文献翻译-PCNN模型及其应用
PCNN时、频域特性研究及其在图像分割中的应用
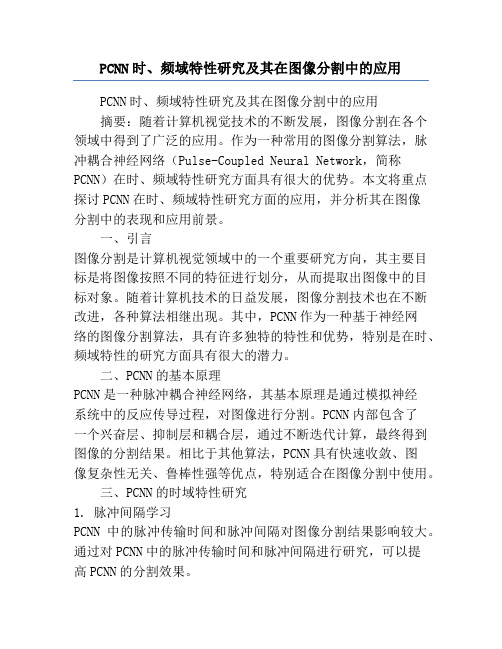
PCNN时、频域特性研究及其在图像分割中的应用PCNN时、频域特性研究及其在图像分割中的应用摘要:随着计算机视觉技术的不断发展,图像分割在各个领域中得到了广泛的应用。
作为一种常用的图像分割算法,脉冲耦合神经网络(Pulse-Coupled Neural Network,简称PCNN)在时、频域特性研究方面具有很大的优势。
本文将重点探讨PCNN在时、频域特性研究方面的应用,并分析其在图像分割中的表现和应用前景。
一、引言图像分割是计算机视觉领域中的一个重要研究方向,其主要目标是将图像按照不同的特征进行划分,从而提取出图像中的目标对象。
随着计算机技术的日益发展,图像分割技术也在不断改进,各种算法相继出现。
其中,PCNN作为一种基于神经网络的图像分割算法,具有许多独特的特性和优势,特别是在时、频域特性的研究方面具有很大的潜力。
二、PCNN的基本原理PCNN是一种脉冲耦合神经网络,其基本原理是通过模拟神经系统中的反应传导过程,对图像进行分割。
PCNN内部包含了一个兴奋层、抑制层和耦合层,通过不断迭代计算,最终得到图像的分割结果。
相比于其他算法,PCNN具有快速收敛、图像复杂性无关、鲁棒性强等优点,特别适合在图像分割中使用。
三、PCNN的时域特性研究1. 脉冲间隔学习PCNN中的脉冲传输时间和脉冲间隔对图像分割结果影响较大。
通过对PCNN中的脉冲传输时间和脉冲间隔进行研究,可以提高PCNN的分割效果。
2. 相位响应研究PCNN在不同的输入相位下,对图像的分割效果也会有所不同。
通过研究PCNN的相位响应特性,可以优化PCNN算法,提高图像分割的准确性和稳定性。
4. 考虑多种时域特性PCNN不仅可以根据图像的相位来进行分割,还可以利用图像的幅度信息、脉冲传输时间等时域特性。
研究多种时域特性对PCNN算法的影响,可以得到更全面的图像分割结果。
四、PCNN的频域特性研究1. 频率响应研究PCNN在不同的输入频率下,对图像的分割效果也会发生变化。
图像分割中PCNN的应用研究
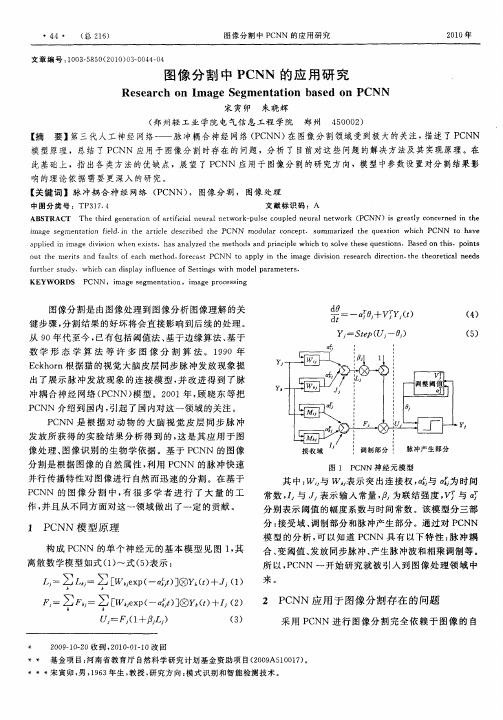
图像分 割是 由图像处理 到图像分 析 图像 理解 的关
键步 骤 , 分割 结果 的好坏将会 直接影 响到后续 的处理 。 从 9 O年代至今 , 已有 包括 阈值法 、 于边 缘算法 、 基 基于 数 学 形 态 学 算 法 等 许 多 图 像 分 割 算 法 。1 9 9 0年 E k on根据猫 的视觉 大脑皮 层同步 脉冲 发放现象 提 ch r
一
T
l "
z )
() 4 () 5
Y =S e 一 ) s tp(
I
卜
1
『
出了展示 脉 冲发放 现象 的连 接模 型 . 改 进得 到 了脉 并 冲耦 合神 经 网络 ( C P NN) 型 。2 0 模 0 1年 , 晓东 等把 顾 P NN 介绍 到 国内, C 引起 了国内对 这 一领域 的关 注 。
此基 础上 ,指 出各类 方法的优缺 点,展望 了P N 应用于 图像 分 割的研 究方 向,模 型 中参数设置对分 割结果影 C N
响 的理论 依据 需要更深入 的研 究。 【 关键词】脉 冲耦合神 经 网络 ( C P NN) .图像 分割 ,图像 处理
中图 分 类 号 :T 3 7 4 P 1 . 文 献标 识 码 :A
图像 分割 中 P NN 的 应 用研 究 C
文 苹 编号 :0 355 (oO 0- 040 1 0 — 8 0 2 I ) 30 4 —4
2 1 矩 00
图 像 分 割 中 P NN 的 应 用 研 究 C
Re e r h o m a e S g e t to a e n PCNN sa c n I g e m n ain b s d o 宋 寅卯 朱晓辉 ( 郑州轻工 业学院 电气信息 工程 学院 郑州 10 0 ) 5 0 2 【 摘 要】第三 代人工神经 网络—— 脉 冲耦合 神经 网络 ( C P NN) 图像分 割领域 受到极 大的关注 , 在 描述 了P NN C 模 型原 理 ,总结 了 P NN 应用于 图像 分 割时存在 的 问题 ,分 析 了 目前对这 些问题 的解决方法及其 实现 原理 。在 C
医学图像分割处理中改进型PCNN模型的应用综述
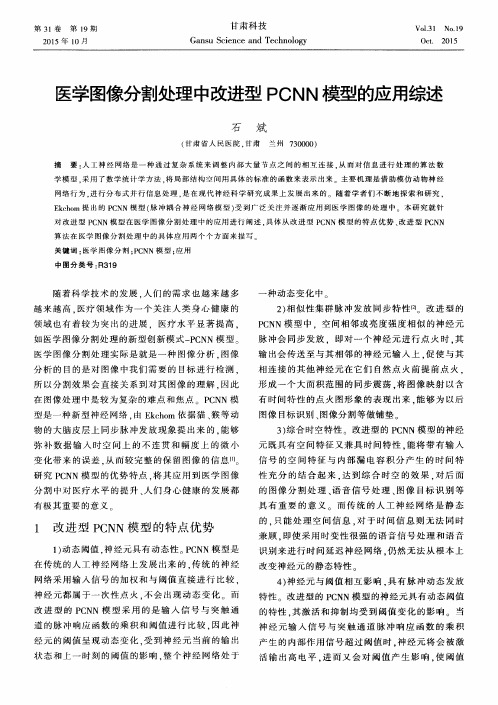
型 是 一种 新 型神 经 网络 , 由E k c h o m依 据 猫 、 猴 等 动 物 的 大脑 皮 层 上 同步 脉 冲发 放 现 象提 出来 的 . 能够
弥 补 数 据 输 入 时 空 间 上 的 不 连 贯 和 幅 度 上 的微 小
石 斌
( 甘肃省人 民医院, 甘肃 兰 州 7 3 0 0 0 0 )
摘 要 : 人 工 神 经 网络 是 一 种 通 过 复 杂 系 统 来 调 整 内 部 大 量 节 点 之 间 的 相 互 连接 , 从 而 对 信 息 进 行 处 理 的 算 法 数 学模型 , 采 用 了 数学 统计 学方 法 , 将 局 部 结 构 空 间 用 具 体 的 标 准 的 函 数 来 表 示 出来 。主 要 机 理 是 借 助模 仿 动 物神 经 网络 行 为 , 进 行 分 布 式并 行信 息 处 理 , 是 在 现 代 神 经 科 学 研 究 成 果 上 发 展 出 来 的 。随 着 学 者 们 不 断地 探 索 和 研 究 ,
改 变神 经元 的静态 特性
1 改进型 P C NN模 型 的特 点 优 势
1 ) 动 态 阈值 . 神经元 具 有动 态性 。 P C N N模 型是
在 传 统 的人 工 神 经 网络 上 发 展 出来 的 . 传 统 的神 经 网络 采 用输 入 信 号 的加 权 和 与 阈值 直 接 进行 比较 . 神 经 元 都 属 于一 次性 点 火 , 不 会 出现动 态 变 化 。而 改 进型的 P C N N 模 型 采 用 的是 输 入 信 号 与 突 触 通
E k c o m提 出 的 P CNN模 型 ( 脉 冲耦 合 神 经 网 络 模 型 ) 受 到 广 泛 关 注 并 逐 渐 应 用 到 医 学 图 像 的 处 理 中 。本 研 究 就 针
自然语言处理中常见的语言模型及其应用(Ⅰ)

自然语言处理中常见的语言模型及其应用引言自然语言处理(NLP)是计算机科学与人工智能领域中的一个重要研究方向,它涉及计算机对人类语言进行理解、生成和处理。
在NLP领域中,语言模型扮演着至关重要的角色,它们被用于诸如自动翻译、语音识别、问答系统等多个应用中。
本文将介绍自然语言处理中常见的语言模型及其应用。
统计语言模型统计语言模型是NLP领域中最早也是最常见的语言模型之一。
它基于概率统计原理,通过分析文本中的词语出现概率来推断句子的结构和语义。
统计语言模型主要包括n-gram模型和神经网络语言模型两种类型。
n-gram模型是一种基于马尔科夫假设的语言模型,它假设一个词语出现的概率只与其前面的n-1个词语相关。
n-gram模型在机器翻译、语音识别和文本生成等方面有着广泛的应用。
例如,在机器翻译中,n-gram模型可以通过分析源语言和目标语言之间的词语搭配,从而提高翻译准确度。
神经网络语言模型是近年来兴起的一种语言模型,它通过神经网络来学习文本中词语之间的语义关系。
神经网络语言模型在语言模型的建模能力和泛化能力方面都有显著的提升,因此在自然语言处理中得到了广泛的应用。
例如,在自动问答系统中,神经网络语言模型可以通过学习大规模的语料库数据,从而更准确地回答用户提出的问题。
词向量模型词向量模型是自然语言处理中另一种常见的语言模型,它通过将词语映射到一个高维的实数向量空间中,从而能够表示词语之间的语义关系。
词向量模型主要包括基于计数的词向量模型和基于预测的词向量模型两种类型。
基于计数的词向量模型是一种经典的词向量表示方法,它通过统计词语在文本中的共现频次来构建词向量空间。
基于计数的词向量模型在文本分类、信息检索和情感分析等任务中有着广泛的应用。
例如,在情感分析中,基于计数的词向量模型可以通过分析文本中词语的频次来判断文本的情感倾向。
基于预测的词向量模型是近年来兴起的一种词向量表示方法,它通过预测上下文词语来学习词向量表示。
PCNN模型的改进及其在医学图像处理中的应用
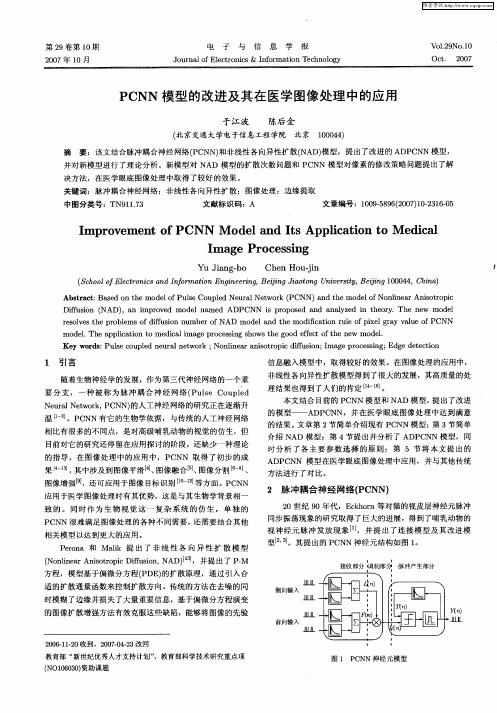
并对新模型进行 了理论分析 。新模型对 NAD模 型的扩散次数 问题和 P NN 模型对像素 的修改策略 问题提 出了解 C 决方法 ,在医学眼底 图像处理 中取得 了较好 的效果 。 关键词:脉冲耦合神经网络 ;非线性各 向异性扩散 ;图像 处理 ;边缘提取
中图分类号:T 9 1 3 N 1. 7
ቤተ መጻሕፍቲ ባይዱ
1 引言
随着生物神经学 的发展 ,作为第三代 神经网络 的一个重 要 分支 ,一种 被称 为 脉冲 耦 合神 经 网络 ( le o p e Pus C u ld Ne rl t ok PC ua w r , NN) Ne 的人工神经 网络 的研 究正在逐渐升 温 [3。P NN有它 的生物 学依据 ,与传统 的人 工神经网络 1】 C - 相 比有很多的不 同点 ,是对高级哺乳动物的视觉的仿生 ,但 目前对它 的研究还停 留在应用探讨 的阶段 ,还缺 少一种理论 的指 导。在图像处 理中的应用 中,P CNN 取得 了初步 的成
Y in — o uJa gb Ch nHo - n e uj i
基于PCNN模型的图像分割技术研究
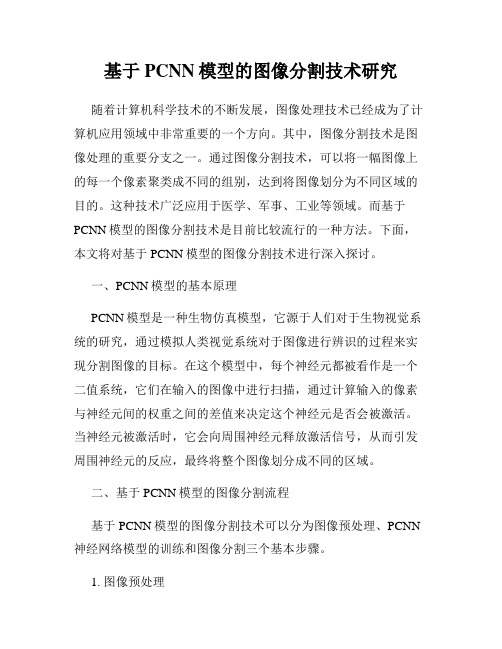
基于PCNN模型的图像分割技术研究随着计算机科学技术的不断发展,图像处理技术已经成为了计算机应用领域中非常重要的一个方向。
其中,图像分割技术是图像处理的重要分支之一。
通过图像分割技术,可以将一幅图像上的每一个像素聚类成不同的组别,达到将图像划分为不同区域的目的。
这种技术广泛应用于医学、军事、工业等领域。
而基于PCNN模型的图像分割技术是目前比较流行的一种方法。
下面,本文将对基于PCNN模型的图像分割技术进行深入探讨。
一、PCNN模型的基本原理PCNN模型是一种生物仿真模型,它源于人们对于生物视觉系统的研究,通过模拟人类视觉系统对于图像进行辨识的过程来实现分割图像的目标。
在这个模型中,每个神经元都被看作是一个二值系统,它们在输入的图像中进行扫描,通过计算输入的像素与神经元间的权重之间的差值来决定这个神经元是否会被激活。
当神经元被激活时,它会向周围神经元释放激活信号,从而引发周围神经元的反应,最终将整个图像划分成不同的区域。
二、基于PCNN模型的图像分割流程基于PCNN模型的图像分割技术可以分为图像预处理、PCNN 神经网络模型的训练和图像分割三个基本步骤。
1. 图像预处理图像预处理是对输入图像进行预先处理以获得更好的分割结果。
在这个步骤中,图像预处理的主要任务是去噪、对比度增强和边缘检测。
常用的图像预处理方法包括高斯滤波、图像增强和边缘检测等。
2. PCNN神经网络模型的训练在实际应用中,人们往往需要训练一个可靠的神经网络模型来实现图像分割。
PCNN神经网络模型的训练需要依赖于现有的图像样本来进行,其中的参数需要在训练过程中进行调整以保证最终的分割效果。
3. 图像分割在得到训练完成的PCNN神经网络模型后,就可以利用这个模型对待分割的图像进行分析和处理了。
在这个步骤中,每个神经元对输入图像进行扫描和处理,通过激活周围神经元来实现对图像的分割。
最终,可以得到图像被分割成不同区域的结果。
三、基于PCNN模型的图像分割技术的特点基于PCNN模型的图像分割技术具备以下几个特点:1. 对于图像中的复杂区域,该方法具备很高的分割精度。
改进遍历过程的PCNN在图像处理中的应用
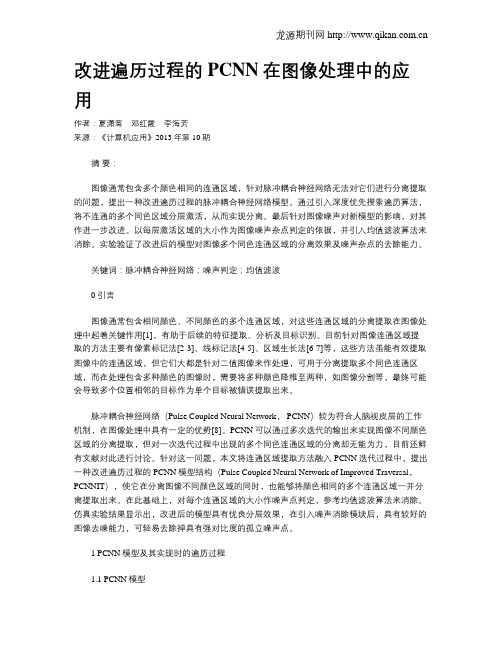
改进遍历过程的PCNN在图像处理中的应用作者:夏潇鸾邓红霞李海芳来源:《计算机应用》2013年第10期摘要:图像通常包含多个颜色相同的连通区域,针对脉冲耦合神经网络无法对它们进行分离提取的问题,提出一种改进遍历过程的脉冲耦合神经网络模型。
通过引入深度优先搜索遍历算法,将不连通的多个同色区域分层激活,从而实现分离。
最后针对图像噪声对新模型的影响,对其作进一步改进。
以每层激活区域的大小作为图像噪声杂点判定的依据,并引入均值滤波算法来消除。
实验验证了改进后的模型对图像多个同色连通区域的分离效果及噪声杂点的去除能力。
关键词:脉冲耦合神经网络;噪声判定;均值滤波0 引言图像通常包含相同颜色、不同颜色的多个连通区域,对这些连通区域的分离提取在图像处理中起着关键作用[1],有助于后续的特征提取、分析及目标识别。
目前针对图像连通区域提取的方法主要有像素标记法[2-3]、线标记法[4-5]、区域生长法[6-7]等,这些方法虽能有效提取图像中的连通区域,但它们大都是针对二值图像来作处理,可用于分离提取多个同色连通区域,而在处理包含多种颜色的图像时,需要将多种颜色降维至两种,如图像分割等,最终可能会导致多个位置相邻的目标作为单个目标被错误提取出来。
脉冲耦合神经网络(Pulse Coupled Neural Network, PCNN)较为符合人脑视皮层的工作机制,在图像处理中具有一定的优势[8]。
PCNN可以通过多次迭代的输出来实现图像不同颜色区域的分离提取,但对一次迭代过程中出现的多个同色连通区域的分离却无能为力,目前还鲜有文献对此进行讨论。
针对这一问题,本文将连通区域提取方法融入PCNN迭代过程中,提出一种改进遍历过程的PCNN模型结构(Pulse Coupled Neural Network of Improved Traversal,PCNNIT),使它在分离图像不同颜色区域的同时,也能够将颜色相同的多个连通区域一并分离提取出来。
中英文双语外文文献翻译:一种基于ARIMA模型、BPNN模型对消费物价指数(CPI)进行预测的新型分治模型
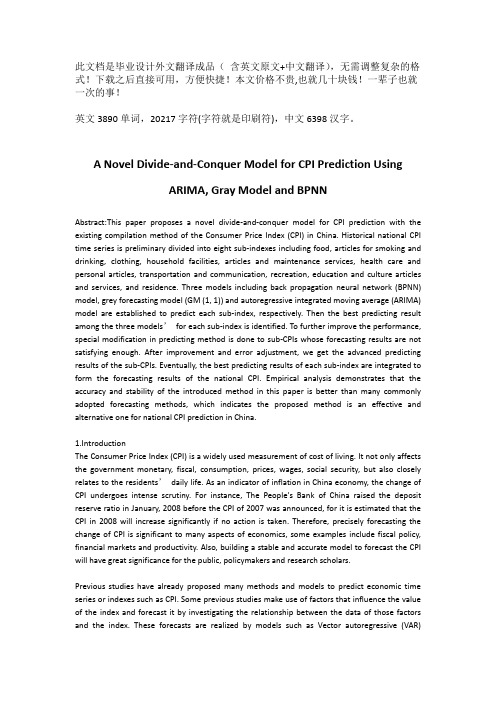
此文档是毕业设计外文翻译成品(含英文原文+中文翻译),无需调整复杂的格式!下载之后直接可用,方便快捷!本文价格不贵,也就几十块钱!一辈子也就一次的事!英文3890单词,20217字符(字符就是印刷符),中文6398汉字。
