Using semi-distributed representations to overcome catastrophic forgetting in connectionist
人工智能领域中英文专有名词汇总
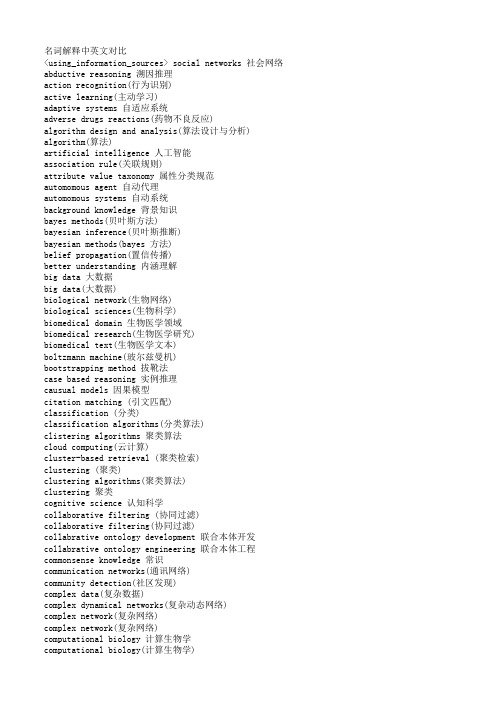
名词解释中英文对比<using_information_sources> social networks 社会网络abductive reasoning 溯因推理action recognition(行为识别)active learning(主动学习)adaptive systems 自适应系统adverse drugs reactions(药物不良反应)algorithm design and analysis(算法设计与分析) algorithm(算法)artificial intelligence 人工智能association rule(关联规则)attribute value taxonomy 属性分类规范automomous agent 自动代理automomous systems 自动系统background knowledge 背景知识bayes methods(贝叶斯方法)bayesian inference(贝叶斯推断)bayesian methods(bayes 方法)belief propagation(置信传播)better understanding 内涵理解big data 大数据big data(大数据)biological network(生物网络)biological sciences(生物科学)biomedical domain 生物医学领域biomedical research(生物医学研究)biomedical text(生物医学文本)boltzmann machine(玻尔兹曼机)bootstrapping method 拔靴法case based reasoning 实例推理causual models 因果模型citation matching (引文匹配)classification (分类)classification algorithms(分类算法)clistering algorithms 聚类算法cloud computing(云计算)cluster-based retrieval (聚类检索)clustering (聚类)clustering algorithms(聚类算法)clustering 聚类cognitive science 认知科学collaborative filtering (协同过滤)collaborative filtering(协同过滤)collabrative ontology development 联合本体开发collabrative ontology engineering 联合本体工程commonsense knowledge 常识communication networks(通讯网络)community detection(社区发现)complex data(复杂数据)complex dynamical networks(复杂动态网络)complex network(复杂网络)complex network(复杂网络)computational biology 计算生物学computational biology(计算生物学)computational complexity(计算复杂性) computational intelligence 智能计算computational modeling(计算模型)computer animation(计算机动画)computer networks(计算机网络)computer science 计算机科学concept clustering 概念聚类concept formation 概念形成concept learning 概念学习concept map 概念图concept model 概念模型concept modelling 概念模型conceptual model 概念模型conditional random field(条件随机场模型) conjunctive quries 合取查询constrained least squares (约束最小二乘) convex programming(凸规划)convolutional neural networks(卷积神经网络) customer relationship management(客户关系管理) data analysis(数据分析)data analysis(数据分析)data center(数据中心)data clustering (数据聚类)data compression(数据压缩)data envelopment analysis (数据包络分析)data fusion 数据融合data generation(数据生成)data handling(数据处理)data hierarchy (数据层次)data integration(数据整合)data integrity 数据完整性data intensive computing(数据密集型计算)data management 数据管理data management(数据管理)data management(数据管理)data miningdata mining 数据挖掘data model 数据模型data models(数据模型)data partitioning 数据划分data point(数据点)data privacy(数据隐私)data security(数据安全)data stream(数据流)data streams(数据流)data structure( 数据结构)data structure(数据结构)data visualisation(数据可视化)data visualization 数据可视化data visualization(数据可视化)data warehouse(数据仓库)data warehouses(数据仓库)data warehousing(数据仓库)database management systems(数据库管理系统)database management(数据库管理)date interlinking 日期互联date linking 日期链接Decision analysis(决策分析)decision maker 决策者decision making (决策)decision models 决策模型decision models 决策模型decision rule 决策规则decision support system 决策支持系统decision support systems (决策支持系统) decision tree(决策树)decission tree 决策树deep belief network(深度信念网络)deep learning(深度学习)defult reasoning 默认推理density estimation(密度估计)design methodology 设计方法论dimension reduction(降维) dimensionality reduction(降维)directed graph(有向图)disaster management 灾害管理disastrous event(灾难性事件)discovery(知识发现)dissimilarity (相异性)distributed databases 分布式数据库distributed databases(分布式数据库) distributed query 分布式查询document clustering (文档聚类)domain experts 领域专家domain knowledge 领域知识domain specific language 领域专用语言dynamic databases(动态数据库)dynamic logic 动态逻辑dynamic network(动态网络)dynamic system(动态系统)earth mover's distance(EMD 距离) education 教育efficient algorithm(有效算法)electric commerce 电子商务electronic health records(电子健康档案) entity disambiguation 实体消歧entity recognition 实体识别entity recognition(实体识别)entity resolution 实体解析event detection 事件检测event detection(事件检测)event extraction 事件抽取event identificaton 事件识别exhaustive indexing 完整索引expert system 专家系统expert systems(专家系统)explanation based learning 解释学习factor graph(因子图)feature extraction 特征提取feature extraction(特征提取)feature extraction(特征提取)feature selection (特征选择)feature selection 特征选择feature selection(特征选择)feature space 特征空间first order logic 一阶逻辑formal logic 形式逻辑formal meaning prepresentation 形式意义表示formal semantics 形式语义formal specification 形式描述frame based system 框为本的系统frequent itemsets(频繁项目集)frequent pattern(频繁模式)fuzzy clustering (模糊聚类)fuzzy clustering (模糊聚类)fuzzy clustering (模糊聚类)fuzzy data mining(模糊数据挖掘)fuzzy logic 模糊逻辑fuzzy set theory(模糊集合论)fuzzy set(模糊集)fuzzy sets 模糊集合fuzzy systems 模糊系统gaussian processes(高斯过程)gene expression data 基因表达数据gene expression(基因表达)generative model(生成模型)generative model(生成模型)genetic algorithm 遗传算法genome wide association study(全基因组关联分析) graph classification(图分类)graph classification(图分类)graph clustering(图聚类)graph data(图数据)graph data(图形数据)graph database 图数据库graph database(图数据库)graph mining(图挖掘)graph mining(图挖掘)graph partitioning 图划分graph query 图查询graph structure(图结构)graph theory(图论)graph theory(图论)graph theory(图论)graph theroy 图论graph visualization(图形可视化)graphical user interface 图形用户界面graphical user interfaces(图形用户界面)health care 卫生保健health care(卫生保健)heterogeneous data source 异构数据源heterogeneous data(异构数据)heterogeneous database 异构数据库heterogeneous information network(异构信息网络) heterogeneous network(异构网络)heterogenous ontology 异构本体heuristic rule 启发式规则hidden markov model(隐马尔可夫模型)hidden markov model(隐马尔可夫模型)hidden markov models(隐马尔可夫模型) hierarchical clustering (层次聚类) homogeneous network(同构网络)human centered computing 人机交互技术human computer interaction 人机交互human interaction 人机交互human robot interaction 人机交互image classification(图像分类)image clustering (图像聚类)image mining( 图像挖掘)image reconstruction(图像重建)image retrieval (图像检索)image segmentation(图像分割)inconsistent ontology 本体不一致incremental learning(增量学习)inductive learning (归纳学习)inference mechanisms 推理机制inference mechanisms(推理机制)inference rule 推理规则information cascades(信息追随)information diffusion(信息扩散)information extraction 信息提取information filtering(信息过滤)information filtering(信息过滤)information integration(信息集成)information network analysis(信息网络分析) information network mining(信息网络挖掘) information network(信息网络)information processing 信息处理information processing 信息处理information resource management (信息资源管理) information retrieval models(信息检索模型) information retrieval 信息检索information retrieval(信息检索)information retrieval(信息检索)information science 情报科学information sources 信息源information system( 信息系统)information system(信息系统)information technology(信息技术)information visualization(信息可视化)instance matching 实例匹配intelligent assistant 智能辅助intelligent systems 智能系统interaction network(交互网络)interactive visualization(交互式可视化)kernel function(核函数)kernel operator (核算子)keyword search(关键字检索)knowledege reuse 知识再利用knowledgeknowledgeknowledge acquisitionknowledge base 知识库knowledge based system 知识系统knowledge building 知识建构knowledge capture 知识获取knowledge construction 知识建构knowledge discovery(知识发现)knowledge extraction 知识提取knowledge fusion 知识融合knowledge integrationknowledge management systems 知识管理系统knowledge management 知识管理knowledge management(知识管理)knowledge model 知识模型knowledge reasoningknowledge representationknowledge representation(知识表达) knowledge sharing 知识共享knowledge storageknowledge technology 知识技术knowledge verification 知识验证language model(语言模型)language modeling approach(语言模型方法) large graph(大图)large graph(大图)learning(无监督学习)life science 生命科学linear programming(线性规划)link analysis (链接分析)link prediction(链接预测)link prediction(链接预测)link prediction(链接预测)linked data(关联数据)location based service(基于位置的服务) loclation based services(基于位置的服务) logic programming 逻辑编程logical implication 逻辑蕴涵logistic regression(logistic 回归)machine learning 机器学习machine translation(机器翻译)management system(管理系统)management( 知识管理)manifold learning(流形学习)markov chains 马尔可夫链markov processes(马尔可夫过程)matching function 匹配函数matrix decomposition(矩阵分解)matrix decomposition(矩阵分解)maximum likelihood estimation(最大似然估计)medical research(医学研究)mixture of gaussians(混合高斯模型)mobile computing(移动计算)multi agnet systems 多智能体系统multiagent systems 多智能体系统multimedia 多媒体natural language processing 自然语言处理natural language processing(自然语言处理) nearest neighbor (近邻)network analysis( 网络分析)network analysis(网络分析)network analysis(网络分析)network formation(组网)network structure(网络结构)network theory(网络理论)network topology(网络拓扑)network visualization(网络可视化)neural network(神经网络)neural networks (神经网络)neural networks(神经网络)nonlinear dynamics(非线性动力学)nonmonotonic reasoning 非单调推理nonnegative matrix factorization (非负矩阵分解) nonnegative matrix factorization(非负矩阵分解) object detection(目标检测)object oriented 面向对象object recognition(目标识别)object recognition(目标识别)online community(网络社区)online social network(在线社交网络)online social networks(在线社交网络)ontology alignment 本体映射ontology development 本体开发ontology engineering 本体工程ontology evolution 本体演化ontology extraction 本体抽取ontology interoperablity 互用性本体ontology language 本体语言ontology mapping 本体映射ontology matching 本体匹配ontology versioning 本体版本ontology 本体论open government data 政府公开数据opinion analysis(舆情分析)opinion mining(意见挖掘)opinion mining(意见挖掘)outlier detection(孤立点检测)parallel processing(并行处理)patient care(病人医疗护理)pattern classification(模式分类)pattern matching(模式匹配)pattern mining(模式挖掘)pattern recognition 模式识别pattern recognition(模式识别)pattern recognition(模式识别)personal data(个人数据)prediction algorithms(预测算法)predictive model 预测模型predictive models(预测模型)privacy preservation(隐私保护)probabilistic logic(概率逻辑)probabilistic logic(概率逻辑)probabilistic model(概率模型)probabilistic model(概率模型)probability distribution(概率分布)probability distribution(概率分布)project management(项目管理)pruning technique(修剪技术)quality management 质量管理query expansion(查询扩展)query language 查询语言query language(查询语言)query processing(查询处理)query rewrite 查询重写question answering system 问答系统random forest(随机森林)random graph(随机图)random processes(随机过程)random walk(随机游走)range query(范围查询)RDF database 资源描述框架数据库RDF query 资源描述框架查询RDF repository 资源描述框架存储库RDF storge 资源描述框架存储real time(实时)recommender system(推荐系统)recommender system(推荐系统)recommender systems 推荐系统recommender systems(推荐系统)record linkage 记录链接recurrent neural network(递归神经网络) regression(回归)reinforcement learning 强化学习reinforcement learning(强化学习)relation extraction 关系抽取relational database 关系数据库relational learning 关系学习relevance feedback (相关反馈)resource description framework 资源描述框架restricted boltzmann machines(受限玻尔兹曼机) retrieval models(检索模型)rough set theroy 粗糙集理论rough set 粗糙集rule based system 基于规则系统rule based 基于规则rule induction (规则归纳)rule learning (规则学习)rule learning 规则学习schema mapping 模式映射schema matching 模式匹配scientific domain 科学域search problems(搜索问题)semantic (web) technology 语义技术semantic analysis 语义分析semantic annotation 语义标注semantic computing 语义计算semantic integration 语义集成semantic interpretation 语义解释semantic model 语义模型semantic network 语义网络semantic relatedness 语义相关性semantic relation learning 语义关系学习semantic search 语义检索semantic similarity 语义相似度semantic similarity(语义相似度)semantic web rule language 语义网规则语言semantic web 语义网semantic web(语义网)semantic workflow 语义工作流semi supervised learning(半监督学习)sensor data(传感器数据)sensor networks(传感器网络)sentiment analysis(情感分析)sentiment analysis(情感分析)sequential pattern(序列模式)service oriented architecture 面向服务的体系结构shortest path(最短路径)similar kernel function(相似核函数)similarity measure(相似性度量)similarity relationship (相似关系)similarity search(相似搜索)similarity(相似性)situation aware 情境感知social behavior(社交行为)social influence(社会影响)social interaction(社交互动)social interaction(社交互动)social learning(社会学习)social life networks(社交生活网络)social machine 社交机器social media(社交媒体)social media(社交媒体)social media(社交媒体)social network analysis 社会网络分析social network analysis(社交网络分析)social network(社交网络)social network(社交网络)social science(社会科学)social tagging system(社交标签系统)social tagging(社交标签)social web(社交网页)sparse coding(稀疏编码)sparse matrices(稀疏矩阵)sparse representation(稀疏表示)spatial database(空间数据库)spatial reasoning 空间推理statistical analysis(统计分析)statistical model 统计模型string matching(串匹配)structural risk minimization (结构风险最小化) structured data 结构化数据subgraph matching 子图匹配subspace clustering(子空间聚类)supervised learning( 有support vector machine 支持向量机support vector machines(支持向量机)system dynamics(系统动力学)tag recommendation(标签推荐)taxonmy induction 感应规范temporal logic 时态逻辑temporal reasoning 时序推理text analysis(文本分析)text anaylsis 文本分析text classification (文本分类)text data(文本数据)text mining technique(文本挖掘技术)text mining 文本挖掘text mining(文本挖掘)text summarization(文本摘要)thesaurus alignment 同义对齐time frequency analysis(时频分析)time series analysis( 时time series data(时间序列数据)time series data(时间序列数据)time series(时间序列)topic model(主题模型)topic modeling(主题模型)transfer learning 迁移学习triple store 三元组存储uncertainty reasoning 不精确推理undirected graph(无向图)unified modeling language 统一建模语言unsupervisedupper bound(上界)user behavior(用户行为)user generated content(用户生成内容)utility mining(效用挖掘)visual analytics(可视化分析)visual content(视觉内容)visual representation(视觉表征)visualisation(可视化)visualization technique(可视化技术) visualization tool(可视化工具)web 2.0(网络2.0)web forum(web 论坛)web mining(网络挖掘)web of data 数据网web ontology lanuage 网络本体语言web pages(web 页面)web resource 网络资源web science 万维科学web search (网络检索)web usage mining(web 使用挖掘)wireless networks 无线网络world knowledge 世界知识world wide web 万维网world wide web(万维网)xml database 可扩展标志语言数据库附录 2 Data Mining 知识图谱(共包含二级节点15 个,三级节点93 个)间序列分析)监督学习)领域 二级分类 三级分类。
ITERATIVELY WEIGHTED MMSE APPROACH TO DISTRIBUTED SUM-UTILITY MAXIMIZATION FOR INTERFERING CHANNEL
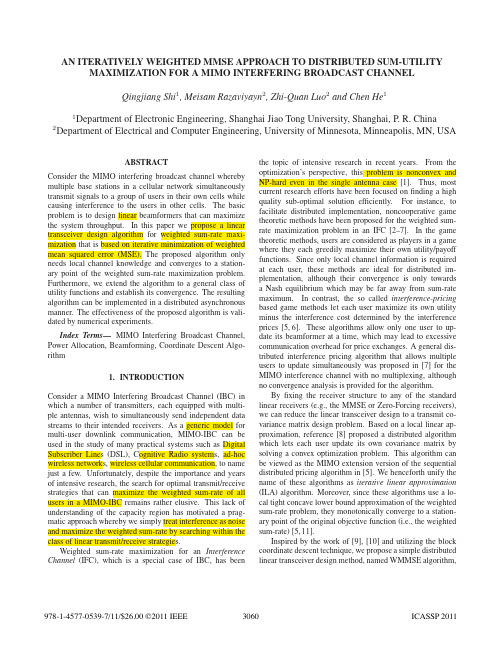
1
Consider the MIMO interfering broadcast channel whereby multiple base stations in a cellular network simultaneously transmit signals to a group of users in their own cells while causing interference to the users in other cells. The basic problem is to design linear beamformers that can maximize the system throughput. In this paper we propose a linear transceiver design algorithm for weighted sum-rate maximization that is based on iterative minimization of weighted mean squared error (MSE). The proposed algorithm only needs local channel knowledge and converges to a stationary point of the weighted sum-rate maximization problem. Furthermore, we extend the algorithm to a general class of utility functions and establish its convergence. The resulting algorithm can be implemented in a distributed asynchronous manner. The effectiveness of the proposed algorithm is validated by numerical experiments. Index Terms— MIMO Interfering Broadcast Channel, Power Allocation, Beamforming, Coordinate Descent Algorithm 1. INTRODUCTION Consider a MIMO Interfering Broadcast Channel (IBC) in which a number of transmitters, each equipped with multiple antennas, wish to simultaneously send independent data streams to their intended receivers. As a generic model for multi-user downlink communication, MIMO-IBC can be used in the study of many practical systems such as Digital Subscriber Lines (DSL), Cognitive Radio systems, ad-hoc wireless networks, wireless cellular communication, to name just a few. Unfortunately, despite the importance and years of intensive research, the search for optimal transmit/receive strategies that can maximize the weighted sum-rate of all users in a MIMO-IBC remains rather elusive. This lack of understanding of the capacity region has motivated a pragmatic approach whereby we simply treat interference as noise and maximize the weighted sum-rate by searching within the class of linear transmit/receive strategies. Weighted sum-rate maximization for an Interference Channel (IFC), which is a special case of IBC, has been
