Spatially Filtering the Binary Confusion Noise for Space Gravitational Wave Detectors
无取样受限波尔兹曼机的图像重建研究
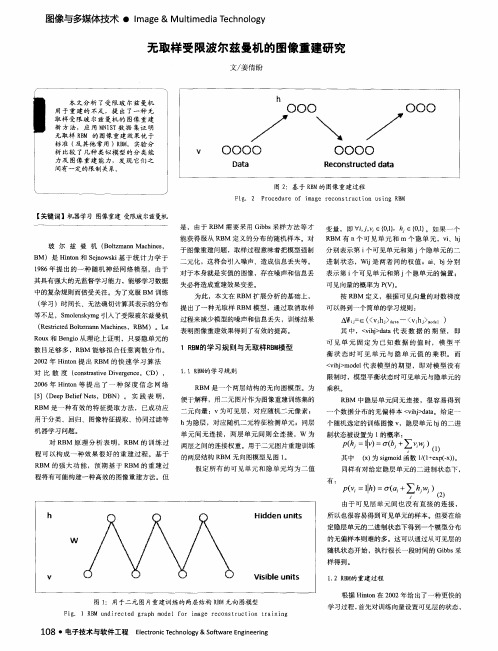
可 见 单 元 固 定为 已 知 数 据 的 值 时 ,模 型 平 衡 状 态 时 可 见 单 元 与 隐 单 元 值 的 乘 积 。 而
< v i h j > mo d e l 代表 模型 的期 望 ,即对 模型 没 有
2 0 0 2年 H i n t o n 提 出R B M 的快 速学 习算 法
对 比散度 ( c o n s t r a s t i v e Di v e r g e n c e ,C D) , 2 0 0 6年 Hi n t o n等提 出 了 一 种 深 度 信 念 网 络 【 5 ]( De e pBe l i e f Ne t s ,DB N)。 实 践 表 明 , R BM 是一种有 效 的特征提 取方法 , 已成功 应 用于分类 、回归 、图像特征提取 、协同过滤等
图像与多媒体技术 ・ I ma g e&Mu l t i me d i a T e c h n o l o g y
无取样 受限波尔兹 曼机 的图像 重建研 究
文/ 姜 倩 盼
本 丈分 析 了受 限玻 尔兹 曼机 用 于 重 建 的 不 足 , 提 出 了一 种 无 取样 受限玻 尔兹曼机 的图像 重建 新 方 法 ,应 用 M N I S T数 据 集 证 明
对R BM 原理 分析 表 明 ,R BM 的 训 练过 程 可 以构成 一种 效果 很好 的重 建 过程 。基 于 R BM 的强大 功 能 ,预 期基 于 RB M 的重建 过
p ( h j = 1 I V ) = ( + ∑ ) …
其中 ( x ) 为s i g m o i d函数 1 / ( 1 + e x p ( 一 x ) ) 。
( R e s t r i c t e d B o l t z ma n n Ma c h i n e s ,R BM)。L e R o u x和 B e n g i o从理论上 证 明,只要 隐单元 的 数 目足够 多 ,RB M 能够 拟合任 意离散 分布 。
1-Bit压缩感知盲重构算法

1-Bit压缩感知盲重构算法张京超;付宁;杨柳【摘要】1-Bit压缩感知(CS)是压缩感知理论的一个重要分支。
该领域中二进制迭代硬阈值(BIHT)算法重构精度高且一致性好,是一种有效的重构算法。
该文针对BIHT 算法重构过程需要信号稀疏度为先验信息的问题,提出一种稀疏度自适应二进制迭代硬阈值算法,简称为SABIHT算法。
该算法修正了BIHT算法,首先通过自适应过程自动调节硬阈值参数,然后利用测试条件估计信号的稀疏度,最终实现不需要确切信号稀疏度的1-Bit压缩感知盲重构。
理论分析和仿真结果表明,该算法较好地实现了未知信号稀疏度的精确重建,并且与 BIHT 算法相比重构精度及算法复杂度均相当。
%1-Bit Compressive Sensing (CS) is an important branch of standard CS. The existing 1-Bit CS algorithm-Binary Iterative Hard Thresholding (BIHT) can perfectly recovery signals with high precision and consistency, which requires exact sparsity level in the recovery phase. Considering this problem, a new Sparsity Adaptive Binary Iterative Hard Thresholding (SABIHT) algorithm without prior information of the sparsity is proposed by modifying the BIHT algorithm. By using the adaptive process of automatically adjusting the hard threshold parameters and test conditions to estimate the sparsity, the proposed algorithm realizes accurate reconstruction and estimates the true supporting set of approximated signal. The analytical theory and simulation results show that the SABIHT algorithm recovers effectively the signals without prior information of signal sparsity and the reconstruction performance is similar to the BIHT algorithm.【期刊名称】《电子与信息学报》【年(卷),期】2015(000)003【总页数】7页(P567-573)【关键词】压缩感知;1-Bit压缩感知;盲重构;二进制迭代硬阈值【作者】张京超;付宁;杨柳【作者单位】哈尔滨工业大学自动化测试与控制系哈尔滨 150080;哈尔滨工业大学自动化测试与控制系哈尔滨 150080;哈尔滨工业大学自动化测试与控制系哈尔滨 150080【正文语种】中文【中图分类】TN911.72压缩感知理论[1-3]是近年发展起来的新的信号获取和信号处理理论。
奇异值分解低通滤波眼底图像归一化
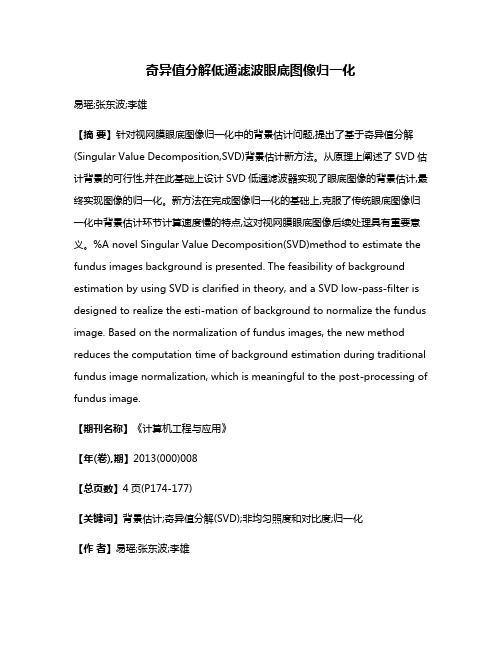
奇异值分解低通滤波眼底图像归一化易瑶;张东波;李雄【摘要】针对视网膜眼底图像归一化中的背景估计问题,提出了基于奇异值分解(Singular Value Decomposition,SVD)背景估计新方法。
从原理上阐述了SVD估计背景的可行性,并在此基础上设计SVD低通滤波器实现了眼底图像的背景估计,最终实现图像的归一化。
新方法在完成图像归一化的基础上,克服了传统眼底图像归一化中背景估计环节计算速度慢的特点,这对视网膜眼底图像后续处理具有重要意义。
