Golay Code Clustering for Mobility Behavior Similarity Classification in Pocket Switched Network
LTE_3GPP_36.213-860(中文版)
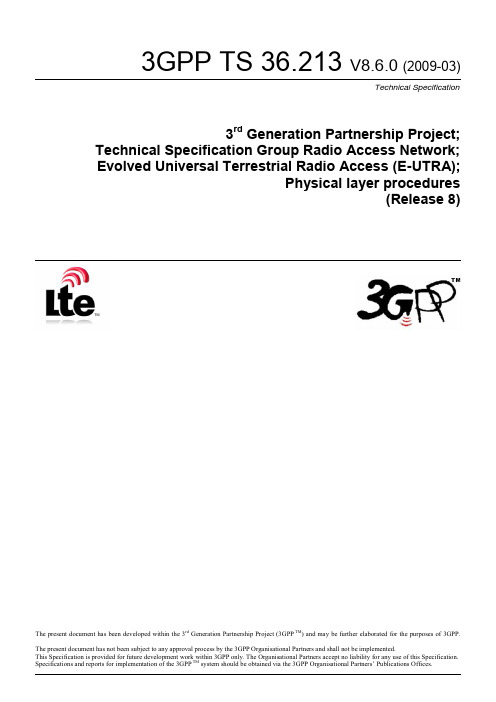
3GPP
Release 8
3
3GPP TS 36.213 V8.6.0 (2009-03)
Contents
Foreword ...................................................................................................................................................... 5 1 2 3
Internet
Copyright Notification No part may be reproduced except as authorized by written permission. The copyright and the foregoing restriction extend to reproduction in all media.
© 2009, 3GPP Organizational Partners (ARIB, ATIS, CCSA, ETSI, TTA, TTC). All rights reserved. UMTS™ is a Trade Mark of ETSI registered for the benefit of its members 3GPP™ is a Trade Mark of ETSI registered for the benefit of its Members and of the 3GPP Organizational Partners LTE™ is a Trade Mark of ETSI currently being registered for the benefit of i ts Members and of the 3GPP Organizational Partners GSM® and the GSM logo are registered and owned by the GSM Association
An Overview of Internet of Vehicles 车联网综述
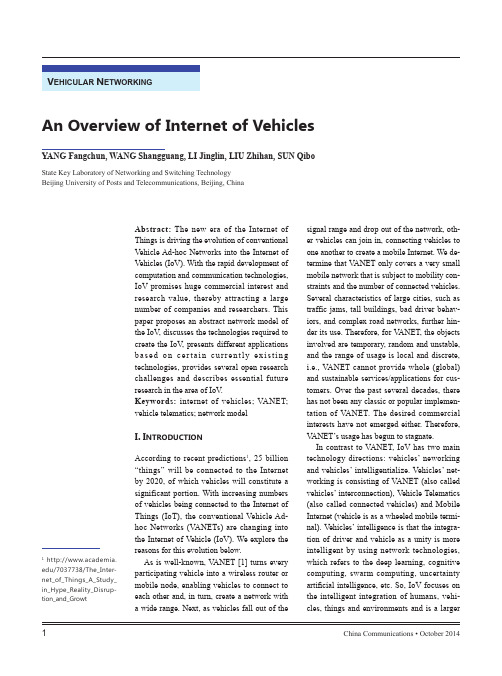
signal range and drop out of the network, oth-er vehicles can join in, connecting vehicles to one another to create a mobile Internet. We de-termine that V ANET only covers a very small mobile network that is subject to mobility con-straints and the number of connected vehicles. Several characteristics of large cities, such as traffic jams, tall buildings, bad driver behav-iors, and complex road networks, further hin-der its use. Therefore, for V ANET, the objects involved are temporary, random and unstable, and the range of usage is local and discrete, i.e., VANET cannot provide whole (global) and sustainable services/applications for cus-tomers. Over the past several decades, there has not been any classic or popular implemen-tation of VANET. The desired commercial interests have not emerged either. Therefore, V ANET’s usage has begun to stagnate.In contrast to VANET, IoV has two main technology directions: vehicles’ neworking and vehicles’ intelligentialize. Vehicles’ net-working is consisting of V ANET (also called vehicles’ interconnection), Vehicle Telematics (also called connected vehicles) and Mobile Internet (vehicle is as a wheeled mobile termi-nal). Vehicles’ intelligence is that the integra-tion of driver and vehicle as a unity is more intelligent by using network technologies, which refers to the deep learning, cognitive computing, swarm computing, uncertainty artificial intelligence, etc. So, IoV focuses on the intelligent integration of humans, vehi-cles, things and environments and is a largerAbstract: The new era of the Internet of Things is driving the evolution of conventional Vehicle Ad-hoc Networks into the Internet of Vehicles (IoV). With the rapid development of computation and communication technologies, IoV promises huge commercial interest and research value, thereby attracting a large number of companies and researchers. This paper proposes an abstract network model of the IoV , discusses the technologies required to create the IoV , presents different applications b a s e d o n c e r t a i n c u r r e n t l y e x i s t i n g technologies, provides several open research challenges and describes essential future research in the area of IoV .Keywords: internet of vehicles; VANET; vehicle telematics; network modelI. I NTRODUCTIONAccording to recent predictions 1, 25 billion “things” will be connected to the Internet by 2020, of which vehicles will constitute a significant portion. With increasing numbers of vehicles being connected to the Internet of Things (IoT), the conventional Vehicle Ad-hoc Networks (VANETs) are changing into the Internet of Vehicle (IoV). We explore the reasons for this evolution below.As is well-known, V ANET [1] turns every participating vehicle into a wireless router or mobile node, enabling vehicles to connect to each other and, in turn, create a network with a wide range. Next, as vehicles fall out of theAn Overview of Internet of VehiclesYANG Fangchun, WANG Shangguang, LI Jinglin, LIU Zhihan, SUN QiboState Key Laboratory of Networking and Switching Technology Beijing University of Posts and Telecommunications, Beijing, ChinaV EHICULAR N ETWORKING1/7037738/The_Inter-net_of_Things_A_Study_in_Hype_Reality_Disrup-tion_and_Growtthe conventional Vehicle Ad-hoc Networks (V ANETs), Vehicle Telematics, and other con-nected vehicle networks have to evolve into the Internet of Vehicle (IoV). The question accordingly arises as to why such systems did not evolve into IoT, Internet or wireless mo-bile networks.The main reason is that some characteris-tics of IoV are different from IoT, Internet or wireless mobile networks. Firstly, in wireless mobile networks, most end-users’ trajectories follow a random walk model. However, in IoV , the trajectory of vehicles is subject to the road distributions in the city. Secondly, IoT focuses on things and provides data-aware-ness for connected things, while the Internet focuses on humans and provides information services for humans. However, IoV focuses on the integration of humans and vehicles, in which, vehicles are an extension of a human’s abilities, and humans are an extension of a vehicle’s intelligence. The network model, the service model, and the behavior model of human-vehicle systems are highly different from IoT, Internet or wireless mobile network. Finally, IoV interconnects humans within and around vehicles, intelligent systems on board vehicles, and various cyber-physical systems in urban environments, by integrating vehi-cles, sensors, and mobile devices into a global network, thus enabling various services to be delivered to vehicles and humans on board and around vehicles. Several researchers have referred to the vehicle as a manned computer with four wheels or a manned large phone in IoV. Thus, in contrast to other networks, ex-isting multi-user, multi-vehicle, multi-thing and multi-network systems need multi-level collaboration in IoV .In this paper, we first provide a network model of IoV using the swarm model and an individual model. We introduce existing re-search work focusing on activation and main-tenance of IoV. Then, we survey the various applications based on some currently existing technologies. Finally, we give several open re-search challenges for both the network model and the service model of human-vehicle sys-network that provides services for large cities or even a whole country. IoV is an open and integrated network system with high manage-ability, controllability, operationalization and credibility and is composed of multiple users, multiple vehicles, multiple things and multiple networks. Based on the cooperation between computation and communication, e.g., col-laborative awareness of humans and vehicles, or swarm intelligence computation and cog-nition, IoV can obtain, manage and compute the large scale complex and dynamic data of humans, vehicles, things, and environments to improve the computability, extensibility and sustainability of complex network systems and information services. An ideal goal for IoV is to finally realize in-depth integration of human-vehicle-thing-environment, reduce social cost, promote the efficiency of trans-portation, improve the service level of cities, and ensure that humans are satisfied with and enjoy their vehicles. With this definition, it is clear that V ANET is only a sub network of IoV. Moreover, IoV also contains Vehicle Telematics [2], which is a term used to define a connected vehicle interchanging electronic data and providing such information services as location-based information services, remote diagnostics, on-demand navigation, and au-dio-visual entertainment content. For IoV , Ve-hicle Telematics is simply a vehicle with more complex communication technologies, and the intelligent transportation system is an applica-tion of IoV , but vehicle electronic systems do not belong to IoV .In the last several years, the emergence of IoT, cloud computing, and Big Data has driven demand from a large number of users. Individ-ual developers and IT enterprises have pub-lished various services/applications. However, because V ANET and Vehicle Telematics lack the processing capacity for handling global (whole) information, they can only be used in short term applications or for small scale ser-vices, which limits the development and popu-lar demand for these applications on consumer vehicles. There is a desperate need for an openand integrated network system. Therefore,people who consume or provide services/ap-plications of IoV . Human do not only contain the people in vehicles such as drivers and pas-sengers but also the people in environment of IoV such as pedestrians, cyclists, and drivers’ family members. Vehicle in IoV terminology refers to all vehicles that consume or provide services/applications of IoV . Thing in IoV ter-minology refers to any element other than hu-man and vehicle. Things can be inside vehicles or outside, such as AP or road. Environment refers to the combination of human, vehicle and thing.The individual model focuses on one vehi-cle. Through the interactions between human and environment, vehicle and environment, and thing and environment, IoV can provide services for the vehicles, the people and the things in the vehicles. In the model, the in-tra-vehicle network is used to support the interaction between human and vehicle, and the interaction between vehicle and thing in that vehicle. The inter-vehicle network is usedtems, i.e., enhanced communication through computation and sustainability of service pro-viding, and outline essential future research work in the area of IoV .The rest of this paper is organized as fol-lows. Section 2 describes o ur proposed net-work model of IoV. The overview of IoV is presented from three different perspectives in Section 3. In Section 4, several open research challenges and essential future research work related to IoV are outlined. Finally, we present this paper’s conclusions in Section 5.II. N ETWORK M ODEL OF IOVAs shown in Fig. 1, we propose a network model of IoV based on our previous work [3], in which the model is composed of a swarm model and an individual model. The key as-pect of the network model is the integration between human, vehicle, thing, and environ-ment.Human in IoV terminology refers to all theFig.1Network model of IoVsignificantly improve the quality of vehicle service, while a bad wireless access may often lead to the breakdown of services. As is well-known, routing technology is the research core of traditional networks. For IoV , while routing is still the core of the inter-vehicle network, it is also essential for delivering the control message. Finally, IoV has the two most im-portant elements, i.e., users and network. For a simple IoV, wireless access is its user, and routing is its network. With the development of IoV , however, these elements might be less important, and other technologies may play a vital role, such as collaboration technology and swarm intelligence computing. However, due to page limitations, a detailed discussion is beyond this paper.Note that the technologies introduced in this section cannot cover the technologies of IoV , and most of them belong to V ANET [4] or Ve-hicle Telematics. The reason is that IoV is an open and integrated network system composed of multiple users, multiple vehicles, multiple things and multiple networks, and an integrat-ed IoV is not described. Hence, this section mainly focuses on existing technologies and applications, even if they do not represent the technologies and applications of IoV .3.1 Activation of IoVThere are many steps in the activation of IoV , but the most important step is to take the vehi-cles into the integrated network of IoV using wireless access technologies. At present, there are many existing wireless access technologies such as WLANs, WiMAX, Cellular Wireless, and satellite communications [5]. As shown in Fig. 1, most of these technologies are used to connect vehicles to each other in IoV .WLAN contains IEEE 802.11a/b/g/n/p standards. IEEE 802.11-based WLAN, which has achieved great acceptance in the market, supports short-range, relatively high-speed data transmission. The maximum achievable data rate in the latest version (802.11n) is ap-proximately 100 Mbps. IEEE 802.11p is a new communication standard in the IEEE 802.11 family which is based on the IEEE 802.11a.to support the interaction between human and environment, vehicle and environment, and thing and environment. Swarm model focus-es on multi-user, multi-vehicle, multi-thing and multi-network scenarios. Through swarm intelligence, crowd sensing and crowd sourc-ing, and social computing, IoV can provide services/applications. Moreover, in this model, the interaction between human and human, ve-hicle and vehicle, and thing and thing, all need an integrated network to collaborate with each other and with the environment. Note that IoV has a computation platform for providing vari-ous decisions for whole network, and there are many virtual vehicles with drivers correspond-ing to physica vehicles and drivers. Then we call the virtual vehicle with driver as Autobot. In the IoV, Autobot can interact with each other by using swarm computing technologies and provide decision-making information for IoV in the computation platform.III. T ECHNOLOGY AND A PPLICATION OF IOVOver a decade ago, both industrial and aca-demic researchers proposed many advanced technologies for the application layer, the mo-bile model & the channel model, the physical layer & the data link layer, the network layer & the transport layer, and security & privacy; these technologies are all used in IoV . In this section, we only focus on giving an overview of the technologies and their applications in IoV, and do not describe the details of the technologies. The overview describes the acti-vation of the IoV , maintenance of the IoV , and IoV applications.For the activation and maintenance of the IoV, we only summarize the wireless access technology and the routing technology. There are several reasons for focusing on these two technologies. Firstly, most researchers working on IoV focus on wireless access and routing, for which the number of proposed re-search works are the highest. Secondly, wire-less access technologies play an important role in IoV . A good wireless access technology canquality of service, even for non-line-of-sight transmissions. The key advantage of WiMAX compared to WLAN is that the channel access method in WiMAX uses a scheduling algo-rithm in which the subscriber station needs to compete only once for initial entry into the network.Cellular wireless comprises of 3G, 4G and LTE. Current 3G networks deliver data at a rate of 384 kbps to moving vehicles, and can go up to 2 Mbps for fi xed nodes. 3G sys-tems deliver smoother handoffs compared to WLAN and WiMAX systems, and many nota-ble works have been proposed. For example, Chao et al. [8] modeled the 3G downloading and sharing problem in integration networks. Qingwen et al. [9] made the first attempt in exploring the problem of 3G-assisted data delivery in V ANETs. However, due to central-ized switching at the mobile switching center (MSC) or the serving GPRS support node (SGSN), 3G latency may become an issue for many applications. Vinel [3] provided an an-IEEE 802.11p is designed for wireless access in the vehicular environment to support intel-ligent transport system applications. The use of wireless LANs in V ANETs requires further research. For example, Wellens et al. [6] pre-sented the results of an extensive measure-ment campaign evaluating the performance of IEEE 802.11a, b, and g in car communication scenarios, and showed that the velocity has a negligible impact, up to the maximum tested speed of 180 km/h. Yuan et al. [7] evaluated the performance of the IEEE 802.11p MAC protocol applied to V2V safety communica-tions in a typical highway environment. Wi-MAX contains IEEE 802.16 a/e/m standards. IEEE 802.16 standard-based WiMAX are able to cover a large geographical area, up to 50 km, and can deliver significant bandwidth to end-users - up to 72 Mbps theoretically. While IEEE 802.16 standard only supports fixed broadband wireless communications, IEEE 802.16e/mobile WiMAX standard supports speeds up to 160 km/h and different classes of Fig.2 Wireless access technologies in IoVAPManagement and Control on the APIEEE 802.16WiMax DatabaseAP Management and Control on the APAPManagement and Control on the APCellular NetworkCellular NetworkDatabaseSatellite NetworkSatellite Network DatabaseAPManagement and Control on the APIEEE 802.11WLAN DatabaseVehicleVehicleVehicleVehicleCentralized Management and Control Unitand Control Unit (CMCU)NetworkDatabaseCMCUIoV3.2 Maintenance of IoVThere are also many aspects to the mainte-nance of IoVs, such as data-awareness, virtual networks, and encoding, but the most im-portant aspect is the switching of the control message for IoV. Routing technology is the suitable solution, and in IoV , is dependent on a number of factors such as velocity, density, and direction of motion of the vehicles. As shown in Fig. 1, vehicles can either be the source or the destination during the process of routing, and various standards have been built to accomplish the task of routing. With the growing needs of the users to access various resources during mobility, efficient techniques are required to support their needs and keep them satisfied.Topology based maintenance: Because of the large overhead incurred for route discovery and route maintenance for highly mobile unco-ordinated vehicles, only a few of the existing routing protocols for inter-vehicle networks are able to handle the requirements of safety applications [10,11]. An important group of routing protocols for ad-hoc networks is based on topology, and needs the establishment of an end-to-end path between the source and the destination before sending any data packet. Due to rapid changes in the network topology and highly varying communication channel conditions, the end-to-end paths determined by regular ad-hoc topology-based routing pro-tocols are easily broken. To solve this prob-lem, several routing protocols have been pro-posed [12,13] [14,15] [16] [17]. For example, Namboodiri and Gao [12] proposed a predic-tion-based routing for V ANETs. The PBR is a reactive routing protocol, which is specifically tailored to the highway mobility scenario, to improve upon routing capabilities without us-ing the overhead of a proactive protocol. The PBR exploits the deterministic motion pat-tern and speeds, to predict roughly how long an existing route between a “node” vehicle and a “gateway” vehicle will last. Using this prediction, the authors pre-emptively create new routes before the existing route lifetimealytical framework which allows comparing 802.11p/WA VE and LTE protocols in terms of the probability of delivering the beacon before the expiration of the deadline. Lei et al. [4] studied the potential use cases and technical design considerations in the operator con-trolled device-to-device communications. The potential use cases were analyzed and classi-fied into four categories. Each use case had its own marketing challenges and the design of related techniques should take these fac-tors into consideration. Gerla and Kleinrock [5] discussed LTE cellular service in a future urban scenario with very high bandwidth and broad range. The so-called cognitive radios will allow the user to be “best connected” all the time. For instance, in a shopping mall or in an airport lounge, LTE will become congested, and the user’s cognitive radio will disconnect from LTE. For vehicles, due to large costs, sat-ellite communications are barely used, except for GPS. It is only a supplement for temporary and emergency uses, when other communica-tion technologies are invalid or unavailable. Looking at the wireless access technologies described above, we think that the 4G or LTE should be the most efficient technology to launch the inter-vehicle network and to acti-vate the IoV . The reasons are as follows. First-ly, 4G or LTE is the most used communication standard, and has been deployed by most countries to provide access services. Obvious-ly, any vehicle can use it to connect to the IoV . Secondly, in the context of high buildings and a complex city environment, the performance of 4G or LTE is the best among all wireless access technologies. Finally, in the past ten years, the development of VANET has been very slow, and can barely be used in the real world. The main reason is that the connected vehicles cannot maintain V ANET in city roads because the goals of drivers are random and different. To maintain the V ANET, all vehicles must access the integrated network of IoV, after which IoV can be activated to provide services for users.which combines store-carry-and-forward tech-nique with routing decisions based on geo-graphic location. These geographic locations are provided by GPS devices. In GeoSpray, authors proposed a hybrid approach, mak-ing use of a multiple copy and a single copy routing scheme. To exploit alternate paths, GeoSpray starts with multiple copy schemes which spread a limited number of bundle cop-ies. Afterwards, it switches to a single copy scheme, which takes advantage of additional opportunities. It improves delivery success and reduces delivery delay. The protocol ap-plies active receipts to clear the delivered bun-dles across the network nodes. Compared with other geographic location-based schemes, and single copy and non-location based multiple copy routing protocols, it was found that Geo-Spray improves delivery probability and re-duces delivery delay. In contrast to the above work, Bernsen and Manivannan proposed [20] a routing protocol for V ANETs that utilizes an undirected graph representing the surrounding street layout, where the vertices of the graph are points at which streets curve or intersect, and the graph edges represent the street seg-ments between those vertices. Unlike existing protocols, it performs real-time, active traffic monitoring and uses these data and other data gathered through passive mechanisms to as-sign a reliability rating to each street edge. Then, considering the different environments, a qualitative survey of position-based rout-ing protocols was made in [21], in which the major goal was to check if there was a good candidate for both environments or not. An-other perspective was offered by Liu et al. [22], who proposed a relative position based message dissemination protocol to guarantee high delivery ratio with acceptable latency and limited overhead. Campolo et al. [23] used the time, space and channel diversity to improve the efficiency and robustness of network ad-vertisement procedures in urban scenarios. Clustering based maintenance: In this type of routing scheme, one of the nodes among the vehicles in the cluster area becomes a clusterhead (CH), and manages the rest ofexpires. Toutouh et al. [13] proposed a well-known mobile ad hoc network routing proto-col for V ANETs to optimize parameter settings for link state routing by using an automatic optimization tool. Nzounta et al.