Abstract Elastic Medical Image Registration Based on Image Intensity
(阅-000)一种基于轮廓的医学图像弹性配准方法

作者简介: 舒小华( 1965- ) , 男, 国防科技大学博士研究生, 研究方向为信号与信息处理、图像处理; 沈振康( 1937- ) , 男, 国防科技大学 ATR 实验室 教授, 博士生导师, 主要研究方向: 数字图像处理、目标识别、信号处理、神经网络等。
C舒om小pu华ter, 沈En振gi康ne:e一rin种g 基an于d 轮Ap廓pli的ca医tio学ns图计像算弹机性工配程准与方应法用
式函数) :
#( r) =p( r) , r∈[0, 1]
( 4)
由于这种径向函数具有紧支撑特性, 且是正定函数。用它
作 为 弹 性 配 准 的 变 换 函 数 , 不 仅 线 性 方 程 T( ui) =vi 的 解 稳 定 、 唯一, 而且方程的求解和插值运算量都不是很大, 因此很适合
应用于基于特征点的弹性配准中。本文采用的 ψ函数:
在变换函数的选取中, 径向基函数对变形图像的弹性配准
是有效的。它们对于能对线性方程 T( ui) =vi 提供稳定、唯 一 的
解。目前所使用的径向基函数有 薄 板 样 条 函 数[6]( RTPS) , 二 次 曲
面样条函数( RM) , 高斯函数( RG) 等:
#r4-dlnr 4- d∈2N
RTPS( r) = r4-d otherwise
$ Rs( x) = &i R( ‖x- ui‖) i=1
( 9) ( 10)
式中 R( r) =R( ‖x- ui‖) 为径向基函数, 其值取决于点 x 与特征 点 ui 间的距离, αi 为待定的配准参数。为了保证无限远处的弹 性变换为零, 需满足约束条件:
n
’&i $j( ui) =0, j=1, …, m i=1
ieee transactions on medical imaging latex模板

ieee transactions on medical imaging latex模板以下是一个简单的IEEE Transactions on Medical Imaging的LaTeX模板,默认字体大小为12pt,页边距为1英寸。
```latex\documentclass[12pt]{article}\usepackage[margin=1in]{geometry}\title{IEEE Transactions on Medical Imaging}\author{Your Name}\date{\today}\begin{document}\maketitle\section{Introduction}This is a sample document for IEEE Transactions on Medical Imaging using LaTeX. In this section, we will briefly introduce the background and motivation of the research topic.\section{Related Work}This section discusses the previous work in the field of medical imaging. We will review and summarize the existing literature and highlight the gaps in the current research.\section{Methods}The methodology section describes the data collection process, experimental setup, and the proposed algorithm or technique. We will present the steps involved in our research and provide detailedexplanations.\section{Results}In this section, we present the results of our experiments and evaluate our proposed method. We will provide quantitative and qualitative analysis of the obtained results and compare them with the baseline methods.\section{Discussion}This section discusses the implications and significance of our findings. We will analyze the limitations of our research and propose possible directions for future work.\section{Conclusion}In conclusion, this paper presents a study on XYZ in the context of medical imaging. We have proposed a novel approach and obtained promising results. Our findings contribute to the field of medical imaging and have potential applications in clinical practice. \section{Acknowledgements}We would like to acknowledge the support and guidance of our advisors and colleagues throughout this research. We are also grateful to the participants for their contribution to our study.\begin{thebibliography}{9}\bibitem{ref1}Author A, Author B, Author C. Title of the paper. \textit{IEEE Transactions on Medical Imaging}, Year, Volume, Issue, Pages.\bibitem{ref2}Author X, Author Y, Author Z. Another title of the paper.\textit{IEEE Transactions on Medical Imaging}, Year, Volume, Issue, Pages.\end{thebibliography}\end{document}```请注意,此模板只是一个基本的示例,并未包含具体的内容。
跨尺度脑影像研究方法介绍
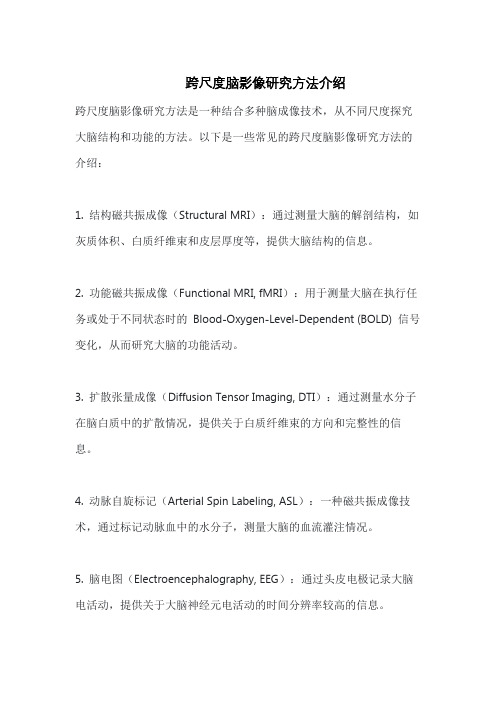
跨尺度脑影像研究方法介绍跨尺度脑影像研究方法是一种结合多种脑成像技术,从不同尺度探究大脑结构和功能的方法。
以下是一些常见的跨尺度脑影像研究方法的介绍:1. 结构磁共振成像(Structural MRI):通过测量大脑的解剖结构,如灰质体积、白质纤维束和皮层厚度等,提供大脑结构的信息。
2. 功能磁共振成像(Functional MRI, fMRI):用于测量大脑在执行任务或处于不同状态时的Blood-Oxygen-Level-Dependent (BOLD) 信号变化,从而研究大脑的功能活动。
3. 扩散张量成像(Diffusion Tensor Imaging, DTI):通过测量水分子在脑白质中的扩散情况,提供关于白质纤维束的方向和完整性的信息。
4. 动脉自旋标记(Arterial Spin Labeling, ASL):一种磁共振成像技术,通过标记动脉血中的水分子,测量大脑的血流灌注情况。
5. 脑电图(Electroencephalography, EEG):通过头皮电极记录大脑电活动,提供关于大脑神经元电活动的时间分辨率较高的信息。
6. 脑磁图(Magnetoencephalography, MEG):利用磁场感应器记录大脑神经元产生的磁场信号,提供与脑电图类似的信息,但具有更好的空间分辨率。
7. 正电子发射断层扫描(Positron Emission Tomography, PET):通过注射放射性示踪剂,测量大脑的代谢活动或神经递质分布。
8. 光学成像技术:包括近红外光谱成像(Near-Infrared Spectroscopy, NIRS)和功能性光学成像(Functional Optical Imaging),用于测量大脑的血液动力学或代谢变化。
这些跨尺度脑影像研究方法可以单独或结合使用,以获取不同尺度下大脑结构和功能的信息。
综合分析这些多模态数据可以提供更全面的了解大脑的工作机制、神经网络连接和病理变化等。
医学图像配准技术 综述
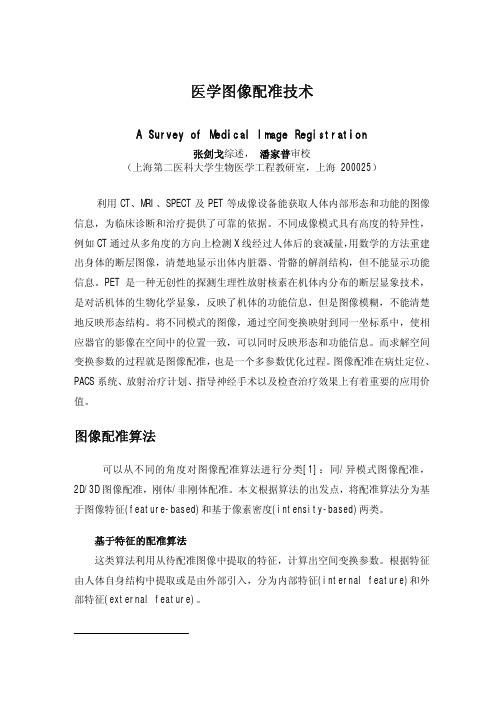
医学图像配准技术A Survey of Medical Image Registration张剑戈综述,潘家普审校(上海第二医科大学生物医学工程教研室,上海 200025)利用CT、MRI、SPECT及PET等成像设备能获取人体内部形态和功能的图像信息,为临床诊断和治疗提供了可靠的依据。
不同成像模式具有高度的特异性,例如CT通过从多角度的方向上检测X线经过人体后的衰减量,用数学的方法重建出身体的断层图像,清楚地显示出体内脏器、骨骼的解剖结构,但不能显示功能信息。
PET是一种无创性的探测生理性放射核素在机体内分布的断层显象技术,是对活机体的生物化学显象,反映了机体的功能信息,但是图像模糊,不能清楚地反映形态结构。
将不同模式的图像,通过空间变换映射到同一坐标系中,使相应器官的影像在空间中的位置一致,可以同时反映形态和功能信息。
而求解空间变换参数的过程就是图像配准,也是一个多参数优化过程。
图像配准在病灶定位、PACS系统、放射治疗计划、指导神经手术以及检查治疗效果上有着重要的应用价值。
图像配准算法可以从不同的角度对图像配准算法进行分类[1]:同/异模式图像配准,2D/3D图像配准,刚体/非刚体配准。
本文根据算法的出发点,将配准算法分为基于图像特征(feature-based)和基于像素密度(intensity-based)两类。
基于特征的配准算法这类算法利用从待配准图像中提取的特征,计算出空间变换参数。
根据特征由人体自身结构中提取或是由外部引入,分为内部特征(internal feature)和外部特征(external feature)。
【作者简介】张剑戈(1972-),男,山东济南人,讲师,硕士1. 外部特征在物体表面人为地放置一些可以显像的标记物(外标记,external marker)作为基准,根据同一标记在不同图像空间中的坐标,通过矩阵运算求解出空间变换参数。
外标记分为植入性和非植入性[2]:立体框架定位、在颅骨上固定螺栓和在表皮加上可显像的标记。
磁共振SWI在诊断脑血管病微出血灶中的应用价值
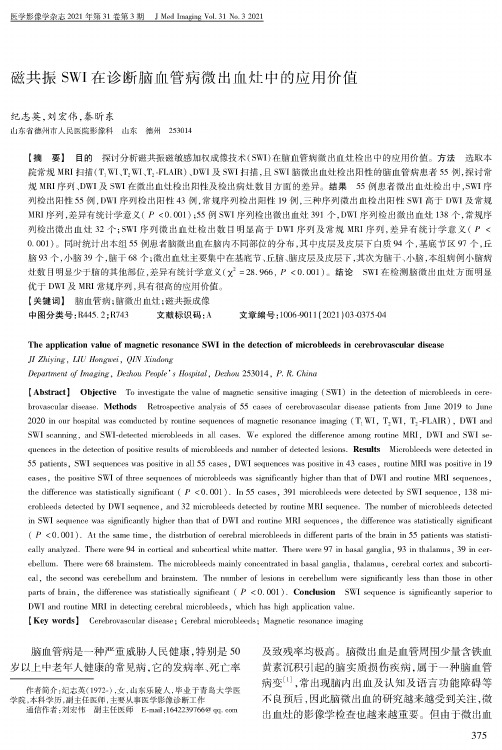
磁共振SWI在诊断脑血管病微出血灶中的应用价值纪志英,刘宏伟,秦昕东山东省德州市人民医院影像科山东德州253014$摘要】目的探讨分析磁共振磁敏感加权成像技术(SWI)在脑血管病微出血灶检出中的应用价值。
方法选取本院常规MRI扫描(T1WI、TWI、T2-FLAIR)、DWI及SWI扫描,且SWI脑微出血灶检出阳性的脑血管病患者55例,探讨常规MRI序列、DWI及SWI在微出血灶检出阳性及检出病灶数目方面的差异(结果55例患者微出血灶检出中,SWI序列检出阳性55例,DWI序列检出阳性43例,常规序列检出阳性19例,三种序列微出血检出阳性SWI高于DWI及常规MRI序列,差异有统计学意义(P<0.001);55例SWI序列检出微出血灶391个,DWI序列检出微出血灶138个,常规序列检出微出血灶32个;SWI序列微出血灶检出数目明显高于DWI序列及常规MRI序列,差异有统计学意义(P<0.001)。
同时统计出本组55例患者脑微出血在脑内不同部位的分布,其中皮层及皮层下白质94个,基底节区97个,丘脑93个,小脑39个,脑干68个;微出血灶主要集中在基底节、丘脑、脑皮层及皮层下,其次为脑干、小脑,本组病例小脑病灶数目明显少于脑的其他部位,差异有统计学意义(#=28.966,P<0.001)(结论SWI在检测脑微出血灶方面明显优于DWI及MRI常规序列,具有很高的应用价值。
$关键词】脑血管病;脑微出血灶;磁共振成像中图分类号:R445.2&R743文献标识码:A文章编号:1006-9011(2021)03-375-4The application value of magnetic resonancc SWI in the detection of microbleers in cerenrovascular disease>I Zhiying,LII Hongwei,QIN XindongDepagment O Imaging,Dezhoo People*s Hospital,Dezhoo253014,P.R.China)Abstract]Objective To investigate the value of magnetic sensitive imaaing(SWI)in the detection of microbleeds in cere-bmvvsculaC disease.Methods Retrospective analysis of55cases of cerebrovvsculaC disease patients from June2019te June 2020in our hospital was comducted by routine sequences of maanetic resonance imaaing(T]WI,T2WI,T2-FLAIR),DWI and SWIscanning,and SWI-dHeced miceobiHdsin a i casHs.WHHipioeHd ehHdi o eHncHamongeoueinHMRI,DWIand SWIsH-quences in the detection of positive results of microbleeds and number of detected lesions.Reselts Microbleeds were detected in 55paeienes,SWIsequenceswasposieivein a i55cases,DWIsequenceswasposieivein43cases,eoueineMRIwasposieivein19 cases,eheposieiveSWIooeheeesequencesoomiceobieedswassignioicaneiyhigheeehan ehaeooDWIand eoueineMRIsequences,ehedi o eeencewasseaeiseica i ysignioicane(P<0.001).In55cases,391miceobieedsweeedeeeceed bySWIsequence,138mi-ceobieedsdeeeceed byDWIsequence,and32miceobieedsdeeeceed byeoueineMRIsequence.Thenumbeeoomiceobieedsdeeeceed in SWIsequencewassignioicaneiyhigheeehan ehaeooDWIand eoueineMRIsequences,ehedi o eeencewasseaeiseica i ysignioicane (P<0.001).Aeehesameeime,ehediseebueion ooceeebeaimiceobieedsin di o eeenepaeesooehebeain in55paeieneswasseaeisei-ca i yanaiyzed.Theeeweee94in coeeicaiand subcoeeicaiwhieema e e e.Theeeweee97in basaigangiia,93in ehaiamus,39in cee-ebe i um.Theeeweee68beainseem.Themiceobieedsmainiyconceneeaeed in basaigangiia,ehaiamus,ceeebeaicoeeeiand subcoeei-cai,ehesecond wasceeebe i um and beainseem.Thenumbeeooiesionsin ceeebe i um weeesignioicaneiyie s ehan ehosein oehee parts of brain,the difference was statisticayu significant(P<0.001).Conclusion SWI sequence is significanUu superior te DWIand oueineMRIin deeeceingceeb aimicLobieeds,which hashigh appiicaeion vaiue.)Key words]CerebmvvsculaC disease;Cerebral microbleeds;Maanetic resonance imaaing脑血管病是一种严重威胁人民健康,特别是50岁以上中老年人健康的常见病,它的发病率、死亡率作者简介:纪志英(1972-),女,山东乐陵人,毕业于青岛大学医学院,本科学历,副主任医师,主要从事医学影像诊断工作通信作者:刘宏伟副主任医师E-mail:1642239766@ 及致残率均极高。
THE INTERNATIONAL JOURNAL OF MEDICAL ROBOTICS AND COMPUTER ASSISTED SURGERY Int J Med Robot
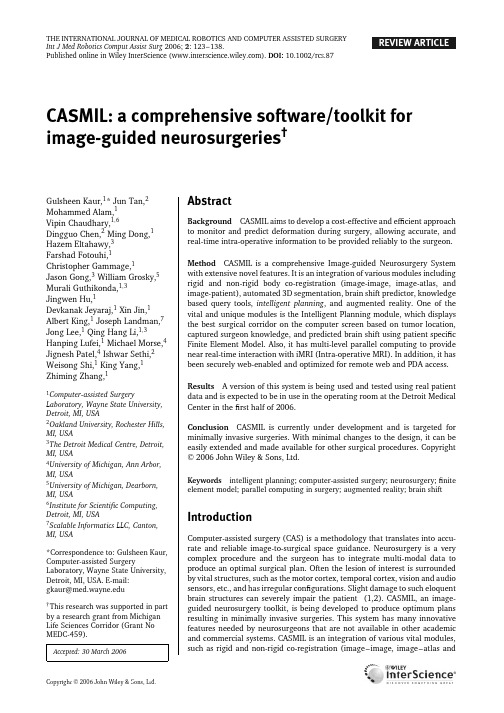
Introduction
Computer-assisted surgery (CAS) is a methodology that translates into accurate and reliable image-to-surgical space guidance. Neurosurgery is a very complex procedure and the surgeon has to integrate multi-modal data to produce an optimal surgical plan. Often the lesion of interest is surrounded by vital structures, such as the motor cortex, temporal cortex, vision and audio sensors, etc., and has irregular configurations. Slight damage to such eloquent brain structures can severely impair the patient (1,2). CASMIL, an imageguided neurosurgery toolkit, is being developed to produce optimum plans resulting in minimally invasive surgeries. This system has many innovative features needed by neurosurgeons that are not available in other academic and commercial systems. CASMIL is an integration of various vital modules, such as rigid and non-rigid co-registration (image–image, image–atlas and
医学图像配准方法及其应用
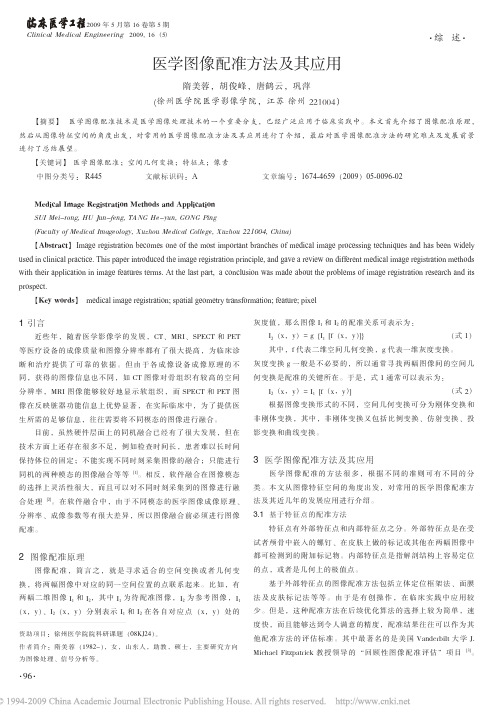
其中, f 代表二维空间几何变换, g 代表一维灰度变换。
灰度变换 g 一般是不必要的, 所以通常寻找两幅图像间的空间几
何变换是配准的关键所在。 于是, 式 1 通常可以表示为:
I2 (x, y) = I1 [f (x, y)]
(式 2)
根据图像变换形式的不同, 空间几何变换可分为刚体变换和
非刚体变换, 其中, 非刚体变换又包括比例变换、 仿射变换、 投
2009 年 5 月第 16 卷第 5 期
Clinical Medical Engineering 2009, 16 (5)
4 总结
近些年, 医学图像配准方法有了很大的发展, 已经应用于 CT、 MR、 PET 和 SPECT 等 图 像 , 并 将 逐 渐 扩 展 到 三 维 图 像 的 融 合 中 。 [10-12] 但 是 由 于 研 究 对 象 的 多 样 性 和 复 杂 性 , 现 在 还 没 有 一 种方法适用于所有的医学图像配准。 在具体应用中, 需要根据不 同的研究对象, 在配准精度、 运算速度和计算量中进行权衡比 较, 选择适合的配准方法。 目前, 多模态成像还只限于两种成像 模态的配准融合, 如 PET 和 CT 图像的配准融合, MR 和 CT 图像 的配准融合, 将来很有可能发展到三种模态成像的融合, 但很多 问题还有待进一步解决。 医学图像配准方法的研究还有漫长的历 程。
但由于各成像设备成像原理的不同获得的图像信息也不同如ct图像对骨组织有较高的空间分辨率mri图像能够较好地显示软组织而spect和pet像在反映脏器功能信息上优势显着在实际临床中为了提供医生所需的足够信息往往需要将不同模态的图像进行融合
2009 年 5 月第 16 卷第 5 期
Clinical Medical Engineering 2009, 16 (5)
深度学习下的医学影像分割算法综述

图像分割与图像分类、目标检测同为图像处理技术在计算机视觉应用下的重要研究分支。
图像分割,具体可分为语义分割与非语义分割两大类。
语义分割,也称像素级分类,是将属于同一对象类的图像部分聚集在一起的任务。
相反,非语义分割仅基于单个对象类的一般特征将像素聚集在一起[1]。
图像分割技术通常用于定位图像中目标和边界的位置,为图像内容的进一步理解打下良好的基础。
传统的图像分割技术包括基于阈值的方法、基于边缘的方法、基于区域的方法、基于特定理论的方法等[2]。
受限于计算机的硬件设备限制,传统的图像分割技术仅能对灰度图像进行处理,后期才逐渐发展到可以对RGB图像进行处理的阶段,但在分割的精度和效率上仍难以达到实际应用的要求。
近些年来,随着集成电路上可容纳的电子元器件数目不断翻倍,GPU的运算性能越来越强,受益于此的深度学习技术也因此迅猛发展,结合深度学习技术的图像分割算法逐渐成为该领域的主流算法,被广泛应用于移深度学习下的医学影像分割算法综述彭璟1,2,罗浩宇1,2,赵淦森1,2,林成创1,2,易序晟1,2,陈少洁1,21.华南师范大学计算机学院,广州5106632.广州市云计算安全与测评技术重点实验室,广州510663摘要:医学影像分割是计算机视觉在医学影像处理中的一个重要应用领域,其目标是从医学影像中分割出目标区域,为后续的疾病诊断和治疗提供有效的帮助。
近年来深度学习技术在图像处理方面取得了巨大进展,基于深度学习的医学影像分割算法逐渐成为该领域研究的重点和热点。
叙述了计算机视觉下的医学影像分割任务及其难点,重点综述了基于深度学习的医学影像分割算法,对当前具有代表性的相关方法进行了分类和总结,介绍了医学影像分割算法常用的评价指标和数据集。
对该技术的发展进行了总结和展望。
关键词:深度学习;计算机视觉;医学影像;图像分割文献标志码:A中图分类号:TP391doi:10.3778/j.issn.1002-8331.2010-0335Survey of Medical Image Segmentation Algorithm in Deep LearningPENG Jing1,2,LUO Haoyu1,2,ZHAO Gansen1,2,LIN Chengchuang1,2,YI Xusheng1,2,CHEN Shaojie1,21.School of Computer Science,South China Normal University,Guangzhou510663,China2.Guangzhou Key Lab on Cloud Computing Security and Assessment Technology,Guangzhou510663,ChinaAbstract:Medical image segmentation is an important application area of computer vision in the medical image processing, its goal is to segment the target area from medical images and provide effective help for subsequent diagnosis and treatment of diseases.Since deep learning technology has made great progress in the image processing,medical image segmentation algorithm based on deep learning has gradually become the focus and hotspot of research in this field.This paper gives a description on the tasks and difficulties of medical image segmentation.Then,it details the deep learning-based medical image segmentation algorithm,classifies and summarizes the current representative methods.Moreover,this paper presents the frequently-used algorithm evaluation indicators and datasets in the field of medical image segmentation.The development of medical image segmentation technology is summarized and forecasted.Key words:deep learning;computer vision;medical image;image segmentation基金项目:国家重点研发计划(2018YFB1404402);广东省重点研发计划(2019B010137003);唯链基金会资金(SCNU-2018-01);广东省科技计划(2018A07071702,201804010314,2016B030305006,201222412)。
imagej用户使用手册第三部分