%A novel Singular Value Decomposition(SVD)method to estimate the fundus images background is presented. The feasibility of background estimation by using SVD is clarified in theory, and a SVD low-pass-filter is designed to realize the esti-mation of background to normalize the fundus image. Based on the normalization of fundus images, the new method reduces the computation time of background estimation during traditional fundus image normalization, which is meaningful to the post-processing of fundus image.【期刊名称】《计算机工程与应用》【年(卷),期】2013(000)008【总页数】4页(P174-177)【关键词】背景估计;奇异值分解(SVD);非均匀照度和对比度;归一化【作者】易瑶;张东波;李雄【作者单位】湘潭大学信息工程学院,湖南湘潭 411105;湘潭大学信息工程学院,湖南湘潭 411105;湘潭大学信息工程学院,湖南湘潭 411105【正文语种】中文【中图分类】TP751视网膜眼底图像由于获取过程中照明、曲面反射[1],普遍存在非均匀照明和对比度现象,如图1(a),眼底图像内和图像之间的这种照明和对比度变化,造成某些区域的病变难以观测,从而严重影响诊断过程和结果,因此有必要在检测前进行归一化处理。
基于加权奇异值分解截断共轭梯度的电容层析图像重建
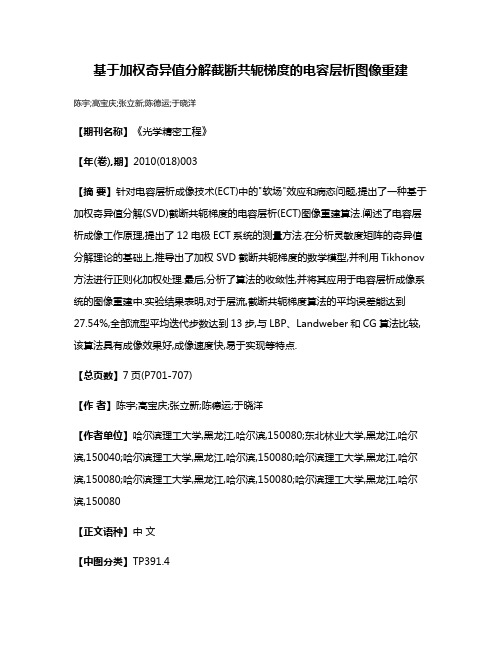
基于加权奇异值分解截断共轭梯度的电容层析图像重建陈宇;高宝庆;张立新;陈德运;于晓洋【期刊名称】《光学精密工程》【年(卷),期】2010(018)003【摘要】针对电容层析成像技术(ECT)中的"软场"效应和病态问题,提出了一种基于加权奇异值分解(SVD)截断共轭梯度的电容层析(ECT)图像重建算法.阐述了电容层析成像工作原理,提出了12电极ECT系统的测量方法.在分析灵敏度矩阵的奇异值分解理论的基础上,推导出了加权SVD截断共轭梯度的数学模型,并利用Tikhonov 方法进行正则化加权处理.最后,分析了算法的收敛性,并将其应用于电容层析成像系统的图像重建中.实验结果表明,对于层流,截断共轭梯度算法的平均误差能达到27.54%,全部流型平均迭代步数达到13步,与LBP、Landweber和CG算法比较,该算法具有成像效果好,成像速度快,易于实现等特点.【总页数】7页(P701-707)【作者】陈宇;高宝庆;张立新;陈德运;于晓洋【作者单位】哈尔滨理工大学,黑龙江,哈尔滨,150080;东北林业大学,黑龙江,哈尔滨,150040;哈尔滨理工大学,黑龙江,哈尔滨,150080;哈尔滨理工大学,黑龙江,哈尔滨,150080;哈尔滨理工大学,黑龙江,哈尔滨,150080;哈尔滨理工大学,黑龙江,哈尔滨,150080【正文语种】中文【中图分类】TP391.4【相关文献】1.一种基于截断奇异值分解正则化的电离层层析成像算法 [J], 欧明;甄卫民;於晓;徐继生;邓忠新2.三项共轭梯度的电容层析成像图像重建算法 [J], 陈宇;孙帆;张健3.基于数据驱动的卷积神经网络电容层析成像图像重建 [J], 孙先亮; 李健; 韩哲哲; 许传龙4.基于改进ALEXNET卷积神经网络的电容层析成像三维图像重建 [J], 李岩;王璐;李佳琪5.基于概率加权共轭梯度算法的混凝土超声波层析成像 [J], 刘建军;许令周因版权原因,仅展示原文概要,查看原文内容请购买。
基于自适应对偶字典的磁共振图像的超分辨率重建
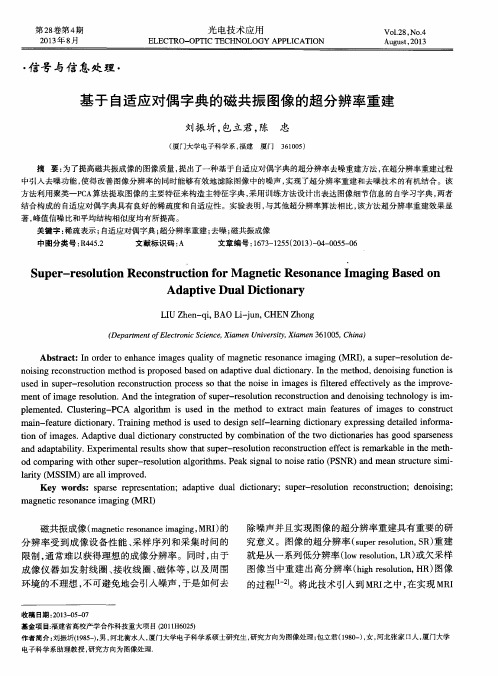
L I U Z h e n - q i , B A 0 L i - j u n , C HE N Z h o n g
r De p a r t m e n t o f E l e c t r o n i c S c i e n c e , X i a me n U n i v e r s i t y , Xi a me n 3 6 1 0 0 5 , C h i n a )
刘振 圻 , 包立君 , 陈 忠
( 厦 门大学电子科 学系, 福建 厦门 3 6 1 0 0 5 )
摘 要: 为了提高磁共振成像的图像 质量 , 提 出了一种基于 自适应对偶字典的超分辨率 去噪重建方法 , 在超分辨率重建过程 中引入去噪功能 , 使 得改善图像 分辨率的同时能够有效地滤除 图像 中的噪声 , 实现 了超分辨率重建和去噪技术 的有机结合 。该 方法利用聚类一P c A算 法提取图像的主要特征来构造主特征字典 , 采用 训练方法设计 出表达图像 细节信 息的 自学 习字 典 , 两者 结合构成的 自适应对偶字典具有 良好 的稀疏度和 自适应性 。实验表 明, 与其他超分辨率算法相 比, 该方法超分辨率重建效果显 著, 峰值信噪 比和平均结构相似度均有所提高。
第2 8 卷第 4 期
2 0 1 3 年8 月
பைடு நூலகம்光 电技术 应 用
EL ECT RO一 0P T I C T ECHNOLOGY AP P LI CAT1 0N
V O1 . 28. NO. 4
Au g u s t , 2 01 3
・
信号 与信息处理 ・
基 于 自适应对偶 字典的磁共振 图像 的超 分辨率重建
用小波限制紧算子逼近奇异积分算子的速度

用小波限制紧算子逼近奇异积分算子的速度
杨奇祥
【期刊名称】《数学进展》
【年(卷),期】2003(032)005
【摘要】根据Beylin-Coifman-Rokhlin和Yahg的观点,用合适的小波基可以刻画性地研究C.Z算子或拟微分算子,这样就可以用小波来计算算子.比如可以从奇异积分算子的B-C-R算法刻画出发,用小波限制紧算子进行逼近.本文旨在计算作为原算子与逼近算子的差的误差算子的H1到L1的连续性范数的最佳衰减速度和在Lp 上的连续性范数的衰减速度的范围.