A Novel Divide-and-Conquer Model for CPI Prediction UsingARIMA, Gray Model and BPNNAbstract:This paper proposes a novel divide-and-conquer model for CPI prediction with the existing compilation method of the Consumer Price Index (CPI) in China. Historical national CPI time series is preliminary divided into eight sub-indexes including food, articles for smoking and drinking, clothing, household facilities, articles and maintenance services, health care and personal articles, transportation and communication, recreation, education and culture articles and services, and residence. Three models including back propagation neural network (BPNN) model, grey forecasting model (GM (1, 1)) and autoregressive integrated moving average (ARIMA) model are established to predict each sub-index, respectively. Then the best predicting result among the three models’for each sub-index is identified. To further improve the performance, special modification in predicting method is done to sub-CPIs whose forecasting results are not satisfying enough. After improvement and error adjustment, we get the advanced predicting results of the sub-CPIs. Eventually, the best predicting results of each sub-index are integrated to form the forecasting results of the national CPI. Empirical analysis demonstrates that the accuracy and stability of the introduced method in this paper is better than many commonly adopted forecasting methods, which indicates the proposed method is an effective and alternative one for national CPI prediction in China.1.IntroductionThe Consumer Price Index (CPI) is a widely used measurement of cost of living. It not only affects the government monetary, fiscal, consumption, prices, wages, social security, but also closely relates to the residents’daily life. As an indicator of inflation in China economy, the change of CPI undergoes intense scrutiny. For instance, The People's Bank of China raised the deposit reserve ratio in January, 2008 before the CPI of 2007 was announced, for it is estimated that the CPI in 2008 will increase significantly if no action is taken. Therefore, precisely forecasting the change of CPI is significant to many aspects of economics, some examples include fiscal policy, financial markets and productivity. Also, building a stable and accurate model to forecast the CPI will have great significance for the public, policymakers and research scholars.Previous studies have already proposed many methods and models to predict economic time series or indexes such as CPI. Some previous studies make use of factors that influence the value of the index and forecast it by investigating the relationship between the data of those factors and the index. These forecasts are realized by models such as Vector autoregressive (VAR)model1 and genetic algorithms-support vector machine (GA-SVM) 2.However, these factor-based methods, although effective to some extent, simply rely on the correlation between the value of the index and limited number of exogenous variables (factors) and basically ignore the inherent rules of the variation of the time series. As a time series itself contains significant amount of information3, often more than a limited number of factors can do, time series-based models are often more effective in the field of prediction than factor-based models.Various time series models have been proposed to find the inherent rules of the variation in the series. Many researchers have applied different time series models to forecasting the CPI and other time series data. For example, the ARIMA model once served as a practical method in predicting the CPI4. It was also applied to predict submicron particle concentrations frommeteorological factors at a busy roadside in Hangzhou, China5. What’s more, the ARIMA model was adopted to analyse the trend of pre-monsoon rainfall data forwestern India6. Besides the ARIMA model, other models such as the neural network, gray model are also widely used in the field of prediction. Hwang used the neural-network to forecast time series corresponding to ARMA (p, q) structures and found that the BPNNs generally perform well and consistently when a particular noise level is considered during the network training7. Aiken also used a neural network to predict the level of CPI and reached a high degree of accuracy8. Apart from the neural network models, a seasonal discrete grey forecasting model for fashion retailing was proposed and was found practical for fashion retail sales forecasting with short historical data and better than other state-of-art forecastingtechniques9. Similarly, a discrete Grey Correlation Model was also used in CPI prediction10. Also, Ma et al. used gray model optimized by particle swarm optimization algorithm to forecast iron ore import and consumption of China11. Furthermore, to deal with the nonlinear condition, a modified Radial Basis Function (RBF) was proposed by researchers.In this paper, we propose a new method called “divide-and-conquer model”for the prediction of the CPI.We divide the total CPI into eight categories according to the CPI construction and then forecast the eight sub-CPIs using the GM (1, 1) model, the ARIMA model and the BPNN. To further improve the performance, we again make prediction of the sub-CPIs whose forecasting results are not satisfying enough by adopting new forecasting methods. After improvement and error adjustment, we get the advanced predicting results of the sub-CPIs. Finally we get the total CPI prediction by integrating the best forecasting results of each sub-CPI.The rest of this paper is organized as follows. In section 2, we give a brief introduction of the three models mentioned above. And then the proposed model will be demonstrated in the section 3. In section 4 we provide the forecasting results of our model and in section 5 we make special improvement by adjusting the forecasting methods of sub-CPIs whose predicting results are not satisfying enough. And in section 6 we give elaborate discussion and evaluation of the proposed model. Finally, the conclusion is summarized in section 7.2.Introduction to GM(1,1), ARIMA & BPNNIntroduction to GM(1,1)The grey system theory is first presented by Deng in 1980s. In the grey forecasting model, the time series can be predicted accurately even with a small sample by directly estimating the interrelation of data. The GM(1,1) model is one type of the grey forecasting which is widely adopted. It is a differential equation model of