道程表(讲义)CN
道教基础知识大全 ppt课件
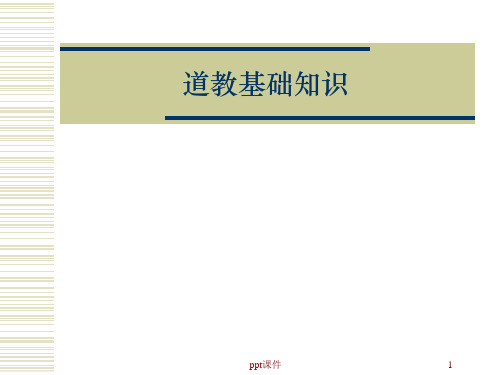
ppt课件
9
ppt课件
10
灵官殿实图
ppt课件
11
三清殿
道教宫观的主殿 主要供奉道家三清
ppt课件
12
Байду номын сангаас清元始天尊
道教最高尊神“三清”之一, 又稱“虛皇道君”。
元始天尊居三十六天之上的 清微天玉清境。
天尊之體常存不滅,至天地 初開,授道屜劫度人。
他主宰和像徵宇宙混沌初顯、 陰陽未判的第一個大世紀, 道教稱“混元”時期。
《三教搜神大全》卷四,说他:幼孤不读,有 管力,性刚暴质直。市中有不平者,直与分忧。 锄硬挞横,国人服其公,且惮其武。曾经焚烧 一江怪古庙,忽怪风大作,适逢萨真人相助作 法反风而灭妖,境界以安。
玉帝封他为豁落王元帅,赐金印,掌监察之职。 老百姓赞曰;“三眼能观天下事,一鞭惊醒世 间人。”
由于王灵官曾被封为“玉枢火府天将”,人们 又把他看成是火神,所以有的灵官像就是一副 火神的模样,火神庙中也供奉着灵官。
ppt课件
26
道教标记
ppt课件
27
张三丰
--修于武当山,创武当太极十三式,为武当派祖师
ppt课件
28
中国四大道教名山
ppt课件
29
湖北武当山
武当山又名太和山,位于鄂西北的 丹江口市境内,是我国的道教名山, 列中国“四大道教名山”之首,又 是武当武术的发源地。
主峰紫霄峰海拔1612米。武当山山 势奇特,雄浑壮阔。有72峰、36岩、 24涧、3潭、9泉、构成了“七十二 峰朝大顶,二十四涧水长流”的秀 丽画境。
名。曾一度被金世宗召至中都(今北京)传教。
后还乡,隐居栖霞山。金兴定三年(1219年),
成吉思汗西征途中,遣使相召。他率弟子
计程表课程设计

计程表课程设计一、课程目标知识目标:1. 学生能理解并掌握计程表的基本概念和构成要素。
2. 学生能够运用公式计算简单的计程费用,并解决相关问题。
3. 学生了解计程表的分类及其在实际生活中的应用。
技能目标:1. 学生能够运用所学的计程表知识,解决实际生活中的问题。
2. 学生通过小组合作,提高沟通协作能力,共同完成计程表的设计与计算。
3. 学生能够运用信息技术工具,如电子表格软件,进行计程费用的计算与数据分析。
情感态度价值观目标:1. 学生对计程表产生兴趣,认识到数学知识在生活中的重要性。
2. 学生在学习过程中,培养积极探究、勤于思考的学习态度。
3. 学生通过学习,增强对公平、诚信的认识,提高社会责任感。
课程性质:本课程为数学学科,结合生活实际,提高学生的应用能力和解决问题的能力。
学生特点:学生为五年级学生,具有一定的数学基础和逻辑思维能力,对生活中的数学问题感兴趣。
教学要求:教师应注重启发式教学,引导学生主动参与,关注学生的个体差异,提高学生的实践操作能力和团队合作能力。
通过课程学习,使学生在知识、技能和情感态度价值观方面取得具体的学习成果。
二、教学内容本节课主要依据教材中“计量与计费”章节内容,围绕计程表相关知识进行展开。
教学内容如下:1. 计程表的基本概念:介绍计程表的定义、构成要素及其在实际生活中的应用。
2. 计程表的分类:分析不同类型的计程表,如按里程计费、按时间计费等,并比较各自的特点。
3. 计程费用的计算方法:讲解计程费用的计算公式,通过实例演示如何运用公式计算计程费用。
4. 实际问题解决:结合生活实际,设计一些与计程表相关的问题,引导学生运用所学知识解决问题。
5. 小组合作设计计程表:分组让学生设计一个简单的计程表,培养学生的实践操作能力和团队协作能力。
教学进度安排:第一课时:计程表的基本概念和分类。
第二课时:计程费用的计算方法及实例分析。
第三课时:实际问题解决及小组合作设计计程表。
roadmap
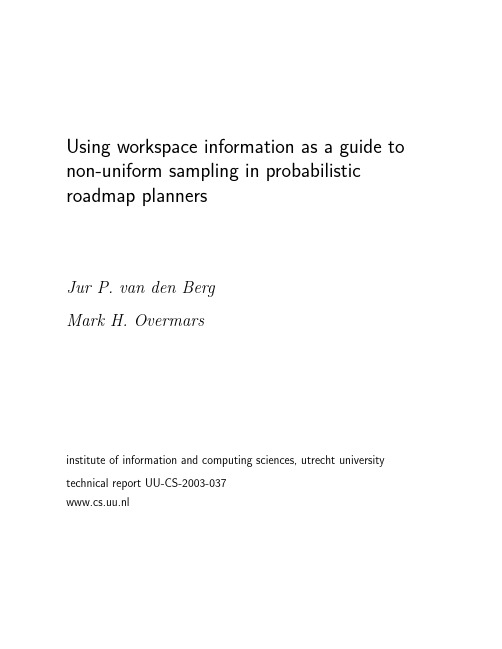
Using workspace information as a guide to non-uniform sampling in probabilistic roadmap plannersJur P.van den BergMark H.Overmarsinstitute of information and computing sciences,utrecht university technical report UU-CS-2003-037www.cs.uu.nlUsing workspace information as a guide to non-uniform sampling in probabilistic roadmap plannersJur P.van den Berg Mark H.OvermarsNovember2003AbstractThe probabilistic roadmap(PRM)planner is a popular method for robot motion planning problems with many degrees of freedom.However,it has been shown that themethod performs less well in situations where the robot has to pass through a narrowpassage in the scene.This is mainly due to the uniformity of the sampling used in theplanner;it places many samples in large open regions and too few in tight passages.In thispaper,a technique based on a robot independent cell decomposition of the free workspaceis proposed to guide the probabilistic sampling,such that the distribution of samples tendsmore toward the interesting regions in the scene.It is shown that this leads to improvedperformance on difficult planning problems in2D and3D workspaces.1IntroductionMotion planning is of great importance in variousfields,such as robotics,virtual environ-ments,maintenance planning,and computer-aided design.Although a number of exact and complete methods have been proposed for the robot motion planning problem(see[16]for an overview),these are seldomly used because they are only applicable to the simplest in-stances of the planning problem.For more complicated problems,where the robot has many degrees of freedom,these methods are computationally infeasible.Therefore,the focus has shifted toward probabilistic and heuristic methods,sacrificing completeness for speed and applicability.A technique often used nowadays is the Probabilistic Roadmap(PRM)planner[2,3,12, 14,17,18].The idea behind it is that a roadmap is created that represents the connectivity of the free part of the configuration space.The nodes of the graph are randomly sampled collision-free configurations that are connected by a simple and fast local planner(typically a straight-line motion in configuration space is used).The method is capable of solving motion planning queries in complex environments,and has been used in many practical situations.However,the method has trouble infinding paths through narrow passages in the scene. This is mainly due to the uniformity of the sampling;it places many samples in open regions and too few in tight passages(a thorough analysis is given in[11]).This problem has received much attention of researchers in thefield.The earliest strategies trying to tackle this prob-lem use information from the roadmap adaptively during construction.They add additional nodes in the neighborhood of nodes that were only connected to a few neighbors[13,15]. Later methods involve sampling more densely near obstacle boundaries[1,6,10],or far from obstacles,on the medial axis[20].Despite the amount of work done on this topic(see the pro-ceedings of