Hierarchical organization of modularity in metabolic networks
叶绿体与蓝藻代谢网络的比较分析及进化研究
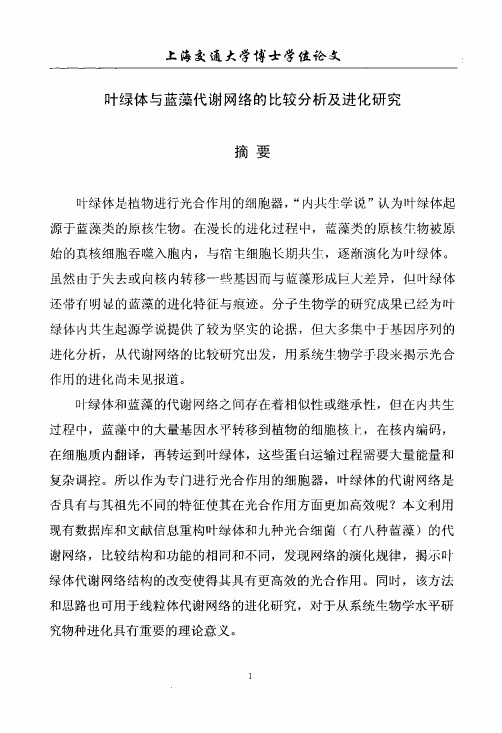
日常生活和食物营养上的100倍…1。
光合作用从根本上改变了地球上的生活环境。
根据绿色植物每年同化1750亿吨碳素计算,每年从光合作用中可以放出大约4700亿吨的游离念的氧气,这样就把原先没有氧的地面环境改变成有氧的环境。
需氧生物出现的先决条件就是光合作用,这对于地球上生物界的进化和发展具有极大的意义。
2.2.2叶绿体的结构叶绿体是植物细胞所特有的能量转换细胞器,其主要功能是进行光合作用。
大多数高等植物的叶肉细胞一般含有50--200个叶绿体,可占细胞质体积的40%,叶绿体的数目因物种、细胞种类和生理状况而不同。
叶绿体由叶绿体膜(chloroplastmembrane或称外被outerenvelope)、类囊体(thylakoid)和基质(stroma)三部分构成。
叶绿体含有三种不同的膜(外膜,内膜和类囊体膜)和三种彼此分隔的空隙(膜空隙、基质和类囊体腔),如图2-1所示m1。
图2.1叶绿体的形态结构图唧1371Fig.2-1Theshapeandstructureofchloroplast17图2-2非循环式光合磷酸化旧mFig.2-2Non-cyclicphotophosphorylation球图2-3循环式光合磷酸化唧F-g.2-3CyclicphotophosphoTyhtion”光合作用[49-52]o图2.7给出了蓝藻与拟南芥之间的基因转移关系以及这些转移来的基因在拟南芥细胞孩和叶绿体中的定位瞄01。
图2-7蓝藻与拟南芥之间的基因转移关系㈨Fig.2-7Genetransferbetweencyanobacteriaandarabidopsisthaliana【酬2.4水平基因转移水平基因转移(horizontalgenetransfer,HGn,又称横向基因转移(1ateralgenetransfer,LGT),是指在差异生物个体之间,或单个细胞内部细胞器之间所进行的遗传物质的交流。
德国最大科研机构亥姆霍兹联合会的演化史
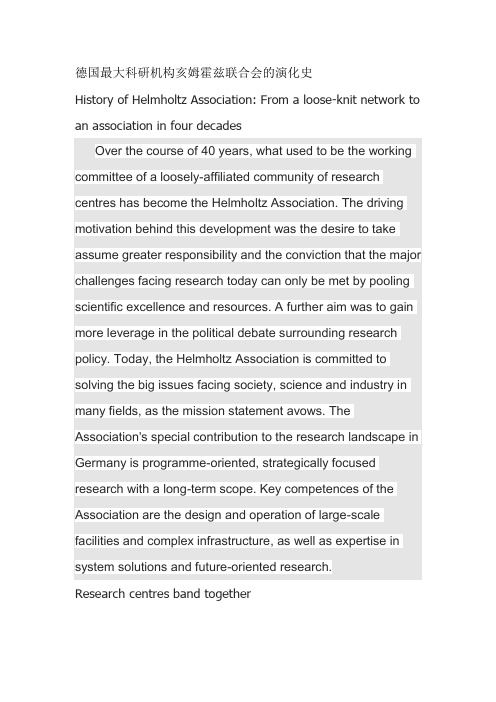
德国最大科研机构亥姆霍兹联合会的演化史History of Helmholtz Association: From a loose-knit network to an association in four decadesOver the course of 40 years, what used to be the working committee of a loosely-affiliated community of research centres has become the Helmholtz Association. The driving motivation behind this development was the desire to take assume greater responsibility and the conviction that the major challenges facing research today can only be met by pooling scientific excellence and resources. A further aim was to gain more leverage in the political debate surrounding research policy. Today, the Helmholtz Association is committed to solving the big issues facing society, science and industry in many fields, as the mission statement avows. The Association's special contribution to the research landscape in Germany is programme-oriented, strategically focused research with a long-term scope. Key competences of the Association are the design and operation of large-scale facilities and complex infrastructure, as well as expertise in system solutions and future-oriented research.Research centres band togetherIn order to accomplish the first great task - developing safe and powerful nuclear reactors - representatives from the Karlsruhe and Jülich research centres, which were still in the process of being established, met in 1958 with the former Society for Nuclear Energy Management in Shipbuilding and Shipping Navigation (known today as Helmholtz-Zentrum Geesthacht Centre for Materials and Coastal Research) and the nuclear research institutes of a number of universities to form the "Working committee for administrative and operational affairs in German reactor control stations". The former Hahn Meitner Institute for Nuclear Research in Berlin joined the committee in the following year, 1959.Initially the committee focused on the exchange of experiences related to operational and safety issues, but soon the focus was expanded to include topics that still concern the large research centres today.. These involve questions of strategic orientation, training, remuneration and patent management, which are all of great importance for the advancement of excellent research and its practical and commercial application. It is for this reason that research centres involved in other scientific fields, but dedicated to solving similar problems joined the Association in the 1960s.These were the Deutsches Elektronen Synchroton (DESY), the Radiation Research Society (now the Helmholtz Zentrum München - German Research Center for Environmental Health), the Max Planck Institute of Plasma Physics, the German Test and Research Institute for Aviation and Space Flight (now the German Aerospace Center), the former Society for Mathematics and Data Processing (part of the Fraunhofer Institute today) and the Society for Heavy Ion Research (GSI).The articles of association and a new structure legitimised this grouping together of research associations, with the aim of avoiding bureaucratic micromanagement andover-interference in research. Today, the government's role in formulating research policy guidelines and the Association's mission to address the big issues facing, science and industry are more clearly defined.The Association's relationship to the German government is definedAt the beginning of 1970, representatives of the research centres formed the Arbeitsgemeinschaft derGroßforschungseinrichtungen" (syndicate of large-scale research institutes), or AGF, and formulated the guidelines that would govern their relationship to the government. Working ina spirit of partnership, the AGF aimed to define the basic tasks the Association would concern itself with; implementing the guidelines would be the responsibility of the individual research centres.The German Cancer Research Centre joined the AGF in 1975, followed a year later by the German Research Centre for Biotechnology (GBF ), now renamed the Helmholtz Centre for Infection Research). In 1983, the Alfred Wegener Institute for Polar and Marine Research became a member. The Association saw further growth as a result of German reunification, when research centres in the new federal states - including the GFZ Geosciences Research Centre in Potsdam, the Max Delbrück Center for Molecular Medicine and the former Leipzig-Halle Centre for Environmental Research (known today as the Helmholtz Centre for Environmental Research, or UFZ) - were admitted as members.Strength and excellence through integration and initiative In 1995, the AGF became the Helmholtz Association of German Research Centres. The research institutes came one step closer to achieving their aim of freedom to govern their own affairs. Since then, a senior council has provided recommendations on major issues relating to content andstructure, such as the practice of appointing professors jointly with universities, principles of the appraisal procedure and research priorities.2001 was a significant milestone in the restructuring of the Association. The loose affiliation was transformed into a registered association with legally independent member centres. The core of the reform with regard to content was the introduction of the programme-oriented advancement of funding, which involved a switch from centre-oriented financing, in which each centre administered its own budget, to superordinate financing at the level of the Association. A total of 30 cross-centre research programmes have been drafted, which are evaluated in detail by internationally-renowned experts with regard to their strategic relevance and scientific excellence. The Helmholtz Association is the only research organisation in recent years to have initiated comparable reforms that have resulted in the reorientation of research efforts towards strategic goals.The reform also gave the Association a full-time president. With the Initiative and Networking Fund, he has an effective tool at his disposal for setting strategic priorities, as well as for promoting scientific excellence and advancing research in keyfields with the required critical mass. The strategically-oriented Initiative and Networking Fund has established an incentive scheme that promotes scientific competition. Along with other funding schemes, it also supports the goals of the Helmholtz Association within the scope of the Pact for Research and Innovation. In concentrating on excellence, new forms of cooperation and networking, promoting the young scientific community and the development of new approaches to further innovation, the Association contribute to economic growth and prosperity in our society.Following the amendment of the articles of association at the end of 2006, each of the Association's different fields of research is represented by a vice president in the newly established executive committee; in addition, there are two vice presidents with administrative duties.The reforms in recent years set the course for the Helmholtz Association to become one of the most important and highly-regarded scientific organisations in Germany today.The Helmholtz-Zentrum Berlin für Materialien und Energie was created on 1 January 2009 when the formerHahn-Meitner-Institut Berlin (HMI) merged with another Berlininstitute, the Elektronenspeicherring-Gesellschaft für Synchrotronstrahlung (BESSY).The newly founded German Center for Neurodegenerative Diseases (DZNE) has been a member of the Helmholtz Association since 30 April 2009. The 16th Research Centre to join, the DZNE is unique in two ways. Firstly, it is not limited to a single location; instead it is made up of a strong interdisciplinary network consisting of the core facility in Bonn and (currently) six outstanding partner locations inRostock/Greifswald, Magdeburg, Göttingen, Witten-Herdecke, Tübingen and Munich. Secondly, unlike other European research institutes in the field, the DZNE does not focus on basic research alone, but also translates research findings into practice. On 1 January 2011 the Forschungszentrum Dresden-Rossendorf (FZD) became the 17th member of the Association, under the new name Helmholtz-Zentrum Dresden Rossendorf (HZDR). A year later on 1 January 2012 the former Leibniz Institute of Marine Sciences (IFM-GEOMAR) converted into GEOMAR | Helmholtz Centre for Ocean Research Kiel due to the transition of the institute into the Helmholtz Association.。
OrganizationStructure组织结构

Integration is achieve through structural mechanisms that enhance collaboration and coordination. Any job activity that links different work units performs an integrative function. Remember, the more highly differentiated your firm, the greater the need for integration among the different units.一体化可以通过促进合作和协调的机制实现。任何一项连接不同工作单位的行动都执行着一体化的职能。
The numerous tasks that must be carried out in an organization make specialization and division of labor necessities. Otherwise the complexity of the overall work of the organization would be too much for any individual.在组织中需要完成众多不同的任务,从而使专业化和劳动分工成为必然,要不然,组织中工作的复杂性是任何个人都难以全部胜任的。Differentiation is high when there are many subunits and many kinds of specialists who think differently.当下属单位数量较多,众多专家的思维方式不同时,差异化程度较高。
Secretaries and accountants specialize in, and perform, different jobs; similarly, marketing, finance, and human resources tasks are divided among those respective departments.秘书和会计具有不同的专长因而从事不同的工作;与此类似,营销、财务、和人力资源工作被划分为不同的部门。
标准文献分类法ics
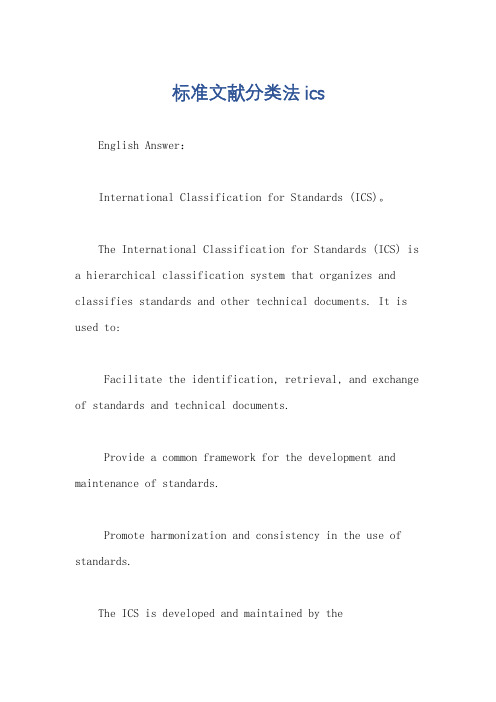
标准文献分类法icsEnglish Answer:International Classification for Standards (ICS)。