which the order is 1 and the number of variable is 1, too. The differential equation is:Introduction to ARIMAAutoregressive Integrated Moving Average (ARIMA) model was first put forward by Box and Jenkins in 1970. The model has been very successful by taking full advantage of time series data in the past and present. ARIMA model is usually described as ARIMA (p, d, q), p refers to the order of the autoregressive variable, while d and q refer to integrated, and moving average parts of the model respectively. When one of the three parameters is zero, the model is changed to model “AR”, “MR”or “ARMR”. When none of the three parameters is zero, the model is given by:where L is the lag number,Ɛt is the error term.Introduction to BPNNArtificial Neural Network (ANN) is a mathematical and computational model which imitates the operation of neural networks of human brain. ANN consists of several layers of neurons. Neurons of contiguous layers are connected with each other. The values of connections between neurons are called “weight”. Back Propagation Neural Network (BPNN) is one of the most widely employed neural network among various types of ANN. BPNN was put forward by Rumelhart and McClelland in 1985. It is a common supervised learning network well suited for prediction. BPNN consists of three parts including one input layer, several hidden layers and one output layer, as is demonstrated in Fig 1. The learning process of BPNN is modifying the weights of connections between neurons based on the deviation between the actual output and the target output until the overall error is in the acceptable range.Fig. 1. Back-propagation Neural Network3.The Proposed MethodThe framework of the dividing-integration modelThe process of forecasting national CPI using the dividing-integration model is demonstrated in Fig 2.Fig. 2.The framework of the dividing-integration modelAs can be seen from Fig. 2, the process of the proposed method can be divided into the following steps: Step1: Data collection. The monthly CPI data including total CPI and eight sub-CPIs are collected from the official website of China’s State Statistics Bureau (/).Step2: Dividing the total CPI into eight sub-CPIs. In this step, the respective weight coefficient of eight sub-CPIs in forming the total CPI is decided by consulting authoritative source .(/). The eight sub-CPIs are as follows: 1. Food CPI; 2. Articles for Smoking and Drinking CPI; 3. Clothing CPI; 4. Household Facilities, Articles and Maintenance Services CPI; 5. Health Care and Personal Articles CPI; 6. Transportation and Communication CPI;7. Recreation, Education and Culture Articles and Services CPI; 8. Residence CPI. The weight coefficient of each sub-CPI is shown in Table 8.Table 1. 8 sub-CPIs weight coefficient in the total indexNote: The index number stands for the corresponding type of sub-CPI mentioned before. Other indexes appearing in this paper in such form have the same meaning as this one.So the decomposition formula is presented as follows:where TI is the total index; Ii (i 1,2, ,8) are eight sub-CPIs. To verify the formula, we substitute historical numeric CPI and sub-CPI values obtained in Step1 into the formula and find the formula is accurate.Step3: The construction of the GM (1, 1) model, the ARIMA (p, d, q) model and the BPNN model. The three models are established to predict the eight sub-CPIs respectively.Step4: Forecasting the eight sub-CPIs using the three models mentioned in Step3 and choosing the best forecasting result for each sub-CPI based on the errors of the data obtained from the three models.Step5: Making special improvement by adjusting the forecasting methods of sub-CPIs whose predicting results are not satisfying enough and get advanced predicting results of total CPI.Step6: Integrating the best forecasting results of 8 sub-CPIs to form the prediction of total CPI with the decomposition formula in Step2.In this way, the whole process of the prediction by the dividing-integration model is accomplished.3.2. The construction of the GM(1,1) modelThe process of GM (1, 1) model is represented in the following steps:Step1: The original sequence:Step2: Estimate the parameters a and u using the ordinary least square (OLS). Step3: Solve equation as follows.Step4: Test the model using the variance ratio and small error possibility.The construction of the ARIMA modelFirstly, ADF unit root test is used to test the stationarity of the time series. If the initial time series is not stationary, a differencing transformation of the data is necessary to make it stationary. Then the values of p and q are determined by observing the autocorrelation graph, partial correlation graph and the R-squared value.After the model is built, additional judge should be done to guarantee that the residual error is white noise through hypothesis testing. Finally the model is used to forecast the future trend ofthe variable.The construction of the BPNN modelThe first thing is to decide the basic structure of BP neural network. After experiments, we consider 3 input nodes and 1 output nodes to be the best for the BPNN model. This means we use the CPI data of time , ,toforecast the CPI of time .The hidden layer level and the number of hidden neurons should also be defined. Since the single-hidden- layer BPNN are very good at non-liner mapping, the model is adopted in this paper. Based on the Kolmogorov theorem and testing results, we define 5 to be the best number of hidden neurons. Thus the 3-5-1 BPNN structure is determined.As for transferring function and training algorithm, we select ‘tansig’as the transferring function for middle layer, ‘logsig’for input layer and ‘traingd’as training algorithm. The selection is based on the actual performance of these functions, as there are no existing standards to decide which ones are definitely better than others.Eventually, we decide the training times to be 35000 and the goal or the acceptable error to be 0.01.4.Empirical AnalysisCPI data from Jan. 2012 to Mar. 2013 are used to build the three models and the data from Apr. 2013 to Sept. 2013 are used to test the accuracy and stability of these models. What’s more, the MAPE is adopted to evaluate the performance of models. The MAPE is calculated by the equation:Data sourceAn appropriate empirical