imagej用户使用手册第三部分I. Introduction to ImageJIn this third part of the ImageJ User Manual, we will explore advanced features and functionalities of ImageJ, an open-source image processing software used for scientific and medical research. This section aims to provide a comprehensive guide for users to maximize the potential of ImageJ in their image analysis workflows.II. ImageJ PluginsOne of the key strengths of ImageJ lies in its extensive collection of plugins, which can be easily installed and utilized to expand the software's capabilities. Plugins offer users a wide range of additional tools and functions tailored to specific image processing requirements. Some popular plugins include:1. Fiji: Fiji is a distribution of ImageJ that comes prepackaged with a vast collection of plugins, macros, and scripts. It offers advanced features like 3D visualization, deconvolution, and machine learning algorithms, making it a powerful tool for image analysis.2. BioFormats: This plugin allows users to import and export various microscopy image file formats, making it compatible with most scientific image data. It supports over 150 file formats, ensuring seamless integration with different imaging modalities.3. CellProfiler: CellProfiler is a plugin designed for the automated analysis of cell images. It offers a user-friendly interface and a range ofbuilt-in algorithms, enabling the extraction of quantitative information from large image datasets.III. Macro ProgrammingImageJ provides a built-in macro language that allows users to automate repetitive tasks and create customized image analysis workflows. Macros are written using a simplified scripting language, which can be easily recorded and edited within the ImageJ interface. This feature simplifies complex image processing tasks and enables batch processing of multiple images.IV. Image Registration and AlignmentImage registration is a crucial step in image analysis, particularly when dealing with multi-channel or time-lapse imaging data. ImageJ offers several plugins and built-in functions to align and register images, compensating for movement or drift during acquisition. Users can choose from a variety of registration algorithms, such as rigid-body, affine, or elastic transformations, to optimize image alignment.V. Image SegmentationSegmentation is the process of partitioning an image into meaningful regions based on defined criteria. ImageJ provides numerous plugins and tools for image segmentation, catering to different applications and image types. These plugins utilize various algorithms, including thresholding, edge detection, or machine learning-based methods, to accurately segment objects of interest within an image.VI. Quantitative AnalysisImageJ excels in quantitative analysis of images, enabling users to extract valuable information from their datasets. This includes measuring object properties such as size, shape, intensity, and spatial distribution. Additionally, ImageJ offers statistical analysis tools for comparing image features, generating histograms, and performing co-localization analysis.VII. 3D Image ProcessingIn addition to its 2D analysis capabilities, ImageJ also supports processing and analysis of 3D image stacks. Users can load and visualize 3D datasets, manipulate data slices, and apply volumetric measurements and processing operations. Plugins like 3D Viewer and 3D Object Counter enhance the 3D analysis capabilities of ImageJ.VIII. Scripting and IntegrationAdvanced users can take advantage of ImageJ's scripting capabilities to extend its functionality or integrate it with other software tools. ImageJ supports several scripting languages, including JavaScript, Python, and BeanShell, allowing users to write custom scripts for their specific requirements. This enables seamless integration with external libraries and facilitates workflow automation.IX. ConclusionIn conclusion, this third part of the ImageJ User Manual has provided an overview of advanced features and functionalities that ImageJ offers. From plugins to macro programming, image registration to quantitative analysis, ImageJ empowers users to perform complex image processing and analysis tasks efficiently. The flexibility and versatility of ImageJ make it anindispensable tool for researchers in various scientific and medical fields. Explore the vast capabilities of ImageJ and unleash the full potential of your image analysis endeavors.。
Deformable Medical Image Registration
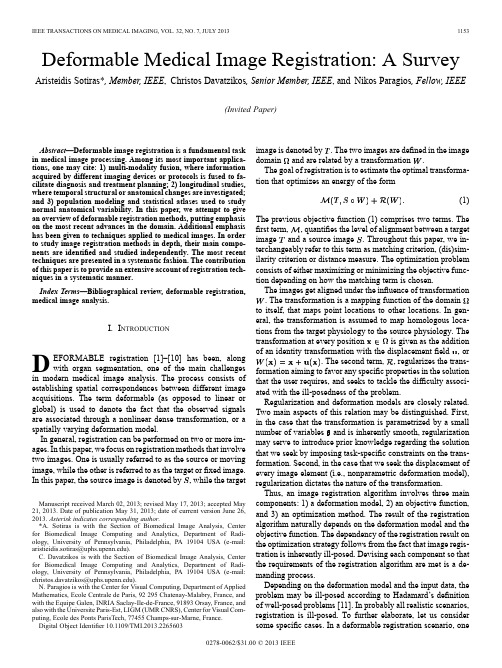
Deformable Medical Image Registration:A Survey Aristeidis Sotiras*,Member,IEEE,Christos Davatzikos,Senior Member,IEEE,and Nikos Paragios,Fellow,IEEE(Invited Paper)Abstract—Deformable image registration is a fundamental task in medical image processing.Among its most important applica-tions,one may cite:1)multi-modality fusion,where information acquired by different imaging devices or protocols is fused to fa-cilitate diagnosis and treatment planning;2)longitudinal studies, where temporal structural or anatomical changes are investigated; and3)population modeling and statistical atlases used to study normal anatomical variability.In this paper,we attempt to give an overview of deformable registration methods,putting emphasis on the most recent advances in the domain.Additional emphasis has been given to techniques applied to medical images.In order to study image registration methods in depth,their main compo-nents are identified and studied independently.The most recent techniques are presented in a systematic fashion.The contribution of this paper is to provide an extensive account of registration tech-niques in a systematic manner.Index Terms—Bibliographical review,deformable registration, medical image analysis.I.I NTRODUCTIOND EFORMABLE registration[1]–[10]has been,alongwith organ segmentation,one of the main challenges in modern medical image analysis.The process consists of establishing spatial correspondences between different image acquisitions.The term deformable(as opposed to linear or global)is used to denote the fact that the observed signals are associated through a nonlinear dense transformation,or a spatially varying deformation model.In general,registration can be performed on two or more im-ages.In this paper,we focus on registration methods that involve two images.One is usually referred to as the source or moving image,while the other is referred to as the target orfixed image. In this paper,the source image is denoted by,while the targetManuscript received March02,2013;revised May17,2013;accepted May 21,2013.Date of publication May31,2013;date of current version June26, 2013.Asterisk indicates corresponding author.*A.Sotiras is with the Section of Biomedical Image Analysis,Center for Biomedical Image Computing and Analytics,Department of Radi-ology,University of Pennsylvania,Philadelphia,PA19104USA(e-mail: aristieidis.sotiras@).C.Davatzikos is with the Section of Biomedical Image Analysis,Center for Biomedical Image Computing and Analytics,Department of Radi-ology,University of Pennsylvania,Philadelphia,PA19104USA(e-mail: christos.davatzikos@).N.Paragios is with the Center for Visual Computing,Department of Applied Mathematics,Ecole Centrale de Paris,92295Chatenay-Malabry,France,and with the Equipe Galen,INRIA Saclay-Ile-de-France,91893Orsay,France,and also with the Universite Paris-Est,LIGM(UMR CNRS),Center for Visual Com-puting,Ecole des Ponts ParisTech,77455Champs-sur-Marne,France. Digital Object Identifier10.1109/TMI.2013.2265603image is denoted by.The two images are defined in the image domain and are related by a transformation.The goal of registration is to estimate the optimal transforma-tion that optimizes an energy of the form(1) The previous objective function(1)comprises two terms.The first term,,quantifies the level of alignment between a target image and a source image.Throughout this paper,we in-terchangeably refer to this term as matching criterion,(dis)sim-ilarity criterion or distance measure.The optimization problem consists of either maximizing or minimizing the objective func-tion depending on how the matching term is chosen.The images get aligned under the influence of transformation .The transformation is a mapping function of the domain to itself,that maps point locations to other locations.In gen-eral,the transformation is assumed to map homologous loca-tions from the target physiology to the source physiology.The transformation at every position is given as the addition of an identity transformation with the displacementfield,or.The second term,,regularizes the trans-formation aiming to favor any specific properties in the solution that the user requires,and seeks to tackle the difficulty associ-ated with the ill-posedness of the problem.Regularization and deformation models are closely related. Two main aspects of this relation may be distinguished.First, in the case that the transformation is parametrized by a small number of variables and is inherently smooth,regularization may serve to introduce prior knowledge regarding the solution that we seek by imposing task-specific constraints on the trans-formation.Second,in the case that we seek the displacement of every image element(i.e.,nonparametric deformation model), regularization dictates the nature of the transformation. Thus,an image registration algorithm involves three main components:1)a deformation model,2)an objective function, and3)an optimization method.The result of the registration algorithm naturally depends on the deformation model and the objective function.The dependency of the registration result on the optimization strategy follows from the fact that image regis-tration is inherently ill-posed.Devising each component so that the requirements of the registration algorithm are met is a de-manding process.Depending on the deformation model and the input data,the problem may be ill-posed according to Hadamard’s definition of well-posed problems[11].In probably all realistic scenarios, registration is ill-posed.To further elaborate,let us consider some specific cases.In a deformable registration scenario,one0278-0062/$31.00©2013IEEEseeks to estimate a vector for every position given,in general, scalar information conveyed by image intensity.In this case,the number of unknowns is greater than the number of constraints. In a rigid setting,let us consider a consider a scenario where two images of a disk(white background,gray foreground)are registered.Despite the fact that the number of parameters is only 6,the problem is ill-posed.The problem has no unique solution since a translation that aligns the centers of the disks followed by any rotation results in a meaningful solution.Given nonlinear and nonconvex objective functions,in gen-eral,no closed-form solutions exist to estimate the registration parameters.In this setting,the search methods reach only a local minimum in the parameter space.Moreover,the problem itself has an enormous number of different facets.The approach that one should take depends on the anatomical properties of the organ(for example,the heart and liver do not adhere to the same degree of deformation),the nature of observations to be regis-tered(same modality versus multi-modal fusion),the clinical setting in which registration is to be used(e.g.,offline interpre-tation versus computer assisted surgery).An enormous amount of research has been dedicated to de-formable registration towards tackling these challenges due to its potential clinical impact.During the past few decades,many innovative ideas regarding the three main algorithmic registra-tion aspects have been proposed.General reviews of thefield may be found in[1]–[7],[9].However due to the rapid progress of thefield such reviews are to a certain extent outdated.The aim of this paper is to provide a thorough overview of the advances of the past decade in deformable registration.Never-theless,some classic papers that have greatly advanced the ideas in thefield are mentioned.Even though our primary interest is deformable registration,for the completeness of the presenta-tion,references to linear methods are included as many prob-lems have been treated in this low-degree-of-freedom setting before being extended to the deformable case.The main scope of this paper is focused on applications that seek to establish spatial correspondences between medical im-ages.Nonetheless,we have extended the scope to cover appli-cations where the interest is to recover the apparent motion of objects between sequences of successive images(opticalflow estimation)[12],[13].Deformable registration and opticalflow estimation are closely related problems.Both problems aim to establish correspondences between images.In the deformable registration case,spatial correspondences are sought,while in the opticalflow case,spatial correspondences,that are associ-ated with different time points,are looked for.Given data with a good temporal resolution,one may assume that the magnitude of the motion is limited and that image intensity is preserved in time,opticalflow estimation can be regarded as a small defor-mation mono-modal deformable registration problem.The remainder of the paper is organized by loosely following the structural separation of registration algorithms to three com-ponents:1)deformation model,2)matching criteria,and3)op-timization method.In Section II,different approaches regarding the deformation model are presented.Moreover,we also chose to cover in this section the second term of the objective function, the regularization term.This choice was motivated by the close relation between the two parts.In Section III,thefirst term of the objective function,the matching term,is discussed.The opti-mization methods are presented in Section IV.In every section, particular emphasis was put on further deepening the taxonomy of registration method by grouping the presented methods in a systematic manner.Section V concludes the paper.II.D EFORMATION M ODELSThe choice of deformation model is of great importance for the registration process as it entails an important compromise between computational efficiency and richness of description. It also reflects the class of transformations that are desirable or acceptable,and therefore limits the solution to a large ex-tent.The parameters that registration estimates through the op-timization strategy correspond to the degrees of freedom of the deformation model1.Their number varies greatly,from six in the case of global rigid transformations,to millions when non-parametric dense transformations are considered.Increasing the dimensionality of the state space results in enriching the de-scriptive power of the model.This model enrichment may be accompanied by an increase in the model’s complexity which, in turns,results in a more challenging and computationally de-manding inference.Furthermore,the choice of the deformation model implies an assumption regarding the nature of the defor-mation to be recovered.Before continuing,let us clarify an important,from imple-mentation point of view,aspect related to the transformation mapping and the deformation of the source image.In the in-troduction,we stated that the transformation is assumed to map homologous locations from the target physiology to the source physiology(backward mapping).While from a theoretical point of view,the mapping from the source physiology to the target physiology is possible(forward mapping),from an implemen-tation point of view,this mapping is less advantageous.In order to better understand the previous statement,let us consider how the direction of the mapping influences the esti-mation of the deformed image.In both cases,the source image is warped to the target domain through interpolation resulting to a deformed