the yearly IEEE International Conference on Robotics and Automation(ICRA)11INTRODUCTION2Figure1:A cell decomposition of the workspace of a2D example scene.The grey cells form the watersheds.and the Workshop on Algorithmic Foundations of Robotics(WAFR)for many contributions), a generic solution has not yet emerged.The general observation remains,however,that the way of sampling is crucial for the result[7,8,9].Whereas previous methods are mainly obstacle-based strategies,we propose a method that uses the shape of free workspace to guide the sampling in configuration rmation from the workspace can only be used effectively when the configuration space more or less resembles the workspace.This means that narrow passages in the configuration space should correspond to narrow passages in the workspace,and that configurations in difficult regions can be mapped straightforwardly to the corresponding points in the workspace.This holds for a large class of problems in robot motion planning:free-flying robots in two or three dimensional scenes.If the size of the robot is not too large compared to the size of the scene,each difficult region in the configuration space can be related to a relatively narrow passage in the workspace.Our method identifies the narrow passages in the workspace using a cell decomposition approach.Because the workspace is only3-dimensional,this can easily be done.Subsequently, cells of the decomposition are grouped into regions of interest by labeling them.The labeling-part is the most crucial in this context.For this,we propose a method called watershed labeling,inspired by a technique from thefield of image processing,called watershed segmentation[19].It is a powerful method that separates large open regions from each other by so-called watersheds.These watersheds are positioned inside the corridors connecting these regions.As a result,the narrow passages are labeled differently than the open regions.See Fig.1for an example.This information is used to effectively steer the sampling toward the most interesting regions of the scene.To this end,each of the labeled regions is assigned a weight indicating the chance that a sample in picked inside this region.To be precise,for a sample we pick the position in the region and the other degrees of freedom randomly.Narrow passage regions will receive relatively larger weights than open regions,which results in more samples in narrow passages.This will lead to faster connection of loose components in the probabilistic roadmap, and hence a quicker convergence toward a roadmap capturing the free space.The global outline of this paper is as follows:in Section2we briefly recall the PRM method;in Section3we describe our global approach.The details arefilled in in Section2PRM BASICS3 4.In Section5we discuss watershed labeling and in Section6we will present experiments showing that the method indeed leads to significant improvements.Finally,we discuss the work presented and put forward some possible future work in Section7.2PRM BasicsThe motion planning problem is generally formulated in terms of the configuration space C,the set of all possible configurations of the robot.The dimension of the configuration space corresponds to the number of the robot’s degrees of freedom.Each obstacle in the workspace,in which the robot actually moves,transforms into an obstacle in configuration space.Together,they form the forbidden part C forb of the configuration space.C free=C\C forb denotes the set of all collision-free configurations.Probabilistic roadmap planners generally work in two stages:a preprocessing stage,some-times referred to as learning stage,and a query stage.In the preprocessing stage,a roadmap is constructed that forms a discrete representation of the connectivity of the free configuration space.The nodes of the roadmap are free samples,randomly chosen from the configuration space.There is an edge between two nodes,if a local plannerfinds a collision-free path be-tween the corresponding configurations.The paths searched for are mostly simple straight line segments in the configuration space.In the query stage,the constructed roadmap is used tofind a path between a start and goal configuration.In general,these configurations are not present in the roadmap,so they are added to the roadmap using the local ing Dijkstra or other graph searching methods,a path between the start and goal configuration is then easily found.An important aspect of PRM is the way samples are picked from the configuration space. In the basic PRM this is done uniform randomly,but this yields many samples in open regions of the free space and few in narrow regions,while