Multiscaling behavior in the volatility return intervals of Chinese indices
教育游戏化:将课堂变成一场协同冒险游戏——以Classcraft为例

28 |
PUBLISHING REFERENCE
海外市场
“对战”形式完成教学评测。学生按时完成任务可 以获得奖励,并用来升级角色的经验值(Experience Points,XP)——这将使其角色提高战斗水平并学 习新的技能。如果一个学生违反了课堂纪律,就会 失去生命值,甚至最终导致角色在“对战”中失败。 如果学生获得经验值点数,对相应角色及其团队都 有益处;相反,如果一个学生失去了生命值点数, 其团队的其他成员角色也会受到伤害,并且大家必 须完成各种额外任务。无论如何,学生们需要共同 努力才能使团队获得成功。一般而言,没有学生愿 意自己的不当行为损害团队利益,导致他人失败。 游戏团队中,学生还可以帮助彼此成长。例如,如 果学生的虚拟角色是一名战士,而队友因为上课迟 到面临生命值点数降低,则该学生可以通过完成额 外的学习任务来挽救队友。学生知道他们在课堂上 的行为会影响整个团队的进度、这会激励他们强化 课堂上的积极行为和团队合作,提升课堂学习效率。 Classcraft 每个月都会发布新的故事情节和场景供教 育工作者选择,帮助提升学生的课堂参与感 [19]。除 了在预制故事中添加课程任务外,Classcraft 还允许 教师自己编写课程,通过上传不同的学习任务来教 授不同的科目。根据在课堂活动中收集的数据,教 师还可以查看学生的行为并进行分析。
是以游戏软件为基础的学习,教育游戏(Educational Games)的设
计与开发是当前研究的主流方向。教育游戏模糊了学习与游戏、正式 学习与非正式学习的边界 [13];但是有别于教育游戏的软件性质(见表
1),教育游戏化是一套解决方案,服务于教育情境中的各类问题,
如激发学习者动机和兴趣、引导学习者面对学业失败、激发其学校生
研究表明,随着游戏在当代文化中的地位日益 提高,其在教育中能够扮演的角色也越来越多样化。 Classcraft 作为受到游戏启发开发的教育解决方案, 它对于学习的积极作用和游戏非常相似。
一种基于词袋模型的新的显著性目标检测方法
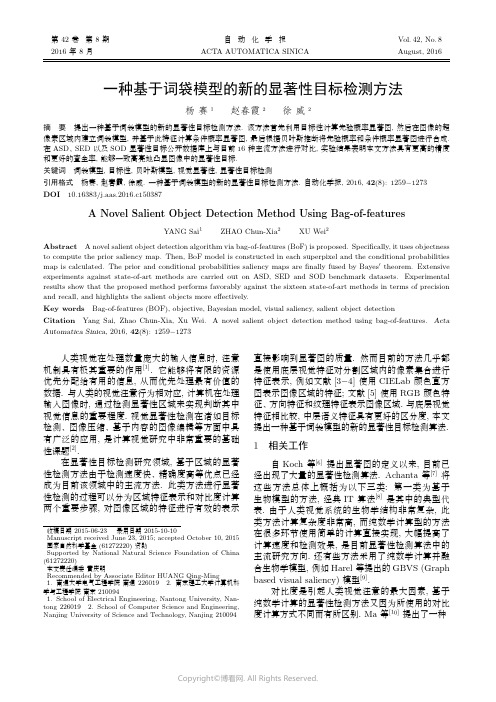
第42卷第8期自动化学报Vol.42,No.8 2016年8月ACTA AUTOMATICA SINICA August,2016一种基于词袋模型的新的显著性目标检测方法杨赛1赵春霞2徐威2摘要提出一种基于词袋模型的新的显著性目标检测方法.该方法首先利用目标性计算先验概率显著图,然后在图像的超像素区域内建立词袋模型,并基于此特征计算条件概率显著图,最后根据贝叶斯推断将先验概率和条件概率显著图进行合成.在ASD、SED以及SOD显著性目标公开数据库上与目前16种主流方法进行对比,实验结果表明本文方法具有更高的精度和更好的查全率,能够一致高亮地凸显图像中的显著性目标.关键词词袋模型,目标性,贝叶斯模型,视觉显著性,显著性目标检测引用格式杨赛,赵春霞,徐威.一种基于词袋模型的新的显著性目标检测方法.自动化学报,2016,42(8):1259−1273DOI10.16383/j.aas.2016.c150387A Novel Salient Object Detection Method Using Bag-of-featuresYANG Sai1ZHAO Chun-Xia2XU Wei2Abstract A novel salient object detection algorithm via bag-of-features(BoF)is proposed.Specifically,it uses objectness to compute the prior saliency map.Then,BoF model is constructed in each superpixel and the conditional probabilities map is calculated.The prior and conditional probabilities saliency maps arefinally fused by Bayes theorem.Extensive experiments against state-of-art methods are carried out on ASD,SED and SOD benchmark datasets.Experimental results show that the proposed method performs favorably against the sixteen state-of-art methods in terms of precision and recall,and highlights the salient objects more effectively.Key words Bag-of-features(BOF),objective,Bayesian model,visual saliency,salient object detectionCitation Yang Sai,Zhao Chun-Xia,Xu Wei.A novel salient object detection method using bag-of-features.Acta Automatica Sinica,2016,42(8):1259−1273人类视觉在处理数量庞大的输入信息时,注意机制具有极其重要的作用[1].它能够将有限的资源优先分配给有用的信息,从而优先处理最有价值的数据.与人类的视觉注意行为相对应,计算机在处理输入图像时,通过检测显著性区域来实现判断其中视觉信息的重要程度.视觉显著性检测在诸如目标检测、图像压缩、基于内容的图像编辑等方面中具有广泛的应用,是计算视觉研究中非常重要的基础性课题[2].在显著性目标检测研究领域,基于区域的显著性检测方法由于检测速度快、精确度高等优点已经成为目前该领域中的主流方法.此类方法进行显著性检测的过程可以分为区域特征表示和对比度计算两个重要步骤,对图像区域的特征进行有效的表示收稿日期2015-06-23录用日期2015-10-10Manuscript received June23,2015;accepted October10,2015国家自然科学基金(61272220)资助Supported by National Natural Science Foundation of China (61272220)本文责任编委黄庆明Recommended by Associate Editor HUANG Qing-Ming1.南通大学电气工程学院南通2260192.南京理工大学计算机科学与工程学院南京2100941.School of Electrical Engineering,Nantong University,Nan-tong2260192.School of Computer Science and Engineering, Nanjing University of Science and Technology,Nanjing210094直接影响到显著图的质量.然而目前的方法几乎都是使用底层视觉特征对分割区域内的像素集合进行特征表示,例如文献[3−4]使用CIELab颜色直方图表示图像区域的特征;文献[5]使用RGB颜色特征、方向特征和纹理特征表示图像区域.与底层视觉特征相比较,中层语义特征具有更好的区分度,本文提出一种基于词袋模型的新的显著性目标检测算法.1相关工作自Koch等[6]提出显著图的定义以来,目前已经出现了大量的显著性检测算法.Achanta等[7]将这些方法总体上概括为以下三类:第一类为基于生物模型的方法,经典IT算法[8]是其中的典型代表.由于人类视觉系统的生物学结构非常复杂,此类方法计算复杂度非常高,而纯数学计算型的方法在很多环节使用简单的计算直接实现,大幅提高了计算速度和检测效果,是目前显著性检测算法中的主流研究方向.还有些方法采用了纯数学计算并融合生物学模型,例如Harel等提出的GBVS(Graph based visual saliency)模型[9].对比度是引起人类视觉注意的最大因素,基于纯数学计算的显著性检测方法又因为所使用的对比度计算方式不同而有所区别.Ma等[10]提出了一种1260自动化学报42卷局部对比度的显著性检测方法,它使用CIELuv颜色表示图像中每个像素的特征,并使用欧式距离度量每个像素与其邻域像素之间的差异程度;MZ方法在计算局部对比度时,将邻域的大小设为固定值,无法实现多尺度的显著性计算,为此Achanta 等[11]提出通过改变感知单元邻域的尺寸大小实行显著性的多尺度计算;LC(Luminance-based con-trast)方法[12]同样是以图像中的每个像素作为基本处理单元,但与MZ不同的是,使用图像像素的灰度特征计算像素在整幅图像上的全局对比度;Cheng 等[3]提出的HC(Histogram-based contrast)方法在CIELab颜色空间的三个通道计算像素在整幅图像上的全局对比度;Achanta等[7]提出的FT(Fre-quency tuned)方法同样也是一种全局对比度计算方法,其所使用的全局信息是图像的平均信息; Goferman等[13]提出的CA(Contex aware)方法也是从感知单元之间的差异性出发计算显著性,但是与上面方法不同的是,CA考虑了感知单元之间的空间位置关系.上述显著性检测方法都是在像素级别计算显著性,而基于区域的显著性检测方法以图像区域为基本处理单元,速度更快,精度更高.此类方法又因为使用不同的分割方法,区域的图像特征表示和显著性计算而有所不同.Cheng等[3]提出的RC (Region-based contrast)方法使用图割对图像进行分割,然后使用颜色直方图表示每个图像区域的特征,在计算每个图像小块的全局对比度的同时考虑了颜色对比度、空间距离和分块大小三个因素;与RC方法基于超像素分割获得图像区域不同,Cheng 等[14]提出的GC(Global cues)方法利用对所有像素进行初始聚类得到的聚类中心计算颜色对比度,利用对高斯成分进行二次聚类得到聚类中心计算颜色空间分布,最后使用文献[15]中的方法将颜色对比度与颜色空间分布相结合得到最终显著图;Mar-golin等[16]提出的PD(Patch distinct)方法通过分析图像小块的内部统计特性,使用主成分分析表示图像小块进而计算图像小块的显著性;Jiang等[4]提出的CBS(Context-based saliency)方法使用图割方法将图像快速分成不同的子区域,使用CIELab 颜色直方图表示图像区域的特征,然后使用距离函数计算每个图像小块与近邻图像小块之间的差异性生成显著图;Shen等[5]提出的LR(Low rank)方法使用RGB颜色特征、方向特征和纹理特征表示图像区域,使用鲁棒PCA(Principal component analysis)算法对特征矩阵进行分解计算显著性.基于区域的显著性检测过程可以分为区域的图像特征表示和对比度计算两个重要步骤,目前此类方法几乎都是使用底层视觉特征进行对比度计算.相对于底层视觉特征,中层语义特征更加符合人类视觉模型,为此本文提出一种基于词袋模型的新的显著性目标检测方法.2本文方法2.1方法描述对于一幅给定的图像I,显著性检测的目的是将图像中任意像素x归于前景目标区域或者背景区域两种可能状态之一,将这两种状态分别简记为S (Salient)和B(Background),它们的先验概率相应地简记为P(S)和P(B),则根据贝叶斯推断原理,像素x的显著性计算公式为:P(S|x)=P(S)P(x|S)P(S)P(x|S)+P(B)P(x|B) P(S)+P(B)=1(1)式中,P(x|S)表示显著区域已知的情况下观测像素x的条件概率密度,P(x|B)表示背景区域已知的情况下观测像素x的条件概率密度.2.2基于目标性的先验概率本文使用目标性计算式(1)中的先验概率,对于图像中的任意像素x,以此像素为中心,随机抽取图像中的W个窗口,文献[17]分别从以下四个方面计算每个窗口的目标性:1)窗口显著性.