The International Classification for Standards (ICS) is a hierarchical classification system that organizes and classifies standards and other technical documents. It is used to:Facilitate the identification, retrieval, and exchange of standards and technical documents.Provide a common framework for the development and maintenance of standards.Promote harmonization and consistency in the use of standards.The ICS is developed and maintained by theInternational Organization for Standardization (ISO) and the International Electrotechnical Commission (IEC). It is based on the following principles:Hierarchy: The ICS is a hierarchical structure, with each level of the hierarchy representing a broader or more general category.Modularity: The ICS is a modular system, with each module representing a specific subject area.Flexibility: The ICS is flexible enough to accommodate new technologies and developments.The ICS is divided into three levels:Level 1: The first level of the ICS is divided into 40 broad subject areas, such as agriculture, construction, and healthcare.Level 2: The second level of the ICS is divided into 140 sub-areas, such as crop production, buildingconstruction, and medical equipment.Level 3: The third level of the ICS is divided into over 6,000 groups, such as specific types of crops, building materials, and medical devices.Each level of the ICS is further divided into sub-groups and sections, allowing for a very specific and detailed classification of standards.The ICS is used by a wide range of organizations, including:Standards development organizations.Technical committees.Testing and certification bodies.Manufacturers.Consumers.Regulatory authorities.The ICS helps these organizations to identify and access the standards that they need, and to ensure that the standards they develop are consistent with other standards in the same field.Chinese Answer:国际标准分类法 (ICS)。
报告人: 林 苑指导老师:章忠志 副教授复旦大学2010730
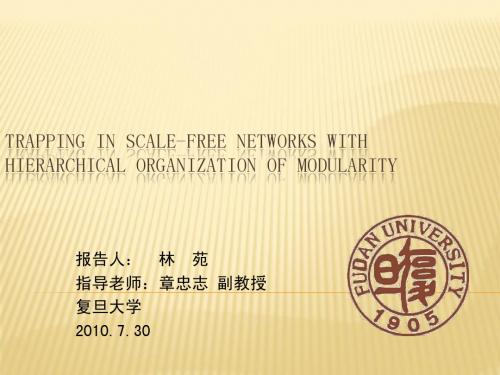
The new external nodes are composed of all the peripheral nodes of M-1 copies of
Hg-1.
FORMULATION OF THE TRAPPING PROBLEM
Xi
First-passage
time (FPT)
Trapping in scale-free networks with hierarchical organization of modularity, Zhang Zhongzhi, Lin Yuan, et al. Physical Review E, 2009, 80: 051120.
Markov chain
aiv F ( X i t) F ( X v t 1) v 1 d i ( g )
Ng
FORMULATION OF THE TRAPPING PROBLEM
Define a generating function
F i ( z) F ( X i t ) z t
GENERIC APPROACH
Markov Chain
Laplacian
matrix
Generating Function
MEASURES
Mean transit time
Tij
≠ Tji
Tij Tii Cij
Mean return time
Cij
Mean commute time
MODULAR SCALE-FREE NETWORKS
We denote by Hg the network model after g iterations. For g>1,
公司等级结构

Advantages of Hierarchical Structure:
1 Using a hierarchical structure establishes clear authority for work and departments. Employees recognize defined levels of leadership within the organization; authority and levels of responsibility are obvious. 2Opportunities for promotion motivat employees to perform well. employees have a clear path to move up the ladder if they want to earn more money and assume more responsibility. 3 Hierarchical structures promote developing employees as specialists. Employees may narrow their field of focus and become experts in specific functions. 4 The hierarchical structure creates clear lines of communication. Subordinates know whom to report to and where to get information and directives. This serves to unify the department, resulting in coordination(协调) between its members.
领导力、组织行为学、SWOT英文翻译

领导力、组织行为学、SWOT英文翻译领导力(Leadership)就是指在管辖的范围内充分的利用人力和客观条件在以最小的成本办成所需的事提高整个团体的办事效率。
Leadership is the accomplishment of a goal at minimum cost by making the best use of human resources and objective conditions in one’s jurisdiction, which improves the efficiency of a team。
组织行为学(Organizational Behavioural Science )所谓组织行为学是研究在组织中以及组织与环境相互作用中,人们从事工作的心理活动和行为的反应规律性的科学。
组织行为学综合运用了心理学、社会学、文化人类学、生理学、生物学,还有经济学、政治学等学科有关人的行为的知识与理论,来研究一定组织中的人的行为规律.近年来出版了很多与组织行为学有关并以此命名的书籍.Organizational Behavioural Science is an academic discipline concerned with researching the reaction regularity of people’s psychology and behaviour in an organisation or in the interaction between organisation and environment. Many academic disciplines related to human behaviour (including psychology,sociology, cultural anthropology,physiology, biology,economics and politics)are synthetically used in organizational behavioural science to research the reaction regularity in a certain organisation. In recent years, many publications related to organizational behavioural science appeared.SWOT是一种分析方法,用来确定企业本身的竞争优势,竞争劣势,机会和威胁,从而将公司的战略与公司内部资源、外部环境有机结合。
世界环保组织英文演讲

世界环保组织英文演讲Ladies and gentlemen,Distinguished guests,I stand before you today as the representative of the World Environmental Organization – an organization committed to protecting our planet and ensuring the sustainability of our future generations. Today, I want to address an issue that is crucial to our survival and well-being –environmental conservation.In recent decades, we have witnessed significant changes in our environment. Rising global temperatures, melting glaciers, deforestation, rising sea levels, and an alarming increase in carbon emissions are but a few of the many challenges we face. The detrimental consequences of these changes are evident in the loss of biodiversity, destruction of ecosystems, and the increasing frequency and intensity of natural disasters.The urgency to take immediate and decisive action has never been greater. We must unite as a global community to address these issues collectively, regardless of our differences. Environmental problems know no boundaries, and their effects will be felt by every individual on this planet.One of the areas that require our immediate attention is climate change. The scientific consensus is clear – human activity, particularly the burning of fossil fuels, is the primary driver of this phenomenon. The increasing concentrations of greenhouse gases in our atmosphere are trapping heat, resulting in a rise in global temperatures. This, in turn, leads to extreme weather events, including hurricanes, droughts, and heatwaves, threatening the lives and livelihoods of communities around the world.To combat climate change, we must transition to a low-carbon economy. This shift entails promoting renewable energy sources and investing in green technologies. By reducing our dependency on fossil fuels and embracing sustainable alternatives, we can not only mitigate the effects of climate change but also create new job opportunities and economic growth. Governments, businesses, and individuals all have a stake in this fight and must work together to implement sustainable practices and policies.Another critical aspect of environmental conservation is the preservation of our forests and oceans. Forests act as carbon sinks, absorbing large amounts of carbon dioxide and releasing oxygen. They are also crucial habitats for numerous species and sources of livelihood for millions of people. Therefore, we must prioritize reforestation efforts, combat illegal logging, and support sustainable forestry practices.Likewise, the health of our oceans is of paramount importance. They produce over half of the oxygen we breathe, regulate our climate, and provide food and livelihoods for countless communities. However, overfishing, pollution, and climate change are threatening their delicate balance. We must act swiftly to protect marine ecosystems by establishing marine protected areas, reducing plastic waste, and implementing sustainable fishing practices.Education and awareness are also key components of our efforts to conserve the environment. We must empower individuals with the knowledge and understanding of the importance of environmental conservation. By instilling a sense of responsibility and inspiring action, we can shape a new generation of environmentally conscious global citizens.Furthermore, international cooperation is vital in addressing environmental challenges. The World Environmental Organization is committed to facilitating partnerships between governments, NGOs, and businesses to exchange knowledge and resources. Together, we can address global issues, build resilience, and promote sustainable development.In closing, it is our collective responsibility to safeguard and preserve the environment for future generations. We must act now to ensure a sustainable and prosperous future for all. By making conscious choices and building a global community committed to environmental conservation, we can overcome any challenge and create a brighter and more sustainable future.Thank you.。
组织行为学双语课堂背诵要点
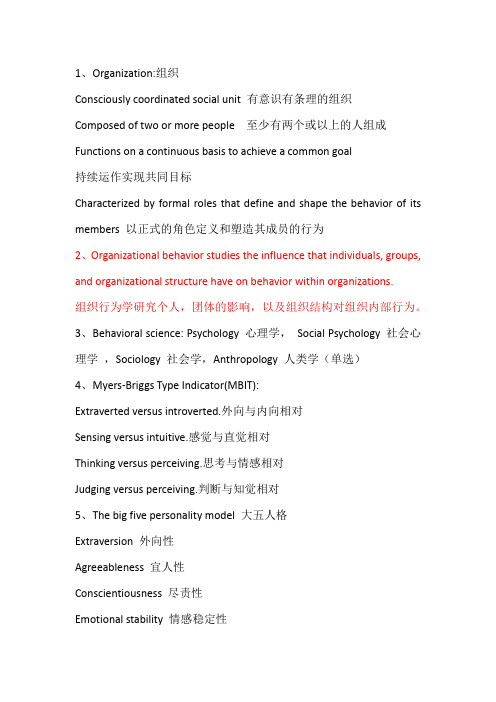
1、Organization:组织Consciously coordinated social unit 有意识有条理的组织Composed of two or more people 至少有两个或以上的人组成Functions on a continuous basis to achieve a common goal持续运作实现共同目标Characterized by formal roles that define and shape the behavior of its members以正式的角色定义和塑造其成员的行为2、Organizational behavior studies the influence that individuals, groups, and organizational structure have on behavior within organizations.组织行为学研究个人,团体的影响,以及组织结构对组织内部行为。
3、Behavioral science: Psychology 心理学,Social Psychology 社会心理学,Sociology 社会学,Anthropology 人类学(单选)4、Myers-Briggs Type Indicator(MBIT):Extraverted versus introverted.外向与内向相对Sensing versus intuitive.感觉与直觉相对Thinking versus perceiving.思考与情感相对Judging versus perceiving.判断与知觉相对5、The big five personality model 大五人格Extraversion 外向性Agreeableness 宜人性Conscientiousness 尽责性Emotional stability 情感稳定性Openness to experience 经验的开放性6、Values represent basic convictions that “a specific mode of conduct or end –state of existence is personally or socially preferable to an opposite or converse mode of conduct or end-state of existence.价值观代表基本的、稳定的信念系统,这个系统指“个人或者社会偏向某种具体的行为模式或者生存的终极目标,而不是队里的或者相反的行为模式或生存的终极目标。
2018翻硕考研:机构组织类英文缩写
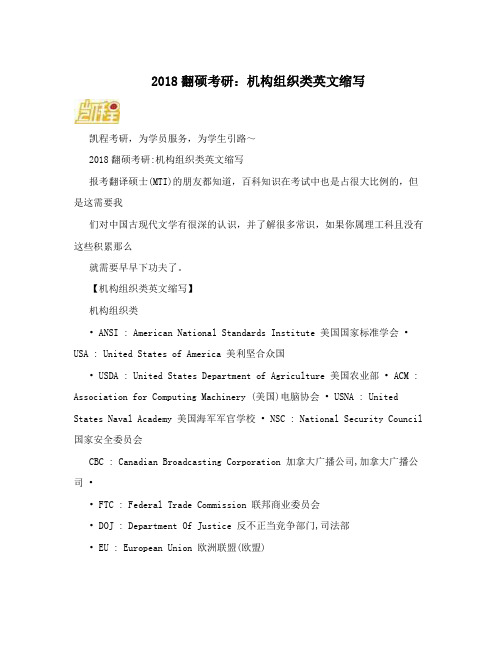
2018翻硕考研:机构组织类英文缩写凯程考研,为学员服务,为学生引路~2018翻硕考研:机构组织类英文缩写报考翻译硕士(MTI)的朋友都知道,百科知识在考试中也是占很大比例的,但是这需要我们对中国古现代文学有很深的认识,并了解很多常识,如果你属理工科且没有这些积累那么就需要早早下功夫了。
