Dynamic reconfiguration of sub-optimal parallel query execution plans
Toward green and soft a 5G perspective
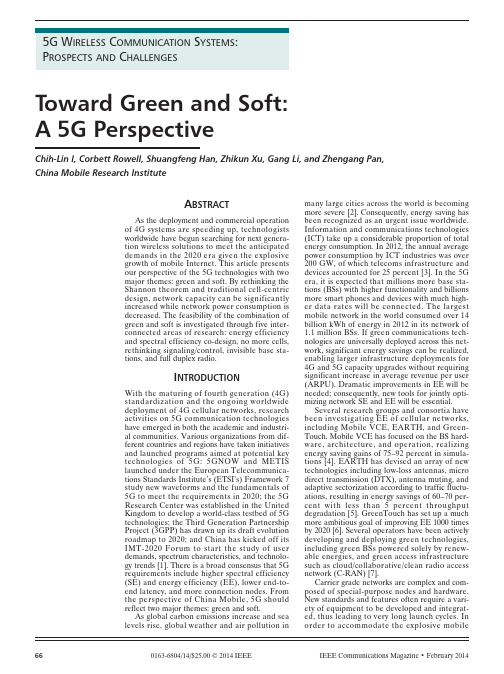
I NTRODUCTIONWith the maturing of fourth generation (4G)standardization and the ongoing worldwide deployment of 4G cellular networks, research activities on 5G communication technologies have emerged in both the academic and industri-al communities. Various organizations from dif-ferent countries and regions have taken initiatives and launched programs aimed at potential key technologies of 5G: 5GNOW and METI S launched under the European Telecommunica-tions Standards Institute’s (ETSI’s) Framework 7study new waveforms and the fundamentals of 5G to meet the requirements in 2020; the 5G Research Center was established in the UnitedKingdom to develop a world-class testbed of 5G technologies; the Third Generation Partnership Project (3GPP) has drawn up its draft evolution roadmap to 2020; and China has kicked off its IMT-2020 Forum to start the study of user demands, spectrum characteristics, and technolo-gy trends [1]. There is a broad consensus that 5G requirements include higher spectral efficiency (SE) and energy efficiency (EE), lower end-to-end latency, and more connection nodes. From the perspective of China Mobile, 5G should reflect two major themes: green and soft.As global carbon emissions increase and sea levels rise, global weather and air pollution inmany large cities across the world is becoming more severe [2]. Consequently, energy saving has been recognized as an urgent issue rmation and communications technologies (ICT) take up a considerable proportion of total energy consumption. In 2012, the annual average power consumption by ICT industries was over 200 GW, of which telecoms infrastructure and devices accounted for 25 percent [3]. In the 5G era, it is expected that millions more base sta-tions (BSs) with higher functionality and billions more smart phones and devices with much high-er data rates will be connected. The largest mobile network in the world consumed over 14billion kWh of energy in 2012 in its network of 1.1 million BSs. If green communications tech-nologies are universally deployed across this net-work, significant energy savings can be realized,enabling larger infrastructure deployments for 4G and 5G capacity upgrades without requiring significant increase in average revenue per user (ARPU). Dramatic improvements in EE will be needed; consequently, new tools for jointly opti-mizing network SE and EE will be essential.Several research groups and consortia have been investigating EE of cellular networks,including Mobile VCE, EARTH, and Green-Touch. Mobile VCE has focused on the BS hard-ware, architecture, and operation, realizing energy saving gains of 75–92 percent in simula-tions [4]. EARTH has devised an array of new technologies including low-loss antennas, micro direct transmission (DTX), antenna muting, and adaptive sectorization according to traffic fluctu-ations, resulting in energy savings of 60–70 per-cent with less than 5 percent throughput degradation [5]. GreenTouch has set up a much more ambitious goal of improving EE 1000 times by 2020 [6]. Several operators have been actively developing and deploying green technologies,including green BSs powered solely by renew-able energies, and green access infrastructure such as cloud/collaborative/clean radio access network (C-RAN) [7].Carrier grade networks are complex and com-posed of special-purpose nodes and hardware.New standards and features often require a vari-ety of equipment to be developed and integrat-ed, thus leading to very long launch cycles. In order to accommodate the explosive mobileA BSTRACTAs the deployment and commercial operation of 4G systems are speeding up, technologists worldwide have begun searching for next genera-tion wireless solutions to meet the anticipated demands in the 2020 era given the explosive growth of mobile Internet. This article presents our perspective of the 5G technologies with two major themes: green and soft. By rethinking the Shannon theorem and traditional cell-centric design, network capacity can be significantly increased while network power consumption is decreased. The feasibility of the combination of green and soft is investigated through five inter-connected areas of research: energy efficiency and spectral efficiency co-design, no more cells,rethinking signaling/control, invisible base sta-tions, and full duplex radio.Chih-Lin I, Corbett Rowell, Shuangfeng Han, Zhikun Xu, Gang Li, and Zhengang Pan, China Mobile Research InstituteT oward Green and Soft: A 5G PerspectiveInternet traffic growth and a large number of new applications/services demanding much short-er times to market, much faster turnaround of new network capabilities is required. Dynamic RAN reconfiguration can handle both temporal and spatial domain variation of mobile traffic without overprovisioning homogeneously. Soft technologies are the key to resolve these issues.By separating software and hardware, control plane and data plane, building software over general-purpose processors (GPPs) via program-ming interfaces and virtualization technology, it is possible to achieve lower cost and higher effi-ciency using software defined networks (SDNs) and network functions virtualization (NFV) [8]. The OpenRoad project at Stanford University introduced Open-flow, FlowVisor, and SNMPVi-sor to wireless networks to enhance the control plane. Base station virtualization from NEC con-centrated on slicing radio resources at the medi-um access control (MAC) layer. CloudEPC from Ericsson modified Long Term Evolution (LTE) control plane to control open-flow switches. CellSDN from Alcatel-Lucent considered a logi-cally centralized control plane, and scalable dis-tributed enforcement of quality of service (QoS) and firewall policies in the data plane. C-RAN implements a soft and virtualized BS with multi-ple baseband units (BBUs) integrated as virtual machines on the same server, supporting multi-ple radio access technologies (RATs). A soft end-to-end solution from the core network to the RAN can enable the 5G goals of spectraland energy efficiency.In the following sections, this article will elab-orate on a green and soft 5G vision. In additionto the traditional emphasis on maximizing SE,EE must be positioned side by side for jointoptimization. We present an EE and SE co-design framework. The concept of no more cellsis highlighted later with user-centric design andC-RAN as key elements of a soft cell infra-structure. The rationale for a fundamentalrethinking of signaling and control design in 5Gis provided. This article further discusses theidea of invisible BSs incorporating line-sideanswer supervision (LSAS) technology. Finally,the fundamental interference management issuesin networks based on full duplex technologiesand potential solutions are identified; we thensummarize this article.R ETHINK S HANNON:EE AND SE C O-D ESIGNGiven limited spectrum and ever increasingcapacity demand, SE has been pursued fordecades as the top design priority of all majorwireless standards, ranging from cellular net-works to local and personal area networks. Thecellular data rate has been improved from kilo-bits per second in 2G to gigabits per second in4G. SE-oriented designs, however, have over-looked the issues of infrastructure power con-sumption. Currently, RANs consume 70 percentof the total power. In contrast to the exponentialgrowth of traffic volume on mobile Internet,both the associated revenue growth and the net-work EE improvement lag by orders of magni-tude. A sustainable future wireless network musttherefore be not only spectral efficient but alsoenergy efficient. Therefore EE and SE jointoptimization is a critical part of 5G research.Looking at traditional cellular systems, thereare many opportunities to become greener, fromequipment level such as more efficient poweramplifiers using envelop tracking, to networklevel such as dynamic operation in line with traf-fic variations both in time and space. For funda-mental principles of EE and SE co-design, onemust first revisit the classic Shannon theory andreformulate it in terms of EE and SE.In classic Shannon theory, the channel capac-ity is a function of the log of the transmit power(P t), noise power spectral density (N0), and sys-tem bandwidth (W). The total system power con-sumption is a sum of P t and the circuit power P c,that is,where r is power amplifier (PA) efficiencydefined as the ratio of the input of the PA to theoutput of the PA. From the definition of EE [9],EE is equal to the channel capacity normalizedby the system power consumption. SE is thechannel capacity normalized by system band-width. The relationship of EE and SE can beshown as a function of PA efficiency and P c inFig. 1a. From Fig. 1a, it can be observed thatwhen P c is zero, there is a monotonic trade-offbetween h EE and h SE as predicted by the classicShannon theory. For nonzero P c, however, h EEincreases in the low SE region and decreases inthe high SE region with h SE(for a given h EE,there are two values of h SE). As P c increases, theEE-SE curve appears flatter. Furthermore, whentaking the derivative of h EE over h SE, the maxi-mum EE (h*EE) and its corresponding SE (h*SE)then satisfy the following: log2h*EE= log2r/(N0ln2) –h*SE. This means there is a linear relation-ship between log2h*EE and h*SE, and the EE-SErelationship at the EE optimal points is indepen-dent of P c. This observation implies that as P cdecreases, an exponential EE gain may beobtained at the cost of linear SE loss.Figure 1b compares the EE-SE performanceof current Global System for Mobile Communi-cations (GSM) and LTE BSs. LTE performs bet-ter than GSM in terms of both SE and EE; both,however, are working in a low SE region, indi-cating room for improvement.Antenna muting is proposed in EARTH toimprove EE, while LSAS stipulates EE improve-ment by increasing the number of antennas. Theseemingly contradicting conclusions are actuallyconsistent with the analysis presented abovewhere the difference is that the former operatesin a low SE region, whereas the latter operatesin a high SE region.While some progress has been made in EEand SE co-design investigation, there is still along way to go to develop a unified frameworkand comprehensive understanding in this area.Ideally, the EE-SE curve in future systemsshould achieve the following criteria:•The EE value should be improved for eachSE operation point.ρ=+PPP,tottcAngle φ (degrees)Angle θ (degrees)80604020040806020806040200020406080arrival/angle of departure (AoA/AoD) and large-scale fading with regard to different antennas are assumed to be the same due to the regular spacing in the traditional 2D array. With irregu-lar antenna arrays, however, the spacing and rel-ative position of each antenna may invalidate the above assumption where AoA /AoD and large-scale fading may be different for each ray with regard to different LSAS antennas; therefore, modification to the current channel modeling is needed.F ULL D UPLEX R ADIOCurrent cellular systems are either frequency-division duplex (FDD) or TDD. To double SE as well as improve EE, full duplex operation should be considered for 5G. A full duplex BS transmits to and receives from different termi-nals simultaneously using the same frequency resource. Self-interference cancellation is the key to the success of a full duplex system since high DL interference will make the receivers unable to detect the UL signal. Significant research progress has been made recently in self-interference cancellation technologies, including antenna placement, orthogonal polarizations, analog cancellation, and digital cancellation [13]. Most of the research, however, has been on either point-to-point relay or a single-cell BS scenario. There is also inter-user UL-to-DL interference in the single-cell full duplex system.To mitigate such interference, the inter-userinterference channel must be measured andreported. The full duplex BS can then scheduleproper UL and DL user pairs, possibly with jointpower control.In the case of a multi-cell full duplex network,interference management becomes significantlymore complex. For current TDD or FDD sys-tems, the DL-to-DL interference received at UEand UL-to-UL interference received at BSs havebeen studied extensively in literature and stan-dardization bodies (e.g., CoMP in 3GPP LTE-Advanced and IEEE 802.16m). In a full duplexsystem, however, there are new interference situ-ations. For example, if there are two BSs, therewill be additional interference in the UL and DLbetween multiple UE mobile devices with thesame frequency and time resources. In additionto intracell interference, there are inter-BS DL-to UL-interference and intercell inter-user UL-to-DL interference. These additional types ofinterference will adversely impact full duplex sys-tem performance. Traditional transmit or receivebeamforming schemes can be applied to mitigateinter-BS DL-to-UL interference. The intracellinterference mitigation can be extended to han-dle intercell inter-user UL-to-DL interference.C ONCLUSIONSThis article has presented five promising areasof research targeting a green and soft 5G system.The fundamental differences between classicShannon theory and practical systems are firstidentified and then harmonized into a frame-work for EE-SE co-design. The characteristics ofno more cells are described from the perspectiveof infrastructure and architecture variations withparticular emphasis on C-RAN as a typical real-ization in order to enable various soft technolo-gies. Rethinking signaling/control based ondiverse traffic profiles and network loading isthen explored, and initial redesign mechanismsand results are discussed. Virtually invisible basestations with irregular LSAS array are envi-sioned to provide much larger capacity at lowerpower in high-density areas when integrated intobuilding signage. Optimal configuration oftransceivers and active antennas is investigatedin terms of EE-SE performance. Finally, newinterference scenarios are identified in fullduplex networks, and several candidate solutionsare discussed. These five areas provide potentialfor fundamental breakthroughs, and togetherwith achievements in other research areas, theywill lead to a revolutionary new generation ofstandards suitable for 2020 5G deployment.A CKNOWLEDGMENTThe authors would like to express gratitude toYami Chen, Jiqing Ni, Chengkang Pan, HualeiWang, and Sen Wang in the Green ResearchCommunication Center of the China MobileResearch Institute.R EFERENCES[1] .[2] .[3] T. C. Group, “Smart 2020: Enabling the Low CarbonEconomy in the Information Age,” 2008.[4] .[5] P. Skillermark and P. Frenger, “Enhancing Energy Effi-ciency in LTE with Antenna Muting,” IEEE VTC Spring’12, 2012, May 2012, pp. 1–9.[6] .[7] C. M. R. Institute, “C-RAN: The Road Towards GreenRAN,” Oct. 2011, available: /cran.[8] M. Chiosi, D. Clarke, and P. Willis, “Network FunctionsVirtualization,” Oct. 2012.[9] G. Y. Li et al., “Energy-Efficient Wireless Communica-tions: Tutorial, Survey, and Open Issues,” IEEE WirelessCommun., vol. 18, no. 6, Dec. 2011, pp. 28–35.[10] M. Gupta et al., “Energy Impact of Emerging MobileInternet Applications on LTE Networks: Issues and Solu-tions,” IEEE Commun. Mag., vol. 51, no. 2, Feb. 2013,pp. 90–97.[11] F. Rusek et al., “Scaling Up MIMO: Opportunities andChallenges with Very Large Arrays,” IEEE Signal Proc.Mag., vol. 30, no. 1, Jan. 2013, pp. 40–60.[12] T. Marzetta, “Noncooperative Cellular Wireless withUnlimited Numbers of Base Station Antennas,” IEEETrans. Wireless Commun., vol. 9, no. 11, Nov. 2010,pp. 3590–3600.