[15] proposed a class of road-based VANET routing proto-cols. These protocols leverage real-time ve-hicular traffic information to create paths. Fur -thermore, geographical forwarding allows the use of any node on a road segment to transfer packets between two consecutive intersections on the path, reducing the path’s sensitivity to individual node movements. Huang et al. [16] examined the efficiency of node-disjoint path routing subject to different degrees of path coupling, with and without packet redundancy. An Adaptive approach for Information Dis-semination (AID) in VANETs was presented in [14], in which each node gathered the in-formation on neighbor nodes such as distance measurements, fixed upper/lower bounds and the number of neighboring nodes. Using this information, each node dynamically adjusts the values of local parameters. The authors of this approach also proposed a rebroadcast-ing algorithm to obtain the threshold value. The results obtained show that AID is better than other conventional schemes in its cate-gory. Fathy et al. [17] proposed a QoS Aware protocol for improving QoS in VANET. The protocol uses Multi-Protocol Label Switching (MPLS), which runs over any Layer 2 technol-ogies; and routers forward packets by looking at the label of the packet without searching the routing table for the next hop.Geographic based maintaining. The geo-graphic routing based protocols rely mainly on the position information of the destination, which is known either through the GPS sys-tem or through periodic beacon messages. By knowing their own position and the destination position, the messages can be routed directly, without knowing the topology of the network or prior route discovery. V . Naumov et al. [18] specifically designed a position-based routing protocol for inter-vehicle communication in a city and/or highway environment. Soares et al. [19] proposed the GeoSpray routing protocol,dynamic transmission range, the direction of vehicles, the entropy, and the distrust value parameters. Wang et al. [26] refined the orig -inal PC mechanism and proposed a passive clustering aided mechanism, the main goal of which is to construct a reliable and stable clus-ter structure for enhancing the routing perfor-mance in V ANETs. The proposed mechanism includes route discovery, route establishment, and data transmission phases. The main idea is to select suitable nodes to become cluster-heads or gateways, which then forward route request packets during the route discovery phase. Each clusterhead or gateway candidate self-evaluates its qualification for clusterhead or gateway based on a priority derived from athe nodes, which are called cluster members. If a node falls in the communication range of two or more clusters, it is called a border node. Different protocols have been proposed for this scheme, and they differ in terms of how the CH is selected and the way the routing is done. R. S. Schwartz et al. [24] proposed a dissemination protocol suitable for both sparse and dense vehicular networks. Suppression techniques were employed in dense networks, while the store-carry-forward communication model was used in sparse networks. A. Daein-abi [25] proposed a novel clustering algorithm - vehicular clustering - based on a weighted clustering algorithm that takes into consider-ation the number of neighbors based on theavoidance. At present, collision avoidance technologies are largely vehicle-based systems offered by original equipment manufacturers as autonomous packages which broadly serve two functions, collision warning and driver assistance. The former warns the driver when a collision seems imminent, while the latter partially controls the vehicle either for steady-state or as an emergency intervention [41]. To be specific, collision warning includes notifi -cations about a chain car accident, warnings about road conditions such as slippery road, and approaching emergency vehicle warning [5]. On the one hand, collision warnings could be used to warn cars of an accident that oc-curred further along the road, thus presenting a pile-up from occurring. On the other hand, they could also be used to provide drivers with early warnings and prevent an accident from happening in the first place. Note that driving near and through intersections is one of the most complex challenges that drivers face be-cause two or more traffic flows intersect, and the possibility of collision is high [42]. The intelligent intersection, where such conven-tional traffic control devices as stop signs and traffic signals are removed, has been a hot area of research for recent years. Vehicles coordi-nate their movement across the intersection through a combination of centralized and dis-tributed real-time decision making, utilizing global positioning, wireless communications and in-vehicle sensing and computation 1. A number of solutions for collision avoidance of multiple vehicles at an intersection have been proposed. A computationally efficient control law [43-45] has been derived from ex-ploitation of the monotonicity of the vehicles’ dynamics, but it has not been applied to more than two vehicles. An algorithm that addresses multi-vehicle collisions, based on abstraction, has been proposed in [46]. An algorithmic ap-proach to enforcing safety based on a time slot assignment, which can handle a larger number of vehicles, is found in [41]. Colombo et al. [47] designed a supervisor for collision avoid-ance, which is based on a hybrid algorithm that employs a dynamic model of the vehiclesweighted combination of the proposed metrics. P. Miao [27] proposed a cooperative commu-nication aware link scheduling scheme, with the objective of maximizing the throughput for a session in C-V ANETs. They let the RSU schedule the multi-hop data transmissions among vehicles on highways by sending small sized control messages.Based on the above overview, we provide a relative comparison of all routing protocols in Tab 1. In this table, Route length is the total distance between source and destination. PDR is the packet delivery ratio. Latency is the in-terval of time between the first broadcast and the end of the last host’s broadcast. Latency includes buffering, queuing, transmission and propagation delays.3.3 IoV applicationsWith the rapid development of numeric infor-mation technology and network technology, it is brought forward that theautomatization and intelligentization of vehicle.This gives birth to lots of applications which combine safe driving with service provision. For example, Apple CarPlay, originally introduced as iOS in vehicles, offer full-on automobile integration for Apple’s Maps and turn-by-turn navigation, phone, iMeessage, and music service 5. Similar to CarPlay, Google Android Auto provides a distraction-free interface that allows drivers enjoy the services by connecting Android de-vices to the vehicle. Chinese Tecent recently launched its homegrown navigation app Lu-bao that features user generated contents and social functions 7. For demonstration purposes, in this paper, IoV applications can be divided into two major categories: Safety applications and User applications. Applications that in-crease vehicle safety and improve the safety of the passengers on the roads by notifying the vehicles about any dangerous situation in their neighborhood are called safety applications. Applications that provide value-added services are called User applications.Technologies to enhance vehicular and passenger safety are of great interest, and one of the important applications is collision。
低频活动漂浮潜水船声探测系统(LFATS)说明书

LOW-FREQUENCY ACTIVE TOWED SONAR (LFATS)LFATS is a full-feature, long-range,low-frequency variable depth sonarDeveloped for active sonar operation against modern dieselelectric submarines, LFATS has demonstrated consistent detection performance in shallow and deep water. LFATS also provides a passive mode and includes a full set of passive tools and features.COMPACT SIZELFATS is a small, lightweight, air-transportable, ruggedized system designed specifically for easy installation on small vessels. CONFIGURABLELFATS can operate in a stand-alone configuration or be easily integrated into the ship’s combat system.TACTICAL BISTATIC AND MULTISTATIC CAPABILITYA robust infrastructure permits interoperability with the HELRAS helicopter dipping sonar and all key sonobuoys.HIGHLY MANEUVERABLEOwn-ship noise reduction processing algorithms, coupled with compact twin line receivers, enable short-scope towing for efficient maneuvering, fast deployment and unencumbered operation in shallow water.COMPACT WINCH AND HANDLING SYSTEMAn ultrastable structure assures safe, reliable operation in heavy seas and permits manual or console-controlled deployment, retrieval and depth-keeping. FULL 360° COVERAGEA dual parallel array configuration and advanced signal processing achieve instantaneous, unambiguous left/right target discrimination.SPACE-SAVING TRANSMITTERTOW-BODY CONFIGURATIONInnovative technology achievesomnidirectional, large aperture acousticperformance in a compact, sleek tow-body assembly.REVERBERATION SUPRESSIONThe unique transmitter design enablesforward, aft, port and starboarddirectional transmission. This capabilitydiverts energy concentration away fromshorelines and landmasses, minimizingreverb and optimizing target detection.SONAR PERFORMANCE PREDICTIONA key ingredient to mission planning,LFATS computes and displays systemdetection capability based on modeled ormeasured environmental data.Key Features>Wide-area search>Target detection, localization andclassification>T racking and attack>Embedded trainingSonar Processing>Active processing: State-of-the-art signal processing offers acomprehensive range of single- andmulti-pulse, FM and CW processingfor detection and tracking. Targetdetection, localization andclassification>P assive processing: LFATS featuresfull 100-to-2,000 Hz continuouswideband coverage. Broadband,DEMON and narrowband analyzers,torpedo alert and extendedtracking functions constitute asuite of passive tools to track andanalyze targets.>Playback mode: Playback isseamlessly integrated intopassive and active operation,enabling postanalysis of pre-recorded mission data and is a keycomponent to operator training.>Built-in test: Power-up, continuousbackground and operator-initiatedtest modes combine to boostsystem availability and accelerateoperational readiness.UNIQUE EXTENSION/RETRACTIONMECHANISM TRANSFORMS COMPACTTOW-BODY CONFIGURATION TO ALARGE-APERTURE MULTIDIRECTIONALTRANSMITTERDISPLAYS AND OPERATOR INTERFACES>State-of-the-art workstation-based operator machineinterface: Trackball, point-and-click control, pull-down menu function and parameter selection allows easy access to key information. >Displays: A strategic balance of multifunction displays,built on a modern OpenGL framework, offer flexible search, classification and geographic formats. Ground-stabilized, high-resolution color monitors capture details in the real-time processed sonar data. > B uilt-in operator aids: To simplify operation, LFATS provides recommended mode/parameter settings, automated range-of-day estimation and data history recall. >COTS hardware: LFATS incorporates a modular, expandable open architecture to accommodate future technology.L3Harrissellsht_LFATS© 2022 L3Harris Technologies, Inc. | 09/2022NON-EXPORT CONTROLLED - These item(s)/data have been reviewed in accordance with the InternationalTraffic in Arms Regulations (ITAR), 22 CFR part 120.33, and the Export Administration Regulations (EAR), 15 CFR 734(3)(b)(3), and may be released without export restrictions.L3Harris Technologies is an agile global aerospace and defense technology innovator, delivering end-to-endsolutions that meet customers’ mission-critical needs. The company provides advanced defense and commercial technologies across air, land, sea, space and cyber domains.t 818 367 0111 | f 818 364 2491 *******************WINCH AND HANDLINGSYSTEMSHIP ELECTRONICSTOWED SUBSYSTEMSONAR OPERATORCONSOLETRANSMIT POWERAMPLIFIER 1025 W. NASA Boulevard Melbourne, FL 32919SPECIFICATIONSOperating Modes Active, passive, test, playback, multi-staticSource Level 219 dB Omnidirectional, 222 dB Sector Steered Projector Elements 16 in 4 stavesTransmission Omnidirectional or by sector Operating Depth 15-to-300 m Survival Speed 30 knotsSize Winch & Handling Subsystem:180 in. x 138 in. x 84 in.(4.5 m x 3.5 m x 2.2 m)Sonar Operator Console:60 in. x 26 in. x 68 in.(1.52 m x 0.66 m x 1.73 m)Transmit Power Amplifier:42 in. x 28 in. x 68 in.(1.07 m x 0.71 m x 1.73 m)Weight Winch & Handling: 3,954 kg (8,717 lb.)Towed Subsystem: 678 kg (1,495 lb.)Ship Electronics: 928 kg (2,045 lb.)Platforms Frigates, corvettes, small patrol boats Receive ArrayConfiguration: Twin-lineNumber of channels: 48 per lineLength: 26.5 m (86.9 ft.)Array directivity: >18 dB @ 1,380 HzLFATS PROCESSINGActiveActive Band 1,200-to-1,00 HzProcessing CW, FM, wavetrain, multi-pulse matched filtering Pulse Lengths Range-dependent, .039 to 10 sec. max.FM Bandwidth 50, 100 and 300 HzTracking 20 auto and operator-initiated Displays PPI, bearing range, Doppler range, FM A-scan, geographic overlayRange Scale5, 10, 20, 40, and 80 kyd PassivePassive Band Continuous 100-to-2,000 HzProcessing Broadband, narrowband, ALI, DEMON and tracking Displays BTR, BFI, NALI, DEMON and LOFAR Tracking 20 auto and operator-initiatedCommonOwn-ship noise reduction, doppler nullification, directional audio。
OSHA现场作业手册说明书
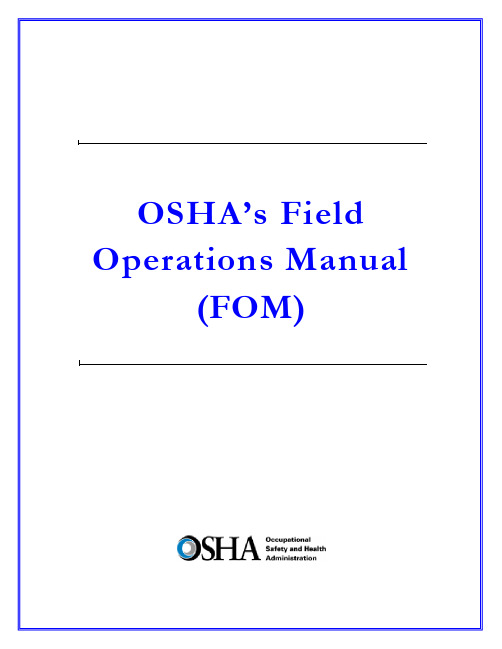
DIRECTIVE NUMBER: CPL 02-00-150 EFFECTIVE DATE: April 22, 2011 SUBJECT: Field Operations Manual (FOM)ABSTRACTPurpose: This instruction cancels and replaces OSHA Instruction CPL 02-00-148,Field Operations Manual (FOM), issued November 9, 2009, whichreplaced the September 26, 1994 Instruction that implemented the FieldInspection Reference Manual (FIRM). The FOM is a revision of OSHA’senforcement policies and procedures manual that provides the field officesa reference document for identifying the responsibilities associated withthe majority of their inspection duties. This Instruction also cancels OSHAInstruction FAP 01-00-003 Federal Agency Safety and Health Programs,May 17, 1996 and Chapter 13 of OSHA Instruction CPL 02-00-045,Revised Field Operations Manual, June 15, 1989.Scope: OSHA-wide.References: Title 29 Code of Federal Regulations §1903.6, Advance Notice ofInspections; 29 Code of Federal Regulations §1903.14, Policy RegardingEmployee Rescue Activities; 29 Code of Federal Regulations §1903.19,Abatement Verification; 29 Code of Federal Regulations §1904.39,Reporting Fatalities and Multiple Hospitalizations to OSHA; and Housingfor Agricultural Workers: Final Rule, Federal Register, March 4, 1980 (45FR 14180).Cancellations: OSHA Instruction CPL 02-00-148, Field Operations Manual, November9, 2009.OSHA Instruction FAP 01-00-003, Federal Agency Safety and HealthPrograms, May 17, 1996.Chapter 13 of OSHA Instruction CPL 02-00-045, Revised FieldOperations Manual, June 15, 1989.State Impact: Notice of Intent and Adoption required. See paragraph VI.Action Offices: National, Regional, and Area OfficesOriginating Office: Directorate of Enforcement Programs Contact: Directorate of Enforcement ProgramsOffice of General Industry Enforcement200 Constitution Avenue, NW, N3 119Washington, DC 20210202-693-1850By and Under the Authority ofDavid Michaels, PhD, MPHAssistant SecretaryExecutive SummaryThis instruction cancels and replaces OSHA Instruction CPL 02-00-148, Field Operations Manual (FOM), issued November 9, 2009. The one remaining part of the prior Field Operations Manual, the chapter on Disclosure, will be added at a later date. This Instruction also cancels OSHA Instruction FAP 01-00-003 Federal Agency Safety and Health Programs, May 17, 1996 and Chapter 13 of OSHA Instruction CPL 02-00-045, Revised Field Operations Manual, June 15, 1989. This Instruction constitutes OSHA’s general enforcement policies and procedures manual for use by the field offices in conducting inspections, issuing citations and proposing penalties.Significant Changes∙A new Table of Contents for the entire FOM is added.∙ A new References section for the entire FOM is added∙ A new Cancellations section for the entire FOM is added.∙Adds a Maritime Industry Sector to Section III of Chapter 10, Industry Sectors.∙Revises sections referring to the Enhanced Enforcement Program (EEP) replacing the information with the Severe Violator Enforcement Program (SVEP).∙Adds Chapter 13, Federal Agency Field Activities.∙Cancels OSHA Instruction FAP 01-00-003, Federal Agency Safety and Health Programs, May 17, 1996.DisclaimerThis manual is intended to provide instruction regarding some of the internal operations of the Occupational Safety and Health Administration (OSHA), and is solely for the benefit of the Government. No duties, rights, or benefits, substantive or procedural, are created or implied by this manual. The contents of this manual are not enforceable by any person or entity against the Department of Labor or the United States. Statements which reflect current Occupational Safety and Health Review Commission or court precedents do not necessarily indicate acquiescence with those precedents.Table of ContentsCHAPTER 1INTRODUCTIONI.PURPOSE. ........................................................................................................... 1-1 II.SCOPE. ................................................................................................................ 1-1 III.REFERENCES .................................................................................................... 1-1 IV.CANCELLATIONS............................................................................................. 1-8 V. ACTION INFORMATION ................................................................................. 1-8A.R ESPONSIBLE O FFICE.......................................................................................................................................... 1-8B.A CTION O FFICES. .................................................................................................................... 1-8C. I NFORMATION O FFICES............................................................................................................ 1-8 VI. STATE IMPACT. ................................................................................................ 1-8 VII.SIGNIFICANT CHANGES. ............................................................................... 1-9 VIII.BACKGROUND. ................................................................................................. 1-9 IX. DEFINITIONS AND TERMINOLOGY. ........................................................ 1-10A.T HE A CT................................................................................................................................................................. 1-10B. C OMPLIANCE S AFETY AND H EALTH O FFICER (CSHO). ...........................................................1-10B.H E/S HE AND H IS/H ERS ..................................................................................................................................... 1-10C.P ROFESSIONAL J UDGMENT............................................................................................................................... 1-10E. W ORKPLACE AND W ORKSITE ......................................................................................................................... 1-10CHAPTER 2PROGRAM PLANNINGI.INTRODUCTION ............................................................................................... 2-1 II.AREA OFFICE RESPONSIBILITIES. .............................................................. 2-1A.P ROVIDING A SSISTANCE TO S MALL E MPLOYERS. ...................................................................................... 2-1B.A REA O FFICE O UTREACH P ROGRAM. ............................................................................................................. 2-1C. R ESPONDING TO R EQUESTS FOR A SSISTANCE. ............................................................................................ 2-2 III. OSHA COOPERATIVE PROGRAMS OVERVIEW. ...................................... 2-2A.V OLUNTARY P ROTECTION P ROGRAM (VPP). ........................................................................... 2-2B.O NSITE C ONSULTATION P ROGRAM. ................................................................................................................ 2-2C.S TRATEGIC P ARTNERSHIPS................................................................................................................................. 2-3D.A LLIANCE P ROGRAM ........................................................................................................................................... 2-3 IV. ENFORCEMENT PROGRAM SCHEDULING. ................................................ 2-4A.G ENERAL ................................................................................................................................................................. 2-4B.I NSPECTION P RIORITY C RITERIA. ..................................................................................................................... 2-4C.E FFECT OF C ONTEST ............................................................................................................................................ 2-5D.E NFORCEMENT E XEMPTIONS AND L IMITATIONS. ....................................................................................... 2-6E.P REEMPTION BY A NOTHER F EDERAL A GENCY ........................................................................................... 2-6F.U NITED S TATES P OSTAL S ERVICE. .................................................................................................................. 2-7G.H OME-B ASED W ORKSITES. ................................................................................................................................ 2-8H.I NSPECTION/I NVESTIGATION T YPES. ............................................................................................................... 2-8 V.UNPROGRAMMED ACTIVITY – HAZARD EVALUATION AND INSPECTION SCHEDULING ............................................................................ 2-9 VI.PROGRAMMED INSPECTIONS. ................................................................... 2-10A.S ITE-S PECIFIC T ARGETING (SST) P ROGRAM. ............................................................................................. 2-10B.S CHEDULING FOR C ONSTRUCTION I NSPECTIONS. ..................................................................................... 2-10C.S CHEDULING FOR M ARITIME I NSPECTIONS. ............................................................................. 2-11D.S PECIAL E MPHASIS P ROGRAMS (SEP S). ................................................................................... 2-12E.N ATIONAL E MPHASIS P ROGRAMS (NEP S) ............................................................................... 2-13F.L OCAL E MPHASIS P ROGRAMS (LEP S) AND R EGIONAL E MPHASIS P ROGRAMS (REP S) ............ 2-13G.O THER S PECIAL P ROGRAMS. ............................................................................................................................ 2-13H.I NSPECTION S CHEDULING AND I NTERFACE WITH C OOPERATIVE P ROGRAM P ARTICIPANTS ....... 2-13CHAPTER 3INSPECTION PROCEDURESI.INSPECTION PREPARATION. .......................................................................... 3-1 II.INSPECTION PLANNING. .................................................................................. 3-1A.R EVIEW OF I NSPECTION H ISTORY .................................................................................................................... 3-1B.R EVIEW OF C OOPERATIVE P ROGRAM P ARTICIPATION .............................................................................. 3-1C.OSHA D ATA I NITIATIVE (ODI) D ATA R EVIEW .......................................................................................... 3-2D.S AFETY AND H EALTH I SSUES R ELATING TO CSHO S.................................................................. 3-2E.A DVANCE N OTICE. ................................................................................................................................................ 3-3F.P RE-I NSPECTION C OMPULSORY P ROCESS ...................................................................................................... 