analysis based on the above discussion can be performed using suitably disaggregated data. We collect the monthly data of sub-CPIs from the website of National Bureau of Statistics of China (/).Particularly, sub-CPI data from Jan. 2012 to Mar. 2013 are used to build the three models and the data from Apr. 2013 to Sept. 2013 are used to test the accuracy and stability of these models.Experimental resultsWe use MATLAB to build the GM (1,1) model and the BPNN model, and Eviews 6.0 to build the ARIMA model. The relative predicting errors of sub-CPIs are shown in Table 2.Table 2.Error of Sub-CPIs of the 3 ModelsFrom the table above, we find that the performance of different models varies a lot, because the characteristic of the sub-CPIs are different. Some sub-CPIs like the Food CPI changes drastically with time while some do not have much fluctuation, like the Clothing CPI. We use different models to predict the sub- CPIs and combine them by equation 7.Where Y refers to the predicted rate of the total CPI, is the weight of the sub-CPI which has already been shown in Table 1and is the predicted value of the sub-CPI which has the minimum error among the three models mentioned above. The model chosen will be demonstrated in Table 3:Table 3.The model used to forecastAfter calculating, the error of the total CPI forecasting by the dividing-integration model is 0.0034.5.Model Improvement & Error AdjustmentAs we can see from Table 3, the prediction errors of sub-CPIs are mostly below 0.004 except for two sub- CPIs: Food CPI whose error reaches 0.0059 and Transportation & Communication CPI 0.0047.In order to further improve our forecasting results, we modify the prediction errors of the two aforementioned sub-CPIs by adopting other forecasting methods or models to predict them. The specific methods are as follows.Error adjustment of food CPIIn previous prediction, we predict the Food CPI using the BPNN model directly. However, the BPNN model is not sensitive enough to investigate the variation in the values of the data. For instance, although the Food CPI varies a lot from month to month, the forecasting values of it are nearly all around 103.5, which fails to make meaningful prediction.We ascribe this problem to the feature of the training data. As we can see from the original sub-CPI data on the website of National Bureau of Statistics of China, nearly all values of sub-CPIs are around 100. As for Food CPI, although it does have more absolute variations than others, its changes are still very small relative to the large magnitude of the data (100). Thus it will be more difficult for the BPNN model to detect the rules of variations in training data and the forecastingresults are marred.Therefore, we use the first-order difference series of Food CPI instead of the original series to magnify the relative variation of the series forecasted by the BPNN. The training data and testing data are the same as that in previous prediction. The parameters and functions of BPNN are automatically decided by the software, SPSS.We make 100 tests and find the average forecasting error of Food CPI by this method is 0.0028. The part of the forecasting errors in our tests is shown as follows in Table 4:Table 4.The forecasting errors in BPNN testError adjustment of transportation &communication CPIWe use the Moving Average (MA) model to make new prediction of the Transportation and Communication CPI because the curve of the series is quite smooth with only a few fluctuations. We have the following equation(s):where X1, X2…Xn is the time series of the Transportation and Communication CPI, is the value of moving average at time t, is a free parameter which should be decided through experiment.To get the optimal model, we range the value of from 0 to 1. Finally we find that when the value of a is 0.95, the forecasting error is the smallest, which is 0.0039.The predicting outcomes are shown as follows in Table5:Table 5.The Predicting Outcomes of MA modelAdvanced results after adjustment to the modelsAfter making some adjustment to our previous model, we obtain the advanced results as follows in Table 6: Table 6.The model used to forecast and the Relative ErrorAfter calculating, the error of the total CPI forecasting by the dividing-integration model is 0.2359.6.Further DiscussionTo validate the dividing-integration model proposed in this paper, we compare the results of our model with the forecasting results of models that do not adopt the dividing-integration method. For instance, we use the ARIMA model, the GM (1, 1) model, the SARIMA model, the BRF neural network (BRFNN) model, the Verhulst model and the Vector Autoregression (VAR) model respectively to forecast the total CPI directly without the process of decomposition and integration. The forecasting results are shown as follows in Table7.From Table 7, we come to the conclusion that the introduction of dividing-integration method enhances the accuracy of prediction to a great extent. The results of model comparison indicate that the proposed method is not only novel but also valid and effective.The strengths of the proposed forecasting model are obvious. Every sub-CPI time series have different fluctuation characteristics. Some are relatively volatile and have sharp fluctuations such as the Food CPI while others are relatively gentle and quiet such as the Clothing CPI. As a result, by dividing the total CPI into several sub-CPIs, we are able to make use of the characteristics of each sub-CPI series and choose the best forecasting model among several models for every sub-CPI’s prediction. Moreover, the overall prediction error is provided in the following formula:where TE refers to the overall prediction error of the total CPI, is the weight of the sub-CPI shown in table 1 and is the forecasting error of corresponding sub-CPI.In conclusion, the dividing-integration model aims at minimizing the overall prediction errors by minimizing the forecasting errors of sub-CPIs.7.Conclusions and future workThis paper creatively transforms the forecasting of national CPI into the forecasting of 8 sub-CPIs. In the prediction of 8 sub-CPIs, we adopt three widely used models: the GM (1, 1) model, the ARIMA model and the BPNN model. Thus we can obtain the best forecasting results for each