[13] E. Aryafar et al., “Midu: Enabling MIMO Full Duplex,”Proc. ACM Mobicom ’12, 2012.B IOGRAPHIESC HIH L IN I(icl@) received her Ph.D. degreein electrical engineering from Stanford University and hasalmost 30 years experience in wireless communications.She has worked at various world-class companies andresearch institutes, including the Wireless CommunicationFundamental Research Department of AT&T Bell Labs; theheadquarters of AT&T, as director of Wireless Communica-tions Infrastructure and Access Technology; ITRI of Taiwan,as director of Wireless Communication Technology; HongKong ASTRI, as vice president and founding group directorof the Communications Technology Domain. She receivedthe IEEE Transactions on Communications Stephen RiceBest Paper Award and is a winner of the CCCP National1000 Talent program. Currently, she is China Mobile’s chiefscientist of wireless technologies in charge of advancedwireless communication R&D efforts of the China MobileResearch Institute. She established the Green Communica-tions Research Center of China Mobile, spearheading majorinitiatives including 5G key technologies R&D; high energyefficiency system architecture, technologies, and devices;green energy; and C-RAN and soft base stations. She was an elected Board Member of IEEE ComSoc, Chair of the ComSoc Meetings and Conferences Board, and Founding Chair of the IEEE WCNC Steering Committee. She is cur-rently an Executive Board Member of GreenTouch and a Network Operator Council Member of ETSI NFV. Her research interests are green communications, C-RAN, net-work convergence, bandwidth refarming, EE-SE co-design,massive MIMO, and active antenna arrays.C ORBETT R OWELL (corbettrowell@) received his B.A. degree (honors) in physics from the University of California Santa Cruz, his M.Phil. degree in electrical and electronic engineering from Hong Kong University of Sci-ence and Technology, and his Ph.D. degree in electrical and electronic engineering from Hong Kong University. He has worked extensively in industry with experience inside startups, research institutes, antenna manufacturers, and operators, designing a wide variety of products including cellular antennas, digital repeaters, radio units, MRI, NFC,MIMO, and base station RF systems. Currently, he is the research director of Antenna and RF Systems in the Green Communication Research Center at the China Mobile Research Institute in Beijing and is designing large-scale antenna systems for TD-LTE and future 5G systems. He has over 30 granted patents and 20 published papers with over 1300 citations. His research interests are digital RF,FPGA, miniature antennas, antenna arrays, active antennas,beamforming, isolation, and sensor arrays.SHUANGFENG H AN (hanshuangfeng@)received his M.S. and Ph.D. degrees in electrical engineer-ing from Tsinghua University in 2002 and 2006 respective-ly. He joined Samsung Electronics in Korea as a senior research engineer in 2006 working on MultiBS MIMO,MIMO codebook design, small cell/HetNet, millimeter wave communication, D2D, and distributed radio over fiber. Cur-rently he is a senior project manager in the Green Commu-nication Research Center at the China Mobile Research Institute. He has over 30 patent applications, and has pub-lished over 10 conference and journal papers. His research interests include green technologies R&D in 5G wireless communication systems, including large-scale antenna sys-tems, active antenna systems, a co-frequency co-time full duplex non-orthogonal multiple access scheme, and EE-SE co-design.Z HIKUN X U (xuzhikun@) received B.S.E. and Ph.D. degrees in electrical and computer engineering from Beihang University in 2007 and 2013, respectively. He was a visiting researcher in the School of Electrical and Com-puter Engineering, Georgia Institute of Technology, from 2009 to 2010. After graduation, he joined the Green Com-munication Research Center of the China Mobile Research Institute as a project manager. His current interests include green technologies, the fundamental relationships between energy efficiency and spectral efficiency, energy-efficient network deployment and operation, cross-layer resource allocation in cellular networks, and advanced signal pro-cessing and transmission techniques.G ANG L I (ligangyf@) received his B.A.degree in telecommunication engineering and M.E. degree in automation engineering from Sichuan University. After graduation, he worked for Lucent Technologies for four years as a team leader and software developer for the core network. He is now a senior researcher at the Green Com-munication Research Center of the China Mobile Research Institute and is working on the key technologies of next generation 5G wireless communication systems. His research interests include radio access network architecture optimization, service-aware signaling/control redesign, and radio and core network convergence.Z HENGANG P AN (panzhengang@) received his B.S.E. from Southeast University in Nanjing and his Ph.D. degree in electrical and electronic engineering from Hong Kong University. After graduation, he worked for NTT DoCoMo Beijing Communication Labs and ASTRI in Hong Kong working on wireless communication (WiFi, WiMax,LTE), mobile digital TV (T-DMB, DVB-T/H, CMMB), and wire-line broadband access (HomePlug, MoCA) for both sys-tem/algorithm design and terminal SoC chip implementation. He is currently a principal staff member of the Green Communication Research Center at the China Mobile Research Institute and is now leading a team work-ing on the key technologies for the next generation 5G wireless communication systems. He has published more than 40 papers in top journals and international confer-ences, and filed 45 patents (20 granted). His research inter-ests are time/frequency/sampling synchronization technology for OFDM/A-based systems, channel estimation,forward error correction coding, MIMO, space-time pro-cessing/coding, and cross-layer optimization.。
Autodesk Nastran 2022 用户手册说明书
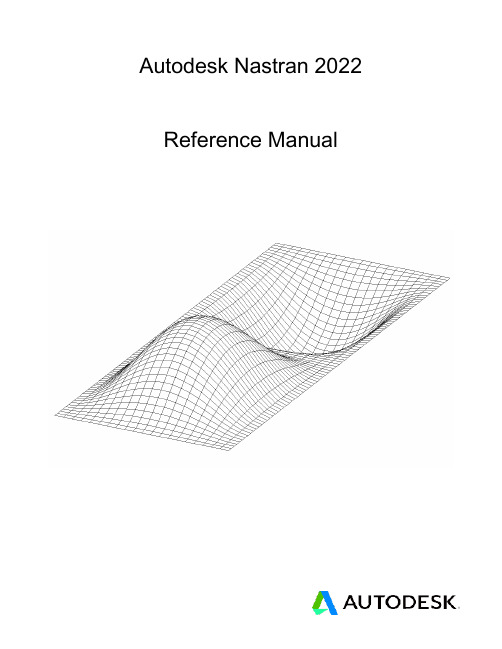
MPA, MPI (design/logo), MPX (design/logo), MPX, Mudbox, Navisworks, ObjectARX, ObjectDBX, Opticore, Pixlr, Pixlr-o-matic, Productstream,
Publisher 360, RasterDWG, RealDWG, ReCap, ReCap 360, Remote, Revit LT, Revit, RiverCAD, Robot, Scaleform, Showcase, Showcase 360,
TrueConvert, DWG TrueView, DWGX, DXF, Ecotect, Ember, ESTmep, Evolver, FABmep, Face Robot, FBX, Fempro, Fire, Flame, Flare, Flint,
ForceEffect, FormIt, Freewheel, Fusion 360, Glue, Green Building Studio, Heidi, Homestyler, HumanIK, i-drop, ImageModeler, Incinerator, Inferno,
Autodesk Nastran 2022
Reference Manual
Nastran Solver Reference Manual
fpga 矩阵运算

fpga 矩阵运算Openn WPFGF"FPGA Matrix Operations: Revolutionizing High-Performance Computing"IntroductionField-Programmable Gate Arrays (FPGAs) have emerged as a game-changer in the world of high-performance computing. With their ability to parallelize complex computations, FPGAs excel at matrix operations - a fundamental task in various domains, such as image processing, machine learning, and scientific simulations. In this article, we will explore the benefits and challenges ofFPGA-based matrix operations, step-by-step, shedding light on the key concepts and techniques involved.1. Understanding FPGAsFPGAs are integrated circuits that allow users to create custom digital circuits by configuring their structure and functionality. Unlike traditional CPUs or GPUs, FPGAs offer a high degree offlexibility since their circuits can be reconfigured on-the-fly. This flexibility is what makes FPGAs a valuable tool for matrix operations, as matrices can be efficiently parallelized across the FPGA's logic gates.2. Why Matrix Operations?Matrix operations form the foundation of many computational tasks. They involve manipulating multiple matrix values, e.g., multiplication, addition, or decomposition. However, performing these operations on large matrices can be computationally demanding and time-consuming. FPGAs provide a significant advantage by enabling the parallel processing of matrix operations, resulting in significant speed-ups compared to traditional processors.3. Matrix Multiplication on FPGAMatrix multiplication is a central operation used in many scientific and engineering applications. Let's discuss a step-by-step process for implementing matrix multiplication on an FPGA:a. Matrix Partitioning: Divide the input matrices into smallersub-matrices, each fitting in the FPGA's memory. This partitioning allows efficient memory access during the computation.b. Memory Allocation: Allocate sufficient on-chip memory in the FPGA to store the input matrices. Specialized memory controllers ensure high-speed access to these on-chip memories.c. Parallel Processing: Assign each FPGA logic gate to compute a specific portion of the resulting matrix. This parallelism significantly speeds up the computation.d. Dataflow and Pipelining: Optimize the data flow within the FPGA by using pipelining techniques. This ensures efficient utilization of resources and maximizes throughput.e. Memory Coalescing: Arrange the memory accesses in a way that minimizes data transfers between the FPGA and external memory, reducing latency and improving performance.4. Challenges in FPGA Matrix OperationsWhile FPGA-based matrix operations offer great potential, they also present several challenges that need to be addressed:a. Design Complexity: FPGA design requires expertise in hardware description languages (HDLs) and low-level circuit design. Developing optimal FPGA implementations for matrix operations demands considerable knowledge and experience.b. High Energy Consumption: FPGAs can consume more power compared to CPUs or GPUs due to their inherently parallel nature. Efficient power management techniques are required to mitigate this issue and optimize energy usage.c. Memory Constraints: FPGAs have limited on-chip memory compared to off-chip memory. Memory partitioning and coalescing techniques are necessary to optimize memory utilization and minimize data transfers.d. Programming Model: Unlike CPUs and GPUs with standardized programming models such as OpenCL or CUDA, FPGAs require specialized programming languages like VHDL or Verilog. This uniqueness demands specialized FPGA programming skills.5. Future DirectionsFPGA-based matrix operations have already shown remarkable progress, but there is still room for innovation and improvement. Some future directions in this field include:a. Higher-Level Abstraction: Developing higher-level programming abstractions for FPGAs can simplify the design process and enable non-experts to leverage FPGA's power for matrix operations.b. Dynamic Reconfiguration: Exploiting FPGA's reconfigurability during runtime can enhance performance by dynamically adjusting hardware components according to the specific matrix operation requirements.c. Machine Learning Acceleration: FPGAs can significantly accelerate machine learning algorithms, which heavily rely on matrix operations. Developing specialized IP cores and frameworks for machine learning on FPGAs will be an area of focus.d. Hybrid Architectures: Combining FPGAs with other specializedprocessors, such as GPUs or ASICs, can create hybrid architectures that leverage the strengths of each technology, enabling more efficient and scalable matrix operations.ConclusionFPGA-based matrix operations have revolutionizedhigh-performance computing by parallelizing complex computations and achieving significant speed-ups. Although challenges exist, ongoing research and innovations promise to overcome these barriers and make FPGA-based matrix operations even more accessible and efficient. As the demand for computation-intensive tasks continues to grow, FPGAs will undoubtedly play an essential role in meeting these requirements and driving future advancements.。
复杂产品的六性指标分析与设计研究

0引言复杂产品是指客户需求复杂、组成复杂、技术复杂、制造过程复杂等类型的产品,其应用非常广泛。
复杂产品的使用环境等需求对其可靠性、维修性、测试性、保障性、安全性和环境适应性等特性(以下简称六性)提出了更高的要求,如何以六性为抓手实施复杂产品的研制全过程管理就成为一项重要问题。
复杂产品可靠性是在规定的时间和条件下完成规定功能的能力。
谭尧等针对复杂系统验收试验前存在多种形式专家信息,考虑系统寿命服从威布尔分布的情况,结合专家信息来计算产品在给定试验方案下的两类风险,得到试验时间较短、风险可控的试验方案[1]。
贾祥考虑不同类型和不同形式的专家经验,通过验前矩拟合的方法将其转化为产品寿命分布参数的验前分布,求得数据融合后产品的可靠度和剩余寿命等可靠性评估结果[2]。
翟亚利针对受不同因素影响导致性能逐步退化的产品,基于扩散过程和累积失效理论,建立多种退化机理作用下产品性能指标退化模型,给出模型中参数的估计方法和性能退化产品可靠度估计方法[3]。
贾详提出了一个基于信息熵函数和Bayes 理论的产品可靠性评估方法,评估结果的精度也优于现有方法[4]。
Mi 等运用故障树和蒙特卡洛模拟等方法研究了复杂系统的可靠性评估问题[5]。
Lavorato 等运用人工神经网络和灰色关联法提出了预测配电设施可靠性综合评估模型[6]。
Wu 提出了一种间接的概率模型用于多体结构可靠性评估[7]。
维修性是在规定的条件下和规定的时间内按照规定的程序和方法进行维修的时候复杂产品保持或恢复到规定状态的能力。
周震愚为了在产品设计中系统全面准确地反映用户需求,提高维修性要求与产品设计特征之间的关联性,提出维修需求到维修性要求再到产品设计特征的规范化映射方法[8]。
徐廷学等分别建立了前期试验阶段维修时间信息向现场试验信息折合的内积模型和相似装备维修时间信息向待评装备维修时间信息折合的线性模型[9]。
韩朝帅等提出构建了基于虚拟现实的产品维修性定量指标验证系统[10]。
转型咨询的术语英文

转型咨询的术语英文Terminology in Transformation ConsultingTransformation consulting is a dynamic field that encompasses a wide range of strategies and methodologies aimed at helping organizations navigate the complexities of change. As the business landscape continues to evolve, the need for effective transformation strategies has become increasingly crucial. To navigate this landscape, professionals in the field of transformation consulting have developed a unique set of terminology that serves to facilitate communication, enhance understanding, and enable the successful implementation of change initiatives.One of the core concepts in transformation consulting is the idea of "organizational transformation." This refers to the comprehensive and strategic process of aligning an organization's people, processes, and technologies to achieve a desired future state. This often involves a deep examination of the organization's current state, the identification of areas for improvement, and the development of a tailored plan to drive the necessary changes.Another key term in the transformation consulting lexicon is "changemanagement." This discipline focuses on the human aspects of organizational change, addressing the psychological and emotional reactions that individuals may experience during periods of transition. Effective change management strategies aim to minimize resistance, foster buy-in, and ensure that the desired outcomes are achieved.The concept of "digital transformation" has also become increasingly prominent in the field of transformation consulting. This refers to the integration of digital technologies, such as cloud computing, big data analytics, and artificial intelligence, to fundamentally alter the way an organization operates and delivers value to its stakeholders. Digital transformation often requires a comprehensive review of an organization's existing processes, systems, and capabilities, and the development of a strategic roadmap to leverage digital technologies for competitive advantage.Closely related to digital transformation is the notion of "business process optimization." This involves the analysis and refinement of an organization's core business processes to improve efficiency, reduce costs, and enhance overall performance. Transformation consultants often employ process mapping, workflow analysis, and automation techniques to identify and address areas for improvement.Another important term in transformation consulting is "organizational culture." This refers to the shared beliefs, values, and behaviors that shape the way an organization functions. Transformation initiatives often require a deep understanding of the existing organizational culture and the development of strategies to align it with the desired future state.The concept of "stakeholder engagement" is also crucial in transformation consulting. This involves the identification, analysis, and active involvement of all individuals and groups who are impacted by or have a vested interest in the change initiative. Effective stakeholder engagement helps to ensure that the transformation process is inclusive, collaborative, and responsive to the needs of all affected parties.Closely related to stakeholder engagement is the idea of "change readiness." This refers to the assessment of an organization's preparedness to undertake a transformation initiative, taking into account factors such as leadership support, employee buy-in, and the availability of necessary resources. Transformation consultants often utilize various assessment tools and frameworks to gauge an organization's change readiness and develop targeted strategies to address any identified gaps.Another key term in the transformation consulting lexicon is "agiletransformation." This approach emphasizes the adoption of agile methodologies, such as Scrum and Kanban, to drive organizational change in a more iterative and responsive manner. Agile transformation focuses on the rapid prototyping, testing, and refinement of change initiatives, allowing organizations to adapt to evolving market conditions and stakeholder needs.The concept of "organizational design" is also crucial in transformation consulting. This involves the strategic reconfiguration of an organization's structure, roles, and responsibilities to align with the desired future state. Transformation consultants often work closely with clients to develop optimal organizational designs that support the achievement of strategic objectives.Finally, the term "transformation roadmap" is used to describe the comprehensive plan that outlines the steps, timelines, and resources required to successfully execute a transformation initiative. This roadmap serves as a guiding framework for the change process, ensuring that all stakeholders are aligned and that progress is monitored and measured effectively.In conclusion, the field of transformation consulting is characterized by a rich and diverse set of terminology that reflects the complexity and nuance of organizational change. By understanding and applying these key concepts, transformation consultants can betternavigate the challenges and opportunities that arise in their work, ultimately helping their clients achieve their desired outcomes and thrive in an ever-changing business landscape.。
有关现值汉密尔顿函数的注解
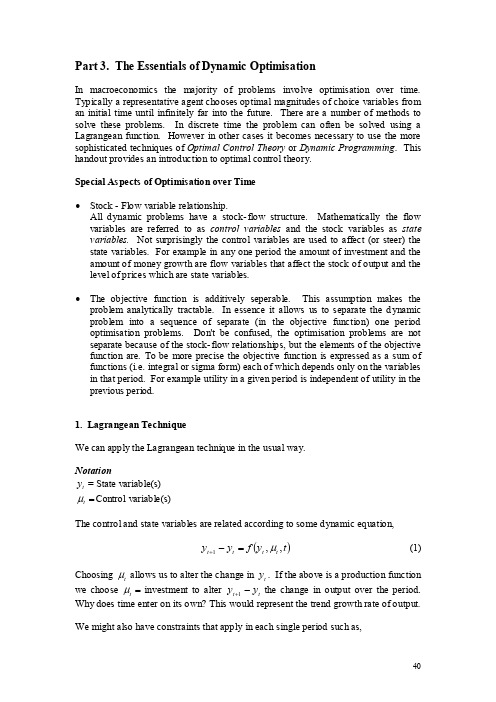