3-5G.P ERSONAL S ECURITY C LEARANCE. ................................................................................................................. 3-5H.E XPERT A SSISTANCE. ........................................................................................................................................... 3-5 III. INSPECTION SCOPE. ......................................................................................... 3-6A.C OMPREHENSIVE ................................................................................................................................................... 3-6B.P ARTIAL. ................................................................................................................................................................... 3-6 IV. CONDUCT OF INSPECTION .............................................................................. 3-6A.T IME OF I NSPECTION............................................................................................................................................. 3-6B.P RESENTING C REDENTIALS. ............................................................................................................................... 3-6C.R EFUSAL TO P ERMIT I NSPECTION AND I NTERFERENCE ............................................................................. 3-7D.E MPLOYEE P ARTICIPATION. ............................................................................................................................... 3-9E.R ELEASE FOR E NTRY ............................................................................................................................................ 3-9F.B ANKRUPT OR O UT OF B USINESS. .................................................................................................................... 3-9G.E MPLOYEE R ESPONSIBILITIES. ................................................................................................. 3-10H.S TRIKE OR L ABOR D ISPUTE ............................................................................................................................. 3-10I. V ARIANCES. .......................................................................................................................................................... 3-11 V. OPENING CONFERENCE. ................................................................................ 3-11A.G ENERAL ................................................................................................................................................................ 3-11B.R EVIEW OF A PPROPRIATION A CT E XEMPTIONS AND L IMITATION. ..................................................... 3-13C.R EVIEW S CREENING FOR P ROCESS S AFETY M ANAGEMENT (PSM) C OVERAGE............................. 3-13D.R EVIEW OF V OLUNTARY C OMPLIANCE P ROGRAMS. ................................................................................ 3-14E.D ISRUPTIVE C ONDUCT. ...................................................................................................................................... 3-15F.C LASSIFIED A REAS ............................................................................................................................................. 3-16VI. REVIEW OF RECORDS. ................................................................................... 3-16A.I NJURY AND I LLNESS R ECORDS...................................................................................................................... 3-16B.R ECORDING C RITERIA. ...................................................................................................................................... 3-18C. R ECORDKEEPING D EFICIENCIES. .................................................................................................................. 3-18 VII. WALKAROUND INSPECTION. ....................................................................... 3-19A.W ALKAROUND R EPRESENTATIVES ............................................................................................................... 3-19B.E VALUATION OF S AFETY AND H EALTH M ANAGEMENT S YSTEM. ....................................................... 3-20C.R ECORD A LL F ACTS P ERTINENT TO A V IOLATION. ................................................................................. 3-20D.T ESTIFYING IN H EARINGS ................................................................................................................................ 3-21E.T RADE S ECRETS. ................................................................................................................................................. 3-21F.C OLLECTING S AMPLES. ..................................................................................................................................... 3-22G.P HOTOGRAPHS AND V IDEOTAPES.................................................................................................................. 3-22H.V IOLATIONS OF O THER L AWS. ....................................................................................................................... 3-23I.I NTERVIEWS OF N ON-M ANAGERIAL E MPLOYEES .................................................................................... 3-23J.M ULTI-E MPLOYER W ORKSITES ..................................................................................................................... 3-27 K.A DMINISTRATIVE S UBPOENA.......................................................................................................................... 3-27 L.E MPLOYER A BATEMENT A SSISTANCE. ........................................................................................................ 3-27 VIII. CLOSING CONFERENCE. .............................................................................. 3-28A.P ARTICIPANTS. ..................................................................................................................................................... 3-28B.D ISCUSSION I TEMS. ............................................................................................................................................ 3-28C.A DVICE TO A TTENDEES .................................................................................................................................... 3-29D.P ENALTIES............................................................................................................................................................. 3-30E.F EASIBLE A DMINISTRATIVE, W ORK P RACTICE AND E NGINEERING C ONTROLS. ............................ 3-30F.R EDUCING E MPLOYEE E XPOSURE. ................................................................................................................ 3-32G.A BATEMENT V ERIFICATION. ........................................................................................................................... 3-32H.E MPLOYEE D ISCRIMINATION .......................................................................................................................... 3-33 IX. SPECIAL INSPECTION PROCEDURES. ...................................................... 3-33A.F OLLOW-UP AND M ONITORING I NSPECTIONS............................................................................................ 3-33B.C ONSTRUCTION I NSPECTIONS ......................................................................................................................... 3-34C. F EDERAL A GENCY I NSPECTIONS. ................................................................................................................. 3-35CHAPTER 4VIOLATIONSI. BASIS OF VIOLATIONS ..................................................................................... 4-1A.S TANDARDS AND R EGULATIONS. .................................................................................................................... 4-1B.E MPLOYEE E XPOSURE. ........................................................................................................................................ 4-3C.R EGULATORY R EQUIREMENTS. ........................................................................................................................ 4-6D.H AZARD C OMMUNICATION. .............................................................................................................................. 4-6E. E MPLOYER/E MPLOYEE R ESPONSIBILITIES ................................................................................................... 4-6 II. SERIOUS VIOLATIONS. .................................................................................... 4-8A.S ECTION 17(K). ......................................................................................................................... 4-8B.E STABLISHING S ERIOUS V IOLATIONS ............................................................................................................ 4-8C. F OUR S TEPS TO BE D OCUMENTED. ................................................................................................................... 4-8 III. GENERAL DUTY REQUIREMENTS ............................................................. 4-14A.E VALUATION OF G ENERAL D UTY R EQUIREMENTS ................................................................................. 4-14B.E LEMENTS OF A G ENERAL D UTY R EQUIREMENT V IOLATION.............................................................. 4-14C. U SE OF THE G ENERAL D UTY C LAUSE ........................................................................................................ 4-23D.L IMITATIONS OF U SE OF THE G ENERAL D UTY C LAUSE. ..............................................................E.C LASSIFICATION OF V IOLATIONS C ITED U NDER THE G ENERAL D UTY C LAUSE. ..................F. P ROCEDURES FOR I MPLEMENTATION OF S ECTION 5(A)(1) E NFORCEMENT ............................ 4-25 4-27 4-27IV.OTHER-THAN-SERIOUS VIOLATIONS ............................................... 4-28 V.WILLFUL VIOLATIONS. ......................................................................... 4-28A.I NTENTIONAL D ISREGARD V IOLATIONS. ..........................................................................................4-28B.P LAIN I NDIFFERENCE V IOLATIONS. ...................................................................................................4-29 VI. CRIMINAL/WILLFUL VIOLATIONS. ................................................... 4-30A.A REA D IRECTOR C OORDINATION ....................................................................................................... 4-31B.C RITERIA FOR I NVESTIGATING P OSSIBLE C RIMINAL/W ILLFUL V IOLATIONS ........................ 4-31C. W ILLFUL V IOLATIONS R ELATED TO A F ATALITY .......................................................................... 4-32 VII. REPEATED VIOLATIONS. ...................................................................... 4-32A.F EDERAL AND S TATE P LAN V IOLATIONS. ........................................................................................4-32B.I DENTICAL S TANDARDS. .......................................................................................................................4-32C.D IFFERENT S TANDARDS. .......................................................................................................................4-33D.O BTAINING I NSPECTION H ISTORY. .....................................................................................................4-33E.T IME L IMITATIONS..................................................................................................................................4-34F.R EPEATED V. F AILURE TO A BATE....................................................................................................... 4-34G. A REA D IRECTOR R ESPONSIBILITIES. .............................................................................. 4-35 VIII. DE MINIMIS CONDITIONS. ................................................................... 4-36A.C RITERIA ................................................................................................................................................... 4-36B.P ROFESSIONAL J UDGMENT. ..................................................................................................................4-37C. A REA D IRECTOR R ESPONSIBILITIES. .............................................................................. 4-37 IX. CITING IN THE ALTERNATIVE ............................................................ 4-37 X. COMBINING AND GROUPING VIOLATIONS. ................................... 4-37A.C OMBINING. ..............................................................................................................................................4-37B.G ROUPING. ................................................................................................................................................4-38C. W HEN N OT TO G ROUP OR C OMBINE. ................................................................................................4-38 XI. HEALTH STANDARD VIOLATIONS ....................................................... 4-39A.C ITATION OF V ENTILATION S TANDARDS ......................................................................................... 4-39B.V IOLATIONS OF THE N OISE S TANDARD. ...........................................................................................4-40 XII. VIOLATIONS OF THE RESPIRATORY PROTECTION STANDARD(§1910.134). ....................................................................................................... XIII. VIOLATIONS OF AIR CONTAMINANT STANDARDS (§1910.1000) ... 4-43 4-43A.R EQUIREMENTS UNDER THE STANDARD: .................................................................................................. 4-43B.C LASSIFICATION OF V IOLATIONS OF A IR C ONTAMINANT S TANDARDS. ......................................... 4-43 XIV. CITING IMPROPER PERSONAL HYGIENE PRACTICES. ................... 4-45A.I NGESTION H AZARDS. .................................................................................................................................... 4-45B.A BSORPTION H AZARDS. ................................................................................................................................ 4-46C.W IPE S AMPLING. ............................................................................................................................................. 4-46D.C ITATION P OLICY ............................................................................................................................................ 4-46 XV. BIOLOGICAL MONITORING. ...................................................................... 4-47CHAPTER 5CASE FILE PREPARATION AND DOCUMENTATIONI.INTRODUCTION ............................................................................................... 5-1 II.INSPECTION CONDUCTED, CITATIONS BEING ISSUED. .................... 5-1A.OSHA-1 ................................................................................................................................... 5-1B.OSHA-1A. ............................................................................................................................... 5-1C. OSHA-1B. ................................................................................................................................ 5-2 III.INSPECTION CONDUCTED BUT NO CITATIONS ISSUED .................... 5-5 IV.NO INSPECTION ............................................................................................... 5-5 V. HEALTH INSPECTIONS. ................................................................................. 5-6A.D OCUMENT P OTENTIAL E XPOSURE. ............................................................................................................... 5-6B.E MPLOYER’S O CCUPATIONAL S AFETY AND H EALTH S YSTEM. ............................................................. 5-6 VI. AFFIRMATIVE DEFENSES............................................................................. 5-8A.B URDEN OF P ROOF. .............................................................................................................................................. 5-8B.E XPLANATIONS. ..................................................................................................................................................... 5-8 VII. INTERVIEW STATEMENTS. ........................................................................ 5-10A.G ENERALLY. ......................................................................................................................................................... 5-10B.CSHO S SHALL OBTAIN WRITTEN STATEMENTS WHEN: .......................................................................... 5-10C.L ANGUAGE AND W ORDING OF S TATEMENT. ............................................................................................. 5-11D.R EFUSAL TO S IGN S TATEMENT ...................................................................................................................... 5-11E.V IDEO AND A UDIOTAPED S TATEMENTS. ..................................................................................................... 5-11F.A DMINISTRATIVE D EPOSITIONS. .............................................................................................5-11 VIII. PAPERWORK AND WRITTEN PROGRAM REQUIREMENTS. .......... 5-12 IX.GUIDELINES FOR CASE FILE DOCUMENTATION FOR USE WITH VIDEOTAPES AND AUDIOTAPES .............................................................. 5-12 X.CASE FILE ACTIVITY DIARY SHEET. ..................................................... 5-12 XI. CITATIONS. ..................................................................................................... 5-12A.S TATUTE OF L IMITATIONS. .............................................................................................................................. 5-13B.I SSUING C ITATIONS. ........................................................................................................................................... 5-13C.A MENDING/W ITHDRAWING C ITATIONS AND N OTIFICATION OF P ENALTIES. .................................. 5-13D.P ROCEDURES FOR A MENDING OR W ITHDRAWING C ITATIONS ............................................................ 5-14 XII. INSPECTION RECORDS. ............................................................................... 5-15A.G ENERALLY. ......................................................................................................................................................... 5-15B.R ELEASE OF I NSPECTION I NFORMATION ..................................................................................................... 5-15C. C LASSIFIED AND T RADE S ECRET I NFORMATION ...................................................................................... 5-16。
里程计和全方位自动导引车的外部传感器(AGV的)同时校准
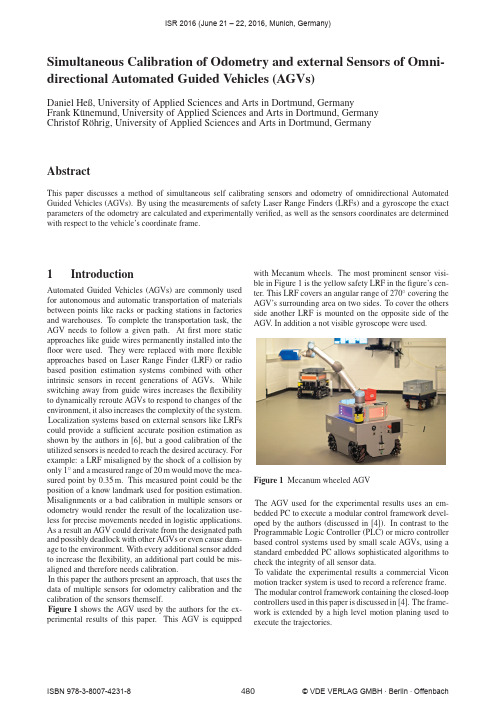
with Mecanum wheels. The most prominent sensor visible in Figure 1 is the yellow safety LRF in the figure’s center. This LRF covers an angular range of 270◦ covering the AGV’s surrounding area on two sides. To cover the others side another LRF is mounted on the opposite side of the AGV. In addition a not visible gyroscope were used.
2.1
Calibration of multiple Laser Range Finder (LRF)
Calibration of multiple LRF or Light Detection and Ranging (LIDAR) sensors was introduced in [2]. This paper discusses the on-line calibration of two LIDAR sensors by using natural features in an outdoor scenario. Through utilizing the described process the sensor data of both sensors is kept aligned. The vehicle used in this paper is a conventional automobile with an Ackermann steering instead of an omnidirectional AGV. Furthermore both scanners are setup in a manner, that create vertical scan lines while the setup discussed in this paper uses scanners creating horizontal scan lines.
EPOCH-II高流量输出单元I说明书
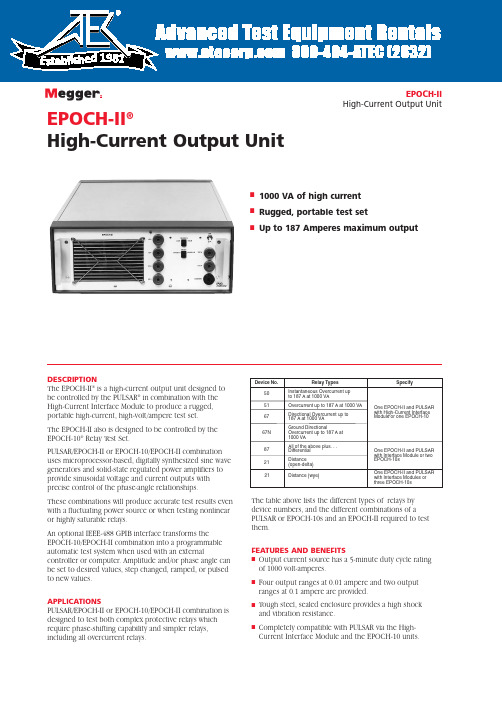
EPOCH-II ®High-Current Output UnitI 1000 VA of high current I Rugged, portable test setIUp to 187 Amperes maximum outputEPOCH-IIHigh-Current Output UnitDESCRIPTIONThe EPOCH-II ®is a high-current output unit designed to be controlled by the PULSAR ®in combination with the High-Current Interface Module to produce a rugged,portable high-current, high-volt/ampere test set. The EPOCH-II also is designed to be controlled by the EPOCH-10®Relay T est Set.PULSAR/EPOCH-II or EPOCH-10/EPOCH-II combination uses microprocessor-based, digitally synthesized sine wave generators and solid-state regulated power amplifiers to provide sinusoidal voltage and current outputs with precise control of the phase-angle relationships.These combinations will produce accurate test results even with a fluctuating power source or when testing nonlinear or highly saturable relays.An optional IEEE-488 GPIB interface transforms the EPOCH-10/EPOCH-II combination into a programmable automatic test system when used with an externalcontroller or computer. Amplitude and/or phase angle can be set to desired values, step changed, ramped, or pulsed to new values.APPLICATIONSPULSAR/EPOCH-II or EPOCH-10/EPOCH-II combination is designed to test both complex protective relays which require phase-shifting capability and simpler relays,including all overcurrent relays.The table above lists the different types of relays by device numbers, and the different combinations of a PULSAR or EPOCH-10s and an EPOCH-II required to test them.FEATURES AND BENEFITSI Output current source has a 5-minute duty cycle rating of 1000 volt-amperes.IFour output ranges at 0.01 ampere and two output ranges at 0.1 ampere are provided.IT ough steel, sealed enclosure provides a high shock and vibration resistance.ICompletely compatible with PULSAR via the High-Current Interface Module and the EPOCH-10 units.Device No.Relay TypesSpecifyOne EPOCH-II and PULSAR with High-Current Interface Module or one EPOCH-10One EPOCH-II and PULSAR with Interface Module or two EPOCH-10sInstantaneous Overcurrent up to 187 A at 1000 VADirectional Overcurrent up to 187 A at 1000 VA All of the above plus. . .Differential Distance (open-delta)50678721One EPOCH-II and PULSAR with Interface Modules or three EPOCH-10sDistance (wye)21Ground DirectionalOvercurrent up to 187 A at 1000 VA67NOvercurrent up to 187 A at 1000 VA 51 1981EPOCH-II High-Current Output UnitSPECIFICATIONSInputInput Voltage (specify one)115 V ±10%, 50/60 Hz, 30 A (at full rated output)OR230 V ±10%, 50/60 Hz, 20 AOutputOutput CurrentT o provide a variety of test circuit impedances, six output taps with two ranges are provided.High Range2.00 to 10.00 A at 100 V max3.00 to 15.00 A at 66.6 V max8.00 to 40.00 A at 25 V max10.00 to 50.00 A at 20 V max20.00 to 100.0 A at 10 V max34.00 to 170.0 A at 5.9 V maxOutput Power:1000 VALow Range2.00 to 10.00 A at 50 V max3.00 to 1 5.00 A at 33.3 V max8.00 to 40.00 A at 12.5 V max10.00 to 50.00 A at 10 V max20.00 to 100.0 A at 5 V max34.00 to 170.0 A at 2.95 V maxOutput Power:500 VAAccuracyT ypical:±0.5% of settingMaximum:±1.0% of settingAlarm will indicate when amplitude, phase angle, or waveform is in error.ResolutionFour ranges:0.01 AT wo ranges:0.l ADuty CycleFive minutes at full rated VA output. Fifteen minutes recovery time.Overrange CapabilityThe EPOCH-II has an overrange capability of +10% for each tap with a maximum output current of 187 A on the 170 A tap. DistortionLess than 1% typical, 3% max.Output of EPOCH-10/EPOCH-II CombinationFrequencyI Synchronized to input power sourceI60 Hz crystal-controlledI50 Hz crystal-controlled AccuracyI Synchronized, tracks input frequencyI±0.006 Hz for 60-Hz crystal control (±001%)I±0.005 Hz for 50-Hz crystal control (±0.01%)Output of PULSAR and Interface Module Connected to EPOCH-II The High-Current Interface Module provides a variable frequency signal to the Epoch-II High-Current Output Unit.Output frequency is continuously displayed for each channel with large, high-intensity LEDs with the following ranges:5.000 to 99.999 Hz100.01 to 999.99 HzFrequency Accuracy:±10 ppm at 23°C, ±2°C)Current Phase Angle ControlAngle is adjusted on the EPOCH-10 control unit by 4-digit, pushbutton control with large LED display of setting.Range:0.0 to 359.9°Resolution:0.1°Accuracy:less than ±0.3°typical, ±1.0°maxControl SectionThe control unit for the EPOCH-II high-current section is PULSAR (with the High-Current Interface Module), an EPOCH-I or EPOCH-10. Thus, the excellent operating and control features of PULSAR and the EPOCH-10 are used to control the EPOCH-II.Note:When the EPOCH-II is in use, the current output of the EPOCH-10 control unit is inoperative. All EPOCH-10s can control an EPOCH-II high-current section. When the high-current section of the EPOCH-II is not needed, the EPOCH-10 can be used independently or slaved together with other EPOCH units. ProtectionThe input line circuit is breaker-protected. The dc power supply is overcurrent-protected. In addition, overvoltage protection is provided on the input line circuit.The power amplifiers are forced-air cooled and are protected by thermal-overload sensors.Audio and visual alarms on the PULSAR and EPOCH-10 control units indicate whenever the current or potential outputs are overloaded.TemperatureOperating32 to 122°F (0 to 50°C)Reduced duty cycle above 113°F (45°C)Storage–13 to +158°F (–25°to +70°C)Dimensions7.6 H x 19.75 W x 21.6 D in.(193 H x 502 W x 549 D mm)Weight115 lb (52.3 kg)EPOCH-II with EPOCH-10 control unitEPOCH-II High-Current Output UnitUKArchcliffe Road Dover CT17 9EN EnglandT +44 (0) 1304 502101 F +44 (0) 1304 207342UNITED STATES4271 Bronze Way DallasTX75237-1017 USAT 800 723 2861 (USA only)T +1 214 330 3203F +1 214 337 3038OTHER TECHNICAL SALES OFFICESValley Forge USA, Toronto CANADA,Mumbai INDIA, Trappes FRANCE,Sydney AUSTRALIA, Madrid SPAINand the Kingdom of BAHRAIN.Registered to ISO 9001:2000 Reg no. Q 09290Registered to ISO 14001 Reg no. EMS 61597EPOCH_II_DS_en_V10The word ‘Megger’ is a registeredtrademark。
