任可澄研究综述
“社会医学”思政元素渗透与教学内容设计探索
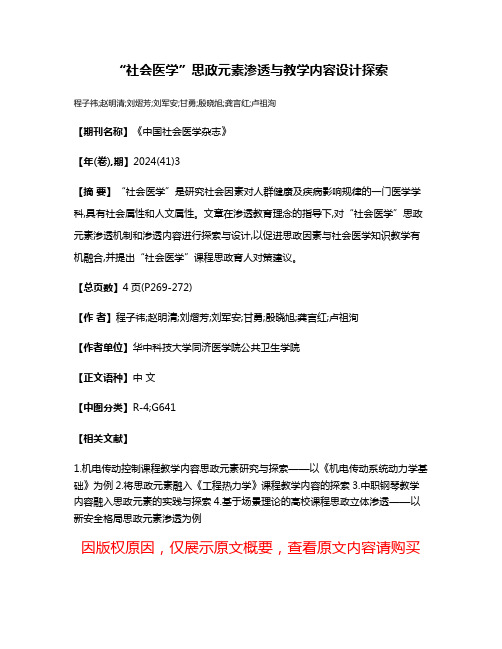
“社会医学”思政元素渗透与教学内容设计探索
程子祎;赵明清;刘熠芳;刘军安;甘勇;殷晓旭;龚言红;卢祖洵
【期刊名称】《中国社会医学杂志》
【年(卷),期】2024(41)3
【摘要】“社会医学”是研究社会因素对人群健康及疾病影响规律的一门医学学科,具有社会属性和人文属性。
文章在渗透教育理念的指导下,对“社会医学”思政元素渗透机制和渗透内容进行探索与设计,以促进思政因素与社会医学知识教学有机融合,并提出“社会医学”课程思政育人对策建议。
【总页数】4页(P269-272)
【作者】程子祎;赵明清;刘熠芳;刘军安;甘勇;殷晓旭;龚言红;卢祖洵
【作者单位】华中科技大学同济医学院公共卫生学院
【正文语种】中文
【中图分类】R-4;G641
【相关文献】
1.机电传动控制课程教学内容思政元素研究与探索——以《机电传动系统动力学基础》为例
2.将思政元素融入《工程热力学》课程教学内容的探索
3.中职钢琴教学内容融入思政元素的实践与探索
4.基于场景理论的高校课程思政立体渗透——以新安全格局思政元素渗透为例
因版权原因,仅展示原文概要,查看原文内容请购买。
(2,6-二戊基)-β环糊精作为毛细管气相色谱固定相分离对映体
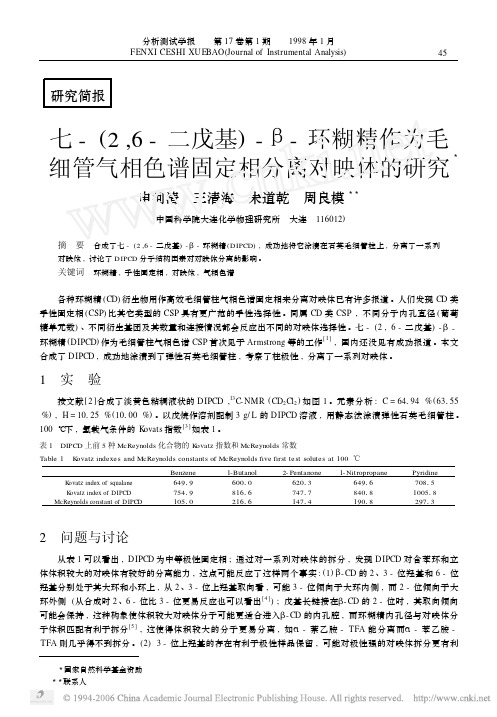
研究简报七-(2,6-二戊基)-β-环糊精作为毛细管气相色谱固定相分离对映体的研究3申河清 王清海 朱道乾 周良模33(中国科学院大连化学物理研究所 大连 116012)摘 要 合成了七-(2,6-二戊基)-β-环糊精(DIPCD ),成功地将它涂渍在石英毛细管柱上,分离了一系列对映体,讨论了DIPCD 分子结构因素对对映体分离的影响。
关键词 环糊精,手性固定相,对映体,气相色谱各种环糊精(CD )衍生物用作高效毛细管柱气相色谱固定相来分离对映体已有许多报道。
人们发现CD 类手性固定相(CSP )比其它类型的CSP 具有更广范的手性选择性。
同属CD 类CSP ,不同分子内孔直径(葡萄糖单元数)、不同衍生基团及其数量和连接情况都会反应出不同的对映体选择性。
七-(2,6-二戊基)-β-环糊精(DIPCD )作为毛细管柱气相色谱CSP 首次见于Armstrong 等的工作[1],国内还没见有成功报道。
本文合成了DIPCD ,成功地涂渍到了弹性石英毛细管柱,考察了柱极性,分离了一系列对映体。
3国家自然科学基金资助33联系人1 实 验按文献[2]合成了淡黄色粘稠液状的DIPCD ,13C -NMR (CD 2Cl 2)如图1。
元素分析:C =64194%(63155%),H =10125%(10100%)。
以戊烷作溶剂配制3g/L 的DIPCD 溶液,用静态法涂渍弹性石英毛细管柱。
100℃下,氢载气条件的K ovats 指数[3]如表1。
表1 DIPCD 上前5种McReynolds 化合物的K ovatz 指数和McReynolds 常数Table 1 K ovatz indexes and McReynolds constants of McReynolds five first test solutes at 100℃Benzene 1-Butanol 2-Pentanone 1-NitropropanePyridine K ovatz index of squalane6491960010620136491670815K ovatz index of DIPCD 75419816167471784018100518McReynolds constant of DIPCD10510216161471419018297132 问题与讨论从表1可以看出,DIPCD 为中等极性固定相;通过对一系列对映体的拆分,发现DIPCD 对含苯环和立体体积较大的对映体有较好的分离能力,这点可能反应了这样两个事实:(1)β-CD 的2、3-位羟基和6-位羟基分别处于其大环和小环上,从2、3-位上羟基取向看,可能3-位倾向于大环内侧,而2-位倾向于大环外侧(从合成时2、6-位比3-位更易反应也可以看出[4]);戊基长链接在β-CD 的2-位时,其取向倾向可能会保持,这种构象使体积较大对映体分子可能更适合进入β-CD 的内孔腔,而环糊精内孔径与对映体分子体积匹配有利于拆分[5],这使得体积较大的分子更易分离,如α-萘乙胺-TFA 能分离而α-苯乙胺-TFA 则几乎得不到拆分。
聚丙烯成核剂的现状与发展趋势
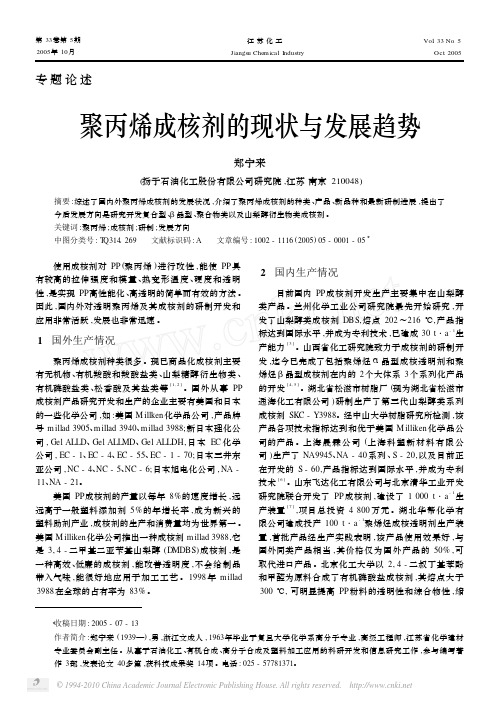
专用成核剂 。所开发成核剂能使 PP在凝固时形成球
形微晶体 ,以提高结晶速率 ,改善光泽和增加透明度 ,
赋予 PP制品多种优良性能 。烟台只楚合成化学有限
公司投资 2 000 多万元 ,在 APEC 中国烟台 (芝罘 ) 科
技工业园内建成 400 t·a - 1第三代 PP 成核剂 ZC - 3
的现代化工厂 [8 ] 。2003年 5月成功推出第三代山梨醇
3 成核剂种类
作为高性能成核剂必须具备条件是 : 成核剂是晶 体 ,在聚合物熔体中不熔 ,尤其在聚合物结晶前不熔 , 含有平行的层状或者排状分子 ,露出非极性表面 ,并将 极性基团限定在中心 ;成核剂允许聚合物附生结晶 ;能 跟聚合物发生反应 ,诱导结晶 。有机类聚丙烯成核剂 化学结构具有以下特点 [9 ] : ( 1) 分子中有苯环结构的 成核剂比脂肪烃结构的成核效果好 ; ( 2 ) 羧酸盐比游 离的羧酸成核效果好 ; ( 3 ) 钠盐比其他盐类成核剂的 成核效果好 ; ( 4) 苯环上有取代基时 ,对位聚代比其他 位取代的成核效果好 ; ( 5 ) 苯环与羧基之间的亚甲基 被长链取代后 ,成核效果变差 。
3收稿日期 : 2005 - 07 - 13 作者简介 :郑宁来 (1939—) ,男 ,浙江文成人 , 1963年毕业于复旦大学化学系高分子专业 ,高级工程师 ,江苏省化学建材 专业委员会副主任 。从事于石油化工 、有机合成 、高分子合成及塑料加工应用的科研开发和信息研究工作 ,参与编写著 作 3部 ,发表论文 40多篇 ,获科技成果奖 14项 。电话 : 025 - 57781371。
50
湖北省松滋市树脂厂
30
上海晟霖公司
200
山东飞达化工有限公司 1 000
《亚麻籽产品中氰苷的去除和SDG的释放及其定量分析研究》范文
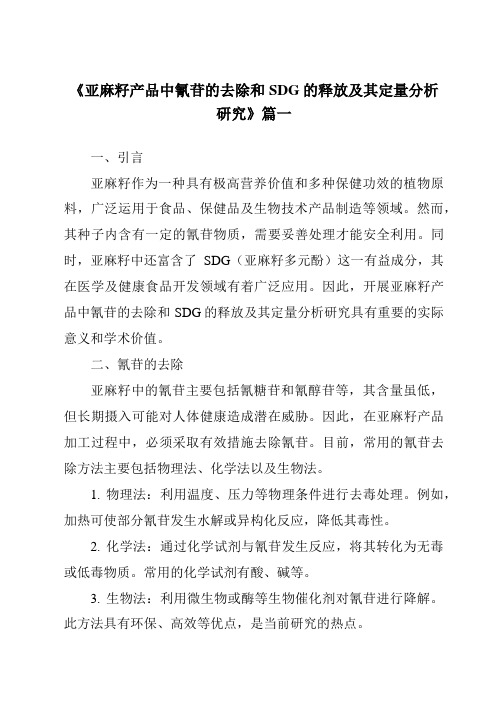
《亚麻籽产品中氰苷的去除和SDG的释放及其定量分析研究》篇一一、引言亚麻籽作为一种具有极高营养价值和多种保健功效的植物原料,广泛运用于食品、保健品及生物技术产品制造等领域。
然而,其种子内含有一定的氰苷物质,需要妥善处理才能安全利用。
同时,亚麻籽中还富含了SDG(亚麻籽多元酚)这一有益成分,其在医学及健康食品开发领域有着广泛应用。
因此,开展亚麻籽产品中氰苷的去除和SDG的释放及其定量分析研究具有重要的实际意义和学术价值。
二、氰苷的去除亚麻籽中的氰苷主要包括氰糖苷和氰醇苷等,其含量虽低,但长期摄入可能对人体健康造成潜在威胁。
因此,在亚麻籽产品加工过程中,必须采取有效措施去除氰苷。
目前,常用的氰苷去除方法主要包括物理法、化学法以及生物法。
1. 物理法:利用温度、压力等物理条件进行去毒处理。
例如,加热可使部分氰苷发生水解或异构化反应,降低其毒性。
2. 化学法:通过化学试剂与氰苷发生反应,将其转化为无毒或低毒物质。
常用的化学试剂有酸、碱等。
3. 生物法:利用微生物或酶等生物催化剂对氰苷进行降解。
此方法具有环保、高效等优点,是当前研究的热点。
三、SDG的释放亚麻籽中的SDG通常与其它多酚物质形成复杂的化合物或与细胞壁结构结合。
因此,要充分释放SDG并发挥其作用,需要在提取和加工过程中采用特定的技术和条件。
目前常见的SDG释放技术包括超声提取、酶解法等。
1. 超声提取法:利用超声波的机械效应和空化效应加速亚麻籽中SDG的释放。
此方法具有操作简便、效率高等优点。
2. 酶解法:通过添加特定酶类(如多酚氧化酶)来催化SDG 的释放。
此方法具有针对性强、反应条件温和等优点。
四、定量分析研究为了准确评估亚麻籽产品中氰苷的去除效果和SDG的含量,需要采用合适的定量分析方法进行检测。
目前常用的分析方法包括光谱法、色谱法和电化学法等。
1. 光谱法:利用紫外-可见光谱、红外光谱等对样品进行定性或定量分析。
此方法具有操作简便、快速等优点。
数字时代学习场域“身体—技术”的可供性生产与响应式协商

数字时代学习场域“身体—技术”的可供性生产与响应式协商作者:杨霞来源:《电化教育研究》2024年第06期基金项目:2023年度国家社会科学基金教育学一般项目“统编教材的中华民族共同体意识建构及其教学实现研究”(项目编号:BHA230135)[摘要] 数字时代“身体—技术”的关系变得更加复杂和不确定。
技术可供性作为解释“技术与人互动关系”的最具代表性的一种阐释框架,可为学习者“身体—技术”关系提供新的分析视角,体现为在特定环境下,技术对学习者身体在生理、心理以及时空方面的可供性生产。
在此基础上,为充分发挥技术对学习主体身体的形塑作用,为学习活动提质增效,需要从双向互构的动态视角探究“身体—技术”的协商关系,一是强化技术具身感知,提升学习主体自我意识;二是构建场景学习模式,延展学习主体过程体验;三是确立个体时空边界,构建学习主体身份认同。
[关键词] 数字时代;技术可供性;技术具身;主体身份[中图分类号] G434 [文献标志码] A[作者简介] 杨霞(1995—),女,重庆人。
博士研究生,主要从事课程与教学基本原理、教师教育研究。
E-mail:*****************。
一、问题的提出当前,虚拟现实、数字孪生、人工智能等技术的发展带来了个体身体与技术的耦合,技术正日益“侵入、重构并愈益支配身体的内容”[1]。
这就为“身体—技术”这一主题提供了新的讨论场域,但也模糊了“身体”与“技术”原本清晰而明确的界限,“虚拟身体”“数字化身”等概念更是挑战了传统的身体观念。
学界势必将重新思考“技术与身体是何种关系”“技术如何影响身体变革”“技术变革过程中如何理解身体与学习”等议题。
近年来,相关研究已经在此类问题上做出了有益尝试,但总体上是将“身体—技术”问题附属于“教育与技术融合”的整体框架之中,较为零散且尚未形成完善的理论体系。
与此同时,不断更新的技术形式和身体实践,也在丰富着学习主体的内涵。
相较于数字技术的快速发展,目前人们对于身体与技术的思考仍然是匮乏的,对如何深入推进身体与技术的共生共进有待进一步探讨。
夹缝中的应对与坚守———论任职民国教育总长之际的任可澄文学研究
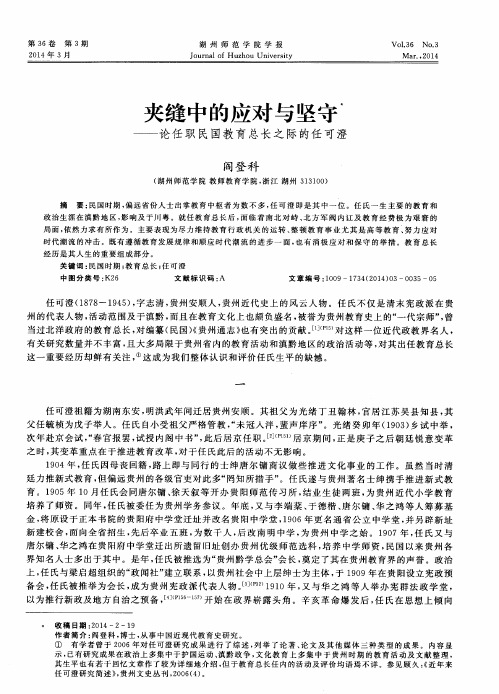
任 可澄 ( 1 8 7 8 —1 9 4 5 ) , 字 志清 , 贵 州安顺 人 , 贵 州近 代史 上 的 风 云人 物 。任 氏不 仅是 清 末 宪政 派 在 贵 州 的代 表人 物 , 活动 范 围及于 滇黔 , 而且 在 教育 文化 上也 颇负 盛名 , 被誉 为 贵州 教育史 上 的“ 一 代宗 师” , 曾 当过 北洋 政府 的教育 总长 , 对 编纂 ( 民国) 《 贵州通 志 》 也有 突 出 的贡献 。 I l l ( P 1 5 ) 对这样 一 位近代 政 教界 名人 , 有关 研究 数 量并 不丰 富 , 且 大 多局 限 于贵州 省 内的教 育 活动 和滇黔 地 区 的政 治 活动 等 , 对 其 出任教 育总 长
第 3 6卷
第 3期
湖 卅 I 师 范 学 院 学 报
J o u r n a l o f Hu z h o u Un i v e r s i t y
Vo l I 3 6 No . 3
Ma r . , 2O1 4
2 01 4年 3月
夹缝 中 的应对 与坚守
— —
论 任 职 民 国教 育 总长 之 际 的任 可澄
这一 重要 经 历却 鲜有 关注 , ① 这 成 为我们 整 体认识 和 评价 任 氏生平 的缺 憾 。
任可 澄祖 籍 为湖 南东 安 , 明洪武 年 间迁 居贵 州安 顺 。其 祖父 为 光 绪 丁丑 翰 林 , 官 居 江苏 吴 县 知县 , 其 父任 毓桢 为戊 子举 人 。任 氏 自小受 祖父 严格 管教 , “ 未 冠入 泮 , 蜚 声庠 序 ” 。光 绪癸 卯 年 ( 1 9 0 3 ) 乡试 中举 , 次年 赴京 会试 , “ 春 官报 罢 , 试授 内阁 中 书” , 此 后 居京 任 职 。 [ 2 ] ( n ”居 京期 间 , 正 是 庚 子 之后 朝1 4—2—1 9
研究生学位论文开题报告-腈水合酶酶学性质、结构、功能及其基因蔟结构的研究
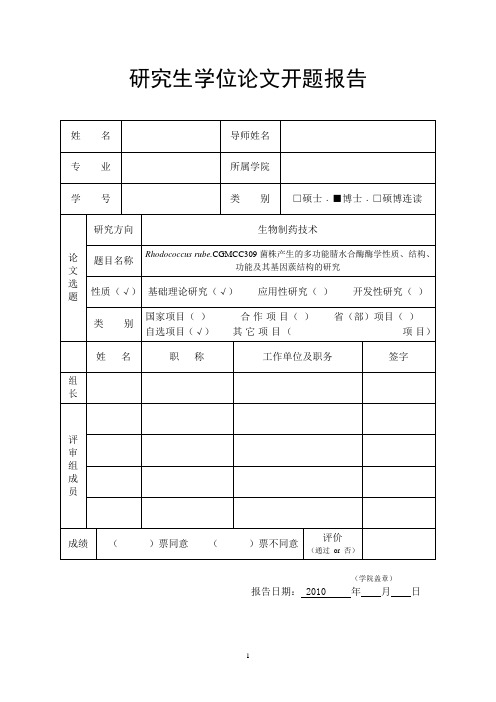
2、本课题研究领域在国内外的研究动态及发展趋势:
2.1 腈水合酶理论的研究动态及发展趋势 腈水合酶(NHase; E.C.4.2.1.84)催化腈的水合作用转变成相应的酰胺。 酰胺然后经酰胺酶 (E.C.3.5.1.4).转化成羟基酸。这组酶之所以受到广泛的研究主要是因为它们作为催化剂成功 地应用于大规模的商业生物转化之中,由Nitto化学公司进行的大规模的工业化转化生产 (6000t/年) 这一成就的取得是由于使用了来自Pseudomonas chlororaphis B23菌株的腈水 。 合酶[6] 。使用Rhodococcus rhodochrous J1产生的腈水合酶,这一过程进一步改进,使得年 产丙烯酰胺达30000吨以上[7]。另一个方面他们对环境生物修复具有潜在的应用价值。生物 催化优于传统方法的优势[8,9]有很多,对环境更加友好,使合成产量更高,并具有化学、区 域和立体选择性,在更加的温和的反应条件下进行的特点。 2.1.1 腈代谢酶的发现、分布和分离 许多微生物菌株可以经亚乙基羟胺水化酶作用下,代谢催化亚乙基羟胺[10]。亚乙基羟 胺是合成天然产物的中间体。 亚乙基羟胺水解酶基因像腈代谢酶 (腈水合酶和酰胺酶) 一样, 具有同样基因蔟和操纵子。该途径的作用是分流亚乙基羟胺进入中心能量代谢机制。在
3
和钴型(Co-type)腈水合酶[32,33]。大多数腈水合酶的最小的功能单元由两个亚基组成,还包括 一个金属离子单位。迄今为止,至少有两个已经被描述的酶有同型二聚体功能单元,以2α 表示(Agrobacterium tumefaciens[34] (Trott et al., 2001) 和Corynebacterium sp. C5 [35] (Yamamoto et al.,1992)),一个有一个单体功能单元。不同亚基α和β的大小范围从23kDa到 30kDa不等。天然分子量在30kDa[36](Rhodococcus equi SHB-121菌)和500-530kDa[37] ( Rhodococcus rhodochrous J1之间。这样腈水合酶就分成了轻型腈水合酶(L-NHase)和重型 腈水合酶(H-NHase),特别是来自于Rhodococcus rhodochrous J1.的酶。几个腈水合酶的晶体 结构已经阐明(Rhodococcus sp. R312,程序数据库登陆号[38]为1AHJ/2AHJ;Rhodococcus sp. N-771[39],Nagashima et al.,1998;Pseudomonas thermophila,程序数据库登陆号[40]为1IRE, Miyanaga et al.,2001;Bacillus smithii SC-J05,程序数据库登陆号[41]为1V29,Hourai et al., 2003)。所有这些酶大体折叠层次是保守的。第一个细致描述的腈水合酶结构,分辨率是 2.65,报道于1997年(Huang et al., 1997)。这个酶属于铁型亚群。酶的α亚基由一个长的延 伸的N-端臂和一个球状的C-端结构域组成。这个C-端的球状结构域很不寻常,详细的有4层 结构,即α-β-β-α。β亚基以30个氨基酸残基的长环开始,在αβ双体内将α亚基包缠起来。随 着是5个螺旋结构域,跟着是一个由6个反平行链组成圆环。Rhodococcus sp. R312的腈水合 酶的α和β亚基形成了一个紧密的二聚体结构,带有一个很大的3800 2内表面积。铁中心位 于内部表面积中的一个大的开放洞穴内部,与金属离子配位的蛋白配基由α亚基提供。 cys110α, cys113α, cys115α的侧链和ser114αand cys115α残基的主链酰胺氮原子形成了配体 球。在晶体结构中观察到的配体球是这样的形式S3N2X,带有5个一致的配体,占据八面体 的5个顶点。第6个位置(由X代表)被推测是一个氢氧化合物或水分子以酶的活性形式存在 和一氧化氮以酶的非活性形式存在[42]。来自于保守残基arg141和arg56的胍基同来自于 cys115α和 cys113α的硫原子形成氢键,这样就保正了他们的正确的位置。来自Rhodococcus sp. N-771菌株的另一个铁型腈水合酶[43] (Nagashima et al., 1998)表明cys114 αand cys112α (相 当于Rhodococcus sp. R312菌株的cys115α和cys113 α)是转录后各自被修饰成半胱次磺酸和 半胱亚磺酸。在这个酶中,arg141β和 arg56β同这些修饰的半胱残基的氧原子形成氢键。现 已表明, α亚基的Val-Cys-Ser(Thr)-Leu-Cys-Ser-Cys序列形成了金属结合模型 (即爪状模型) , [44] 在所有腈水合酶中 都具有高度的保守性。钴型腈水合酶有一个苏氨酸替代了铁型腈水合 酶的丝氨酸。 这种取代方式一般认为是对于不同的辅因子有不同的偏好。 由金属离子占据的 开放洞穴接近弥散的溶胶中, 被认为是酶的可能的活性位点。 这种观点已经受到了底物类似 物(碘乙腈)进入Rhodococcus sp. R312菌株(Huang et al.,1997)晶体结构的活性位点口袋成功 模拟的支持。 根据这种模型已经提出了三种不同的催化机理。 所有机制都需要金属离子充当 路易斯酸作用激活腈的水合反应。由于不需要配体交换,一般认为,这种机制最可能是腈的 间接性激活。三价离子的配体交换十分缓慢,致使此机制很少受到欢迎。现已阐明[45]R. rhodochrous sp. J1菌株的钴型腈水合酶与Rhodococcus sp. R312菌株的铁型腈水合酶在相同 的反应条件下,水解丙腈的反应效率几乎一致。由于低自旋铁(III)和钴(III)的配体交换动力 学特征明显不同, 这两种酶类似反应性质暗示这些酶反应机制不需要配体的交换。 在由黄等 人推崇的机制中, 即所谓的外球体机制, 有一个氢氧离子充当一般的碱在活性位点激活水分 子,这样的水分子反过来攻击腈。已经报道嗜热菌株Pseudonocardia thermophila JCM 3095 的钴型腈水合酶晶体结构[46]。该结构(分辨率:1.8)显示与适温腈水合酶有很高的结构 相似性。 然而两种酶的明显区别在于P. thermophila腈水合酶的α和β亚基之间有附加的相互作 用, 这是由两个额外的螺旋引起的, 这表明这种额外的相互作用很可能有助于酶的热稳定性。 钴型腈水合酶的活性中心(金属结合位点)像铁型酶一样有相同的爪状模型,与钴离子配位 的配体是由cys108α , cys111α and cys113α残基的硫原子和ser112α and cys113 α的主链酰胺氮 原子之间形成的。电子密度峰指出cys111α and cys113α在转录后分别氧化成半胱次磺酸和半 胱亚磺酸,这已经确定无疑[47]。在Rhodococcus sp. N-771腈水合酶[48]中,由于来自这些修饰
抗体药物Fc段结构域改造研究进展

抗体药物Fc段结构域改造研究进展
刘爽;孙钰芳;邱熙然;安毛毛;慎慧
【期刊名称】《中国临床医学》
【年(卷),期】2024(31)1
【摘要】抗体是生物体内应对外来物质入侵时产生的主要保护物质,是保护生物体的重要分子。
对抗体的Fc段进行改造可有效延长抗体的半衰期,大大减少给药剂量;Fc段介导的抗体效应子的功能也可以根据疾病特殊性进行上调或下调,实现治疗效果最大化。
同时,仅有Fc片段的抗体,也可具备结合抗原的能力,通过缩小抗体分子的大小,可大大减少抗体分子量大的限制,为抗体药物的开发提供了新的方向。
此外,除抗肿瘤作用外,抗体分子在抗感染领域也发挥了重要的作用。
对抗感染中和抗体Fc区进行工程改造,可有效促进保护性的细胞免疫效应,增强抗病毒活性并延长半衰期。
本文总结了抗体Fc结构域的功能特性及优化策略,并介绍Fc结构域优化改造所介导的生物学效应及在临床治疗中的应用与发展。
【总页数】11页(P143-153)
【作者】刘爽;孙钰芳;邱熙然;安毛毛;慎慧
【作者单位】同济大学附属第十人民医院药学部;同济大学附属第十人民医院检验科
【正文语种】中文
【中图分类】R915
【相关文献】
1.猪IgGⅡB类Fc受体剪接异构体胞外区及结构域的原核表达与抗体制备
2.人IgE-Fc结构域的基因克隆、原核表达及单克隆抗体的制备
3.变应性鼻炎IgE高亲和力可溶型Fc段受体1α(sFcε1α)蛋白的制备及血清中相应抗体的检测
4.Fc段改造单克隆抗体在肿瘤治疗中的应用
因版权原因,仅展示原文概要,查看原文内容请购买。
智能响应型Pickering乳液的制备及在物质分离中的应用进展

化工进展CHEMICAL INDUSTRY AND ENGINEERING PROGRESS2021年第40卷第4期智能响应型Pickering 乳液的制备及在物质分离中的应用进展程芳琴,焦玉花,李恩泽,康锦,王旭明(山西大学资源与环境工程研究所,山西太原030006)摘要:Pickering 乳液是指由微纳米固体粒子代替传统表面活性剂作为乳化剂而稳定的乳液,具有较强的稳定性和超高油/水界面,能够为多相界面反应和物质传输提供高效稳定的场所。
Pickering 乳液的乳滴结构和性质与固体颗粒的尺寸形貌及表面性质密不可分,通过调控固体颗粒本身或表面的性质可以赋予Pickering 乳液特定的响应性功能,拓宽其应用领域。
本文对近年来不同响应型(磁性、CO 2、pH 、光、温度等响应型)的Pickering 乳液的主要研究成果进行了综述,重点介绍了Pickering 乳液的稳定性原理、响应型Pickering 乳液的制备方法和结构调控策略,以及近年来Pickering 乳液在物质分离提取中的应用研究进展,最后对智能响应型Pickering 乳液应用研究的发展趋势进行了展望。
关键词:Pickering 乳液;粒子;响应型;稳定原理;表面;结构调控;传质中图分类号:TD98文献标志码:A文章编号:1000-6613(2021)04-2206-09Progress on the preparation of intelligent responsive Pickering emulsionsand their applications in matter separationCHENG Fangqin ,JIAO Yuhua ,LI Enze ,KANG Jin ,WANG Xuming(Institute of Resources and Environmental Engineering,Shanxi University,Taiyuan 030006,Shanxi,China)Abstract:Pickering emulsion refers to an emulsion that is stabilized by micro-nano solid particles instead of traditional surfactants as an emulsifier.It has strong stability and an ultra-high oil/water interface,which can provide an efficient and stable place for multi-phase interface reactions and mass transport.The structure of emulsion droplet and properties of Pickering emulsion depends on the size,morphology and surface properties of the solid particles.Pickering emulsions can be endowed with specific responsive functions via adjusting the properties of the solid particles or their surfaces,resulting in wide application fields.This paper reviewed the main research results of Pickering emulsions with different response types (magnetic,CO 2,pH,light,temperature and other response types),focusing on the stability principle of Pickering emulsions,the preparation methods and structural regulation of response Pickering emulsions,and the recent application research progress of Pickering emulsion in matter separation.Finally,the development trend of the application research of Pickering emulsion was prospected.Keywords:Pickering emulsions;particles;responsive;stabilization principle;surface;structure regulation;mass transfer综述与专论DOI :10.16085/j.issn.1000-6613.2020-1048收稿日期:2020-06-10;修改稿日期:2020-07-07。