Part 3. The Essentials of Dynamic OptimisationIn macroeconomics the majority of problems involve optimisation over time. Typically a representative agent chooses optimal magnitudes of choice variables from an initial time until infinitely far into the future. There are a number of methods to solve these problems. In discrete time the problem can often be solved using a Lagrangean function. However in other cases it becomes necessary to use the more sophisticated techniques of Optimal Control Theory or Dynamic Programming . This handout provides an introduction to optimal control theory.Special Aspects of Optimisation over Time∙ Stock - Flow variable relationship.All dynamic problems have a stock-flow structure. Mathematically the flow variables are referred to as control variables and the stock variables as state variables. Not surprisingly the control variables are used to affect (or steer) the state variables. For example in any one period the amount of investment and the amount of money growth are flow variables that affect the stock of output and the level of prices which are state variables.∙ The objective function is additively seperable. This assumption makes the problem analytically tractable. In essence it allows us to separate the d ynamic problem into a sequence of separate (in the objective function) one period optimisation problems. Don't be confused, the optimisation problems are not separate because of the stock-flow relationships, but the elements of the objective function are. To be more precise the objective function is expressed as a sum of functions (i.e. integral or sigma form) each of which depends only on the variables in that period. For example utility in a given period is independent of utility in the previous period.1. Lagrangean TechniqueWe can apply the Lagrangean technique in the usual way.Notationt y = State variable(s) =t μControl variable(s)The control and state variables are related according to some dynamic equation,()t y f y y t t t t ,,1μ=-+ (1)Choosing t μ allows us to alter the change in t y . If the above is a production function we choose =t μ investment to alter t t y y -+1 the change in output over the period. Why does time enter on its own? This would represent the trend growth rate of output.We might also have constraints that apply in each single period such as,()0,,≤t y G t t μ(2)The objective function in discrete time is of the form,()∑=Tt ttt y F 0,,μ(3)The first order conditions with respect to t y are,1. Optimal Control TheorySuppose that our objective is maximise the discounted utility from the use of an exhaustible resource over a given time interval. In order to optimise we would have to choose the optimal rate of extraction. That is we would solve the following problem,()()dt e E S U Max t TEρ-⎰0subject to,()t E dtdS-= )0(S S =()free T S =Where ()t S denotes the stock of a raw material and ()t E the rate of extraction. By choosing the optimal rate of extraction we can choose the optimal stock of oil at each period of time and so maximise utility. The rate of extraction is called the control variable and the stock of the raw material the state variable. By finding the optimal path for the control variable we can find the optimal path for the state variable. This is how optimal control theory works.The relationship between the stock and the extraction rate is defined by a differential equation (otherwise it would not be a dynamic problem). This differential equation is called the equation of motion . The last two are conditions are boundary conditions. The first tells us the current stock, the last tells us we are free to choose the stock at the end of the period. If utility is always increased by using the raw material this must be zero. Notice that the time period is fixed. This is called a fixed terminal time problem.The Maximum PrincipleIn general our prototype problem is to solve,()dt u y t F V Max Tu⎰=0,,()u y t f ty,,=∂∂ ()00y y =To find the first order conditions that define the extreme values we apply a set of condition known as the maximum principle.Step 1. Form the Hamiltonian function defined as,()()()()u y t f t u y t F u y t H ,,,,,,,λλ+=Step 2. Find,),,,(λu y t H Max uOr if as is usual you are looking for an interior solution, apply the weaker condition,0),,,(=∂∂uu y t H λAlong with,()∙=∂∂y u y t H λλ,,,()∙=∂∂λλy u y t H ,,,()0=T λStep 3. Analyse these conditions.Heuristic Proof of the Maximum PrincipleIn this section we can derive the maximum principle , a set of first order conditions that characterise extreme values of the problem under consideration.The basic problem is defined by,()dt u y t F V Max Tu⎰=0,,()u y t f ty,,=∂∂()00y y =To derive the maximum principle we use attempt to solve the problem using the 'Calculus of Variations'. Essentially the approach is as follows. The dynamic problem is to find the optimal time path for ()t y , although that we can use ()t u to steer ()t y . It ought to be obvious that,()()0=∂∂t u t VWill not do. This simply finds the best choice in any one period without regard to any future periods. Think of the trade off between consumption and saving. We need to choose the paths of the control (state) variable that gives us the highest value of the integral subject to the constraints. So we need to optimise in every time period, given the linkages across periods and the constraints. The Calculus of Variations is a way to transform this into a static optimisation problem.To do this let ()*t u denote the optimal path of the control variable and consider each possible path as variations about the optimal path.()()()t P t u t u ε+=*(3)In this case ε is a small number (the maths sense) and ()t P is a perturbing curve. It simply means all paths can be written as variations about the optimal path. Since we can write the control path this way we can also (must) write the path of the state variable and boundary points in the same way.()()()t q t y t y ε+=*(4)T T T ∆+=*ε(5)T T T y y y ∆+=*ε(6)The trick is that all of the possible choice variables that define the integral path are now functions of .ε As ε varies we can vary the whole path including the endpoints so this trick essentially allows us to solve the dynamic problem as a function of ε as a static problem. That is to find the optimum (extreme value) path we choose the value of ε that satisfies,0=∂∂εV(7) given (3) to (6).Since every variable has been written as a function of ε, (7) is the only necessary condition for an optimum that we need. When this condition is applied it yields the various conditions that are referred to as the maximum principle .In order to show this we first rewrite the problem in a way that allows us to include the Hamiltonian function,()()()dt y u y t f t u y t F V Max Tu ⎪⎪⎭⎫ ⎝⎛-+=∙⎰,,,, 0λWe can do this because the term inside the brackets is always zero provided the equation of motion is satisfied. Alternatively as,()()dt y t u y t H V Max Tu∙-=⎰λ0,,(1)Integrating (by parts)1the second term in the integral we obtain,()()()()()T T u y T y dt t y t u y t H V Max λλλ-+⎭⎬⎫⎩⎨⎧+=⎰∙000,,(2)Now we apply the necessary condition (7) given (3) to (6).Recall that to differentiate an Integral by a Parameter we use Leibniz's rule, (page 9). After simplification this yields,()()()()[]()0,,,0=∆-∆+⎭⎬⎫⎩⎨⎧∂∂+⎥⎦⎤⎢⎣⎡+∂∂=∂∂=∙⎰T T t T y T T u y t H dt t p u H t q y H V λλλεε (3)The 3 components of this integral provide the conditions defining the optimum. In particular,()()()⎰=⎭⎬⎫⎩⎨⎧∂∂+⎥⎦⎤⎢⎣⎡+∂∂∙ελT dt t p u Ht q y H 00requires that,∙-=∂∂λyHand 0=∂∂u HWhich is a key part of the maximum principle.The Transversality Condition1Just letdt y y dt y T TT⎰⎰∙∙-=0λλλTo derive the transversality condition we have to analyse the two terms,()[]()0,,,=∆-∆=T Tt y T T u y t H λλFor out prototype problem (fixed terminal time) we must have .0=∆T Therefore the transversality condition is simply that,()0=T λThe first two conditions always apply for 'interior' solutions but the transversality condition has to be defined by the problem at hand. For more on this see Chiang pages 181-184.The Current Value HamiltonianIt is very common in economics to encounter problems in which the objective function includes the following function t e ρ-. It is usually easier to solve these problems using the current value Hamiltonian. For example an optimal consumption problem may have an objective function looking something like,()()dt et C U tρ-∞⎰0Where ρ represents the rate of time discount. In general the Hamiltonian for such problems will be of the form,()()()()u y t f t e u y t F u y t H t ,,,,,,,λλρ+=-The current value Hamiltonian is defined by,()()()u y t f t m u y t F H CV ,,,,+=(1)Where ()()t e t t m ρλ-=. The advantage of the current value Hamiltonian is that the system defined by the first order equations is usually easier to solve. In addition to (1) an alternative is to write,()()()t t CV e u y t f t m e u y t F H ρρ--+=,,,,(2)The Maximum ConditionsWith regard to (2) the first two conditions are unchanged. That is,μμ∂∂=∂∂CVH H and λλ∂∂=∂∂CV H H (3)The third condition is also essentially the same since,∙=∂∂=∂∂λyH y H CVHowever it is usual to write this in terms of ∙m . Since,t t me e m ρρρλ--∙∙-=We can write the third condition as,m yH m CVρ+∂∂-=∙(4)The endpoint can similarly be stated in terms of m since t e m ρλ= the conditio n ()0=T λmeans that,()()0==T e T T m ρλOr,()0=-T e T m ρ(5)Ramsey model of optimal savingIn the macroeconomics class you have the following problem,Choose consumption to maximise,()()dt t c u e B U t t ⎰∞=-=0βWhere ()g θηρβ---=1 subject to the following constraint,()()()()()()t k g t c t k f t k +--=∙ηIn this example ()t c u =, ()t k y =. The Hamiltonian is,()()()()()()()[]g n t c t k f t t C u Be H t +--+=-λβThe basic conditions give us,()()()()0=-'=∂∂-t t c u Be t c Ht λβ (1)()()()()()[]()t g n t k f t t k H λλ =+-'-=∂∂ (2)Plus,()()()()()()t k g t c t k f t k+--=∙η(3)Now we must solve these. Differentiate the right-hand side of (1) with respect to time.This gives you ()t λwhich can then be eliminated from (2). The combined condition can then be rewritten as,()()()()()()[]g t r t c u t c u t cθρ--'''-=This is the Euler equation. If you assume the instantaneous utility function is CRRA as in class and you calculate the derivatives you should get the same expression.。
基于云-边协同的配电网快速供电恢复智能决策方法

第51卷第19期电力系统保护与控制Vol.51 No.19 2023年10月1日Power System Protection and Control Oct. 1, 2023 DOI: 10.19783/ki.pspc.221918基于云-边协同的配电网快速供电恢复智能决策方法蔡田田1,姚 浩1,杨英杰1,张子麒2,冀浩然2,李 鹏2(1.南方电网数字电网研究院有限公司,广东 广州 510700;2.智能电网教育部重点实验室(天津大学),天津 300072)摘要:分布式电源高渗透率接入对配电网故障自愈能力提出了更高的要求。
基于模型的供电恢复方法利用精准的网络参数构建优化模型,可以实现供电恢复策略的准确制定。
但在配电网实际运行中,精准的配电网络参数往往难以获取,导致基于模型的供电恢复方法应用受限。
云-边协同运行模式可作为配电网快速供电恢复的一种实现方案。
提出一种基于云-边协同的配电网快速供电恢复智能决策方法。
首先,在云端基于图卷积神经网络建立配电网快速供电恢复智能决策模型,包括网络重构模块和潮流模拟模块。
当故障发生后,云端利用网络重构模块,快速制定网络重构策略,经过破圈法/避圈法验校后下发至配电网边缘侧的边缘计算装置。
边缘侧根据云端的网络重构策略利用潮流模拟模块就地制定负荷恢复策略,实现系统的快速供电恢复。
最后,依托改进的IEEE33节点配电网算例对所提模型进行分析,验证了所提方法可有效提升配电网的供电恢复能力。
关键词:配电网;云-边协同;供电恢复;分布式电源;图卷积神经网络Cloud-edge collaboration-based supply restoration intelligent decision-making methodCAI Tiantian1, YAO Hao1, YANG Yingjie1, ZHANG Ziqi2, JI Haoran2, LI Peng2(1. Digital Grid Research Institute, China Southern Power Grid, Guangzhou 510700, China; 2. Key Laboratory ofSmart Grid of Ministry of Education (Tianjin University), Tianjin 300072, China) Abstract: The high-penetration integration of distributed generators (DGs) makes higher demands on the self-healing ability of a distribution network. The model-based supply restoration methods build the optimization model with accurate network parameters, which can realize the accurate formulation of restoration strategies. However, the accurate network parameters are often difficult to acquire in practical operation, which may limit the application of the model-based methods. The cloud-edge collaboration control mode can be used as an implementation scheme for fast supply restoration.A fast supply restoration intelligent decision-making method for distribution network based on cloud-edge collaborationis proposed. First, an intelligent decision-making model is established based on a graph convolutional neural network (GCN) on the cloud, containing network reconstruction and power flow simulation modules. When a failure occurs, the network reconstruction module is used to customize the reconstruction strategy on the cloud. After correction by loop-breaking/loop-avoiding method, the reconstruction strategy will be sent to the edge calculation device of distribution network edge side. With the power flow simulation module, the supply recovery strategy can be determined rapidly at the edge side to realize a fast supply restoration. Finally, the proposed strategy is analyzed using the modified IEEE 33-node system. The results show that the proposed method can effectively improve the supply restoration ability of a distribution network.This work is supported by the National Key Research and Development Program of China (No. 2020YFB0906000 and No. 2020YFB0906002).Key words: distribution network; cloud-edge collaboration; supply restoration; distributed generators (DGs); graph convolutional neural networks (GCN)0 引言配电网中设备种类繁多、控制策略复杂[1],尤基金项目:国家重点研发计划项目资助(2020YFB0906000,2020YFB0906002) 其是当分布式电源(distributed generators, DGs)高渗透率接入后,配电网的运行特性发生巨大变化[2],对配电网故障自愈能力提出了更高的要求[3]。
基于双指标有序时段划分的配电网动态重构

3 动态重构时段划分
3. 1 单指标不足
由于每个时段对应一条节点负荷曲线ꎬ因此曲
线聚类结果即重构时段划分结果ꎮ 聚类分析是将一
文提出一种同时考虑节点负荷曲线幅值和形态相似
群对象根据相似度高低划分为不同类别的过程ꎬ为
性的双指标有序时段划分方法ꎮ 该方法根据节点负
c =1
(6)
式中ꎬh c 和 h c + 1 - 1 表示第 c 个分段的上下界ꎮ
3. 3 双指标有序时段划分
3. 3. 1 目标函数
设重构时段有 m 个基本时段ꎬ每个基本时段内
负荷保持不变ꎬ配电网络有 i 个节点ꎬ则系统在第 h
个时段的负荷状态可以用 X h = [ x hꎬ1 ꎬx hꎬ2 ꎬꎬx hꎬi ꎬ
proposed. Based on the idea of Fisher optimal partition to orderly divide the reconfiguration periodꎬthe node load
curve amplitude and shape similarity are used as the division indexꎬ transforming the dynamic reconfiguration prob ̄
lem of continuous period into the static reconfiguration problem of multiple discrete periods. In view of the common
problems of low optimization efficiency and local convergence in reconfiguration algorithmsꎬ the beetle antennae
含多区域综合能源系统的主动配电网双层博弈优化调度策略
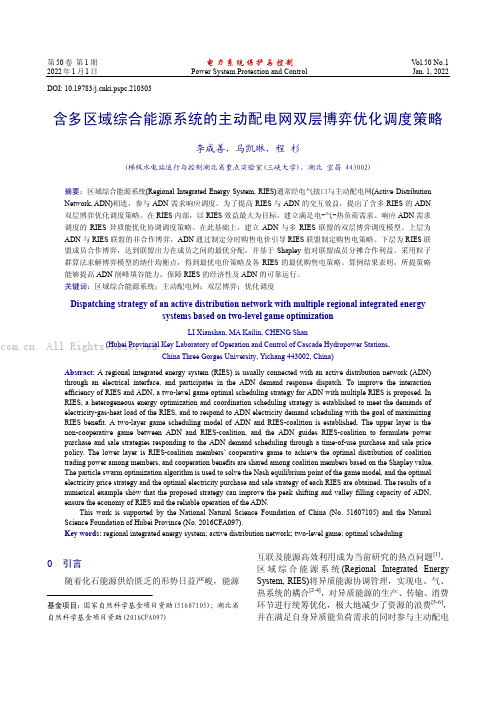
第50卷第1期电力系统保护与控制Vol.50 No.1 2022年1月1日Power System Protection and Control Jan. 1, 2022 DOI: 10.19783/ki.pspc.210303含多区域综合能源系统的主动配电网双层博弈优化调度策略李咸善,马凯琳,程 杉(梯级水电站运行与控制湖北省重点实验室(三峡大学),湖北 宜昌 443002)摘要:区域综合能源系统(Regional Integrated Energy System, RIES)通常经电气接口与主动配电网(Active Distribution Network, ADN)相连,参与ADN需求响应调度。
为了提高RIES与ADN的交互效益,提出了含多RIES的ADN 双层博弈优化调度策略。
在RIES内部,以RIES效益最大为目标,建立满足电-气-热负荷需求、响应ADN需求调度的RIES异质能优化协调调度策略。
在此基础上,建立ADN与多RIES联盟的双层博弈调度模型。
上层为ADN与RIES联盟的非合作博弈,ADN通过制定分时购售电价引导RIES联盟制定购售电策略。
下层为RIES联盟成员合作博弈,达到联盟出力在成员之间的最优分配,并基于Shapley值对联盟成员分摊合作利益。
采用粒子群算法求解博弈模型的纳什均衡点,得到最优电价策略及各RIES的最优购售电策略。
算例结果表明,所提策略能够提高ADN削峰填谷能力,保障RIES的经济性及ADN的可靠运行。
关键词:区域综合能源系统;主动配电网;双层博弈;优化调度Dispatching strategy of an active distribution network with multiple regional integrated energysystems based on two-level game optimizationLI Xianshan, MA Kailin, CHENG Shan(Hubei Provincial Key Laboratory of Operation and Control of Cascade Hydropower Stations,China Three Gorges University, Yichang 443002, China)Abstract: A regional integrated energy system (RIES) is usually connected with an active distribution network (ADN) through an electrical interface, and participates in the ADN demand response dispatch. To improve the interaction efficiency of RIES and ADN, a two-level game optimal scheduling strategy for ADN with multiple RIES is proposed. In RIES, a heterogeneous energy optimization and coordination scheduling strategy is established to meet the demands of electricity-gas-heat load of the RIES, and to respond to ADN electricity demand scheduling with the goal of maximizing RIES benefit. A two-layer game scheduling model of ADN and RIES-coalition is established. The upper layer is the non-cooperative game between ADN and RIES-coalition, and the ADN guides RIES-coalition to formulate power purchase and sale strategies responding to the ADN demand scheduling through a time-of-use purchase and sale price policy. The lower layer is RIES-coalition members’ cooperative game to achieve the optimal distribution of coalition trading power among members, and cooperation benefits are shared among coalition members based on the Shapley value.The particle swarm optimization algorithm is used to solve the Nash equilibrium point of the game model, and the optimal electricity price strategy and the optimal electricity purchase and sale strategy of each RIES are obtained. The results of a numerical example show that the proposed strategy can improve the peak shifting and valley filling capacity of ADN, ensure the economy of RIES and the reliable operation of the ADN.This work is supported by the National Natural Science Foundation of China (No. 51607105) and the Natural Science Foundation of Hubei Province (No. 2016CFA097).Key words: regional integrated energy system; active distribution network;two-level game; optimal scheduling0 引言随着化石能源供给匮乏的形势日益严峻,能源基金项目:国家自然科学基金项目资助(51607105);湖北省自然科学基金项目资助(2016CFA097) 互联及能源高效利用成为当前研究的热点问题[1]。
电力英语文献---配电网络中较少损耗的实际方法
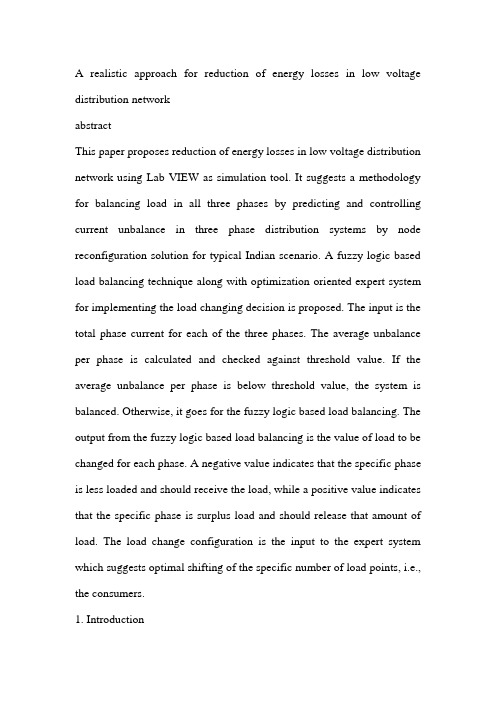