image.When the forward mapping is estimated, every voxel of the source image is pushed forward to its esti-mated position in the deformed image.On the other hand,when the backward mapping is estimated,the pixel value of a voxel in the deformed image is pulled from the source image.The difference between the two schemes is in the difficulty of the interpolation problem that has to be solved.In thefirst case,a scattered data interpolation problem needs to be solved because the voxel locations of the source image are usually mapped to nonvoxel locations,and the intensity values of the voxels of the deformed image have to be calculated.In the second case,when voxel locations of the deformed image are mapped to nonvoxel locations in the source image,their intensities can be easily cal-culated by interpolating the intensity values of the neighboring voxels.The rest of the section is organized by following coarsely and extending the classification of deformation models given 1Variational approaches in general attempt to determine a function,not just a set of parameters.SOTIRAS et al.:DEFORMABLE MEDICAL IMAGE REGISTRATION:A SURVEY1155Fig.1.Classi fication of deformation models.Models that satisfy task-speci fic constraints are not shown as a branch of the tree because they are,in general,used in conjunction with physics-based and interpolation-based models.by Holden [14].More emphasis is put on aspects that were not covered by that review.Geometric transformations can be classi fied into three main categories (see Fig.1):1)those that are inspired by physical models,2)those inspired by interpolation and ap-proximation theory,3)knowledge-based deformation models that opt to introduce speci fic prior information regarding the sought deformation,and 4)models that satisfy a task-speci fic constraint.Of great importance for biomedical applications are the con-straints that may be applied to the transformation such that it exhibits special properties.Such properties include,but are not limited to,inverse consistency,symmetry,topology preserva-tion,diffeomorphism.The value of these properties was made apparent to the research community and were gradually intro-duced as extra constraints.Despite common intuition,the majority of the existing regis-tration algorithms are asymmetric.As a consequence,when in-terchanging the order of input images,the registration algorithm does not estimate the inverse transformation.As a consequence,the statistical analysis that follows registration is biased on the choice of the target domain.Inverse Consistency:Inverse consistent methods aim to tackle this shortcoming by simultaneously estimating both the forward and the backward transformation.The data matching term quanti fies how well the images are aligned when one image is deformed by the forward transformation,and the other image by the backward transformation.Additionally,inverse consistent algorithms constrain the forward and backward transformations to be inverse mappings of one another.This is achieved by introducing terms that penalize the difference between the forward and backward transformations from the respective inverse mappings.Inverse consistent methods can preserve topology but are only asymptotically symmetric.Inverse-consistency can be violated if another term of the objective function is weighted more importantly.Symmetry:Symmetric algorithms also aim to cope with asymmetry.These methods do not explicitly penalize asym-metry,but instead employ one of the following two strategies.In the first case,they employ objective functions that are by construction symmetric to estimate the transformation from one image to another.In the second case,two transformation functions are estimated by optimizing a standard objective function.Each transformation function map an image to a common domain.The final mapping from one image to another is calculated by inverting one transformation function and composing it with the other.Topology Preservation:The transformation that is estimated by registration algorithms is not always one-to-one and cross-ings may appear in the deformation field.Topology preserving/homeomorphic algorithms produce a mapping that is contin-uous,onto,and locally one-to-one and has a continuous inverse.The Jacobian determinant contains information regarding the injectivity of the mapping and is greater than zero for topology preserving mappings.The differentiability of the transformation needs to be ensured in order to calculate the Jacobian determi-nant.Let us note that Jacobian determinant and Jacobian are in-terchangeably used in this paper and should not be confounded with the Jacobian matrix.Diffeomorphism:Diffeomoprhic transformations also pre-serve topology.A transformation function is a diffeomorphism,if it is invertible and both the function and its inverse are differ-entiable.A diffeomorphism maps a differentiable manifold to another.1156IEEE TRANSACTIONS ON MEDICAL IMAGING,VOL.32,NO.7,JULY2013In the following four subsections,the most important methods of the four classes are presented with emphasis on the approaches that endow the model under consideration with the above desirable properties.A.Geometric Transformations Derived From Physical Models Following[5],currently employed physical models can be further separated infive categories(see Fig.1):1)elastic body models,2)viscousfluidflow models,3)diffusion models,4) curvature registration,and5)flows of diffeomorphisms.1)Elastic Body Models:a)Linear Models:In this case,the image under deforma-tion is modeled as an elastic body.The Navier-Cauchy Partial Differential Equation(PDE)describes the deformation,or(2) where is the forcefield that drives the registration based on an image matching criterion,refers to the rigidity that quanti-fies the stiffness of the material and is Lamésfirst coefficient. Broit[15]first proposed to model an image grid as an elastic membrane that is deformed under the influence of two forces that compete until equilibrium is reached.An external force tries to deform the image such that matching is achieved while an internal one enforces the elastic properties of the material. Bajcsy and Kovacic[16]extended this approach in a hierar-chical fashion where the solution of the coarsest scale is up-sam-pled and used to initialize thefiner one.Linear registration was used at the lowest resolution.Gee and Bajscy[17]formulated the elastostatic problem in a variational setting.The problem was solved under the Bayesian paradigm allowing for the computation of the uncertainty of the solution as well as for confidence intervals.Thefinite element method(FEM)was used to infer the displacements for the ele-ment nodes,while an interpolation strategy was employed to es-timate displacements elsewhere.The order of the interpolating or shape functions,determines the smoothness of the obtained result.Linear elastic models have also been used when registering brain images based on sparse correspondences.Davatzikos[18]first used geometric characteristics to establish a mapping be-tween the cortical surfaces.Then,a global transformation was estimated by modeling the images as inhomogeneous elastic ob-jects.Spatially-varying elasticity parameters were used to com-pensate for the fact that certain structures tend to deform more than others.In addition,a nonzero initial strain was considered so that some structures expand or contract naturally.In general,an important drawback of registration is that when source and target volumes are interchanged,the obtained trans-formation is not the inverse of the previous solution.In order to tackle this shortcoming,Christensen and Johnson[19]pro-posed to simultaneously estimate both forward and backward transformations,while penalizing inconsistent transformations by adding a constraint to the objective function.Linear elasticity was used as regularization constraint and Fourier series were used to parametrize the transformation.Leow et al.[20]took a different approach to tackle the incon-sistency problem.Instead of adding a constraint that penalizes the inconsistency error,they proposed a unidirectional approach that couples the forward and backward transformation and pro-vides inverse consistent transformations by construction.The coupling was performed by modeling the backward transforma-tion as the inverse of the forward.This fact was also exploited during the optimization of the symmetric energy by only fol-lowing the gradient direction of the forward mapping.He and Christensen[21]proposed to tackle large deforma-tions in an inverse consistent framework by considering a se-quence of small deformation transformations,each modeled by a linear elastic model.The problem was symmetrized by consid-ering a periodic sequence of images where thefirst(or last)and middle image are the source and target respectively.The sym-metric objective function thus comprised terms that quantify the difference between any two successive pairs of images.The in-ferred incremental transformation maps were concatenated to map one input image to another.b)Nonlinear Models:An important limitation of linear elastic models lies in their inability to cope with large defor-mations.In order to account for large deformations,nonlinear elastic models have been proposed.These models also guar-antee the preservation of topology.Rabbitt et al.[22]modeled the deformable image based on hyperelastic material properties.The solution of the nonlinear equations was achieved by local linearization and the use of the Finite Element method.Pennec et al.[23]dropped the linearity assumption by mod-eling the deformation process through the St Venant-Kirchoff elasticity energy that extends the linear elastic model to the non-linear regime.Moreover,the use of log-Euclidean metrics in-stead of Euclidean ones resulted in a Riemannian elasticity en-ergy which is inverse consistent.Yanovsky et al.[24]proposed a symmetric registration framework based on the St Venant-Kir-choff elasticity.An auxiliary variable was added to decouple the regularization and the matching term.Symmetry was im-posed by assuming that the Jacobian determinants of the defor-mation follow a zero mean,after log-transformation,log-normal distribution[25].Droske and Rumpf[26]used an hyperelastic,polyconvex regularization term that takes into account the length,area and volume deformations.Le Guyader and Vese[27]presented an approach that combines segmentation and registration that is based on nonlinear elasticity.The authors used a polyconvex regularization energy based on the modeling of the images under deformation as Ciarlet-Geymonat materials[28].Burger et al.[29]also used a polyconvex regularization term.The au-thors focused on the numerical implementation of the registra-tion framework.They employed a discretize-then-optimize ap-proach[9]that involved the partitioning voxels to24tetrahedra.2)Viscous Fluid Flow Models:In this case,the image under deformation is modeled as a viscousfluid.The transformation is governed by the Navier-Stokes equation that is simplified by assuming a very low Reynold’s numberflow(3) These models do not assume small deformations,and thus are able to recover large deformations[30].Thefirst term of theSOTIRAS et al.:DEFORMABLE MEDICAL IMAGE REGISTRATION:A SURVEY1157Navier-Stokes equation(3),constrains neighboring points to de-form similarly by spatially smoothing the velocityfield.The velocityfield is related to the displacementfield as.The velocityfield is integrated in order to estimate the displacementfield.The second term al-lows structures to change in mass while and are the vis-cosity coefficients.Christensen et al.[30]modeled the image under deformation as a viscousfluid allowing for large magnitude nonlinear defor-mations.The PDE was solved for small time intervals and the complete solution was given by an integration over time.For each time interval a successive over-relaxation(SOR)scheme was used.To guarantee the preservation of topology,the Jaco-bian was monitored and each time its value fell under0.5,the deformed image was regridded and a new one was generated to estimate a transformation.Thefinal solution was the con-catenation of all successive transformations occurring for each regridding step.In a subsequent work,Christensen et al.[31] presented a hierarchical way to recover the transformations for brain anatomy.Initially,global affine transformation was per-formed followed by a landmark transformation model.The re-sult was refined byfluid transformation preceded by an elastic registration step.An important drawback of the earliest implementations of the viscousfluid models,that employed SOR to solve the equa-tions,was computational inefficiency.To circumvent this short-coming,Christensen et al.employed a massive parallel com-puter implementation in[30].Bro-Nielsen and Gramkow[32] proposed a technique based on a convolutionfilter in scale-space.Thefilter was designed as the impulse response of the linear operator defined in its eigen-function basis.Crun et al.[33]proposed a multi-grid approach towards handling anisotropic data along with a multi-resolution scheme opting forfirst recovering coarse velocity es-timations and refining them in a subsequent step.Cahill et al.[34]showed how to use Fourier methods to efficiently solve the linear PDE system that arises from(3)for any boundary condi-tion.Furthermore,Cahill et al.extended their analysis to show how these methods can be applied in the case of other regu-larizers(diffusion,curvature and elastic)under Dirichlet,Neu-mann,or periodic boundary conditions.Wang and Staib[35]usedfluid deformation models in an atlas-enhanced registration setting while D’Agostino et al. tackled multi-modal registration with the use of such models in[36].More recently,Chiang et al.