Some generalized aggregating operators with linguistic information and their application
Some geometric aggregation operators based on intuitionistic fuzzy sets

高二英语区块链技术单选题50题

高二英语区块链技术单选题50题1. The ______ of blockchain technology has brought significant changes to the financial industry.A. introductionB. productionC. conclusionD. decision答案:A。
本题考查名词的词义辨析。
“introduction”意为“引入,引进”;“production”意为“生产”;“conclusion”意为“结论”;“decision”意为“决定”。
区块链技术是被引入到金融行业,带来了重大变化,所以选择A 选项。
2. The company is trying to ______ a new blockchain-based system to improve its business processes.A. developB. destroyC. deliverD. decline答案:A。
本题考查动词的词义辨析。
“develop”意为“开发,发展”;“destroy”意为“破坏”;“deliver”意为“交付,投递”;“decline”意为“下降,拒绝”。
公司是要开发新的基于区块链的系统来改进业务流程,所以选A 选项。
3. The blockchain technology is highly ______ and secure.A. efficientB. expensiveC. exhaustedD. extensive答案:A。
本题考查形容词的词义辨析。
“efficient”意为“高效的”;“expensive”意为“昂贵的”;“exhausted”意为“精疲力竭的”;“extensive”意为“广泛的”。
区块链技术是高效且安全的,所以选A 选项。
4. We need to ______ the advantages of blockchain technology to solve this problem.A. utilizeB. uniteC. updateD. upset答案:A。
GRE考试逻辑阅读部分翻译与解析(3)
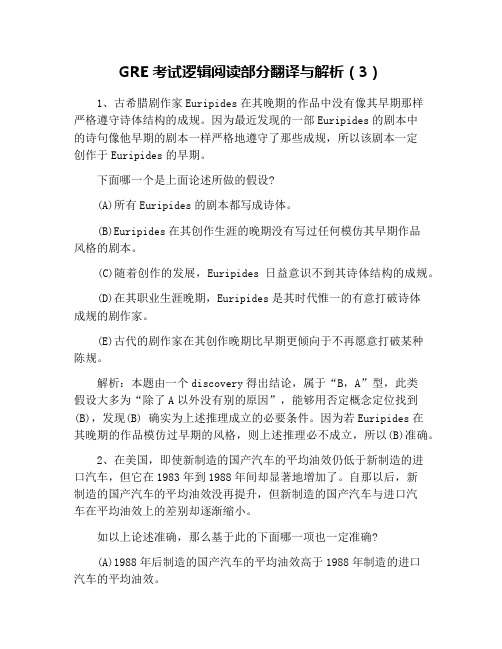
GRE考试逻辑阅读部分翻译与解析(3)1、古希腊剧作家Euripides在其晚期的作品中没有像其早期那样严格遵守诗体结构的成规。
因为最近发现的一部Euripides的剧本中的诗句像他早期的剧本一样严格地遵守了那些成规,所以该剧本一定创作于Euripides的早期。
下面哪一个是上面论述所做的假设?(A)所有Euripides的剧本都写成诗体。
(B)Euripides在其创作生涯的晚期没有写过任何模仿其早期作品风格的剧本。
(C)随着创作的发展,Euripides日益意识不到其诗体结构的成规。
(D)在其职业生涯晚期,Euripides是其时代惟一的有意打破诗体成规的剧作家。
(E)古代的剧作家在其创作晚期比早期更倾向于不再愿意打破某种陈规。
解析:本题由一个discovery得出结论,属于“B,A”型,此类假设大多为“除了A以外没有别的原因”,能够用否定概念定位找到(B),发现(B) 确实为上述推理成立的必要条件。
因为若Euripides在其晚期的作品模仿过早期的风格,则上述推理必不成立,所以(B)准确。
2、在美国,即使新制造的国产汽车的平均油效仍低于新制造的进口汽车,但它在1983年到1988年间却显著地增加了。
自那以后,新制造的国产汽车的平均油效没再提升,但新制造的国产汽车与进口汽车在平均油效上的差别却逐渐缩小。
如以上论述准确,那么基于此的下面哪一项也一定准确?(A)1988年后制造的国产汽车的平均油效高于1988年制造的进口汽车的平均油效。
(B)新制造的国产汽车的平均油效从1988年后逐渐趋向缩小。
(C)新制造的进口汽车的平均油效从1988年以后趋向缩小。
(D)新制造的进口汽车的平均油效在1983年后趋向增加。
(E)1983年制造的进口汽车的平均油效高于1988年制造的进口汽车的平均油效。
解析:本题属于数学相关类的归纳题,能够用简单的数学思维去判断。
通过读题,发现重点为“新的国产汽车自1988年后平均油效未提升,而与进口汽车的平均油效的差别却减小了”,所以能够得出结论,进口汽车在1988年后的平均油效减小了。
Lukasiewicz型直觉模糊推理三I方法的性质分析

Lukasiewicz型直觉模糊推理三I方法的性质分析李骏;刘岩【摘要】直觉模糊推理的两个基本模型是Intuitionistic Fuzzy Modus Ponens(IFMP)和Intuitionistic Fuzzy Modus Tollens(IFMT).首先利用经典模糊集之间的自然距离定义了直觉模糊集间的一种距离.其次,证明了基于Lukasiewicz 直觉模糊蕴涵的IFMP和IFMT问题的三I方法关于该距离都具有连续性,并且分别给出了IFMP和IFMT问题的三I方法满足逼近性的充分条件.%The two basic reasoning models of intuitionistic fuzzy reasoning are Intuitionistic Fuzzy Modus Ponens(IFMP) and Intuitionistic Fuzzy ModusTollens(IFMT)respectively.A kind of distance between intuitionistic fuzzy sets is intro-duced by the natural distance between classical fuzzy sets in the present paper.It is proven that both the triple I methods for solving IFMP and IFMT problems based on Lukasiewicz intuitionistic fuzzy implication are continuous with respect to this distance.Some sufficient conditions to guarantee the approximation property of the triple I methods for solving IFMP and IFMT are given respectively.【期刊名称】《计算机工程与应用》【年(卷),期】2018(054)008【总页数】5页(P44-47,54)【关键词】直觉模糊集;直觉模糊推理;三I方法;连续性;逼近性【作者】李骏;刘岩【作者单位】兰州理工大学理学院,兰州730050;兰州理工大学理学院,兰州730050【正文语种】中文【中图分类】TP181;O1591 引言模糊推理作为模糊控制的核心,在模糊信息的处理过程中起着举足轻重的作用。
Ch06练习答案
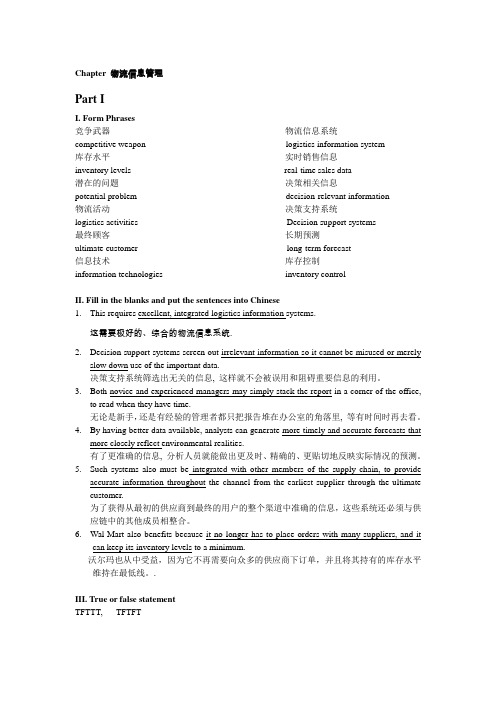
Chapter 物流信息管理Part II. Form Phrases竞争武器物流信息系统competitive weapon logistics information system库存水平实时销售信息inventory levels real-time sales data潜在的问题决策相关信息potential problem decision-relevant information物流活动决策支持系统logistics activities Decision support systems最终顾客长期预测ultimate customer long-term forecast信息技术库存控制information technologies inventory controlII. Fill in the blanks and put the sentences into Chinese1.This requires excellent, integrated logistics information systems.这需要极好的、综合的物流信息系统.2.Decision support systems screen out irrelevant information so it cannot be misused or merelyslow down use of the important data.决策支持系统筛选出无关的信息, 这样就不会被误用和阻碍重要信息的利用。
3.Both novice and experienced managers may simply stack the report in a corner of the office,to read when they have time.无论是新手,还是有经验的管理者都只把报告堆在办公室的角落里, 等有时间时再去看。
2015CMA中文考试-part2-真题-题库精选易错题题(wiley)

题目3:2D1-CQ02某公司正在构建风险分析体系,以量化数据中心面临的各种类型风险的威胁程度。
在调整保险赔偿金后,下列哪项将代表年度最高损失?发生的频率:1年损失金额:15000美元保险责任范围:85%。
发生的频率:100年损失金额:400000美元保险责任范围:50%。
发生的频率:8年损失金额:75000美元保险责任范围:80%。
发生的频率:20年损失金额:200000美元保险责任范围:80%。
预计的年度损失应该是12,750 [15,000×(0.85)]。
频率为8,预计的年度损失= (75,000/8)×(0.8) = 9,375×(0.8) = 7,500;频率为20,预计的年度损失=(200,000/20)×(0.8) = 100,000×(0.8) = 8,000;频率为100,预计的年度损失= (400,000/100)×(0.5) = 4,000×(0.5) = 2,000。
34.衍生金融工具的名义金额指的是:合同的初始购买价格。
标的资产的数量。
行使未到期合同的费用。
合同的固定价格。
衍生金融工具是交易双方之间的合同,单个付款(或多个付款)是在双方之间进行的。
合同的名义金额(或面值)可以是由一个特定的事件引发的预先确定的数量,也可以是标的资产价值数量的变化。
衍生金融工具的“名义金额”既指一定数量的货币金额,也可能指一定数量的股份,还可能指衍生金融工具合同所约定的一定数量的其他项目。
5.斯坦利公司的会计师利用以下的信息计算公司的加权平均资本成本(WACC)。
得出的公司加权平均资本成本是多少?17% 。
13.4% 。
10.36% 。
11.5% 。
加权平均资金成本计算如下:加权平均资本成本=长期债务的权重×长期债务的税后成本+普通股的权重×普通股的成本+留存收益的权重×留存收益的成本长期债务、普通股和留存收益的总额=10,000,000美元+ 10,000,000美元+ 5,000,000美元=25,000,000美元。
operators with intuitionistic fuzzy information and their application to group decision making
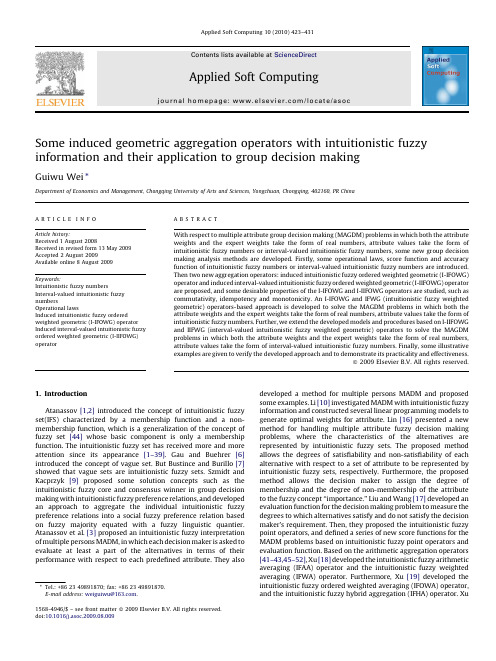
Some induced geometric aggregation operators with intuitionistic fuzzy information and their application to group decision makingGuiwu Wei*Department of Economics and Management,Chongqing University of Arts and Sciences,Yongchuan,Chongqing,402160,PR China1.IntroductionAtanassov[1,2]introduced the concept of intuitionistic fuzzy set(IFS)characterized by a membership function and a non-membership function,which is a generalization of the concept of fuzzy set[44]whose basic component is only a membership function.The intuitionistic fuzzy set has received more and more attention since its appearance[1–39].Gau and Buehrer[6] introduced the concept of vague set.But Bustince and Burillo[7] showed that vague sets are intuitionistic fuzzy sets.Szmidt and Kacprzyk[9]proposed some solution concepts such as the intuitionistic fuzzy core and consensus winner in group decision making with intuitionistic fuzzy preference relations,and developed an approach to aggregate the individual intuitionistic fuzzy preference relations into a social fuzzy preference relation based on fuzzy majority equated with a fuzzy linguistic quantier. Atanassov et al.[3]proposed an intuitionistic fuzzy interpretation of multiple persons MADM,in which each decision maker is asked to evaluate at least a part of the alternatives in terms of their performance with respect to each predefined attribute.They also developed a method for multiple persons MADM and proposed some examples.Li[10]investigated MADM with intuitionistic fuzzy information and constructed several linear programming models to generate optimal weights for attribute.Lin[16]presented a new method for handling multiple attribute fuzzy decision making problems,where the characteristics of the alternatives are represented by intuitionistic fuzzy sets.The proposed method allows the degrees of satisfiability and non-satisfiability of each alternative with respect to a set of attribute to be represented by intuitionistic fuzzy sets,respectively.Furthermore,the proposed method allows the decision maker to assign the degree of membership and the degree of non-membership of the attribute to the fuzzy concept‘‘importance.’’Liu and Wang[17]developed an evaluation function for the decision making problem to measure the degrees to which alternatives satisfy and do not satisfy the decision maker’s requirement.Then,they proposed the intuitionistic fuzzy point operators,and defined a series of new score functions for the MADM problems based on intuitionistic fuzzy point operators and evaluation function.Based on the arithmetic aggregation operators [41–43,45–52],Xu[18]developed the intuitionistic fuzzy arithmetic averaging(IFAA)operator and the intuitionistic fuzzy weighted averaging(IFWA)operator.Furthermore,Xu[19]developed the intuitionistic fuzzy ordered weighted averaging(IFOWA)operator, and the intuitionistic fuzzy hybrid aggregation(IFHA)operator.XuApplied Soft Computing10(2010)423–431A R T I C L E I N F OArticle history:Received1August2008Received in revised form13May2009 Accepted2August2009Available online8August2009Keywords:Intuitionistic fuzzy numbersInterval-valued intuitionistic fuzzy numbersOperational lawsInduced intuitionistic fuzzy ordered weighted geometric(I-IFOWG)operator Induced interval-valued intuitionistic fuzzy ordered weighted geometric(I-IIFOWG) operator A B S T R A C TWith respect to multiple attribute group decision making(MAGDM)problems in which both the attribute weights and the expert weights take the form of real numbers,attribute values take the form of intuitionistic fuzzy numbers or interval-valued intuitionistic fuzzy numbers,some new group decision making analysis methods are developed.Firstly,some operational laws,score function and accuracy function of intuitionistic fuzzy numbers or interval-valued intuitionistic fuzzy numbers are introduced. Then two new aggregation operators:induced intuitionistic fuzzy ordered weighted geometric(I-IFOWG) operator and induced interval-valued intuitionistic fuzzy ordered weighted geometric(I-IIFOWG)operator are proposed,and some desirable properties of the I-IFOWG and I-IIFOWG operators are studied,such as commutativity,idempotency and monotonicity.An I-IFOWG and IFWG(intuitionistic fuzzy weighted geometric)operators-based approach is developed to solve the MAGDM problems in which both the attribute weights and the expert weights take the form of real numbers,attribute values take the form of intuitionistic fuzzy numbers.Further,we extend the developed models and procedures based on I-IIFOWG and IIFWG(interval-valued intuitionistic fuzzy weighted geometric)operators to solve the MAGDM problems in which both the attribute weights and the expert weights take the form of real numbers, attribute values take the form of interval-valued intuitionistic fuzzy numbers.Finally,some illustrative examples are given to verify the developed approach and to demonstrate its practicality and effectiveness.ß2009Elsevier B.V.All rights reserved.*Tel.:+862349891870;fax:+862349891870. E-mail address:weiguiwu@.Contents lists available at ScienceDirect Applied Soft Computingj o ur n a l ho m e pa g e:w w w.e l s e vi e r.c om/l o ca t e/as o c1568-4946/$–see front matterß2009Elsevier B.V.All rights reserved.doi:10.1016/j.asoc.2009.08.009[20]developed some geometric aggregation operators,such as the intuitionistic fuzzy weighted geometric(IFWG)operator,the intuitionistic fuzzy ordered weighted geometric(IFOWG)operator, and the intuitionistic fuzzy hybrid geometric(IFHG)operator and gave an application of the IFHG operator to multiple attribute group decision making with intuitionistic fuzzy information.Xu[21] defined some new intuitionistic preference relations,such as the consistent intuitionistic preference relation,incomplete intuitio-nistic preference relation and acceptable intuitionistic preference relation,and studied their properties and also developed a method for group decision making with intuitionistic preference relations and a method for group decision making with incomplete intuitionistic preference relations,respectively.Xu[22]investigated the group decision making problems in which all the information provided by the decision makers is expressed as intuitionistic fuzzy decision matrices where each of the elements is characterized by intuitionistic fuzzy number,and the information about attribute weights is partially known,which may be constructed by various forms.Li[14]extended the linear programming techniques for multidimensional analysis of preference(LINMAP)to develop a new methodology for solving MADM problems in intuitionistic fuzzy environments.Xu[23]investigated the intuitionistic fuzzy MADM with the information about attribute weights is incompletely known or completely unknown,a method based on the ideal solution was ter,Atanassov and Gargov[4,5]further introduced the interval-valued intuitionistic fuzzy set(IVIFS),which is a general-ization of the IFS.The fundamental characteristic of the IVIFS is that the values of its membership function and non-membership function are intervals rather than exact numbers.Burillo[8]defined the concepts of correlation and correlation coefficient of IVIFSs,and developed two decomposition theorems of the correlation of IVIFSs in terms of the correlation of interval-valued fuzzy sets and the entropy of the intuitionistic fuzzy sets,and the correlation of intuitionistic fuzzy sets.Hong[31]generalized the concepts of correlation and correlation coefficient of IVIFSs in a general probability space.Hung and Wu[32]proposed a method to calculate the correlation coefficient of IVIFSs by means of‘‘centroid’’. Xu[24]proposed a new approach to deriving the correlation coefficients of IVIFSs.The prominent characteristic of the approach is that it can guarantee that the correlation coefficient of any two IVIFSs equals one if and only if these two IVIFSs are the same,and can relieve the influence of the unfair arguments on thefinal results. Grzegorzewski[33]proposed some distances between intuitionistic fuzzy sets and/or interval-valued fuzzy sets based on the Hausdorff metric.Wang[34]used evidential reasoning algorithms to solve MADM in which the information on the attribute’s weights is incomplete and attribute’s values is interval intuitionistic fuzzy numbers.Xu[25]proposed the interval-valued intuitionistic fuzzy weighted averaging(IIFWA)operator.Furthermore,Xu[25] developed the interval-valued intuitionistic fuzzy ordered weighted averaging(IIFOWA)operator and the interval-valued intuitionistic fuzzy hybrid aggregation(IIFHA)operator and gave an application of the IIFHA operator to multiple attribute group decision making with interval-valued intuitionistic fuzzy information.Xu[28]developed the interval-valued intuitionistic fuzzy weighted geometric(IIFWG) operator.Furthermore,Xu[26]developed the interval-valued intuitionistic fuzzy ordered weighted geometric(IIFOWG)operator and the interval-valued intuitionistic fuzzy hybrid geometric(IIFHG) operator.In this paper,we investigate the MAGDM problems,in which both the attribute weights and the expert weights take the form of real numbers,attribute values take the form of intuitionistic fuzzy numbers or interval-valued intuitionistic fuzzy numbers.Then,we propose two new aggregation operators called induced intuitio-nistic fuzzy ordered weighted geometric(I-IFOWG)operator and induced interval-valued intuitionistic fuzzy ordered weighted geometric(I-IIFOWG)operator which are an extension of induced ordered weighted geometric(IOWG)operator proposed by Xu and Da[27]and study some desirable properties of the I-IFOWG and I-IIFOWG operators,such as commutativity,idempotency and monotonicity.An I-IFOWG and IFWG(intuitionistic fuzzy weighted geometric)operators-based approach is developed to solve the MAGDM problems in which both the attribute weights and the expert weights take the form of real numbers,attribute values take the form of intuitionistic fuzzy numbers.Further,we extend the developed models and procedures based on I-IIFOWG and IIFWG(interval-valued intuitionistic fuzzy weighted geo-metric)operators to solve the MAGDM problems in which both the attribute weights and the expert weights take the form of real numbers,attribute values take the form of interval-valued intuitionistic fuzzy numbers.In order to do so,the remainder of this paper is set out as follows.In the next section,we introduce some basic concepts related to intuitionistic fuzzy sets and a new aggregation operator called induced intuitionistic fuzzy ordered weighted geometric (I-IFOWG)operator is proposed,and some desirable properties of the I-IFOWG operators are studied,such as commutativity, idempotency and monotonicity.In Section3,An I-IFOWG and IFWG(intuitionistic fuzzy weighted geometric)operators-based approach is developed to solve the MAGDM under the intuitio-nistic fuzzy environment.In Section4a new aggregation operator called induced interval-valued intuitionistic fuzzy ordered weighted geometric(I-IIFOWG)operator is proposed,and some desirable properties of the I-IIFOWG operators are studied,such as commutativity,idempotency and monotonicity.In Section5,An I-IIFOWG and IIFWG(interval-valued intuitionistic fuzzy weighted geometric)operators-based approach is developed to solve the MAGDM under the interval-valued intuitionistic fuzzy environ-ment.In Section6,some illustrative examples are pointed out.In Section7we conclude the paper and give some remarks.2.Induced intuitionistic fuzzy ordered weighted geometric (I-IFOWG)operatorIn the following,we shall introduce some basic concepts related to intuitionistic fuzzy sets.Definition1.Let X be a universe of discourse,then a fuzzy set is defined as:A¼x;m A xðÞh i x2Xjf g(1) which is characterized by a membership function m A:X!0;1½ , where m A xðÞdenotes the degree of membership of the element x to the set A[44].Atanassov[1,2]extended the fuzzy set to the IFS,shown as follows:Definition2.An IFS A in X is given byA¼x;m A xðÞ;n A xðÞh i x2Xjf g(2) where m A:X!0;1½ and n A:X!0;1½ ,with the condition0m A xðÞþn A xðÞ1;8x2XThe numbers m A xðÞand n A xðÞrepresent,respectively,the membership degree and non-membership degree of the element x to the set A.Definition3.For each IFS A in X,ifp A xðÞ¼1ÀmAxðÞÀn A xðÞ;8x2X(3) Then p A xðÞis called the degree of indeterminacy of x to A[1,2].G.Wei/Applied Soft Computing10(2010)423–431 424Definition 4.Let ˜a¼m ;n ðÞbe an intuitionistic fuzzy number,a score function S of an intuitionistic fuzzy value can be represented as follows [39]:S ˜aðÞ¼m Àn ;S ˜aðÞ2À1;1½ :(4)Definition 5.Let ˜a¼m ;n ðÞbe an intuitionistic fuzzy number,an accuracy function H of an intuitionistic fuzzy value can be repre-sented as follows [31]:H ˜aðÞ¼m þn ;H ˜aðÞ20;1½ (5)to evaluate the degree of accuracy of the intuitionistic fuzzy value˜a¼m ;n ðÞ,where H ˜a ðÞ20;1½ .The larger the value of H ˜a ðÞ,the more the degree of accuracy of the intuitionistic fuzzy value ˜a.As presented above,the score function S and the accuracyfunction H are,respectively,defined as the difference and the sum of the membership function ˜m A x ðÞand the non-member-ship function ˜nA x ðÞ.Hong and Choi [31]showed that the relation between the score function S and the accuracy function H is similar to the relation between mean and variance in statistics.Based on the score function S and the accuracy function H ,in the following,Xu [20]give an order relation between two intuitionistic fuzzy values,which is defined as follows:Definition 6.Let ˜a1¼m 1;n 1ðÞand ˜a 2¼m 2;n 2ðÞbe two intuitio-nistic fuzzy values,s ˜a1ðÞ¼m 1Àn 1and s ˜a 2ðÞ¼m 2Àn 2be the scores of ˜aand ˜b ,respectively,and let H ˜a 1ðÞ¼m 1þn 1and H ˜a 2ðÞ¼m 2þn 2be the accuracy degrees of ˜aand ˜b ,respectively,then if S ˜aðÞ<S ˜b,then ˜a is smaller than ˜b ,denoted by ˜a <˜b ;if S ˜aðÞ¼S ˜b ,then (1)if H ˜a ðÞ¼H ˜b ,then ˜a and ˜b represent the same information,denoted by ˜a¼˜b ;(2)if H ˜a ðÞ<H ˜b,˜a is smaller than ˜b,denoted by ˜a <˜b .Definition 7.Let ˜aj ¼m j ;n jj ¼1;2;ÁÁÁ;n ðÞbe a collection of intuitionistic fuzzy values,and let IFWG:Q n !Q ,ifIFWG v ˜a 1;˜a 2;ÁÁÁ;˜a n ðÞ¼Yn j ¼1˜a j v j ¼Y n j ¼1m j v j ;1ÀY n j ¼11Àn jÀÁv j;0@1A(6)wherev ¼v 1;v 2;ÁÁÁ;v n ðÞTbethe weight vector of˜aj j ¼1;2;ÁÁÁ;n ðÞ,and v j >0,Xn j ¼1v j ¼1,then IFWG is calledthe intuitionistic fuzzy weighted geometric (IFWG)operator[20].Example 1.Assume v ¼0:3;0:4;0:2;0:1ðÞ,˜a1¼0:3;0:5ðÞ,˜a 2¼0:2;0:6ðÞ,˜a 3¼0:7;0:2ðÞ,and ˜a 4¼0:4;0:3ðÞ,then IFWG v ˜a1;˜a 2;˜a 3;˜a 4ðÞ¼0:30:3Â0:20:4Â0:70:2Â0:40:1;1Àð1À0:5Þ0:3Âð1À0:6Þ0:4Âð1À0:2Þ0:2Âð1À0:3Þ0:1Þ¼0:311;0:480ðÞDefinition 8.Let ˜aj ¼m j ;n jj ¼1;2;ÁÁÁ;n ðÞbe a collection of intuitionistic fuzzy values,an intuitionistic fuzzy ordered weighted geometric (IFOWG)operator of dimension n is a mapping IFOWG:Q n !Q ,that has an associated weight vector w ¼w 1;w 2;ÁÁÁ;w n ðÞTsuch that w j >0and X n j ¼1w j ¼1.Furthermore,IFOWG w ˜a 1;˜a 2;ÁÁÁ;˜a n ðÞ¼Yn j ¼1˜as j ðÞw j ¼Y n j ¼1m s j ðÞw j ;1ÀY n j ¼11Àn s j ðÞÀÁwj 0@1A(7)where s 1ðÞ;s 2ðÞ;ÁÁÁ;s n ðÞðÞis a permutation of 1;2;ÁÁÁ;n ðÞ,suchthat ˜a s j À1ðÞ!˜a s j ðÞfor all j ¼2;ÁÁÁ;n .