首先利用任意显著性检测方法计算得到图像中每个像素的显著值I(p),则窗口w∈W的显著性计算公式为:S(w,θs)={p∈W|I(P)≥θs}I(p)×{p∈W|I(P)≥θs}|w|(2)式中,θs表示待学习的显著性阈值参数.2)颜色对比度.对于窗口w∈W,以θcc为固定倍数在每个方向将其扩展到周围区域得到某一矩形区域Surr(w,θcc),则窗口w在此区域内的颜色对比度计算公式为:CC(w,θcc)=χ2(h(w),h(Surr(w,θcc)))(3)式中,h(w)、h(Surr(w,θcc))分别表示窗口w与矩形区域Surr(w,θcc)的颜色直方图,χ2(·)表示卡方距离函数.3)边缘密度.对于窗口w∈W,以θED为固定倍数将其收缩到内部环状区域Inn(w,θED),则此窗口w在区域Inn(w,θED)内的边缘性计算公式为: ED(w,θED)=p∈Inn(w,θED)I ED(p)Len(Inn(w,θED))(4)8期杨赛等:一种基于词袋模型的新的显著性目标检测方法1261式中,I ED(p)表示使用Canny算子得到的二值图,Len(·)表示计算区域Inn(w,θED)的周长.4)轮廓闭合性.首先将图像分割为若干超像素S,则窗口w∈W的轮廓闭合性的计算公式为:SS(w)=1−s∈S min(|s\w|,|s∩w|)|w|(5)式中,s∈S表示图像中的第s个超像素,|s\w|表示超像素s位于窗口w之外的面积,而|s∩w|表示超像素s位于窗口w内部的面积.将上述得到的窗口显著性S(w,θs)、颜色对比度CC(w,θcc)、边缘密度ED(w,θED)以及轮廓闭合性SS(w)进行融合就得到每个窗口被判定为显著性目标的概率值P(w),那么基于目标性的先验概率计算公式为:P s(x)=w∈W∩x∈WP(w x)(6) 2.3超像素词袋特征已知一个图像数据集D={d1,d2,···,d N},由于CIELab颜色模型能够将亮度和色度分量分开,相关研究工作[3−4,7,16]也表明在此颜色空间进行检测得到的显著图的准确度更高,因此将图像变换到CIELab颜色空间,然后随机抽取其中的300k个像素的颜色特征组成局部特征集合X,对X进行聚类得到视觉词典V=[v1,v2,···,v K]∈R D×K, v k∈R D×1,k=1,2,···,K表示第k个视觉单词向量,K为视觉单词数目,D为像素颜色特征的维数.在得到视觉词典后,使用硬分配编码方法对图像中的每个像素进行编码[18].对于数据集中任意一幅图像,c j∈R D×1表示第j个像素颜色特征,其对应的编码矢量U j∈R K×1第k维值的计算公式为:U jk=1,若j=arg min j=1,2,···,K c j−v k 2 0,其他(7)式中,矢量c j与v k之间的距离计算采用欧氏函数.完成对图像中所有像素的编码操作之后,使用SLIC(Simple linear iterative clustering)方法对图像进行分割,如图1(b)所示,图像被相应地分割成为N个尺寸均匀的超像素,假设其中第n个超像素区域内共有P n个像素,则此区域内所有像素编码矢量的总和统计值为:BoF n=P nj=1U j(8)式中,U j表示超像素区域内第j个像素颜色特征的编码矢量,可以利用式(7)计算其第k维值,则BoF n就为图像中第n个超像素的词袋特征.(a)原图像(a)Original image(b)超像素分割(b)Superpixel of original image(c)背景区域(c)Background regions图1背景超像素示意图Fig.1Illustration of background s superpixels2.4条件概率为了估计式(1)中观测像素x的条件概率密度,本文假定图像周边的超像素区域为背景区域,如图1(c)所示.假设背景区域内超像素的数目为N b,背景超像素词袋特征记为BoF B,其中第j个超像素区域的词袋特征表示为BoF Bj,使用Parzen窗法[19]得到背景超像素特征BoF B的概率密度分布,表达式为:P(ˆBoF B)=1N bσKN bj=1KBoF B−BoF Bjσ(9)1262自动化学报42卷式中,K为核函数,σ为窗宽,K为背景超像素特征的维数,即词袋特征的维数.如果核函数选用高斯核函数,式(9)变为:P(ˆBoF B)=1N bσKN bj=1exp−BoF B−BoF Bj 22σ2(10)式中, · 2表示l2范数,则在背景区域已知的情况下,图像中任意超像素区域R n的条件概率密度计算公式为:P(ˆR i|B)=1N bσKN bj=1exp(− BoF i−BoF Bj 22σ2)P(R i|ˆS)=1−P(ˆR i|B)(11)将区域的显著性值传递给此区域内的所有像素就得到了基于中层语义特征的条件概率显著图P(x|B)和P(x|S).将式(6)中得到的先验概率和式(11)中得到的条件概率P(x|B)和P(x|S)代入式(1)中就得到了图像的最终显著图.3实验与分析3.1数据库及评价准则本节实验在4个显著性目标公开数据库上验证本文方法的性能.第一个为瑞士洛桑理工大学Achanta等建立的ASD数据库[7],该数据库是MSRA-5000数据库的一个子集,共有1000幅图像,是目前最为广泛使用的、已经人工精确标注出显著性目标的显著性检测算法标准测试库.第二个和第三个为SED1和SED2数据库[20],这两个数据库都包含共100幅图像,并且提供了3个不同用户给出的精确人工标注,也是目前广泛使用的显著性检测算法标准测试库.这两个数据库的主要区别在于前者每幅图像包含一个目标物体,而后者包含两个目标物体.第四个为SOD数据库[21],该数据库是由伯克利图像分割数据集的300幅图像所组成,提供了七个不同用户给出的精确人工标注.在第一个评价准则中,假设使用某一固定阈值t 对显著图进行分割,得到二值分割后的图像,t的取值范围为[0,255].将二值分割(Binary segmenta-tion,BS)图像与人工标注图像(Groud-truth,GT)进行比较得到查准率(Precision)和查全率(Recall),计算公式为:P recision=(x,y)GT(x,y)BS(x,y)(x,y)BS(x,y)(12)Recall=(x,y)GT(x,y)BS(x,y)(x,y)GT(x,y)(13)式中,GT和BS分别表示人工标注图像和二值分割后的图像.将阈值t依次设定为1到255对数据库中的所有显著图进行二值分割,计算出相应的平均查准率和查全率,以查全率为横坐标,以查准率为纵坐标,就得到了关于阈值t在整个数据库上的PR(Precision-recall)曲线.在第二个评价准则中,使用文献[3,5,7]中的自适应阈值确定方法对图像进行二值分割,同样与人工标注图像进行比较,得到查准率和查全率,并计算F度量值(F-measure),计算公式为:Fβ=(1+β2)×P recision×Recallβ2×P recision+Recall(14)与文献[3,5,7]一致,本文也将β2设为0.3,并且将自适应阈值设为图像显著值的整数倍,即:tα=KW×HWx=1Hy=1S(x,y)(15)式中,W、H分别表示显著图的宽度和长度,S为显著图,K的经验值为2.为了进一步评价F度量值的综合性能,在区间[0.1,6]中以0.1为采样步长均匀选取一系列K的值,利用式(14)计算不同K值对应的平均F度量值,然后以K值为横坐标,F值为纵坐标,相应地画出Fβ−K曲线.由于查准率和查全率不能度量显著图中被正确标注为前景像素和背景像素的精确数目,为了更加全面均衡地对显著性检测方法进行客观评价,使用文献[22]中的平均绝对误差(Mean absolute error,MAE)作为第三个评价准则,该准则计算未进行二值分割的连续显著图S与人工标注图GT所有像素之间的绝对误差的平均值,计算公式为:MAE=1W×HWx=1Hy=1|S(x,y)−GT(x,y)|(16)式中,W、H分别表示S以及GT的宽度和长度.3.2参数本文方法中的重要参数为超像素的数目N和视觉单词数目K,使用第二个度量准则中的平均F度量值衡量各种参数对检测性能的影响.首先固定K=50,将N分别设为100、150、200、250、300、350、400、450、500、600,不同超像素数目下的平均F度量值如图2所示,由此可知,ASD、SED1、SED2以及SOD四个数据库上,当N大于200之后,各个超像素数目之间的性能相差不大.当超像素数目分别为200、350、250、350时,本文方法取得最高的F值,因此接下来的实验在8期杨赛等:一种基于词袋模型的新的显著性目标检测方法1263四个数据库上将N分别设为200、350、250、350.将K分别设为10、20、30、40、50、60、70、80、90、100,不同单词数目下的平均F度量值如图3所示,由此可知,ASD数据库上各个单词数目之间的性能相差很小,单词数目为70时,本文方法取得了最高的F值.SED1和SED2数据库上F值的最高与最低之差分别为0.011和0.013,单词数目分别为80和20时,本文方法取得了最高的F值.SOD数据库上的最高值与最低值之差超过0.02,这主要是因为此数据集比较复杂,当视觉单词数目比较少时,不能充分编码图像中的颜色特征,从而加剧了视觉单词数目之间的性能之差.单词数为90时,本文方法取得了最高的F值.因此在接下来的实验中, ASD、SED1、SED2以及SOD数据库上的单词数目分别被设为70、80、20和90.3.3与其他显著性检测算法的比较将本文方法与16种流行的显著性检测方法进行性能比较.为了便于对比,本文将这16种流行算法分为:1)在图像像素级别上进行显著性计算的方法,包括IT[8]、MZ[10]、AC[11]、LC[12]、HC[3]、FT[7]、CA[13]、GBVS[9],这类方法是本领域引用次数较多的经典方法;2)在图像区域级别上进行显著性计算的方法,包括RC[3]、GC[14]、PD[16]、CBS[4]、LR[5],这类方法是近三年出现在顶级期刊上的方法;3)基于贝叶斯模型的方法,包括SUN(Saliency using natual statistics)[23]、SEG(Segmentation)[24]、CHB(Convex hull and Bayesian)[25],此类方法是与本文方法最为相关的显著性计算方法.3.3.1定量对比图4至图7给出了本文算法与16种流行算法的PR曲线.本文方法在ASD、SED1、SED2以及SOD四个数据库上都取得了最优的性能.当分割阈值t为0时,所有方法具有相同的查准率,在ASD、SED1、SED2以及SOD数据库上的数值分别为0.1985、0.2674、0.2137、0.2748,即表明数据库中分别平均有19.85%、26.74%、21.37%、27.48%的像素属于显著性区域.当分割阈值t为255时查全率达到最小值.此时本文方法的查准率在ASD、SED1、SED2、SOD数据库上分别达到了0.9418、0.8808、0.9088、0.7781.当查全率为0.85时,本文方法在ASD数据库上的查准率保持在0.9以上,在SED1、SED2两个数据库上保持在0.75以上,在SOD数据库上也高于0.5,表明本文方法能够以更高精度检测到显著区域的同时覆盖更大的显著性区域.除此之外,将式(15)中K值设为2计算自适应阈值,使用式(12)∼(14)分别计算平均查准率、查全率和F值,本文方法与16种流行算法的的对比结果见图8.