An Iterative Receiver Algorithm for Space-Time Encoded Signals
latex英文模板
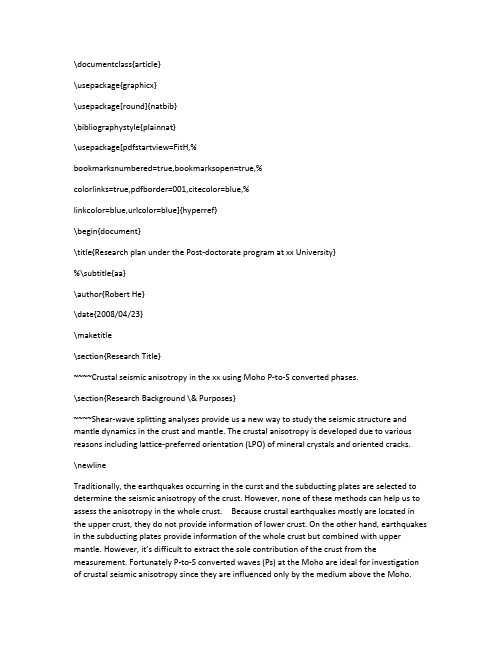
\documentclass{article}\usepackage{graphicx}\usepackage[round]{natbib}\bibliographystyle{plainnat}\usepackage[pdfstartview=FitH,%bookmarksnumbered=true,bookmarksopen=true,%colorlinks=true,pdfborder=001,citecolor=blue,%linkcolor=blue,urlcolor=blue]{hyperref}\begin{document}\title{Research plan under the Post-doctorate program at xx University}%\subtitle{aa}\author{Robert He}\date{2008/04/23}\maketitle\section{Research Title}~~~~Crustal seismic anisotropy in the xx using Moho P-to-S converted phases.\section{Research Background \& Purposes}~~~~Shear-wave splitting analyses provide us a new way to study the seismic structure and mantle dynamics in the crust and mantle. The crustal anisotropy is developed due to various reasons including lattice-preferred orientation (LPO) of mineral crystals and oriented cracks.\newlineTraditionally, the earthquakes occurring in the curst and the subducting plates are selected to determine the seismic anisotropy of the crust. However, none of these methods can help us to assess the anisotropy in the whole crust. Because crustal earthquakes mostly are located in the upper crust, they do not provide information of lower crust. On the other hand, earthquakes in the subducting plates provide information of the whole crust but combined with upper mantle. However, it’s difficult to extract the sole contri bution of the crust from the measurement. Fortunately P-to-S converted waves (Ps) at the Moho are ideal for investigation of crustal seismic anisotropy since they are influenced only by the medium above the Moho.Moho. Figure \ref{crustalspliting}~schematically shows the effects of shear wave splitting on Moho Ps phases. Initially, a near-vertically incident P wave generates a radially polarized converted shear wave at the crust-mantle boundary. The phases, polarized into fast and slow directions, progressively split in time as they propagate through the anisotropic media. Here, the Ps waves can be obtained from teleseismic receiver function analysis.%%\begin{figure}[htbp]\begin{center}\includegraphics[width=0.47\textwidth]{crustalsplit.png}\caption{The effects of shear wave splitting in the Moho P to S converted phase. Top shows a schematic seismogram in the fast/slow coordinate system with split horizontal Ps components.(cited from: McNamara and Owens, 1993)}\label{crustalspliting}\end{center}\end{figure}%%The Korean Peninsula is composed of three major Precambrian massifs, the Nangrim, Gyeongii, and Yeongnam massifs(Fig.\ref{geomap}). The Pyeongbuk-Gaema Massif forms the southern part of Liao-Gaema Massif of southern Manchuria, and the Gyeonggi and Mt. Sobaeksan massifs of the peninsula are correlated with the Shandong and Fujian Massifs of China.%\begin{figure}[htbp]\begin{center}\includegraphics[width=0.755\textwidth]{geo.png}\caption{Simplified geologic map. NCB: North China block; SCB: South China block.(cited from: Choi et al., 2006)}\label{geomap}\end{center}\end{figure}%Our purpose of the study is to measure the shear wave splitting parameters in the crust of the Korean Peninsula. The shear wave splitting parameters include the splitting time of shear energybetween the fast and slow directions, as well as fast-axis azimuthal direction in the Korean Peninsula. These two parameters provide us constraints on the mechanism causing the crustal anisotropy. From the splitting time, the layer thickness of anisotropy will be estimated. Whether crustal anisotropy mainly contributed by upper or lower crustal or both will be determined. Based on the fast-axis azimuthal direction, the tectonic relation between northeastern China and the Korean peninsula will be discussed.\section{Research Methods}~~~~Several methods have been introduced for calculation of receiver functions. An iterative deconvolution technique may be useful for this study since it produces more stable receiver function results than others. The foundation of the iterative deconvolution approach is aleast-squares minimization of the difference between the observed horizontal seismogram and a predicted signal generated by the convolution of an iteratively updated spike train with the vertical-component seismogram. First, the vertical component is cross-correlated with the radial component to estimate the lag of the first and largest spike in the receiver function (the optimal time is that of the largest peak in the absolute sense in the cross-correlation signal). Then the convolution of the current estimate of the receiver function with the vertical-component seismogram is subtracted from the radial-component seismogram, and the procedure is repeated to estimate other spike lags and amplitudes. With each additional spike in the receiver function, the misfit between the vertical and receiver-function convolution and the radial component seismogram is reduced, and the iteration halts when the reduction in misfit with additional spikes becomes insignificant.\newlineFor all measurement methods of shear-wave splitting, time window of waveform should be selected. Conventionally the shear-wave analysis window is picked manually. However, manual window selection is laborious and also very subjective; in many cases different windows give very different results.\newlineIn our study, the automated S-wave splitting technique will be used, which improves the quality of shear-wave splitting measurement and removes the subjectivity in window selection. First, the splitting analysis is performed for a range of window lengths. Then a cluster analysis isapplied in order to find the window range in which the measurements are stable. Once clusters of stable results are found, the measurement with the lowest error in the cluster with the lowest variance is presented for the analysis result.\section{Expected results \& their contributions}~~~~First, the teleseismic receiver functions(RFs) of all stations including radial and transverse RFs can be gained. Based on the analysis of RFs, the crustal thickness can be estimated in the Korean Peninsula. Then most of the expected results are the shear-wave splitting parameters from RFs analysis in the crust beneath the Korean Peninsula. The thickness of anisotropic layer will be estimated in the region when the observed anisotropy is assumed from a layer of lower crustal material.All the results will help us to understand the crustal anisotropy source.\newlineCrustal anisotropy can be interpreted as an indicator of the crustal stress/strain regime. In addition, since SKS splitting can offer the anisotropy information contributed by the upper mantle but combined with the crust, the sole anisotropy of the upper mantle can be attracted from the measurement of SKS splitting based on the crustal splitting result.%\cite{frogge2007}%%%\citep{frogge2008}%%%\citep{s-frogge2007}% 5. References\begin{thebibliography}{99}\item Burdick, L. J. and C. A. Langston, 1977, Modeling crustal structure through the use of converted phases in teleseismic body waveforms, \textit{Bull. Seismol. Soc. Am.}, 67:677-691.\item Cho, H-M. et al., 2006, Crustal velocity structure across the southern Korean Peninsula from seismic refraction survey, \textit{Geophy. Res. Lett.} 33, doi:10.1029/2005GL025145.\item Cho, K. H. et al., 2007, Imaging the upper crust of the Korean peninsula by surface-wave tomography, \textit{Bull. Seismol. Soc. Am.}, 97:198-207.\item Choi, S. et al., 2006, Tectonic relation between northeastern China and the Korean peninsula revealed by interpretation of GRACE satellite gravity data, \textit{Gondwana Research}, 9:62-67.\item Chough, S. K. et al., 2000, Tectonic and sedimentary evolution of the Korean peninsula: a review and new view, \textit{Earth-Science Reviews}, 52:175-235.\item Crampin, S., 1981, A review of wave motion in anisotropic and cracked elastic-medium, \textit{Wave Motion}, 3:343-391.\item Fouch, M. J. and S. Rondenay, 2006, Seismic anisotropy beneath stable continental interiors, \textit{Phys. Earth Planet. Int.}, 158:292-320.\item Herquel, G. et al., 1995, Anisotropy and crustal thickness of Northern-Tibet. New constraints for tectonic modeling, \textit{Geophys. Res. Lett.}, 22(14):1 925-1 928.\item Iidaka, T. and F. Niu, 2001, Mantle and crust anisotropy in the eastern China region inferred from waveform splitting of SKS and PpSms, \textit{Earth Planets Space}, 53:159-168.\item Kaneshima, S., 1990, Original of crustal anisotropy: Shear wave splitting studies in Japan, \textit{J. Geophys. Res.}, 95:11 121-11 133.\item Kim, K. et al., 2007, Crustal structure of the Southern Korean Peninsula from seismic wave generated by large explosions in 2002 and 2004, \textit{Pure appl. Geophys.}, 164:97-113.\item Kosarev, G. L. et al., 1984, Anisotropy of the mantle inferred from observations of P to S converted waves, \textit{Geophys. J. Roy. Astron. Soc.}, 76:209-220.\item Levin, V. and J. Park, 1997, Crustal anisotropy in the Ural Mountains foredeep from teleseismic receiver functions, \textit{Geophys. Res. Lett.}, 24(11):1 283 1286.\item Ligorria, J. P. and C. J. Ammon, 1995, Iterative deconvolution and receiver-function estimation. \textit{Bull. Seismol. Soc. Am.}, 89:1 395-1 400.\item Mcnamara, D. E. and T. J. Owens, 1993, Azimuthal shear wave velocity anisotropy in the basin and range province using Moho Ps converted phases, \textit{J. Geophys. Res.}, 98:12003-12 017.\item Peng, X. and E. D. Humphreys, 1997, Moho dip and crustal anisotropy in northwestern Nevada from teleseismic receiver functions, \textit{Bull. Seismol. Soc. Am.}, 87(3):745-754.\item Sadidkhouy, A. et al., 2006, Crustal seismic anisotropy in the south-central Alborz region using Moho Ps converted phases, \textit{J. Earth \& Space Physics}, 32(3):23-32.\item Silver, P. G. and W. W. Chan, 1991, Shear wave splitting and subcontinental mantle deformation, \textit{J. Geophys. Res.},96:16 429-16454.\item Teanby, N. A. et al., 2004, Automation of shear wave splitting measurement using cluster analysis, \textit{Bull. Seismol. Soc. Am.}, 94:453-463.\item Vinnik, L. and J-P. Montagner, 1996, Shear wave splitting in the mantle Ps phases,\textit{Geophys. Res. Lett.}, 23(18):2 449- 2 452.\item Yoo, H. J. et al., 2007, Imaging the three-dimensional crust of the Korean peninsula by joint inversion of surface-wave dispersion of teleseismic receiver functions, \textit{Bull. Seismol. Soc. Am.}, 97(3):1 002-1 011.\item Zhu, L., and H. Kanamori, 2000, Moho depth variation in Southern California from teleseismic receiver functions, \textit{J. Geophys. Res.}, doi :10.1029/1999JB900322, 105:2 969-2 980.%%%%\end{document}。
英语学术论文写作

*
instances will be regarded as the same class
and be connected with the same weight,
which in turn pulls these missing instances
together in the low-dimensional subspace
●*
T
H
A
N
K
Y
O
U
The proposed method significantly outperforms the other methods on the above multi-view databases with all kinds of incomplete cases. For instance, on the handwritten digit database (Table II), the proposed method achieves 3% and 6% improvement of ACC and NMI in comparison with the second best method. 【Clarifying experimental results and analyze the results by giving an example. 】
Example two
*
Complexity
Academic writing is gramatically more complex than other forms of writing.
Inspired by this motivation, Gao et al. [30]
*
WRCP

Achieving fast convergence for max-min fair rate allocation in Wireless Sensor NetworksAvinash Sridharan and Bhaskar Krishnamachari{asridhar,bkrishna}@Dept.of Electrical Engineering,University of Southern CaliforniaABSTRACTThe state of the art congestion control algorithms for wire-less sensor networks respond to coarse-grained feedback re-garding available capacity in the network with an additive in-crease multiplicative decrease mechanism to set source rates. Providing precise feedback is challenging in wireless net-works because link capacities vary with traffic on interfering links.We address this challenge by applying a receiver ca-pacity model that associates capacities with nodes instead of links,and use it to develop an iterative algorithm that prov-ably converges to lexicographic max-min fair rate allocation. We use this algorithm as the basis for the design and imple-mentation of thefirst explicit and precise distributed rate-based congestion control protocol for wireless sensor net-works—the wireless rate control protocol(WRCP),which can operate in asynchronous networks with dynamic traffic conditions.We show through extensive results from experi-ments that WRCP offers substantial improvements over the state of the art inflow completion times as well as in end-to-end packet delays.1.INTRODUCTIONIn event driven sensor networks,one of the primary modes of operations is for nodes to remain dormant for long dura-tions,and becoming active for short durations on sensing an event.In order to reduce the lag between sensing at the edges and detection at the base station,it is imperative to get the sensed data efficiently to base station within the uptime of the network.The duration of uptime in these networks has significant ramifications on the network life time.Apart from event driven sensor networks,networks that are con-tinuously monitoring the environment need to employ duty cycling when they require to achieve network life times to the order of years.For such networks,data sensed during the dormant phase is stored on boardflash,bulk data trans-fer then takes place during the periodic wake up phase of the network.A characteristic of sensor networks in general, is the low data rates that these networks operate at(∼250 kbps/sec).Due to interference,even in networks that are as small as20nodes,the per node available rate is to the order of2pkts/sec(where the packet size is as small as40 bytes).Given the constrained bandwidth resources available,for these class of sensor networks which need to maintain small uptime,it is imperative to have rate control mecha-nisms for fast and efficient delivery of data.In the absence of rate control mechanisms the networks might experience congestion collapse if nodes are greedily aggressive in try-ing to minimize their up time.The occurrence of congestion collapse in event driven systems in the absence of rate con-trol mechanisms has been highlighted in[19]and[18].Fur-ther,for these class of networks,given the requirement of maintaining short uptime,it is important that rate control al-gorithms are responsive and hence exhibit fast convergence times allowing the sources to send at the achievable rate as fast as possible.In wireless sensor networks the philosophy of performing congestion control has largely been based on a router-centric approach which uses explicit congestion feedback from in-termediate nodes.However,existing schemes still assume a lack of knowledge of achievable network capacity.The key reason has been the difficulty in computing capacity given that the bandwidth of each link is affected by interference from other links in its vicinity.The core AIMD mechanism is therefore a component of nearly all distributed conges-tion control protocols proposed for wireless sensor networks (ARC[25],CODA[23],FUSION[12],IFRC[19]).AIMD-based schemes have the advantage that the proto-col is agnostic to the underlying link layer,allowing for mod-ular protocol design.They can also be designed to guaran-tee efficient and fair rate allocation in steady state.However, AIMD mechanisms take a long time to converge and can ex-hibit long queue backlogs as the rates constantly exceed the available capacity(which is required to obtain a signal from the network that it is time to cut back).This is illustrated in Figure1,which presents the performance of IFRC[19], the state of the art for congestion control in wireless sensor networks,in a simple4-node experiment(see Figure6).In this particular example it can be observed that the rate allo-cation takes more than300seconds to converge,and queue sizes routinely reach8-10packets.We therefore believe that a fresh look at the problem of rate control design for sensor networks is required,with emphasis on convergence times and end-to-end packet delays.Our principal contribution in this work is the design of aFigure1:The behavior of allocated rate and queue back logs for IFRC.distributed rate control protocol,that we refer to as the Wire-less Rate Control Protocol(WRCP),which uses an ap-proximation of the available capacity in order to provide ex-plicit and precise feedback to sources.This approximation is obtained by exploiting knowledge of the performance of the underlying CSMA MAC protocol.The key idea in our ap-proach is to associate a constant capacity with nodes instead of links.The gains of this approach in terms of convergence times(few tens of seconds for WRCP as compared to hun-dreds of seconds for IFRC)and smaller queue back logs are highlighted in Figure2.The fast convergence times trans-lates to fastflow completion times and the reduced queue size improves end-to-end packet delays.The rest of the paper is organized as follows.First,in section2,we present a useful notion of capacity in a wire-less sensor network operating a CSMA protocol,which is referred to as receiver capacity.This determines the con-straints that define an achievable rate region forflows in a collection tree.In Section3we describe a idealized syn-chronous algorithm,which acts as the motivation for the WRCP protocol,and which provably converges to a lexico-graphic max-min fair rate allocation under this receiver ca-pacity ing the intuition presented by the idealized algorithm(section3)we design the Wireless Rate Control Protocol,presented in Section4,which handles real-world concerns of asynchrony,traffic-dynamics and stochastic in the underlying network,link and physical layers.We then describe the software architecture of WRCP(in Section5), which is designed to be integrated easily with the TinyOS2.x collection tree architecture for wireless sensor networks.We undertake an experimental evaluation of WRCP on Tmote Sky devices using the IEEE802.15.4-compliant CC2420ra-dios.We give details of our experimental methodology in Section6.We then compare our protocol extensively over both static and dynamic-traffic scenarios with IFRC,the state of the art distributed rate control protocol for wireless sensor networks,over a20-node experimental testbed(Section7). The results show substantial improvements inflowcomple-Figure2:The behavior of allocated rate,queue back logs for WRCP.tion times and end-to-end packet delays.We place our con-tributions in light of prior work in Section8,and present concluding comments on future work in Section9.2.RECEIVER CAPACITYThe primary requirement for designing an explicit and precise rate control algorithm is a usable notion of achiev-able capacity.In traditional wired networks,the notion of capacity is associated with a link existing between any two nodes.Allflows traversing the link are assumed to linearly share the constant capacity of the link.In wireless networks the capacity of a link is not constant,but rather affected by activity on interfering links in its vicinity.We therefore need to redefine the notion of capacity.Each node can be perceived as having a receiver domain consisting of all transmitting nodes within range,including itself.The crux of our approach is to associate the concept of capacity with nodes instead of links,we refer to this as receiver capacity.This capacity is to be shared linearly by allflows traversing the corresponding receiver’s domain.Al-though in general the region of achievable rates in a given re-ceiver domain is not linear,we approximate it with a linear rate region by making the receiver capacity a constant that depends only upon on the number of neighboring nodes(not their rates).Although we believe on intuitive grounds that this is a good approximation for wireless sensor networks due to the small packet sizes(≈40bytes)that are prevalent in such networks,we do not prove here rigorously how good this approximation is.We rely on the empirical results pre-sented in our evaluation of WRCP which is designed based on this approximation,to show that it is extremely useful in practice.Most sensor networks today use a randomized CSMA MAC as the de facto data link layer.In order to associate a value to the receiver capacity,we equate the capacity of a receiver with the saturation throughput of the CSMA MAC.The sat-uration throughput[5]of a CSMA MAC is defined as the throughput observed by the receiver when all senders are10 20 30 40 50 60 70 80 90 100 110 120 130 140 150 160 170 180 190 200 1 2 3 4 5 6 7 8 9 10 11 12 13 14 15 16 17 18 19 20 21 22 23 24 25S a t u r a t i o n T h r o u g h p u t (P k t s /s e c )Number of SendersFigure 3:Saturation throughput