A realistic approach for reduction of energy losses in low voltage distribution networkabstractThis paper proposes reduction of energy losses in low voltage distribution network using Lab VIEW as simulation tool. It suggests a methodology for balancing load in all three phases by predicting and controlling current unbalance in three phase distribution systems by node reconfiguration solution for typical Indian scenario. A fuzzy logic based load balancing technique along with optimization oriented expert system for implementing the load changing decision is proposed. The input is the total phase current for each of the three phases. The average unbalance per phase is calculated and checked against threshold value. If the average unbalance per phase is below threshold value, the system is balanced. Otherwise, it goes for the fuzzy logic based load balancing. The output from the fuzzy logic based load balancing is the value of load to be changed for each phase. A negative value indicates that the specific phase is less loaded and should receive the load, while a positive value indicates that the specific phase is surplus load and should release that amount of load. The load change configuration is the input to the expert system which suggests optimal shifting of the specific number of load points, i.e., the consumers.1. IntroductionAmong three functional areas of electrical utility namely, generation, transmission and distribution, the distribution sector needs more attention as it is very difficult to standardize due to its complexity. Transmission and distribution losses in India have been consistently on the higher side in the range of 21–23%. Out of these losses, 19% is at distribution level in which 14% is contributed by technical losses. This is due to inadequate investments for system improvement work. To reduce technical losses, the important parameters are inadequate reactive compensation, unbalance of current and voltage drops in the system. There are two main distribution network lines namely, primary distribution lines (33 kV/22 kV/11 kV) and secondary distribution lines (415 V line voltage). Primary distribution lines are feeding HT consumers and are regularized by insisting the consumers to maintain power factor of 0.9 and above and their loads in all three phases is mostly balanced. The energy loss control becomes a critical task in secondary distribution network due to the very complex nature of the network.Distribution Transformer caters to the needs of varying consumers namely Domestic, Commercial, Industrial, Public lighting, Agricultural, etc. Nature of load also varies as single phase load and three phase load. The system is dynamic and ever expanding. It requires fast response to changes in load demand, component failures and supply outages. Successful analysis of load data at the distribution level requiresprocedures different from those typically in use at the transmission system level. Several researchers have proposed methods for node reconfiguration in primary distribution network [1–11]. Two types of switches used in primary distribution systems are normally closed switches (sectionalizing switches) and normally open switches (tie switches). Those two types of switches are designed for both protection and configuration management and by altering the open/ closed status of switches loss reduction and optimization of primary distribution network can be achieved. Siti et al. [12] discussed reconfiguration algorithms in secondary distribution network with load connections done via a switching matrix with triacs and hence costly alternative for developing countries. Much work needs to be done in the secondary distribution network where lack of information is an inherent characteristic. For example in most of the developing countries (India, China, Brazil, etc.) the utilities charge the consumers based on their monthly electric energy consumption. It does not reflect the day behaviour of energy consumption and such data are insufficient for distribution system analysis.Conventionally, to reduce the unbalance current in a feeder the load connections are changed manually after field measurement and software analysis. Although this process can improve the phase current unbalance, this strategy is more time consuming and erroneous. The more scientific process of node reconfiguration of LV network which involves thearrangement of loads or transfer of load from heavily loaded area to the less loaded area is needed. This paper focuses on this objective. In the first stage, the energy meter reading from secondary of Distribution Transformer is downloaded and is applied as input to Lab-VIEW based distribution simulation package to study the effects of daily load patterns of a typical low voltage network (secondary distribution network). The next stage is to develop an intelligent model capable of estimating the load unbalance on a low voltage network in any hour of day and suggesting node reconfiguration to balance the currents in all three phases.Objectives are to:Study the daily load pattern of low voltage network of Distribution Transformer by using Lab VIEW.Study the unbalance of current in all three phases and power factor compensation in individual phases.Develop distribution simulation package.The distribution simulation package contains fuzzy logic based load balancing technique and fuzzy expert system to shift the number of consumers from over loaded phase to under loaded phase.2. Existing systemIn the existing system of distribution network, the energymeters are provided for energy accounting, but there is no means of sensingunbalance currents, voltage unbalance and power factor correction requirement for continuous 24 h in three phases of LT feeder. In other words, instantaneous load curves, voltage curves, energy curves and power factor curves for individual three phases are not available for monitoring, analyzing and controlling the LV network. The individual phase of Distribution Transformer could be monitored only by taking reading whenever required and if there is unequal distribution of load in three phases, the consumer loads are shifted from overloaded phase to under loaded phase of distribution LT feeder by the field staff in charge of the Distribution Transformer. There is no scientific methodology at present.3. Proposed systemIn the proposed system, Lab VIEW is used as software simulation tool [13]. In the existing system of distribution network, the Distribution Transformers are fixed with energy meters in the Secondary of the Distribution Transformer and energy meter readings can be downloaded with Common Meter Reading Instrument (CMRI instrument). The energy meter reading includes VI profile and it can be used for the power measurement.4. Monitoring parametersThe phase voltages and the line currents of all three phases are available every half an hour and the voltage curve and load curve are obtained fromthese values. The active, the reactive and the apparent power are computed from these quantities after the phase angle is determined. The following parameters are plotted:1. Individual phase voltage.2. Individual phase current.3. Individual phase active power.4. Individual phase reactive power.5. Individual phase apparent power.6. Individual phase power factor.With the above concepts, the front panel and block diagram are developed for unbalanced three phase loads by downloading the VI profile from energy meter installed in the Distribution Transformer and simulating the setup using practical values. From the actual values obtained load unbalance is predicted using fuzzy logic and node reconfiguration is suggested using expert system.The Lab VIEW front panel displays the VI profile on a particular date with power and energy measurement as in Table 1. The Lab VIEW reads the VI profile and computes the real power, reactive power, apparent power and energy, kWh.4.1. Prediction of current unbalanceThe maximum current consumption in each phase is IRmax, IYmax, and IBmax. The optimum current (Iopt) is given in the following equation:()3max max max B Y R opt I I I I ++=The difference between opt I and m ax R I is then determined. Similarly thedifference between opt I and max Y I , opt I and max B I is computed. If thedifference is positive then that phase is considered as overloaded and if the difference is negative then that phase is considered to be under loaded. If the difference is within the threshold value, then that load is perfectly balanced.To balance the current in three phases, if the difference between opt I and m ax R I is less than threshold value then that phase is left as such.Otherwise, if the difference is greater than threshold value, some of the consumers are suggested reconfiguration from overloaded phase to under loaded phase using expert system.5. Fuzzy based load balancingA fuzzy logic based load balancing technique is proposed along with combinatorial optimization oriented expert system for implementing the load changing decision. The flowchart of the proposed system is shown in Fig. 1. Here the input is the total phase current for each of the three phases. Typical loads on low voltage networks are stochastic by nature. However it has been ensured that there is similarity in stochastic nature throughout the day as seen from load graph of Distribution Transformer as shown in Fig. 6. It has been verified that if R phase is overloaded followed by Y phase and thenB phase the same load pattern continuesthroughout the day.The average unbalance per phase is calculated as (IRmax _ Iopt) for R phase, (IYmax _ Iopt) for Y phase and (IBmax _ Iopt) for B phase and is checked against a threshold value (allowed unbalance current) of 10 A. If the average unbalance per phase is below 10 A, it can be assumed that the system is more or less balanced and discard any further load balancing. Otherwise, it goes for the fuzzy logic based load balancing. The output from the fuzzy logic based load-balancing step is the load change values for each phase.This load change configuration is the input to the expert system, which tries to optimally suggest shifting of specific number of load points. However, sometimes the expert system may not be able to execute the exact amount of load change as directed by the fuzzy step. This is because the actual load points for any phase might not result in a combination which sums up to the exact change value indicated by the fuzzy controller however optimization is achieved because of balancing attempted during peak hours of the day of the load graph.5.1. Fuzzy controller: input and outputTo design the fuzzy controller, at first the input and output variables are to be designed. For the load balancing purpose, the inputs selected are ‘phase current’ i.e., the individual phase current for each of the three phases and optimum current required and the output as ‘change’, i.e., thechange of load (positive or negative) to be made for each phase. For the input variable, Table 2 and Fig. 2 show the fuzzy nomenclature and the triangular fuzzy membership functions. And for the output variable, Table 3 shows the fuzzy nomenclature and Fig. 3 the corresponding triangular fuzzy membership functions.The IF-THEN fuzzy rule set governing the input and output variable is described in Table 4.5.2. Fuzzy expert systemA fuzzy expert system is an expert system that uses a collection of fuzzy membership functions and rules, instead of Boolean logic, to reason out data. The rules in a fuzzy expert system are usually of a form similar to the following:If x is low and y is high then z = mediumwhere x and y are input variables (names for known data values), z is an output variable (a name for a data value to be computed), low is a membership function (fuzzy subset) defined on x, high is a membership function defined on y, and medium is a membership function defined on z .The antecedent (the rule’s premise) describes to what degree the rule applies, while the conclusion (the rule’s consequent) assigns a membership function to each of one or more output variables. Most tools for working with fuzzy expert systems allow more than one conclusion per rule. The set of rules in a fuzzy expert system is known as the rulebase or knowledge base.The load change configuration is the input to the expert system which tries to optimally shift the specific number of load points. The following are the objectives of the expert system:_ Minimum switching._ Minimum losses._ Satisfying the voltage and current constraints.Fg. 4 shows the block diagram of the expert system. The inputs to the expert system are the value added or subtracted to that particular phase from the fuzzy controller and the current consumption of the individual consumers on that particular phase. The expert system should display which of the consumers are to be shifted from the overloaded phase to under loaded phase and also displays the message NO CHANGE if that phase is balanced.6. Simulation resultsTable 1 shows the display of output of Lab-VIEW based power and energy measurement [14]. It asks for the Distribution Transformer secondary reading, date, tolerance value (threshold value), and fuzzy conditioner of three phases for load balancing. It then displays the date, time, voltage, current, power factor, real power, reactive power, apparent power.Fig. 5 shows the line voltage curve for R, Y and B phases. It alsoindicates the voltage drop during peak hours of the day. The current curve for R, Y and B phases is shown in Fig. 6. It indicates the current unbalance in the existing supply network. The load graph from typical Distribution Transformer for entire day indicates interesting similarity in load patterns of consumers. Hence load balancing attempted during peak load band yielded fruitful result for the entire day.Fig. 7 displays the results of fuzzy logic based load balancing technique. Fuzzy toolkit in Lab VIEW is used for simulation. Mamdani fuzzy inference technique is applied and centroid based defuzzication technique is employed in the load balancing system. The output from the fuzzy controller is the value that is to be subtracted or added to a particular phase. The positive value indicates that the specific phase is overloaded and it should release the amount of load. The negative value indicates that the specific phase is under loaded and it should receive the amount of load. The value less than 10 A indicate that phase is perfectly loaded. Fig. 8 show the expert system output for all three phases. It gives the Service connection number (SC No.) and current consumption of individual consumer. The output of the fuzzy controller is applied as the input to the expert system. If the output of the fuzzy controller is a positive value then the expert system should inform which of the consumers are to be shifted from that phase.From Fig. 8, the R phase is overloaded, so the expert system informs thatthe SC No.’s 56 and 23 should be shifted. The output of the fuzzy controller for the Y phase is less than threshold value 10 A so that phase is perfectly loaded. The output of the fuzzy controller for B phase is a negative value; hence it receives the load from R-phase. There is no shifting of consumer in Y phase and B phase therefore the entries are indicated by zero values. There is no switching arrangement in secondary low voltage distribution network in Indian scenario and hence shifting to be done manually.The suggested approach has been tested practically on 70 nodes (70 consumers) low voltage distribution network and results are as shown in Fig. 9 (before balancing) and Fig. 10 (after balancing). Single phase customers physically reconfigured from overloaded phase into under loaded phase and then test results studied. Unbalancing has been observed for 10 days and then balancing attempted. Balanced network was studied and then results obtained. There is a percentage reduction in Energy loss from 9.695% to 8.82% though there is increase in cumulative kWh from 1058.95 to 1065.9. This Distribution Transformer belongs to urban area of a typical Indian city and has 41 single phase consumers and 29 three-phase consumers and three-phase consumers have balanced loads. In rural areas where number of single phase consumers are predominant and scattered around lengthy distribution lines this balancing technique will be much more beneficial than the tested study indicates.This research is significant to the Indian scenario considering the fact that there are 180,763 Distribution Transformers (www.tneb.in) and 2,07,00,000 consumers and length of secondary distribution network 5,17,604 km in one state, Tamil Nadu alone, 1% saving in energy loss per transformer per day will save few crores of rupees for a month to electrical utility.7. ConclusionIn this paper, the complete online monitoring of low voltage distribution network is done by using Lab VIEW and the fuzzy logic based load balancing technique is presented. With the results obtained from Lab VIEW, currents in individual phases are predicted and unbalance pattern is studied without actually measuring instantaneous values from consumer premise.A fuzzy logic based load balancing is implemented to balance the current in three phases and expert system to reconfigure some of the consumers from over loaded phase to under loaded phase. The input to the fuzzy controller is the individual phase current. The output of the fuzzy controller is the load change value, negative value for load receiving and positive value for load releasing. Expert system performs the optimal interchanging of the load points between the releasing and receiving phases.The proposed phase balancing system using fuzzy logic and expertsystem is effective for reducing the phase unbalance in the low voltage secondary distribution network. The energy losses are reduced and efficiency of the distribution network is improved and has been practically studied in typical Distribution Transformer of electrical utility.图一图2图3 图4图5图6图7图8图9图10。
Labview图形化编程语言中英文对照外文翻译文献