由图中的数据可知,ASD数据与SED1数据库上取得了一致的结果,与基于像素的和基于区域的13种检测方法相比,本文方法具有最高的查准率、查全率和F值,说明本文方法能够以最高的图2不同超像素数目下的平均F值Fig.2F-measure under different superpixel numbers图3不同单词数目下的平均F值Fig.3F-measure under different visual words numbers1264自动化学报42卷(a)与基于像素的检测算法的对比(a)Comparison with algorithmsbased on pixels(b)与基于区域的检测算法的对比(b)Comparison with algorithmsbased on regions(c)与基于贝叶斯模型的检测算法的对比(c)Comparison with algorithms basedon Bayesian model图4ASD数据库上本文方法与其他16种流行算法的PR曲线Fig.4Precision-recall curves of our method and sixteen state-of-the-art methods on ASD database(a)与基于像素的检测算法的对比(a)Comparison with algorithmsbased on pixels(b)与基于区域的检测算法的对比(b)Comparison with algorithmsbased on regions(c)与基于贝叶斯模型的检测算法的对比(c)Comparison with algorithms basedon Bayesian model图5SED1数据库上本文方法与其他16种流行算法的PR曲线Fig.5Precision-recall curves of our method and sixteen state-of-the-art methods on SED1database(a)与基于像素的检测算法的对比(a)Comparison with algorithmsbased on pixels(b)与基于区域的检测算法的对比(b)Comparison with algorithmsbased on regions(c)与基于贝叶斯模型的检测算法的对比(c)Comparison with algorithms basedon Bayesian model图6SED2数据库上本文方法与其他16种流行算法的PR曲线Fig.6Precision-recall curves of our method and sixteen state-of-the-art methods on SED2database精度检测显著性目标,同时能够最大覆盖显著性目标所在区域.与基于贝叶斯模型的显著性检测方法相比,本文方法具有最高的查准率,但是查全率仅仅低于CHB方法,这主要是因为CHB使用角点检测显著性区域作为先验信息时,很多角点会落在背景区域,造成检测到的显著性区域过大,如图22(d)中第4排、图23(d)中第2排所示.但本文方法仍然具有最高F度量值,说明仍具有更优的检测性能.在SED2和SOD数据库上取得了一致结果,与所有对比方法相比较,本文方法具有最高的查全率和F 值,但是查准率却分别低于SEG方法和CBS方法.为了更进一步评价F度量值的综合性能,将式8期杨赛等:一种基于词袋模型的新的显著性目标检测方法1265 (15)中K值分别设为[0.1:0.1:6]计算自适应阈值,使用式(14)计算得到一系列F值,以K值为横坐标,以F值为纵坐标得到Fβ−K曲线.本文方法与16种流行算法的Fβ−K曲线分别见图9∼图12.由图中的结果可知,在ASD数据库上,与基于像素的和基于区域的13种检测方法相比,本文方法在每个K值处都具有最高的F值,与基于贝叶斯模型的显著性检测算法相比较,在K∈[5.7,6]这个区间时(如图9(c)所示),本文方法的F值低于CHB方法,在K取其他值时,本文方法的F值仍然最高,这是因为CHB方法的检测结果会出现显著范围过大的现象.SED1、SED2和SOD数据库上取得了一致的结果,相较于所有对比方法,本文方法在每个K值处都具有最高的F值.(a)与基于像素的检测算法的对比(a)Comparison with algorithmsbased on pixels(b)与基于区域的检测算法的对比(b)Comparison with algorithmsbased on regions(c)与基于贝叶斯模型的检测算法的对比(c)Comparison with algorithms basedon Bayesian model图7SOD数据库上本文方法与其他16种流行算法的PR曲线Fig.7Precision-recall curves of our method and sixteen state-of-the-art methods on SOD database(a)ASD数据库(a)ASD database(b)SED1数据库(b)SED1database(c)SED2数据库(c)SED2database(d)SOD数据库(d)SOD database图8本文方法与16种流行算法的平均查准率、平均查全率、F度量值对比图Fig.8Precision,recall and F-measure of our method and sixteen state-of-the-art methods1266自动化学报42卷(a)与基于像素的检测算法的对比(a)Comparison with algorithms basedon pixels(b)与基于区域的检测算法的对比(b)Comparison with algorithms basedon regions(c)与基于贝叶斯模型的检测算法的对比(c)Comparison with algorithms basedon Bayesian model图9ASD数据库上本文方法与16种流行算法的Fβ−K曲线Fig.9Fβ−K curves of our method and sixteen state-of-the-art methods on ASD database(a)与基于像素的检测算法的对比(a)Comparison with algorithms basedon pixels(b)与基于区域的检测算法的对比(b)Comparison with algorithms basedon regions(c)与基于贝叶斯模型的检测算法的对比(c)Comparison with algorithms basedon Bayesian model图10SED1数据库上本文方法与16种流行算法的Fβ−K曲线Fig.10Fβ−K curves of our method and sixteen state-of-the-art methods on SED1database(a)与基于像素的检测算法的对比(a)Comparison with algorithms basedon pixels(b)与基于区域的检测算法的对比(b)Comparison with algorithms basedon regions(c)与基于贝叶斯模型的检测算法的对比(c)Comparison with algorithms basedon Bayesian model图11SED2数据库上本文方法与16种流行算法的Fβ−K曲线Fig.11Fβ−K curves of our method and sixteen state-of-the-art methods on SED2database为了全面评价显著性检测方法的性能,根据式(16)计算显著图与人工标注图之间的MAE值,本文方法与16种流行算法在所有数据库上的对比结果分别见图13.由图中的结果可知,四个数据库上取得了一致的结果,本文方法具有最低的MAE值, SUN方法的MAE值最高.在ASD、SED1以及SOD数据库上,所有对比方法中,GC的MAE值最低,与该方法相比较,本文方法的MAE值又分别降低了22%、12%和17%;在SED2数据库上,所有对比方法中,HC的MAE值最低,与该方法相比较,本文方法的MAE值又降低了13%.3.3.2视觉效果对比本文方法与基于像素的显著性检测算法的视觉对比结果见图14∼17.由图14(b)和14(i)、图8期杨赛等:一种基于词袋模型的新的显著性目标检测方法1267(a)与基于像素的检测算法的对比(a)Comparison with algorithms basedon pixels(b)与基于区域的检测算法的对比(b)Comparison with algorithms basedon regions(c)与基于贝叶斯模型的检测算法的对比(c)Comparison with algorithms basedon Bayesian model图12SOD数据库上本文方法与16种流行算法的Fβ−K曲线Fig.12Fβ−K curves of our method and sixteen state-of-the-art methods on SOD database(a)ASD数据库(a)ASD database(b)SED1数据库(b)SED1database(c)SED2数据库(c)SED2database(d)SOD数据库(d)SOD database图13本文方法与其他16种流行算法的MAE值对比图Fig.13MAE of our method and sixteen state-of-the-art methods15(b)和15(i)、图16(b)和16(i)以及图17(b)和17(i)可知,IT和GBVS方法得到的显著图分辨率比较低,这是因为IT方法采用下采样的方式实现多尺度显著性计算,而GBVS方法中的马尔科夫链平衡状态的计算复杂度比较高,同样需要减小图像的分辨率实现快速计算.由图14(c)、图15(c)、图16(c)以及图17(c)可知,MZ方法得到的显著图过分强调显著性目标边缘部分,这是因为在计算局部对比度时使用的邻域比较少.相对于MZ方法,AC 方法是一种多尺度局部对比度方法,多个尺度的范围比较大,如图14(d)、图15(d)、图16(d)以及图17(d)所示,该方法能够检测到整个显著性目标.LC 和HC都是使用颜色的全局对比度,导致稀有颜色占优,只能检测到显著性目标的部分区域,例如图14(e)和14(f)的第4排,两种方法只将鸡蛋中最明亮的颜色检测出来.图15(e)和15(f)中的第1排1268自动化学报42卷图14ASD 数据库上本文方法与基于像素的典型显著性检测算法的视觉效果对比图Fig.14Visual comparison with detection methods based on pixels on ASDdatabase图15SED1数据库上本文方法与基于像素的典型显著性检测算法的视觉效果对比图Fig.15Visual comparison with detection methods based on pixels on SED1database图16SED2数据库上本文方法与基于像素的典型显著性检测算法的视觉效果对比图Fig.16Visual comparison with detection methods based on pixels on SED2database图17SOD 数据库上本文方法与基于像素的典型显著性检测算法的视觉效果对比图Fig.17Visual comparison with detection methods based on pixels on SOD database和第2排也出现了相同的现象,两种方法将图像中颜色最明亮的水面和草地错误地检测为显著性区域.与MZ 方法相比,CA 方法考虑了像素之间的距离因素,检测性能有很大的提高,但是仍然只是使用K 个近邻计算局部对比度,因此同样会过分强调显著性目标边缘,如图14(h)、图15(h)、图16(h)以及图17(h)所示.与基于像素的典型显著性检测算法相比,本文方法以区域为处理单位,如图14(j)、图15(j)、图16(j)以及图17(j)所示,显著图具有很高的分辨率,能够一致高亮地凸显图像中的显著。
Processability and automaticity

2022.10.18
CONTENT
Processability Theory • Introduction Automaticity Theory • Key constructs • Conclusion • References
INTRODUCTION OF PT
• Processability Theory claims that the mechanisms of the human mind impose constraints on how learners develop the ability to process such linguistic information.
• Processability theory is a theory and a model of second language acquisition( main focus) developed by Manfred Pienemann that touches the linguistic structures that surface in the learning of a second language.