语义分析的一些方法

语义分析的一些方法语义分析的一些方法(上篇)•5040语义分析,本文指运用各种机器学习方法,挖掘与学习文本、图片等的深层次概念。
wikipedia上的解释:In machine learning, semantic analysis of a corpus is the task of building structures that approximate concepts from a large set of documents(or images)。
工作这几年,陆陆续续实践过一些项目,有搜索广告,社交广告,微博广告,品牌广告,内容广告等。
要使我们广告平台效益最大化,首先需要理解用户,Context(将展示广告的上下文)和广告,才能将最合适的广告展示给用户。
而这其中,就离不开对用户,对上下文,对广告的语义分析,由此催生了一些子项目,例如文本语义分析,图片语义理解,语义索引,短串语义关联,用户广告语义匹配等。
接下来我将写一写我所认识的语义分析的一些方法,虽说我们在做的时候,效果导向居多,方法理论理解也许并不深入,不过权当个人知识点总结,有任何不当之处请指正,谢谢。
本文主要由以下四部分组成:文本基本处理,文本语义分析,图片语义分析,语义分析小结。
先讲述文本处理的基本方法,这构成了语义分析的基础。
接着分文本和图片两节讲述各自语义分析的一些方法,值得注意的是,虽说分为两节,但文本和图片在语义分析方法上有很多共通与关联。
最后我们简单介绍下语义分析在广点通“用户广告匹配”上的应用,并展望一下未来的语义分析方法。
1 文本基本处理在讲文本语义分析之前,我们先说下文本基本处理,因为它构成了语义分析的基础。
而文本处理有很多方面,考虑到本文主题,这里只介绍中文分词以及Term Weighting。
1.1 中文分词拿到一段文本后,通常情况下,首先要做分词。
分词的方法一般有如下几种:•基于字符串匹配的分词方法。
此方法按照不同的扫描方式,逐个查找词库进行分词。
综述Representation learning a review and new perspectives

explanatory factors for the observed input. A good representation is also one that is useful as input to a supervised predictor. Among the various ways of learning representations, this paper focuses on deep learning methods: those that are formed by the composition of multiple non-linear transformations, with the goal of yielding more abstract – and ultimately more useful – representations. Here we survey this rapidly developing area with special emphasis on recent progress. We consider some of the fundamental questions that have been driving research in this area. Specifically, what makes one representation better than another? Given an example, how should we compute its representation, i.e. perform feature extraction? Also, what are appropriate objectives for learning good representations?
基于弹性网和直方图相交的非负局部稀疏编码

DOI: 10. 11772 / j. issn. 1001-9081. 2018071483
基于弹性网和直方图相交的非负局部稀疏编码
*பைடு நூலகம்
万 源,张景会 ,陈治平,孟晓静
( 武汉理工大学 理学院,武汉 430070) ( * 通信作者电子邮箱 Jingzhang@ whut. edu. cn)
摘 要: 针对稀疏编码模型在字典基的选择时忽略了群效应,且欧氏距离不能有效度量特征与字典基之间距离 的问题,提出基于弹性网和直方图相交的非负局部稀疏编码方法( EH-NLSC) 。首先,在优化函数中引入弹性网模型, 消除字典基选择数目的限制,能够选择多组相关特征而排除冗余特征,提高了编码的判别性和有效性。然后,在局部 性约束中引入直方图相交,重新定义特征与字典基之间的距离,确保相似的特征可以共享其局部的基。最后采用多 类线性支持向量机进行分类。在 4 个公共数据集上的实验结果表明,与局部线性约束的编码算法( LLC) 和基于非负 弹性网的稀疏编码算法( NENSC) 相比,EH-NLSC 的分类准确率分别平均提升了 10 个百分点和 9 个百分点,充分体现 了其在图像表示和分类中的有效性。
Key words: sparse coding; elastic net model; locality; histogram intersection; image classification
0 引言
图像分类是计算机视觉领域的一个重要研究方向,广泛 应用于生物特征识别、网络图像检索和机器人视觉等领域,其 关键在于如何提取特征对图像有效表示。稀疏编码是图像特 征表示 的 有 效 方 法。考 虑 到 词 袋 ( Bag of Words,BoW) 模 型[1]和空 间 金 字 塔 匹 配 ( Spatial Pyramid Matching,SPM) 模 型[2]容易造成量化误差,Yang 等[3] 结合 SPM 模型提出利用 稀疏编 码 的 空 间 金 字 塔 的 图 像 分 类 算 法 ( Spatial Pyramid Matching using Sparse Coding,ScSPM) ,在图像的不同尺度上 进行稀疏编码,取得了较好的分类效果。在稀疏编码模型中, 由于 1 范数在字典基选择时只考虑稀疏性而忽略了群体效 应,Zou 等[4]提出一种新的正则化方法,将弹性网作为正则项 和变量选择方法。Zhang 等[5]提出判别式弹性网正则化线性
半监督深度学习图像分类方法研究综述

半监督深度学习图像分类方法研究综述吕昊远+,俞璐,周星宇,邓祥陆军工程大学通信工程学院,南京210007+通信作者E-mail:*******************摘要:作为人工智能领域近十年来最受关注的技术之一,深度学习在诸多应用中取得了优异的效果,但目前的学习策略严重依赖大量的有标记数据。
在许多实际问题中,获得众多有标记的训练数据并不可行,因此加大了模型的训练难度,但容易获得大量无标记的数据。
半监督学习充分利用无标记数据,提供了在有限标记数据条件下提高模型性能的解决思路和有效方法,在图像分类任务中达到了很高的识别精准度。
首先对于半监督学习进行概述,然后介绍了分类算法中常用的基本思想,重点对近年来基于半监督深度学习框架的图像分类方法,包括多视图训练、一致性正则、多样混合和半监督生成对抗网络进行全面的综述,总结多种方法共有的技术,分析比较不同方法的实验效果差异,最后思考当前存在的问题并展望未来可行的研究方向。
关键词:半监督深度学习;多视图训练;一致性正则;多样混合;半监督生成对抗网络文献标志码:A中图分类号:TP391.4Review of Semi-supervised Deep Learning Image Classification MethodsLYU Haoyuan +,YU Lu,ZHOU Xingyu,DENG XiangCollege of Communication Engineering,Army Engineering University of PLA,Nanjing 210007,ChinaAbstract:As one of the most concerned technologies in the field of artificial intelligence in recent ten years,deep learning has achieved excellent results in many applications,but the current learning strategies rely heavily on a large number of labeled data.In many practical problems,it is not feasible to obtain a large number of labeled training data,so it increases the training difficulty of the model.But it is easy to obtain a large number of unlabeled data.Semi-supervised learning makes full use of unlabeled data,provides solutions and effective methods to improve the performance of the model under the condition of limited labeled data,and achieves high recognition accuracy in the task of image classification.This paper first gives an overview of semi-supervised learning,and then introduces the basic ideas commonly used in classification algorithms.It focuses on the comprehensive review of image classification methods based on semi-supervised deep learning framework in recent years,including multi-view training,consistency regularization,diversity mixing and semi-supervised generative adversarial networks.It summarizes the common technologies of various methods,analyzes and compares the differences of experimental results of different methods.Finally,this paper thinks about the existing problems and looks forward to the feasible research direction in the future.Key words:semi-supervised deep learning;multi-view training;consistency regularization;diversity mixing;semi-supervised generative adversarial networks计算机科学与探索1673-9418/2021/15(06)-1038-11doi:10.3778/j.issn.1673-9418.2011020基金项目:国家自然科学基金(61702543)。
关于双语心理词库的表征结构
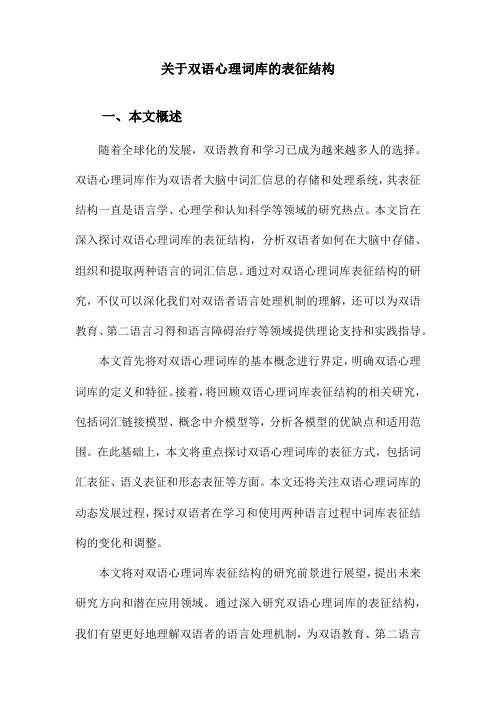
关于双语心理词库的表征结构一、本文概述随着全球化的发展,双语教育和学习已成为越来越多人的选择。
双语心理词库作为双语者大脑中词汇信息的存储和处理系统,其表征结构一直是语言学、心理学和认知科学等领域的研究热点。
本文旨在深入探讨双语心理词库的表征结构,分析双语者如何在大脑中存储、组织和提取两种语言的词汇信息。
通过对双语心理词库表征结构的研究,不仅可以深化我们对双语者语言处理机制的理解,还可以为双语教育、第二语言习得和语言障碍治疗等领域提供理论支持和实践指导。
本文首先将对双语心理词库的基本概念进行界定,明确双语心理词库的定义和特征。
接着,将回顾双语心理词库表征结构的相关研究,包括词汇链接模型、概念中介模型等,分析各模型的优缺点和适用范围。
在此基础上,本文将重点探讨双语心理词库的表征方式,包括词汇表征、语义表征和形态表征等方面。
本文还将关注双语心理词库的动态发展过程,探讨双语者在学习和使用两种语言过程中词库表征结构的变化和调整。
本文将对双语心理词库表征结构的研究前景进行展望,提出未来研究方向和潜在应用领域。
通过深入研究双语心理词库的表征结构,我们有望更好地理解双语者的语言处理机制,为双语教育、第二语言习得和语言障碍治疗等领域提供更有效的理论支持和实践指导。
二、双语心理词库的理论背景双语心理词库的研究是在语言学、心理学、认知科学等多个学科的交叉背景下逐渐发展起来的。
其理论背景主要源于以下几个方面:双语心理词库的研究受到了认知心理学中信息加工理论的影响。
信息加工理论认为,人类大脑在进行信息处理时,会形成一个复杂的认知结构,即心理词库。
这一理论为双语心理词库的研究提供了基础,使我们能够从信息加工的角度去理解和描述双语者的词汇存储和加工过程。
双语心理词库的研究还受到了语言学中词汇理论的影响。
词汇理论认为,词汇是语言的基础,是语言理解和表达的关键。
在双语者的语言中,词汇的存储和加工是一个复杂的过程,涉及到两种语言的词汇之间的相互影响和相互作用。
代数英语

(0,2) 插值||(0,2) interpolation0#||zero-sharp; 读作零井或零开。
0+||zero-dagger; 读作零正。
1-因子||1-factor3-流形||3-manifold; 又称“三维流形”。
AIC准则||AIC criterion, Akaike information criterionAp 权||Ap-weightA稳定性||A-stability, absolute stabilityA最优设计||A-optimal designBCH 码||BCH code, Bose-Chaudhuri-Hocquenghem codeBIC准则||BIC criterion, Bayesian modification of the AICBMOA函数||analytic function of bounded mean oscillation; 全称“有界平均振动解析函数”。
BMO鞅||BMO martingaleBSD猜想||Birch and Swinnerton-Dyer conjecture; 全称“伯奇与斯温纳顿-戴尔猜想”。
B样条||B-splineC*代数||C*-algebra; 读作“C星代数”。
C0 类函数||function of class C0; 又称“连续函数类”。
CA T准则||CAT criterion, criterion for autoregressiveCM域||CM fieldCN 群||CN-groupCW 复形的同调||homology of CW complexCW复形||CW complexCW复形的同伦群||homotopy group of CW complexesCW剖分||CW decompositionCn 类函数||function of class Cn; 又称“n次连续可微函数类”。
Cp统计量||Cp-statisticC。
词向量算法的使用教程及语义关联分析
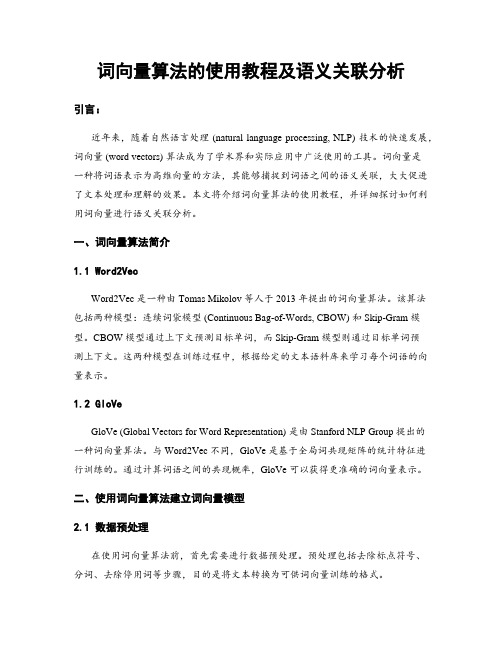
词向量算法的使用教程及语义关联分析引言:近年来,随着自然语言处理 (natural language processing, NLP) 技术的快速发展,词向量 (word vectors) 算法成为了学术界和实际应用中广泛使用的工具。
词向量是一种将词语表示为高维向量的方法,其能够捕捉到词语之间的语义关联,大大促进了文本处理和理解的效果。
本文将介绍词向量算法的使用教程,并详细探讨如何利用词向量进行语义关联分析。
一、词向量算法简介1.1 Word2VecWord2Vec 是一种由 Tomas Mikolov 等人于 2013 年提出的词向量算法。
该算法包括两种模型:连续词袋模型 (Continuous Bag-of-Words, CBOW) 和 Skip-Gram 模型。
CBOW 模型通过上下文预测目标单词,而 Skip-Gram 模型则通过目标单词预测上下文。
这两种模型在训练过程中,根据给定的文本语料库来学习每个词语的向量表示。
1.2 GloVeGloVe (Global Vectors for Word Representation) 是由 Stanford NLP Group 提出的一种词向量算法。
与 Word2Vec 不同,GloVe 是基于全局词共现矩阵的统计特征进行训练的。
通过计算词语之间的共现概率,GloVe 可以获得更准确的词向量表示。
二、使用词向量算法建立词向量模型2.1 数据预处理在使用词向量算法前,首先需要进行数据预处理。
预处理包括去除标点符号、分词、去除停用词等步骤,目的是将文本转换为可供词向量训练的格式。
2.2 训练词向量模型使用预处理后的文本语料库,我们可以开始训练词向量模型。
对于 Word2Vec算法,可以选择使用 CBOW 模型或 Skip-Gram 模型。
通过调整模型参数,如窗口大小、向量维度等,可以优化词向量模型的性能。
2.3 优化词向量模型在训练词向量模型之后,我们可以通过一些优化算法进一步改进词向量的性能。
distributed representations of words and phrases and their compositionality
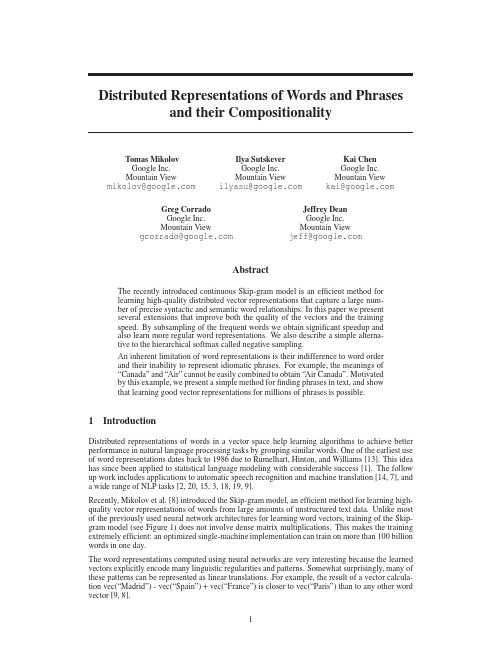
Tomas MikolovGoogle Inc.Mountain View mikolov@Ilya SutskeverGoogle Inc.Mountain Viewilyasu@Kai ChenGoogle Inc.Mountain Viewkai@Greg CorradoGoogle Inc.Mountain View gcorrado@Jeffrey DeanGoogle Inc.Mountain View jeff@AbstractThe recently introduced continuous Skip-gram model is an efficient method forlearning high-quality distributed vector representations that capture a large num-ber of precise syntactic and semantic word relationships.In this paper we presentseveral extensions that improve both the quality of the vectors and the trainingspeed.By subsampling of the frequent words we obtain significant speedup andalso learn more regular word representations.We also describe a simple alterna-tive to the hierarchical softmax called negative sampling.An inherent limitation of word representations is their indifference to word orderand their inability to represent idiomatic phrases.For example,the meanings of“Canada”and“Air”cannot be easily combined to obtain“Air Canada”.Motivatedby this example,we present a simple method forfinding phrases in text,and showthat learning good vector representations for millions of phrases is possible.1IntroductionDistributed representations of words in a vector space help learning algorithms to achieve better performance in natural language processing tasks by grouping similar words.One of the earliest use of word representations dates back to1986due to Rumelhart,Hinton,and Williams[13].This idea has since been applied to statistical language modeling with considerable success[1].The follow up work includes applications to automatic speech recognition and machine translation[14,7],and a wide range of NLP tasks[2,20,15,3,18,19,9].Recently,Mikolov et al.[8]introduced the Skip-gram model,an efficient method for learning high-quality vector representations of words from large amounts of unstructured text data.Unlike most of the previously used neural network architectures for learning word vectors,training of the Skip-gram model(see Figure1)does not involve dense matrix multiplications.This makes the training extremely efficient:an optimized single-machine implementation can train on more than100billion words in one day.The word representations computed using neural networks are very interesting because the learned vectors explicitly encode many linguistic regularities and patterns.Somewhat surprisingly,many of these patterns can be represented as linear translations.For example,the result of a vector calcula-tion vec(“Madrid”)-vec(“Spain”)+vec(“France”)is closer to vec(“Paris”)than to any other word vector[9,8].Figure1:The Skip-gram vector representations that are good at predictingIn this paper we We show that sub-sampling of frequent(around2x-10x),and improves accuracy of we present a simpli-fied variant of Noise model that results in faster training and better vector representations for frequent words,compared to more complex hierarchical softmax that was used in the prior work[8].Word representations are limited by their inability to represent idiomatic phrases that are not com-positions of the individual words.For example,“Boston Globe”is a newspaper,and so it is not a natural combination of the meanings of“Boston”and“Globe”.Therefore,using vectors to repre-sent the whole phrases makes the Skip-gram model considerably more expressive.Other techniques that aim to represent meaning of sentences by composing the word vectors,such as the recursive autoencoders[15],would also benefit from using phrase vectors instead of the word vectors.The extension from word based to phrase based models is relatively simple.First we identify a large number of phrases using a data-driven approach,and then we treat the phrases as individual tokens during the training.To evaluate the quality of the phrase vectors,we developed a test set of analogi-cal reasoning tasks that contains both words and phrases.A typical analogy pair from our test set is “Montreal”:“Montreal Canadiens”::“Toronto”:“Toronto Maple Leafs”.It is considered to have been answered correctly if the nearest representation to vec(“Montreal Canadiens”)-vec(“Montreal”)+ vec(“Toronto”)is vec(“Toronto Maple Leafs”).Finally,we describe another interesting property of the Skip-gram model.We found that simple vector addition can often produce meaningful results.For example,vec(“Russia”)+vec(“river”)is close to vec(“V olga River”),and vec(“Germany”)+vec(“capital”)is close to vec(“Berlin”).This compositionality suggests that a non-obvious degree of language understanding can be obtained by using basic mathematical operations on the word vector representations.2The Skip-gram ModelThe training objective of the Skip-gram model is tofind word representations that are useful for predicting the surrounding words in a sentence or a document.More formally,given a sequence of training words w1,w2,w3,...,w T,the objective of the Skip-gram model is to maximize the average log probability1training time.The basic Skip-gram formulation defines p(w t+j|w t)using the softmax function:exp v′w O⊤v w Ip(w O|w I)=-2-1.5-1-0.5 0 0.511.5 2-2-1.5-1-0.5 0 0.5 1 1.5 2Country and Capital Vectors Projected by PCAChinaJapanFranceRussiaGermanyItalySpainGreece TurkeyBeijingParis Tokyo PolandMoscow Portugal Berlin Rome Athens MadridAnkara Warsaw LisbonFigure 2:Two-dimensional PCA projection of the 1000-dimensional Skip-gram vectors of countries and their capital cities.The figure illustrates ability of the model to automatically organize concepts and learn implicitly the relationships between them,as during the training we did not provide any supervised information about what a capital city means.which is used to replace every log P (w O |w I )term in the Skip-gram objective.Thus the task is to distinguish the target word w O from draws from the noise distribution P n (w )using logistic regres-sion,where there are k negative samples for each data sample.Our experiments indicate that values of k in the range 5–20are useful for small training datasets,while for large datasets the k can be as small as 2–5.The main difference between the Negative sampling and NCE is that NCE needs both samples and the numerical probabilities of the noise distribution,while Negative sampling uses only samples.And while NCE approximately maximizes the log probability of the softmax,this property is not important for our application.Both NCE and NEG have the noise distribution P n (w )as a free parameter.We investigated a number of choices for P n (w )and found that the unigram distribution U (w )raised to the 3/4rd power (i.e.,U (w )3/4/Z )outperformed significantly the unigram and the uniform distributions,for both NCE and NEG on every task we tried including language modeling (not reported here).2.3Subsampling of Frequent WordsIn very large corpora,the most frequent words can easily occur hundreds of millions of times (e.g.,“in”,“the”,and “a”).Such words usually provide less information value than the rare words.For example,while the Skip-gram model benefits from observing the co-occurrences of “France”and “Paris”,it benefits much less from observing the frequent co-occurrences of “France”and “the”,as nearly every word co-occurs frequently within a sentence with “the”.This idea can also be applied in the opposite direction;the vector representations of frequent words do not change significantly after training on several million examples.To counter the imbalance between the rare and frequent words,we used a simple subsampling ap-proach:each word w i in the training set is discarded with probability computed by the formulaP (w i )=1− f (w i )(5)Method Syntactic[%]Semantic[%]NEG-563549761 HS-Huffman53403853NEG-561583661 HS-Huffman5259/p/word2vec/source/browse/trunk/questions-words.txtNewspapersNHL TeamsNBA TeamsAirlinesCompany executives.