Fourier Spectrum Analysis of the New Solar Neutrino Capture Rate Data for the Homestake Exp
傅里叶变换红外光谱仪英文

傅里叶变换红外光谱仪英文Fourier Transform Infrared SpectrometerIntroduction:The Fourier Transform Infrared (FTIR) spectrometer is an essential tool in the field of spectroscopy. It utilizes the mathematical technique known as Fourier transform to analyze infrared light, enabling scientists to study the molecular composition and structure of various substances. In this article, we will explore the principles behind the Fourier Transform Infrared Spectrometer and its applications in scientific research.Principles of Fourier Transform Infrared Spectroscopy:Fourier Transform Infrared Spectroscopy is based on the interaction between infrared light and matter. When a substance is exposed to infrared radiation, the energy absorbed by the molecules causes them to vibrate. These vibrations are specific to each molecule and are dependent on the molecular bonds present within the substance.The spectrometer operates by passing an infrared beam through the sample and measuring the amount of light absorbed at different wavelengths. This absorption spectrum is then transformed using Fourier analysis, producing a highly detailed and accurate representation of the substance's molecular structure.Advantages of Fourier Transform Infrared Spectroscopy:1. High Speed and Sensitivity: Fourier Transform Infrared Spectroscopy offers rapid analysis times due to its ability to gather a full range ofwavelengths simultaneously. This allows for efficient data collection, making it ideal for high-throughput applications. Additionally, the technique is highly sensitive, capable of detecting even small quantities of sample material.2. Broad Analytical Range: FTIR spectroscopy covers a wide range of wavelengths, from near-infrared (NIR) to mid-infrared (MIR). This versatility enables the analysis of various substances, including organic and inorganic compounds, polymers, pharmaceuticals, and biological samples.3. Non-destructive Analysis: One of the key advantages of FTIR spectroscopy is that it is a non-destructive technique. Samples do not require any special preparation and can be analyzed directly, allowing for subsequent analysis or retesting if required.Applications of Fourier Transform Infrared Spectrometers:1. Pharmaceutical Analysis: FTIR spectroscopy plays a vital role in drug discovery and development. It is used to identify and characterize the molecular composition of active pharmaceutical ingredients (APIs), excipients, and impurities. By comparing spectra, scientists can ensure the quality and purity of pharmaceutical products.2. Environmental Analysis: Fourier Transform Infrared Spectrometers are employed in environmental monitoring to analyze air, water, and soil samples. It aids in detecting pollutants, identifying unknown substances, and assessing the impact of human activities on the environment.3. Forensic Science: FTIR spectroscopy has proven to be a valuable tool in forensic science. It assists in the analysis of various evidence, such asfibers, paints, and drugs. FTIR spectra can provide crucial information in criminal investigations, helping to identify unknown substances and link them to potential sources.4. Food and Beverage Industry: The FTIR spectrometer allows for the analysis of food quality, safety, and authenticity. It can identify contaminants, detect adulteration, and verify product labeling claims. Both raw materials and finished products can be analyzed using this technique, ensuring compliance with industry regulations.Conclusion:The Fourier Transform Infrared Spectrometer has revolutionized the field of spectroscopy by providing accurate and detailed information about a substance's molecular structure. Its speed, sensitivity, and versatility make it a crucial analytical tool in various scientific disciplines. With ongoing advancements in technology, FTIR spectroscopy continues to contribute to new discoveries and advancements in research.。
抗坏血酸升温氧化过程的二维相关红外光谱分析

抗坏血酸升温氧化过程的二维相关红外光谱分析华 瑞 孙素琴3 周 群徐永群(清华大学化学系,北京100084)(黄冈师范学院化学系,黄冈438000)摘 要 采用傅里叶变换红外光谱法(FTIR )和二维相关分析(2D C orrelation Analysis )技术研究了固态抗坏血酸在20℃~160℃的升温氧化过程。
结果表明:抗坏血酸随着温度升高的红外光谱的特征峰在一维图上变化不明显。
借助于二维相关分析,不仅提高了谱图的分辨率,将一维红外谱图上1674cm -1处的单峰分解为两个自相关峰,显示了抗坏血酸的互变异构体中酮式结构的C O 基团和醇式结构的C C 基团的振动;还揭示了分子内各官能团之间的相互作用,反映了在升温氧化过程中这些基团之间的协同关系和变化的先后顺序。
抗坏血酸分子中C O 、C C 和C O C 基团发生了相互作用,其变化的顺序是C C 先于C O C发生偶极矩的变化,最后是C O 发生变化,此现象与抗坏血酸的氧化反应机理相一致。
总之,二维相关红外分析可以作为研究抗坏血酸升温氧化过程中结构动态变化的一种新方法,也为抗坏血酸在氧化过程中的机理研究提供了一个重要的理论依据。
关键词 二维相关分析,红外光谱,抗坏血酸,氧化 2002203223收稿;2002209230接受本文系国家中医药管理局科技重大资助项目(国中医药科2001Z DZX 01)1 引 言1986年,Noda 提出并构建了二维红外光谱(2D IR S pectra )的概念1。
在二维红外光谱中,谱图是将两个独立的波数轴的红外谱经过数学相关分析而得到的,根据二维图上的谱峰可以分析在外部扰动下分子内基团的变化。
二维光谱有两个优点:一是可以得到在实验过程中分子内及分子间不同基团之间相互变化的相互作用及先后关系,二是提高谱图的分辨率。
最初用来进行二维红外光谱分析的外部扰动都是与时间有关的,后来扩展到更加广义的扰动,且微扰可以是光、热、电、磁、化学或者机械的多种方式2~4。
电压闪变与波动的外文翻译

Measurement of a power system nominal voltage, frequency and voltage flicker parametersA.M. Alkandari a, S.A. Soliman b,*a b s t r a c t:We present, in this paper, an approach for identifying the frequency and amplitude of voltage flicker signal that imposed on the nominal voltage signal, as well as the amplitude and frequency of the nominal signal itself. The proposed algorithm performs the estimation in two steps; in the first step the original voltage signal is shifted forward and backward by an integer number of sample, one sample in this paper.The new generated signals from such a shift together with the original one is used to estimate the amplitude of the original signal voltage that composed of the nominal voltage and flicker voltage. The average of this amplitude gives the amplitude of the nominal voltage; this amplitude is subtracted from the original identified signal amplitude to obtain the samples of the flicker voltage. In the second step, the argument of the signal is calculated by simply dividing the magnitude of signal sample with the estimated amplitude in the first step. Calculating the arccosine of the argument, the frequency of the nominal signal as well as the phase angle can be computing using the least error square estimation algorithm. Simulation examples are given within the text to show the features of the proposed approach.Keywords: Power quality Nominal frequency and amplitude measurements Voltage flicker Frequency and amplitude estimation Forward and backward difference1.IntroductionV oltage flicker and harmonics are introduced to power system as a result of arc furnace operation, and power utilities are concern about their effects. As such an accurate model for the voltage flicker is needed. The