KEY CONSTRUCTS
1. Processing hierarchy theory: the key of processability theory • A kind of language information processing program rated by grade. • The basis is transfer of grammatical information between sentences or phrases. • Eg. She gives him a book.( the grammatical information of the singular third person isR源自PRESENTATIVES OF AT
融合多尺度通道注意力的开放词汇语义分割模型SAN
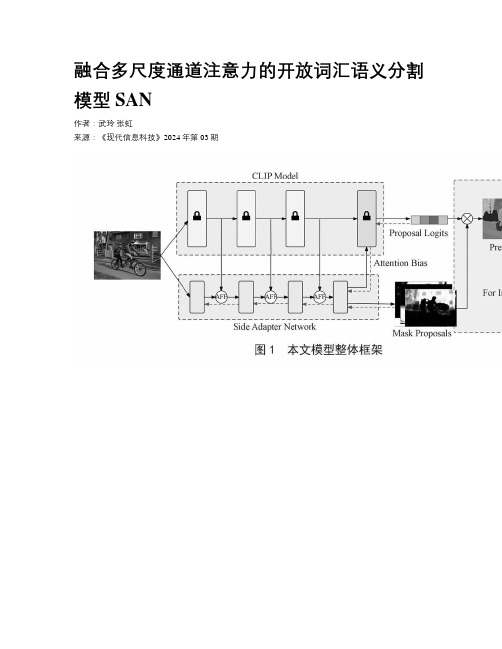
融合多尺度通道注意力的开放词汇语义分割模型SAN作者:武玲张虹来源:《现代信息科技》2024年第03期收稿日期:2023-11-29基金项目:太原师范学院研究生教育教学改革研究课题(SYYJSJG-2154)DOI:10.19850/ki.2096-4706.2024.03.035摘要:随着视觉语言模型的发展,开放词汇方法在识别带注释的标签空间之外的类别方面具有广泛应用。
相比于弱监督和零样本方法,开放词汇方法被证明更加通用和有效。
文章研究的目标是改进面向开放词汇分割的轻量化模型SAN,即引入基于多尺度通道注意力的特征融合机制AFF来改进该模型,并改进原始SAN结构中的双分支特征融合方法。
然后在多个语义分割基准上评估了该改进算法,结果显示在几乎不改变参数量的情况下,模型表现有所提升。
这一改进方案有助于简化未来开放词汇语义分割的研究。
关键词:开放词汇;语义分割;SAN;CLIP;多尺度通道注意力中图分类号:TP391.4;TP18 文献标识码:A 文章编号:2096-4706(2024)03-0164-06An Open Vocabulary Semantic Segmentation Model SAN Integrating Multi Scale Channel AttentionWU Ling, ZHANG Hong(Taiyuan Normal University, Jinzhong 030619, China)Abstract: With the development of visual language models, open vocabulary methods have been widely used in identifying categories outside the annotated label. Compared with the weakly supervised and zero sample method, the open vocabulary method is proved to be more versatile and effective. The goal of this study is to improve the lightweight model SAN for open vocabularysegmentation, which introduces a feature fusion mechanism AFF based on multi scale channel attention to improve the model, and improve the dual branch feature fusion method in the original SAN structure. Then, the improved algorithm is evaluated based on multiple semantic segmentation benchmarks, and the results show that the model performance has certain improvement with almost no change in the number of parameters. This improvement plan will help simplify future research on open vocabulary semantic segmentation.Keywords: open vocabulary; semantic segmentation; SAN; CLIP; multi scale channel attention 0 引言識别和分割任何类别的视觉元素是图像语义分割的追求。
模式识别与机器学习 复习资料 温雯 老师
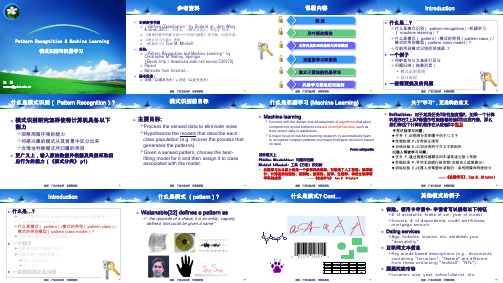
温雯
一些需要提及的问题
温雯 广东工业大学 计算机学院 23
温雯
广东工业大学
计算机学院
21
温雯
广东工业大学
模式识别系统的复杂性 – An Example
“利用光学传感器采集信息,对 传送带上的鱼进行种类的自动 区分” Fish Classification: Sea Bass / Salmon
一个例子
将鲈鱼与三文鱼进行区分 问题归纳(抽象而言) • 模式识别系统 • 设计流程
Preprocessing involves:
广东工业大学 计算机学院 28
Overlap in the histograms is small compared to length feature 温雯 广东工业大学 计算机学院
27
温雯
判定边界
错误分类的代价
模型的复杂度
Generalization (推广能力)
Partition the feature space into two regions by finding the decision boundary (判定边界)that minimizes the error.
Optical Character Recognition (typography)
A v t u I h D U w K
一种新的人机交互系统 你,从中看到模式识别吗?
Vision
语言的群体间偏差研究
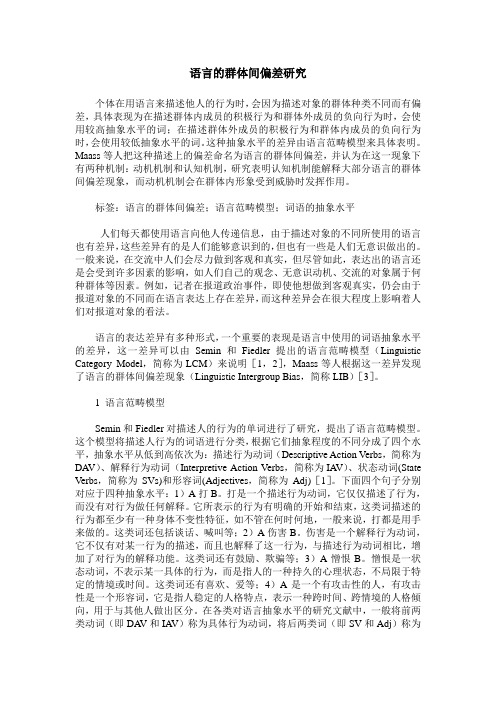
语言的群体间偏差研究个体在用语言来描述他人的行为时,会因为描述对象的群体种类不同而有偏差,具体表现为在描述群体内成员的积极行为和群体外成员的负向行为时,会使用较高抽象水平的词;在描述群体外成员的积极行为和群体内成员的负向行为时,会使用较低抽象水平的词。
这种抽象水平的差异由语言范畴模型来具体表明。
Maass等人把这种描述上的偏差命名为语言的群体间偏差,并认为在这一现象下有两种机制:动机机制和认知机制,研究表明认知机制能解释大部分语言的群体间偏差现象,而动机机制会在群体内形象受到威胁时发挥作用。
标签:语言的群体间偏差;语言范畴模型;词语的抽象水平人们每天都使用语言向他人传递信息,由于描述对象的不同所使用的语言也有差异,这些差异有的是人们能够意识到的,但也有一些是人们无意识做出的。
一般来说,在交流中人们会尽力做到客观和真实,但尽管如此,表达出的语言还是会受到许多因素的影响,如人们自己的观念、无意识动机、交流的对象属于何种群体等因素。
例如,记者在报道政治事件,即使他想做到客观真实,仍会由于报道对象的不同而在语言表达上存在差异,而这种差异会在很大程度上影响着人们对报道对象的看法。
语言的表达差异有多种形式,一个重要的表现是语言中使用的词语抽象水平的差异,这一差异可以由Semin和Fiedler提出的语言范畴模型(Linguistic Category Model,简称为LCM)来说明[1,2],Maass等人根据这一差异发现了语言的群体间偏差现象(Linguistic Intergroup Bias,简称LIB)[3]。
1 语言范畴模型Semin和Fiedler对描述人的行为的单词进行了研究,提出了语言范畴模型。
这个模型将描述人行为的词语进行分类,根据它们抽象程度的不同分成了四个水平,抽象水平从低到高依次为:描述行为动词(Descriptive Action Verbs,简称为DA V)、解释行为动词(Interpretive Action Verbs,简称为IA V)、状态动词(State Verbs,简称为SVs)和形容词(Adjectives,简称为Adj)[1]。
选择与坚持:跨期选择与延迟满足之比较
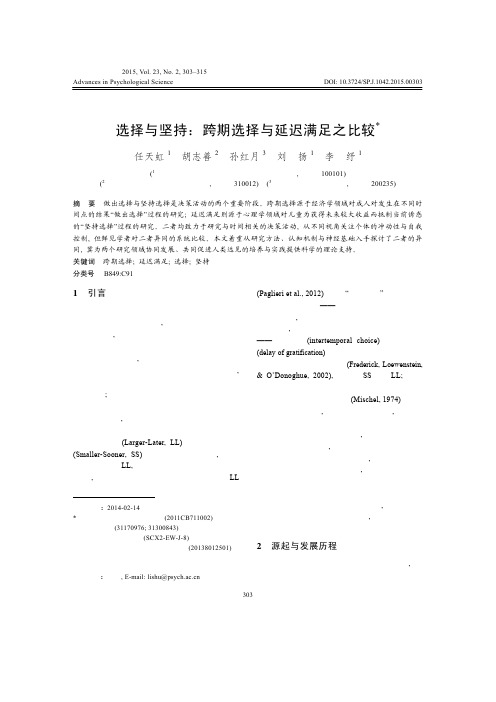
第2期
任天虹等 : 选择与坚持:跨期选择与延迟满足之比较
305
会通过预实验选择一对较为恰当的 SS 与 LL, 以 保证 SS 与 LL 之间的差异既要大到足以使被试愿 意选择后者 , 又要小到使 SS 对儿童有足够的诱惑 , 从而避免等待时间的天花板效应与地板效应 (Mischel & Underwood, 1974)。 动物实验在跨期选择与延迟满足的研究中都 有 所 涉 及 , 这 些动 物研 究 的 实验 范式 直 观 易 懂 , 对儿童被试的研究也颇有启示意义。随着研究内 容的扩展与研究范式的改进 , 跨期选择与延迟满 足在研究对象上有融合的趋势。 3.2 研究内容 跨期选择关注被试的时间折扣 (time discounting), 而延迟满足则更关注被试在等待时间上的 个 体 差 异 、 自 我 控 制 策 略 及 其 有 效 性 (Ainslie, 1975; Mischel et al., 1989)。如果说跨期选择的研 究者将其研究重点放在了计算、分析、推理、权 衡等较为高级的认知过程上 , 那么延迟满足的研 究者则将其重点放在了情绪、意志力、动机强度 等更为基础的本能反应上。 时间折扣是跨期选择研究的基本假设 , 也是 其研究的重要内容 , 它是指在跨期选择中 , 个体 首先会对延迟结果的价值根据其延迟的时间进行 一定的折扣, 然后再对两个结果进行比较(Frederick et al., 2002; Scholten & Read, 2010)。经济学家力 图找到某一通用的公式来描述折扣程度与结果及 延 迟 时 间 之间 的 关 系 , 表 现 为 数 理 模 型 的 优化 ; 心理学家则更关注外界因素对个体时间折扣程度 的影响 , 表现为认知神经机制的揭示。 延迟满足的研究者并不关注折扣程度 , 他们 更加关注等待时间上的个体差异 , 并在实验中细 致地检验被试自我控制策略的选择与使用状况 , 这些研究者热衷于以追踪研究揭示儿童在实验中 的表现与其个性及行为特征之间的关系 (Mischel et al., 1989)。 3.3 研究范式 跨期选择的研究关注被试的选择过程 , 它要 求被试做出一系列选择 ; 延迟满足则更关注被试 的坚持过程 , 要求被试坚持完成选择后的等待过 程。尽管在跨期选择的部分任务中也涉及到等待 过程 , 但是它与延迟满足有本质的区别。被试在 延迟满足任务中能够自主选择中止等待 , 而在跨 期选择任务中则只能消极等待延时结束 (Evans & Beran, 2007)。