(6)count(w i)×count(w j)Theδis used as a discounting coefficient and prevents too many phrases consisting of very infre-quent words to be formed.The bigrams with score above the chosen threshold are then used as phrases.Typically,we run2-4passes over the training data with decreasing threshold value,allow-ing longer phrases that consists of several words to be formed.We evaluate the quality of the phrase representations using a new analogical reasoning task that involves phrases.Table2shows examples of thefive categories of analogies used in this task.This dataset is publicly available on the web2.4.1Phrase Skip-Gram ResultsStarting with the same news data as in the previous experiments,wefirst constructed the phrase based training corpus and then we trained several Skip-gram models using different hyper-parameters.As before,we used vector dimensionality300and context size5.This setting already achieves good performance on the phrase dataset,and allowed us to quickly compare the Negative Sampling and the Hierarchical Softmax,both with and without subsampling of the frequent tokens. The results are summarized in Table3.The results show that while Negative Sampling achieves a respectable accuracy even with k=5, using k=15achieves considerably better performance.Surprisingly,while we found the Hierar-chical Softmax to achieve lower performance when trained without subsampling,it became the best performing method when we downsampled the frequent words.This shows that the subsampling can result in faster training and can also improve accuracy,at least in some cases.Dimensionality10−5subsampling[%]30027NEG-152730047Table3:Accuracies of the Skip-gram models on the phrase analogy dataset.The models were trained on approximately one billion words from the news dataset.HS with10−5subsamplingLingsugurGreat Rift ValleyRebbeca NaomiRuegenchess grandmasterVietnam+capital Russian+riverkoruna airline Lufthansa Juliette Binoche Check crown carrier Lufthansa Vanessa Paradis Polish zoltyflag carrier Lufthansa Charlotte Gainsbourg CTK Lufthansa Cecile De Table5:Vector compositionality using element-wise addition.Four closest tokens to the sum of two vectors are shown,using the best Skip-gram model.To maximize the accuracy on the phrase analogy task,we increased the amount of the training data by using a dataset with about33billion words.We used the hierarchical softmax,dimensionality of1000,and the entire sentence for the context.This resulted in a model that reached an accuracy of72%.We achieved lower accuracy66%when we reduced the size of the training dataset to6B words,which suggests that the large amount of the training data is crucial.To gain further insight into how different the representations learned by different models are,we did inspect manually the nearest neighbours of infrequent phrases using various models.In Table4,we show a sample of such comparison.Consistently with the previous results,it seems that the best representations of phrases are learned by a model with the hierarchical softmax and subsampling. 5Additive CompositionalityWe demonstrated that the word and phrase representations learned by the Skip-gram model exhibit a linear structure that makes it possible to perform precise analogical reasoning using simple vector arithmetics.Interestingly,we found that the Skip-gram representations exhibit another kind of linear structure that makes it possible to meaningfully combine words by an element-wise addition of their vector representations.This phenomenon is illustrated in Table5.The additive property of the vectors can be explained by inspecting the training objective.The word vectors are in a linear relationship with the inputs to the softmax nonlinearity.As the word vectors are trained to predict the surrounding words in the sentence,the vectors can be seen as representing the distribution of the context in which a word appears.These values are related logarithmically to the probabilities computed by the output layer,so the sum of two word vectors is related to the product of the two context distributions.The product works here as the AND function:words that are assigned high probabilities by both word vectors will have high probability,and the other words will have low probability.Thus,if“V olga River”appears frequently in the same sentence together with the words“Russian”and“river”,the sum of these two word vectors will result in such a feature vector that is close to the vector of“V olga River”.6Comparison to Published Word RepresentationsMany authors who previously worked on the neural network based representations of words have published their resulting models for further use and comparison:amongst the most well known au-thors are Collobert and Weston[2],Turian et al.[17],and Mnih and Hinton[10].We downloaded their word vectors from the web3.Mikolov et al.[8]have already evaluated these word representa-tions on the word analogy task,where the Skip-gram models achieved the best performance with a huge margin.Model Redmond ninjutsu capitulate (training time)Collobert(50d)conyers reiki abdicate (2months)lubbock kohona accedekeene karate rearmJewell gunfireArzu emotionOvitz impunityMnih(100d)Podhurst-Mavericks (7days)Harlang-planning Agarwal-hesitatedVaclav Havel spray paintpresident Vaclav Havel grafittiVelvet Revolution taggers/p/word2vecReferences[1]Yoshua Bengio,R´e jean Ducharme,Pascal Vincent,and Christian Janvin.A neural probabilistic languagemodel.The Journal of Machine Learning Research,3:1137–1155,2003.[2]Ronan Collobert and Jason Weston.A unified architecture for natural language processing:deep neu-ral networks with multitask learning.In Proceedings of the25th international conference on Machine learning,pages160–167.ACM,2008.[3]Xavier Glorot,Antoine Bordes,and Yoshua Bengio.Domain adaptation for large-scale sentiment classi-fication:A deep learning approach.In ICML,513–520,2011.[4]Michael U Gutmann and Aapo Hyv¨a rinen.Noise-contrastive estimation of unnormalized statistical mod-els,with applications to natural image statistics.The Journal of Machine Learning Research,13:307–361, 2012.[5]Tomas Mikolov,Stefan Kombrink,Lukas Burget,Jan Cernocky,and Sanjeev Khudanpur.Extensions ofrecurrent neural network language model.In Acoustics,Speech and Signal Processing(ICASSP),2011 IEEE International Conference on,pages5528–5531.IEEE,2011.[6]Tomas Mikolov,Anoop Deoras,Daniel Povey,Lukas Burget and Jan Cernocky.Strategies for TrainingLarge Scale Neural Network Language Models.In Proc.Automatic Speech Recognition and Understand-ing,2011.[7]Tomas Mikolov.Statistical Language Models Based on Neural Networks.PhD thesis,PhD Thesis,BrnoUniversity of Technology,2012.[8]Tomas Mikolov,Kai Chen,Greg Corrado,and Jeffrey Dean.Efficient estimation of word representationsin vector space.ICLR Workshop,2013.[9]Tomas Mikolov,Wen-tau Yih and Geoffrey Zweig.Linguistic Regularities in Continuous Space WordRepresentations.In Proceedings of NAACL HLT,2013.[10]Andriy Mnih and Geoffrey E Hinton.A scalable hierarchical distributed language model.Advances inneural information processing systems,21:1081–1088,2009.[11]Andriy Mnih and Yee Whye Teh.A fast and simple algorithm for training neural probabilistic languagemodels.arXiv preprint arXiv:1206.6426,2012.[12]Frederic Morin and Yoshua Bengio.Hierarchical probabilistic neural network language model.In Pro-ceedings of the international workshop on artificial intelligence and statistics,pages246–252,2005. [13]David E Rumelhart,Geoffrey E Hintont,and Ronald J Williams.Learning representations by back-propagating errors.Nature,323(6088):533–536,1986.[14]Holger Schwenk.Continuous space language puter Speech and Language,vol.21,2007.[15]Richard Socher,Cliff C.Lin,Andrew Y.Ng,and Christopher D.Manning.Parsing natural scenes andnatural language with recursive neural networks.In Proceedings of the26th International Conference on Machine Learning(ICML),volume2,2011.[16]Richard Socher,Brody Huval,Christopher D.Manning,and Andrew Y.Ng.Semantic CompositionalityThrough Recursive Matrix-Vector Spaces.In Proceedings of the2012Conference on Empirical Methods in Natural Language Processing(EMNLP),2012.[17]Joseph Turian,Lev Ratinov,and Yoshua Bengio.Word representations:a simple and general method forsemi-supervised learning.In Proceedings of the48th Annual Meeting of the Association for Computa-tional Linguistics,pages384–394.Association for Computational Linguistics,2010.[18]Peter D.Turney and Patrick Pantel.From frequency to meaning:Vector space models of semantics.InJournal of Artificial Intelligence Research,37:141-188,2010.[19]Peter D.Turney.Distributional semantics beyond words:Supervised learning of analogy and paraphrase.In Transactions of the Association for Computational Linguistics(TACL),353–366,2013.[20]Jason Weston,Samy Bengio,and Nicolas Usunier.Wsabie:Scaling up to large vocabulary image annota-tion.In Proceedings of the Twenty-Second international joint conference on Artificial Intelligence-Volume Volume Three,pages2764–2770.AAAI Press,2011.。
海康威视网络录像机快速入门指南说明书
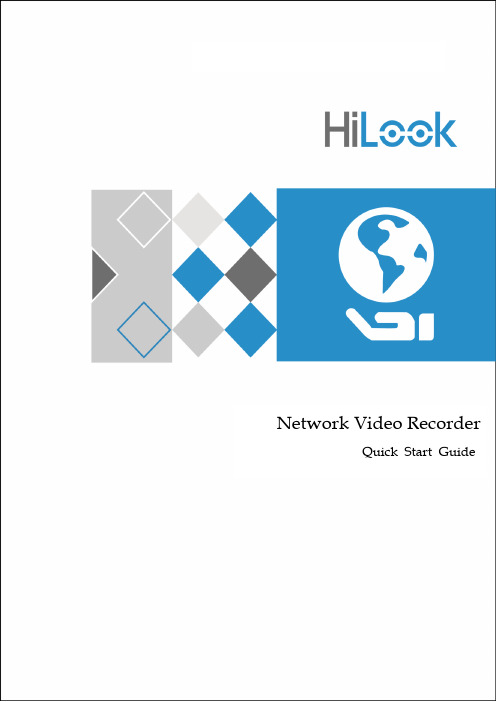
Network Video RecorderQuick Start GuideTABLE OF CONTENTSChapter1 Panels Description (8)1.1 Front Panel (8)1.2 Rear Panel (9)NVR-100H-D and NVR-100MH-D Series (9)NVR-100H-D/P and NVR-100MH-D/P Series (10)Chapter 2 Installation and Connections (11)2.1 NVR Installation (11)2.2 Hard Disk Installation (11)2.3 HDD Storage Calculation Chart (13)Chapter 3 Menu Operation (14)3.1 Startup and Shutdown (14)3.2 Activate Your Device (14)3.3 Set the Unlock Pattern for Login (15)3.4 User Login (16)3.5 Network Settings (16)3.6 Add IP Cameras (17)3.7 Live View (18)3.8 Recording Settings (18)3.9 Playback (19)Chapter 4 Accessing by Web Browser (21)Quick Start GuideCOPYRIGHT ©2019 Hangzhou Hikvision Digital Technology Co., Ltd.ALL RIGHTS RESERVED.Any and all information, including, among others, wordings, pictures, graphs are the properties of Hangzhou Hikvision Digital Technology Co., Ltd. or its subsidiaries (hereinafter referred to be “Hikvision”). This user manual (hereinafter referred to be “the Manual”) cannot be reproduced, changed, translated, or distributed, partially or wholly, by any means, without the prior written permission of Hikvision. Unless otherwise stipulated, Hikvision does not make any warranties, guarantees or representations, express or implied, regarding to the Manual.About this ManualThis Manual is applicable to Network Video Recorder (NVR).The Manual includes instructions for using and managing the product. Pictures, charts, images and all other information hereinafter are for description and explanation only. The information contained in the Manual is subject to change, without notice, due to firmware updates or other reasons. Please find the latest version in the company website (/en/).Please use this user manual under the guidance of professionals.Trademarks Acknowledgementand other Hikvision’s trademarks and logos are the properties of Hikvision in various jurisdictions. Other trademarks and logos mentioned below are the properties of their respective owners.The terms HDMI and HDMI High-Definition Multimedia Interface, and the HDMI Logoare trademarks or registered trademarks of HDMI Licensing Administrator, Inc. in the United States and other countries.Legal DisclaimerTO THE MAXIMUM EXTENT PERMITTED BY APPLICABLE LAW, THE PRODUCT DESCRIBED, WITH ITS HARDWARE, SOFTWARE AND FIRMWARE, IS PROVIDED “AS IS”, WITH ALL FAULTS AND ERRORS, AND HIKVISION MAKES NO WARRANTIES, EXPRESS OR IMPLIED, INCLUDING WITHOUT LIMITATION, MERCHANTABILITY, SATISFACTORY QUALITY, FITNESS FOR A PARTICULAR PURPOSE, AND NON-INFRINGEMENT OF THIRD PARTY. IN NO EVENT WILL HIKVISION, ITS DIRECTORS, OFFICERS, EMPLOYEES, OR AGENTS BE LIABLE TO YOU FOR ANY SPECIAL, CONSEQUENTIAL, INCIDENTAL, OR INDIRECT DAMAGES, INCLUDING, AMONG OTHERS, DAMAGES FOR LOSS OF BUSINESS PROFITS, BUSINESS INTERRUPTION, OR LOSS OF DATA OR DOCUMENTATION, IN CONNECTION WITH THE USE OF THIS PRODUCT, EVEN IF HIKVISION HAS BEEN ADVISED OF THE POSSIBILITY OF SUCH DAMAGES.REGARDING TO THE PRODUCT WITH INTERNET ACCESS, THE USE OF PRODUCT SHALL BE WHOLLY AT YOUR OWN RISKS. HIKVISION SHALL NOT TAKE ANY RESPONSIBILITES FOR ABNORMAL OPERATION, PRIVACY LEAKAGE OR OTHER DAMAGES RESULTING FROM CYBER ATTACK, HACKER ATTACK, VIRUS INSPECTION, OR OTHER INTERNET SECURITY RISKS; HOWEVER, HIKVISION WILL PROVIDE TIMELY TECHNICAL SUPPORT IF REQUIRED.SURVEILLANCE LAWS VARY BY JURISDICTION. PLEASE CHECK ALL RELEVANT LAWS IN YOUR JURISDICTION BEFORE USING THIS PRODUCT IN ORDER TO ENSURE THAT YOUR USE CONFORMSTHE APPLICABLE LAW. HIKVISION SHALL NOT BE LIABLE IN THE EVENT THAT THIS PRODUCT IS USED WITH ILLEGITIMATE PURPOSES.IN THE EVENT OF ANY CONFLICTS BETWEEN THIS MANUAL AND THE APPLICABLE LAW, THE LATER PREVAILS.Regulatory InformationFCC InformationPlease take attention that changes or modification not expressly approved by the party responsible for compliance could void the user’s authority to operate the equipment.FCC compliance: This equipment has been tested and found to comply with the limits for a Class A digital device, pursuant to part 15 of the FCC Rules. These limits are designed to provide reasonable protection against harmful interference when the equipment is operated in a commercial environment. This equipment generates, uses, and can radiate radio frequency energy and, if not installed and used in accordance with the instruction manual, may cause harmful interference to radio communications. Operation of this equipment in a residential area is likely to cause harmful interference in which case the user will be required to correct the interference at his own expense.FCC ConditionsThis device complies with part 15 of the FCC Rules. Operation is subject to the following two conditions:1. This device may not cause harmful interference.2. This device must accept any interference received, including interference that may cause undesired operation.EU Conformity StatementThis product and - if applicable - the supplied accessories too are marked with "CE" and comply therefore with the applicable harmonized European standards listed under the EMC Directive 2014/30/EU, the LVD Directive 2014/35/EU, the RoHS Directive 2011/65/EU.2012/19/EU (WEEE directive): Products marked with this symbol cannot be disposed of as unsorted municipal waste in the European Union. For proper recycling, return this product to your local supplier upon the purchase of equivalent new equipment, or dispose of it at designated collection points. For more information see: 2006/66/EC (battery directive): This product contains a battery that cannot be disposed of as unsorted municipal waste in the European Union. See the product documentation for specific battery information. The battery is marked with this symbol, which may include lettering to indicate cadmium (Cd), lead (Pb), or mercury (Hg). For proper recycling, return the battery to your supplier or to a designated collection point. For more information see: Industry Canada ICES-003 ComplianceThis device meets the CAN ICES-3 (A)/NMB-3(A) standards requirements.Applicable ModelsThis manual is applicable to the models listed in the following table.Series ModelNVR-100H-D NVR-104H-D NVR-108H-DNVR-100H-D/P NVR-104H-D/4P NVR-108H-D/8PNVR-100MH-D NVR-104MH-D NVR-108MH-DNVR-100MH-D/P NVR-104MH-D/4P NVR-108MH-D/8PSymbol ConventionsThe symbols that may be found in this document are defined as follows.Symbol DescriptionProvides additional information to emphasize or supplementimportant points of the main text.Indicates a potentially hazardous situation, which if not avoided,could result in equipment damage, data loss, performancedegradation, or unexpected results.Indicates a hazard with a high level of risk, which if not avoided, willresult in death or serious injury.Safety Instructions●Proper configuration of all passwords and other security settings is the responsibility of theinstaller and/or end-user.