for multi-ple senders for the CC2420CSMA MAC on TinyOS-2.0.2.2backlogged and are within each others interference range.While this does not cover all possible sender-configurations possible in a receiver domain,our experience with WRCP shows that although this estimate is potentially conservative,it leads to equal or better performance in terms of achievable goodput compared to the state of the art.Our implementation is performed on the Tmote sky mote [1],which use the CC2420radios,running TinyOS-2.0.2.2.Fig-ure 3presents the empirically measured saturation through-put for this platform as the number of senders in-range is varied.This graph allows us to associate a value with the receiver capacity for each node in a WSN collection ing the notion of receiver capacity,we can determine constraints on rate allocation to flows in a WSN collection tree.Let N i be the set of all neighbors of i (consisting of i itself,all its immediate children,and all other nodes in its interference-range);C i the set denoting the subtree rooted at i (including itself);r i the rate at which data generated at source node i is being transmitted;and B i the value of node i ’s receiver capacity.The receiver capacity constraint at a node i is then given as follows:j ∈N ik ∈C jr k ≤B i (1)We explain this with an example.Figure 4shows an 8node topology.The solid lines indicate a parent-child rela-tionship in the tree.The dashed lines are interference links.Rates indicated on interference links 1quantify the amount of interference generated by a neighboring node when it is transmitting data to its parent.Thus,when node 2sends its data to node 1at some rate,node 2not only consumes the corresponding amount of capacity at node 1but also at node 3;the rate label on interference link 2→3is the same as that on link 2→1.Based on our model,the constraint on the rates at node 3would be as follows:r tot 2+r tot 3+r 6≤B 3(2)where B 3is the receiver capacity of node 3and r 6is theDefinition 2.Max-Min Fair Rate Vector We say a feasible rate vector is max-min fair,if for each i,r i cannot be increased while maintaining feasibility without decreasing r j for some source j for which r j≤r i Intuitively,a max-min fair rate allocation ensures that the most-starved node gets as much rate as possible;conditioned on this,it then ensures that the second most-starved node gets as much rate as possible;and so on.Wefirst present an illustrative example to show how this objective can be achieved in the toy8node topology of Figure4.We then present a general iterative distributed algorithm and prove that it will ensure that source rates converge to a rate alloca-tion that is max-min fair for a WSN collection tree under the receiver capacity model.3.1An Illustrative ExampleTo determine the max-min fair rate allocation for the topol-ogy of Figure4,wefirst need to calculate the perflow avail-able capacity at each node.For this calculation we divide the receiver capacity at each node by the total number offlows incident and exiting the node.For example there are4flows incident on node2(2from4and5,and2from node3),and 3flows exiting node2(2from its children and1of its own), giving a totalflow count at node2of7.Thus,the perflow available capacity for node2is B26,for node7it would be B74,B53and B87≥B37since the sum would add up toB2and allflows at node2would get an equal share.In the given topology this would apply toflows from nodes2,3, 4,5and6.Flows from nodes7and8do not consume node 2’s bandwidth and hence their rates should be constrained bynode7(assuming B73),resulting inflows from7and8being allocated a max-min rate of B7Pj∈N iF j2.γmini(n+1)=minj∈Sγj(n+1)3.γpathi(n+1)=minj∈A iγj(n+1)4.ifγpathi(n+1)>05.then r i(n+1)=r i(n)+γmini(n+1)•F i is the the total number offlows forwarded by nodei,whoseγpath>0.F i counts only thoseflows whodo not have a single node in their path whose capacityhas been exhausted.•S is the set of all nodes i whoseγi>0.Theorem 1.Algorithm MMF-RC converges in afi-nite number of steps.Proof.At every time step n,in step2of MMF-RC a node i is picked whoseγi(n)>0and is the minimum amongst the set S.In step3every source k calculates itsγpathk(n),which corresponds to the minimum of the avail-able perflow capacity amongst all nodes in the set A k,whosebandwidth source k consumes.Every source k whoseγpathk> 0increments its allocated rate r k(n)byγi(n).Thus,at time step n since allflows in the set F i have increased their rates byγi(n)>0,at time step n+1,γi(n+1)=0.Thus, at time step n+1all sources k which have i∈A k willhaveγpathk=0and hence will not increase their rate after time step n+1.Since i was any arbitrary node and n was any arbitrary time step,for n>0at every n+1time stepthere will be at least1source k,whoseγpathk(n+1)=0. Since the total number of sources in the system arefinite, in afinite number of steps N′>N,where N is the total number of source’s in the system,all sources i in the systemwill haveγpathi(N′)=0and hence MMF-RC will have converged.Definition 3.We say a node i is a bottleneck node with respect to a source j whoseflow consumes band-width at i if its receiver capacity is completely consumed and r j≥r k for all sources k whoseflow consumes band-width at i.Lemma 1.A feasible rate vector is max-min fair if and only if in the rate vector every source has a bottle-neck node.We omit a proof of this lemma due to space limitations, but note that it can be proved by a fairly straightforward modification of the similar proposition in Section6.5.2of [4]which applies to max-min fair rate allocation in wired networks.The key differences are in substituting bottleneck nodes instead of bottleneck links,and the set A i of all nodes whose bandwidth is consumed by aflow instead of the set of links on the path.Theorem 2.The rate vector that Algorithm MMF-RC converges to is max-min fair.Proof.From lemma1,it suffices to show that each node has a bottleneck node when??converges.In theorem1it was shown that at every step n>0,there will be at least one source k whoseγpathk(n)=0,such thatγpath k (n−1)>0.Also,the node k would have increasedits allocated rate by someγmin(n−1)at time step n−1sinceγpathk (n−1)>0.We claim that the node i,whoseγi(n−1)=γmin(n−1),will be the bottleneck node for node k,since at time step nγi(n)=0and till time step n all flows j who were consuming capacity at node i,and whoseγpath j (n−1)>0were experiencing equal increments.Thus,there can be noflow j,for whom i∈A j such that r j(N′)> r k(N′)for N′>n.Hence our claim that i is the bottleneck node of k is justified.Further,since for some N′′>N,where N is the totalnumber of sources,for all sources j,γpathj (N′′)=0,atN′′all sources j will have a bottleneck node.Thus,from lemma1,for some N′′>N MMF-RC converges to the max-min fair rate vector.4.THE WIRELESS RATE CONTROL PRO-TOCOLIn Section3,we presented the MMF-RC algorithm and showed that it achieves max-min fairness over a collection tree.However that algorithm operates in an idealized setting;it assumes synchronous execution,rapid global coordina-tion,constant-bit-rate staticflows from backlogged sources, and lossless links.A real world sensor network on the other hand would have asynchronous communication,stochastic introduced due to randomized CSMA MAC,lossy links and dynamicflows which might result from the on-demand na-ture of the sensing application.To implement the algorithmin a practical setting,we need to relax these assumptions.To this end we have designed the Wireless Rate Control Protocol(WRCP)which incorporates a number of mech-anisms to handle these real-world concerns.A single time step execution of the protocol is presented in the form of the WRCP algorithmSome additional notation used in the description of the WRCP algorithm is as follows;P i is the parent of node i;r i is the maximum allocated rate at whichflows consuming ca-pacity at node i can operate;r tot i is total transmission rateof node i,it is essential to note that for a source its de-mand might be less then its allocated rate,which implies that r tot i≤ j∈C i,∀j r i.Algorithm WRCP1.Calculate Per Flow Available Capacity:2.γi((n+1)T)=B i(nT)−Pj∈N i(nT)r totj(nT)Pj∈N i(nT)F j(nT)7.RateT hresh=0.1×MaxRate8.if r i(nT)>r Pi(nT)9.then r i(nT)=r Pi(nT)10.if r i(nT)≤MaxRate11.then ifγmini((n+1)T)<−RateT hresh12.orγmini((n+1)T)>013.thenα=αdef ault14.elseα=015.if r i(nT)>MaxRate16.then ifγmini((n+1)T)<017.thenα=0.518.else ifγmini((n+1)T)>RateT hresh19.thenα=αdef ault20.elseα=021.r i((n+1)T)=r i(nT)+α×γmini((n+1)T)22.Broadcast to j∈N i:23.γi((n+1)T),γmini((n+1)T),r i((n+1)T)WRCP retains the essential elements of the MMF-RC algorithm(steps2,4,21)and builds on it.Wedescribe the essential components of this protocol be-low.4.1Local TimersWRCP relies on a T second timer to present nodeswith an interval to calculate their rate updates.Intu-itively T should be large enough for a node to be ablegather information from all nodes in the set A i in orderto perform accurate rate updates.Thus,T should beatleast as large as the delay in sending information fromthe sink to the leaf at the largest depth.4.2Estimating Receiver CapacityAs mentioned earlier,we approximate the receivercapacity by the saturation throughput,which is a func-tion of the number of senders in the receiver’s range.The saturation throughput function is pre-calculatedand stored as a lookup table.Figure3shows that thereceiver capacity will almost remain a constant as longas the number of senders is greater than4.4.3Estimating Active Flow CountsIn a dynamic environment,the number of active neigh-bors and the number of activeflows in a given neighbor-hood is going to change.To handle the ephemeralflowcount,an activeflow state tag is associated with eachneighbor entry and aging this entry in the absence ofpackets from the neighbor helps give a correct estimateof activeflows in the network.The number offlows in aneighborhood determine the perflow available capacityat a receiver.A conservativeflow count will calculateflows from all the senders that a node has heard fromwithout regard for their link quality.The active neigh-bor andflow count is estimated by looking up both thetotal number of active neighbors,and the active sourcesthat each neighbor is forwarding.However,recent em-pirical results have shown that capture effects are quitedominant in these networks[21].These results suggest that nodes with stronger links will cause more inter-ference(or consume more capacity)than nodes with weaker links.We therefore take an optimistic approach and weigh the number offlows from a sender j to a re-ceiver i by its link quality p ij∈[0,1].Our experimental results(section7)show that this gives us a much better estimate of the achievable max-min rate than adoptinga conservative approach.4.4Estimating Transmission RatesDue to the stochastic introduced by random access CSMA schemes which are common in sensor networks, as well as stochastic that might be introduced due to non CBR traffic,instantaneous estimates of transmis-sion rates would be erroneous.Hence we maintain an exponential weighted moving average of transmission rates instead of instantaneous estimates as follows:P kts T ransmitted r tot i((n+1)T)=(1−β)r tot i(nT)+βFigure5:Software Architecture for WRCP guarantee the stability and performance of WRCP as well.Since the primary objective of this work is to show that explicit capacity rate control algorithms can be de-signed for sensor networks using a simple model such as the receiver capacity model,we perceive the complete evaluation of parameter selection process for WRCP tobe out of the scope of the current work and target it as part of our future work.For empirical evaluation,we have set the parameters for WRCP intuitively.SinceT needs to cater to the largest delay in the maximum size network that WRCP is going to be operational in, we set it to1000ms(the largest network we have con-sidered is a20node network with4hops).To ensure stabilityαneeds to be set to a small value and the moving average needs to be conservative.Thus,for our evaluation we setα=0.1andβ=0.8.5.SOFTWARE ARCHITECTURE FOR WRCPIN TINYOS-2.XFigure5shows the software architecture of WRCP implementation in TinyOS-2.0.2.2.TinyOS2.0.2.2,al-ready provides a framework for building collection treesin the form of the collection tree protocol(CTP)(TEP 123[2]).Since WRCP aims to achieve lexicographic max-min fairness among sources over a collection tree, we have integrated WRCP with the collection tree pro-tocol.The only modification required to the existing MAC (the CC2420CSMA MAC)was the addition offieldsto the header to support rate control information and provide acknowledgements to broadcast packets using the software acknowledgement feature of TinyOS2.0.2.2 (TEP126[3]).We also modified the routing engine in CTP to store additional per-neighbor information re-quired by WRCP(current transmission rate,per-flow available capacity,current allocated per-flow rate,ac-tiveflow state,number of descendants).We also had to make a major modification to the forwarding engine of CTP,since the default forwardingFigure6:A4-node fully connected linear topol-ogy.engine of the collection tree protocol does not imple-ment a FIFO queue.It implements a form of priority queuing which restricts the number of packets originat-ing from an application in the node itself to one,giving higher priority toflows from its descendants.Since,our algorithm explicitly assumes that the forwarding engine treats allflows equally,we needed to implement a vari-ant of the forwarding engine that implements a FIFO queue.In our software architecture,the core functionalityof WRCP is implemented in the Rate Controller.The Rate Controller performs the per-flow rate calculations, and sets the token generation rate on the leaky bucket[4]. The Flow Controller then uses tokens from the leaky bucket to admit packets into the system.6.EXPERIMENTAL METHODOLOGY6.1Basic Experimental SetupOur implementation is performed on the Tmote sky motes,which have CC2420radios,running TinyOS-2.0.2.2.The experiments are conducted over a small stand alone4-node topology(Figure6)and a larger20-node topology(Figure7)on the USC TutorNet testbed[15]. The smaller topology highlights the behavior of WRCP when a small number offlows exist,pertaining to a scenario when the perflow available capacity is high. The larger topology is representative of a network witha large number offlows,pertaining to a scenario witha small available perflow capacity.Experiments were conducted over a period of few months to ascertain any change in the performance of the protocols due to link quality variations in the topologies.It was observed that the link quality variations for the topologies over this large time frame were negligible,lending validity to the results.The state of the art for distributed rate control in wireless sensor networks is the Interference Aware Rate Control Protocol(IFRC)[19].We therefore use IFRCas the benchmark for evaluating the performance of WRCP.IFRC is an AIMD scheme and hence a com-parison of WRCP and IFRC will clearly highlight the advantages of designing a rate control protocol in a sensor network setting in terms of allocated rates,Figure7:A20node topology. convergence times,flow completion times and end-to-end delays.To have an unbiased comparison between IFRC and WRCP,we ported IFRC from its TinyOS-1.x2implementation to TinyOS-2.x using the same software architecture used for WRCP(figure5). Similar to WRCP,IFRC was implemented as the rate controller block in the given software architecture.Due to space constraints we omit the description of IFRC and refer the reader to[19]for protocol specific details. For comparative evaluation between IFRC and WRCP we consider3scenarios,a static scenario and two dy-namic scenarios.The static scenario pertains to the case whenflows are active for the complete duration of the experiment.The two dynamic scenarios highlight the protocol behavior whenflow joins andflow departures are taking place during the operation of the network. We believe these three cases test the correctness of the protocols(in terms of achieving max-min fair rates)and capture a broad class offlow dynamics that might exist in real sensor networks.7.BENCHMARKING RESULTSFor all experimental results presented in this section, the size of the payload was10bytes.WRCP adds16 bytes,where as IFRC adds26bytes of overhead to each packet.Since both protocols exchange control informa-tion over the data path using a promiscuous mode of operation,WRCP exhibits better overhead efficiency. For the purposes of comparison we have set the IFRC parameters r init=0.1pkts/sec,φ=0.0125,ǫ=0.02. The upper queue threshold was set to8packets.These parameters were calculated as per the analysis presented in[19]for a20node topology,since this is the maximum size network we are dealing with in our experiments. For WRCP we setα=0.1,as per the arguments pre-sented in section4.6,and T was set to1000ms to cater2 4 6 8 10 12 14 16 18 20 22 24 26 28 30 0 200 400 600 800 1000 1200 1400A l l o c a t e d R a t e (P k t s /s e c )Time (secs)Src 2 Allocated Src 3 Allocated Src 4 Allocated(a)IFRC rate alloca-tion. 02 4 6 8 10 12 14 16 18 20 22 24 26 28 30 0100200300400500600700800900100011001200130014001500A l l o c a t e d R a t e (P k t s /s e c )Time (secs)Src 2 Allocated Src 3 Allocated Src 4 Allocated(b)WRCP rate alloca-tion. 05000 10000 15000 20000 2500030000 01002003004005006007008009001000110012001301400N u m b e r o f P a c k e t s R e c i e v e d a t B STime (secs)WRCP Src 4 Flow Completion IFRC Src 2 Flow Completion(c)WRCP and IFRC flow completion times.Node ID(d)WRCP and IFRC goodput.(e)WRCP and IFRC end-to-end packet delayFigure 8:The convergence time of the allocated rate,flow completion time,goodput and end-to-end packet delay for WRCP and IFRC on the 4-node fully connected topology,in a static scenario.2 4 6 8 10 030060090012001500A l l o c a t e d R a t e (P k t s /s e c )Time (secs)Src 2 Allocated (One hop)Src 18 Allocated (Two hops)Src 19 Allocated (Three hop)Src 20 Allocated (Four hops)(a)IFRC rate alloca-tion. 02 4 6 8 10 030060090012001500A l l o c a t e d R a t e (P k t s /s e c )Time (secs)Src 2 Allocated (One Hop)Src 18 Allocated (Two Hops)Src 19 Allocated (Three Hops)Src 20 Allocated (Four Hops)(b)WRCP rate alloca-tion. 01000 2000 3000 4000 5000 6000 0100200300400500600700800900100011001200130014001500N u m b e r o f P a c k e t s R e c i e v e d a t B STime (secs)WRCP Src 2 Packet Completion IFRC Src 2 Packet Completion WRCP Src 17 Packet Completion IFRC Src 17 Packet Completion(c)Flow completion times.A v e r a g e R a t e (P k t s /s e c )Node ID(d)Goodput.A v e r a g e D e l a y (m s )Node ID(e)End-to-End packetdelays.Figure 9:The convergence time of the allocated rate,flow completion time,goodput and average delay for WRCP and IFRC on the 20-node topology when all flows start simultaneously (static scenario).and ??.The flow dynamics for this experiment are as follows:•Flows from sources 2,3,8and 12(first hop sources)start at the beginning of the experiment and re-main active for the complete duration of the ex-periment.•Flows from sources 5,6,9,11,14,15,18,4,10(second hop sources)become active after the first 1000seconds.•Flows from sources 7,13,16and 19(third hop sources)become active after the first 2000seconds.•Flows from sources 17and 20(fourth hop sources)become active after the first 3000seconds.In this experiment all nodes keep their rate controllers on from the start of the experiments.For IFRC,the advantage of the rate controllers always being on,irre-spective of whether a node is sending out a flow or not,is that rates are pre-computed,and hence,when a flow joins the network it starts a much higher rate exceeding network capacity and forcing sources to perform multi-plicative decrease (since the rates computed before the flow joined the network was for a smaller number of flows).This leads to faster convergence times in terms of the allocated rate.However,it leads to a patho-logical case which can be seen in Figure 10(a)at 3000Node IDFigure 11:Goodput (Case 1).seconds.If the congestion is large,due to multiple flow joins,and the multiplicative decrease of IFRC is not ag-gressive enough,it might lead to the multiplicative de-crease setting the threshold value([19])quite low.Since the rate controllers have always been on,all nodes in the network are in the additive increase phase.In this phase,since the rate of increase is solely dependent on the threshold value value,the slope of the increments is severely affected by the excessive congestion experi-enced by sources.For WRCP,the above scenario does not present a problem since the number of flows are actively com-puted and even though flows start at a higher rate the decrements are aggressive and accurate,since the。
Wavelet计算信号处理说明书