definition of voltage flicker in IEEE standards is the ‘‘impression of fluctuating brightness or color, when the frequency observed variation lies between a few hertz and the fusion frequency of image” [1]. The flicker phenomenon may be divided into two general categories, cyclic flicker and non-cyclic flicker. Cyclic flicker is repetitive one and is caused by periodic voltage fluctuations due to the operation of loads such as spot welders, compressors, or arc welders. Non-cyclic flicker corresponds to occasional voltage fluctuations, such as starting of large motors, some of loads will cause both cyclic andnon-cyclic flicker, such as arc furnace, welder, and ac choppers.Over the past three decades, many digital algorithms have been developed and tested to measure power system frequency and rate of change of frequency. Ref. [2] presents the application of the continuous Wavelet transform for power quality analysis. The transform appears to be reliable for detecting and measuring voltage sags, flicker and transients in power quality analysis. Ref. [3] pays attention to the fast Fourier transform and its pitfalls. A low pass.digital filter is used, and the effects of system voltage deviation on the voltage - flicker measurements by direct FFT are studied. The DC component leakage effect on the flicker components in the spectrum analysis of the effective value of the voltage and the windowing effect on the data acquisition of the voltage signal are discussed as well.A digital flicker meter is proposed in Ref. [6] based on forward and inverse FFT and on filtering, in the frequency domain, for the implementation of the functional blocks of simulation of lamp–eye–brain response. Refs. [5–7] propose a method based on Kalman filtering algorithms to measure the low frequency modulation of the 50/60 Hz signal. The method used, in these references, allows for random and deterministic variation of the modulation. The approach utilizes a combination of linear and non-linear Kalman filter modes.Ref. [8] presents a method for direct calculation of flicker level from digital measurements of voltage waveforms. The direct digital implementation uses Fast Fourier transform (FFT) as the first step in computation. A pruned FFT, customized for the flicker level computation, is also proposed. Presented in Ref. [9] is a static state estimation algorithm based on least absolute value error (LA V) for measurement of voltage flicker level. The waveform for the voltage signal is assumed to have, for simplicity, one flicker component. This algorithm estimates accurately the nominal voltage waveform and the voltage flicker component. An application of continuous wavelet transform (CWT) for analysis of voltage flicker-generated signals is proposed in Ref. [10] With the time-frequency localization characteristics embedded in the wavelets, the time and frequency information of a waveform can be integrally presentedRef. [11] presents an arc furnace model that implemented in the Simulink environment by using chaotic and deterministic elements. This model is obtained by solving the corresponding differential equation, which yields dynamic and multivalued v i characteristics of the arc furnace load. In order to evaluate the flicker in the simulated arc furnace voltage, the IEC flicker meter is implemented based on the IEC 1000-4-15 standard in Matlab environment.Ref. [12] presents an approach to estimate voltage flicker components magnitudes and frequencies, based on Lp norms (p = 1,2 and 1) and Taylor’s’ series linearization. It has been found that it is possible to design an Lp estimator to identify flicker frequency and amplitude from time series measurements. The Teager energy operator (TEO) and the Hilbert transform (HT) are introduced in Ref. [13] as effective approaches for tracking the voltage flicker levels. It has been found that TEO and HT are capable of tracking the amplitude variations of the voltage flicker and supply frequency in industrial systems with an average error 3%.Ref. [14] presents a control technique for flicker mitigation. This technique is based on the instantaneous tracking of the measured voltage envelope. The ADALINE (ADAptive LINear) neuron algorithm and the Recursive Least Square (RLS) algorithm are introduced for the flicker envelope tracking. In Ref. [15], an algorithm for tracking the voltage envelope based on calculating the energy operator of a sinusoidal waveform is presented. It is assumed that the frequency of the sinusoidal waveform is known and a lead-lag network with unity gain is used. Ref. [16] develops an enhanced method for estimating the voltage fluctuation (DV10) of the electric arc furnace (EAF). The method proposed considers the reactive power variation and also the active power variation in calculating DV10 value of ac and dc LEAFsControl and protection of power systems requires accurate measurement of system frequency. A system operates at nominal frequency, 50/60 Hz means a balance in the active power, i.e. The power generated equals the demand power plus losses. Imbalance in the active power causes the frequency to change. A frequency less than the nominal frequency means that the demand load plus losses are greater than the power generated, but a frequency greater than nominal frequency means that the system generation is greater than the load demand plus losses. As such, frequency can be used as a measure of system power balance.Ref. [17] presents a numerical differentiation-based algorithm for high-accuracy, wide-range frequency estimation of power systems. The signal, using this algorithm, includes up to 31st-order harmonic components. Ref. [18] presents a method for estimation of power frequency and its rate of change. The proposed methodaccommodates the inherent non-linearity of the frequency estimation problem. The estimator is based on a quadrature phase-look loop concept.An approach for designing a digital algorithm for local system frequency estimation is presented in Ref. [19]. The algorithm is derived using the maximum likelihood method. A recursive Newtontype algorithm suitable for various measurement applications in power system is developed in Ref. [20] and is used for power system frequency and spectra estimation. A precise digital algorithm based on discrete Fourier transforms (DFT) to estimate the frequency of a sinusoid with harmonics in real-time is proposed in Ref. [21]. This algorithm is called smart discrete Fourier transform (SDFT) that avoids the errors due to frequency deviation and keeps all the advantages of the DFT.Ref. [22] presents an algorithm for frequency estimation from