集成主动学习方法的半监督多视图聚类

集成主动学习方法的半监督多视图聚类引言在当今的信息时代,数据的爆炸性增长使得数据挖掘和聚类成为了研究和应用领域中的重要问题。
聚类是一种无监督学习方法,它将数据集中相似的对象归为一类。
然而,传统的聚类方法在处理大规模和高维度数据时存在一些问题,例如维度灾难和样本稀疏性。
为了解决这些问题,研究者们提出了多视图聚类方法,并结合半监督学习技术来提高聚类效果。
本文将介绍一种集成主动学习方法的半监督多视图聚类算法。
首先,我们将介绍多视图聚类和半监督学习的基本概念和技术。
然后,我们将详细介绍集成主动学习方法,并解释它在半监督多视图聚类中的应用。
一、多视图聚类1.1 基本概念传统上,每个样本只有一个特征向量来描述其属性。
然而,在现实世界中,一个对象往往可以从不同角度进行描述,并且每个描述角度都可以看作是一个视图。
多视图聚类是一种利用多个视图进行聚类的方法。
通过融合不同的视图信息,多视图聚类可以提供更准确和全面的聚类结果。
1.2 多视图聚类方法目前,有许多不同的多视图聚类方法被提出,包括谱聚类、共识谱聚类、子空间聚类等。
这些方法通过将不同视图的相似度矩阵进行融合,来实现更好的聚类效果。
二、半监督学习2.1 基本概念半监督学习是介于无监督学习和有监督学习之间的一种学习方式。
它利用有标签和无标签样本来进行训练,并通过利用无标签样本中包含的信息来提高分类或者聚类效果。
2.2 半监督学习方法目前,半监督学习领域中存在许多不同的方法,包括自训练、协同训练、主动学习等。
这些方法通过利用无标签样本中潜在的信息来提高分类或者聚类效果。
三、集成主动学习方法3.1 基本概念集成主动学习是一种结合主动学习和集成学习的方法。
主动学习是一种主动选择有用样本进行标注的方法,而集成学习是一种通过结合多个分类器来提高分类效果的方法。
集成主动学习通过结合多个主动学习器来提高聚类效果。
3.2 集成主动学习方法在半监督多视图聚类中的应用在半监督多视图聚类中,集成主动学习方法可以通过选择有用样本进行标注,并结合多个视图信息来提高聚类效果。
q阶正交模糊有序加权几何算子在多属性决策中的应用

1引言在多属性决策中,决策者很难根据某些标准准确表达对备选方案的偏好,特别是在依赖于不确定和不完整信息时。
为了处理多属性决策中的不确定信息,将一些不确定数学工具应用到多属性决策中,如模糊集(FSs )[1]、直觉模糊集(IFSs )、毕达哥拉斯模糊集(PFSs )等。
虽然,IFSs 和PFSs 可以有效地描述多属性决策中复杂的决策信息,但也有不少情况不能满足IFSs 和PFSs 。
例如,如果隶属度和非隶属度的平方和大于1,那么决策信息不能由IFSs 或PFSs 来表达。
2017年,Yager [2]提出了q 阶正交模糊集(q -ROFSs ),要求隶属度的q 次方和非隶属度的q 次方之和小于等于1。
当q =1时,q -ROFSs 退化为IFSs ;当q =2时,q -ROFSs 退化为PFSs 。
显然,随着q 的增加,q -ROFSs 的应用范围越来越大,为决策者提供了更多的表达自由。
由于其独特的优势,q -ROFSs 越来越受到人们的关注。
集成算子是多属性决策方法中的一个核心问题。
几何集成算子作为一种重要的信息集结算子,它能突出决策信息集成过程中单个数据的作用,在处理多属性决策问题时更具灵活性和适用性。
基于此,针对q 阶正交模糊环境下的信息集成问题,给出了q 阶正交模糊有序加权几何集成算子,建立了q 阶正交模糊多属性决策方法。
2预备知识定义1[2]:设X 为一个论域,X 上的q 阶正交模糊集G 的定义为:G ={<x ,μG (x ),νG (x)>|x ∈X }(1)其中,μG (x )和νG (x )为取值在[0,1]上的元素集合,分别表示G 中元素x ∈X 的隶属度和非隶属度,使得0≤μG (x )q+νG (x)q≤1,q ≥1。
定义2[2]:设α=(μa ,νa )和β=(μβ,νβ)为任意两个q 阶正交模糊数(q -ROFN ),则α和β的得分函数和精确函数可分别定义为S (α)=(μqa -νqa ),S (β)=(μqβ-νqβ),H (α)=(μqa +νqa ),H (β)=(μqβ+νqβ),那么:①如果S (α)>S (β),那么α>β。
《2024年基于多模态注意力机制的视觉问答研究》范文

《基于多模态注意力机制的视觉问答研究》篇一一、引言随着人工智能技术的快速发展,视觉问答(Visual Question Answering,VQA)成为了计算机视觉与自然语言处理领域的热门研究方向。
视觉问答的目标是使机器能够理解图像或视频中的内容,并能够根据这些内容回答相关的问题。
然而,由于图像和文本的复杂性,单一的视觉或语言模型往往难以准确理解并回答复杂的问题。
因此,基于多模态注意力机制的视觉问答研究应运而生,旨在通过融合图像和文本信息,提高视觉问答的准确性和鲁棒性。
二、多模态注意力机制概述多模态注意力机制是一种有效的跨模态信息融合方法,通过将图像和文本信息进行相互关注,实现对多模态信息的有效利用。
在视觉问答任务中,多模态注意力机制能够根据问题的语义信息,自动关注图像中与问题相关的区域,从而提取出更有利于问题解答的信息。
此外,多模态注意力机制还能够根据图像的视觉特征,对问题中的关键词进行重新加权,提高问题理解的准确性。
三、基于多模态注意力机制的视觉问答模型本文提出了一种基于多模态注意力机制的视觉问答模型。
该模型主要包括以下几个部分:1. 图像特征提取:采用卷积神经网络(CNN)对图像进行特征提取,得到图像的视觉特征。
2. 文本特征提取:采用循环神经网络(RNN)对问题进行语义理解,得到问题的文本特征。
3. 多模态注意力机制:将图像和文本特征进行融合,通过多模态注意力机制实现相互关注。
具体地,根据问题的文本特征,对图像中的每个区域进行加权,提取出与问题相关的图像区域;同时,根据图像的视觉特征,对问题中的关键词进行重新加权,提高问题理解的准确性。
4. 答案预测:将加权后的图像和文本特征进行融合,输入到全连接层进行答案预测。
四、实验与分析为了验证本文提出的基于多模态注意力机制的视觉问答模型的有效性,我们在公开的视觉问答数据集上进行了一系列实验。
实验结果表明,相比传统的视觉问答模型,本文提出的模型在准确率和鲁棒性方面均有明显提升。
多智能体系统 交互结构特征
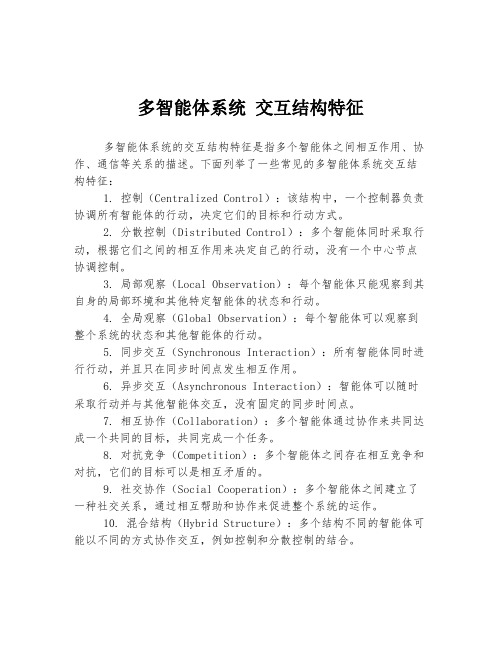
多智能体系统交互结构特征
多智能体系统的交互结构特征是指多个智能体之间相互作用、协作、通信等关系的描述。
下面列举了一些常见的多智能体系统交互结构特征:
1. 控制(Centralized Control):该结构中,一个控制器负责协调所有智能体的行动,决定它们的目标和行动方式。
2. 分散控制(Distributed Control):多个智能体同时采取行动,根据它们之间的相互作用来决定自己的行动,没有一个中心节点协调控制。
3. 局部观察(Local Observation):每个智能体只能观察到其自身的局部环境和其他特定智能体的状态和行动。
4. 全局观察(Global Observation):每个智能体可以观察到整个系统的状态和其他智能体的行动。
5. 同步交互(Synchronous Interaction):所有智能体同时进行行动,并且只在同步时间点发生相互作用。
6. 异步交互(Asynchronous Interaction):智能体可以随时采取行动并与其他智能体交互,没有固定的同步时间点。
7. 相互协作(Collaboration):多个智能体通过协作来共同达成一个共同的目标,共同完成一个任务。
8. 对抗竞争(Competition):多个智能体之间存在相互竞争和对抗,它们的目标可以是相互矛盾的。
9. 社交协作(Social Cooperation):多个智能体之间建立了一种社交关系,通过相互帮助和协作来促进整个系统的运作。
10. 混合结构(Hybrid Structure):多个结构不同的智能体可能以不同的方式协作交互,例如控制和分散控制的结合。
一种基于词共现图的受限领域自动问答系统
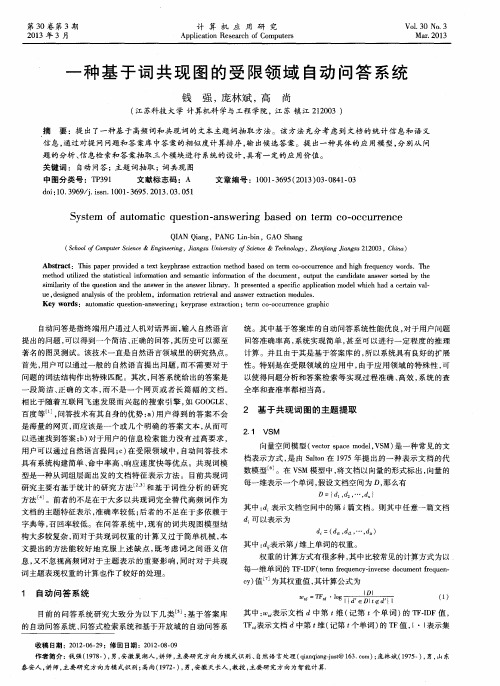
( 江 苏科技 大 学 计算机 科 学与 工程 学院 ,江 苏 镇 江 2 1 2 0 0 3)
摘
要 :提 出 了一 种基 于 高频 词和 共现词 的文本 主题词 抽 取 方法 。该 方 法 充分 考 虑到 文 档 的统 计 信 息和 语 义
信息, 通过 对提 问 问题 和答 案库 中答 案的相 似度 计算排 序 , 输 出候 选答 案 。提 出一种具 体的 应 用模 型 , 分 别从 问
u e , d e s i g n e d a n a l y s i s o f t h e p r o b l e m ,i n f o ma r t i o n r e t r i e v a l a n d ns a w e r e x t r a c t i o n mo d u l e s .