A. Aldroubi, Oblique and hierarchical multiwavelet bases, To appear in Applied and Camp. Harmonic Analysis, 1997.
J. Allen, Cochlear modeling, IEEE ASSP Magazine, 2:3-29, 1985.
[BMG92] A. Baskurt, I. E. Magnin, and R. Goutte, Adaptive discrete cosine transform coding algorithm for digital mammography, Optical Engineering, 31:1922-1928, Sept. 1992.
A. Aldroubi and M. Unser, Sampling procedures in function spaces and asymptotic equivalence with Shannon's sampling theory, Numer. Funct. Anal. Optimiz., 15:1-21, 1994.
[BT92]
J. J. Benedetto and A. Teolis, An auditory motivated time scale signal representation, IEEE-SP International Symposium on Time-Frequency and Time-Scale analysis, Oct. 1992.
An integrated approach to inverse kinematics and path planning for redundant manipulators

I. I NVERSE K INEMATICS AND PATH P LANNING Service robots and especially humanoid robots are expected to perform complex manipulation tasks in dynamic environments. This precludes the use of preprogrammed trajectories, and instead necessitates general and flexible techniques for autonomous manipulation planning. Solving a manipulation planning problem involves computing some sequence of grasping, regrasping, and manipulation operations applied to a set of movable objects [1]–[4]. In this paper, we focus on the reaching subtask, which involves computing a trajectory for the manipulator arm to move from some initial configuration to a goal configuration with the end-effector in a position to grasp the object. Reaching subtasks have traditionally been further subdivided into the problems of grasp selection, arm configuration selection, and arm trajectory planning. This division of the computation exists for both historical and practical reasons. Conventional path planning algorithms require a specific goal configuration as input. Thus, a method for calculating the joint angles that correspond to the desired workspace posture of the endeffector is needed. This is the classic inverse kinematics (IK) problem, which has a long history in the robotics literature. Apart from special cases, there currently exist no known analytical methods for solving the inverse kinematics of a general redundant mechanism (greater than six degrees of freedom) [5]. Iterative, numerical techniques based on the calculation of the pseudo-inverse of the Jacobian J + [6], [7] are typically used instead. These methods have several drawbacks:
Xilinx ISE自带的除法器IP核数据手册
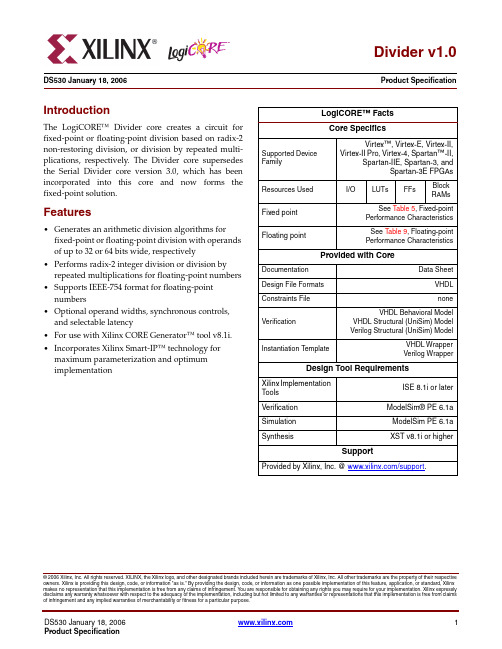
© 2006 Xilinx, Inc. All rights reserved. XILINX, the Xilinx logo, and other designated brands included herein are trademarks of Xilinx, Inc. All other trademarks are the property of their respective owners. Xilinx is providing this design, code, or information "as is." By providing the design, code, or information as one possible implementation of this feature, application, or standard, Xilinx makes no representation that this implementation is free from any claims of infringement. Y ou are responsible for obtaining any rights you may require for your implementation. Xilinx expressly disclaims any warranty whatsoever with respect to the adequacy of the implementation, including but not limited to any warranties or representations that this implementation is free from claimsof infringement and any implied warranties of merchantability or fitness for a particular purpose.IntroductionThe LogiCORE™ Divider core creates a circuit for fixed-point or floating-point division based on radix-2non-restoring division, or division by repeated multi-plications, respectively. The Divider core supersedes the Serial Divider core version 3.0, which has been incorporated into this core and now forms the fixed-point solution.Features•Generates an arithmetic division algorithms for fixed-point or floating-point division with operands of up to 32 or 64 bits wide, respectively •Performs radix-2 integer division or division by repeated multiplications for floating-point numbers •Supports IEEE-754 format for floating-point numbers •Optional operand widths, synchronous controls, and selectable latency •For use with Xilinx CORE Generator™ tool v8.1i. •Incorporates Xilinx Smart-IP™ technology for maximum parameterization and optimum implementationDivider v1.0DS530 January 18, 2006Product SpecificationLogiCORE™ Facts Core SpecificsSupported Device FamilyVirtex™, Virtex-E, Virtex-II,Virtex-II Pro, Virtex-4, Spartan™-II,Spartan-IIE, Spartan-3, andSpartan-3E FPGAsResources UsedI/OLUTsFFsBlock RAMsFixed point See Table 5,Fixed-point Performance Characteristics Floating pointSee T able 9,Floating-point Performance CharacteristicsProvided with CoreDocumentation Data SheetDesign File Formats VHDL Constraints File noneVerificationVHDL Behavioral ModelVHDL Structural (UniSim)Model Verilog Structural (UniSim)ModelInstantiation TemplateVHDL Wrapper Verilog WrapperDesign Tool RequirementsXilinx Implementation Tools ISE 8.1i or later Verification ModelSim®PE 6.1a Simulation ModelSim PE 6.1a SynthesisXST v8.1i or higherSupportProvided by Xilinx, Inc. @ /support .OverviewThe Divider core selects an implementation depending on the algorithm_type parameter. Currently,the following two division implementations are supported:•Fixed-point. Radix-2, non-restoring integer division using fixed-point operands, allowing a remainder to be generated.•Floating-point. Division by repeated multiplications. Works on normalized operands; in effect, a floating-point implementation.A detailed explanation of each implementation is provided in a later section of this data sheet.ApplicationsDivision is the most complex of the four basic arithmetic operations. Because hardware solutions arecorrespondingly larger and more complex than the solutions for the other operations, it is best to min-imize the number of divisions in any algorithm. There are many forms of division implementation,which can be separated into two broad categories: fixed-point algorithms and floating-point algo-rithms. This core provides one example of each category.The radix-2 non-restoring algorithm solves one bit of the quotient per cycle using addition and subtrac-tion. For this reason, it can achieve very high clock speeds at the expense of relatively high latency.However, the design is fully pipelined, so can achieve a throughput of one division per clock cycle. Theresulting circuit is relatively large, however, so if the throughput is smaller, the divisions per clockparameter allows compromises of throughput and resource use. This algorithm naturally generates aremainder, so is the choice for applications requiring remainders or modulus results.The repeated multiplications algorithm is an iterative method using successive approximations to thereciprocal of the denominator. The number of bits of the quotient solved doubles per iteration, so thisalgorithm is well suited to applications requiring precise results. Also, because this core makes use ofembedded multipliers, the overall resource use is less than that for the radix-2 algorithm. Again, thedesign is fully pipelined to allow a throughput of one division per clock cycle. This algorithm does notnaturally yield a remainder.2DS530 January 18, 2006Generic XCO and VHDL ParametersThe descriptions below refer to generic VHDL parameters. Table2 defines the parameters, legal values,and meaning of the XCO parameters and VHDL generics, which are broadly equivalent.•c_family (string) and c_xdevicefamily (string): Together, these generics identify the specific FPGA device family the core is targeting. Table1 the values for each of the supported families.T able 1: Relationship between Target FPGA Family, c_family and c_xdevicefamilyTarget FPGA Family c_family c_xdevicefamily Virtex/Virtex-E"virtex""virtex"Spartan-II/Spartan-II E"virtex""spartan2"Virtex-II"virtex2""virtex2"Virtex-II Pro"virtex2p""virtex2p"Spartan-3"spartan3""spartan3"Spartan-3E"spartan3""spartan3e"Virtex-4"virtex4""virtex4"•algorithm_type (integer): Specifies the division algorithm to use. The choice is 1 for radix-2(fixed-point notation) or 2 for division by repeated multiplications (floating-point notation).•signed_b (integer): 0 for unsigned operands, 1 for signed (2’s complement) operands. Applies to fixed-point notation only.•fractional_b (integer): 0 (no remainder) or 1 (has remainder). Applies to fixed-point only.•dividend_width (integer): 2 to 32 (fixed). Specifies the width of both dividend and quotient.•fractional_width (integer): 2 to 32 (fixed-point only).•c_has_ce (integer): 0 (no ce), or 1 (has ce).•c_has_aclr (integer): 0 (no ce), or 1 (has ce).•c_has_sclr (integer): 0 (no ce), or 1 (has ce).•divclk_sel (integer): 1, 2, 4, or 8. Specifies the number of clocks between division results for the fixed-point case only. A higher number results in lower-circuit area at the cost of lower throughput.•latency (integer): 1 to 99(float only). Specifies the circuit latency in terms of enabled clock (ce) cycles.•divisor_width (integer):2 to 32 (fixed)•bias (integer): Specifies the bias on the exponent, according to IEEE-754 format. A value of -1 results in a bias value in the mid-point of the exponent range.•mantissa_width (integer): 2 to 64. Specifies the width of the mantissae of all operands (floating-point only).•exponent_width (integer): 2 to 16. Specifies the width of the exponents of all operands (floating-point only).4DS530 January 18, 2006T able 2: Common Generic Parameters XCO ParameterXCO ValuesGeneric VHDL Parameter Generic ValuesDescriptionCommon GenericsAlgorithm T ype Fixed, Float algorithm_type (1),21 = fixed-point division (radix-2)2 = floating-point division CE false, true c_has_ce (0),10 = no CE 1 = has CE ACLR false, true c_has_aclr (0),10 = no ACLR 1 = has ACLR SCLRfalse, true c_has_sclr(0),10 = no SCLR 1 = has SCLRSCLR/CE Priority SCLR_overrides _CE, CE_overrides_SCLRc_sync_enable (0),10 = SCLR overrides CE 1 = CE overrides SCLRSerial-divider Generics (Fixed-point)Dividend and Quotient width 2 to 32 (16)dividend_width 2 to 32(16)Width of dividend and quotient (fixed only)Divisor width 2 to 32 (16)divisor_width 2 to 32 (16)Width of divisor (fixed only)Remainder type remainder, fractional fractional_b 0,10 = remainder,1 = fractional Fractional width 2 to 32 (16)fractional_width 2 to 32 (16)Width of fraction (fractional only)Operand signunsigned, signedsigned_b0,10 = unsigned 1 = signedClocks per division 1,2,4,8divclk_sel (1),2,4,8Throughput (interval between input opportunities)Low-latency Generics (Floating-point)Mantissa width 2 to 64mantissa_width 2 to 64 (16)Width of mantissa Exponent width2 to 16exponent_width2 to 16 (8)Width of exponent Latency 1 to 99latency 1 to 99(1)Latency of division Bias-1 to 2^Exponent_width -1bias-1 to 2^exponent_width -1Exponent biasFeature Summary Fixed-point Solution•Divides dividend by divisor to provide the quotient with integer or fractional remainder •Pipelined architecture for increased throughput •Pipeline reduction for size versus throughput selections •Dividend width from 1 to 32 bits •Divisor width from 3 to 32 bits•Fractional remainder width from 3 to 32 bits•Independent dividend, divisor and fractional bit widths •Fully synchronous design using a single clock•Supports unsigned or two’s complement signed numbers •Can implement 1/X (reciprocal) function •Fully registered outputsOverview Fixed-point SolutionThis parameterized module divides an M-bit-wide variable dividend by an N-bit-wide variable divi-sor. The output consists of the quotient and either the integer remainder or the fractional result (quo-tient continued past the binary point). In the integer remainder case, the result of the division is an M-bit-wide quotient with an N-bit-wide integer remainder (Equation 1). In the fractional case, the result is an M-bit-wide quotient with an F-bit-wide fractional remainder (Equation 2). When both frac-tional and signed are selected, the top bit of the fractional result is a two’s complement sign bit, result-ing in one less bit of magnitude result (Equation 3). It is an efficient, high-speed, parallel implementation. The core can be configured for unsigned or signed data.Equation 1: Integer remainder case.Equation 2: F-bit-wide fractional remainder in the unsigned caseEquation 3: F-bit-wide fractional remainder in the signed caseNote that for signed mode with integer remainder, the sign of the quotient and remainder correspond exactly to Equation 1.Dividend = quotient * divisor + remainderFractRmd=IntRmd *2FDivisorFractRmd=IntRmd *2( F-1)DivisorThus6/-4 = -1 REMD 2whereas-6/4 = -1 REMD –2For signed mode with fractional remainder, the sign bit is present both in the quotient and the remain-der. For example, for a four-bit dividend, divisor and fractional remainder we have:-9/4 = 9/-4 = -(2 1/4)This corresponds to:(1)0111 / 0100 or 1001/1100Giving the result:Quotient = 1110 (= -2)Remainder = 1110 (= -1/4)For division by zero, the quotient, remainder, and fractional results are undefined.The design is highly pipelined. The amount of pipelining can be reduced to decrease the area of thedesign at the expense of throughput. In the fully pipelined mode the design outputs the result of onedivision operation per clock cycle after an initial latency. The design also supports the options of 2, 4,and 8 clock cycles per division after an initial latency, as shown in Table4.The dividend and divisor bit widths can be set independently. The bit width of the quotient is equal tothe bit width of the dividend. The bit width of the integer remainder is equal to the width of the divisor.For fractional output, the remainder bit width is also independent of the dividend and divisor. The corewill handle data ranges of 3 to 32 bits for the dividend, divisor and fractional output.The divider can be used to implement the 1/X function; that is, the reciprocal of the variable X. To dothis, the dividend bit width is set to 1 for unsigned or 2 for signed data and fractional mode is selected.The dividend input is tied high within the user’s design.6DS530 January 18, 2006Pinout of Fixed-point SolutionThe fixed-point core pinout and signal names are shown in Figure 1 and defined in Table 3.Figure 1: Core Pinout DiagramTable 3: Fixed-point Signal PinoutSignalDirectionDescriptionDIVIDEND[Dividend Width-1:0]Input Dividend (parallel data in). Data bit width determined by the Dividend width generic or XCO parameter.DIVISOR[Divisor Width -1:0]InputDivisor (parallel data in). Data bit width determined by the Divisor width generic or XCO parameter.CLK InputClock . With the exception of ACLR, control and data inputs are captured and new output data formed on rising clock transitions.ACLRInput (optional)Asynchronous Clear (ACLR). Optional input pin. All control signals are synchronous to the rising edge of CLK except ACLR. When ACLR is asserted (High), all the core flip-flops are asynchronously initialized. The core remain in this state until ACLR is negated.SCLRInput (optional)Synchronous Clear (SCLR). Optional input pin. When asserted (high), all the core flip-flops are synchronously initialized (synchronous to the clock). The core remains in this state until SCLR is deasserted. When both SCLR and CE exist, the sync_enable parameter determines whether SCLR is qualified by CE or whether SCLR overrides CE (that is, will clear the module on the clock edge even if CE is deasserted).CEInput (optional)Clock Enable (CE). Optional input pin. When deasserted (low), all the synchronous inputs are ignored and the core remains in its current state.8DS530 January 18, 2006Following a power-on reset, SCLR , or ACLR , the outputs QUOTIENT and REMAINDER output all zeroes until new results appear.Waveforms of Fixed-point SolutionThe total latency (number of clocks required to get the first output) is a function of the bit width of the dividend. If fractional output is required, the latency is also a function of the fractional bit width. If clock enable is selected, latency is in terms of enabled clock cycles.When ‘clocks per division’ is set to 2, 4, or 8, the RFD output indicates the cycle in which input data is sampled (Figure 2), and therefore from when latency is measured. Ready for data should be qualified by clock enable if used externally.In general:Latency is of the order M for integer remainder dividers Latency is of the order M + F for fractional remainder dividersRFDOutputReady for Data (RFD). An output that indicates the cycle in which input data is sampled by the core. This is only applicable to cores where divclk_sel is not 1. For the case of divclk_sel = 1 the core is fully pipelined and samples the inputs on every enabled clock rising edge; hence, RFD will always be high.When divclk_sel = 2, 4 or 8, the core only samples data on every 2nd, 4th or 8th enabled clock rising edge respectively. The cycle on which data is sampled isimportant for the definition of latency, as shown in figure3. RFD will only change on enabled (CE input) clock rising edges for a core that has CE input (has_ce = True).QUOTIENT[Dividend width-1:0]OutputQuotient . The result of the integer division of dividend by divisor (dividend DIV divisor). The bit width of the quotient is equal to the dividend. For signed operation, the quotient is in two’s complement form. Parallel data out. Data bit width determined by the dividend width generic or XCO parameter.REMAINDER[n:0]REMAINDER[f:0]OutputRemainder . The integer remainder of the integer division of dividend by divisor (dividend MOD divisor) when the core is not fractional. For a fractional core, this output is the fractional part of the division result.For either case, if the core is signed, the output is in two’s complement form.