Survey of clustering data mining techniques
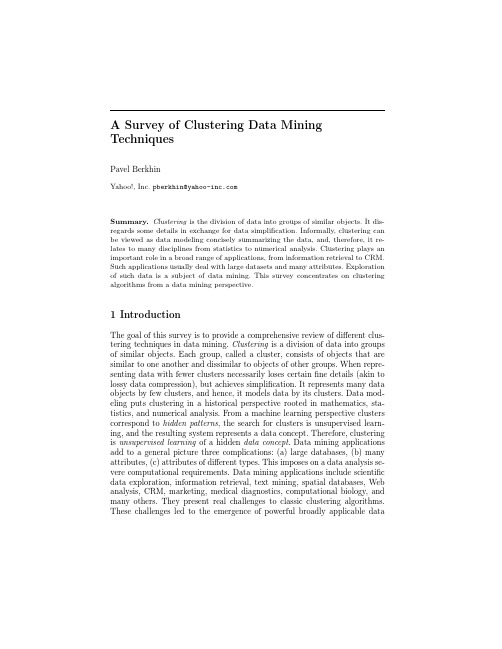
A Survey of Clustering Data Mining TechniquesPavel BerkhinYahoo!,Inc.pberkhin@Summary.Clustering is the division of data into groups of similar objects.It dis-regards some details in exchange for data simplifirmally,clustering can be viewed as data modeling concisely summarizing the data,and,therefore,it re-lates to many disciplines from statistics to numerical analysis.Clustering plays an important role in a broad range of applications,from information retrieval to CRM. Such applications usually deal with large datasets and many attributes.Exploration of such data is a subject of data mining.This survey concentrates on clustering algorithms from a data mining perspective.1IntroductionThe goal of this survey is to provide a comprehensive review of different clus-tering techniques in data mining.Clustering is a division of data into groups of similar objects.Each group,called a cluster,consists of objects that are similar to one another and dissimilar to objects of other groups.When repre-senting data with fewer clusters necessarily loses certainfine details(akin to lossy data compression),but achieves simplification.It represents many data objects by few clusters,and hence,it models data by its clusters.Data mod-eling puts clustering in a historical perspective rooted in mathematics,sta-tistics,and numerical analysis.From a machine learning perspective clusters correspond to hidden patterns,the search for clusters is unsupervised learn-ing,and the resulting system represents a data concept.Therefore,clustering is unsupervised learning of a hidden data concept.Data mining applications add to a general picture three complications:(a)large databases,(b)many attributes,(c)attributes of different types.This imposes on a data analysis se-vere computational requirements.Data mining applications include scientific data exploration,information retrieval,text mining,spatial databases,Web analysis,CRM,marketing,medical diagnostics,computational biology,and many others.They present real challenges to classic clustering algorithms. These challenges led to the emergence of powerful broadly applicable data2Pavel Berkhinmining clustering methods developed on the foundation of classic techniques.They are subject of this survey.1.1NotationsTo fix the context and clarify terminology,consider a dataset X consisting of data points (i.e.,objects ,instances ,cases ,patterns ,tuples ,transactions )x i =(x i 1,···,x id ),i =1:N ,in attribute space A ,where each component x il ∈A l ,l =1:d ,is a numerical or nominal categorical attribute (i.e.,feature ,variable ,dimension ,component ,field ).For a discussion of attribute data types see [106].Such point-by-attribute data format conceptually corresponds to a N ×d matrix and is used by a majority of algorithms reviewed below.However,data of other formats,such as variable length sequences and heterogeneous data,are not uncommon.The simplest subset in an attribute space is a direct Cartesian product of sub-ranges C = C l ⊂A ,C l ⊂A l ,called a segment (i.e.,cube ,cell ,region ).A unit is an elementary segment whose sub-ranges consist of a single category value,or of a small numerical bin.Describing the numbers of data points per every unit represents an extreme case of clustering,a histogram .This is a very expensive representation,and not a very revealing er driven segmentation is another commonly used practice in data exploration that utilizes expert knowledge regarding the importance of certain sub-domains.Unlike segmentation,clustering is assumed to be automatic,and so it is a machine learning technique.The ultimate goal of clustering is to assign points to a finite system of k subsets (clusters).Usually (but not always)subsets do not intersect,and their union is equal to a full dataset with the possible exception of outliersX =C 1 ··· C k C outliers ,C i C j =0,i =j.1.2Clustering Bibliography at GlanceGeneral references regarding clustering include [110],[205],[116],[131],[63],[72],[165],[119],[75],[141],[107],[91].A very good introduction to contem-porary data mining clustering techniques can be found in the textbook [106].There is a close relationship between clustering and many other fields.Clustering has always been used in statistics [10]and science [158].The clas-sic introduction into pattern recognition framework is given in [64].Typical applications include speech and character recognition.Machine learning clus-tering algorithms were applied to image segmentation and computer vision[117].For statistical approaches to pattern recognition see [56]and [85].Clus-tering can be viewed as a density estimation problem.This is the subject of traditional multivariate statistical estimation [197].Clustering is also widelyA Survey of Clustering Data Mining Techniques3 used for data compression in image processing,which is also known as vec-tor quantization[89].Datafitting in numerical analysis provides still another venue in data modeling[53].This survey’s emphasis is on clustering in data mining.Such clustering is characterized by large datasets with many attributes of different types. Though we do not even try to review particular applications,many important ideas are related to the specificfields.Clustering in data mining was brought to life by intense developments in information retrieval and text mining[52], [206],[58],spatial database applications,for example,GIS or astronomical data,[223],[189],[68],sequence and heterogeneous data analysis[43],Web applications[48],[111],[81],DNA analysis in computational biology[23],and many others.They resulted in a large amount of application-specific devel-opments,but also in some general techniques.These techniques and classic clustering algorithms that relate to them are surveyed below.1.3Plan of Further PresentationClassification of clustering algorithms is neither straightforward,nor canoni-cal.In reality,different classes of algorithms overlap.Traditionally clustering techniques are broadly divided in hierarchical and partitioning.Hierarchical clustering is further subdivided into agglomerative and divisive.The basics of hierarchical clustering include Lance-Williams formula,idea of conceptual clustering,now classic algorithms SLINK,COBWEB,as well as newer algo-rithms CURE and CHAMELEON.We survey these algorithms in the section Hierarchical Clustering.While hierarchical algorithms gradually(dis)assemble points into clusters (as crystals grow),partitioning algorithms learn clusters directly.In doing so they try to discover clusters either by iteratively relocating points between subsets,or by identifying areas heavily populated with data.Algorithms of thefirst kind are called Partitioning Relocation Clustering. They are further classified into probabilistic clustering(EM framework,al-gorithms SNOB,AUTOCLASS,MCLUST),k-medoids methods(algorithms PAM,CLARA,CLARANS,and its extension),and k-means methods(differ-ent schemes,initialization,optimization,harmonic means,extensions).Such methods concentrate on how well pointsfit into their clusters and tend to build clusters of proper convex shapes.Partitioning algorithms of the second type are surveyed in the section Density-Based Partitioning.They attempt to discover dense connected com-ponents of data,which areflexible in terms of their shape.Density-based connectivity is used in the algorithms DBSCAN,OPTICS,DBCLASD,while the algorithm DENCLUE exploits space density functions.These algorithms are less sensitive to outliers and can discover clusters of irregular shape.They usually work with low-dimensional numerical data,known as spatial data. Spatial objects could include not only points,but also geometrically extended objects(algorithm GDBSCAN).4Pavel BerkhinSome algorithms work with data indirectly by constructing summaries of data over the attribute space subsets.They perform space segmentation and then aggregate appropriate segments.We discuss them in the section Grid-Based Methods.They frequently use hierarchical agglomeration as one phase of processing.Algorithms BANG,STING,WaveCluster,and FC are discussed in this section.Grid-based methods are fast and handle outliers well.Grid-based methodology is also used as an intermediate step in many other algorithms (for example,CLIQUE,MAFIA).Categorical data is intimately connected with transactional databases.The concept of a similarity alone is not sufficient for clustering such data.The idea of categorical data co-occurrence comes to the rescue.The algorithms ROCK,SNN,and CACTUS are surveyed in the section Co-Occurrence of Categorical Data.The situation gets even more aggravated with the growth of the number of items involved.To help with this problem the effort is shifted from data clustering to pre-clustering of items or categorical attribute values. Development based on hyper-graph partitioning and the algorithm STIRR exemplify this approach.Many other clustering techniques are developed,primarily in machine learning,that either have theoretical significance,are used traditionally out-side the data mining community,or do notfit in previously outlined categories. The boundary is blurred.In the section Other Developments we discuss the emerging direction of constraint-based clustering,the important researchfield of graph partitioning,and the relationship of clustering to supervised learning, gradient descent,artificial neural networks,and evolutionary methods.Data Mining primarily works with large databases.Clustering large datasets presents scalability problems reviewed in the section Scalability and VLDB Extensions.Here we talk about algorithms like DIGNET,about BIRCH and other data squashing techniques,and about Hoffding or Chernoffbounds.Another trait of real-life data is high dimensionality.Corresponding de-velopments are surveyed in the section Clustering High Dimensional Data. The trouble comes from a decrease in metric separation when the dimension grows.One approach to dimensionality reduction uses attributes transforma-tions(DFT,PCA,wavelets).Another way to address the problem is through subspace clustering(algorithms CLIQUE,MAFIA,ENCLUS,OPTIGRID, PROCLUS,ORCLUS).Still another approach clusters attributes in groups and uses their derived proxies to cluster objects.This double clustering is known as co-clustering.Issues common to different clustering methods are overviewed in the sec-tion General Algorithmic Issues.We talk about assessment of results,de-termination of appropriate number of clusters to build,data preprocessing, proximity measures,and handling of outliers.For reader’s convenience we provide a classification of clustering algorithms closely followed by this survey:•Hierarchical MethodsA Survey of Clustering Data Mining Techniques5Agglomerative AlgorithmsDivisive Algorithms•Partitioning Relocation MethodsProbabilistic ClusteringK-medoids MethodsK-means Methods•Density-Based Partitioning MethodsDensity-Based Connectivity ClusteringDensity Functions Clustering•Grid-Based Methods•Methods Based on Co-Occurrence of Categorical Data•Other Clustering TechniquesConstraint-Based ClusteringGraph PartitioningClustering Algorithms and Supervised LearningClustering Algorithms in Machine Learning•Scalable Clustering Algorithms•Algorithms For High Dimensional DataSubspace ClusteringCo-Clustering Techniques1.4Important IssuesThe properties of clustering algorithms we are primarily concerned with in data mining include:•Type of attributes algorithm can handle•Scalability to large datasets•Ability to work with high dimensional data•Ability tofind clusters of irregular shape•Handling outliers•Time complexity(we frequently simply use the term complexity)•Data order dependency•Labeling or assignment(hard or strict vs.soft or fuzzy)•Reliance on a priori knowledge and user defined parameters •Interpretability of resultsRealistically,with every algorithm we discuss only some of these properties. The list is in no way exhaustive.For example,as appropriate,we also discuss algorithms ability to work in pre-defined memory buffer,to restart,and to provide an intermediate solution.6Pavel Berkhin2Hierarchical ClusteringHierarchical clustering builds a cluster hierarchy or a tree of clusters,also known as a dendrogram.Every cluster node contains child clusters;sibling clusters partition the points covered by their common parent.Such an ap-proach allows exploring data on different levels of granularity.Hierarchical clustering methods are categorized into agglomerative(bottom-up)and divi-sive(top-down)[116],[131].An agglomerative clustering starts with one-point (singleton)clusters and recursively merges two or more of the most similar clusters.A divisive clustering starts with a single cluster containing all data points and recursively splits the most appropriate cluster.The process contin-ues until a stopping criterion(frequently,the requested number k of clusters) is achieved.Advantages of hierarchical clustering include:•Flexibility regarding the level of granularity•Ease of handling any form of similarity or distance•Applicability to any attribute typesDisadvantages of hierarchical clustering are related to:•Vagueness of termination criteria•Most hierarchical algorithms do not revisit(intermediate)clusters once constructed.The classic approaches to hierarchical clustering are presented in the sub-section Linkage Metrics.Hierarchical clustering based on linkage metrics re-sults in clusters of proper(convex)shapes.Active contemporary efforts to build cluster systems that incorporate our intuitive concept of clusters as con-nected components of arbitrary shape,including the algorithms CURE and CHAMELEON,are surveyed in the subsection Hierarchical Clusters of Arbi-trary Shapes.Divisive techniques based on binary taxonomies are presented in the subsection Binary Divisive Partitioning.The subsection Other Devel-opments contains information related to incremental learning,model-based clustering,and cluster refinement.In hierarchical clustering our regular point-by-attribute data representa-tion frequently is of secondary importance.Instead,hierarchical clustering frequently deals with the N×N matrix of distances(dissimilarities)or sim-ilarities between training points sometimes called a connectivity matrix.So-called linkage metrics are constructed from elements of this matrix.The re-quirement of keeping a connectivity matrix in memory is unrealistic.To relax this limitation different techniques are used to sparsify(introduce zeros into) the connectivity matrix.This can be done by omitting entries smaller than a certain threshold,by using only a certain subset of data representatives,or by keeping with each point only a certain number of its nearest neighbors(for nearest neighbor chains see[177]).Notice that the way we process the original (dis)similarity matrix and construct a linkage metric reflects our a priori ideas about the data model.A Survey of Clustering Data Mining Techniques7With the(sparsified)connectivity matrix we can associate the weighted connectivity graph G(X,E)whose vertices X are data points,and edges E and their weights are defined by the connectivity matrix.This establishes a connection between hierarchical clustering and graph partitioning.One of the most striking developments in hierarchical clustering is the algorithm BIRCH.It is discussed in the section Scalable VLDB Extensions.Hierarchical clustering initializes a cluster system as a set of singleton clusters(agglomerative case)or a single cluster of all points(divisive case) and proceeds iteratively merging or splitting the most appropriate cluster(s) until the stopping criterion is achieved.The appropriateness of a cluster(s) for merging or splitting depends on the(dis)similarity of cluster(s)elements. This reflects a general presumption that clusters consist of similar points.An important example of dissimilarity between two points is the distance between them.To merge or split subsets of points rather than individual points,the dis-tance between individual points has to be generalized to the distance between subsets.Such a derived proximity measure is called a linkage metric.The type of a linkage metric significantly affects hierarchical algorithms,because it re-flects a particular concept of closeness and connectivity.Major inter-cluster linkage metrics[171],[177]include single link,average link,and complete link. The underlying dissimilarity measure(usually,distance)is computed for every pair of nodes with one node in thefirst set and another node in the second set.A specific operation such as minimum(single link),average(average link),or maximum(complete link)is applied to pair-wise dissimilarity measures:d(C1,C2)=Op{d(x,y),x∈C1,y∈C2}Early examples include the algorithm SLINK[199],which implements single link(Op=min),Voorhees’method[215],which implements average link (Op=Avr),and the algorithm CLINK[55],which implements complete link (Op=max).It is related to the problem offinding the Euclidean minimal spanning tree[224]and has O(N2)complexity.The methods using inter-cluster distances defined in terms of pairs of nodes(one in each respective cluster)are called graph methods.They do not use any cluster representation other than a set of points.This name naturally relates to the connectivity graph G(X,E)introduced above,because every data partition corresponds to a graph partition.Such methods can be augmented by so-called geometric methods in which a cluster is represented by its central point.Under the assumption of numerical attributes,the center point is defined as a centroid or an average of two cluster centroids subject to agglomeration.It results in centroid,median,and minimum variance linkage metrics.All of the above linkage metrics can be derived from the Lance-Williams updating formula[145],d(C iC j,C k)=a(i)d(C i,C k)+a(j)d(C j,C k)+b·d(C i,C j)+c|d(C i,C k)−d(C j,C k)|.8Pavel BerkhinHere a,b,c are coefficients corresponding to a particular linkage.This formula expresses a linkage metric between a union of the two clusters and the third cluster in terms of underlying nodes.The Lance-Williams formula is crucial to making the dis(similarity)computations feasible.Surveys of linkage metrics can be found in [170][54].When distance is used as a base measure,linkage metrics capture inter-cluster proximity.However,a similarity-based view that results in intra-cluster connectivity considerations is also used,for example,in the original average link agglomeration (Group-Average Method)[116].Under reasonable assumptions,such as reducibility condition (graph meth-ods satisfy this condition),linkage metrics methods suffer from O N 2 time complexity [177].Despite the unfavorable time complexity,these algorithms are widely used.As an example,the algorithm AGNES (AGlomerative NESt-ing)[131]is used in S-Plus.When the connectivity N ×N matrix is sparsified,graph methods directly dealing with the connectivity graph G can be used.In particular,hierarchical divisive MST (Minimum Spanning Tree)algorithm is based on graph parti-tioning [116].2.1Hierarchical Clusters of Arbitrary ShapesFor spatial data,linkage metrics based on Euclidean distance naturally gener-ate clusters of convex shapes.Meanwhile,visual inspection of spatial images frequently discovers clusters with curvy appearance.Guha et al.