the inverse would be preferable.In this paper,we will focus on a sampling strategy yielding more samples in interesting regions.3Global approachThe idea of our method is to use the shape of the free workspace to guide the sampling in configuration space.For this purpose,the preprocessing stage of PRM is subdivided in two phases,a datastructure phase and a sampling phase.In the datastructure phase,a robot independent datastructure is built upon the workspace,identifying narrow and interesting regions.This proceeds as follows:First,the free workspace,denoted by W free,is decomposed into a collection of cells.Sec-ond,cells are grouped into regions of interest by labeling the cells according to some local properties.The set of cells having the same label is called a labeled region.The subdivision of the workspace is used to steer the sampling.So,each of the labeled regions is assigned a weight indicating the chance that a sample in picked inside this re-gion.Since narrow passages should receive relatively more samples than open regions,narrow passage regions are assigned a relatively large weight.The datastructure phase is algorithmically depicted in Algorithm1.In Section3.2and 3.3we will discuss the datastructure phase in detail.The sampling phase is straightforward.A sample is picked with its translational degrees of freedom inside a labeled region that is selected according to the weight distribution.The1:Decompose F into cells2:Group cells into labeled regions by assigning a label to each of the cells3:Assign each of the labeled regions a weight defining its sampling probability3.2Cell decompositionIn thefirst step of our algorithm the free workspace is decomposed into a set of cells.Let this{χ∈X}.Two set of cells be denoted by X and the union of all the cells by D,i.e.:D=categories of methods to decompose a space into cells exist:exact and approximate ones.Exact cell decomposition methods result in a set of cells whose union exactly equals the free space of the scene,i.e.D=W free.Examples are triangulations(tetrahedralizations in3D),trapezoidal maps and generalized Voronoi diagrams[4].These methods extensively use the geometry of the obstacles in the scene.Since scenes can consist of tens of thousands of obstacles,geometric processing is often not feasible within the tight runtime demands.Therefore,we will focus on approximate cell decompositions.Examples are octrees and binary space partitions[16].Approximate cell decompositions result in subsets of the free space,i.e.D⊆W free.Mostly, they have a recursive nature.A coarse approximation of the free space becomesfiner at each level,by subdividing cells that partially overlap both free and forbidden space.This may continue until a desired level of detail is reached.This is formalized as follows:Definition3.1.Let B p(ǫ)denote the ball of radiusǫat position p.A cell decomposition is calledǫ-detailed if∀B p(ǫ)⊂W free:p∈D.Decompositions satisfying this criterion are denoted by Dǫ.We require the cell decomposition technique to be able to produce anǫ-detailed decom-position for everyǫ>0.It is easy to see that the region in configuration space corresponding to the r in-detailed totally contains the free configuration space:decomposition D rinan r in-detailed decomposition Theorem3.3.Let r in be the inner radius of the robot,and D rin).Such cell decompositions are said to be totally covering the of W free.Then C free⊂C(D rinfree configuration space.This observation is used later to prove the probabilistic completeness of our method.The cell decomposition over the workspace should in principle be robot independent.This means that D has to be detailed enough for every robot.This is of course not possible,but when we choose a small enoughǫ,a subset of Dǫcan be used for robots with an inner radius larger thanǫ.For robots that have no inscribed ball(r in=0),e.g.a point robot or a line-segment robot,approximate cell decomposition techniques cannot produce a totally covering decomposition.For this kind of robots,however,a weaker statement can be made: Theorem3.4.Let Dǫbe anǫ-detailed cell decomposition of W free.Then,∀c∈C free\C(Dǫ): cl(c)≤ǫ.This fact is used later to prove a weaker form of probabilistic completeness for our method.3.3Labeling and weightsApproximate cell decompositions usually result in a large number of relatively small cells, especially near the boundary of the free workspace.This is,however,not what we want; we want a clear distinction between different regions of interest in the scene,for instance open regions and corridors between them.Therefore,cells should be grouped to create larger regions.This is done by labeling each cell:cells belonging