[20].Example 2.Let ˜a 1¼0:5;0:3ðÞ,˜a 2¼0:4;0:5ðÞ,˜a 3¼0:8;0:1ðÞ,and ˜a 4¼0:6;0:3ðÞbe four intuitionistic fuzzy values,by (4),we calcu-late the scores of ˜aj j ¼1;2;3;4ðÞ:S ˜a1ðÞ¼0:5À0:3¼0:2;S ˜a 2ðÞ¼0:4À0:5¼À0:1S ˜a 3ðÞ¼0:8À0:1¼0:7;S ˜a 4ðÞ¼0:6À0:3¼0:3SinceS ˜a 3ðÞ>S ˜a 4ðÞ>S ˜a 1ðÞ>S ˜a 2ðÞthus˜as 1ðÞ¼0:8;0:1ðÞ;˜a s 2ðÞ¼0:6;0:3ðÞ;˜as 3ðÞ¼0:5;0:3ðÞ;˜as 4ðÞ¼0:4;0:5ðÞSuppose that w ¼0:2;0:3;0:4;0:1ðÞis the weighting vector of the IFOWG operator.Then,by (7),it follows thatIn the following,we shall develop an induced intuitionisticfuzzy ordered weighted geometric (I-IFOWG)operator which is an extension of induced ordered weighted averaging (IOWG)operator proposed by Xu and Da [27].Definition 9.An induced intuitionistic fuzzy ordered weighted geometric (I-IFOWG)operator is defined as follows:where w ¼w 1;w 2;ÁÁÁ;w n ðÞT is a weighting vector,such thatw j >0,Xn j ¼1w j ¼1,j ¼1;2;ÁÁÁ;n ,˜as j ðÞ¼m s j ðÞ;n s j ðÞ is the ˜a i value of the IFOWG pair u i ;˜ai h i having the j th largest u i u i 20;1½ ðÞ,and u i in u i ;˜ai h i is referred to as the order IFOWG w ˜a1;˜a 2;˜a 3;˜a 4ðÞ¼0:80:2Â0:60:3Â0:50:4Â0:40:1;1Àð1À0:1Þ0:2Âð1À0:3Þ0:3Âð1À0:3Þ0:4Âð1À0:5Þ0:1¼0:567;0:288ðÞI-IFOWG w u 1;˜a 1h i ;u 2;˜a 2h i ;ÁÁÁ;u n ;˜a n h i ðÞ¼Yn j ¼1˜as j ðÞw j ¼Y n j ¼1m s j ðÞw j ;1ÀY n j ¼11Àn s j ðÞÀÁwj 0@1A(8)G.Wei /Applied Soft Computing 10(2010)423–431425inducing variable and ˜ai ˜a i ¼m i ;n i ðÞðÞas the intuitionistic fuzzy values.The I-IFOWG operator has the following properties similar to those of the IOWG operator [27].Theorem 1(Commutativity ).I-IFOWG w u 1;˜a1h i ;u 2;˜a 2h i ;ÁÁÁ;u n ;˜a n h i ðÞ¼I-IFOWG w u 1;˜a 01;u 2;h À˜a02i ;ÁÁÁ;u n ;˜a 0n Þwhere u 1;˜a 01 ;u 2;˜a 02 ;ÁÁÁ;u n ;˜a 0n ÀÁis any per-mutation of u 1;˜a 1h i ;u 2;˜a 2h i ;ÁÁÁ;u n ;˜a n h i ðÞ.Proof.LetI-IFOWG w u 1;˜a 1h i ;u 2;˜a 2h i ;ÁÁÁ;u n ;˜a n h i ðÞ¼Yn j ¼1˜a s j ðÞw j I-IFOWG wu 1;˜a01;u 2;˜a 02 ;ÁÁÁ;u n ;˜a 0n ÀÁ¼Y n j ¼1˜a0s j ðÞ w jSince u 1;˜a 01 ;u 2;˜a 02 ;ÁÁÁ;u n ;˜a 0n ÀÁis any permutation ofu 1;˜a1h i ;u 2;˜a 2h i ;ÁÁÁ;u n ;˜a n h i ðÞ,we have ˜a s j ðÞ¼˜a 0s j ðÞj ¼1;2;ÁÁÁ;ðn Þ,and thenI-IFOWG w u 1;˜a 1h i ;u 2;˜a 2h i ;ÁÁÁ;u n ;˜a n h i ðÞ¼I-IFOWG w u 1;˜a 01 ;u 2;˜a 02 ;ÁÁÁ;u n ;˜a 0n ÀÁ&Theorem 2(Idempotency ).If ˜aj ˜a j ¼m j ;n j¼˜a ˜a ¼m ;n ðÞðÞfor all j ,then I-IFOWG w u 1;˜a 1h i ;ðu 2;˜a2h i ;ÁÁÁ;u n ;˜a n h iÞ¼˜a Proof.Since ˜aj ¼˜a for all j ,we have I-IFOWG w u 1;˜a1h i ;u 2;˜a 2h i ;ÁÁÁ;u n ;˜a n h i ðÞ¼Yn j ¼1˜a w j ¼Y n j ¼1m w j ;1ÀY n j ¼11Àn ðÞw j 0@1A ¼mP nj ¼1w j ;1À1Àn ðÞP nj ¼1w j ¼m ;n ðÞ¼˜a &Theorem 3(Monotonicity ).If ˜aj ˜a 0j for all j ,then I-IFOWG w u 1;˜a 1h i ;u 2;˜a 2h i ;ÁÁÁ;u n ;˜a n h i ðÞ I-IFOWG w u 1;˜a 01 ;u 2;˜a 02 ;ÁÁÁ;u n ;˜a 0n ÀÁProof.LetI -IFOWG w u 1;˜a 1h i ;u 2;˜a 2h i ;ÁÁÁ;u n ;˜a n h i ðÞ¼Yn j ¼1˜as j ðÞw j I -IFOWG wu 1;˜a01 ;u 2;˜a 02 ;ÁÁÁ;u n ;˜a 0n ÀÁ¼Y n j ¼1˜a0s j ðÞ w jSince ˜aj ˜a 0j for allj ,itfollows that˜as j ðÞ ˜a 0s j ðÞj ¼1;2;ÁÁÁ;n ðÞ,thenI-IFOWG w u 1;˜a 1h i ;u 2;˜a 2h i ;ÁÁÁ;u n ;˜a n h i ðÞ I-IFOWG w u 1;˜a 01 ;u 2;˜a 02 ;ÁÁÁ;u n ;˜a 0n ÀÁ&Example 3.Assume we have four IFOWG pairs u j ;˜a jgiven u 1;˜a1h i ¼0:4;0:5;0:3ðÞh i ;u 2;˜a 2h i ¼0:2;0:4;0:5ðÞh i u 3;˜a3h i ¼0:8;0:6;0:2ðÞh i ;u 3;˜a 3h i ¼0:3;0:4;0:3ðÞh i That we desire to aggregate using the weighting vectorw ¼0:2;0:4;0:1;0:3ðÞ.Performing the ordering the IFOWG pairs with respect to the first component,we get u s 1ðÞ;˜as 1ðÞ¼0:8;0:6;0:2ðÞh i u s 2ðÞ;˜a s 2ðÞ ¼0:4;0:5;0:3ðÞh i u s 3ðÞ;˜a s 3ðÞ ¼0:3;0:4;0:3ðÞh i u s 4ðÞ;˜a s 4ðÞ ¼0:2;0:4;0:5ðÞh i This ordering includes the ordered intuitionistic fuzzy argu-ments˜as 1ðÞ¼0:6;0:2ðÞ;˜as 2ðÞ¼0:5;0:3ðÞ;˜as 3ðÞ¼0:4;0:3ðÞ;˜as 4ðÞ¼0:4;0:5ðÞAnd from this,we get an aggregated value3.An approach to group decision making based onintuitionistic fuzzy informationLet A ¼A 1;A 2;ÁÁÁ;A m f g be a discrete set of alternatives,and G ¼G 1;G 2;ÁÁÁ;G n f g be the set of attributes,v ¼v 1;v 2;ÁÁÁ;v n ðÞis the weighting vector of the attribute G j j ¼1;2;ÁÁÁ;n ðÞ,wherev j >0,Xn j ¼1v j ¼1.Let D ¼D 1;D 2;ÁÁÁ;D t f g be the set of decisionmakers,n ¼n 1;n 2;ÁÁÁ;n n ðÞbe the weighting vector of decisionmakers,with n k >0,X t k ¼1n k ¼1.Suppose that ˜R k ¼˜r k ðÞi j m Ân¼m k ðÞi j ;n k ðÞi jm Ânis the intuitionistic fuzzy decision matrix,wherem k ðÞi jindicates the degree that the alternative A i satisfies the attribute G j given by the decision maker D k ,n k ðÞi j indicates the degree that the alternative A i does not satisfy the attribute G j given by the decision maker D k ,m k ðÞi j &0;1½ ,n k ðÞi j &0;1½ ,m k ðÞi j þn k ðÞi j 1,i ¼1;2;ÁÁÁ;m ,j ¼1;2;ÁÁÁ;n ,k ¼1;2;ÁÁÁ;t .In the following,we apply the I-IFOWG and IFWG operator to multiple attribute group decision making based on intuitionistic fuzzy information.The method involves the following steps (Procedure &):Step 1.Utilize the decision information given in matrix ˜Rk ,and the I-IFOWG operator which has associated weighting vector w ¼w 1;w 2;ÁÁÁ;w n ðÞT˜ri j ¼m i j ;n i j ¼I-IFOWG w u 1;˜r 1ðÞi j D E ;u 2;˜r 2ðÞi j D E ;ÁÁÁ;u t ;˜r t ðÞi j D E;i ¼1;2;ÁÁÁ;m ;j ¼1;2;ÁÁÁ;n(9)to aggregate all the decision matrices ˜Rk k ¼1;2;ÁÁÁ;t ðÞinto a collective decision matrix ˜R¼˜r i j ÀÁm Ân ,where u ¼u 1;u 2;ÁÁÁ;f u t g be the weighting vector of decision makers.Step 2.Utilize the decision information given in matrix ˜R,and the IFWG operator˜ri ¼m i ;n i ðÞ¼IFWG v ˜r i 1;˜r i 2;ÁÁÁ;˜r in ðÞ;i ¼1;2;ÁÁÁ;m (10)I-IFOWG w 0:4;0:5;0:3ðÞh i ;0:2;0:4;0:5ðÞh i ;ð0:8;0:6;0:2ðÞh i ;0:3;0:4;0:3ðÞh iÞ¼0:60:2Â0:50:4Â0:40:1Â0:40:3;1Àð1À0:2Þ0:2Âð1À0:3Þ0:4Âð1À0:3Þ0:1Âð1À0:5Þ0:3¼0:474;0:350ðÞG.Wei /Applied Soft Computing 10(2010)423–431426to derive the collective overall preference values ˜ri i ¼1;ð2;ÁÁÁ;m Þof the alternative A i ,where v ¼v 1;v 2;ÁÁÁ;v n ðÞT is the weighting vector of the attributes.Step 3.Calculate the scores S ˜ri ðÞi ¼1;2;ÁÁÁ;m ðÞof the collective overall intuitionistic fuzzy preference values ˜ri i ¼1;2;ÁÁÁ;m ðÞto rank all the alternatives A i i ¼1;2;ÁÁÁ;ðm Þand then to select the best one(s)(if there is no difference between two scores S ˜ri ðÞand S ˜r j ÀÁ),then we need to calculate the accuracy degrees H ˜ri ðÞand H ˜r j ÀÁof the collective overall intuitionistic fuzzy preference values ˜ri and ˜r j ,respectively,and then rank the alternatives A i and A j in accordance with the accuracy degrees H ˜ri ðÞand H ˜r j ÀÁ.Step 4.Rank all the alternatives A i i ¼1;2;ÁÁÁ;m ðÞand select thebest one(s)in accordance with S ˜ri ðÞand H ˜r i ðÞi ¼1;2;ÁÁÁ;m ðÞ:Step 5.End.In what follows,we shall extend the developed models and procedures to solve the MAGDM problems in which both the attribute weights and the expert weights take the form of real numbers,attribute values take the form of interval-valued intuitionistic fuzzy numbers.4.Induced interval-valued intuitionistic fuzzy ordered weighted geometric (I-IIFOWG)operatorAtanassov and Gargov [4,5]further introduced the interval-valued intuitionistic fuzzy set (IVIFS),which is a generalization ofthe IFS.The fundamental characteristic of the IVIFS is that thevalues of its membership function and non-membership function are intervals rather than exact numbers.Definition 10.Let X be a universe of discourse,An IVIFS ˜Aover X is an object having the form [4,5]:˜A ¼x ;˜m A x ðÞ;˜nA x ðÞh i x 2X j f g (11)where ˜m A x ðÞ&0;1½ and ˜nA x ðÞ&0;1½ are interval numbers,and 0 sup ˜m A x ðÞðÞþsup ˜nA x ðÞðÞ 1;8x 2X For convenience,let ˜m A x ðÞ¼a ;b ½ ,˜n A x ðÞ¼½c ;d ,so ˜A ¼a ;b ½ ;ðc ;d ½ ÞDefinition 11.Let ˜a¼a ;b ½ ;c ;d ½ ðÞbe an interval-valued intuitio-nistic fuzzy number,a score function S of an interval-valued intuitionistic fuzzy value can be represented as follows [25,28]:S ˜aðÞ¼a Àc þb Àd;S ˜aðÞ2À1;1½ (12)Definition 12.Let ˜a¼a ;b ½ ;c ;d ½ ðÞbe an interval-valued intuitio-nistic fuzzy number,a accuracy function H of an interval-valued intuitionistic fuzzy value can be represented as follows [25,28]:H ˜aðÞ¼a þb þc þd2;H ˜aðÞ20;1½ (13)to evaluate the degree of accuracy of the interval-valuedintuitionistic fuzzy value ˜a¼a ;b ½ ;c ;d ½ ðÞ,where H ˜a ðÞ20;1½ .The larger the value of H ˜aðÞ,the more the degree of accuracy of the interval-valued intuitionistic fuzzy value ˜a.As presented above,the score function S and the accuracyfunction H are,respectively,defined as the difference and the sum of the membership function ˜m A x ðÞand the non-membership function ˜nA x ðÞ.Xu [25]showed that the relation between the score function S and the accuracy function H is similar to the relation between mean and variance in statistics.Based on the score function S and the accuracy function H ,in the following,Xu [25,28]give an order relation between two interval-valued intuitionistic fuzzy values,which is defined as follows:Definition 13.Let ˜a1¼a 1;b 1½ ;c 1;d 1½ ðÞand ˜a 2¼a 2;b 2½ ;c 2;d 2½ ðÞbe two interval-valued intuitionistic fuzzy values,s ˜a1ðÞ¼ða 1Àc 1þb 1Àd 1Þ=2and s ˜a2ðÞ¼ða 2Àc 2þb 2Àd 2Þ=2be the scores of ˜a and ˜b,respectively,and let H ˜a 1ðÞ¼ða 1þc 1þb 1þd 1Þ=2and H ˜a 2ðÞ¼ða 2þc 2þb 2þd 2Þ=2be the accuracy degrees of ˜aand ˜b ,respectively,then if S ˜a ðÞ<S ˜b ,then ˜a is smaller than ˜b ,denotedby ˜a<˜b ;if S ˜a ðÞ¼S ˜b ,then if (1)H ˜a ðÞ¼H ˜b ,then ˜a and ˜b represent the same information,denoted by ˜a ¼˜b ;(2)if H ˜aðÞ<H ˜b,˜a is smaller than ˜b ,denoted by ˜a <˜b .Xu [28]developed the interval-valued intuitionistic fuzzyweighted geometric (IIFWG)operator.Definition 14.Let ˜aj ¼a j ;b j ÂÃ;c j ;d j ÂÃÀÁj ¼1;2;ÁÁÁ;n ðÞbe a col-lection of interval-valued intuitionistic fuzzy values,and let IIFWG:Q n !Q ,ifwhere v ¼v 1;v 2;ÁÁÁ;v n ðÞT be the weight vector of˜a j j ¼1;2;ÁÁÁ;n ðÞ,and v j >0,P nj ¼1v j ¼1,then IIFWG is called the interval-valued intuitionistic fuzzy weighted geometric (IIFWG)operator.Example 4.Assume v ¼0:2;0:3;0:1;0:4ðÞ,˜a1¼0:3;0:5½ ;0:2;½ð0:3 Þ,˜a2¼0:4;0:7½ ;0:1;0:2½ ðÞ,˜a 3¼0:1;0:2½ ;0:7;0:8½ ðÞand ˜a 4¼0:5;0:7½ ;0:1;0:3½ ðÞ,then IIFWG v ˜a1;˜a 2;˜a 3;˜a 4ðÞ¼ð½0:30:2Â0:40:3Â0:10:1Â0:50:4;0:50:2Â0:70:3Â0:20:1Â0:70:4 ;½1Àð1À0:2Þ0:2Âð1À0:1Þ0:3Âð1À0:7Þ0:1Âð1À0:1Þ0:4 ;½1Àð1À0:3Þ0:2Âð1À0:2Þ0:3Âð1À0:8Þ0:1Âð1À0:3Þ0:4¼ ½0:3594;0:5774 ;½0:2124;0:3574Furthermore,Xu [26]developed the interval-valued intuitio-nistic fuzzy ordered weighted geometric (IIFOWG)operator.Definition 15.Let ˜aj ¼a j ;b j ÂÃ;c j ;d j ÂÃÀÁj ¼1;2;ÁÁÁ;n ðÞbe a col-lection of interval-valued intuitionistic fuzzy values.An interval-valued intuitionistic fuzzy ordered weighted geometric (IIFOWG)operator of dimension n is a mapping IIFOWG:Q n !Q ,that has an associated vector w ¼w 1;w 2;ÁÁÁ;w n ðÞT such that w j >0and P nj ¼1w j ¼1.Furthermore,IIFOWG w ˜a 1;˜a 2;ÁÁÁ;˜a n ðÞ¼Yn j ¼1˜a s j ðÞw j ¼Y n j ¼1a s j ðÞw j ;Y n j ¼1b s j ðÞwj 2435;1ÀY n j ¼11Àc s j ðÞÀÁw j ;1ÀY n j ¼11Àd s j ðÞÀÁw j 24350@1A(15)IIFWG v ˜a1;˜a 2;ÁÁÁ;˜a n ðÞ¼Y n j ¼1˜a j v j ¼Y n j ¼1a j v j ;Y n j ¼1b j vj 2435;1ÀY n j ¼11Àc jÀÁv j;1ÀY n j ¼11Àd jÀÁv j24350@1A(14)G.Wei /Applied Soft Computing 10(2010)423–431427。
2021IBM_L2考试真题模拟及答案(2)

2021IBM_L2考试真题模拟及答案(2)1、对于生产过程中引入的有机溶剂,下面哪种说法正确?()(多选题)A. 应在后续的生产环节给予有效去除B. 正文已明确列有残留溶剂检查的品种,必须对生产过程中引入的有机溶剂依法进行该项目检查C. 未在残留溶剂项下明确列出的有机溶剂,可以不检查D. 未在正文中列有此项检查的各品种,如生产过程中引入或产品中残留有机溶剂,均应按通则残留溶剂测定法检查并应符合相应溶剂的限度规定试题答案:A,B,D2、某客户每天要进行备份的应用多达25个,每个应用需要备份的数据量都不大。
之前采用物理磁带库进行数据备份,配置了2个LTO3的磁带驱动器。
据用户反映,目前存在备份窗口内无法完成备份任务的问题。
在这种情况下,推荐用户怎样解决当前问题?()(单选题)A. 将LTO3磁带驱动器替换为LTO4B. 再增加2个LTO4磁带驱动器C. 增加磁带槽位D. 推荐用户使用虚拟带库提高并行备份任务数试题答案:D3、工艺规程如需更改,应当按照()修订、审核、批准。
(单选题)A. 注册批件B. 相关的操作规程C. 质量要求D. 部门规定试题答案:B4、TS3100支持的最大槽位数为()?(单选题)A. 1个B. 9个C. 24个D. 48个试题答案:C5、文件应当()、条理分明,便于查阅。
(单选题)A. 分类存放B. 编号管理C. 分类发放D. 逐份存放试题答案:A6、在某些情况下,性能确认可与()结合进行。
(多选题)A. 运行确认B. 安装确认C. 工艺验证D. 设备确认试题答案:A,C7、为了避免筛网、冲具污染到生产物料,下列做法正确的是()。
(多选题)A. 不用筛网、冲具进行生产B. 挑选不易脱落的材质C. 应定期更换D. 有相应的保护措施试题答案:B,C,D8、一个客户有一个IO比较敏感,比较耗用缓存的应用,下面哪方面可能对性能影响比较明显?()(单选题)A. 写缓存B. 服务器中的高速缓存C. 磁盘驱动器机械臂D. 磁盘阵列中的高速缓存试题答案:C9、中药饮片生产企业可从下列哪些途径购入中药材?()(多选题)A. 供货商B. 农户C. 药材市场D. 农贸市场试题答案:A,B,C,D10、DS5300最多支持的主机登录数量是()。
2014年6月英语六级真题及答案
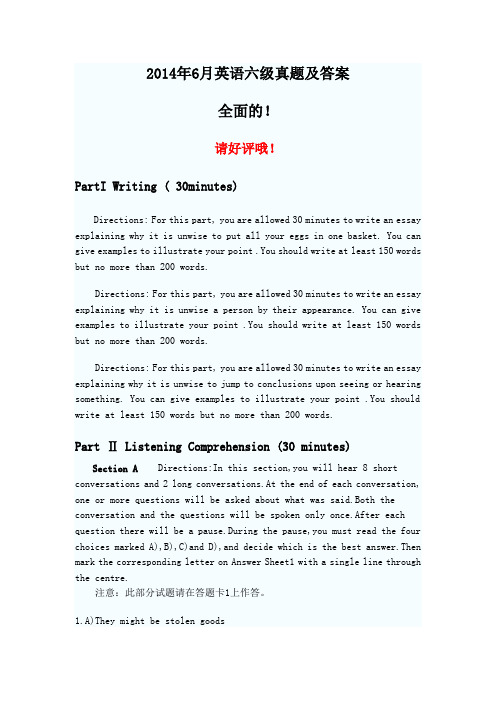
2014年6月英语六级真题及答案全面的!请好评哦!PartI Writing ( 30minutes)Directions: For this part, you are allowed 30 minutes to write an essay explaining why it is unwise to put all your eggs in one basket. You can give examples to illustrate your point .You should write at least 150 words but no more than 200 words.Directions: For this part, you are allowed 30 minutes to write an essay explaining why it is unwise a person by their appearance. You can give examples to illustrate your point .You should write at least 150 words but no more than 200 words.Directions: For this part, you are allowed 30 minutes to write an essay explaining why it is unwise to jump to conclusions upon seeing or hearing something. You can give examples to illustrate your point .You should write at least 150 words but no more than 200 words.Part Ⅱ Listening Comprehension (30 minutes)Section A Directions:In this section,you will hear 8 short conversations and 2 long conversations.At the end of each conversation, one or more questions will be asked about what was said.Both the conversation and the questions will be spoken only once.After each question there will be a pause.During the pause,you must read the four choices marked A),B),C)and D),and decide which is the best answer.Then mark the corresponding letter on Answer Sheet1 with a single line through the centre.注意:此部分试题请在答题卡1上作答。
Pruning in ordered bagging ensembles
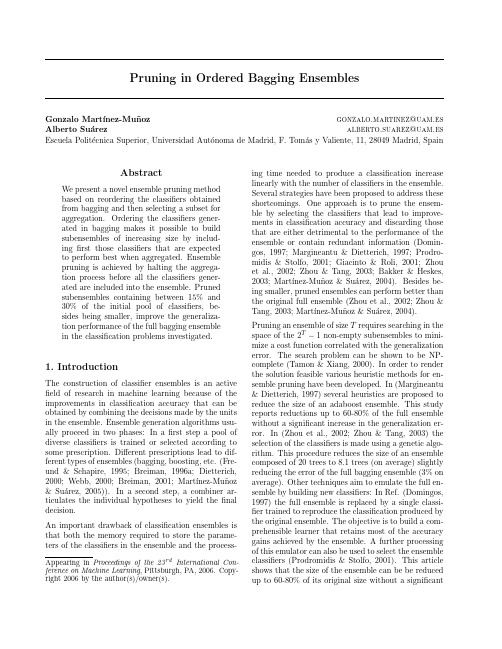
Pruning in Ordered Bagging EnsemblesGonzalo Mart´ınez-Mu˜n oz gonzalo.martinez@uam.es Alberto Su´a rez alberto.suarez@uam.es Escuela Polit´e cnica Superior,Universidad Aut´o noma de Madrid,F.Tom´a s y Valiente,11,28049Madrid,SpainAbstractWe present a novel ensemble pruning methodbased on reordering the classifiers obtainedfrom bagging and then selecting a subset foraggregation.Ordering the classifiers gener-ated in bagging makes it possible to buildsubensembles of increasing size by includ-ingfirst those classifiers that are expectedto perform best when aggregated.Ensemblepruning is achieved by halting the aggrega-tion process before all the classifiers gener-ated are included into the ensemble.Prunedsubensembles containing between15%and30%of the initial pool of classifiers,be-sides being smaller,improve the generaliza-tion performance of the full bagging ensemblein the classification problems investigated.1.IntroductionThe construction of classifier ensembles is an active field of research in machine learning because of the improvements in classification accuracy that can be obtained by combining the decisions made by the units in the ensemble.Ensemble generation algorithms usu-ally proceed in two phases:In afirst step a pool of diverse classifiers is trained or selected according to some prescription.Different prescriptions lead to dif-ferent types of ensembles(bagging,boosting,etc.(Fre-und&Schapire,1995;Breiman,1996a;Dietterich, 2000;Webb,2000;Breiman,2001;Mart´ınez-Mu˜n oz &Su´a rez,2005)).In a second step,a combiner ar-ticulates the individual hypotheses to yield thefinal decision.An important drawback of classification ensembles is that both the memory required to store the parame-ters of the classifiers in the ensemble and the process-Appearing in Proceedings of the23rd International Con-ference on Machine Learning,Pittsburgh,PA,2006.Copy-right2006by the author(s)/owner(s).ing time needed to produce a classification increase linearly with the number of classifiers in the ensemble. Several strategies have been proposed to address these shortcomings.One approach is to prune the ensem-ble by selecting the classifiers that lead to improve-ments in classification accuracy and discarding those that are either detrimental to the performance of the ensemble or contain redundant information(Domin-gos,1997;Margineantu&Dietterich,1997;Prodro-midis&Stolfo,2001;Giacinto&Roli,2001;Zhou et al.,2002;Zhou&Tang,2003;Bakker&Heskes, 2003;Mart´ınez-Mu˜n oz&Su´a rez,2004).Besides be-ing smaller,pruned ensembles can perform better than the original full ensemble(Zhou et al.,2002;Zhou& Tang,2003;Mart´ınez-Mu˜n oz&Su´a rez,2004). Pruning an ensemble of size T requires searching in the space of the2T−1non-empty subensembles to mini-mize a cost function correlated with the generalization error.The search problem can be shown to be NP-complete(Tamon&Xiang,2000).In order to render the solution feasible various heuristic methods for en-semble pruning have been developed.In(Margineantu &Dietterich,1997)several heuristics are proposed to reduce the size of an adaboost ensemble.This study reports reductions up to60-80%of the full ensemble without a significant increase in the generalization er-ror.In(Zhou et al.,2002;Zhou&Tang,2003)the selection of the classifiers is made using a genetic algo-rithm.This procedure reduces the size of an ensemble composed of20trees to8.1trees(on average)slightly reducing the error of the full bagging ensemble(3%on average).Other techniques aim to emulate the full en-semble by building new classifiers:In Ref.(Domingos, 1997)the full ensemble is replaced by a single classi-fier trained to reproduce the classification produced by the original ensemble.The objective is to build a com-prehensible learner that retains most of the accuracy gains achieved by the ensemble.A further processing of this emulator can also be used to select the ensemble classifiers(Prodromidis&Stolfo,2001).This article shows that the size of the ensemble can be be reduced up to60-80%of its original size without a significantdeterioration of the generalization performance of the pruned ensemble.Adopting a different strategy,one can apply clustering to the classifiers/regressors in the ensemble and select a single representative classifier for every cluster that has been identified(Giacinto& Roli,2001;Bakker&Heskes,2003).Our approach to ensemble pruning is to modify the original random aggregation ordering in the ensemble assuming that near-optimal subensembles of increas-ing size can be constructed incrementally by incor-porating at each step the classifier that is expected to produce the maximum reduction in the generaliza-tion error(Margineantu&Dietterich,1997;Mart´ınez-Mu˜n oz&Su´a rez,2004).After ordering,only a frac-tion of the inducers in the ordered ensemble is retained. The pruned ensemble obtained in this manner shows significant improvements in classification accuracy on test examples.In this work we propose a new criterion to guide the ordering of the units in the ensemble.The goal is to selectfirst those classifiers that bring the ensemble closer to an ideal classification performance.In or-der to accomplish this,each inducer is characterized by a signature vector whose dimension is equal to the size of the training set.The components of this vector are calculated in terms of the error made by the corre-sponding classifier on a particular labeled example(+1 if the example is correctly classified,-1if it is incor-rectly classified).The classifier is then incorporated into the ensemble according to the deviation of the orientation of the corresponding signature vector from a reference vector.This reference vector represents the direction toward which the signature vector of the ensemble(calculated as the average of the signature vectors of the ensemble elements)should be modified to achieve a perfect classification performance on the training set.In an ensemble of size T,the ordering op-eration can be performed with a quick-sort algorithm, which has an average running time of O(T log(T)).If we are only interested in the selection of theτ-best classifiers a quick-select algorithm can also be applied. Thus,the complexity of the ordering or of the selection operation is linear,in contrast to the quadratic time-complexity of the algorithms proposed in(Mart´ınez-Mu˜n oz&Su´a rez,2004),where the selection of each classifier involves an evaluation over all the remaining classifiers.The proposed ordering method also makes it possible to give a criterion for selecting a subset of classifiers to be considered for the inclusion in thefinal ensemble.This avoids the use of a pruning percentage that isfixed beforehand.The article is structured as follows:In Section2we introduce the ordering procedure in bagging ensem-bles.Section3presents the proposed criterion for or-dering.The results of experiments that illustrate the performance of the pruned ensembles on several UCI datasets(Blake&Merz,1998)are discussed in Sec-tion4.Finally,the conclusions of this research are presented.2.Ordering Bagging EnsemblesLet L=(x i,y i),i=1,2,...,N be a collection of N labeled instances.The training examples are charac-terized by a vector of attributes x i∈χand a discrete class label y i∈φ≡{1,2,...,C}.Consider a learning algorithm that constructs a classifier,h,from a given training set L.This classifier produces a classification y∈φof a new instance x∈χby a mapping h:χ→φ. In bagging(Breiman,1996a)a collection of classifiers is generated by training each inducer with a different dataset.These datasets are obtained by sampling with replacement from the original training set L with N extractions(bootstrap sampling).Thefinal classifica-tion is obtained by combining with equal weights the decisions of the individual classifiers in the ensemble. An instance x is thus classified according to the rule argmaxkTt=1I(h t(x)=k) :k=1,2,...,C,(1)where T is the number of classifiers and I is the indi-cator function such that I(T rue)=1,I(F alse)=0. The order in which classifiers are aggregated in bag-ging is irrelevant for the classification given by the full ensemble.The rationale for modifying the aggregation ordering in a bagging ensemble is to construct small subensem-bles with good classification performance.As stated in the introduction,the combinatorial problem of iden-tifying the optimal subensemble is NP-complete(Ta-mon&Xiang,2000)and becomes intractable for rela-tively small ensembles(T>30).Instead of solving the optimization problem exactly,we use an approximate procedure:Assume we have identified a subensemble composed ofτ−1classifiers,which is close to being optimal.A near-optimal ensemble of sizeτis built by selecting from the pool of remaining classifiers(of size T−(τ−1))the classifier that maximizes a quantity that is correlated with the generalization performance of the ensemble of sizeτ.We have performed exten-sive experiments using exhaustive search for small en-sembles(up to31classifiers)and global optimization tools,such as genetic algorithms,for larger ensembles, that show that this greedy search is efficient infinding near-optimal ensembles0.02 0.04 0.06 0.08 0.10.12 0.14 0.16 0 20 40 6080 100 120 140 160 180 200e r r o rnumber of classifierssegmentbagging orderedFigure 1.Average test and train error for the Segment dataset for bagging and ordered bagging according to the proposed heuristic.Figure 1shows the typical dependence of the classifica-tion error with the ensemble size in randomly ordered bagging (the standard version of bagging,where the order in which classifiers are aggregated is dictated by the bootstrap procedure)and in ordered bagging en-sembles.This figure displays the error curves for both the training and the test set (Segment dataset).Re-sults are averaged over 100executions of bagging with random ordering (solid line)and of ordered bagging (long trait line).The error for bagging ensembles with random ordering generally decreases monotonically as the number of classifiers included in the ensemble in-creases,approaching saturation at a constant error level for large ensembles.For the ordered ensembles both the test and training error curves reach a min-imum at an intermediate number of classifiers.The error rate at this minimum is lower than the error of the full ensemble.Note that the minimum of the error curve for the training set is achieved for smaller ensem-bles than in the test set.This means that the location of the minimum in the training error curve cannot be directly used to determine the optimal subensemble size.In this example the minimum in the training set error is achieved with 14classifiers,whereas the best results for the test set are obtained in ensembles that contain 44classifiers.It is in general difficult to give a reliable estimate of the optimal number of classifiers to get the best generalization accuracies.Nonetheless,Fig.1also shows that the minimum in the test error is fairly broad,and that,for a large range of sizes,the ordered subensembles have a generalization error that is under the final bagging error.This implies that it should be easy to identify pruned ensembles with im-proved classification accuracy.3.Orientation OrderingFor the ordering procedure to be useful the quantity that guides the selection of the classifiers should be a reliable indicator of the generalization performance of the ensemble.Measures based on individual properties of the classifiers (for instance,selecting first classifiers with a lower training error)are not well correlated with the classification performance of the subensem-ble.It is necessary to employ measures,such as diver-sity (Margineantu &Dietterich,1997),that contain in-formation of the complementariness of the classifiers.In this work,the quantity proposed measures how a given classifier maximizes the alignment of a signa-ture vector of the ensemble with a direction that cor-responds to perfect classification performance on the training set.Consider a dataset L tr composed of N tr examples.De-fine c t ,the signature vector of the classifier h t for the dataset L tr ,as the N tr -dimensional vector whose com-ponents arec ti =2I (h t (x i )=y i )−1,i =1,2,...,N tr ,(2)where c ti is equal to +1if h t (i.e.the t th unit in the ensemble)correctly classifies the i th example of L tr and −1otherwise.The average signature vector of the ensemble isc ens=1T Ttc t .