• Integer Remainder. Result data bit width determined by divisor width generic or XCO parameter.• Fractional Remainder. Result data bit width determined by Fractional Width generic or XCO parameter.Table 3: Fixed-point Signal Pinout (Continued)SignalDirectionDescriptionTable 4 provides a list of the latency formula for divider selections and Figure 2 illustrates how latency is defined. Latency is expressed in clock cycles for dividers with no clock enable input and otherwise in enabled clock cycles.The divclk_sel parameter allows a range of choices of throughput versus area. With divclk_sel = 1, the core is fully pipelined, so it will have maximal throughput of one division per clock cycle, but will occupy the most area. The divclk_sel selections of 2, 4 and 8 reduce the throughput by those respective factors for smaller core sizes.Figure 2: Latency Example (Clocks per Division = 4)T able 4: Latency of Fixed-point Solution Based on Divider ParametersSignedFractionalClks/DivLatencyFalse False 1M+2False False >1M+3False True 1M+F+2False True >1M+F+3True False 1M+4True False >1M+5True True 1M+F+4TrueTrue>1M+F+5Note: M=dividend width, F=fractional remainder width.clk dividend divisorrfd quot remda ba divb a rem blatencyce c div d c rem dc de f10DS530 January 18, 2006Performance Characteristics of Fixed-point SolutionTable 5 defines performance characteristics for cases run on a Virtex-4, speed grade 10 device, and are intended to provide an indication of resources used and achievable clock speed. Generics not specified are at their default values.T able 5: Fixed-point Performance CharacteristicsDivisor WidthDividend WidthDivclk_selSlices usedSpeed (MHz)DSP48s usedBlock Memories881129385008821002850088475322008886233000323211666203Feature Summary Floating-point Solution•Performs division by repeated multiplications for floating-point numbers•Supports IEEE-754 format for floating-point numbers•Optional operand widths, synchronous controls, selectable latencyOverview Floating-point SolutionThe floating-point implementation performs division by repeated multiplications. The design is fully pipelined for maximal throughput. The two operands, divisor and dividend, are entered in sign-man-tissa-exponent form. A single sign bit per operand determines the sign of the mantissa. The mantissa width is configurable, as is the exponent width. The bias of the exponent is also configurable. Following IEEE754, certain combinations of exponent and mantissa are interpreted as zero, infinity and NaN (not a number). The result is expressed in the same form as the inputs. Overflow and underflow outputs are given for those results whose magnitudes lie above or below (respectively) the range which can be expressed with the specified mantissa and exponent widths.The format of number representation follows IEEE754. The mantissas, both on input and output have an implicit leading ’1.’ The mantissa describes a number in the range 0.5(inclusive) to 1.0(not inclusive). For example, the number 0.75 is 0.11000... in binary. The mantissa to describe this would be 10000... (to the specified width). The leading 1 indicates the number is in the range 0.5 to just less than 1.0. Since the number representation requires this normalization the leading 1 is not required as an input since it car-ries no information.The underflow and overflow outputs are provided to show if the result of a calculation resulted in and exponent outside the range allowed by the width of the exponent, that is, <0 or >2^exponent_width. Table6 defines special values recognized by the core.T able 6: Special ValuesExponent Mantissa Special ValueAll ’1’s Not all ’0’s Not a Number (NaN)All ’1’s All ’0’s InfinityAll ’0’s All ’0’s ZeroTable7 defines division results involving any of the special values described in Table6.T able 7: Division Results Involving Special ValuesDividend Divisor QuotientNaN Any Value NaNAny Value NaN NaNInfinity Infinity NaNZero Zero NaNAny value Infinity Zero12DS530 January 18, 2006Pinout of Floating-point SolutionThe floating-point core pinout and signal names are displayed in Figure 3 and defined in Table 8.Any Value Zero Infinity Zero Any Value Zero InfinityAny ValueInfinityFigure 3: Floating-point Schematic SymbolTable 8: Pinout of Floating-point SolutionSignalDirectionDescriptionCLK Input Clock. Rising edge clock signal CE Input (optional)Clock Enable ACLR Input (optional)Asynchronous Clear SCLRInput (optional)Synchronous Clear DIVIDEND _MANTISSA [Mantissa Width-1:0]Input Dividend mantissa DIVISOR _MANTISSA [Mantissa Width-1:0]Input Divisor mantissaDIVISOR_SIGNInputSign of Divisor mantissa (0 for +ve, 1 for -ve)T able 7: Division Results Involving Special Values (Continued)DividendDivisorQuotientWaveforms of Floating-point SolutionThe functional timing characteristics of the floating-point solution are very simple. Because the design has a throughput of one division per clock cycle, no handshaking signals (Ready for Data, New Data,output Ready) are required. Latency is selectable and is defined in the same manner as for the fixed-point solution. For this reason, outputs occur following the n th enabled rising clock edge after the inputs, where n is the latency value.Performance Characteristics of Floating-point SolutionTable 9 defines performance characteristics for cases run on a Virtex-4, speed grade 10 device and are intended to provide an indication of resources used and achievable clock speed. Generics not specified are at their default values.Note that when the core does not have asynchronous clear nor synchronous clear, use can be made of SRL16 primitives, leading to a substantial reduction in circuit size. For this reason, the use of SCLR or ACLR is not recommended.DIVIDEND_SIGNInput Sign of Dividend mantissa (0 for +ve, 1 for -ve)DIVISOR _EXPONENT [Exponent Width-1:0]Input Exponent of Divisor DIVIDEND _EXPONENT [Exponent Width-1:0]Input Exponent of Dividend QUOTIENT _MANTISSA [Mantissa Width-1:0]Output Quotient mantissa QUOTIENT_SIGNOutput Sign of Quotient QUOTIENT _EXPONENT [Exponent Width-1:0]Output Exponent of Quotient OVERFLOW Output Float overflow indication UNDERFLOWOutputFloat underflow indicationNoteAll control inputs are Active High. If an Active Low input is required for a particular control pin, an inverter must be placed in the path to the pin. The inverter will be absorbed appropriately during synthesis and/or mapping.T able 9: Floating-point Performance CharacteristicsMantissa WidthExponent WidthLatencySlices usedSpeed (MHz)DSP48s usedBlock Memories1581842510115810231941011583027227610124826(no SCLR)399283101Table 8: Pinout of Floating-point Solution (Continued)SignalDirectionDescription14DS530 January 18, 2006Generating the CoreThe Divider core can be included in your design in two ways: Using the CORE Generator graphical user interface (GUI), or using direct instantiation.Method 1: GUIThe CORE Generator system produces several files when a core is generated. Instructions about how to instantiate a core using this method are automatically produced in the .vho file. An example of a section of a .vho file is provided below:-- The following code must appear in the VHDL architecture header:------------- Begin Cut here for COMPONENT Declaration ------ COMP_TAG component div_gen_v1_0 port (clk: IN std_logic;...(other ports));end component;-- COMP_TAG_END ------ End COMPONENT Declaration -- The following code must appear in the VHDL architecture -- body. Substitute your own instance name and net names.------------- Begin Cut here for INSTANTIATION Template ----- INST_TAG your_instance_name : div_gen_v1_0 port map ( clk => clk, q => q);-- INST_TAG_END ------ End INSTANTIATION Template -- You must compile the wrapper file counter.vhd when simulating24826(with SCLR)720310101428157388821142845937211211T able 9: Floating-point Performance Characteristics (Continued)Mantissa WidthExponent WidthLatencySlices usedSpeed (MHz)DSP48s usedBlock Memories-- the core, counter. When compiling the wrapper file, be-- sure to reference the XilinxCoreLib VHDL simulation-- library. For detailed-- instructions, please see the CORE Generator User Guide.Method 2: Direct InstantiationThe CORE Generator now allows cores to be directly instantiated into user code. To do this, add the fol-lowing lines to the head of your VHDL file:Library Xilinxcorelib;Use Xilinxcorelib.div_gen_v1_0_comp.all;and instantiate the Divider core with appropriate values for the generics and your local signals:i_instance: div_gen_v1_0generic map(c_dividend_width => 16,c_has_ce => 1etc.);port map(clk => clk ,sclr => sclr ,quotient => output);Note that generics do not need to be specified if the default value suits your application.CORE Generator Parameter ScreensThe Divider core GUI provides three screens for selecting core parameters.•Main screen. Describes parameters common to both implementations, such as SCLR and CE, and allows the selection of the divider implementation.•Fixed-point implementation options. Provides configuration options for the fixed-point divider configuration. Note that this screen is displayed only if Fixed-point is selected on the main screen. •Floating-point implementation options. Provides configuration options for the floating-point divider configuration. Note that this screen is displayed only if Floating-point is selected on the main screen.16DS530 January 18, 2006Main Screen•Component Name. The base name of the output files generated for the core. Names must begin with a letter and be composed of any of the following characters: a to z, 0 to 9 and “_”.Figure 4: Main ScreenFixed-point Implementation OptionsFloating-point Implementation OptionsFigure 5: Fixed-point Implementation OptionsFigure 6: Floating-Point Implementation OptionsVerificationThe Divider core is supplied with a VHDL functional behavioral model, and the CORE Generator canalso produce a UniSim-based Verilog model if desired.SimulationWhen the Divider core is generated using the CORE Generator, a VHDL functional behavioral model is alsogenerated. The VHDL behavioral model is a pre-defined, parameterized model of the core, which is copiedto the project directory. A Verilog wrapper is also provided for the VHDL model for mixed-language simu-lation. If a Verilog model is selected, the CORE Generator produces a UniSim-based model of the core.Important Note: The VHDL Behavioral model provided for the floating-point solution does not exactlyreproduce the behavior of the synthesized core. The models quotients may differ by the least significant bitof the mantissa. For an exact match, the structural (UniSim) behavioral model must be used.References"Computer Arithmetic Algorithms and Hardware Designs," Behrooz Parhami. Oxford Press © 2000.LicensingThe Divider core does not require a license.Ordering InformationThis core may be downloaded from the Xilinx IP Center for use with the Xilinx CORE Generator systemv8.1i and higher. The Xilinx CORE Generator system is bundled with the ISE Foundation software at noadditional charge. To inquire about other Xilinx products, contact your local Xilinx sales representative.SupportXilinx provides technical support for this LogiCORE product when used as described in the productdocumentation. Xilinx cannot guarantee timing, functionality, or support of product if implemented indevices not listed in the documentation, or if customized beyond that allowed in the productdocumentation, or if any changes are made in sections of design marked as DO NOT MODIFY.Related InformationXilinx products are not intended for use in life-support appliances, devices, or systems. Use of a Xilinxproduct in such application without the written consent of the appropriate Xilinx officer is prohibited.Revision HistoryDate Version Revision1/18/06 1.0Initial Xilinx release.18DS530 January 18, 2006。
04 Iterative multiuser receiver in sparse code multipleaccess systems

Fig. 1: Block diagram of an uplink SCMA system.
be represented by a sparse factor graph. By carefully designing the factor graph and mapping functions, SCMA can perform better than LDS with similar decoding complexity [ 1 1]. Inspired by the turbo principle [ 12], iterative multiuser receivers have been investigated in CDMA systems [13], [14] and also in the LDS system [ 15]. In this paper, we consider an uplink SCMA system employing channel coding, and develop an iterative multiuser receiver for the SCMA system. It will be shown that how the soft decisions are exchanged between the SCMA decoder and channel decoders to fully utilize the diversity gain and coding gain, and how the decoding complexity can be reduced by exploiting the special structure of SCMA codebook and tailoring the factor graph during the iterations. The simulation results demonstrate the superiority of the proposed iterative receiver over the non-iterative one. The simulation results also show that SCMA can work well in highly overloaded scenario, and the performance will not degrade even if the load is as high as 300%. In this paper, the set of binary and complex numbers are denoted by lR and C, respectively. We use x, x, and X to represent a scalar, a vector and a matrix. The rest of the paper is organized as follows. Section II introduces the system model. Section III presents the details of the iterative multiuser receiver. In Section IV, the receiver performance is evaluated. Section V concludes the paper. II.
