and lines
Nagao-Matsuyama filter
Calculate the variance of 9 subwindows within a 5 x 5 moving window Output pixel is the mean of the subwindow with the lowest variance
后处理的专业英文术语
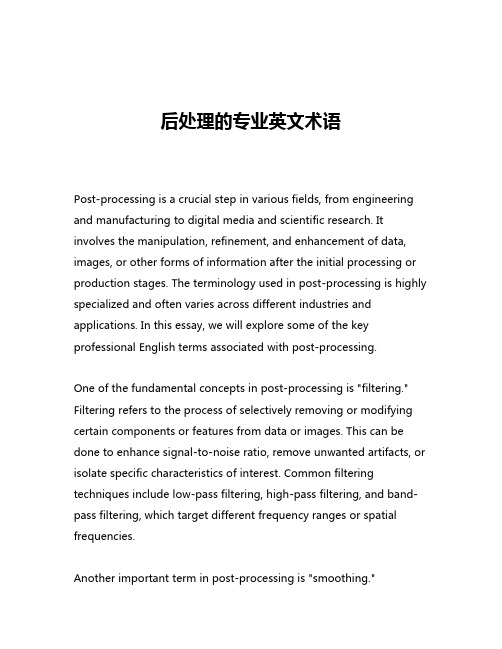
后处理的专业英文术语Post-processing is a crucial step in various fields, from engineering and manufacturing to digital media and scientific research. It involves the manipulation, refinement, and enhancement of data, images, or other forms of information after the initial processing or production stages. The terminology used in post-processing is highly specialized and often varies across different industries and applications. In this essay, we will explore some of the key professional English terms associated with post-processing.One of the fundamental concepts in post-processing is "filtering." Filtering refers to the process of selectively removing or modifying certain components or features from data or images. This can be done to enhance signal-to-noise ratio, remove unwanted artifacts, or isolate specific characteristics of interest. Common filtering techniques include low-pass filtering, high-pass filtering, and band-pass filtering, which target different frequency ranges or spatial frequencies.Another important term in post-processing is "smoothing."Smoothing involves the application of algorithms or algorithms to reduce the roughness or unevenness in data or images, often to improve the visual or analytical quality. This can be particularly useful in applications such as image processing, where smoothing can help to reduce noise or artifacts, or in data analysis, where smoothing can help to identify underlying trends or patterns."Interpolation" is another key concept in post-processing. Interpolation is the process of estimating intermediate values between known data points or pixels, allowing for the creation of new data or the enhancement of existing information. This is commonly used in image scaling, where interpolation algorithms are employed to generate additional pixels and maintain image quality when resizing or upscaling an image."Segmentation" is another important term in post-processing, particularly in the context of image and video analysis. Segmentation refers to the process of partitioning an image or video into distinct regions or objects, often based on characteristics such as color, texture, or shape. This can be useful for tasks such as object detection, scene understanding, or medical image analysis."Registration" is a crucial process in post-processing, especially in fields such as remote sensing, medical imaging, and computer vision. Registration involves the alignment of two or more images ordatasets, ensuring that corresponding features or elements are properly aligned. This can be used for tasks such as change detection, image fusion, or the integration of data from multiple sources."Denoising" is another important concept in post-processing, particularly in the context of signal processing and image analysis. Denoising refers to the process of removing or reducing unwanted noise or interference from a signal or image, often to improve the signal-to-noise ratio and enhance the quality of the data."Sharpening" is a post-processing technique used to enhance the clarity and definition of images or other visual data. Sharpening algorithms amplify the high-frequency components of an image, effectively increasing the contrast between edges and details, resulting in a more crisp and focused appearance."Warping" is a post-processing technique used to geometrically transform or distort an image or dataset. This can be used for tasks such as image rectification, where distortions caused by camera lenses or perspective are corrected, or for the alignment of images or data to a specific coordinate system or reference frame."Compression" is another important post-processing technique, particularly in the context of digital media and data storage. Compression involves the reduction of the file size or data volume ofan image, video, or other digital content, often through the use of specialized algorithms that identify and remove redundant or unnecessary information."Rendering" is a post-processing step in computer graphics and visualization, where the final appearance of a 3D scene or model is determined. Rendering involves the application of lighting, shading, and texturing algorithms to create a realistic or stylized representation of the scene.These are just a few examples of the many professional English terms associated with post-processing. The specific terminology used in post-processing can vary widely depending on the industry, application, and underlying technologies involved. However, understanding these key concepts and their associated terminology is essential for effective communication and collaboration in the field of post-processing.。
Digital Signal Processing and Filter Design

Digital Signal Processing and FilterDesignDigital Signal Processing (DSP) is a crucial aspect of modern technology, playing a significant role in various fields such as telecommunications, audio processing, image processing, and control systems. One of the key components of DSP is filter design, which involves the creation of algorithms and systems to manipulate digital signals for specific purposes such as noise reduction, signal enhancement, and data compression. However, filter design can be a complex and challenging task, requiring a deep understanding of signal processing theory, mathematical algorithms, and practical implementation techniques. From atechnical perspective, filter design involves the application of mathematical concepts such as convolution, Fourier analysis, and z-transforms to manipulate digital signals. This process requires a solid understanding of signal processing theory and a strong mathematical background to effectively design and implement filters. Additionally, filter design often involves the use of specialized software tools such as MATLAB, Python, or Simulink, which require proficiency in programming and algorithm development. Moreover, the implementation of filters in real-world applications requires careful consideration of factors such as computational complexity, hardware limitations, and real-time processing constraints. Furthermore, filter design also encompasses a practical aspect, as engineers and researchers must consider the specific requirements and constraints of the application. For instance, in audio processing, the design of digitalfilters must take into account the frequency response, phase characteristics, and group delay to ensure high-quality sound reproduction. Similarly, in telecommunications, filter design plays a crucial role in channel equalization, interference rejection, and signal demodulation, requiring careful consideration of factors such as signal-to-noise ratio, bandwidth requirements, and transmission efficiency. Moreover, filter design also involves a creative element, as engineers and researchers must often innovate and develop novel solutions to address complex signal processing challenges. This may involve the exploration of new algorithms, the integration of multiple filtering techniques, or theadaptation of existing methods to new applications. As such, filter design requires a combination of technical expertise, practical experience, and creative problem-solving skills to effectively meet the demands of modern signal processing applications. In conclusion, digital signal processing and filter design are integral components of modern technology, with a wide range of applications in various fields. The process of filter design involves a combination of technical, practical, and creative elements, requiring a deep understanding of signal processing theory, mathematical algorithms, and real-world application requirements. As technology continues to advance, the importance of filter design in shaping the future of digital signal processing will only continue to grow, making it a critical area of study and research for engineers and researchers alike.。
纹理物体缺陷的视觉检测算法研究--优秀毕业论文
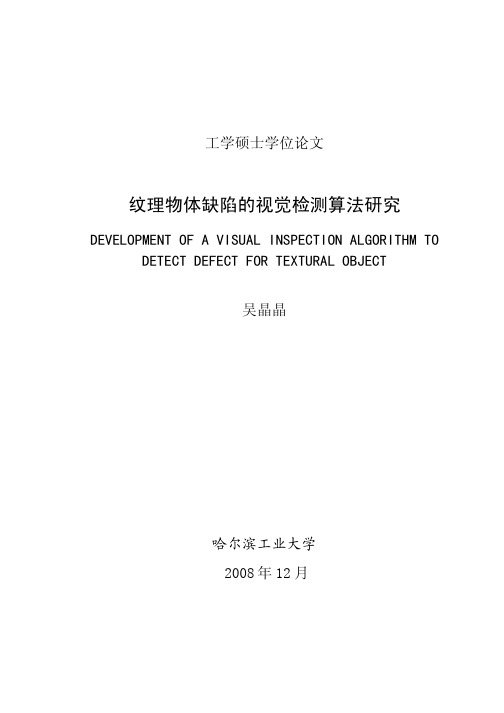
摘 要
在竞争激烈的工业自动化生产过程中,机器视觉对产品质量的把关起着举足 轻重的作用,机器视觉在缺陷检测技术方面的应用也逐渐普遍起来。与常规的检 测技术相比,自动化的视觉检测系统更加经济、快捷、高效与 安全。纹理物体在 工业生产中广泛存在,像用于半导体装配和封装底板和发光二极管,现代 化电子 系统中的印制电路板,以及纺织行业中的布匹和织物等都可认为是含有纹理特征 的物体。本论文主要致力于纹理物体的缺陷检测技术研究,为纹理物体的自动化 检测提供高效而可靠的检测算法。 纹理是描述图像内容的重要特征,纹理分析也已经被成功的应用与纹理分割 和纹理分类当中。本研究提出了一种基于纹理分析技术和参考比较方式的缺陷检 测算法。这种算法能容忍物体变形引起的图像配准误差,对纹理的影响也具有鲁 棒性。本算法旨在为检测出的缺陷区域提供丰富而重要的物理意义,如缺陷区域 的大小、形状、亮度对比度及空间分布等。同时,在参考图像可行的情况下,本 算法可用于同质纹理物体和非同质纹理物体的检测,对非纹理物体 的检测也可取 得不错的效果。 在整个检测过程中,我们采用了可调控金字塔的纹理分析和重构技术。与传 统的小波纹理分析技术不同,我们在小波域中加入处理物体变形和纹理影响的容 忍度控制算法,来实现容忍物体变形和对纹理影响鲁棒的目的。最后可调控金字 塔的重构保证了缺陷区域物理意义恢复的准确性。实验阶段,我们检测了一系列 具有实际应用价值的图像。实验结果表明 本文提出的纹理物体缺陷检测算法具有 高效性和易于实现性。 关键字: 缺陷检测;纹理;物体变形;可调控金字塔;重构
Keywords: defect detection, texture, object distortion, steerable pyramid, reconstruction
II
Signal Processing For A Sagnac Interferometer

发明人:Ralph A. Bergh 申请号:US1168254 9 申请日:20070306 公开号:US20080218765A1 公开日:20080911 专利附图:
摘要:Disclosed is a method and apparatus for modulating the phase difference between a pair of optical waves that exit a Sagnac interferometer, and, more particularly, one that is commonly employed as a fiber gyro, and includes a detector responsive to
phase difference between the pair of waves that exit the interferometer, and a phase modulator that forms part of two control loops that are instrumental in deriving an accurate measurement of rotation rate. As disclosed herein, phase modulation applied equally to the pair of waves as they counter-propagate through the phase modulator induces modulation of the phase-difference between the two waves as they exit the interferometer. This phase difference modulation includes (i) a bias phase-difference modulation component having a selected frequency, amplitude, and waveform, (ii) a rotation-rate feedback phase-difference component that is equal in magnitude to, and opposite in sign of, the Sagnac phase difference, so that the sum thereof is controlled to be substantially zero, and (iii) a calibration feedback phase-difference modulation component that is characterized by a predetermined phase difference magnitude having substantially alternating positive and negative sign values.