【机构组织类英文缩写】机构组织类• ANSI : American National Standards Institute 美国国家标准学会• USA : United States of America 美利坚合众国• USDA : United States Department of Agriculture 美国农业部• ACM : Association for Computing Machinery (美国)电脑协会• USNA : United States Naval Academy 美国海军军官学校• NSC : National Security Council 国家安全委员会CBC : Canadian Broadcasting Corporation 加拿大广播公司,加拿大广播公司•• FTC : Federal Trade Commission 联邦商业委员会• DOJ : Department Of Justice 反不正当竞争部门,司法部• EU : European Union 欧洲联盟(欧盟)• AAAS : American Association for the Advancement of Science 美国科学促进(协)会• BEA : Bureau of Economic Analysis 经济分析局• IEC : International Electrotechnical Commission 国际电子技术委员会• USMA : United States Military Academy 美国陆军军官学校• ACS : American Cancer Society 美国癌症学会• AMA : American Medical Association 美国医学协会• US : United States 美国• HMO : Health Maintenance Organization 卫生维护组织• EFTA : European Free Trade Association 欧洲自由贸易联盟• AP : Associated Press 美国联合通讯社,美联社,美联社• APA : American Psychological Association 美国心理学协会• WIPO : World Intellectual Property Organization 世界知识产权组织• NCIC : National Crime Information Center 国家犯罪信息中心/国家刑事犯罪情报中心• SPCA : Society for the Prevention of Cruelty to Animals 英国动物保护协会• ADA : American Dental Association 美国牙医学会• MS : Microsoft 微软• APL : Applied Physics Laboratory 应用物理试验室[美] • NASD : National Association of Securities Dealers 全国证券交易商协会• BBB : Better Business Bureau 商务改善协会• NGS : National Geographic Society 全国地理学会第 1 页共 1 页凯程考研,为学员服务,为学生引路~• who : world health organization 世界卫生组织• ABA : American Bar Association 美国律师协会• UN : United Nations 联合国• AKC : American Kennel Club 美国养狗爱好者俱乐部• VFD : Volunteer Fire Department 志愿消防队ABC : Australian Broadcasting Corporation 澳大利亚广播公司•• ACLU : American Civil Liberties Union 美国公民自由协会• BBC : British Broadcasting Corporation 英国广播公司• CNN : Cable News Network 美国有线电视台• ECMA : European Compu ter Manufacturers Association 欧洲计算机制造商协会• ASPCA : American Society for the Prevention of Cruelty to Animals 美国防止虐待动物协会• MADD : Mothers Against Drunk Driving 抗议司机酒后驾驶母亲协会• YMCA : Young Men's Christian Association 基督教青年会• BA : British Airways 英国航空公司• ECB : European Central Bank 欧洲中央银行• UPS : United Parcel Service 联合包裹快递• IDC : International Data Corporation 国际数据公司• NAS : National Academy of Sciences 国家科学院• SIG : Special Interest Group 共同利益组织• ASE : American Stock Exchange 美国证券交易所• AAA : Ame rican Automobile Association 美国汽车协会• AHL : American Hockey League 美国曲棍球联盟• EMU : European Monetary Union 欧洲货币联盟• ABA : American Bankers Association 美国银行家协会• ALA : American Library Association 美国图书馆协会• ARC : American Red Cross 美国红十字会• IATA : Intern ational Air Transport Association 国际航空运输协会• MLB : Major League Baseball 美国全国棒球协会和盟国棒球协会• NPR : National Public Radio 美国全国公共电台• AFL : American Federation of Labor 美国劳工联合会• CERT : Computer Emergency Response Team 计算机紧急情况反应小组• NBA : National Basketball Association (美国)国家篮球协会• NHL : National Hockey League 全国曲棍球联盟• APC : American Power Conversion 美国能源转换• IAFF : International Association of Fire Fighters 国际消防战斗员协会• NEA : National Education Association 全国教育协会• PBS : Public Broadcasting Service 公共广播事务局• IRC : International Red Cross 国际红十字会• NEA : National Endowment for the Arts 全国艺术捐赠基金会• GCC : Gulf Cooperation Council 海湾合作委员会• GM : General Motors 通用汽车公司(美国)• AA : American Airlines 美国美洲航空• WWF : World Wrestling Federation 世界摔角联盟第 2 页共 2 页凯程考研,为学员服务,为学生引路~• AMC : American Motors Corporation 美国汽车公司• AAL : American Airlines 美国航空公司• WTO : World Tourism Organization 世界旅游组织• DA : Department of the Army (美国)陆军部• OSS : Office of Strategic Services 战略情报局ASC : American Society of Cinematographers 美国电影摄影师学会•• NPF : National Park Foundation 国家公园基金会• AAA : Agricultural Adjustment Administration 美国家业调节局• ABA : American Booksellers Association 美国书商协会• ACU : Australian Catholic University 澳大利亚天主教大学• NOW : National Organization for Women (美国)全国妇女组织• HP : Hewlett-Packard 惠普公司• SFO : San Francisco 三藩市• AFI : American Film Institute 美国电影学会• CEC : Commission of the European Communities 欧盟委员会• LWV : League of Women Voters 女选民联盟• AR : Argentina 阿根廷• JP : Japan 日本• FEDEX : Federal Ex press 联邦快递• FHA : Federal Housing Administration 联邦住宅管理局• IRE :Ireland 爱尔兰• PE : Peru 秘鲁• CAB : Civil Aeronautics Board 民航局• IR : Iran 伊朗• SP : Spanish 西班牙• RFD : Rural Free Delivery 乡村免费邮递处• CAN : Canada 加拿大第 3 页共 3 页。
复杂网络上的社区发现算法研究
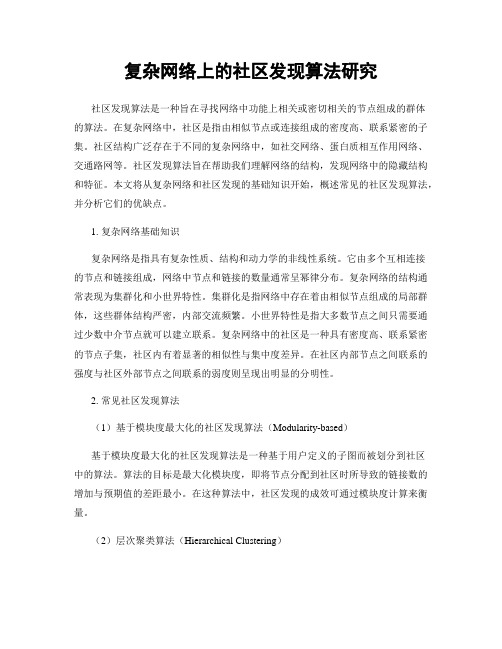
复杂网络上的社区发现算法研究社区发现算法是一种旨在寻找网络中功能上相关或密切相关的节点组成的群体的算法。
在复杂网络中,社区是指由相似节点或连接组成的密度高、联系紧密的子集。
社区结构广泛存在于不同的复杂网络中,如社交网络、蛋白质相互作用网络、交通路网等。
社区发现算法旨在帮助我们理解网络的结构,发现网络中的隐藏结构和特征。
本文将从复杂网络和社区发现的基础知识开始,概述常见的社区发现算法,并分析它们的优缺点。
1. 复杂网络基础知识复杂网络是指具有复杂性质、结构和动力学的非线性系统。
它由多个互相连接的节点和链接组成,网络中节点和链接的数量通常呈幂律分布。
复杂网络的结构通常表现为集群化和小世界特性。
集群化是指网络中存在着由相似节点组成的局部群体,这些群体结构严密,内部交流频繁。
小世界特性是指大多数节点之间只需要通过少数中介节点就可以建立联系。
复杂网络中的社区是一种具有密度高、联系紧密的节点子集,社区内有着显著的相似性与集中度差异。
在社区内部节点之间联系的强度与社区外部节点之间联系的弱度则呈现出明显的分明性。
2. 常见社区发现算法(1)基于模块度最大化的社区发现算法(Modularity-based)基于模块度最大化的社区发现算法是一种基于用户定义的子图而被划分到社区中的算法。
算法的目标是最大化模块度,即将节点分配到社区时所导致的链接数的增加与预期值的差距最小。
在这种算法中,社区发现的成效可通过模块度计算来衡量。
(2)层次聚类算法(Hierarchical Clustering)层次聚类算法是一种通过递归层次划分,并同时保留了局部和整体结构性质的算法。
聚类过程不断地从原始集群中剥离最小的聚类子集,形成一棵树状表示,其中根节点表示整个网络,叶节点则表示最小的聚类。
树状结构本身可用于挖掘不同尺度上的社区结构。
(3)基于Louvain算法的社区发现算法(Louvain-based)Louvain算法是一种快速发现社区结构的常见算法。
复杂网络中的社团发现算法综述

复杂网络中的社团发现算法综述随着社会网络的日益发达,社交网络成为了现代社会的重要组成部分。
然而,这些网络往往都是由大量的节点和边构成,而且具有非常复杂的拓扑结构。
对于这样的复杂网络,如何有效地发现其中的社团结构一直是研究的热点之一。
社团结构是指在网络中存在一些密度较高、连通性较强的子图,其中节点之间的联系比较紧密,而与其他社团的节点则联系较松散。
社团结构的发现可以帮助我们了解网络中的相互作用关系,为社交网络的数据挖掘和信息推荐提供基础理论和方法。
社团发现算法按照算法思想的不同,可以分为基于模型的方法、基于聚类的方法和基于图分割的方法。
其中,基于模型的方法是使用概率模型描述网络,然后利用统计学方法推导出社团结构;基于聚类的方法是将网络中的节点聚类成若干个社团,每个社团内节点之间的相似性要求较高;基于图分割的方法则是将网络切分为若干个部分,使得每个部分内的节点之间的连通性要求较强。
下面将分别介绍一些经典的社团发现算法:1. 基于模型的方法(1) 随机游走社团发现算法(Random Walk Community Detection Algorithm,RWCD)RWCD是基于随机游走模型的社团发现算法,它将节点的相似性定义为它们之间的转移概率,然后使用PageRank算法迭代计算各节点的权值,在一定阈值下将权值较高的节点聚合成社团。
RWCD算法可以充分利用网络中的拓扑结构,对大型网络具有较好的扩展性。
(2) 右奇社团发现算法(Modularity Optimization Algorithm,MOA)MOA算法是一种基于模块度优化的社团发现算法,它将社团内节点的连接强度与所有节点的连接强度相比较,然后计算模块度值,寻找最大模块度值时的节点聚类。
MOA算法的思想简单易懂,但需要耗费大量的计算资源。
2. 基于聚类的方法(1) K-means社团发现算法K-means算法是一种常用的聚类算法,它将网络中的节点分成K个组,每个组是一个社团。
Modularity and community structure in networks
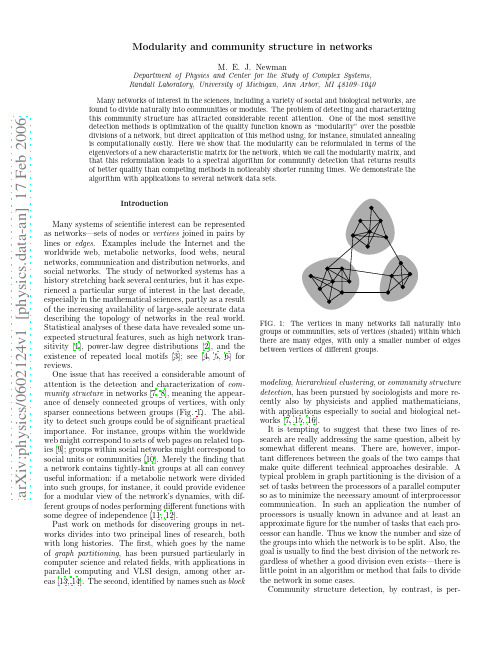
a r X i v :p h y s i c s /0602124v 1 [p h y s i c s .d a t a -a n ] 17 F eb 2006Modularity and community structure in networksM. E.J.NewmanDepartment of Physics and Center for the Study of Complex Systems,Randall Laboratory,University of Michigan,Ann Arbor,MI 48109–1040Many networks of interest in the sciences,including a variety of social and biological networks,are found to divide naturally into communities or modules.The problem of detecting and characterizing this community structure has attracted considerable recent attention.One of the most sensitive detection methods is optimization of the quality function known as “modularity”over the possible divisions of a network,but direct application of this method using,for instance,simulated annealing is computationally costly.Here we show that the modularity can be reformulated in terms of the eigenvectors of a new characteristic matrix for the network,which we call the modularity matrix,and that this reformulation leads to a spectral algorithm for community detection that returns results of better quality than competing methods in noticeably shorter running times.We demonstrate the algorithm with applications to several network data sets.IntroductionMany systems of scientific interest can be represented as networks—sets of nodes or vertices joined in pairs by lines or edges .Examples include the Internet and the worldwide web,metabolic networks,food webs,neural networks,communication and distribution networks,and social networks.The study of networked systems has a history stretching back several centuries,but it has expe-rienced a particular surge of interest in the last decade,especially in the mathematical sciences,partly as a result of the increasing availability of large-scale accurate data describing the topology of networks in the real world.Statistical analyses of these data have revealed some un-expected structural features,such as high network tran-sitivity [1],power-law degree distributions [2],and the existence of repeated local motifs [3];see [4,5,6]for reviews.One issue that has received a considerable amount of attention is the detection and characterization of com-munity structure in networks [7,8],meaning the appear-ance of densely connected groups of vertices,with only sparser connections between groups (Fig.1).The abil-ity to detect such groups could be of significant practical importance.For instance,groups within the worldwide web might correspond to sets of web pages on related top-ics [9];groups within social networks might correspond to social units or communities [10].Merely the finding that a network contains tightly-knit groups at all can convey useful information:if a metabolic network were divided into such groups,for instance,it could provide evidence for a modular view of the network’s dynamics,with dif-ferent groups of nodes performing different functions with some degree of independence [11,12].Past work on methods for discovering groups in net-works divides into two principal lines of research,both with long histories.The first,which goes by the name of graph partitioning ,has been pursued particularly in computer science and related fields,with applications in parallel computing and VLSI design,among other ar-eas [13,14].The second,identified by names such as blockFIG.1:The vertices in many networks fall naturally into groups or communities,sets of vertices (shaded)within which there are many edges,with only a smaller number of edges between vertices of different groups.modeling ,hierarchical clustering ,or community structure detection ,has been pursued by sociologists and more re-cently also by physicists and applied mathematicians,with applications especially to social and biological net-works [7,15,16].It is tempting to suggest that these two lines of re-search are really addressing the same question,albeit by somewhat different means.There are,however,impor-tant differences between the goals of the two camps that make quite different technical approaches desirable.A typical problem in graph partitioning is the division of a set of tasks between the processors of a parallel computer so as to minimize the necessary amount of interprocessor communication.In such an application the number of processors is usually known in advance and at least an approximate figure for the number of tasks that each pro-cessor can handle.Thus we know the number and size of the groups into which the network is to be split.Also,the goal is usually to find the best division of the network re-gardless of whether a good division even exists—there is little point in an algorithm or method that fails to divide the network in some cases.Community structure detection,by contrast,is per-2haps best thought of as a data analysis technique used to shed light on the structure of large-scale network datasets,such as social networks,Internet and web data, or biochemical munity structure meth-ods normally assume that the network of interest divides naturally into subgroups and the experimenter’s job is to find those groups.The number and size of the groups is thus determined by the network itself and not by the experimenter.Moreover,community structure methods may explicitly admit the possibility that no good division of the network exists,an outcome that is itself considered to be of interest for the light it sheds on the topology of the network.In this paper our focus is on community structure