distorted signals. The proposed algorithm is based on the extended complex Kalman filter, which uses discrete values of a three-phase voltage that are transformed into the well-known ab-transform Using such a transformation a non-linear state space formulation is obtained for the extended Kalman filter. This algorithm is iterative and complex and needs much computing time and uses the three-phase voltage measurements, to calculate the power system voltage frequency.Ref. [23] describes design, computational aspect, and implementation aspects of a digital signal processing technique for measuring the operating frequency of a power system. It is suggested this technique produces correct and noise-free estimates for near nominal, nominal and off-nominal frequencies in about 25 ms, and it requires modest computation. The proposed technique uses per-phase digitized voltage samples and applies orthogonal FIR digital filters with the least errors square (LES) algorithm to extract the system frequency.Ref. [24] presents an iterative technique for measuring power system frequency to a resolution of 0.01–0.02 Hz for near nominal, nominal and off-nominal frequencies in about 20 ms. The algorithm in this reference uses per-phase digitized voltage samples together with a FIR filter and the LES algorithm to extract iteratively the signal frequency.This algorithm has beneficial features including fixed sampling rate, fixed data window size and easy implementationRefs. [25,26] present a new pair of orthogonal filters for phasor computation; the technique proposed extracts accurately the fundamental component of fault voltageand current signal. Ref. [27] describes an algorithm for power system frequency estimation. The algorithm, applies orthogonal signal component obtained with use of two orthogonal FIR filters. The essential property of the algorithm proposed is outstanding immunity to both signals orthogonal component magnitudes and FIR filter gain variations. Again this algorithm uses the per-phase digitized voltage samples.Ref. [28] presents a method of measuring the power system frequency, based on digital filtering and Prony’s estimation. The discrete Fourier transform with a variable data window is used to filter out the noise and harmonics associated with the signal. The results obtained using this algorithm are more accurate than when applying the method based on the measurement of angular velocity of the rotating voltage phasor. The response time of the proposed method equals to three to four periods of the fundamental components. This method uses also per phase digitized voltage samples to compute the system frequency from harmonics polluted voltage signal. Ref. [29] implements a digital technique for the evaluation of power system frequency. The algorithm is suitable for microprocessor implementation and uses only standard hardware. The algorithm works with any relative phase of the input signal and produces a new frequency estimate for every new input sample. This algorithm uses the orthogonal sine and cosine- filtering algorithm..A frequency relay, which is capable of under/over frequency and rate of change of frequency measurements using an instantaneous frequency-measuring algorithm, is presented in Ref. [30]. It has been shown that filtering the relay input signal could adversely affect the dynamic frequency evaluation response. Misleading frequency behavior is observed in this method, and an algorithm has been developed to improve this behavior. The under/over frequency function of the relay will cause it to operate within 30 ms.Digital state estimation is implemented to estimate the power system voltage amplitude and normal frequency and its rate of change. The techniques employed for static state estimation are least errors square technique [31–33], least absolute value technique [34–36]. While linear and non-linear Kalman filtering algorithms are implemented for tracking the system operating frequency, rate of change of frequency and power system voltage magnitude from a harmonic polluted environment of the system voltage at the relay location. Most of these techniques use the per-phasedigitized voltage samples, and assume that the three-phase voltages are balanced and contain the same noise and harmonics, which is not the case in real-time, especially in the distribution systems, where different single phase loads are supplied from different phases.An approach for identifying the frequency and amplitude of flicker signal that imposed on the nominal voltage signal, as well as the amplitude and frequency of the nominal signal itself is presented in this text. The proposed algorithm performs the estimation in two steps. While, in the first step the original signal is shifted forward and backward by an integer number of sample, one sample in this paper. The generated signals from such shift together with the original one are used to estimate the amplitude of the original voltage signal that composed of the nominal voltage and the flicker voltage, the average of this amplitude gives the amplitude of the nominal voltage. This amplitude is subtracted from the original identified amplitude to obtain the samples of the flicker voltage. In the second step, the argument of the signal is calculated by simply dividing the magnitude of signal sample with the estimated amplitude in step one. Computing the arccosine of the argument, the frequency of the nominal signal as well as the phase angle can be calculated using the least error square estimation algorithm. Simulation examples are given within the text to show the features of the proposed approach.2. Flicker voltage identificationGenerally speaking, the voltage during the time of flicker can be expressed as [2]:where AO is the amplitude of the nominal power system voltage, xO is the nominal power frequency, and /O is the nominal phase angle. Furthermore, Ai is the amplitude of the flicker voltage, xfi its frequency, and /fi its phase angle and M is the expected number of flicker voltage signal in the voltage waveform. This type of voltage signal is called amplitude modulated (AM) signal.2.1. Signal amplitude measurement::The first bracket in Eq. (1) is the amplitude of the signal, A(t) which can be written as:As such Eq. (1) can be rewritten asAssume that the signal is given forward and backward shift by an angle equals an integral number of the sampling angle. Then Eq. (3) can be written in the forward direction as:While for the backward direction, it can be written as:where h is the shift angle and is given byN is the number of samples required for the shift, fO is the signal frequency