●In the use of the product, you must be in strict compliance with the electrical safetyregulations of the nation and region. Please refer to technical specifications for detailedinformation.●Input voltage should meet both the SELV (Safety Extra Low Voltage) and the Limited PowerSource with 100~240 VAC, 48 VDC or 12 VDC according to the IEC60950-1 standard. Please refer to technical specifications for detailed information.●Do not connect several devices to one power adapter as adapter overload may causeover-heating or a fire hazard.●Please make sure that the plug is firmly connected to the power socket.●If smoke, odor or noise rise from the device, turn off the power at once and unplug the powercable, and then please contact the service center.●If the POE ports of device do not comply with Limited Power Source, the additional equipmentconnected to POE ports shall have fire enclosure.●The USB interface of the /P devices can be connected with the mouse and U-flash disk storagedevice only.Preventive and Cautionary TipsBefore connecting and operating your device, please be advised of the following tips:●Ensure unit is installed in a well-ventilated, dust-free environment.●Unit is designed for indoor use only.●Keep all liquids away from the device.●Ensure environmental conditions meet factory specifications.●Ensure unit is properly secured to a rack or shelf. Major shocks or jolts to the unit as a result ofdropping it may cause damage to the sensitive electronics within the unit.●Use the device in conjunction with an UPS if possible.●Power down the unit before connecting and disconnecting accessories and peripherals.● A factory recommended HDD should be used for this device.●Improper use or replacement of the battery may result in hazard of explosion. Replace withthe same or equivalent type only. Dispose of used batteries according to the instructionsprovided by the battery manufacturer.Power Supply InstructionsUse only power supplies listed in the user instructions.NVR Models Standard Power Supply Models ManufacturerNVR-104H-D NVR-108H-D NVR-104MH-D NVR-108MH-D EuropeanMSA-C1500IC12.0-18P-DE MOSO Power Supply Technology Co., LtdADS-26FSG-12 12018EPG Shenzhen HONOR Electronic Co., LtdKL-AD3060VA Xiamen Keli Electronics Co., LtdKPD-018-VI Channel Well Technology Co., Ltd BritishADS-25FSG-12 12018GPB Shenzhen HONOR Electronic Co., LtdMSA-C1500IC12.0-18P-GB MOSO Power Supply Technology Co., LtdADS-26FSG-12 12018EPB Shenzhen HONOR Electronic Co., LtdNVR-104H-D/4PNVR-108H-D/8P NVR-104MH-D/4P NVR-108MH-D/8P UniversalMSP-Z1360IC48.0-65W MOSO Power Supply Technology Co., LtdMSA-Z1040IS48.0-65W-Q MOSO Power Supply Technology Co., LtdMSA-Z1360IS48.0-65W-QMOSO Power Supply Technology Co., Ltd●The power supplies list above is for EU countries only.●The power supplies list is subject to change without prior notice.Chapter1 Panels Description 1.1 Front PanelFigure 1-1NVR-100H-D (/P) SeriesFigure 1-2NVR-100MH-D (/P) SeriesTable 1-1Description of Front Panel No. Icon Description1 Indicator turns red when NVR is powered up.2 Indicator lights in red when data is being read from or written to HDD.3 Indicator blinks blue when network connection is functioning properly.1.2 Rear PanelNVR-100H-D and NVR-100MH-D SeriesFigure 1-3NVR-100H-D Rear PanelFigure 1-4NVR-100MH-D Rear PanelNo. Item Description1 Power Supply 12 VDC power supply.2 VGA Interface DB9 connector for VGA output. Display local videooutput and menu.3 HDMI Interface HDMI video output connector.4 USB Interface Universal Serial Bus (USB) ports for additional devicessuch as USB mouse and USB Hard Disk Drive (HDD).5 LAN Network Interface 10/100 Mbps self-adaptive Ethernet interface.6 Ground Ground (needs to be connected when NVR starts up).NVR-100H-D/P and NVR-100MH-D/P SeriesFigure 1-5NVR-100H-D/P Rear PanelFigure 1-6NVR-100MH-D/P Rear PanelTable 1-3Description of Rear Panel No. Item Description1 Power Supply 12 VDC power supply.2 VGA Interface DB9 connector for VGA output. Display local videooutput and menu.3 HDMI Interface HDMI video output connector.4 USB Interface Universal Serial Bus (USB) ports for additional devicessuch as USB mouse and USB Hard Disk Drive (HDD).5 LAN Network Interface 10/100 Mbps self-adaptive Ethernet interface.6 Ground Ground (needs to be connected when NVR starts up).7 Network Interfaces withPoE functionNetwork interfaces for the cameras and to providepower over Ethernet.4 interfaces for /4P models and 8 interfaces for /8Pmodels.Chapter 2 Installation and Connections2.1 NVR InstallationDuring installation of the NVR:●Use brackets for rack mounting.●Ensure ample room for audio and video cables.●When routing cables, ensure that the bend radius of the cables are no less than five times thanits diameter.●Connect the alarm cable.●Allow at least 2cm (≈0.75-inch) of space between racks mounted devices.●Ensure the NVR is grounded.●Environmental temperature should be within the range of -10 to +55º C, +14 to +131º F.●Environmental humidity should be within the range of 10% to 90%.2.2 Hard Disk InstallationBefore you start:Disconnect the power from the NVR before installing a hard disk drive (HDD). A factory recommended HDD should be used for this installation.Tools Required: Screwdriver.Step 1Remove the cover from the device by unfastening the screws on the bottom.Figure 2-1Remove the CoverStep 2Place the HDD on the bottom of the device and then fasten the screws on the bottom to fix the HDD.Figure 2-2Fix the HDDStep 3Connect one end of the data cable to the motherboard of NVR and the other end to the HDD.Step 4Connect the power cable to the HDD.Figure 2-3Connect CablesStep 5Re-install the cover of the NVR and fasten screws.2.3 HDD Storage Calculation ChartThe following chart shows an estimation of storage space used based on recording at one channel for an hour at a fixed bit rate.Bit Rate Storage Used96K42M128K56M160K70M192K84M224K98M256K112M320K140M384K168M448K196M512K225M640K281M768K337M896K393M1024K450M1280K562M1536K675M1792K787M2048K900M4096K 1.8G8192K 3.6G16384K 7.2GPlease note that supplied values for storage space used is just for reference. The storage values in the chart are estimated by formulas and may have some deviation from actual value.Chapter 3 Menu Operation3.1 Startup and ShutdownProper startup and shutdown procedures are crucial to expanding the life of the NVR.To start your NVR:Step 1Check the power supply is plugged into an electrical outlet. It is HIGHLY recommended that an Uninterruptible Power Supply (UPS) be used in conjunction with the device. The Powerbutton) on the front panel should be red, indicating the device is receiving the power.Step 2Press the power switch on the panel. The Power LED should turn blue. The unit will begin to start.After the device starts up, the wizard will guide you through the initial settings, including modifying password, date and time settings, network settings, HDD initializing, and recording.To shut down the NVR:Step 1Go to Menu > Shutdown.Figure 3-1ShutdownStep 2Select Shutdown.Step 3Click Yes.3.2 Activate Your DevicePurpose:For the first-time access, you need to activate the device by setting an admin password. No operation is allowed before activation. You can also activate the device via Web Browser, SADP or client software.Step 1Input the same password in Create New Password and Confirm New Password.Step 2(Optional) Use customized password to activate and add network camera(s) connected to the device.1)Uncheck Use Channel Default Password.2)Enter a password in IP Camera Activation.Figure 3-2Set Admin PasswordSTRONG PASSWORD RECOMMENDED–We highly recommend you create a strong password of your own choosing (Using a minimum of 8 characters, including at least three of the following categories: upper case letters, lower case letters, numbers, and special characters.) in order to increase the security of your product. And we recommend you reset your password regularly, especially in the high security system, resetting the password monthly or weekly can better protect your product.Step 3Click OK.3.3 Set the Unlock Pattern for LoginAdmin can use the unlock pattern for device login.For devices with PoE function, you can draw the device unlock pattern after activation. For other devices, the unlock pattern interface will show after the first-time login.Step 1Use the mouse to draw a pattern among the 9 dots on the screen. Release the mouse when the pattern is done.Figure 3-3Draw the Pattern●Connect at least 4 dots to draw the pattern.●Each dot can be connected for once only.Step 2Draw the same pattern again to confirm it. When the two patterns match, the pattern is configured successfully.3.4 User LoginPurpose:If NVR has logged out, you must login the device before operating the menu and other functions. Step 1Select the User Name in the dropdown list.Figure 3-4LoginStep 2Input Password.Step 3Click OK.In the Login dialog box, if you enter the wrong password 7 times, the current user account will be locked for 60 seconds.3.5 Network SettingsPurpose:Network settings must be properly configured before you operate NVR over network.Step 1Enter the general network settings interface.Menu > Configuration > Network > GeneralFigure 3-5Network SettingsStep 2Configure the following settings: NIC Type, IPv4 Address, IPv4 Gateway, MTU and DNS Server.Step 3If the DHCP server is available, you can check the checkbox of DHCP to automatically obtain an IP address and other network settings from that server.Step 4Click Apply.3.6 Add IP CamerasPurpose:Before you can get live video or record the video files, you should add the network cameras to the connection list of the device.Before you start:Ensure the network connection is valid and correct, and the IP camera to add has already been activated. Please refer to the User Manual for activating the inactive IP camera.You can select one of the following three options to add the IP camera.OPTION 1:Step 1Click to select an idle window in the live view mode.Step 2Click in the center of the window to pop up the Add IP Camera interface.Figure 3-6Add IP CameraStep 3Select the detected IP camera and click Add to add it directly, and you can click Search to refresh the online IP camera manually.Or you can choose to custom add the IP camera by editing the parameters in thecorresponding text field and then click Add to add it.3.7 Live ViewIcons are provided on screen in Live View mode to indicate camera status. These icons include: Live View IconsIn the live view mode, there are icons at the upper-right corner of the screen for each channel, showing the status of the record and alarm in the channel for quick reference.Alarm (video loss, tampering, motion detection, VCA or sensor alarm)Record (manual record, continuous record, motion detection, VCA or alarm triggered record)Alarm and RecordEvent/Exception (event and exception information, appears at the lower-left corner of the screen.)3.8 Recording SettingsBefore you start:Make sure that the disk has already been installed. If not, please install a disk and initialize it. You may refer to the user manual for detailed information.Purpose:Two kinds of record types are introduced in the following section, including Instant Record andAll-day Record. And for other record types, you may refer to the user manual for detailed information.After rebooting all the manual records enabled are canceled.Step 1On the live view window, right lick the window and move the cursor to the Start Recording option, and select Continuous Record or Motion Detection Record on your demand.Figure 3-7Start Recording from Right-click MenuStep 2Click Yes in the pop-up Attention message box to confirm the settings. All the channels will start to record in the selected mode.3.9 PlaybackThe recorded video files on the hard disk can be played back in the following modes: instant playback, all-day playback for the specified channel, and playback bynormal/event/smart/tag/sub-periods/external file search.Step 1Enter playback interface.Click Menu > Playback or from the right-click menuStep 2Check the checkbox of channel(s) in the channel list and then double-click to select a date on the calendar.Step 3You can use the toolbar in the bottom part of Playback interface to control playing progress.Figure 3-8 Playback InterfaceStep 4 Select the channel(s) to or execute simultaneous playback of multiple channels.Chapter 4 Accessing by Web BrowserYou shall acknowledge that the use of the product with Internet access might be under network security risks. For avoidance of any network attacks and information leakage, please strengthen your own protection. If the product does not work properly, please contact with your dealer or the nearest service center.Purpose:You can get access to the device via web browser. You may use one of the following listed web browsers: Internet Explorer 6.0, Internet Explorer 7.0, Internet Explorer 8.0, Internet Explorer 9.0, Internet Explorer 10.0, Internet Explorer 11.0, Apple Safari, Mozilla Firefox, and Google Chrome. The supported resolutions include 1024*768 and above.Step 1Open web browser, input the IP address of the device and then press Enter.Step 2Login to the device.If the device has not been activated, you need to activate the device first before login.Figure 4-1Set Admin Password1)Set the password for the admin user account.2)Click OK.STRONG PASSWORD RECOMMENDED–We highly recommend you create a strong password of your own choosing (using a minimum of 8 characters, including upper case letters, lower case letters, numbers, and special characters) in order to increase the security of your product. And we recommend you reset your password regularly, especially in the high security system, resetting the password monthly or weekly can better protect your product.If the device is already activated, enter the user name and password in the login interface, and click Login.Figure 4-2LoginStep 3Install the plug-in before viewing the live video and managing the camera. Please follow the installation prompts to install the plug-in.You may have to close the web browser to finish the installation of the plug-in.After login, you can perform the operation and configuration of the device, including the live view, playback, log search, configuration, etc.03041041090702。