sub-CPI. Furthermore, we make special improvement by adjusting the forecasting methods of sub-CPIs whose predicting results are not satisfying enough and get the advanced predicting results of them. Finally, the advanced predicting results of the 8 sub- CPIs are integrated to formthe forecasting results of the total CPI.Furthermore, the proposed method also has several weaknesses and needs improving. Firstly, The proposed model only uses the information of the CPI time series itself. If the model can make use of other information such as the information provided by factors which make great impact on the fluctuation of sub-CPIs, we have every reason to believe that the accuracy and stability of the model can be enhanced. For instance, the price of pork is a major factor in shaping the Food CPI. If this factor is taken into consideration in the prediction of Food CPI, the forecasting results will probably be improved to a great extent. Second, since these models forecast the future by looking at the past, they are not able to sense the sudden or recent change of the environment. So if the model can take web news or quick public reactions with account, it will react much faster to sudden incidence and affairs. Finally, the performance of sub-CPIs prediction can be higher. In this paper we use GM (1, 1), ARIMA and BPNN to forecast sub-CPIs. Some new method for prediction can be used. For instance, besides BPNN, there are other neural networks like genetic algorithm neural network (GANN) and wavelet neural network (WNN), which might have better performance in prediction of sub-CPIs. Other methods such as the VAR model and the SARIMA model should also be taken into consideration so as to enhance the accuracy of prediction.References1.Wang W, Wang T, and Shi Y. Factor analysis on consumer price index rising in China from 2005 to 2008. Management and service science 2009; p. 1-4.2.Qin F, Ma T, and Wang J. The CPI forecast based on GA-SVM. Information networking and automation 2010; p. 142-147.3.George EPB, Gwilym MJ, and Gregory CR. Time series analysis: forecasting and control. 4th ed. Canada: Wiley; 20084.Weng D. The consumer price index forecast based on ARIMA model. WASE International conferenceon information engineering 2010;p. 307-310.5.Jian L, Zhao Y, Zhu YP, Zhang MB, Bertolatti D. An application of ARIMA model to predict submicron particle concentrations from meteorological factors at a busy roadside in Hangzhou, China. Science of total enviroment 2012;426:336-345.6.Priya N, Ashoke B, Sumana S, Kamna S. Trend analysis and ARIMA modelling of pre-monsoon rainfall data forwestern India. Comptesrendus geoscience 2013;345:22-27.7.Hwang HB. Insights into neural-network forecasting of time seriescorresponding to ARMA(p; q) structures. Omega 2001;29:273-289.am A. Using a neural network to forecast inflation. Industrial management & data systems 1999;7:296-301.9.Min X, Wong WK. A seasonal discrete grey forecasting model for fashion retailing. Knowledge based systems 2014;57:119-126.11. Weimin M, Xiaoxi Z, Miaomiao W. Forecasting iron ore import and consumption of China using grey model optimized by particleswarm optimization algorithm. Resources policy 2013;38:613-620.12. Zhen D, and Feng S. A novel DGM (1, 1) model for consumer price index forecasting. Greysystems and intelligent services (GSIS)2009; p. 303-307.13. Yu W, and Xu D. Prediction and analysis of Chinese CPI based on RBF neural network. Information technology and applications2009;3:530-533.14. Zhang GP. Time series forecasting using a hybrid ARIMA and neural network model. Neurocomputing 2003;50:159-175.15. Pai PF, Lin CS. A hybrid ARIMA and support vector machines model in stock price forecasting. Omega 2005;33(6):497-505.16. Tseng FM, Yu HC, Tzeng GH. Combining neural network model with seasonal time series ARIMA model. Technological forecastingand social change 2002;69(1):71-87.17.Cho MY, Hwang JC, Chen CS. Customer short term load forecasting by using ARIMA transfer function model. Energy management and power delivery, proceedings of EMPD'95. 1995 international conference on IEEE, 1995;1:317-322.译文:一种基于ARIMA、灰色模型和BPNN对CPI(消费物价指数)进行预测的新型分治模型摘要:在本文中,利用我国现有的消费者价格指数(CPI)的计算方法,提出了一种新的CPI预测分治模型。
基于Handel-C的PCNN模型研究及应用

的明确描 述 ,导致 实验 结果 的分析 比较 困难 [] 6。 2 ch r模 型应 用 时众 多参 数需 要 设置 [] a gn t等人 根 )E kon 6 。H SR na ah 据E ko n 型提 出 了一组 较为 简洁 的PN 模 型的数 学表 达式 :模 型结 构如 e hr模 CN
10在式6一10中sij代表图像像素灰度值b是连接域的连接系数vfvl和ve分别是反馈输入域连接域和动态门限的放大系数afal和ae分别是反馈输入域连接域和动态门限的时间衰减常数mijk和ijkl分别为反馈输入域和耦合连接域的连接矩阵is
信 息
科 学
V A
一 -
基 于Ha e- n l C的PCNN模 型 研 究 及 应 用 d
在 式 ( )一 (0 6 1 )中 ,sj 表 图像 像 素灰度 值 , B是 连接 域的 连接 系 i 代
数 ,V、V和 V分 别 是反馈 输 入 域 、连接 域 和动 态 门 限的 放大 系数 , a 、 F L E F
a 和 a 分 别是 反 馈 输入 域 、 连接 域 和动 态 门 限 的时 间衰 减 常数 ,Mj 和 L E il k Wj分 别 为反馈 输入 域和 耦合连 接域 的连 接矩 阵 [] i1 k 6。
FG , 即现场 可编 程 逻辑 阵列 ;它 基于 PL A 、CL 等 可编程 器件 PA A 、G L PD 的基 础 上进 一 步发 展 的一种 专用 半 定制 电路 芯 片 。当前 , 生产 FG 芯片 的 FA
厂家 主 要 有A tr 、X ln 等 当 前 ,FG 可 以使 用V D 、Hn l— 等语 le a i ix PA H L ad eC 言来 进 行应 用 开发 。本 文 所用 的F G开 发 芯 片是x ln 公 司 的X2 I0 , PA i ix C VO0 如 图 2 示 。X 2 I0拥 有 10 个 系 统 门 ,4 ×3 个 可 配 置 逻 辑单 元 , 所 CV O0 0万 0 2 4个 lX1 is O 8 b t乘法 器 ,70b t A ,8 DM 块 [] 8 2 K isRM + C 模 9
基于加性耦合连接的PCNN模型

基于加性耦合连接的PCNN模型荣剑;赵同林;赵毅力;张晴晖【摘要】The pulse coupled neural network (PCNN) is better than the traditional neural network. It can imitate biological neurons with considering space and time accumulation. When plus-coupled is proposed instead of multiplicative-coupled in PCNN, the difference of image processing effect is tiny. The entropy of plus-coupled is approximate compared with that of coupled and non-coupled PCNN, but PCNN with the plus-coupled connection overcomes the periodicity of non-coupled PCNN, provides the stop conditions, and maintains rotation invariant, scaling invariance and some other characteristics of PCNN with the multiplicative-coupled connection. Furthermore, it maintains the time and space accumulation. It is more suitable for hardware implementation due to easy computation.%脉冲耦合神经网络(PCNN)比传统神经网络更好地模仿了生物神经元,既考虑空间累加,又考虑了时间累加.在此用加性耦合代替乘性耦合,在图像处理效果上与耦合和无耦合PCNN的效果相比,其差别较小,熵与耦合PCNN处理后图像的熵基本一致.但加性耦合连接的PCNN,改变了无耦合PCNN的周期特性,提供了停止条件,保持了PCNN的旋转不变性、缩放不变性等一些特性,同时保持了时间和空间上的积累,且迭代次数少,计算量相对较小,更适合于硬件实现.【期刊名称】《现代电子技术》【年(卷),期】2011(034)022【总页数】3页(P126-128)【关键词】PCNN;时间签名;熵;加性耦合;乘性耦合【作者】荣剑;赵同林;赵毅力;张晴晖【作者单位】西南林业大学计算机与信息学院,云南昆明650224;西南林业大学计算机与信息学院,云南昆明650224;西南林业大学计算机与信息学院,云南昆明650224;西南林业大学计算机与信息学院,云南昆明650224【正文语种】中文【中图分类】TN919-34;TP391.7;TP751.10 引言Eckhorn在1990年根据猫的大脑视觉皮层同步脉冲发放现象,提出了展示脉冲发放现象的连接模型[1]。