de-tection in network datasets representing real-world sys-tems of interest.However,both the similarities and differences between community structure methods and graph partitioning will motivate many of the develop-ments that follow.The method of optimal modularity Suppose then that we are given,or discover,the struc-ture of some network and that we wish to determine whether there exists any natural division of its vertices into nonoverlapping groups or communities,where these communities may be of any size.Let us approach this question in stages and focus ini-tially on the problem of whether any good division of the network exists into just two communities.Perhaps the most obvious way to tackle this problem is to look for divisions of the vertices into two groups so as to mini-mize the number of edges running between the groups. This“minimum cut”approach is the approach adopted, virtually without exception,in the algorithms studied in the graph partitioning literature.However,as discussed above,the community structure problem differs crucially from graph partitioning in that the sizes of the commu-nities are not normally known in advance.If community sizes are unconstrained then we are,for instance,at lib-erty to select the trivial division of the network that puts all the vertices in one of our two groups and none in the other,which guarantees we will have zero intergroup edges.This division is,in a sense,optimal,but clearly it does not tell us anything of any worth.We can,if we wish,artificially forbid this solution,but then a division that puts just one vertex in one group and the rest in the other will often be optimal,and so forth.The problem is that simply counting edges is not a good way to quantify the intuitive concept of commu-nity structure.A good division of a network into com-munities is not merely one in which there are few edges between communities;it is one in which there are fewer than expected edges between communities.If the num-ber of edges between two groups is only what one would expect on the basis of random chance,then few thought-ful observers would claim this constitutes evidence of meaningful community structure.On the other hand,if the number of edges between groups is significantly less than we expect by chance—or equivalently if the number within groups is significantly more—then it is reasonable to conclude that something interesting is going on. This idea,that true community structure in a network corresponds to a statistically surprising arrangement of edges,can be quantified using the measure known as modularity[17].The modularity is,up to a multiplicative constant,the number of edges falling within groups mi-nus the expected number in an equivalent network with edges placed at random.(A precise mathematical formu-lation is given below.)The modularity can be either positive or negative,with positive values indicating the possible presence of com-munity structure.Thus,one can search for community structure precisely by looking for the divisions of a net-work that have positive,and preferably large,values of the modularity[18].The evidence so far suggests that this is a highly effective way to tackle the problem.For instance, Guimer`a and Amaral[12]and later Danon et al.[8]op-timized modularity over possible partitions of computer-generated test networks using simulated annealing.In di-rect comparisons using standard measures,Danon et al. found that this method outperformed all other methods for community detection of which they were aware,in most cases by an impressive margin.On the basis of con-siderations such as these we consider maximization of the modularity to be perhaps the definitive current method of community detection,being at the same time based on sensible statistical principles and highly effective in practice.Unfortunately,optimization by simulated annealing is not a workable approach for the large network problems facing today’s scientists,because it demands too much computational effort.A number of alternative heuris-tic methods have been investigated,such as greedy algo-rithms[18]and extremal optimization[19].Here we take a different approach based on a reformulation of the mod-ularity in terms of the spectral properties of the network of interest.Suppose our network contains n vertices.For a par-ticular division of the network into two groups let s i=1 if vertex i belongs to group1and s i=−1if it belongs to group2.And let the number of edges between ver-tices i and j be A ij,which will normally be0or1,al-though larger values are possible in networks where mul-tiple edges are allowed.(The quantities A ij are the el-ements of the so-called adjacency matrix.)At the same time,the expected number of edges between vertices i and j if edges are placed at random is k i k j/2m,where k i and k j are the degrees of the vertices and m=14m ijA ij−k i k j4m s T Bs,(1)where s is the vector whose elements are the s i.The leading factor of1/4m is merely conventional:it is in-cluded for compatibility with the previous definition of modularity[17].We have here defined a new real symmetric matrix B with elementsk i k jB ij=A ij−FIG.2:Application of our eigenvector-based method to the “karate club”network of Ref.[23].Shapes of vertices indi-cate the membership of the corresponding individuals in the two known factions of the network while the dotted line indi-cates the split found by the algorithm,which matches the fac-tions exactly.The shades of the vertices indicate the strength of their membership,as measured by the value of the corre-sponding element of the eigenvector.groups,but to place them on a continuous scale of“how much”they belong to one group or the other.As an example of this algorithm we show in Fig.2the result of its application to a famous network from the so-cial science literature,which has become something of a standard test for community detection algorithms.The network is the“karate club”network of Zachary[23], which shows the pattern of friendships between the mem-bers of a karate club at a US university in the1970s. This example is of particular interest because,shortly after the observation and construction of the network, the club in question split in two as a result of an inter-nal dispute.Applying our eigenvector-based algorithm to the network,wefind the division indicated by the dotted line in thefigure,which coincides exactly with the known division of the club in real life.The vertices in Fig.2are shaded according to the val-ues of the elements in the leading eigenvector of the mod-ularity matrix,and these values seem also to accord well with known social structure within the club.In partic-ular,the three vertices with the heaviest weights,either positive or negative(black and white vertices in thefig-ure),correspond to the known ringleaders of the two fac-tions.Dividing networks into more than two communities In the preceding section we have given a simple matrix-based method forfinding a good division of a network into two parts.Many networks,however,contain more than two communities,so we would like to extend our method tofind good divisions of networks into larger numbers of parts.The standard approach to this prob-lem,and the one adopted here,is repeated division into two:we use the algorithm of the previous sectionfirst to divide the network into two parts,then divide those parts,and so forth.In doing this it is crucial to note that it is not correct, afterfirst dividing a network in two,to simply delete the edges falling between the two parts and then apply the algorithm again to each subgraph.This is because the degrees appearing in the definition,Eq.(1),of the mod-ularity will change if edges are deleted,and any subse-quent maximization of modularity would thus maximize the wrong quantity.Instead,the correct approach is to define for each subgraph g a new n g×n g modularity matrix B(g),where n g is the number of vertices in the subgraph.The correct definition of the element of this matrix for vertices i,j isB(g)ij=A ij−k i k j2m ,(4)where k(g)i is the degree of vertex i within subgraph g and d g is the sum of the(total)degrees k i of the vertices in the subgraph.Then the subgraph modularity Q g=s T B(g)s correctly gives the additional contribution to the total modularity made by the division of this subgraph.In particular,note that if the subgraph is undivided,Q g is correctly zero.Note also that for a complete network Eq.(4)reduces to the previous definition for the modu-larity matrix,Eq.(2),since k(g)i→k i and d g→2m in that case.In repeatedly subdividing our network,an important question we need to address is at what point to halt the subdivision process.A nice feature of our method is that it provides a clear answer to this question:if there exists no division of a subgraph that will increase the modular-ity of the network,or equivalently that gives a positive value for Q g,then there is nothing to be gained by divid-ing the subgraph and it should be left alone;it is indi-visible in the sense of the previous section.This happens when there are no positive eigenvalues to the matrix B(g), and thus our leading eigenvalue provides a simple check for the termination of the subdivision process:if the lead-ing eigenvalue is zero,which is the smallest value it can take,then the subgraph is indivisible.Note,however,that while the absence of positive eigen-values is a sufficient condition for indivisibility,it is not a necessary one.In particular,if there are only small positive eigenvalues and large negative ones,the terms in Eq.