一种计算水溶解度的经验加合模型的适用范围与局限

一种计算水溶解度的经验加合模型的适用范围与局限段宝根李嫣李婕程铁军王任小*(中国科学院上海有机化学研究所,生命有机化学国家重点实验室,上海200032)摘要:我们发展了一种用于预测有机小分子化合物水溶解度(log S )的经验方法XLOGS.它本质上是一种加合模型,采用83种原子/基团类型和3个校正因子作为模型的描述符.该方法还可以根据一个合适的参照分子的log S 实验值来计算未知化合物的log S 值.我们将XLOGS 模型在由4171个化合物组成的训练集上进行了参数化,多元线性回归获得的相关系数(R 2)和标准偏差(SD)分别为0.82和0.96单位.将该训练集进一步分为仅含液体化合物和仅含固体化合物的两个子集.XLOGS 模型在这两个子集上的回归结果显示前者优于后者(标准偏差分别为0.65单位和0.94单位).还利用log1/S 和log P (脂水分配系数)之间的差值来研究XLOGS 方法在液体和固体化合物数据集上的表现.研究结果表明:XLOGS 等加合法模型更适合应用于这两者差值接近于0的化合物.还将XLOGS 和其它三种流行的log S 计算模型(包括Qikprop,MOE-log S 和ALOGPS)在一个含有132个类药化合物的独立测试集上进行了比较.总体而言,我们的研究结果为加合法模型在水溶解度预测方面的合理应用提供了指导.关键词:水溶解度;加合法模型;XLOGS中图分类号:O645An Empirical Additive Model for Aqueous Solubility Computation:Success and LimitationsDUAN Bao-GenLI YanLI JieCHENG Tie-JunWANG Ren-Xiao *(State Key Laboratory of Bioorganic Chemistry,Shanghai Institute of Organic Chemistry,Chinese Academy of Sciences,Shanghai 200032,P .R.China )Abstract:We have developed a new empirical model,namely XLOGS,for computing aqueous solubility (log S )of organic compounds.This model is essentially an additive model,which employs a total of 83atom/group types and three correction factors as descriptors.Furthermore,it computes the log S value of a query compound by using the known log S value of an appropriate reference molecule as a starting point.XLOGS was calibrated on a training set of 4171compounds with known log S values.The squared correlation coefficient (R 2)and standard deviation (SD)in regression were 0.82and 0.96log units,respectively.The entire training set was further split into one subset containing liquid compounds only and another subset containing solid compounds only.Regression results of XLOGS were obviously better on the former subset (SD=0.65vs 0.94log units).The difference between log1/S and log P (partition coefficient,the ratio of concentrations of a compound in a mixture of water and n -octanol at equilibrium)was used as an indicator to investigate the performance of XLOGS on liquid compounds and solid compounds.Our results suggested that an additive model like XLOGS performed most satisfactorily when this difference was close to zero.Three other log S models,including Qikprop,MOE-log S ,and ALOGPS,were also compared with XLOGS on an independent test set of 132drug-like compounds.Put together,our study provides some[Article]doi:10.3866/PKU.WHXB201209171物理化学学报(Wuli Huaxue Xuebao )Acta Phys.-Chim.Sin .2012,28(10),2249-2257October Received:July 16,2012;Revised:September 12,2012;Published on Web:September 17,2012.∗Corresponding author.Email:wangrx@;Tel:+86-21-54925128.The project was supported by the National Natural Science Foundation of China (81172984,21072213,21002117,21102168,21102165)and National High-Tech Research and Development Program of China (863)(2012AA020308).国家自然科学基金(81172984,21072213,21002117,21102168,21102165)和国家高技术研究发展计划(863)(2012AA020308)资助项目ⒸEditorial office of Acta Physico-Chimica Sinica2249Acta Phys.-Chim.Sin.2012Vol.28 general guidance for applying additive models to computation of aqueous solubility.Key Words:Aqueous solubility;Additive model;XLOGS1IntroductionAqueous solubility is perhaps the most important physico-chemical property for orally available drugs since it affects ab-sorption,distribution,metabolism,and elimination processes.1,2 Poor solubility is often associated with poor druggability.Tra-ditionally,solubility of a compound is measured experimental-ly through an equilibrium approach.However,this approach re-quires a fair amount of sample(1-2mg)and is time-consum-ing(tens of hours to complete properly).3Besides,compound stability could be a serious issue in such measurement.Other approaches,such as nephelometry,4provide a kinetic solubility measurement with a low amount of sample,but they require a reliable dimethyl sulfoxide(DMSO)stock solution and multi-ple repeats to achieve accuracy.3Since experimental measurement of solubility is often diffi-cult to carry out,especially in a high-throughput scenario,theo-retical methods serve as an alternative approach to predict solu-bility.Many methods have been proposed for this purpose.So far the most popular methods are empirical methods.Such methods can be classified roughly into two categories.(i) Quantitative structure-property relationships(QSPR)models. Aqueous solubility is either correlated with other experimental properties,5-7such as partition coefficient,melting point and so on,or molecular descriptors,8-12such as topological indices,sol-vent accessible surface area,and the numbers of donor and ac-ceptor of hydrogen bonds and so on,through all sorts of data mining approaches.(ii)Atom/group additive models.13-16These models are based on the basic assumption that the physico-chemical properties of a molecule can be described as a sum of the contributions from its parts,i.e.,chemical fragments.In oth-er words,structural building blocks are directly used in such methods as descriptors to correlate with solubility or other properties.In addition,some“correction factors”are intro-duced to compensate any derivations from pure addition in or-der to improve accuracy further.Empirical methods are convenient to use in practice.Never-theless,a potential disadvantage of these methods is that their application may be limited by their training sets.If a query molecule is outside the training set of an empirical method, then the prediction made by this method is often less reason-able.Furthermore,these methods,especially QSPR models, cannot provide physical insights into the solvation process.A more fundamental approach to solubility computation is based on free energy calculations and thermodynamics relations.17 There have