convolve函数
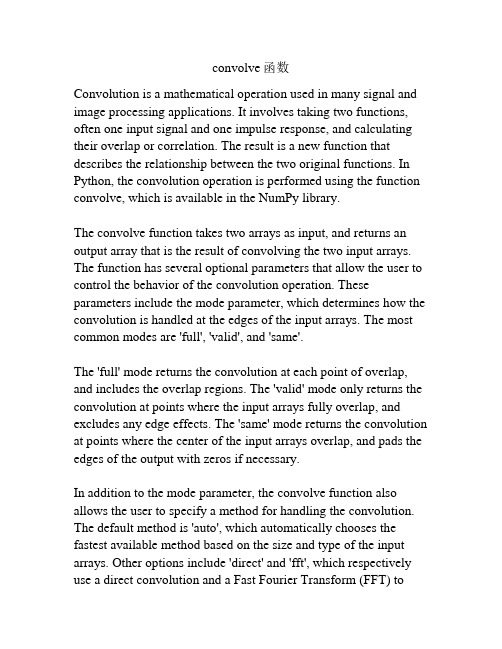
convolve函数Convolution is a mathematical operation used in many signal and image processing applications. It involves taking two functions, often one input signal and one impulse response, and calculating their overlap or correlation. The result is a new function that describes the relationship between the two original functions. In Python, the convolution operation is performed using the function convolve, which is available in the NumPy library.The convolve function takes two arrays as input, and returns an output array that is the result of convolving the two input arrays. The function has several optional parameters that allow the user to control the behavior of the convolution operation. These parameters include the mode parameter, which determines how the convolution is handled at the edges of the input arrays. The most common modes are 'full', 'valid', and 'same'.The 'full' mode returns the convolution at each point of overlap, and includes the overlap regions. The 'valid' mode only returns the convolution at points where the input arrays fully overlap, and excludes any edge effects. The 'same' mode returns the convolution at points where the center of the input arrays overlap, and pads the edges of the output with zeros if necessary.In addition to the mode parameter, the convolve function also allows the user to specify a method for handling the convolution. The default method is 'auto', which automatically chooses the fastest available method based on the size and type of the input arrays. Other options include 'direct' and 'fft', which respectively use a direct convolution and a Fast Fourier Transform (FFT) toperform the convolution.The convolve function can be used to perform a wide range of signal and image processing operations. For example, it can be used to filter out noise from an audio signal, to sharpen or blur an image, or to detect edges in an image. In addition to its applications in signal and image processing, convolution is also used in many other areas of science and engineering, including physics, biology, and economics.In conclusion, the convolve function is an important tool for performing convolution operations in Python. It allows the user to easily perform a wide range of signal and image processing operations, and provides a range of options for controlling the behavior of the convolution. Whether you are working in the field of signal processing, computer vision, or another area of science and engineering, the convolve function is an essential tool for your toolkit.。
于慧敏,浙江大学,教授,博士生导师。主要研究方向为图像视频处理与
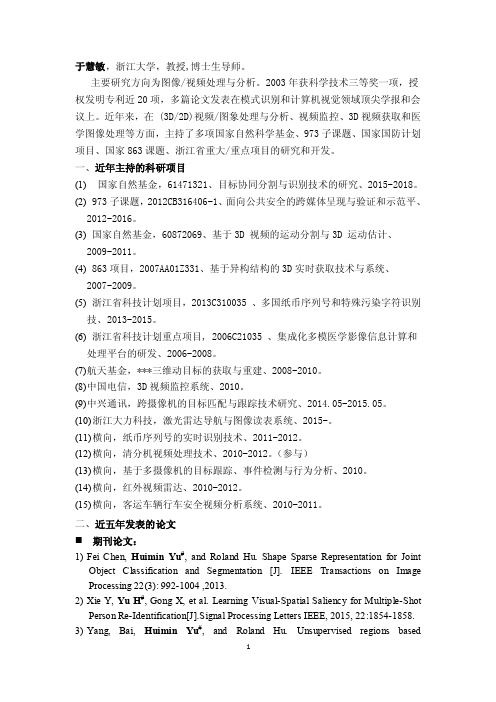
于慧敏,浙江大学,教授,博士生导师。
主要研究方向为图像/视频处理与分析。
2003年获科学技术三等奖一项,授权发明专利近20项,多篇论文发表在模式识别和计算机视觉领域顶尖学报和会议上。
近年来,在 (3D/2D)视频/图象处理与分析、视频监控、3D视频获取和医学图像处理等方面,主持了多项国家自然科学基金、973子课题、国家国防计划项目、国家863课题、浙江省重大/重点项目的研究和开发。
一、近年主持的科研项目(1)国家自然基金,61471321、目标协同分割与识别技术的研究、2015-2018。
(2) 973子课题,2012CB316406-1、面向公共安全的跨媒体呈现与验证和示范平、2012-2016。
(3)国家自然基金,60872069、基于3D 视频的运动分割与3D 运动估计、2009-2011。
(4) 863项目,2007AA01Z331、基于异构结构的3D实时获取技术与系统、2007-2009。
(5)浙江省科技计划项目,2013C310035 、多国纸币序列号和特殊污染字符识别技、2013-2015。
(6)浙江省科技计划重点项目, 2006C21035 、集成化多模医学影像信息计算和处理平台的研发、2006-2008。
(7)航天基金,***三维动目标的获取与重建、2008-2010。
(8)中国电信,3D视频监控系统、2010。
(9)中兴通讯,跨摄像机的目标匹配与跟踪技术研究、2014.05-2015.05。
(10)浙江大力科技,激光雷达导航与图像读表系统、2015-。
(11)横向,纸币序列号的实时识别技术、2011-2012。
(12)横向,清分机视频处理技术、2010-2012。
(参与)(13)横向,基于多摄像机的目标跟踪、事件检测与行为分析、2010。
(14)横向,红外视频雷达、2010-2012。
(15)横向,客运车辆行车安全视频分析系统、2010-2011。
二、近五年发表的论文期刊论文:1)Fei Chen, Huimin Yu#, and Roland Hu. Shape Sparse Representation for JointObject Classification and Segmentation [J]. IEEE Transactions on Image Processing 22(3): 992-1004 ,2013.2)Xie Y, Yu H#, Gong X, et al. Learning Visual-Spatial Saliency for Multiple-ShotPerson Re-Identification[J].Signal Processing Letters IEEE, 2015, 22:1854-1858.3)Yang, Bai, Huimin Yu#, and Roland Hu. Unsupervised regions basedsegmentation using object discovery, Journal of Visual Communication and Image Representation, 2015,31: 125-137.4)Fei Chen, Roland Hu, Huimin Yu#, Shiyan Wang: Reduced set density estimatorfor object segmentation based on shape probabilistic representation. J. Visual Communication and Image Representation,2012, 23(7): 1085-1094.5)Fei Chen, Huimin Yu#, Jincao Yao , Roland Hu ,Robust sparse kernel densityestimation by inducing randomness[J],Pattern Analysis and Applications: Volume 18, Issue 2 (2015), Page 367-375.6)赵璐,于慧敏#,基于先验形状信息和水平集方法的车辆检测,浙江大学学报(工学版),pp.124-129,2010.1。
遥感期刊_国外

7. 期刊名称:ISPRS JOURNAL OF PHOTOGRAMMETRY AND REMOTE SENSING
ISSN: 0924-2716
出版频率: Quarterly
出版社: ELSEVIER SCIENCE BV, PO BOX 211, AMSTERDAM, NETHERLANDS, 1000 AE
出版社网址: http://www.elsevier.nl
期刊网址: http://www.elsevier.nl/locate/isprsjprs
期刊网址: /
影响因子: 0.102(2002)
主题范畴: GEOSCIENCES来自 MULTIDISCIPLINARY; REMOTE SENSING; ENGINEERING, CIVIL
3. 期刊名称:PHOTOGRAMMETRIC RECORD
7. International Journal of Geographical Information
国际地理系统杂志,是1999新创刊的,我觉得比较好,特别是有一次看到一个关于spatial analysis的专刊,德国施普林格出版社有英文全文,可以免费看到文章摘要,南大有2000年后的杂志,季刊。
出版社网址: /
期刊网址: /publications.html
影响因子: 0.841(2001);1.176(2002)
主题范畴: GEOGRAPHY, PHYSICAL; GEOSCIENCES, MULTIDISCIPLINARY; REMOTE SENSING; PATHOLOGY
11. Surveying and land information system
Signalandimageprocessing
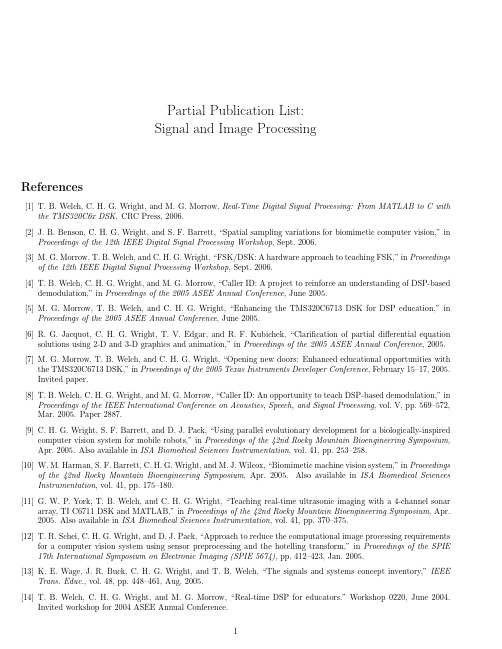
Partial Publication List:Signal and Image ProcessingReferences[1]T.B.Welch,C.H.G.Wright,and M.G.Morrow,Real-Time Digital Signal Processing:From MATLAB to C withthe TMS320C6x DSK.CRC Press,2006.[2]J.B.Benson,C.H.G.Wright,and S.F.Barrett,“Spatial sampling variations for biomimetic computer vision,”inProceedings of the12th IEEE Digital Signal Processing Workshop,Sept.2006.[3]M.G.Morrow,T.B.Welch,and C.H.G.Wright,“FSK/DSK:A hardware approach to teaching FSK,”in Proceedingsof the12th IEEE Digital Signal Processing Workshop,Sept.2006.[4]T.B.Welch,C.H.G.Wright,and M.G.Morrow,“Caller ID:A project to reinforce an understanding of DSP-baseddemodulation,”in Proceedings of the2005ASEE Annual Conference,June2005.[5]M.G.Morrow,T.B.Welch,and C.H.G.Wright,“Enhancing the TMS320C6713DSK for DSP education,”inProceedings of the2005ASEE Annual Conference,June2005.[6]R.G.Jacquot,C.H.G.Wright,T.V.Edgar,and R.F.Kubichek,“Clarification of partial differential equationsolutions using2-D and3-D graphics and animation,”in Proceedings of the2005ASEE Annual Conference,2005.[7]M.G.Morrow,T.B.Welch,and C.H.G.Wright,“Opening new doors:Enhanced educational opportunities withthe TMS320C6713DSK,”in Proceedings of the2005Texas Instruments Developer Conference,February15–17,2005.Invited paper.[8]T.B.Welch,C.H.G.Wright,and M.G.Morrow,“Caller ID:An opportunity to teach DSP-based demodulation,”inProceedings of the IEEE International Conference on Acoustics,Speech,and Signal Processing,vol.V,pp.569–572, Mar.2005.Paper2887.[9]C.H.G.Wright,S.F.Barrett,and D.J.Pack,“Using parallel evolutionary development for a biologically-inspiredcomputer vision system for mobile robots,”in Proceedings of the42nd Rocky Mountain Bioengineering Symposium, Apr.2005.Also available in ISA Biomedical Sciences Instrumentation,vol.41,pp.253–258.[10]W.M.Harman,S.F.Barrett,C.H.G.Wright,and M.J.Wilcox,“Biomimetic machine vision system,”in Proceedingsof the42nd Rocky Mountain Bioengineering Symposium,Apr.2005.Also available in ISA Biomedical Sciences Instrumentation,vol.41,pp.175–180.[11]G.W.P.York,T.B.Welch,and C.H.G.Wright,“Teaching real-time ultrasonic imaging with a4-channel sonararray,TI C6711DSK and MATLAB,”in Proceedings of the42nd Rocky Mountain Bioengineering Symposium,Apr.2005.Also available in ISA Biomedical Sciences Instrumentation,vol.41,pp.370–375.[12]T.R.Schei,C.H.G.Wright,and D.J.Pack,“Approach to reduce the computational image processing requirementsfor a computer vision system using sensor preprocessing and the hotelling transform,”in Proceedings of the SPIE 17th International Symposium on Electronic Imaging(SPIE5674),pp.412–423,Jan.2005.[13]K.E.Wage,J.R.Buck,C.H.G.Wright,and T.B.Welch,“The signals and systems concept inventory,”IEEEc.,vol.48,pp.448–461,Aug.2005.[14]T.B.Welch,C.H.G.Wright,and M.G.Morrow,“Real-time DSP for educators.”Workshop0220,June2004.[15]T.B.Welch,M.G.Morrow,and C.H.G.Wright,“Reach out and DSP someone!,”in Proceedings of the2004ASEEAnnual Conference,June2004.Paper3620-06.[16]T.B.Welch,M.G.Morrow,C.H.G.Wright,and R.W.Ives,“commDSK:a tool for teaching modem design andanalysis,”ASEE c.J.,vol.XIV,pp.82–89,Apr.2004.[17]T.B.Welch,M.G.Morrow,and C.H.G.Wright,“Using DSP hardware to control your world,”in Proceedings ofthe IEEE International Conference on Acoustics,Speech,and Signal Processing,vol.V,pp.1041–1044,May2004.Paper1146.