【免费下载】10机器学习 课程教案

Machine Learning Course PlanLecture OneTitle: IntroductionContent:●Basic information about this course: books, TA, office, homework, projectand test form…●Introduce the definition of learning systems.●Give an overview of applications to show the goals of machine learning.●Introduce the aspects of developing a learning system: training data, conceptrepresentation, function approximation.Targets:●Understand the background of machine learning;●Remember the basic function of machine learning;●Get the general ideas of machine learning’s problem and point; Processions:●What is Machine Learning?●Applications of ML●Disciplines relevant to ML●Well-Posed Learning Problems●Designing a Learning System●Perspectives and Issues In Machine Learning●How To Read This BookDifficulties:●How to design a learning system;●The understand of concept.Lecture TwoTitle: Concept Learning and the General-to-Specific OrderingContents:●What is the concept learning task? Where is it applied to?●Make students understand concept learning is equivalent to search through ahypothesis space.●Illustrate step by step the procedure of general-to-specific ordering ofhypotheses, to find the maximally specific hypothesesTargets:●Understand the background of concept learning;●Remember the basic concept of concept learning, version space, etc; Processions:●Introduction● A Concept Learning Task●Concept Learning as Search●Find-S:Finding a Maximally Specific HypothesisDifficulties:●What’s the process of concept learning;●Remember the concept of concept learning;Lecture ThreeTitle: Candidate elimination and Inductive biasContents:●Introduce the definition of version spaces and the candidate eliminationalgorithm.●How to learning conjunctive concepts?●Introduce and emphasize the importance of inductive bias.Targets:●Remember the process of candidate elimination algorithm;●Get the basic idea of the useless of unbiased learning;Processions:●Version Spaces and the Candidate-Elimination Algorithm●Remarks On VS and C-E●Inductive BiasDifficulties:●The understand of version space;●The idea of bias;●The under stand of Find-S Algorithm and Candidate-Elimination Algorithm; Assignments:●EX. 2.1●EX. 2.4Lectrue FourTitle: Decision Tree Learning(1)Contents:●Development of Decision tree learning, the role it plays in the history ofincrecemental learning●Show the students how to representing concepts as decision trees.●Introduce recursive induction of decision trees.Targets:●Understand the background of Decision Tree;●Remember the basic concept of decision tree, over fitting, etc; Processions:●Introduction●Decision Tree Representation●Appropriate Problems for Decision Tree LearningDifficulties:●One of the most widely used and practical methods for inductive inference● A method for approximating discrete-valued functions●Robust to noisy data●Capable of learning disjunctive expressionsLectrue FiveTitle: Decision Tree Learning(2)Contents:●Introduce recursive induction of decision trees.●Picking the best splitting attribute: entropy and information gain. Emphasizethis part, let students do exercise to practice the procedureTargets:●Remember the process of the learning algorithm of decision tree; Processions:●The Basic Decision Tree Learning Algorithm●Hypothesis Space Search ID3Difficulties:●ID3, Assistant, C4.5Lectrue SixTitle: Decision Tree Learning(3)Contents:●What is Overfitting? When will is happen? What damage will it cause toclassifiers? What should be done in case of noisy data? Why and how to prune?●How to apply the decision tree to continuous attributes and missing values. Targets:●Get the basic idea of solving the problems;Processions:●Inductive Bias in Decision Tree Learning●Issue In Decision Tree LearningDifficulties:●Inductive bias is a preference for small trees over large trees●Can also be re-presented as sets of if-then rulesLectrue SevenTitle: Artificial Neural Networks(1)Contents:●What is Neurons? What is the biological motivation of Artificial NeuralNetworks?●What are linear threshold units and their functions?●Introduce the principle of perceptrons: representational limitation andgradient descent training.Targets:●Understand the background of Neutral network;●Remember the basic concept of neutral network, over fitting, etc; Processions:●Introduction●Neural Network Representations●Appropriate Problems For Neural Network Learning●PerceptronLectrue EightTitle: Artificial Neural Networks(2)Contents:●Introduce the component of an Artificial Neural Networks:●Multilayer networks and backpropagation. Hidden layers and constructingintermediate, distributed representations.Targets:●Remember the process of the learning algorithm of neutral network; Processions:●Multilayer Networks And The Back propagation Algorithm●Notation SpecificationLectrue NineTitle: Artificial Neural Networks(3)Contents:●The problem of Overfitting●How to learn network structure, recurrent networks.●Face Recognition exampleTargets:●Get the basic idea of solving over fitting;●Learn how to slove real problem with ANN;Processions:●An Illustrative Example: Face Recognition●Alternative Error FunctionsProjects:●Face Recognition●CheckerLecture TenTitle: Evaluation HypothesisContents:●Motivation for Evaluation Hypothesis●Estimating Hypothesis Accuracy●Basics of Sampling Theory● A General Approach for Deriving Confidence Intervals●Difference in Error of Two Hypotheses●Comparing Learning AlgorithmTargets:●Given the observed accuracy of a hypothesis over a limited sample of data,estimate accuracy over additional examples;●Know how to Compare performance of different algorithms;●Understand the best way to use those limited data to learn a hypothesis andestimate its accuracy;Processions:●Motivation●Estimating hypothesis accuracy●Sample Error and True Error●Confidence intervals for Discrete-valued hypotheses●Basics og sampling theory● A general approach for deriving donfidence intervals●Difference in error of two hypotheses●Comparing learning algorithmsrLecture ElevenTitle: Bayesian Learning(1)Contents:●Give a brief introduction to the following definitions and theories:✧Probability theory, Bayes rule, and MAP concept learning.✧Naive Bayes learning algorithm.Targets:●Understand the background of Bayes;●Remember the basic concept of Naive Bayes learning algorithm, etc; Processions:●Introduction●Bayes Theorem●Bayes Theorem and Concept Learning●Maximum likelihood and least-squared error hypotheses●Maximum Likelihood Hypotheses For Predicting ProbabilityLecture TwelveTitle: Bayesian Learning(2)Contents:●Give a brief introduction to the following definitions and theories:✧Bayes nets and representing dependencies.✧Bayes optimal classifers.✧Minimum description length principal.Targets:●Remember the process of the Bayes optimal classifers;●Get the basic idea of Bayes nets;Processions:●Minimum Description Length Principle●Bayes Optimal Classifier●Gibbs Algorithm●Naïve Bayes Classifier●An Example: Learning To Classify Text●Experimental Results●Bayesian Belief Network●EM algorithmLecture ThirteenTitle: Genetic AlgorithmsContents:●Overview the theory of Genetic algorithm.●Genetic algorithm provide an approach learning that is based loosely onsimulated evolution.●How Hypotheses are described by bit strings whose interpretation depends onthe application?●The search begins with a population of initial hypotheses. The next generationof population is generated by means of operations such as random mutationand crossover●Introduce the fitness function.●ApplicationTargets:●Know the importance of GA.●Understand the process of GA, and get the idea of how to interpret thehypothesis●Understand the selection, crossover and mutation.Processions:●Introduction the motivation of GA● A prototypical genetic algorithm●Representing of Hypotheses●Genetic operators: selection, crossover and mutation●The fitness function and selection。
Procedings of the IASTED International Conference APPLIED SIMULATION AND MODELLING

Procedings of the IASTED International ConferenceAPPLIED SIMULATION AND MODELLINGSeptember3-5,2003,Marbella,SpainA time-frequency approach to blind separation of under-determinedmixture of sourcesA.MANSOURLab.E I,ENSIETA,29806Brest cedex09,(FRANCE).mansour@M.KAW AMOTODept.of Electronic andControl Systems Eng.,Shimane University,Shimane690-8504,(JAPAN)kawa@ecs.shimane-u.ac.jpC.PuntonetDepartamento de Arquitectura yTecnologia de computadores,Universidad de Granada,18071Granada,(SPAIN).carlos@atc.ugr.esABSTRACTThis paper deals with the problem of blind separation of under-determined or over-complete mixtures(i.e.more sources than sensors).Atfirst a global scheme to sepa-rate under-determined mixtures is presented.Then a new approach based on time-frequency representations(TFR) is discussed.Finally,some experiments are conducted and some experimental results are given.KEY WORDSICA,BSS,Time-Frequency domain,over-complete or under-determined mixtures1.IntroductionBlind separation of sources problem is a recent and an im-portant signal processing problem.This problem involves recovering unknown sources by only observing some mixed signals of them[1].Generally,researchers assume that the sources are statistically independent from each other and at most one of them can be a Gaussian signal[2]. Other assumptions can be also founded in the literature concerning the nature of the transmission channel(i.e.an instantaneous or a memoryless channel,a convolutive or a memory channel,and a non-linear channel).In addition,a widely used assumption considers that the number of sen-sors should be equal or greater(for subspace approaches) than the number of sources.These assumptions are fairly satisfied in many divers applications such as robotics, telecommunication,biomedical engineering,radars,etc., see[3].In recent applications linked to special scenarios in telecommunication(as satellite communication in double-talk mode),robotics(for exemple,robots which imitate human behavior)or radar(in ELectronic INTelli-gence”ELINT”applications),the assumption about the number of sensors can not be satisfied.In fact,in the latter applications the number of sensors is less than the number of sources and often we should deal with a mono-sensor system with two or more sources.Recently,few authors have considered the under-determined mixtures.Thus by using overcomplete repre-sentations,Lewicki and Sejnowski in[4]present an algo-rithms to learn overcomplete basis.Their algorithm uses a Gaussian approximation of probability density function (PDF)to maximize the probability of the data given the model.Their approach can be considered as a generaliza-tion of the Independent Component Analysis(ICA)[2]in the case of instantaneous mixtures.However,in this ap-proach,the sources should be sparse enough to get good ex-perimental results,otherwise the sources are being mapped down to a smaller subspace and there is necessary a loss of ing the previous approach,Lee et al.[5] separate successfully three speech signals using two micro-phones.On the other hand,When the sources are sparsely distributed,at any time t,at most one of sources could be significantly different from zero.In this case,estimating the mixing matrix[6,7,8]consists offinding the direc-tions of maximum data density by simple clustering ing Reimannian metrics and Lie group structures on the manifolds of over-complet mixture matrices,Zhang et al.[9]present a theoretical approach and develop an al-gorithm which can be considered as a generalization of the one presented in[10].The algorithm of Zhang et al.up-date the weight matrix by minimizing a kullback-Leibler divergence by using natural learning algorithm[11].In the general case,one can consider that separation of over-complete mixtures still a real challenge for the sci-entific community.However,some algorithms have been proposed to deal with particular applications.Thus for bi-nary signals used in digital communication,Diamantaras and Chassioti[12,13]propose an algorithm based on the PDF of the observed mixed signals.The pdf of the ob-servation signals have been modeled by Gaussian pdf and estimated from the histogram of the observed -ing differential correlation function,Deville and Savoldelli [14]propose an algorithm to separate two sources from noisy convolutive mixtures.The proposed approach re-quires the sources to be long-term non-stationary signals and the noise should be long-terme stationary ones.The previous statement means that the sources(resp.noise) should have different(resp.identically)second order statis-tics at different instances separated by a long period.2.Channel ModelHereinafter,we consider that the sources are non Gaus-sian signals and statistically independent from each other.In addition,we assume that the noise is an additive whiteGaussian noise (AWGN).Letdenote the source vector at any time t,is mixing vector and is a AWGN vector.The channel is represented by a full rank real and constant matrix ().H ( )Channel+B(t)S(t)Figure 1.General structure.The separation is considered achieved when the sources are estimated up to a scale factor and a permuta-tion.That means the global matrix can be written as:here,is a weight matrix,is a permutation matrix and is a non-zero diagonal matrix.For a sake of simplic-ity and without loss of generality,we will consider in the following that:Where is an invertible matrix and is a full rankrectangular matrix.3.A Separation SchemeIn the case of over-complete mixtures (),the invert-ibility of the mixing matrix becomes an ill-conditioned problem.That means the Independent Component Analy-sis (ICA)will be reduced to extract independent signals which are not necessarily the origine sources,i.e.the sep-aration can not give a unique