been many studies in this field during the past20 years.For example,Lindfors et al.18-21developed a model for estimating the amorphous solubility of drugs in water.They firstly computed the free energy of solvation in pure melt at 673.17K using Monte Carlo simulations.Secondly,melting drug crystals and then rapidly cooled the melt to obtain the amorphous phase.The free energy associated with this process was computed.Then,the free energy change in bringing a drug molecule from the vapor into a pure drug amorphous phase was obtained,plus the hydration free energy allowed the solu-bility of amorphous drugs to be determined in water.Mitchell et al.22reported methods to predict the intrinsic solubility of crystalline organic molecules with two different thermodynam-ic cycles.They found that a mixed model of direct computa-tions and informatics,which relied on the calculated thermody-namic properties and a few more key descriptors,yielded good results.On an external test set containing drug molecules,their model yielded R2=0.77and RMSE=0.71log units between ex-perimental and calculated data.Here,R2is coefficient of deter-mination,which reflects the goodness of curve fitting.The val-ue closer to1means better fitting between the regression equa-tion and the input data.RMSE is the abbreviation for root-mean-square-error,which is used to measure the deviations be-tween the fitted value and the experimental data.The smaller RMSE indicates better fitting effect.Chebil and co-workers23 used experimental data and all-atom molecular dynamics simu-lation to predict quercetin solubility in different solvents.If the experimental solubility of quercetin in one solvent is known, plus the hydration free energy computed from all-atom molecu-lar dynamics simulation,its solubility in other solvent can be determined.Compared to empirical models,methods based on thermody-namic energy computation can explain the solvation mechanics from a physical point of view.However,they are computation-ally much more expensive and thus not suitable for high-throughput tasks.Moreover,lattice energy,which needs to be compensated in the solvation of a solid compound,is still diffi-cult to be computed accurately.These problems certainly limit the application of such methods in a wider range.Thus,empiri-cal methods and first principles-based methods will co-exist in this field in the foreseeable future.In this study,we aimed at improving the accuracy of addi-tive model.Here,we describe a new model for log S computa-tion,i.e.,XLOGS,by combining a knowledge-based approach with a conventional additive model.This approach is based on the assumption that compounds with similar chemical struc-tures are associated with similar properties,a strategy that has been successfully applied in some research.24-27By this ap-proach,the log S value of a given compound is computed based on the known log S value of an appropriate reference molecule.2Methods2250DUAN Bao-Gen et al.:An Empirical Additive Model for Aqueous Solubility Computation:Success and Limitations No.102.1Data set preparationA set of organic molecules with experimental aqueous solu-bility data was necessary for calibrating our empirical model. This training set was selected from the PHYSPROP database (/esc/physdemo.htm),which is probably the largest compilation of such data available to the public.Accept-ed molecules were selected with the following criteria.Firstly, only the molecules with experimental aqueous solubility data were considered since not every molecule included in the PHYSPROP database has this information.Secondly,only sol-ubility data measured at room temperature,i.e.,20-25°C,were considered.Thirdly,each qualified molecule must not contain atoms other than hydrogen,carbon,oxygen,nitrogen,sulfur, phosphorus,and halogen atoms.As a result,a total of4544 molecules were selected.The chemical structures of these mol-ecules provided by the PHYSPROP database were then manu-ally examined.Gas molecules,salts,or mixtures at room tem-perature were excluded.False molecular structures were cor-rected.Finally,a total of4217molecules were included in our training set.An independent test set was also employed to verify the pre-dictive power of our model.It was cited from the“solubility challenge”launched by Llinas et al.28,29recently.This set con-tains132drug-like compounds(Table1),which are generally more complex than those in the training set.Molecular structur-al files of this set of molecules were downloaded directly from PubChem(/).30Note that a to-tal of46molecules in this test set overlapped with our training set described above.Thus,these molecules were removed from the training set,leaving the total number of molecules in our training set to be4171.This final training set will be referred to as“Set I”throughout this article.Set I was further classified according to the state informa-tion(liquid or solid)of each compound.State information is available in the PHYSPROP database for3569molecules in Set I.Among them,989molecules that are liquid at room tem-perature were assembled as“Set II”.Among the compounds that are solid at room temperature,experimentally measured melting point data were available for2357of them.They will be referred to as“Set III”throughout this article.In our study, Set II and Set III were also employed for deriving additive models specifically applicable to liquid and solid compounds, respectively.Some basic features of the training sets and the test set used in this study are listed in Table1.2.2The pure addictive model:XLOGS-AAThe additive model described in this study,i.e.XLOGS,is based essentially on the use of some atom types