[18]T.B.Welch,C.H.G.Wright,and M.G.Morrow,“Experiences in offering a DSP-based communication laboratory,”in Proceedings of the11th IEEE Digital Signal Processing Workshop and the3rd IEEE Signal Processing Education Workshop,Aug.2004.[19]K.E.Wage,J.R.Buck,and C.H.G.Wright,“Obstacles in signals and systems conceptual learning,”in Proceedingsof the11th IEEE Digital Signal Processing Workshop and the3rd IEEE Signal Processing Education Workshop,Aug.2004.[20]C.H.G.Wright,S.F.Barrett,D.J.Pack,T.R.Schei,J.R.Anderson,and M.J.Wilcox,“Computational imageprocessing for a computer vision system using biomimetic sensors and eigenspace object models,”in Proceedings of the SPIE16th International Symposium on Electronic Imaging(SPIE5299),pp.327–336,Jan.2004.[21]D.J.Pack and C.H.G.Wright,“Object recognition of occluded targets using a subspace graph search method.”Online Presentation,2004./electrical/research/wispr/pubs/subspace.pdf. [22]T.B.Welch,C.H.G.Wright,and M.G.Morrow,“Real-time DSP for educators.”Workshop0220,June2003.Invited workshop for2003ASEE Annual Conference.[23]T.B.Welch,M.G.Morrow,C.H.G.Wright,and R.W.Ives,“commDSK:a tool for teaching modem design andanalysis,”in Proceedings of the2003ASEE Annual Conference,June2003.Session2420.[24]C.H.G.Wright,T.B.Welch,D.M.Etter,and M.G.Morrow,“Teaching DSP:Bridging the gap from theory toreal-time hardware,”ASEE c.J.,vol.XIII,pp.14–26,July2003.[25]C.H.G.Wright,T.B.Welch,and M.G.Morrow,“An inexpensive method to teach hands-on digital communi-cations,”in Proceedings of the IEEE/ASEE Frontiers in Education Annual Conference,pp.F2E19–F2E24,Nov.2003.[26]T.B.Welch,R.W.Ives,M.G.Morrow,and C.H.G.Wright,“Using DSP hardware to teach modem designand analysis techniques,”in Proceedings of the IEEE International Conference on Acoustics,Speech,and Signal Processing,vol.III,pp.769–772,Apr.2003.[27]D.J.Pack and C.H.G.Wright,“A fast object recognition system using subspace representation,”2003.in review,IEEE Trans.Image Proc.[28]D.J.Pack and C.H.G.Wright,“Comparing two occluded object recognition systems,”in Proceedings of the9thIASTED International Conference on Signal and Image Processing,The International Association of Science and Technology for Development,August2003.[29]C.H.G.Wright,T.B.Welch,D.M.Etter,and M.G.Morrow,“Teaching DSP:Bridging the gap from theory toreal-time hardware,”in Proceedings of the2002ASEE Annual Conference,June2002.[30]K.E.Wage,J.R.Buck,T.B.Welch,and C.H.G.Wright,“The signals and systems concept inventory,”inProceedings of the2002ASEE Annual Conference,June2002.[31]G.W.P.York,C.H.G.Wright,M.G.Morrow,and T.B.Welch,“Teaching real-time sonar with the C6711DSKand MATLAB,”ASEE c.J.,vol.XII,pp.79–87,July2002.[32]C.H.G.Wright,T.B.Welch,D.M.Etter,and M.G.Morrow,“A systematic model for teaching DSP,”in Proceedingsof the IEEE International Conference on Acoustics,Speech,and Signal Processing,vol.IV,pp.4140–4143,May2002.[33]C.H.G.Wright,T.B.Welch,D.M.Etter,and M.G.Morrow,“Teaching hardware-based DSP:Theory to practice,”in Proceedings of the IEEE International Conference on Acoustics,Speech,and Signal Processing,vol.IV,pp.4148–4151,May2002.Paper4024(invited).[34]K.E.Wage,J.R.Buck,T.B.Welch,and C.H.G.Wright,“The continuous time signals and systems conceptinventory,”in Proceedings of the IEEE International Conference on Acoustics,Speech,and Signal Processing,vol.IV, pp.4112–4115,IEEE,May2002.Paper2985.[35]S.F.Barrett,M.J.Wilcox,T.E.Olson,D.J.Pack,and C.H.G.Wright,“Modelling a parallel L4neuron arrayof thefly(Musca domestica)vision system with a sequential processor,”ISA Biomedical Sciences Instrumentation, vol.38,pp.477–482,Apr.2002.[36]M.G.Morrow,T.B.Welch,and C.H.G.Wright,“A tool for real-time DSP demonstration and experimentation,”in Proceedings of the10th IEEE Digital Signal Processing Workshop,Oct.2002.Paper4.8.[37]K.E.Wage,J.R.Buck,T.B.Welch,and C.H.G.Wright,“Testing and validation of the signals and systemsconcept inventory,”in Proceedings of the10th IEEE Digital Signal Processing Workshop,Oct.2002.Paper4.6. [38]T.B.Welch,D.M.Etter,C.H.G.Wright,M.G.Morrow,and G.J.Twohig,“Experiencing DSP hardware priorto a DSP course,”in Proceedings of the10th IEEE Digital Signal Processing Workshop,Oct.2002.Paper8.5. [39]D.J.Pack and C.H.G.Wright,“Object recognition of occluded targets using a subspace graph search method,”inProceedings of the6th World Multiconference on Systemics,Cybernetics and Informatics(SCI2002),International Institute of Informatics and Systemics,July2002.[40]S.F.Barrett,C.H.G.Wright,H.Zwick,M.Wilcox,B.A.Rockwell,and E.Naess,“Efficiently tracking a movingobject in two-dimensional image space,”SPIE Journal of Electronic Imaging,vol.10,pp.785–793,July2001. [41]T.B.Welch,M.G.Morrow,and C.H.G.Wright,“Teaching practical hands-on DSP with MATLAB and the C31DSK,”ASEE c.J.,vol.XI,pp.13–20,Apr.2001.[42]M.G.Morrow,T.B.Welch,C.H.G.Wright,and G.W.P.York,“Demonstration platform for real-time beamform-ing,”in Proceedings of the IEEE International Conference on Acoustics,Speech,and Signal Processing,May2001.Paper1146.[43]M.G.Morrow,T.B.Welch,and C.H.G.Wright,“An introduction to hardware-based DSP using winDSK6,”inProceedings of the2001ASEE Annual Conference,June2001.Session1320.[44]T.B.Welch,C.T.Field,and C.H.G.Wright,“A signal analyzer for teaching signals and systems,”in Proceedingsof the2001ASEE Annual Conference,June2001.Session2793.[45]C.H.G.Wright,T.B.Welch,and M.G.Morrow,“Teaching transfer functions with MATLAB and real-time DSP,”in Proceedings of the2001ASEE Annual Conference,June2001.Session1320.[46]G.W.P.York,M.G.Morrow,T.B.Welch,and C.H.G.Wright,“Teaching real-time sonar with the C6711DSKand MATLAB,”in Proceedings of the2001ASEE Annual Conference,June2001.Session1320.[47]M.G.Morrow,T.B.Welch,and C.H.G.Wright,“An inexpensive software tool for teaching real-time DSP,”inProceedings of the1st IEEE DSP in Education Workshop,IEEE Signal Processing Society,Oct.2000.[48]T.B.Welch,M.G.Morrow,and C.H.G.Wright,“Teaching practical hands-on DSP with MATLAB and the C31DSK,”in Proceedings of the2000ASEE Annual Conference,June2000.Paper1320-03.[49]C.H.G.Wright,T.B.Welch,M.G.Morrow,and W.J.Gomes III,“Teaching real-world DSP using Matlab andthe TMS320C31DSK,”ASEE c.J.,vol.X,pp.28–35,Jan.2000.[50]T.B.Welch,C.H.G.Wright,and M.G.Morrow,“Poles and zeroes and Matlab,oh my!,”ASEE c.J.,vol.X,pp.70–72,Apr.2000.[51]M.G.Morrow,T.B.Welch,C.H.G.Wright,and G.York,“Teaching real-time beamforming with the C6211DSKand Matlab,”in Proceedings of the2000Texas Instruments DSP Educators and Third-Party Conference,August[52]T.B.Welch,C.H.G.Wright,and M.G.Morrow,“Poles and zeroes and Matlab,oh my!,”in Proceedings of the1999ASEE Annual Conference,June1999.Paper1320-02.[53]C.H.G.Wright,T.B.Welch,M.G.Morrow,and W.J.Gomes III,“Teaching real-world DSP using Matlab andthe TMS320C31DSK,”in Proceedings of the1999ASEE Annual Conference,June1999.Paper1320-06.[54]T.B.Welch,B.Jenkins,and C.H.G.Wright,“Computer interfaces for teaching the Nintendo generation,”inProceedings of the1999ASEE Annual Conference,June1999.Paper3532-02.[55]C.H.G.Wright and T.B.Welch,“Teaching real-world DSP using Matlab,”ASEE c.J.,vol.IX,pp.1–5,Jan–Mar1999.[56]C.H.G.Wright,T.B.Welch,and M.G.Morrow,“Making DSP fun for students using Matlab and the c31DSK”,”in Proceedings of the1999Texas Instruments DSP Educators and Third-Party Conference,August4–6,1999. [57]C.H.G.Wright and T.B.Welch,“Teaching DSP concepts using Matlab and the TMS320C31DSK,”in Proceedingsof the IEEE International Conference on Acoustics,Speech,and Signal Processing,Mar.1999.Paper1778.[58]C.H.G.Wright,P.W.de Graaf,and S.F.Barrett,“Signal processing for robotically assisted laser photocoagulationof the retina,”in Proceedings of the SPIE International Society for Optical Engineering Annual Conference,July 1999.Paper3809-20.[59]C.H.G.Wright and T.B.Welch,“Teaching real-world DSP using Matlab,”in Proceedings of the1998ASEEAnnual Conference,June1998.Paper1220-03.[60]C.H.G.Wright and T.B.Welch,“Teaching DSP concepts using Matlab and the TMS320C5x,”in Proceedings ofthe1998Texas Instruments DSP Educators and Third-Party Conference,August6–8,1998.[61]C.H.G.Wright,E.J.Delp,and N.C.Gallagher,“Nonlinear target enhancement algorithms to counter the hostilenuclear environment,”IEEE Trans.Aerosp.Elect.Syst.,vol.AES-26,pp.122–145,Jan.1990.[62]C.H.G.Wright,E.J.Delp,and N.C.Gallagher,“Morphological based target enhancement algorithms,”in postersession at IEEE6th Workshop on Multidimensional Signal Processing,Sept.1989.[63]C.H.G.Wright,E.J.Delp,and N.C.Gallagher,“Morphological based target enhancement algorithms to counterthe hostile nuclear environment,”in Proceedings of the IEEE International Conference on Systems,Man,and Cy-bernetics,Nov.1989.[64]C.H.G.Wright and E.J.Delp,“A study of target enhancement algorithms to counter the hostile nuclear environ-ment,”Tech.Rep.TR-EE88-20,Purdue University,May1988.。