生物信息学主要英文术语及释义(续完)
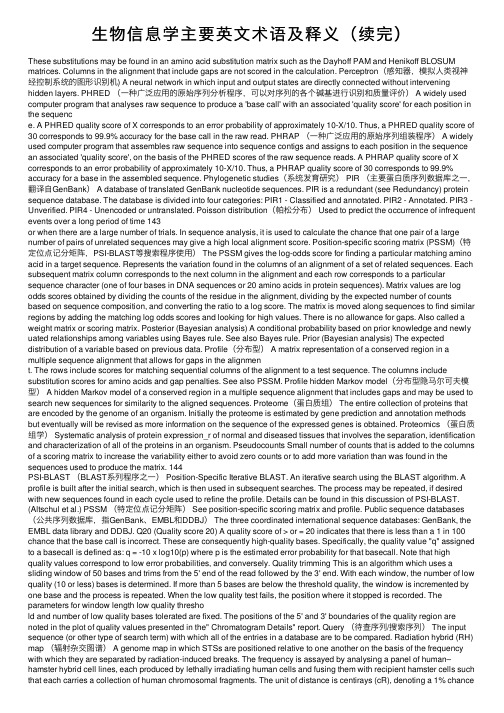
⽣物信息学主要英⽂术语及释义(续完)These substitutions may be found in an amino acid substitution matrix such as the Dayhoff PAM and Henikoff BLOSUM matrices. Columns in the alignment that include gaps are not scored in the calculation. Perceptron(感知器,模拟⼈类视神经控制系统的图形识别机) A neural network in which input and output states are directly connected without intervening hidden layers. PHRED (⼀种⼴泛应⽤的原始序列分析程序,可以对序列的各个碱基进⾏识别和质量评价) A widely used computer program that analyses raw sequence to produce a 'base call' with an associated 'quality score' for each position in the sequence. A PHRED quality score of X corresponds to an error probability of approximately 10-X/10. Thus, a PHRED quality score of30 corresponds to 99.9% accuracy for the base call in the raw read. PHRAP (⼀种⼴泛应⽤的原始序列组装程序) A widely used computer program that assembles raw sequence into sequence contigs and assigns to each position in the sequence an associated 'quality score', on the basis of the PHRED scores of the raw sequence reads. A PHRAP quality score of X corresponds to an error probability of approximately 10-X/10. Thus, a PHRAP quality score of 30 corresponds to 99.9% accuracy for a base in the assembled sequence. Phylogenetic studies(系统发育研究) PIR (主要蛋⽩质序列数据库之⼀,翻译⾃GenBank) A database of translated GenBank nucleotide sequences. PIR is a redundant (see Redundancy) protein sequence database. The database is divided into four categories: PIR1 - Classified and annotated. PIR2 - Annotated. PIR3 -Unverified. PIR4 - Unencoded or untranslated. Poisson distribution(帕松分布) Used to predict the occurrence of infrequent events over a long period of time 143or when there are a large number of trials. In sequence analysis, it is used to calculate the chance that one pair of a large number of pairs of unrelated sequences may give a high local alignment score. Position-specific scoring matrix (PSSM)(特定位点记分矩阵,PSI-BLAST等搜索程序使⽤) The PSSM gives the log-odds score for finding a particular matching amino acid in a target sequence. Represents the variation found in the columns of an alignment of a set of related sequences. Each subsequent matrix column corresponds to the next column in the alignment and each row corresponds to a particular sequence character (one of four bases in DNA sequences or 20 amino acids in protein sequences). Matrix values are log odds scores obtained by dividing the counts of the residue in the alignment, dividing by the expected number of counts based on sequence composition, and converting the ratio to a log score. The matrix is moved along sequences to find similar regions by adding the matching log odds scores and looking for high values. There is no allowance for gaps. Also called a weight matrix or scoring matrix. Posterior (Bayesian analysis) A conditional probability based on prior knowledge and newly uated relationships among variables using Bayes rule. See also Bayes rule. Prior (Bayesian analysis) The expected distribution of a variable based on previous data. Profile(分布型) A matrix representation of a conserved region in a multiple sequence alignment that allows for gaps in the alignment. The rows include scores for matching sequential columns of the alignment to a test sequence. The columns include substitution scores for amino acids and gap penalties. See also PSSM. Profile hidden Markov model(分布型隐马尔可夫模型) A hidden Markov model of a conserved region in a multiple sequence alignment that includes gaps and may be used to search new sequences for similarity to the aligned sequences. Proteome(蛋⽩质组) The entire collection of proteins that are encoded by the genome of an organism. Initially the proteome is estimated by gene prediction and annotation methods but eventually will be revised as more information on the sequence of the expressed genes is obtained. Proteomics (蛋⽩质组学) Systematic analysis of protein expression_r of normal and diseased tissues that involves the separation, identification and characterization of all of the proteins in an organism. Pseudocounts Small number of counts that is added to the columns of a scoring matrix to increase the variability either to avoid zero counts or to add more variation than was found in the sequences used to produce the matrix. 144PSI-BLAST (BLAST系列程序之⼀) Position-Specific Iterative BLAST. An iterative search using the BLAST algorithm. A profile is built after the initial search, which is then used in subsequent searches. The process may be repeated, if desired with new sequences found in each cycle used to refine the profile. Details can be found in this discussion of PSI-BLAST. (Altschul et al.) PSSM (特定位点记分矩阵) See position-specific scoring matrix and profile. Public sequence databases (公共序列数据库,指GenBank、EMBL和DDBJ) The three coordinated international sequence databases: GenBank, the EMBL data library and DDBJ. Q20 (Quality score 20) A quality score of > or = 20 indicates that there is less than a 1 in 100 chance that the base call is incorrect. These are consequently high-quality bases. Specifically, the quality value "q" assigned to a basecall is defined as: q = -10 x log10(p) where p is the estimated error probability for that basecall. Note that high quality values correspond to low error probabilities, and conversely. Quality trimming This is an algorithm which uses a sliding window of 50 bases and trims from the 5' end of the read followed by the 3' end. With each window, the number of low quality (10 or less) bases is determined. If more than 5 bases are below the threshold quality, the window is incremented by one base and the process is repeated. When the low quality test fails, the position where it stopped is recorded. The parameters for window length low quality threshold and number of low quality bases tolerated are fixed. The positions of the 5' and 3' boundaries of the quality region are noted in the plot of quality values presented in the" Chromatogram Details" report. Query (待查序列/搜索序列) The input sequence (or other type of search term) with which all of the entries in a database are to be compared. Radiation hybrid (RH) map (辐射杂交图谱) A genome map in which STSs are positioned relative to one another on the basis of the frequency with which they are separated by radiation-induced breaks. The frequency is assayed by analysing a panel of human–hamster hybrid cell lines, each produced by lethally irradiating human cells and fusing them with recipient hamster cells such that each carries a collection of human chromosomal fragments. The unit of distance is centirays (cR), denoting a 1% chanceof a break occuring between two loci Raw Score (初值,指最初得到的联配值S) The score of an alignment, S, calculated as the sum of substitution and gap scores. Substitution scores are given by a look-up table (see PAM, BLOSUM). Gap scores are typically calculated as the sum of G, the gap opening penalty 145and L, the gap extension penalty. For a gap of length n, the gap cost would be G+Ln. The choice of gap costs, G and L is empirical, but it is customary to choose a high value for G (10-15)and a low value for L (1-2). Raw sequence (原始序列/读胶序列) Individual unassembled sequence reads, produced by sequencing of clones containing DNA inserts. Receiver operator characteristic The receiver operator characteristic (ROC) curve describes the probability that a test will correctly declare the condition present against the probability that the test will declare the condition present when actually absent. This is shown through a graph of the tesls sensitivity against one minus the test specificity for different possible threshold values. Redundancy (冗余) The presence of more than one identical item represents redundancy. In bioinformatics, the term is used with reference to the sequences in a sequence database. If a database is described as being redundant, more than one identical (redundant) sequence may be found. If the database is said to be non-redundant (nr), the database managers have attempted to reduce the redundancy. The term is ambiguous with reference to genetics, and as such, the degree of non-redundancy varies according to the database manager's interpretation of the term. One can argue whether or not two alleles of a locus defines the limit of redundancy, or whether the same locus in different, closely related organisms constitutes redundency. Non-redundant databases are, in some ways, superior, but are less complete. These factors should be taken into consideration when selecting a database to search. Regular expression_rs This computational tool provides a method for expressing the variations found in a set of related sequences including a range of choices at one position, insertions, repeats, and so on. For example, these expression_rs are used to characterize variations found in protein domains in the PROSITE catalog. Regularization A set of techniques for reducing data overfitting when training a model. See also Overfitting. Relational database(关系数据库)Organizes information into tables where each column represents the fields of informa-tion that can be stored in a single record. Each row in the table corresponds to a single record. A single database can have many tables and a query language is used to access the data. See also Object-oriented database. Scaffold (⽀架,由序列重叠群拼接⽽成) The result of connecting contigs by linking information from paired-end reads from plasmids, paired-end reads from BACs, known messenger RNAs or other sources. The contigs in a scaffold are ordered and oriented with respect to one another. 146 Scoring matrix(记分矩阵) See Position-specific scoring matrix. SEG (⼀种蛋⽩质程序低复杂性区段过滤程序) A program for filtering low complexity regions in amino acid sequences. Residues that have been masked are represented as "X" in an alignment. SEG filtering is performed by default in the blastp subroutine of BLAST 2.0. (Wootton and Federhen) Selectivity (in database similarity searches)(数据库相似性搜索的选择准确性) The ability of a search method to locate members of a protein family without making a false-positive classification of members of other families. Sensitivity (in database similarity searches)(数据库相似性搜索的灵敏性) The ability of a search method to locate as many members of a protein family as possi-ble, including distant members of limited sequence similarity. Sequence Tagged Site (序列标签位点) Short cDNA sequences of regions that have been physically mapped. STSs provide unique landmarks, or identifiers, throughout the genome. Useful as a framework for further sequencing. Significance(显著⽔平) A significant result is one that has not simply occurred by chance, and therefore is prob-ably true. Significance levels show how likely a result is due to chance, expressed as a probability. In sequence analysis, the significance of an alignment score may be calcu-lated as the chance that such a score would be found between random or unrelated sequences. See Expect value. Similarity score (sequence alignment) (相似性值) Similarity means the extent to which nucleotide or protein sequences are related. The extent of similarity between two sequences can be based on percent sequence identity and/or conservation. In BLAST similarity refers to a positive matrix score. The sum of the number of identical matches and conservative (high scoring) substitu-tions in a sequence alignment divided by the total number of aligned sequence charac-ters. Gaps are usually ignored. Simulated annealing A search algorithm that attempts to solve the problem of finding global extrema. The algorithm was inspired by the physical cooling process of metals and the freezing process in liquids where atoms slow down in movement and line up to form a crystal. The algorithm traverses the energy levels of a function, always accepting energy levels that are smaller than previous ones, but sometimes accepting energy levels that are greater, according to the Boltzmann probability distribution. Single-linkage cluster analysis An analysis of a group of related objects, e.g., similar proteins in different genomes to identify both close and more distant relationships, represented on a tree or dendogram. The method joins the most closely related pairs by the neighbor-joining algorithm by representing these pairs as outer branches on 147the tree. More distant objects are then pro-gressively added to lower tree branches. The method is also used to predict phylogenet-ic relationships by distance methods. See also Hierarchical clustering, Neighbor-joining method. Smith-Waterman algorithm(Smith-Waterman算法) Uses dynamic programming to find local alignments between sequences. The key fea-ture is that all negative scores calculated in the dynamic programming matrix are changed to zero in order to avoid extending poorly scoring alignments and to assist in identifying local alignments starting and stopping anywhere with the matrix. SNP (单核苷酸多态性) Single nucleotide polymorphism, or a single nucleotide position in the genome sequence for which two or more alternative alleles are present at appreciable frequency (traditionally, at least 1%) in the human population. Space or time complexity(时间或空间复杂性) An algorithms complexity is the maximum amount of computer memory or time required for the number of algorithmic steps to solve a problem. Specificity (in database similarity searches)(数据库相似性搜索的特异性) The ability of a search method to locate members of one protein family, including dis-tantly related members. SSR (简单序列重复) Simple sequence repeat, a sequence consisting largely of a tandem repeat of a specific k-mer (such as (CA)15). Many SSRs are polymorphic and have been widely used in genetic mapping. Stochastic context-free grammar A formal representation of groups of symbols in different parts of a sequence; i.e., not in the same context. An example is complementary regions in RNA that will form sec-ondary structures. The stochastic feature introduces variability into such regions. Stringency Refers to the minimum number of matches required within a window. See also Filtering. STS (序列标签位点的缩写) See Sequence Tagged Site Substitution (替换) The presence of a non-identical amino acid at a given position in an alignment. If the aligned residues have similar physico-chemical properties the substitution is said to be "conservative". Substitution Matrix (替换矩阵) A substitution matrix containing values proportional to the probability that amino acid i mutates into amino acid j for all pairs of amino acids. such matrices are constructed by assembling a large and diverse sample of verified pairwise alignments of amino acids. If the sample is large enough to be statistically significant, the resulting matrices should reflect the true probabilities of mutations occuring through a period of evolution. 148Sum of pairs method Sums the substitution scores of all possible pair-wise combinations of sequence charac-ters in one column of a multiple sequence alignment. SWISS-PROT (主要蛋⽩质序列数据库之⼀) A non-redundant (See Redundancy) protein sequence database. Thoroughly annotated and cross referenced. A subdivision is TrEMBL. Synteny The presence of a set of homologous genes in the same order on two genomes. Threading In protein structure prediction, the aligning of the sequence of a protein of unknown structure with a known three-dimensional structure to determine whether the amino acid sequence is spatially and chemically compatible with that structure. TrEMBL (蛋⽩质数据库之⼀,翻译⾃EMBL) A protein sequence database of Translated EMBL nucleotide sequences. Uncertainty(不确定性) From information theory, a logarithmic measure of the average number of choices that must be made for identification purposes. See also Information content. Unified Modeling Language (UML) A standard sanctioned by the Object Management Group that provides a formal nota-tion for describing object-oriented design. UniGene (⼈类基因数据库之⼀) Database of unique human genes, at NCBI. Entries are selected by near identical presence in GenBank and dbEST databases. The clusters of sequences produced are considered to represent a single gene. Unitary Matrix (⼀元矩阵) Also known as Identity Matrix.A scoring system in which only identical characters receive a positive score. URL(统⼀资源定位符) Uniform resource locator. Viterbi algorithm Calculates the optimal path of a sequence through a hidden Markov model of sequences using a dynamic programming algorithm. Weight matrix See Position-specifc scoring matrix.。
soft actor-critic 的解释 -回复
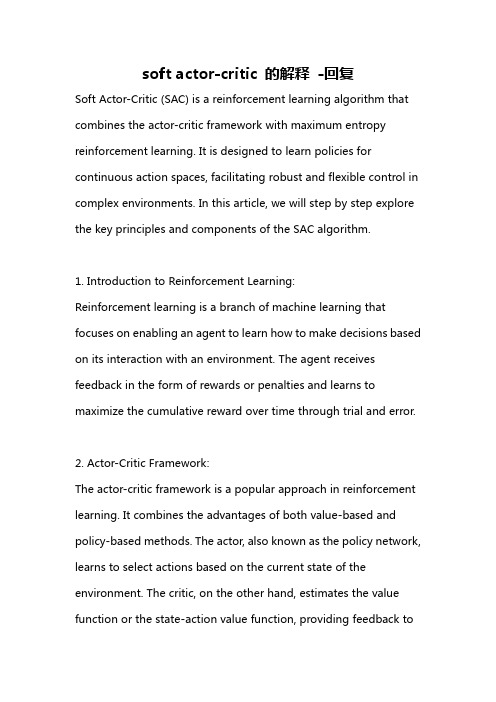