and m is the total number of samples over the data window size. Using Eqs. (4)–(6), one obtainsThe recursive equation for the amplitude A(k) is given by:Having identified the amplitude A(k), the amplitude of the nominal voltage signal of frequency x O can be calculated, just by taking the average over complete data window size as:Having identified the power signal amplitude AO, then the flicker voltage components can be determined by;This voltage flicker signal can be written as;where DT is the sampling time that is the reciprocal of the sampling frequency.2.2. Measurement of flicker frequencyWithout loss of generality, we assume that the voltage flicker signal has only one component given by, i = 1To determine the flicker amplitude Vf1(k) and the frequency xf1 from the available m samples, we may use the algorithm explained in Ref. [9]. The frequency is calculated fromWhile the amplitude can be calculated as:In the above equations v0 are the fist and second derivative of the flicker signal, they can be calculated, using the central forward and backward difference [9] as:2.3. Nominal voltage signal frequency and phase angleThe signal argument can be calculated fromwhere AR(k) is given byIn the above equation W0, u are the two parameters to be estimated from the available m samples of the argument AR(k). At least two samples are required for such a linear estimation.Eq. (17) can be written, for m samples, asIn vector form, Eq. (19) can be written as:where Z is m 1 measurements vector for the argument samples, H is m 2 measurements matrix the element of this matrix depend on the sampling time, sampling frequency, X is the 2 1 parameters vector to be estimated and f is m 1 error vector to be minimized. The minimum of f based on least error squares occurs when:The above two equations give directly the frequency and phase angle, in closed forms, for the signal under study. To have a practical approach those formulas should not be sensitive to noise and harmonics. One way to reduce those sensitivities is to use of least error squares algorithm, as we explained in Eq. (21), for the frequency estimation in the paper. In the following section we offer examples from the area of power system voltage flickers that can be considered as amplitude modulated signals.puter experimentsThe above algorithm is tested using amplitude modulated signal with one voltage flicker signal given by;The signal is sampled at 10000 Hz and is giving a forward shift and backward shift by one sample, h = 7.2 and 1000 samples are used. The power system voltage, 50 Hz signal, amplitude is estimated using the algorithm explained earlier, using Eqs. (8) and (9), and it has been found to that the proposed algorithm is succeeded in estimating this amplitude with great accuracy and is found be AO = 1.0. Fig. 1 gives the actualvoltage signal, the tracked signal and the voltage signal amplitude. Examining this figure reveals the following:The power voltage signal amplitude, 50 Hz, is almost 1 p.u., the average value of A(t), as that calculated using Eq. (9).The proposed technique tracked the actual signal exactly.The flicker signal frequency is estimated using 200 samples only with Eq. (13). Fig. 2 gives the estimated flicker voltage frequency at each sampling step. Examining this figure reveals that the proposed algorithm estimates the flicker frequency with great accuracy. The spikes, in these curves, are due to the value of the voltage flicker signal at this time of sampling which is very small, and looking to Eq. (13) one can notice that to calculate the frequency we divide by this value ceeded to estimate the flicker amplitude, except at the points of spikes, as we explained earlier in the frequency estimation.Another example has been solved, where the voltage signal has two flicker signals with different amplitude and frequency. The voltage signal in this case is given by The signal is sampled at 500 Hz and is giving a forward shift and backward shift by one sample, h = 7.2 and 500 samples are used. The voltage nominal amplitude is estimated using the technique explained earlier and has found to be one per unit, and the tracking voltage, using this technique, tracks the signal exactly as shown in Fig. 4.4.ConclusionsAn approach for identifying the frequency and amplitude of flicker signal that imposed on the nominal voltage signal, as well as the amplitude and frequency of the nominal signal itself is presented in this paper. The proposed algorithm performs the estimation in two steps; in the first step the original signal is shifted forward and backward by an integer number of sample, at least one sample. The new generated signals from such a shift together with the original one is used to estimate the amplitude of the original voltage signal that composed of the nominal voltage and theflicker voltage. The average of this amplitude gives the amplitude of the nominal voltage; this amplitude is subtracted from the original identified amplitude to obtain the samples of the flicker voltage. The frequency of the flicker voltage is calculated using the forward and backward difference for the first and second derivatives for the voltage flicker signal.In the second step, the argument of the signal is calculated by simply dividing the magnitude of signal sample with the estimated amplitude in step one. Calculating the arccosine of the argument, the frequency of the nominal signal as well as the phase angle can be calculated using the least error square estimation algorithm. Simulation examples are given. It has been shown that the proposed algorithm is succeeded in estimating the voltage flicker frequency and amplitude as well as the amplitude and frequency of the power voltage signal.The proposed algorithm can be used off-line as well as on-line. In the on-line mode we recommend the usage of a digital lead- lag circuit. Wile in the off-line mode; just shift the registration counter on sample in the backward direction and another on in the forward direction to obtain the required sample of the data window size.电力系统的额定电压,频率和电压闪变参数的测量摘要我们提出,在本文中,用于识别施加于标称电压信号电压闪变信号,以及标称信号本身的振幅和频率的频率和幅度的方法。
信号与系统目录(Signal and system directory)
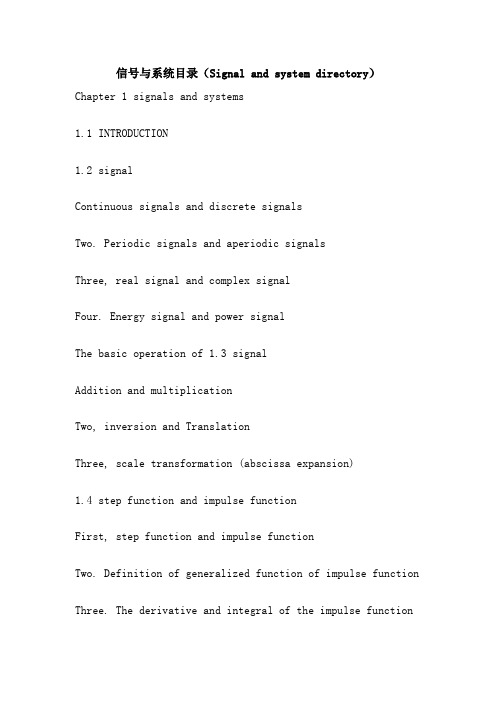