图像处理类相关英文文献

Journal of VLSI Signal Processing39,295–311,2005c 2005Springer Science+Business Media,Inc.Manufactured in The Netherlands.Parallel-Beam Backprojection:An FPGA Implementation Optimizedfor Medical ImagingMIRIAM LEESER,SRDJAN CORIC,ERIC MILLER AND HAIQIAN YU Department of Electrical and Computer Engineering,Northeastern University,Boston,MA02115,USAMARC TREPANIERMercury Computer Systems,Inc.,Chelmsford,MA01824,USAReceived September2,2003;Revised March23,2004;Accepted May7,2004Abstract.Medical image processing in general and computerized tomography(CT)in particular can benefit greatly from hardware acceleration.This application domain is marked by computationally intensive algorithms requiring the rapid processing of large amounts of data.To date,reconfigurable hardware has not been applied to the important area of image reconstruction.For efficient implementation and maximum speedup,fixed-point implementations are required.The associated quantization errors must be carefully balanced against the requirements of the medical community.Specifically,care must be taken so that very little error is introduced compared tofloating-point implementations and the visual quality of the images is not compromised.In this paper,we present an FPGA implementation of the parallel-beam backprojection algorithm used in CT for which all of these requirements are met.We explore a number of quantization issues arising in backprojection and concentrate on minimizing error while maximizing efficiency.Our implementation shows approximately100times speedup over software versions of the same algorithm running on a1GHz Pentium,and is moreflexible than an ASIC implementation.Our FPGA implementation can easily be adapted to both medical sensors with different dynamic ranges as well as tomographic scanners employed in a wider range of application areas including nondestructive evaluation and baggage inspection in airport terminals.Keywords:backprojection,medical imaging,tomography,FPGA,fixed point arithmetic1.IntroductionReconfigurable hardware offers significant potentialfor the efficient implementation of a wide range ofcomputationally intensive signal and image process-ing algorithms.The advantages of utilizing Field Pro-grammable Gate Arrays(FPGAs)instead of DSPsinclude reductions in the size,weight,performanceand power required to implement the computationalplatform.FPGA implementations are also preferredover ASIC implementations because FPGAs have moreflexibility and lower cost.To date,the full utility ofthis class of hardware has gone largely unexploredand unexploited for many mainstream applications.In this paper,we consider a detailed implementa-tion and comprehensive analysis of one of the mostfundamental tomographic image reconstruction steps,backprojection,on reconfigurable hardware.While weconcentrate our analysis on issues arising in the useof backprojection for medical imaging applications,both the implementation and the analysis we providecan be applied directly or easily extended to a widerange of otherfields where this task needs to be per-formed.This includes remote sensing and surveillanceusing synthetic aperture radar and non-destructiveevaluation.296Leeser et al.Tomography refers to the process that generates a cross-sectional or volumetric image of an object from a series of projections collected by scanning the ob-ject from many different directions[1].Projection data acquisition can utilize X-rays,magnetic resonance,ra-dioisotopes,or ultrasound.The discussion presented here pertains to the case of two-dimensional X-ray ab-sorption tomography.In this type of tomography,pro-jections are obtained by a number of sensors that mea-sure the intensity of X-rays travelling through a slice of the scanned object.The radiation source and the sen-sor array rotate around the object in small increments. One projection is taken for each rotational angle.The image reconstruction process uses these projections to calculate the average X-ray attenuation coefficient in cross-sections of a scanned slice.If different structures inside the object induce different levels of X-ray atten-uation,they are discernible in the reconstructed image. The most commonly used approach for image recon-struction from dense projection data(many projections, many samples per projection)isfiltered backprojection (FBP).Depending on the type of X-ray source,FBP comes in parallel-beam and fan-beam variations[1].In this paper,we focus on parallel-beam backprojection, but methods and results presented here can be extended to the fan-beam case with modifications.FBP is a computationally intensive process.For an image of size n×n being reconstructed with n projec-tions,the complexity of the backprojection algorithm is O(n3).Image reconstruction through backprojection is a highly parallelizable process.Such applications are good candidates for implementation in Field Pro-grammable Gate Array(FPGA)devices since they pro-videfine-grained parallelism and the ability to be cus-tomized to the needs of a particular implementation. We have implemented backprojection by making use of these principles and shown approximately100times speedup over a software implementation on a1GHz Pentium.Our architecture can easily be expanded to newer and larger FPGA devices,further accelerating image generation by extracting more data parallelism.A difficulty of implementing FBP is that producing high-resolution images with good resemblance to in-ternal characteristics of the scanned object requires that both the density of each projection and their total num-ber be large.This represents a considerable challenge for hardware implementations,which attempt to maxi-mize the parallelism in the implementation.Therefore, it can be beneficial to usefixed-point implementations and to optimize the bit-width of a projection sample to the specific needs of the targeted application domain. We show this for medical imaging,which exhibits distinctive properties in terms of requiredfixed-point precision.In addition,medical imaging requires high precision reconstructions since visual quality of images must not be compromised.We have paid special attention to this requirement by carefully analyzing the effects of quan-tization on the quality of reconstructed images.We have found that afixed-point implementation with properly chosen bit-widths can give high quality reconstructions and,at the same time,make hardware implementation fast and area efficient.Our quantization analysis inves-tigates algorithm specific and also general data quanti-zation issues that pertain to input data.Algorithm spe-cific quantization deals with the precision of spatial ad-dress generation including the interpolation factor,and also investigates bit reduction of intermediate results for different rounding schemes.In this paper,we focus on both FPGA implemen-tation performance and medical image quality.In pre-vious work in the area of hardware implementations of tomographic processing algorithms,Wu[2]gives a brief overview of all major subsystems in a com-puted tomography(CT)scanner and proposes loca-tions where ASICs and FPGAs can be utilized.Ac-cording to the author,semi-custom digital ASICs were the most appropriate due to the level of sophistica-tion that FPGA technology had in1991.Agi et al.[3]present thefirst description of a hardware solu-tion for computerized tomography of which we are aware.It is a unified architecture that implements for-ward Radon transform,parallel-and fan-beam back-projection in an ASIC based multi-processor system. Our FPGA implementation focuses on backprojection. Agi et al.[4]present a similar investigation of quanti-zation effects;however their results do not demonstrate the suitability of their implementation for medical ap-plications.Although theirfiltered sinogram data are quantized with12-bit precision,extensive bit trunca-tion on functional unit outputs and low accuracy of the interpolation factor(absolute error of up to2)ren-der this implementation significantly less accurate than ours,which is based on9-bit projections and the max-imal interpolation factor absolute error of2−4.An al-ternative to using specially designed processors for the implementation offiltered backprojection(FBP)is pre-sented in[5].In this work,a fast and direct FBP al-gorithm is implemented using texture-mapping hard-ware.It can perform parallel-beam backprojection of aParallel-Beam Backprojection 297512-by-512-pixel image from 804projections in 2.1sec,while our implementation takes 0.25sec for 1024projections.Luiz et al.