Labview图形化编程语⾔中英⽂对照外⽂翻译⽂献中英⽂资料外⽂翻译National Instruments LabVIEW: A Programming Environment for Laboratory Automation and Measurement .National Instruments LabVIEW is a graphical programming language that has its roots in automation control and data acquisition. Its graphical representation, similar to a process flow diagram, was created to provide an intuitive programming environment for scientists and engineers. The language has matured over the last 20 years to become a general purpose programming environment. LabVIEW has several key features which make it a good choice in an automation environment. These include simple network communication, turnkey implementation of common communication protocols (RS232, GPIB, etc.), powerful toolsets for process control and data fitting, fast and easy user interface construction, and an efficient code execution environment. We discuss the merits of the language and provide an example application suite written in-house which is used in integrating and controlling automation platforms.Keywords: NI LabVIEW; graphical programming; system integration; instrument control; component based architecture; robotics; automation; static scheduling; dynamic scheduling; databaseIntroductionCytokinetics is a biopharmaceutical company focused on the discovery of small molecule therapeutics that target the cytoskeleton. Since inception we have developed a robust technology infrastructure to support our drug discovery efforts. The infrastructure provides capacity to screen millions of compounds per year in tests ranging from multiprotein biochemical assays that mimic biological function to automated image-based cellular assays with phenotypic readouts. The requirements for processing these numbers and diversity of assays have mandated deployment of multiple integrated automation systems. For example, we have several platforms for biochemical screening, systems for live cell processing, automated microscopy systems, and an automated compound storage and retrieval system. Each in-house integrated system is designed around a robotic arm and contains an optimal set of plate-processing peripherals (such as pipetting devices, plate readers, and carousels) depending on its intended range of use. To create the most flexible, high performance, and cost-effective systems, we have taken the approach of building our own systems in-house. This has given us the ability to integrate the most appropriate hardware and software solutions regardless of whether they are purchased from a vendor or engineered de novo, and hence we can rapidly modify systems as assay requirements change.To maximize platform consistency and modularity, each of our 10 automated platforms is controlled by a common, distributed application suite that we developed using National Instruments (NI) LabVIEW. This application suite described in detail below, enables our end users to create and manage their own process models (assayscripts) in a common modeling environment, to use these process models on any automation system with the required devices, and allows easy and rapid device reconfiguration. The platform is supported by a central Oracle database and can run either statically or dynamically scheduled processes.NI LabVIEW BackgroundLabVIEW, which stands for Laboratory Virtual Instrumentation Engineering Workbench is a graphical programming language first released in 1986 by National Instruments (Austin, TX). LabVIEW implements a dataflow paradigm in which the code is not written, but rather drawn or represented graphically similar to a flowchart diagram Program execution follows connector wires linking processing nodes together. Each function or routine is stored as a virtual instrument (VI) having three main components: the front panel which is essentially a form containing inputs and controls and can be displayed at run time, a block diagram where the code is edited and represented graphically, and a connector pane which serves as an interface to the VI when it is imbedded as a sub-VI.The top panel (A) shows the front panel of the VI. Input data are passed through “Controls” which are shown to the left. Included here are number inputs, a file path box, and a general error propagation cluster. When the VI runs, the “Indicator”outputs on the right of the panel are populated with output data. In this example, data include numbers (both as scalar and array), a graph, and the output of the error cluster. In the bottom panel (B) the block diagram for the VI is shown. The outer case structure executes in the “No Error” case (VIs can make internal errors o r if called as a sub-VI the caller may propagate an error through the connector pane).Unlike most programming languages, LabVIEW compiles code as it is created thereby providing immediate syntactic and semantic feedback and reducing the time required for development and testing.2Writing code is as simple as dragging and droppingfunctions or VIs from a functions palette onto the block diagram within process structures (such as For Loops, or Case Structures) and wiring terminals (passing input values, or references). Unit testing is simplified because each function is separately encapsulated; input values can be set directly on the front panel without having to test the containing module or create a separate test harness. The functions that generate data take care of managing the storage for the data.NI LabVIEW supports multithreaded application design and executes code in an inherently parallel rather than sequential manner; as soon as a function or sub-VI receives all of its required inputs, it can begin execution. In Figure 1b, all the sub-VIs receive the array input simultaneously as soon as the For Loop is complete, and thus they execute in parallel. This is unique from a typical text-based environment where the control flows line by line within a function. When sequential execution is required, control flow can be enforced by use of structures such as Sequences, Events, or by chaining sub-VIs where output data from one VI is passed to the input of the next VI.Similar to most programming languages, LabVIEW supports all common data types such as integers, floats, strings, and clusters (structures) and can readily interface with external libraries, ActiveX components, and .NET framework. As shown in Figure 1b, each data type is graphically represented by wires of different colors and thickness. LabVIEW also supports common configuration management applications such as Visual SourceSafe making multideveloper projects reasonable to manage.Applications may be compiled as executables or as Dynamic Link Libraries (DLLs) that execute using a run-time engine similar to the Java Runtime Environment. The development environment provides a variety of debugging tools such as break-points, trace (trace), and single-step. Applications can be developed using a variety of design patterns such as Client-Server, Consumer-Producer, andState-Machine. There are also UML (Unified Modeling Language) modeling tools that allow automated generation of code from UML diagrams and state diagrams.Over the years, LabVIEW has matured into a general purpose programming language with a wider user base.NI LabVIEW as a Platform for Automation and InstrumentationOur experience creating benchtop instrumentation and integrated automation systems has validated our choice of LabVIEW as an appropriate tool. LabVIEW enables rapid development of functionally rich applications appropriate for both benchtop applications and larger integrated systems. On many occasions we have found that project requirements are initially ill defined or change as new measurements or new assays are developed.. There are several key features of the language that make it particularly useful in an automation environment for creating applications to control and integrate instrumentation, manage process flow, and enable data acquisition.Turnkey Measurement and Control FunctionLabVIEW was originally developed for scientists and engineers .The language includes a rich set of process control and data analysis functions as well as COM, .NET, and shared DLL support. Out of the box, it provides turnkey solutions to a variety of communication protocols including RS232, GPIB, and TCP/IP. Control structures such as timed While Loops allow synchronized and timed data acquisition from a variety of hardware interfaces such as PCI, USB, and PXI. DataSocket and VI ServerDeployment of an integrated system with multiple control computers requires the automation control application to communicate remotely with instrument drivers existing on remote computers. LabVIEW supports a distributed architecture by virtue of enabling seamless network communication through technologies such as VI Server and DSTP (data sockets transfer protocol). DSTP is an application layer protocol similar to http based on Transmission Control Protocol/Internet Protocol (TCP/IP). Data sockets allow easy transfer of data between remote computers with basic read and write functions. Through VI server technology, function calls can be made to VIs residing on remote computers as though they are residing on the local computer. Both Datasockets and VI server can be configured to control accesses privileges.Simple User Interface (UI) ImplementationIn addition to common interface controls such as text boxes, menu rings, and check-boxes, LabVIEW provides a rich set of UI controls (switches, LEDs, gauges, array controls, etc.) that are pertinent to laboratory equipment. These have their origins in LabVIEWs laboratory roots and help in development of interfaces which give scientists a clear understanding of a system's state. LabVIEW supports UI concepts including subpanels (similar to the Multiple Document Interface), splitter bars, and XControls (analogous to OCX controls).Multithreaded Programming EnvironmentThe inherent parallel environment of LabVIEW is extremely useful in the control of laboratory equipment. Functions can have multiple continuous While Loops where one loop is acquiring data rapidly and the other loop processes the data at a much slower rate. Implementing such a paradigm in other languages requires triggering an independent function thread for each process and developing logic to manage synchronization. Through timed While Loops, multiple independent While Loops can be easily synchronized to process at a desired period and phase relative to one another. LabVIEW allows invoking multiple instances of the same function witheach maintaining its own data space. For instance, we could drag many instances of the Mean sub-VI onto the block diagramin Figure 1b and they would all run in parallel, independent of one another. To synchronize or enforce control flow within the dataflow environment, LabVIEW also provides functions such as queues, semaphores, and notification functions.NI LabVIEW Application Example: The Open System Control Architecture (OSCAR)OSCAR is a LabVIEW-based (v7.1) automation integration framework and task execution engine designed and implemented at Cytokinetics to support application development for systems requiring robotic task management. OSCAR is organized around a centralized Oracle database which stores all instrumentation configuration information used to logically group devices together to create integrated systems (Fig. 2). The database also maintains Process Model information from which tasks and parameters required to run a particular process on a system can be generated and stored to the database. When a job is started, task order and parameter data are polled by the Execution Engine which marshals tasks to each device and updates task status in the database in real time. Maintaining and persisting task information for each system has two clear benefits. It allows easy job recovery in the event of a system error, and it also provides a process audit trail that can be useful for quality management and for troubleshooting process errors or problems.Each OSCAR component is distributed across the company intranet and communicates with a central database. Collections of physical devices controlled through OSCAR Instrument packages (OIP) make up systems. Users interact with systems through one of the several applications built on OSCAR. Each application calls the RTM which marshals tasks from the database to each OIP. OSCAR has sets of tools for managing system configurations, creating Process Models, monitoring running processes, recovering error-state systems, and managing plate inventory in storage devices.OSCAR uses a loosely coupled distributed component architecture, enabled in large part by LabVIEWs DSTP and remote VI technologies that allow system control to be extended beyond the confines of the traditional central control CPU model. Any networked computer or device can be integrated and controlled in an OSCAR system regardless of its physical location. This removes the proximity constraints of traditional integrated systems and allows for the utilization of remote data crunchers, devices, or even systems. The messaging paradigm used shares many similarities with current Service Oriented Architectures or Enterprise Service Bus implementations without a lot of required programming overhead or middleware; a centralized server is not required to direct the XML packets across the network. An additional benefit to this loosely coupled architecture is the flexibility in front-end application design. OSCAR encapsulates and manages all functionality related to task execution and device control, which frees the developer to focus on the unique requirements of a given application. For example, an application being created for the purpose of compound storage and retrieval can be limited in scope to requirements such as inventory management and LIMS integration rather than device control, resource allocation, and task synchronization.The OSCAR integration framework consists of multiple components that enable device and system configuration, process modeling, process execution, and process monitoring. Below are descriptions of key components of the framework. Integration PlatformThe Oscar Instrument Package (OIP) is the low level control component responsible for communicating with individual devices. It can support any number of devices on a system (including multiple independent instances of the same type of device) and communicates to the Runtime Manager (RTM) via serialized XMLstrings over DSTP. This allows the device controller and RTM components to exist on separate networked computers if necessary. Additionally, the OIP controller communicates with a device instance via LabVIEW remote VI calls which provide a lower level of distribution and allow the device drivers to exist on a separate networked computer from the controller. At Cytokinetics, we currently support approximately 100 device instances of 30 device types which are distributed across 10 integrated systems.System ManagementAn OSCAR system is a named collection of device instances which is logically represented in the database. The interface for each device (commands and parameters) is stored in the database along with the configuration settings for each device instance (i.e., COM port, capacity). The System Manager component provides the functionality to easily manipulate this information (given appropriate permissions). When a physical device is moved from one system to another, or a processing bottleneck alleviated by addition of another similar device, system configuration information is changed without affecting the processes that may be run on the system.Process ModelingA process model is the logical progression of a sequence of tasks. For example, a biochemical assay might include the following steps (1) remove plate from incubator, (2) move plate to pipettor, (3) add reagent, (4) move plate to fluorescent reader, (5) read plate, and (6) move plate to waste. The Process Modeler component allows the end user to choose functions associated with devices and organize them into a sequence of logical tasks. The resulting process model is then scheduled via a static schedule optimization algorithm or saved for dynamic execution (Fig. 3). Aprocess model is not associated with a physical system, but rather a required collection of devices. This has two importantbenefits: (1) the scientist is free to experiment with virtual system configurations to optimize the design of a future system or the reconfiguration of an existing system, and (2) any existing process model can be executed on any system equipped with the appropriate resources.The top panel (A) shows the Process Schedule Modeler, an application that graphically displays statically scheduled processes. Each horizontal band represents a task group which is the collection of required tasks used by a process; tasks are color coded by device. The bottom panel (B) shows the UI from the Automated Imaging System application. The tree structure depicts the job hierarchy for an imaging run. Jobs (here AIS_Retrieval and AIS_Imaging) are composed of task groups. As the systems runs, the tasks in the task group are executed and their status is updated in the database.Process ExecutionProcess execution occurs by invoking the OSCAR RTM. The RTM is capable of running multiple differing processes on a system at the same time allowing multiple job types to be run in parallel. The RTM has an application programming interface (API) which allows external applications to invoke its functionality and consists of two main components, the Task Generator Module (TGM) and the Execution Engine. External applications invoke an instance of a Process Model through the TGM at which point a set of tasks and task parameters are populated in the OSCAR database. The Execution Engine continually monitors the database for valid tasks and if a valid task is found it is sent to the appropriate device via the OIP. The OSCAR system supports running these jobs in either a static or dynamic mode. For processes which must meet strict time constraints (often due to assay requirements), or require the availability of a given resource, a static schedule is calculated and stored for reuse.The system is capable of optimizing the schedule based on actual task operation times (stored in the database).Other types of unconstrained processes benefit more from a dynamic mode of operation where events trigger the progress of task execution as resources become available in real-time. When operating dynamically, intelligent queuing of tasks among multiple jobs allows optimal use of resources minimizing execution time while allowing for robust error handling.Process MonitoringAll systems and jobs can be monitored remotely by a distributed application known as the Process Monitor. This application allows multiple users to monitor active jobs across all systems for status and faults and provides email notification for fault situations.ConclusionCytokinetics has built and maintains an automation software infrastructure using NI LabVIEW. The language has proven to be a powerful tool to create both rapid prototype applications as well as an entire framework for system integration and process execution. LabVIEW's roots in measurement instrumentation and seamless network communication protocols have allowed systems to be deployed containing multiple control computers linked only via the network. The language continues to evolve and improve as a general purpose programming language and develop a broad user base.。