soft actor-critic 的解释-回复Soft Actor-Critic (SAC) is a reinforcement learning algorithm that combines the actor-critic framework with maximum entropy reinforcement learning. It is designed to learn policies for continuous action spaces, facilitating robust and flexible control in complex environments. In this article, we will step by step explore the key principles and components of the SAC algorithm.1. Introduction to Reinforcement Learning:Reinforcement learning is a branch of machine learning that focuses on enabling an agent to learn how to make decisions based on its interaction with an environment. The agent receives feedback in the form of rewards or penalties and learns to maximize the cumulative reward over time through trial and error.2. Actor-Critic Framework:The actor-critic framework is a popular approach in reinforcement learning. It combines the advantages of both value-based and policy-based methods. The actor, also known as the policy network, learns to select actions based on the current state of the environment. The critic, on the other hand, estimates the value function or the state-action value function, providing feedback tothe actor's policy learning process.3. Continuous Action Spaces:Many real-world problems, such as robotics control or autonomous driving, involve continuous action spaces. In contrast to discrete action spaces where there are a finite number of actions to choose from, continuous action spaces allow for an infinite number of actions within a specific range. Traditional policy-based methods struggle with continuous actions due to the curse of dimensionality.4. Maximum Entropy Reinforcement Learning:Maximum entropy reinforcement learning aims to learn policies that are not only optimal but also stochastic. Introducing stochasticity in the policy allows for exploration and probabilistic decision-making, enabling the agent to handle uncertainties in the environment. This approach helps prevent the agent from getting trapped in local optima.5. Soft Q-Learning:Soft Q-learning is a variant of the Q-learning algorithm that leverages maximum entropy reinforcement learning principles. Itseeks to learn a soft state-action value function, which combines the typical expected reward with an entropy term. The entropy term encourages exploration by discouraging over-reliance on deterministic policies.6. Policy Optimization with Soft Actor-Critic:In SAC, the actor is responsible for learning the policy distribution, parametrized by a neural network. The critic learns the Q-function, estimating the state-action values. The training procedure consists of sampling actions based on the current policy, collecting trajectories or episodes, and using these samples to update the policy and Q-function.7. Entropy Regularization:SAC utilizes entropy regularization to ensure exploration and stochastic decision-making. The entropy term acts as a regularizer added to the objective function during policy optimization. By maximizing the entropy, the agent strives to maintain a diverse set of actions and explore the full action space.8. Soft Actor-Critic Architecture:The SAC architecture involves three main components: the actornetwork, the critic network, and target networks. The actor network is responsible for learning the policy distribution, while the critic network estimates the Q-function for value estimation. Target networks are used to stabilize the learning process by providing temporally consistent value estimates.9. Experience Replay:Experience replay is a technique employed in SAC to improve sample efficiency and mitigate potential non-stationarity issues. Instead of updating the policy and value function using immediate samples, experience replay stores and replays past experiences. This approach enables the agent to learn from a diverse range of experiences, leading to more robust policy learning.10. Exploration Strategies:Exploration is critical for reinforcement learning, as it allows the agent to discover new and potentially better policies. SAC employs a combination of exploration strategies, including adding noise to the policy parameters or actions. This noise injection encourages the agent to explore different solutions, improving the chance of finding the optimal policy.In conclusion, Soft Actor-Critic is a powerful reinforcement learning algorithm for continuous action spaces. By incorporating maximum entropy reinforcement learning principles, SAC enables robust and flexible control in complex environments. Its actor-critic framework, with entropy regularization, allows for policy optimization and exploration, making it well-suited for real-world problems. Additionally, the use of experience replay and exploration strategies enhances the learning process, leading to better performance and more efficient policy learning.。
Accelerated iterative hard thresholding
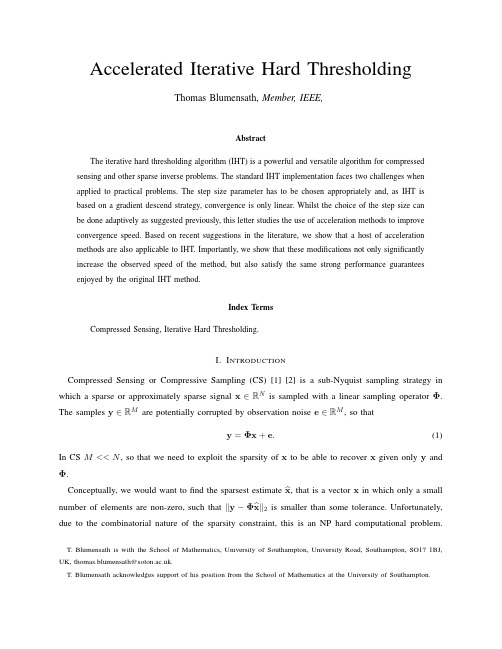
Accelerated Iterative Hard ThresholdingThomas Blumensath,Member,IEEE,AbstractThe iterative hard thresholding algorithm(IHT)is a powerful and versatile algorithm for compressed sensing and other sparse inverse problems.The standard IHT implementation faces two challenges when applied to practical problems.The step size parameter has to be chosen appropriately and,as IHT is based on a gradient descend strategy,convergence is only linear.Whilst the choice of the step size can be done adaptively as suggested previously,this letter studies the use of acceleration methods to improve convergence speed.Based on recent suggestions in the literature,we show that a host of acceleration methods are also applicable to IHT.Importantly,we show that these modifications not only significantly increase the observed speed of the method,but also satisfy the same strong performance guarantees enjoyed by the original IHT method.Index TermsCompressed Sensing,Iterative Hard Thresholding.I.I NTRODUCTIONCompressed Sensing or Compressive Sampling(CS)[1][2]is a sub-Nyquist sampling strategy in which a sparse or approximately sparse signal x∈R N is sampled with a linear sampling operatorΦ. The samples y∈R M are potentially corrupted by observation noise e∈R M,so thaty=Φx+e.(1) In CS M<<N,so that we need to exploit the sparsity of x to be able to recover x given only y and Φ.Conceptually,we would want tofind the sparsest estimate x,that is a vector x in which only a small number of elements are non-zero,such that y−Φ x 2is smaller than some tolerance.Unfortunately, due to the combinatorial nature of the sparsity constraint,this is an NP hard computational problem.T.Blumensath is with the School of Mathematics,University of Southampton,University Road,Southampton,SO171BJ, UK,thomas.blumensath@.T.Blumensath acknowledges support of his position from the School of Mathematics at the University of Southampton.Instead,CS reconstruction is typically solved using either a convex relaxation of the recovery problem [1]such asminxx 1: y−Φ x 2≤ ,(2) or a greedy algorithm such as the Compressive Sampling Matching Pursuit(CoSaMP)[3]or the Iterative Hard Thresholding(IHT)algorithm[4],[9].The IHT algorithm is an iterative methodx n+1=H k(x n+µΦT(y−Φx n)),(3) where H k is the hard thresholding operator that sets all but the k largest(in magnitude)elements1in a vector to zero.IHT is a very simple algorithm and yet,it can be shown that,under certain conditions,IHT can recover sparse and approximately sparse vectors with near optimal accuracy[4].However,in practice,there are two issues with this simple scheme.1)The step sizeµhas to be chosen appropriately to avoid instability of the method and2)IHT has only a linear rate of convergence.Recently,several approaches have been proposed to address these issues([5],[6],[7][8]).In[10],a normalised IHT(NIHT)algorithm was suggested that choosesµadaptively in each iteration.This was shown to guarantees the stability of NIHT.In[10],the step size is set toµ=ΦTΓn(y−Φx n) 22ΦΓnΦT(y−Φx n) 22(4)in each iteration,whereΓn is the support set of x n.Whilst this is sufficient to guarantee convergence under certain RIP conditions[10],if these conditions fail,then an additional line search was proposed in[10] to guarantee stability.A similar approach was suggested in[8],where againµis calculated as in(4),but this time,the setΓis the union of the support of x n and the support of H k(ΦT(y−Φx n)),which again guarantees stability and performance under RIP conditions.Qiu and Dogandzic[5]proposed another approach and analysed the Expectation-Conditional Maximisation Either(ECME)algorithm which is similar to,though not quite identical with,the‘iterative thresholding with inversion’algorithm studied by Maleki[6]x n+1=H k(x n+ΦT(ΦΦT)−1(y−Φx n)),(5) which is guaranteed to converge,as the use of the inverse matrix(ΦΦT)−1guarantees stability,thus circumventing the need to tuneµ.Importantly,as pointed out in[5],if(ΦΦT)is the identity matrix(that1In case the k largest elements are not defined uniquely,Hkis allowed to choose from the offending elements in an arbitrary way.For example,it can use a respecified ordering or random selection.is,if the rows ofΦare orthonormal),then the ECME algorithm is identical to the IHT algorithm with µ=1.Thus,ifΦhas orthonormal rows,then the IHT algorithm withµ=1is guaranteed to be stable (that is,the automatic step-size selection step in IHT is not required in this case).However,if(ΦΦT)is not diagonal,then the ECME algorithm requires the pre-computation and storage of the inverse matrix (ΦΦT)−1,which might not be feasible for certain large scale problems.For these problems,the NIHT algorithm remains an important alternative.Qui and Dogandzic further suggested a double over-relaxation scheme[5]to address the convergence speed issue.After calculating an update x as in(5), x is combined with the two previous estimates x n and x n−1to reduce a specific cost function.The new estimate is then again thresholded.If this newly thresholded estimate has a lower cost than x n+1itself,then this new estimate is accepted,whilst x n+1 is used otherwise.This double relaxation approach(abbreviated DORE)led to a significant improvement in the convergence speed of the method as compared to the IHT algorithm.Furthermore,Qui and Dogandzic[5]provided a performance bound for sparse recovery under a‘2k-sparse subspace quotient condition’2ρ2k=minx: x 0≤2k ΦT(ΦΦT)−1Φx 22x 22>0.5.(6)Inspired by these results and related work in[7]and[8],this letter studies the use of similar acceleration schemes in IHT.As it is not clear when the subspace quotient condition of Qui and Dogandzic holds and how to construct matrices with this property,our main contribution is to analyse the accelerated IHT algorithms based on the Restricted Isometry Property commonly used in CS theory.Importantly,we can show that the accelerated IHT algorithms have exactly the same strong,near optimal recovery results enjoyed by standard IHT.This result is a direct generalisation of a similar result by Foucart derived in [7].II.A CCELERATION OF IHTAs in IHT,we define an accelerated IHT algorithm(AIHT)as any method that calculates an initial updatex n+1=H k(x n+µΦT(y−Φx n)).(7) However,instead of continuing the iterative process with x n+1,following the same reasoning as in[5], we suggest the use of a strategy that tries tofind an estimate x n+1,that satisfies two conditions:2Here and throughout, x 0denotes the number of non-zero elements in the vector x.1)x n+1is k-sparse,2)x n+1satisfies y−Φx n+1 2≤ y−Φ x n+1 2.Any algorithm that calculates such an estimate will be called an accelerated IHT algorithm.One can envisage a range of different approaches to update x n+1.These can be roughly split into two categories,methods that only update the non-zero elements in x n+1and methods that are allowed to update all elements of x n+1but which use a second thresholding step to guarantee the new estimate is k-sparse.Thefirst type of approach is conceptually the simplest.For example,assume the set of non-zero elements in x n+1is Γ.IfΦ Γis the matrix with the columns not in the set Γremoved and if x n+1Γisdefined similarly,then all we need to do is to optimise the cost function y−ΦΓ x 22.This approach hasfirst been proposed and analysed by Foucart in[7].This optimisation can be done for example witha gradient(as in[7])or conjugate gradient algorithm,which when initialised with x n+1Γ,will always produce estimates that satisfy the condition2)above.Importantly,in practice,it is advisable to only run a small number of gradient or conjugate gradient steps in each IHT iteration so not to spend too much time in optimising the cost function in the inner loop(see below).The double-overrelaxation approach of[5]falls into the second category of approaches.The double-over-relaxation approach of[5]uses two relaxation stepsx n+11= x n+1+a1( x n+1−x n),(8) andx n+12= x n+11+a2( x n+11−x n−1),(9) where,for the AIHT algorithm,the line search parameters a1and a2can be calculated in closed form tominimise the quadratic cost function y−Φ x n+11 22and y−Φ x n+1222respectively.With this approach,x n+12is no longer guaranteed to be k-sparse,so that the optimisation step needs to be followed by an additional thresholding step,which in turn is likely to increase the quadratic cost.It can thus happen that y−ΦH k( x n+12) 22> y−Φ x n+1 22,which would violate our second condition.Thus,if y−ΦH k( x n+12) 22> y−Φ x n+1 22,we set x n+1= x n+1whilst we use x n+1=H k( x n+12)otherwise.III.RIP ANALYSIS OF AIHTThe advantage of AIHT methods is that,as long as each estimate x n+1satisfies the two conditions given above,then AIHT has the same performance guarantees as IHT itself.In CS,these guarantees aretypically stated in terms of the Restricted Isometry Constant (RIP).For a given matrix Φ,the Restricted Isometry Constants of order 2k are the largest α2k and smallest β2k ,such thatα2k x 1+x 2 22≤ Φ(x 1+x 2) 22≤β2k x 1+x 2 22(10)holds for all k sparse vectors x 1and x 2.AIHT satisfies the following performance bound that states that,as long as Φhas RIP constants that are not too different,then AIHT can recover any signal x to near optimal accuracy.Theorem 1:For arbitrary x ,given y =Φx +e where Φsatisfies the RIP with β2k ≤µ−1<1.5α2k ,after n = 2log( e 2/ x k 2)log(2/(µα2k )−2)(11)iterations,the AIHT algorithm calculates a solution x n satisfyingx −x n2≤(1+c β2k ) x −x k 2+c β2k x −x k 1√k +c e 2.(12)where c ≤ 43α2k −2µ+1, e =Φ(x −x k )+e and x k is the best k -term approximation to x .Proof:The proof is an extension of the proof in [11]and establishes an upper bound on x −x n +1 2.We here only summarise the main steps,concentrating on those areas that differ from [11].As in [11],we havex −x n +1 2≤ x k −x n +1 2+2α2k ( y −Φx n +1 22+ e 22),(13)where e =Φ(x −x k )+e .The proof of [11]is modified by realising that,by the second condition of the acceleration scheme,any AIHT algorithm satisfiesy −Φx n +1 22≤ y −Φ x n +1 22.