基于PCNN和指数型模糊加权熵的医学图像融合

基于PCNN和指数型模糊加权熵的医学图像融合姚丽莎;赵海峰;罗斌【摘要】In order to integrate CT and MRI images to be better,this paper presents a new CT and MRI medical image fusion method.This method proposes adaptive exponentially weighted fuzzy entropy fusion rules and improved regional information PCNN fusion rules.It is in the framework of multi-wavelet for CT and MRI medical image fusion.The images are decomposed based on multi-wavelets.Different image fusion algorithms are used for different frequency components.Experiments show that the proposed algorithm is superior to other fusion algorithms.lt improves the clarity of the image and retains the details of the image in large extent,with the advantages of prominent edge information and high brightness and contrast.%为了更好地对CT和MRI图像进行融合,提出了一种指数型模糊加权熵自适应融合规则和改进的PCNN区域信息融合规则在多小波基的框架下进行CT和MRI医学图像的融合方法.对待融合图像进行多小波基的分解,对不同频率分量采用不同融合算法.实验表明,算法明显优于其他融合算法.它提高了图像的清晰度,较大程度保留了细节信息,具有边缘信息突出,亮度对比度高的优点.【期刊名称】《计算机工程与应用》【年(卷),期】2011(047)025【总页数】4页(P198-201)【关键词】指数型模糊熵;多小波基;脉冲耦合神经网络;图像融合【作者】姚丽莎;赵海峰;罗斌【作者单位】安徽大学计算智能与信号处理教育部重点实验室,合肥230039;安徽大学计算智能与信号处理教育部重点实验室,合肥230039;安徽大学计算智能与信号处理教育部重点实验室,合肥230039【正文语种】中文【中图分类】TP391.411 引言医学成像已经成为现代医疗不可或缺的一部分。
PCNN在图像增强中的应用
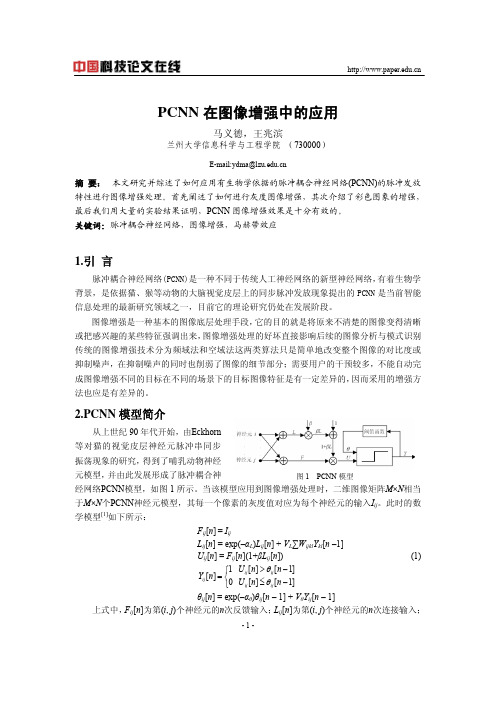
PCNN 在图像增强中的应用马义德,王兆滨兰州大学信息科学与工程学院 (730000)E-mail:ydma@摘 要: 本文研究并综述了如何应用有生物学依据的脉冲耦合神经网络(PCNN)的脉冲发放特性进行图像增强处理。
首先阐述了如何进行灰度图像增强,其次介绍了彩色图象的增强,最后我们用大量的实验结果证明,PCNN 图像增强效果是十分有效的。
关键词:脉冲耦合神经网络,图像增强,马赫带效应1.引 言脉冲耦合神经网络(PCNN)是一种不同于传统人工神经网络的新型神经网络,有着生物学背景,是依据猫、猴等动物的大脑视觉皮层上的同步脉冲发放现象提出的PCNN 是当前智能信息处理的最新研究领域之一,目前它的理论研究仍处在发展阶段。
图像增强是一种基本的图像底层处理手段,它的目的就是将原来不清楚的图像变得清晰或把感兴趣的某些特征强调出来,图像增强处理的好坏直接影响后续的图像分析与模式识别传统的图像增强技术分为频域法和空域法这两类算法只是简单地改变整个图像的对比度或抑制噪声,在抑制噪声的同时也削弱了图像的细节部分;需要用户的干预较多,不能自动完成图像增强不同的目标在不同的场景下的目标图像特征是有一定差异的,因而采用的增强方法也应是有差异的。
2.PCNN 模型简介图1 PCNN 模型从上世纪90年代开始,由Eckhorn等对猫的视觉皮层神经元脉冲串同步振荡现象的研究,得到了哺乳动物神经元模型,并由此发展形成了脉冲耦合神经网络PCNN 模型,如图1所示。
当该模型应用到图像增强处理时,二维图像矩阵M ×N 相当于M ×N 个PCNN 神经元模型,其每一个像素的灰度值对应为每个神经元的输入I ij 。
此时的数学模型[1]如下所示:F ij [n ] = I ijL ij [n ] = exp(–αL )L ij [n ] + V L ∑W ijkl Y kl [n –1]U ij [n ] = F ij [n ](1+βL ij [n ]) (1)]1[][]1[][01][−≤>⎩⎨⎧=n n U n n U n Y ij ij ij ij ij θθ− θij [n ] = exp(–αθ)θij [n – 1] + V θY ij [n – 1]上式中,F ij [n ]为第(i , j )个神经元的n 次反馈输入;L ij [n ]为第(i , j )个神经元的n 次连接输入; - 1 -I ij为外部输入刺激信号(这里为图像像素构成的矩阵中第(i, j)个像素的灰度值);U ij[n]为神经元内部活动项;Y ij[n]是PCNN脉冲输出序列;θij[n]为动态门限值;β为突触之间连接强度常数;W为内部连接矩阵,W ijkl为L ij[n]中Y kl[n –1]的加权系数;V L、Vθ分别为L ij[n]、θij[n]中的固有电势,αL、αθ分别为L ij[n]、θij[n]的衰减时间常数。
基于自动波的PCNN模型及其应用
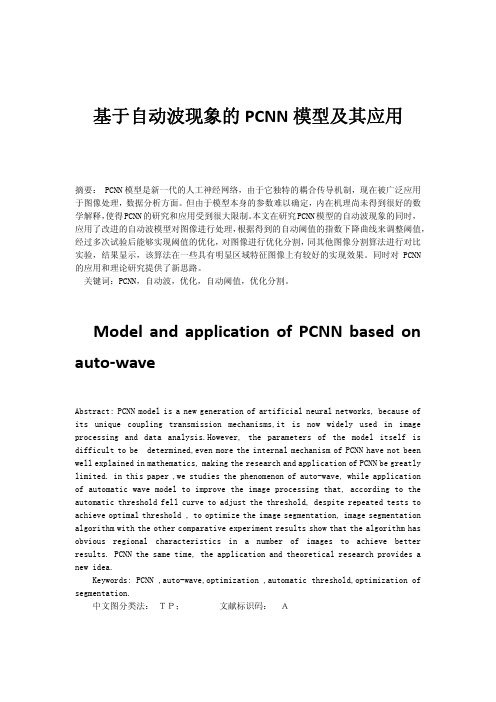
基于自动波现象的PCNN模型及其应用摘要: PCNN模型是新一代的人工神经网络,由于它独特的耦合传导机制,现在被广泛应用于图像处理,数据分析方面。
但由于模型本身的参数难以确定,内在机理尚未得到很好的数学解释,使得PCNN的研究和应用受到很大限制。
本文在研究PCNN模型的自动波现象的同时,应用了改进的自动波模型对图像进行处理,根据得到的自动阈值的指数下降曲线来调整阈值,经过多次试验后能够实现阈值的优化,对图像进行优化分割,同其他图像分割算法进行对比实验,结果显示,该算法在一些具有明显区域特征图像上有较好的实现效果。
同时对PCNN 的应用和理论研究提供了新思路。
关键词:PCNN,自动波,优化,自动阈值,优化分割。
Model and application of PCNN based on auto‐waveAbstract: PCNN model is a new generation of artificial neural networks, because of its unique coupling transmission mechanisms,it is now widely used in image processing and data analysis.However, the parameters of the model itself is difficult to be determined,even more the internal mechanism of PCNN have not been well explained in mathematics, making the research and application of PCNN be greatly limited. in this paper ,we studies the phenomenon of auto-wave, while application of automatic wave model to improve the image processing that, according to the automatic threshold fell curve to adjust the threshold, despite repeated tests to achieve optimal threshold , to optimize the image segmentation, image segmentation algorithm with the other comparative experiment results show that the algorithm has obvious regional characteristics in a number of images to achieve better results. PCNN the same time, the application and theoretical research provides a new idea.Keywords: PCNN ,auto-wave,optimization ,automatic threshold,optimization of segmentation.中文图分类法: TP; 文献标识码: A一:PCNN模型1,PCNN模型的诞生与 应用Pcnn模型是在猫和猴子的视觉皮层实验中根据视觉神经的脉冲簇现象而提出来的,从生理学上来说,当一些在空间上相邻同时在强度上相近的神经刺激会使神经细胞趋向于用同样的频率来发放脉冲。
PCNN原理及其在图像处理中的应用研究
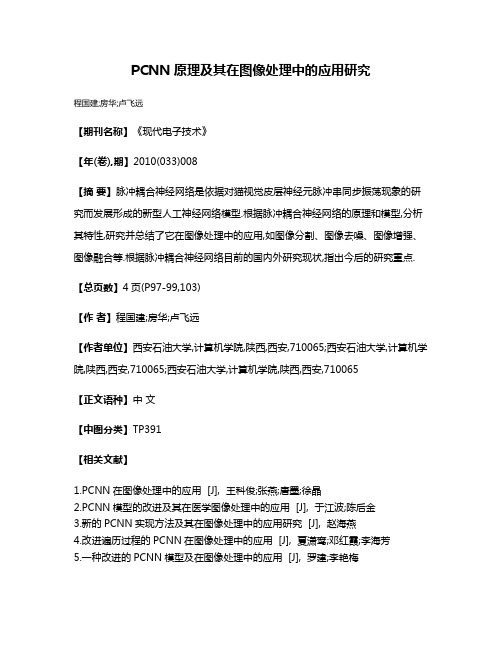
PCNN原理及其在图像处理中的应用研究
程国建;房华;卢飞远
【期刊名称】《现代电子技术》
【年(卷),期】2010(033)008
【摘要】脉冲耦合神经网络是依据对猫视觉皮层神经元脉冲串同步振荡现象的研究而发展形成的新型人工神经网络模型.根据脉冲耦合神经网络的原理和模型,分析其特性,研究并总结了它在图像处理中的应用,如图像分割、图像去噪、图像增强、图像融合等.根据脉冲耦合神经网络目前的国内外研究现状,指出今后的研究重点.【总页数】4页(P97-99,103)
【作者】程国建;房华;卢飞远
【作者单位】西安石油大学,计算机学院,陕西,西安,710065;西安石油大学,计算机学院,陕西,西安,710065;西安石油大学,计算机学院,陕西,西安,710065
【正文语种】中文
【中图分类】TP391
【相关文献】
1.PCNN在图像处理中的应用 [J], 王科俊;张燕;唐墨;徐晶
2.PCNN模型的改进及其在医学图像处理中的应用 [J], 于江波;陈后金
3.新的PCNN实现方法及其在图像处理中的应用研究 [J], 赵海燕
4.改进遍历过程的PCNN在图像处理中的应用 [J], 夏潇鸾;邓红霞;李海芳
5.一种改进的PCNN模型及在图像处理中的应用 [J], 罗建;李艳梅
因版权原因,仅展示原文概要,查看原文内容请购买。
基于强度 PCNN 的静态图像人脸识别

基于强度 PCNN 的静态图像人脸识别常莎;邓红霞;李海芳【期刊名称】《太原理工大学学报》【年(卷),期】2015(46)1【摘要】In order to reduce the influence of the change of faceposture ,facial expression and light in the face image on face recognition ,a novel feature extracting method is quoted for face recognition based on the strength of Pulse Coupled Neural network (PCNN ) .Different faceima_ges have different grayscale characteristics ,every image can form a specific pulse intensity matrix after being put into the new PCNNmodel .Experiments use pulse intensity matrix as facial fea_tures ,and combine the distance classifier—cosine distance for facerecognition .Simulation results show that ,the characteristics extracted by the strength of PCNN model can portray the details of the face .For different posture ,facial expression and facial mask of the face image ,good recogni_tion result is obtained .This method has strong robustness in the aspact of feature extraction for complex face image .%为了减少人脸图像中姿势、表情和光照等因素对人脸识别的影响,引用了一种基于脉冲发放强度的脉冲耦合神经网络(PCNN ,pulse coupled neural network)的人脸特征提取方法。
基于视觉信息的PCNN参数自适应设定及模型改进
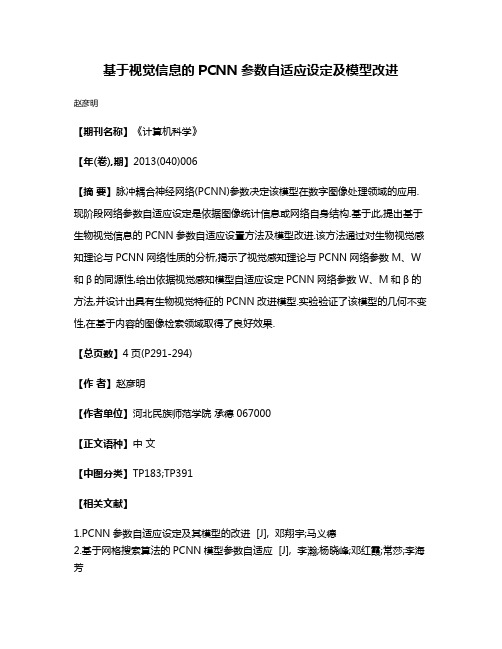
基于视觉信息的PCNN参数自适应设定及模型改进
赵彦明
【期刊名称】《计算机科学》
【年(卷),期】2013(040)006
【摘要】脉冲耦合神经网络(PCNN)参数决定该模型在数字图像处理领域的应用.现阶段网络参数自适应设定是依据图像统计信息或网络自身结构.基于此,提出基于生物视觉信息的PCNN参数自适应设置方法及模型改进.该方法通过对生物视觉感知理论与PCNN网络性质的分析,揭示了视觉感知理论与PCNN网络参数M、W 和β的同源性,给出依据视觉感知模型自适应设定PCNN网络参数W、M和β的方法,并设计出具有生物视觉特征的PCNN改进模型.实验验证了该模型的几何不变性,在基于内容的图像检索领域取得了良好效果.