加州大学伯克利动态优化讲义Dynamic Optimization in Continuous-Time Economic Models
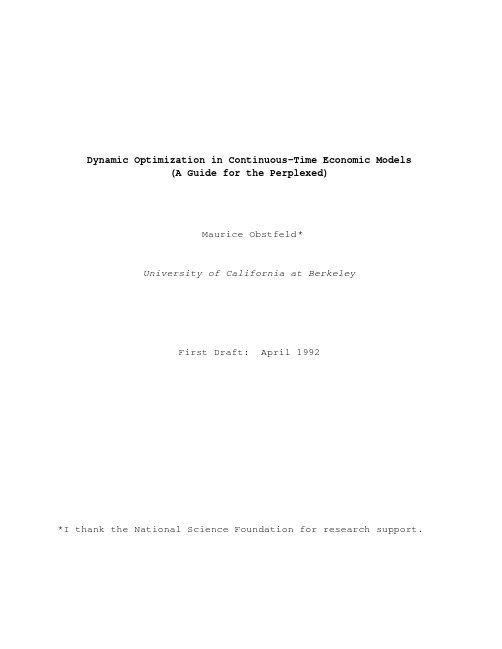
Dynamic Optimization in Continuous-Time Economic Models(A Guide for the Perplexed)Maurice Obstfeld*University of California at BerkeleyFirst Draft:April1992*I thank the National Science Foundation for research support.I.IntroductionThe assumption that economic activity takes placecontinuously is a convenient abstraction in many applications.In others,such as the study of financial-market equilibrium,the assumption of continuous trading corresponds closely to reality. Regardless of motivation,continuous-time modeling allows application of a powerful mathematical tool,the theory ofoptimal dynamic control.The basic idea of optimal control theory is easy to grasp--indeed it follows from elementary principles similar to thosethat underlie standard static optimization problems.The purpose of these notes is twofold.First,I present intuitivederivations of the first-order necessary conditions that characterize the solutions of basic continuous-time optimization problems.Second,I show why very similar conditions apply in1deterministic and stochastic environments alike.A simple unified treatment of continuous-time deterministic and stochastic optimization requires some restrictions on theform that economic uncertainty takes.The stochastic models I discuss below will assume that uncertainty evolves continuously^according to a type of process known as an Ito(or Gaussian------------------------------------------------------------------------------------------------------1When the optimization is done over a finite time horizon,the usual second-order sufficient conditions generalize immediately. (These second-order conditions will be valid in all problems examined here.)When the horizon is infinite,however,some additional"terminal"conditions are needed to ensure optimality.I make only passing reference to these conditions below,even though I always assume(for simplicity)that horizons areinfinite.Detailed treatment of such technical questions can be found in some of the later references.1diffusion)process.Once mainly the province of finance^theorists,Ito processes have recently been applied tointeresting and otherwise intractable problems in other areas of economics,for example,exchange-rate dynamics,the theory of the firm,and endogenous growth theory.Below,I therefore include a brief and heuristic introduction to continuous-time stochastic processes,including the one fundamental tool needed for this^type of analysis,Ito’s chain rule for stochastic differentials.^Don’t be intimidated:the intuition behind Ito’s Lemma is nothard to grasp,and the mileage one gets out of it thereaftertruly is amazing.II.Deterministic Optimization in Continuous TimeThe basic problem to be examined takes the form:Maximize8i-----d t(1)2e U[c(t),k(t)]dtjsubject toQ(2)k(t)=G[c(t),k(t)],k(0)given,where U(c,k)is a strictly concave function and G(c,k)is concave.In practice there may be some additional inequality constraints on c and/or k;for example,if c stands for consumption,c must be nonnegative.While I will not deal in any detail with such constraints,they are straightforward to22incorporate.In general,c and k can be vectors,but I will concentrate on the notationally simpler scalar case.I call cthe control variable for the optimization problem and k the state variable.You should think of the control variable as a flow--for example,consumption per unit time--and the state variable as a stock--for example,the stock of capital,measured in units of consumption.The problem set out above has a special structure that we can exploit in describing a solution.In the above problem, planning starts at time t=0.Since no exogenous variablesenter(1)or(2),the maximized value of(1)depends only onk(0),the predetermined initial value of the state variable.In other words,the problem is stationary,i.e.,it does not change3in form with the passage of time.Let’s denote this maximized value by J[k(0)],and call J(k)the value function for the8problem.If{c*(t)}stands for the associated optimal path oft=084the control and{k*(t)}for that of the state,then byt=0definition,------------------------------------------------------------------------------------------------------2The best reference work on economic applications of optimal control is still Kenneth J.Arrow and Mordecai Kurz,Public Investment,the Rate of Return,and Optimal Fiscal Policy (Baltimore:Johns Hopkins University Press,1970).3Nonstationary problems often can be handled by methods analogous to those discussed below,but they require additional notation to keep track of the exogenous factors that are changing.4According to(2),these are related bytik*(t)=2G[c*(s),k*(s)]ds+k(0).j38i-----d tJ[k(0)]=2e U[c*(t),k*(t)]dt.jThe nice structure of this problem relates to the following property.Suppose that the optimal plan has been followed until a time T>0,so that k(T)is equal to the value k*(T)given in the last footnote.Imagine a new decision maker who maximizesthe discounted flow of utility from time t=T onward,8i---d(t---T)(3)2e U[c(t),k(t)]dt,jTsubject to(2),but with the intial value of k given by k(T)=k*(T).Then the optimal program determined by this new decision maker will coincide with the continuation,from time T onward,of the optimal program determined at time0,given k(0).You should construct a proof of this fundamental result,which is intimately related to the notion of"dynamic consistency."You should also convince yourself of a key implicationof this result,thatTi-----d t----d T(4)J[k(0)]=2e U[c*(t),k*(t)]dt+e J[k*(T)],jwhere J[k*(T)]denotes the maximized value of(3)given that k(T) =k*(T)and(2)is respected.Equation(4)implies that we can think of our original,t=0,problem as the finite-horizon problem of maximizing4Ti---d t----d Te U[c(t),k(t)]dt+e J[k(T)]jsubject to the constraint that(2)holds for0<t<T.Of course,in practice it may not be so easy to determine thecorrect functional form for J(k),as we shall see below!Nonetheless,this way of formulating our problem--which is known as Bellman’s principle of dynamic programming--leadsdirectly to a characterization of the optimum.Because this characterization is derived most conveniently by starting in discrete time,I first set up a discrete-time analogue of our basic maximization problem and then proceed to the limit of continuous time.Let’s imagine that time is carved up into discrete intervals of length h.A decision on the control variable c,which is a flow,sets c at some fixed level per unit time over an entire period of duration h.Furthemore,we assume that changes in k, rather than accruing continuously with time,are"credited"only at the very end of a period,like monthly interest on a bank account.We thus consider the problem:Maximize8s---d th(5)e U[c(t),k(t)]htt=0subject to5(6)k(t+h)---k(t)=hG[c(t),k(t)],k(0)given.Above,c(t)is the fixed rate of consumption over period t whilek(t)is the given level of k that prevails from the very end of period t-----1until the very end of t.In(5)[resp.(6)]I have multiplied U(c,k)[resp.G(c,k)]by h because the cumulative flowof utility[resp.change in k]over a period is the product of a fixed instantaneous rate of flow[resp.rate of change]and the period’s length.Bellman’s principle provides a simple approach to the preceding problem.It states that the problem’s value function is given by()----d h(7)J[k(t)]=max{U[c(t),k(t)]h+e J[k(t+h)]},c(t)90subject to(6),for any initial k(t).It implies,in particular, that optimal c*(t)must be chosen to maximize the term in braces. By taking functional relationship(7)to the limit as h L0,we5will find a way to characterize the continuous-time optimum.We will make four changes in(7)to get it into useful form. First,subtract J[k(t)]from both sides.Second,impose the----------------------------------------------------------------------------------------------------------------5All of this presupposes that a well-defined value functionexists--something which in general requires justification.(Seethe extended example in this section for a concrete case.)Ihave also not proven that the value function,when it does exist,is differentiable.We know that it will be for the type ofproblem under study here,so I’ll feel free to use the value function’s first derivative whenever I need it below.With somewhat less justification,I’ll also use its second and third derivatives.6constraint(6)by substituting for k(t+h),k(t)+hG[c(t),k(t)].-----d hThird,replace e by its power-series representation,1----d h+2233(d h)/2-----(d h)/6+....Finally,divide the whole thing by h.The result is&2(8)0=max U(c,k)---[d-----(d h/2)+...]J[k+hG(c,k)]7c*+{J[k+hG(c,k)]----J(k)}/h,8 where implicitly all variables are dated t.Notice thatJ[k+hG(c,k)]-----J(k){J[k+hG(c,k)]----J(k)}G(c,k)-----------------------------------------------------------------------------------------=------------------------------------------------------------------------------------------------------------------.h G(c,k)hIt follows that as h L0,the left-hand side above approachesJ’(k)G(c,k).Accordingly,we have proved the followingPROPOSITION II.1.At each moment,the control c*optimalfor maximizing(1)subject to(2)satisfies the Bellman equation(9)0=U(c*,k)+J’(k)G(c*,k)----d J(k)()=max{U(c,k)+J’(k)G(c,k)-----d J(k)}.c90This is a very simple and elegant formula.What is its interpretation?As an intermediate step in interpreting(9), define the Hamiltonian for this maximization problem as7(10)H(c,k)_U(c,k)+J’(k)G(c,k).In this model,the intertemporal tradeoff involves a choicebetween higher current c and higher future k.If c isconsumption and k wealth,for example,the model is one in which the utility from consuming now must continuously be traded off against the utility value of savings.The Hamiltonian H(c,k)can be thought of as a measure of the flow value,in current utility terms,of the consumption-savings combination implied by the consumption choice c,given the predetermined value of k.TheHamiltonian solves the problem of"pricing"saving in terms ofQ current utility by multiplying the flow of saving,G(c,k)=k,byJ’(k),the effect of an increment to wealth on total lifetime utility.A corollary of this observation is that J’(k)has a natural interpretation as the shadow price(or marginal current utility)of wealth.More generally,leaving our particular example aside,J’(k)is the shadow price one should associatewith the state variable k.This brings us back to the Bellman equation,equation(9). Let c*be the value of c that maximizes H(c,k),given k,which is arbitrarily predetermined and therefore might not have been6chosen optimally.Then(9)states that(11)H(c*,k)=max{H(c,k)}=d J(k).c------------------------------------------------------------------------------------------------------6It is important to understand clearly that at a given point in time t,k(t)is not an object of choice(which is why we call it a state variable).Variable c(t)can be chosen freely at time t (which is why it is called a control variable),but its level influences the change in k(t)over the next infinitesimal time interval,k(t+dt)-----k(t),not the current value k(t).8In words,the maximized Hamiltonian is a fraction d of anoptimal plan’s total lifetime value.Equivalently,the instantaneous value flow from following an optimal plan divided by the plan’s total value--i.e.,the plan’s rate of return--must equal the rate of time preference,d.Notice that if we were to measure the current payout of the plan by U(c*,k)alone,we would err by not taking proper account of the value of the current increase in k.This would be like leaving investment out of our measure of GNP!The Hamiltonian solves this accounting problem by valuing the increment to k using the shadow price J’(k).To understood the implications of(9)for optimalconsumption we must go ahead and perform the maximization that it specifies(which amounts to maximizing the Hamiltonian).As aby-product,we obtain the Pontryagin necessary conditions for optimal control.7 Maximizing the term in braces in(9)over c,we get(12)U(c*,k)=-----G(c*,k)J’(k).c cThe reason this condition is necessary is easy to grasp.At each moment the decision maker can decide to"consume"a bit more,but at some cost in terms of the value of current"savings."A unitof additional consumption yields a marginal payoff of U(c*,k),cbut at the same time,savings change by G(c*,k).The utilityc------------------------------------------------------------------------------------------------------7I assume interior solutions throughout.9value of a marginal fall in savings thus is---G(c*,k)J’(k);andcif the planner is indeed at an optimum,it must be that this marginal cost just equals the marginal current utility benefit from higher consumption.In other words,unless(12)holds,there will be an incentive to change c from c*,meaning that c* cannot be optimal.Let’s get some further insight by exploiting againthe recursive structure of the problem.It is easy to see from (12)that for any date t the optimal level of the control,c*(t),depends only on the inherited state k(t)(regardless of whether k(t)was chosen optimally in the past).Let’s write this functional relationship between optimal c and k as c* =c(k),and assume that c(k)is differentiable.(For example,if c is consumption and k total wealth,c(k)will be the household’s consumption function.)Functions like c(k) will be called optimal policy functions,or more simply,policy functions.Because c(k)is defined as the solution to(9),it automatically satisfies0=U[c(k),k]+J’(k)G[c(k),k]-----d J(k).Equation(12)informs us as to the optimal relation between c and k at a point in time.To learn about the implied optimalbehavior of consumption over time,let’s differentiate the preceding equation with respect to k:100=[U(c*,k)+J’(k)G(c*,k)]c’(k)+U(c*,k)c c k+[G(c*,k)----d]J’(k)+J"(k)G(c*,k).kThe expression above,far from being a hopeless quagmire,is actually just what we’re looking for.Notice first that the left-hand term multiplying c’(k)drops out entirely thanks to (12):another example of the envelope theorem.This leaves us with the rest,(13)U(c*,k)+J’(k)[G(c*,k)-----d]+J"(k)G(c*,k)=0.k kEven the preceding simplified expression probably isn’t totally reassuring.Do not despair,however.A familiar economic interpretation is again fortunately available.We saw earlier that J’(k)could be usefully thought of as the shadow price of the state variable k.If we think of k as an asset stock(capital,foreign bonds,whatever),this shadow price corresponds to an asset price.Furthermore,we know that under perfect foresight,asset prices adjust so as to equate the asset’s total instantaneous rate of return to some required or benchmark rate of return,which in the present context can only be the time-preference rate,d.As an aid to clear thinking, let’s introduce a new variable,l,to represent the shadow price J’(k)of the asset k:l_J’(k).11Our next step will be to rewrite(13)in terms of l.Thekey observation allowing us to do this concerns the last term on the right-hand side of(13),J"(k)G(c*,k).The chain rule of calculus implies thatdJ’(k)dk d l dk d l QJ"(k)G(c*,k)=--------------------------*---------=---------*--------=--------=l;dk dt dk dt dtand with this fact in hand,it is only a matter of substitution to express(13)in the formQU+l G+lk k(14)-----------------------------------------------=d.lThis is just the asset-pricing equation promised in thelast paragraph.Can you see why this last assertion is true?To understand it,let’s decompose the total return to holding a unit of stock k into"dividends"and"capital gains."The"dividend"is the sum of two parts,the direct effect of an extra unit of k on utility,U,and its effect on the rate of increase of k,l G.