[37]proposed an inverse consistent variant offluid registration to register Diffusion Tensor images.Symmetrized Kullback-Leibler(KL)diver-gence was used as the matching criterion.Inverse consistency was achieved by evaluating the matching and regularization criteria towards both directions.3)Diffusion Models:In this case,the deformation is mod-eled by the diffusion equation(4) Let us note that most of the algorithms,based on this transforma-tion model and described in this section,do not explicitly state the(4)in their objective function.Nonetheless,they exploit the fact that the Gaussian kernel is the Green’s function of the diffu-sion equation(4)(under appropriate initial and boundary condi-tions)to provide an efficient regularization step.Regularization is efficiently performed through convolutions with a Gaussian kernel.Thirion,inspired by Maxwell’s Demons,proposed to perform image matching as a diffusion process[38].The proposed algo-rithm iterated between two steps:1)estimation of the demon forces for every demon(more precisely,the result of the appli-cation of a force during one iteration step,that is a displace-ment),and2)update of the transformation based on the cal-culated forces.Depending on the way the demon positions are selected,the way the space of deformations is defined,the in-terpolation method that is used,and the way the demon forces are calculated,different variants can be obtained.The most suit-able version for medical image analysis involved1)selecting all image elements as demons,2)calculating demon forces by considering the opticalflow constraint,3)assuming a nonpara-metric deformation model that was regularized by applying a Gaussianfilter after each iteration,and4)a trilinear interpo-lation scheme.The Gaussianfilter can be applied either to the displacementfield estimated at an iteration or the updated total displacementfield.The bijectivity of the transformation was en-sured by calculating for every point the difference between its initial position and the one that is reached after composing the forward with the backward deformationfield,and redistributing the difference to eachfield.The bijectivity of the transformation can also be enforced by limiting the maximum length of the up-date displacement to half the voxel size and using composition to update the transformation.Variants for the contour-based reg-istration and the registration between segmented images were also described in[38].Most of the algorithms described in this section were inspired by the work of Thirion[38]and thus could alternatively be clas-sified as“Demons approaches.”These methods share the iter-ative approach that was presented in[38]that is,iterating be-tween estimating the displacements and regularizing to obtain the transformation.This iterative approach results in increased computational efficiency.As it will be discussed later in this section,this feature led researchers to explore such strategies for different PDEs.The use of Demons,as initially introduced,was an efficient algorithm able to provide dense correspondences but lacked a sound theoretical justification.Due to the success of the algo-rithm,a number of papers tried to give theoretical insight into its workings.Fischer and Modersitzki[39]provided a fast algo-rithm for image registration.The result was given as the solution of linear system that results from the linearization of the diffu-sion PDE.An efficient scheme for its solution was proposed while a connection to the Thirion’s Demons algorithm[38]was drawn.Pennec et al.[40]studied image registration as an energy minimization problem and drew the connection of the Demons algorithm with gradient descent schemes.Thirion’s image force based on opticalflow was shown to be equivalent with a second order gradient descent on the Sum of Square Differences(SSD) matching criterion.As for the regularization,it was shown that the convolution of the global transformation with a Gaussian。
改良“轴保持短缩法”单人操作结肠镜在结肠术后患者检查中的临床应用

·临床研究·doi :10.3969/j.issn.1006-5725.2011.02.016基金项目:解放军总医院苗圃基金项目(编号:09MP05)作者单位:100853北京市,解放军总医院南楼消化内镜诊疗科通信作者:王志强E-mail :yxmnc1956@163.com结肠镜是检查大肠疾病可靠、有效的检查方法[1],自从日本消化内镜专家工藤进英提出“轴保持短缩法”及“Jiggling 手技法”[2]以来,结肠镜单人操作技术日趋完美。
因其操作安全、简便、患者痛苦小、成功率高、患者反应良好、又节省人力,单人操作结肠镜已经逐渐成为国际上结肠镜插入法的主流技术。
结肠术后患者由于肠管部分切除、吻合手术造成结肠正常生理走形改变,肠管之间、肠管与腹壁之间可能存在粘连,给结肠镜的插入带来不便[3]。
我们改良了工藤进英教授提出的单人操作结肠镜“轴保持短缩法”,对结肠术后患者进行检查,与传统单人操作结肠镜方法进行对比,探讨该方法对结肠术后患者检查的临床作用。
1资料与方法1.1一般资料2009年4-12月来我院消化内镜中心进行随访的结肠术后患者512例,其中男317改良“轴保持短缩法”单人操作结肠镜在结肠术后患者检查中的临床应用李明阳王志强令狐恩强卢忠生黄启阳摘要目的:探讨改良“轴保持短缩法”单人操作结肠镜在结肠术后患者检查中的临床应用。
方法:对2009年4-12月来我院消化内镜中心进行随访的512例结肠术后患者,分别应用改良“轴保持短缩法”单人操作法以及常规单人操作结肠镜进行检查,对两种方法的成功率、进镜时间、疼痛评分等指标进行比较。
结果:常规单人操作结肠镜和改良“轴保持短缩法”单人操作结肠镜到达回盲部(或结肠-小肠吻合口)的成功率分别为93.8%和99.1%。
常规单人操作结肠镜检查和改良“轴保持短缩法”单人操作结肠镜的平均进镜时间分别为7.6min 和3.5min (P <0.05)。
常规单人操作结肠镜和改良“轴保持短缩法”单人操作结肠镜检查后,采用数字评定量表(NRS )评定疼痛程度的平均分数分别为6.7分和3.8分(P <0.05)。
Multimodality Image Registration by Maximization of Mutual Information
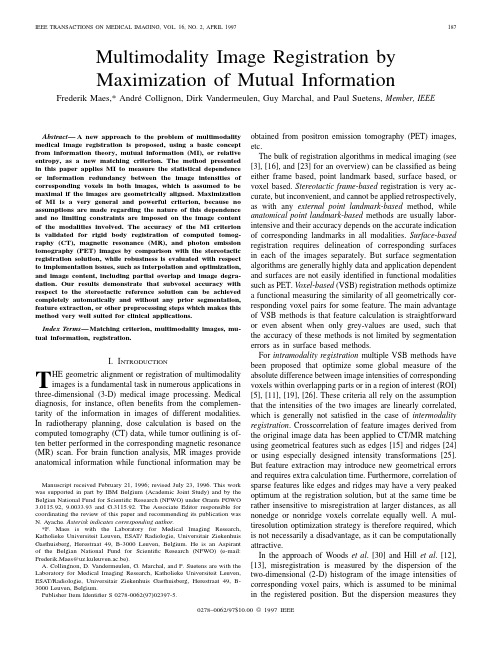
Multimodality Image Registration byMaximization of Mutual Information Frederik Maes,*Andr´e Collignon,Dirk Vandermeulen,Guy Marchal,and Paul Suetens,Member,IEEEAbstract—A new approach to the problem of multimodality medical image registration is proposed,using a basic concept from information theory,mutual information(MI),or relative entropy,as a new matching criterion.The method presented in this paper applies MI to measure the statistical dependence or information redundancy between the image intensities of corresponding voxels in both images,which is assumed to be maximal if the images are geometrically aligned.Maximization of MI is a very general and powerful criterion,because no assumptions are made regarding the nature of this dependence and no limiting constraints are imposed on the image content of the modalities involved.The accuracy of the MI criterion is validated for rigid body registration of computed tomog-raphy(CT),magnetic resonance(MR),and photon emission tomography(PET)images by comparison with the stereotactic registration solution,while robustness is evaluated with respect to implementation issues,such as interpolation and optimization, and image content,including partial overlap and image degra-dation.Our results demonstrate that subvoxel accuracy with respect to the stereotactic reference solution can be achieved completely automatically and without any prior segmentation, feature extraction,or other preprocessing steps which makes this method very well suited for clinical applications.Index Terms—Matching criterion,multimodality images,mu-tual information,registration.I.I NTRODUCTIONT HE geometric alignment or registration of multimodality images is a fundamental task in numerous applications in three-dimensional(3-D)medical image processing.Medical diagnosis,for instance,often benefits from the complemen-tarity of the information in images of different modalities. In radiotherapy planning,dose calculation is based on the computed tomography(CT)data,while tumor outlining is of-ten better performed in the corresponding magnetic resonance (MR)scan.For brain function analysis,MR images provide anatomical information while functional information may beManuscript received February21,1996;revised July23,1996.This work was supported in part by IBM Belgium(Academic Joint Study)and by the Belgian National Fund for Scientific Research(NFWO)under Grants FGWO 3.0115.92,9.0033.93and G.3115.92.The Associate Editor responsible for coordinating the review of this paper and recommending its publication was N.Ayache.Asterisk indicates corresponding author.*F.Maes is with the Laboratory for Medical Imaging Research, Katholieke Universiteit Leuven,ESAT/Radiologie,Universitair Ziekenhuis Gasthuisberg,Herestraat49,B-3000Leuven,Belgium.He is an Aspirant of the Belgian National Fund for Scientific Research(NFWO)(e-mail: Frederik.Maes@uz.kuleuven.ac.be).A.Collingnon,D.Vandermeulen,G.Marchal,and P.Suetens are with the Laboratory for Medical Imaging Research,Katholieke Universiteit Leuven, ESAT/Radiologie,Universitair Ziekenhuis Gasthuisberg,Herestraat49,B-3000Leuven,Belgium.Publisher Item Identifier S0278-0062(97)02397-5.obtained from positron emission tomography(PET)images, etc.The bulk of registration algorithms in medical imaging(see [3],[16],and[23]for an overview)can be classified as being either frame based,point landmark based,surface based,or voxel based.Stereotactic frame-based registration is very ac-curate,but inconvenient,and cannot be applied retrospectively, as with any external point landmark-based method,while anatomical point landmark-based methods are usually labor-intensive and their accuracy depends on the accurate indication of corresponding landmarks in all modalities.Surface-based registration requires delineation of corresponding surfaces in each of the images separately.But surface segmentation algorithms are generally highly data and application dependent and surfaces are not easily identified in functional modalities such as PET.Voxel-based(VSB)registration methods optimize a functional measuring the similarity of all geometrically cor-responding voxel pairs for some feature.The main advantage of VSB methods is that feature calculation is straightforward or even absent when only grey-values are used,such that the accuracy of these methods is not limited by segmentation errors as in surface based methods.For intramodality registration multiple VSB methods have been proposed that optimize some global measure of the absolute difference between image intensities of corresponding voxels within overlapping parts or in a region of interest(ROI) [5],[11],[19],[26].These criteria all rely on the assumption that the intensities of the two images are linearly correlated, which is generally not satisfied in the case of intermodality registration.Crosscorrelation of feature images derived from the original image data has been applied to CT/MR matching using geometrical features such as edges[15]and ridges[24] or using especially designed intensity transformations[25]. But feature extraction may introduce new geometrical errors and requires extra calculation time.Furthermore,correlation of sparse features like edges and ridges may have a very peaked optimum at the registration solution,but at the same time be rather insensitive to misregistration at larger distances,as all nonedge or nonridge voxels correlate equally well.A mul-tiresolution optimization strategy is therefore required,which is not necessarily a disadvantage,as it can be computationally attractive.In the approach of Woods et al.[30]and Hill et al.[12], [13],misregistration is measured by the dispersion of the two-dimensional(2-D)histogram of the image intensities of corresponding voxel pairs,which is assumed to be minimal in the registered position.But the dispersion measures they0278–0062/97$10.00©1997IEEEpropose are largely heuristic.Hill’s criterion requires seg-mentation of the images or delineation of specific histogram regions to make the method work [20],while Woods’criterion is based on additional assumptions concerning the relationship between the grey-values in the different modalities,which reduces its applicability to some very specific multimodality combinations (PET/MR).In this paper,we propose to use the much more general notion of mutual information (MI)or relative entropy [8],[22]to describe the dispersive behavior of the 2-D histogram.MI is a basic concept from information theory,measuring the statistical dependence between two random variables or the amount of information that one variable contains about the other.The MI registration criterion presented here states that the MI of the image intensity values of corresponding voxel pairs is maximal if the images are geometrically aligned.Because no assumptions are made regarding the nature of the relation between the image intensities in both modalities,this criterion is very general and powerful and can be applied automatically without prior segmentation on a large variety of applications.This paper expands on the ideas first presented by Collignon et al .[7].Related work in this area includes the work by Viola and Wells et al .[27],[28]and by Studholme et al .