(3)In a binary classification problem,the i th component of this ensemble signature vector is equal to the clas-sification margin for the i th example (the margin is defined as the difference between the number of votes for the correct class and the number of votes for the most common incorrect class,normalized to the inter-val [−1,1](Schapire et al.,1998)).In general multi-class classification problems,it is equal to 1−2edge (i )of the ensemble for the i th example (the edge is defined as the difference between the number of votes for the correct class and the number of votes for all incor-rect classes,normalized to the interval [0,1](Breiman,1997)).The i th example is correctly classified by the ensemble if the i th component of the average vector c ens is positive.That is,an ensemble whose average signature vector is in the first quadrant of the N tr -dimensional space will correctly classify all examples of the L tr dataset.This study presents an ordering criterion based on the orientation of the signature vector of the indi-vidual classifiers with respect to a reference direction.This direction,coded in a reference vector,c ref ,is0 50010001500200025003000-50 0 50 100150 200 250 300zxbagging ordered-20 020406080100-500 50 100150 200 250 300yxbagging ordered-5050 100150200250300-2020406080100500 1000 1500 2000 2500 3000bagging orderedxyzFigure 2.Projection of the unordered and ordered bagging signature vectors onto:two dimensions c ens (z axis)and c ref (x axis)(top plot ),two dimensions c ref and an axis perpendicular to c ref and c ens (y axis)(middle plot )and in the three dimensions previously defined (bottom plot ).Plots are for the Waveform problem.the projection of the first quadrant diagonal onto the hyper-plane defined by c ens .The classifiers are or-dered by increasing values of the angle between the signature vectors of the individual classifiers and the reference vector c ref .Finally,the fraction of the clas-sifiers whose angle is less than π/2(i.e.those withinthe quadrant defined by c ref and c ens )are included in the final ensemble.The reference vector,c ref ,is chosen to maximize the torque on c ens (which repre-sents the central tendency of the full ensemble)along the direction that corresponds to the ideal classifica-tion performance.This effect is obtained by choosing c ref =o +λc ens ,where o is a vector oriented along the diagonal of the first quadrant,and λis a constant such that c ref is perpendicular to c ens (c ref ⊥c ens ).As an example,consider a training set composed of three examples and an ensemble with c ens ={1,0.5,−0.5},meaning that the first example is cor-rectly classified by all the classifiers of the ensem-ble,the second by 75%of classifiers and the third by 25%of classifiers.Then the projection is calcu-lated considering that c ref =o +λc ens and c ref ⊥c ens ,which gives λ=−o ·c ens /|c ens |2.Hence,λ=−2/3and c ref ={1/3,2/3,4/3}.In the ordering phase,a stronger pull will be felt along the dimensions corre-sponding to examples that are harder to classify by the full ensemble (i.e.the third and second examples).However,c ref becomes unstable when the vectors that define the projection (i.e.c ens and the diagonal of the first quadrant)are close to each other.This makes the selection of c ref less reliable and renders the or-dering process less efficient.This is the case for en-sembles that quickly reach zero training error,such as boosting or bagging composed of unpruned trees,which do not show significant improvements in classi-fication performance when reordered according to the proposed heuristic.In Figure 2the learning processes in bagging and in or-dered bagging are depicted.A 200classifier ensemble is trained to solve the Waveform problem (Breiman et al.,1984)using 300data examples (i.e.the sig-nature vectors have 300dimensions).These plots show 2and 3-dimensional projections of the walks fol-lowed by the incremental sum of the signature vec-tors ( τt =1c t ;τ=1,2,...,T )in the randomly or-dered ensemble (solid line)and in the ordered ensem-ble (long trait line).In the top plot the ensemble vec-tors are projected onto the plane defined by c ens (z axis)and by c ref (x axis).The middle plot shows a 2-dimensional projection onto a plane perpendicular to c ens ,defined by c ref (x axis)and a vector perpen-dicular to both c ens and c ref (y axis).This plot is a projection into a plane that is perpendicular to the vector that defines the ensemble,c ens ;therefore any path including all classifiers starts and finishes at the origin.Finally,the bottom plot shows a 3-dimensional projection onto the previously defined x,y and z axis.For bagging (solid lines)it can be observed that the in-cremental sum of the signature vectors follows a paththat can be seen as a Brownian bridge starting at the origin and with afinal value of T×c ens.The ordering algorithm(long trait line)rearranges the steps of the original random path in such a way that thefirst steps are the ones that approximate the walker the most to c ref:Hence the characteristic form of the ordered path,which appears elongated toward the direction of c ref.These plots show the stochastic nature of the bagging learning process((Breiman,2001;Esposito& Saitta,2004))and how this process can be altered by re-ordering its classifiers.4.Experimental ResultsIn order to assess the performance of the ordering procedure described in the previous section,experi-ments are carried out in18classification problems from the UCI-repository(Blake&Merz,1998)and from Refs.(Breiman,1996b;Breiman et al.,1984).The datasets have been selected to test the performance of the pruning procedure on a wide variety of problems, including synthetic and real-world data from various applicationfields with different numbers of classes and attributes.Table1shows the characteristics of the sets investigated.For each dataset this table presents the number of examples used to train and test the en-sembles,the number of attributes and the number of classes.The subdivisions into training and testing are made using approximately2/3of the set for training and1/3for testing except for the Image Segmentation set,where the sizes specified in its documentation are used.For the synthetic sets(Waveform and Twonorm) different training and testing sets were generated in every execution of the algorithm.For each dataset100executions were carried out,each involving the following steps:(i)Generate a stratified random partition(independent sampling for the syn-thetic datasets)into training and testing sets whose sizes are given in Table1.(ii)Using bootstrap sam-pling,200CART decision trees are generated from the training set.The decision trees are pruned accord-ing to the CART10-fold cross validation procedure (Breiman et al.,1984).The ensemble generalization error is estimated in the unseen test set.This test error is calculated for a bagging ensemble that uses thefirst100classifiers generated and for a bagging en-semble containing all200trees.(iii)The trees in the ensemble are then ordered according to the rule de-scribed in Section3using the training subset.Finally, we calculate the average of the signature vector angles for vectors whose angle with respect to c ref is lower thanπ/2.Only classifiers whose signature vector an-gle is less than this average are included in the pruned Table1.Characteristics of the datasets used in the exper-iments.Dataset Train Test Attribs.Classes Audio140866924 Australian500190142 Breast W.50019992 Diabetes46830082 German600400202 Heart170100132 Horse-Colic244124212 Ionosphere234117342 Labor3720162 New-thyroid1407553 Segment2102100197 Sonar13870602 Tic-tac-toe60035892 Twonorm3005000202 Vehicle564282184 Vowel6003901011 Waveform3005000213 Wine10078133 subensemble.This rule gives a reasonable estimate of the number of classifiers needed for an optimal general-ization performance.In fact,any rule that selects the first20-30%of the classifiers in the ordered ensemble achieves a similar generalization accuracy.Figure3displays ensemble error curves for3of the classification problems considered.The behavior of the ensemble error curves is similar for the remaining problems.These plots show the dependence of the av-erage train(bottom curves in each plot)and test(top curves in each plot)errors on the number of classi-fiers included in the subensemble for randomly ordered bagging(solid line)and for orientation ordered bag-ging using100(dotted line)and200trees(trait line). As expected,in unordered bagging the error decreases monotonically as the number of classifiers in the en-semble grows and reaches a constant error rate asymp-totically.In contrast to this monotonic behavior,error curves in ordered bagging ensembles exhibit a typical shape where the error initially decreases with the num-ber of classifiers,reaches a minimum,and eventually rises and reaches the error of the full bagging ensem-ble.This characteristic shape is reproduced in both the train and test error curves and for ensembles of 100and200trees.It is important to note that this minimum is achieved for a smaller number of classifiers in the training set than in the test set.In the training set the minima appear generally for a fraction of clas-sifiers that is under10%of the initial pool.For the test curves the minima appear for subensemble whose0.05 0.1 0.15 0.2 0.25 0.3 0.35 0.4 0 20 40 6080 100 120 140 160 180 200e r r o rnumber of classifiersaudiobagging ordered 200ordered 1000.10.12 0.14 0.16 0.18 0.2 0.22 0.24 0.26 0.28 0.3 0.32 020406080 100 120 140160 180 200e r r o rnumber of classifiersgermanbagging ordered 200ordered 1000.050.1 0.15 0.20.250.30.350 20 40 6080 100 120 140 160 180 200e r r o rnumber of classifierswaveformbagging ordered 200ordered 100Figure 3.Train and test error curves for Bagging (solid line ),ordered bagging with 200trees (trait line )and 100trees (dotted line )for Audio ,German and Waveform clas-sification problems.size ranges between 20%-40%of the original classifiers.This fact makes it difficult to use directly the train-ing error curve minimum to estimate the number of classifiers that produce the best generalization error.Furthermore,this estimation becomes more difficult when considering each execution individually (instead of the smooth averaged curves)since the curves are more bumpy and do not always show clear minima.In any case,given that the minima are fairly flat,the range of valid pruning values that lead to a reduction the mean generalization error of bagging is broad.Table 2shows the results for the classification prob-lems investigated.The values reported are averages over 100executions.Note that the figures displayed in Table 2and the values of the test curves shown in figure 3do not always coincide,since the former is an average for different subensemble sizes,whereas the latter is an average for a fixed number of classifiers.The second column displays the test error when con-sidering the full ensemble of size 200and the third col-umn gives the test error for the ordered ensemble using the corresponding fraction of classifiers.The average number of classifiers used for calculating the general-ization accuracy of the ordered ensembles is shown in the fourth column.As a reference,we run the reduce-error (RE)pruning algorithm without back-fitting 1(Margineantu &Dietterich,1997),using the same en-sembles and with a near-optimal pruning rate of 80%(i.e.41classifiers of 200and 21of 100).This heuristic chooses at each ordering step the classifier that re-duces most the training error of the already selected subensemble.The test error of the reduce-error algo-rithm is shown in fifth column.The proposed method always reduces the average gen-eralization error for the studied datasets using a small subset of the classifiers of the full ensemble.This num-ber of classifiers in the pruned ensembles varies from 33of 200for the German dataset to 58of 200for Vowel .The improvements in classification accuracy of the presented method with respect to bagging are sta-tistically significant at a 99.99%confidence level (us-ing a paired two tailed Student’s t-test)in all problems investigated,with the exception of Australian and Di-abetes .For these sets the differences are significant for ensembles of 200trees but with a lower confi-dence level (95%).However,we should be cautious about the confidence levels in the real-world datasets since the statistical test may overestimate its signif-icance (Nadeau &Bengio,2003).For the synthetic datasets the confidence levels are perfectly valid as the experiements were carried out using independent sam-pling.In comparison with reduce-error pruning the proposed method obtains similar or slightly better re-sults.Its generalization error is lower in 11out of 18datasets,equal on 3and worse on 4.1We choose not to show the results with back-fitting because for this experiment configuration not using back-fitting is the most efficient selection.The generalization error with and without back-fitting are equivalent (within ±0.3%)and the execution time increases substantially when using back-fitting.Table2.Average test error in%.bagging(200trees)bagging(100trees)full ordered size re-41full ordered size re-21 Audio30.2±4.124.4±3.738.624.4±3.930.2±3.924.8±3.719.125.0±4.0 Australian14.5±2.114.1±2.238.013.7±2.314.5±2.114.3±2.218.914.0±2.3 Breast W. 4.7±1.5 4.1±1.340.9 4.1±1.3 4.7±1.5 4.2±1.320.2 4.1±1.3 Diabetes24.9±1.824.5±2.036.624.4±1.924.9±1.724.7±1.918.524.6±2.1 German26.6±1.625.4±1.732.925.1±1.726.6±1.725.6±1.716.825.5±1.7 Heart20.4±4.318.5±3.740.918.9±3.620.3±4.219.0±3.320.019.6±3.4 Horse-colic17.7±2.916.0±2.832.915.5±2.417.5±2.916.3±2.916.415.8±2.5 Ionosphere9.3±2.57.4±2.338.57.6±2.59.4±2.47.7±2.419.37.6±2.4 Labor14.4±7.810.0±6.745.712.3±7.614.6±7.710.0±6.723.012.1±7.6 New-Thyroid7.3±3.1 5.7±2.644.2 6.2±2.67.5±3.1 5.8±2.522.0 6.1±2.8 Segment9.7±1.77.8±1.141.78.0±1.19.8±1.78.0±1.121.18.2±1.1 Sonar24.7±4.720.7±5.147.121.5±4.824.6±4.721.7±4.623.221.9±4.7 Tic-tac-toe 2.7±1.1 2.0±0.848.8 2.3±1.0 2.7±1.1 2.3±0.924.5 2.6±1.1 Twonorm9.3±3.1 6.5±1.051.38.7±2.09.5±3.17.5±1.025.69.6±1.8 Vehicle29.6±2.226.5±2.141.026.5±1.929.5±2.226.9±2.020.726.9±2.0 Vowel13.7±2.212.1±2.058.313.6±2.114.0±2.212.8±2.129.214.1±2.2 Waveform22.8±2.519.6±1.242.020.0±1.323.0±2.420.3±1.220.720.6±1.3 Wine 6.5±4.0 4.8±2.944.7 5.8±3.5 6.6±4.2 5.1±2.922.4 6.2±3.6In a second batch of experiments we investigate how the number of classifiers in the original bagging ensem-ble affects the performance of the ordered ensembles. For these experiments the generated ensembles were re-evaluated using thefirst100trees of the randomly ordered bagging ensemble of size200.The ordering algorithm is then applied to this smaller pool of clas-sifiers.The average generalization error curve for the randomly ordered ensemble of size100and for the or-dered one are shown in Table2in the sixth and sev-enth columns,respectively.The number of classifiers in the pruned subensemble selected from the ordered ensembles of size100are shown in the eighth column. In the datasets investigated,a bagging ensemble with 100trees seems to be large enough to achieve the best possible classification performance of bagging.Slight improvements are observed for some sets(New-thyroid, Sonar,Waveform,...)when using the larger ensemble but also small error increases(Heart and Horse-colic). For ordered ensembles,the results reported in Table 2show that there are small but systematic improve-ments in classification accuracy for the larger ensem-bles,at the expense of using pruned ensembles with approximately twice as many classifiers.The curves plotted in Figure3,show that initially there is a steep parallel descent of the error curves for both ordered ensembles of size100and200and for both train and test curves up to a point that depends on the dataset. From this point onwards,the curves of the smaller en-sembles slow their descent until they reach a minimum. The error curves for the ordered ensemble of size200Table3.Average ordering time(s)for orientation ordering (OO)and reduce-error(RE)for different ensemble sizes. Size501002004008001600 OO0.0120.0250.0480.0890.1770.355 RE0.268 1.053 4.18116.6966.83268.5continue to decrease with a smaller negative slope and finally reach aflatter minimum.Finally,Table3shows the time needed for pruning using orientation ordering(OO)and reduce-error or-dering without back-fitting(RE)(Margineantu&Di-etterich,1997)for50,100,200,400,800and1600trees and for the Pima Indian Diabetes set.The values re-ported in this table are averages over10executions using a Pentium IV at3.2MHz.The results displayed in this table clearly show the approximately linear be-havior of the proposed method,in contrast to longer execution times and quadratic dependence on the size of the ensemble for the reduce-error pruning.5.ConclusionsThis article presents a novel method for pruning bag-ging ensembles that consistently reduces the general-ization error for the studied datasets by using a frac-tion of the classifiers of the complete bagging ensemble. The fraction of selected classifiers varies from15%to。
A Corpus-based Analysis for the Ordering of Clause Aggregation Operators
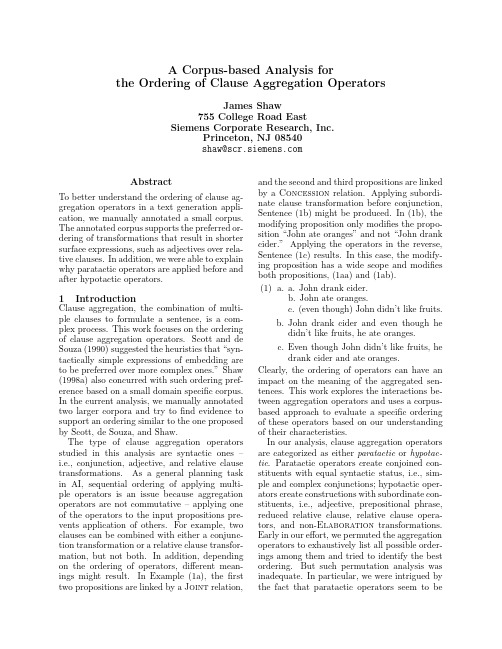
A Corpus-based Analysis forthe Ordering of Clause Aggregation OperatorsJames Shaw755College Road EastSiemens Corporate Research,Inc.Princeton,NJ08540shaw@AbstractTo better understand the ordering of clause ag-gregation operators in a text generation appli-cation,we manually annotated a small corpus. The annotated corpus supports the preferred or-dering of transformations that result in shorter surface expressions,such as adjectives over rela-tive clauses.In addition,we were able to explain why paratactic operators are applied before and after hypotactic operators.1IntroductionClause aggregation,the combination of multi-ple clauses to formulate a sentence,is a com-plex process.This work focuses on the ordering of clause aggregation operators.Scott and de Souza(1990)suggested the heuristics that“syn-tactically simple expressions of embedding are to be preferred over more complex ones.”Shaw (1998a)also concurred with such ordering pref-erence based on a small domain specific corpus. In the current analysis,we manually annotated two larger corpora and try tofind evidence to support an ordering similar to the one proposed by Scott,de Souza,and Shaw.The type of clause aggregation operators studied in this analysis are syntactic ones–i.e.,conjunction,adjective,and relative clause transformations.As a general planning task in AI,sequential ordering of applying multi-ple operators is an issue because aggregation operators are not commutative–applying one of the operators to the input propositions pre-vents application of others.For example,two clauses can be combined with either a conjunc-tion transformation or a relative clause transfor-mation,but not both.In addition,depending on the ordering of operators,different mean-ings might result.In Example(1a),thefirst two propositions are linked by a Joint relation,and the second and third propositions are linked by a Concession relation.Applying subordi-nate clause transformation before conjunction, Sentence(1b)might be produced.In(1b),the modifying proposition only modifies the propo-sition“John ate oranges”and not“John drank cider.”Applying the operators in the reverse, Sentence(1c)results.In this case,the modify-ing proposition has a wide scope and modifies both propositions,(1aa)and(1ab).(1) a.a.John drank cider.b.John ate oranges.c.