【总页数】4页(P291-294)
【作者】赵彦明
【作者单位】河北民族师范学院承德067000
【正文语种】中文
【中图分类】TP183;TP391
【相关文献】
1.PCNN参数自适应设定及其模型的改进 [J], 邓翔宇;马义德
2.基于网格搜索算法的PCNN模型参数自适应 [J], 李瀚;杨晓峰;邓红霞;常莎;李海芳
3.一种基于PCNN的参数自适应图像分割方法 [J], 汤灿明;张欣;杨贝贝;贺建婷
4.基于参数自适应PCNN和卷积稀疏的多聚焦图像融合 [J], 李致金;顾鹏;钱百青
5.基于参数自适应PCNN和卷积稀疏的多聚焦图像融合 [J], 李致金;顾鹏;钱百青因版权原因,仅展示原文概要,查看原文内容请购买。
一种用于紫外成像系统的PCNN图像融合算法
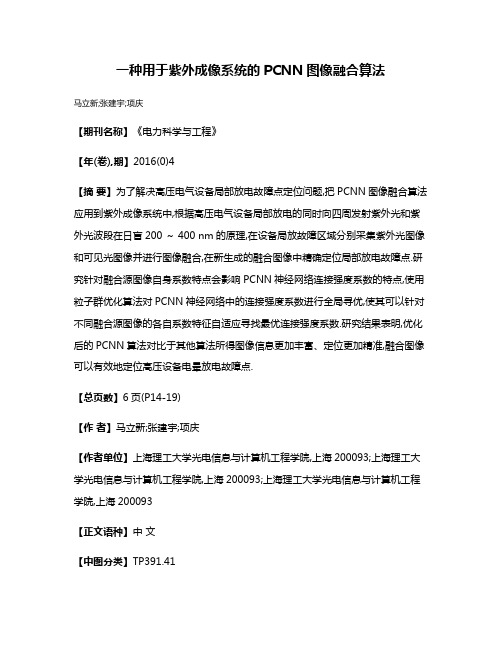
一种用于紫外成像系统的PCNN图像融合算法
马立新;张建宇;项庆
【期刊名称】《电力科学与工程》
【年(卷),期】2016(0)4
【摘要】为了解决高压电气设备局部放电故障点定位问题,把PCNN图像融合算法应用到紫外成像系统中,根据高压电气设备局部放电的同时向四周发射紫外光和紫外光波段在日盲200 ~ 400 nm的原理,在设备局放故障区域分别采集紫外光图像和可见光图像并进行图像融合,在新生成的融合图像中精确定位局部放电故障点.研究针对融合源图像自身系数特点会影响PCNN神经网络连接强度系数的特点,使用粒子群优化算法对PCNN神经网络中的连接强度系数进行全局寻优,使其可以针对不同融合源图像的各自系数特征自适应寻找最优连接强度系数.研究结果表明,优化后的PCNN算法对比于其他算法所得图像信息更加丰富、定位更加精准,融合图像可以有效地定位高压设备电晕放电故障点.
【总页数】6页(P14-19)
【作者】马立新;张建宇;项庆
【作者单位】上海理工大学光电信息与计算机工程学院,上海200093;上海理工大学光电信息与计算机工程学院,上海200093;上海理工大学光电信息与计算机工程学院,上海200093
【正文语种】中文
【中图分类】TP391.41
【相关文献】
1.一种基于NSCT和PCNN的图像融合算法 [J], 石瀚洋;杨静;赵焱
2.一种改进的PCNN 图像融合算法 [J], 郭传奇;汪文革;储彬彬
3.两类基于PCNN的图像融合算法综述 [J], 贾紫婷
4.一种改进的基于NSST-SPCNN医学图像融合算法 [J], 常春红;王雷;郝本利;邢艺馨
5.基于改进PCNN模型的偏振图像融合算法 [J], 姜兆祯;韩裕生;任帅军;张延厚因版权原因,仅展示原文概要,查看原文内容请购买。
基于 PCNN的自动图像分割
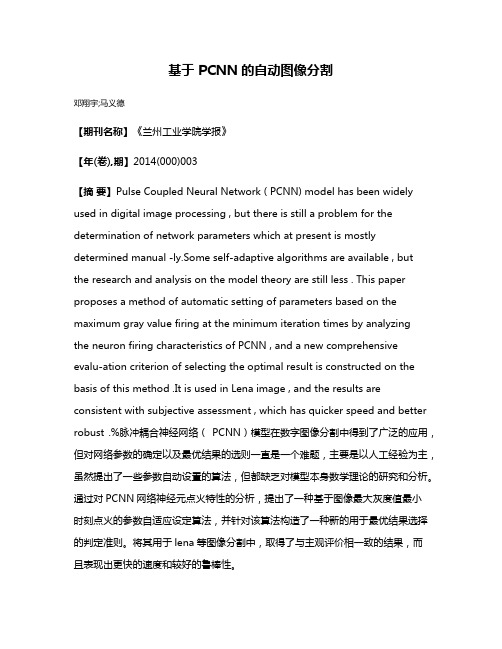
基于 PCNN的自动图像分割邓翔宇;马义德【期刊名称】《兰州工业学院学报》【年(卷),期】2014(000)003【摘要】Pulse Coupled Neural Network ( PCNN) model has been widely used in digital image processing , but there is still a problem for the determination of network parameters which at present is mostly determined manual -ly.Some self-adaptive algorithms are available , butthe research and analysis on the model theory are still less . This paper proposes a method of automatic setting of parameters based on the maximum gray value firing at the minimum iteration times by analyzingthe neuron firing characteristics of PCNN , and a new comprehensiveevalu-ation criterion of selecting the optimal result is constructed on the basis of this method .It is used in Lena image , and the results are consistent with subjective assessment , which has quicker speed and better robust .%脉冲耦合神经网络(PCNN)模型在数字图像分割中得到了广泛的应用,但对网络参数的确定以及最优结果的选则一直是一个难题,主要是以人工经验为主,虽然提出了一些参数自动设置的算法,但都缺乏对模型本身数学理论的研究和分析。
- 1、下载文档前请自行甄别文档内容的完整性,平台不提供额外的编辑、内容补充、找答案等附加服务。
- 2、"仅部分预览"的文档,不可在线预览部分如存在完整性等问题,可反馈申请退款(可完整预览的文档不适用该条件!)。
- 3、如文档侵犯您的权益,请联系客服反馈,我们会尽快为您处理(人工客服工作时间:9:00-18:30)。
PCNN模型及其应用约翰·L·约翰逊和玛丽娄帕吉特,会员,IEEE摘要-本文将描述脉冲耦合神经网络模型。
其链接领域调制术语显示其网络模型是生物基础性树状模型的普遍特征。
本文将综述和回顾神经网络模型的应用与实现并且基于应用程序的变化与简化进行总结。
本文将在新的细节方面对神经网络图像图解进行阐释。
关键词-树状模型,脉冲神经网络模型因式分解,脉冲耦合神经网络1 介绍从霍金和赫胥黎的开创性研究到最近关于内部树状脉冲生成研究,神经元电化学动态研究使模型越来越精转化和细节化。
生物模型到算法模型的转录引出了广泛的文献数据处理系统,其系统最初主要关注于将自适应算法进运用于数据分类器。
关于脉冲神经元动态研究,不论适应与否,是最近的研究项目。
早期的一篇论文描述了一个基于一对耦合振荡器的动态链接架构。
同步脉冲在猫视觉皮层的爆发的实验观察鞭策了更多的关于生物基础性脉冲动态系统的研究。
1990年eckhorn网络连接系统以现象学模型系统被介绍并展示了同步脉冲迸发。
它用一个叫神经元模型的脉冲生成器,一个调制耦合项和一个作为漏水容器的突出链接建模。
中央新概念是次要接受域和链接域的引入,它的整合引入是通过内部细胞电路调节远处喂养接受域。
这提供了一个简单,有效的仿真工具并研究同步脉冲的动态网络,很快就被认为是在图像处理的重要应用。
大量的变形与变体被引入到链接领域模型是为了调整作为图像处理算法的表现,而这些被统称为脉冲耦合神经网络。
这种链接调节能够适用于高阶网络和一种新型的图像融合,并进一步容许在单个神经上进行任意复杂模糊的逻辑规则系统建设。
脉冲耦合神经网络的两种基本属性正是脉冲产品的应用。
较后的属性直接来源于原创链接领域作为其基本耦合机制。
这是一个由其他神经元输入的不对称的调制。
选择调节耦合而不是常见的添加剂耦合的优点是如果一个神经元没有主要输入则不能被神经元耦合输入激活,这个特点在图像处理功能上是很重要的。
添加剂耦合是一个在并联的突触电流的主要生物机制,因此添加剂,有实验证据表明,脉冲产品和空间信息的时间编码可以同等重要。
候选人机制产生乘法耦合来自于下面动力学区划模型的树状计算。
第二部分首先详细检查有两个输入,相的最小数量的分为若干部分的模型。
它显示输入的调节耦合,随着时间的信号如何即使在非常简单的模型细胞提供一个优雅的独特编码信息。
然后讨论了脉冲发生器机制,结果表明,神经元模型和integrate-and-fire(及)模型在脉冲的产生破裂有一个重要区别。
然后讨论了脉冲发生器机制,结果表明,神经元模型和integrate-and-fire(及)模型在脉冲的产生破裂有一个重要区别。