【总页数】6页(P547-552)
【作者】杨奇祥
【作者单位】武汉大学数学学院,武汉,湖北,430072,中国
【正文语种】中文
【中图分类】O177.2
【相关文献】
1.一类奇异积分算子与无界连续函数逼近 [J], 郑成德
2.紧算子的正逼近 [J], 裴君莹;杜鸿科;刘三阳
3.基于小波分析方法的一类函数的限制逼近 [J], 张玲玲
4.一类拟总体列紧算子逼近的正则值 [J], 孟京华
5.Мираквян奇异积分算子与无界连续函数逼近 [J], 郑成德;王仁宏
因版权原因,仅展示原文概要,查看原文内容请购买。
随机森林算法改进综述
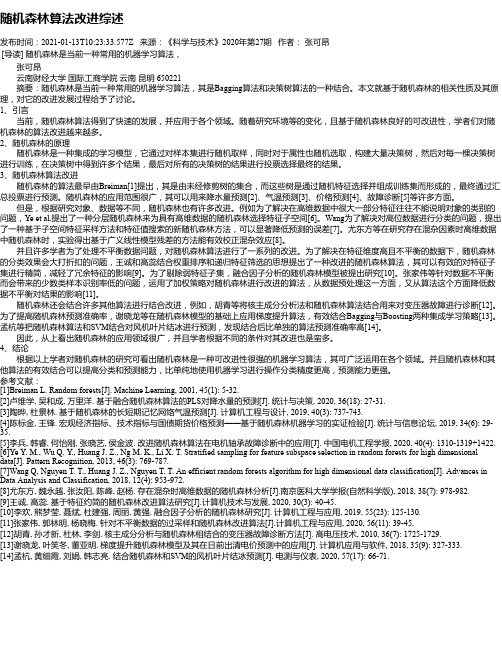
随机森林算法改进综述发布时间:2021-01-13T10:23:33.577Z 来源:《科学与技术》2020年第27期作者:张可昂[导读] 随机森林是当前一种常用的机器学习算法,张可昂云南财经大学国际工商学院云南昆明 650221摘要:随机森林是当前一种常用的机器学习算法,其是Bagging算法和决策树算法的一种结合。
本文就基于随机森林的相关性质及其原理,对它的改进发展过程给予了讨论。
1、引言当前,随机森林算法得到了快速的发展,并应用于各个领域。
随着研究环境等的变化,且基于随机森林良好的可改进性,学者们对随机森林的算法改进越来越多。
2、随机森林的原理随机森林是一种集成的学习模型,它通过对样本集进行随机取样,同时对于属性也随机选取,构建大量决策树,然后对每一棵决策树进行训练,在决策树中得到许多个结果,最后对所有的决策树的结果进行投票选择最终的结果。
3、随机森林算法改进随机森林的算法最早由Breiman[1]提出,其是由未经修剪树的集合,而这些树是通过随机特征选择并组成训练集而形成的,最终通过汇总投票进行预测。
随机森林的应用范围很广,其可以用来降水量预测[2]、气温预测[3]、价格预测[4]、故障诊断[5]等许多方面。
但是,根据研究对象、数据等不同,随机森林也有许多改进。
例如为了解决在高维数据中很大一部分特征往往不能说明对象的类别的问题,Ye et al.提出了一种分层随机森林来为具有高维数据的随机森林选择特征子空间[6]。
Wang为了解决对高位数据进行分类的问题,提出了一种基于子空间特征采样方法和特征值搜索的新随机森林方法,可以显著降低预测的误差[7]。
尤东方等在研究存在混杂因素时高维数据中随机森林时,实验得出基于广义线性模型残差的方法能有效校正混杂效应[8]。
并且许多学者为了处理不平衡数据问题,对随机森林算法进行了一系列的改进。
为了解决在特征维度高且不平衡的数据下,随机森林的分类效果会大打折扣的问题,王诚和高蕊结合权重排序和递归特征筛选的思想提出了一种改进的随机森林算法,其可以有效的对特征子集进行精简,减轻了冗余特征的影响[9]。
尔曼滤波器(贝叶斯算法
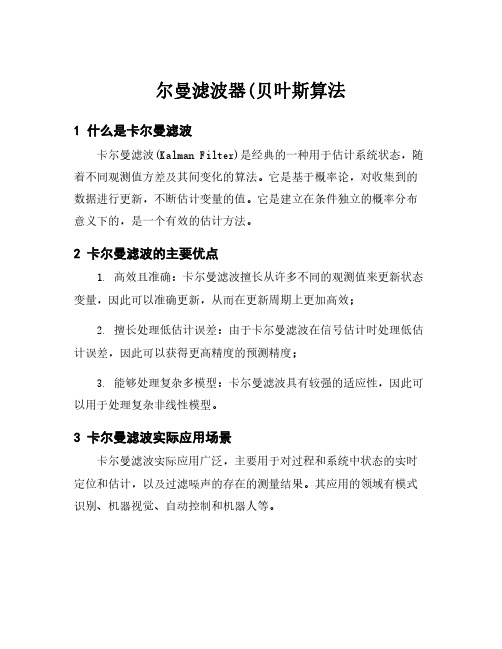
尔曼滤波器(贝叶斯算法
1什么是卡尔曼滤波
卡尔曼滤波(Kalman Filter)是经典的一种用于估计系统状态,随着不同观测值方差及其间变化的算法。
它是基于概率论,对收集到的数据进行更新,不断估计变量的值。
它是建立在条件独立的概率分布意义下的,是一个有效的估计方法。
2卡尔曼滤波的主要优点
1.高效且准确:卡尔曼滤波擅长从许多不同的观测值来更新状态变量,因此可以准确更新,从而在更新周期上更加高效;
2.擅长处理低估计误差:由于卡尔曼滤波在信号估计时处理低估计误差,因此可以获得更高精度的预测精度;
3.能够处理复杂多模型:卡尔曼滤波具有较强的适应性,因此可以用于处理复杂非线性模型。
3卡尔曼滤波实际应用场景
卡尔曼滤波实际应用广泛,主要用于对过程和系统中状态的实时定位和估计,以及过滤噪声的存在的测量结果。
其应用的领域有模式识别、机器视觉、自动控制和机器人等。
4贝叶斯算法
贝叶斯算法是一种基于贝叶斯定理的机器学习算法。
它的核心思想是利用已经掌握的知识,来推断新的事件的可能性,同时从已知的概率性质中推测新发生事件的概率。
它是由英国数学家Thomas Bayes 在18世纪发明的一种用于处理模糊性和不可靠信息的统计方法。
贝叶斯算法能够根据已有结果,得出新的概率分布,从而有效的解决分类和推断的问题。
它的优点在于可以利用已有知识,对未知事件进行推断预测,常用于文本分类、垃圾邮件过滤、条件概率分布求解以及基因分析等领域。
超定情况下的基于稀疏成分分析的盲图像源分离
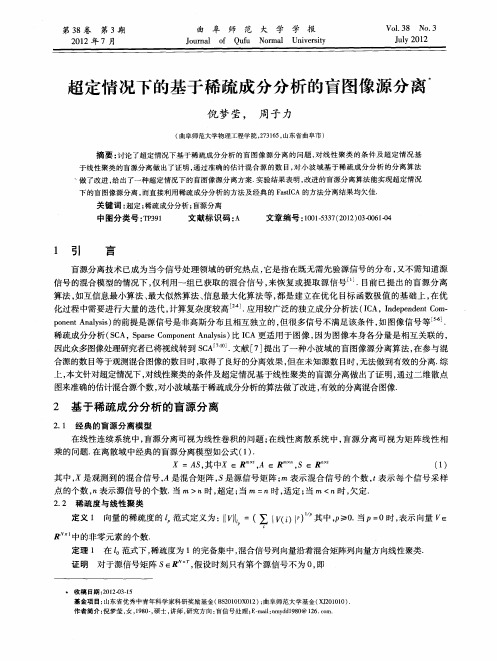
发现 , 只有 同列 之 间元 素存 在 关系 , 而与 同行元 素间 大小无 关 . 其它适 定 情况 以此类 推.