(even though)John didn’t like fruits.b.John drank cider and even though hedidn’t like fruits,he ate oranges.c.Even though John didn’t like fruits,hedrank cider and ate oranges. Clearly,the ordering of operators can have an impact on the meaning of the aggregated sen-tences.This work explores the interactions be-tween aggregation operators and uses a corpus-based approach to evaluate a specific ordering of these operators based on our understanding of their characteristics.In our analysis,clause aggregation operators are categorized as either paratactic or hypotac-tic.Paratactic operators create conjoined con-stituents with equal syntactic status,i.e.,sim-ple and complex conjunctions;hypotactic oper-ators create constructions with subordinate con-stituents,i.e.,adjective,prepositional phrase, reduced relative clause,relative clause opera-tors,and non-Elaboration transformations. Early in our effort,we permuted the aggregation operators to exhaustively list all possible order-ings among them and tried to identify the best ordering.But such permutation analysis was inadequate.In particular,we were intrigued by the fact that paratactic operators seem to beapplied more frequently to input propositions than hypotactic ones,i.e.,paratactic,hypotac-tic,and then paratactic again.In theory,for most rhetorical relations,a rhetorical relation can be realizes by either a paratactic operator or a hypotactic operator, but not both.In practice,it is difficult for a content planner to specify rhetorical relations in such a way so that the sentence planner can sim-ply perform a one to one transformation.For example,since in general only constituents of the same syntactic type can be conjoined and a content planner lacks detailed syntactic infor-mation,a content planner cannot always cor-rectly specify Joint relations to all the modify-ing propositions which will be transformed into a conjoined constituent in thefinal sentence. In Section4,we will describe an example in which despite no Joint relation specified among the input propositions,the conjunctor“and,”a clear surface marker of Joint relation,appears in thefinal surface form.Section2describes the corpus-based method-ology used to evaluate the effectiveness of our proposed sequential ordering of the aggregation operators.Section3provides a brief description of related work.The markup language used for this annotation is described in Section4.Sec-tion5presents the result of the analysis and evaluates our proposed ordering based on the annotated corpus.Section6provides a ratio-nale for our ordering preference.2MethodologyAfter realizing that permutation analysis can-not be used tofind the optimal ordering of clause aggregation operators,we settled on a more modest goal–to show that our proposed ordering of clause aggregation operators works well in reconstructing human-written sentences. Given a specific aggregation operator ordering, evaluation was performed using a manually an-notated corpus to determine if applying the op-erators in the specific order will reconstruct the original sentences.The operator ordering used in the current evaluation is shown in Figure1. If such an ordering works well for the annotated corpus,researchers can be confident that NLG systems using such an ordering will work well. In our evaluation,a special corpus was used. To increase the chance of encountering sen-tences that underwent both paratactic and hy-1.Adjective(conjunction optional)2.Prepositional phrase(conjunction op-tional)3.Reduced relative clause,including appo-sition(conjunction optional)4.Relative clause(conjunction optional)5.Transformations for other rhetorical rela-tions(conjunction optional)6.Simple conjunctionplex conjunctionFigure1:Our proposed ordering of clause ag-gregation operators.and negative evidence will indicate how well the proposed ordering works.The current analysis focuses on the opera-tor ordering among different types of operators. When the same operator is applied multiple times,such as a sequence of adjective transfor-mations,the ordering decision among the same operator is outside the scope of the current an-notation effort.Malouf(2000)addressed such linearization issue using other corpus-base ap-proaches.Among different types of hypotactic operators,we assumed that the operators ap-plied earlier should be closer to the head than the constituent results from operators applied later.For example,if a reduced relative clause operator is applied before a relative clause op-erator,the reduced relative clause will appear closer to the head than the relative clause at the surface level.3Related WorkThe type of corpus annotation performed in this analysis is similar to discourse annotations such as RST analysis(Mann and Thompson,1988)or cohesion analysis(Halliday and Hasan,1976). Such effort has always been quite time con-suming and laborious.To facilitate discourse annotation effort,various graphical tools have been developed(O’Donnell,2000;Garside and Rayson,1997).Recently,eXtensible Markup Language(XML)has been gaining popularity as the meta-language for such annotation,i.e., LT XML tool and MATE workbench from the Edinburgh Language Technology Group.De-spite attempts to automate the process(Marcu, 2000),the type of annotation performed in this work must be done manually.In particular, the recovering of elided constituents during the de-aggregation process is difficult to automate. Similar to other works in clause aggregation (Scott and de Souza,1990;Moser and Moore, 1995;R¨o sner and Stede,1992),the current work uses rhetorical relations extensively.Our focus is on issues related to combining operations that transform linked clauses into sentences based on these rhetorical relations.4The AnnotationSection4.1describes the markup language used for annotation.Section4.2provides details on the concept of proposition set or propset,a use-ful device that facilitates our annotation effort.4.1The Markup LanguageThe de-aggregated sentences are annotated us-ing XML notation.Each sentence entry consists offive parts.Thefirst part is the original sen-tence.The second part is a list of de-aggregated propositions after manual reconstruction of the ellided constituents.These propositions are enclosed in a propset1,which might contain nested propsets.The third section specifies the rhetorical relations which linked the de-aggregated propositions or propsets to create cohesion.The number of rhetorical relations in a sentence entry is always one less than the number of propositions.The fourth section is a sequence of transformations that can be ap-plied to the de-aggregated propositions to re-construct the original sentence.Thefifth sec-tion contains annotator’s comments.One of them,seqordering tag,indicates whether the sequence of the transformations in the transfor-mation annotation section violates or adheres to the proposed aggregation operator ordering. The conj tag indicates whether a conjunctor “and”in the original sentence contains a col-lective or distributive reading.Following anno-tated sentence entry is an example taken from our corpus.<sentence id="s32">Local sports fans themselves,long knownfor their passive demeanor at games and propensity to leave early,don’t resistthe image.<propset id="pset32-1"><prop id="p32-1">Local sports fans don’t resist theimage.</prop><prop id="p32-2">Local sports fans are long known fortheir passive demeanor at games.</prop><prop id="p32-3">Local sports fans are long known fortheir propensity to leave early.</prop></propset><focus entity=’local sports fans’/><rst-rel id="r32-1"name="elab"nuc="p32-1"sat="p32-2"ref="no"/><rst-rel id="r32-2"name="elab"nuc="p32-1"sat="p32-3"ref="no"/><trans id="tx32-1"name="conj-simp"nuc="p32-2"sat="p32-3"/><trans id="tx32-2"name="rel-reduced-del-wh-be"nuc="p32-1"sat="tx32-1"/><seqorder valid="true"/><conj id="c32-1"type="dist"/></sentence>In this example,the original sentence is bro-ken into three propositions,with propositions p32-2and p32-3modifying p32-1with Elabo-ration relations,r32-1and r32-2.Both propo-sition p32-2and p32-3can be transformed into reduced relative clauses modifying p32-1,“[who are]long known for their passive...”and“[who are]long known for their propensity...”Using the ordering specified in Figure1,reduced rel-ative clause operator is appliedfirst.Because p32-2and p32-3are syntactically similar and can be conjoined using conjunction,the optional conjunction operator is activated before the re-duced relative clause operator is applied.After thefirst simple conjunction transformation,the intermediate results can be expressed as the fol-lowing:<prop id="p32-1">Local sports fans don’t resist theimage.</prop><prop id="tx32-1">Local sports fans are long known fortheir passive demeanor at games andpropensity to leave early.</prop>with<prop id=”tx32-1”>contains the result of applying simple conjunction transformation to p32-2and p32-3.The combined result <prop id=”tx32-1”>undergoes further trans-formation in<trans id=”tx32-2”>as a satel-lite proposition to nucleus proposition<prop id=”p32-1”>.The reduced relative clause transformation deletes”who”and”be”,and the original sentence is reproduced:<prop id="tx32-2">Local sports fans,long known for their passive demeanor at games and propensity to leave early,don’t resist the image.</prop>As explained earlier in Section1,because of lack of detailed syntactic information,it is difficult for content planners to specify Joint relations to all the modifying propositions which might be combined and appear as a conjoined con-stituent in thefinal sentence.Instead of per-forming such task in content planners,in our system,the sentence planner opportunistically uses the conjunctor,“and,”to combine these two syntactically similar modifying propositions in thefinal surface form.4.2The Proposition Set ConceptIn our preliminary effort to annotate the se-lected sentences with rhetorical relations,we re-alized that simply specifying rhetorical relations among the de-aggregated propositions did not seem to provide sufficient information to repro-duce the original sentence.For example,the propositions in Sentence(1a)in Section1can be realized as either Sentence(2a)or(2b)de-pending on whether a hypotactic operator or a conjunction operator is appliedfirst.(2) a.John drank cider and even though hedidn’t like fruits,he ate oranges.b.Even though John didn’t like fruits,hedrank cider and ate oranges.In Sentence(2a),the third proposition(1ac) only modifies second proposition(1ab),not thefirst(1aa).The Joint relation between the event(1aa)and(1ab)describes merely events and one of them is in conflict with the fact“John didn’t like fruits.”While in Sen-tence(2b),the proposition(1ac)has a wide scope and modifying both propositions(1aa) and(1ab).To clarify the scope of such mod-ifying construction,we came up with the con-cept of proposition set,or propset which facili-tates the specification of the scope of modifying proposition,as shown below.<sentence id="s1"><propset id="pset1-1"><prop id="p1-1">John drank cider.</prop><propset id="pset1-2"><prop id="p1-2">John ate oranges.</prop><prop id="p1-3">(even though)John didn’t likefruits.</prop></propset></propset><focus entity=’John’/><rst-rel id="r1-1"name="joint"nuc="p1-1"sat="pset1-2"ref="no"/><rst-rel id="r1-2"name="concession"nuc="p1-2"sat="p1-3"ref="no"/></sentence>The annotation for sentence s1specified a nar-row scope for the modifying proposition(p1-3), as in Sentence(2a).In rhetorical relation,r1-2,the second and third propositions are linked by a Concession relation.Together,they are linked to p1-1through a Joint relation in r1-1.<sentence id="s2"><propset id="pset1-1"><propset id="pset1-2"><prop id="p1-1">John drank cider.</prop><prop id="p1-2">John ate oranges.</prop></propset><prop id="p1-3">(even though)John didn’t likefruits.</prop></propset><focus entity=’John’/><rst-rel id="r1-1"name="joint"nuc="p1-1"sat="p1-2"ref="no"/><rst-rel id="r1-2"name="concession"nuc="pset1-2"sat="p1-3"ref="no"/></sentence>In the second annotation for sentence s2,the modifying proposition,p1-3,has a wide scope. To specify that a modifying proposition modi-fies both p1-1and p1-2,propset is used to group the two propositions before they are jointly modified by p1-3.As a result of making the scope clear in the de-aggregated proposition, our system can present either Sentence(2a)or (2b)and ensures correct scopings of modifying propositions are conveyed.Incorporating the concept of propset into an-notation provided several benefits:•Specify certain propositions are more tightly related.Tightly related events are grouped together in a propset,such as events related to a patient’s smoking habit,“he was a smoker”and“he quit10years ago.”The system treats propositions in a propset as one proposition and will combine themfirst before aggregating the combined proposition with others.•Simplify the annotation process for cer-tain rmation contained in the embedded S-structure of verbs like “said”or“believe”can be extracted and an-alyzed as a propset.For example,“John be-lieved Tim invested in stock and real estate.”Without using propset,the subject and verb of the main clause would appear multiple times in the de-aggregated propositions;i.e.,“John believed Tim invested in stock”and“John be-lieved Tim invested in real estate.”By elimi-nating such recurrences of the same main sub-jects and verbs,aggregation analysis is sim-plified.The transformations which combine all the propositions in the propset of an em-bedded S-structure are annotated as“ARG”transformations.They are just cosmetic arti-facts and unlikely to have any impact on the analysis of the ordering of the operators.•Minimize scope ambiguity.The earlier examples,(2a)and(2b),illustrate this pointwell.By using propset,the scope of the mod-ifying proposition can be made explicit.•Minimize redundant specification ofmultiple modifying rhetorical relations. When a proposition modifies multiple proposi-tions at the same time,the propositions being modified can be grouped under a propset so that only one rhetorical relation need be spec-ified between the modifying proposition and the propset being modified.If in such case, multiple rhetorical relations are specified be-tween each modifying proposition and propo-sitions being modified,the number of rhetori-cal relations could be greater than the number of propositions.Since transformation opera-tors are directly related to rhetorical relations, extra or redundant specifications of rhetorical relations would introduce complications to the implementation of the aggregation operators. The elimination of specifying multiple rhetorical relations for a single proposition is particularly important because it makes one transformation operator corresponds to a single rhetorical rela-tion.This simplification makes the aggregation task more manageable.5The ResultsThe200-sentence corpus was de-aggregated into 763clauses,about3.8clauses per sentence.Af-ter specifying the transformation operators for the sentences according to our proposed sequen-tial ordering,the majority of the sentences can be resynthesized from the de-aggregated propo-sitions using our ordering of aggregation op-erators(195out of200).The percentage is quite high because the incorporation of propset in the annotation takes care of many cases which would have violated our proposed order-ing.This result provides evidence supporting our claim that the proposed ordering shown in Figure1is effective.Excluding the40“ARG”relations in our analysis,there are20different types of rhetori-cal relations identified in the corpus,with a to-tal of523rhetorical relations.The interesting one for our analysis are Elaboration,Joint, and Sequence.Together,these three rhetor-ical relations made up of440of523rhetori-cal relations.Except for a few of them(e.g., joint-collective,alternative,and comparative), the other rhetorical relations are hypotactic in nature.The full annotated corpus is available through the Web(/˜shaw/col02). In the annotated corpus,excluding“ARG”transformations which are not involved in the ordering of aggregation operators,there are523 transformations used,roughly2.6transforma-tions for each sentence.Of the523transforma-tions,417(80%)of them are transformations re-lated to Joint,Elaboration,and Sequence, while106(20%)of them are not implemented at all.The transformations which were not imple-mented in our system include“or”,parenthesis, using“with”for paratactic operation,or any transformation which involves extraction.In the analysis,we did not remove sentences con-taining transformations which our system does not handle because doing so would eliminate many complex sentences appropriate for our analysis.Instead,unhandled transformations are categorized as either hypotactic and parat-actic,and they are mapped to the closest type of transformations during evaluation.Since the sentences selected for analysis are not random because they all contain the word“and,”this bias might create a tendency to select sentences with transformations our system handles well, such as paratactic transformation(62%contains conjunctor“and”).Given that our goal was to find as much interactions between hypotactic and paratactic operators as possible,this bias is reasonable.Of thefive unsupported cases,two of them involved application of relative clause transfor-mation before reduced relative clause.One such sentence is shown below:The patient was a38-year-old woman fromthe Dominican Republic[who presented tothe Cardiology Clinic in11/90][complainingof dyspnea on exertion and palpitations].In this example,the relative clause(“who pre-sented...”)is closer to its head,“woman,”than the reduced relative clause(“complain-ing of...”).Based on their surface ordering, the constituents in the original sentences indi-cated that a relative clause transformation is applied before the reduced relative clause trans-formation,and violates our ordering preference shown in Figure 1.The other unsupported cases involved realizing Joint relations using hypotactic constructions or realizing Elabo-ration relations using paratactic construction. Since these transformations are not the ex-pected transformation operators for the rhetor-ical relations,they violated our proposed order-ing.Overall,unsupported cases are rare.6Rationale for the OrderingBefore the current annotation effort was under-way,the ordering of operators used in our sys-tem was paratactic operators,hypotactic oper-ators,and then paratactic operators again.It was not clear why paratactic operators are ap-plied multiple times while hypotactic operators only once.It was not clear if there were different types of Joint relations connecting the proposi-tions which resulted in multiple applications of paratactic operators.After preliminary anno-tation of the corpus using propset,the answer became clear–thefirst application of parat-actic operators is a sub-step of the hypotac-tic operations which combine satellite proposi-tions with subordinate rhetorical relations(i.e., Elaboration)that have similar structures and modify the same entity in their nucleus proposi-tion.The ordering of clause aggregation opera-tors should be hypotactic operators followed by paratactic operators,but inside the hypotactic operators,paratactic operators are also applied to specific configurations of satellite proposi-tions as an optimization.We believe the ordering of hypotactic and paratactic operators might be related to the lo-cality of their operations.The operations to in-sert a modifying constituent into a sentence is a local operation because such insertion can be done without considering other constituents in the sentence which are not being modified.For example,attaching a prepositional phrase“with deep pocket”to the sentence“Bob Morgan is a reputable stock-broker who is interested in dot coms.”can be performed without considering how“with deep pocket”interacts with adjec-tives or the relative clause,or where the entity being modified,“stock-broker”,appears in the sentence.In contrast,paratactic operators are global in nature because they are very sensitive to constituents that are identical across all the propositions being combine.Due to directional constraint(Ross,1970;Shaw,1998b),the dele-tion of identical constituents cannot be made locally but must wait until the surface order-ing of the identical constituents is known.Incomparison,hypotactic operations have fewer constraints and should be applied earlier.The proposed sequence in Figure1applies all hypotactic operations before paratactic opera-tions.The ordering for intra-hypotactic opera-tors is chosen to produce the most concise sen-tence by applying operators producing short-est transformed constituentsfirst.Similarly, simple conjunction operator is applied before complex conjunction operator because the sim-ple conjunction operator produces more concise expressions.Other hypotactic transformations for non-Elaboration relation are treated sim-ilarly as relative clause transformations.Since there is little or no deletion result from such constructions(“Because John likes fruit,he ate oranges.”has no deletion),they are low on the priority and thus become the last one of the hy-potactic transformations.7ConclusionCurrent work made two signification observa-tions.First,in Section6we explained why some paratactic operators are applied before the hypotactic operators while others are ap-plied later.Secondly,propset was used for an-notating propositions during the de-aggregation process.The importance of rhetorical relations in clause aggregation operations were noted by many researchers(Scott and de Souza,1990; Moser and Moore,1995;R¨o sner and Stede, 1992),but the concept of propset was not men-tioned in previous literature related to rhetor-ical relations.It facilitates annotation during the de-aggregation process and allows annota-tor to ensure that both the number of transfor-mations and rhetorical relations is always one smaller than the number of propositions.This kept both the de-aggregation and aggregation process manageable.The goal of this work is tofind evidence to support the proposed ordering of the aggrega-tion operators for synthesizing grammatical and concise sentences.By imposing our proposed ordering onto de-aggregated propositions and trying to re-synthesize the original sentences, we determined the proposed ordering works well based on a human-written ing such ordering information and ensuring the content planner can specify propositions,propset,and rhetorical relations as the markup in the anno-tated corpus,the research community can incor-porate clause aggregation operations into nat-ural language generation systems and expect grammatical,concise sentences to be automati-cally generated.ReferencesRoger Garside and Paul Rayson.1997.Higher-level annotation tools.In R.Garside, G.Leech,and A.McEnery,editors,Cor-pus Annotation:Linguistic Information from Computer Text Corpora.Michael A.K.Halliday and R.Hasan.1976. Cohesion in English.Robert Malouf.2000.The order of prenominal adjectives in natural language generation.In Proc.of the38th ACL.William C.Mann and Sandra A.Thompson. 1988.Rhetorical structure theory:Toward a functional theory of text organization.Text, 8(3):243–281.Daniel Marcu.2000.The rhetorical parsing of unrestricted texts:A surface-based approach. Computational Linguistics,26(3):395–448. Megan Moser and Johanna D.Moore.1995.In-vestigating cue selection and placement in tu-torial discourse.In Proc.of the33rd ACL. Michael O’Donnell.2000.RSTTool 2.4–a markup tool for rhetorical structure theory. In Proc.of the1st INLG Conference. Dietmar R¨o sner and Manfred Stede.1992.Cus-tomizing RST for the automatic production of technical manuals.In R.Dale,E.Hovy, D.R¨o sner,and O.Stock,editors,Aspects of Automated Natural Language Generation. John Robert Ross.1970.Gapping and the order of constituents.In M.Bierwisch and K.Heidolph,editors,Progress in Linguistics. Donia R.Scott and Clarisse S.de Souza.1990. Getting the message across in RST-based text generation.In R.Dale,C.Mellish,and M.Zock,editors,Current Research in Natu-ral Language Generation.James Shaw.1998a.Clause aggregation us-ing linguistic knowledge.In Proc.of the9th INLG.James Shaw.1998b.Segregatory coordination and ellipsis in text generation.In Proc.of the 17th COLING and the36th ACL.。