(We mustk kmultiply G by the shadow price l in order to express thekQphysical effect of k on k in the same terms as U,that is,inkterms of utility.)The"capital gain"is just the increase inQthe price of k,l.The sum of dividend and capital gain,divided by the asset price l,is just the rate of return on k,which,by12(14)must equal d along an optimal path.-------------------------------------------------------------------------------------------------------------------------------------------------------------------------------------------------------------------------------------------------------------ExampleLet’s step back for a moment from this abstract setting toconsolidate what we’ve learned through an example.Consider the8----d tstandard problem of a consumer who maximizes i e U[c(t)]dtQsubject to k=f(k)-----c(where c is consumption,k capital,andf(k)the production function).Now U=0,G(c,k)=f(k)----c,Gk c=-----1,and G=f’(k).In this setting,(14)becomes thekstatement that the rate of time preference should equal the marginal product of capital plus the rate of accrual of utility capital gains,Q ld=f’(k)+-----.lCondition(12)becomes U’(c)=l.Since this last equalityQ Qimplies that l=U"(c)c,we can express the optimal dynamics of c and k as a nonlinear differential-equation system:U’(c)q eQ Q(15)c=----------------------2f’(k)-----d2,k=f(k)---- c.U"(c)z cYou can see the phase diagram for this system in figure 1. (Be sure you can derive it yourself!The diagram assumes thatlim f’(k)=0,so that a steady-state capital stock exists.) k L8The diagram makes clear that,given k,any positive initial c13initiates a path along which the two preceding differential equations for c and k are respected.But not all of these paths are optimal,since the differential equations specify conditions that are merely necessary,but not sufficient,for optimality.Indeed,only one path will be optimal in general:we can write the associated policy function as as c*=c(k)(it is graphed in figure1).For given k,paths with initialconsumption levels exceeding c(k)imply that k becomes negative after a finite time interval.Since a negative capital stock is nonsensical,such paths are not even feasible,let alone optimal. Paths with initial consumption levels below c(k)imply that kgets to be too large,in the sense that the individual couldraise lifetime utility by eating some capital and never replacing it.These"overaccumulation"paths violate a sort of terminal condition stating that the present value of the capital stock should converge to zero along an optimal path.I shall not take the time to discuss such terminal conditions here.If we take1---(1/e)c-----1U(c)=------------------------------------,f(k)=rk,1----(1/e)where e and r are positive constants.we can actually findan algebraic formula for the policy function c(k).Let’s conjecture that optimal consumption is proportional to wealth,that is,that c(k)=h k for some constant h to be14determined.If this conjecture is right,the capital stock k Qwill follow k=(r-----h)k,or,equivalently,Q k------=r---h.kThis expression gives us the key clue for finding h.If c= h k,as we’ve guessed,then alsoQ c------=r---h.cQ cBut necessary condition(15)requires that----=e(r----d),cwhich contradicts the last equation unless(16)h=(1---e)r+ed.Thus,c(k)=[(1-----e)r+ed]k is the optimal policy function.In the case of log utility(e=1),we simply have h=d.We getthe same simple result if it so happens that r and d are equal.Equation(16)has a nice interpretation.In Milton Friedman’s permanent-income model,where d=r,people consume the annuity value of wealth,so that h=r=d.This ruleresults in a level consumption path.When d$r,however,the optimal consumption path will be tilted,with consumption rising over time if r>d and falling over time if r<d.By writing15(16)ash=r-----e(r-----d)we can see these two effects at work.Why is the deviation fromthe Friedman permanent-income path proportional to e?Recallthat e,the elasticity of intertemporal substitution,measures an individual’s willingness to substitute consumption today for consumption in the future.If e is high and r>d,for example, people will be quite willing to forgo present consumption to take advantage of the relatively high rate of return to saving;andthe larger is e,certeris paribus,the lower will be h.Alert readers will have noticed a major problem with all this.If r> d and e is sufficiently large,h,and hence"optimal" consumption,will be negative.How can this be?Where has our analysis gone wrong?The answer is that when h<0,no optimum consumption plan exists!After all,nothing we’ve done demonstrates existence:our arguments merely indicate some properties that an optimum,if one exists,will need to have.No optimal consumption path exists when h<0for thefollowing reason.Because optimal consumption growth necessarily Qsatisfies c/c=e(r-----d),and e(r-d)>r in this case,optimal consumption would have to grow at a rate exceeding the rate ofQreturn on capital,r.Since capital growth obeys k/k=r----(c/k),however,and c>0,the growth rate of capital,and hence16that of output,is at most r.With consumption positive andgrowing at 3percent per year,say,but with capital growing at a lower yearly rate,consumption would eventually grow to begreater than total output--an impossibility in a closed economy.So the proposed path for consumption is not feasible.This means that no feasible path--other than the obviously suboptimal path with c(t)=0,for all t--satisfies the necessary conditions for optimality.Hence,no feasible path is optimal:no optimal path exists.Let’s take our analysis a step further to see how the value function J(k)looks.Observe first that at any time t,k(t)=(r ------h )t e (r ----d )t k(0)e =k(0)e ,where k(0)is the starting capital stock and h is given by (16).Evidently,the value function at t=0is just8q e -1()2211i -----d t 1----(1/e )J[k(0)]=22{2e [h k(t)]dt -------}1---------j 22d e 90z c 08q e -1()2211i -----d t e (r ----d )t 1----(1/e )=22{2e [h k(0)e ]dt --------}1----------j 22d e 90z c 0q e 1-----(1/e )-1()22[h k(0)]11=22{-----------------------------------------------------------------------------------}.1---------22d ----(e -----1)(r ----d )d e 90zc So the value function J(k)has the same general form as the utility function,but with k in place of c.This is not the last 17time we’ll encounter this property.Alert readers will again notice that to carry out the final step of the last calculation, I had to assume that the integral in braces above is convergent, that is,that d---(e-----1)(r-----d)>0.Notice,however,that d---(e------1)(r-----d)=r-----e(r-----d)=h,so the calculation is valid provided an optimal consumption program exists.If one doesn’t, the value function clearly doesn’t exist either:we can’t specify the maximized value of a function that doesn’t attain a maximum. This counterexample should serve as a warning against blithely assuming that all problems have well-defined solutions and value functions.-------------------------------------------------------------------------------------------------------------------------------------------------------------------------------------------------------------------------------------------------------------------------------Return now to the theoretical development.We have seen how to solve continuous-time determinstic problems using Bellman’s method of dynamic programming,which is based on the valuefunction J(k).We have also seen how to interpret the derivative of the value function,J’(k),as a sort of shadow asset price, denoted by l.The last order of business is to show that we have8 actually proved a simple form of Pontryagin’s Maximum Principle: PROPOSITION II.2.(Maximum Principle)Let c*(t)solve the problem of maximizing(1)subject to(2).Then there exist variables l(t)--called costate variables--such that the Hamiltonian------------------------------------------------------------------------------------------------------8First derived in L.S.Pontryagin et al.,The Mathematical Theory of Optimal Processes(New York and London:Interscience Publishers,1962).18H[c,k(t),l(t)]_U[c,k(t)]+l(t)G[c,k(t)]is maximized at c=c*(t)given l(t)and k(t);that is,d H(17)------------(c*,k,l)=U(c*,k)+l G(c*,k)=0c cd cat all times(assuming,as always,an interior solution).Furthermore,the costate variable obeys the differential equationd HQ(18)l=ld-------------(c*,k,l)=ld-----[U(c*,k)+l G(c*,k)]k kd kQ9for k=G(c*,k)and k(0)given.------------------------------------------------------------------------------------------------------------9You should note that if we integrate differential-equation (18),we get the general solution8d Hi-----d(s-----t)d tl(t)=2e------[c*(s),k(s),l(s)]ds+Ae,j d ktwhere A is an arbitrary constant.[To check this claim,just differentiate the foregoing expression with respect to t:if theQintegral in the expression is I(t),we find that l=d I---(d H/d k)d t+d Ae=dl---(d H/d k).]I referred in the prior example to an additional terminal condition requiring the present value of the capital stock to converge to zero along an optimal path.Since l(t)is the price of capital at time t,this terminal condition-----d tusually requires that lim e l(t)=0,or that A=0in thet L819You can verify that if we identify the costate variable with the derivative of the value function,J’(k),the Pontryagin necessary conditions are satisfied by our earlier dynamic-programming solution.In particular,(17)coincides with(12) and(18)coincides with(14).So we have shown,in a simple stationary setting,why the Maximum Principle"works."The principle is actually more broadly applicable than you might guess from the foregoing discussion--it easily handles nonstationary environments,side constraints,etc.And it has a10stochastic analogue,to which I now turn.-----------------------------------------------------------------------------------------------------------------------solution above.The particular solution that remains equates the shadow price of a unit of capital to the discounted stream of its shadow"marginal products,"where the latter are measured by partial derivatives of the flow of value,H,with respect to k. 10For more details and complications on the deterministic Maximum Principle,see Arrow and Kurz,op.cit.20。
新一代大型运载火箭大推力直接入轨高精度姿态控制方法

2021年第2期 导 弹 与 航 天 运 载 技 术 No.2 2021 总第379期 MISSILES AND SPACE VEHICLES Sum No.379收稿日期:2021-01-29;修回日期:2021-02-22文章编号:1004-7182(2021)02-0021-04 DOI :10.7654/j.issn.1004-7182.20210205新一代大型运载火箭大推力直接入轨高精度姿态控制方法黄 聪,张 宇,王 辉,李学锋,王 硕(北京航天自动控制研究所,北京,100854)摘要:为解决一级半构型的千吨级大推力新一代大型运载火箭直接入轨时刻5m 直径机架变形结构干扰大、20吨级巨大载荷条件下刚晃与弹晃交联耦合严重、百吨级低温发动机氧涡轮泵停转后效干扰大、主发动机关机后姿态控制能力显著不足等难题,提出了一种分时段多维增益自适应调整技术,动态调整关机后效段姿控系统滚动通道增益,可以有效提升载荷分离时刻姿态控制精度,确保20吨级有效载荷分离安全。
关键词:新一代大型运载火箭;大推力入轨;姿态控制 中图分类号:V448.1 文献标识码:AA High Precision Attitude Control Method for High Thrust Direct Orbit Entry of New Generation Large Launch VehicleHuang Cong, Zhang Yu, Wang Hui, Li Xue-feng, Wang Shuo(Beijing Aerospace Automatic Control Institute, Beijing, 100854)Abstract: In order to solve the problems that the large interference of the 5 meter diameter frame deformation structure, thesevere cross linking coupling of rigid-sloshing and elastic-sloshing under the 20 ton huge load, the large interference of the oxygen turbo pump of the hundred ton level low temperature after engine cutoff, the significant insufficient attitude control ability after the main engine shutdown. A time-segmented multi-dimensional gain adaptive technology is proposed, which can dynamically adjusts the roll channel gain of the attitude system after the core-level main engine shutdown, and this method can effectively improve the attitude control accuracy of the load separation moment, which ensures the safety of the 20 ton load separation.Key words: new generation; large launch vehicle; high thrust orbit entry; attitude control0 引 言长征五号B 运载火箭(以下简称CZ-5B 火箭)是长征五号(以下简称CZ-5火箭)的一级半构型,由芯一级+助推器+整流罩组成,没有单独的调姿和末速修正过程,CZ-5B 火箭利用一级火箭直接将空间站的核心舱和实验舱等送入预定轨道,在一级发动机关机时,约 1400 kN 的推力在3~6 s 之内消失,相当于一辆高速行驶的火车突然“刹车”,还要稳稳停靠在指定位置,姿态控制难度极大[1,2]。
制冷外文文献

Keywords:Steady-state simulation、Semi—empirical model、Domestic refrigerators、Experimental validation1。
IntroductionA household refrigerator is composed of a thermally insulated cabinet and a vapor—compression refrigeration loop as shown in Fig. 1。
These refrigeration systems, on the whole,consume a large amount of energy since hundreds of millions are currently in use, and dozens of millions are coming onto the market every year。
An understanding of the operational characteristics of a refrigeration system is vital for any energy optimization study,not only to predict its performance,but also to aid the decision making during the design process。
The refrigerator performance is usually assessed by one of the following approaches: (i) simplified calculations based on component characteristics; (ii) component analyzes through commercial CFD packages;and (iii)standardized experiments. Although the first two techniques play important roles in component design,they do not provide enough information on component matching and system behavior,which is only obtained by testing the refrigeratorin a controlled environment chamber. These tests,however, are time consuming and expensive。
DYNAMIC SETTING OF OPTIMAL BUFFER SIZES IN IP NETW

专利名称:DYNAMIC SETTING OF OPTIMAL BUFFERSIZES IN IP NETWORKS发明人:Jay J. Lee,Thomas Tan,Deepak Kakadia,EmerM. Delos Reyes,Maria G. Lam申请号:US12181042申请日:20080728公开号:US20100020686A1公开日:20100128专利内容由知识产权出版社提供专利附图:摘要:A communications system provides a dynamic setting of optimal buffer sizes in IP networks. A method for dynamically adjusting buffer capacities of a router may includesteps of monitoring a number of incoming packets to the router, determining a packet arrival rate, and determining the buffer capacities based at least partially on the packet arrival rate. Router buffers are controlled to exhibit the determined buffer capacities, e.g. during writing packets into and reading packets from each of the buffers as part of a packet routing performed by the router. In the disclosed examples, buffer size may be based on the mean arrival rate and one or more of mean packet size and mean waiting time.申请人:Jay J. Lee,Thomas Tan,Deepak Kakadia,Emer M. Delos Reyes,Maria G. Lam 地址:San Ramon CA US,San Jose CA US,Union City CA US,Martinez CA US,Irvine CA US 国籍:US,US,US,US,US更多信息请下载全文后查看。
具有鲁棒性和抗毁性的卫星网络重构策略

具有鲁棒性和抗毁性的卫星网络重构策略作者:彭秀媛张文波潘成胜来源:《数字技术与应用》2009年第11期[摘要]根据卫星网络的特性并结合其管理方式,提出了具有鲁棒性和抗毁性的卫星网络重构策略。
针对重构触发条件采取相应重构策略,对网络故障触发的重构将依据卫星网络体系结构从管理站重构和管理域内重构两个方面进行讨论。
采用基于管理域划分的重构策略能够有重点的实施网络重构,使网络尽快得到最大限度的恢复。
管理站的重构主要采用备份。
管理域内重构从三个方面进行:中继卫星重构、应用卫星重构、链路重构。
[关键词]卫星网络网络重构备份迂回路由策略鲁棒性和抗毁性[中图分类号]TN927[文献标识码]A[文章编号]1007-9416(2009)11-0098-03卫星网络自身的特点,向动态变化的卫星网络的重构提出了严峻的挑战:组成卫星网络的核心节点由于其运行的轨道周期特性以及由于节点的相对移动性而发生的通信链路重建或拆除、新节点的入网、卫星节点或网络链路的故障和通信链路的质量下降而导致无法正常通信或通信中断,都将导致网络拓扑发生改变。
要使这样一个复杂的网络正常运行,有必要对具有鲁棒性和抗毁性的卫星网络重构策略进行研究。
卫星网络体系从逻辑组成上包含:总管理站、各个分管理站、星簇、单星节点。
如图1所示。
中心管理站是卫星网络管理的最高层,负责对网络运行进行总体规划和状态监控。
各个分管理站作为中心管理站的逻辑下级,对星簇和单星节点执行包含配置、性能、故障和安全等管理功能域的管理,通常设置在地基,它向中心管理站报告网络状态,接受总管理站的管理。
分管理域内的星簇中由簇首管理任务星簇成员。
针对以上的卫星网络体系结构,为有效实现卫星网络的重构,我们提出了卫星网络重构策略:对于网络故障触发的网络重构,我们从管理域内重构和管理站的重构两方面来考虑。
1 管理站重构由于总管理站和分管理站位于地面,管理站的故障只能是由自身的失效以及管理站之间的通信失效而引起的,所以管理站的重构策略主要采取管理站的备份启用以及管理站间链路的备份的方法,保证卫星网络管理系统具有一定的抗毁性(见图1)。
自动切换数据流量原理

自动切换数据流量原理English:Automatic switching of data traffic is achieved through the use of load balancing algorithms and intelligent network technologies. Load balancing algorithms are designed to distribute the data traffic across multiple network links in a way that optimizes the use of available bandwidth and minimizes congestion. These algorithms monitor the traffic flow in real-time and dynamically adjust the distribution of traffic based on factors such as link utilization, latency, and packet loss. Intelligent network technologies, such as Software-Defined Networking (SDN) and Virtual Private Networks (VPN), also play a crucial role in automating the switching of data traffic. SDN enables central control of the network infrastructure, allowing for dynamic reconfiguration of network paths and routing rules to accommodate changes in traffic patterns. VPNs, on the other hand, provide secure and reliable connections for data traffic by creating virtualized network environments that can be easily reconfigured to reroute traffic as needed. Together, load balancing algorithms and intelligent network technologies work in tandem to automaticallyswitch data traffic in a way that ensures optimal performance and reliability of the network.Translated content:数据流量的自动切换是通过负载平衡算法和智能网络技术实现的。
FUJITSU Software BS2000 VM2000 V11.5 虚拟化白皮书说明书