[99]introduced the hierarchical agglomerative clustering algo-rithm CURE (Clustering Using REpresentatives).This algorithm has a num-ber of novel features of general importance.It takes special steps to handle outliers and to provide labeling in assignment stage.It also uses two techniques to achieve scalability:data sampling (section 8),and data partitioning.CURE creates p partitions,so that fine granularity clusters are constructed in parti-tions first.A major feature of CURE is that it represents a cluster by a fixed number,c ,of points scattered around it.The distance between two clusters used in the agglomerative process is the minimum of distances between two scattered representatives.Therefore,CURE takes a middle approach between the graph (all-points)methods and the geometric (one centroid)methods.Single and average link closeness are replaced by representatives’aggregate closeness.Selecting representatives scattered around a cluster makes it pos-sible to cover non-spherical shapes.As before,agglomeration continues until the requested number k of clusters is achieved.CURE employs one additional trick:originally selected scattered points are shrunk to the geometric centroid of the cluster by a user-specified factor α.Shrinkage suppresses the affect of outliers;outliers happen to be located further from the cluster centroid than the other scattered representatives.CURE is capable of finding clusters of different shapes and sizes,and it is insensitive to outliers.Because CURE uses sampling,estimation of its complexity is not straightforward.For low-dimensional data authors provide a complexity estimate of O (N 2sample )definedA Survey of Clustering Data Mining Techniques9 in terms of a sample size.More exact bounds depend on input parameters: shrink factorα,number of representative points c,number of partitions p,and a sample size.Figure1(a)illustrates agglomeration in CURE.Three clusters, each with three representatives,are shown before and after the merge and shrinkage.Two closest representatives are connected.While the algorithm CURE works with numerical attributes(particularly low dimensional spatial data),the algorithm ROCK developed by the same researchers[100]targets hierarchical agglomerative clustering for categorical attributes.It is reviewed in the section Co-Occurrence of Categorical Data.The hierarchical agglomerative algorithm CHAMELEON[127]uses the connectivity graph G corresponding to the K-nearest neighbor model spar-sification of the connectivity matrix:the edges of K most similar points to any given point are preserved,the rest are pruned.CHAMELEON has two stages.In thefirst stage small tight clusters are built to ignite the second stage.This involves a graph partitioning[129].In the second stage agglomer-ative process is performed.It utilizes measures of relative inter-connectivity RI(C i,C j)and relative closeness RC(C i,C j);both are locally normalized by internal interconnectivity and closeness of clusters C i and C j.In this sense the modeling is dynamic:it depends on data locally.Normalization involves certain non-obvious graph operations[129].CHAMELEON relies heavily on graph partitioning implemented in the library HMETIS(see the section6). Agglomerative process depends on user provided thresholds.A decision to merge is made based on the combinationRI(C i,C j)·RC(C i,C j)αof local measures.The algorithm does not depend on assumptions about the data model.It has been proven tofind clusters of different shapes,densities, and sizes in2D(two-dimensional)space.It has a complexity of O(Nm+ Nlog(N)+m2log(m),where m is the number of sub-clusters built during the first initialization phase.Figure1(b)(analogous to the one in[127])clarifies the difference with CURE.It presents a choice of four clusters(a)-(d)for a merge.While CURE would merge clusters(a)and(b),CHAMELEON makes intuitively better choice of merging(c)and(d).2.2Binary Divisive PartitioningIn linguistics,information retrieval,and document clustering applications bi-nary taxonomies are very useful.Linear algebra methods,based on singular value decomposition(SVD)are used for this purpose in collaborativefilter-ing and information retrieval[26].Application of SVD to hierarchical divisive clustering of document collections resulted in the PDDP(Principal Direction Divisive Partitioning)algorithm[31].In our notations,object x is a docu-ment,l th attribute corresponds to a word(index term),and a matrix X entry x il is a measure(e.g.TF-IDF)of l-term frequency in a document x.PDDP constructs SVD decomposition of the matrix10Pavel Berkhin(a)Algorithm CURE (b)Algorithm CHAMELEONFig.1.Agglomeration in Clusters of Arbitrary Shapes(X −e ¯x ),¯x =1Ni =1:N x i ,e =(1,...,1)T .This algorithm bisects data in Euclidean space by a hyperplane that passes through data centroid orthogonal to the eigenvector with the largest singular value.A k -way split is also possible if the k largest singular values are consid-ered.Bisecting is a good way to categorize documents and it yields a binary tree.When k -means (2-means)is used for bisecting,the dividing hyperplane is orthogonal to the line connecting the two centroids.The comparative study of SVD vs.k -means approaches [191]can be used for further references.Hier-archical divisive bisecting k -means was proven [206]to be preferable to PDDP for document clustering.While PDDP or 2-means are concerned with how to split a cluster,the problem of which cluster to split is also important.Simple strategies are:(1)split each node at a given level,(2)split the cluster with highest cardinality,and,(3)split the cluster with the largest intra-cluster variance.All three strategies have problems.For a more detailed analysis of this subject and better strategies,see [192].2.3Other DevelopmentsOne of early agglomerative clustering algorithms,Ward’s method [222],is based not on linkage metric,but on an objective function used in k -means.The merger decision is viewed in terms of its effect on the objective function.The popular hierarchical clustering algorithm for categorical data COB-WEB [77]has two very important qualities.First,it utilizes incremental learn-ing.Instead of following divisive or agglomerative approaches,it dynamically builds a dendrogram by processing one data point at a time.Second,COB-WEB is an example of conceptual or model-based learning.This means that each cluster is considered as a model that can be described intrinsically,rather than as a collection of points assigned to it.COBWEB’s dendrogram is calleda classification tree.Each tree node(cluster)C is associated with the condi-tional probabilities for categorical attribute-values pairs,P r(x l=νlp|C),l=1:d,p=1:|A l|.This easily can be recognized as a C-specific Na¨ıve Bayes classifier.During the classification tree construction,every new point is descended along the tree and the tree is potentially updated(by an insert/split/merge/create op-eration).Decisions are based on the category utility[49]CU{C1,...,C k}=1j=1:kCU(C j)CU(C j)=l,p(P r(x l=νlp|C j)2−(P r(x l=νlp)2.Category utility is similar to the GINI index.It rewards clusters C j for in-creases in predictability of the categorical attribute valuesνlp.Being incre-mental,COBWEB is fast with a complexity of O(tN),though it depends non-linearly on tree characteristics packed into a constant t.There is a similar incremental hierarchical algorithm for all numerical attributes called CLAS-SIT[88].CLASSIT associates normal distributions with cluster nodes.Both algorithms can result in highly unbalanced trees.Chiu et al.[47]proposed another conceptual or model-based approach to hierarchical clustering.This development contains several different use-ful features,such as the extension of scalability preprocessing to categori-cal attributes,outliers handling,and a two-step strategy for monitoring the number of clusters including BIC(defined below).A model associated with a cluster covers both numerical and categorical attributes and constitutes a blend of Gaussian and multinomial models.Denote corresponding multivari-ate parameters byθ.With every cluster C we associate a logarithm of its (classification)likelihoodl C=x i∈Clog(p(x i|θ))The algorithm uses maximum likelihood estimates for parameterθ.The dis-tance between two clusters is defined(instead of linkage metric)as a decrease in log-likelihoodd(C1,C2)=l C1+l C2−l C1∪C2caused by merging of the two clusters under consideration.The agglomerative process continues until the stopping criterion is satisfied.As such,determina-tion of the best k is automatic.This algorithm has the commercial implemen-tation(in SPSS Clementine).The complexity of the algorithm is linear in N for the summarization phase.Traditional hierarchical clustering does not change points membership in once assigned clusters due to its greedy approach:after a merge or a split is selected it is not refined.Though COBWEB does reconsider its decisions,its。
机器人服务行业智能机器人操作系统考核试卷

A. rviz
B. gazebo
C. urdf_to_graphiz
D. xacro_to_urdf
8.以下哪些功能包与ROS中的导航相关?( )
A. move_base
B. amcl
C. gmapping
D. navfn
9. ROS中以下哪些功能包与机器人的路径规划有关?( )
B. roscore
C. rosnet
D. roswtf
20.以下哪些是ROS社区推荐的编程语言?( )
A. C++
B. Python
C. Lisp
D. Java
(注:以上试题内容仅供参考,实际考试内容以正式试卷为准。)
三、填空题(本题共10小题,每小题2分,共20分,请将正确答案填到题目空白处)
1. ROS的全称是_______。()
B. Real-time Operating System
C. Random Operating System
D. Robot Object-oriented System
2.以下哪项不是ROS的主要特性?( )
A.开源
B.跨平台
C.实时性
D.面向服务
3.在ROS中,用于描述机器人硬件接口的文件是:( )
2.描述ROS中话题(Topics)、服务(Services)和动作(Actions)通信的区别和特点,并给出一个实际应用场景中这三种通信方式的例子。(10分)
3.请解释URDF(Unified Robot Description Format)的作用,以及如何使用URDF和XACRO来描述一个简单的机器人模型。(10分)
HORIBA A-TEEM 分子指纹分析技术说明书

ELEMENTAL ANALYSISFLUORESCENCEOPTICAL COMPONENTSCUSTOM SOLUTIONSSPR IMAGINGAqualog®A-TEEM TMIntroducing the NEW HMMP tool for easybatch regression and discrimination analysis ofAqualog A-TEEM dataHORIBA’s patentedA-TEEM molecularfingerprinting isan ideal opticaltechnique forproductcharacterizationinvolvingcomponent quantification and identification. The HMMPAdd-In tool, powered by Eigenvector Inc. Solo, ideallycomplements the A-TEEM by supporting the developmentand batch wise application of methods for an unlimitednumber of component regression models as well asdiscrimination models. The HMMP breaks the time- andlabor-consuming barrier of analyzing individual modelsand collating results into a cohesive report to meet therequirements of industrial QA/QC applications. TheHMMP tool facilitates administrator level method modeldevelopment but more importantly push-button operator-level application and report generation.The HMMP tool is exclusive to the Aqualog A-TEEM andsupports enhanced model robustness by combining theabsorbance and fluorescence excitation-emission matrix(EEM) data using the Solo Multiblock Model tools! HMMPincorporates a direct, exclusive link to the Aqualog’s batchfile output directory for trouble-free file browsing andautomatic concatenation of absorbance and EEM data aswell as all model-dependent pre-processing.The HMMP tool mates seamlessly with data collectedusing the Fast-01 autosampler as well as any othersampling method that employs the Aqualog SampleQtoolbox.The HMMP tool supports an unlimited number ofregression models in a given method to providecomprehensive reports of all parameters of interest.Discrimination model methods with multiple class groupsare also supported to facilitate product characterizationas functions of unique compositions and component orcontaminant threshold concentrations among other QA/QC scenarios. The HMMP tool can employ a wide rangeof algorithms for discrimination and regression includingPrincipal Components Analysis (PCA), Partial LeastSquares (PLS), Artificial Neural Networks (ANN), SupportVector Machine (SVM) and Extreme Gradient Boost (XGB).Key applications supported include wine quality chemistry,water contamination and pharmaceutical productidentification and composition among many others.Key Features and Benefits• Easy, Rapid Operator Level Analysis• Facilitated Administration of Method Model Developmentand Editing• Complete Parameter Profile and Classification Reports• HMMP Add-In Fully Integrated into Eigenvector Inc.Solo/Solo+Mia and Exclusively Activated and Supportedby HORIBA Instruments Inc.• HMMP Reports include all required parameter informationand are saved in a comma separated format for LIMSsystem compatibility.• The HMMP tool is provided with ample online Helpsupport powered by the Eigenvector Inc. Wiki platformand HORIBA’s fully featured user manual.Aqualog A-TEEM Spectrometerwith FAST-01 AutosamplerPowered by Solo Predictor software fromEigenvector Research, IncorporatedHMMP SpecificationsTo learn more about theA-TEEM molecular fingerprinting technique, applications and uses of this autosampler, refer also to *******************/scientificUSA: +1 732 494 8660 France: +33 (0)1 69 74 72 00 Germany: +49 (0) 6251 8475 0UK: +44 (0)1604 542 500 Italy: +39 06 51 59 22 1 Japan: +81(75)313-8121 China: +86 (0)21 6289 6060 India: +91 80 41273637 Singapore: +65 (0)6 745 8300Taiwan: +886 3 5600606Brazil: +55 (0)11 2923 5400 Ot h er:+33 (0)1 69 74 72 00The HMMP user interface facilitates method development and selection, fully articulated data file browsing with data integrity warnings and push-button report generation.。
基于算法融合的高速公路断面交通量预测

0引言在现代综合交通运输系统中,高速公路是联结普通国省道等干线公路的重要通道。
高速公路的快速发展,为综合运输体系的建立开辟了道路和创造了有利条件[1]。
然而随着经济的高速发展,汽车保有量不断增长,使高速公路交通供需失衡,许多交通往来频繁的高速公路出现了严重拥堵的现象。
目前,我国交通管理者主要通过两种途径来缓解高速公路的交通拥堵[2]:一是加大交通供给,通过新建高速公路、扩宽原有道路等方式提高路网的容纳能力;二是从交通需求方面入手,采用先进的交通管理手段,科学、合理、有效地诱导车辆通行,充分地利用现有道路资源,提升高速公路网络的服务水平。
实践证明,不断加大线网密度、“按需增供”的方法不仅需要投入大量的人力物力,其增长速度也难以满足快速增长的交通需求[3],因此,缓解交通拥堵的措施越来越倾向于提高道路交通管理水平[4]。
作为交通流理论的重要参数,交通量是智能交通系统构建的基础。
合理、准确地预测交通量,可为未来的交通状态预判提供数据支撑,提高交通管理水平。
目前,我国高速公路系统积累了大量数据,如何基于这些数据分析交通流的特性并采用合适的算法对未来的交通量进行实时、有效地预测,是智能交通系统在高速公路系统内应用的重要研究课题。
李文勇等[5]基于改进的ARIMA 模型对交通流进行了预测,杨兆升等[6]考虑与交通流相关的非线性特征,采用SVM 模型对交通量进行预测,结果表明该模型在预测精度、泛化能力等方面的表现优于BP 神经网络模型,钟颖等[7]基于XGBoost 模型建立时间序列预测模型以及时空序列预测模型,并与线性回归模型、神经网络模型预测结果进行了比较。
本论文依据路段交通饱和度对交通流进行服务水平划分,基于ARIMA 、XGBoost 、SVR 、BP 和GRU 五种模型对高速公路交通量进行预测,并提出融合SVR 、BP 和GRU 模型的预测模型,提高了高速公路断面交通量预测的准确率。
1数据预处理选取济南市绕城高速上的38个门架作为研究对象,统计其每15分钟的交通量。
INTERNATIONAL JOURNAL OF WIRELESS AND MOBILE COMPUTING (IJWMC) 1 A Biologically Inspired Qo
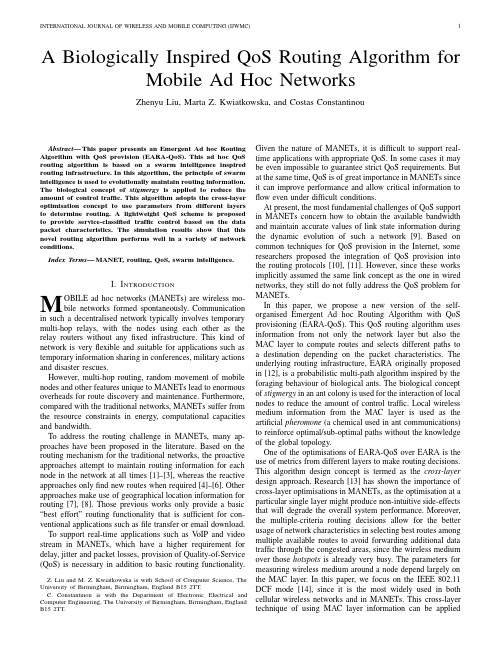
A Biologically Inspired QoS Routing Algorithm forMobile Ad Hoc NetworksZhenyu Liu,Marta Z.Kwiatkowska,and Costas ConstantinouAbstract—This paper presents an Emergent Ad hoc Routing Algorithm with QoS provision(EARA-QoS).This ad hoc QoS routing algorithm is based on a swarm intelligence inspired routing infrastructure.In this algorithm,the principle of swarm intelligence is used to evolutionally maintain routing information. The biological concept of stigmergy is applied to reduce the amount of control traffic.This algorithm adopts the cross-layer optimisation concept to use parameters from different layers to determine routing.A lightweight QoS scheme is proposed to provide service-classified traffic control based on the data packet characteristics.The simulation results show that this novel routing algorithm performs well in a variety of network conditions.Index Terms—MANET,routing,QoS,swarm intelligence.I.I NTRODUCTIONM OBILE ad hoc networks(MANETs)are wireless mo-bile networks formed munication in such a decentralised network typically involves temporary multi-hop relays,with the nodes using each other as the relay routers without anyfixed infrastructure.This kind of network is veryflexible and suitable for applications such as temporary information sharing in conferences,military actions and disaster rescues.However,multi-hop routing,random movement of mobile nodes and other features unique to MANETs lead to enormous overheads for route discovery and maintenance.Furthermore, compared with the traditional networks,MANETs suffer from the resource constraints in energy,computational capacities and bandwidth.To address the routing challenge in MANETs,many ap-proaches have been proposed in the literature.Based on the routing mechanism for the traditional networks,the proactive approaches attempt to maintain routing information for each node in the network at all times[1]–[3],whereas the reactive approaches onlyfind new routes when required[4]–[6].Other approaches make use of geographical location information for routing[7],[8].Those previous works only provide a basic “best effort”routing functionality that is sufficient for con-ventional applications such asfile transfer or email download. To support real-time applications such as V oIP and video stream in MANETs,which have a higher requirement for delay,jitter and packet losses,provision of Quality-of-Service (QoS)is necessary in addition to basic routing functionality. Z.Liu and M.Z.Kwiatkowska is with School of Computer Science,The University of Birmingham,Birmingham,England B152TT.C.Constantinou is with the Department of Electronic Electrical and Computer Engineering,The University of Birmingham,Birmingham,England B152TT.Given the nature of MANETs,it is difficult to support real-time applications with appropriate QoS.In some cases it may be even impossible to guarantee strict QoS requirements.But at the same time,QoS is of great importance in MANETs since it can improve performance and allow critical information to flow even under difficult conditions.At present,the most fundamental challenges of QoS support in MANETs concern how to obtain the available bandwidth and maintain accurate values of link state information during the dynamic evolution of such a network[9].Based on common techniques for QoS provision in the Internet,some researchers proposed the integration of QoS provision into the routing protocols[10],[11].However,since these works implicitly assumed the same link concept as the one in wired networks,they still do not fully address the QoS problem for MANETs.In this paper,we propose a new version of the self-organised Emergent Ad hoc Routing Algorithm with QoS provisioning(EARA-QoS).This QoS routing algorithm uses information from not only the network layer but also the MAC layer to compute routes and selects different paths to a destination depending on the packet characteristics.The underlying routing infrastructure,EARA originally proposed in[12],is a probabilistic multi-path algorithm inspired by the foraging behaviour of biological ants.The biological concept of stigmergy in an ant colony is used for the interaction of local nodes to reduce the amount of control traffic.Local wireless medium information from the MAC layer is used as the artificial pheromone(a chemical used in ant communications) to reinforce optimal/sub-optimal paths without the knowledge of the global topology.One of the optimisations of EARA-QoS over EARA is the use of metrics from different layers to make routing decisions. This algorithm design concept is termed as the cross-layer design approach.Research[13]has shown the importance of cross-layer optimisations in MANETs,as the optimisation at a particular single layer might produce non-intuitive side-effects that will degrade the overall system performance.Moreover, the multiple-criteria routing decisions allow for the better usage of network characteristics in selecting best routes among multiple available routes to avoid forwarding additional data traffic through the congested areas,since the wireless medium over those hotspots is already very busy.The parameters for measuring wireless medium around a node depend largely on the MAC layer.In this paper,we focus on the IEEE802.11 DCF mode[14],since it is the most widely used in both cellular wireless networks and in MANETs.This cross-layer technique of using MAC layer information can be appliedeasily to other MAC protocols.In addition to the basic routing functionality,EARA-QoS supports an integrated lightweight QoS provision scheme.In this scheme,traffic flows are classified into different service classes.The classification is based on their relative delay bounds.Therefore,the delay sensitive traffic is given a higher priority than other insensitive traffic flows.The core technique of the QoS provision scheme is a token bucket queuing scheme,which is used to provide the high priority to the real-time traffic,and also to protect the lower-priority traffic from star-vation.Experimental results from simulation of mobile ad hoc networks show that this QoS routing algorithm performs well over a variety of environmental conditions,such as network size,nodal mobility and traffic loads.II.B ACKGROUNDIn this section,we give a brief introduction to background knowledge on ant colony heuristics,and the QoS provision techniques in MANETs.A.Foraging Strategies in AntsOne famous example of biological swarm social behaviour is the ant colony foraging [15](see Figure 1).Many ant species have a trail-laying,trail-following behaviour when foraging:individual ants deposit a chemical substance called pheromone as they move from a food source to their nest,and foragers follow such pheromone trails.Subsequently,more ants are attracted by these pheromone trails and in turn reinforce them even more.As a result of this auto-catalytic effect,the optimal solution emerges rapidly.In this food searching process a phenomenon called stigmergy plays a key role in developing and manipulating local information.It describes the indirect communication of individuals through modifying theenvironment.Fig.1.All Ants Attempt to Take the Shortest PathFrom the self-organisation theory point of view,the be-haviour of the social ant can be modelled based on four elements:positive feedback,negative feedback,randomness and multiple interactions [16].This model of social ants using self-organisation theories provides powerful tools to transfer knowledge about the social insects to the design of intelligent decentralised problem-solving systems.B.Quality-of-Service in MANETsQuality-of-Service (QoS)provision techniques are used to provide some guarantee on network performance,such as average delay,jitter,etc.In wired networks,QoS provision can generally be achieved with the over-provisioning of re-sources and with network traffic engineering [17].With the over-provisioning approach,resources are upgraded (e.g.fibre optic data link,advanced routers and network cards)to make networks more resistant to resource demanding applications.The advantage of this approach is that it is easy to be implemented.The main disadvantage of this approach is that all the applications still have the same priority,and the network may become unpredictable during times of bursting and peak traffic.In contrast,the idea of the traffic engineering approach is to classify applications into service classes and handle each class with a different priority.This approach overcomes the defect of the former since everyone is following a certain rule within the network.The traffic engineering approach has two complemen-tary means to achieve QoS provisioning,Integrated Services (IntServ)and Differentiated Services (DiffServ).IntServ [18]provides guaranteed bandwidth for flows,while DiffServ [19]provides hard guarantees for service classes.Both of the approaches rely on the possibility to make bandwidth reservations.The former was used in ATM (Asynchronous Transfer Mode)[20]and is today the method of achieving QoS in RSVP-IntServ [21].On the other hand,in the DiffServ approach,no reservation is done within the network.Instead,QoS is achieved by mechanisms such as Admission Control ,Policy Manager ,Traffic Classes and Queuing Schedulers .These mechanisms are used to mark a packet to receive a particular forwarding or dropping treatment at each node.Based on QoS provision techniques in wired networks,many QoS approaches are proposed to provide QoS services for MANETs.Flexible QoS Model for MANETs (FQMM)[22],is the first QoS approach for MANETs,which combines knowledge on IntServ/DiffServ in wired networks with con-sideration of MANETs.As an essential component to achieve the QoS provisioning,QoS routing algorithms tightly integrate QoS provisioning into routing protocols.The QoS version of AODV (QoS-AODV)[23],the Core-Extraction Distributed Ad Hoc Routing (CEDAR)protocol [10],the Multimedia Support for Mobile Wireless Networks (MMWN)protocol [11],and the ticket-based protocols [24]are examples of QoS routing algorithms proposed for MANETs.On the other hand,QoS signaling techniques are inde-pendent of the underlying routing protocols.The In-band Signalling for QoS in Ad-Hoc Mobile Networks (INSIGNIA)algorithm [25]is the typical signaling protocol designed exclusively for MANETS.The idea of CEDAR,MMWN,and ticket-based protocols is to disseminate link-state information across the network in order to enable other nodes to find routes that meet certain QoS criteria,like the minimum bandwidth.On the other hand,INSIGNIA piggybacks resource reservations onto data packets,which can be modified by intermediate nodes to inform the communication endpoint nodes in case of lack ofresources.All those approaches are based on the idea that the wireless links between mobile nodes have certain QoS related properties,in particular a known amount of available bandwidth,and that nodes are able to give guarantees for traffic traversing these links.III.C RITIQUE OF E XISTING Q O S A PPROACHES INMANET SNowadays,most of the QoS provisioning techniques are derived from the QoS approaches of the wired networks. However,QoS support approaches proposed in wired networks are based on the assumption that the link characteristics such as bandwidth,delay,loss rate and error rate must be available and manageable.However,given the challenges of MANETs, e.g.dynamic topology and time-varying link capacity,this assumption does not apply any longer.Thus,applying the concepts of wired traffic engineering QoS approaches directly to MANETs is extremely difficult.Generally,the situation in MANETs is completely different from those in wired networks.In wireless networks,the available bandwidth undergoes fast time-scale variations due to channel fading and errors from physical obstacles.These effects are not present in wired networks.In MANETs,the wireless channel is a shared-access medium,and the available bandwidth even varies with the number of hosts contending for the channel.Below we analyse why the IntServ/DiffServ models are not appropriate for MANETs