rather than chemical fragments.The advantage of an atom-based model is that a molecular structure can always be dissected into atoms without any ambiguity.Such a model is also much more straightforward to implement and is in principle free of the missing-fragment problem.In XLOGS method,83basic atom/group types are defined according to the rules set by the XLOGP3method25for Sets I and III,and73atom/group types for Set II.Details of atom types are given in Table S1in Supporting Information.In addi-tion,three correction factors are introduced in XLOGS.The first correction factor(“HB”)accounts for intramolecular hy-drogen bonds,which weaken the interactions between solute and water.The second correction factor(“AA”)is used on or-ganic compounds withα-amino acid moieties.Such com-pounds usually exist in a zwitterionic form at the neutral pH condition,which is certainly very different from the corre-sponding neutral form in solvation.The third correction factor (“HDHA”)is the product of the number of hydrogen bond do-nors and the number of hydrogen bond acceptors on the solute molecule.It is an indication of the favorable polar interactions between solute and water.The additive model described above will be referred to as XLOGS-AA throughout the rest of the ar-ticle for the convenience.2.3The knowledge-based modelThe key idea of XLOGS is to calculate the log S value of a given compound from the known log S of a molecule with simi-lar structure.A conventional additive model computes log S aslog S=∑i=1M a i A i+∑j=1N c j C j(1) Here,a i and A i are the contribution and occurrence of the i th at-om/group type in the given compound,respectively.c j and C j are the contribution and the occurrence of the j th correction fac-tor.M is the total number of defined atom/group types and N is the total number of defined correction factors.The XLOGS method is to compute the log S of a given compound from the known log S of a structural analog,i.e.,the reference molecule. log S of the reference molecule is computed by an additive mod-el as;log S0=∑i=1M a i A0i+∑j=1N c j C0j(2) By subtracting Eq.(2)from Eq.(1),one getslog S=log S0+∑i=1M a i()A i-A0i+∑j=1N c j()C j-C0j(3) Here,S0means the log S value of the reference molecule mea-sured by experiment.A0and C0are calculated contributions ofSet I(N=4171) Set II(N=989) Set III(N=2357) test set(N=132)Molecular weight220.6(±78.4)a154.5(±60.0)247.3(±74.3)300.1(±72.2)Average number of heavy atoms14.3(±5.1)9.8(±3.8)16.1(±4.6)20.6(±5.1)Average number of oxygen and nitrogen atoms3.3(±2.0)1.6(±1.3)3.7(±1.9)4.5(±1.9)Experimental log S-2.80(±1.76)-1.99(±1.59)-3.30(±1.89)-3.34(±1.17)a The data outside brackets are mean values;the data inside brackets are standard deviations.Table1Some basic properties of the data sets considered in this study2251Acta Phys.-Chim.Sin.2012Vol.28log S for the reference molecule by atom/group types and cor-rection factors,respectively.Then,the log S value of a given compound can be computed by Eq.(3)based on the known log S value of a reference molecule.This model is called XLOGS-full in our paper.This concept is illustrated in Fig.1with an example.In principle,the reference molecule should be found among a large set of organic compounds with known log S values.For the sake of convenience,in our study the training set used for calibrating XLOGS-AA was also employed as the knowledge set for finding the appropriate reference molecule.Obviously,the reference molecule should resemble the query compound as much as possible in terms of chemical structure.In our study,the structural similarity between any two molecules was computed with an algorithm based on topological torsion de-scriptors described previously.25,31For a given query molecule,a similarity threshold of 50%was applied to search the entire knowledge set.The molecule with the highest similarity score was selected to be the reference.If no molecule in the knowl-edge set met the similarity threshold of 50%,the pure additive model,i.e.,XLOGS-AA,was applied for instead to compute the log S value of the query.2.4Analysis of the relationship between solubilityand partition coefficientPartition coefficient (log P )is the ratio of concentrations of a compound between n -octanol and water phases.Conventional additive models work well for calculating log P .But this is not the case for solubility,where the effects like electron donating/accepting contributions of substituents,and intramolecular hy-drogen bonding can play an important role.Such complex ef-fects cannot be properly described solely by fragment contribu-tions.32If these complex effects on solubility are dominating,the prediction accuracy of an additive model may be poor.Some studies have attempted to correlate log S with log P .5,33-36In these studies,the log P value came from either experiment or the computational methods.It was also found that the main fac-tor in solubility was due to its lipophilicity for a particular scaf-fold of compounds.37In brief,the relationship between log S and log P can reflect the predictive power of an additive model of solubility to some extents.For example,a simple parameter called ΔSL was used to define the threshold of lipophilicity on solubility in Faller ʹs study:32ΔSL =log 1/S -log P (4)Here,log1/S is the reciprocal solubility value in molar terms.This parameter was introduced to separate lipophilicity from other contributions to solubility.If the ΔSL value is close to ze-ro,it indicates that solubility is dominated by lipophilicity.Then,additive models have their advantages in solubility pre-diction.When the ΔSL value deviates from zero,it indicates that some nonlinear effects