to the same region are given the same label.The set of cells that is given a same particular label i is called a labeled regionR i.The union of all the labeled regions should form the total cell decomposition.This means that all cells should be given a label.Each of the labeled regions should be assigned a weight to steer the sampling,beled regions have a chance to receive a sample with a probability proportional to their weights.If each labeled region would be given its volume as its weight,the resulting sampling distribution is uniform over the total cell decomposition.So,to give narrow regions relatively more samples and open regions relatively less,their weights should be raised or lowered respectively.None of the labeled regions should be given a weight of0;each portion of the configuration space must have a probability>0to receive a sample,in order for the method to remain probabilistically complete,which we will prove below.3.4SamplingThe sampling scheme we propose on the datastructure created above is simple:first,select a labeled region R according to the weight distribution.Second,a sample is picked uniform randomly inside C(R).This is done as follows:the labeled region R consists of a number of cells,so a cell is picked randomly from R,with a probability for each cell proportional to its volume.Then,the translational degrees of freedom are chosen inside this cell.The other degrees of freedom are chosen randomly.Algorithmically,it looks as follows:1:Choose randomly a labeled region R according to the weight distribution2:Choose randomly a cell inside R according to the cell volumes3:Create a new sample:pick its position inside the cell and the other degrees of freedom uniform randomly4:Treat this sample as in standard PRMFor cell decompositions not totally covering the free configuration space,a weaker state-ment can be made.Theorem3.6.Let Dǫbe anǫ-detailed cell decomposition of W free,and assume a path with clearance>ǫexists.Then,the above sampling scheme willfind a path.Proof Theorem3.4implies that any path with clearance>ǫin C free is contained in C(Dǫ). Since C(Dǫ)is totally sampled,every small ball with radius>0in C(Dǫ)will contain a sample if the sampling continues long enough.This implies that the method will eventually find a path[18].4FILLING IN THE DETAILS7 4Filling in the detailsA number of important details have yet to befilled in,such as the used approximate cell decomposition technique and the way the cells are labeled and assigned weights.Let us recall the requirements posed upon these choices:We require that•The cell decomposition technique is able for every scene to produce anǫ-detailed de-composition for everyǫ>0.•The union of the labeled regions forms the total cell decomposition,i.e.all cells must be given a label.•No weight assigned to a labeled region is0.4.1Cell decompositionA well-known and obvious approximate cell decomposition datastructure is the octree.An octree is a rooted tree in which every internal node has eight children.Every node in the tree corresponds to a cube.If a node has children,then their corresponding cubes are the eight octants of the cube of the parent node.A node of the octree is subdivided when the cell contains both obstacles and free space.If the cell only contains free space or obstacles,then it is marked as free or full respectively,and it is not subdivided.The subdivision of the mixed cells may propagate down until some desired level of detail is reached.The root of the octree corresponds to a cube or rectangloid covering the entire workspace.The leaves of the tree with label free together form the approximate cell-decomposition of the workspace.Note that all cells in the octree are congruent to the initial cell covering the total scene.It is easily shown that the octree decomposition technique fulfills our requirement: Theorem4.1.For every scene and for everyǫ>0,the octree cell decomposition technique is able to produce anǫ-detailed decomposition.Many related decomposition techniques exist that satisfy the above criterion as well,such as the binary space partition.For the sake of simplicity,however,we will use the octree cell decomposition in this paper.4.2Labeling and weightsThe simplest instance of labeling cells is to give each cell a different label.In other words,each cell is treated as a different labeled region.The simplest weight distribution for this labeling is to give each labeled region a weight according to its volume.This results in uniform sampling over the free space,and in that sense our method of using cell decompositions can be seen as a generalization of standard PRM.Another obvious distribution of weights is