respectively. IntServ based approaches are not applicable for MANETs mainly due to two factors,huge resource consumption and computation power limitation.Firstly,to support IntServ,a huge amount of link state information has to be built and main-tained for each mobile node.The amount of state information increases proportionally with the number offlows,which is also a problem with the current IntServ QoS scheme.Secondly, current wireless networks employ two major MAC techniques, the single-channel approach and the multiple channel ap-proach.With single-channel approach(e.g.IEEE802.11[14]), all nodes share the same channel and therefore potentially interfere with each other.With a multiple-channel approach (e.g.Bluetooth[26]or CDMA[27]),nodes can communicate on several channels simultaneously.Both of the two MAC techniques have a similar bandwidth reservation mechanism. This common mechanism requires a transmission schedule to define time slots,in which nodes take their turns periodically. For each slot,its duration and a set of possible simultaneous transmissions must be defined.However,in wireless networks, the problem offinding an optimal schedule is proved to be NP-complete[28],which is a fundamental limitation of QoS provisioning in wireless networks.On the other hand,the DiffServ approach is a lightweight QoS model for interior routers since individual stateflows are aggregated into sets of service classes whose packets are treated differently at the routing nodes.This makes routing a lot easier in the network.Thus this approach could be a potential solution for MANETs.Even though it is not practical to provide a hard separation of different service classes in MANETs,relative prioritisation is possible in such a way that traffic of a certain class is given a higher or lower priority than traffic of other service classes.One solution would be to divide the traffic into a predefined set of service classes that are defined by their relative delay bounds,such as delay sensitive(realtime)and insensitive(bulk)traffic.Realtime traffic should be given higher priority than bulk traffic.No absolute bandwidth guarantees are provided.Some work based on service differentiation rather than resource reservations in MANETs already exists[29].IV.D ESCRIPTION OF EARA-Q O SEARA-QoS is an on-demand multipath routing algorithm for MANETs,inspired by the ant foraging intelligence.This algorithm incorporates positive feedback,negative feedback and randomness into the routing computation.Positive feed-back originates from destination nodes to reinforce the existing pheromone on good paths.Ant-like packets,analogous to the ant foragers,are used to locallyfind new paths.Artificial pheromone is laid on the communication links between nodes and data packets are biased towards strong pheromone,but the next hop is chosen probabilistically.To prevent old routing solutions from remaining in the current network status,expo-nential pheromone decay is adopted as the negative feedback. Each node using this algorithm maintains a probabilistic routing table.In this routing table,each route entry for the destination is associated with a list of neighbour nodes.A probability value in the list expresses the goodness of node as the next hop to the destination.For each neighbour, the shortest hop distance to the destination and the largest sequence number seen so far are also recorded.In addition to the routing table,each node also possesses a pheromone table.This table tracks the amount of pheromone on each neighbour link.The table may be viewed as a ma-trix with rows corresponding to neighbourhood and columns to destinations.There are three threshold values controlling the bounds on pheromone in the table.They are the upper pheromone that prevents extreme differences in pheromone, the lower pheromone,below which data traffic cannot be forwarded,and the initial pheromone that is assigned when a new route is found.In addition to the routing data structures present above,the following control packets are used in EARA-QoS to perform routing computation:Route Request Packet(RQ)containing destination ad-dress,source address and broadcast ID.Route Reply Packet(RP)containing source address,des-tination address,sequence number,hop account and life-time.Reinforcement Signal(RS)containing destination ad-dress,pheromone value and sequence number.Local Foraging Ant(LFA)containing source address (the node that sent LFA),the least hop distance from the source to the destination,stack of intermediate node address and hop count.Hello Packet(HELLO)containing source(the node that sent Hello)address and hop count(set to0).A.Parameters of Lower Layers1)The Average MAC Layer Utilisation:Thefirst metric is the average MAC layer utilisation for a node.This metric measures the usage of the wireless medium around that node. As the instantaneous MAC layer utilisation at a node is either (busy)or(idle),we average this value over a period of time window as follows:(1) where is the time when the medium is busy in the window.This average MAC utilisation indicates the degree to which the wireless medium around that node is busy or idle.We consider the instantaneous MAC layer utilisation level at a node to be1when the wireless medium around that node either detects physical carrier to be present or is deferring due to virtual carrier sensing,inter-frame spacing,or backoff.In addition,we also consider the medium is busy at any time when the node has at least one packet in the transmission queue.2)The Transmission Queue Heuristic:The second metric isa heuristic value that is calculated with the network interface transmission queue length in the current node.Apart from the media status,the transmission queue length is also a key factor that can affect the packet latency or packet drop due to the size limit on the queue length.We define the heuristic value with the following rules.If the outgoing network interface employs a single queue scheme,the heuristic value is defined as:(2) where is the length(in bytes waiting to be sent)of the interface queue in node,and is the maximum packet bytes allowed in the queue.If the network interface employs the multiple virtual queue scheme for each outgoing link,the heuristic value is defined as:(3)where is the length(in bytes waiting to be sent)of the virtual queue of the link in node and denotes the neighbourhood of node as a next-hop to some destination.3)The Average MAC Layer Delay:The last metric is the MAC layer delay for the link.The MAC layer delay is defined as the interval from when the RTS frame is sent at node to when the data frame is received successfully at node.The average MAC delay is obtained by averaging these values over a time window as follows:(4)where is the time interval in the window,and is a coefficient.This average MAC delay indicates the degree of interference.In regions where there is a lot of interference from other nodes,MAC delay is high due to the contentionof the channel.B.Data PropagationWhen multiple virtual queue scheme is employed,the rout-ing probability value is computed by the composition ofthe pheromone values,the local heuristic values and the linkdelays as follows:(5) where,and()are tunable parametersthat control the relative weight of pheromone trail,MAC delay and heuristic value,and is the neighbourhood as a next-hop to some destination.Incorporating the heuristic value and link delay in the rout-ing computation makes this algorithm possess the congestionawareness property.Based on the probabilistic routing table, data traffic will be distributed according to the probabilitiesfor each neighbour in the routing table.The routing algorithmexhibits load balancing behaviour.Nodes with a large number of packets in the buffer are avoided.The EARA-QoS algorithm consists of several components.They are the route discovery procedure,the positive and neg-ative reinforcement,and the local connectivity management.C.Route DiscoveryWe use a similar route discovery procedure as describedin[12].On initialisation,a neighbourhood for each node is built using the single-hop HELLO messages.Whenever atraffic source needs a route to a destination,it broadcastsroute request packets(RQ)across the network.Rather than simplyflooding the RQ packets,we adopt the probabilisticbroadcast scheme explored in[30]combined with the MAClayer utilisation.When a nodefirst receives a packet,with probability it broadcasts the packet to its neighbours,andwith probability it discards the packet.The probabilityvalue is calculated as(6) where()is the coefficient.This broadcast scheme helps to discover new routes avoiding congestion areas,but atthe cost of missing potential routes to the destination. During the course offlooding RQ packets to the destination ,the intermediate node receiving a RQ packetfirst sets up reverse paths to the source by recording the source addressand the previous hop node in the message cache.If a validroute to the destination is available,that is,there is at least one link associated with the pheromone trail greater than the lower pheromone bound,the intermediate node generates a route reply(RP).The RP is routed back to the source via the reverse paths.Otherwise,the RQ is rebroadcast.Other than just establishing a single forward path,whenthe destination node receives RQs it will send a RP to allthe neighbours from which it sees a RQ.In order to maintain multiple loop-free paths at each intermediate node,node(b) Path Reinforcement(c) Local Repair(a) Initial Pheromone Setup Fig.2.Illustrating Working Mechanism of EARA-QoSmust record all new forward paths that possess the latest sequence number but hold a lower hop-count in its routing table,and also send a RP to all the neighbours from which it saw a RQ.During the course of the RP tracking back to the source,an initial pheromone value is assigned to the corresponding neighbour node,which indicates a valid route to the destination.This process is illustrated in Figure2(a).D.Route ReinforcementAfter the destination node receives the data traffic sent by the source node,it begins to reinforce some good neighbour(s)in order to“pull”more data traffic through the good path(s)by sending reinforcement signal packets(RS) whenever it detects new good paths.When node receives a RS,it knows it has an outgoing link toward the destination ,which is currently deemed a good path.Subsequently, node updates the corresponding pheromone table entry with the value and forwards a RS packet to(at least one) selected neighbour locally based on its message cache,e.g.the neighbour(s)that saw the least hops of the incoming packets. The amount of the pheromone used to positively rein-force the previous hop neighbour is computed as follows.If the RS packet is sent by the destination to node,then is calculated using the upper bound pheromone value ,(7) If the RS packet is sent by an intermediate node towards node,the is calculated using the current largest pheromone value max()in node with the next hop to the destination in the pheromone table,max(8) where,and are parameters that control the relative weight of the relative source hop distance,the rel-ative packet number and the local queue heuristic. Incorporating the congestion-measuring metric into the reinforcement can lead data traffic to avoid the congestion areas.The relative source hop distance is calculated as follows:(9) where is the shortest hop distance from the source to the current node through node,and is the shortest hop distance from to.This parameter is used to ensure that paths with shorter hop distance from the source node to the current node are reinforced with more pheromone.The relative packet number is calculated as follows:(10) where is the number of incoming packets from neighbour to the destination,and is the total number of incomingpackets towards the destination.This parameter is used to indicate that the data forwarding capacity of a link also affects the reinforcement.The more data arrives,the stronger reinforcement is generated for the corresponding link.On receiving the RS from a neighbour,node needs to positively increase the pheromone of the link towards node.If the sequence number in the RS is greater than the one recorded in the pheromone table,node updates its corresponding pheromone with the value of carried on the RS:(11) If the sequence number is equal to the current one,then:ifotherwise(12)If the sequence number in RS is less than the current one in the pheromone table,then this RS is just discarded.Node also has to decide to reinforce(at least)one of its neighbours by sending the RS message based on its own message cache.This process will continue until reaching the source node.As a result of this reinforcement,good quality routes emerge,which is illustrated in Figure2(b).The same procedure can apply to any intermediate node to perform local link error repair as long as it has pheromone value that is greater than the lower bound.For instance,if an intermediate node detects a link failure from one of its upstream links, it can apply the reinforcement rules to discover an alternative path as shown in Figure2(c).There is also an implicit negative reinforcement for the pheromone values.Within every time interval,if there is no data towards a neighbour node,its corresponding pheromone value decays by a factor as follows:(13)E.Local Foraging AntsIn a dynamic network like MANET,the changes of the net-work topology create chances for new good paths to emerge.In order to make use of this phenomenon,this algorithm launcheslocal foraging ants(LFA)with a time interval to locallysearch for new routes whenever all the pheromone trails of a node towards some destination drop below the threshold.The LFA will take a random walk from its original node. During the course of its walk,if the LFA detects congestionaround a node(the average channel utilisation is greaterthan a predefined threshold value),then the LFA dies to avoid increasingly use the wireless medium.Otherwise,theLFA pushes the address of the nodes that it has travelledinto its memory stack.To avoid forming of loops,LFA will not choose to travel to the node that is already in.Before reaching the maximum hop,if LFA canfind a node with pheromone trails greater than and the hop distanceto destination not greater than the one from its original nest,itreturns to its’nest’following its memory stack and updates the corresponding paths with.Otherwise,it simply dies.F.Local Connectivity ManagementNodes maintain their local connectivity in two ways.When-ever a node receives a packet from a neighbour,it updates its local connectivity information to ensure that it includes thisneighbour.In the event that a node has not sent any packets toits neighbours within a time interval,it has to broadcast a HELLO packet to its neighbours.Failure to receive packetsfrom the neighbourhood in indicates changes in the local connectivity.If HELLO packets are not received from the nexthop along an active path,the node that uses that next hop issent notification of link failure.In case of a route failure occurring at node,cannot for-ward a data packet to the next hop for the intended destination .Node sends a RS message that sets ROUTE RERR tag to inform upstream nodes of the link failure.This RS signalassigns to the corresponding links the lower bound.Here, RS plays the role of an explicit negative feedback signal to negatively reinforce the upstream nodes along the failure path. This negative feedback avoids causing buffer overflow due to caching on-flight packets from upstream nodes. Moreover,the use of HELLO packets can also help to ensure that only nodes with bidirectional connectivity are deemed as neighbours.For this purpose,the HELLO packet sent by a node has an option to list the nodes from which it has heard HELLO packets,and nodes that receive the HELLO check to ensure that it uses only routes to neighbours that have sent HELLO packets.G.The QoS Provision SchemeThis section describes a lightweight approach to DiffServ. The basic idea is to classifyflows into a predefined set of service classes by their relative delay bounds.Admission control only works at the source node.There is no session orflow state information maintained at intermediate nodes. Once a realtime session is admitted,its packets are marked as RT(realtime service)and otherwise they are considered as best-effort bulk packets.As depicted in Figure3,each of these traffic classes is buffered in a logically separate queue.A simple novel queuing strategy,based on the token bucket scheme,provides high priority to realtime traffic,and also protects the lower-priority traffic from starvation.No absolute bandwidth guarantees are provided in this scheme.We explain this queuing strategy and its novelty below.The queues are scheduled according to a token bucket scheme.In this scheme,prioritisation is achieved with token balancing.Each traffic class has a balance of tokens,and the class with higher balance has a higher priority when dequeuing the next packet for transmission.For each transmission of a packet of class,an amount of tokens is subtracted from the class’token balance and an equal fraction thereof is added to every other class’balance such that the sum of all tokens is always the same.The weight value reflects the delay sensitivity assigned to the different classes.A higher weight value corresponds to a lower delay sensitivity.The size of the token balance together with the value determines the maximal length of a burst of traffic from one class.In this scheme,as long as the amount of delay-sensitive traffic does not grow too large,it is forwarded as quickly as possible,and if it does grow too large,starvation of other traffic classes is prevented.Setting the upper bound of a class’token balance depending on its delay-sensitivity enables further tuning of the describedmethod.Fig.3.Overview of Service Differentiation SchemeIn this packet scheduling scheme,routing protocol pack-ets are given unconditional priority before other packets. Moreover,realtime applications normally have stringent delay bounds for their traffic.This means that packets arriving too late are useless.From the application’s point of view,there is no difference between late and lost packets.This implies that it is actually useless to forward realtime packets that stay in a router for more than a threshold amount of time,because they will be discarded at the destination anyway.Dropping those packets instead has the advantage of reducing the load in the network.To our knowledge,this service classification based queuing scheme is the simplest implemented QoS provisioning technique designed exclusively for MANETs so far.V.C HARACTERISTICS OF THE A LGORITHMThis proposed protocol,implementing the cross-layer design concept,exhibits some properties that show itsfitness as a solution for mobile ad hoc networks:Loop-freeness:during the route discovery phase,the nodes record the unique sequence number of RP packets.。
MAX14878-MAX14880 高速传输接收器的中文名字说明书
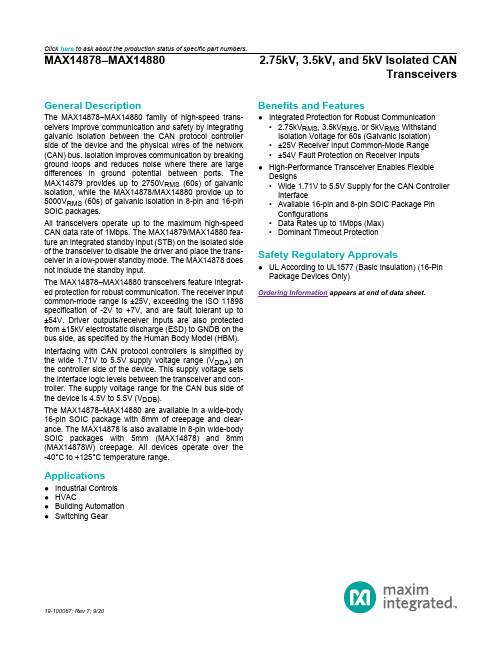
General DescriptionThe MAX14878–MAX14880family of high-speed trans-ceivers improve communication and safety by integrating galvanic isolation between the CAN protocol controller side of the device and the physical wires of the network (CAN)bus.Isolation improves communication by breaking ground loops and reduces noise where there are large differences in ground potential between ports.The MAX14879provides up to2750V RMS(60s)of galvanic isolation,while the MAX14878/MAX14880provide up to 5000V RMS(60s)of galvanic isolation in8-pin and16-pin SOIC packages.All transceivers operate up to the maximum high-speed CAN data rate of1Mbps.The MAX14879/MAX14880fea-ture an integrated standby input(STB)on the isolated side of the transceiver to disable the driver and place the trans-ceiver in a low-power standby mode.The MAX14878does not include the standby input.The MAX14878–MAX14880transceivers feature integrat-ed protection for robust communication.The receiver input common-mode range is±25V,exceeding the ISO11898 specification of-2V to+7V,and are fault tolerant up to ±54V.Driver outputs/receiver inputs are also protected from±15kV electrostatic discharge(ESD)to GNDB on the bus side, as specified by the Human Body Model (HBM). Interfacing with CAN protocol controllers is simplified by the wide1.71V to5.5V supply voltage range(V DDA)on the controller side of the device.This supply voltage sets the interface logic levels between the transceiver and con-troller.The supply voltage range for the CAN bus side of the device is 4.5V to 5.5V (V DDB).The MAX14878–MAX14880are available in a wide-body 16-pin SOIC package with8mm of creepage and clear-ance.The MAX14878is also available in8-pin wide-body SOIC packages with5mm(MAX14878)and8mm (MAX14878W)creepage.All devices operate over the -40°C to +125°C temperature range.Applications●Industrial Controls●HVAC●Building Automation●Switching Gear Benefits and Features●Integrated Protection for Robust Communication• 2.75kV RMS, 3.5kV RMS, or 5kV RMS Withstand Isolation Voltage for 60s (Galvanic Isolation)•±25V Receiver Input Common-Mode Range•±54V Fault Protection on Receiver Inputs●High-Performance Transceiver Enables FlexibleDesigns•Wide 1.71V to 5.5V Supply for the CAN Controller Interface•Available 16-pin and 8-pin SOIC Package Pin Configurations•Data Rates up to 1Mbps (Max)•Dominant Timeout ProtectionSafety Regulatory Approvals●UL According to UL1577 (Basic Insulation) (16-PinPackage Devices Only)Ordering Information appears at end of data sheet.Click here to ask about the production status of specific part numbers.MAX14878–MAX14880 2.75kV, 3.5kV, and 5kV Isolated CANTransceiversSimplified Block DiagramAbsolute Maximum RatingsV DDA to GNDA.........................................................-0.3V to +6V V DDB to GNDB.........................................................-0.3V to +6V TXD to GNDA...........................................................-0.3V to +6V RXD to GNDA...........................................-0.3V to (V DDA+ 0.3V) STB to GNDB...........................................................-0.3V to +6V I.C. to GNDB.............................................-0.3V to (V DDB+ 0.3V) CANH or CANL to GNDB, (Continuous).................-54V to +54V Short-Circuit Duration (CANH to CANL).....................Continuous Short-Circuit Duration (RXD to GNDA or V DDA)........Continuous Continuous Power Dissipation (T A= +70ºC)16-pin W SOIC (derate 14.1mW/°C above +70°C)..1126.8mW 8-pin W SOICW8MS+1 (derate 9.39mW/°C above +70°C)........751.17mW W8MS+5 (derate 11.35mW/°C above +70°C)......908.06mW Operating Temperature Range.............................-40ºC to 125ºC Junction Temperature.......................................................+150ºC Storage Temperature Range..............................-60ºC to +150ºC Lead Temperature (soldering, 10s)...................................+300ºC Soldering Temperature (reflow)........................................+260ºCNOTE:See the Isolation section of the Electrical Characteristics table for maximum voltage from GNDA to GNDBStresses beyond those listed under “Absolute Maximum Ratings” may cause permanent damage to the device. These are stress ratings only, and functional operation of the device at these or any other conditions beyond those indicated in the operational sections of the specifications is not implied. Exposure to absolute maximum rating conditions for extended periods may affect device reliability.Package InformationFor the latest package outline information and land patterns (footprints), go to /packages. Note that a “+”, “#”, or “-” in the package code indicates RoHS status only. Package drawings may show a different suffix character, but the drawing pertains to the package regardless of RoHS status.Package thermal resistances were obtained using the method described in JEDEC specification JESD51-7, using afour-layer board. For detailed information on package thermal considerations, refer to / thermal-tutorial.Electrical Characteristics(V DDA=1.71V to5.5V,V DDB=4.5V to5.5V,T A=-40°C to+125°C,STB or I.C.