[21].The theoretical concept of MI is presented in Section II,while the implementation of the registration algorithm is described in Section III.In Sections IV,V,and VI we evaluate the accuracy and the robustness of the MI matching criterion for rigid body CT/MR and PET/MR registration.Section VII summarizes our current findings,while Section VIII gives some directions for further work.In the Appendexes,we discuss the relationship of the MI registration criterion to other multimodality VSB criteria.II.T HEORYTwo randomvariables,,with marginal probabilitydistributions,and:.MI,and(1)MI is related to entropy by theequationsandgivengiven(5)(7)Theentropy,whilewhenknowingby the knowledge of another randomvariablecontainsaboutandandand.The MI registration criterion states that the images are geometrically aligned by thetransformation forwhichMAES et al.:MULTIMODALITY IMAGE REGISTRATION BY MAXIMIZATION OF MUTUAL INFORMATION189(a)(b)Fig.1.Joint histogram of the overlapping volume of the CT and MR brain images of dataset A in Tables II and III:(a)Initial position:I (CT;MR )=0:46,(b)registered position:I (CT;MR )=0:89.Misregistration was about 20mm and 10 (see the parameters in Table III).If both marginaldistributionsand,the MI criterion reduces to minimizing the jointentropyor ,which is the case if one of the images is always completely contained in the other,the MI criterion reduces to minimizing the conditionalentropyisvariedandand.The MI criterion takes this into accountexplicitly,as becomes clear in (2),which can be interpreted as follows [27]:“maximizing MI will tend to find as much as possible of the complexity that is in the separate datasets (maximizing the first two terms)so that at the same time they explain each other well (minimizing the last term).”For.Thisrequiresis varied,which will be the case if the image intensity values are spatially correlated.This is illustrated by the graphs in Fig.2,showing the behaviorofaxis along the row direction,theaxis along the plane direction.One of the images is selected to be the floatingimage,are taken and transformed intothe referenceimage,or a sub-or superset thereof.Subsampling of the floating image might be used to increase speed performance,while supersampling aims at increasing accuracy.For each value of the registrationparameterfalls inside the volumeofis a six-component vector consisting of three rotationanglestoimage(8)with3diagonal matrixes representing thevoxel sizes ofimages,respectively (inmillimeters),3rotation matrix,with thematrixes-,-axis,respectively,and190IEEE TRANSACTIONS ON MEDICAL IMAGING,VOL.16,NO.2,APRIL1997Fig.3.Graphical illustration of NN,TRI,and PV interpolation in 2-D.NN and TRI interpolation find the reference image intensity value at position T s and update the corresponding joint histogram entry,while PV interpolation distributes the contribution of this sample over multiple histogram entries defined by its NN intensities,using the same weights as for TRI interpolation.B.CriterionLetatposition.The joint image intensityhistogramis computed by binning the image intensitypairs forallbeing the total number of bins in the joint histogram.Typically,weusewill not coincide with a grid pointofis generally insufficient to guaranteesubvoxel accuracy,as it is insensitive to translations up to one voxel.Other interpolation methods,such as trilinear (TRI)interpolation,may introduce new intensity values which are originally not present in the reference image,leading tounpredictable changes in the marginaldistributionof the reference image for small variationsof,the contribution of the imageintensityofon the gridofis varied.Estimations for the marginal and joint image intensitydistributionsis then evaluatedby(12)and the optimal registrationparameter is foundfrom,using Brent’s one-dimensional optimization algorithm for the line minimizations [18].The direction matrix is initialized with unit vectors in each of the parameter directions.An appropriate choice for the order in which the parameters are optimized needs to be specified,as this may influence optimization robustness.For instance,when matching images of the brain,the horizontal translation and the rotation around the vertical axis are more constrained by the shape of the head than the pitching rotation around the left-to-right horizontal axis.There-fore,first aligning the images in the horizontal plane by first optimizing the in-planeparameters may facilitate the optimization of the out-of-planeparametersMAES et al.:MULTIMODALITY IMAGE REGISTRATION BY MAXIMIZATION OF MUTUAL INFORMATION 191TABLE IID ATASETS U SEDIN THEE XPERIMENTS D ISCUSSED IN S ECTIONS VANDVIIV.E XPERIMENTSThe performance of the MI registration criterion was eval-uated for rigid-body registration of MR,CT,and PET images of the brain of the same patient.The rigid-body assumption is well satisfied inside the skull in 3-D scans of the head if patient related changes (due to for instance interscanning operations)can be neglected,provided that scanner calibration problems and problems of geometric distortions have been minimized by careful calibration and scan parameter selection,respectively.Registration accuracy is evaluated in Section V by comparison with external marker-based registration results and other retrospective registration methods,while the robust-ness of the method is evaluated in Section VI with respect to implementation issues,such as sampling,interpolation and op-timization,and image content,including image degradations,such as noise,intensity inhomogeneities and distortion,and partial image overlap.Four different datasets are used in the experiments described below (Table II).Dataset A 1contains high-resolution MR and CT images,while dataset B was obtained by smoothing and subsampling the images of dataset A to simulate lower resolution data.Dataset C 2contains stereotactically acquired MR,CT,and PET images,which have been edited to remove stereotactic markers.Dataset D contains an MR image only and is used to illustrate the effect of various image degradations on the registration criterion.All images consist of axial slices and in all casestheaxis is directedhorizontally front to back,andthedirection.In all experiments,the joint histogram size is256axis (0.7direction due to an offset inthedirection for the solution obtainedusing PV interpolation due to a 1rotation parameter.For MR to PET as well as for PET to MR registration,PV interpolation yields the smallest differences with the stereotactic reference solution,especially inthedirection due to offsets inthe192IEEE TRANSACTIONS ON MEDICAL IMAGING,VOL.16,NO.2,APRIL 1997TABLE IIIR EFERENCE AND MI R EGISTRATION P ARAMETERS FOR D ATASETS A,B,AND C AND THE M EAN AND M AXIMAL A BSOLUTE D IFFERENCE E V ALUATED AT E IGHT P OINTS N EAR THE B RAIN SURFACEvolume as the floating image and using different interpolation methods.For each combination,various optimization strate-gies were tried by changing the order in which the parameters were optimized,each starting from the same initial position with all parameters set to zero.The results are summarized in Fig.5.These scatter plots compare each of the solutions found (represented by their registrationparameterson the horizontal axis (using mm and degreesfor the translation and rotation parameters,respectively)and by the difference in the value of the MI criterion(MI)on the vertical axis.Although the differences are small for each of the interpolation methods used,MR to CT registration seems to be somewhat more robust than CT to MR registration.More importantly,the solutions obtained using PV interpolation are much more clustered than those obtained using NN or TRI interpolation,indicating that the use of PV interpolation results in a much smoother behavior of the registration criterion.This is also apparent from traces in registration space computed around the optimal solution for NN,TRI,and PV interpolation (Fig.6).These traces look very similar when a large parameter range is considered,but in the neighborhood of the registration solution,traces obtained with NN and TRI interpolation are noisy and show manylocal maxima,while traces obtained with PV interpolation are almost quadratic around the optimum.Remark that the MI values obtained using TRI interpolation are larger than those obtained using NN or PV interpolation,which can be interpreted according to (2):The TRI averaging and noise reduction of the reference image intensities resulted in a larger reduction of the complexity of the joint histogram than the corresponding reduction in the complexity of the reference image histogram itself.B.SubsamplingThe computational complexity of the MI criterion is pro-portional to the number of samples that is taken from the floating image to compute the joint histogram.Subsampling of the floating image can be applied to increase speed perfor-mance,as long as this does not deteriorate the optimization behavior.This was investigated for dataset A by registration of the subsampled MR image with the original CT image using PV interpolation.Subsampling was performed by takingsamples on a regular grid at sample intervalsofand direction,respectively,using NNinterpolation.No averaging or smoothing of the MR image before subsampling was applied.Weused,and .The same optimization strategy was used in each case.RegistrationsolutionsandMAES et al.:MULTIMODALITY IMAGE REGISTRATION BY MAXIMIZATION OF MUTUAL INFORMATION193(a)(b)Fig.5.Evaluation of the MI registration robustness for dataset A.Horizontal axis:norm of the difference vector j 0 3j for different optimization strategies,using NN,TRI,and PV interpolation. 3corresponds to the registration solution with the best value for the registration criterion for each of the interpolation schemes applied.Vertical axis:difference in the registration criterion between each solution and the optimal one.(a)Using the CT image as the floating image.(b)Using the MR image as the floatingimage.(a)(b)(c)(d)Fig.6.MI traces around the optimal registration position for dataset A:Rotation around the x axis in the range from 0180to +180 (a)and from 00.5to +0.5 (bottom row),using NN (b),TRI (c),and PV (d)interpolation.intheand 0.2mm off from the solutionfound without subsampling.C.Partial OverlapClinically acquired images typically only partially overlap,as CT scanning is often confined to a specific region to minimize the radiation dose while MR protocols frequently image larger volumes.The influence of partial overlap on the registration robustness was evaluated for dataset A for CT to MR registration using PV interpolation.The images were initially aligned as in the experiment in Section V and the same optimization strategy was applied,but only part of the CT data was considered when computing the MI criterion.More specifically,three 50-slice slabs were selected at the bottom (the skull basis),the middle,and the top part of the dataset.The results are summarized in Table IV and compared with the solution found using the full dataset by the mean and194IEEE TRANSACTIONS ON MEDICAL IMAGING,VOL.16,NO.2,APRIL 1997TABLE IVI NFLUENCEOFP ARTIAL O VERLAPONTHE R EGISTRATION R OBUSTNESSFORCTTOMR R EGISTRATIONOFD ATASETAFig.7.Effect of subsampling the MR floating image of dataset A on the registration solution.Horizontal axis:subsampling factor f ,indicating that only one out of f voxels was considered when evaluating the MI criterion.Vertical axis:norm of the difference vector j 0 3j . 3corresponds to the registration solution obtained when no subsampling is applied.maximal absolute difference evaluated over the full image at the same eight points as in Section V.The largest parameter differences occur for rotation aroundthedirection,resulting in maximal coordinate differencesup to 1.5CT voxel inthe direction,but on average all differences are subvoxel with respect to the CT voxel sizes.D.Image DegradationVarious MR image degradation effects,such as noise,in-tensity inhomogeneity,and geometric distortion,alter the intensity distribution of the image which may affect the MI registration criterion.This was evaluated for the MR image of dataset D by comparing MI registration traces obtained for the original image and itself with similar traces obtained for the original image and its degraded version (Fig.8).Such traces computed for translation inthewas alteredinto(15)(a)(b)(c)(d)Fig.8.(a)Slice 15of the original MR image of dataset D,(b)zero mean noise added with variance of 500grey-value units,(c)quadratic inhomogeneity (k =0:004),and (d)geometric distortion (k =0:00075).with being the image coordinates of the point around which the inhomogeneity is centeredand.All traces for all param-eters reach their maximum at the same position and the MI criterion is not affected by the presence of the inhomogeneity.3)Geometric Distortion:Geometricdistortions(16)(17)(18)withthe image coordinates of the center of each image planeandtranslation parameter proportionalto the averagedistortionMAES et al.:MULTIMODALITY IMAGE REGISTRATION BY MAXIMIZATION OF MUTUAL INFORMATION195(a)(b)(c)(d)Fig.9.MI traces using PV interpolation for translation in the x direction of the original MR image of dataset D over its degraded version in the range from 010to +10mm:(a)original,(b)noise,(c)intensity inhomogeneity,and (d)geometric distortion.VII.D ISCUSSIONThe MI registration criterion presented in this paper assumes that the statistical dependence between corresponding voxel intensities is maximal if both images are geometrically aligned.Because no assumptions are made regarding the nature of this dependence,the MI criterion is highly data independent and allows for robust and completely automatic registration of multimodality images in various applications with min-imal tuning and without any prior segmentation or other preprocessing steps.The results of Section V demonstrate that subvoxel registration differences with respect to the stereo-tactic registration solution can be obtained for CT/MR and PET/MR matching without using any prior knowledge about the grey-value content of both images and the correspondence between them.Additional experiments on nine other datasets similar to dataset C within the Retrospective Registration Evaluation Project by Fitzpatrick et al .