Prioritized intuitionistic fuzzy aggregation operators
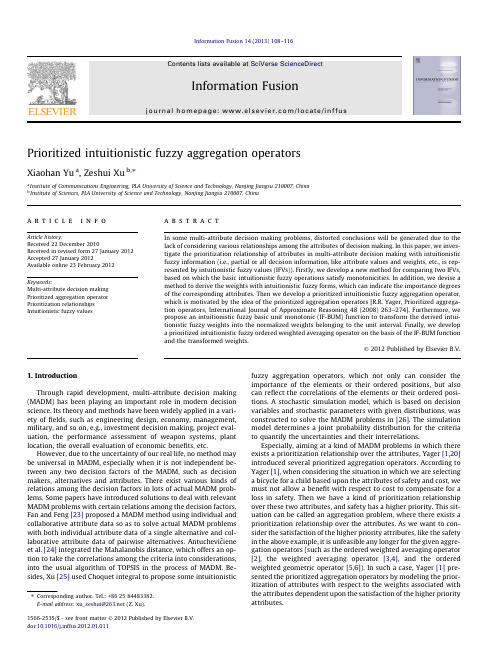
Prioritized intuitionistic fuzzy aggregation operatorsXiaohan Yu a ,Zeshui Xu b ,⇑a Institute of Communications Engineering,PLA University of Science and Technology,Nanjing Jiangsu 210007,China bInstitute of Sciences,PLA University of Science and Technology,Nanjing Jiangsu 210007,Chinaa r t i c l e i n f o Article history:Received 22December 2010Received in revised form 27January 2012Accepted 27January 2012Available online 23February 2012Keywords:Multi-attribute decision making Prioritized aggregation operator Prioritization relationships Intuitionistic fuzzy valuesa b s t r a c tIn some multi-attribute decision making problems,distorted conclusions will be generated due to the lack of considering various relationships among the attributes of decision making.In this paper,we inves-tigate the prioritization relationship of attributes in multi-attribute decision making with intuitionistic fuzzy information (i.e.,partial or all decision information,like attribute values and weights,etc.,is rep-resented by intuitionistic fuzzy values (IFVs)).Firstly,we develop a new method for comparing two IFVs,based on which the basic intuitionistic fuzzy operations satisfy monotonicities.In addition,we devise a method to derive the weights with intuitionistic fuzzy forms,which can indicate the importance degrees of the corresponding attributes.Then we develop a prioritized intuitionistic fuzzy aggregation operator,which is motivated by the idea of the prioritized aggregation operators [R.R.Yager,Prioritized aggrega-tion operators,International Journal of Approximate Reasoning 48(2008)263–274].Furthermore,we propose an intuitionistic fuzzy basic unit monotonic (IF-BUM)function to transform the derived intui-tionistic fuzzy weights into the normalized weights belonging to the unit interval.Finally,we develop a prioritized intuitionistic fuzzy ordered weighted averaging operator on the basis of the IF-BUM function and the transformed weights.Ó2012Published by Elsevier B.V.1.IntroductionThrough rapid development,multi-attribute decision making (MADM)has been playing an important role in modern decision science.Its theory and methods have been widely applied in a vari-ety of fields,such as engineering design,economy,management,military,and so on,e.g.,investment decision making,project eval-uation,the performance assessment of weapon systems,plant location,the overall evaluation of economic benefits,etc.However,due to the uncertainty of our real life,no method may be universal in MADM,especially when it is not independent be-tween any two decision factors of the MADM,such as decision makers,alternatives and attributes.There exist various kinds of relations among the decision factors in lots of actual MADM prob-lems.Some papers have introduced solutions to deal with relevant MADM problems with certain relations among the decision factors.Fan and Feng [23]proposed a MADM method using individual and collaborative attribute data so as to solve actual MADM problems with both individual attribute data of a single alternative and col-laborative attribute data of pairwise alternatives.Antuchevicˇiene et al.[24]integrated the Mahalanobis distance,which offers an op-tion to take the correlations among the criteria into considerations,into the usual algorithm of TOPSIS in the process of MADM.Be-sides,Xu [25]used Choquet integral to propose some intuitionistic fuzzy aggregation operators,which not only can consider the importance of the elements or their ordered positions,but also can reflect the correlations of the elements or their ordered posi-tions.A stochastic simulation model,which is based on decision variables and stochastic parameters with given distributions,was constructed to solve the MADM problems in [26].The simulation model determines a joint probability distribution for the criteria to quantify the uncertainties and their interrelations.Especially,aiming at a kind of MADM problems in which there exists a prioritization relationship over the attributes,Yager [1,20]introduced several prioritized aggregation operators.According to Yager [1],when considering the situation in which we are selecting a bicycle for a child based upon the attributes of safety and cost,we must not allow a benefit with respect to cost to compensate for a loss in safety.Then we have a kind of prioritization relationship over these two attributes,and safety has a higher priority.This sit-uation can be called an aggregation problem,where there exists a prioritization relationship over the attributes.As we want to con-sider the satisfaction of the higher priority attributes,like the safety in the above example,it is unfeasible any longer for the given aggre-gation operators (such as the ordered weighted averaging operator [2],the weighted averaging operator [3,4],and the ordered weighted geometric operator [5,6]).In such a case,Yager [1]pre-sented the prioritized aggregation operators by modeling the prior-itization of attributes with respect to the weights associated with the attributes dependent upon the satisfaction of the higher priority attributes.1566-2535/$-see front matter Ó2012Published by Elsevier B.V.doi:10.1016/j.inffus.2012.01.011Corresponding author.Tel.:+862584483382.E-mail address:xu_zeshui@ (Z.Xu).However,Yager[1]just discussed the attribute values and weights in real-valued environments.In practical applications the attribute values may be represented by fuzzy or uncertain argu-ments,like interval values[7,8],intuitionistic fuzzy values(IFVs) [9],and linguistic labels[10,11],because of the imprecision of assessment information which results from the decision maker’s level of knowledge,loose description to objects and subjective preferences.For example,when selecting a student to join a math contest from several candidates,we focus on the abilities of Mathe-matics and English expression,and the former is of course more important than the latter in a math contest.Meanwhile,the abilities of Mathematics and English expression,which can be represented by the IFVs(each of which is characterized by a membership degree and a non-membership degree),are judged from all of the test scores with respect to the course of Mathematics and English respectively.For a student,in terms of one course aforementioned, we calculate the membership degree and the non-membership degree of the IFVs according to the good scores and the bad scores respectively,and consider the rest as the hesitancy degree.If there is a student who is excellent both in Mathematics and English,it is no doubt that we will choose him/her,but this kind of student is not always existent.In the case,we usually choose a student who is excellent in Mathematics rather than one who is just good at English,because during the math contest the ability of Mathe-matics is more important,i.e.the ability of Mathematics,whose loss cannot be compensated by the benefit with respect to the ability of English expression,has a higher priority.The problem stated in the example is a MADM problem with a prioritization relationship over the attributes,which is represented by the IFVs rather than exact real numbers,and thus,it is necessary for us to develop some prior-itized aggregation operators for aggregating intuitionistic fuzzy information.In order to do that,in this paper,wefirst develop a new method for ranking IFVs based on which intuitionistic fuzzy operations satisfy monotonicities,and therefore an IFV can also indicate the importance degree of an attribute.In this case we devel-op a prioritized intuitionistic fuzzy aggregation operator by extend-ing the prioritized aggregation operators presented by Yager[1]. Furthermore,considering the intuitionistic fuzzy weights are not common,we propose an intuitionistic fuzzy basic unit monotonic (IF-BUM)function to transform the intuitionistic fuzzy weights into the normal weights belonging to the unit interval[0,1].Finally,we develop a prioritized intuitionistic fuzzy ordered weighted averag-ing operator by utilizing the IF-BUM function.2.PreliminariesThe concept of intuitionistic fuzzy set(IFS)was introduced by Atanassov[12,13],which can be defined as follows:An IFS A in X is an object having the following form:A¼f<x;l AðxÞ;t AðxÞ>j x2X gð1Þwhich is characterized by a membership function l A and a non-membership function v A,wherelA:X!½0;1 ;x2X!l AðxÞ2½0;1t A:X!½0;1 ;x2X!t AðxÞ2½0;1with the condition:lAðxÞþt AðxÞ1;for all x2XFor each IFS A in X,ifp AðxÞ¼1ÀlAðxÞÀt AðxÞ;for all x2Xð2Þthen p A(x)is called a hesitancy degree of x to A[12].Obviously, 06p A(x)61,for all x e X.For convenience,we call a=(l a,t a)an intuitionistic fuzzy value (IFV)[9],wherela2½0;1 ;t a2½0;1 ;l aþt a1ð3Þand we introduce some operational laws and aggregation operators for IFVs.Definition 2.1.[12,14,30].Let a¼ðl a;t aÞ;a1¼ðl a1;t a1Þand a2¼ðl a2;t a2Þbe the IFVs,then(1)a1^a2¼ðminðl a1;l a2Þ;maxðt a1;t a2ÞÞ;(2)a1_a2¼ðmaxðl a1;l a2Þ;minðt a1;t a2ÞÞ;(3)a1Èa2¼ðl a1þl a2Àl a1la2;t a1t a2Þ;(4)a1 a2¼ðl a1la2;t a1þt a2Àt a1t a2Þ;(5)k a¼ð1Àð1Àl aÞk;t k aÞ;k>0;(6)a k¼ðl k a;1Àð1Àt aÞkÞ;k>0.As we know,for real numbers,their operational laws,like addi-tion and multiplication operations,usually satisfy monotonicity, i.e.,c1+d1P c2+d2or c1Âd1P c2Âd2if c1P c2and d1P d2for c1;c2;d1;d12R(R denotes the set of all real numbers).Similarly, if a method for comparing two IFVs,which will be introduced in Section3,is adopted,then we usually expect that the operation results of the bigger IFVs will be larger than those of the smaller IFVs,which is called the monotonicity of the operational laws in this paper.For example,if a1P b1and a2P b2,then it should be tenable:(1)a1^a2P b1^b2;(2)a1_a2P b1_b2;(3) a1Èa2P b1Èb2;(4)a1 a2P b1 b2;(5)k a1!kb1;and(6) a k1!b k1,where‘‘P’’denotes‘‘no less than’’in the adopted method. Generally speaking,if the operational laws satisfy the monotonic-ity by using a method for comparing two IFVs,the method will be more practical and feasible.It is the reason why we shall design a new method for the comparison of a pair of IFVs in Section3.For convenience,suppose that a i(i=1,2,...,n)are IFVs,we then define_ni¼1a i¼a1_a2_..._a nand^ni¼1a i¼a1^a2^...^a nDefinition 2.2[14].Let X be the set of all IFVs,and a i¼ðl ai;t a iÞ(i=1,2,...,n)be n IFVs,and let IFWA:X n?X,if IFWA wða1;a2;...;a nÞ¼w1a1Èw2a2È...Èw n a nð4Þthen the function IFWA is called an intuitionistic fuzzy weighted averaging(IFWA)operator of dimension n,where w=(w1,w2,..., w n)T is the weight vector of a i,with w i e[0,1]andP ni¼1w i¼1.Definition2.3[15].Let(a1,a2,...,a n)be a collection of IFVs and let IFWC:X n?X,ifIFWC wða1;a2;...;a nÞ¼_ni¼1ðw i^a iÞð5Þthen the function IFWC is called an intuitionistic fuzzy weighted combination(IFWC)operator with dimension n,where w=(w1, w2,...,w n)T is the weight vector of the IFVs a i(i=1,2,...,n),and w i as well as a i(i=1,2,...,n)are IFVs.In this definition,we consider the weight w i as the importance degree corresponding to a i,and the larger w i is,the more important a i is.Differing from the IFWA operator,the weights of the IFWC operator are IFVs but not real numbers.Generally speaking,the former is of higher sensitivity than the latter,i.e.,a minor changeX.Yu,Z.Xu/Information Fusion14(2013)108–116109of an argument must influence the result of the IFWA operator,but the result of the IFWC may remain constant.Thus,the IFWA and IFWC operators should be used in different kinds of problems.Definition 2.4[14].Let X be the set of all IFVs and a i ¼ðl a i ;t a i Þ(i =1,2,...,n )be IFVs,and let IFOWA :X n ?X .IfIFOWA x ða 1;a 2;...;a n Þ¼x 1a ind ð1ÞÈx 2a ind ð2ÞÈÁÁÁÈx n a ind ðn Þð6Þthen the function IFOWA is called an intuitionistic fuzzy ordered weighted averaging (IFOWA)operator of dimension n ,where x =(x 1,x 2,...,x n )T is the weight vector,with x i e [0,1]and P ni ¼1x i ¼1,and ind (j )represents the index of the j th largest a i (i =1,2,...,n ).A basic unit interval and monotonic (BUM)function was intro-duced by Yager [21]:Definition 2.5[21].A BUM function is a mapping:f :[0,1]?[0,1]such that f (0)=0,f (1)=1and f (x )P f (y )if x >y .According to Yager [21],if there are n alternatives a 1,a 2,...,a n in a MADM problem,we can assign weights to them by using a BUM function:w i ¼f i Àf i À1 ;i ¼1;2;...;n3.A new method for the comparison between two IFVs With the development of intuitionistic fuzzy theory,a variety ofmethods for ranking IFVs have been proposed.In [16],Chen and Tan introduced the concept of the score function S (a )=l a Àt a for an IFV a =(l a ,t a ).The function S is used to measure the score of an IFV.It is clear that the score of a is directly related to the devi-ation between l a and t a ,i.e.,the higher the degree of deviation be-tween l a and t a ,the bigger the score of a ,and thus,the larger the IFV a .Later,Hong and Choi [17]defined an accuracy function H to evaluate the degree of accuracy of the IFV a =(l a ,t a )as H (a )=l a +t a .According to the score function and the accuracy function,Xu [14]gave a procedure for ranking IFVs,which can be defined as follows:Definition 3.1[14].Let a =(l a ,t a )and b =(l b ,t b )be two IFVs,S (a )=l a Àt a and S (b )=l b Àt b be the scores of a and b ,respec-tively,H (a )=l a +t a and H (b )=l b +t b be the accuracy degrees of a and b ,then(1)if S (a )<S (b ),then a is smaller than b ,denoted by a <b ;(2)if S (a )=S (b ),then(a)if H (a )=H (b ),then a and b represent the same informa-tion,i.e.,l a =l b and t a =t b ,denoted by a =b ;(b)if H (a )<H (b ),then a is smaller than b ,denoted by a <b .However,the main problem of the above two methods is not in accordant with the monotonicity of the intuitionistic fuzzy operational laws in Definition 2.1.For example,let three IFVs a 1=(0.4,0.1),a 2=(0.5,0.4)and a 3=(0.3,0.1),then a 2<a 3because S (a 2)=0.1<S (a 3)=0.2.But a 1_a 2=(0.5,0.1)>a 1_a 3=(0.4,0.1),which is not correct.Additionally,there is another method for ranking IFVs by using the intuitionistic fuzzy point operator [18],in which Liu and Wang introduced a new score function:J n ða Þ¼l a þrp a þr ð1Àr Àh Þp a þÁÁÁþr ð1Àr Àh Þn À1p a¼l a þrp a 1Àð1Àr Àh Þnr ;n ¼1;2;3...ð7ÞJ 1ða Þ¼l a þr r p að8Þwhere a =(l a ,t a )is an IFV whose hesitancy degree p a =1Àl a Àt a ,r ,h e [0,1]and r +h 61.In this way,the larger the value of J n (a ),the more priority should be given in ranking.In practical applications,the decision maker can choose the suitable parameters r and h according to the actual demands.However,if we assume r =h =1/2,thenJ n ða Þ¼l a þ12p a ¼12ð1þl a Àt a Þ¼12ð1þS ða ÞÞwhere S (a )is the score function defined above.In this case,themethod also does not accord with the monotonicity of the intuition-istic fuzzy operational laws.Differing from the above methods,there is a method satisfying the monotonicity.In [19],Deschrijver and Kerre showed that IFSs can also be seen as L -fuzzy sets in the sense of Goguen [22]and de-fined a complete lattice as a partially ordered set ðL Ã; L ÃÞ.A tradi-tional relation on the lattice L ⁄, L Ã,defined bya L Ãb ()l a l b and t a !t bð9Þfor two IFVs a and b .But as pointed out by Xu and Da [6],in some situations,(9)cannot be used to compare IFVs.For example,let a =(l a ,t a )=(0.2,0.4)and b =(l b ,t b )=(0.4,0.5)be two IFVs,where l a =0.2<l b =0.4and t a =0.4<t b =0.5.Then it is impossible to know which one is bigger by using (9).In the following,we improve the method in [19]to develop a new method for the comparison between two IFVs.As we know,when comparing two IFVs,an IFV which has the larger member-ship degree and the smaller non-membership degree should be prior.Thus,if there are two IFVs a =(l a ,t a )and b =(l b ,t b ),we have the following conclusions:(1)If l a P l b and t a <t b ,then a >b .(2)If l a <l b and t a P t b ,then a <b .(3)If l a =l b and t a =t b ,then a =b .However,if l a <l b and t a <t b ,we cannot determine the or-dered relation between the two IFVs a and b by the method above,but a is possibly smaller than b .In this case,we can give the fol-lowing definition:Definition 3.2.Let a =(l a ,t a )and b =(l b ,t b )be two IFVs,then (1)If l a =l b and t a =t b ,then a =b .(2)If l a P l b ,t a 6t b ,then a strongly dominates b ,denoted asa P 1b .(3)If l a P l b ,then a weakly dominates b ,denoted asa P db .According to Definition 3.2,if there exits strong dominance relation between two IFVs,then we can compare them certainly;otherwise,if there exits just weak dominance relation between them,it is vague and ambiguous that one IFV is larger than the other.Here,we define a parameter d e [0,1],called the domi-nance degree.For two IFVs a and b ,the dominance degree d can be considered as a probability that a is larger than b ,if a weakly dominates b ,then we denote the weak dominance rela-tion as P d .Specially,if d =1when comparing two IFVs a and b ,then we can certainly determine which one is larger,in other words,there exists the strong dominance relation between a and b ,thus the strong dominance relation is a special case of the weak dominance relation.110X.Yu,Z.Xu /Information Fusion 14(2013)108–116In what follows,we will verify the monotonicity of all opera-tions in Definition2.1based on the new method for ranking IFVs in Definition3.2:Theorem 3.1.Let a=(l a,t a),a0¼ðl a0;t a0Þ,b=(l b,t b)and b0¼ðl b0;t b0Þbe IFVs,and k>0.If a P d a0and b P d b0,then(1)a^b P d a0^b0;(2)a_b P d a0_b0;(3)aÈb P d a0Èb0;(4)a b P d a0 b0;(5)k a!d k a0;(6)a k!d a0k.Proof.Since a P d a0and b P d b0,then l a!l a0and l b!l b0. Also(1)a^b=(min(l a,l b),max(t a,t b))and a0^b0¼ðminðl a0;lb0Þ;maxðt a0;t b0ÞÞ,then minðl a;l bÞP minðl a0;l b0Þ,and thus,a^b P d a0^b0.(2)a_b=(max(l a,l b),min(t a,t b))and a0_b0¼ðmaxðl a0;lb0Þ;minðt a0;t b0ÞÞ,then maxðl a;l bÞP maxðl a0;l b0Þ,and thus,a_b P d a0_b0.(3)aÈb¼ðl aþl bÀl a l b;t a t bÞand a0Èb0¼ðl a0þl b0Àl a0lb0;t a0t b0Þ,then l aþl bÀl a l b¼1Àð1Àl aÞð1Àl bÞP 1Àð1Àl a0Þð1Àl b0Þ¼l a0þl b0Àl a0l b0and thus,aÈb P d a0Èb0.(4)a b=(l a l b,t a+t bÀt a t b)and a0 b0¼ðl a0l b0;t a0þtb0Àt a0t b0Þ,then l a l b P l a0l b0,and thus,a b P d a0 b0.(5)k a¼ð1Àð1Àl aÞk;t k aÞand k a¼ð1Àð1Àl a0Þk;t k a0Þ,then1Àð1Àl aÞk P1Àð1Àl a0Þk,and thus,k a P d k a0.(6)a k¼ðl k a;1Àð1Àt aÞkÞand a0k¼ðl k a0;1Àð1Àt a0ÞkÞ,thenl kaP l k a0,and thus,a k P d a0k.Similar to Theorem3.1,we haveTheorem 3.2.Let a=(l a,t a),a0¼ðl a0;t a0Þ,b¼ðl b;t bÞand b0¼ðl b0;t b0Þbe IFVs,and k>0.If a P1a0and b P1b0,then(1)a^b P1a0^b0;(2)a_b P1a0_b0;(3)aÈb P1a0Èb0;(4)a b P1a0 b0;(5)k a!1k a0;(6)a k!1a0k.In the following,we give a way to calculate the dominance degree:Defintion3.3.Let a=(l a,t a)and b=(l b,t b)be two IFVs,and let a P d b but a–b.(1)If l a>l b,then we can calculate the dominance degreed byd¼laÀl blalbt a t bð10Þ(2)If l a=l b,then(a)If t a<t b,then d=1;(b)If t a>t b,then d=0.For example,if there are two IFVs a=(0.4,0.6)and b=(0.2,0.5), then we know that a weakly dominates b,and according to Defini-tion3.3,we calculate the dominance degree d=2/3.In this case,we can denote a weakly dominates b as a P2/3b.Generally speaking, we have the following properties for the calculation formula of the dominance degree in(10):Theorem 3.3.Let a=(l a,t a)and b=(l b,t b)be two IFVs,and l a>l b,t a P t b,then(1)if l aÀl b isfixed,then the smaller t aÀt b,the larger thedominance degree d,and d=1if t aÀt b=0;(2)if t aÀt b isfixed,then the larger l aÀl b,the larger d,andd?0if l aÀl b?0;and(3)if l aÀt a=l bÀt b,then d=0.5;where d is the dominance degree of a over b calculated by(10),and ‘‘?’’denotes‘‘approach to’’.Considering IFVs cannot be ranked determinately in some situ-ations,in the above,we have developed a new method for ranking IFVs by introducing the concept of dominance degree.According to the method,we can compare two IFVs vaguely and ambiguously if the priority of the two IFVs cannot be determined absolutely.It is found that the main issues of the existing methods for ranking IFVs can be well overcome by using the new method.4.Prioritized intuitionistic fuzzy aggregation operatorIn this section,we shall introduce the prioritized aggregation operators developed by Yager[1],and then develop the prioritized intuitionistic fuzzy aggregation(PIFA)operators by extending the prioritized aggregation operators.At length,after transforming intuitionistic fuzzy weights into real-valued weights,we develop a prioritized intuitionistic fuzzy ordered weighted averaging operator.4.1.Prioritized aggregation operatorsSuppose that we have a collection of attributes partitioned into q distinct categories H1,H2,...,H q such that H i¼f a i1;a i2;...;a inig. Here a ij(j=1,2,...,n i)are the attributes in the category H i.We also assume a prioritization relationship among these categories:H1>H2>...>H qThe attributes in the category H i have a higher priority than those in H k if i<k.Then,the universal set of attributes is A¼S qi¼1H i.Assume that n¼P qi¼1n i is the total number of attri-butes.According to Yager[1],if the above assumptions hold in a MADM problem,then it is a MADM problem with prioritization relationships over the attributes.According to Yager[1],the weights can be associated with an attribute dependent upon the satisfaction of the higher priority attributes by modeling the prioritization between attributes.In this case,wefirst definei i¼1;i¼0/ða i1;a i2;...;a iniÞ;i¼1;2;...;qð11Þwhere/is an alternative function for calculating i i,such as the maximum or minimum function,the OWA aggregation function, and so on,which was mentioned in[1].Moreover,we can calculate the weights by means of i i:w i¼Y ik¼1ikÀ1;i¼1;2;...;qð12ÞLet a ij(x)be the attribute values of the alternative x with respect to the attribute a ij,and a(x)be the overall attribute value of the alternative x.Generally speaking,any attribute value of x belongs to[0,1]in this paper.However,in some practical problems,the attribute values are real numbers.In this case,we can alwaysX.Yu,Z.Xu/Information Fusion14(2013)108–116111devise a function mapping from R to [0,1]so as to transform the real attribute values into the values in [0,1].Then we have the fol-lowing definition:Definition 4.1[1].Let F :[0,1]n ?