to give each labeled region the same weight, i.e.they all have the same chance to be sampled.This weight distribution has a rationale, since cells,and thus the labeled regions,tend to be smaller when they are closer to obstacles, for instance in narrow passages.Yet each of the cells has the same chance to contribute a sample,leading to a larger density of samples in narrow passages when compared to uniform sampling over the entire configuration space.A problem of this method is that also many small cells are generated along the boundary of obstacles,leading to many samples close to boundaries.It lacks a way to make a distinction between narrow passages and regions close to obstacles.A similar problem was observed in previous attempts to deal with the narrow passage problem[1,6].Yet,experiments show that this method already works better than basic PRM in some scenes.To improve this,we need a way to distinguish cells in narrow passages from cells near a boundary in an open area.In the next section,we will describe a watershed approach to achieve this.5Watershed labelingThe simple labeling scheme discussed above is of course not favorable;we want to be able to really distinguish regions of interest in the scene.For such a labeling method,we let us inspire by image segmentation methods from thefield of image processing.In image segmentation, the problem is roughly the beled regions should be formed of pixels that somehow belong together,according to a property defined in terms of the image itself.The watershed transform[19]is a well-known segmentation method.It has shown to be a powerful method in many applications.Watershed segmentation yields nice and convincing labeled regions.It separates open regions from each other by watersheds.The watershed transform may very well be used as a basis for our labeling scheme,where the watershed regions represent narrow passages and the regions they separate represent the open regions in the scene.5.1Watersheds in topographical landscapesThe watershed method was originally developed for discrete binary images(2D or3D).So, we need to adapt the algorithm to make it suitable for approximate cell decompositions.We concentrate on octrees here,but we believe that it is extendible to other cell decompositions as well.We use an analogon with a topographical landscape to explain the algorithm for2D cell decompositions.The algorithm is easily extended to higher dimensions.We interpret the sizes of the cells in the decomposition as elevations in a topographical rge cells correspond to low elevations(see Fig.2for an impression).In the landscape we may distinguish different catchment basins.These are areas in the landscape associated with a local minimum,such that every imaginary rain drop falling in the catchment basin would end up in the particular local minimum.The boundaries between the catchment basins are called watersheds.Tofind the watershed regions,the landscape isflooded.Starting from the minima of low-est elevation(the largest free cells),the water will progressivelyfill up the different catchment basins.Now,at each cell where the water coming from two different minima would merge,we build a watershed.At the end of thisflooding procedure,each minimum is completely sur-rounded by watersheds,which delimit its associated catchment basin.The catchment basins now each form an open labeled region and the watersheds separating the catchment basins identify the narrow passages between open labeled regions.Figure2:An impression of the topographical landscape induced by the cell decomposition of the example scene.5.2Watersheds for cell decompositionsIn terms of an octree cell decomposition,each of the hierarchy levels in the octree correspond to aflood level in the topographical landscape,and rising theflood level corresponds to processing the cells in the next hierarchy level of the octree.Local minima correspond to isolated groups of cells of locally maximal sizes.These are given a unique label.The algorithm iteratively processes each of the hierarchy levels in the octree.We start with the highest hierarchy level,which contains the largest cells.Processing a hierarchy level goes as follows:for each of the cells in the level,it is checked if it has an already labeled(larger)neighboring cell.If this is the case,the cell is appended to afirst-in-first-out queue(see Algorithm3,lines2...4).Subsequently,each cell in the queue is processed.Again,its neighbors are inspected:if the neighbor cells that are already labeled all have the same label,the cell is given that label too.If there are neighbors with different labels,the cell is labeled as a watershed cell.Neighboring cells