题 的分析 、 信 息检 索和 答案抽 取 三个模 块进 行 系统的设 计 , 具 有一 定的 应用价 值 。 关键 词 : 自动 问答 ;主题 词抽 取 ; 词 共现 图
中图分类 号 :T P 3 9 1 文献 标志 码 :A 文章编 号 :1 0 0 1 . 3 6 9 5 ( 2 0 1 3 ) o 3 — 0 8 4 1 . 0 3
Q I A N Q i a n g , P A N G L i n — b i n , G A O S h a n g
( S c h o o l o fC o m p u t e r S c i e n c e& E n g i n e e r i n g ,J i a n g s u U n i v e r s i t y fS o c i e n c e& T e c h n o l o g y , Z h e n j i a n g J i a n g s u 2 1 2 0 0 3,C h i n a )
网路银行消费者行为之研究--创新扩散理论
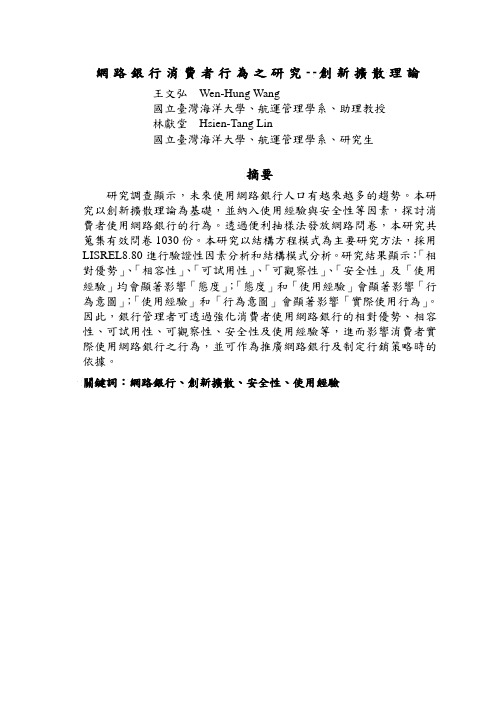
網路銀行消費者行為之研究--創新擴散理論王文弘Wen-Hung Wang國立臺灣海洋大學、航運管理學系、助理教授林獻堂Hsien-Tang Lin國立臺灣海洋大學、航運管理學系、研究生摘要研究調查顯示,未來使用網路銀行人口有越來越多的趨勢。
本研究以創新擴散理論為基礎,並納入使用經驗與安全性等因素,探討消費者使用網路銀行的行為。
透過便利抽樣法發放網路問卷,本研究共蒐集有效問卷1030份。
本研究以結構方程模式為主要研究方法,採用LISREL8.80進行驗證性因素分析和結構模式分析。
研究結果顯示:「相對優勢」、「相容性」、「可試用性」、「可觀察性」、「安全性」及「使用經驗」均會顯著影響「態度」;「態度」和「使用經驗」會顯著影響「行為意圖」;「使用經驗」和「行為意圖」會顯著影響「實際使用行為」。
因此,銀行管理者可透過強化消費者使用網路銀行的相對優勢、相容性、可試用性、可觀察性、安全性及使用經驗等,進而影響消費者實際使用網路銀行之行為,並可作為推廣網路銀行及制定行銷策略時的依據。
關鍵詞:網路銀行、創新擴散、安全性、使用經驗A n E m p i r i c a l R e s e a r c h o f C u s t o m e rB e h a v i o r o f We b-B a n k i n g S e r v i c e,I n n o v a t i o n o f D i f f u s i o n T h e o r yAbstractWith the rapid growth internet users, the demand of web-banking is eager. Based on innovation of diffusion theory, this research wants to discuss the factors that influence consumer web-bank using behavior. Data collected from online survey and got 1030 usable samples.With the analysis of SEM, the analytic results showed that “Relative Advantage,” “Compatibility,” “Trialibility,” “Observability,” “Security,” and “Experience,” have positive significant effect on “Attitude;”in the meanwhile, both “Attitude,” and “E xperience” have positive significant on “Behavioral Intention;” furthermore, both “E xperience” and “Behavioral Intention” have positive significant effect on “Usage.”Based on the analytic results,suggestions are the managers of banks should enhance the attributes of web-bank by “Relative Advantage,”“Compatibility,”“Trialibility,” “Observability,” “Security” and “Experience,”in order to achieve both elevation of using behavior of web-bank and financial performance.Keywords: Web-Bank, Innovation of Diffusion, Security, Experience緒論現今台灣的金融環境,正面臨著一系列的改革與挑戰。
多维事件的的表述方法
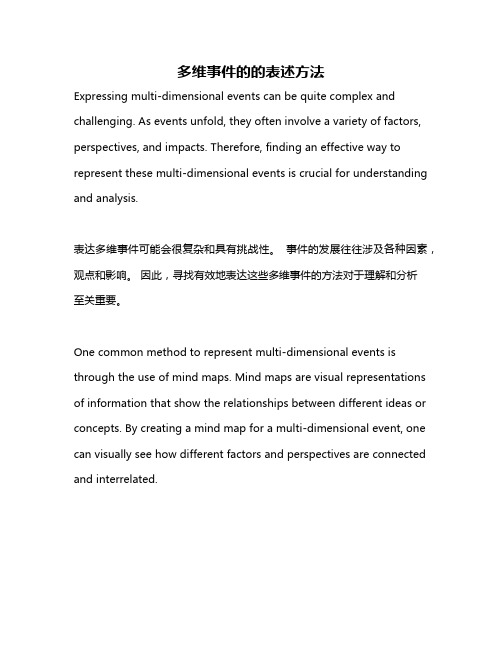
多维事件的的表述方法Expressing multi-dimensional events can be quite complex and challenging. As events unfold, they often involve a variety of factors, perspectives, and impacts. Therefore, finding an effective way to represent these multi-dimensional events is crucial for understanding and analysis.表达多维事件可能会很复杂和具有挑战性。
事件的发展往往涉及各种因素,观点和影响。
因此,寻找有效地表达这些多维事件的方法对于理解和分析至关重要。
One common method to represent multi-dimensional events is through the use of mind maps. Mind maps are visual representations of information that show the relationships between different ideas or concepts. By creating a mind map for a multi-dimensional event, one can visually see how different factors and perspectives are connected and interrelated.表示多维事件的一种常见方法是通过使用头脑图。
头脑图是信息的视觉表示,显示不同想法或概念之间的关系。
通过为多维事件创建一个头脑图,人们可以直观地看到不同因素和观点是如何相互联系和相关的。
Another method to represent multi-dimensional events is through the use of matrices. Matrices are mathematical structures that can be used to organize and display complex data in a structured manner. By creating a matrix for a multi-dimensional event, one can categorize different factors, perspectives, and impacts, allowing for a more systematic analysis and understanding of the event.另一种表示多维事件的方法是通过使用矩阵。
多粒度直觉二元语义的多准则群决策方法
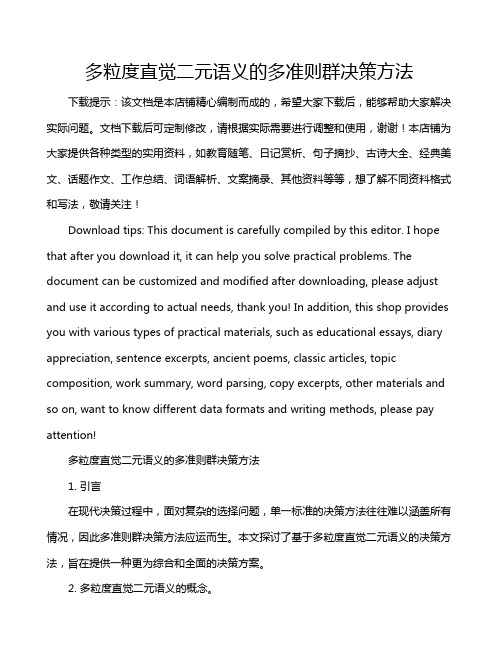
多粒度直觉二元语义的多准则群决策方法下载提示:该文档是本店铺精心编制而成的,希望大家下载后,能够帮助大家解决实际问题。
文档下载后可定制修改,请根据实际需要进行调整和使用,谢谢!本店铺为大家提供各种类型的实用资料,如教育随笔、日记赏析、句子摘抄、古诗大全、经典美文、话题作文、工作总结、词语解析、文案摘录、其他资料等等,想了解不同资料格式和写法,敬请关注!Download tips: This document is carefully compiled by this editor. I hope that after you download it, it can help you solve practical problems. The document can be customized and modified after downloading, please adjust and use it according to actual needs, thank you! In addition, this shop provides you with various types of practical materials, such as educational essays, diary appreciation, sentence excerpts, ancient poems, classic articles, topic composition, work summary, word parsing, copy excerpts, other materials and so on, want to know different data formats and writing methods, please pay attention!多粒度直觉二元语义的多准则群决策方法1. 引言在现代决策过程中,面对复杂的选择问题,单一标准的决策方法往往难以涵盖所有情况,因此多准则群决策方法应运而生。
多维状态无聊量表在中国和加拿大大学生中的因素等值性研究

语言学Categorization宋

Categorization
Definition:
Categorization is the process of classifying our experiences into different categories based on commonalities and differences. Categorization is a major ingredient in the creation of human knowledge, and it allows us to relate present experiences to past one. 范畴化是基于人类经验的异同将我们的经验 划分为不同的类型
2.They are much less informative relative to their immediate superior category.(它们的信息相对比他们的中间 层次上位范畴少)
3.They are frequently polymorphemic, the most common pattern being modifier-head.(它们是多词素性的,而最普遍 的格式是修饰—中心语结构)
Dogs
Dogs are different, but they still share enough to be distinguished from cats, birds, snakes, primates, etc..