[10]have verified these results [29],[14].Moreover,Section VI-C demonstrated the robustness of the method with respect to partial over-lap,while it was shown in Section VI-D that large image degradations,such as noise and intensity inhomogeneities,have no significant influence on the MI registration crite-rion.Estimations of the image intensity distributions were ob-tained by simple normalization of the joint histogram.In all experiments discussed in this paper,the joint histogram was computed from the entire overlapping part of both images,using the original image data and a fixed number of bins of256andand .For low-resolutionimages,the optimization often did not converge to the global optimum if a different parameter order was specified,due to the occurrence of local optima especially forthe196IEEE TRANSACTIONS ON MEDICAL IMAGING,VOL.16,NO.2,APRIL1997theand40mm,but we have not extensivelyinvestigated the robustness of the method with respect to theinitial positioning of the images,for instance by using multiplerandomised starting estimates.The choice of thefloating imagemay also influence the behavior of the registration criterion.In the experiment of Section VI-A,MR to CT matching wasfound to be more robust than CT to MR matching.However,it is not clear whether this was caused by sampling andinterpolation issues or by the fact that the MR image is morecomplex than the CT image and that the spatial correlation ofimage intensity values is higher in the CT image than in theMR image.We have not tuned the design of the search strategy towardspecific applications.For instance,the number of criterionevaluations required may be decreased by taking the limitedimage resolution into account when determining convergence.Moreover,the results of Section VI-B demonstrate that forhigh-resolution images subsampling of thefloating imagecan be applied without deteriorating optimization robustness.Important speed-ups can,thus,be realized by using a mul-tiresolution optimization strategy,starting with a coarselysampled image for efficiency and increasing the resolution asthe optimization proceeds for accuracy[20].Furthermore,thesmooth behavior of the MI criterion,especially when usingPV interpolation,may be exploited by using gradient-basedoptimization methods,as explicit formulas for the derivativesof the MI function with respect to the registration parameterscan be obtained[27].All the experiments discussed in this paper were for rigid-body registration of CT,MR,and PET images of the brainof the same patient.However,it is clear that the MI criterioncan equally well be applied to other applications,using moregeneral geometric transformations.We have used the samemethod successfully for patient-to-patient matching of MRbrain images for correlation of functional MR data and forthe registration of CT images of a hardware phantom to itsgeometrical description to assess the accuracy of spiral CTimaging[14].MI measures statistical dependence by comparing the com-plexity of the joint distribution with that of the marginals.Bothmarginal distributions are taken into account explicitly,whichis an important difference with the measures proposed by Hillet al.[13](third-order moment of the joint histogram)andCollignon et al.[6](entropy of the joint histogram),whichfocus on the joint histogram only.In Appendexes A and B wediscuss the relationship of these criteria and of the measureof Woods et al.[30](variance of intensity ratios)to the MIcriterion.MI is only one of a family of measures of statisticaldependence or information redundancy(see Appendix C).We have experimentedwith,the entropy correlation coefficient[1].In some cases these measures performed better thanthe original MI criterion,but we could not establish a clearpreference for either of these.Furthermore,the use of MIfor multimodality image registration is not restricted to theoriginal image intensities only:other derived features such asedges or ridges can be used as well.Selection of appropriatefeatures is an area for further research.VIII.C ONCLUSIONThe MI registration criterion presented in this paper allowsfor subvoxel accurate,highly robust,and completely automaticregistration of multimodality medical images.Because themethod is largely data independent and requires no userinteraction or preprocessing,the method is well suited to beused in clinical practice.Further research is needed to better understand the influenceof implementation issues,such as sampling and interpolation,on the registration criterion.Furthermore,the performance ofthe registration method on clinical data can be improved bytuning the optimization method to specific applications,whilealternative search strategies,including multiresolution andgradient-based methods,have to be investigated.Finally,otherregistration criteria can be derived from the one presented here,using alternative information measures applied on differentfeatures.A PPENDIX AWe show the relationship between the multimodality reg-istration criterion devised by Hill et al.[12]and the jointentropy th-order moment of thescatter-plot[22]with the following properties.1)andand with。
ieee transactions on medical imaging的审稿流程
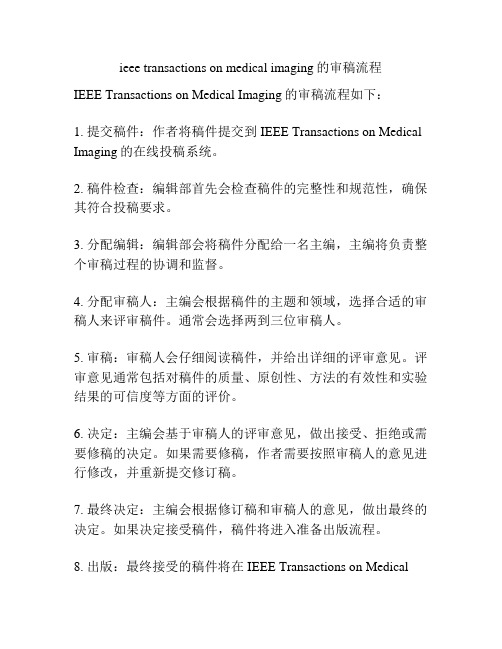
ieee transactions on medical imaging的审稿流程
IEEE Transactions on Medical Imaging的审稿流程如下:
1. 提交稿件:作者将稿件提交到IEEE Transactions on Medical Imaging的在线投稿系统。
2. 稿件检查:编辑部首先会检查稿件的完整性和规范性,确保其符合投稿要求。
3. 分配编辑:编辑部会将稿件分配给一名主编,主编将负责整个审稿过程的协调和监督。
4. 分配审稿人:主编会根据稿件的主题和领域,选择合适的审稿人来评审稿件。
通常会选择两到三位审稿人。
5. 审稿:审稿人会仔细阅读稿件,并给出详细的评审意见。
评审意见通常包括对稿件的质量、原创性、方法的有效性和实验结果的可信度等方面的评价。
6. 决定:主编会基于审稿人的评审意见,做出接受、拒绝或需要修稿的决定。
如果需要修稿,作者需要按照审稿人的意见进行修改,并重新提交修订稿。
7. 最终决定:主编会根据修订稿和审稿人的意见,做出最终的决定。
如果决定接受稿件,稿件将进入准备出版流程。
8. 出版:最终接受的稿件将在IEEE Transactions on Medical
Imaging上正式出版,并获得DOI编号。
整个审稿流程通常需要数周至数个月的时间,具体时间会因实际情况而有所变化。
ieee transactions on medical imaging的审稿流程
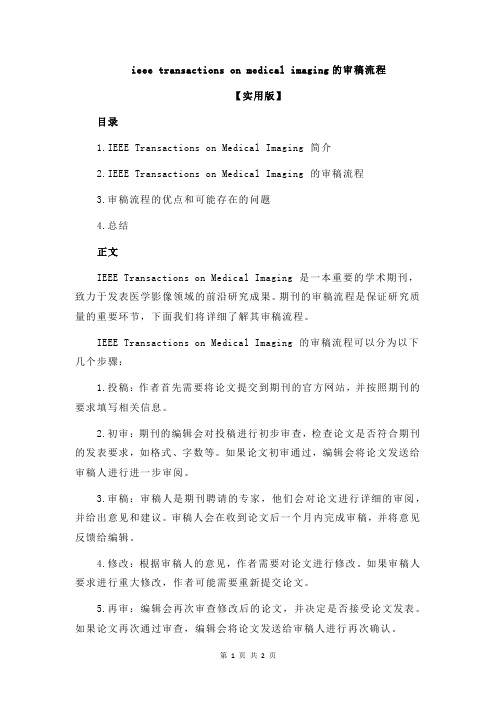
ieee transactions on medical imaging的审稿流程【实用版】目录1.IEEE Transactions on Medical Imaging 简介2.IEEE Transactions on Medical Imaging 的审稿流程3.审稿流程的优点和可能存在的问题4.总结正文IEEE Transactions on Medical Imaging 是一本重要的学术期刊,致力于发表医学影像领域的前沿研究成果。
期刊的审稿流程是保证研究质量的重要环节,下面我们将详细了解其审稿流程。
IEEE Transactions on Medical Imaging 的审稿流程可以分为以下几个步骤:1.投稿:作者首先需要将论文提交到期刊的官方网站,并按照期刊的要求填写相关信息。
2.初审:期刊的编辑会对投稿进行初步审查,检查论文是否符合期刊的发表要求,如格式、字数等。
如果论文初审通过,编辑会将论文发送给审稿人进行进一步审阅。
3.审稿:审稿人是期刊聘请的专家,他们会对论文进行详细的审阅,并给出意见和建议。
审稿人会在收到论文后一个月内完成审稿,并将意见反馈给编辑。
4.修改:根据审稿人的意见,作者需要对论文进行修改。
如果审稿人要求进行重大修改,作者可能需要重新提交论文。
5.再审:编辑会再次审查修改后的论文,并决定是否接受论文发表。
如果论文再次通过审查,编辑会将论文发送给审稿人进行再次确认。
6.接受发表:如果审稿人再次确认论文质量,编辑会接受论文发表,并将论文排版出版。
IEEE Transactions on Medical Imaging 的审稿流程具有以下优点:1.严格保证论文质量:通过多轮审稿,期刊可以确保发表的论文具有较高的学术价值。
2.公正公平:期刊采用双盲审稿制度,确保审稿过程公正公平。
3.提供反馈:审稿人会给出详细的意见和建议,帮助作者改进论文。
然而,审稿流程也可能存在一些问题,如审稿时间较长、审稿人意见不一等。
腰椎重力线在腰椎间盘病变患者中的变化分析及腰腿痛影像的相关性研究

腰椎重力线在腰椎间盘病变患者中的变化分析及腰腿痛影像的相关性研究潘广松谢承佳覃振党韦岑谢尚I谢其德马城(来宾市人民医院,广西546100)扌%要:目的:探讨腰椎重力线在腰椎间盘病变患者中的变化分析及腰腿痛影像的相关性°方法:选择2018年4月-2020年1月腰椎间盘病变及腰腿痛患者224例为研究对象,所有患者入院后均进行腰椎正侧位DR检查及腰椎CT 或MRI检查,完成患者腰椎重力线测定;采用视觉模拟疼痛(/AS)量表对患者疼痛进行评估;采用SPSSPearson相关性分析对腰椎间盘膨出、突出及腰椎滑脱患者腰椎重力线与腰腿痛进行相关性分析°结果:224例腰椎间盘膨出、突出及腰椎滑脱患者均完成腰椎重力线测定,腰椎重力线平均值为(0.24±0.04);重力线分级排在前两位的分别为重力线III级和重力线II级,分别占39.73%和33.04%;所有患者均完成腰腿痛评估,/AS评分为(5.39±0.69)分$SPSSPearson相关性分析结果表明:腰椎间盘病变腰椎重力线分级与腰腿痛VAS评分呈正相关性(P<0.05)o结论:腰椎重力线改变能参与腰椎间盘病变的发生、发展,且与腰腿痛存在相关性"加强腰椎重力线测定能指导临床诊疗,减轻患者疼痛与不适"关键词:腰椎重力线改变;腰椎间盘病变;腰腿痛;相关性中图分类号:R816.8文献标识码:B D0I:10.3969/j.issn.1001-0270.2021.01.11Analysis of Changes in Lumbar Spine Gravity Line in Patients with Lumbar Disc Disease and its Correlation with Low Back and Leg Pain ImagingPAN Guang-song,XIE Cheng-jia,QIN Zhen-Pang,WEI Cen,XIE Shang-meng,XIE Qi-de,MA Cheng (Laibin People,s Hospital,Guangxi546100,China)Abstract:Objective:To investigate changes in lumbar spine gravity line in patients with lumbar disc disease and its correlation with low back and leg pain imaging.Methods:224patients with lumbar disc disease and lumbocrural pain in April2018and January2020were selected as subjects.After admission,all patients were given lumbar forward and lateral DR examination and lumbar CT or MRI examination,and the measurement of lumbar gravity line was completed.Visual analogue pain scale (VAS)was used to evaluate the pain of patients.SPSSPearson correlation analysis was used to analyze the correlation between lumbar gravity line and lumbar leg pain in patients with lumbar disc bulge, protrusion and lumbar spondylolisthesis.Results:All the224patients with lumbar intervertebral disc bulge,protrusion and lumbar spondylolisthesis completed the measurement of the lumbar gravity line, and the mean value of the lumbar gravity line was(0.24±0.04).The top two gravity line grades are gravity line III and gravity line II,accounting for39.73%and33.04%,respectively.All patients completed the low back and leg pain assessment,VAS score was(5.39±0.69),SPSSPearson correlation收稿日期:2021-01-04analysis showed that there was a positive correlation between lumbar gravity line grading and VAS score of lumbar leg pain(P<0.05).Conclusion:The change of lumbar gravimetric line can participate in the occurrence and development of lumbar intervertebral disc disease,and is correlated with lumbo-leg pain.Strengthening the measurement of lumbar gravimetric line can guide clinical diagnosis and treatment,and relieve the pain and discomfort of patients.Key words:Changes in the Lumbar Gravity Line;Lumbar Disc Disease;Lumbocrural Pain;The Correlation腰椎间盘病变属于发病率较高的骨科疾病,临床以神经功能障碍、坐骨神经痛"腰紙部疼痛为主,且随着病情的不断发展,会引起患者姿势变化、活动受限对于急性期腰椎间盘病变患者,则会产生剧烈的疼痛感,不仅会影响患者工作,亦可影响其生活能力。
用弹簧分子系统进行弹性医学图像配准
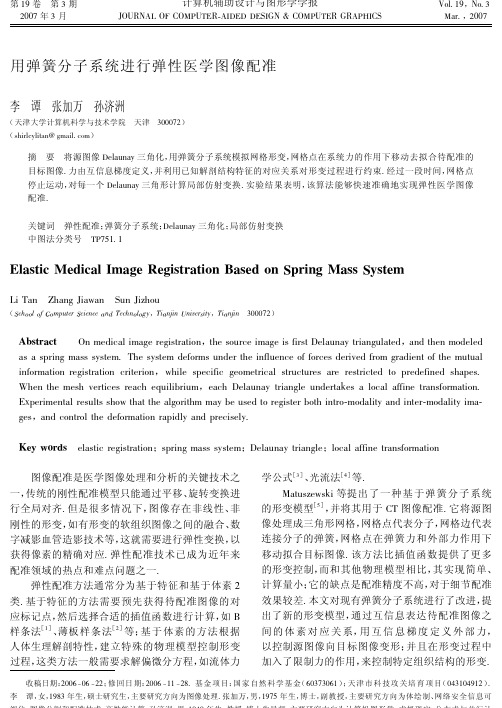
第19卷第3期2007年3月计算机辅助设计与图形学学报JOURNAL OF COMPUTER-AIDED DESIGN &COMPUTER GRAPHICSVoi.19,No.3Mar.,2007收稿日期:2006-06-22;修回日期:2006-11-28.基金项目:国家自然科学基金(60373061);天津市科技攻关培育项目(043104912).李 谭,女,1983年生,硕士研究生,主要研究方向为图像处理.张加万,男,1975年生,博士,副教授,主要研究方向为体绘制、网络安全信息可用弹簧分子系统进行弹性医学图像配准李 谭 张加万 孙济洲(天津大学计算机科学与技术学院 天津 300072)(shirieyiitan@ )摘要 将源图像Deiaunay 三角化,用弹簧分子系统模拟网格形变,网格点在系统力的作用下移动去拟合待配准的目标图像.力由互信息梯度定义,并利用已知解剖结构特征的对应关系对形变过程进行约束.经过一段时间,网格点停止运动,对每一个Deiaunay 三角形计算局部仿射变换.实验结果表明,该算法能够快速准确地实现弹性医学图像配准.关键词 弹性配准;弹簧分子系统;Deiaunay 三角化;局部仿射变换中图法分类号 TP751.1Elastic Medical Image Registration Based on Spring Mass SystemLi Tan Zhang Jiawan Sun Jizhou(School of Computer Science and Technology ,Tianjin Uniuersity ,Tianjin 300072)Abstract On medicai image registration ,the source image is first Deiaunay trianguiated ,and then modeiedas a spring mass system.The system deforms under the infiuence of forces derived from gradient of the mutuai information registration criterion ,whiie specific geometricai structures are restricted to predefined shapes.When the mesh vertices reach eguiiibrium ,each Deiaunay triangie undertakes a iocai affine transformation.Experimentai resuits show that the aigorithm may be used to register both intro-modaiity and inter-modaiity ima-ges ,and controi the deformation rapidiy and preciseiy.Key words eiastic registration ;spring mass system ;Deiaunay triangie ;iocai affine transformation 图像配准是医学图像处理和分析的关键技术之一,传统的刚性配准模型只能通过平移、旋转变换进行全局对齐.但是很多情况下,图像存在非线性、非刚性的形变,如有形变的软组织图像之间的融合、数字减影血管造影技术等,这就需要进行弹性变换,以获得像素的精确对应.弹性配准技术已成为近年来配准领域的热点和难点问题之一.弹性配准方法通常分为基于特征和基于体素2类.基于特征的方法需要预先获得待配准图像的对应标记点,然后选择合适的插值函数进行计算,如B样条法[1]、薄板样条法[2]等;基于体素的方法根据人体生理解剖特性,建立特殊的物理模型控制形变过程,这类方法一般需要求解偏微分方程,如流体力学公式[3]、光流法[4]等.Matuszewski 等提出了一种基于弹簧分子系统的形变模型[5],并将其用于CT 图像配准.它将源图像处理成三角形网格,网格点代表分子,网格边代表连接分子的弹簧,网格点在弹簧力和外部力作用下移动拟合目标图像.该方法比插值函数提供了更多的形变控制,而和其他物理模型相比,其实现简单、计算量小;它的缺点是配准精度不高,对于细节配准效果较差.本文对现有弹簧分子系统进行了改进,提出了新的形变模型,通过互信息表达待配准图像之间的体素对应关系,用互信息梯度定义外部力,以控制源图像向目标图像变形;并且在形变过程中加入了限制力的作用,来控制特定组织结构的形变.实验结果证明,本文算法配准精度更高,速度更快,对于多模态图像也能够有效地进行配准.!"形变模型设D表示待变形的源图像区域,对其进行Deiaunay三角化,分别为每一个Deiaunay三角形!k 计算变换T k:T k:P-P',V P E!k ,U!k=D(l)其中P和P'分别是三角形顶点的初始位置和新位置.图像形变由网格形变唯一确定,网格点位移轨迹用力学方程d2Pj d z2=fj+a(z)fSj+b(z)fgj+c(z)fj,V j E M(2)描述.其中,M是所有可移动网格点的集合;Pj表示点j的位置;fj 是作用于点j的耗散力;fSj表示j受到相邻顶点的弹簧力作用;外部力fgj表达待配准图像的体素相似性;限制力fj反映网格形变的约束条件;a(z),b(z)和c(z)分别表示各个作用力的权重.初始时刻,网格模型处于平衡状态,外部力和限制力作用均为0.随着时间的推移,在外部力和限制力的作用下,源图像逐渐向目标图像形变.文献[6]给出了fj ,fSj和fgj的计算公式,其中外部力fgj利用归一化相关系数反映图像之间的体素对应关系,精度不高,特别对于多模态图像,作用效果存在较大偏差.为了更精确地控制形变模型,本文对外部力进行了改进,同时给出了限制力fj的定义.!#!"外部力弹簧分子系统通过匹配局部纹理寻找源图像和目标图像之间的体素对应关系.互信息是一种基于图像灰度信息的统计度量标准,它精度高、稳健性强,对单模态和多模态图像都能取得很好的配准效果.本文采用归一化互信息(normaiized mutuai in-formation,NMI)[7]衡量局部纹理相似性.外部力是控制源图像向目标图像形变的动力.对于源图像上以网格点j为中心的局部图像窗口WS{j},设目标图像上与之最相似的纹理,即归一化互信息值最大的图像窗口为WT{j'},窗口中心为j'.认为j'是j在目标图像上的对应点,j在外部力的作用向j'移动,如图l所示.设在z时刻,j移动到j(z),外部力fgj=d NMI(WT{j(z)},WS{j})d z,NMI WT{j(z)},WS{j()}=H WT{j(z)()}+H WS{j()}H WT{j(z)},WS{j()};其中WT{}和WS{}分别表示源图像和目标图像上以某点为中心的局部图像窗口,它们之间的互信息由联合直方图计算得到.图l 外部力作用用互信息梯度定义外部力能够有效地实现网格点的运动效果.如果j(z)的运动轨迹朝向j',互信息呈增加趋势,fgj>0,j(z)沿当前方向继续移动;若j(z)的运动轨迹背离j',互信息降低,fgj<0,促使j(z)朝相反方向移动.j(z)离j'越远,互信息梯度值越大,外部力作用效果越明显;随着j(z)-j',互信息梯度-0,fgj-0,网格点移动速度越来越小.当j(z)移动到j'时,外部力作用为0,网格点停止运动.!#$"限制力局部图像纹理匹配只考虑平移变换,而忽略了旋转、缩放和局部扭曲的影响,配准精度不高,仅靠外部力很难精确地控制待配准图像的拟合.将源图像看成由2个部分组成:具有明显解剖结构特征的区域和非特征区域.后者在外部力的作用下自由变形,而前者需要遵循特定的形变限制.限制力的作用就是利用待配准图像的结构特征信息约束弹簧分子系统,提高配准精度.限制力的作用效果如图2所示,其中S和S'分别为同一器官在源图像和目标图像上的轮廓.配准前二者形状存在明显差异,在限制力的作用下,源图像轮廓S逐渐向目标图像轮廓S'形变,直至二者形状大致相同.假设已经从待配准图像中提取出了组对应轮廓特征C-C',k=l,2,…,;253计算机辅助设计与图形学学报2007年!"j =-c D(#j,$'k)c t,if j E$k,k=l,2,…,I0,if j G Ck,k=l,2,…,{I(3)其中,Ck 和C'k分别表示源图像和目标图像上的轮廓曲线,D(#j ,$'k)表示点j到$'k的距离函数.根据式(3),只有源图像上的特征轮廓点才受到限制力的作用,限制力大小等于该点和对应目标轮廓之间距离函数的梯度大小,方向与梯度方向相反.图像轮廓形状一般较为复杂,很难用数学函数进行描述.本文将轮廓曲线表示为离散轮廓点的集合,距离函数D(#j ,$'k)定义为D(#j ,$'k)=mini E C'k(pj-pi).图2 限制力作用!"算法实现流程弹簧力保证网格形变的一致性,基于互信息梯度的外部力对图像内部组织结构进行配准,而限制力主要实现针对轮廓特征的配准,弹簧力和外部力直接由待配准图像计算得到.为了计算限制力,需要从待配准图像上提取出对应轮廓特征,确定形变初始网格.尤其对于多模态图像,应选择合适的轮廓提取算法,找到不同模态下对应的轮廓曲线.本文采用如下轮廓提取算法:先用基于直方图的阈值分割方法消除图像背景噪声,然后施加Can-ny算子进行边缘检测,再用边界跟踪方法提取轮廓.通常将源图像和目标图像的外轮廓看成是一组对应特征,内部组织结构的轮廓对应关系通过手工标记获得.对于同模态和异模态图像,上述步骤都能够有效地提取出对应轮廓曲线.基于提取出的轮廓特征,本文采用限制性Deiaunay三角化算法[8]实现网格化操作,将提取出的源图像轮廓曲线作为Deiaunay三角网格的边界限制条件,轮廓曲线经过重采样,采样比例为41l.重采样的目的是为了减少生成的网格数量,节省计算时间.背景区域的Deiaunay三角形不需要进行形变,合.对于所有网格点,计算以该点为中心的局部图像均值:如果局部图像均值大于全局图像均值,认为该点属于可移动点集合M;否则,该点属于背景区域,它在形变过程中位置固定不变.对所有的可移动点求解式(2)所示的二阶微分方程.由于微分方程的数值解法速度较慢,本文采用了一种加速实现算法,步骤如下:Stepl.对于落在源图像特征轮廓上的网格点,计算限制力和外部力的作用.设网格点j E$k,在目标图像对应轮廓上寻找使局部互信息取最大值的点j'=arg maxi,i E C'kNMI(WT{i},WS{j}),令#'j=#j'.Step2.对于源图像上的其他可移动网格点计算外部力的作用.设网格点j G$k&j E M,且以j为中心的局部图像方差足够大,在目标图像该位置附近寻找使局部互信息取最大值的点j'=arg maxi,i E Ieighborhood(j)NMI(WT{i},WS{j}),令#'j=#j'.Step3.将外部力和限制力设为0,用四阶龙格-库塔方法求解式(2),直至分子弹簧系统在弹簧力和耗散力的作用下达到平衡状态.Step4.在得到网格点新位置#'后,对源图像上的每个Deiaunay三角形,如式(l)所示进行局部仿射变换,由Deiau-nay三角形顶点的初始位置和新位置计算变换参数.Step5.设配准前源图像和目标图像之间的互信息值为NMI,配准后为NMI'.如果NMI'>NMI,则将变换后的源图像作为新的源图像,转Stepl进行下一次迭代;否则,算法停止.在计算局部互信息时需要选择合适的窗口值.若窗口太小,互信息缺少足够的统计信息量,不能准确地匹配局部纹理;否则,计算量增加.设wj表示以j为中心的局部窗口值,wj=arg maxw min S w S w maxlNZi E W{j,w}(gi-g-)2(4)其中,g-=lNZi E W{j,w}gi;wmax和wmin分别是预定义的窗口值上界和下界;W{j,w}表示以j为中心、大小为w的局部图像区域,区域内共有N个像素,灰度值为gi.式(4)为每一个局部图像确定合适的窗口值,保证局部图像有足够的纹理信息.#"实验结果与分析我们分别进行单模态和多模态图像进行仿真实验,实验数据来自美国Vancerbiit大学RREP(retro-Spective regiStration evaiuation project)提供的国际通用脑部图像.在进行弹性配准之前,待配准图像已经3533期李谭等:用弹簧分子系统进行弹性医学图像配准采用RREP 提供的一组已配准的多模态数据作为标准图像,将本文算法配准结果和标准图像进行比较,衡量算法误差.选择某模态的标准图像!,对其施加弹性变换后,作为待配准的源图像 !.选择某模态的图像作为目标图像",将 !与"进行配准.如果!:",进行单模态配准;如果!和"异模态,进行多模态配准.将配准后的图像!'和!进行比较,二者的灰度差异越小,说明配准精度越高.配准误差定义为mse =Z f'E !',f E !(f'-f )2!.为了更清晰地表达灰度差异,本文中的差值图像都进行了适当的灰度调整.3.1 单模态配准从RREP 提供的三维人脑CT 数据中提取出二维切片作为标准图像,对其施加弹性变换,将变换前的数据作为目标图像,变换后的数据作为待配准的源图像,如图3所示,图像大小均为256>256.图3 单模态待配准图像图4所示为从脑组织中提取出的3组轮廓特征,将其作为形变模型的对应轮廓特征,分别采用文献[6]的算法和本文算法进行配准.图5,6所示分别为网格形变效果和配准结果,配准图像和标准图像的差值图像如图7所示.