solution.Therefore,further assumptions should be considered and in consequence suit-able algorithms could be developed.Thus,two strategies can be considered:At first one can identify the mixing matrix then us-ing this estimated matrix along with important infor-mation about the nature or the distributions of the sources,we should retrieve the original sources.In many applications (such as speech signals,telecom-munications,etc ),one can assume the sources havespecial features (constant modulus,frequency prop-erties,etc ).Using sources’specifics,the separation becomes possible in the classic manner,i.e.up to per-mutation and a scale factor.Beside the algorithms cited and discussed in the intro-duction of our manuscript,few more algorithms can be founded in the literature.The latter publications are dis-cussed in this section.3.1Identification &SeparationOne of the first publications on the identification of under-determined mixtures was proposed by Cardoso [15].In his manuscript,Cardoso proposed an algorithm based only on fourth-order cumulant.In fact,using the symmetries of quadricovariance tensor,an identification method based on the decomposition of the quadricovariance was proposed.Recently,Comon [16]proved using an algebraic approach,that the identification of static MIMO (Multiple Inputs Multiple Outputs)with fewer outputs than inputs is possible.In other words,he proved that the CANonical Decomposition (CAND)of a fourth-order cross-cumulant tensor can be considered to achieve the identification.In addition,he proved that ICA is a symmetric version of ing a Sylveter’s theorem in multilinear algebra and the fourth order cross cumulant tensor,he proposed an algorithm to identify the mixing matrix in the general case.To recover d-psk sources,comon proposes alsoa non-linear inversion ofby adding some non-linear equations and using the fact that the d-psk signals satisfyspecial polynomial properties (i.e.).Later on,Comon and Grellier [17]proposed an extension of the previous algorithm to deal with different communication signals (MSK,QPSK and QAM4).Similar approach was also proposed by De Lathauwer et al.,see [18].Finally,Taleb [19]proposes a blind identification al-gorithm of M-inputs and 2-outputs channel.He proved thatthe coefficients of the mixing matrixare the roots of a polynomial equations based on the derivative of the sec-ond characteristic function of the observed signals.The uniqueness of the solution is proved using Darmois’Theo-rem [20].3.2Direct SeparationHere,we discuss methods to separate special signals.As it is mentioned in the previous subsection that Comon et al.[16,17]proposed an algorithm to separate communication signals.Nakadai et al.[21,22]addressed the problem of a blind separation of three mixed speech signals with the help of two microphones by integrating auditory and vi-sual processing in real world robot audition systems.Theirapproach is based on direction pass-filters which are imple-mented using the interaural phase difference and the inter-aural intensity difference in each sub-band -ing Dempster-Shafer theory,they determine the direction of each sub-band frequency.Finally,the waveform of one sound can be obtained by a simple inverse FFT applied to the addition of the sub-band frequencies issued by the spe-cific direction of that speaker.Their global system can per-form sound source localization,separation and recognition by using audio-visual integration with active movements.4.Time-Frequency ApproachThe algorithm proposed in this section is based on time-frequency distributions of the observed signals.To our knowledge,few time-frequency methods have been devoted to the blind separation of MIMO channel.In fact,for MIMO channel with more sensors than sources, Belouchrani and Moeness[23]proposed a time-frequency separation method exploiting the difference in the time-frequency signatures of the sources which are assumed to be nonstationary multi-variate process.Their idea consists on achieving a joint diagonalization of a combined set of spatial time-frequency distributions which have been defined in their paper.It is clear from the discussion of the previous sections that the identification of MIMO channel is possible.How-ever,the separation is not evident in the general case.The few published algorithms for the under-determined matter are very linked to signal features of theirs applications.In our applications,an instantaneous static under-determined mixture of speech signals is considered.This problem can be divided into two steps:Atfirst an identification algorithm should be applied.For the moment,we didn’t develop a specific identi-fication algorithm.Therefore,any identification algo-rithms previously mentioned can be used.Let us assume that the coefficient of the mixing matrixhave been estimated.The question becomes How can we recover the sources from fewer sensors?To answer this question,we consider in this section the separation of a few speech signals(for the instance, we are considering just two or at most three sources) using the output of a single microphone(i.e.Multiple Inputs Single output,MISO channel).Recently,time-frequency representations(TFR) have been developed by many researchers[24]and they are considered as very powerful signal processing tools.In the literature,many different TFR have been developed as Wigner-Ville,Pseudo-Wigner-Ville,Smooth Pseudo-Wigner-Ville,Cho-Willims,Born-Jordan,etc.In a previous study[25],we found that for simplicity and performance reasons,the Pseudo-Wigner-Ville can be considered as a good TFR candidate.Here we present a new algorithm based on time-frequency representations of the observed signals(TFR)to separate a MISO channel with speech sources.It is known that speech signals are non-stationary signals.However within phonemes(about80ms of duration)the statistics of the signal are relatively constant[26].On the other hand,It is well known that voiced speech are quasi-periodic signals and the non-voiced signals can be considered as white filtered noise[27].Within a small window corresponding to51ms,the pitch can be slightly change.Therefore,one can use this property to pick up the frequency segments of a speaker.The pitch can be estimated using divers techniques[28].Using the previous facts and Pseudo-Wigner-Ville representations,one can separate up to three speech signals from one observed mixed signal of them.To achieve that goal,we assume that the time-frequency signatures of the sources are disjoints.Atfirst,one should calculate the TFR of the observed signal.Then,in the time-frequency space, we plot a regular grilled.The dimensions of the a small cell of the grilled are evaluated based on the properties of the speech signals and the sampling frequency.Therefore, these dimensions can be considered as10to20ms in length (i.e.time axis)and5to10%of the sampling frequency value in the vertical axis.Once we plot the grilled,we estimate the energy average in each cell and a threshold is applied to distinguish noisy cells from other.Then the cell with the maximum energy is considered as a potential pitch of one speaker and it is pointed out.After that,we merge in a set of cells,all cells with high level of energy in the neighborhood of the previous cell.At least one har-monic of the pitch should be also selected.The previous steps should repeated as necessary.Finally,the obtained map can be considered as a bi-dimensional time-frequency filters which should be applied on the mixed -ing a simple correlation maximization algorithm,one can find the different pieces corresponding to the speech of one speaker.5.Experimental ResultsTo demonstrate the validity of the proposed algorithm men-tioned in section4,many computer simulations were con-ducted.Some results are shown in this section.We consid-ered the following two-input and one-output system.(1)The sources were male and female voices which were recorded by8[KHz]sampling fre-quency.The TFR was calculated by using128data of the observed signal.Figure2shows the results obtained by applying the proposed algorithm(last paragraph in section4)to the ob-served signal.From thisfigure,one might think that the estimated signals are different from the original signals. However,if one hear the estimated signals,one can see that the two original sources and are separated from the observed signal by the proposed algorithm.6.ConclusionThis paper deals with the problem of blind separation of under-determined(or over-complete)mixtures(i.e.more sources than sensors).Atfirst,a survey on blind separation algorithms for under-determined mixtures is given.A sep-aration scheme based on identification or direct separation is discussed.A new time-frequency algorithm to separate speech signals has been proposed.Finally,some experi-ments have been conducted and the some experimental re-sults are given.Actually,we are working on a project con-cern the separation of under-determined mixtures.Further results will be the subject of future communications.References[1]A.Mansour, A.Kardec Barros,and N.Ohnishi,“Blind separation of sources:Methods,assumptions and applications.,”IEICE Transactions on Funda-mentals of Electronics,Communications and Com-puter Sciences,vol.E83-A,no.8,pp.1498–1512, August2000.[2]on,“Independent component analysis,a newconcept?,”Signal Processing,vol.36,no.3,pp.287–314,April1994.[3]A.Mansour and M.Kawamoto,“Ica papers classi-fied according to their applications&performances.,”IEICE Transactions on Fundamentals of Electronics, Communications and Computer Sciences,vol.E86-A,no.3,pp.620–633,March2003.[4]M.Lewicki and T.J.Sejnowski,“Learning non-linear overcomplete representations for efficient cod-ing,”Advances in neural Information Processing Sys-tems,vol.10,pp.815–821,1998.[5]T.W.Lee,M.S.Lewicki,M.Girolami,and T.J.Se-jnowski,“Blind source separation of more sources than mixtures using overcomplete representations,”IEEE Signal Processing Letters,vol.6,no.4,pp.87–90,April1999.[6]P.Bofill and M.Zibulevsky,“Blind separation ofmore sources than mixtures using sparsity of their short-time fourier transform,”in International Work-shop on Independent Component Analysis and blind Signal Separation,Helsinki,Finland,19-22June 2000,pp.87–92.[7]P.Bofill and M.Zibulevsky,“Underdetermined blindsource separation using sparse representations,”Sig-nal Processing,vol.81,pp.2353–2363,2001. [8]P.Bofill,“Undetermined blind separation of delayedsound sources in the frequency domain,”NeuroCom-puting,p.To appear,2002.[9]L.Q.Zhang,S.I.Amari,and A.Cichocki,“Nat-ural gradient approach to blind separation of over-and under-complete mixtures,”in First International Workshop on Independent Component Analysis and signal Separation(ICA99),J.F.Cardoso,Ch.Jutten, and Ph.loubaton,Eds.,Aussois,France,11-15Jan-uary1999,pp.455–460.[10]M.Lewicki and T.J.Sejnowski,“Learning overcom-plete representations,”Neural Computation,vol.12, no.2,pp.337–365,2000.[11]S.I.Amari,A.Cichocki,and H.H.Yang,“A newlearning algorithm for blind signal separation,”in Neural Information Processing System8,Eds.D.S.Toureyzky et.al.,1995,pp.757–763.[12]K.Diamantaras and E.Chassioti,“Blind separationof n binary sources from one observation:A deter-ministic approach,”in International Workshop on In-dependent Component Analysis and blind Signal Sep-aration,Helsinki,Finland,19-22June2000,pp.93–98.[13]K.Diamantaras,“Blind separation of multiple binarysources using a single linear mixture,”in Proceed-ings of International Conference on Acoustics Speech and Signal Processing2001,ICASSP2000,Istanbul, Turkey,Jun2000,pp.2889–2892.[14]Y.Deville and S.Savoldelli,“A second order dif-ferential approach for underdetermined convolutive source separation,”in Proceedings of International Conference on Acoustics Speech and Signal Process-ing2001,ICASSP2001,Salt Lake City,Utah,USA, May7-112001.[15]J.F.Cardoso,“Super-symetric decomposition of thefourth-order cumulant tensor.blind identification of more sources than sensors.,”in Proceedings of Inter-national Conference on Speech and Signal Process-ing1991,ICASSP’91,Toronto-Canada,May1991, pp.3109–3112.[16]on,“Blind channel identification and extrac-tion of more sources than sensors,”in In SPIE Confer-ence on Advanced Algorithms and Architectures for Signal Processing,San Diego(CA),USA,July19-24 1998,pp.2–13,Keynote address.[17]on and O.Grellier,“Non-linear inversion ofunderdetermined mixtures,”in First International Workshop on Independent Component Analysis andFigure2.Simulations Results:(a)Source signal(b)Source signal(c)Observed signal(d)Estimated signal of(e)Estimated signal ofsignal Separation(ICA99),J.F.Cardoso,Ch.Jut-ten,and Ph.loubaton,Eds.,Aussois,FRANCE,11-15 January1999,pp.461–465.[18]L.De Lathauwer,on,B.De Moor,and J.Van-dewalle,“ICA algorithms for3sources and2sen-sors,”in IEEE SP Int Workshop on High Order Statis-tics,HOS99,Caeserea,Israel,12-14June1999,pp.116–120.[19]A.Taleb,“An algorithm for the blind identification ofn independent signals with2sensors,”in Sixth Inter-national Symposium on Signal Processing and its Ap-plications(ISSPA2001),M.Deriche,Boashash,and W.W.Boles,Eds.,Kuala-Lampur,Malaysia,August 13-162001.[20]G.Darmois,“Analyse g´e n´e rale des liaisons stochas-tiques,”Rev.Inst.Intern.Stat.,vol.21,pp.2–8,1953.[21]K.I.Nakadai,K.Hidai,H.G.Okuno,and H.ki-tano,“Real-time speaker localization and speech sep-aration by audio-visual integration,”in17th inter-national Joint Conference on Artificial Intelligence (IJCAI-01),Seatle,USA,August2001,pp.1425–1432.[22]H.G.Okuno,K.Nakadai,T.Lourens,and H.kitano,“Separating three simultaneous speeches with two microphones by integrating auditory and visual pro-cessing,”in European Conference on Speech Process-ing,Aalborg,Denmark,September2001,pp.2643–2646.[23]A.Belouchrani and M.G.Amin,“Blind source sep-aration based on time-frequency signal representa-tions,”IEEE Trans.on Signal Processing,vol.46, no.11,pp.2888–2897,1998.[24]P.Flandrin,Time-Frequency/Time-Scale analysis,Academic Press,Paris,1999.[25]D.Le Guen and A.Mansour,“Automatic recogni-tion algorithm for digitally modulated signals,”in6th Baiona workshop on signal processing in communi-cations,Baiona,Spain,25-28June2003,p.To ap-pear.[26]J.Thiemann,Acoustic noise suppression for speechsignals using auditory masking effects,Ph.D.thesis, Department of Electrical&Computer Engineering, McGill University,Canada,July2001.[27]R.Le Bouquin,Traitemnet pour la reduction du bruitsur la parole application aux communications radio-mobiles.,Ph.D.thesis,L’universit´e de Rennes I,July 1991.[28]A.Jefremov and B.Kleijn,“Sline-based continuous-time pitch estimation,”in Proceedings of Interna-tional Conference on Acoustics Speech and Signal Processing2002,ICASSP2002,Orlando,Florida, U.S.A,13-17May2002.。
稀疏表示
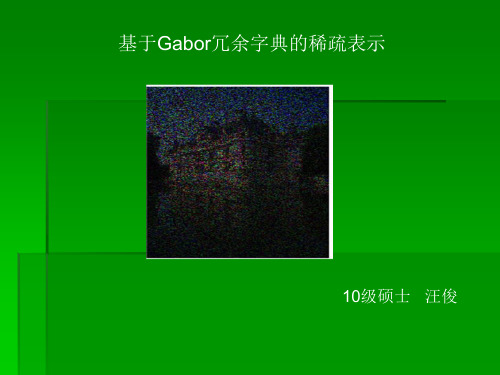
( D) 2 || ||0
上面的符号表示:最小的线性相关的列向量所含的向量个 数。那么对于0范数优化问题就会有一个唯一的解。可即便是 证明了唯一性,求解这个问题仍然是NP-Hard。
时间继续来到2006年,华裔的数学家Terrence Tao出现, Tao和Donoho的弟子Candes合作证明了在RIP条件下,0范 数优化问题与以下1范数优化问题具有相同的解:
谢谢!