Characteristics of Basic level:
基于用户潜在状态及依赖关系学习的时序行为推荐
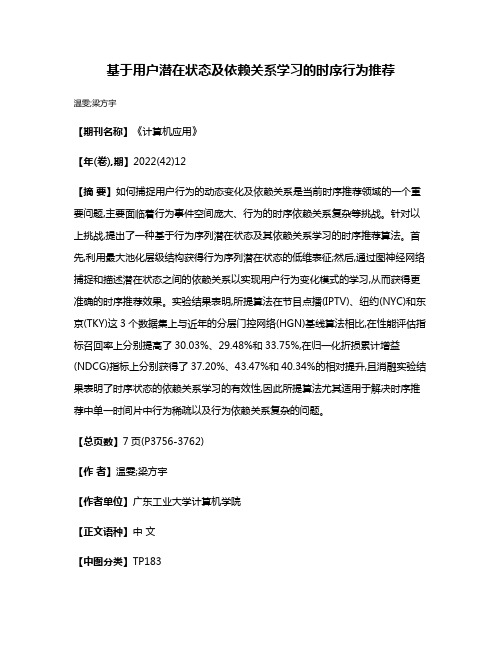
基于用户潜在状态及依赖关系学习的时序行为推荐
温雯;梁方宇
【期刊名称】《计算机应用》
【年(卷),期】2022(42)12
【摘要】如何捕捉用户行为的动态变化及依赖关系是当前时序推荐领域的一个重要问题,主要面临着行为事件空间庞大、行为的时序依赖关系复杂等挑战。
针对以上挑战,提出了一种基于行为序列潜在状态及其依赖关系学习的时序推荐算法。
首先,利用最大池化层级结构获得行为序列潜在状态的低维表征;然后,通过图神经网络捕捉和描述潜在状态之间的依赖关系以实现用户行为变化模式的学习,从而获得更准确的时序推荐效果。
实验结果表明,所提算法在节目点播(IPTV)、纽约(NYC)和东京(TKY)这3个数据集上与近年的分层门控网络(HGN)基线算法相比,在性能评估指标召回率上分别提高了30.03%、29.48%和33.75%,在归一化折损累计增益(NDCG)指标上分别获得了37.20%、43.47%和40.34%的相对提升,且消融实验结果表明了时序状态的依赖关系学习的有效性,因此所提算法尤其适用于解决时序推荐中单一时间片中行为稀疏以及行为依赖关系复杂的问题。
【总页数】7页(P3756-3762)
【作者】温雯;梁方宇
【作者单位】广东工业大学计算机学院
【正文语种】中文
【中图分类】TP183
【相关文献】
1.学习系统中基于用户行为分析的推荐算法研究
2.基于用户行为的产品相似性推荐模型及用户、产品标签化推荐模型
3.基于深度学习的用户行为推荐方法研究
4.融合学习者时序行为和认知水平的个性化学习资源推荐算法
5.面向群组用户时序行为的动态推荐算法
因版权原因,仅展示原文概要,查看原文内容请购买。
- 1、下载文档前请自行甄别文档内容的完整性,平台不提供额外的编辑、内容补充、找答案等附加服务。
- 2、"仅部分预览"的文档,不可在线预览部分如存在完整性等问题,可反馈申请退款(可完整预览的文档不适用该条件!)。
- 3、如文档侵犯您的权益,请联系客服反馈,我们会尽快为您处理(人工客服工作时间:9:00-18:30)。
a r X i v :0809.0250v 1 [p h y s i c s .s o c -p h ] 1 S e p 2008EPJ manuscript No.(will be inserted by the editor)Multiscaling behavior in the volatility return intervals of Chinese indicesFei Ren 1,2,a and Wei-Xing Zhou 1,2,3,4,b1School of Business,East China University of Science and Technology,Shanghai 200237,China2Research Center for Econophysics,East China University of Science and Technology,Shanghai 200237,China 3School of Science,East China University of Science and Technology,Shanghai 200237,China4Research Center of Systems Engineering,East China University of Science and Technology,Shanghai 200237,ChinaReceived September 1,2008/Revised version:Abstract.We investigate the probability distribution of the return intervals τbetween successive 1-min volatilities of two Chinese indices exceeding a certain threshold q .The Kolmogorov-Smirnov (KS)tests show that the two in-dices exhibit multiscaling behavior in the distribution of τ,which follows a stretched exponential form f q (τ/ τ )∼e −a (τ/ τ )γwith different correlation exponent γfor different threshold q ,where τ is the mean return interval cor-responding to a certain value of q .An extended self-similarity analysis of the moments provides further evidence of multiscaling in the return intervals.PACS.89.65.Gh Economics;econophysics,financial markets,business and management –89.75.Da Systems obeying scaling laws –05.45.Tp Time series analysis1IntroductionThe analysis of the waiting time between two successive events is helpful to understand the dynamics of stock markets,which has drawn much attention.A variety of waiting time variables have been raised by different definitions of event to characterize the stock markets from different view angles,such as the per-sistence probability [1,2,3],the exit time [4,5,6,7,8,9],and the intertrade duration [10,11,12,13].Recently the return intervals between successive extreme events exceeding a certain thresh-old q have been investigated for numerous complex systems,including rainfalls,floods,temperatures and earthquakes [14,15,16,17,18].Similar analysis was subsequently carried out concerning the volatility return intervals,which are defined as the waiting times between successive volatilities exceeding a certain threshold in stock markets.Yamasaki et al.and Wang et ed the daily data and in-traday data of US stocks to study the properties of the volatility return intervals [19,20,21,22].They found that the distribution of return intervals τbetween successive volatilities greater than a certain threshold q showed scaling behavior.This scaling be-havior is expected to be of great importance for the risk assess-ment of large price fluctuations.Similar scaling behavior was observed in the return intervals of daily and 1-min volatilities of thousands Japanese stocks [23].Qiu,Guo and Chen analyzed the high-frequency intraday data of four liquid stocks traded in the emerging Chinese market,and found that the returninterval2Fei Ren,Wei-Xing Zhou:Multiscaling behavior in volatility return intervals of Chinese indicesThe paper is organized as follows.In Section2,we explain the database analyzed and how the volatility return intervals are calculated.Section 3examines the scaling behavior and the curve fitting of the return interval distributions using the KS tests.In Section 4,we further study the multiscaling behavior by analyzing the moments of the scaled return intervals.Sec-tion 5concludes.2Preprocessing the data setsOur analysis is based on the high-frequency intraday data of two Chinese indices,the Shanghai Stock Exchange Composite Index (SSEC)and the Shenzhen Stock Exchange Composite Index (SZCI).Each composite index is constructed based on all the stocks listed on the corresponding exchange.The indices are recorded every six to eight seconds from January 2004to June 2006.We define the volatility as the magnitude of loga-rithmic index return between two consecutive minutes,that is R (t )=|ln Y (t )−ln Y (t −1)|,where the index Y is the closest tick to a minute mark.Thus the sampling time is one minute,and the volatility data size is about 140,000.The intraday volatilities of both indices exhibit a L-shaped intraday pattern [30],similar to the individual stocks [30,29].When dealing with intraday data,this pattern should be re-moved [20,21,22,26,24].Otherwise,the return intervals dis-tribution will exhibit daily periodicity for large thresholds.The intraday pattern A (s )is defined asA (s )=1A (s ).(2)Then we normalize the volatility by dividing its standard devi-ationv (t )=R ′(t )τf q (τ/ τ ),(4)where τ is the mean return interval that depends on the thresh-old q .If the function f q (x )is independent of q ,there exists a universal function f (x )such that f q (x )=f (x )for differ-ent values of q .In other words,the probability distributions f q (τ/ τ )of the scaled return intervals collapse onto a single curve f (τ/ τ )and the return intervals exhibit scaling behav-ior.To investigate whether the return interval distributions of the two Chinese indices exhibit scaling behavior,we plot in Figure 1the empirical probability distributions f q (τ/ τ )=P q (τ) τ as a function of the scaled return intervals τ/ τ for a wide range of threshold q =2,3,4,5.It is evident that the curves for different thresholds q show systematic deviations from each other and do not collapse onto a single curve,es-pecially for the Shanghai Composite Index.This indicates that the distributions of return intervals for both indices could not be approximated by a scaling relation.With the increase of the threshold q ,there are more large scaled return intervals and the distribution becomes broader.Fig.1.(Color online)Empirical probability distributions of scaled return intervals for different threshold q =2,3,4,5for the Shanghai Composite Index and the Shenzhen Composite Index.The solid curvesare the fitted functions ce −ax γwith the parameters listed in Table 2.Fei Ren,Wei-Xing Zhou:Multiscaling behavior in volatility return intervals of Chinese indices 3The observation that there is no scaling behavior in the volatility return interval distributions is consistent with there-sults of a previous study ofindividual Chinesestocks [29].TheKolmogorov-Smirnov test shows that only 12stocks out of 30most liquid Chinese stocks exhibit scaling behaviors in the re-turn interval distributions for different thresholds q ,while the other 18stocks do not show scaling behavior [29].3.2Kolmogorov-Smirnov test of scaling in return interval distributionsThe eyeballing of the probability distributions offers a qualita-tive way of distinguishing scaling and nonscaling behaviors.Here we further adopt a quantitative approach based on the Kolmogorov-Smirnov test.The standard KS test is designed to test the hypothesis that the distribution of the empirical data is equal to a particular distribution by comparing their cumulative distribution functions (CDFs).Our hypothesis is that the two return interval distributions for any two different q values do not differ at least in the common region of the scaled return in-tervals [23].Suppose that F q i is the CDF of return intervals for q i and F q j is the CDF of return intervals for q j ,where q i =q j .We calculate the KS statistic by comparing the two CDFs in the overlapping region:KS =max|F q i −F q j | ,q i =q j .(5)When the KS statistic is less than a critical value CV ,the hy-pothesis is accepted and we can assume that the distribution forq i is coincident with the distribution for q j .The critical value at the significance level of 5%is CV =1.36/q i q j KS CV 230.03210.0211240.07200.0296250.05350.0408340.04360.0329350.04440.0432450.04450.050620.802.050.590.800.673.510.470.0232.1314.200.380.631.5514.850.370.5440.925.790.430.581.7122.010.340.7251.0514.190.350.741.5625.060.330.85The p value could be regarded as the probability that the empirical distribution consists with its best fit.Consider the4Fei Ren,Wei-Xing Zhou:Multiscaling behavior in volatility return intervals of Chinese indicessignificance level of 1%.If the p -value of an index for a cer-tain threshold q is less than 1%,then the null hypothesis that the empirical PDF of this index can be wellfitted by a stretched ex-ponential is rejected.According to Table 2,the null hypotheses for all the q values are accepted for both two Chinese indices.It is noteworthy to point out that the p -values for all the q values (except for q =2for the Shenzhen Composite Index)are very large,implying high goodness-of-fit of the stretched exponen-tial to the empirical PDFs.At the significance level of 5%,the stretched exponential is rejected when q =2for the Shenzhen Composite Index.To show how good the stretched exponen-tial fits the data,we illustrate in Figure 1the fitted stretched exponential with the parameters listed in Table 2.It is obvi-ous that the empirical PDFs could be well fitted by a stretched exponential.In principle,the stretched exponential fits the em-pirical PDF better when the p -value is larger.For instance,the stretched exponential fits the empirical PDF for the Shanghai Composite Index better than the Shenzhen Composite Index when q =2.According to Table 2,the parameters differ from one an-other,providing further evidence supporting our conclusion that the return interval distributions do not have a scaling form.On average,the exponent γdecreases with increasing threshold q ,which is in line with the US stocks [27].4Moments of scaled return intervalsThe distributions of return intervals exhibit multiscaling behav-ior and show a systematic tendency with the threshold q .To fur-ther study this tendency of the interval distribution with q ,we compute the moments of the scaled return intervals x =τ/ τ defined asµm = (τ/ τ )m 1/m = ∞0x mf q (x )dx 1/m.