(14)It is thus sufficient to bound y −Φ x n +1 22,which can be done as follows (where g =2Φ∗(y −Φx n )).y −Φ x n +1 22− y −Φx n 22=− ( x n +1−x n ),g + Φ( x n +1−x n ) 22≤−2µ ( x n +1−x n ),µ2g +1µ ( x n +1−x n ) 22=1µ x n +1−x n −µ2g 22−µ2 g 22 =1µ inf x : x 0≤0 x −x n −µ2g 22+−µ2g 22=inf x : x 0≤0− (x −x n ),g +1µ (x −x n ) 22 ≤− (x k −x n ),g +1µ(x k −x n ) 22=−2 (x k −x n ),Φ∗(y −Φx n ) +α x k −x n 22+(1µ−α) x k −x n 22≤−2 (x k −x n ),Φ∗(y −Φx n ) + Φ(x k −x n ) 22+(1µ−α) x k −x n 22= y −Φx k 22− y −Φx n 22+(1µ−α) x k −x n 22= e 22− y −Φx n 22+(1µ−α) (x k −x n ) 22.The inequalities are due to (from top to bottom)1)the RIP condition and the choice of β≤1µ,2)the fact that x k is k -sparse and 3)the RIP condition again.The third equality is due to the definition of x n +1=H k (x n +µ2g ).Thus,wrapping up as in [11],we get the bound x −x n +1 22≤ 2µα2k −2 (x k −x n ) 22+4α2ke 22+ x k −x 2(15)Therefore,the condition 2(1µα2k−1)<1implies that x −x n 2≤ 2µα2k −2 n/2 x k 2+√c e 2+ x k −x 2so that the theorem follows using Lemma 6.1in [3].IV.N UMERICAL S IMULATIONSTwo experiments were conducted.In the firs,random matrices Φ∈R 256×512were created with i.i.d.normal entries followed by normalisation of the columns of Φ.For each sparsity k in the interval from 1to 128,1000matrices were generated and k -sparse vectors x were drawn with the k non-zero entries also drawn from the unit variance normal distribution.No noise was added.Two accelerated IHT approach were compared.In the first approach three conjugate gradient steps were used per outer iteration (AIHT CG ),whilst the other approach used the double-over-relaxation method of [5](AIHT DORE ).TheIHT algorithm(NIHT)and the ECME algorithm with the double-over-relaxation(DORE)as proposed in[5]were also used.Both the AIHT as well as the IHT methods used the automatic step-size selection approach which we slightly modified here to reduce the number of line searches.In each iteration,the current proposed step-size was compared to the previously used step-size and the smaller of the two was used.We also relaxed the line search criterion in[10]so that a line search was only initialised when the proposed step sizeµ>1.5( x n+1−x n 22)/( Φ(x n+1−x n) 22).These two modification reduced the number of line searches.For the ECME algorithm,the matrix inverse was precomputed,the cost of which was counted toward the computation time shown.All algorithms were stopped once x n+1−x n 22/N<10−9.The code for the simulations is available on the authors webpage.Figure1shows the average Signal to Noise Ratio(SNR)(top panel)and the average computation time in seconds(lower panel)for each sparsity level k/M.The simulations were run in Matlab on a Intel Core2Duo CPU E85003.16GHz PC.It is clear that both acceleration methods work well with IHT.Both significantly improve the convergence speed of the method,however,ECME is still somewhat faster in this example and also works somewhat better in terms of signal recovery when k/M≈0.35.Though this needs to be contrasted with the fact that the AIHT implementation used here did not require the computation and storage of an inverse matrix,which in some applications can be a significant advantage. Figure2,which shows the average computation time for the above experiment(run on a8GB2.8 GHz Intel Core i7Macbook Pro computer)when AIHT uses1,3,5and10conjugate gradient steps, demonstrate that it is advisable to only use a small number of such steps.The second example used the Shepp-Logan image of size512×512(seefigure3),where between50 to70radial slices were sampled from the2D-Fourier transform of the image which were then used as the measurements y.The image was assumed to be k sparse in the Haar Wavelet domain with k=3769. The algorithms were run with the same parameters as before but stopped once x n+1−x n 22/N<10−16. Figure3,which also gives the results obtained by back-projection,shows the Peak Signal to Noise Ratio(PSNR)for each estimate as well as the computation time(run on a8GB2.8GHz Intel Core i7 Macbook Pro computer).NIHT is seen to be significantly slower than the other approaches.In contrast, using three iterations of a conjugate gradient solver per iteration to accelerate the NIHT algorithm not only significantly reduces the computation time but also lead to significantly better PSNR values.The DORE algorithm,which in this example does not have to use matrix inversion due to the orthogonality of the observation matrix(and is thus identical to our AIHT DORE method),shows comparable performance.Fig.1.Average SNR(20log10 x 2/ x−ˆx 2)and computation time(in seconds)for the four algorithms usingΦ∈R256×512 with i.i.d.normal entries and normalised rows with x only k non-zero entries drawn form a unit variance normal distribution.parison of average computation time for AIHT with1,3,6and10conjugate gradient steps.V.D ISCUSSION AND CONCLUSIONThe Iterative Hard Thresholding algorithms is a simple yet powerful tool to reconstruct sparse signals. Not only does it give near optimal recovery guarantees under the RIP,it is also very versatile and can be easily adapted to a range of constraint sets[11]as well as to non-linear measurement systems[12].Fig.3.Reconstruction accuracy and computation time for the512×512Shepp-Logan phantom image which was sampled taking between50to70equally spaced radial slices from the2D Fourier transform of the image and reconstructed assuming sparsity in the Haar wavelet domain.Shown are the PSNR(20log10 x ∞/ x−ˆx 2)and the computation time in seconds for different ratios of sparsity(k)to number of observations(M).Inspired by the recently developed ECME algorithm,we have here introduced and analysed an accelerated IHT framework.We have in particular looked at two acceleration strategies,the use of a conjugate gradient method and the use of the double-over-relaxation approach of[5],though other approaches can equally well be slotted into the AIHT algorithm.Our main contribution here was to show that, if done correctly,then any accelerated IHT algorithm inherits the strong performance guarantees from the IHT algorithm.Furthermore,combining these acceleration methods with NIHT significant increased the algorithm’s convergence speed,making the accelerated NIHT algorithm a strong competitor to the ECME method.Importantly,the accelerated NIHT method is extremely simple to implement and does not require the computation,storage and repeated use of matrix inverses.This is an advantage in many compressed sensing applications where the measurement matrix is often based on fast transforms such as the wavelet and Fourier transform.R EFERENCES[1] E.Cand`e s and J.Romberg,“Practical signal recovery from random projections,”in Proc.SPIE Conf.,Wavelet Applicationsin Signal and Image Processing XI,Jan.2005.[2] D.Donoho,“Compressed sensing,”IEEE Trans.on Information Theory,vol.52,no.4,pp.1289–1306,2006.[3]Needell D,Tropp JA.CoSaMP:Iterative signal recovery from incomplete and inaccurate samples.Applied andComputational Harmonic Analysis.2008May;26(3):301–321.[4]T.Blumensath and M.Davies,“Iterative hard thresholding for compressed sensing,”Applied and Computational HarmonicAnalysis,vol.27,no.3,.pp.265–2742009.[5]K.Qiu and A.Dogandzic,“ECME Thresholding Methods for Sparse Signal Reconstruction,”arXiv,no.1004.4880v3.[6] A.Maleki“Coherence Analysis of Iterative Thresholding Algorithms,”sin Proc.of the47th Annual Allerton Conferenceon Communication,Control,and Computing,pp.236–241,2009[7]S.Foucart“Hard thresholding pursuit:an algorithm for compressive sensing,”submitted[8]V.Cevher“On Accelerated Hard Thresholding Methods for Sparse Approximation,”EPFL Tec Rep.,2011.[9]T.Blumensath and M.E.Davies,“Iterative thresholding for sparse approximations,”Journal of Fourier Analysis andApplications,vol.14,no.5,pp.629–654,2008.[10]T.Blumensath and M.E.Davies,“Normalised Iterative Hard Thresholding;guaranteed stability and performance,”IEEEJournal of Selected Topics in Signal Processing,vol.4,no.2,pp.298–309,2010.[11]T.Blumensath,“Sampling and reconstructing signals from a union of linear subspaces,”to appear in IEEE Transactionson Information Theory,2011.[12]T.Blumensath,“Compressed Sensing with Nonlinear Observations,”submitted,2011.。
匹配滤波算法的英文

匹配滤波算法的英文Title: Understanding and Implementing Matched Filtering AlgorithmMatched filtering is a signal processing technique used in many fields such as radar, sonar, and digital communication systems. This algorithm has proven to be extremely useful for detecting known signals buried under noise. In this document, we will discuss the concept of matched filtering, its mathematical principles, and how it can be implemented.1. IntroductionA matched filter is an optimal linear filter that maximizes the signal-to-noise ratio (SNR) when the signal waveform is known in advance. It is widely used in detection of weak signals in noisy environments by correlating the received signal with a replica of the expected signal.2. Mathematical PrinciplesThe matched filter is based on the cross-correlation function, which measures the similarity between two signals. The output of a matched filter is given by the convolution of the input signal with a time-reversed version of the known signal. If the input signal contains the known signal, the output of the matched filter will be a peak at the location where the known signal begins.3. ImplementationThe implementation of a matched filter involves the following steps:- Generate a replica of the known signal.- Reverse the replica in time.- Convolve the input signal with the reversed replica.- Locate the peak in the output to find the start of the known signal.4. AdvantagesThe main advantage of the matched filter is its ability to detect weak signals in noise. It provides the best possible SNR for any linear filter when the signal waveform is known. Additionally, it is relatively simple to implement computationally.5. ConclusionMatched filtering is a powerful tool in signal processing. Its effectiveness in enhancing the SNR makes it particularly useful in applications where weak signals need to be detected in noisy environments. Despite its simplicity, the matched filter remains a fundamental component in various fields including radar, sonar, and digital communications.。
Research Interests

Shashibhushan P. Borade70 Pacific Street, Apt. 612, Cambridge MA 02139.Phone: 857-222-8502, Email:spb@/spb/www/EducationTechnology 2004–6/2008 (expected)ofMassachusettsInstitutePh.D. Candidate, EECS.GPA: 5.0/5.0Advisor: Prof. Lizhong ZhengThesis: An information theoretic approach to unequal error protectionMassachusetts Institute of Technology 2002–2004M.S., EECS. GPA: 5.0/5.0Advisors: Prof. Lizhong Zheng and Prof.Robert GallagerThesis: Maximizing degrees of freedom in wireless networksIndian Institute of Technology, Bombay 1998–2002 B.Tech., EE. GPA: 9.72/10.0Ranked first in the department and third in the institute amongst 420 students.Research InterestsInformation Theory: role of Geometry and Large Deviations, Feedback Channels, Networks, Wireless Communications Awards and Honors•Hewlett Packard Graduate Fellowship 2005 – 2007 •MIT Presidential Fellowship 2002 – 2003 •Institute Silver Medal for the highest GPA in Electrical Engineering at IIT Bombay 2002•National Talent Scholarship by the Government of India (about 750 awards per year across India). 1996 Research ExperienceLaboratory for Information and Decision Systems (LIDS), MIT 2002–Present Graduate ResearcherUnequal error protection•Classical information theory assumes that all information is equally important and aims to protect it uniformly well. However, in many scenarios such as wireless networks, where sufficient error protection becomes a luxury, providing such a uniform protection to all the information may be either wasteful or infeasible. Instead, it is more efficient to protect a crucial part of information better than the rest. This research developed a general theoretical framework for a variety of such situations, characterized the fundamental limits, and found optimal strategies. Geometry in Information Theory•Problems in multi-user information theory often involve optimization of Kullback-Leibler divergence over probability distributions. Viewing probability distributions as points on a manifold, a geometric approach reveals the structure of these optimum solutions. We took this approach and studied error exponent problems based on a simple Pythgoras-like theorem for KL divergence. We later used a Euclidean approximation for KL divergence to simplify this geometry. With this simplification, we solved the open problem of broadcasting with degraded message sets as a canonical example of network information theory problems.Wireless Communication at Low SNR (“Writing on Fading Paper”)•Developed optimal communication schemes for a low power wireless system, where the receiver is dumb and the transmitter is smart but has limited power. Also resolved an open problem about dirty-paper coding with causal channel state information.Page 1 of 3Value of Coordination in a Network•Investigated the effects of limited coordination between relay nodes in a wireless network and showed that some simple relay operations are optimal at low noise levels. Also found some new properties for eigenvalues of a product of matrices.Swiss Federal Institute of Technology (EPFL), Switzerland. Summer 2004 Visiting Research Intern – Laboratory of Information TheoryMentor: Prof. Emre TelatarUsing Feedback for Communication at Large Noise Levels•In the limit of low power, characterized the information theoretic capacity of a wireless channel with feedback.Note that signal estimation is difficult at large noise levels but is crucial nonetheless for higher energy efficiency. EE, IIT Bombay 2001 – 2002 Undergraduate researchAdaptive Algorithms for Signal Estimation•Developed a fast adaptive algorithm for multi-user detection in wireless systems using minimum entropy method. Swiss Federal Institute of Technology (EPFL), Switzerland. Summer 2001 Summer Intern – Laboratory of Information TheoryMentors: Prof. Emre Telatar and Prof. Rüdiger UbrankeNetwork Information Flow•Resolved the converse for the network coding problem conjectured by Ahlswede et al. For a large network class, it proved the optimality of traditional routing—no need for any network coding.Teaching ExperienceEECS, MIT Spring 2004 Teaching Assistant – Wireless CommunicationsInstructors: Prof. Gregory Wornell and Prof. Lizhong Zheng•Involved in development of this new course in its first year. Developed class notes from course lectures, which were distributed weekly to the class. Developed new problems for exams and problem sets. Graded exams.EECS, MIT Fall 2003 Teaching Assistant – Digital CommunicationsInstructor: Prof. Robert Gallager•Designed new problems for exams and problem sets, provided solutions, and managed course logistics. Graded problem sets. Tutored the class of 40 students through office hours and review sessions.Professional ExperienceD. E. Shaw and Co., New York, NY. Summer 2007 Quantitative Analyst Intern•Applied information theoretic concepts for efficiently predicting very noisy data in a causal manner. Also investigated numerous other approaches from machine learning, signal processing, and statistics.Hewlett Packard Laboratories,Palo Alto, CA. Summer 2006 Visiting Research Intern – Media Systems LabsMentor: Dr. Mitchell Trott•Designed optimal schedulers for broadcasting common media to wireless users using convex and linear programming to. Computational cost was reduced using set theory and coding theory.Qualcomm Inc., San Diego, CA. Summer 2005 Engineering Intern – Corporate R & D division•Developed an iterative receiver algorithm for wireless systems. It reduced the computational complexity without any performance.degradationinPage 2 of 3Professional Service•Coordinator of Annual LIDS Colloquium Series in 2006-2007•Coordinator of LIDS Student Conference 2003.•Reviewer for IEEE Transactions on Information Theory, IEEE International Symposium on Information Theory, IEEE International Conference on Communications.PublicationsJournal Papers:•S. Borade, L. Zheng, R. Gallager, “Amplify and forward in wireless relay networks: rate, diversity and network size”, IEEE Trans. on Info. Theory, Special Issue on Relaying and Cooperation in Comm. Networks, Oct. 2007.•S. Borade, L. Zheng, “Unequal error protection: an information theoretic approach", to be submitted.•S. Borade, L. Zheng, “Euclidean information theory”, to be submitted.•S. Borade, L. Zheng, “Wideband fading channels with feedback: writing on fading paper and other optimal strategies”, to be submitted.Conference Papers:•S. Borade, L. Zheng, B. Nakiboglu “Unequal error protection: some fundamental limits and optimal strategies”, Information Theorey and Applications Workshop, UCSD, Jan. 2008.•S. Borade, L. Zheng, “Euclidean information theory”, Allerton Conference, Sept. 2007. (Invited)•S. Borade, L. Zheng, and M. Trott, “Multilevel broadcast networks”, IEEE Intl. Symp. on Info. Theory, June 2007.•S. Borade, L. Zheng, "On geometry of error exponents", Allerton Conference, Oct. 2006. (Invited)•S. Borade, L. Zheng, "Writing on fading paper and causal transmitter CSI", IEEE Intl. Symp.on Info. Theory, 2006.•S. Borade, L. Zheng, "Wideband Fading Channels with Feedback", Allerton Conference, Oct. 2004. (Invited)•S. Borade, L. Zheng and R. Gallager, "Maximizing Degrees of Freedom in Wireless Networks", Allerton Conference, Oct. 2003.•S. Borade, "Network information flow: limits and achievability", IEEE Intl. Symp. on Info. Theory,July 2002. ReferencesAvailable upon requestPage 3 of 3。
布谷鸟算法里发现概率英文表达
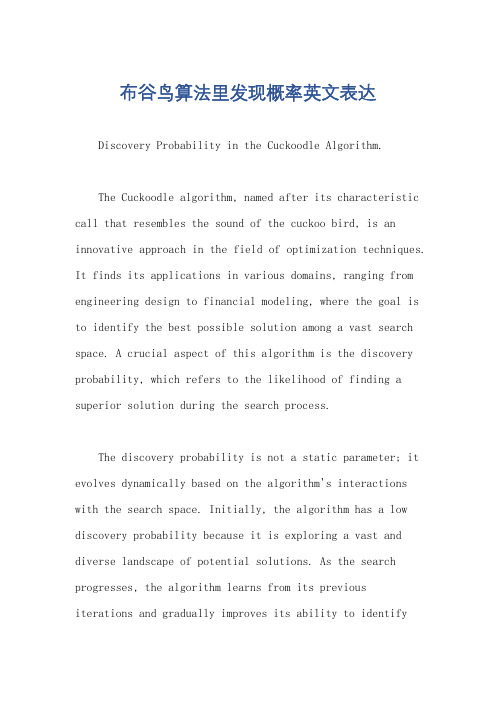