=GNDB.Typical values are at T A=+25°C with GNDA = GNDB, V DDA= 3.3V, V DDB= 5V. (Notes 1, 2)PARAMETER SYMBOL CONDITIONS MIN TYP MAX UNITS POWERProtocol Controller Side(A-Side) Voltage SupplyV DDA 1.71 5.5V CAN Bus Side (B-Side)Voltage SupplyV DDB 4.5 5.5VV DDA Supply Current I DDA V DDA= 5V0.340.83mA V DDA= 3.3V0.340.76V DDA= 1.8V0.330.64V DDB Supply Current I DDB V DDB= 5V, TXD = GNDA,R L= open4.37.3mA V DDB= 5V, TXD = GNDA, R L= 60Ω47.667.3V DDB= 5V, TXD = V DDA, R L= 60Ω 3.2V DDB= 5V, CANH shorted to CANL,TXD = V DDA3.2V DDB= 5V, CANH shorted to CANL,TXD = GNDA94140V DDB= 5V, TXD = V DDA, R L= 60Ω,STB = V DDB(MAX14879/MAX14880)0.40.8V DDA UndervoltageLockout Threshold,RisingV DDAUVLO_R 1.66VV DDA Undervoltage-Lockout Threshold,FallingV DDAUVLO_F 1.3 1.55VV DDB Undervoltage-Lockout Threshold,RisingV DDBUVLO_R 4.25VV DDB Undervoltage-Lockout Threshold,FallingV DDBUVLO_F 3.45V CANH, CANL TRANSMITTERDominant Output Voltage V O(DOM)V TXD= 0V,R L= 50Ω to 65ΩCANH 2.75 4.5VCANL0.5 2.25Electrical Characteristics (continued)(V DDA=1.71V to5.5V,V DDB=4.5V to5.5V,T A=-40°C to+125°C,STB or I.C.=GNDB.Typical values are at T A=+25°C with GNDA = GNDB, V DDA= 3.3V, V DDB= 5V. (Notes 1, 2)PARAMETER SYMBOL CONDITIONS MIN TYP MAX UNITSDominant Differential Bus Output Voltage V OD(V CANH- V CANL),V TXD= 0V,R L= 50Ω to65Ω, Figure 1R CM is open 1.53V (V CANH- V CANL),V TXD= 0V,R L= 50Ω to65Ω, Figure 2R CM= 1.25kΩ,-17V < V CM<+17V1.53(V CANH- V CANL),V TXD= 0V,R L= 50Ω to65Ω, Figure 3RCM = 1.25kΩ,-25V < VCM <+25V1.13Recessive Voltage Output V ORV TXD= V DDA,No loadCANH23VCANL23Short-Circuit Current I SHORT V TXD= 0V CANH shorted toGNDB5075100mA CANL shorted toVDDB5075100Recessive Differential Bus Output Voltage V ODR(V CANH- V CANL),V TXD= V DDARL is open-500+50mVRL = 60Ω-120+12CANH/CANL OutputVoltage in Standby Mode V STBMAX14879/MAX14880 only,V TXD= V DDA, No load, STB = V DDB70175mVDC BUS RECEIVER (CANH and CANL externally driven)Common Mode Input Range V CMCANH or CANL toGNDB, RXDoutput validNormal operation-25+25VStandby mode(MAX14879/MAX14880 only)-12+12Differential Input Voltage V DIFF V TXD= V DDA Recessive0.5V Dominant, No load0.9Differential InputHysteresisV DIFF(HYST)125mVStandby Mode Differential Input Voltage MAX14879/MAX14880 only,V TXD= V DDA,V STB= V DDBRecessive0.45VDominant 1.15Common-Mode Input Resistance R INV TXD= V DDA, R IN= ΔV/∆I,∆V = +300mV, V STB= GNDB(MAX14879/MAX14880)1050kΩDifferential Input Resistance R IDV TXD= V DDA, R IN= ∆V/∆I,∆V = +300mV, V STB= GNDB(MAX14879/MAX14880)20100kΩInput Leakage Current I LKG V DDB= 0V, V CANH= V CANL= 5V310μA Input Capacitance C IN CANH or CANL to GNDB (Note 3)14.420pFElectrical Characteristics (continued)(V DDA=1.71V to5.5V,V DDB=4.5V to5.5V,T A=-40°C to+125°C,STB or I.C.=GNDB.Typical values are at T A=+25°C with GNDA = GNDB, V DDA= 3.3V, V DDB= 5V. (Notes 1, 2)PARAMETER SYMBOL CONDITIONS MIN TYP MAX UNITS Differential InputCapacitanceC IND CANH to CANL (Note 3)7.210pF LOGIC INTERFACE (RXD, TXD, STB)Input High Voltage V IH TXD 1.71V ≤ V DDA<2.25V0.75 xV DDAV 2.25V ≤ V DDA≤5.5V0.7 xV DDASTB (MAX14879/MAX14880 only)0.7 xV DDBInput Low Voltage V IL TXD, 1.71 ≤ V DDA< 2.25V0.7V TXD, 2.25V ≤ V DDA≤ 5.5V0.8STB (MAX14879/MAX14880 only)0.8Output High Voltage V OH RXD, I SOURCE= 4mA V DDA-0.4VOutput Low Voltage V OL RXD, I SINK= 4mA0.4V Input Pullup Current I PU TXD-10-5-1.5μA Input PulldownResistanceR PD STB (MAX14879/MAX14880 only)75250kΩInput Capacitance5pF PROTECTIONFault Protection Range CANH to GNDB, CANL to GNDB-54+54VESD Protection (CANH and CANL to GNDB)IEC 61000-4-2 Air-Gap Discharge±10kV IEC 61000-4-2 Contact Discharge±5Human Body Model±15ESD Protection (CANH and CANL to GNDA)IEC 61000-4-2 Contact Discharge±3kV IEC 61000-4-2 Air Gap Discharge, 330pFcapacitor connected between GNDA andGNDB±10ESD Protection (AllOther Pins)Human body model±2kV Thermal ShutdownThresholdTemperature rising+160°C Thermal ShutdownHysteresis13°CElectrical Characteristics - Switching(V DDA=1.71V to5.5V,V DDB=4.5V to5.5V,T A=-40°C to+125°C,STB or I.C.=GNDB.Typical values are at T A=+25°C with GNDA = GNDB, V DDA= 3.3V, V DDB= 5V, STB = GNDB.)PARAMETER SYMBOL CONDITIONS MIN TYP MAX UNITSDifferential Driver Output Rise Time t RR L= 60Ω, C L= 100pF, R CM isopen, Figure 120nsElectrical Characteristics - Switching (continued)(V DDA=1.71V to5.5V,V DDB=4.5V to5.5V,T A=-40°C to+125°C,STB or I.C.=GNDB.Typical values are at T A=+25°C with GNDA = GNDB, V DDA= 3.3V, V DDB= 5V, STB = GNDB.)PARAMETER SYMBOL CONDITIONS MIN TYP MAX UNITSDifferential Driver Output Fall Time t FR L= 60Ω, C L= 100pF, R CM isopen, Figure 133nsTXD to RXD Loop Delay t LOOP R L= 60Ω, C L= 100pF,C RXD= 15pF, Dominant to recessive andrecessive to dominant. Figure 2210nsTXD Propagation Delay t PDTXD_RDR L= 60Ω,C L= 100pF,R CM open,Figure 1Recessive toDominant95ns t PDTXD_DRR L= 60Ω,C L= 100pF,R CM open,Figure 2Dominant toRecessive95RXD Propagation Delay t PDRXD_RDC L= 15pF,Figure 3Recessive toDominant115ns t PDRXD_DRC L= 15pF,Figure 4Dominant toRecessive115TXD Dominant Timeout t DOM(Note 4) 1.4 4.8msUndervoltage Detection Time to Normal Operation t UV(VDDA),t UV(VDDB)110230μsWake-up Time to Dominant State t WAKEMAX14879/MAX14880 only, Instandby mode (V STB= V DDB), Figure 40.55μsStandby Propagation Delay MAX14879/MAX14880 only, RXD,Dominant to recessive, V STB= V DDB,C L= 15pF285500nsStandby to NormalMode Delayt EN MAX14879/MAX14880 only40μsNormal to Standby Dominant Mode Delay MAX14879/MAX14880 only,(V CANH- V CANL) > 1.2V65μsElectrical Characteristics–Package Insulation and Safety Related Specifications: W 16-SOIC(V DDA=1.71V to5.5V,V DDB=4.5V to5.5V,T A=-40°C to+125°C,STB or I.C.=GNDB.Typical values are at T A=+25°C with GNDA = GNDB, V DDA= 3.3V, V DDB= 5V, STB = GNDB.) (Note 5)PARAMETER SYMBOL CONDITIONS MIN TYP MAX UNITS Insulation Resistance RIO T A= 25°C, V IO= 500V>1012ΩBarrier Capacitance C IO GNDA to GNDB2pF Minimum CreepageDistanceCPG8mm Minimum ClearanceDistanceCLR8mm Internal Clearance Distance through insulation0.015mmElectrical Characteristics–Package Insulation and Safety Related Specifications: W 16-SOIC (continued)(V DDA=1.71V to5.5V,V DDB=4.5V to5.5V,T A=-40°C to+125°C,STB or I.C.=GNDB.Typical values are at T A=+25°C with GNDA = GNDB, V DDA= 3.3V, V DDB= 5V, STB = GNDB.) (Note 5)PARAMETER SYMBOL CONDITIONS MIN TYP MAX UNITS Comparative TrackingIndexCTI550Electrical Characteristics–Package Insulation and Safety Related Specifications: W 8-SOIC(V DDA-V GNDA=1.71V to5.5V,V DDB-V GNDB=1.71V to5.5V,C L=15pF,T A=-40°C to+125°C,unless otherwise noted.Typical values are at V DDA- V GNDA= 3.3V, V DDB- V GNDB= 3.3V, GNDA = GNDB, T A= 25°C, unless otherwise noted.) (Notes 2,3) PARAMETER SYMBOL CONDITIONS MIN TYP MAX UNITS Insulation Resistance RIO T A= 25°C, V IO= 500V> 1012ΩBarrier Capacitance C IO GNDA to GNDB2pFMinimum Creepage Distance CPGMAX14878 5.5mm MAX14878W8Minimum Clearance Distance CLRMAX14878 5.5mm MAX14878W8Internal Clearance Distance through insulation0.015mm Comparative TrackingIndexCTI>400Electrical Characteristics–Insulation Characteristics (As Defined by VDE 0884-10): W 16-SOIC(V DDA=1.71V to5.5V,V DDB=4.5V to5.5V,T A=-40°C to+125°C,STB or I.C.=GNDB.Typical values are at T A=+25°C with GNDA = GNDB, V DDA= 3.3V, V DDB= 5V, STB = GNDB.) (Note 5)PARAMETER SYMBOL CONDITIONS MIN TYP MAX UNITSPartial Discharge V PR Method B1 =V IORM x 1.875(t = 1s, partialdischarge < 5pC)MAX148791182V PMAX14878/MAX148802250Maximum Repetitive Peak Voltage V IORMMAX14879630V P MAX14878/MAX148801200Maximum Working Voltage V IOWMGNDA to GNDBcontinuousMAX14879445V RMSMAX14878/MAX14880848Maximum Transient Overvoltage V IOTMMAX148794600V P MAX14878/MAX148808400Isolation Voltage V ISO GNDA to GNDB for60sMAX14879 2.75kV RMSMAX14878/MAX148805Electrical Characteristics–Insulation Characteristics (As Defined by VDE 0884-10): W 16-SOIC (continued)(V DDA=1.71V to5.5V,V DDB=4.5V to5.5V,T A=-40°C to+125°C,STB or I.C.=GNDB.Typical values are at T A=+25°C with GNDA = GNDB, V DDA= 3.3V, V DDB= 5V, STB = GNDB.) (Note 5)PARAMETER SYMBOL CONDITIONS MIN TYP MAX UNITS Maximum SurgeIsolation VoltageV IOSM IEC 61000-4-5, Basic insulation10kV Barrier Resistance R S T A= +150°C, V IO= 500V>109ΩClimate Category 40/125/21Pollution Degree DIN VDE 0110, Table 12Electrical Characteristics–Insulation Characteristics: W 8-SOIC(V DDA=1.71V to5.5V,V DDB=4.5V to5.5V,T A=-40°C to+125°C,STB or I.C.=GNDB.Typical values are at T A=+25°C with GNDA = GNDB, V DDA= 3.3V, V DDB= 5V, STB = GNDB.) (Note 5)PARAMETER SYMBOL CONDITIONS MIN TYP MAX UNITSMaximum Repetitive Peak Voltage V IORMMAX14878630V P MAX14878W1200Maximum Working Voltage V IOWMGNDA to GNDBcontinuousMAX14878445V RMSMAX14878W848Maximum Transient Overvoltage V IOTMMAX148785000V P MAX14878W8400Isolation Voltage V ISO GNDA to GNDB for60sMAX14878 3.5kV RMSMAX14878W5Maximum SurgeIsolation VoltageV IOSM IEC 61000-4-5, Basic insulation10kV Barrier Resistance R S T A= +150°C, V IO= 500V>109ΩClimate Category 40/125/21Pollution Degree2Note 1:All devices 100% production tested at T A= +25°C. Specifications over temperature are guaranteed by design.Note 2:All currents into the device are positive.All currents out of the device are negative.All voltages referenced to their respective ground (GNDA or GNDB), unless otherwise noted.Note 3:Not production tested. Guaranteed at T A= +25°C.Note 4:The dominant timeout feature releases the bus when TX is held low longer than t DO.CAN protocol guarantees a maximum of11successive dominant bits in any transmission.The minimum data rate allowed by the dominant timeout,then,is11/ t DO(min).Note 5:All16-pin package devices are100%production tested for high voltage conditions(this does not apply to the8-pin MAX14878AWA).Typical Operating Characteristics(V DDA= 3.3V, V DDB= 5V, 60Ω load between CANH and CANL, T A= +25°C, unless otherwise noted.)Typical Operating Characteristics (continued)(V DDA= 3.3V, V DDB= 5V, 60Ω load between CANH and CANL, T A= +25°C, unless otherwise noted.)Pin DescriptionPINNAME FUNCTIONREFSUPPLYTYPEMAX14878 16-PIN MAX148788-PINMAX14879,MAX14880CONTROLLER SIDE (A-SIDE)131V DDA Power Supply Input for theController Side/A-Side. BypassV DDA to GNDA with 0.1μFcapacitor as close to the deviceas possible.V DDA Power2, 842, 8GNDA Controller Side/A-Side Ground V DDA Ground315RXD Receiver Output. RXD is highwhen the bus is in the recessivestate. RXD is low when the bus isin the dominant state.V DDA Digital Output4, 5, 7-4, 6, 7N.C.No Connection. Not internally connected. Connect to GNDA, V DDA, or leave unconnected.623TXD Transmit Data Input. CANH andCANL are in the dominant statewhen TXD is low. CANH andCANL are in the recessive statewhen TXD is high.V DDA Digital InputCAN BUS SIDE (B-SIDE)9, 1559, 15GNDB CAN Bus Side/B-Side Ground V DDB Ground10, 14-10I.C.Internally Connected. Connect to GNDB or leave unconnected.11-11I.C Internally Connected. Connect to GNDB, V DDB, or leave unconnected.12612CANL Low-Level CAN Differential BusLineV DDBDifferentialI/O13713CANH High-Level CAN Differential BusLineV DDBDifferentialI/OPin Description (continued)PINNAME FUNCTIONREFSUPPLYTYPEMAX14878 16-PIN MAX148788-PINMAX14879,MAX14880--14STB Standby Input, Active High. DriveSTB high to disable the CAN busdriver and place the transceiverin low-power standby mode.Drive STB low for normaloperation.V DDB Digital Input16816V DDB Power Supply Input for the CANBus Side/B-Side. Bypass V DDBto GNDB with a 0.1μF capacitoras close to the device aspossible.V DDB PowerDetailed DescriptionThe MAX14878–MAX14880isolated controller area network(CAN)transceivers provide2750V RMS or5000V RMS(60s) of galvanic isolation between the cable side(B-side)of the transceiver and the controller side(A-side).These devices allow up to1Mbps communication across an isolation barrier when a large potential exists between grounds on each side of the barrier.CANH and CANL outputs are short-circuit current limited and are protected against excessive power dissipation by thermal shutdown circuitry that places the driver outputs in a high-impedance state.IsolationData isolation is achieved using integrated capacitive isolation that allows data transmission between the controller side and cable side of the transceiver.Fault ProtectionThe MAX14878–MAX14880feature±54V fault protection on the CANH and CANL bus lines.When CANH or CANL is pulled above+30V(typ)or below-30V(typ),the I/O is set to high impedance.This wide fault protection range simplifies selecting external TVS components for surge protection.TransmitterThe transmitter converts a single-ended input signal(TXD)from the CAN controller to differential outputs for the bus lines (CANH, CANL). The truth table for the transmitter and receiver is given in Table 1.Transmitter Dominant TimeoutThe MAX14878–MAX14880feature a transmitter-dominant timeout(t DOM)that prevents erroneous CAN controllers from clamping the bus to a dominant level by maintaining a continuous low TXD signal.When TXD remains in the dominant state (low) for greater than t DOM, the transmitter is disabled, releasing the bus to a recessive state (Table 1).After a dominant timeout fault, normal transmitter function is re-enabled on the rising edge of a TXD.The transmitter-dominant timeout limits the minimum possible data rate to 9kbps for standard CAN protocol.Driver Output ProtectionThe MAX14878–MAX14880feature integrated circuitry to protect the transmitter output stage against a short-circuit to a positive or negative voltage by limiting the driver current.The transmitter returns to normal operation once the short is removed.Thermal shutdown further protects the transceiver from excessive temperatures that may result from a short by setting the transmitter outputs to high impedance when the junction temperature exceeds+160°C(typ).The transmitter returns to normal operation when the junction temperature falls below the thermal shutdown hysteresis.ReceiverThe receiver reads the differential input from the bus(CANH,CANL)and transfers this data as a single-ended output (RXD)to the CAN controller.During normal operation,a comparator senses the difference between CANH and CANL, V DIFF=(V CANH-V CANL),with respect to an internal threshold of0.7V(typ).If V DIFF>0.9V,a logic-low is present on RXD. If V DIFF< 0.5V, a logic-high is present.The CANH and CANL common-mode range is±25V.RXD is logic-high when CANH and CANL are shorted or terminated and undriven.Thermal ShutdownIf the junction temperature exceeds+160°C(typ),the device is switched off.During thermal shutdown,CANH and CANL are high-impedance and all IC functions are disabled.The transmitter outputs are re-enabled and the device resumes normal operation when the junction temperature drops below 147°C (typ).Table 1. Transmitter and Receiver Truth Table When Not Connected to the Bus TXD TXD LOW TIME CANH CANL BUS STATE RXD LOW< t DOM HIGH LOW DOMINANT LOW LOW> t DOM V DDB/2V DDB/2RECESSIVE HIGH HIGH X V DDB/2V DDB/2RECESSIVE HIGHApplications InformationReduced EMI and ReflectionsIn multidrop CAN applications,it is important to maintain a single linear bus of uniform impedance that is properly terminated at each end. A star configuration should never be used.Any deviation from the end-to-end wiring scheme creates a stub.High-speed data edges on a stub can create reflections back down the bus.These reflections can cause data errors by eroding the noise margin of the system.Although stubs are unavoidable in a multidrop system,care should be taken to keep these stubs as short as possible,especially when operating with high data rates.Typical Operating CircuitOrdering InformationPART NUMBER ISOLATION VOLTAGE (kV RMS)STANDBY OPERATING TEMPERATURE PACKAGE MAX14878AWA+ 3.5NO-40°C to +125°C W 8-SOIC MAX14878AWA+T 3.5NO-40°C to +125°C W 8-SOIC MAX14878AWE+5NO-40°C to +125°C W 16-SOIC MAX14878AWE+T5NO-40°C to +125°C W 16-SOIC MAX14878WAWA+5NO-40°C to +125°C W 8-SOIC MAX14878WAWA+T5NO-40°C to +125°C W 8-SOIC MAX14879AWE+ 2.75YES-40°C to +125°C W 16-SOIC MAX14879AWE+T 2.75YES-40°C to +125°C W 16-SOIC MAX14880AWE+5YES-40°C to +125°C W 16-SOIC MAX14880AWE+T5YES-40°C to +125°C W 16-SOICRevision HistoryREVISION NUMBER REVISIONDATEDESCRIPTIONPAGESCHANGED06/17Initial release—18/17Updated parameters in Electrical Characteristics table and added Typical Operating Circuit7, 14 210/17Corrected the Pin Description section for internally connected pins; updated Figure 29, 12 33/18Updated the Safety Regulatory Approvals section1 46/18Updated Pin Description table1253/19Updated the General Description, Benefits and Features, Package Information, Electrical Characteristics, Pin Configuration, and Pin Description to add a Wide 8-Pin SOIC package;added MAX14878AWA+ and MAX14878AWA+T to the Ordering Information table1, 3, 8,12, 1465/19Updated the General Description, Benefits and Features, Safety Regulatory Approvals,Electrical Characteristics–Package Insulation and Safety Related Specifications: W 8-SOIC, Electrical Characteristics–Insulation Characteristics (As Defined by VDE 0884-10): W16-SOIC, Electrical Characteristics–Insulation Characteristics: W 8-SOIC, and OrderingInformation sections1–16709/20Updated the General Description, Absolute Maximum Ratings, Package Information,Electrical Characteristics–Package Insulation and Safety Related Specifications: W 8-SOIC,Electrical Characteristics–Insulation Characteristics: W 8-SOIC, and Ordering Informationsections1, 3, 8-9,19For pricing, delivery, and ordering information, please visit Maxim Integrated’s online storefront at https:///en/storefront/storefront.html. Maxim Integrated cannot assume responsibility for use of any circuitry other than circuitry entirely embodied in a Maxim Integrated product. No circuit patent licenses are implied. Maxim Integrated reserves the right to change the circuitry and specifications without notice at any time. The parametric values (min and max limits) shown in the Electrical Characteristics table are guaranteed. Other parametric values quoted in this data sheet are provided for guidance.。
3GPP TS 25.211 3GPP协议标准 通信
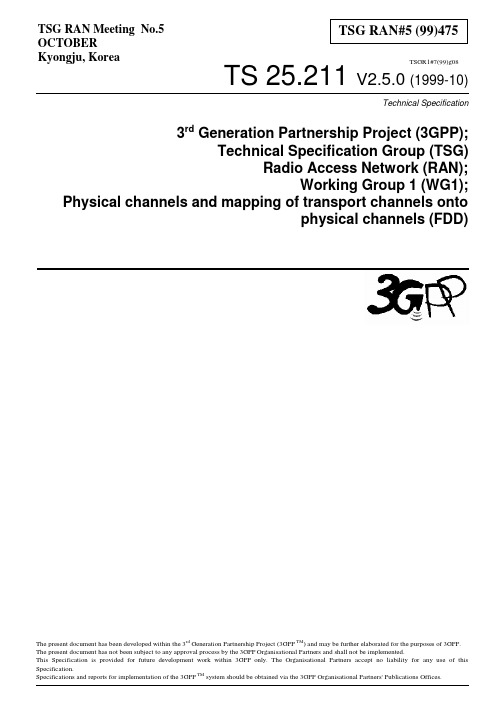
Technical SpecificationTS 25.211 V2.5.0 (1999-10)3rd Generation Partnership Project (3GPP);Technical Specification Group (TSG)Radio Access Network (RAN);Working Group 1 (WG1);Physical channels and mapping of transport channels ontophysical channels (FDD)The present document has been developed within the 3rd Generation Partnership Project (3GPP TM ) and may be further elaborated for the purposes of 3GPP.The present document has not been subject to any approval process by the 3GPP Organisational Partners and shall not be implemented.This Specification is provided for future development work within 3GPP only. The Organisational Partners accept no liability for any use of this Specification.Specifications and reports for implementation of the 3GPP TM system should be obtained via the 3GPP Organisational Partners' Publications Offices.TSGR1#7(99)g08TSG RAN#5 (99)475TSG RAN Meeting No.5OCTOBER Kyongju, KoreaReference<Workitem> (<Shortfilename>.PDF)Keywords<keyword[, keyword]>3GPPPostal addressOffice addressInternetsecretariat@Individual copies of this deliverablecan be downloaded fromCopyright NotificationNo part may be reproduced except as authorized by written permission. The copyright and the foregoing restriction extend to reproduction in all media.©All rights reserved.ContentsContents (4)Intellectual Property Rights (5)Foreword (5)1Scope (5)2References (6)3Definitions, symbols and abbreviations (6)3.1Definitions (6)3.2Symbols (6)3.3Abbreviations (6)4Transport channels (7)4.1Dedicated transport channels (7)4.1.1DCH – Dedicated Channel (7)4.2Common transport channels (7)4.2.1BCH – Broadcast Channel (8)4.2.2FACH – Forward Access Channel (8)4.2.3PCH – Paging Channel (8)4.2.4RACH – Random Access Channel (8)4.2.5CPCH – Common Packet Channel (8)4.2.6DSCH – Downlink Shared Channel (8)5Physical channels (8)5.1The physical resource (8)5.2Uplink physical channels (9)5.2.1Dedicated uplink physical channels (9)5.2.2Common uplink physical channels (12)5.2.2.1Physical Random Access Channel (PRACH) (12)5.2.2.1.1RACH transmission (12)5.2.2.1.2RACH preamble part (12)5.2.2.1.3RACH message part (13)5.2.2.2Physical Common Packet Channel (PCPCH) (14)5.2.2.2.1CPCH transmission (14)5.2.2.2.2CPCH access preamble part (14)5.2.2.2.3CPCH collision detection preamble part (15)5.2.2.2.4CPCH power control preamble part (15)5.2.2.2.5CPCH message part (15)5.3Downlink physical channels (15)5.3.1Downlink Transmit Diversity (15)5.3.1.1Open loop transmit diversity (16)5.3.1.1.1Space time block coding based transmit antenna diversity (STTD) (16)5.3.1.1.2Time Switched Transmit Diversity for SCH (TSTD) (17)5.3.2Dedicated downlink physical channels (17)5.3.2.1STTD for DPCH (21)5.3.2.2Dedicated channel pilots with closed loop mode transmit diversity (22)5.3.2.3DL-DPCCH for CPCH (22)5.3.3Common downlink physical channels (22)5.3.3.1Common Pilot Channel (CPICH) (22)5.3.3.1.1Primary Common Pilot Channel (23)5.3.3.1.2Secondary Common Pilot Channel (23)5.3.3.2Primary Common Control Physical Channel (P-CCPCH) (24)5.3.3.2.1Primary CCPCH structure with STTD encoding (24)5.3.3.3Secondary Common Control Physical Channel (S-CCPCH) (25)5.3.3.3.1Secondary CCPCH structure with STTD encoding (27)5.3.3.4Synchronisation Channel (SCH) (28)5.3.3.4.1SCH transmitted by TSTD (28)5.3.3.5Physical Downlink Shared Channel (PDSCH) (29)5.3.3.6Acquisition Indication Channel (AICH) (30)5.3.3.7Page Indication Channel (PICH) (30)6Mapping of transport channels onto physical channels (32)7Timing relationship between physical channels (32)7.1General (32)7.2PICH/S-CCPCH timing relation (33)7.3PRACH/AICH timing relation (34)7.4PCPCH/AICH timing relation (35)7.5DPCH/PDSCH timing (36)7.6DPCCH/DPDCH timing relations (36)7.6.1Uplink (36)7.6.2Downlink (36)7.6.3Uplink/downlink timing at UE (36)History (37)Intellectual Property Rights<IPR notice shall be provided once correct notice is available within 3GPP>ForewordThis Technical Specification has been produced by the 3GPP.The contents of the present document are subject to continuing work within the TSG and may change following formal TSG approval. Should the TSG modify the contents of this TS, it will be re-released by the TSG with an identifying change of release date and an increase in version number as follows:Version 3.y.zwhere:x the first digit:1presented to TSG for information;2presented to TSG for approval;3Indicates TSG approved document under change control.y the second digit is incremented for all changes of substance, i.e. technical enhancements, corrections, updates, etc.z the third digit is incremented when editorial only changes have been incorporated in the specification;1 ScopeThis specification describes the characteristics of the Layer 1 transport channels and physicals channels in the FDD mode of UTRA. The main objectives of the document are to be a part of the full description of the UTRA Layer 1, and to serve as a basis for the drafting of the actual technical specification (TS).2 ReferencesThe following documents contain provisions which, through reference in this text, constitute provisions of the present document.