(9)where the mean interval τ is dependent of the threshold q .When m =1,we have µ1=1by definition,independent of q .If there is a scaling behavior that f q (x )=f (x ),the m -th moment µm is a univariate function of the order m and is in-dependent of any other variables including the threshold q and the mean return interval τ .On the contrary,the m -th moment µm is not constant with respect to τ for m =1,when there is no scaling in the return interval distributions.4.1Dependence of moment on mean return interval We first investigate the relation between µm and τ .To better quantify the dependence of µm on τ ,we calculate the mo-ments in a certain medium range of τ to avoid the finite size effect and discreteness effect [26].Figure 2illustrates the mo-ments µm for m =0.25,0.5,1.5,2.0versus τ for the two Chinese indices.We investigate µm for a range of τ corre-sponding to 1≤q ≤5.Each curve of the moments µm sig-nificantly deviate from a horizontal line,confirming the multi-scaling behavior in the return interval distributions.The mo-ment function µm decreases with the increase of τ when m <1,and shows an increasing tendency with the increaseof τ when m >1.These two types of moment functions are delimited by the horizontal line µ1=1.Fig.2.(Color online)Moment µm vs τ for the two Chinese indices:Shanghai Composite Index and Shenzhen Composite Index.For each data set,four moments m =0.25,0.5,1.5,2.0are presented.Take a careful look at µm in Figure 2,for m <1(m >1)µm first decreases (increases)rapidly with the increase of τ ,and then starts to decrease (increase)relatively slowly at τ =10.The discreteness of the records of τresponds to the rapid increase (decrease)of µm for small τ ( τ <10).The moment for extremely large τ ,i.e., τ >100( τ is in units of standard deviations),will increase (decrease)for m <1(m >1)due to the finite size effect.We choose to study µm in a medium region 10< τ <100where in the effects of finite size and discreteness are small and µm shows a clear power-law-like trend with τ .We use a power law to fit the moment in this medium region,µm ∼ τ α.(10)If the PDF of return intervals follow a scaling form,µm is inde-pendent of τ according to Eq.(9)and the exponent αshould be some value very close to 0.If the exponent αis significantly different from 0,it implies that the PDF of return intervals may show multiscaling behavior.Fei Ren,Wei-Xing Zhou:Multiscaling behavior in volatility return intervals of Chinese indices 5Figure 3plots the exponent αas a function of order m for the two Chinese indices.This figure shows that the exponent αdiffers from 0in a systematic fashion.The exponents for the two indices are very close to each other when m is small.For m <1,αis negative.The exponent αincreases with m when m <3and decreases afterwards owning to the finite size effect.For large order m ,the αvalue for the Shenzhen Com-posite Index is greater than that for the Shanghai Composite Index.This implies that large τ tends to occur with greater probability for the Shenzhen Composite Index than the Shang-hai Composite Index,since large τ contributes more for high order µm .Fig.3.(Color online)Exponent αof µm in the region 10< τ <100for the two Chinese indices:Shanghai Composite Index and Shen-zhen Composite Index presented by circles and squares respectively.The above analysis according to Eq.(10)can be related to the extended self-similarity (ESS)analysis [33],which readsτm ∼ τnξ(m,n ).(11)If the generalized variable of µmµm,n =ττn 1/n(12)scales asµm,n ∼τn 1/nα(13)together with Eq.(11),we have(α+1)/n =ξ(m,n )/m.(14)If the return interval distribution can be scaled as followsP q (τ)=1τn 1/n,(15)we obtain thatξ(m,n )=m/n.(16)In this case,we haveα=0.(17)In other words,µm,n is independent of τn 1/n .Our empirical test focuses on the case that n =1.This ESS framework was also used to investigate scaling in the exit times in turbulence [34]and intertrade durations [35].According to Eq.(14)α(m )=ξ(m,1)/m −1.(18)Since ξ(1,1)=1,we have α(1)=0when m =1.This is wellverified by Figure 3.4.2Dependence of moment on order mThe moment µm not only display a significant dependence on τ ,but also shows a systematic tendency with m as shown in Figure 2.It is interesting to investigate the relation between µm and m directly.For a fixed τ ,one can study the moment of τof various orders m .If the return interval distribution strictly obeys a scaling form,µm should not depend on τ ,and the µm curves for different τ should all collapse onto a single curve.We plot in Figure 4(a)the moment µm as a function of m for fixed τ =10,30,100corresponding to q =2.0,3.2,4.8for the Shenzhen Composite Index.One sees the curves for dif-ferent τ exhibit substantial deviations from a single curve,Fig.4.(Color online)(a)Moment µm vs m for Shenzhen Composite Index for τ =10,30,100corresponding to q =2.0,3.2,4.8,pre-sented by circles,squares,triangles respectively.(b)Analytical mo-ments obtained from the stretched exponential distributions with pa-rameters fitted from empirical data of Shenzhen Composite Index for q =2,3,5.6Fei Ren,Wei-Xing Zhou:Multiscaling behavior in volatility return intervals of Chinese indices which demonstrates the multiscaling behavior of return inter-vals.For small m(m<1),µm decreases with the increase ofτ ,while for big m(m>1)µm tends to increase with theincrease of τ .This is not difficult to understand since smallτdominatesµm for small order m and largeτdominatesµm forlarge order m.The situation for the Shanghai Composite Indexis very similar.We have demonstrated that the return interval distributionfollows a stretched exponential form with different parametersfor various thresholds q in Section3.3.For the data perfectlyfollow a stretched exponential distribution,we can calculatethe analytical result of the momentµm by substituting Eq.(6)to Eq.(9)and considering the normalization condition of prob-ability density.It follows immediately that[26]µ=1Γ(1/γ)1/m.(19)In Figure4(b),the analytical curves ofµm versus m for three stretched exponential distributionsfitted from empirical data of Shenzhen Composite Index for q=2,3,5are plotted.As one can see that the analytical results are similar to that of the em-pirical data,which supports the multiscaling of the empirical return intervals.5Summary and conclusionsWe have studied the multiscaling properties of the distributions of volatility return intervals for two Chinese indices,together with their moments.The Kolmogorov-Smirnov test is adopted to examine the scaling behavior of the return interval distribu-tions as well as the particular form of the distribution.Wefind that the return intervals of the two indices exhibit multiscal-ing behaviors,and their distributions for different thresholds q can be well approximated by stretched exponential functions f q(x)∼e−axγbut with different values of the correlation ex-ponentγ.An ESS-like moment analysis confirms the existence of multiscaling rather than monoscaling.This result is consis-tent with previous analysis on individual Chinese stocks[29] and help us better understand the properties of volatility return intervals in the Chinese stock markets.This work was partially supported by the Shanghai Educational De-velopment Foundation(No.2008CG37),the National Natural Science Foundation of China(No.70501011),the Fok Ying Tong Education Foundation(No.101086),and the Program for New Century Excel-lent Talents in University(No.NCET-07-0288).References1. B.Zheng,Mod.Phys.Lett.B16,775(2002)2. F.Ren,B.Zheng,Phys.Lett.A313,312(2003)3. F.Ren,B.Zheng,H.Lin,L.Wen,S.Trimper,Physica A350,439(2005)4.I.Simonsen,M.H.Jensen,A.Johansen,Eur.Phys.J.B27,583(2002)5.M.H.Jensen,A.Johansen,I.Simonsen,Int.J.Modern Phys.B17,4003(2003)6.M.H.Jensen,A.Johansen,I.Simonsen,Physica A324,338(2003)7.W.X.Zhou,W.K.Yuan,Physica A353,433(2005)8.M.Załuska-Kotur,K.Karpio, A.Orłowski,Acta PhysicaPolonica B37,3187(2006)9.K.Karpio,M.A.Załuska-Kotur,A.Orłowski,Physica A375,599(2007)10. E.Scalas,R.Gorenflo,H.Luckock,F.Mainardi,M.Mantelli,M.Raberto,Quant.Financ.4,695(2004)11.P.C.Ivanov,A.Yuen,B.Podobnik,Y.K.Lee,Phys.Rev.E69,056107(2004)12.N.Sazuka,Physica A376,500(2007)13.Z.Q.Jiang,W.Chen,W.X.Zhou,Physica A387,in press(2008)14. F.Schmitt,C.Nicolis,Fractals10,285(2002)15. A.Bunde,J.F.Eichner,S.Havlin,J.W.Kantelhardt,Physica A330,1(2003)16. A.Bunde,J.F.Eichner,S.Havlin,J.W.Kantelhardt,Physica A342,308(2004)17. A.Bunde,J.F.Eichner,J.W.Kantelhardt,S.Havlin,Phys.Rev.Lett.94,048701(2005)18. A.Saichev,D.Sornette,Phys.Rev.Lett.97,078501(2006)19.K.Yamasaki,L.Muchnik,S.Havlin,A.Bunde,H.E.Stanley,A102,9424(2005)20. F.Z.Wang,K.Yamasaki,S.Havlin,H.E.Stanley,Phys.Rev.E73,026117(2006)21. F.Wang,P.Weber,K.Yamasaki,H.S.,H.E.Stanley,Eur.Phys.J.B55,123C133(2007)22.I.V odenska-Chitkushev, F.Z.Wang,P.Weber,K.Yamasaki,S.Havlin,H.E.Stanley,Eur.Phys.J.B61,217(2008)23.W.S.Jung,F.Z.Wang,S.Havlin,T.Kaizoji,H.T.Moon,H.E.Stanley,Eur.Phys.J.B62,113(2008)24.T.Qiu,L.Guo,G.Chen(2008),arXiv:0805.219425.J.W.Lee,K.E.Lee,P.A.Rikvold,J.Korean Phys.Soc.48,S123(2006)26. F.Z.Wang,K.Yamasaki,S.Havlin,H.E.Stanley,Phys.Rev.E77,016109(2008)27. F.Z.Wang,K.Yamasaki,S.Havlin,H.E.Stanley(2008),arXiv:0808.320028.Z.Q.Jiang,L.Guo,W.X.Zhou,Eur.Phys.J.B57,347(2007)29. F.Ren,L.Guo,W.X.Zhou(2008),arXiv:0807.181830.X.H.Ni,W.X.Zhou(2007),arXiv:0710.240231. A.Clauset, C.R.Shalizi,M.E.J.Newman(2007),arXiv:0706.106232.M.C.Gonzalez,C.A.Hidalgo,A.L.Barab´a si,Nature453,779(2008)33.R.Benzi,S.Ciliberto,R.Tripiccione,C.Baudet,F.Massaioli,S.Succi,Phys.Rev.E48,R29(1993)34.W.X.Zhou,D.Sornette,W.K.Yuan,Physica D214,55(2006)35.Z.Eisler,J.Kert´e sz,Eur.Phys.J.B51,145(2006)。