[6]investigated residue number systems (RNS)for the implementation of con-volution based backprojection to speedup the process-ing.Unfortunately,extra binary-to-RNS and RNS-to-binary conversions are introduced.Other approaches to accelerating the backprojection algorithm have been investigated [7,8].One approach [7]presents an order O (n 2log n )and merits further study.The suitability to medical image quality and hardware implementation of these approaches[7,8]needs to be demonstrated.There are also a lot of interests in the area of fan-beam and cone-beam reconstruction using hardware implementa-tion.An FPGA-based fan-beam reconstruction module [9]is proposed and simulated using MAX +PLUS2,version 9.1,but no actual FPGA implementation is mentioned.Moreover,the authors did not explore the potential parallelism for different projections as we do,which is essential for speed-up.More data and com-putation is needed for 3D cone-beam FBP.Yu’s PC based system [10]can reconstruct the 5123data from 288∗5122projections in 15.03min,which is not suit-able for real-time.The embedded system described in [11]can do 3D reconstruction in 38.7sec with the fastest time reported in the literature.However,itisFigure 1.(a)Illustration of the coordinate system used in parallel-beam backprojection,and (b)geometric explanation of the incremental spatial address calculation.based on a Mercury RACE ++AdapDev 1120devel-opment workstation and need many modifications for a different platform.Bins et al.[12]have investigated precision vs.error in JPEG compression.The goals of this research are very similar to ours:to implement de-signs in fixed-point in order to maximize parallelism and area utilization.However,JPEG compression is an application that can tolerate a great deal more error than medical imaging.In the next section,we present the backprojection algorithm in more detail.In Section 3we present our quantization studies and analysis of error introduced.Section 4presents the hardware implementation in de-tail.Finally we present results and discuss future di-rections.An earlier version of this research was pre-sented [13].This paper provides a fuller discussion of the project and updated results.2.Parallel-Beam Filtered BackprojectionA parallel-beam CT scanning system uses an array of equally spaced unidirectional sources of focused X-ray beams.Generated radiation not absorbed by the object’s internal structure reaches a collinear array of detectors (Fig.1(a)).Spatial variation of the absorbed298Leeser et al.energy in the two-dimensional plane through the ob-ject is expressed by the attenuation coefficient µ(x ,y ).The logarithm of the measured radiation intensity is proportional to the integral of the attenuation coef-ficient along the straight line traversed by the X-ray beam.A set of values given by all detectors in the array comprises a one-dimensional projection of the attenu-ation coefficient,P (t ,θ),where t is the detector dis-tance from the origin of the array,and θis the angle at which the measurement is taken.A collection of pro-jections for different angles over 180◦can be visualized in the form of an image in which one axis is position t and the other is angle θ.This is called a sinogram or Radon transform of the two-dimensional function µ,and it contains information needed for the reconstruc-tion of an image µ(x ,y ).The Radon transform can be formulated aslog e I 0I d= µ(x ,y )δ(x cos θ+y sin θ−t )dx dy≡P (t ,θ)(1)where I 0is the source intensity,I d is the detected inten-sity,and δ(·)is the Dirac delta function.Equation (1)is actually a line integral along the path of the X-ray beam,which is perpendicular to the t axis (see Fig.1(a))at location t =x cos θ+y sin θ.The Radon transform represents an operator that maps an image µ(x ,y )to a sinogram P (t ,θ).Its inverse mapping,the inverse Radon transform,when applied to a sinogram results in an image.The filtered backprojection (FBP)algo-rithm performs this mapping [1].FBP begins by high-pass filtering all projections be-fore they are fed to hardware using the Ram-Lak or ramp filter,whose frequency response is |f |.The dis-crete formulation of backprojection isµ(x ,y )=πK Ki =1 θi(x cos θi +y sin θi ),(2)where θ(t )is a filtered projection at angle θ,and K is the number of projections taken during CT scanning at angles θi over a 180◦range.The number of val-ues in θ(t )depends on the image size.In the case of n ×n pixel images,N =√n D detectors are re-quired.The ratio D =d /τ,where d is the distance between adjacent pixels and τis the detector spac-ing,is a critical factor for the quality of the recon-structed image and it obviously should satisfy D >1.In our implementation,we utilize values of D ≈1.4and N =1024,which are typical for real systems.Higher values do not significantly increase the image quality.Algorithmically,Eq.(2)is implemented as a triple nested “for”loop.The outermost loop is over pro-jection angle,θ.For each θ,we update every pixel in the image in raster-scan order:starting in the up-per left corner and looping first over columns,c ,and next over rows,r .Thus,from (2),the pixel at loca-tion (r ,c )is incremented by the value of θ(t )where t is a function of r and c .The issue here is that the X-ray going through the currently reconstructed pixel,in general,intersects the detector array between detec-tors.This is solved by linear interpolation.The point of intersection is calculated as an address correspond-ing to detectors numbered from 0to 1023.The frac-tional part of this address is the interpolation factor.The equation that performs linear interpolation is given byint θ(i )=[ θ(i +1)− θ(i )]·I F + θ(i ),(3)where IF denotes the interpolation factor, θ(t )is the 1024element array containing filtered projection data at angle θ,and i is the integer part of the calculated address.The interpolation can be performed before-hand in software,or it can be a part of the backpro-jection hardware itself.We implement interpolation in hardware because it substantially reduces the amount of data that must be transmitted to the reconfigurable hardware board.The key to an efficient implementation of Eq.(2)is shown in Fig.1(b).It shows how a distance d between square areas that correspond to adjacent pixels can be converted to a distance t between locations where X-ray beams that go through the centers of these areas hit the detector array.This is also derived from the equa-tion t =x cos θ+y sin θ.Assuming that pixels are pro-cessed in raster-scan fashion,then t =d cos θfor two adjacent pixels in the same row (x 2=x 1+d )and sim-ilarly t =d sin θfor two adjacent pixels in the same column (y 2=y 1−d ).Our implementation is based on pre-computing and storing these deltas in look-up tables(LUTs).Three LUTs are used corresponding to the nested “for”loop structure of the backprojection algorithm.LUT 1stores the initial address along the detector axis (i.e.along t )for a given θrequired to update the pixel at row 1,column 1.LUT 2stores the increment in t required as we increment across a row.LUT 3stores the increment for columns.Parallel-Beam Backprojection299Figure2.Major simulation steps.3.QuantizationMapping the algorithm directly to hardware will not produce an efficient implementation.Several modifica-tions must be made to obtain a good hardware realiza-tion.The most significant modification is usingfixed-point arithmetic.For hardware implementation,narrow bit widths are preferred for more parallelism which translates to higher overall processing speed.How-ever,medical imaging requires high precision which may require wider bit widths.We did extensive analy-sis to optimize this tradeoff.We quantize all data and all calculations to increase the speed and decrease the re-sources required for implementation.Determining al-lowable quantization is based on a software simulation of the tomographic process.Figure2shows the major blocks of the simulation. An input image isfirst fed to the software implementa-tion of the Radon transform,also known as reprojection [14],which generates the sinogram of1024projections and1024samples per projection.Thefiltering block convolves sinogram data with the impulse response of the rampfilter generating afiltered sinogram,which is then backprojected to give a reconstructed image.All values in the backprojection algorithm are real numbers.These can be implemented as eitherfloating-point orfixed-point values.Floating-point represen-tation gives increased dynamic range,but is signifi-cantly more expensive to implement in reconfigurable hardware,both in terms of area and speed.For these reasons we have chosen to