[1]TS 25.201: “Physical layer - general description”[2]TS 25.211: “Physical channels and mapping of transport channels onto physical channels (FDD)”[3]TS 25.212: “Multiplexing and channel coding (FDD)”[4]TS 25.213: “Spreading and modulation (FDD)”[5]TS 25.214: “Physical layer procedures (FDD)”[6]TS 25.221: “Transport channels and physical channels (TDD)”[7]TS 25.222: “Multiplexing and channel coding (TDD)”[8]TS 25.223: “Spreading and modulation (TDD)”[9]TS 25.224: “Physical layer procedures (TDD)”[10]TS 25.231: “Measurements”[11]TS 25.301: “Radio Interface Protocol Architecture”[12]TS 25.302: “Services Provided by the Physical Layer”[13]TS 25.401: “UTRAN Overall Description”3 Definitions, symbols and abbreviations3.1 DefinitionsFor the purposes of the present document, the [following] terms and definitions [given in ... and the following] apply. <defined term>: <definition>.example: text used to clarify abstract rules by applying them literally.3.2 SymbolsFor the purposes of the present document, the following symbols apply:<symbol><Explanation>3.3 AbbreviationsFor the purposes of the present document, the following abbreviations apply:<ACRONYM><Explanation>A I Acquisition IndicatiorA ICH Acquisition Indication ChannelA P Access PreambleB CH Broadcast ChannelC CPCH Common Control Physical ChannelC CTrCH Coded Composite Transport ChannelC D Collision DetectionC PCH Common Packet ChannelC PICH Common Pilot ChannelD CH Dedicated ChannelD PCCH Dedicated Physical Control ChannelD PCH Dedicated Physical ChannelD PDCH Dedicated Physical Data ChannelD SCH Downlink Shared ChannelF ACH Forward Access ChannelF BI Feedback InformationM UI Mobile User IdentifierP CH Paging ChannelP-CCPCH Primary Common Control Physical ChannelP CPCH Physical Common Packet ChannelP DSCH Physical Downlink Shared ChannelP I Page IndicatiorP ICH Page Indication ChannelP RACH Physical Random Access ChannelP SC Primary Synchronisation CodeR ACH Random Access ChannelR NC Radio Network ControllerS-CCPCH Secondary Common Control Physical ChannelS CH Synchronisation ChannelS F Spreading FactorS FN System Frame NumberS SC Secondary Synchronisation CodeS TTD Space Time Transmit DiversityT FCI Transport Format Combination IndicatorT STD Time Switched Transmit DiversityT PC Transmit Power ControlU E User EquipmentU TRAN UMTS Terrestrial Radio Access Networkchannels4 TransportT ransport channels are the services offered by Layer 1 to the higher layers. General concepts about transport channels are described in [12].A transport channel is defined by how and with what characteristics data is transferred over the air interface. A general classification of transport channels is into two groups:•Dedicated Channels•Common Channels4.1 Dedicated transport channelsT here exists only one type of dedicated transport channel, the Dedicated Channel (DCH).4.1.1 DCH – Dedicated ChannelT he Dedicated Channel (DCH) is a downlink or uplink transport channel. The DCH is transmitted over the entire cell or over only a part of the cell using beam-forming antennas. The Dedicated Channel (DCH) is characterized by the possibility of fast rate change (every 10ms), fast power control and inherent addressing of UEs.4.2 Common transport channelsThere are six types of common transport channels: BCH, FACH, PCH, RACH, CPCH and DSCH.4.2.1 BCH – Broadcast ChannelT he Broadcast Channel (BCH) is a downlink transport channel that is used to broadcast system- and cell-specific information. The BCH is always transmitted over the entire cell with a low fixed bit rate.4.2.2 FACH – Forward Access ChannelT he Forward Access Channel (FACH) is a downlink transport channel. The FACH is transmitted over the entire cell or over only a part of the cell using beam-forming antennas. The FACH uses slow power control.4.2.3 PCH – Paging ChannelT he Paging Channel (PCH) is a downlink transport channel. The PCH is always transmitted over the entire cell. The transmission of the PCH is associated with the transmission of a physical layer signal, the Paging Indicator, to support efficient sleep-mode procedures.4.2.4 RACH – Random Access ChannelT he Random Access Channel (RACH) is an uplink transport channel. The RACH is always received from the entire cell. The RACH is characterized by a limited size data field, a collision risk and by the use of open loop power control.4.2.5 CPCH – Common Packet ChannelT he Common Packet Channel (CPCH) is an uplink transport channel. The CPCH is a contention based random access channel used for transmission of bursty data traffic. CPCH is associated with a dedicated channel on the downlink which provides power control for the uplink CPCH.4.2.6 DSCH – Downlink Shared ChannelThe downlink shared channel (DSCH) is a downlink transport channel shared by several UEs The DSCH is associated with a DCH.channels5 PhysicalP hysical channels typically consist of a three-layer structure of superframes, radio frames, and time slots, although this is not true for all physical channels. Depending on the symbol rate of the physical channel, the configuration of radio frames or time slots varies.-Superframe: A Superframe has a duration of 720ms and consists of 72 radio frames. The superframe boundaries are defined by the System Frame Number (SFN):-The head radio frame of superframe :SFN mod 72=0.-The tail radio frame of superframe:SFN mod 72=71.-Radio frame: A Radio frame is a processing unit which consists of 15 time slots.-Time slot: A Time slot is a unit which consists of fields containing bits. The number of bits per time slot depends on the physical channel.5.1 The physical resourceT he basic physical resource is the code/frequency plane. In addition, on the uplink, different information streams may be transmitted on the I and Q branch. Consequently, a physical channel corresponds to a specific carrier frequency, code, and, on the uplink, relative phase (0 or π/2).5.2 Uplink physical channels5.2.1Dedicated uplink physical channelsT here are two types of uplink dedicated physical channels, the uplink Dedicated Physical Data Channel (uplink DPDCH) and the uplink Dedicated Physical Control Channel (uplink DPCCH).T he DPDCH and the DPCCH are I/Q code multiplexed within each radio frame (see [4]).T he uplink DPDCH is used to carry dedicated data generated at Layer 2 and above, i.e. the dedicated transport channel (DCH). There may be zero, one, or several uplink DPDCHs on each Layer 1 connection.T he uplink DPCCH is used to carry control information generated at Layer 1. The Layer 1 control information consists of known pilot bits to support channel estimation for coherent detection, transmit power-control (TPC) commands,feedback information (FBI), and an optional transport-format combination indicator (TFCI). The transport-format combination indicator informs the receiver about the instantaneous parameters of the different transport channels multiplexed on the uplink DPDCH, and corresponds to the data transmitted in the same frame. It is the UTRAN that determines if a TFCI should be transmitted, hence making it is mandatory for all UEs to support the use of TFCI in the uplink. There is one and only one uplink DPCCH on each Layer 1 connection.F igure 1 shows the frame structure of the uplink dedicated physical channels. Each frame of length 10 ms is split into 15slots, each of length T slot = 2560 chips, corresponding to one power-control period. A super frame corresponds to 72consecutive frames, i.e. the super-frame length is 720 ms.Figure 1: Frame structure for uplink DPDCH/DPCCH.The parameter k in Figure 1 determines the number of bits per uplink DPDCH/DPCCH slot. It is related to the spreading factor SF of the physical channel as SF = 256/2k . The DPDCH spreading factor may thus range from 256 down to 4.Note that an uplink DPDCH and uplink DPCCH on the same Layer 1 connection generally are of different rates, i.e.have different spreading factors and different values of k.The exact number of bits of the different uplink DPCCH fields (N pilot , N TFCI , N FBI , and N TPC ) is determined in Table 2.The field order and total number of bits/slot are fixed, though the number of bits per field may vary during a connection.The values for the number of bits per field are given in Table 1 and Table 2. The channel bit and symbol rates given in Table 1 are the rates immediately before spreading. The pilot patterns are given in Table 3 and Table 4, the TPC bit pattern is given in Table 5.Pilot N pilot bitsTPC N TPC bitsData N data bits Slot #0Slot #1Slot #i Slot #14Frame #0Frame #1Frame #i Frame #71T slot = 2560 chips, 10*2k bits (k=0..6)T f = 10 msT super = 720 msDPDCH DPCCHFBI N FBI bitsTFCI N TFCI bitsThe N FBI bits are used to support techniques requiring feedback between the UE and the UTRAN Access Point (=cell transceiver), including closed loop mode transmit diversity and site selection diversity (SSDT). The exact details of the FBI field are shown in Figure 2 and described below.S field D fieldN FBIFigure 2: Details of FBI field.The S field is used for SSDT signalling, while the D field is used for FB Mode Transmit Diversity Signalling. Each of the S and D fields can be length 0, 1 or 2, with a total FBI field size N FBI according to Table 2 (DPCCH fields). Simultaneous use of SSDT power control and FB Mode Transmit Diversity requires that both the S and D fields be of length 1. The use of these FBI fields is described in [5].Table 1: DPDCH fields.Slot Format#i Channel BitRate (kbps)Channel SymbolRate (ksps)SF Bits/FrameBits/SlotN data01515256150101013030128300202026060646004040312012032120080804240240162400160160548048084800320320696096049600640640There are two types of Uplink Dedicated Physical Channels; those that include TFCI(e.g. for several simultaneous services) and those that do not include TFCI(e.g. for fixed-rate services). These types are reflected by the duplicated rows of Table 2. The channel bit and symbol rates given in Table 2 are the rates immediately before spreading.Table 2: DPCCH fields.Slot Format#i Channel BitRate (kbps)Channel SymbolRate (ksps)SF Bits/FrameBits/SlotN pilot N TFCI N FBI N TPC01515256150106202 11515256150108002 21515256150105212 31515256150107012 41515256150106022 51515256150105221The pilot bit pattern is described in Table 3 and Table 4. The shadowed part can be used as frame synchronization words. (The value of the pilot bit other than the frame synchronization word shall be “1”.)Table 3: Pilot bit patterns for uplink DPCCH with N pilot = 5 and 6.N pilot = 5N pilot = 6Bit #01234012345Slot #0123456789 10 11 12 13 14111111111111111111111111111111111111111111111111111111111111111111111111111111111111111111111111111111111 Table 4: Pilot bit patterns for uplink DPCCH with N pilot = 7 and 8.N pilot = 7N pilot = 8Bit #012345601234567Slot #0123456789 10 11 12 13 14111111111111111111111111111111111111111111111111111111111111111111111111111111111111111111111111111111111111111111111111111111111111111111111111111111111111111111111The relationship between the TPC bit pattern and transmitter power control command is presented in Table 5.Table 5: TPC Bit Pattern.TPC Bit PatternN TPC = 1N TPC = 2Transmitter power control command1 011001For slot formats using TFCI, the TFCI value in each radio frame corresponds to a certain combination of bit rates of the DCHs currently in use. This correspondence is (re-)negotiated at each DCH addition/removal. The mapping of the TFCI bits onto slots is described in [3].Multi-code operation is possible for the uplink dedicated physical channels. When multi-code transmission is used,several parallel DPDCH are transmitted using different channelization codes, see [4]. However, there is only one DPCCH per connection.5.2.2Common uplink physical channels5.2.2.1Physical Random Access Channel (PRACH)The Physical Random Access Channel (PRACH) is used to carry the RACH.5.2.2.1.1 RACH transmissionThe random-access transmission is based on a Slotted ALOHA approach with fast acquisition indication. The UE can start the transmission at a number of well-defined time-offsets, denoted access slots. There are 15 access slots per two frames and they are spaced 5120 chips apart. Timing information on the access slots and the acquisition indication is given in section 7.3. Figure 3 shows the access slot numbers and their spacing to each other. Information on what access slots are available in the current cell is given by higher layers.#0#1#2#3#4#5#6#7#8#9#10#11#12#13#145120 chips radio frame: 10 msradio frame: 10 msAccess slot #0Random Access TransmissionAccess slot #1Access slot #7Access slot #14Random Access TransmissionRandom Access TransmissionRandom Access TransmissionAccess slot #8Figure 3: RACH access slot numbers and their spacing.The structure of the random-access transmission is shown in Figure 4. The random-access transmission consists of one or several preambles of length 4096 chips and a message of length 10 ms.Message partPreamble 4096 chips10 msPreamblePreambleFigure 4: Structure of the random-access transmission.5.2.2.1.2 RACH preamble partThe preamble part of the random-access burst consists of 256 repetitions of a signature. There are a total of 16 different signatures, based on the Hadamard code set of length 16 (see [4] for more details).5.2.2.1.3 RACH message partFigure 5 shows the structure of the Random-access message part. The 10 ms message is split into 15 slots, each of length T slot = 2560 chips. Each slot consists of two parts, a data part that carries Layer 2 information and a control part that carries Layer 1 control information. The data and control parts are transmitted in parallel.The data part consists of 10*2k bits, where k=0,1,2,3. This corresponds to a spreading factor of 256, 128, 64, and 32respectively for the message data part.The control part consists of 8 known pilot bits to support channel estimation for coherent detection and 2 TFCI bits.This corresponds to a spreading factor of 256 for the message control part. The pilot bit pattern is described in Table 8.The total number of TFCI bits in the random-access message is 15*2 = 30. The TFCI value corresponds to a certain transport format of the current Random-access message.Pilot N pilot bitsData N data bitsSlot #0Slot #1Slot #iSlot #14T slot = 2560 chips, 10*2k bits (k=0..3)Random-access messageT RACH = 10 msData ControlTFCI N TFCI bitsFigure 5: Structure of the random-access message part.Table 6: Random-access message data fields.Slot Format#iChannel Bit Rate (kbps)Channel Symbol Rate (ksps)SF Bits/FrameBits/Slot N data0151525615010101303012830020202606064600404031201203212008080Table 7: Random-access message control fields.Slot Format#iChannel Bit Rate (kbps)Channel Symbol Rate (ksps)SF Bits/FrameBits/Slot N pilotN TFCI15152561501082Table 8: Pilot bit patterns for RACH message part with N pilot = 8.N pilot = 8Bit #01234567Slot #01234567891011121314111111*********100011110101100111111*********101001101110000111111*********110001*********111111*********0010100001110115.2.2.2 Physical Common Packet Channel (PCPCH)The Physical Common Packet Channel (PCPCH) is used to carry the CPCH.5.2.2.2.1 CPCH transmissionThe CPCH transmission is based on DSMA-CD approach with fast acquisition indication. The UE can start transmission at a number of well-defined time-offsets, relative to the frame boundary of the received BCH of the current cell. The access slot timing and structure is identical to RACH in section 5.2.2.1.1. The structure of the CPCH random access transmission is shown in Figure 6. The CPCH random access transmission consists of one or several Access Preambles [A-P] of length 4096 chips, one Collision Detection Preamble (CD-P) of length 4096 chips, a [10] ms DPCCH Power Control Preamble (PC-P) and a message of variable length Nx10 ms.4096 chipsP 0P 1P jP jCollision Resolution PreambleAccess Preamble DPCCH DPDCH[10] msecN*10 msecMessage PartFigure 6: Structure of the CPCH random access transmission.5.2.2.2.2 CPCH access preamble partSimilar to 5.2.2.1.2 (RACH preamble part). The RACH preamble signature sequences are used. The number ofsequences used could be less than the ones used in the RACH preamble. The scrambling code could either be chosen to be a different code segment of the Gold code used to form the scrambling code of the RACH preambles (see [4] for more details) or could be the same scrambling code in case the signature set is shared.5.2.2.2.3 CPCH collision detection preamble partSimilar to 5.2.2.1.2 (RACH preamble part). The RACH preamble signature sequences are used. The scrambling code is chosen to be a different code segment of the Gold code used to form the scrambling code for the RACH and CPCH preambles (see [4] for more details).5.2.2.2.4 CPCH power control preamble partThe power control preamble segment is a [10] ms DPCCH Power Control Preamble (PC-P). The following Table 9 is identical to Rows 2 and 4 of Table 2 in 5.2.1. Table 9 defines the DPCCH fields which only include Pilot, FBI and TPC bits. The Power Control Preamble length is ffs.Table 9: DPCCH fields for CPCH power control preamble segment.Slot Format#i Channel BitRate (kbps)Channel SymbolRate (ksps)SF Bits/FrameBits/SlotN pilot N TFCI N FBI N TPC01515256150108002115152561501070125.2.2.2.5 CPCH message partFigure 1 in 5.2.1 shows the structure of the CPCH message part. Each message consists of up to N_Max_frames 10 ms frames. N_Max_frames is a higher layer parameter. Each 10 ms frame is split into 15 slots, each of length T slot = 2560 chips. Each slot consists of two parts, a data part that carries higher layer information and a control part that carries Layer 1 control information. The data and control parts are transmitted in parallel.The data part consists of 10*2k bits, where k = 0, 1, 2, 3, 4, 5, 6, corresponding to spreading factors of 256, 128, 64, 32, 16, 8, 4 respectively. Note that various rates might be mapped to different signature sequences.The spreading factor for the UL-DPCCH (message control part ) is 256. The entries in Table 1 corresponding to spreading factors of 256 and below and Table 2 [both in section 5.2.1] apply to the DPDCH and DPCCH fields respectively for the CPCH message part.5.3 Downlink physical channels5.3.1 Downlink Transmit DiversityTable 10 summarizes the possible application of open and closed loop Transmit diversity modes on different downlink physical channels.Table 710: Application of Tx diversity modes on downlink physical channels.Channel Open loop modeClosed loop modeCPICH X N/A P-CCPCH X N/A SCH X N/A S-CCPCH X N/A DPCH X X PICHX N/A PDSCH (associated with DPCH)X X AICHXN/AN/A = Not applied X = Can be applied5.3.1.1Open loop transmit diversity5.3.1.1.1Space time block coding based transmit antenna diversity (STTD)Tx.Antenna 2M U XData Tx.Antenna 1Channelization code andlong scrambling code C ,spreading length =M Ant1Ant2Ant1Ant2TPC TFIPilotM U XChannelEncoderInterleaverSTTD EncoderRate MatchingQPSK symbolsDiversity PilotThe open loop downlink transmit diversity employs a space time block coding based transmit diversity (STTD). The STTD encoding is optional in UTRAN. STTD support is mandatory at the UE. Ablock diagram of the transmitter and a generic STTD encoder are shown in the Figure 6Figure 7 and Figure 8Figure 8 below. Channel coding, rate matching and interleaving is done as in the non-diversity mode.Figure 67: Block diagram of the transmitter (STTD).S 1S 2STTD encoderS 1S 2-S 2*S 1*T 2TT 2TAnt 1Ant 2Mobile AntennaPath 1Path jN dataFigure 8: Block diagram of STTD encoder. The symbols S 1, S 2 are QPSK or discontinuoustransmission (DTX) symbols and T denotes the symbol time.5.3.1.1.2 Time Switched Transmit Diversity for SCH (TSTD)TSTD is optional in UTRAN. TSTD support is mandatory at the UE. A block diagram of the transmitter using TSTD for SCH and STTD for P-CCPCH is shown in Figure 9.TSWSW0&1slotBCHSW0Control signal for SW0&1SW1Control signal for TSW S-SCHP-SCHTSWAnt1Ant2channelisation and scrambling codeSTTD EncoderInter-leaver Channel Encoder G S-SCHG P-SCHSTTD schemeTSTD schemeG P-SCH: Gain factor of Primary SCH G S-SCH: Gain factor of Secondary SCHFigure 9: Multiplexing scheme of SCH (TSTD) and P-CCPCH (STTD).5.3.2 Dedicated downlink physical channelsThere is only one type of downlink dedicated physical channel, the Downlink Dedicated Physical Channel (downlink DPCH).Within one downlink DPCH, dedicated data generated at Layer 2 and above, i.e. the dedicated transport channel (DCH),is transmitted in time-multiplex with control information generated at Layer 1 (known pilot bits, TPC commands, and an optional TFCI). The downlink DPCH can thus be seen as a time multiplex of a downlink DPDCH and a downlink DPCCH, compare Section 5.2.1. It is the UTRAN that determines if a TFCI should be transmitted, hence making it is mandatory for all UEs to support the use of TFCI in the downlink.Figure 10 shows the frame structure of the downlink DPCH. Each frame of length 10 ms is split into 15 slots, each of length T slot = 2560 chips, corresponding to one power-control period. A super frame corresponds to 72 consecutive frames, i.e. the super-frame length is 720 ms.。
轨迹 原始节点编码

轨迹原始节点编码是指对轨迹数据中的每个节点进行唯一标识的一种编码方式。
在轨迹数据中,节点通常表示位置信息,而轨迹则是通过一系列有序的节点连接而成的。
为了对轨迹数据进行有效的处理和分析,需要一种方式来区分和标识不同的节点。
轨迹原始节点编码可以采用多种方式,具体取决于应用场景和数据特点。
以下是一些常见的轨迹原始节点编码方式:
1.顺序编码:按照节点在轨迹中的顺序进行编号,这是一种简单而直观的编码方式。
由于节点是按
照顺序存储的,因此可以快速访问和遍历整个轨迹数据。
然而,顺序编码对于不同的轨迹来说不具备唯一性,因此不适合用于跨轨迹匹配等应用场景。
2.哈希编码:将节点的相关信息进行哈希计算,从而得到一个唯一的哈希值作为节点编码。
哈希编
码可以保证节点编码的唯一性,适用于跨轨迹匹配等应用场景。
然而,哈希编码可能会存在冲突的情况,即不同的节点计算得到相同的哈希值。
因此,在实际应用中需要注意哈希冲突的问题。
3.空间编码:将节点的空间位置信息(如经度和纬度)进行编码,从而得到唯一的节点编码。
空间
编码方式适合于具有空间位置信息的轨迹数据。
它可以有效地将空间位置信息转换为数字形式,方便计算机处理和分析。
Go汇编语言

Go汇编语⾔Go的汇编器继承⾃Plan9的汇编器,但与Plan9汇编器仍有很多不同之处。
Plan9并不是Go语⾔中特有的东西,⽽是指贝尔实验室中开发的⼀个操作系统。
贝尔实验室九号项⽬(英语:Plan 9 from Bell Labs,常简称为Plan 9)是⼀个分布式操作系统,由贝尔实验室的计算科学研究中⼼在1980年代中期⾄2002年开发,以作为UNIX的后继者。
它现在仍然被操作系统的研究者和爱好者开发使⽤。
Go汇编并不是对底层机器的直接表⽰,相反,它是⼀种半抽象的汇编语⾔,是Go编译器的输出,并作为Go汇编器的输⼊,Go汇编器会将Go汇编表⽰的指令翻译成具体平台的机器码。
所以使⽤Go汇编编写的程序,在编译完成后可能和原来并不相同。
例如Go汇编的MOV指令,在编译完成后可能会变成清除指令或加载指令,再例如,在arm中,为了⽅便编写汇编,可能使⽤⼀条DIV或MOD指令来做算数操作,但实际上其对应的arm指令可能不⽌⼀条。
另外,Go汇编也不是个独⽴的语⾔,⽆法独⽴使⽤,必须结合Go代码。
Go汇编必须以Go包的⽅式组织,同时包中⾄少要有⼀个Go源⽂件⽤于指明当前包名等基本包信息。
Go使⽤Plan9汇编意在提供更好的跨平台特性,让汇编与机器⽆关,但是并没能实现。
对于⼀些通⽤的,例如内存操作指令和过程调⽤指令,由于这些指令在许多平台上基本相同,所以可以进⾏抽象,但是对⼀些平台独有的指令,Go汇编却并不能进⾏抽象。
在命名上,Go汇编必须以CPU的体系结构作为⽂件名的后缀,如_amd64、arm等。
(?)在Go源码中,src/cmd⽬录下是⼀些与Go相关的命令实现,如go fmt、go doc等,⽽在src/cmd/compile⽬录下,便是Go编译器的实现。
对于⼀个Go语⾔⽂件,可以使⽤该⽬录下的⼀些⼯具来查看⽣成的汇编。
如可以使⽤下⾯的命令查看⼀个Go源⽂件⽣成的Go汇编。
$ GOOS=linux GOARCH=amd64 go tool compile -S main.go或$ go build -gcflags -S main.go要看最终⽣成的机器相关的汇编,可以使⽤go tool objdump:$ go build -o a.out main.go$ go tool objdump -s main.main a.out伪寄存器在Go汇编中,预定义了4个伪寄存器。
gomobile 编译
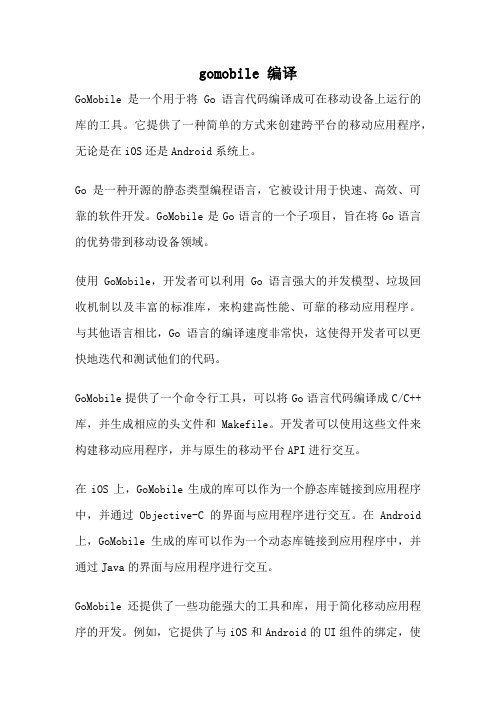
gomobile 编译GoMobile是一个用于将Go语言代码编译成可在移动设备上运行的库的工具。
它提供了一种简单的方式来创建跨平台的移动应用程序,无论是在iOS还是Android系统上。
Go是一种开源的静态类型编程语言,它被设计用于快速、高效、可靠的软件开发。
GoMobile是Go语言的一个子项目,旨在将Go语言的优势带到移动设备领域。
使用GoMobile,开发者可以利用Go语言强大的并发模型、垃圾回收机制以及丰富的标准库,来构建高性能、可靠的移动应用程序。
与其他语言相比,Go语言的编译速度非常快,这使得开发者可以更快地迭代和测试他们的代码。
GoMobile提供了一个命令行工具,可以将Go语言代码编译成C/C++库,并生成相应的头文件和Makefile。
开发者可以使用这些文件来构建移动应用程序,并与原生的移动平台API进行交互。
在iOS上,GoMobile生成的库可以作为一个静态库链接到应用程序中,并通过Objective-C的界面与应用程序进行交互。
在Android 上,GoMobile生成的库可以作为一个动态库链接到应用程序中,并通过Java的界面与应用程序进行交互。
GoMobile还提供了一些功能强大的工具和库,用于简化移动应用程序的开发。
例如,它提供了与iOS和Android的UI组件的绑定,使开发者可以使用Go语言来构建用户界面。
它还提供了对移动平台的各种传感器和设备功能的访问,如相机、GPS、陀螺仪等。
与其他跨平台开发工具相比,GoMobile具有一些独特的优势。
首先,由于Go语言本身的性能优势,使用GoMobile构建的应用程序具有更高的性能和可靠性。
其次,GoMobile提供了对原生移动平台API 的直接访问,开发者可以充分利用移动平台的功能和特性。
最后,GoMobile生成的库可以与现有的移动应用程序进行集成,无需进行大规模重写。
虽然GoMobile在移动应用程序开发领域还比较新,但它已经被越来越多的开发者采用。
Go微服务架构实战-中篇1.k8s架构介绍
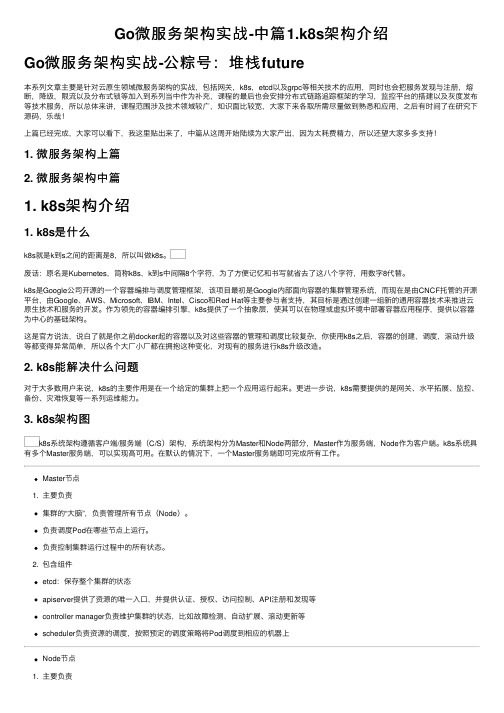
Go微服务架构实战-中篇1.k8s架构介绍Go微服务架构实战-公粽号:堆栈future本系列⽂章主要是针对云原⽣领域微服务架构的实战,包括⽹关,k8s,etcd以及grpc等相关技术的应⽤,同时也会把服务发现与注册,熔断,降级,限流以及分布式锁等加⼊到系列当中作为补充,课程的最后也会安排分布式链路追踪框架的学习,监控平台的搭建以及灰度发布等技术服务,所以总体来讲,课程范围涉及技术领域较⼴,知识⾯⽐较宽,⼤家下来各取所需尽量做到熟悉和应⽤,之后有时间了在研究下源码,乐哉!上篇已经完成,⼤家可以看下,我这⾥贴出来了,中篇从这周开始陆续为⼤家产出,因为太耗费精⼒,所以还望⼤家多多⽀持!1. 微服务架构上篇2. 微服务架构中篇1. k8s架构介绍1. k8s是什么k8s就是k到s之间的距离是8,所以叫做k8s。
废话:原名是Kubernetes,简称k8s,k到s中间隔8个字符,为了⽅便记忆和书写就省去了这⼋个字符,⽤数字8代替。
k8s是Google公司开源的⼀个容器编排与调度管理框架,该项⽬最初是Google内部⾯向容器的集群管理系统,⽽现在是由CNCF托管的开源平台,由Google、AWS、Microsoft、IBM、Intel、Cisco和Red Hat等主要参与者⽀持,其⽬标是通过创建⼀组新的通⽤容器技术来推进云原⽣技术和服务的开发。
作为领先的容器编排引擎,k8s提供了⼀个抽象层,使其可以在物理或虚拟环境中部署容器应⽤程序,提供以容器为中⼼的基础架构。
这是官⽅说法,说⽩了就是你之前docker起的容器以及对这些容器的管理和调度⽐较复杂,你使⽤k8s之后,容器的创建,调度,滚动升级等都变得异常简单,所以各个⼤⼚⼩⼚都在拥抱这种变化,对现有的服务进⾏k8s升级改造。
2. k8s能解决什么问题对于⼤多数⽤户来说,k8s的主要作⽤是在⼀个给定的集群上把⼀个应⽤运⾏起来。
更进⼀步说,k8s需要提供的是⽹关、⽔平拓展、监控、备份、灾难恢复等⼀系列运维能⼒。