信号与系统目录(Signal and system directory)Chapter 1 signals and systems1.1 INTRODUCTION1.2 signalContinuous signals and discrete signalsTwo. Periodic signals and aperiodic signalsThree, real signal and complex signalFour. Energy signal and power signalThe basic operation of 1.3 signalAddition and multiplicationTwo, inversion and TranslationThree, scale transformation (abscissa expansion)1.4 step function and impulse functionFirst, step function and impulse functionTwo. Definition of generalized function of impulse functionThree. The derivative and integral of the impulse functionFour. Properties of the impulse functionDescription of 1.5 systemFirst, the mathematical model of the systemTwo. The block diagram of the systemCharacteristics and analysis methods of 1.6 systemLinearTwo, time invarianceThree, causalityFour, stabilityOverview of five and LTI system analysis methodsExercise 1.32The second chapter is the time domain analysis of continuous systemsThe response of 2.1LTI continuous systemFirst, the classical solution of differential equationTwo, about 0- and 0+ valuesThree, zero input responseFour, zero state responseFive, full response2.2 impulse response and step responseImpulse responseTwo, step response2.3 convolution integralConvolution integralTwo. The convolution diagramThe properties of 2.4 convolution integralAlgebraic operations of convolutionTwo. Convolution of function and impulse function Three. Differential and integral of convolutionFour. Correlation functionExercise 2.34The third chapter is the time domain analysis of discretesystemsThe response of 3.1LTI discrete systemsDifference and difference equationsTwo. Classical solutions of difference equationsThree, zero input responseFour, zero state response3.2 unit sequence and unit sequence responseUnit sequence and unit step sequenceTwo, unit sequence response and step response3.3 convolution sumConvolution sumTwo. The diagram of convolution sumThree. The nature of convolution sum3.4 deconvolutionExercise 3.27The fourth chapter is Fourier transform and frequency domainanalysis of the systemThe 4.1 signal is decomposed into orthogonal functions Orthogonal function setTwo. The signal is decomposed into orthogonal functions 4.2 Fourier seriesDecomposition of periodic signalsTwo, Fourier series of odd even functionThree. Exponential form of Fu Liye seriesThe spectrum of 4.3 period signalFrequency spectrum of periodic signalTwo, the spectrum of periodic matrix pulseThree. The power of periodic signal4.4 the spectrum of aperiodic signalsFirst, Fu Liye transformTwo. Fourier transform of singular functionsProperties of 4.5 Fourier transformLinearTwo, parityThree, symmetryFour, scale transformationFive, time shift characteristicsSix, frequency shift characteristicsSeven. Convolution theoremEight, time domain differential and integral Nine, frequency domain differential and integral Ten. Correlation theorem4.6 energy spectrum and power spectrumEnergy spectrumTwo. Power spectrumFourier transform of 4.7 periodic signals Fourier transform of sine and cosine functionsTwo. Fourier transform of general periodic functionsThree 、 Fu Liye coefficient and Fu Liye transformFrequency domain analysis of 4.8 LTI systemFrequency responseTwo. Distortionless transmissionThree. The response of ideal low-pass filter4.9 sampling theoremSampling of signalsTwo. Time domain sampling theoremThree. Sampling theorem in frequency domainFourier analysis of 4.10 sequencesDiscrete Fourier series DFS of periodic sequencesTwo. Discrete time Fourier transform of non periodic sequences DTFT4.11 discrete Fu Liye and its propertiesDiscrete Fourier transform (DFT)Two. The properties of discrete Fourier transformExercise 4.60The fifth chapter is the S domain analysis of continuous systems 5.1 Laplasse transformFirst, from Fu Liye transform to Laplasse transformTwo. Convergence domainThree, (Dan Bian) Laplasse transformThe properties of 5.2 Laplasse transformLinearTwo, scale transformationThree, time shift characteristicsFour, complex translation characteristicsFive, time domain differential characteristicsSix, time domain integral characteristicsSeven. Convolution theoremEight, s domain differential and integralNine, initial value theorem and terminal value theorem5.3 Laplasse inverse transformationFirst, look-up table methodTwo, partial fraction expansion method5.4 complex frequency domain analysisFirst, the transformation solution of differential equation Two. System functionThree. The s block diagram of the systemFour 、 s domain model of circuitFive, Laplasse transform and Fu Liye transform5.5 bilateral Laplasse transformExercise 5.50The sixth chapter is the Z domain analysis of discrete systems 6.1 Z transformFirst, transform from Laplasse transform to Z transformTwo, z transformThree. Convergence domainProperties of 6.2 Z transformLinearTwo. Displacement characteristicsThree, Z domain scale transformFour. Convolution theoremFive, Z domain differentiationSix, Z domain integralSeven, K domain inversionEight, part sumNine, initial value theorem and terminal value theorem 6.3 inverse Z transformFirst, power series expansion methodTwo, partial fraction expansion method6.4 Z domain analysisThe Z domain solution of difference equationTwo. System functionThree. The Z block diagram of the systemFour 、 the relation between s domain and Z domainFive. Seeking the frequency response of discrete system by means of DTFTExercise 6.50The seventh chapter system function7.1 system functions and system characteristicsFirst, zeros and poles of the system functionTwo. System function and time domain responseThree. System function and frequency domain responseCausality and stability of 7.2 systemsFirst, the causality of the systemTwo, the stability of the system7.3 information flow graphSignal flow graphTwo, Mason formulaStructure of 7.4 systemFirst, direct implementationTwo. Implementation of cascade and parallel connectionExercise 7.39The eighth chapter is the analysis of the state variables of the system8.1 state variables and state equationsConcepts of state and state variablesTwo. State equation and output equationEstablishment of state equation for 8.2 continuous systemFirst, the equation is directly established by the circuit diagramTwo. The equation of state is established by the input-output equationEstablishment and Simulation of state equations for 8.3discrete systemsFirst, the equation of state is established by the input-output equationTwo. The system simulation is made by the state equationSolution of state equation of 8.4 continuous systemFirst, the Laplasse transform method is used to solve the equation of stateTwo, the system function matrix H (z) and the stability of the systemThree. Solving state equation by time domain methodSolution of state equation for 8.5 discrete systemsFirst, the time domain method is used to solve the state equations of discrete systemsTwo. Solving the state equation of discrete system by Z transformThree, the system function matrix H (z) and the stability of the systemControllability and observability of 8.6 systemsFirst, the linear transformation of state vectorTwo, the controllability and observability of the systemExercise 8.32Appendix a convolution integral tableAppendix two convolution and tableAppendix three Fourier coefficients table of commonly used periodic signalsAppendix four Fourier transform tables of commonly used signalsAppendix five Laplasse inverse exchange tableAppendix six sequence of the Z transform table。