on solubility become more impor-tant,and thus additive models may not be appropriate in this case.In addition,a variable (ΔRMSE)was defined in our study to measure the change in the accuracy of an additive model with the change in the contents of data set:ΔRMSE =RMSE new -RMSE oldN new -N old(5)Here,RMSE old and RMSE new are the RMSE values of a given ad-ditive model before and after some molecules with large ΔSL values are added to the data set,respectively;N old and N new are the size of the original data set and the new data set,respective-ly.2.5Other log S methods under evaluationIn order to make a comparison,three popular models for sol-ubility calculation were applied to the same data sets for test-ing XLOGS,including the function in the molecular descriptor module in MOE software (version 2010),Qikprop in Schro-dinger software (version 2011),and ALOGPS (version 2.1)available online at /lab/alogps/start.html.3Results and discussion3.1Regression results and model validationFig.1Illustration of the basic computational procedure ofXLOGS2252DUAN Bao-Gen et al .:An Empirical Additive Model for Aqueous Solubility Computation:Success and Limitations No.10A total of 86descriptors were used in XLOGS-AA for Set I,including 83basic atom/group types and three correction fac-tors.Contributions of all descriptors were obtained by multivar-iate linear regression analysis on the 4171compounds in Set I (Table S1(Supporting Information)).The R 2between experi-mental and calculated values is 0.82,and the RMSE is 0.96log units (Fig.2).A standard leave-one-out cross-validation was conducted to test the predictive power of this model,producing a Q 2(cross validation coefficient,which indicates the predic-tion power of the model)value of 0.81,and a RMSE value of 0.98log units.The results of leave-one-out cross-validation test are very close to the ones obtained from regression analy-sis,indicating that XLOGS-AA is not an over-fitted model.Moreover,the XLOGS method (Eq.(3))was also applied to the entire Set I.A threshold of 50%was adopted in similarity comparison.Among all of the 4171compounds in Set I,quali-fied reference molecules were found for 2386compounds,ac-counting for 57.2%of the entire data set.Other compounds were still computed with the pure additive XLOGS-AA model.The final regression results of the XLOGS model are:R 2=0.83,RMSE=0.94log pared to the results produced by XLOGS-AA,the improvement is rather limited.The reason is that many compounds in this data set cannot find the appropri-ate reference molecule,and thus the power of XLOGS is not fully verified.To make a comparison,three log S models in popular commer-cial software,including MOE-log S ,Qikprop,and ALOGPS,were also applied to Set I.The statistical results are summa-rized in Table 2.One can see that the performance of both XLOGS and XLOGS-AA are marginally better than the other three models.As described in the Methods section,the “solubility chal-lenge ”data set 28,29were adopted as an independent test set to test XLOGS.Among the 132compounds in this data set,elev-en compounds were excluded in our study because their aque-ous solubility values were not accurate.28,29The XLOGS model was applied to the remaining 121compounds,producing a R 2value between experimental and calculated values of 0.47,and a RMSE value of 1.08log units (Fig.3).Correlations between the experimental values and the calculated values by MOE-log S ,Qikprop,and ALOGPS are not high either (Table 3).Nev-ertheless,the statistical results produced by these three models are marginally better than XLOGS on this test set.This could be a coincidence since this test set is relatively small.It should be mentioned that XLOGS was actually applied to only 28mol-ecules in this test set while the remaining molecules were pro-cessed by XLOGS-AA due to lack of appropriate reference molecules in Set I.Thus,there is no significant difference be-tween the statistical results produced by XLOGS and XLOGS-AA.3.2Performance of additive model on Set II and Set III Dissolution of a compound in water is controlled by two types of interactions:3one is solute -solvent interaction,and the other is the internal interactions between solute molecules.Basically,solute -solvent interactions need to be strong enough to compensate solute -solute interactions in order to make a molecule soluble in water.As for liquid compounds,their solu-bility is mainly affected by the first type of interaction.As forFig.2Experimental log S values versus calculated values byXLOGS-AA on the entire training setN =4171,R 2=0.82,SD=0.96logunitsTable 2Results of different log S methods on three training setsSD:standard deviation in regression;AUE:average unsigned error between calculated and experimental dataModel XLOGS-full XLOGS-AA ALOGPS MOE-log S QikpropSet I (N =4171)R20.830.820.800.780.75SD 0.940.961.001.071.14AUE 0.690.710.700.770.84Set II (N =989)R20.890.890.860.840.83SD 0.650.650.760.810.82AUE 0.470.470.520.610.57Set III (N =2357)R 20.850.840.800.790.75SD 0.930.941.071.091.18AUE 0.690.700.750.790.90Table 3Comparison of the performance of several log S modelsFig.3Experimental log S values versus calculated values byXLOGS on the test setN =121,R 2=0.47,RMSE=1.08log units2253Acta Phys.