α=(0,0,0.75)
α=(0,0.24,0.75)
α=(0,0.24,0.75)
α=(0,0.24,0.65)
对于上面求内积找最匹配原子的一步,当时鉴于原 子个数太多,就想了可否在这里做个优化,就用了PSO (粒子群优化算法)查找最优原子,这个比遗传算法要 简单,我觉得这个算法也还挺有意思的。 基于学习的方法:
输入的刺激即照片不一样,则响应神经元也不一样
模拟人类视觉系统的感知机制来形成对于图像的稀疏表 示,将字典中的每个原子看作一个神经元,整个字典则对应 人类视觉皮层中神经元整体,并且字典中原子具有类似视觉 皮层中神bor函数作为简单细胞的感受野 函数,刻画其响应特性。
2 2 2 x k y x g K ( ) exp( ) cos(2 ) 2 2
( x x0 ) cos ( y y0 ) sin x
( x x0 ) sin ( y y0 ) cos y
Gabor函数
稀疏表示的应用 图像恢复,又左侧图像恢复出右侧结果
图像修补,左侧图像修补得到右侧结果
图像去模糊左上为输入模糊图像,右下为输出清晰图像, 中间均为迭代过程
物体检测
自行车,左侧输入图像,中间为位置概率图,右侧为检测结果
distributed representations 嵌入方法

distributed representations 嵌入方法Distributed Representations,也称为分布式表示或分布式嵌入,是一种在自然语言处理和机器学习领域广泛使用的技术。
其核心思想是将每个单词或实体表示为一个高维向量,这些向量在向量空间中捕捉单词或实体的语义和上下文信息。
这种方法与传统的独热编码(One-Hot Encoding)相比,具有更高的表示能力和灵活性。
Distributed Representations的主要优势在于它能够捕捉单词之间的语义相似性。
由于向量空间中的单词表示是通过上下文信息学习得到的,因此语义上相似的单词在向量空间中的位置也会相近。
这种特性使得Distributed Representations在多种NLP任务中表现出色,如词义消歧、信息检索、文本分类等。
常见的Distributed Representations嵌入方法包括Word2Vec、GloVe和FastText等。
其中,Word2Vec是一种基于神经网络的方法,通过训练大量的文本语料库来学习单词的向量表示。
GloVe则是一种基于全局词频统计的方法,它通过构建一个共现矩阵来捕捉单词之间的关联信息。
FastText则是一种结合了Word2Vec和n-gram思想的嵌入方法,它可以更好地处理词序和语义信息。
在实际应用中,Distributed Representations嵌入方法已经被广泛应用于各种NLP任务中。
例如,在文本分类任务中,我们可以使用预训练的词向量作为输入特征,提高分类器的性能。
在信息检索任务中,我们可以通过计算查询词和文档词向量之间的余弦相似度来评估文档的相关性。
此外,Distributed Representations还可以用于生成词向量空间模型,用于可视化和分析文本数据。
总之,Distributed Representations是一种强大的自然语言处理技术,它通过将单词表示为高维向量来捕捉单词之间的语义和上下文信息。
深度学习研究综述
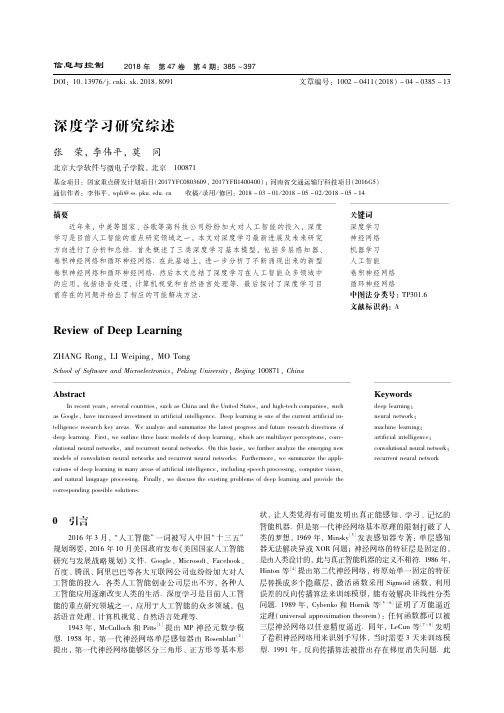
的第 i个神经元被激活函数作用之前的值,Wlji是第 l层的
第 j个神经元与第 l+1层的第 i个神经元之间的权重,bli
是偏置,f(·)是非线性激活函数,常见的有径向基函数、
ReLU、PReLU、Tanh、Sigmoid等.
如果采用均方误差(meansquarederror),则损失函数为
∑ J=
Keywords
deeplearning; neuralnetwork; machinelearning; artificialintelligence; convolutionalneuralnetwork; recurrentneuralnetwork
0 引言
2016年 3月,“人工智能”一词被写入中国“十三五” 规划纲要,2016年 10月美国政府发布《美国国家人工智能 研究与发展 战 略 规 划 》文 件.Google、大对人 工智能的投入.各类人工智能创业公司层出不穷,各种人 工智能应用逐渐改变人类的生活.深度学习是目前人工智 能的重点研究领域之一,应用于人工智能的众多领域,包 括语音处理、计算机视觉、自然语言处理等.
适合处理空间数据,在计算机视觉领域应用广泛.一维卷
积神经网络也被称 为 时 间 延 迟 神 经 网 络 (timedelayneural network),可以用来处理一维数据.CNN的设计思想受到 了视觉神经 科 学 的 启 发,主 要 由 卷 积 层 (convolutionallay er)和池化层(poolinglayer)组成.卷积层能够保持图像的 空间连续性,能将图像的局部特征提取出来.池化层可以 采用最大 池 化 (maxpooling)或 平 均 池 化 (meanpooling), 池化层能降低中间隐藏层的维度,减少接下来各层的运算 量,并提供了旋转不变性.卷积与池化操作示意图如图 3 所示,图中采用 3×3的卷积核和 2×2的 pooling.
分布式表达和语言模型

分布式表达和语言模型
分布式表达和语言模型是自然语言处理领域中的两个重要概念。
分布式表达(Distributed Representation)是一种将高维稠密向量表示为低维稀疏向量的方法,即将一个词或概念表示为一个向量。
这种方法的好处是能够将语义相似或相关的词或概念表示为相近的向量,从而方便进行相似度计算和聚类等操作。
例如,可以将“猫”、“狗”、“鸟”等动物相关的词汇表示为相近的向量。
语言模型是一种概率模型,用于描述语言中词或句子的出现概率。
语言模型通常采用上下文无关语法或上下文有关语法来定义语言中的词或句子,并使用概率分布来表示它们出现的可能性。
语言模型广泛应用于自然语言处理任务,如文本分类、机器翻译、语音识别等。
分布式表达和语言模型之间的关系在于,分布式表达可以用于构建语言模型。
具体来说,可以使用分布式表达来表示词或句子的概率分布,从而构建一个概率模型。
这种概率模型可以用于自然语言处理任务,例如文本分类、机器翻译等。
因此,分布式表达和语言模型在自然语言处理中起着非常重要的作用,并且两者之间的关系密切。
视频运营方案英文缩写
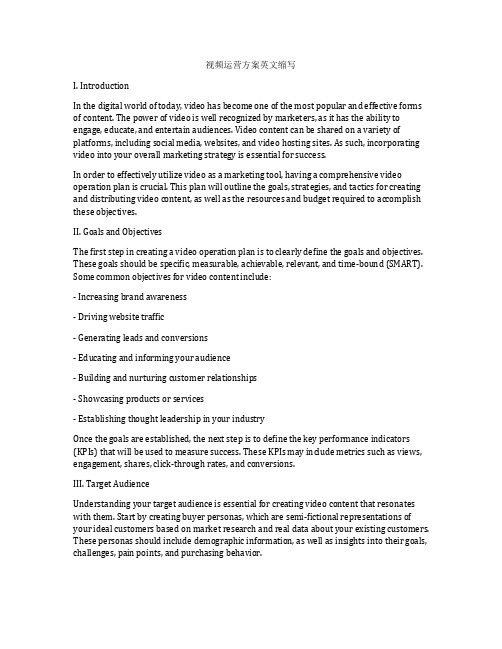
视频运营方案英文缩写I. IntroductionIn the digital world of today, video has become one of the most popular and effective forms of content. The power of video is well recognized by marketers, as it has the ability to engage, educate, and entertain audiences. Video content can be shared on a variety of platforms, including social media, websites, and video hosting sites. As such, incorporating video into your overall marketing strategy is essential for success.In order to effectively utilize video as a marketing tool, having a comprehensive video operation plan is crucial. This plan will outline the goals, strategies, and tactics for creating and distributing video content, as well as the resources and budget required to accomplish these objectives.II. Goals and ObjectivesThe first step in creating a video operation plan is to clearly define the goals and objectives. These goals should be specific, measurable, achievable, relevant, and time-bound (SMART). Some common objectives for video content include:- Increasing brand awareness- Driving website traffic- Generating leads and conversions- Educating and informing your audience- Building and nurturing customer relationships- Showcasing products or services- Establishing thought leadership in your industryOnce the goals are established, the next step is to define the key performance indicators (KPIs) that will be used to measure success. These KPIs may include metrics such as views, engagement, shares, click-through rates, and conversions.III. Target AudienceUnderstanding your target audience is essential for creating video content that resonates with them. Start by creating buyer personas, which are semi-fictional representations of your ideal customers based on market research and real data about your existing customers. These personas should include demographic information, as well as insights into their goals, challenges, pain points, and purchasing behavior.Additionally, it's important to consider the buyer's journey when planning video content. Different types of videos will resonate with audiences at different stages of the buyer's journey, such as awareness, consideration, and decision. Tailoring video content to meet the needs of audiences at each stage of the buyer's journey will help move them through the sales funnel.IV. Content StrategyWith the goals and target audience established, the next step is to create a content strategy that outlines the types of videos that will be produced, as well as the topics, formats, and distribution channels. Some popular types of videos that can be used as part of a video operation plan include:- Brand videos: These videos are designed to introduce your brand and tell your story. They can be used to build brand awareness and establish an emotional connection with your audience.- How-to and instructional videos: Educational videos that showcase how to use your product or service. These are great for providing value to your audience and establishing thought leadership.- Testimonials and case studies: Customer success stories that demonstrate the benefits of your products or services. These videos can help build trust and credibility with potential customers.- Product demonstrations: Videos that showcase your products in action, highlighting their features and benefits. These can be used to educate prospects and drive sales.- Behind-the-scenes videos: These videos offer a glimpse into your company's culture, processes, and people. They can help humanize your brand and build relationships with your audience.- Live videos: Real-time videos that allow you to connect with your audience in a personal and interactive way. These can be used to host Q&A sessions, product announcements, or behind-the-scenes tours.When creating a content strategy, it's important to consider the different stages of the buyer's journey and create videos that address the needs of audiences at each stage. For example, awareness stage videos may focus on general educational content, while decision stage videos may be more product-focused and promotional.V. Production and DistributionOnce the content strategy is in place, the next step is to produce the videos and distribute them across the appropriate channels. Depending on the resources and budget available, videos may be produced in-house or outsourced to a production company. It's important toconsider factors such as production costs, time constraints, and the quality of the content when making this decision.When it comes to distribution, there are a variety of channels that can be used to share video content, including:- Social media platforms (e.g. Facebook, Instagram, LinkedIn, Twitter)- Video hosting sites (e.g. YouTube, Vimeo)- Your company website and blog- Email marketing campaigns- Paid advertising (e.g. Google AdWords, Facebook ads)- Industry-specific forums and communitiesIt's important to tailor the distribution strategy to the preferences and behaviors of your target audience. For example, if your audience spends a lot of time on Instagram, it makes sense to prioritize that platform for video distribution.VI. Performance Tracking and OptimizationOnce videos are produced and distributed, it's important to closely monitor their performance and make adjustments as necessary. This may involve tracking metrics such as views, engagement, shares, and conversions, as well as gathering feedback from the audience.Based on this data, the content strategy can be optimized to improve performance. This may involve creating more of the types of videos that are resonating with the audience, testing different formats, or refining the distribution strategy. Performance tracking is an ongoing process that should be continuously iterated upon to achieve the best results.VII. ConclusionIn conclusion, a comprehensive video operation plan is essential for effectively utilizing video as a marketing tool. By clearly defining goals and objectives, understanding the target audience, creating a content strategy, producing and distributing videos, and tracking performance, organizations can create and share video content that engages and converts their target audience. With the right plan in place, video has the power to drive brand awareness, website traffic, leads, and conversions, ultimately leading to business growth and success.。
- 1、下载文档前请自行甄别文档内容的完整性,平台不提供额外的编辑、内容补充、找答案等附加服务。
- 2、"仅部分预览"的文档,不可在线预览部分如存在完整性等问题,可反馈申请退款(可完整预览的文档不适用该条件!)。
- 3、如文档侵犯您的权益,请联系客服反馈,我们会尽快为您处理(人工客服工作时间:9:00-18:30)。
In Proceedings of the 13th Annual Cognitive Science Society Conference, 1991, NJ:LEA, 173-178.Using Semi-Distributed Representations to OvercomeCatastrophic Forgetting in Connectionist NetworksRobert M. FrenchPsychology Department, University of LiègeLiège, Belgium, rfrench@ulg.ac.beAbstractIn connectionist networks, newly-learned information destroys previously-learnedinformation unless the network is continually retrained on the old information. Thisbehavior, known as catastrophic forgetting, is unacceptable both for practical purposesand as a model of mind. This paper advances the claim that catastrophic forgetting is adirect consequence of the overlap of the system’s distributed representations and can bereduced by reducing this overlap. A simple algorithm is presented that allows astandard feedforward backpropagation network to develop semi-distributedrepresentations, thereby significantly reducing the problem of catastrophic forgetting.1 IntroductionCatastrophic forgetting is the inability of a neural network to retain old information in the presence of new. New information destroys old unless the old information is continually relearned by the net. McCloskey & Cohen [1990] and Ratcliff [1989] have demonstrated that this is a serious problem with connectionist networks. A related problem is that connectionist networks are not sensitive to overtraining. A network trained 1000 times to associate a pattern A with a pattern A' will forget that fact just as quickly as would a network trained on that association for 100 cycles. Clearly, this behavior is unacceptable as a model of mind, as well as from a purely practical standpoint. Once a network has thoroughly learned a set of patterns, it should be able to learn a completely new set and still be able to recall the first set with relative ease. In this paper I will suggest that catastrophic forgetting arises because of the overlap of distributed representations and I will present an algorithm that will allow a standard feedforward backpropagation (FFBP) network to overcome to a significant extent the problems of catastrophic forgetting and insensitivity to overtraining.2 Catastrophic forgetting and the overlap of representationsI suggest the following relation between catastrophic forgetting and representations in a distributed system:Catastrophic forgetting is a direct consequence of the overlap ofdistributed representations and can be reduced by reducing thisoverlap.Very local representations will not exhibit catastrophic forgetting because there is little interaction among representations. Consider the extreme example of a look-up table where there is no overlap at all among representations. There is no catastrophic forgetting; new information can be added without interfering at all with old information. However, because of its completely local representations, a look-up table lacks the all-important ability to generalize.At the other extreme are fully distributed networks where there is considerable interaction among representations. This interaction is responsible for the networks' generalization ability. On the other hand, these networks are severely affected by catastrophic forgetting.The moral of the story is that you can’t have it both ways. A system that develops highly distributed representations will be able to generalize but will suffer from castastrophic forgetting; conversely, a system that develops very local representations will not suffer from catastrophic forgetting, but will lose some of its ability to generalize. The challenge is to develop systems capable of producing semi-distributed representations that are local enough to overcome catastrophic forgetting yet that are sufficiently distributed to nonetheless allow generalization.In what follows, I will examine two distributed systems that do not suffer from catastrophic forgetting. Both of these systems work because their representations are not fully distributed over the entire memory, but rather are semi-distributed and hence exhibit limited representation overlap, at least prior to memory saturation. Finally, I will present a simple method that allows standard layered feedforward backpropagation networks to develop semi-distributed representations in the hidden layer. Not only does this method appear to dramatically reduce catastrophic forgetting but it also allows the system’s representations to partially reflect the degree to which a particular pattern has been learned. Even after a particular pattern has been learned, overlearning continues to modify connection weights in such a way that unlearning of the pattern will be made more difficult.3 Two examples of semi-distributed representationsI will briefly examine two systems that produce semi-distributed representations. In both systems, assuming that they are not saturated, there is little overlap of the representations produced. For this reason, they exhibit little catastrophic forgetting.3.1 Sparse Distributed MemorySparse Distributed Memory (hereafter, SDM [Kanerva 1988]) is an auto-associative, content-addressable memory typically consisting of one million 1000-bit “memory locations”. The memory is called “sparse” because it uses only one million locations out of a possible 21000 (i.e., 106of approximately 10300 possible locations). At each of these locations there is a vector of 1000 integers, called “counters”. New data are represented in the system as follows: If we wish to write a particular 1000-bit string to this memory, we select all memory locations that are within a Hamming distance of 450 bits of the write address. This gives us approximately 1000 locations (i.e. 0.1% of all of the entire address space). Wherever there is a 1 in the bit-string to be written to memory, we increment the corresponding counter in each of the vectors at the 1000 memory locations; wherever there is a 0, we decrement the corresponding counter. This is clearly a semi-distributed representation of the input data: storage of the bit-string is distributed over 1000 different memory locations but these 1000 memory locations account for a mere 0.1% of the total available memory.This system can easily store new information without interfering with previously stored information as long as the representations do not overlap too much. As soon as the memory starts to become saturated (at somewhat less than 100,000 words written to memory), there is interference among representations, and learning new information begins to interfere with the old. In this case, not only is there forgetting of the old information but the new information cannot be stored either.3.2 ALCOVEALCOVE [Kruschke 1990] is a computer memory model based on Nosofsky’sexemplar memory model [Nosofsky 1984]. This model does not suffer from the phenomenon of catastrophic forgetting noted by Ratcliff and McCloskey & Cohen. As we will see, ALCOVE, like SDM, uses semi-distributed representations.ALCOVE is a three-layer feed-forward network in which the activation of a node in the hidden layer is inversely exponentially proportional to the distance between the hidden node position and the input stimulus position. The hidden layer can be regarded as a “covering” of the input layer. The inverse exponential activation function has the effect of producing a localized receptive field around each hidden node, causing it