fourier analysis an introduction
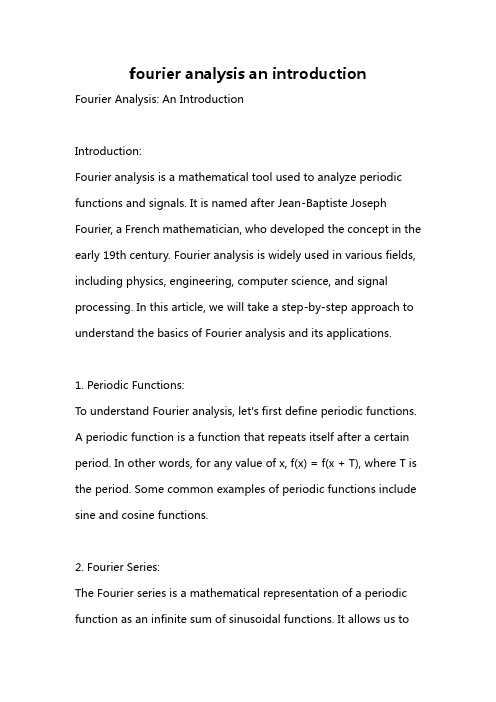
fourier analysis an introductionFourier Analysis: An IntroductionIntroduction:Fourier analysis is a mathematical tool used to analyze periodic functions and signals. It is named after Jean-Baptiste Joseph Fourier, a French mathematician, who developed the concept in the early 19th century. Fourier analysis is widely used in various fields, including physics, engineering, computer science, and signal processing. In this article, we will take a step-by-step approach to understand the basics of Fourier analysis and its applications.1. Periodic Functions:To understand Fourier analysis, let's first define periodic functions.A periodic function is a function that repeats itself after a certain period. In other words, for any value of x, f(x) = f(x + T), where T is the period. Some common examples of periodic functions include sine and cosine functions.2. Fourier Series:The Fourier series is a mathematical representation of a periodic function as an infinite sum of sinusoidal functions. It allows us todecompose a complex periodic function into simpler sinusoidal components. The formula for the trigonometric Fourier series for a periodic function f(x) with period T is given by:f(x) = a0 + Σ[an*cos(nωx) + bn*sin(nωx)]where a0 is the DC component, an and bn are the Fourier coefficients, n is an integer, and ω= 2π/T.3. Fourier Coefficients:The Fourier coefficients an and bn determine the amplitude of each component in the Fourier series. To calculate these coefficients, we can use the formulas:an = (2/T) * ∫[f(x)*cos(nωx)] dxbn = (2/T) * ∫[f(x)*sin(nωx)] dxwhere ∫represents integration over one period.4. Frequency Spectrum:The frequency spectrum is a graphical representation of the Fourier series, showing the amplitude of each sinusoidal component as afunction of frequency. The frequency spectrum helps us understand the distribution of frequency components in the original signal. The DC component (a0), representing the average value of the signal, is located at zero frequency.5. Fast Fourier Transform (FFT):Computing the Fourier coefficients directly using integrals can be computationally expensive for large data sets. The Fast Fourier Transform (FFT) is an algorithm used to efficiently compute discrete Fourier transforms. The FFT reduces the computational complexity from O(n^2) to O(n log n), making it practical for real-time processing of signals.6. Applications of Fourier Analysis:Fourier analysis finds numerous applications in various fields. Some of the key areas where Fourier analysis is used include:- Signal Processing: Fourier analysis is used to extract various frequency components from signals, enabling filtering, noise reduction, and compression.- Image Processing: The Fourier Transform is used to analyze and enhance images, such as in image compression algorithms.- Communication Systems: Fourier analysis helps in the modulation, demodulation, and encoding of signals in various communication systems.- Quantum Mechanics: Fourier analysis is used to describe and analyze wave functions in quantum mechanics.- Music and Audio Processing: Fourier analysis is used for audio compression, synthesis, and analysis of music signals.Conclusion:Fourier analysis is a powerful mathematical tool for analyzing periodic functions and signals. Its ability to decompose complex signals into simple sinusoidal components allows us to gain insights into the frequency content of a signal and enables various applications such as signal processing, image processing, communication systems, and more. Understanding the basics of Fourier analysis provides a solid foundation for exploring its advanced concepts and applications in different fields.。
振动方面的专业英语及词汇
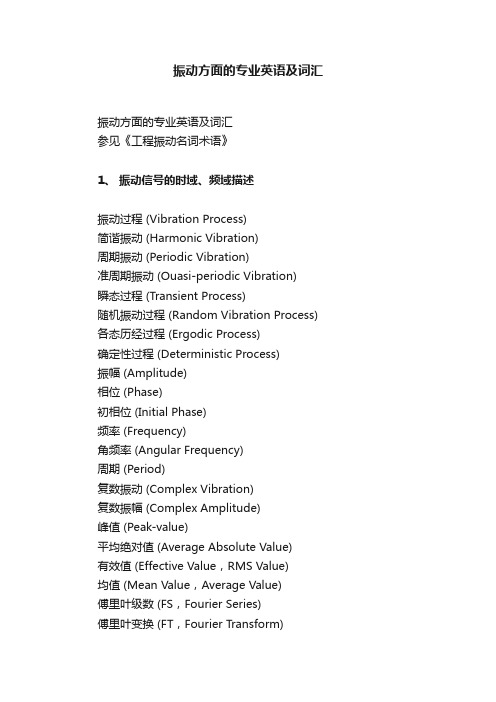
振动方面的专业英语及词汇振动方面的专业英语及词汇参见《工程振动名词术语》1、振动信号的时域、频域描述振动过程 (Vibration Process)简谐振动 (Harmonic Vibration)周期振动 (Periodic Vibration)准周期振动 (Ouasi-periodic Vibration)瞬态过程 (Transient Process)随机振动过程 (Random Vibration Process) 各态历经过程 (Ergodic Process)确定性过程 (Deterministic Process)振幅 (Amplitude)相位 (Phase)初相位 (Initial Phase)频率 (Frequency)角频率 (Angular Frequency)周期 (Period)复数振动 (Complex Vibration)复数振幅 (Complex Amplitude)峰值 (Peak-value)平均绝对值 (Average Absolute Value)有效值 (Effective Value,RMS Value)均值 (Mean Value,Average Value)傅里叶级数 (FS,Fourier Series)傅里叶变换 (FT,Fourier Transform)傅里叶逆变换 (IFT,Inverse Fourier Transform) 离散谱 (Discrete Spectrum)连续谱 (Continuous Spectrum)傅里叶谱 (Fourier Spectrum)线性谱 (Linear Spectrum)幅值谱 (Amplitude Spectrum)相位谱 (Phase Spectrum)均方值 (Mean Square Value)方差 (Variance)协方差 (Covariance)自协方差函数 (Auto-covariance Function)互协方差函数 (Cross-covariance Function)自相关函数 (Auto-correlation Function)互相关函数 (Cross-correlation Function)标准偏差 (Standard Deviation)相对标准偏差 (Relative Standard Deviation)概率 (Probability)概率分布 (Probability Distribution)高斯概率分布 (Gaussian Probability Distribution) 概率密度 (Probability Density)集合平均 (Ensemble Average)时间平均 (Time Average)功率谱密度 (PSD,Power Spectrum Density)自功率谱密度 (Auto-spectral Density)互功率谱密度 (Cross-spectral Density)均方根谱密度 (RMS Spectral Density)能量谱密度 (ESD,Energy Spectrum Density)相干函数 (Coherence Function)帕斯瓦尔定理 (Parseval''''s Theorem)维纳,辛钦公式 (Wiener-Khinchin Formula2、振动系统的固有特性、激励与响应振动系统 (Vibration System)激励 (Excitation)响应 (Response)单自由度系统 (Single Degree-Of-Freedom System) 多自由度系统 (Multi-Degree-Of- Freedom System) 离散化系统 (Discrete System)连续体系统 (Continuous System)刚度系数 (Stiffness Coefficient)自由振动 (Free Vibration)自由响应 (Free Response)强迫振动 (Forced Vibration)强迫响应 (Forced Response)初始条件 (Initial Condition)固有频率 (Natural Frequency)阻尼比 (Damping Ratio)衰减指数 (Damping Exponent)阻尼固有频率 (Damped Natural Frequency)对数减幅系数 (Logarithmic Decrement)主频率 (Principal Frequency)无阻尼模态频率 (Undamped Modal Frequency)模态 (Mode)主振动 (Principal Vibration)振型 (Mode Shape)振型矢量 (Vector Of Mode Shape)模态矢量 (Modal Vector)正交性 (Orthogonality)展开定理 (Expansion Theorem)主质量 (Principal Mass)模态质量 (Modal Mass)主刚度 (Principal Stiffness)模态刚度 (Modal Stiffness)正则化 (Normalization)振型矩阵 (Matrix Of Modal Shape)模态矩阵 (Modal Matrix)主坐标 (Principal Coordinates)模态坐标 (Modal Coordinates)模态分析 (Modal Analysis)模态阻尼比 (Modal Damping Ratio)频响函数 (Frequency Response Function)幅频特性 (Amplitude-frequency Characteristics)相频特性 (Phase