of the same hierarchy level that neither are already in the queue,nor are already labeled,are appended to the queue(see Algorithm3,lines6...15).This process repeats until the queue is empty.Then,there may still be a number of cells in the current hierarchy level that have no label yet.These cells form new local minima and are given new labels.Groups of adjacent unlabeled cells are given the same label(see Algorithm 3,lines17...26).They form together a local minimum.After this,the algorithm proceeds to the next hierarchy level,carrying out the same process.This may continue until some desired level of detail is reached.Note that the water-shed algorithm can be carried out simultaneously with a breadth-first construction of the cell decomposition.The algorithm is given in pseudocode in Algorithm3.Afirst-in-first-out queue is used in the algorithm.Three functions are defined on this queue:•fifofirst():Returns the cell at the front of the queue and removes it from the queue •fifo5WATERSHED LABELING10 Furthermore,a function N(χ)is used.It returns a set containing all free neighboring cells ofχin the octree.Note that the function is only used for cells whose neighbors are either larger or have the same size,so this function is easily implemented.1:for all hierarchy levels of the cell decomposition do2:for all cellsχin the current hierarchy level do3:if there existχ′∈N(χ)that is already labeled then4:fifoempty()do7:χ←fifoadd(χ′)16:17:for all cellsχin the current hierarchy level do18:ifχis unlabeled then19:Giveχa new label20:fifoempty()do22:χ′←fifoadd(χ′′)Figure3:(a)Labeled regions in the example scene.The grey squares form watershed regions.(b)Distribution of samples in the example scene when each labeled region is given the same weight.The sample density in open region5is clearly higher than the sample density in open region2.workspace,the alleys are marked as narrow passage on the’street scale’,and doors separating rooms are marked as narrow passages on the’house scale’.In theory,every narrowing,small or not,is detected and marked as narrow passage. Fortunately,the watershed method allows for setting some thresholds,for instance a narrow passage should be a factor x more narrow than the regions it connects,or a narrow passage should be narrower than the absolute value of y.For the cell decomposition variant of the watershed algorithm,this problem is less pregnant,since cells of different levels already differ a constant factor in size.5.3Assigning weightsAssigning weights to the labeled regions can be done in many ways.We restrict ourselves to a simple weighting approach in this paper:each labeled region is given the same weight. Experiments showed that this works quite well for most scenes.In Fig.3b an impression is given of how the samples are distributed using this method.More complicated weighting schemes are the subject of further research.6Experimental resultsWe experimented with our method on four different scenes:rooms,clutter,hole and office (see Fig.4).rooms in this scene there are three rooms with two narrow doors between them.The table must move through the doors to the other room.The watershed labeling yields three open regions in each of the rooms and two watershed regions in the doors between them.clutter the clutter scene consists of500uniformly distributed tetrahedra.The configu-ration space will consist almost solely of narrow passages,so for this scene uniform random sampling is expected to work best.We test it with an L-shaped robot that has to travelfrom one corner to the opposite.The watershed labeling yields a disorganized distribution ofFigure4:The scenes used in the experiments.(a)Rooms.(b)Clutter.(c)Hole.(d)Office.‘open’and watershed regions between the tetrahedra.In total,there are296different labeled regions.hole this scene clearly has a narrow passage which is relatively hard for any kind of robot.A method to steer the sampling toward narrow passages should work very well in this case. We use a complicated robot having four legs.The watershed labeling yields two open regions on each side of the wall and a watershed region inside the hole.office this scene has a large hall with two offices similar to the rooms scene in each of the corners.The robot(a sphere)has to move from one to the other office.We expect that denser sampling of smaller open regions can be of particular interest in this scene.The watershed labeling yields eight open regions.Three smaller ones in each of the offices and two large ones in the central hall.6.1Experimental setupOur method was integrated in the motion planning system SAMPLE(System for Advanced Motion PLanning Experiments),implemented in Visual