[0,1].For any alternative x ,ifa ðx Þ¼F w ða ij ðx ÞÞ¼Xi ;jw i a ij ðx Þ¼X q i ¼1w iX n ij ¼1a ij ðx Þ!ð13Þwhere w =(w 1,w 2,...,w q )T can be calculated by (12),then the func-tion F is called a prioritized scoring operator.If the weights w i (i =1,2,...,q )in (12)have been normalized,then we should call F in Definition 4.1a prioritized averaging oper-ator.However,as illustrated in [1],the prioritized averaging oper-ator does not always guarantee a monotonic aggregation.Only if the priority relationship between the attributes is a linear ordering,no ties allowed,we can obtain a prioritized averaging operator.Therefore,we use the prioritized scoring operator rather than the prioritized averaging operator in practical applications.Before introducing the prioritized ordered weighted averaging (POWA)operator,according to Yager [20],we assume a collection of attributes A ={a 1,a 2,...,a n }which are prioritized such that a i >a j if i <j .For any alternative x ,let i i =a i (x )(i =1,2,...,n )and i 0=1,where a i (x )denotes the attribute value of x with respect to the attribute a i ,then we may calculate the weights just like (12):u i ¼Y i k ¼1i k À1;i ¼1;2;...;nð14ÞUsing this we are able to obtain the normalized priorities basedweights:r i ¼u iP nj ¼1u j;i ¼1;2;...;n ð15ÞThe next step is to order the attributes by their satisfactions andthen aggregate them,which will generate a prioritized ordered weighted averaging (POWA)operator.Definition 4.2[20].On the basis of a BUM function f (see Definition 2.5),we can calculate w k =f (R k )Àf (R k À1)(k =1,2,...,n ),where R k ¼P k i ¼1r ind ði Þand R 0=0.Moreover,let F :[0,1]n?[0,1],for any alternative x ,ifa ðx Þ¼F w ða i ðx ÞÞ¼X n i ¼1w i a ind ði Þð16Þwhere the weight vector w =(w 1,w 2,...,w n )T ,and the function indis assumed as an index function so that ind (j )is the index of the j th largest of the a i (x )(i =1,2,...,n ),then F is called a prioritized or-dered weighted averaging (POWA)operator.In the following subsections,we will develop the prioritized intuitionistic fuzzy aggregation operators motivated by the above operators.4.2.Prioritized intuitionistic fuzzy aggregation (PIFA)operator We take the MADM problems into account here,whose attri-butes are assessed by intuitionistic fuzzy information.When there are prioritization relationships over the attributes in an intuitionis-tic fuzzy MADM problem,it will be unavailable any longer for the common MADM methods.Therefore,it is essential to develop some new aggregation methods to solve intuitionistic fuzzy MADM problems with prioritized attributes,and thus,we will put forward a prioritized intuitionistic fuzzy aggregation (PIFA)operator by extending the prioritized aggregation operators in this subsection.We first introduce intuitionistic fuzzy MADM problems with prioritized attributes as follows:Suppose that we have a set of attributes,based on which we assess several alternatives making use of the IFVs,and there exist prioritization relationships over these attributes.According to the prioritization relationships,we partition the attributes into q dis-tinct categories,e H 1;e H 2;...;e H q ,such that e H i ¼f a i 1;a i 2;...;a in ig and e H1>e H 2>...>e H q ,i.e.,the attributes in the category e H i have a higher priority than those in e Hk if i <k .Here a ij (j =1,2,...,n i )are the attributes in category e Hi .Then the universal set of attributes is ~A ¼S q i ¼1e H i ,furthermore,we assume that n ¼P q i ¼1n i is the total number of attributes.When considering the alternatives x 1,x 2,...,x m under these attributes,we express the attribute values of the alternatives as a ij (x k )(i =1,...,q ;j =1,...,n i ;k =1,...,m ).In the remainder of this paper,we will make an in-depth exploi-tation with respect to the solutions to intuitionistic fuzzy MADM problems with prioritized attributes.In order to solve these MADM problems,the key is to calculate the weights by modeling the prioritization of attributes and then aggregate the prioritized attributes.The weight w i may be obtained by calculating the attributes inthe i th attribute category e Hi .We assume that there is a certain function ~/:X n !X ,based on which we can synthesize all the attribute values in the same category into an IFV ~i i :~i i ¼ð1;0Þ;i ¼0~/ða i 1;a i 2;...;a in iÞ;i ¼1;2;...;qð17ÞFor example,we may take the function ~/as the minimum,max-imum or average function,etc.Thereafter,based on ~i i ði ¼0;1;2;...;q Þ,we can calculate the weights as:w i ¼ ik ¼1~i k À1¼~i 0 ~i 1 ... ~i i À1ð18ÞFrom (17)and (18),we know that:(1)the weights w i (i =1,2,...,q )are IFVs;(2)the weight of the attribute with the higher priority strongly dominates that of the lowly prior attribute,i.e.,w i P 1w k if i <k ;and (3)the weight vectors are generally not the same for dif-ferent alternatives.Suppose that for an attribute value a (x )there are two different weights w 1and w 2represented by IFVs.Then accord-ing to Theorem 3.1,if w 1P d w 2,then (1)w 1^a (x )P d w 2^a (x );(2)w 1_a (x )P d w 2_a (x );(3)w 1Èa (x )P d w 2Èa (x );and (4)w 1 a (x )P d w 2 a (x ).Additionally,according to Theorem 3.2,if w 1P 1w 2,then (1)w 1^a (x )P 1w 2^a (x );(2)w 1_a (x )P 1w 2_a (x );(3)w 1Èa (x )P 1w 2Èa (x );and (4)w 1 a (x )P 1w 2 a (x ).Thus,we can also indicate the importance degree of an attribute value by means of the intuitionistic fuzzy weight.What we next want to do is to aggregate the attribute values together with the ob-tained weights to an overall one for a certain alternative.To do so,we develop an aggregation function ~F:X n !X ,called a prioritized intuitionistic fuzzy aggregation (PIFA)operator:a ðx k Þ¼~F w ðx kÞðe H 1;e H 2;...;e H q Þ;k ¼1;2;...;m ð19Þwhere the PIFA operator is a monotonic function,and w (x k )=(w 1(x k ),w 2(x k ),...,w q (x k ))T (k =1,2,...,m )are the weight vectors corresponding to the alternatives x k (k =1,2,...,m ).For illustration,we give a special PIFA operator as follows:Let ~/be the minimum function,and then by (17),we have ~i i ðx k Þ¼1;i ¼0^jða ij ðx k ÞÞ;i ¼1;2;...;q(ð20Þbased on which and (18),we can calculate the weights w i (x k )(i =1,2,...,q ).Furthermore,let the function e F be the IFWC operator (see (5)),then we utilize the IFWC operator to aggregate the priori-tized attribute values and the weights so as to get the overall attri-bute values a (x k )(k =1,2,...,m )for the alternatives x k (k =1,2,...,m )respectively:112X.Yu,Z.Xu /Information Fusion 14(2013)108–116。
国家电网公司英语职称考试要点(短文判断)(2)
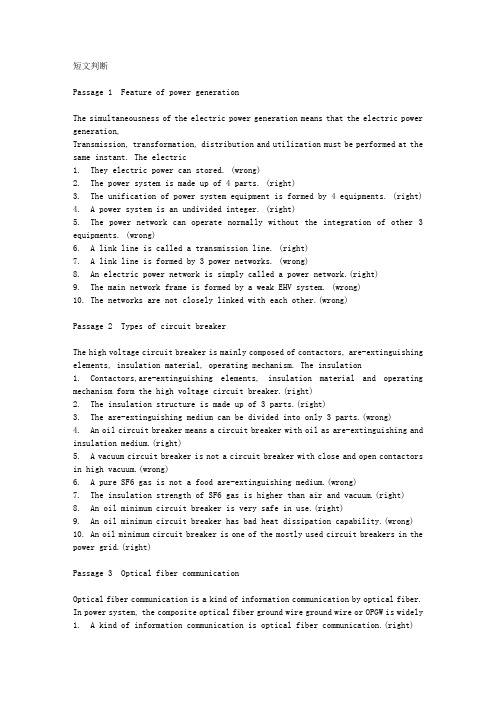
短文判断Passage 1 Feature of power generationThe simultaneousness of the electric power generation means that the electric power generation,Transmission, transformation, distribution and utilization must be performed at the same instant. The electric1. They electric power can stored. (wrong)2. The power system is made up of 4 parts. (right)3. The unification of power system equipment is formed by 4 equipments. (right)4. A power system is an undivided integer. (right)5. The power network can operate normally without the integration of other 3 equipments. (wrong)6. A link line is called a transmission line. (right)7. A link line is formed by 3 power networks. (wrong)8. An electric power network is simply called a power network.(right)9. The main network frame is formed by a weak EHV system. (wrong)10. The networks are not closely linked with each other.(wrong)Passage 2 Types of circuit breakerThe high voltage circuit breaker is mainly composed of contactors, are-extinguishing elements, insulation material, operating mechanism. The insulation1. Contactors,are-extinguishing elements, insulation material and operating mechanism form the high voltage circuit breaker.(right)2. The insulation structure is made up of 3 parts.(right)3. The are-extinguishing medium can be divided into only 3 parts.(wrong)4. An oil circuit breaker means a circuit breaker with oil as are-extinguishing and insulation medium.(right)5. A vacuum circuit breaker is not a circuit breaker with close and open contactors in high vacuum.(wrong)6. A pure SF6 gas is not a food are-extinguishing medium.(wrong)7. The insulation strength of SF6 gas is higher than air and vacuum.(right)8. An oil minimum circuit breaker is very safe in use.(right)9. An oil minimum circuit breaker has bad heat dissipation capability.(wrong)10. An oil minimum circuit breaker is one of the mostly used circuit breakers in the power grid.(right)Passage 3 Optical fiber communicationOptical fiber communication is a kind of information communication by optical fiber. In power system, the composite optical fiber ground wire ground wire or OPGW is widely 1. A kind of information communication is optical fiber communication.(right)2. The composite optical fiber ground wire is seldom used in power system.(wrong)3. One of the transmission tasks of the optical fiber main network is to transmit administration telephones from power system.(right)4. Optical fiber communication has been the important communication method in power communication system.(right)5. Large capacity of communication is not one of the advantages of optical fiber communication.(wrong)6. The communication capacity of optical fiber is indirectly proportion to the carrier frequency.(wrong)7. The carrier frequency of power line is more than 300kHz.(right)8. The carrier frequency of microwave is between 3000Mha and 500MHz.(wrong)9. The main problem of the fiber-optic channel is the high price of fiber-optic equipment(right)10. The cables made by optical fiber are not expensive.(wrong)Passage 4 Power plantAccording to the mode of energy conversion, power plants can be classified into fossil-fired, hydraulic, nuclear, wind, solar, geothermal and tide power plants and so1. Base on energy conversion, power plants are divided into more than 7 categories.(right )2. A power plant tries to transform energy source into electric power. (wrong )3. The power plant generating electricity and supplying steam is called a co-generation plant. (right )4. A power net plan for short and medium term is one of factors in selecting a site (wrong)5. Two important factors for a new plant plan are fuel supply and ash disposal.(right)6. Environmental protection is less considered in building a new power plant(wrong)7. Water sources and transportation conditions are not needed to consider in selecting a new site(wrong)8. The total capacity in a newly scheduled plant arranges from 1200MW to 3600MW(right)9. The number of units exceeds more than six.(wrong)10. The ranks of capacity should be limited within two.(right)Passage 5 Selection of metal material for the boiler in units of 1000MW gradeTaking a general view of the 1000MW grade high-efficiency supercritical unit designed and made in China, the temperature of main steam and reheated steam are1. The 1000MW grade high-efficiency supercritical unit is designed and made in China.(right)2. The temperature of main steam and reheated steam reach basically between 570 to650℃.(wrong)3. The tube wall temperature of superheater and reheater can reach more than 600 to 650 ℃ (right)4. The tube wall temperature steam oxidation performance at tube inner surface is highly demanded.(rig ht)5. The anti-high temperature flue gas corrosion performance at tube outside surface is not highly demanded.(wrong)6. The temperature limit of T91 for use is about 593℃(right)7. The temperature limit of T92 for use is less than 620℃(wrong)8. The temperature of austenite stainless steel for normal use is about 650℃(right)9. The temperature limit of austenite stainless steel is less than 700℃(wrong)10. Only heat-resisting steel can be adopted for use.(wrong)Passage 6 The role of the condenserThe condenser is a surface heat exchanger in which cooling water passing through the tubes removes the vaporization heat from the exhaust steam which is passing over 1. A condenser is a heat exchanger removing the vaporization heat from the exhaust steam and condensing the exhaust steam into water.(right)2. It is suggested that the water should not be cooled below the saturation temperature.(right)3. The saturation temperature would not remove excess energy from the system and reduce the overall efficiency.(wrong)4. It is unnecessary to reduce the steam to water so that it can be pumped back through the system.(wrong)5. The condenser does not control back-pressure at the turbine exhaust.(wrong)6. The important factor controlling the turbine backpressure is the temperature of the cooling water passing through the condenser tubes.(right)7. The temperature of cooling water will increase its temperature between 15oF and 20oF as it passes through the condenser.(right)8. The terminal difference means the temperature difference between the cooling water and the turbine exhaust steam.(right)9. The temperature increase of the cooling water and the terminal difference depend on 3 factors.(wrong)10. The exhaust steam temperature does not rely on the temperature of the cooling water which enters the condenser.(wrong)Passage 7 Hydraulic structureThe selected type dam of hydraulic power plant depends principally on topographic, geologic, hydrologic, climatic conditions, constructional materials, lay out plan, cost1. The type of dam for hydraulic power plant depends on many factors.(right)2. The main purpose of the dam safety monitoring is to discover the normality ofdam in time.(right)3. The equipment installed inside the dam is to discover the normality of dam in time.(wrong)4. Horizontal displacement is more important than vertical displacement.(wrong)5. Temperature stress and strain are the two main monitoring items of dam.(wrong)6. The monitoring method is the suitable points on the dam and its foundation.(right)7. Some monitoring devices are movable for periodical supervision.(wrong)8. The monitoring result result shall be analyzed and studied by computers.(wrong)Passage 8 Heat treatmentThe purpose of post-weld heat treatment is: to diminish the residual stress in the welded joints; to improve the organization and property of the welded joints.1. The post-weld heat treatments have two main purposes.(right)2. One of the purposes is to diminish the residual stress in the welded joints.(right)3. The other purpose is to improve the organization of the welded joints.(wrong)4. The welding crack can be classified into 5 categories.(right)5. To change the internal structure of the work piece is one of purposes for heat treatment.(right)6. There are 5 kinds of steel heat treatment.(wrong)7. The processes of heat treatment include 4 procedures.(right)8. The electric resistance furnace is not widely used.(wrong)9. Heat treatment furnaces are not composed of electric resistance furnace, combustion furnace and surface heating device.(wrong)10. The control device for heat treatment temperature is composed of temperature measuring element, and control device.(wrong)Passage 9 Business and risksMarx once quoted a famous saying in his work capitalism, “once ther’s appropriate profit, capital will become bold. For 10% profit, it will guarantee its being fully1. The work Capitalism is written by Carl Marx.(right)2. For 20% profit, capital will take risks.(wrong)3. For 100%, capital dares to break any human laws.(right)4. For 200%, capital dares to commit any crime and even risks being hanged. (wrong)5. The pursuit of profits forces human beings to take any risks.(right)6. The pursuit of profit is much more encouraged than the pursuit of friendship.(wrong)7. There are two ownerships existing in Chinese economy.(right)8. Only bright manufacturer or manager can predict the future of his enterprises.(wrong)Passage10 ElectricityElectricity may be dangerous. It always takes the shortest way to the ground. So it needs to find something like metal or water to carry it to the ground. These things are1. Electricity is not dangerous as people think.(wrong)2. Dry wood can carry electricity to the ground. u(wrong)3. Conductors like metal or water can carry electricity to the ground.(right)4. Human body is a good conductor because it has 90% water in the body.(wrong)5. You can use a hairdryer far from bath and water.(right)6. Wet hands are good conductors for transmitting electricity.(right)7. The best tool to push a shocked person away from the power is to use a non-conductor.(right)Passage 11 Undersea LifeThe undersea world is very mysterious. In the daytime, there is enough light. Under the sea, everything is blue and green. Today scuba diving is a new sport and many1. Everything under the sea becomes blue and green. (right)2. Now people can dive into the water to explore the secrets of the sea.(right)3. You can stay in deep blue sea for a long time if you have bottles of air on your back. (right)4. It is warm but dark in deeper water. (wrong)5. Under about 3000 feet, there is no light at all. (right)6. Many fish under deep water have eyes like normal fish in water. (wrong )7. The sea is a dangerous place for all organisms.(right)8. Deep sea animals must follow the rule: to eat or be eaten.(right)Passage 12 Advice on FriendshipWe all need friends. Without friends we may feel empty and sad. It is not difficult for most people to make friends. But you may feel shy and not want to make the first1. People can’t live well if they have no friends. (right)2. If you want to keep a friend forever, you should greet him with a smile.(right)3. Cooking some soup for a sick neighbour makes you’re the sick neighbour feel much better.(right)4. If you have a close friend, It doesn’t matter to be rude to him.(wrong )5. If you want to be a loyal friend, you should understand their ideas and learn from them. (right)Passage 13 AustraliaAustralia is a vast contient,the sixth largest in the world.It is approximately 7700000 square kilometers in size and has a coastline almost 37000 kilometers long.1. Australia is a large country and has a relatively lagre population. (wrong )2. The majority of people living in Australia are city dwellers. (right)3. The delivery of education services to people living in remote areas is unimaginable for almost a century. (wrong )4. The attempt to provide educational services to distant dwellers did not take place until 1916. (right)5. The schoolwork was delivered and returned through sealed roads,regular bus routes and air services in the beginning. (wrong )Passage 14 BiomassBiomass is a cost-effective source of energy.1、.As a source of energy, biomass can bring (Right)2、.One of the advantages of using biomass(Right)3、.Biomass doesn′,t produce enough carbon(wrong)4、4Despite its advantages ,biomass is(Right)5、.Only trees and crops can be used(wrong)Passage 15 Nuclear radiationNuclear power,′s danger to health, safety, and1、The mystery about nuclear radiation(Right)2、We cannot sense radio activity without(wrong)3、Common radio waves are harmless to(Right)4、.Even at the lowest levels, radiation(wrong)5、Victims of nuclear radiati0n may die(Right)Passage 16 Livestock′s Long shadowWhen you think about the growth of human1、With the increase of human population(wrong)2、It was not big the number of (wrong)3、Rain forests are decreasing as a result(Right)4、The global livestock contribute more(Right)5、According to the passage ,the only(wrong)Passage 17 Pain managementYears ago, doctors often said that pain was1、Pain is a natural part of aging(wrong)2、Today, it is believed that chronic(Right)3、To provide a comprehensive therapy(Right)4、Our respect for pain management(wrong)5、The drugs which helped patients(Right)Passage 18 The obama administration′s bankAmong the criticisms of the obama administration′s1、The obama administration, bank rescue(wrong)2、The word “float”(Para.3) (wrong)3、According to the author ,ordinary(Right)4、The bailout plan is so complex(wrong)5、The last paragraph indicates that(Right)。
Weak Magnetic Dipole Moments in the MSSM
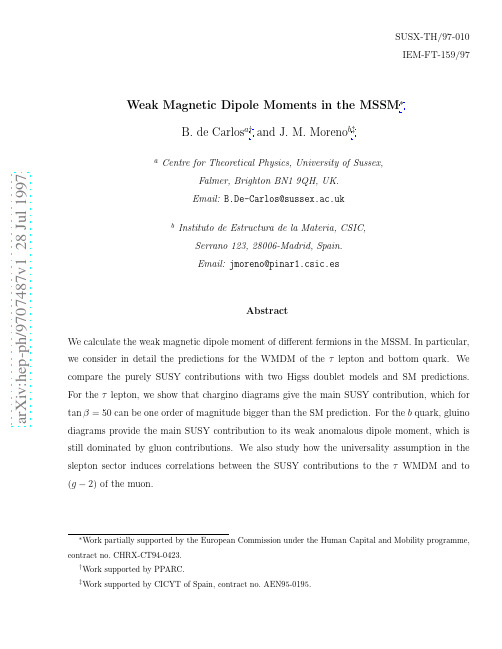
1
Introduction
One of the most promising candidates for physics beyond the so–called Standard Model (SM) is that of supersymmetry (SUSY) [1]. SUSY has the highly attractive properties of giving a natural explanation to the hierarchy problem of how it is possible to have a low energy theory containing light scalars (the Higgs) when the ultimate theory must include states with masses of order of the Planck mass. On the other hand, the spectrum of new particles predicted by SUSY seems to lie beyond the region explored by present colliders. The information coming from direct searches is nicely complemented by the one provided by precision measurements. The predicted values for these measurements are sensitive to supersymmetric, virtual, contributions. An example of this kind of observables is given by magnetic dipole moments (WMDM). In a renormalizable theory, they are generated by quantum corrections, and then virtual effects from new physics appear at the same level as SM weak contributions. Due to the chiral nature of these observables, we expect that the induced corrections will be suppressed by some power of mf /M , where mf is the mass of the fermion and M is the typical scale of new physics. In that case, heavy third generation fermions would be the preferred candidates to look for this kind of quantum corrections. In this paper we present a complete calculation of the WMDM of the τ lepton and bottom quark within the Minimal Supersymmetric Standard Model (MSSM) framework. We will compare the contributions from the three different sectors: the electroweak one, the two higgs doublet sector and the purely supersymmetric one involving charginos, neutralinos and sfermions. We will analyze the regions of the supersymmetric parameter space where these new contributions could be more relevant. Finally, we will also study other observables such as (g − 2)µ that could get potentially large supersymmetric contributions in the region where the SUSY corrections to the WMDM are enhanced.