-Chim.Sin.2012Vol.28solid compounds,the entire solvation process can be dissected into two steps in a thermodynamic point of view:first,crystal melts into pure liquid phase,and then liquid phase is parti-tioned into water.The first step is dominated by solute-solute interactions whereas the second step is the same as solvation of liquid compounds.Therefore,the performance of additive mod-els on liquid and solid compounds is different.In our study,both liquid and solid compounds were included in Set I.We attempted to treat compounds separately according to their states as follows.Set I was filtered further to extract liq-uid compounds(Set II)and solid compounds(Set III),respec-tively.For understandable reasons,Set II consists of compounds with relatively simple structures.Thus,some atom types in our general atom typing scheme(Table S1)were absent or had very low occurrence on this data set.Such atom types were re-moved to obtain a valid regression model.Finally,73atom/ group types and one correction factor,i.e.,intermolecular hy-drogen bonds,were included in the regression model.The con-tribution of each descriptor was obtained through multivariate regression(Table S1).Regression results(R2=0.89,SD=0.65 log units)indicated that the fitted log S values have a good rela-tionship with the experimental data(Fig.4).Results of leave-one-out cross-validation(Q2=0.87,RMSE=0.73log units)indi-cate that this regression model is not over-fitted.As a compari-son,three other log S methods were also applied to Set II.The results indicated that our XLOGS model was superior to others (Table2).Similarly,a regression model of86descriptors was obtained on Set III,i.e.,solid compounds with known log S values.Sta-tistical results of regression were:R2=0.84,SD=0.94log units (Fig.5).Statistical results of leave-one-out cross-validation were:Q2=0.83,RMSE=1.00log units,which indicated that this regression model was also not parison of the performance of XLOGS and three other log S methods on Set III are summarized in Table2.One can see that the perfor-mance of XLOGS is also better on this data set.Nevertheless, its performance on this data set is not as good as that on Set II.This difference was actually expected by us due to the more complicated solvation process of solid compounds.One way to estimate the penalty of crystal energy to log S of solid compounds is to use melting points.Yalkowsky et al.33,38 estimated solubility of solid non-electrolytes with an empirical equation including melting point and obtained reasonable re-sults:log S=0.5-0.01(MP-25)-log P(6) In the above equation,MP is the experimental value of melting point.In our study,we tested this method on Set III.In our cal-culation,log P values were all calculated using the XLOGP3 method25although the experimental log P values of many com-pounds in this data set are known.The statistical results are: R2=0.76,RMSE=1.17log units.One can see that this method does not produce better results than our additive model XLOGS.Moreover,this method is not very practical since melting point and log P values are needed to carry out computa-tion.Although computed melting points and log P values can be used for instead,it will introduce additional uncertainty into the final estimations of log S values by doing so.In particular, reliable estimation of melting points is as challenging as esti-mation of log S itself.3.3Relationship betweenΔSL and accuracy ofadditive modelIt has been demonstrated in the above discussion that an ad-ditive model like XLOGS is less successful in estimating log S values of solid compounds,primarily due to the inadequate consideration of crystal energy.TheΔSL parameter(Eq.(4))re-flects the deviation between water solubility and octanol-water partition coefficient.The rationale is that partition of a solute between octanol phase and water phase does not involve crys-tal break and there is a“pure”process.It was used in our study to investigate the performance of additive model in log S com-putation.The relationships between log1/S exp and log P cal for liquid compounds(Fig.6(a))and solid compounds(Fig.6(b))were are firstly studied.Note that calculated log P values by XLOGP3 were used here because the experimental log P values of someFig.4Experimental log S values versus calculated values by XLOGS-AA on the liquid compounds in Set IIN=989,R2=0.89,SD=0.65log units Fig.5Experimental log S values versus calculated values by XLOGS-AA on the solid compounds in Set IIIN=2357,R2=0.84,SD=0.94logunits 2254DUAN Bao-Gen et al .:An Empirical Additive Model for Aqueous Solubility Computation:Success and Limitations No.10compounds in our data are not available.One can see in Fig.6that the correlation between log1/S exp and log P cal for liquid com-pounds is closer to unity than the corresponding scenario re-garding solid compounds.Distribution of ΔSL values (Fig.7)also shows that ΔSL values have a larger fluctuation zone around zero for solid compounds.Based on the above analysis,aqueous solubility of liquid compounds is more relevant to lipophilicity than other factors.In fact,the so-called general solubility equation (GSE)37devel-oped for liquid compounds previously only correlates solubili-ty with partition coefficient.But it is not the case for solid com-pounds.For solid compounds,some other factors other than li-pophilicity,such as crystal energy,are not described adequate-ly by additive models.But it also needs to be pointed out that solute -solute interactions are not completely ignored by addi-tive models.Such interactions are also reflected in fragment contributions implicitly to some extents.That is why our XLOGS model still produced acceptable results on solid com-pounds.In our study,the ΔSL parameter was used as an indicator to judge whether an additive model is suitable for computing aqueous solubility.Although solid compounds have larger ΔSL distributions than liquid compounds in our data set,there aresome solid compounds whose ΔSL values are close to zero.We further studied the performance of additive model on a data set