可以看出,文献[6]算法配准后的脑内部组织结构之间仍存在较大差异,本文算法利用提取出的轮廓特征限制网格形变,脑内的细节部分也得到了较好的配准.图5 单模态配准网格形变效果图6 配准结果图7 配准图像和标准图像的差值图像表l 所示为2种算法的性能比较.虽然本文采用的归一化互信息比文献[6]的归一化相关系数计算量大,但是算法迭代次数有明显减少.总体而言,本文算法速度较快而且配准误差更小,精度更高.表1 2种算法性能比较mse >l03NMI 迭代次数配准前5.6077l.3006-文献[6]算法l.9442l.36353本文算法l.l536l.3705l3.2 多模态配准我们分别从RREP 的CT 和MR 数据中提取出对应二维切片,将CT 作为标准图像.目标图像选择MR ,源图像是施加弹性形变后的CT 数据,如图8所453计算机辅助设计与图形学学报2007年图8 多模态待配准图像提取不同模态下脑组织图像的外轮廓作为形变模型的对应轮廓特征,如图9所示.用本文算法进行配准,网格形变效果和配准结果分别如图10,11所示.源图像配准前后和标准图像的差值图像如图12所示.可以看出,对于CT和MR,本文算法有效地对齐了整个脑部结构.配准误差和迭代次数如表2所示.图9 多模态配准对应轮廓特征图10 多模态配准网格形变效果图12 差值图像表2 多模态配准结果mse X103NMI迭代次数配准前 5.6077 1.1736-本文算法 2.4075 1.193734 结论弹簧分子系统是一种基于网格的弹性配准形变模型.本文对现有弹簧分子系统进行了改进,利用归一化互信息定义新的外部力公式,更好地匹配局部纹理;同时加入限制力的约束,控制源图像上的特定组织结构向目标图像上的对应结构特征进行形变.在二维人脑数据上进行仿真实验结果表明:与现有算法相比,本文算法能够更精确地控制弹性形变,配准精度更高、速度更快.本文算法不仅适用于单模态图像,对于多模态图像也能够有效地进行配准.参考文献[1]Kybic Jan,Unser Michaei.Fast parametric eiastic image registra-tion[J].IEEE Transactions on Image Processing,2003,12(11):1427-1442[2]Li Feng,Zhang Mingju,He Mingfeng,et al.A non-rigid medi-cai image registration approach based on hierarchicai mutuai in-formation and thin-piate spiine[J].Journai of Computer-AidedDesign&Computer Graphics,2005,17(7):1492~1496(inChinese)(冯林,张名举,贺明峰,等.用分层互信息和薄板样条实现医学图像弹性自动配准[J].计算机辅助设计与图形学学报,2005,17(7):1492~1496)[3]D'A gostino Emiiiano,Maes Frederik,Vandermeuien Dirk,et al.A viscous fiuid modei for muitimodai non-rigid image registrationusing mutuai information[J].Medicai Image Anaiysis,2003,7(4):565-575[4]Lef bure Martin,Cohen Laurent D.Image registration,opticai fiow and iocai rigidity[J].Journai of Mathematicai Imaging andVision,2001,14(2):131-1475533期李谭等:用弹簧分子系统进行弹性医学图像配准(康琦,申功.全场测速技术进展[J].力学进展,1997,27(1):106-121)[2]Gao Dianrong,Wang Yigun,Shen Gongxin.DPIV technigue and its appiication in fiow fieid measurement[J].Hydrauiics Pneu-matics&Seais,2001(5):30-33(in Chinese)(高殿荣,王益群,申功.DPIV技术及其在流场测量中的应用[J].液压气动与密封,2001(5):30-33)[3]Ohyama R,kumeta M,ueda A,!"#$.Mechanism of dieiectric fiuid transport by air-wave type eiectrohydrodynamic pumps[C]Proceedings of IEEE Internationai Conference on Dieiectric Lig-uids,Coimbra,2005:185-188[4]Parry J P,Shephard J D,Jones J D C,!"#$.Speckie contrast re-duction from a iarge core fibre deiivering O-switched puises[C]Proceedings of Conference on Lasers and Eiectro-Optics,Baiti-more,2005:516[5]Sun Heguan,kang Haigui.Processing of DPIV data[J].Jour-nai of Daiian university of Technoiogy,2000,40(3):364-367(in Chinese)(孙鹤泉,康海贵.DPIV流场测试技术中的数据处理[J].大连理工大学学报,2000,40(3):364-367)[6]Xia Yongguan,Liu Zhengdong,Yang Jingyu.Appiication of mo-ment invariant approach in region matching[J].Journai of Com-puter-Aided Design&Computer Graphics,2005,17(10):2152-2156(in Chinese)(夏永泉,刘正东,杨静宇.不变矩方法在区域匹配中的应用[J].计算机辅助设计与图形学学报,2005,17(10):2152-2156)[7]Rui Ting,Ding Jian,Wang Jinyan,!"#$.Fast image matching method based on SVD[J].Journai of Computer-Aided Design&Computer Graphics,2006,18(2):212-216(in Chinese)(芮挺,丁健,王金岩,等.SVD特征的快速景像匹配方法[J].计算机辅助设计与图形学学报,2006,18(2):212-216)[8]Wu Longhua,Yan Zhongmin,Tang Hongwu.Determination of the correiation window sizes in correiation anaiysis of DPIV[J].Advances in Water Science,2002,13(5):594-598(in Chi-nese)(吴龙华,严忠民,唐洪武.DPIV相关分析中相关窗口大小的确定[J].水科学进展,2002,13(5):594-598)[9]Adrian R J.Particie imaging technigues for experimentai fiuid mechanics[J].Annuai Review of Fiuid Mechanics,1991,23(1):261-304[10]Wiiiert C E,Gharib M.Digitai particie image veiocimetry[J].Experiments in Fiuids,1991,10(4):181-193[11]Wang Canxing,Lin Jianzhong,YamamotoFujio.An aigorithm for two-dimensionai PIV images[J].Journai of Hydrodynamics,2001,16(4):399-404(in Chinese)(王灿星,林建忠,山本富士夫.二维PIV图像处理算法[J].水动力学研究与进展,2001,16(4):399-404)[12]Gao Chao,Cao Ying,Guo Yongcai.Particie image veiocimetry technigues used in biood fiow fieid anaiysis[J].Opto-eiectronicEngineering,2004,31(8):37-40(in Chinese)(高潮,曹英,郭永彩.PIV血流场显示测速技术[J].光电工程,2004,31(8):37-40)(上接第!""页)[5]Matuszewski Bogdan J,Shen Jian-kun,Shark Lik-kwan.Eiastic image matching with embedded rigid structures using spring-masssystem[C]Proceedings of the Internationai Conference on ImageProcessing,Barceiona,Cataionia,2003,2:937-940[6]Shen Jian-kun,Matuszewski Bogdan J,Shark Lik-kwan.De-formabie image registration[C]Proceedings of the InternationaiConference on Image Processing,Genoa,2005,3:III-1112-1115[7]Piuim Josien P W,Maintz J B Antoine,Viergever Max A.Image registration by maximization of combined mutuai information andgradient information[J].IEEE Transactions on Medicai Ima-ging,2000,19(8):809-814[8]Shewchuk Jonathan Richard.Deiaunay refinement aigorithms for trianguiar mesh generation[J].Computationai Geometry:Theoryand Appiications,2002,22(1-3):21-24063计算机辅助设计与图形学学报2007年炘炘。
ieee transactions on medical imaging的审稿流程

ieee transactions on medical imaging的审稿流程摘要:1.IEEE Transactions on Medical Imaging 的概述2.IEEE Transactions on Medical Imaging 的审稿流程3.IEEE Transactions on Medical Imaging 的影响力和地位4.对未来审稿流程的展望正文:一、IEEE Transactions on Medical Imaging 的概述IEEE Transactions on Medical Imaging 是一份由IEEE(Institute of Electrical and Electronics Engineers,电气和电子工程师协会)出版的学术期刊,专注于医学影像领域的研究。
该期刊接收关于医学影像理论、技术、应用和相关领域的原创研究论文、综述文章以及技术报告。
二、IEEE Transactions on Medical Imaging 的审稿流程IEEE Transactions on Medical Imaging 的审稿流程分为以下几个步骤:1.作者提交论文:作者首先需要将论文提交到期刊的官方网站。
2.编辑部初审:编辑部会对提交的论文进行初步审查,检查论文是否符合期刊的发表要求,如格式、字数限制等。
3.送审:如果论文通过了初审,编辑部会将论文发送给合适的审稿人进行评审。
通常,期刊会邀请具有相关专业知识和经验的专家或者学者来担任审稿人。
4.审稿人评审:审稿人会对论文进行详细的审查,包括论文的研究方法、数据分析、结论等方面。
审稿人会在评审结束后向编辑部提交评审意见和建议。
5.编辑部决策:根据审稿人的评审意见和建议,编辑部会做出是否接受论文发表的决定。
如果论文被接受,编辑部会通知作者进行修改和完善;如果论文被拒绝,编辑部会向作者说明拒绝的原因。
6.作者修改和完善:如果论文被接受,作者需要根据审稿人的建议进行修改和完善,并提交最终稿给编辑部。
考研 英语二 大纲词汇

考研英语二大纲词汇abandon, ability, able, abolish, aboard, abrupt, absence, absolute, absorb, abstract, absurd, abuse, access, accompany, accumulate, accuracy, accurate, accuse, accustomed, achieve, acknowledge, acquire, adapt, add, address, adequate, adhere, adjacent, adjust, administration, admire, admit, adopt, advance, advantage, advise, advocate, afford, afraid, aggregate, aggressive, agree, agreeable, ahead, airline, alarm, album,alcohol, alike, allocate, alternative appliance, appreciate, approach, appropriate, approve, arbitrary, argue, arise, arrange, articulate, artificial, assess, assign, associate, assumption, assure, at, atmosphere, attach, attitude, auction.audible, audience, author, authority, automate, autonomy, available, average, avoid, aware, awkward, babble, baby, bachelor,back, backbone, bacon, badminton, bag, bake, balance, balcony, ball, balloon, ballot, banana, ban, banner, bargain, barrel, base, basement, basis,bat, batch, bathrobe, battery, bean, beard, bearer, bear, beat, bid, bicycle, bill, billion, bind, biology,blade, blanket, blast, blaze, bloc, block, blot, blur, board, bold, bolt, bomb, bonus, book, boom, borough, borrow, botany, bracelet, branch, brand, brave, breed,brief, brilliant, bulk, bundle, burden, bureaucracy, button, calendar, campaign, cancel, candidate, capability, capacity, captain, capsule, capture, carbohydrate, carbon, card, career, carrier, case, castle, catalog, catastrophe, category,cattle,cell, center, central, century, ceremony, challenge, chamber, chance, change, chaos, characteristic, character, charter,cheap, cheerful, chemist, chemistry, chest, chicken, chief, childhood, choice, choose, chronicle,circumstance,circular, circumstance, cite, citizen, civil, civilian,claim, clarify, class, classic, classification, classroom, claw, clay, clean, clearance, clear,clerk, clever,climate, cling, clinic, clinical, clockwise, closure,clue, cluster,coach,coal, coast, code, cocktail, coin, coincide,colleague,collect, collection, collective, collide, collision, column, combination, combine, come, comedian, comfort, comic, command, comment, commit, commodity, communicate, community, companion, compare, comparison, compartment, compatible, compete, competitive, compile, complaint, complete, complex, component, compose, comprehensive, compress, compute, computer, concentrate,concept, concern, conclude, conclusion, concrete, condition, conduct, conference, confess,confidence,confirm, conflict, conform, conscious, consensus, consequence, conservative, consider, considerable,consist, consistent,console, consolidate,constant,constitute, constitution, constrain,construct, construct, consult, consumer, contact, contain, container, contemporary, context, continue, contract,contradict, contrary, contrast, contribute, control, conventional,convey,convince, coordinate, cope, copy, core,corn,corner, corporate, correct, correlate, correspond, corridor,cost, cotton, council, counsellor, count, counter, country, couple, courage, course, court, cover,cow,crack, craft, crane, crazy, create, creation, creative, creature, credit, creep, crew, criminal, crisis, criticize, crop,cross, crowd,crucial,cruise,crush,crystal,cube,cultural,culture,cuis ine,currency, current, customary,custom, cycle,cylinder,damage, damn, dance, data, database, date, daughter, day, dead, deal, dear, debate, decade, deceive,decide,declare,decorate, decrease, dedicate, deep, defeat, defence, define, definite, definition, degree, delay,delicate, delight,deliver,demand, democracy, democratic, denote, depart, depend, depth, derive, describe, desert, deserve, design, desire, desk,despite,destroy,detail,detect, develop, device, devote, diagram, dialect, dialogue, diameter, dictate, diet, differ, difference, different, digital, dilemma, dimension, diminish, dinner, diploma, diplomacy, direct, direction, director, disable,disaster,disciple,discount,discourage,discourse,discover,discover,discretion,discriminate,discussion,disease,disguise,disguise,disgust,dish, dismiss, disorder, display, dispose, distant,distinct,distinguish,distribute, district, distribute, disturb, divide, divine, documentary, document, dominate, donate, door, double, doubt, draft, drag, dramatic, dramatic, dramatize,draw, dreadful, drink, drive, drop, drone, drought,drown,drug,drum,due,dull,dump,duplicate,duration,durable,dust,duty,dynamic,eager,earnest,earth,ease,east,easy,ecology,economy,edge, edit, education, educational, effective, efficiency, efficient, effort,elastic,elect,electric,electric,electronic,elegant,element, observe, obstacle, omit, once, online, open, operate, operation, opinion, oppose, opposition, opportunity, opposite, oppress, optic, option, or, orange, orbit, order, organization, originate, original, outcome, outside, outward, oven,overall,overheat,overlap,overlook,overseer,overwhelm,oxy gen,pace,package,packet,page,painting,palace,pale,panel,panic,pant,paper,parade,paragraph,parallel,parameter,parcel,parent,parish,park,parliament,part,partial,participate,particle,par ticular,passage,passenger,past, pastor, paste, patent, path, patient, pattern, pause, pave, payment, peak, peasant, peculiar,pedestrian,penalize,penalty,penguin,peninsula,perceive,percentage,perfect,perform,performance,permanent,permissio n,permit,persist,personal,personality,perspective,persuade,pe ssimist,petty,phase,phenomenon,philosophy,phonetic,phrase,phy sical,physician,piano,picture,piece,pillar,pillow,pilot,pin,p ioneer,pipe,pitch,pixel,pizza,place,plan,planet,plant,plastic,platform,plead,pleasure,plot,plus,pocket ,poem,poet,poison,polar,pole,police,policy,political,polygon, pool,popular,population,porcelain,portable,portion, portrait, pose,positive,possess,possibility,possible,post,potato,potent ial,pound,pour,powder,power,powerful,practical, practice, praise, predict, prefer, preference, prefix, pregnancy, pregnant,premise,prepare,presence,present,presentation,presid ent,press,pressure,pretend,pretty,preview,previous,price,prim ary,prime,principle,print,priority,prison,privacy,private,pri ze,probably,problem,procedure,process,produce,profession,prof essional,professor,profile,profit,program,progress,project,pr omote,prompt,proof,property,prophet,proportion,proposal,propo se,prosperous,protect,protection,protest,provide,provision,ps ychology,public,publish,pudding,pulley,punch,purchase,pure,pu rify,purpose,pursue,push,put,qualify,qualitative,quantity,qua ntify,quarter,queen,question,quick,quite,quote,race,radical,r adius,rain,rainbow,random,range,rank,rant,rapid,rare,rate,rat her,rational,ray,razor,react,reaction,read, reader, readable, ready, real, reality, reasonable, recall, receive, recent, recent, recipe,recognize,recommend, recover,recreation,recruit,reduce,reduction,reflect,reflection,reform ,refugee,refuseregard,region,register,regular,regulation,rein force,reject,relative,relax,release,relevant,relief,rely,rema in,remark,remember,remind,remove,render,renew,rent,repair,rep eat,replace,report,republic,repress,reproduce,request,require ,rescue,research,reserve,residence,resident,resist,resolution,resolve,respect,respond,restrict,result,retain,retire,retrea t,reveal,revenge,reverse,review,revise,revolution,rich,ridicu lous,right,rigid,ring,riot,robot,role,roll,rotate,rough,round,route,routine,row,royal,rub,ruin ,rule,rulerrural, rush,rust,safety,sail,sale,salmon,salon,sample,sanction,satellite, satisfy,savage,scale,scandal,scarce,scare,scene,scheme,schola r,scholarship,science,scientific,scope,score,。
- 1、下载文档前请自行甄别文档内容的完整性,平台不提供额外的编辑、内容补充、找答案等附加服务。
- 2、"仅部分预览"的文档,不可在线预览部分如存在完整性等问题,可反馈申请退款(可完整预览的文档不适用该条件!)。
- 3、如文档侵犯您的权益,请联系客服反馈,我们会尽快为您处理(人工客服工作时间:9:00-18:30)。