■ 一
哪 豫 嚣
.
r . 5 7 7 0 7 6 2 0 0 3 6 8 4 5 91 0 3 0 3 8 2 2 7 6] 439 782 9O 0 . 8 4 9 8 8 1 4 .9970 333 -
l = alSl+a1S 1 2 2, X2 = a 】 2S】+ a 2 2 2S .
尊
嘲
s置 五 奇2l , 一 a 1 2l . 1 一 一a 2 a 1 一一
3 I 测试 中随 机产 生的混 合矩 阵 A及 聚类 .
l a 12 一 2 1 … 2 a .
因此众多图像处理研究者已将视线转到 S A7 文献 [ ] C l1. -3 0 7 提出了一种小波域的盲图像源分离算法 , 在参与混 合源的数 目等于观{混合图像的数 目时, 贝 0 取得了良好的分离效果 , 但在未知源数 目时 , 无法做到有效的分离. 综 上, 本文针对 超定情况下 , 性聚类 的条件及 超定 情况 基 于线性 聚类 的盲 源分离 做 出了证 明 , 对线 通过 二维 散点 图来准确的估计?合源个数 , 昆 对小波域基于稀疏成分分析的算法做了改进 , 有效的分离混合图像.
3期
倪 梦 莹 , : 定情 况下 的基 于稀 疏成 分分 析 的盲 图像 源分 离 等 超
6 3
定理 2 在忽略分离时序和幅度大小及适定的前提下 , 源信号 s 复的正确与否只与混合矩阵 A的列 恢
方 向有 关 , 行方 向无 关. 与
证 2=2 ,和写行量 式有 ]口:[, 为 明1 n为将 S 向的 , 【= 主展  ̄ =例 为 形 则 主 【 1 】开 m a 12 】
一种新的低复杂度基于变换域的图像差错掩盖算法
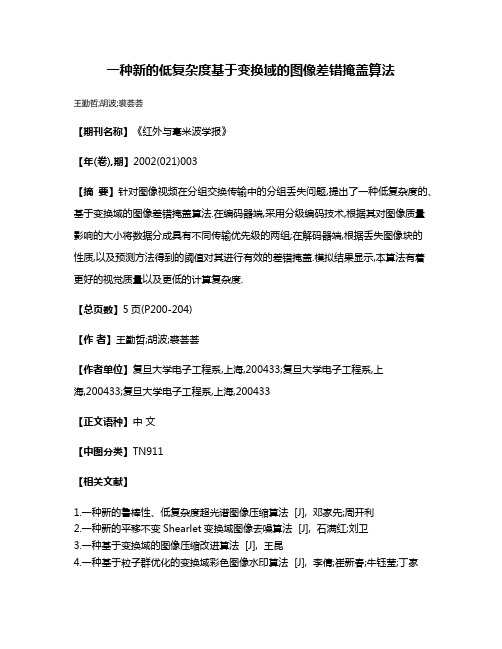
一种新的低复杂度基于变换域的图像差错掩盖算法
王勤哲;胡波;裘荟荟
【期刊名称】《红外与毫米波学报》
【年(卷),期】2002(021)003
【摘要】针对图像视频在分组交换传输中的分组丢失问题,提出了一种低复杂度的、基于变换域的图像差错掩盖算法.在编码器端,采用分级编码技术,根据其对图像质量影响的大小将数据分成具有不同传输优先级的两组;在解码器端,根据丢失图像块的
性质,以及预测方法得到的阈值对其进行有效的差错掩盖.模拟结果显示,本算法有着更好的视觉质量以及更低的计算复杂度.
【总页数】5页(P200-204)
【作者】王勤哲;胡波;裘荟荟
【作者单位】复旦大学电子工程系,上海,200433;复旦大学电子工程系,上
海,200433;复旦大学电子工程系,上海,200433
【正文语种】中文
【中图分类】TN911
【相关文献】
1.一种新的鲁棒性、低复杂度超光谱图像压缩算法 [J], 邓家先;周开利
2.一种新的平移不变Shearlet变换域图像去噪算法 [J], 石满红;刘卫
3.一种基于变换域的图像压缩改进算法 [J], 王昆
4.一种基于粒子群优化的变换域彩色图像水印算法 [J], 李倩;崔新春;牛钰莹;丁家
琳
5.一种新的彩色图像变换域全息水印算法 [J], 李晨璐;孙刘杰
因版权原因,仅展示原文概要,查看原文内容请购买。
非局部主成分分析极大似然估计MRI图像Rician噪声去噪
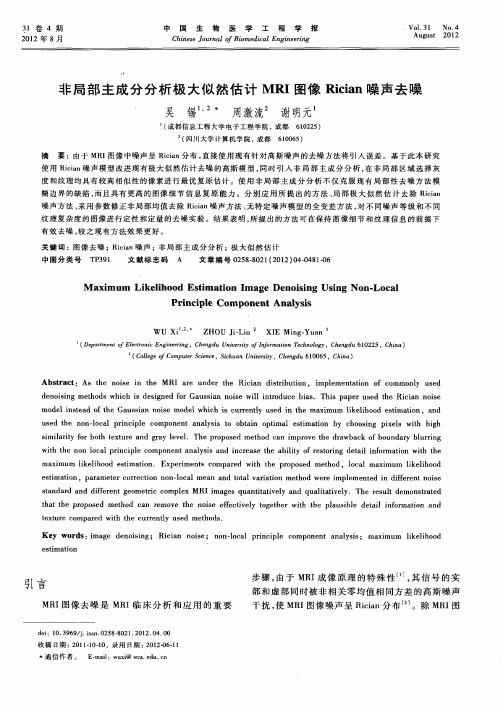
W U Xi, 一
Z HOU J— i iL u
XI i gYu n E M n — a
( e at n o l t ncE gn eig hn d nvr t I o m t n T c n l y h n d 1 2 5 h n ) Dp r me tfE e r i n i i ,C e g u U i s y o n r ai eh oo ,C e g u6 0 2 ,C ia co e' n ei f f o g
( oeeo o p t c ne ScunU i rt,C eg u6 0 6 ,C ia C lg C m ue Si c, i a nv sy hn d 10 5 hn ) l f r e h ei
Abs r c :As t e o s i t e ta t h n ie n h MRI r u d r h Ri i n iti u i n, i l me t t n f c mmo l u e a e n e t e c a d srb t o mp e n a i o o o ny s d
噪声方法 、 采用参数修正非局部 均值去除 R c n噪声方法 、 特定噪声模型 的全变差方 法 , 不同 噪声 等级 和不 同 ia i 无 对
纹 理 复 杂 度 的 图像 进 行 定 性 和 定 量 的 去 噪 实 验 。结 果 表 明 , 提 出 的 方 法 可 在 保 持 图 像 细 节 和 纹 理 信 息 的 前 提 下 所
M a i u k lh o tm a i n I a e D e ii g U sng No Lo a x m m Li ei o d Esi to m g no sn i n- c l
Pr n i l m po ntAna y i i c p e Co ne l ss
采用双树复小波和混合概率模型的光学相干层析图像去噪
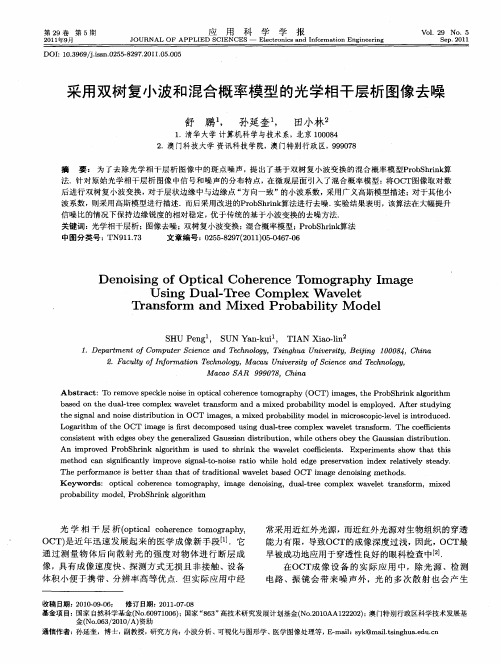
An i p o e o S rn lo ih i s d t h i k t e wa ee o m ce t. Ex e i n s s o t t t i m r v d Pr b h ik ag rt m S u e o s rn h v lt c e in s p rme t h w ha hs