(3)for negativeβi may outweigh those for positive.It is straightforward to guard against this possibility,how-ever:we simply calculate the modularity contribution for each proposed split directly and confirm that it is greater than zero.Thus our algorithm is as follows.We construct the modularity matrix for our network andfind its leading (most positive)eigenvalue and eigenvector.We divide the network into two parts according to the signs of the elements of this vector,and then repeat for each of the parts.If at any stage wefind that the proposed split makes a zero or negative contribution to the total mod-5ularity,we leave the corresponding subgraph undivided. When the entire network has been decomposed into in-divisible subgraphs in this way,the algorithm ends. One immediate corollary of this approach is that all “communities”in the network are,by definition,indi-visible subgraphs.A number of authors have in the past proposed formal definitions of what a community is[9,16,24].The present method provides an alter-native,first-principles definition of a community as an indivisible subgraph.Further techniques for modularity maximization In this section we describe briefly another method we have investigated for dividing networks in two by mod-ularity optimization,which is entirely different from our spectral method.Although not of especial interest on its own,this second method is,as we will shortly show,very effective when combined with the spectral method.Let us start with some initial division of our vertices into two groups:the most obvious choice is simply to place all vertices in one of the groups and no vertices in the other.Then we proceed as follows.Wefind among the vertices the one that,when moved to the other group, will give the biggest increase in the modularity of the complete network,or the smallest decrease if no increase is possible.We make such moves repeatedly,with the constraint that each vertex is moved only once.When all n vertices have been moved,we search the set of in-termediate states occupied by the network during the operation of the algorithm tofind the state that has the greatest modularity.Starting again from this state,we repeat the entire process iteratively until no further im-provement in the modularity results.Those familiar with the literature on graph partitioning mayfind this algo-rithm reminiscent of the Kernighan–Lin algorithm[25], and indeed the Kernighan–Lin algorithm provided the inspiration for our method.Despite its simplicity,wefind that this method works moderately well.It is not competitive with the best pre-vious methods,but it gives respectable modularity val-ues in the trial applications we have made.However, the method really comes into its own when it is used in combination with the spectral method introduced ear-lier.It is a common approach in standard graph par-titioning problems to use spectral partitioning based on the graph Laplacian to give an initial broad division of a network into two parts,and then refine that division us-ing the Kernighan–Lin algorithm.For community struc-ture problems wefind that the equivalent joint strategy works very well.Our spectral approach based on the leading eigenvector of the modularity matrix gives an ex-cellent guide to the general form that the communities should take and this general form can then befine-tuned by our vertex moving method,to reach the best possible modularity value.The whole procedure is repeated to subdivide the network until every remaining subgraph is indivisible,and no further improvement in the modular-ity is possible.Typically,thefine-tuning stages of the algorithm add only a few percent to thefinal value of the modularity, but those few percent are enough to make the difference between a method that is merely good and one that is, as we will see,exceptional.Example applicationsIn practice,the algorithm developed here gives excel-lent results.For a quantitative comparison between our algorithm and others we follow Duch and Arenas[19] and compare values of the modularity for a variety of networks drawn from the literature.Results are shown in Table I for six different networks—the exact same six as used by Duch and Arenas.We compare mod-ularityfigures against three previously published algo-rithms:the betweenness-based algorithm of Girvan and Newman[10],which is widely used and has been incor-porated into some of the more popular network analysis programs(denoted GN in the table);the fast algorithm of Clauset et al.[26](CNM),which optimizes modularity using a greedy algorithm;and the extremal optimization algorithm of Duch and Arenas[19](DA),which is ar-guably the best previously existing method,by standard measures,if one discounts methods impractical for large networks,such as exhaustive enumeration of all parti-tions or simulated annealing.The table reveals some interesting patterns.Our al-gorithm clearly outperforms the methods of Girvan and Newman and of Clauset et al.for all the networks in the task of optimizing the modularity.The extremal opti-mization method on the other hand is more competitive. For the smaller networks,up to around a thousand ver-tices,there is essentially no difference in performance be-tween our method and extremal optimization;the mod-ularity values for the divisions found by the two algo-rithms differ by no more than a few parts in a thousand for any given network.For larger networks,however,our algorithm does better than extremal optimization,and furthermore the gap widens as network size increases, to a maximum modularity difference of about a6%for the largest network studied.For the very large networks that have been of particular interest in the last few years, therefore,it appears that our method for detecting com-munity structure may be the most effective of the meth-ods considered here.The modularity values given in Table I provide a use-ful quantitative measure of the success of our algorithm when applied to real-world problems.It is worthwhile, however,also to confirm that it returns sensible divisions of networks in practice.We have given one example demonstrating such a division in Fig.2.We have also checked our method against many of the example net-works used in previous studies[10,17].Here we give two more examples,both involving network representationsmodularity Q network GN CNM DA this paper3419845311331068027519maximal value of the quantity known as modularity over possible divisions of a network.We have shown that this problem can be rewritten in terms of the eigenval-ues and eigenvectors of a matrix we call the modularity matrix,and by exploiting this transformation we have created a new computer algorithm for community de-tection that demonstrably outperforms the best previ-ous general-purpose algorithms in terms of both quality of results and speed of execution.We have applied our algorithm to a variety of real-world network data sets, including social and biological examples,showing it to give both intuitively reasonable divisions of networks and quantitatively better results as measured by the modu-larity.AcknowledgmentsThe author would like to thank Lada Adamic,Alex Arenas,and Valdis Krebs for providing network data and for useful comments and suggestions.This work was funded in part by the National Science Foundation un-der grant number DMS–0234188and by the James S. McDonnell Foundation.[1]D.J.Watts and S.H.Strogatz,Collective dynamics of‘small-world’networks.Nature393,440–442(1998). [2]A.-L.Barab´a si and R.Albert,Emergence of scaling inrandom networks.Science286,509–512(1999).[3]o,S.Shen-Orr,S.Itzkovitz,N.Kashtan,D.Chklovskii,and U.Alon,Network motifs:Simplebuilding blocks of complex networks.Science298,824–827(2002).[4]R.Albert and A.-L.Barab´a si,Statistical mechanics ofcomplex networks.Rev.Mod.Phys.74,47–97(2002).[5]S.N.Dorogovtsev and J.F.F.Mendes,Evolution ofnetworks.Advances in Physics51,1079–1187(2002). [6]M.E.J.Newman,The structure and function of complexnetworks.SIAM Review45,167–256(2003).[7]M.E.J.Newman,Detecting community structure in net-works.Eur.Phys.J.B38,321–330(2004).[8]L.Danon,J.Duch, A.Diaz-Guilera,and A.Arenas,Comparing community structure identification.J.Stat.Mech.p.P09008(2005).[9]G.W.Flake,wrence,C.L.Giles,and F.M.Co-etzee,Self-organization and identification of Web com-munities.IEEE Computer35,66–71(2002).[10]M.Girvan and M.E.J.Newman,Community structurein social and biological networks.Proc.Natl.Acad.Sci.USA99,7821–7826(2002).[11]P.Holme,M.Huss,and H.Jeong,Subnetwork hierar-chies of biochemical pathways.Bioinformatics19,532–538(2003).[12]R.Guimer`a and L.A.N.Amaral,Functional cartogra-phy of complex metabolic networks.Nature433,895–900 (2005).[13]U.Elsner,Graph partitioning—a survey.Technical Re-port97-27,Technische Universit¨a t Chemnitz(1997). [14]P.-O.Fj¨a llstr¨o m,Algorithms for graph partitioning:Asurvey.Link¨o ping Electronic Articles in Computer and Information Science3(10)(1998).[15]H.C.White,S.A.Boorman,and R.L.Breiger,Socialstructure from multiple networks:I.Blockmodels of roles and positions.Am.J.Sociol.81,730–779(1976). [16]S.Wasserman and K.Faust,Social Network Analysis.Cambridge University Press,Cambridge(1994).[17]M.E.J.Newman and M.Girvan,Finding and evaluat-ing community structure in networks.Phys.Rev.E69, 026113(2004).[18]M.E.J.Newman,Fast algorithm for detecting com-munity structure in networks.Phys.Rev.E69,066133 (2004).[19]J.Duch and A.Arenas,Community detection in complexnetworks using extremal optimization.Phys.Rev.E72, 027104(2005).[20]F.R.K.Chung,Spectral Graph Theory.Number92in CBMS Regional Conference Series in Mathematics, American Mathematical Society,Providence,RI(1997).[21]M.Fiedler,Algebraic connectivity of graphs.Czech.Math.J.23,298–305(1973).[22]A.Pothen,H.Simon,and K.-P.Liou,Partitioning sparsematrices with eigenvectors of graphs.SIAM J.Matrix Anal.Appl.11,430–452(1990).[23]W.W.Zachary,An informationflow model for conflictandfission in small groups.Journal of Anthropological Research33,452–473(1977).[24]F.Radicchi,C.Castellano,F.Cecconi,V.Loreto,andD.Parisi,Defining and identifying communities in net-A101,2658–2663 (2004).[25]B.W.Kernighan and S.Lin,An efficient heuristic proce-dure for partitioning graphs.Bell System Technical Jour-nal49,291–307(1970).[26]A.Clauset,M.E.J.Newman,and C.Moore,Findingcommunity structure in very large networks.Phys.Rev.E70,066111(2004).[27]P.Gleiser and L.Danon,Community structure in jazz.Advances in Complex Systems6,565–573(2003). [28]H.Jeong,B.Tombor,R.Albert,Z.N.Oltvai,and A.-L.Barab´a si,The large-scale organization of metabolic networks.Nature407,651–654(2000).[29]H.Ebel,L.-I.Mielsch,and S.Bornholdt,Scale-free topol-ogy of e-mail networks.Phys.Rev.E66,035103(2002).[30]X.Guardiola,R.Guimer`a,A.Arenas,A.Diaz-Guilera,D.Streib,and L. A.N.Amaral,Macro-and micro-structure of trust networks.Preprint cond-mat/0206240 (2002).[31]M.E.J.Newman,The structure of scientific collabora-tion A98,404–409 (2001).[32]L.A.Adamic and N.Glance,The political blogosphereand the2004us election.In Proceedings of the WWW-2005Workshop on the Weblogging Ecosystem(2005).。
微观组织英语
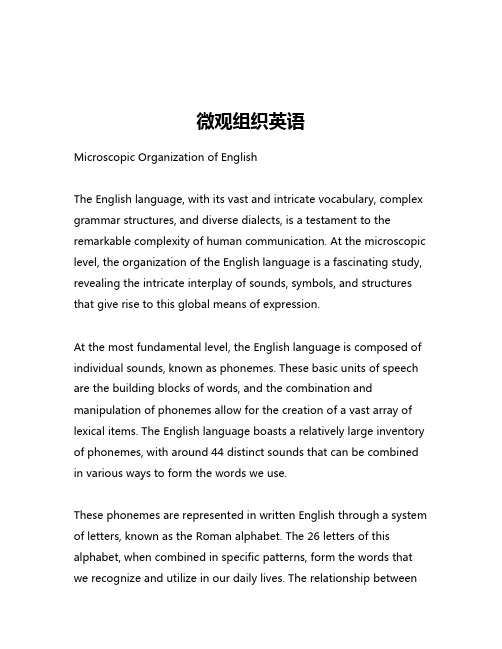