Elastic Medical Image Registration Based on Image Intensity Xiu Ying Wang1,3, David Dagan Feng1,2, Jesse Jin11 Biomedical and Multimedia Information Technology (BMIT) GroupSchool of Information Technologies, F09The University of Sydney, NSW 2006, Australia2Center for Multimedia Signal ProcessingDepartment of Electronic and Information EngineeringHong Kong Polytechnic University3 School of Computer ScienceHeilongjiang University, Harbin 150080, P.R.Chinaxying_wang@.auAbstractA two-step elastic medical image registration approach is proposed, which is based on the image intensity. In the first step, the global affine medical image registration is used to establish one-to-one mapping between the two images to be registered. After this first step, the images are registered up to small local elastic deformation. Then the mapped images are used as inputs in the second step, during which, the study image is modeled as elastic sheet by being divided into several subimages. Moving the individual subimage in the reference image, the local displacement vectors are found and the global elastic transformation is achieved by assimilating all of the local transformation into a continuous transformation. This algorithm has been tested on both simulated and tomographic images. Keywords: image registration, medical imaging, elastic registration, affine transformation1 IntroductionMedical imaging modalities can be divided into two major categories: anatomical and functional modalities. Anatomical modalities, depicting primarily morphology, include X-ray, CT, MRI, US etc. Functional modalities, depicting primarily information on the metabolism of the underlying anatomy, include SPECT, PET, which together make up the nuclear medicine imaging modalities. Since information gained from two images acquired in the clinical track of events is usually of a complementary nature, proper integration of useful data obtained from the separate images is often desired. The process of registration is a fundamental task in image processing which seeks to match two data sets, which may differ in such things as time of acquisition, image properties, or viewpoint [1][2][13]. Registration of both intra-subject and inter-subject images has been the subject of extensive study in medical imaging literatures. It is important for several reasons. Firstly, images obtained from different modalities can be superposed only after accurate registration. Visualization and Copyright © 2002, Australian Computer Society, Inc. This paper appeared at the Pan-Sydney Area Workshop on Visual Information Processing (VIP2001), Sydney, Australia. Conferences in Research and Practice in Information Technology, Vol. 11. David Dagan Feng, Jesse Jin, Peter Eades, Hong Yan, Eds. Reproduction for academic, not-for profit purposes permitted provided this text is included.quantitative analysis can be improved by such superposition. Secondly, atlas images accurately registered to patient data can be used for automatic identification of brain anatomy and for lesion location. Thirdly, image registration can assist in monitoring treatment by, for example, allowing accurate comparison of brain tumor size and location during treatment over time. Finally, it is hypothesized that proper statistical study of the morphology of both normal and diseased brains can be conducted only with good registration across diverse population.Registration is the determination of a one-to-one transformation between two image spaces which maps each point of an image onto corresponding points of another image. Generally, the transformations can be divided into rigid and non-rigid transformations. The rigid methods can be used to cope with rotation and translation differences between the registered images. Specific problems arise due to differences in patient posture for different studies, possible differences in organ shape and volume (e.g., stomach and bladder content), so elastic or non-rigid methods are required to cope with local differences between the images. The elastic transformations are based on models from elasticity theory and describe local deformations. The central idea behind elastic registration is to consider images as continuous bodies and to model the geometric differences between images such that they have been caused by elastic deformation [9]. Principally, registration approaches can be distinguished into landmark-based and intensity-based schemes. Landmark-based schemes first extract landmarks (e.g., points, curves, surfaces) from images and then compute a transformation based on these features. With intensity-based schemes, the image intensities are directly exploited to compute the transformation.The approach we propose in this paper has two main advantages over existing registration methods: firstly, it can cope with rigid deformation and local elastic deformation as well; secondly, on the basis of image intensities, the approach requires no human intervention.2 Registration MethodIntensity-based approaches for elastic registration of medical images directly exploit the image intensities. The main advantage of these schemes is that an explicit segmentation of the images is not required. However, for global elastic transformation, the number of parameters to be optimized is generally too large to be feasible in practice. In this paper, we present the two-step registration approach based on raw image intensities.2.1 First Step: Global Affine RegistrationA global affine registration is used to initialize the robust voxel-to-voxel matching algorithm. The affine registration, even if not extremely accurate, is a good initial guess of the final registration.2.1.1 MethodWe use the rapid algorithm developed by Woods[4],[5] which calculates the ratio of one image to another at each voxel in the brain and then aligns the two images such that the variance of this ratio across all voxels is minimized. We register the two images using affine transformation which have nine degrees of freedom (three rotations, three translation and three scaling degrees of freedom) instead of rigid transformation which only has six degrees of freedom.2.1.2 OptimizationThis affine registration step is carried out using Powell’s optimization method [6]. We assume that on the basis of such a global affine registration, the images are registered up to small local elastic deformation.2.2 Second Step: Elastic RegistrationIn this step, the matched images are used as inputs and the study image is modeled as elastic sheet by being divided into equal size windows which number is less than the number of voxels. A local transformation is found in each individual window and the global elastic transformation is achieved by assimilating all of the local transformation into continuous transformation.2.2.1 MethodThe elastic registration is performed by the following procedures:a. Finding the best position for individual study subimageThe study image is modeled as elastic sheet by being divided into some number (less than the number of voxels) of square windows. Optimizing the local similarity with the subimage of the reference image by moving the individual window on the reference image within a searching rang iterativelyb. The determination of local displacement field Displacement vectors for each voxel in the individual subimage are determined by the position differences between the original and the new subimage voxels. LettiV be the voxel position of subimage (window) i at iteration t. We use the transformation (in this paper, we limit it to translation) t T to transform t i V into1+t i V.)(1tittiVTV=+ (1) The local displacement field in the window i is:})({}{1titittitiiVVTVVD−=−=+ (2)c. Global Elastic TransformationThe local displacement fields found are translated into global elastic transformation by cubic interpolation. The elastic registration is achieved by applying the global elastic transformation to the study image.2.2.2 CriterionAny automatic registration method requires an objective criterion whose role is to measure the similarity of the study image with respect to reference image. SupposethatswiI(x,y,z) andRwiI(x,y,z) are the ith sumimages of study image and its corresponding same size neighbourhood in the reference image respectively. Taking a warping function g, we can get a warped version of the subimage of the study image.)),,((),,(zyxgIzyxIswiwswi= (3)We want to find such a warping function g so that the warped subimages of study image are as close as possible to the corresponding subimages of the reference image, in the sense of sum squared distance (SSD):()∑∑∈−==IzyxRwiwswizyxIzyxIeE),,(2 2),,(),,((4)3 Experimental ResultsIn this section, some experimental results achieved by our two-step elastic approach will be presented. We carry out experiments using 2-D images3.1 Registration of phantom imagesThe experiments are carried out by the following procedures:(1) Designate a phantom imagerI as the reference image;(2) Create the study imagesI bya. Firstly, elastically deformingrI usingpolynomial warping;b. Secondly, rotating the image by θ=5;c. Finally, translating the image by (dx,dy)=(5,5)(3) Register the images using our algorithm.Fig. 1a Fig.1bFig.1c Fig.1dFig.1e Fig.1fFig.1g Fig.1hFigure 1. Experiment of elastic registration ofphantom imagesFig.1a and Fig.1b are the reference and study images respectively; Fig.1c is the difference between the two images; Fig.1d is the result of the first-step registration; Fig.1e is the study image after the affine transformation; Fig.1f is global displacement field; Fig.1g is the result of second-step registration with the registration deformation grid overlaid; Fig.1h is the difference between the two images after elastic registration3.2 Experiments using MR image andartificial deformed MR imageIn this series of experiments we adapt the experimental procedure slightly in the sense thatrI is now a MR image. The rest of the procedure as described in the previous experiment remains unaltered. We register the two images using windows of 4*4 pixels.Fig.2a Fig.2bFig.2c Fig.2dFig.2e Fig.2fFig.2g Fig.2hFigure 2. Experiment of elastic registration of MRimagesFig.2a and Fig.2b are the reference and study images respectively; Fig.2c is the difference between the two images; Fig.2d is the result of the first-step registration; Fig.2e is the study image after the affine transformation; Fig.2f is global displacement field; Fig.2g is the result of second-step registration with the registration deformation grid overlaid; Fig.2h is the difference between the two images after elastic registration4 Summary and Further workWe have described an elastic medical image registration algorithm where the images are firstly registered using global affine transformation and then through the second step, the local elastic deformations are matched.We have implemented the algorithm and we have presented several experiment results involving synthetic images and medical images as well. The experimental results show that our algorithm has good performance in coping both rigid and local elastic deformations.An attractive feature of this registration approach is that it can register the medical images with high performance. The other attractive feature is that it is an automatic algorithm, using the raw intensity as feature space, the algorithm need no human intervention and can perform registration automatically.Further research will address the development of a more efficient elastic registration model which can register the more deformable organs images of liver, stomach and bladder.5 AcknowledgementThis work was partially supported by CSC (Chinese Scholarship Council) , UGC and ARC.6 References[1] VAN DEN ELSEN, P.A., POL, E.J.D. and VIERGEVER, M.A.(1993): Medical Image matching a Review with classification. IEEE Eng.Med.Biol., vol.12,pp.26-39,1993[2] MAINTZ, J.B.A. and VIERGEVER, M.A.(1998): A survey of Medical Image Registration. Medical Image Analysis, vol. 2, no.1, pp.1-36,1998[3] PELIZZARI, C.A., CHEN, G.T.Y.,et.al.(1989): Accurate three-dimensional registration of CT, PET and/or MR images of brain. puter Assist.Tomogr., vol.13,pp.20-26,1989[4] WOODS, R.P., MAZZIOTTA, J.C. and ChERRY, S.R.(1993): MRI-PET registration with automated algorithm. p. Assist. Tomogr., vol.19, no.4, pp.536-546, 1993[5] WOODS, R.P., GRAFTON S.T., CHERRY, S.R. and MAZZIOTTA, J.C.(1998): Automated image registration: I.General methods and intrasubject, intramodality validation. p. Assist. Tomogr., vol.22, no.1, pp.139-152, 1998[6] POWELL, M.J.D.(1964): An efficient method for finding the minimum of a function of several variables without calculating derivatives. Comput.J.,vol.7, pp.155-163, 1964[7] ALPERT, N. M., BRADSHAW, J. F., KENNEDY, D. and. CORREIA, J.A.(1990): The principal axes transformation - A method for image registration. J. Nucl. Med., 31:1717-1722.[8] ARDEKANI, B. A., BRAUN, M., HUTTON, B. F., KANNO, I. and IIDA H.,(1995): A fully automatic multimodality image registration algorithm. J. Comput. Assist. Tomogr., 19:615-623.[9] ROHR,K.,(2000): Elastic Registration of Multimodal medical images: A Survey. Auszug aus: Kunstliche Intelligenz, Heft3/2000:11-17.[10] COLLIGNON, A., MAES, F., DELAERE, D., VANDERMEULEN, D., SUETENS, P.and MARCHAL, G., (1995): Automated multimodality image registration using information theory. In Bizais, Y. and Barillot, C. (eds), Information Processing in Medical Imaging, pp. 263-274, Kluwer Academic Pulishers, Dordrecht.[11] FITZPATRICK, J. M., WEST, J. B. and MAURER,C. R. (1998): Predicting error in rigid-body point-based registration. IEEE Trans. Med. Imag., 17:694-702. [12] LIN, K. P., Huang, S. C. et al. (1994): A general technique for interstudy registration of multifunction and multimodality images. IEEE Trans. Nucl. Sci., 41:2850-2855.[13] MAURER, C. R. and FITZPATRICK J. M. (1993):A review of medical image registration. In Maciunas, R. J. (ed.), Interactive Imageguided Neurosurgery, pp. 17-44, American Association of Neurological Surgeons, Parkridge, IL.[14] KYBIC, J., THEVENAZ, P., NIRKKO, A. and UNSER, M.(2000): Unwarping of unindirectionally distorted EPI images. IEEE Transactions on Medical Imaging, vol.19,pp.80-93,Feb.2000.[15] ROUET, J.M., JACQ, J.J and ROUX,C.(2000):Genetic Algorithms for a Robust 3-D MR-CT Registration. IEEE Trans. Inform. Technol. Biomed., vol.21,pp:126-136, June.2000.[16] WANG, H. S., FENG, D., YEH, E. and HUANG, S.C.,(2001): Objective assessment of image registration results using statistical confidence intervals. IEEE Transactions on Nuclear Science, 48:106-110, 2001.。