C++under Windows XP.The ex-periments were run on a2.4GHz Pentium4processor with1GB internal memory.As basic collision checking package we use Solid[5].In the experiments we consider the time needed to construct a covering roadmap for the scene.A roadmap is considered good enough when a pair of predefined query configurations is connected via the roadmap.For each scene such a query pair is devised(see Fig.4).Because randomness is involved,we averaged the running times of100independent runs.Only for the hole scene,we performed50runs.Since our method uses a preprocessing step to build a datastructure guiding the sam-pling,the actual running time is not directly comparable to traditional methods,where such a datastructure step is not present.Since our datastructure is robot independent,i.e.:for dif-ferent types of robots a roadmap can be constructed using the same datastructure,the time needed to construct the datastructure is not added to the time needed to build the roadmap, but reported separately.For the rooms,clutter,hole and office scene it took respectively0.55, 3.9,0.06and2.0seconds to build a watershed labeled cell decomposition with an appropriate level of detail.We believe that these times can be improved,but we did not concentrate on that.We compare our method to previously proposed techniques that aim to better capture narrow passages in the sampling.In a recent publication[9],various sampling methods were compared to each other,among other things with the focus on narrow passages.Two conclu-sions can be drawn from that work:uniform random sampling performs quite well in many cases,and an obstacle-based variant in which only near-obstacle configurations are sampled, called nearest contact,appears to work well for narrow passage problems.So,in our experiments we compare our method to these two sampling strategies.Fig.5 summarizes our results.6.2ResultsThe results show that our method works particularly well for the rooms scene.This is because the watershed regions capture the narrow passages very well and enable an effective steering of the sampling.This results in considerably better performance of our method than uniform random and nearest contact.。
道次第学习表格-深信业果
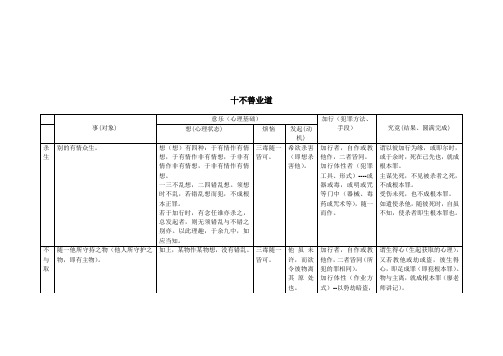
十不善业道
此中意业思(思:造作,审虑思、决定思、动身发语思)者,是业非业道。
身语七支者是业,以是思惟所履之事故,亦是业道。
贪心之三者,是业道而非业也。
依不善业论业果轻重
十不善业果报差别
业的种类
完美人身的因果差别
----异熟功德及业用、异熟之因
如何得殊胜异熟?----修八因(见上表),具三缘(本表)
正文:彼八种中,若具三缘,当得殊胜异熟。
三缘者(一心清净,二加行清净,三田力清净)。
心清净中(复分自他之二),观待于自有二:(一)修诸善因,回向无上菩提,不希异熟。
(二)及意乐至诚猛利而修诸因也(以纯厚意、决定意、猛利修善)。
观待于他有二:(一)见同法者(同法者,如自行施,他亦行施,同心一德者是),上中下三(品),断除嫉妒、校量、毁誉(轻侮比赛之心),心生随喜(勤修随喜)。
(二)若不能者,亦应日日于其所行,多次观察焉(即不能如是,亦应日日多次观察他所作事,见贤思齐)。
加行清净中(亦分二):(一)观待于自,长时无间猛利所作(自方对八因长时殷重而作)。
(二)观待他者,未受正行,赞美令受。
诸已受者,赞美令喜,常恒无间,安住不舍(他方未修八因者,赞彼令修,已修者,赞之令增)。
田清净者,即彼二种意乐加行,获多美果等同田也(田清净中,即彼意乐加行所得之二果,美妙繁多,其力如田,故称田清净)。
四力净修之法。
标准预防课件幻灯片
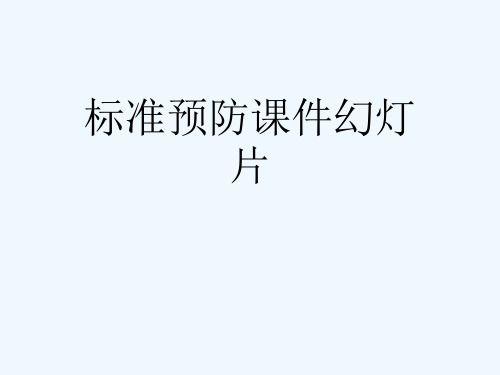
标准预防措施5
2、接触隔离、防护隔离
(1)进入隔离病室接触病人包括接触病人的血液、体液、分泌 物、排泄物等物质时,应戴手套。 (2)离开隔离病室前,接触污染物品后摘除手套,洗手和/或手 消毒。 (3)进入病室,从事可能污染工作服的操作时,应穿隔离衣; 离开病室前,脱下隔离衣,按要求悬挂,或使用一次性隔离 衣,用后按医疗废物管理要求进行处置。 (4)隔离室应有隔离标志,并限制人员的出入。
三、医院标准预防制度
认定病人的血液、体液、分泌物、排泄物均具有传染性, 必须进行隔离,不论是否有明显的血迹污染或是否接触不完 整的皮肤与粘膜,接触上述物质者,必须采取防护措施。其 基本特点为:
1、既要防止血源性疾病的传播,也要防止非血源性疾 病的传播。
2、强调双向防护,即防止疾病从病人传至医务人员, 又防止疾病从医务人员传至病人。
标准预防措施5
空气隔离
1、 空气传播是指病原微生物经由悬浮在空气中的微 粒(粒径小于5um)一气溶胶来传播的方式,这种微粒 能在空气中悬浮较长时间,并可随气流漂浮到较远处, 所以可造成多人感染,甚至导致医院感染暴发流行。 因此,病人所处的环境需要屏蔽,可使用单人房间、 专门的空气处理系统和通风设备防ቤተ መጻሕፍቲ ባይዱ空气传播。
二、确诊感染的措施
医务人员和进入该环境的人员应使用呼吸 道保护装置。如果病人确诊或可疑感染了经空 气传播的疾病,如结核、流行性脑膜炎、腮腺 炎、水痘、麻疹、肺鼠疫、肺出血热等,在标 准预防的基础上还要采用空气传播的隔离预防, 要采用以下隔离措施:1、病人的隔离; 2、空气隔离、防护隔离 ;3、飞沫隔离
( 4 )一次性使用的利器,如针头等放置在防刺、防渗漏的容器内进行 无害化处理。
标准预防措施4
道程表B、A制作
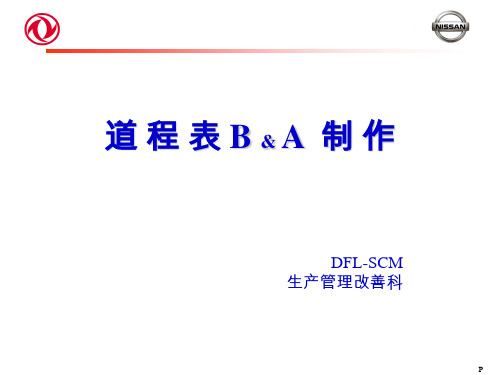
库
动态值
存
静态值
时间
P
一、道程表B
3、道程表B应用符号
Байду номын сангаас
分类 信息
符号
判断内容 文件名 电脑名
实物的
n
流动
n
n
n
说明 工长或作业员的判断及实行的内容
电脑输出等
电脑终端界面
<加工> 冲压、去毛刺、热处理、 切削、装配等
1台加工设备就用一个符号标记。 <检查> 内外径测定、目视等
<停止>
检查设备1台、全数检查场所1处 用1个符号标记。
工序通过时间
生产批量图示
A B C D 一个流或确定了顺序的生产 A +B C+D 以班为单位下达生产计划
A+B+C+D A+B+C+D
以日为单位下达生产计划 以日以上为单位下达生产计划
P
二、道程表A
提示: 道程表B制作时要从线头开始向后编制和测算 道程表A制作时要从线尾开始向前绘制和测算
P
道 程 表 B &A 制 作
DFL-SCM 生产管理改善科
P
一、道程表B
1、道程表B的作用
道程表B真实反映(类似于拍照的方式)某时点的生产过程实物 流状态,通过数据化的描述每个管理点的数量、品种、节拍…等要 素,来分析测算生产周期L/T并寻找改善点。
2、道程表B制作时点的选择
道程表B制作的时点应该选择在一天的生产结束之后或生产开始 之前。生产过程是个动态的过程,每个管理点的库存也是动态变化的。
5、数据采集要点
a、将管理要点表分段,确定点数人员数量,每段2-3人负责; b、选择参与点数人员,点数人员必须熟悉现场的品种、存放地; c、确定点数时间,确保在生产线开动前能够完成; d、多品种混流线要全部清点在线品种数量(制作B表时排序); e、点数结果上交并留底,以备核对; f、节拍必须采用实测数据; g、其他数据:生产班次、SNP、搬运频次、换模时间、生产批量…
道程表(讲义)CN
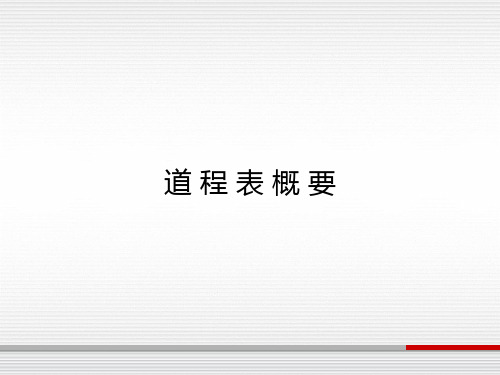
工程改善着眼点
『E C R S』
①排除(Eliminate) 「该工程是否一定需要」
②结合(Combine) 「合并2个以上的工程,变成1个。」
③順序替换(Replace) 「变更工程順序,是否可以去取消一些工程、合并一些」
④简化(Simplify) 「必要工程或作业进行简化」
道程表 事例
工程: 工程A 改善项目表
道程表 事例
工程: 工程A 改善项目表
效果 现状 19节 改善后
【现状物体流】