frequency Characteristics)共振 (Resonance)半功率点 (Half power Points)波德图(Bodé Plot)动力放大系数 (Dynamical Magnification Factor)单位脉冲 (Unit Impulse)冲激响应函数 (Impulse Response Function)杜哈美积分(Duhamel’s Integral)卷积积分 (Convolution Integral)卷积定理 (Convolution Theorem)特征矩阵 (Characteristic Matrix)阻抗矩阵 (Impedance Matrix)频响函数矩阵 (Matrix Of Frequency Response Function) 导纳矩阵 (Mobility Matrix)冲击响应谱 (Shock Response Spectrum)冲击激励 (Shock Excitation)冲击响应 (Shock Response)冲击初始响应谱 (Initial Shock Response Spectrum)冲击剩余响应谱 (Residual Shock Response Spectrum) 冲击最大响应谱 (Maximum Shock Response Spectrum) 冲击响应谱分析 (Shock Response Spectrum Analysis)3 、模态试验分析模态试验 (Modal Testing)机械阻抗 (Mechanical Impedance)位移阻抗 (Displacement Impedance)速度阻抗 (Velocity Impedance)加速度阻抗 (Acceleration Impedance)机械导纳 (Mechanical Mobility)位移导纳 (Displacement Mobility)速度导纳 (Velocity Mobility)加速度导纳 (Acceleration Mobility)驱动点导纳 (Driving Point Mobility)跨点导纳 (Cross Mobility)传递函数 (Transfer Function)拉普拉斯变换 (Laplace Transform)传递函数矩阵 (Matrix Of Transfer Function)频响函数 (FRF,Frequency Response Function)频响函数矩阵 (Matrix Of FRF)实模态 (Normal Mode)复模态 (Complex Mode)模态参数 (Modal Parameter)模态频率 (Modal Frequency)模态阻尼比 (Modal Damping Ratio)模态振型 (Modal Shape)模态质量 (Modal Mass)模态刚度 (Modal Stiffness)模态阻力系数 (Modal Damping Coefficient)模态阻抗 (Modal Impedance)模态导纳 (Modal Mobility)模态损耗因子 (Modal Loss Factor)比例粘性阻尼 (Proportional Viscous Damping)非比例粘性阻尼 (Non-proportional Viscous Damping) 结构阻尼 (Structural Damping,Hysteretic Damping) 复频率 (Complex Frequency)复振型 (Complex Modal Shape)留数 (Residue)极点 (Pole)零点 (Zero)复留数 (Complex Residue)随机激励 (Random Excitation)伪随机激励 (Pseudo Random Excitation)猝发随机激励 (Burst Random Excitation)稳态正弦激励 (Steady State Sine Excitation)正弦扫描激励 (Sweeping Sine Excitation)锤击激励 (Impact Excitation)频响函数的H1 估计 (FRF Estimate by H1)频响函数的H2 估计 (FRF Estimate by H2)频响函数的H3 估计 (FRF Estimate by H3)单模态曲线拟合法 (Single-mode Curve Fitting Method) 多模态曲线拟合法 (Multi-mode Curve Fitting Method) 模态圆 (Mode Circle)剩余模态 (Residual Mode)幅频峰值法 (Peak Value Method)实频-虚频峰值法 (Peak Real/Imaginary Method)圆拟合法 (Circle Fitting Method)加权最小二乘拟合法 (Weighting Least Squares Fitting method) 复指数拟合法 (Complex Exponential Fitting method)4、振动测试的名词术语1 )传感器测量系统传感器测量系统 (Transducer Measuring System)传感器 (Transducer)振动传感器 (Vibration Transducer)机械接收 (Mechanical Reception)机电变换 (Electro-mechanical Conversion)测量电路 (Measuring Circuit)惯性式传感器 (Inertial Transducer,Seismic Transducer)相对式传感器 (Relative Transducer)电感式传感器 (Inductive Transducer)应变式传感器 (Strain Gauge Transducer)电动力传感器 (Electro-dynamic Transducer)压电式传感器 (Piezoelectric Transducer)压阻式传感器 (Piezoresistive Transducer)电涡流式传感器 (Eddy Current Transducer)伺服式传感器 (Servo Transducer)灵敏度 (Sensitivity)复数灵敏度 (Complex Sensitivity)分辨率 (Resolution)频率范围 (Frequency Range)线性范围 (Linear Range)频率上限 (Upper Limit Frequency)频率下限 (Lower Limit Frequency)静态响应 (Static Response)零频率响应 (Zero Frequency Response)动态范围 (Dynamic Range)幅值上限 Upper Limit Amplitude)幅值下限 (Lower Limit Amplitude)最大可测振级 (Max.Detectable Vibration Level)最小可测振级 (Min.Detectable Vibration Level)信噪比 (S/N Ratio)振动诺模图 (Vibration Nomogram)相移 (Phase Shift)波形畸变 (Wave-shape Distortion)比例相移 (Proportional Phase Shift)惯性传感器的稳态响应(Steady Response Of Inertial Transducer)惯性传感器的稳击响应 (Shock Response Of Inertial Transducer) 位移计型的频响特性(Frequency Response Characteristics Vibrometer)加速度计型的频响特性(Frequency Response Characteristics Accelerometer)幅频特性曲线 (Amplitude-frequency Curve)相频特性曲线 (Phase-frequency Curve)固定安装共振频率 (Mounted Resonance Frequency)安装刚度 (Mounted Stiffness)有限高频效应 (Effect Of Limited High Frequency)有限低频效应 (Effect Of Limited Low Frequency)电动式变换 (Electro-dynamic Conversion)磁感应强度 (Magnetic Induction, Magnetic Flux Density)磁通 (Magnetic Flux)磁隙 (Magnetic Gap)电磁力 (Electro-magnetic Force)相对式速度传 (Relative Velocity Transducer)惯性式速度传感器 (Inertial Velocity Transducer)速度灵敏度 (Velocity Sensitivity)电涡流阻尼 (Eddy-current Damping)无源微(积)分电路 (Passive Differential (Integrate) Circuit) 有源微(积)分电路 (Active Differential (Integrate) Circuit)运算放大器 (Operational Amplifier)时间常数 (Time Constant)比例运算 (Scaling)积分运算 (Integration)微分运算 (Differentiation)高通滤波电路 (High-pass Filter Circuit)低通滤波电路 (Low-pass Filter Circuit)截止频率 (Cut-off Frequency)压电效应 (Piezoelectric Effect)压电陶瓷 (Piezoelectric Ceramic)压电常数 (Piezoelectric Constant)极化 (Polarization)压电式加速度传感器 (Piezoelectric Acceleration Transducer) 中心压缩式 (Center Compression Accelerometer)三角剪切式 (Delta Shear Accelerometer)压电方程 (Piezoelectric Equation)压电石英 (Piezoelectric Quartz)电荷等效电路 (Charge Equivalent Circuit)电压等效电路 (Voltage Equivalent Circuit)电荷灵敏度 (Charge Sensitivity)电压灵敏度 (Voltage Sensitivity)电荷放大器 (Charge Amplifier)适调放大环节 (Conditional Amplifier Section)归一化 (Uniformization)电荷放大器增益 (Gain Of Charge Amplifier)测量系统灵敏度 (Sensitivity Of Measuring System)底部应变灵敏度 (Base Strain Sensitivity)横向灵敏度 (Transverse Sensitivity)地回路 (Ground Loop)力传感器 (Force Transducer)力传感器灵敏度 (Sensitivity Of Force Transducer)电涡流 (Eddy Current)前置器 (Proximitor)间隙-电压曲线 (Voltage vs Gap Curve)间隙-电压灵敏度 (Voltage vs Gap Sensitivity)压阻效应 (Piezoresistive Effect)轴向压阻系数 (Axial Piezoresistive Coefficient)横向压阻系数 (Transverse Piezoresistive Coefficient)压阻常数 (Piezoresistive Constant)单晶硅 (Monocrystalline Silicon)应变灵敏度 (Strain Sensitivity)固态压阻式加速度传感器(Solid State Piezoresistive Accelerometer)体型压阻式加速度传感器(Bulk Type Piezoresistive Accelerometer)力平衡式传感器 (Force Balance Transducer)电动力常数 (Electro-dynamic Constant)机电耦合系统 (Electro-mechanical Coupling System)2)检测仪表、激励设备及校准装置时间基准信号 (Time Base Signal)李萨茹图 (Lissojous Curve)数字频率计 (Digital Frequency Meter)便携式测振表 (Portable Vibrometer)有效值电压表 (RMS Value Voltmeter)峰值电压表 (Peak-value Voltmeter)平均绝对值检波电路 (Average Absolute Value Detector) 峰值检波电路 (Peak-value Detector)准有效值检波电路 (Quasi RMS Value Detector)真有效值检波电路 (True RMS Value Detector)直流数字电压表 (DVM,DC Digital Voltmeter)数字式测振表 (Digital Vibrometer)A/D 转换器 (A/D Converter)D/A 转换器 (D/A Converter)相位计 (Phase Meter)电子记录仪 (Lever Recorder)光线示波器 (Oscillograph)振子 (Galvonometer)磁带记录仪 (Magnetic Tape Recorder)DR 方式(直接记录式) (Direct Recorder)FM 方式(频率调制式) (Frequency Modulation)失真度 (Distortion)机械式激振器 (Mechanical Exciter)机械式振动台 (Mechanical Shaker)离心式激振器 (Centrifugal Exciter)电动力式振动台 (Electro-dynamic Shaker)电动力式激振器 (Electro-dynamic Exciter)液压式振动台 (Hydraulic Shaker)液压式激振器 (Hydraulic Exciter)电液放大器 (Electro-hydraulic Amplifier)磁吸式激振器 (Magnetic Pulling Exciter)涡流式激振器 (Eddy Current Exciter)压电激振片 (Piezoelectric Exciting Elements)冲击力锤 (Impact Hammer)冲击试验台 (Shock Testing Machine)激振控制技术 (Excitation Control Technique)波形再现 (Wave Reproduction)压缩技术 (Compression Technique)均衡技术 (Equalization Technique)交越频率 (Crossover Frequency)综合技术 (Synthesis Technique)校准 (Calibration)分部校准 (Calibration for Components in system)系统校准 (Calibration for Over-all System)模拟传感器 (Simulated Transducer)静态校准 (Static Calibration)简谐激励校准 (Harmonic Excitation