b s d o h u lt e o a e n t e d a - r e c mplx wa e e r n f r a d a m i e r b b l y m o e Se e v lt t a so m n x d p o a i t d l mply d.Afe t dy n i i oe t rs u i g t e sg a n o s it i u i n i h i n l d n ie d s rb t o n 0CT m a e . x d p o a l y m o e n m i r s o i -e e n r d c d. a i g s a mi e r b bi t d li c o c p c l v l s i t o u e i i Lo a ihm ft e 0CT ma e i r t d c mpo e sn u lt e o p e v l t t a so m .Th o f ce t g rt o h i g s f s e o i s d u i g d a - r e c m lx wa e e r n f r ec e iin s c n it n t d e be h e e a i e a s i n d s rb t o wh l t e s o y t e Ga s i n d s rb t o o ss e t wih e g s o y t e g n r l d G u sa it i u i n, z i o h r be h u sa it i u i n. e
小波包变换广义回归神经网络同时分辨三种有机化合物的重叠光谱

2008年 1 0月
光
谱
学
与
光
谱
分
析
* 1 8N .0p 9— 9 C . ,o 1,N322 5 o2 3
Oc o e ,2 0 tb r 0 8
S e to c p n p c r lAn l ss p c r s o y a d S e ta ay i
层和输出层 。 R N结构简图如图 1 GN 所示 , 入层 不处理信 输
息 ,只为模式层分配输 入信 息 。输入 层与模 式层 全部相 连。
模式层 中每一神经元代 表一个径 向基 函数 。一个通用的高斯
核心 函数应用于模式层 中。
H() p x一 厂
‘ L ‘
旦]
_ J
第 1 期 O
N
光谱 学与光谱分析
2 9 33
对的 。 求 层中 H() 单 别 经元 算 数目 在 和 ∑ 经由 个特 神 计 x
f 1 一
以 试剂 空 白为 参 比 , 得 对 硝基 苯 胺 和 萘 胺 和联 苯 胺 测
光谱严重重叠的三种有 机化合物进行 同时测定 。 该法结 合小波包变换 ( T) WP 和广义 回归神经 网络 ( R G NN) 改进 了除噪质量和预测能力 。通过最佳 化 , 选择了小波 函数 、 波包分解 水平及 GR N 的平滑 因子 。偏最 小 N
小二乘 ( L ) P S 法用 于 比较研究 ,编制 了三个程序 ( WP R P TG NN,P RN 和 P L ) G N P S 进行 相关计算 。结果表
明, WFF RN G N法是成功的且优于 GR NN及 P S方法 , GR L 与 NN方 法 比较所有组分质 量浓度的预测值 与 实际值 的相对预测标准误差由 4O 降低为 2 3 。 . .
Collaborative

Collaborative filteringCollaborative filtering,即协同过滤,是⼀种新颖的技术。
最早于1989年就提出来了,直到21世纪才得到产业性的应⽤。
应⽤上的代表在国外有,Last.fm,Digg等等。
最近由于毕业论⽂的原因,开始研究这个题⽬,看了⼀个多星期的论⽂与相关资料之后,决定写篇总结来总结⼀下最近这段时间资料收集的成果。
在微软1998年的那篇关于协同过滤的论⽂[1]中,将协同过滤分成了两个流派,⼀个是Memory-Based,⼀个是Model-Based。
关于Memory-Based的算法,就是利⽤⽤户在系统中的操作记录来⽣成相关的推荐结果的⼀种⽅法,主要也分成两种⽅法,⼀种是User-Based,即是利⽤⽤户与⽤户之间的相似性,⽣成最近的邻居,当需要推荐的时候,从最近的邻居中得到推荐得分最⾼的⼏篇⽂章,⽤作推荐;另外⼀种是Item-Based,即是基于item之间的关系,针对item来作推荐,即是使⽤这种⽅法,使⽤⼀种基本的⽅法来得到不俗的效果。
⽽实验结果也表明,Item-Based的做法⽐User-Based更有效[2]。
⽽对于Model-Based的算法,即是使⽤机器学习中的⼀些建模算法,在线下对于模型进⾏预计算,在线上能够快速得出结果。
主要使⽤的算法有 Bayesian belief nets , clustering , latent semantic , 最近⼏年⼜出现了使⽤SVM 等的CF算法。
最近⼏年⼜提出⼀种新的分类,content-based,即是对于item的内容进⾏分析,从⽽进⾏推荐。
⽽现阶段,⽐较优秀的⼀些应⽤算法,则是将以上⼏种⽅法,混合使⽤。
⽐较说Google News[3],在它的系统中,使⽤了⼀种将Memory-Based与Model-Based两种⽅法混合的算法来处理。
在Google的那篇论⽂⾥⾯,它提到了如何构建⼀个⼤型的推荐系统,其中Google的⼀些⾼效的基础架构如:BigTable,MapReduce等得到很好的应⽤。
复数小波与椭圆窗维纳滤波结合图像去噪方法
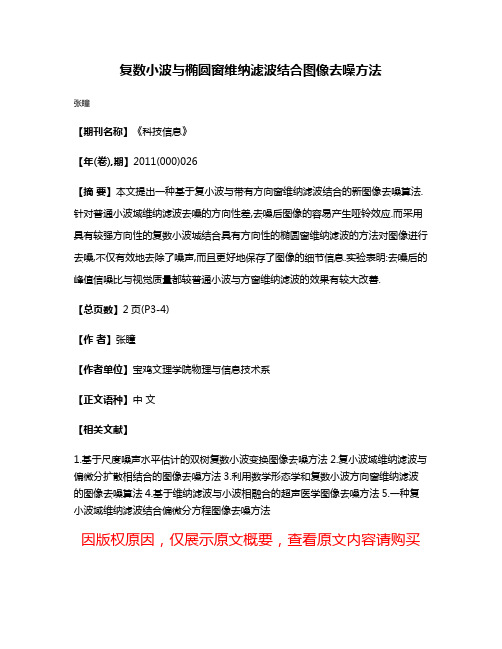
复数小波与椭圆窗维纳滤波结合图像去噪方法
张瞳
【期刊名称】《科技信息》
【年(卷),期】2011(000)026
【摘要】本文提出一种基于复小波与带有方向窗维纳滤波结合的新图像去噪算法.针对普通小波域维纳滤波去嗓的方向性差,去噪后图像的容易产生哑铃效应.而采用具有较强方向性的复数小波城结合具有方向性的椭圆窗维纳滤波的方法对图像进行去嗓,不仅有效地去除了嗓声,而且更好地保存了图像的细节信息.实验表明:去嗓后的峰值信噪比与视觉质量都较普通小波与方窗维纳滤波的效果有较大改善.