White paperFUJITSU Software BS2000 VM2000 V11.5 Virtualization of BS2000 within the Dynamic Data Center.Introduction 2 VM2000 as base for different system environments 2 Optimal use of resources with VM2000 3 Support of Live Migration 4 Further functional enhancements with VM2000 V11.5 4 Version overview 5 Summary of VM2000 benefits 5IntroductionA virtual infrastructure like FUJITSU Software BS2000 VM2000 reduces IT costs by increasing efficiency,flexibility and response capability. It provides IT resource allocation on-the-fly in response to newbusiness requirements and service requests. Extremely high levels of server utilization are abyproduct.VM2000 supports the simultaneous operation of different, totally segregated system environmentson one server. The operating resources (CPU power and main memory) of one real server can bedistributed across up to 32 BS2000 guest systems. This distribution can be modified dynamically. Theconfiguration of peripherals, including their connections (channels), and other devices can bemodified or extended during live operation.The advantage of using VM2000 as compared with the use of multiple servers is the possibility ofconsolidation with the aim of providing more efficient use of hardware resources, human resourcesand infrastructure.VM2000 as base for different system environmentsCustomers are increasingly faced with the need to handle different system environments simultaneously on one server in order to cope most effectively with the wide variety of IT tasks they have to deal with.The reasons are:⏹Optimization of costs⏹Simple and uniform handling and administration⏹Parallel operation of production, development, test and version updates⏹Automation and operational reliability⏹Differentiated systems, for example for service data centers⏹Availability of backup systems⏹Separation of sensitive applicationsVM2000 V11.5 is exclusively released for the FUJITSU Server BS2000 SE series and supports the current versions of the BS2000 operating system BS2000 OSD/BC as guest systems.The provision of different system environments fulfills VM2000 in a flexible manner by the following features:Simultaneous operation of many guest systemsOn Server Unit x86 up to 32 BS2000 guest systems (incl. monitor system) can run simultaneously (on Server Unit /390 the number of guest systems is limited to 15).Full separation of guest systemsAccess to memory areas and devices of other guest systems is not possible. Faults in operation on one guest system do not affect the other guest systems, even if these errors cause the system to crash.Flexible assignment of resources to the guest systemsMemory, devices, CPU power and global store can be assigned to guest system s “on the fly”. The granularity of assignment is optimally small. The Capacity-on-Demand feature is offered: through connection of extra CPUs on the fly, CPU power can be increased for a certain time. Increased reliability and availabilityWhen the guest system (or the monitor system) fails, it can be automatically restarted. A manual restart of the monitor system can also be initiated. This does not affect the remaining guest systems.When one CPU fails, VM2000 automatically activates the available spare CPU (on servers unit /390), system performance remains unchanged. The same applies to possible affected guest systems: a virtual spare CPU will be switched on – the guest system performance remains the same. With this technique, the availability of mono guest systems is equal to the availability level of multiprocessor guest systems.BS2000 guest systems have the same functionality as systems in native modeThe instruction set, network communication options as well as test and diagnostic utilities of all guest systems running under VM2000 correspond to operation without VM2000.Performance of guest systems is comparable with native modeThe guest systems access the CPUs directly, with only minor emulation required.The memory is assigned permanently to the guest system and necessary address conversion is done by hardware.The guest systems execute the IO’s normally directly.Important guest systems can be prioritized, thus enabling a flexible response to customer requirements.The management of I/O peripherals can be done VM2000 spanning: the reconfiguration and the dynamic expansion of peripheral objects is done in common for all guest systems from the monitor system.Optimal use of resources with VM2000VM2000 allows data center service providers to install one or a small number of high-performance servers that can run several operating systems for a variety of external customers.This enables detailed capacity planning throughout the organization. Obvious knock-on effects of this include cost savings in relation to operating staff and space requirements for computers. The virtualization of resources such as CPU, main memory and global storage guarantees a high level of efficiency and optimum use of resources.The billing of the consumed CPU power can be done in two different ways:⏹Usage basedVM2000 writes VM-specific accounting records. They show the consumed CPU and the time periods of resource assignment.⏹Service level agreementsthe customer is guaranteed a certain CPU power, for which a RPF-based constant pricing is determined. The amount of CPU power used can be limited using the VM2000 function MAX-CPU-UTILIZATION.Formation of CPU poolsCPUs can be combined dynamically CPU pools. For such a pool VMs can be determined, which only can use this pool. The CPUs and VMs of the pool form a part of the Server Unit, which is provided to a customer.Dedicated CPUsIf the number of connected real CPUs in a CPU pool is greater or equal to the sum of connected virtual CPUs, then VM2000 assigns each virtual CPU of a VM one real CPU.The solid CPU assignment is optimal for a sufficient number of real CPUs in terms of performance, because each virtual CPU is always running on one and the same real CPU.Limitation of the CPU power for a group of guest systemsMultiple VMs on a Server Unit /390 can be combined into a VM group for which CPU scheduling specifications (CPU-Quota andMAX-CPU-UTILIZATION) can be effected comprehensive. The first step is to determine which CPU performance and CPU power limitation receives the VM group. In the second step, the power distribution within the VM group is determined. VM2000 provides for priority allocation of the group planned CPU power within the group. Service data centers can thus organize guaranteed computing performance for customers with multiple VMs.Granularity setting of CPU-QUOTA and MAX-CPU-UTILIZATIONThe two attributes for controlling the VM’s performance can be s pecified with two decimal places. This means that for very big Server Units definitions are possible in the one-digit RPF area up to one percent of the CPU capacity.Operation of several guest systems under VM2000 on Server Unit /390Support of Live MigrationLive Migration provides on SE servers an uninterruptable relocation of a running BS2000 guest system from one Server Unit to another. This enables a simple relocation of the guest systems with running applications to another server, for example prior to planned maintenance work respectively updates for hardware or firmware, including the reverse relocation of the systems or changing the load distribution between two servers. Those take place without affecting the users.With the new VM2000 command MIGRATE-VM a virtual machine (BS2000-VM) can be relocated from the local Server Unit to another Server Unit (target SU) of the same SU cluster in a running guest system operation while maintaining the operating resources.The Live Migration of a BS2000-VM between two Server Units /390 in SE network is fully executed by VM2000. The target SU is still located in another SE server. The LM functionality of Xen/X2000 in SU x86 is encased by VM2000 commands and messages. During VM migration of an SU x86 the target SU can be located in the same or a different SE server.In addition to the Live Migration of BS2000-VM (VM status RUNNING or INIT-ONLY/DOWN) VM2000 V11.5 supports also the migration of a VM definition (VM status DEFINED-ONLY) between two Server Units of a SU cluster using the command MIGRATE-VM-DEFINITION.The command CHECK-VM-INTEGRATION can check if a Live Migration of a VM is currently feasible.In addition to VM2000 V11.5 the Live Migration functionality requires SE V6.2 (M2000, X2000, HNC) and ONETSERV V4.0 on all guest systems. Further functional enhancements with VM2000 V11.5Besides Live Migration the following new features are released with VM2000 V11.5:Support of VM recoveryWith the new command RECOVER-VM-DEFINITION a VM definition of a shutdowned or failed Server Unit of the same SU cluster can be adopted. Subsequently, the VM definition can be activated on the local Server Unit and the BS2000 guest system can be booted. The command is currently supported on SU / 390.Update of VM2000 disk configurationService technicians can perform configuration changes in a disk storage system during operation. The new commandCHECK-VM-DISK-CONFIGURATION starts an update of VM2000 disk configuration, in order to implement appropriate configuration changes. The virtual machine system FUJITSU Software BS2000 VM2000 V11.5 exclusively supports the SE servers. On S and SQ servers VM2000 V11.5 has not been released.Version overviewServer models supported by VM2000:*in VM2000 V10.0 the new functions for the SE servers are not availableVersion of operating systems supported by VM2000 V11.0Summary of VM2000 benefits⏹Parallel operation of several BS2000 systems, respectively on Server Unit x86 also Linux/Windows systems on one server ⏹Support for version upgrades of the operating system, system-specific software and application systems⏹More flexible resource distribution than is possible on multi-server configurations⏹Provision of backup systems⏹Price advantages compared to several servers (consolidation)⏹Minimization of planned downtimes by uninterruptable relocation of running BS2000 guest systemsContact:FujitsuBarbara StadlerMies-van-der-Rohe-Str. 8, 80807 Munich GermanyEmail:**************************.com Web site: September 30, 2019 EM EN Copyright © 2019 Fujitsu Technology Solutions GmbHFujitsu and the Fujitsu Logo are trademarks or registered trademarks of Fujitsu Limited in Japan and in other countries. Other company, product or service names can be trademarks or registered trademarks of the respective owner.Delivery subject to availability; right of technical modifications reserved. No liability or warranty assumed for completeness, validity and accuracy of the specified data and illustrations.All designations used may be trademarks and/or copyrights, use of these by third parties for their own purposes could violate the rights of the respective owners.。
FPGA可编程逻辑器件芯片EP4CE6F17I8L中文规格书

Figure 113.GUI for Initial Adaptation PMA Configuration Setup1.The PMA Adaptation tab is used to configure the transceiver PMA parameters to compensate the channel loss profile.2.To enable this IP feature, enable adaptation load soft IP .3.You can select the PMA configuration that configures the PMA AFE parameters to the required settings before initiating initial adaptation and continuous adaptation.The PMA configurations listed have been validated across PVT as per IEEE 802.3bs/bj specifications. If you have a different test setup, you must tune some of the parameters to achieve the optimal performance across the PVT .4.Initial adaptation and continuous adaptation PMA parameter options are:8.Dynamic Reconfiguration ExamplesUG-20056 | 2021.02.10Send Feedback8.12. Configuring a PMA Parameter Using Native PHY IP 8.12.1. PMA Bring Up Flow Using Native PHY IPFigure 112.Configuring a PMA Parameter Using Native PHY IP Flow ChartNote:1. Refer to "PMA Adaptation" for PMA Adaptation Tab details.2. Refer the "PMA Adaptation Options" table for details.3. Refer to "PMA Bring Up Flow."4. Refer to "PMA Parameters."5. Refer to the "Configuring a PMA Parameter Using Native PHY IP" design example for details.Related Information•PMA Parameters on page 34•PMA Adaptation Parameters on page 438.Dynamic Reconfiguration ExamplesUG-20056 | 2021.02.10Send Feedback20.Write 0x86[7:0] = 0xEC.21.Write 0x87[7:0] = 0x00.22.Write 0x90[0] = 1'b1.23.Read 0x8A[7]. It should be 1.24.Read 0x8B[0], until it changes to 0.25.Write 0x8A[7] to 1 to clear the 0x8A[7] flag.Set the PMA parameter so that it is not overwritten by the adaptive tuning engine by configuring the PMA attribute code 0x2C to attribute value 0x108 and PMA attribute code 0x6C to attribute value 0x20.26.Write 0x84[7:0] = 0x08.27.Write 0x85[7:0] = 0x01.28.Write 0x86[7:0] = 0x2C.29.Write 0x87[7:0] = 0x00.30.Write 0x90[0] = 1'b1.31.Read 0x8A[7]. It should be 1.32.Read 0x8B[0], until it changes to 0.33.Write 0x8A[7] to 1 to clear the 0x8A[7] flag.34.Write 0x84[7:0] = 0x20.35.Write 0x85[7:0] = 0x00.36.Write 0x86[7:0] = 0x6c.37.Write 0x87[7:0] = 0x00.38.Write 0x90[0] = 1'b1.39.Read 0x8A[7]. It should be 1.40.Read 0x8B[0], until it changes to 0.41.Write 0x8A[7] to 1 to clear the 0x8A[7] flag.Refer to the Register Map for more details.Related InformationRegister Map on page 2178.Dynamic Reconfiguration ExamplesUG-20056 | 2021.02.10Send Feedback。
- 1、下载文档前请自行甄别文档内容的完整性,平台不提供额外的编辑、内容补充、找答案等附加服务。
- 2、"仅部分预览"的文档,不可在线预览部分如存在完整性等问题,可反馈申请退款(可完整预览的文档不适用该条件!)。
- 3、如文档侵犯您的权益,请联系客服反馈,我们会尽快为您处理(人工客服工作时间:9:00-18:30)。
1
Dynamic Recon guration of Sub-Optimal Parallel Query Execution Plans
2
1 Introduction
Growing demands for decision support and data mining against massive databases can result in very long-running queries that require parallel processing to deliver reasonable performance. During query evaluation, changes of system con guration and resource availability in a workstation farm environment are not uncommon. On the other hand, the introduction of abstract data types (ADTs) and associated user-de ned functions (UDFs) in an extensible object-relational DBMS for nontraditional application domains (e.g., time-series data analysis, image processing, and geoscience information systems) makes it more di cult to estimate intermediate data sizes. Furthermore, knowing little about the UDFs operation semantics, query optimizers often treat a UDF subexpression as an opaque operation that is not considered during optimization. As a consequence, static optimization methods that optimize a query once before execution do not satisfy today's query processing requirements. A parallel query execution plan (QEP) speci es the parallel process of manipulating data and producing results for a given query. The subtasks of the QEP are called operators. The conguration of a QEP refers to the composition of operators, connections among operators, degree of data parallelism and operator assignment to di erent processors. In this paper, we present a novel approach that improves the performance of query processing in an extensible and distributed Object-Relational DBMS { dynamic recon guring sub-optimal parallel QEPs. The objective is to take advantage of up-to-date cost estimates which come from more precise information on query statistics (e.g., selectivity of a quali cation), as well as to adapt to changing system con guration and resource availability (e.g., availability of machines, other workloads, etc.). As a consequence, available computing resources can be more e ciently utilized and the evaluation time of long running queries can be minimized. Recon guration includes options such as reassigning tasks to di erent processors, and changing the degree of data parallelism. An important part of performing a recon guration is to ensure the correctness and the completeness of query results { which means no erroneous result is produced and all correct results are generated. Therefore, con guration changes are required to leave the QEP in a consistent state. Informally, a consistent QEP state is one from which the QEP execution can continue and such that prior output plus future output constitute the correct result. The evaluation of a QEP can be viewed as moving the QEP from one consistent state to another.
Dynamic Recon guration of Sub-Optimal Parallel Query Execution Plans
Kenneth W. Ng Zhenghao Wang Richard R. Muntz
fkenneth,zwang,muntzg@
Computer Science Department University of California Los Angeles, CA 90095-1596 September 21, 1998
Abstract
Existing query optimization methods do not satisfy some of today's query processing requirements. Typically, only coarse or inaccurate estimates of database statistics are available prior to query evaluation. On the other hand, massive database sizes and growing demands for sophisticated processing result in long-running queries in extensible Object-Relational DBMS, particularly in decision support, and in data warehousing analysis applications. Therefore changes in system con guration and resource availability during query evaluation are not unexpected and can result in deteriorated query performance. Considering a parallel query evaluation environment, we propose dynamic recon guration of sub-optimal parallel query execution plans (QEPs) to adapt QEPs to the environment as well as to re ned estimates of data and query characteristics. To ensure correct query evaluation in the face of modi cation of the QEP, we propose an algorithm to coordinate the steps in a recon guration. We also present a taxonomy of user-de ned operators which allow the dynamic optimizer to plan a recon guration and systematically evaluate alternatives for execution context checkpointing and restoration. A syntactic extension of SQL to expose the relevant characteristics of user de ned functions in support of dynamic recon guration is proposed. An example from the experimental system is presented.