微观组织英语Microscopic Organization of EnglishThe English language, with its vast and intricate vocabulary, complex grammar structures, and diverse dialects, is a testament to the remarkable complexity of human communication. At the microscopic level, the organization of the English language is a fascinating study, revealing the intricate interplay of sounds, symbols, and structures that give rise to this global means of expression.At the most fundamental level, the English language is composed of individual sounds, known as phonemes. These basic units of speech are the building blocks of words, and the combination and manipulation of phonemes allow for the creation of a vast array of lexical items. The English language boasts a relatively large inventory of phonemes, with around 44 distinct sounds that can be combined in various ways to form the words we use.These phonemes are represented in written English through a system of letters, known as the Roman alphabet. The 26 letters of this alphabet, when combined in specific patterns, form the words that we recognize and utilize in our daily lives. The relationship betweenthe spoken sounds and their written counterparts is not always straightforward, however, as the English language has a complex system of orthography that can sometimes defy the expected correspondence between letters and sounds.Delving deeper into the microscopic organization of English, we find that the language is structured around a hierarchical system of units. At the most basic level, individual phonemes are combined to form morphemes, which are the smallest meaningful units of language. Morphemes can be further grouped into words, which are the primary building blocks of sentences and discourse.Words themselves can be broken down into smaller components, known as morphemes, which are the smallest units of meaning within a language. These morphemes can be root words, prefixes, or suffixes, and the combination and manipulation of these elements allow for the creation of new words and the expression of complex ideas.The grammatical structure of the English language is another important aspect of its microscopic organization. English utilizes a system of parts of speech, such as nouns, verbs, adjectives, and adverbs, which are combined according to specific rules to form coherent sentences and paragraphs. These grammatical structures, while often complex, provide the framework for the clear andeffective communication of ideas.At the level of syntax, the English language follows a relatively rigid word order, with the typical structure of a sentence being subject-verb-object. This consistency in sentence structure, combined with the use of function words like articles, prepositions, and conjunctions, helps to ensure that the meaning of a message is conveyed clearly and unambiguously.The lexical diversity of the English language is another remarkable feature of its microscopic organization. English has a vast vocabulary, with estimates ranging from 500,000 to over 1 million words, many of which have been borrowed from other languages over the course of the language's history. This rich lexical landscape allows for the precise and nuanced expression of ideas, emotions, and concepts, and contributes to the language's versatility and adaptability.The study of the microscopic organization of English also reveals the dynamic nature of the language. Words are constantly being added, modified, or removed from the language, reflecting the evolution of human thought and the changing societal and cultural contexts in which the language is used. The processes of word formation, including derivation, compounding, and blending, further contribute to the ongoing expansion and diversification of the English vocabulary.In conclusion, the microscopic organization of the English language is a complex and fascinating subject of study. From the basic building blocks of phonemes and morphemes, to the intricate structures of grammar and syntax, the language is a testament to the remarkable capacity of the human mind to create and manipulate systems of communication. By understanding the microscopic details of English, we can gain deeper insights into the nature of language itself, and appreciate the remarkable achievements of the countless individuals who have contributed to the development and evolution of this global means of expression.。
合成生物学研究与进展

合成生物学——生物燃料
Company
Ethanol Algenol Biofuels,Naples,Florida
Process Photosynthesis in contained bioreactor
Diesel Aurora Biofuels, alameda, California
必需基因
必需基因(essential genes)是指在一定环境条件 下,维持某种生物体的生命活动所必不可少的基 因。这些基因所编码蛋白质的功能被认为是生命 的基础,去除一个必需基因将使生物体无法继续 存活。
目前用于必需基因研究的实验方法主要有:
转座子突变技术(transposon mutagenesis)、 RNA干扰技术(RNA interference)和T-DNA插入 (T-DNA insertion)。
single-gene deletions single-gene deletions transposon mutagenesis genetic footprint and single-gene deletions genetic footprint and single-gene deletions genetic footprint and single-gene deletions transposon mutagenesis transposon mutagenesis transposon mutagenesis transposon mutagenesis transposon mutagenesis transposon mutagenesis
Photosynthesis Photosynthesis Photosynthesis in close system photobioreactor Combined phtosynthetic production and secretion
外文翻译 组织承诺,人力资源实践和组织特征大学论文
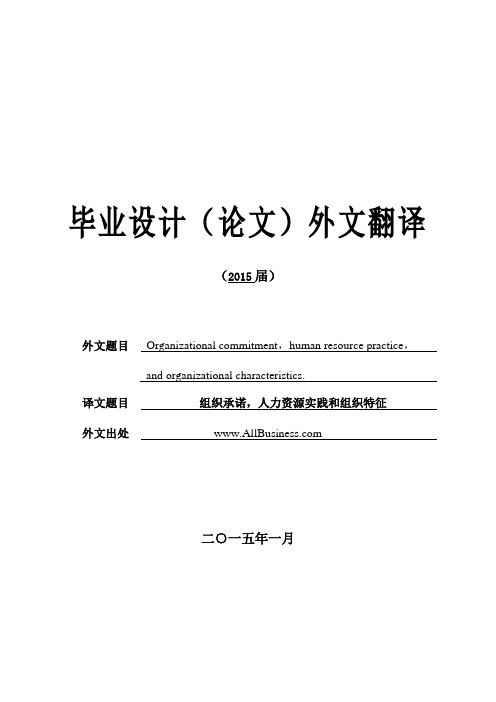
毕业设计(论文)外文翻译(2015届)外文题目Organizational commitment,human resource practice,and organizational characteristics.译文题目组织承诺,人力资源实践和组织特征外文出处二○一五年一月中文5335字组织承诺,人力资源实践和组织特征作者:Fiorito,Jack,Bozeman,Dennis P.,Young,Angela,Meurs,James A.2007年6月22日发表于组织承诺在研究就业、组织和相关领域中是一个主要变量。
Article-First数据库显示,在学术资源中有202篇出版在2001年和2004年之间的文章引用了“组织承诺”作为文章和摘要标题。
并且,组织承诺对管理学者来说仍然是一个长期的课题(比如这些学者在近年进行了研究:Hammer和Avgar,2005;Jandeska和Kraimer,2005;Payne和Huffman,2005)。
之所以有很多人研究承诺,是因为学者们认为它会影响组织绩效。
例如,组织承诺的一个主要方面是角色外行为。
组织通常把员工贡献评价为员工的主动性和自觉性行为。
虽然组织赞赏超出工作要求的个人表现,但是很少人能说工作描述可以充分详细说明所有有价值的贡献。
许多研究表明,组织承诺能预测重要的变量,包括缺勤、组织公民、绩效和营业额(例如Mathieu和Zajac,1990;Morrow,1993;Wright和Bonett,2002,这些学者得出过这样的结论)。
Colbert和Kwon 在2000年的研究中指出,组织承诺与意图寻找工作的替代品和有意图离职的行为都呈负相关(1994年Quarles的研究中也有这样的观点)。
此外,Farrell和Stamm(1988)提出,组织承诺能降低缺勤的频率。
还有,组织承诺与更积极的组织结果有关,包括Williams和Hazer(1986)提出的组织承诺与工作满意度有关,Burton等人(2002)提出的组织承诺和出勤的动机有关。
模联专业词汇扫盲
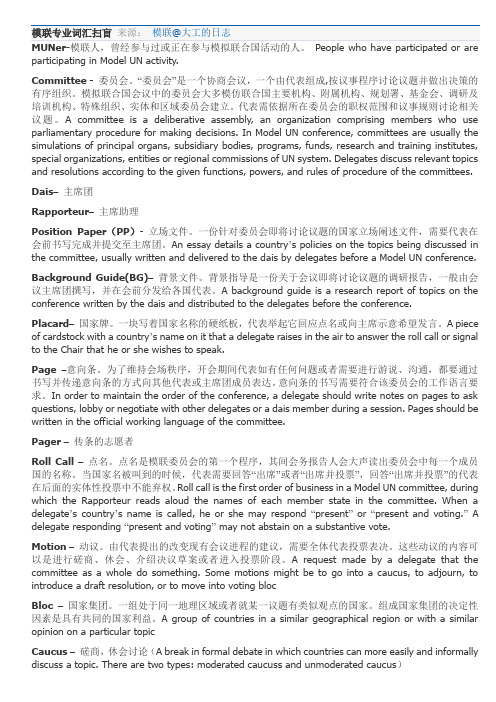
participating in Model UN activity.Committee - 委员会。
“委员会”是一个协商会议,一个由代表组成,按议事程序讨论议题并做出决策的有序组织。
模拟联合国会议中的委员会大多模仿联合国主要机构、附属机构、规划署、基金会、调研及培训机构、特殊组织、实体和区域委员会建立。
代表需依据所在委员会的职权范围和议事规则讨论相关议题。
A committee is a deliberative assembly, an organization comprising members who use parliamentary procedure for making decisions. In Model UN conference, committees are usually the simulations of principal organs, subsidiary bodies, programs, funds, research and training institutes, special organizations, entities or regional commissions of UN system. Delegates discuss relevant topics and resolutions according to the given functions, powers, and rules of procedure of the committees.Dais–主席团Rapporteur–主席助理Position Paper(PP)- 立场文件。
一份针对委员会即将讨论议题的国家立场阐述文件,需要代表在会前书写完成并提交至主席团。
An essay details a country’s policies on the topics being discussed in the committee, usually written and delivered to the dais by delegates before a Model UN conference.Background Guide(BG)–背景文件。
- 1、下载文档前请自行甄别文档内容的完整性,平台不提供额外的编辑、内容补充、找答案等附加服务。
- 2、"仅部分预览"的文档,不可在线预览部分如存在完整性等问题,可反馈申请退款(可完整预览的文档不适用该条件!)。
- 3、如文档侵犯您的权益,请联系客服反馈,我们会尽快为您处理(人工客服工作时间:9:00-18:30)。
Hierarchical organization of modularity in metabolic networksE. Ravasz1, A.L. Somera2, D.A. Mongru2, Z.N. Oltvai2 & A.-L. Barabási11Department of Physics, University of Notre Dame, Notre Dame, IN 465562Department of Pathology, Northwestern University, Chicago, IL 60611Spatially or chemically isolated functional modules composed of several cellular components and carrying discrete functions are considered fundamental building blocks of cellular organization, but their presence in highly integrated biochemical networks lacks quantitative support. Here we show that the metabolic networks of 43 distinct organisms are organized into many small, highly connected topologic modules that combine in a hierarchical manner into larger, less cohesive units, their number and degree of clustering following a power law. Within Escherichia coli the uncovered hierarchical modularity closely overlaps with known metabolic functions. The identified network architecture may be generic to system-level cellular organization.The identification and characterization of system-level features of biological organization is a key issue of post-genomic biology (1, 2, 3). The concept of modularity assumes that cellular functionality can be seamlessly partitioned into a collection of modules. Each module is a discrete entity of several elementary components and performs an identifiable task, separable from the functions of other modules (1, 4, 5, 6, 7, 8). Spatially and chemically isolated molecular machines or protein complexes (such as ribosomes and flagella) are prominent examples of such functional units, but more extended modules, such as those achieving their isolation through the initial binding of a signaling molecule (9) are also apparent.Simultaneously, it is now widely recognized that the thousands of components of a living cell are dynamically interconnected, so that the cell’s functional properties are ultimately encoded into a complex intracellular web of molecular interactions (2, 3, 4, 5, 6, 8). This is perhaps most evident when inspecting cellular metabolism, a fully connected biochemical network in which hundreds of metabolic substrates are densely integrated via biochemical reactions. Within this network, however, modular organization (i.e., clear boundaries between sub-networks) is not immediately apparent. Indeed, recent studies have demonstrated that the probability that a substrate can react with k other substrates (the degree distribution P(k) of a metabolic network) decays as a power law P(k) ~ k-J with J# 2.2 in all organisms (10, 11) suggesting that metabolic networks have a scale-free topology (12). A distinguishing feature of such scale-free networks is the existence of a few highly connected nodes (e.g., pyruvate or CoA), which participate in a very large number of metabolic reactions. With a large number of links, these hubs integrate all substrates into a single, integrated web in which the existence of fully separated modules is prohibited by definition (Fig. 1a).Yet, the dilemma of a modular- versus a highly integrated module-free metabolic network organization remains. A number of approaches for analyzing the functional capabilities of metabolic networks clearly indicate the existence of separable functional elements (13, 14). Also, from a purely topologic perspective the metabolic network of Escherichia coli is known to possess a high clustering coefficient (11), a property that is suggestive of a modular organization (see below). In itself, this implies that the metabolism of E. coli has a modular topology, potentially comprising several densely interconnected functional modules of varying sizes that are connected by few inter-module links (Fig. 1b). However, such clear-cut modularity imposes severe restrictions on the degree distribution, implying that most nodes have approximately the same number of links, which contrasts with the metabolic network’s scale-free nature (10, 11).To determine if such a dichotomy is indeed a generic property