usefixed-point arithmetic. An important issue,especially in medical imaging,is how much numerical accuracy is sacrificed whenfixed-point values are used.Here,we present the methods used tofind appropriate bit-widths for maintaining suf-ficient numerical accuracy.In addition,we investigate possibilities for bit reduction on the outputs of certain functional units in the datapath for different rounding schemes,and what influence that has on the error intro-duced in reconstructed images.Our analysis shows that medical images display distinctive properties with re-spect to how different quantization choices affect their reconstruction.We exploit this and customize quan-tization to bestfit medical images.We compute the quantization error by comparing afixed-point image reconstruction with afloating-point one.Fixed-point variables in our design use a general slope/bias-encoding,meaning that they are represented asV≈V a=SQ+B,(4) where V is an arbitrary real number,V a is itsfixed-point approximation,Q is an integer that encodes V,S is the slope,and B is the bias.Fixed-point versions of the sinogram and thefiltered sinogram use slope/bias scaling where the slope and bias are calculated to give maximal precision.The quantization of these two vari-ables is calculated as:S=max(V)−min(V)max(Q)−min(Q)=max(V)−min(V)2,(5) B=max(V)−S·max(Q)orB=min(V)−S·min(Q),(6) Q=roundV−BS,(7)where ws is the word size in bits of integer Q.Here, max(V)and min(V)are the maximum and mini-mum values that V will take,respectively.max(V) was determined based on analysis of data.Since sinogram data are unsigned numbers,in this case min(V)=min(Q)=B=0.The interpolation factor is an unsigned fractional number and uses radix point-only scaling.Thus,the quantized interpolation factor is calculated as in Eq.(7),with saturation on overflow, with S=2−E where E is the number of fractional bits, and with B=0.For a given sinogram,S and B are constants and they do not show up in the hardware—only the quan-tized value Q is part of the hardware implementation. Note that in Eq.(3),two data samples are subtracted from each other before multiplication with the inter-polation factor takes place.Thus,in general,the bias B is eliminated from the multiplication,which makes quantization offiltered sinogram data with maximal precision scaling easily implementable in hardware.300Leeser etal.Figure 3.Some of the images used as inputs to the simulation process.The next important issue is the metric used for evalu-ating of the error introduced by quantization.Our goal was to find a metric that would accurately describe vi-sual differences between compared images regardless of their dynamic range.If 8-bit and 16-bit versions of a single image are reconstructed so that there is no vis-ible difference between the original and reconstructed images,the proper metric should give a comparable estimate of the error for both bit-widths.The proper metric should also be insensitive to the shift of pixel value range that can emerge for different quantization and rounding schemes.Absolute values of single pix-els do not effect visual image quality as long as their relative value is preserved,because pixel values are mapped to a set of grayscale values.The error metric we use that meets these criteria is the Relative Error (RE):RE = M i =1 (x i −¯x )− y F P i−¯y F P 2M i =1 y F P i−¯y F P ,(8)Here,M is the total number of pixels,x i and y F Pi are the values of the i -th pixel in the quantized and floating-point reconstructions respectively,and ¯x,¯y FP are their means.The mean value is sub-tracted because we only care about the relative pixel values.Figure 3shows some characteristic images from a larger set of 512-by-512-pixel images used as inputs to the simulation process.All images are monochrome 8-bit images,but 16-bit versions are also used in simu-lations.Each image was chosen for a certain reason.For example,the Shepp-Logan phantom is well known and widely used in testing the ability of algorithms to accu-rately reconstruct cross sections of the human head.It is believed that cross-sectional images of the human head are the most sensitive to numerical inaccuracies and the presence of artifacts induced by a reconstruction algo-rithm [1].Other medical images were Female,Head,and Heart obtained from the visible human web site [15].The Random image (a white noise image)should result in the upper bound on bit-widths required for a precise reconstruction.The Artificial image is unique because it contains all values in the 8-bit grayscale range.This image also contains straight edges of rect-angles,which induce more artifacts in the reconstructed image.This is also characteristic of the Head image,which contains a rectangular border around the head slice.Figure 4shows the detailed flowchart of the simu-lated CT process.In addition to the major blocks des-ignated as Reproject,Filter and Backproject,Fig.4also includes the different quantization steps that we have investigated.Each path in this flowchart rep-resents a separate simulation cycle.Cycle 1gives aParallel-Beam Backprojection301Figure 4.Detailed flowchart of the simulation process.floating-point (FP)reconstruction of an input image.All other cycles perform one or more type of quan-tization and their resulting images are compared to the corresponding FP reconstruction by computing the Relative Error.The first quantization step converts FP projection data obtained by the reprojection step to a fixed-point representation.Simulation cycle 2is used to determine how different bit-widths for quantized sino-gram data affect the quality of a reconstructed image.Our research was based on a prototype system that used 12-bit accurate detectors for the acquisition of sino-gram data.Simulations showed that this bit-width is a good choice since worst case introduced error amounts to 0.001%.The second quantization step performsthe Figure 5.Simulation results for the quantization of filtered sinogram data.conversion of filtered sinogram data from FP to fixed-point representation.Simulation cycle 3is used to find the appropriate bit-width of the words representing a filtered sinogram.Figure 5shows the results for this cycle.Since we use linear interpolation of projection values corresponding to adjacent detectors,the interpo-lation factor in Eq.(3)also has to be quantized.Figure 6summarizes results obtained from simulation cycle 4,which is used to evaluate the error induced by this quantization.Figures 5and 6show the Relative Error metric for different word length values and for different simula-tion cycles for a number of input images.Some input images were used in both 8-bit and 16-bit versions.302Leeser etal.Figure 6.Simulation results for the quantization of the interpolation factor.Figure 5corresponds to the quantization of filtered sinogram data (path 3in Fig.4).The conclusion here is that 9-bit quantization is the best choice since it gives considerably smaller error than 8-bit quantiza-tion,which for some images induces visible artifacts.At the same time,10-bit quantization does not give vis-ible improvement.The exceptions are images 2and 3,which require 13bits.From Fig.6(path 4in Fig.4),we conclude that 3bits for the interpolation factor (mean-ing the maximum error for the spatial address is 2−4)Figure 7.Relative error between fixed-point and floating-point reconstruction.is sufficiently accurate.As expected,image 1is more sensitive to the precision of the linear interpolation be-cause of its randomness.Figure 7shows that combining these quantization schemes results in a very small error for image “Head”in Fig.3.We also investigated whether it is feasible to discard some of the least significant bits (LSBs)on outputs of functional units (FUs)in the datapath and still not introduce any visible artifacts.The goal is for the re-constructed pixel values to have the smallest possibleParallel-Beam Backprojection 303bit-widths.This is based on the intuition that bit re-duction done further down the datapath will introduce a smaller amount of error in the result.If the same bit-width were obtained by simply quantizing filtered projection data with fewer bits,the error would be mag-nified by the operations performed in the datapath,es-pecially by the multiplication.Path number 5in Fig.4depicts the simulation cycles that investigates bit reduc-tion at the outputs of three of the FUs.These FUs imple-ment subtraction,multiplication and addition that are all part of the linear interpolation from Eq.