【总页数】2页(P3-4)
【作者】张瞳
【作者单位】宝鸡文理学院物理与信息技术系
【正文语种】中文
【相关文献】
1.基于尺度噪声水平估计的双树复数小波变换图像去噪方法
2.复小波域维纳滤波与偏微分扩散相结合的图像去噪方法
3.利用数学形态学和复数小波方向窗维纳滤波的图像去噪算法
4.基于维纳滤波与小波相融合的超声医学图像去噪方法
5.一种复小波域维纳滤波结合偏微分方程图像去噪方法
因版权原因,仅展示原文概要,查看原文内容请购买。
人声分离算法

人声分离算法人声分离是一种音频信号处理技术,旨在从混合音频中分离或提取出特定的人声信号。
这项任务通常是在语音处理、音乐处理以及音频增强等领域中应用的重要技术。
以下是一些常见的人声分离算法:1. 基于深度学习的方法:• Deep Clustering:使用深度学习模型,如深度聚类网络(Deep Clustering Network, DCN),学习在频谱域对音频进行聚类,以实现音源分离。
该方法在训练过程中将相似的频谱点聚类在一起,从而使网络能够学到不同音源的表示。
• Deep attractor network (DAN):通过学习音源的吸引子表示,这种方法使得模型能够在频谱上分离不同的音源。
2. 基于短时傅立叶变换(STFT)的方法:• Non-negative Matrix Factorization (NMF):将音频信号表示为非负矩阵的乘积,其中一个矩阵表示基础音源,另一个矩阵表示每个时间点的激活系数。
通过调整这两个矩阵,可以分离出人声信号。
• Independent Component Analysis (ICA):基于统计模型,假设混合信号是独立的非高斯过程,通过最大似然估计方法来分离不同的源信号。
3. 基于时域处理的方法:• Ideal Binary Mask (IBM):通过分析语音和非语音的频谱差异,生成一个二进制掩码,用于选择性地过滤和分离人声信号。
• Phase-sensitive Reconstruction (PSR):基于相位信息的处理,通过在频域上对信号进行修复和重新构建来分离人声。
4. 基于卷积神经网络(CNN)的方法:• U-Net Architecture:基于 U-Net 结构的深度学习模型,通过卷积层和上采样层实现对音频信号的高级特征学习和重建。
请注意,人声分离是一个复杂的问题,其效果受到许多因素的影响,如音频质量、混合信号的复杂性以及算法的设计。
选择合适的方法取决于实际应用的要求和环境。
贝叶斯超参数优化 多层感知器

贝叶斯超参数优化是一种用于自动调整机器学习模型超参数的优化技术。
它使用贝叶斯概率理论来估计超参数的最佳值,以优化模型的性能。
多层感知器(MLP)是一种常用的神经网络模型,由多个隐藏层组成,每个层包含多个神经元。
MLP可以用于分类、回归等多种任务。
当使用贝叶斯超参数优化来调整MLP的超参数时,通常会选择一些常见的超参数,如学习率、批量大小、迭代次数等。
贝叶斯优化器会根据这些超参数的性能,选择下一个可能的最佳值。
它通过在每个步骤中随机选择少量的超参数组合,而不是搜索每个可能的组合,来提高效率。
在实践中,贝叶斯超参数优化通常使用一种称为高斯过程回归(Gaussian Process Regression)的方法,该方法可以估计每个超参数的可能值以及它们的概率分布。
然后,根据这些信息选择下一个超参数的值,以最大化模型性能的预期改善。
使用贝叶斯超参数优化可以自动调整超参数,避免了手动调整的困难和耗时。
此外,它还可以帮助找到更好的超参数组合,从而提高模型的性能和准确性。
这对于机器学习任务的实验和开发非常重要,因为它可以帮助快速找到最佳的模型配置。
基于正交小波变换的稀疏权盲均衡算法
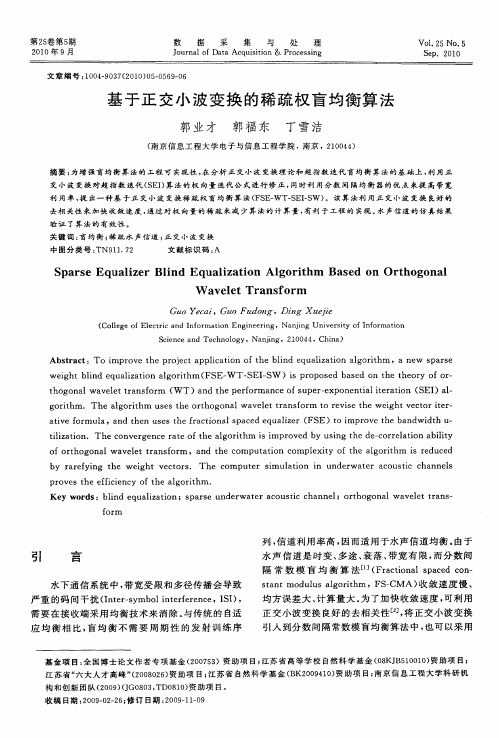
21 0 0年 9月
数
据
采
集
与
处
理
VO . 5 No. 12 5 S p 2 1 e. 00
J u n | fDa aAc usto o r a o t q iiin& Prc sig o esn
文 章 编 号 :0 49 3 ( 0 0 0 —5 90 10 —0 7 2 1 )50 6— 6
验证 了算 法 的 有 效 性 。
关 键 词 : 均衡 ; 疏 水 声信 道 ; 交 小 波 变 换 盲 稀 正
中图分类号 : TN9 1 7 1. 2 文献标 识码 : A
S a s u lz r Blnd Eq a i a i n Al o i h s d o r h g na p r e Eq a i e i u lz to g r t m Ba e n O t o o l
S in ea d Te h oo y,Na j g,2 0 4 ,Chn ) ce c n c n lg ni n 104 ia
Ab ta t To i r v h r jc p l a in o h l d e u l ai n ag rt m ,a n w p r e sr c : mp o e t e p o e ta p i to ft eb i q ai t lo i c n z o h e s a s
we g t b i d e u l a i n a g rt m ( E— T— EIS ) i p o o e a e n t e t e r fo — i h l q a i t l o ih FS W n z o S —W s r p s d b s d o h h o y o r t o o a v l t r n f r ( T)a d t e p r o ma c f u e — x o e ta t r to ( EI l h g n l wa e e a s o m W t n h e f r n e o p r e p n n i l e a i n S )a — s i
人工神经网络与神经网络优化算法
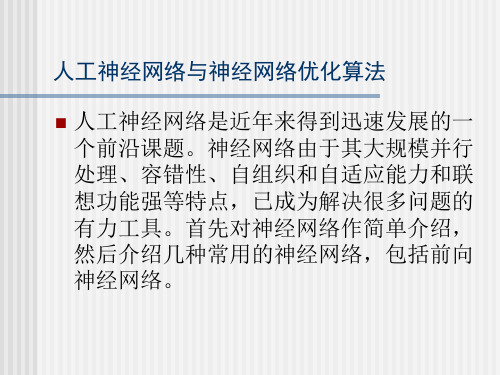
其中P为样本数,t j, p 为第p个样本的第j个输
出分量。
感知器网络
1、感知器模型 2、学习训练算法 3、学习算法的收敛性 4.例题
感知器神经元模型
感知器模型如图Fig2.2.1 I/O关系
n
y wipi bi
i 1
y {10
y0 y0
图2.2.1
单层感知器模型如图2.2.2
定义加权系数
10.1 人工神经网络与神经网络优化算法
③第 l 1层第 i个单元到第个单元的权值表为
; l1,l ij
④第 l 层(l >0)第 j 个(j >0)神经元的
输入定义为 , 输出定义 Nl1
x
l j
y l 1,l ij
l 1 i
为
yLeabharlann l jf (xlj )
, 其中 i0 f (•)为隐单元激励函数,
人工神经网络与神经网络优化算法
自20世纪80年代中期以来, 世界上许多国 家掀起了神经网络的研究热潮, 可以说神 经网络已成为国际上的一个研究热点。
1.构成
生物神经网
枝蔓(Dendrite)
胞体(Soma)
轴突(Axon) 胞体(Soma)
2.工作过程
突触(Synapse)
生物神经网
3.六个基本特征: 1)神经元及其联接; 2)神经元之间的联接强度决定信号传递的强
函数的饱和值为0和1。
4.S形函数
o
a+b
c=a+b/2
(0,c)
net
a
2.2.3 M-P模型
McCulloch—Pitts(M—P)模型, 也称为处理单元(PE)
x1 w1
- 1、下载文档前请自行甄别文档内容的完整性,平台不提供额外的编辑、内容补充、找答案等附加服务。
- 2、"仅部分预览"的文档,不可在线预览部分如存在完整性等问题,可反馈申请退款(可完整预览的文档不适用该条件!)。
- 3、如文档侵犯您的权益,请联系客服反馈,我们会尽快为您处理(人工客服工作时间:9:00-18:30)。
a r X i v :g r -q c /0110052v 1 9 O c t 2001Spatially Filtering the Binary Confusion Noise for Space Gravitational Wave DetectorsRonald W.HellingsMontana State UniversityBozeman MT 59715ABSTRACT:For the last fifteen years,the limiting noise source at the low frequency end of the sensitivity window for space gravitational wave detectors has been expected to be the confusion background of overlapping galactic binary stars.Here,we present results of a study that investigates the correlation between binary star signals and conclude that there is a spatial filter in the position-dependent Doppler shift of each binary that sharply reduces the contribution of the galactic binary confusion to the noise in the detector when a monochromatic source is being detected.The sensitivity is thus determined by the instrument alone,and the confusion noise may effectively be ignored.It has been nearly 15years since the first paper [1]was published recognizing the prob-lem that thousands of compact binaries would present in the detection of low frequency (LF)gravitational waves.Since that time,all discussions of the sensitivity of the detectors to grav-itational waves have had to take these binaries into account,concluding that at periods longer than about 300seconds the detectors will likely be limited not by the instruments themselves,but by the inability of the detectors to separate a weak gravitational wave of interest from the background of the gravitational waves from these other,less interesting sources.In this paper we show,for the case where the source of interest is itself a particular monochromatic binary,that there is an inherent spatial filter due to the phase modulation produced by the motion of the detector around the sun that sharply reduces the confusion noise,typically to a level below the instrument noise of the detector.Therefore,for monochromatic sources like these,the confusion noise may simply be ignored.We will consider the two different configurations of space gravitational detectors that have been proposed.These are the ecliptic plane configuration (like the proposed OMEGA [2]detector in which independent probes orbit the earth in the ecliptic plane)and the precessing plane case (like the proposed LISA [3]mission where the heliocentric orbits for the probes are chosen so that the plane of the detector is inclined 60◦to the ecliptic