有序加权集结算子的赋权方法
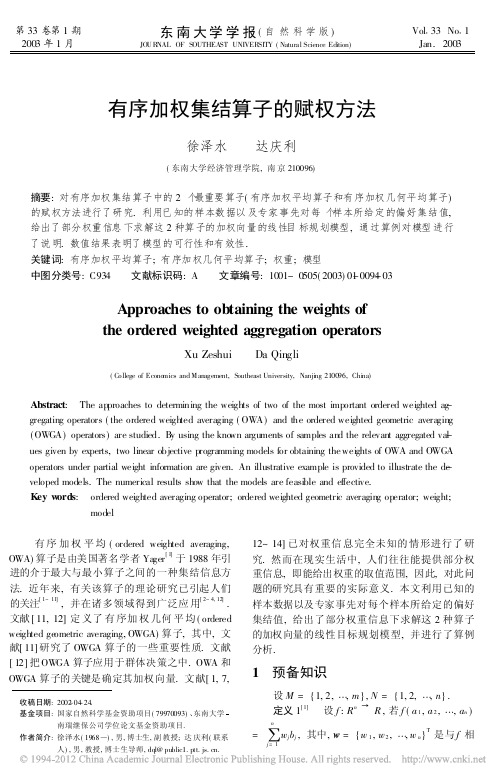
第33卷第1期2003年1月东南大学学报(自然科学版)JO UR NAL OF SOUTHEA ST UNIVER SITY (Natural Science Edition)Vol 133No 11Jan.2003有序加权集结算子的赋权方法徐泽水 达庆利(东南大学经济管理学院,南京210096)摘要:对有序加权集结算子中的2个最重要算子(有序加权平均算子和有序加权几何平均算子)的赋权方法进行了研究.利用已知的样本数据以及专家事先对每个样本所给定的偏好集结值,给出了部分权重信息下求解这2种算子的加权向量的线性目标规划模型,通过算例对模型进行了说明.数值结果表明了模型的可行性和有效性.关键词:有序加权平均算子;有序加权几何平均算子;权重;模型中图分类号:C934 文献标识码:A 文章编号:1001-0505(2003)01-0094-03Approaches to obtaining the weights ofthe ordered weighted aggregation operatorsXu Zeshui Da Qingli(College of Econo mics and M anagement,Southeast University,Nanji ng 210096,Chi na)Abstract : The approaches to determining the weights of two of the most important ordered weighted ag -gregating operators (the ordered weighted averaging (OW A)and the ordered weighted geometric avera ging (OW GA)operators)are studied.B y using the kno wn arguments of samples and the relevant aggregated va-l ues given by experts,two linear objective progra mming models for obtaining the weights of OWA and OWGA operators under partial weight information are given.An illustrative example is provided to illustrate the de -veloped models.The numerical results show that the models are feasible and effective.Key w ords : ordered weighted averaging operator;ordered weighted geometric averaging operator;weight;model收稿日期:2002-04-24.基金项目:国家自然科学基金资助项目(79970093)、东南大学南瑞继保公司学位论文基金资助项目.作者简介:徐泽水(1968)),男,博士生,副教授;达庆利(联系人),男,教授,博士生导师,dql@.有序加权平均(ordered weighted averaging,OWA)算子是由美国著名学者Ya ger [1]于1988年引进的介于最大与最小算子之间的一种集结信息方法.近年来,有关该算子的理论研究已引起人们的关注[1~11],并在诸多领域得到广泛应用[2~4,12].文献[11,12]定义了有序加权几何平均(ordered weighted ge ometric averaging,OWGA)算子,其中,文献[11]研究了OWGA 算子的一些重要性质.文献[12]把OW GA 算子应用于群体决策之中.OW A 和OWGA 算子的关键是确定其加权向量.文献[1,7,12~14]已对权重信息完全未知的情形进行了研究.然而在现实生活中,人们往往能提供部分权重信息,即能给出权重的取值范围,因此,对此问题的研究具有重要的实际意义.本文利用已知的样本数据以及专家事先对每个样本所给定的偏好集结值,给出了部分权重信息下求解这2种算子的加权向量的线性目标规划模型,并进行了算例分析.1 预备知识设M ={1,2,,,m },N ={1,2,,,n }.定义1[1]设f :R ny R ,若f (a 1,a 2,,,a n )=Enj =1w j b j ,其中,w ={w 1,w 2,,,w n }T是与f 相关联的加权向量,w j I[0,1],E n j=1w j=1,且b j是数据集合{a1,a2,,,a n}的降序排列中的第j个元素,则称函数f是n维有序加权平均算子,简称为OW A算子.定义2[11,12]设g:R+n y R+,若g(a1,a2, ,,a n)=F nj=1b w j j,其中,w={w1,w2,,,w n}T是与g相关联的加权向量,w j I[0,1],E n j=1w j=1,且b j是数据集合{a1,a2,,,a n}的降序排列中的第j个元素,则称函数g是n维有序加权几何平均算子,简称为OWGA算子.由于OWA和OWGA算子的加权向量与数据集结过程中的位置相关联,因此又称为位置向量.文献[1,7,12~14]已对权重信息完全未知的情形进行了研究:方法1[1,12]由下列公式确定算子的加权向量w j=Q jn -Q j-1nj I N式中,模糊语义量化算子Q由下式给出:Q(r)=0r<ar-ab-aa[r[b 1r>b式中,a,b,r I[0,1].对应于模糊语义量化准则: /大多数0、/至少半数0、/尽可能多0的算子Q中参数对(a,b)分别为(0.3,0.8),(0,0.5),(0.5,1).方法2[13,14]算子的加权向量由下列非线性规划模型获得:M1max-E n i=1w i ln w is.t.1n-1E ni=1(n-i)w i=A0[A[1 E ni=1w i=10[w i[1,i I N式中,参数A事先由决策者给定.文献[14]利用拉格朗日乘子法把模型M1转变为一个多项式方程来确定加权向量w.文献[7]利用一个复杂的无约束非线性规划模型来确定算子的加权向量,其计算量太大,因此实用性也较差(略).2主要结果由于客观事物的复杂性和不确定性,OWA和OWGA算子中的权重有时可能完全未知或只能给出其取值范围.对此,利用线性目标规划模型为OW A和OWGA算子进行赋权.先考虑OWGA算子:设有m个样本,每个样本是一个n维数据向量{a k1,a k2,,,a kn},k I M,并打算通过OWGA算子对其进行有序加权集结.记H为已知部分权重信息所确定的可能权重集合H=w={w1,w2,,,w n}T0[A j[w j[B j[1,j I N,E n j=1w j=1且专家对每个样本存在一定的偏好,并事先给出每个样本偏好集结值p k,k I M.因此,目标是获得OWGA算子的加权向量w,使得下列一组等式尽可能地满足g(a k1,a k2,,,a kn)=p k k I M即F nj=1b w j kj=p k k I M(1)式中,b kj是数据向量{a k1,a k2,,,a kn}的降序排列中的第j个元素.由于式(1)是非线性形式,用其求解加权向量w较为困难,把式(1)两边取对数,则有E n j=1w j ln b kj =ln p k,k I M,并引入偏差函数f k=E n j=1w j ln b kj -ln p k,k I M.显然,为了得到合理的加权向量w={w1,w2,,,w n}T,上述偏差函数值总是越小越好,因此可构造下列多目标最优化模型:M2min f k=E n j=1w j ln b kj-ln p k k I Ms.t.w I H为了求解多目标最优化模型M2,考虑到所有的目标函数是公平竞争的,且每个目标函数f k希望达到的期望值为0,可将M2转化为下列线性目标规划模型:M3min J=E m k=1(d+k+d-k)s.t.E n j=1w j ln b kj-ln p k-d+k+d-k=0k I M w I H;d+k\0;d-k\0k I M式中,d+k为E n j=1w j ln b kj-ln p k高于期望值0的上偏差变量;d-k为E n j=1w j ln b kj-ln p k低于期望值0的下偏差变量.利用目标单纯形法[15]可求解该模型M3,从而得到OWGA算子的加权向量w.类似地,可以通过求解下列模型M4,即可得95第1期徐泽水,等:有序加权集结算子的赋权方法到OWA 算子的加权向量w :M4min J =E mk =1(e+k+e -k )s.t.E nj =1w jbkj-p k -e +k +e -k =0 k I Mw I H ;e +k \0;e -k \0 k I M 若专家事先没有给出每个样本偏好集结值,则在部分权重信息下,把模型M1推广为模型M5M5max -Eni =1w i ln w is.t.1n -1E ni =1(n -i )w i =A 0[A [1 w I H其中,参数A 事先由决策者给出.利用Frank -Wolfe 算法[15]即可求解模型M5,从而获得加权向量w .3 算例分析设样本的数据向量及专家给出的偏好集结值如表1所示,不妨利用OWGA 算子进行说明:表1 数据值样本数据向量偏好集结值1{4,3,8} 4.52{3,4,7} 4.03{8,3,5} 6.54{5,9,4}5.0设H ={w ={w 1,w 2,w 3}T0.2[w 1[0.4,0.3[w 2[0.6,0.1[w 3[0.3,w 1+w 2+w 3=1},利用模型M3,可建立下列模型:min J =E 4k =1(d+k+d -k )s.t.ln 8w 1+ln 4w 2+ln 3w 3-ln 4.5-d +1+d -1=0ln 7w 1+ln 4w 2+ln 3w 3-ln 4-d +2+d -2=0ln 8w 1+ln 5w 2+ln 3w 3-ln 6.5-d +3+d -3=0ln 9w 1+ln 5w 2+ln 4w 3-ln 5-d +4+d -4=00.2[w 1[0.4;0.3[w 2[0.6;0.1[w 3[0.3;w 1+w 2+w 3=1d +k \0;d -k \0;k =1,2,3,4求解该模型,得到加权向量w ={0.400,0.454,0.146}T ,因此g (4,3,8)=80.400@40.454@30.146= 5.06类似地,可得:g (3,4,7)= 4.80,g (8,3,5)=5.60,g (5,9,4)= 6.12.上述数值结果既充分利用了已有的客观权重信息,又照顾到决策者的主观愿望,从而体现了主观与客观的统一.4 结 语本文利用已知的样本数据以及专家对每个样本事先所给定的偏好集结值,给出了部分权重信息下求解2种算子的加权向量的线性目标规划模型.算例分析表明:模型是可行且有效的.参考文献(References )[1]Yager R R.On ordered weighted averaging aggregation oper -ators in multicri teria decision makin g [J].IEEE Transactionson Systems ,Man ,an d Cybernetics ,1988,18:183190.[2]Yager R R,Kacprzyk J.The ordered weighted a vera ging op-erators :theory and a pplications [M].Norwell,MA:Klu wer,1997.10100.[3]徐泽水.一种不确定型OWA 算子及其在群决策中的应用[J].东南大学学报(自然科学版),2002,32(1):147150.Xu Zeshui.Uncertain ordered weighted averaging (OWA )operator and i ts application to group decision making [J].Journal of Southeast University (N atural Science Edition ),2002,32(1):147150.(in Chinese)[4]徐泽水,达庆利.一种组合加权几何平均算子及其应用[J].东南大学学报(自然科学版),2002,32(3):506509.Xu Zeshui,Da bined weighted geometric averag -ing operator and i ts application [J].Jou rnal of Southeast University (N atural Science Edition ),2002,32(3):506509.(in Chinese)[5]T orra V.The weighted OWA operator [J].Inte r na tionalJournal of Intelligen t Systems ,1997,12:153166.[6]Mitchell H B,Estrakh D D.An OWA operator with fuzzyranks [J].I nternational Journa l o f I ntelligent Systems ,1998,13:6981.[7]Filev D,Yager R R.On the issue of obtaining OWA operatorweights[J].Fuzzy Sets and Systems ,1998,94:157169.[8]Schaefer P A,Mitchell H B.A generalized OWA operator[J].I n ternational Journal of I n telligent Systems ,1999,14:123143.[9]Yager R R,Filev D P.Induced ordered weighted averagingoperators [J].IEEE Transaction on Systems ,Man ,and Cybernetics ,1999,29:141150.[10]Xu Z S,Da Q L.The uncertain OWA operator [J].Inte r na -tional J ournal o f I n telligent Systems ,2002,17:569575.[11]Xu Z S,Da Q L.The ordered wei ghted geometric averagingoperators [J].Internationa l J ournal of Intelligent Systems ,2002,17:709716.[12]Herrera F,Herrera -Vied ma E,Chiclana F.Multiperson de -cision -maki ng based on multiplicative preference relations[J].Europ ean Jou r na l of O p erational Resea rch ,2001,129:372385.[13]O .Hagan M.Aggregating template or rule antecedents inrea-l time expert systems wi th fuzzy set logic [A].In:Proc 22nd Annual IEEE Asilomar Con f On Signa ls ,Systems and Computers [C].Pacific Grove,C A,1988.681689.[14]Full r R,Majlender P.An analytic approach for obtainingmaxi mal entropy OWA operator weights [J].Fu zzy Sets and Systems ,2001,124:5357.[15]胡毓达.实用多目标最优化[M ].上海:上海科学技术出版社,1990.18596东南大学学报(自然科学版)第33卷。
高级国际财务管理师考试复习题
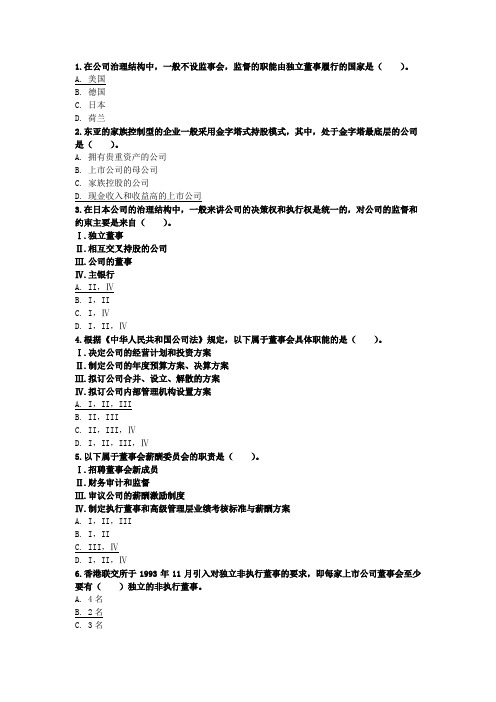
1.在公司治理结构中,一般不设监事会,监督的职能由独立董事履行的国家是()。
A. 美国B. 德国C. 日本D. 荷兰2.东亚的家族控制型的企业一般采用金字塔式持股模式,其中,处于金字塔最底层的公司是()。
A. 拥有贵重资产的公司B. 上市公司的母公司C. 家族控股的公司D. 现金收入和收益高的上市公司3.在日本公司的治理结构中,一般来讲公司的决策权和执行权是统一的,对公司的监督和约束主要是来自()。
Ⅰ.独立董事Ⅱ.相互交叉持股的公司Ⅲ.公司的董事Ⅳ.主银行A. II,ⅣB. I,IIC. I,ⅣD. I,II,Ⅳ4.根据《中华人民共和国公司法》规定,以下属于董事会具体职能的是()。
Ⅰ.决定公司的经营计划和投资方案Ⅱ.制定公司的年度预算方案、决算方案Ⅲ.拟订公司合并、设立、解散的方案Ⅳ.拟订公司内部管理机构设置方案A. I,II,IIIB. II,IIIC. II,III,ⅣD. I,II,III,Ⅳ5.以下属于董事会薪酬委员会的职责是()。
Ⅰ.招聘董事会新成员Ⅱ.财务审计和监督Ⅲ.审议公司的薪酬激励制度Ⅳ.制定执行董事和高级管理层业绩考核标准与薪酬方案A. I,II,IIIB. I,IIC. III,ⅣD. I,II,Ⅳ6.香港联交所于1993年11月引入对独立非执行董事的要求,即每家上市公司董事会至少要有()独立的非执行董事。
A. 4名B. 2名C. 3名D. 1名7.独立董事在行使职权的同时,也要承担一定的责任,独立董事在公司重大事项发表意见时的失误可能对公司造成重大影响,不仅对股东利益造成损害而且可能使自己身败名裂,承担连带责任。
为了避免独立董事逃避责任,消极对待其工作,目前国际上通行的做法是()。
A. 为独立董事设置固定薪金制度B. 独立董事持有公司的股票C. 建立独立董事保险制度D. 建立延期支付计划8.以下说法正确的是()。
Ⅰ.公司控制权转移市场的主要功能是当股东大会和董事会充分发挥作用时,通过并购更换公司经理层和董事会Ⅱ.在众多的公司治理决定因素中,所有权结构和法律制度是公司治理模式的主要决定因素Ⅲ.公司治理作为一套完整的流程和机制,确保公司管理层对其决策负责,使公司达到有效的资源配置Ⅳ.在不同的经济制度条件下,不同的治理系统拥有非常相似的结构特征和机制A. I, IIIB. II,IIIC. II,III,ⅣD. I,II,III,Ⅳ9.在公司治理结构中,作为所有者的代理人,成为联系公司所有者和管理者桥梁的是()。
GMS-BIQ考试-20190613
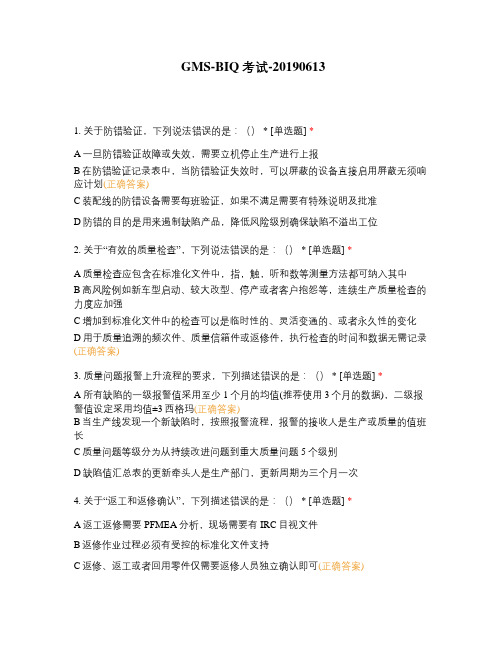
GMS-BIQ考试-201906131. 关于防错验证,下列说法错误的是:() * [单选题] *A一旦防错验证故障或失效,需要立机停止生产进行上报B在防错验证记录表中,当防错验证失效时,可以屏蔽的设备直接启用屏蔽无须响应计划(正确答案)C装配线的防错设备需要每班验证,如果不满足需要有特殊说明及批准D防错的目的是用来遏制缺陷产品,降低风险级别确保缺陷不溢出工位2. 关于“有效的质量检查”,下列说法错误的是:() * [单选题] *A质量检查应包含在标准化文件中,指,触,听和数等测量方法都可纳入其中B高风险例如新车型启动、较大改型、停产或者客户抱怨等,连续生产质量检查的力度应加强C增加到标准化文件中的检查可以是临时性的、灵活变通的、或者永久性的变化D用于质量追溯的频次件、质量信箱件或返修件,执行检查的时间和数据无需记录(正确答案)3. 质量问题报警上升流程的要求,下列描述错误的是:() * [单选题] *A 所有缺陷的一级报警值采用至少1个月的均值(推荐使用3个月的数据),二级报警值设定采用均值±3西格玛(正确答案)B当生产线发现一个新缺陷时,按照报警流程,报警的接收人是生产或质量的值班长C质量问题等级分为从持续改进问题到重大质量问题5个级别D缺陷值汇总表的更新牵头人是生产部门,更新周期为三个月一次4. 关于“返工和返修确认”,下列描述错误的是:() * [单选题] *A返工返修需要PFMEA分析,现场需要有IRC目视文件B返修作业过程必须有受控的标准化文件支持C返修、返工或者回用零件仅需要返修人员独立确认即可(正确答案)D返工返修人员要获得适合的培训和资格确认5. 关于“信息反馈/前馈”,下列描述错误的是:() * [单选题] *A下游内部客户发生的问题可以口头交流,不需要进行记录(正确答案)B操作工位发现来料缺陷应反馈现场质量和供应商质量,并启动筛选和遏制措施C在评审工位/CARE和制造班组长之间,以及制造班组之间,有一个快速反馈/前馈机制D发生缺陷超报警值报警时,由质量工程师进行判断是否进行前/反馈信息,并签字确认6. 关于“快速响应”下列描述错误的是:() * [单选题] *A工厂应设置快速响应交流区域(质量行动中心)B质量经理每日牵头召开区域快速响应中心交流会C相关功能块每日参加中午质量例会,并对当前问题和遗留问题进行回顾D标准化文件没有修改之前,质量问题添加到JES背面即可,无须高关注(正确答案)7. BIQ Next中工厂对所有PTR零件发运前使用(),阻止PTR审批前发运 [单选题] *A PTR标识B Flexnet系统HOLD(正确答案)C 工厂现场标识D PTR系统8. PFMEA在()中有一个计划展示改进和推荐行动,并进行分析改进,制定3年提升改进计划 [单选题] *A PFMEA工艺文件中B 工厂汇报材料C SQP(战略质量计划)(正确答案)D 部门BPD中9. 每年通过评审Bypass的PFMEA,通过()来减少Bypass项目 [单选题] *A风险评估/改进措施(正确答案)B质量例会C快速响应会10. Field Action(市场行动)的指标要求中 Word Class要求是:() [单选题] *A工厂12-24个月内无制造相关的市场行动B ≥ 36月没有任何(制造,工程,供应商责任的)市场行动(正确答案)C >48月无任何市场行动11. Field Action(市场行动)的指标要求中 GOOD要求是:() [单选题] *A 工厂12-24个月内无制造相关的市场行动(正确答案)B ≥ 36月没有任何(制造,工程,供应商责任的)市场行动C >48月无任何市场行动12. 工厂清洁度改进必须有一个过程来跟踪()的实施状况 [单选题] *A杂质消除清单(BOSE)(正确答案)B清洁度改进C清洁度评审问题改进13. 工厂和每个车间必须具有建立和跟踪()(全部包含)与其实际车间表现和制造财务计算(GM账户7000)联系的过程。
- 1、下载文档前请自行甄别文档内容的完整性,平台不提供额外的编辑、内容补充、找答案等附加服务。
- 2、"仅部分预览"的文档,不可在线预览部分如存在完整性等问题,可反馈申请退款(可完整预览的文档不适用该条件!)。
- 3、如文档侵犯您的权益,请联系客服反馈,我们会尽快为您处理(人工客服工作时间:9:00-18:30)。
Some generalized aggregating operators with linguistic information and their application to multiple attribute group decision making qGui-Wu Wei ⇑Department of Economics and Management,Chongqing University of Arts and Sciences,Yongchuan,Chongqing 402160,PR Chinaa r t i c l e i n f o Article history:Received 26June 2010Received in revised form 28November 2010Accepted 14February 2011Available online 16February 2011Keywords:Multiple attribute group decision making Linguistic informationGeneralized 2-tuple weighted average (G-2TWA)operatorGeneralized 2-tuple ordered weighted average (G-2TOWA)operatorInduced generalized 2-tuple ordered weighted average (IG-2TOWA)operatora b s t r a c tWith respect to multiple attribute group decision making problems with linguistic information,some new decision analysis methods are proposed.Firstly,we develop three new aggregation operators:generalized 2-tuple weighted average (G-2TWA)operator,generalized 2-tuple ordered weighted average (G-2TOWA)operator and induced generalized 2-tuple ordered weighted average (IG-2TOWA)operator.Then,a method based on the IG-2TOWA and G-2TWA operators for multiple attribute group decision making is presented.In this approach,alternative appraisal values are calculated by the aggregation of 2-tuple linguistic information.Thus,the ranking of alternative or selection of the most desirable alterna-tive(s)is obtained by the comparison of 2-tuple linguistic information.Finally,a numerical example is used to illustrate the applicability and effectiveness of the proposed method.Ó2011Elsevier Ltd.All rights reserved.1.IntroductionMultiple attribute decision making (MADM)is a usual task in human activities (Merigó,2010;Merigó&Casanovas,2009,2010;Merigó,Casanovas,&Martínez,2010;Merigó&Gil-Lafuente,2010;Wei,2008,2009a,b;Wei,2010a–d;Wei,Lin,Zhao,&Wang,2010;Wei,Zhao,&Lin,2010;Wei,Zhao,Wang,&Lin,2010;Xu,2009;Wang,Luo,and Liu,2007).It consists of finding the most desirable alternative(s)from a given alternative set.However,un-der many conditions,for the real multiple attribute decision mak-ing problems,the decision information about alternatives is usually uncertain or fuzzy due to the increasing complexity of the socio-economic environment and the vagueness of inherent subjec-tive nature of human thinking,thus,numerical values are inade-quate or insufficient to model real-life decision problems.Indeed,human judgments including preference information may be stated in linguistic terms.Several methods have been proposed for dealing with linguistic information.The approximative computational model based on the Exten-sion Principle (Degani &Bortolan,1988).This model transforms linguistic assessment information into fuzzy numbers and usesfuzzy arithmetic to make computations over these fuzzy num-bers.The use of fuzzy arithmetic increases the vagueness.The results obtained by the fuzzy arithmetic are fuzzy numbers that usually do not match any linguistic term in the initial term set. The ordinal linguistic computational model (Delgado,Verdegay,&Vila,1993).This model is also called symbolic model which makes direct computations on labels using the ordinal structure of the linguistic term sets.But symbolic method easily results in a loss of information caused by the use of the round operator. The 2-tuple linguistic computational model (Herrera &Martí-nez,2000a,b,2001;Herrera,Martínez,&Sánchez,2005).This model uses the 2-tuple linguistic representation and computa-tional model to make linguistic computations.Thus,multiple attribute decision making problems under 2-linguistic environment is an interesting research topic having received more and more attention from researchers during the last several years.Herrera and Martínez (1991)show 2-tuple linguistic information processing manner can effectively avoid the loss and distortion of information.It has a distinct advantage over other lin-guistic processing methods in accuracy and reliability.Herrera and Martínez (2000a)developed 2-tuple arithmetic average (TAA)oper-ator,2-tuple weighted average (TWA)operator,2-tuple ordered weighted average (TOWA)operator and extended 2-tuple weighted average (ET-WA)operator.Jiang and Fan (2003)proposed the 2-tuple ordered weighted geometric (TOWG)operator.Wang and0360-8352/$-see front matter Ó2011Elsevier Ltd.All rights reserved.doi:10.1016/j.cie.2011.02.007qThis manuscript was processed by area editor Imed Kacem.⇑Tel.:+862349891870.E-mail address:weiguiwu@Fan(2003)proposed a Technique for Order Preference by Similarity to Ideal Solution(TOPSIS)method for solving multiple attribute group decision making problems with linguistic assessment infor-mation.Herrera-Viedma,Martinez,Mata,and Chiclana(2005)pre-sented a model of consensus support system to assist the experts in all phases of the consensus reaching process of group decision mak-ing problems with multi-granular linguistic preference relations. Herrera et al.(2005)presented a group decision making process for managing non-homogeneous information.The non-homoge-neous information can be represented as values belonging to do-mains with different nature as linguistic,numerical and interval valued or can be values assessed in label sets with different granu-larity,multi-granular linguistic information.Liao,Li,and Lu(2007) presented a model for selecting an ERP system based on linguistic information processing.Herrera,Herrera-Viedma,and Martínez (2008)proposed a fuzzy linguistic methodology to deal with unbal-anced linguistic term sets.Jiang,Fan,and Ma(2008)developed a method for group decision making with multi-granularity linguistic assessment information.Tai and Chen(2009)developed a evalua-tion model for intellectual capital based on computing with linguis-tic variable.Wang(2009)presented a2-tuple fuzzy linguistic computing approach to deal with heterogeneous information and information loss problems during the processes of subjective eval-uation integration which is based on the group decision-making scenario to assist business managers to measure the performance of New Product Development(NPD)manipulates the heteroge-neous integration processes and avoids the information loss effec-tively.Zhang and Chu(2009)developed fuzzy group decision making for multi-format and multi-granularity linguistic judg-ments in quality function deployment.Fan,Feng,Sun,and Ou (2009)evaluated knowledge management capability of organiza-tions by using a fuzzy linguistic method.Fan and Liu(2010)devel-oped a method for group decision making based on multi-granularity uncertain linguistic information.Wei(2010a)extended Technique for Order Preference by Similarity to Ideal Solution(TOP-SIS)method to2-tuple linguistic multiple attribute group decision making with incomplete weight information.Wei(2010b)pro-posed some extended geometric aggregating operators with2-tu-ple linguistic information.Chang and Wen(2010)developed a approach for Design Failure Mode and Effect Analysis(DFMEA) combining2-tuple and the OWA operator.The traditional generalized aggregating operators and induced generalized aggregating operators are generally suitable for aggre-gating the information taking the form of numerical values,and yet they will fail in dealing with linguistic information.The aim of this paper is to extend the traditional generalized aggregating opera-tors to linguistic environment and develop three new generalized aggregation operators:generalized2-tuple weighted average(G-2TWA)operator,generalized2-tuple ordered weighted average (G-2TOWA)operator and induced generalized2-tuple ordered weighted average(IG-2TOWA)operator.Furthermore,a method based on the IG-2TOWA and G-2TWA operators for multiple attri-bute group decision making is presented.In this approach,alterna-tive appraisal values are calculated by the aggregation of2-tuple linguistic information.Thus,the ranking of alternative or selection of the most desirable alternative(s)is obtained by the comparison of2-tuple linguistic information.In doing so,the remainder of this paper is set out as follows.In the next section,we introduce some basic concepts and operational laws of2-tuple linguistic variables. In Section3we develop some generalized weighted average oper-ator with linguistic assessment information.In Section4we devel-op some generalized ordered weighted average operator with linguistic assessment information.In Section5we develop some induced generalized ordered weighted average operator with lin-guistic assessment information.In Section6we apply the IG-2TOWA and G-2TWA operator to multiple attribute group decision making with linguistic assessment information.In Section7,we give an illustrative example about risk analysis to verify the devel-oped approach and to demonstrate its feasibility and practicality. In Section8we conclude the paper and give some remarks.2.PreliminariesLet S¼s if i¼1;2;...;tj g be a linguistic term set with odd cardi-nality.Any label,s i represents a possible value for a linguistic var-iable,and it should satisfy the following characteristics(Herrera& Martínez,2000a;Herrera&Martínez,2000b;Herrera&Martínez, 2001):(1)The set is ordered:s i>s j,if i>j;(2)Max operator:max(-s i,s j)=s i,if s i P s j;(3)Min operator:min(s i,s j)=s i,if s i6s j.For example,S can be defined asS¼f s1¼extremely poor;s2¼very poor;s3¼poor;s4¼medium;s5¼good;s6¼very good;s7¼extremely good gHerrera and Martinez(2000a,2000b)developed the2-tuple fuzzy linguistic representation model based on the concept of sym-bolic translation.It is used for representing the linguistic assess-ment information by means of a2-tuple(s i,a i),where s i is a linguistic label from predefined linguistic term set S and a i is the value of symbolic translation,and a i2½À0:5;0:5Þ.Definition1.Let b be the result of an aggregation of the indices of a set of labels assessed in a linguistic term set S,i.e.,the result of a symbolic aggregation operation,b e[1,t],and t the cardinality of S. Let i=round(b)and a=bÀi be2values,such that i e[1,t]and a2À0:5;0:5Þ½;then a is called a symbolic translation(Herrera& Martínez,2000a;Herrera&Martínez,2000b).Definition 2.Let S={s1,s2,...,s t}be a linguistic term set and b e[1,t]be a value representing the result of a symbolic aggrega-tion operation;then2-tuple that expresses the equivalent informa-tion to b is obtained with the following function:D:½1;t !S½À0:5;0:5Þð1ÞDðbÞ¼s i;i¼roundðbÞalpha¼bÀi;a2½À0:5;0:5Þ&ð2Þwhere round(Á)is the usual round operation,s i has the closest index label to b and a is the value of the symbolic translation(Herrera& Martínez,2000a,2000b).Definition 3.Let S={s1,s2,...,s t}be a linguistic term set and (s i,a i)be a2-tuple;a function DÀ1can be defined,such that,from a 2-tuple(s i,a i)it return its equivalent numerical value b e[1,t]&R, which is obtained with the following function:(Herrera&Martínez, 2000a;Herrera&Martínez,2000b)DÀ1:SÂÀ0:5;0:5Þ½!