of all metabolic networks we first calculated the average clustering coefficient for 43 different organisms (15, 16) as a function of the number of distinct substrates, N, present in their metabolism. The clustering coefficient, defined as C i = 2n/k i(k i-1), where n denotes the number of direct links connecting the k i nearest neighbors of node i (17), is equal to one for a node at the center of a fully inter-linked cluster, while it is zero for a metabolite that is part of a loosely connected group (Fig. 2a). Therefore, C i averaged over all nodes i of a metabolic network is a measure of the network’s potential modularity. We find that for all 43 organisms the clustering coefficient is about an order of magnitude larger than that expected for a scale-free network of similar size (Fig. 2b), suggesting that metabolic networks in all organisms are characterized by a high intrinsic potential modularity. We also observe that in contrast with the prediction of the scale-free model, for which the clustering coefficient decreases as N-0.75(18), the clustering coefficient of metabolic networks' is independent of their size (Fig. 2b).Taken together, these results demonstrate a fundamental conflict between the predictions of the current models of metabolic organization. The high, size independent clustering coefficient offers strong evidence for modularity, while the power law degree distribution of all metabolic networks (10, 11) strongly support the scale-free model and rule out a manifestly modular topology. To resolve this apparent contradiction we propose a simple heuristic model of metabolic organization, which we refer to as a “hierarchical” network (Fig. 1c) (19). In such a model network, our starting point is a small cluster of four densely linked nodes. Next we generate three replicas of this hypothetical module and connect the three external nodes of the replicated clusters to the central node of the old cluster, obtaining a large 16-node module. Subsequently, we again generate three replicas of this 16-node module, and connect the peripheral nodes to the central node of the old module (Fig. 1c). These replication and connection steps can be repeated indefinitely, in each step quadrupling the number of nodes in the system. The architecture of such a network integrates a scale-free topology with an inherent modular structure. It has a power law degree distribution with degree exponent J = 1 + ln4/ln3 = 2.26, in agreement with the J = 2.2 observed in metabolic networks. Its clustering coefficient, C # 0.6, is also comparable with that observed for metabolic networks. Most importantly, the clustering coefficient of the model is independent of the size of the network, in agreement with the results of Fig. 2b.A unique feature of the proposed network model, not shared by either the scale-free (Fig. 1a) or modular (Fig. 1b) models, is its hierarchical architecture. This hierarchy, which is evident from a visual inspection, is intrinsic to the assembly by repeated quadrupling of the system. The hierarchy can be characterized quantitatively by using the recent observation (20) that in deterministic scale-free networks the clustering coefficient of a node with k links follows thescaling law C(k) ~ k -1. This scaling law quantifies the coexistence of a hierarchy of nodes with different degrees of modularity, as measured by the clustering coefficient, and is directly relevant to our model (Fig. 1c). Indeed, the nodes at the center of the numerous 4-node modules have a clustering coefficient C=3/4. Those at the center of a 16-node module have k = 13 and C = 2/13, while those at the center of the 64 node modules have k = 40 and C = 2/40, indicating that the higher a node’s connectivity the smaller is its clustering coefficient, asymptotically following the 1/k law.To investigate if such hierarchical organization is present in cellular metabolism we measured the C(k) function for the metabolic networks of all 43 organisms. As shown in Fig. 2c-f, for each organism C(k) is well approximated by C(k) ~ k-1, in contrast to the k-independent C(k) predicted by both the scale-free and modular networks. This provides direct evidence for an inherently hierarchical organization. Such hierarchical modularity reconciles within a single framework all the observed properties of metabolic networks: their scale-free topology; high, system size independent clustering coefficient and the power-law scaling of C(k).A key issue from a biological perspective is whether the identified hierarchical architecture reflects the true functional organization of cellular metabolism. To uncover potential relationships between topological modularity and the functional classification of different metabolites we concentrate on the metabolic network of Escherichia coli, whose metabolic reactions have been exhaustively studied, both biochemically and genetically (21). With a previously established graph-theoretical representation in hand (10), we first subjected E. coli’s metabolic organization to a three step reduction process, replacing non-branching pathways with equivalent links, allowing us to decrease its complexity without altering the network topology (16). Next, we calculated the topological overlap matrix, O T(i,j), of the condensed metabolicnetwork (Fig. 3a). A topological overlap of one between substrates i and j implies that they are connected to the same substrates, while a zero value indicates that i and j do not share links to common substrates among the metabolites they react with. The metabolites that are part of highly integrated modules have a high topological overlap with their neighbors, and we find that the larger the overlap between two substrates within the E. coli metabolic network the more likely it is that they belong to the same functional class.As the topological overlap matrix is expected to encode the comprehensive functional relatedness of the substrates forming the metabolic network, we investigated whether potential functional modules encoded in the network topology can be uncovered automatically. Initial application of an average-linkage hierarchical clustering algorithm (22) to the overlap matrix of the small hypothetical network shown in Fig. 3a placed those nodes that have a high topological overlap close to each other (Fig. 3b). Also, the method has clearly identified the three distinct modules built into the model of Fig. 3a, as illustrated by the fact that the EFG and HIJK modules are closer to each other in a topological sense than the ABC module (Fig. 3b). Application of the same technique on the E. coli overlap matrix O T(i,j) provides a global topologic representation of E. coli metabolism (Fig. 4a). Groups of metabolites forming tightly interconnected clusters are visually apparent, and upon closer inspection the hierarchy of nested topologic modules of increasing sizes and decreasing interconnectedness are also evident. To visualize the relationship between topological modules and the known functional properties of the metabolites, we color coded the branches of the derived hierarchical tree according to the predominant biochemical class of the substrates it produces, using the standard, small molecule biochemistry based classification of metabolism (15). As shown in Fig. 4a, and in the three dimensional representation in Fig. 4b, we find that most substrates of a given small molecule class aredistributed on the same branch of the tree (Fig. 4a) and correspond to relatively well-delimited regions of the metabolic network (Fig. 4b). Therefore, there are strong correlations between shared biochemical classification of metabolites and the global topological organization of E. coli metabolism (Fig. 4a, bottom, and Ref. 16).To correlate the putative modules obtained from our graph theory-based analysis to actual biochemical pathways, we concentrated on the pathways involving the pyrimidine metabolites. Our method divided these pathways into four putative modules (Fig. 4c), which represent a topologically well-limited area of E. coli metabolism (Fig. 4b, circle). As shown in Fig. 4d, all highly connected metabolites (Fig. 4d, red-boxes) correspond to their respective biochemical reactions within pyrimidine metabolism, together with those substrates that were removed during the original network reduction procedure, and then re-added (Fig. 4d, green boxes). However, it is also apparent that putative module boundaries do not always overlap with intuitive ‘biochemistry-based’ boundaries. For instance, while the synthesis of UMP from L-glutamine is expected to fall within a single module based on a linear set of biochemical reactions, the synthesis of UDP from UMP leaps putative module boundaries. Thus, further experimental and theoretical analyses will be needed to understand the relationship between the decomposition of E. coli metabolism offered by our topology-based approach, and the biologically relevant sub-networks.The organization of metabolic networks is likely to combine a capacity for rapid flux reorganization with a dynamic integration with all other cellular function (11). Here we show that the system-level structure of cellular metabolism is best approximated by a hierarchical network organization with seamlessly embedded modularity. In contrast to current, intuitive views of modularity (Fig. 1b) which assume the existence of a set of modules with a non-uniform size potentially separated from other modules, we find that the metabolic network has an inherent self-similar property: there are many highly integrated small modules, which group into a few larger modules, which in turn can be integrated into even larger modules. This is supported by visual inspection of the derived hierarchical tree (Fig. 4a), which offers a natural breakdown of metabolism into several large modules, which are further partitioned into smaller, but more integrated sub-modules.The mathematical framework proposed here to uncover the presence or absence of such hierarchical modularity, and to delineate the modules based on the network topology could apply to other cellular- and complex networks as well. As scale-free topology has been found at many different organizational levels, ranging from protein interaction, to genetic (23) and protein domain (24) networks, it is possible that biological networks are always accompanied by a hierarchical modularity. Some non-biological networks, ranging from the World Wide Web to the Internet, often combine a scale-free topology with a community structure (i.e., modularity) (25, 26, 27), therefore these networks are also potential candidates for hierarchical modularity. For biological systems hierarchical modularity is consistent with the notion that evolution may act at many organizational levels simultaneously: the accumulation of many local changes, that affect the small, highly integrated modules, could slowly impact the properties of the larger, less integrated modules. The emergence of the hierarchical topology via copying and reusing existing modules (1) and motifs (8), a process reminiscent of the results of gene duplication (28, 29), offers a special role to the modules that appeared first in the network. While the model of Fig. 1c reproduces the large-scale features of the metabolism, understanding the evolutionary mechanism that explains the simultaneous emergence of the observed hierarchical and scale-free topology of the metabolism, and its generality to cellular organization, is now a prime challenge.References and Notes1. L. H. Hartwell, J. J. Hopfield, S. Leibler, A. W. Murray, Nature402, C47 (1999).2. H. Kitano, Science295, 1662-1664 (2002).3. Y. I. Wolf, G. Karev, E. V. Koonin, Bioessays24, 105 (2002).4. D. A. Lauffenburger, Proc Natl Acad Sci U S A 97, 5031 (2000).5. C. V. Rao, A. P. Arkin, Annu Rev Biomed Eng3, 391 (2001).6. N. S. Holter, A. Maritan, M. Cieplak, N. V. Fedoroff, J. R. Banavar, Proc Natl AcadSci Sci U S A98, 1693 (2001).7. J. Hasty, D. McMillen, F. Isaacs, J. J. Collins, Nat Rev Genet2, 268 (2001).8. S. S. Shen-Orr, R. Milo, S. Mangan, U. Alon, Nature Genetics31, 64 (2002).9. U. Alon, M. G. Surette, N. Barkai, S. Leibler, Nature397, 168 (1999).10. H. Jeong, B. Tombor, R. Albert, Z. N. Oltvai, A.