and precesses around the ecliptic pole once per year).A binary star of total mass M and reduced mass µ,with circular orbit at an angular frequency ω,lying in a direction given by angular coordinates Θand Φ(measured in the plane of the detector)and with the orbit plane inclined by i to the line-of-sight,will produce a signal in the detector given byh (t )=−√c 4µM 2/3ω2/3× (1+cos 2i )12(1+cos 2Θ)cos 2Φsin 2Ψ+cos Θsin 2Φcos 2Ψ sin(2φr ) ,(1)where φr is orbital phase at reception,given byφr =ωt −ωRindependent interferometers that are formed in each configuration may be combined in a time-dependent linear combination to simulate two non-rotating detectors,each of which is sensitive to a particular gravitational wave polarization.To simplify the correlation of signals from different directions,we will eliminate the signal modulation produced by the in-plane rotation of the detector and will consider a single non-rotating interferometer responding to a single polarization.The information content with this simplification is unchanged from that in the rotating case.For the ecliptic plane configuration,the relationship between the detector coordinates of the binary,Θand Φ,and its ecliptic coordinates,θand φ,is simplyΘ=θand Φ=φ+α,(3)where αis the angle between the detector centerline and the zero of ecliptic longitude at the vernal equinox.For the precessing plane case the situation is more complicated.The relationship between {Θ,Φ}and {θ,φ}iscos Θ=133cos θ+sin θcos β3sin βcos ψ+(√√3cos θcos β+sin θ)cos ψ(4c )for the precessing plane case.The key to the spatial filter we are analyzing is Eq.(2),the position-dependent phase modulation of each binary’s signal by an amount Rω/c at frequency Ω.Although the grav-itational wave from a binary star is monochromatic with a fixed polarization,the motion of the detector and,in the precessing plan case,the rotation of the detector plane produce a complicated signal in the detector.A simple Fourier power spectrum,therefore,will not detect individual sources.To detect a binary,one must disentangle the complicated spectrum by estimating all of the relevant parameters.This parameter estimation process is equivalent to a cross-correlation of the signal with a set of parameter-dependent templates,looking for the template that produces significant correlation.In what follows,we present the results of numerical simulations of the correlation of a signal from a particular direction with a background of signals from many directions.First,to demonstrate the effect,we consider a single source in the ecliptic at φ=0,with inclination i =90◦and polarization angle ψ=0,and correlate this signal,first with itself and then with other sources with identical parameters but at varying ecliptic longitudes.We use Eqs.(1),(2),and (3)or (4)to generate the signals.The computer code used to generate these signals was derived from that used in Moore and Hellings [4].To illustrate thedependence of the spatialfiltering on frequency,we calculate the correlations for sources and backgrounds at frequencies from10−4Hz to10−2Hz.Results of these simulations are shown in Figs.2a and2b.In Figs.2,the comparison sources all had the same inclination and polarization as the reference source atφ=0.This should produce the greatest correlated signal for comparison sources that lie in nearly the same direction as the reference source.However,as sources are found further away on the celestial sphere,different inclinations and polarizations may actually correlate more strongly.Thus,we would expect a set of comparison sources with random directions,inclinations,polarizations,and phases to have correlations that lie inside the envelope of the Fig.2curves when the sources are close to the reference source,but that often lie outside it when the sources are further away.Figs.3a and3b illustrate this.For thesefigures,a single f=3×10−3Hz reference source was chosen in the ecliptic plane atφ=0,and250comparison sources,each at the same frequency and intrinsic amplitude but with other parameters randomly chosen to provide even distribution over the sky,were correlated with the reference source.The simple sum of all the correlations in each case is given in eachfigure.Thus,for example,the correlation of the sum of all250sources with the single source in the ecliptic plane case(Fig.2a)is only2.23times the amplitude of the√single source itself.Since the amplitude of the250combined sources is∼250=0.141lower than the confusion noise.Although these numbers are exact only for the particular example displayed in thefigures,they are typical of the∼75 random cases that were run.WE have found that the two configurations(ecliptic-plane and precessing-plane)have nearly the same average correlations over the whole sky.The precessing-plane case is fairly uniform over the sky,while the ecliptic plane case is somewhat worse near the ecliptic plane (the2.23in Fig.3a compared with1.30in Fig.3b)and somewhat better for a source near the pole(See Fig.4).Finally,we have simulated the actual data analysis process.We have generated a signal made up of the combined signals from all the sources used to produce Fig.3and have added to it a single source,stronger than the individual sources that made up the background but weaker than the sum of the250background sources.That total signal was then correlated with a template representing the source and the correlation with the correct template was compared with that from other templates.We have chosen the precessing plane configuration for the simulation.Seen in the detector,the rms amplitude of the confusion background was 7.6×10−22,while that of the source was5.5×10−22.The spectral content of the confusion background