(3).When some LSBs are discarded,the remaining part of a binary word can be rounded in different ways.We investigate two different rounding schemes,specifically rounding to nearest and truncation (or rounding to floor).Round-ing to nearest is expected to introduce the smallest er-ror,but requires additional logic resources.Truncation has no resource requirements,but introduces a nega-tive shift of values representing reconstructed pixels.Bit reduction effectively optimizes bit-widths of FUs that are downstream in the data flow.Figure 8shows tradeoffs of bit reduction and the two rounding schemes after multiplication for medi-cal images.It should be noted that sinogram data are quantized to 12bits,filtered sinogram to 9bits,and the interpolation factor is quantized to 3bits (2−4pre-cision).Similar studies were done for the subtraction and addition operations and on a broader set of im-ages.It was determined that medical images suffer the least amount of error introduced by combining quanti-zations and bit reduction.For medical images,in case of rounding to nearest,there is very little difference inthe Figure 8.Bit reduction on the output of the interpolation multiplier.introduced error between 1and 3discarded bits after multiplication and addition.This difference is higher in the case of bit reduction after addition because the multiplication that follows magnifies the error.For all three FUs,when only medical images are considered,there is a fixed relationship between rounding to near-est and truncation.Two least-significant bits discarded with rounding to nearest introduce an error that is lower than or close to the error of 1bit discarded with trun-cation.Although rounding to nearest requires logic re-sources,even when only one LSB is discarded with rounding to nearest after each of three FUs,the overall resource consumption is reduced because of savings provided by smaller FUs and pipeline registers (see Figs.11and 12).Figure 9shows that discarding LSBs introduces additional error on medical images for this combination of quantizations.In our case there was no need for using bit reduction to achieve smaller resource consumption because the targeted FPGA chip (Xilinx Virtex1000)provided sufficient logic resources.There is one more quantization issue we considered.It pertains to data needed for the generation of the ad-dress into a projection array (spatial address addr )and to the interpolation factor.As described in the intro-duction,there are three different sets of data stored in look-up tables (LUTs)that can be quantized.Since pixels are being processed in raster-scan order,the spa-tial address addr is generated by accumulating entries from LUTs 2and 3to the corresponding entry in LUT 1.The 10-bit integer part of the address addr is the index into the projection array θ(·),while its fractional part is the interpolation factor.By using radix point-only。
第四届国际高光谱影像与信号处理学术研讨会(WHISPERS2012)

第四届国际高光谱影像与信号处理学术研讨会(WHISPERS 2012)4th Workshop on Hyperspectral Image and Signal Processing:Evolution in Remote Sensing2012年6月4日-7日,中国,上海会议网址:/ /WHISPERS研讨会旨在汇聚从事高光谱数据处理与应用的专业人员开展学术交流。
“高光谱数据”意指:----信号:由光谱仪直接提供的未经成像的信号;----影像:从地面成像光谱仪、到航空或卫星传感器获取的影像、到太空高光谱影像。
----模型:传感器或感知场景的模型,包括物理模型。
“处理”意指从数据获取、定标到分析的所有操作(图象处理、信号处理、几何和辐射处理、特征提取、降维、混合像元分解、源信号分离、分类、异常检测、定量参数反演等),尤其欢迎围绕以下方向的最新研究成果进行投稿:----光谱仪与高光谱传感器设计与定标----物理建模和物理分析----噪声估计和去除----降维与特征提取----混合像元分解,源信号分离,端元提取----分类与分割----高性能计算与数据压缩WHISPERS会议欢迎面向“应用”的论文,涉及到光谱学应用的不同方向,包括:----航空与卫星遥感对地观测(airborne and satellite remote sensing for Earth observation)----环境与污染监测(monitoring of the environment, pollution)----精细农林业(precision agriculture and forestry)----化学(chemistry)----生物与医学影像(biomedical imagery )----国防应用(defense application)----工业监视(industrial inspection)----天文物理学(astrophysics)----地质与矿产勘探(geology and mineral exploration)----食品安全(food security)重要日期2012年2月15日4页全文投稿(请从网站下载模板)2012年3月35日录用通知2012年4月15日最终稿提交2012年5月1日提前注册结束2012年6月4日会议注册,培训(P rofessor Antonio J. Plaza, S pectral Unmixing of Hyperspectral Data Dr. Xiuping Jia , Feature Mining from Hyperspectral Data)2012年6月5日-7日会议召开4th Workshop on Hyperspectral Image and Signal Processing:Evolution in Remote Sensing2012年6月4日-7日,中国,上海/ /The aim of this workshop is to bring together all the people involved in hyperspectral data processing, generally speakingBy "data", we mean:- signals, as provided by spectrometers and processed individually- images, from the ground using microscopes and imaging spectrometers to airborne or satellite sensors, up to astrophysical data- models: models of the sensors or of the sensed scene, including physical considerationsBy "processing", we mean everything from the acquisition, the calibration to the analysis (image processing, signal processing, feature extraction, dimension reduction, unmixing and source separation, classification, anomaly detection, quantitative inversion, data fusion, and so on).People are invited to submit new research results on the following suggested topics:- Spectrometers and hyperspectral sensors: design and calibration- Physical modeling, physical analysis- Noise estimation and reduction- Dimension reduction- Unmixing, source separation, endmember extraction- Segmentation, classification- Anomaly detection- Quantitative inversion- Fusion of hyperspectral data with other modality data (e.g., LiDAR, SAR, etc.)- High performance computing and compressionApplications oriented papers are also welcome. As a matter of fact, spectrometry is now used in a wide range of domains, including:- airborne and satellite remote sensing-based Earth observation- monitoring of the environment, pollution- precision agriculture and forestry- chemistry- biomedical imagery- defense application- industrial inspection- astrophysics- geology and mineral exploration- food security。
Signal and Image Processing
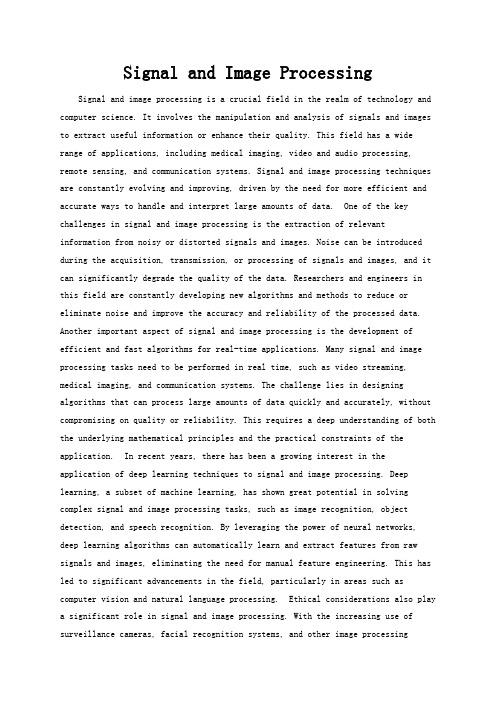
Signal and Image Processing Signal and image processing is a crucial field in the realm of technology and computer science. It involves the manipulation and analysis of signals and images to extract useful information or enhance their quality. This field has a wide range of applications, including medical imaging, video and audio processing, remote sensing, and communication systems. Signal and image processing techniques are constantly evolving and improving, driven by the need for more efficient and accurate ways to handle and interpret large amounts of data. One of the key challenges in signal and image processing is the extraction of relevant information from noisy or distorted signals and images. Noise can be introduced during the acquisition, transmission, or processing of signals and images, and it can significantly degrade the quality of the data. Researchers and engineers in this field are constantly developing new algorithms and methods to reduce or eliminate noise and improve the accuracy and reliability of the processed data. Another important aspect of signal and image processing is the development of efficient and fast algorithms for real-time applications. Many signal and image processing tasks need to be performed in real time, such as video streaming, medical imaging, and communication systems. The challenge lies in designing algorithms that can process large amounts of data quickly and accurately, without compromising on quality or reliability. This requires a deep understanding of both the underlying mathematical principles and the practical constraints of the application. In recent years, there has been a growing interest in theapplication of deep learning techniques to signal and image processing. Deep learning, a subset of machine learning, has shown great potential in solving complex signal and image processing tasks, such as image recognition, object detection, and speech recognition. By leveraging the power of neural networks, deep learning algorithms can automatically learn and extract features from raw signals and images, eliminating the need for manual feature engineering. This has led to significant advancements in the field, particularly in areas such as computer vision and natural language processing. Ethical considerations also play a significant role in signal and image processing. With the increasing use of surveillance cameras, facial recognition systems, and other image processingtechnologies, there are concerns about privacy, surveillance, and the potential misuse of these technologies. It is essential for researchers and engineers inthis field to consider the ethical implications of their work and ensure thattheir technologies are used responsibly and in compliance with privacy and data protection laws. In conclusion, signal and image processing is a dynamic and challenging field with a wide range of applications and implications. The ongoing advancements in this field are driven by the need for more efficient and accurate ways to handle and interpret large amounts of data, as well as the increasing use of deep learning techniques and the ethical considerations surrounding the use of these technologies. As technology continues to evolve, signal and image processing will remain a critical area of research and development, with the potential to revolutionize various industries and improve the quality of life for people around the world.。