布谷鸟算法里发现概率英文表达Discovery Probability in the Cuckoodle Algorithm.The Cuckoodle algorithm, named after its characteristic call that resembles the sound of the cuckoo bird, is an innovative approach in the field of optimization techniques. It finds its applications in various domains, ranging from engineering design to financial modeling, where the goal is to identify the best possible solution among a vast search space. A crucial aspect of this algorithm is the discovery probability, which refers to the likelihood of finding a superior solution during the search process.The discovery probability is not a static parameter; it evolves dynamically based on the algorithm's interactions with the search space. Initially, the algorithm has a low discovery probability because it is exploring a vast and diverse landscape of potential solutions. As the search progresses, the algorithm learns from its previousiterations and gradually improves its ability to identifypromising regions. This improvement is reflected in an increasing discovery probability.The Cuckoodle algorithm employs several strategies to enhance its discovery probability. One such strategy is the utilization of heuristic rules, which guide the search towards regions that are more likely to contain optimal solutions. These rules are derived from past experiences and domain-specific knowledge, enabling the algorithm to make informed decisions about where to explore next.Another key aspect is the balance between exploration and exploitation. Exploration involves searching for new and potentially better solutions, while exploitation focuses on refining the current best solution. The Cuckoodle algorithm strikes a careful balance between these two objectives, ensuring that it doesn't get stuck in local optima while also maintaining the ability to discover globally optimal solutions.The discovery probability is also influenced by the diversity of the search population. In the Cuckoodlealgorithm, a population of candidate solutions evolves over time, with each individual representing a potentialsolution to the problem. By maintaining a diverse population, the algorithm increases its chances of discovering novel and innovative solutions. Techniques such as crossover and mutation are employed to introduce genetic diversity among the population members, enabling the algorithm to explore a broader range of solutions.The evaluation function plays a crucial role in determining the discovery probability. This function assigns a fitness score to each candidate solution, indicating its proximity to the optimal solution. By continuously evaluating and comparing the fitness scores, the algorithm can identify regions that are rich in promising solutions, thus increasing the discovery probability.The Cuckoodle algorithm also incorporates learning mechanisms that enable it to adapt and improve its discovery probability over time. By analyzing thehistorical data and patterns, the algorithm can learn fromits past successes and failures, refining its search strategies and becoming more efficient at finding optimal solutions.In summary, the discovery probability is a fundamental aspect of the Cuckoodle algorithm that governs its ability to find the best possible solution in a given search space. Through dynamic adaptation, heuristic rules, exploration-exploitation balance, population diversity, evaluation functions, and learning mechanisms, the algorithm continuously enhances its discovery probability, ensuring efficient and effective optimization.。
Surveying and comparing simultaneous sparse

Surveying and comparing simultaneous sparse approximation (or group-lasso) algorithms
A. Rakotomamonjy1,∗
LITIS EA4108, University of Rouen
Abstract In this paper, we survey and compare different algorithms that, given an overcomplete dictionary of elementary functions, solve the problem of simultaneous sparse signal approximation, with common sparsity profile induced by a ℓp − ℓq mixed-norm. Such a problem is also known in the statistical learning community as the group lasso problem. We have gathered and detailed different algorithmic results concerning these two equivalent approximation problems. We have also enriched the discussion by providing relations between several algorithms. Experimental comparisons of several of the detailed algorithms have also been carried out. The main lesson learned from these experiments is that depending on the performance measure, greedy approaches and iterative reweighted algorithms are the efficient algorithms either in term of computational complexities or in term of sparsity recovery. Keywords: simultaneous sparse approximation, block sparse regression, group lasso, iterative reweighted algorithms
rtk定位算法流程
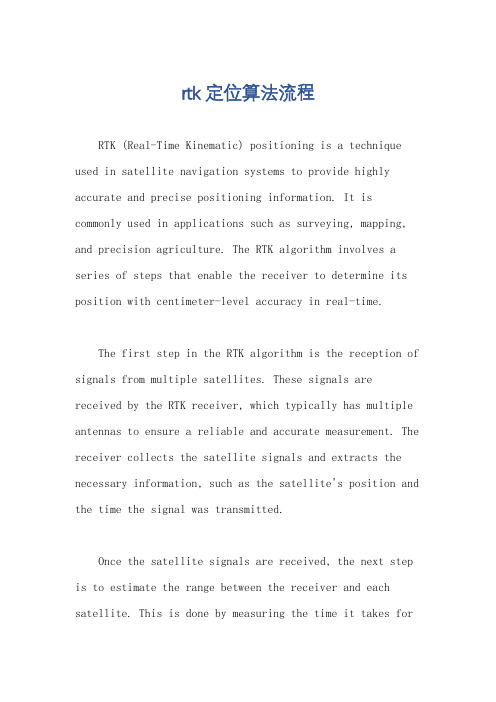
rtk定位算法流程RTK (Real-Time Kinematic) positioning is a technique used in satellite navigation systems to provide highly accurate and precise positioning information. It is commonly used in applications such as surveying, mapping, and precision agriculture. The RTK algorithm involves a series of steps that enable the receiver to determine its position with centimeter-level accuracy in real-time.The first step in the RTK algorithm is the reception of signals from multiple satellites. These signals are received by the RTK receiver, which typically has multiple antennas to ensure a reliable and accurate measurement. The receiver collects the satellite signals and extracts the necessary information, such as the satellite's position and the time the signal was transmitted.Once the satellite signals are received, the next step is to estimate the range between the receiver and each satellite. This is done by measuring the time it takes forthe signals to travel from the satellite to the receiver. By knowing the speed of light, the receiver can calculate the distance between itself and each satellite. However, this initial range measurement is subject to errors due to factors such as atmospheric conditions and clock errors.To improve the accuracy of the range measurements, the RTK algorithm employs a technique called carrier phase measurement. In this technique, the receiver measures the phase of the carrier wave of the satellite signal. By comparing the phase measurements over time, the receiver can determine the carrier phase ambiguity, which is an integer number of carrier wave cycles. Resolving this ambiguity is crucial for achieving centimeter-level accuracy in RTK positioning.The next step in the RTK algorithm is the estimation of the receiver's position. This is done by solving a set of equations that relate the range measurements to the receiver's position. The equations take into account the positions of the satellites, the range measurements, and the carrier phase measurements. The receiver uses aniterative algorithm, such as the least squares method, to find the best estimate of its position that minimizes the residuals between the predicted and measured ranges.Once the receiver's position is estimated, the final step in the RTK algorithm is the correction of any remaining errors. These errors can be caused by factors such as ionospheric and tropospheric delays, multipath interference, and satellite clock errors. To correct for these errors, the receiver uses information from a reference station, which is a stationary receiver with known coordinates. The reference station measures the same satellite signals as the mobile receiver but does notsuffer from the same errors. The reference station sends correction data to the mobile receiver, which applies these corrections to improve the accuracy of its position estimate.In conclusion, the RTK positioning algorithm involves a series of steps, including satellite signal reception, range measurement, carrier phase measurement, position estimation, and error correction. These steps work togetherto provide real-time, centimeter-level accuracy in determining the receiver's position. The algorithm combines measurements from multiple satellites and uses iterative techniques and correction data from a reference station to improve the accuracy and reliability of the positioning solution. RTK positioning has revolutionized applications that require high-precision positioning, enabling advancements in fields such as surveying, mapping, and precision agriculture.。
迭代检测算法的具体检测流程
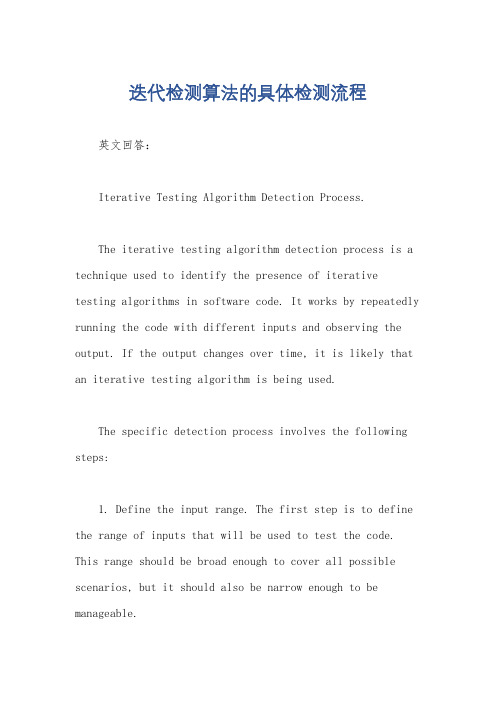
迭代检测算法的具体检测流程英文回答:Iterative Testing Algorithm Detection Process.The iterative testing algorithm detection process is a technique used to identify the presence of iterativetesting algorithms in software code. It works by repeatedly running the code with different inputs and observing the output. If the output changes over time, it is likely that an iterative testing algorithm is being used.The specific detection process involves the following steps:1. Define the input range. The first step is to define the range of inputs that will be used to test the code. This range should be broad enough to cover all possible scenarios, but it should also be narrow enough to be manageable.2. Run the code with the input range. Once the input range has been defined, the code should be run with each input in the range. The output of each run should be recorded.3. Compare the outputs. After the code has been run with each input, the outputs should be compared. If the outputs change over time, it is likely that an iterative testing algorithm is being used.4. Identify the iterative testing algorithm. Once it has been determined that an iterative testing algorithm is being used, the next step is to identify the specific algorithm that is being used. This can be done by examining the code and looking for patterns that are characteristic of iterative testing algorithms.中文回答:迭代检测算法检测流程。
基于信道信息的无线通信接入认证技术研究

1. 提出了一种基于信道信息的物理层认证方案,与上层认证技术相结合,构 成一种跨层认证方案。该方案选取基于基扩展模型的信道估计算法,用于探测时变 信道信息,提高时变信道下物理层认证的成功率。仿真实验还表明,基于基扩展模 型信道信息的物理层认证方案随着信噪比的增加,检测率不断提高,并且该方案在 多普勒频移较高时,认证性能依然很好,适用于时变环境中。
3. 将物理层认证用于检测 MIMO-OFDM 中的欺骗攻击。本文针对检测门限难
以确定的问题,提出一种基于-贪婪策略的检测门限确定方案。最后,在软件无线
电外设平台上搭建欺骗检测的环境,用于检测 MIMO-OFDM 系统中用物理层认证 进行欺骗检测的性能。
关键词:物理层认证,认证和密钥协商, 基扩展模型,信道估计,欺骗检测
2. 针对目前 3G/4G 采用认证和密钥协商协议存在的安全缺陷,本文提出将轻 量级的物理层认证运用到 5G 系统的两种方案。两种方案中物理层认证的首个数据 帧均需要上层认证协议完成,方案一后续数据帧增加物理层认证进行完整性保护, 方案二在双向认证中同时使用上层认证和物理层认证来增强安全性,两种方案分 别满足不同场景认证需求。
II
ABSTRACT
authentication scheme based on channel information improves with the increase of the signal-to-noise ratio and when the Doppler frequency shift is high, the authentication performan this scheme is applicable to time-varying environments.
OFDM系统中的一种低复杂度带状ICI抑制算法

OFDM系统中的一种低复杂度带状ICI抑制算法陈东华;仇洪冰【摘要】Aiming at mitigating intercarrier interference (ICI) caused by channel time variation in orthogonal frequency division multiplexing (OFDM) systems, a low-complexity doubly iterative equalization scheme is proposed by exploiting the approximately banded structure of channel frequency response (CFR) matrix. The scalability of band size of CFR matrix enables a good tradeoff between performance and complexity. In this scheme, a linear ICI canceller is used to reduce performance degradation caused by the band approximation of CFR matrix, and an iterative equalizer with soft interference cancellation is employed to gain the Doppler diversity induced by channel time variation. Theoretic analysis and simulation results indicate that the proposed technique has both performance and complexity advantages over the classic linear minimum mean square error (MMSE) equalizer in time varying channels.%针对时变信道OFDM系统中的子载波间干扰(ICI)抑制问题,基于时变信道频城响应矩阵(CFR)的带状近似,提出一种低复杂度双迭代均衡方案.通过调整CFR矩阵的带宽大小,有效实现了性能和复杂度之间的良好折中.在检测过程中,首先利用线性ICI抵消降低了由于CFR矩阵的带状近似造成的性能恶化,其次通过迭代软干扰抵消检测算法来获得由信道时变带来的多普勒分集增益.理论分析和仿真结果表明,同传统线性最小均方误差时变信道均衡算法相比,该算法同时具有性能和复杂度的优势.【期刊名称】《电子科技大学学报》【年(卷),期】2011(040)004【总页数】5页(P519-523)【关键词】均衡器;子载波间干扰;正交频分复用;时变信道【作者】陈东华;仇洪冰【作者单位】华侨大学信息科学与工程学院福建厦门362021;西安电子科技大学通信工程学院西安710071;华侨大学信息科学与工程学院福建厦门362021;桂林电子科技大学信息与通信学院广西桂林541004【正文语种】中文【中图分类】TN911.5在高速移动通信中,无线信道的时变引起的多普勒频移会破坏OFDM系统子载波之间的正交性,导致子载波间干扰(ICI)并恶化系统性能[1]。
LTE论文:迭代接收技术在LTE中的应用

LTE论文:迭代接收技术在LTE中的应用【中文摘要】随着无线通信技术的快速发展,LTE技术已逐渐走上商用的道路,LTE技术的提出是为了满足信息社会人们对数据传输的大规模需求,为此,LTE标准采用了MIMO、OFDM等先进技术以在峰值速率、传输时延和频谱利用率等方面达到一个新的高度,LTE的目标是为未来十年或十年以上提供有竞争力的无线通讯解决方案。
在LTE接收端传统的实现方案中,MIMO检测与Turbo译码是单独进行的,随着基于概率的软判决迭代检测思想的广泛应用,若能在LTE接收端将MIMO检测与Turbo译码以迭代接收机的形式进行迭代检测将获得巨大的检测增益,提升接收端的性能,因此有必要研究LTE系统中的迭代接收机技术。
本文首先介绍了LTE系统中迭代接收机的系统模型,然后分别研究了迭代接收机中的Turbo迭代译码技术和软输入软输出MIMO检测技术。
本文给出了Turbo迭代译码中Log-Map译码算法的常见简化方法,并针对LLR计算单元提出了一种简化算法,仿真结果表明,相比Max-Log-Map算法,该算法在增加有限的复杂度下取得了0.2dB的性能提升。
本文采用CUDA平台研究实现了基于GPU的Turbo迭代译码器,测试结果表明该迭代译码器能取得4.8Mbps的净吞吐率,能满足低速实时数据传输需求,采用GPU实现Turbo迭代译码器能降低开发成本同时缩短开发周期,该技术可用于软件无线电中,也可用于Turbo码的仿真加速领域中,能上百倍地降低Turbo码的仿真时间。
针对LTE系统支持高速数据传输的需求,本文研究实现了基于FPGA的高吞吐率Turbo迭代译码器,该译码器能适应LTE系统中的全部188种码长,在该Turbo迭代译码器中,本文针对LTE中QPP交织器的特点提出了一种适合于并行译码的QPP交织器实现方法与硬件架构,相比基于存储的方法所需要的8Mbits左右的存储资源,本文提出的方法只需要1692bits的存储资源,具有很大的优越性;结果表明,本文设计的Turbo迭代译码器在资源消耗,译码延时,数据吞吐率等各项指标上都满足了LTE的需求。
- 1、下载文档前请自行甄别文档内容的完整性,平台不提供额外的编辑、内容补充、找答案等附加服务。
- 2、"仅部分预览"的文档,不可在线预览部分如存在完整性等问题,可反馈申请退款(可完整预览的文档不适用该条件!)。
- 3、如文档侵犯您的权益,请联系客服反馈,我们会尽快为您处理(人工客服工作时间:9:00-18:30)。
1. duction
The use of multiple transmit antennas in conjunction with space-time coding techniques, has in recent years been recognized as a powerful method to combat the e ects of signal fading in wireless communications 1 . For example, it has been demonstrated that for the transmit diversity scheme in 2 , using 2 transmit and m receive antennas, a diversity order of 2m is obtained. A generalization to more than two transmit antennas is given in 3 . The method in 2 assumes that perfect channel state information CSI is available at the receiver. Receiver algorithms for the realistic case when channel state information is not available, have been presented in 4, 5 . In 4 , it was concluded that the lack of CSI incurred approximately a 3 dB penalty in performance relative to the ideal case. An
2. Signal Model and Algorithm
We consider a wireless communication scenario where K users each transmit space-time encoded symbol sequences using n antennas. The noisy superpo-
xt =
K X √ k=1
In 2, we assume that user-1 is the desired signal and the quantity, K k=2 Hk Ck + N, is the multi-user interference plus AWGN. The receiver assumes no knowledge of array geometry or array calibration, and only works with the unstructured m × 2 channel Hk , which is implicitly dened in the model. Note that the assumption of K synchronous users is only made for purposes of formulating the model, and that the algorithm to be presented only requires synchronization to the desired signal. The user frame is partitioned into Np training symbols and N information symbols for a total frame length of NT = Np + N . The desired user code matrix is partitioned as C1 = [Cp |Cu ], where Cp is a 2 × Np matrix containing the encoded training symbols, and Cu = [c1,1 , . . . , c1,N ] is a 2 × N information symbol matrix, see Figure 2. Likewise, the received data is partitioned as X = [Xp |Xu ], where Xp is a m × Np matrix, and Xu = [x1 , . . . , xN ] is a m × N matrix.
θ2 θ1
X = [x1 , · · · , xNT ], N = [n1 , · · · , nNT ], and Ck = [ck,1 , · · · , ck,NT ], and for a total frame length of NT symbols, we may write 1 as
1
11 00
K
1
X = H1 C1 +
k=2
Hk Ck + N.
2
Figure 1. Diagram of receiving array and two users.
sition of all user signals impaired by frequency- at Rayleigh fading are received by an array of m antennas. The fading is assumed to be caused by scatterers in the neighborhood of the transmitting antennas. From the perspective of the receiving array, the fading is identical for each user transmit antenna. This models the fading seen from base stations located on high towers. Since the array elements cooperate on discriminating among the di erent users by providing spatial ltering, the receive array elements are correlated. Hence, only a diversity of order n is obtained. In what follows, we assume n = 2 transmit antennas for ease of presentation. A diagram of the receiving array with two users in the eld is presented in Figure 1, where the angles of arrival and angles of separation for user k are denoted by θk and δk , respectively. With negligible delay-spread, the synchronously sampled output of the receiver matched- lter at time t can thus be written as
An Iterative Receiver Algorithm for Space-Time Encoded Signals∗
Anders Ranheim†, Andr P. des Rosiers‡, Paul H. Siegel‡ Department of Signals and Systems Chalmers University of Technology, 412 96 G teborg, Sweden. ‡ Signal Transmission and Recording Group Department of Electrical and Computer Engineering University of California, San Diego, La Jolla, CA 92093-0407. Email: ranheim@s2.chalmers.se, adesrosi, psiegel @
†
Abstract
An iterative receiver is proposed for a wireless communication system employing multiple transmit and receive antennas. The transmitted symbol sequences are space-time encoded. By exploiting the inherent structure in the space-time encoded data sequence, the receiver is able to signi cantly improve the initial estimate of the unknown channel leading to significant performance gains in terms of the bit-error-rate BER as a function of the signal-to-noise ratio SNR. Computer simulations demonstrate the e cacy of the scheme in single-user and multi-user environments.
∗
expectation-maximization EM scheme was suggested in 5 and studied the e ect of pilot-symbol spacing in fading environments. These references are concerned with single-user scenarios in the presence of additive white Gaussian noise AWGN. Multiuser methods using space-time block codes for interference suppression have been presented in 6 , but assume perfect CSI at the receiver. The method presented here carries out detection and decoding of the transmitted symbols based on the initial channel estimates from a few training symbols. The quality of the CSI is then improved by employing the detected and re-encoded symbols, leading to signi cant improvement in BER relative to the known channel case. The proposed method is block based and assumes that the fading parameters are constant during each frame and vary from one frame to another block fading. Simulation results indicate that our method performs similarly to the EM technique for the case of a single user, while relying on fewer assumptions and less side-information, speci cally the transmit power. Furthermore, in a scenario with several users, results show that relying only on training symbols gives very poor performance. The proposed algorithm is able to signi cantly reduce the BER with only a small number of iterations. Computer simulation examples include both space-time block and trellis coded signals.