雨棚 材料
叉车
手
OP1
手
干燥
手
削減数
OP2
OP3
手
手
担当者: 必要工数:
编制:*年*月*日
OP4
手
OP5
完成
装配
手
手
480
80
1
3
1
1
1
1
1
60
2
【改善后物体流】
雨棚 材料 OP1+干燥
OP2
OP3+OP4+OP5
完成
装配
号。
<停滞>
仓库、卸货场、搬运等待 等。
记号
<道程表示例> ※道程表内一个一个记号称为“节”。
绘制例子 ①节(工程名、搬运手段)②在制数 ③配员 ④距离
0.5人
仓库
叉车
线侧
50
10
手搬运
加工机
1
手搬运
50P (35m) ※「P」为1歩 70cm 3P
检查
手搬运 完成品上架
去毛刺+打磨+检查 电动安装
喷雾器
去毛刺、工件安装+打磨+检查
2021年教科版二年级科学下册期中试卷及答案免费
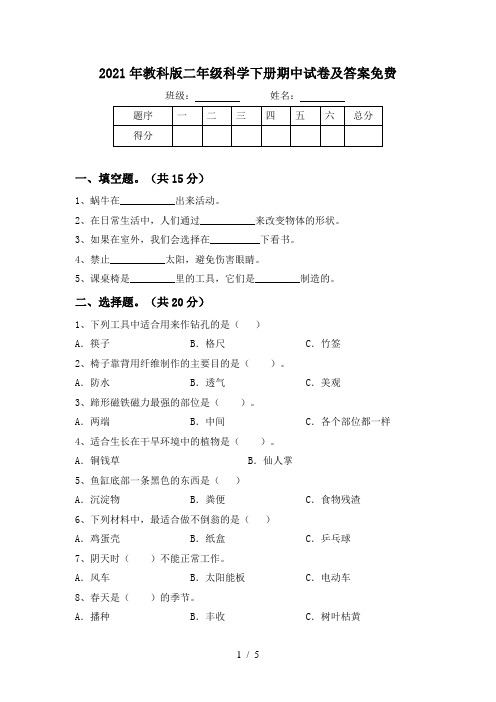
2021年教科版二年级科学下册期中试卷及答案免费班级:姓名:一、填空题。
(共15分)1、蜗牛在___________出来活动。
2、在日常生活中,人们通过___________来改变物体的形状。
3、如果在室外,我们会选择在__________下看书。
4、禁止___________太阳,避免伤害眼睛。
5、课桌椅是_________里的工具,它们是_________制造的。
二、选择题。
(共20分)1、下列工具中适合用来作钻孔的是()A.筷子B.格尺C.竹签2、椅子靠背用纤维制作的主要目的是()。
A.防水B.透气C.美观3、蹄形磁铁磁力最强的部位是()。
A.两端B.中间C.各个部位都一样4、适合生长在干旱环境中的植物是()。
A.铜钱草B.仙人掌5、鱼缸底部一条黑色的东西是()A.沉淀物B.粪便C.食物残渣6、下列材料中,最适合做不倒翁的是()A.鸡蛋壳B.纸盒C.乒乓球7、阴天时()不能正常工作。
A.风车B.太阳能板C.电动车8、春天是()的季节。
A.播种B.丰收C.树叶枯黄9、想要知道花朵是什么颜色,需要()A.用耳朵听B.用眼睛看C.用鼻子闻D.用手摸10、校园里,绝大部分的树叶,()是绿色。
A.秋天B.春天三、判断题。
(共20分)1、相同的磁极会吸在一起。
()2、白天和黑夜对动物、植物、人类的生活都有影响。
()3、猫能通过听觉发现食物。
()4、我们吃的鸡蛋、水果都来自植物。
()5、皮肤是人体器官之一。
()6、钥匙掉进桶里的声音是清脆的。
()7、指甲剪短后不可以再生长。
()8、鞋面用布料做比较透气。
()9、光和热是太阳送给我们的最重要的礼物。
()10、磁铁不仅可以吸铁,还可以吸塑料。
()四、连线题。
(共10分)1、这两位同学分别是用什么力使小车动起来的?推力拉力磁力五、简答题。
(共15分)1、在我们的生活中还有哪些废旧物品可以再利用?(列举三个例子)2、我们的动、植物资源是用不完的吗?你认为在利用和保护动物、植物资源方面应该怎么做?3、健康成长需要良好的生活习惯。
03长输管道通用资料表格概要解读

通用资料表格1、图纸会审记录(一)2、图纸会审记录(二)3、图纸会审记录(三)4、设计交底记录5、交工项目表6、交工主要实物量表7、水工保护工程施工验收单8、技术核定(联络)单9、单位(子单位)工程质量竣工验收申请报告10、单位(子单位)工程质量验收记录11、单位(子单位)工程质量控制资料核查记录表12、检验批质量验收记录13、分项工程验收记录14、分部(子分部)工程质量验收记录15、工序质量报验单16、管沟开挖检验批质量验收记录17、管口组对检验批质量验收记录18、管道焊接检验批质量验收记录19、聚乙烯防腐层现场补口补伤检验批质量验收记录20、管道下沟检验批质量验收记录21、管沟回填检验批质量验收记录22、管道穿越地下管道、线缆检验批质量验收记录23、管道清管、测径检验批质量验收记录24、管道水压试验检验批质量验收记录25、管道气压试验检验批质量验收记录26、输气管道干燥检验批质量验收记录27、管道连头检验批质量验收记录28、牺牲阳极阴极保护检验批质量验收记录29、外加电流阴极保护检验批质量验收记录30、桩墩工程检验批质量验收记录31、线路保护构筑物工程检验批质量验收记录32、导向孔扩孔检验批质量验收记录33、导向孔钻进检验批质量验收记录34、管道回拖检验批质量验收记录图纸会审记录(一)图纸会审记录(二)共页第页图纸会审记录(三)设计交底记录交工项目表交工主要实物量表水工保护工程施工验收单技术核定(联络)单表E.1 _________单位(子单位)工程质量竣工验收申请报告_______________________:(建设单位名称)下列单位(子单位)工程已完工,预试运合格。
根据国家和行业工程施工质量验收规范的规定,下列单位(子单位)工程质量检查评定合格,质量控制资料符合要求。
特申请下列单位(子单位)工程质量竣工验收。
表F.1 _________单位(子单位)工程质量验收记录注:大型项目设计单位必须参加单位(子单位)工程质量验收,中小型项目设计单位可不参加单位(子单位)工程质量验收。
梅霞道人象吉通书全集上兀、下兀、大败、六不成
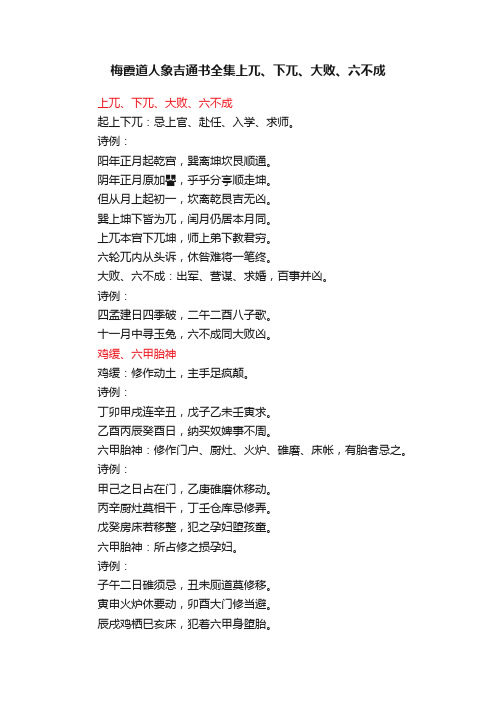
梅霞道人象吉通书全集上兀、下兀、大败、六不成
上兀、下兀、大败、六不成
起上下兀:忌上官、赴任、入学、求师。
诗例:
阳年正月起乾宫,巽离坤坎艮顺通。
阴年正月原加譻,乎乎分亭顺走坤。
但从月上起初一,坎离乾艮吉无凶。
巽上坤下皆为兀,闰月仍居本月同。
上兀本宫下兀坤,师上弟下教君穷。
六轮兀内从头诉,休咎难将一笔终。
大败、六不成:出军、营谋、求婚,百事并凶。
诗例:
四孟建日四季破,二午二酉八子歌。
十一月中寻玉兔,六不成同大败凶。
鸡缓、六甲胎神
鸡缓:修作动土,主手足疯颠。
诗例:
丁卯甲戌连辛丑,戊子乙未壬寅求。
乙酉丙辰癸酉日,纳买奴婢事不周。
六甲胎神:修作门户、厨灶、火炉、碓磨、床帐,有胎者忌之。
诗例:
甲己之日占在门,乙庚碓磨休移动。
丙辛厨灶莫相干,丁壬仓库忌修弄。
戊癸房床若移整,犯之孕妇堕孩童。
六甲胎神:所占修之损孕妇。
诗例:
子午二日碓须忌,丑未厕道莫修移。
寅申火炉休要动,卯酉大门修当避。
辰戌鸡栖巳亥床,犯着六甲身堕胎。
- 1、下载文档前请自行甄别文档内容的完整性,平台不提供额外的编辑、内容补充、找答案等附加服务。
- 2、"仅部分预览"的文档,不可在线预览部分如存在完整性等问题,可反馈申请退款(可完整预览的文档不适用该条件!)。
- 3、如文档侵犯您的权益,请联系客服反馈,我们会尽快为您处理(人工客服工作时间:9:00-18:30)。
装配工程
去毛刺、工件安装+打磨+检查
其他请参考 B表绘制标准书 —(IE专家教育基础课程)
OP1
材料 SNP:80 完成品 SNP: 12
干燥台
OP2
手搬运
在库:5盘×12
OP5
OP4
OP3
装配工程
道程表 事例
工程: 效果
雨棚
工程A
现状
改善项目表
改善后 削減数
OP3
担当者: 必要工数:
OP4 OP5
编制:*年*月*日
19节
【现状物体流】
材料 OP1 干燥 OP2 完成 装配 叉车
手8Βιβλιοθήκη 1道程表 事例工程: 效果
雨棚
工程A
现状
改善项目表
改善后 削減数
OP3
担当者: 必要工数:
OP4 OP5
编制:*年*月*日
19节
【现状物体流】
材料 OP1 干燥 OP2 完成 装配 叉车
手
80 1
手
3
手
1
手
1
手
1
手
1
手
1 2
手
60
480
【改善后物体流】
【改善项目】
【课题・未决事项】
改善着眼点 : 排除(Eliminate)・结合(Combine)・顺序替换(Replace)・简化(Simplify)
手
1 2 60
480
80
2
1
2
【改善项目】 ①干燥工程与OP1统一。 ②干燥用灯得热量〃蒸发水分。 ③OP3,OP4,OP5统一。
【课题、未决事项】
改善着眼点 : 排除(Eliminate)・结合(Combine)・顺序替换(Replace)・简化(Simplify)
道のり表 事例
在库:6盘×80
工程布局图
フォーク
OP1
干燥台
完成品 SNP: 12 材料 SNP:8 0
OP2
手運搬
在库:5盘×12
OP5
OP4
OP3
装配工程
道程表 事例(追求姿态)
追求姿态的布局&改善案
在库:6盘×80
排气管
OP1〒干燥
底涂 干燥
区域传感器
底涂+干燥工位
OP2
S/B
去毛刺+打磨+检查 喷雾器
电动安装
在库:5盘×12OP3+OP4〒OP5
记号
<道程表示例>
0.5人
仓库 叉车 线侧
※道程表内一个一个记号称为“节”。
绘制例子 ①节(工程名、搬运手段)②在制数
手搬运 加工机 手搬运
③配员
检查 手搬运
④距离
完成品上架
50
10
1
3P
1
2P
1
50P (35m) ※「P」为1歩 70cm
「道程表绘制顺序」
道程表事例
在库:6盘×80
工程布局图
叉车
道程表概要
我们可以从这样的地图中找到自己需要的信息
改善手法的种类
目的 見方 角度 宏观 (整体)
工程分析 (道程表)
*ECRS
→
微观 (细部)
动作分析
*动作经济4原 则
(寻找最合适有效的 优良作业方法)
方法研究
M/M组合图
*设备与人的组 合
作业测定
(作业定量化)
稼动分析
*主体作业和附 随比率
速度調査
*作业偏差
要素作業分析
*分析损失
<重点> 目的不同,改善手法分成很多种。要根据目的,选择合适手法。
「道程表定义」
「道程表」是了解「物体流」和「信息流」的工 具。
道程表示例
※道程表用以下记号表示物体流。
<加工> <检查> <停滞>
仓库、卸货场、搬运等待 等。
<搬运>
台车、叉车、手搬运等。
冲压、去毛刺、热处理、 内外径测定、目视等。 切削、装配等 每台检查设备、每个全检场 加工设备每一台一个记 所一个记号。 号。
道程表 事例
工程: 效果
雨棚
工程A
现状
改善项目表
改善后 削減数
OP3
担当者: 必要工数:
OP4 OP5
编制:*年*月*日
19节
【现状物体流】
材料 OP1 干燥 OP2 完成 装配 叉车
手
80 1
手
3
手
1
手
1
手
1
手
1
手
1 2
手
60
480
【改善后物体流】
雨棚 叉车 材料 OP1〒干 燥 手 OP2 手 OP3+OP4+OP5 手 手 完成 装配
手
3
手
1
手
1
手
1
手
1
手
1 2
手
60
480
【改善后物体流】
重点:
不受范围的限制。
【改善项目】 【课题・未决事项】
改善着眼点 : 排除(Eliminate)・结合(Combine)・顺序替换(Replace)・简化(Simplify)
改善着眼点
工程改善着眼点
『E C R S』
①排除(Eliminate) 「该工程是否一定需要」 ②结合(Combine) 「合并2个以上的工程,变成1个。」 ③順序替换(Replace) 「变更工程順序,是否可以去取消一些工程、合并一些」 ④简化(Simplify) 「必要工程或作业进行简化」