第三部分回顾了基本链接;再利用的大多是基于领域模型,多脉冲和单脉冲体制,和一些有用方面的脉冲耦合神经网络。
这些包括逻辑规则、图像融合、规模定义连接强度,标志性的时间信号,脉冲耦合神经元网络树状图,混乱的结构。
第四部分是一个描述脉冲耦合神经元网络技术和不同变体给予定义不同的简短回顾, 第五部分致力于脉冲耦合神经元网络的应用和实现。
因为脉冲耦合神经元网络通常用作非适应处理器、连通性的要求很低,实际构建高速电子芯片。
第六部分, 脉冲耦合神经元网络的算法描述,包括对那些感兴趣开发简单的软件代码的版本。
它显示了用在许多图像处理应用程序的主要简化和快捷键。
伪代码包括保付模式的新细节。
第七部分给出了这些变化的应用和脉冲耦合神经元网络保付特点的示例。
这个分析的目的是要表明,分流诱导调节耦合是一个通用的和普遍的生物脉冲耦合机制对于脉冲产品来说它不是独家机制。
沿着密集树突突触输入非线性增强效应最近被实验证实。
激活通道交互的电导具有电压依赖性。
它在输入复杂的细胞中提供了一个乘法效应,并与钠的活跃的树突峰值有关。
像分流效果,它允许加法和乘法耦合。
而不是选择添加剂耦合或乘法耦合,自然选择。
脉冲耦合神经元网络和标准霍金赫胥黎神经模型模型的主要区别是在脉冲发生器的选择上。
用于原始Eckhorn模型的神经元模型非常类似于生物integrate-and-fire 脉冲发生器整体性能,但它有一些基本的差异。
稍后将讨论这些差异。
脉冲耦合神经元网络是基于在至少两个不同的输入。
区划的模型单元由一个树突部分和第二部分包含integrate-and-fire脉冲发生器,与每个隔间单个突触输入组成,提供数学透明度和充分性的正确组合。
这个模型包含了大量的简化和近似生物更为精确的模型,他们反过来是真正神经元的不完整模型。
这些因素不考虑多个离子突触渠道,激活渠道,细胞老化,长期和短期适应,连续树突几何图形和树枝,不应期(作为一个附加词除外),和温度的影响。
每个隔间是漏电容。
漏了三个主要部分组成:内在的,突触和活跃。
只有firsttwo被用在这里。
突触可以接收一个脉冲输入或稳态输入。
该车厢体生成输出脉冲。
该室由一个串联连接阻力。
不存在轴突隔室。
图。
图1(a)示出了胞体和树突区室,和它们的突触输入电导F分别标有L和。
图。
图1(b)示的等效电路。
标记的框表示脉冲发生器。
是在细胞内的静息电位,名义上大约70毫伏,是突触回来的潜力,通常为20毫伏,并且是隔室电容,毫微数量级的,而并且所述膜固有泄漏电导和纵向电导分别。
突触和固有电导是megaMhos的顺序,而纵向电导的范围可以从10到一千倍[18]。
隔室电压由所描述这突触传导的波形往往建模为阿尔法函数[19],其中。
这是代表一个输入脉冲。
电导可以有其它形式也是如此。
它可以是一个常数,一个方波脉冲,或Delta函数。
同样,该输出脉冲函数可以有几种形式。
阶跃函数步骤为恒定步离散时间模拟有用,形成脉冲在两个时间的步骤。
是门槛pulseinitiation。
一种变化是使用阶梯函数,作为栅极一个脉冲串被设置在最大允许脉冲速率,所建议的Eckhorn[7]。
第三个表示是使用的冲激函数一笔接管了开通时间,这超过时,被定义为突触分流调制项是最容易看出,在稳态情况下,其中内部活动已达到低于阈值进行实弹射击的均衡水平。
对于恒定的输入和输出,并没有脉冲,调制产物立即从所获得的nullcline(1)其中()的形式为这是一个饱和的活动功能。
它有一个内在的漏术语,线性项和一个乘积项。
因为是负数和为正时,泄漏项是负的,并设置一阈值进行实弹射击。
由于,的系数线性分子联项是肯定的,因为是其余条款。
在尺寸方面,电导占主导地位的内在和输入电导。
这使得常数和分子和分母的线性项的所有大小的顺序相同,而产物系数是在漏电导的纵向比电导。
这些对应于连接强度因子,并且是0.1〜0.001或更小的数量级。
可以看出,该突触分流方面引起的线性和乘法术语的内部活动,而后者可以是一个显著的实力。
0.1连接强度的因素给予大幅修改脉冲活动模式[20]。
该调制是对称的输入(不像的原始链接字段的模型),并且该活动将饱和的大投入。
(1)对于一个一般的树突系统与nullcline 无数的投入,多分枝,渠道和树木同样给出了线性和乘法计算的组合在一个饱和的比例。
然而,这将是复杂得多。
它包含的产品和求和的所有可能组合,而事实上将包括一个一般的高阶模型神经元其顺序等于投入的总数达到了整个神经元,它可以是几千输入。
该highestlevel 产品会因的分离减毒最遥远的突触上的树突树,与调制其中本地组更加明显。
现在,考虑该单元产生脉冲的情况下,仍然不断的投入和。
该解决方案很容易由2×2矩阵对角化得到的对角化变换矩阵为一般22 矩阵是这得到解决得到其中时间的依赖关系是在脉冲函数(2)。
并且对角化的特征值矩阵。
尽管两室,两输入端的简单性系统,它通常不被解析地求解。
Bressloff和泰勒21]审查的一般问题,包括分流效应,和迭代的方法,涉及的时间有序运营商是给出。
在实施例部分,然而,只有一个单输入,twocompartment nonshunting解决方案提出。
因此,未讨论的调产物的存在,在那里,也不由Bressloff[22],后者参考的延伸[21]。
拉尔[17]等人[16],[18]将重点放在数值方法。
对于这种情况,其中调制产物得到的解析解,再次忽略。
这是尽管实验证据[15]对脉冲的产品训练时间信号。
这些调制产品提供的关键功能逻辑AND结合,从而使结构对单个神经元的大型,复杂的逻辑规则系统和生物实施的Σ-PI网,高阶网,以及PCNN网。
由于Eckhorn链接字段的内部活动调节耦合被看作是一个不对称的版本标准房室的稳态内部活动模型,从而提供了一个基本的生物接地连接域和所有PCNN的改编自它。
它进一步支持McClurkin等人的实验结果。
[15] 通过示出,有一个明确的通用机制形成输入时间信号的产品在标准信元模型。
甚至不断投入受限的情况下显示了一些有趣的动力学性质。
这种情况下,将对应到该接收nonpulsed输入从视网膜神经节细胞早期的视网膜层,输入是大致恒定在视觉固定的时期。
唯一的轮廓代数解,则由细节,而直未来,是繁琐和冗长。
从只是个脉冲刚过后的整合(3)本次的脉冲。
申请条件是电压incompartment1刚复位后在其最低。
这给出了两个不同的方程组的电压中的枝晶隔层,一个输入每一个函数,并和次峰峰时间间隔。
其中一个公式是在时刻;另一种是在时间这些可以合并,得到各峰峰时间间隔在输入端,蜂窝的几何形状和电化学条款结构,而以前的峰峰间隔时间对于前面给出的电导和电压值制度,这些功能是其中,功能涉及投入条款及房室几何。
图。
图2示出了可能的情况。
对于情况(一),如果曲线不相交并且只有一个脉冲。
对于情况(b)和(c)条,如果曲线相交。
在这种情况下,如果进一步(b)所示,脉冲序列可以达到点吸引子,极限环,或在有限的可以终止脉冲数,而如果(c)中的序列将任何一个点吸引或极限环。
审议该递推关系的显示,即使对于恒定的输入单元将产生一个复杂的输出秒杀火车图案的信息编码在峰峰结构。
这与McClurkin等人的研究结果。
[15] 每个色调都有自己的脉冲时间信号结构,的实验结果,是很难解释,否则。
此外,时间信号还包含有关信息从它的起源细胞的结构,信息的可以被看作是该细胞或细胞类型的识别码。
最后,由于通过Bressloff说明[22]的细胞反应是输入的时间信号的时域卷积和细胞的响应函数是一个无法回避的结论,即真正的神经元有生成,发送,接收的能力,和响应信息承载时间信号。
1)耦合振荡器:这是更普遍和复杂包含其他两个振荡信号发生器作为特殊情况。
输出信号可以是接近纯谐波功能,倾斜的周期函数被boundarymatched 指数衰减/激励,或尖锐,穗状功能,所有依赖于模型参数的选择和响应功能。
它们由两个“细胞”,每个具有其自身的响应函数。
第一个单元格接收系统的输入,然后发送其输出到所述第二小区的依次将信号发送回第一单元。