to respond only to a limited part of the input field. This kind of localization does not exist in standard FFBP networks. This system therefore represents its inputs in a semi-distributed manner, with only a few hidden nodes taking part in the representation of a given input.The architecture of ALCOVE is such that the representation of new inputs, especially of new inputs that are not close to already-learned patterns, will not overlap significantly with the old representations. This means that the set of weights that produced the old representations will remain largely unaffected by new input.As in SDM, the representations in ALCOVE are also somewhat distributed, conferring on the system its ability to generalize. When the width of the receptive fields at each node is increased, thereby making each representation more distributed and causing greater overlap among representations, the amount of interference among representations increases.4 Semi-distributed representations in FFBP networksIf catastrophic forgetting could be reduced, the order in which inputs are presented to the network would be less important. Training could be done either sequentially or concurrently. In other words, the artificial constraint of requiring training data to be presented to the network in an interleaved fashion could be relaxed. If, in addition, the representations also reflected the amount of training required to produce them, it might be possible to produce a system that would better model overlearning than standard FFBP networks. An initial attempt to reduce catastrophic forgetting with semi-distributed representations by differentially modifying the learning rates of the connections in the network was described in [French & Jones 1991]. While this technique gave promising results on very small networks, it failed to scale up to larger networks. The algorithm presented below, using a different technique, allows semi-distributed representations to evolve that significantly reduce catastrophic forgetting.5 Activation overlap and representational interference in FFBP networksCatastrophic forgetting is closely related to the much-studied phenomenon of crosstalk. The discussion of crosstalk has traditionally involved the capacity of a network to store information [Willshaw 1981]: above a certain capacity, distributed networks can no longer store new information without destroying old. In standard backpropagation models, there is a much more serious problem. As things currently stand, FFBP networks will not work at all without artificially interleaved training sets. Even when the network is nowhere near its theoretical storage capacity, learning a single new input can completely disrupt all of the previously learned information. Catastrophic forgetting is crosstalk with a vengeance.A feedforward backpropagation network represents its inputs as activation patterns of units in the hidden layer. The amount of interaction among representations will be measured by their degree of “activation overlap”. The activation overlap of a number of representations in the hidden layer is defined as their average shared activation over all of the units in the hidden layer. For example, if there are four hidden units and the representation for one input is (0.2, 0.1, 0.9, 0.1) and for a second is (0.2, 0.0, 1.0, 0.2), we calculate activation overlap by summing the smaller of the twoactivations (the “shared” activation) of each unit and averaging over all of the units. Here the activation overlap would be (0.2 + 0.0 + 0.9 + 0.1)/4 = 0.3.I suggest that the amount that two representations interfere with one another is directly proportional to their amount of activation overlap. For example, consider the two following activation patterns: (1, 0, 0, 0) and (0, 0, 1, 0). Their activation overlap is 0. Regardless of the weights of the connections between the hidden layer and the output layer, there will be no interference in the production of two separate output patterns. But as activation overlap increases, so does the level of interference.Therefore, if we can find a way to coax the network to produce representations with as little activation overlap as possible, we should be able to significantly reduce catastrophic forgetting.6 Sharpening the activation of hidden unitsA technique that I call “activation sharpening” will allow an FFBP system to gradually develop semi-distributed representations in the hidden layer. Activation sharpening consists of increasing the activation of some number of the most active hidden units by a small amount, slightly decreasing the activation of the other units in a similar fashion, and then changing the input-to-hidden layer weights to accommodate these changes. The new activation for nodes in the hidden layer is calculated as follows:A new = A old + α(1 - A old)for the nodes to be sharpened;A new = A old – αA old for the other nodes;where α is the sharpening factor.The idea behind this is the following. Nodes whose activation values are close to 1 will have a far more significant effect on the output, on average, than nodes with activations close to 0. If the system could evolve representations with a few highly activated nodes, rather than many nodes with average activation levels, this would reduce the average amount of activation overlap among representations. This should result in a decrease in catastrophic forgetting. In addition, because sharpening occurs gradually over the course of learning and continues even after a particular association has been learned, the representations developed will reflect the amount of training that it took to produce them.Let us consider one-node sharpening. On each pass we find the most active node, increase its activation slightly and decrease the activations of the other nodes. To preserve these changes we then backpropagate the difference between the pre-sharpened activation and the sharpened activation to the weights between the input layer and the hidden layer. Here are the details of the algorithm for k-node sharpening:•Perform a forward-activation pass from the input layer to the hidden layer. Record the activations in the hidden layer;• “Sharpen” the activations of k nodes;•Using the difference between the old activation and the sharpened activation on each node as "error", backpropagate this error to theinput layer, modifying the weights between the input layer and thehidden layer appropriately;•Do a full forward pass from the input layer to the output layer.•Backpropagate as usual from the output layer to the input layer;•Repeat.7 Experimental resultsThe experiments consisted of training (and overtraining) an 8–8–8 feedforwardbackpropagation network on a set of eleven associations. The learning rate was 0.2 and momentum 0.9. The network was then presented with a new association. After this new association had been learned, one of the associations from the first set was chosen and tested to see how well the system remembered it. On the first presentation of this previously learned association, the network invariably did very badly. The maximum error over all output nodes was almost always greater than 0.95 and the average error greater than 0.5. The amount of memory refresh required for a standard backpropagation network to relearn this association was recorded and compared to a network with one-node, two-node, three-node, etc. sharpening. In each case the sharpening factor was 0.2. The results are given in Figure 1a. (Note: 0-node sharpening is standard backpropagation.) It can be seen that one-node, two-node and three-node sharpening perform dramatically better than a standard FFBP network.Over twenty separate runs, the standard FFBP network required an average of 330 cycles to relearn the previously-learned association. This figure dropped to 81 cycles for one-node sharpening and to 53 cycles for two-node sharpening. (Note: all runs were terminated at 500 cycles.) When the activations of three or more nodes were sharpened, the amount of relearning began to rise again. With three-node sharpening 175 cycles were required. With four-node (326 cycles) and five-node (346 cycles) sharpening, the modified system does no better than standard backpropagation. Above this, it does significantly worse. [Figure 1a]The two graphs in Figures 1a and 1b suggest that amount of memory refresh required varies directly with the amount of activation overlap among representations. Figure 1b shows the amount of activation overlap of the eleven originally-learned inputs with various degrees of activation-sharpening. (As before “0 nodes sharpened”indicates standard backpropagation.) In general, the less activation overlap, the less the catastrophic forgetting as measured by the number of cycles required to relearn a previously-learned pattern.In Figure 2 we can see the effect of this sharpening algorithm on the representations of one association. For each of twenty runs, the activation patterns on the hidden nodes at the end of the initial training period were recorded. The nodes in each of the twenty runs were sorted according to their activation levels and these figures were then averaged. As might be expected, for standard backpropagation the distribution of activations over the eight nodes was approximately uniform. This gives an activation profile from the most active nodes to least active nodes of approximately constant slope. However, the result of one-node sharpening is quite dramatic; one of the eight nodes was much more active than the other seven. The same phenomenon can be observed for the other experiments where two or more nodes where sharpened.8. Why does activation sharpening work?Let us examine why activation sharpening reduces catastrophic forgetting. Consider two-node sharpening. As the system learns the first set of associations, it develops a set of sharpened representations in the hidden layer. A new association is then presented to the network. Activation sharpening immediately starts to coax the new representation into a sharpened form where two of the eight hidden nodes are highly active and six are not. Thus, very early on, the newly developing representation will have less chance of activation overlap with the already-formed representations than in standard backpropagation, where the activation is spread out over all eight nodes.Sharpened activation patterns interfere less with the weights of the network than unsharpened ones. The reason for this has to do with the way the backpropagation algorithm changes weights. When the activation of a node is near zero, the weight changes of the links associated with it are small. Thus, if a significant number of the nodes in the new representation have a very low activation, then the weights on the1234567R e l e a r n i n g t i m e (c y c l e s )No. of nodes sharpenedEffect of Sharpening onAmount of Memory Refresh0.10.20.30.40.50.60.70.80.912A c t i v a t i o n o v e r l a pNo. of nodes sharpened Effect of Sharpening on 34567Activation OverlapFigure 1aFigure 1bconnections to and from that node will be modified much less, on average, than the weights associated with a highly active node. Therefore, the only representations significantly affected by the new representation will be those in which highly active nodes overlap. Consequently, if we reduce the probability of this overlap by activation sharpening, there will be a decrease in the amount of disruption of the old weights and catastrophic interference will be reduced. The idea is to sharpen new activation patterns as quickly as possible, thereby decreasing their potential to interfere with already learned patterns. Keeping the learning rate low (≤ 0.2) with a relatively high sharpening factor (0.2) allows new activation patterns to become sharpened before they have a chance to do much damage to previously-learned weights. Preliminary experiments in fact indicate that as the learning rate is decreased with the sharpening factor held constant, catastrophic forgetting decreases.It seems likely that semi-distributed representations will cost the network some of its ability to generalize. Optimal generalization depends on as much information as possible taking part in mapping from the input space to the output space. Any mechanism tending to reduce the amount of information brought to bear on a new association would most likely reduce the quality of the mapping. In some sense,activation sharpening forces the input data through a representational bottleneck and this results in information being lost. The extent and severity of this loss and its effect on generalization is a subject of ongoing study.9 How many nodes should be sharpened?This is an open question. For n nodes in the hidden layer, the answer might be k where k is the smallest integer such that n C k is greater than the number of inputs. In other words, a sufficient number of nodes should be sharpened to allow the existence of enough distinct sharpened representations to cover the input space. To minimize the activation overlap, the least such sufficient number of sharpened nodes should be chosen. If the number of input patterns to be learned is not known in advance, itA c t i v a t i o nA c t i v a t i o nA c t i v a t i o nThree-node sharpening0.20.40.60.8A c t i v a t i o nEffect of Sharpening on Hidden-LayerActivation ProfilesFigure 2: Activation Profiles with various node-sharpeningsmight be reasonable to sharpen approximately log n nodes. This estimate is based on work on crosstalk [Willshaw 1981]. This work indicates that in a distributed memory crosstalk can be avoided when the number of active units for each input pattern isproportional to the logarithm of the total number of units. It would seem reasonable to apply this result to the sharpening of hidden-unit activations.10 ConclusionIn this paper I have argued that catastrophic forgetting in distributed systems is a direct consequence of the amount of overlap of representations in that system. I have further suggested that the trade-off between catastrophic forgetting and generalization is inevitable. It is claimed that one way to maintain generalization capabilities while reducing catastrophic forgetting is to use semi-distributed representations. To this end,I presented a simple method to allow a feedforward backpropagation network to dynamically evolve its own semi-distributed representations.AcknowledgmentsI would like to thank Mark Weaver for his invaluable assistance with the ideas and emphasis of this paper. I would also like to thank David Chalmers, Terry Jones, and the members of CRCC and SESAME for their many helpful comments.BibliographyFeldman, J. A., [1988], “Connectionist Representation of Concepts”, In C o n n e c t i o n i s t Models and Their Implications, Waltz, D. and Feldman, J. (eds.), 341–363.French, R. M. and Jones, T. C., [1991], “Differential hardening of link weights: A simple method for decreasing catastrophic forgetting in neural networks”, CRCC Technical Report 1991–50.Hetherington, P. A. and Seidenberg, M. S., [1989], “Is there ‘catastrophic interference’in connectionist networks?”, Proceedings of the 11th Annual Conference of the Cognitive Science Society, Hillsdale, NJ: Erlbaum, 26–33.Kanerva, Pentti, [1988], Sparse Distributed Memory, Cambridge, MA: MIT Press.Kortge, Chris A., [1990]. “Episodic Memory in Connectionist Networks”, Proceedings of the 12th Annual Conference of the Cognitive Science Society, Hillsdale, NJ: Erlbaum, 764-771.Kruschke, J. K., [1990], “ALCOVE: A exemplar-based connectionist model of category learning”, Indiana University Cognitive Science Research Report 19, February 22, 1991.McCloskey, M. and Cohen, N. J., [1989], “Catastrophic interference in connectionist networks: The sequential learning problem”, The Psychology of Learning and Motivation,Vol. 24, 109-165.Nosofsky, R. M., [1984], “Choice, similarity and the context theory of classification”, J. Exp. Psych. Learning, Memory and Cognition, Vol. 10, 104-114.Ratcliff, R., [1990], “Connections models of recognition memory: Constraints imposed by learning and forgetting function”, Psychological Review, Vol. 97, 285-308.Sloman, S. and Rumelhart, D., [1991], “Reducing interference in distributed memories through episodic gating”, In A. Healy, S. Kosslyn, and R. Shiffrin (eds.), Essays in Honor of W. K. Estes (in press).Weaver, M., [1990], “An active symbol connectionist model of concept learning”(unpublished manuscript).Willshaw, D., [1981], “Holography, associative memory, and inductive generalization”, In G.E. Hinton & J. A. Anderson (eds.), Parallel models of associative memory. Hillsdale, NJ: Erlbaum.。