½1;t ð3ÞDÀ1ðs i;aÞ¼iþa¼bð4ÞDefinition4.(Herrera&Martínez,2000a).Let(s k,a k)and(s l,a l)be two2-tuples,with each one representing a linguistic assessment:(1)If k<l then(s k,a k)is smaller than(s l,a l).(2)If k=l then(i)if a k=a l,then(s k,a k)=s l,a l);(ii)if a k<a l then(s k,a k)<(s l,a l);(iii)if a k>a l then(s k,a k)>(s l,a l).G.-W.Wei/Computers&Industrial Engineering61(2011)32–38333.Generalized weighted average operator with linguistic informationZhao,Xu,Ni,and Liu (2010)proposed the generalized weighted average (GWA)operator which is the generalization of the WA operator.Definition 5.A GWA operator of dimension n is a mapping GWA:R n ?R ,such thatGWA ða 1;a 2;...;a n Þ¼Xn j ¼1x j a k j!1=kð5Þwhere x =(x 1,x 2,...,x n )T be the weight vector ofa j (j =1,2,...,n ),and x j >0,Pn j ¼1x j ¼1and k is a parameter such that k 2ðÀ1;þ1Þ.It’s obvious that GWA operator include a wide range of aggrega-tion operators such as the WA,the weighted geometric (WG)oper-ator,the weighted harmonic average (WHA)operator (Chen,Liu,&Sheng,2004),the weighted quadratic average (WQA),and a lot of other cases.In the following,we extend the GWA operator to linguistic envi-ronment and develop the generalized 2-tuple weighted average (G-2TWA)operator.Definition 6.Let {(r 1,a 1),(r 2,a 2),...,(r n ,a n )}be a set of 2-tuple,An G-2TWA operator of dimension n is a mapping G-2TWA:S n ?S ,furthermore,G-2TWA ððr 1;a 1Þ;ðr 2;a 2Þ;...;ðr n ;a n ÞÞ¼D X n j ¼1x j D À1ðr j ;a j Þk!1=k 0@1A ð6Þwhere x =(x 1,x 2,...,x n )T be the weight vector of (r j ,a j )(j =1,2,...,n ),and x j >0,P nj ¼1x j ¼1,and k is a parameter such that k 2ðÀ1;þ1Þ.Now we consider four special cases of the G-2TWA operator:(1)When k ¼1,the G-2TWA operator become the 2TWA oper-ator(Herrera &Martínez,2000a).2TWA ððr 1;a 1Þ;ðr 2;a 2Þ;...;ðr n ;a n ÞÞ¼DX n j ¼1x j D À1ðr j ;a j Þ!ð7ÞIn particular,if x j ¼1;for all (r j ,a j ),we get the 2TA operator.(2)When k !0,the G-2TWA operator become the 2TWGoperator.2TWG ððr 1;a 1Þ;ðr 2;a 2Þ;...;ðr n ;a n ÞÞ¼DY n j ¼1ðD À1ðr j ;a j ÞÞx j!ð8ÞIn particular,if x j ¼1n;for all (r j ,a j ),we get the 2TG operator.(3)When k ¼À1,the G-2TWA operator become the 2TWHAoperator.2TWHA ððr 1;a 1Þ;ðr 2;a 2Þ;...;ðr n ;a n ÞÞ¼D 1P nj ¼1x jD À1ðr j ;a j ÞB BB @1C C C Að9ÞIn particular,if x j ¼1n;for all (r j ,a j ),we get the 2THA operator.(3)When k ¼2,the G-2TWA operator become the 2TWQAoperator.2TWQA ððr 1;a 1Þ;ðr 2;a 2Þ;...;ðr n ;a n ÞÞ¼D X n j ¼1x j D À1ðr j ;a j Þ2!1=20@1Að10ÞIn particular,if x j ¼1n ;for all (r j ,a j ),we get the 2TQA operator.4.Generalized ordered weighted average operator with linguistic informationThe GOWA operator (Yager,2004)is a generalization of the OWA operator (Yager,1988,Yager,2007a,2007b;Chen,Liu and Sheng,2004;Liu,2006a,2006b,2007;Wu,Li,Li,&Duan,2009)by using generalized means.It can be defined as follows.Definition 7.A GOWA operator of dimension n is a mapping GOWA:R n ?R that has an associated weight vectorw =(w 1,w 2,...,w n )T such that w j >0and Pn j ¼1w j ¼1.Furthermore,GOWA ða 1;a 2;...;a n Þ¼X n j ¼1w j a k r ðj Þ!1=kð11Þwhere (r (1),r (2),ÁÁÁ,r (n ))is a permutation of (1,2,ÁÁÁ,n ),suchthat a r ðj À1ÞP a r ðj Þfor all j =2,ÁÁÁ,n and k is a parameter such that k 2ðÀ1;þ1Þ:It’s obvious that GOWA operator include a wide range of aggregation operators such as the OWA operator (Yager,1988),the ordered weighted geometric (OWG)operator (Chiclana,Herrera,&Herrera-Viedma,2000;Xu &Da,2003),the ordered weighted harmonic average (OWHA)operator (Chen,Liu and Sheng,2004),the ordered weighted quadratic average (OWQA),and a lot of other cases.In the following,we extend the GOWA operator to linguistic environment and develop the generalized 2-tuple weighted aver-age (G-2TOWA)operator.Definition 8.Let {(r 1,a 1),(r 2,a 2),...,(r n ,a n )}be a set of 2-tuple,An G-2TOWA operator of dimension n is a mapping G-2TOWA:S n ?S that has an associated weight vector w =(w 1,w 2,...,w n )Tsuch that w j >0and Pn j ¼1w j ¼1.Furthermore,G-2TOWA ððr 1;a 1Þ;ðr 2;a 2Þ;...;ðr n ;a n ÞÞ¼D Xn j ¼1w j D À1ðr r ðj Þ;a r ðj ÞÞk!1=k 0@1A ð12Þwhere (r (1),r (2),ÁÁÁ,r (n ))is a permutation of (1,2,ÁÁÁ,n ),suchthat ðr r ðj À1Þ;a r ðj À1ÞÞP ðr r ðj Þ;a r ðj ÞÞfor all j =2,ÁÁÁ,n ,and k is a param-eter such that k 2ðÀ1;þ1Þ:Now we consider four special cases of the G-2TOWA operator:(1)When k ¼1,the G-2TOWA operator reduces to the 2TOWAoperator(Herrera &Martínez,2000a).2TOWA ððr 1;a 1Þ;ðr 2;a 2Þ;...;ðr n ;a n ÞÞ¼DX n j ¼1w j D À1ðr r ðj Þ;a r ðj ÞÞ!ð13Þ(2)When k !0;the G-2TOWA operator reduces to the 2TOWGoperator (Jiang &Fan,2003).2TOWG ððr 1;a 1Þ;ðr 2;a 2Þ;...;ðr n ;a n ÞÞ¼DY n j ¼1ðD À1ðr r ðj Þ;a r ðj ÞÞÞw j!ð14Þ(3)When k ¼À1;the G-2TOWA operator reduces to the 2TOW-HA operator.2TOWHA ððr 1;a 1Þ;ðr 2;a 2Þ;...;ðr n ;a n ÞÞ¼D 1Pj ¼1w jD ðr r ðj Þ;a r ðj ÞÞBBB @1C C C Að15Þ34G.-W.Wei /Computers &Industrial Engineering 61(2011)32–38(4)When k ¼2;the G-2TOWA operator reduces to the 2TOWQAoperator.2TWQA ððr 1;a 1Þ;ðr 2;a 2Þ;...;ðr n ;a n ÞÞ¼D Xn j ¼1w j D À1ðr r ðj Þ;a r ðj ÞÞ2!1=20@1A ð16Þ5.Induced generalized ordered weighted average operator withlinguistic informationThe induced generalized OWA (IGOWA)operator was intro-duced by Merigóand Gil-Lafuente (2009)and it represents an extension of the GOWA operator,with the difference that the reor-dering step of the IGOWA operator is not defined by the values of the arguments a i ,but rather by order-inducing variables u i ,where the ordered position of the arguments a i depends upon the values of the u i .It can be defined as follows:Definition 9.An IGOWA operator of dimension n is a mapping IGOWA:R n ?R defined by an associated weight vectorw =(w 1,w 2,...,w n )T such that w j >0and P nj ¼1w j ¼1,a set of order-inducing variables u i ,and a parameter k 2½À1;þ1 ,accord-ing to the following formula:IGOWA ðh u 1;a 1i ;h u 2;a 2i ;ÁÁÁ;h u n ;a n iÞ¼X n j ¼1w j a k r ðj Þ!1=kð17Þa r (j )is the a i value of the GOWA pair h u i ,a i i having the j th largestu i (u i e [0,1]),and u i in h u i ,a i i is referred to as the order-inducing var-iable and a i are the argument variables.It’s obvious that IGOWA operator include a wide range of aggre-gation operators such as the IOWA operator (Yager &Filev,1999),the induced ordered weighted geometric (IOWG)operator (Chicl-ana,Herrera,Herrera-Viedma,&Alonso,2004;Xu &Da,2003),the induced ordered weighted harmonic average (IOWHA)opera-tor (Chen,2004),the induced ordered weighted quadratic average (IOWQA),and a lot of other cases.In the following,we shall extend the IGOWA operators to accommodate the situations where the input arguments are lin-guistic variables and develop the induced generalized 2-tuple or-dered weighted averaging (IG-2TOWA)operator.Definition 10.Let (h u 1,(r 1,a 1)i ,h u 2,(r 2,a 2)i ,...,h u n ,(r n ,a n )i )be a set of 2-tuple,An IG-2TOWA operator of dimension n is a mapping IG-2TOWA:S n ?S ,furthermore,IG-2TOWA w ðh u 1;ðr 1;a 1Þi ;h u 2;ðr 2;a 2Þi ;ÁÁÁ;h u n ;ðr n ;a n ÞiÞ¼D Xn j ¼1w j D À1ðr r ðj Þ;a r ðj ÞÞk !1=k 0@1A ð18Þwhere w =(w 1,w 2,ÁÁÁ,w n )T is a weighting vector,such that w j >0,P nj ¼1w j ¼1,j =1,2,ÁÁÁ,n ,(r r (j ),a r (j ))is the (r i ,a i )value of the IG-2TOWA pair h u i ,(r i ,a i )i having the jth largest u i (u i e [0,1]),and u i in h u i ,(r i ,a i )i is referred to as the order-inducing variable and (r i ,a i )as the linguistic variables.Now we consider four special cases of the IG-2TOWA operator:(1)When k ¼1,the IG-2TOWA operator reduces to the I-2TOWA operator.I-2TOWA w ðh u 1;ðr 1;a 1Þi ;h u 2;ðr 2;a 2Þi ;ÁÁÁ;h u n ;ðr n ;a n ÞiÞ¼DPn j ¼1w j D À1ðr r ðj Þ;a r ðj Þ !ð19Þ(2)When k !0;the IG-2TOWA operator reduces to the I-2TOWG operator.I-2TOWG w ðh u 1;ðr 1;a 1Þi ;h u 2;ðr 2;a 2Þi ;ÁÁÁ;h u n ;ðr n ;a n ÞiÞ¼D Y n j ¼1D À1ðr r ðj Þ;a r ðj ÞÞÀÁw j!ð20Þ(3)When k ¼À1;the IG-2TOWA operator reduces to the I-2TOWHA operator.I-2TOWHA w ðh u 1;ðr 1;a 1Þi ;h u 2;ðr 2;a 2Þi ;ÁÁÁ;h u n ;ðr n ;a n ÞiÞ¼D 1Pnj ¼1w jD À1ðr r ðj Þ;a r ðj ÞÞ0BB B @1C C C Að21Þ(4)When k ¼2;the IG-2TOWA operator reduces to the I-2TOW-QA operator.I-2TOWQA w ðh u 1;ðr 1;a 1Þi ;h u 2;ðr 2;a 2Þi ;ÁÁÁ;h u n ;ðr n ;a n ÞiÞ¼D Xn j ¼1w j D À1ðr r ðj Þ;a r ðj ÞÞ2!1=20@1A ð22ÞEspecially,if w =(1/n ,1/n ,...,1/n )T ,then IG-2TOWA is reduced to the generalized 2-tuple averaging (G-2TA)operator;if u i =i ,forall i ,where i is the ordered position of the ~ai ,the IG-2TOWA is re-duced to the generalized 2-tuple weighted averaging (G-2TWA)operator;if u i ¼~ai ,for all i ,then IG-2TOWA is reduced to the gen-eralized 2-tuple ordered weighted averaging (G-2TOWA)operator.6.An approach to multiple attribute group decision making with linguistic assessment informationLet A ={A 1,A 2,...,A m }be a discrete set of alternatives,and G ={G 1,G 2,...,G n }be the set of attributes,x =(x 1,x 2,...,x n )is the weighting vector of the attributes G j (j =1,2,...,n ),wherex j e [0,1],Pn j ¼1x j ¼1.Let D ={D 1,D 2,...,D t }be the set of decision makers,and m =(m 1,m 2,...,m t )be the weight vector of decisionmakers,where m k e [0,1],P tk ¼1m k ¼1.Suppose that ~R k ¼ð~r ðk Þij Þm Ânis the decision matrix,where ~r ðk Þij 2~S is a preference value,which takes the form of linguistic variables,given by the decision maker D k e D ,for the alternative A i e A with respect to the attribute G j e G .To get the best alternative(s),the follows steps are involved:In the following,we apply the IG-2TOWA and G-2TWA operator to multiple attribute group decision making with linguistic assess-ment information.The method involves the following steps:Step 1.Transforming linguistic decision matrix ~R k ¼ð~r ðk Þij Þm Âninto 2-tuple linguistic decision matrix ~R k ¼ð~r ðk Þij Þm Ân ¼ðr ðk Þij ;0Þm Ân .Step 2.Utilize the decision information given in matrix ~Rk ,and the IG-2TOWA operator which has associated weighting vector w =(w 1,w 2,ÁÁÁ,w t )T~r ij ¼ðr ij ;a ij Þ¼IG-2TOWA w ðh m 1;ð~r ð1Þij ;0Þi ;h m 2;ð~r ð2Þij ;0Þi ;ÁÁÁ;h m t ;ð~r ðt Þij ;0ÞiÞ;i ¼1;2;...;m ;j ¼1;2;...;n :ð23Þto aggregate all the decision matrices ~Rk ðk ¼1;2;...;t Þinto a collec-tive decision matrix ~R ¼ð~r ij Þm Ân ,where m =(m 1,m 2,...,m t )be theweighting vector of decision makers.Step 3.Utilize the decision information given in matrix ~R,and the G-2TWA operator~r i ¼ðr i ;a i Þ¼G2TWA x ð~r i 1;~r i 2;...;~r in Þ;ði ¼1;2;...;m Þð24Þto derive the collective overall preference values ~r i ði ¼1;2;...;m Þof the alternative A i ,where x =(x 1,x 2,...,x n )Tis the weighting vector of the attributes.G.-W.Wei /Computers &Industrial Engineering 61(2011)32–3835Step4.Rank all the alternatives A i(i=1,2,...,m)and select the best one(s)in accordance with~r iði¼1;2;...;mÞ.If any alternative has the highest~r i value,then,it is the most important alternative.Step5.End.7.Illustrative exampleLet us suppose there is an investment company,which wants to invest a sum of money in the best option(adapted from Herrera and Martínez(2000b)).There is a panel withfive possible alternatives to invest the money:r A1is a car company;s A2is a food company; t A3is a computer company;u A4is an arms company;v A5is a TV company.The investment company must take a decision accord-ing to the following six attributes:r G1is thefinancial risk;s G2is the technical risk;t G3is the production risk;u G4is the market risk;v G5is the management risk;w G6is the environment risk. Thefive possible alternatives A iði¼1;2;...;5Þare to be evaluated using the linguistic term setS¼f s1¼extremely poor;s2¼very poor;s3¼poor;s4¼medium;s5¼good;s6¼very good;s7¼extremely good gby the three decision makers D kðk¼1;2;3Þ(whose weighting vec-tor m=(0.35,0.40,0.25)T)under the above six attributes,and con-struct,respectively,the linguistic decision matrices are shown in Tables1–3.In the following,we shall utilize the proposed approach in this paper getting the most desirable alternative(s):Firstly,transforming linguistic decision information given in Ta-bles1–3into2-tuple linguistic decision matrix and utilizing the IG-2TOWA operator which has associated weighting vector w=(0.30, 0.50,0.20)T,the aggregating results are shown in Tables4–7.Secondly,by utilizing the decision information given in Tables 4–7,and the G-2TWA operators,x=(0.12,0.15,0.18,0.25,0.2, 0.1)T is the weighting vector of the attributes,we derive the collec-tive overall preference values of the alternatives.The aggregating results are shown in Table8.Finally,according to the aggregating results shown in Table8, the ordering of the alternatives are shown in Table9.Note that> means‘‘preferred to’’.As we can see,depending on the aggregation operators used,the ordering of the strategies is slightly different. Therefore,depending on the aggregation operators used,the re-sults may lead to different decisions.However,the best alternative is A3.From the above analysis,wefind an interesting phenomena that there are some common advantages in these operators:(1)From Definitions6and8,we know that the G-2TWA operator weights the linguistic arguments while the G-2TOWA operator weights the ordered positions of the linguistic arguments instead of weighting the arguments themselves;From Definitions10,we know that the main difference of the IG-2TOWA operator is that the reordering step of the IG-2TOWA is carried out with order-inducing variables,rather than depending on the values of the lin-guistic arguments.(2)Both the G-2TWA and the G-2TOWA opera-tors are special case of the IG-2TOWA operator.However,we also find difference among these operators,for the same multiple attri-bute group decision making problems with linguistic information, if we emphasize the individual influence,the methods based on the geometric aggregating operators are available;if we emphasize the group’s influence,the methods based on the arithmetic aggregat-ing operators are available;if we emphasize to aggregate central tendency data with linguistic information,the methods based on the harmonic aggregating operators are available.8.ConclusionThe traditional generalized aggregation operators and induced generalized aggregation operators are generally suitable for aggre-gating the information taking the form of numerical values,and yet they will fail in dealing with linguistic information.In this paper, with respect to multiple attribute group decision making(MAG-DM)problems in which both the attribute weights and the expert weights take the form of real numbers,attribute values take the form of linguistic variables,some new decision making analysis methods are developed.Firstly,some operational laws of linguistic variables are introduced.Then,we develop three new aggregation operators:generalized2-tuple weighted average(G-2TWA)opera-tor,generalized2-tuple ordered weighted average(G-2TOWA) operator and induced generalized2-tuple ordered weightedTable1Decision matrix~R1.G1G2G3G4G5G6A1P M VP VP EP PA2VP EP G G VP MA3VG G VG EG M VGA4EG VP VP M VG GA5P VP M VP P VGTable2Decision matrix~R2.G1G2G3G4G5G6A1M VP P P M VPA2P VP M P VG MA3G EG G EG G VGA4VG P P G M GA5EG EP VP M P EPTable3Decision matrix~R3.G1G2G3G4G5G6A1G P VP M EP EPA2VP G P G M MA3VG EG G EG G VGA4G VG EG VP EG PA5M VP M VP P EPTable4Decision matrix~R(k¼1).G1G2G3G4G5G6A1(M,À0.30)(P,0.20)(VP,0.30)(P,À0.30)(VP,À0.10)(VP,0.30) A2(VP,0.30)(VP,0.10)(M,0.30)(M,0.40)(M,À0.40)(M,0.00) A3(VG,À0.30)(VG,0.00)(VG,À0.10)(EG,0.00)(G,À0.50)(VG,0.00) A4(VG,0.30)(P,0.10)(P,0.30)(M,À0.10)(VG,À0.40)(G,À0.40) A5(M,0.40)(VP,À0.30)(P,0.40)(P,À0.40)(P,0.00)(M,À0.50) 36G.-W.Wei/Computers&Industrial Engineering61(2011)32–38。