Signal Processing and Linear Systems
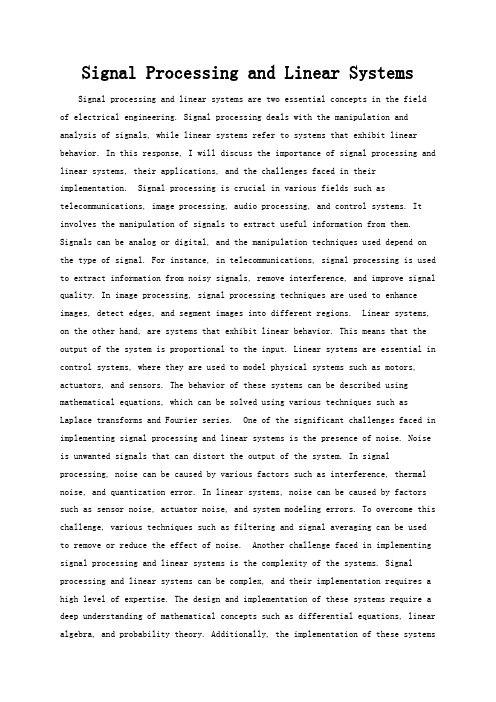
Signal Processing and Linear Systems Signal processing and linear systems are two essential concepts in the field of electrical engineering. Signal processing deals with the manipulation and analysis of signals, while linear systems refer to systems that exhibit linear behavior. In this response, I will discuss the importance of signal processing and linear systems, their applications, and the challenges faced in their implementation. Signal processing is crucial in various fields such as telecommunications, image processing, audio processing, and control systems. It involves the manipulation of signals to extract useful information from them. Signals can be analog or digital, and the manipulation techniques used depend on the type of signal. For instance, in telecommunications, signal processing is used to extract information from noisy signals, remove interference, and improve signal quality. In image processing, signal processing techniques are used to enhance images, detect edges, and segment images into different regions. Linear systems, on the other hand, are systems that exhibit linear behavior. This means that the output of the system is proportional to the input. Linear systems are essential in control systems, where they are used to model physical systems such as motors, actuators, and sensors. The behavior of these systems can be described using mathematical equations, which can be solved using various techniques such as Laplace transforms and Fourier series. One of the significant challenges faced in implementing signal processing and linear systems is the presence of noise. Noise is unwanted signals that can distort the output of the system. In signal processing, noise can be caused by various factors such as interference, thermal noise, and quantization error. In linear systems, noise can be caused by factors such as sensor noise, actuator noise, and system modeling errors. To overcome this challenge, various techniques such as filtering and signal averaging can be used to remove or reduce the effect of noise. Another challenge faced in implementing signal processing and linear systems is the complexity of the systems. Signal processing and linear systems can be complex, and their implementation requires a high level of expertise. The design and implementation of these systems require a deep understanding of mathematical concepts such as differential equations, linear algebra, and probability theory. Additionally, the implementation of these systemsrequires specialized software tools, such as MATLAB, which can be expensive and require extensive training. Despite the challenges, signal processing and linear systems have numerous applications in various fields. In telecommunications, signal processing is used to improve signal quality, remove interference, and detect errors. In control systems, linear systems are used to model physical systems such as motors, actuators, and sensors. In image and audio processing, signal processing techniques are used to enhance images and audio quality. In biomedical engineering, signal processing is used to analyze biological signals such as EEG and ECG signals. In conclusion, signal processing and linear systems are essential concepts in electrical engineering. They have numerous applications in various fields and are crucial in the design and implementation of various systems. However, their implementation requires a high level of expertise and specialized software tools. The challenges faced in implementing these systems include the presence of noise and the complexity of the systems. Despite these challenges, signal processing and linear systems remain crucial in various fields and will continue to play a significant role in the advancement of technology.。
- 1、下载文档前请自行甄别文档内容的完整性,平台不提供额外的编辑、内容补充、找答案等附加服务。
- 2、"仅部分预览"的文档,不可在线预览部分如存在完整性等问题,可反馈申请退款(可完整预览的文档不适用该条件!)。
- 3、如文档侵犯您的权益,请联系客服反馈,我们会尽快为您处理(人工客服工作时间:9:00-18:30)。
* Some useful references for this tutorial:
(2) “Information Processing for Remote Sensing”, edited by C.H. Chen World Scientific Publishing, 1999. (981-02-3737-5) (3) “Frontiers of Remote Sensing Information Processing”, edited by C.H. Chen, World Scientific Publishing 2003. (981-238-344-10-1)
Signal and Image Processing for Remote Sensing
Prof. C.H. Chen Univ. of Massachusetts Dartmouth Electrical and Computer Engineering Dept. N. Dartmouth, MA 02747 USA
7
Performance Comparison of Principal Components Transforms
“Radiance Reconstruction”
“Temperature Profile Estimation”
8
Some references on PCA in remote sensing
1. J.B. Lee, A.S. Woodyatt and M. Berman, “Enhancement of high spectral resolution remote sensing data by a noise adjusted principal component transform”, IEEE Trans. on Geoscience and Remote Sensing, vol. 28, pp. 295-304, May 1990. 2. W.J. Blackwell, “Retrieval of cloud-cleared atmospheric temperature profiles for hyperspectral infrared and microwave observations”, Ph.D. dissertation, EECS Dept., MIT, June 2002. 3. W.J. Blackwell, “Retrieval of atmospheric profiles form hyperspectral sounding data using PCA and a neural network”, Technical talk given at University of Pittsburgh ECE Seminar, Feb. 27, 2008.
9
(Left) AVIRIS RGB image for the Linden, CA scene collected on 20-Aug-1992, denoting location of various features of interest and (Right) a plot of the spectral distribution of the apparent reflectance for those features.
6
Comments on PCA and related transforms
* PC Transform relies on the covariance matrix estimated from data available. In the presence of noise, the covariance matrix is the sum of the noise free covariance and the noise covariance. The coefficients of the PC transform components are statistically uncorrelated. The reduced rank reconstruction error is minimized with respect to the data. * NAPC Transform requires a good knowledge of the noise statistics which often cannot be estimated accurately. * PPC reconstruction of noise free data yields lower distortion (i.e. reconstruction error) than the PC and NAPC Transforms. The next slide on PC transforms performance comparison is from Dr. Balckwell in his talk at the Univ. of Pittsburgh.
10
The 1st, 2nd and 5th principal components of AVIRIS data for the Linden scene. It is apparent that the first two components contain background and the 5th component shows an anomaly. HSI data (Hsu, et al. 2003)
(1) “Signal and Image Processing for Remote Sensing”, edited by C.H. Chen, CRC Press, 2006. (0-8453-5091-3). Referred to as the Book.
This tutorial is based mainly on this book. Split volume books 2007: Signal Processing for Remote Sensing (ISBN 1-42006666-8), Image Processing for Remote Sensing (ISBN1-4200-6664-1)
cchen@
IGARSS2008 Tutorial, July 6, 2008 in Boston
Introduction:
•
Objective of the Tutorial: to introduce the image and signal processing as well as pattern recognition algorithms in remote sensing.
Acknowledgement: I thank all authors of the book chapters of the three books listed above for the use of their materials in this tutorial. My special thanks go to Dr. Blackwell, Dr. Escalante, Dr. Long, Dr. Moser, Dr. Nasrabadi and Dr. Serpico for the use of their power points in this tutorial.
Part 1: PCA, ICA and Related Transforms
* Definition: y = Vx; V = [ v1, v2 , …, vn ]
V is usually an orthogonal matrix for linear transforms. The reconstruction error is minimized such as in PCA. Data reconstruction (m<n): i 1 • Let yi be an element of y. In a non-linear transform, replace yi by a function of yi, gi (yi).
3
Outline:
* * * * Part 1: PCA, ICA and Related Transforms Part 2: Change Detection for SAR Imagery Part 3a: The Classification Problems Part 3b: The Classification Problems continued * Part 4: Contextual Classification in Remote Sensing * Part 5: Other topics
1st PC (Clouds/background)
2nd PC (Hot area)
5th PC (Fire)
11
Classification (by visual identification) result using the 1st, 2nd and 5th principal components. All major atmospheric and surface features are identified as to
Apparent Reflectance
1.0
Smoke (sm. part.) Smoke (lg. part.) Shadow
Grass Fire Hot Area Cloud Soilຫໍສະໝຸດ 0.1Smoke -
small part.
400
700
1000
1300
1600
1900
2200
2500
Wavelength (nm)