consisting of molecules with smaller ΔSL values extracted from Set III.These molecules were selected in a stepwise pro-cedure as follows.Firstly,the molecules with ΔSL values rang-ing from 0to 1were extracted from Set III to form an initial da-ta set.Then,the molecules with ΔSL value lower than 2in the remaining part of Set III were added into the current data set to form a new one.Then,some molecules with even larger ΔSL values were added to extend the range of ΔSL values.At each step,leave-one-out cross-validation was applied to analyze the predictive power of the additive model on the new data set.Sta-tistical results obtained in different ΔSL spaces during this step-wise procedure are summarized in Table 4.As for the mole-cules with negative ΔSL values,the same strategy for data set compilation was employed.Leave-one-out cross-validation re-sults on each version of data set are summarized in Table 5.As one can see in Table 4and Table 5,the performance of an additive model is less satisfactory on solid compounds with larger absolute values of ΔSL.Nevertheless,it still produces very acceptable results on solid compounds with ΔSL valuesFig.6Scatter plot of log1/S exp and log P cal values for (a)the liquid compounds in Set II (N =989),and (b)the solid compounds in Set III (N =2357)The diagonal line is colored in blue;while the linear fitting line is colored inred.Fig.7Distributions of the ΔSL parameter for the liquid compounds in Set II (N =989)and the solid compoundsin Set III (N=2357)Table 5Leave-one-out cross-validation results of XLOGS-AA on different subsets of solid compounds (ΔSL<0)Table 4Leave-one-out cross-validation results of XLOGS-AA on 2255。
1-(4-羟基苯基)-1-丙酮合成方法改进

1-(4-羟基苯基)-1-丙酮合成方法改进
莫芬珠;任进知;胡敏致
【期刊名称】《中国医药工业杂志》
【年(卷),期】1999(30)11
【总页数】2页(P517-518)
【关键词】丙酮;羟基苯基;合成;抗早产药;中间体
【作者】莫芬珠;任进知;胡敏致
【作者单位】中国药科大学计划生育药物研究中心;中国药科大学计划生育药物研究中心有机教研室;江西医药学校
【正文语种】中文
【中图分类】TQ463.24
【相关文献】
1.1-(4-甲基)苯基-3-苯基-3-(N-苯基)胺基-1-丙酮的合成及波谱分析 [J], 强根荣;金红卫;王海滨;范铮;单尚
2.1-(4-氨基苯基)-2-(1-哌啶基)-1-丙酮盐酸盐的合成及其光引发性能 [J], 黄杰;谢川;潘固平;王倩
3.公安部商务部国家卫生和计划生育委员会海关总署国家安全生产监督管理总局国家食品药品监督管理总局关于将N-苯乙基-4-哌啶酮、4-苯胺基-N-苯乙基哌啶、N-甲基-1-苯基-1-氯-2-丙胺、溴素、卜苯基-1-丙酮5种物质列人易制毒化学品管理的公告 [J], 公安部; 商务部; 卫生计生委; 海关总署; 国家安全监管总局; 国家食品药品监管总局
4.3-羟基-3-(4-硝基苯基)-1-苯基-1-丙酮的制备
——一个有机制备实验的设计 [J], 文巍;李朝星
5.国务院办公厅关于同意将N-苯乙基-4-哌啶酮、4-苯胺基-N-苯乙基哌啶、N-甲基-1-苯基-1-氯-2-丙胺、溴素、1-苯基-1-丙酮列入易制毒化学品品种目录的函[J],
因版权原因,仅展示原文概要,查看原文内容请购买。
再论新质生产力的内涵特征与形成路径——以马克思生产力理论为视角

再论新质生产力的内涵特征与形成路径——以马克思生产力
理论为视角
胡莹;方太坤
【期刊名称】《浙江工商大学学报》
【年(卷),期】2024()2
【摘要】在唯物史观视角下,马克思生产力理论为进一步深入探究新质生产力提供了一个系统性的理论基础。
生产力三大基本要素及其优化组合的新质跃升是新质生产力的基本内涵,新质生产力实质上是人更高阶地创造性认识和改造自然的现实能力,是以科技创新引领全要素生产率提升的复杂系统,新质生产力与新型生产关系相互联系和作用。
基于新质生产力的丰富内涵,新质生产力从四个维度分别展现出以脑力劳动者为主的主体特征、颠覆性创新驱动的技术特征、多要素渗透融合的结构特征、数智化和绿色化产业的形态特征。
加快形成新质生产力,要充分发挥社会主义制度优势促进高水平科技创新,推动数字技术对生产要素全方位渗透融合,不断培养适应新质生产力发展的高素质人才,着力培育和壮大战略性新兴产业和未来产业,助力高质量发展。
【总页数】13页(P39-51)
【作者】胡莹;方太坤
【作者单位】中山大学马克思主义学院
【正文语种】中文
【中图分类】F124.3
【相关文献】
1.再论新质生产力:认识误区、形成条件与实现路径
2.面向新质生产力发展的现代新国企:内涵特征、功能目标与打造路径
3.论新质生产力的内涵、特征和意义——兼论马克思主义生产力理论的创新发展
4.新质生产力的时代内涵和形成路径
5.中国技术经济学会举办“以新促质,蓄势赋能——新质生产力内涵特征、形成机理及实现进路”专家座谈会
因版权原因,仅展示原文概要,查看原文内容请购买。
- 1、下载文档前请自行甄别文档内容的完整性,平台不提供额外的编辑、内容补充、找答案等附加服务。
- 2、"仅部分预览"的文档,不可在线预览部分如存在完整性等问题,可反馈申请退款(可完整预览的文档不适用该条件!)。
- 3、如文档侵犯您的权益,请联系客服反馈,我们会尽快为您处理(人工客服工作时间:9:00-18:30)。
化教 育事 业作 出的贡献 。
2 、人物传记
刘毅翔著 《 贵州辛亥革命人物传稿 》“ ;贵 阳市政协文史委编 《 筑人行迹——贵阳历史
文化人物传略》 ;冯祖贻等主编 《 辛亥革命——贵州事典 》” 、魇思纯著 《 民国贵州风云人物 》H 对任可
・
l 1 0・
贵州文史丛刊
2 0 1 6 年
第贰期
任可澄研究综 述
谢 孝明
( 贵州省社会科学院 历史研究所 贵州 贵 阳 5 5 0国影响力的贵 州近代社会政 治活动家、教育家、学者、诗
人 ,是贵 州近代 史、 中 国近代 史研 究 的重要 对 象。本 文 旨在 通过 对任 可澄研 究作 一尽 可 能 详 细的 学术 史回顾 与考 察 ,来 总结这 一研 究 已取得 的成果 与创 获 ,条分 缕析其 同样 存在 的 问题 、 不足及 其原 因,以适 应 “ 总结过 去 ,开创 未 来”之要 求 ,推 动任 可澄研 究 向纵深拓
展。 关键 词 :任 可澄 研究 回顾 综 述
中图分 类号 :K 8 1 文献标 识码 :A 文章编 号 :1 0 0 0 — 8 7 0 5( 2 0 1 6)0 2 — 1 1 0 —1 1 7
任可澄 ( 1 8 7 9 — 1 9 4 5 年) ,字志清 ,原名文燥 ,字仲湘 ,别号匏叟 ,贵州安顺普定人。任可澄是具有 全国影响力的贵州近代社会政治活动家、教育家 、学者 、诗人。他的一生主要从事了三件大事 ,即兴教、
想及实践 》 ;罗正副 《 任可澄及其< 黔南丛书> 》控 ;罗正副 《 任可澄文献思想与实践 》∞ ;顾久 《 近年来
任可澄研究简述 》 ;陈琳 《 任可澄与< 黔南丛书> 析》 ;何静梧 《 贵州著名文人任可澄 》拍 ;何幼兰 《 任
1 周春元 、何长风 、张祥光主编 : 《 贵州近代史 》,贵州人 民出版社 ,1 9 8 7 年9 月。
从政 、修志 。任 可 澄生 活在 晚清 民 国新 旧交替 、变 革 动荡 、内忧外 患交 逼 、民族 觉醒 的特 殊 时代 ,他 的身
上无疑负载着这一时代浓重的气息 ,也打上了这一时代深深 的烙印。同时 ,他的家世 ,他的兴教 、从政 、 修志的人生轨迹 ,他 的教育理念 、政治理想、学术追求 、价值判断 、道德操持 ,既具有他个人 的特殊性 , 也具有他同时代千万儒家士大夫在社会历史转型时期所具有 的共性 。通过对任可澄的研究 ,可以使人们能 够更深入地 了解晚清民国转型时期的社会生态和人文生态。任可澄是一个富含学术价值的历史文化名人 , 以此他成 了贵州近代史 、中国近代史研究 的重要对象。
六 十七 。逝世之地为 贵阳里第。任泰乃任可澄长 子 , 按 过去的规矩 ,父母去世 ,由家中长子主事 ,任泰倩人 为父 作事略行状之类 自是分内
之 事。向义 、姜风翔 等为任可澄高弟 ,出于敬重 师德 、感念师恩而为师拟私谥也 是情 理之中事 。长子 、门生 皆任 氏最亲近之人 , 故 其所言 所述可据 以为 实,信 以为是 。参见 :贵州省文史馆编 《 民国贵州文献大 系》第三辑上册任可澄著 《 匏斋集 》,2 0 1 5 年版 ,第3 4 8 — 3 4 9 页。
任可澄研究综述
在。
・ 1 l 1 ・
( 一) 研 究著述 二十世纪八十年代 ,贵州史学界前辈在编纂 《 贵州近代史 》 《 贵州军 阀史 》 《 西南
军阀史 》等专史时都有涉及任可澄内容的篇章 ,这可视为任可澄研究的肇始。而作为任可澄的的专论则迟
到二十世纪九十年代。迄今任可澄研究表现有如下几种形态 :
1 、专史著述 《 贵州近代史 》 《 贵州军阀史 》 《 西南军阀史 》 《 贵州通史 》 第四卷 《 贵州古代教 育史 》 《 新学人黔——贵州近代教育 的源流及发展 》 ,都有涉及任可澄的相关内容 ,但未列专门章节。 孑 L 令 中主编的 《 贵州教育史 》’ 、贵 阳市政协文史委编的 《 贵阳历史人物丛 书 ・ 文化教育卷 》 《 贵州文化
澄的生平都作 了传记。 3 、期刊文章 截至2 0 1 5 年1 2 月底 ,我们能够搜集 到介绍和研究任可澄生平事迹 的期刊文章 、论文有
十余篇 ,现依文章发表 的时间顺序罗列如下 :彦夫 《 任可澄生平一夕谈 》 ;鉴真 《 贵州近代改 良派的代 表 、著名教育家 、学者——任可澄 》 ;刘毅翔 《 戴戡 、任可澄与护国运动 》" ;邓汉祥 《 对任 可澄的点 滴 回忆 》 徐泽庶 《 任可澄先生传略 》 ;何幼兰 《 缅怀外祖父任可澄 》加 ;何幼兰 《 任可澄先生的教育思
一
、
任 可澄 研究 已取得 的创 获与成 果
作 为贵州乃至 中国近代史上 的重要人物 ,尽管人们对他有 不同的评价 ,但是在研究和书写贵州近 代史 以及 与之紧密相关 联的专史贵州辛亥革命史 、贵州近代教育史 、贵州近代文化史 、贵州军 阀史 、 西南 军 阀史 等之 时 ,他都 是一个 绕不开 的关 键人物 。这也 正是任 可澄对 于学术研究 的价值 和意义所
作者简介 : 谢 孝明 ,湖南桂 阳人 ,贵州省社 会科学院历史研究所副研究员 ,历史学博 士 ,主要研究领域 :中国思想文化史 、中国经济 思想史 、书院与儒学传播 、湖湘文化与湖南人才群体研究 、 湘黔文化交流史等 。 基金项 目:普定县人 民政府乡贤文化研究项 目之 “ 任 可澄研究”课题 。 1 关 于任可澄 的生 卒时间与逝世 之地 ,各种记述多有 矛盾 出入之处 。根据 白之翰 《 代任 泰撰任公志清事 略 》与 向义 《 拟文定先生 私谥 议 》所言 ,任 可澄生于清光绪四年戊寅腊月二十二 日,即公元 1 8 7 9 年1 月1 4 日,卒于 民国三十 四年 ( 1 9 4 5 ) 十二月九 日,享年六 十六 ,虚岁