-L. Barabási, Nature407, 651(2000).11. A. Wagner, D. A. Fell, Proc R Soc Lond B Biol Sci268, 1803 (2001).12. A.-L. Barabási, R. Albert, Science286, 509 (1999).13. C. H. Schilling, D. Letscher, B. O. Palsson, J Theor Biol203, 229 (2000).14. S. Schuster, D. A. Fell, T. Dandekar, Nat Biotechnol18, 326 (2000).15. R. Overbeek et al., Nucleic Acids Res28, 123 (2000).16. Supporting Online Material, available at/~networks/cell/index.html.17. D. J. Watts, S. H. Strogatz, Nature393, 440 (1998).18. R. Albert, A. L. Barabási, Rev Mod Phys74, 47 (2002).19. A.-L. Barabási, E. Ravasz, T. Vicsek, Physica A299, 559 (2001).20. S. N. Dorogovtsev, A. V. Goltsev, J. F. F. Mendes, in press (available at/abs/cond-mat/0112143) (2001).21. P. D. Karp, M. Riley, S. M. Paley, A. Pellegrini-Toole, M. Krummenacker, Nucleic Acids Res30, 56 (2002).22. M. B. Eisen, P. T. Spellman, P. O. Brown, D. Botstein, Proc Natl Acad Sci U S A95, 14863 (1998).23. D. E. Featherstone, K. Broadie, Bioessays24 (3), 267 (2002).24. S. Wuchty, Mol Biol Evol18, 1694 (2001).25. G. W. Flake, S. Lawrence, C. L. Giles, F. M. Coetzee, Computer53, 66 (2002).26. M. Girvan, M. E. J. Newman, Proc. Natl. Acad. Sci. USA99, 7821 (2002).27. A. Vazquez, R. Pastor-Satorras, A. Vespignani, in press (available at/abs/cond-mat/0112400) (2001).28. A. Vazquez, A. Flammini, A. Maritan, A. Vespignani, in press (available at/abs/cond-mat/0108043)(2001).29. R. P. Solé, D. E. Smith, R. Pastor-Satorras, T. Kepler, Santa Fe Preprint (available at /sfi/publications/wpabstract/200108041).30. Acknowledgement. We thank T. Vicsek and I.J. Farkas for discussions on the hierarchical model. We also thank C. Waltenbaugh and J. W. Campbell for comments on the manuscript and the WIT project for making their database publicly available. Research at the University of Notre Dame and at Northwestern University was supported by grants from the Department of Energy and the National Institute of Health Correspondence and request for materials should be addressed to A.-L.B. (e-mail:alb@) or Z.N.O. (e-mail: zno008@).Supporting Online Material/~networks/cell1. Network Models2. Graph Theoretic Characterization of the Metabolism3. Clustering and Functional Characterization4. Figs. S1 to S15Figure LegendsFigure 1. Complex network models.(a) Left: A schematic illustration of a scale-free network, whose degree distribution follows a power law. In such a network a few highly connected nodes, or hubs (blue circles) play an important role in keeping the whole network together. On the right, a typical configuration of a scale-free network with 256 nodes is shown, obtained using the scale-free model, which requires adding a new node at each time such, that existing nodes with higher degrees of connectivity have a higher chance of being linked to the new nodes (12). The nodes are arranged in space using a standard clustering algorithm (http://vlado.fmf.uni-lj.si/pub/networks/pajek/) to illustrate the absence of an underlying modularity. (b) Left: Schematic illustration of a manifestly modular network made of four highly interlinked modules connected to each other by a few links. This intuitive topology does not have a scale-free degree distribution, as most of its nodes have a similar number of links, and hubs are absent. A standard clustering algorithm easily uncovers thenetwork’s inherent modularity (right panel) by partitioning a modular network of N = 256 nodes into the four isolated structures built into the system. (c) Left: The hierarchical network has a scale-free topology with embedded modularity, the increasing hierarchical levels labeled with blue, green and red, respectively. As shown on the right, standard clustering algorithms are less successful in uncovering the network’s underlying modularity. For a detailed quantitative characterization of the three network models see Ref. 16.Figure 2.Evidence of hierarchical modularity in metabolic networks.(a) The clustering coefficient offers a measure of the degree of interconnectivity in the neighborhood of a node (17). For example, a node whose neighbors are all connected to each other has C = 1 (left), while a node with no links between its neighbors has C = 0 (right). (b) The average clustering coefficient, C(N), for 43 organisms (10) is shown as a function of the number of substrates N present in each of them. Species belonging to Archae (purple), Bacteria (green), and Eukaryotes (blue) are shown. The dashed line indicates the dependence of the clustering coefficient on the network size for a module-free scale-free network, while the diamonds denoteC for a scale-free network with the same parameters (N and number of links) as observed in the43 organisms. (c-e): The dependence of the clustering coefficient on the node’s degree in three organisms: Aquidex Aeolicus (archaea)(c), Escherichia coli (bacterium) (d), and S. cerevisiae (eukaryote) (e). In (f) the C(k) curves averaged over all 43 organisms is shown, while the inset displays all 43 species together. The data points are color coded as above. In (c-f) the dashed lines correspond to C(k) ~ k-1, and in (c-e) the diamonds represent C(k) expected for a scale-free network (Fig. 1a) of similar size, indicating the absence of scaling. The wide fluctuations are due to the small size of the network.Figure 3. Uncovering the underlying modularity of a complex network.(a) Topological overlap illustrated on a small hypothetical network. For each pair of nodes, i and j, we define the topological overlap O T(i,j) = J n(i,j)/min(k i,k j), where J n(i,j) denotes the number of nodes to which both i and j are linked to (plus one if there is a direct link between i and j)and min(k i,k j) is the smaller of the k i and k j degrees. On each link we indicate the topological overlap for the connected nodes and in parenthesis next to each node we indicate the node’s clustering coefficient. (b) The topological overlap matrix corresponding to the small network shown in (a). The rows and columns of the matrix were reordered by the application of an average linkage clustering method (22) to its elements, allowing us to identify and place close to each other those nodes that have high topological overlap. The color code denotes the degree of topological overlap between the nodes (see sidebar). The associated tree clearly reflects the three distinct modules built into the model of Fig. 3a, as well as the fact that the EFG and HIJK modules are closer to each other in topological sense that the ABC module.Figure 4.Identifying the functional modules in E. coli metabolism.(a) The topologic overlap matrix corresponding to the E. coli metabolism, together with the corresponding hierarchical tree (top) that quantifies the relationship between the different modules. The branches of the tree are color coded to reflect the functional classification of their substrates. The biochemical classes we used to group the metabolites represent carbohydrate metabolism (blue), nucleotide and nucleic acid metabolism (red), protein, peptide and amino acid metabolism (green), lipid metabolism (cyan), aromatic compound metabolism (dark pink), monocarbon compound metabolism (yellow) and coenzyme metabolism (light orange) (15). Thecolor code of the matrix denotes the degree of topological overlap shown in the matrix. On the bottom we show the large-scale functional map of the metabolism, as suggested by the hierarchical tree. (b) 3-D representation of the reduced E. coli metabolic network. Each node is color coded by the functional class to which it belongs, and is identical to the color code applied to the branches of the tree shown in (a). Note that the different functional classes are visibly segregated into topologically distinct regions of metabolism. The blue-shaded region denotes the nodes belonging to pyrimidine metabolism, discussed below. (c) Enlarged view of the substrate module of pyrimidine metabolism. The colored boxes denote the three-level, nested modularity suggested by the hierarchical tree. (d) A detailed diagram of the metabolic reactions that surround and incorporate the pyrimidine metabolic module. Red boxes denote the substrates directly appearing in the reduced metabolism and the tree shown in (c). Substrates in green boxes are internal to pyrimidine metabolism, but represent members of non-branching pathways or end pathways branching from a metabolite with multiple connections (16). Blue and black boxes show the connections of pyrimidine metabolites to other parts of the metabolic network. Black boxes denote core substrates belonging to other branches of the metabolic tree (a), while blue boxes denote non-branching pathways (if present) leading to those substrates. Note, that with the exception of carbamoyl-phosphate and S-dihydroorotate, all pyrimidine metabolites are connected with a single biochemical reaction. The shaded boxes around the reactions highlight the modules suggested by the hierarchical tree. The shaded blue boxes along the links display the enzymes catalyzing the corresponding reactions, and the arrows show the direction of the reactions according to the WIT metabolic maps (15).。