and of the source are shown in Fig.5,where the complicated spectrum in the moving detector of what is inherently a pure monochromatic signal at3×10−3Hz is evident.The data recovery simulation then proceeded as follows.Three sources were taken,all in the ecliptic plane,one atφ=0,one atφ=60◦,and one atφ=90◦.These were added separately to the confusion background to produce three signals.Each of the three signals were then cross-correlated with three templates,each template appropriate to one of the sources.Results are shown in Fig.6.In Fig.6a,for example,the signal contained the source atφ=0.It was then correlated with templates forφ=0,φ=60◦,andφ=90◦.The strong correlation with the proper template compared with the correlation with the other templates is seen clearly in thefigure.Similar plots are shown for the other two sources in Figs.5b and5c.We reiterate that the particular sources in each case actually have amplitudes lowerthan the background,but have correlated amplitudes well above it.We conclude with a few remarks about the choices we have made for this study.First, in generating Figs.3−6,we have used sources with frequency3×10−3Hz.This is the presumed upper edge of the frequencies where the confusion background spectrum dominates the instrument noise spectrum.If the presumptions about the white dwarf binary background are correct,then the spatialfilter does not enhance sensitivity above this frequency.However, other assumptions about the white dwarf binary background[5,6]would produce a confusion noise at frequencies well above this.In this case confusion noise would dominate over much more of the sensitivity window,and the spatialfilter becomes even more important(and is more effective).Second,at frequencies below3×10−3Hz,the factor by which the spatial filter suppresses noise is reduced,the average factor of10at3×10−3Hz falling to an average factor of5at1×10−3Hz and dropping to about unity(i.e.no spatialfiltering)at1×10−4Hz. Thus,comparing the residual binary confusion noise to the estimated instrument noise for, say,LISA[3],we see that the confusion noise drops below the instrument noise across most of the band from10−4Hz to10−2Hz,the only exception being in a small range around10−3Hz where the residual binary noise does remain slightly above the instrument noise.Finally, our choice to use250sources,even at the higher frequencies where the number of sources would be much lower,was made in order to get the correct statistical results.That is,the probability of a single strong source lying in a particular direction is small,so the amplitude times the probability for each element of a statistical ensemble is equivalent to a weak source, and the ensemble average is well-modeled by adding many weak sources,as we have done in this study.Of course,one might be unlucky and the one or two competing noise sources might lie close in the sky to the source of interest,but an unbiased prediction of sensitivity should not give undue weight to this worst case.We reiterate that the results we have found in this study apply to detection of a single monochromatic binary in the presence of the presumed galactic binary confusion noise.In a future paper,we will consider the application of these ideas to the detection of a chirping and coalescing binary over the same background.The author would like to thank Tom Moore for discussions on modifying the previous code.This work was supported by NASA grant NCC5-579.References:[1]D.Hils,P.L.Bender,J.E.Faller,and R.F.Webbink,in11th Int.Conf.on General Rela-tivity and Gravitation:Abstracts of Contributed Papers,Vol.2(University of Stockholm, 1986),509.[2]R.W.Hellings et al.,Orbiting Medium Explorer for Gravitational Astrophysics(OMEGA),proposal to NASA Medium Explorer program(1998,unpublished).[3]P.Bender et al.,LISA Pre-Phase A Report(second edition)(1998).[4]T.A.Moore and R.W.Hellings,Phys.Rev.D,to be published(2001).[5]E.D.Webbink,Ap.J.277355-360(1984).[6]W.A.Hiscock et al.,Ap.J.Lett.540L5-L8(2000).CAPTIONS:Figure1.The geometry of the source position in the plane of the detector.The two legs forming a single Michelson interferometer are shown,the centerline of the detector forming the x-axis of the detector coordinate system.The binary orbit plane depicted here is at inclination i=0.Figure2.Correlation between a reference source atφ=0(with i=ψ=0,andθ=90◦)and other sources with varyingφ,but with all other parameters the same.Four curves are shown in eachfigure,one for each of the chosen frequencies(10−2Hz,3×10−3Hz,10−3Hz,and 10−4Hz).Figure2a is for the ecliptic plane configuration and Figure2b is for the precessing plane configuration.Figure3.Correlation between a reference source atφ=0(with f=3×10−3,i=ψ=0, andθ=90◦)and250other sources with the same frequency,but with random values for all other parameters.Figure3a is for the ecliptic plane configuration and Figure3b is for the precessing plane configuration.Figure4.Correlation,for the ecliptic plane case,between a reference source atθ=0(with f=3×10−3and i=ψ=0)and250other sources with the same frequency,but with random values for all other parameters.Figure5.Periodograms for:(a)the background of250random sources with f=3×10−3Hz and(b)a single source atφ=0,f=3×10−3Hz,i=ψ=0,andθ=90◦.The horizontal axis gives the number of frequency bins,each of width∆f=1/yr,offset from the fundamental f=3×10−3Hz.Figure6.The results of cross-correlation of templates with a signal composed of binary confusion noise plus a single source in the ecliptic plane at:(a)φ=0,(b)φ=60◦,or(c)φ=90◦.Three templates are used for each signal,representing sources at0,60◦,and90◦, labeled T00,T60,and T90,respectively.binary orbit planeΘdetector orbit planeΦx-axis。