Calibration)绝对校准 (Absolute Calibration)相对校准 (Relative Calibration)比较校准 (Comparison Calibration)标准振动台 (Standard Vibration Exciter)读数显微镜法 (Microscope-streak Method)光栅板法 (Ronchi Ruling Method)光学干涉条纹计数法 (Optical Interferometer Fringe Counting Method)光学干涉条纹消失法(Optical Interferometer Fringe Disappearance Method)背靠背安装 (Back-to-back Mounting)互易校准法 (Reciprocity Calibration)共振梁 (Resonant Bar)冲击校准 (Impact Exciting Calibration)摆锤冲击校准 (Ballistic Pendulum Calibration)落锤冲击校准 (Drop Test Calibration)振动和冲击标准 (Vibration and Shock Standard)迈克尔逊干涉仪 (Michelson Interferometer)摩尔干涉图象 (Moire Fringe)参考传感器 (Reference Transducer)3 )频率分析及数字信号处理带通滤波器 (Band-pass Filter)半功率带宽 (Half-power Bandwidth)3 dB 带宽 (3 dB Bandwidth)等效噪声带宽 (Effective Noise Bandwidth)恒带宽 (Constant Bandwidth)恒百分比带宽 (Constant Percentage Bandwidth)1/N 倍频程滤波器 (1/N Octave Filter)形状因子 (Shape Factor)截止频率 (Cut-off Frequency)中心频率 (Centre Frequency)模拟滤波器 (Analog Filter)数字滤波器 (Digital Filter)跟踪滤波器 (Tracking Filter)外差式频率分析仪 (Heterodyne Frequency Analyzer) 逐级式频率分析仪 (Stepped Frequency Analyzer)扫描式频率分析仪 (Sweeping Filter Analyzer)混频器 (Mixer)RC 平均 (RC Averaging)平均时间 (Averaging Time)扫描速度 (Sweeping Speed)滤波器响应时间 (Filter Response Time)离散傅里叶变换 (DFT,Discrete Fourier Transform) 快速傅里叶变换 (FFT,Fast Fourier Transform)抽样频率 (Sampling Frequency)抽样间隔 (Sampling Interval)抽样定理 (Sampling Theorem)抗混滤波 (Anti-aliasing Filter)泄漏 (Leakage)加窗 (Windowing)窗函数 (Window Function)截断 (Truncation)频率混淆 (Frequency Aliasing)乃奎斯特频率 (Nyquist Frequency)矩形窗 (Rectangular Window)汉宁窗 (Hanning Window)凯塞-贝塞尔窗 (Kaiser-Bessel Window)平顶窗 (Flat-top Window)平均 (Averaging)线性平均 (Linear Averaging)指数平均 (Exponential Averaging)峰值保持平均 (Peak-hold Averaging)时域平均 (Time-domain Averaging)谱平均 (Spectrum Averaging)重叠平均 (Overlap Averaging)栅栏效应 (Picket Fence Effect)吉卜斯效应 (Gibbs Effect)基带频谱分析 (Base-band Spectral Analysis)选带频谱分析 (Band Selectable Sp4ctralAnalysis)细化 (Zoom)数字移频 (Digital Frequency Shift)抽样率缩减 (Sampling Rate Reduction)功率谱估计 (Power Spectrum Estimate)相关函数估计 (Correlation Estimate)频响函数估计 (Frequency Response Function Estimate) 相干函数估计 (Coherence Function Estimate)冲激响应函数估计 (Impulse Response Function Estimate) 倒频谱 (Cepstrum)功率倒频谱 (Power Cepstrum)幅值倒频谱 (Amplitude Cepstrum)倒频率 (Quefrency)4 旋转机械的振动测试及状态监测状态监测 (Condition Monitoring)故障诊断 (Fault Diagnosis)转子 (Rotor)转手支承系统 (Rotor-Support System)振动故障 (Vibration Fault)轴振动 (Shaft Vibration)径向振动 (Radial Vibration)基频振动 (Fundamental Frequency Vibration)基频检测 (Fundamental Frequency Component Detecting) 键相信号 (Key-phase Signal)正峰相位 ( Peak Phase)高点 (High Spot)光电传感器 (Optical Transducer)同相分量 (In-phase Component)正交分量 (Quadrature Component)跟踪滤波 (Tracking Filter)波德图 (Bode Plot)极坐标图 (Polar Plot)临界转速 (Critical Speed)不平衡响应 (Unbalance Response)残余振幅 (Residual Amplitude)方位角 (Attitude Angle)轴心轨迹 (Shaft Centerline Orbit)正进动 (Forward Precession)同步正进动 (Synchronous Forward Precession) 反进动 (Backward Precession)正向涡动 (Forward Whirl)反向涡动 (Backward Whirl)油膜涡动 (Oil Whirl)油膜振荡 (Oil Whip)轴心平均位置 (Average Shaft Centerline Position) 复合探头 (Dual Probe)振摆信号 (Runout Signal)电学振摆 (Electrical Runout)机械振摆 (Mechanical Runout)慢滚动向量 (Slow Roll Vector)振摆补偿 (Runout Compensation)故障频率特征 (Frequency Characteristics Of Fault) 重力临界 (Gravity Critical)对中 (Alignment)双刚度转子 (Dual Stiffness Rotor)啮合频率 (Gear-mesh Frequency)间入简谐分量 (Interharmonic Component)边带振动 (Side-band Vibration)三维频谱图 (Three Dimensional Spectral Plot)瀑布图 (Waterfall Plot)级联图 (Cascade Plot)阶次跟踪 (Order Tracking)阶次跟踪倍乘器 (Order Tracking Multiplier)监测系统 (Monitoring System)适调放大器 (Conditional Amplifier)趋势分析 (Trend Analysis)倒频谱分析 (Cepstrum Analysis)直方图 (Histogram)确认矩阵 (Confirmation Matrix) 通频幅值 (Over-all Amplitude) 幅值谱 (Amplitude Spectrum) 相位谱 (Phase Spectrum)报警限 (Alarm Level)来源网络,侵删。
非等间隔采样信号傅里叶频谱分析方法

Fourier spectrum analysis for non-uniform sampled sigAO Xuesong。 fSchool ofInformation Science and Engineering,Wuhan Universit ̄ ofScience and Technology,Wuhan髓 430081,China)
目前 ,车载激光雷达 都只测量 目标距 离而不测 量 目标 速 度 。文献[4—5]提 出使 用连 续波 的方式 实现激 光 雷达 同步 测 量 目标距 离和速度 ,但 是工作 于连续波 模式 下的激光器 发 射 峰值 功率不可能太高 ,特别是 在使用半 导体激 光器 的情 况 下 ,峰值发射功率 只有几毫瓦 。由于道路 目标 的多样性 ,在发 射峰值功率很低 的情况下 ,探测 低反射 率 的 目标 时极有 可能 达 到 激 光 雷 达 的 理 论 探 测 极 限 。 因 此 ,实 用 的 车 载 激 光 雷 达
DOI:10.11772/j.issn.1001—9081.2016.02.04 92
非等 间隔 采样 信 号傅 里 叶频 谱 分 析方 法
方 建 超 ,毛 雪松 ’
(武汉科技大学 信息科学与工程学院,武汉 430081) (¥通信作者 电子邮箱 XSflaO@wust.edu.Cf1)
摘 要 :针 对伪随机 (PN)码调制的 多普勒激光 雷达 中固有 的对外差信 号不能等 间隔采样的 问题 ,提 出一种新 的 非均 匀采样信号 的离散傅 里叶 变换 (DFT)方法。首先 ,给 出距 离速度 同步测量 多普勒激光雷达 系统模 型 ,指 出对外 差 信号不 能等 间隔采样 的原 因;然后 ,通过理论推导 ,提 出一种新的非均 匀采样信 号的频谱 分析方 法;最后 ,通过仿真验 证该方 法可 用于分析 非等 间隔采样信号 的频 谱。结果表 明 ,在道路运 动 目标 产生 的多普勒频 率范 围 内,接 收信号信 噪 比(SNR)为 O dB的情况下 ,该 方法仍可有效分析 出不等间隔采样 多普勒信 号的频 率。
Fourier transform emission spectroscopy of some new bands of ReN

Fourier transform emission spectroscopy of some new bands of ReNR.S.Rama,*,P.F.Bernatha,b,c,W.J.Balfourda Department of Chemistry,University of Arizona,Tucson,AZ 85721,USA bDepartment of Chemistry,University of York,Heslington,York YO105DD,UK cDepartment of Chemistry,University of Waterloo,Waterloo,Ont.,Canada N2L 3G1dDepartment of Chemistry,University of Victoria,Victoria,BC,Canada V8W 3V6Received 5July 2007;in revised form 21September 2007Available online 1October 2007AbstractThe emission spectrum of ReN has been reinvestigated in the visible region using a Fourier transform spectrometer.Two new bands have been identified with band origins near 22110and 22224cm À1.These bands have a common lower state and have been assigned as the 0+–A 1and 0À–A 1transitions.After rotational analysis it was noted that the new 0+–A 1transition also has its upper state in common with the upper state of the [24.7]0+–X 0+transition reported previously [W.J.Balfour,J.Cao,C.X.W.Qian,S.J.Rixon,J.Mol.Spec-trosc.183(1997)113–118.].This observation provides T 00=2616.26cm À1for the A 1state.It is likely that the A 1and X 0+states are two spin components of the 3R Àground state.Ó2007Elsevier Inc.All rights reserved.Keywords:Fourier transform spectroscopy;High resolution electronic spectroscopy;Diatomic transition metal nitrides1.IntroductionIn recent years there has been interest in the study of transition metal nitrides because of their importance in catalysis,surface science,ab initio calculations and organo-metallic chemistry [1–5].The diatomic transition metal nitrides serve as simple models for the study of metal–nitrogen bonding in inorganic chemistry.The experimental data on these molecules are being used to test the quality of ab initio calculations aimed at predicting the spectroscopic properties of small molecules accurately.In the last few years,considerable progress has been made in the study of ReN.The first observation of ReN was made in 1994[6]when a DX =1transition assigned as [23.8]1–X 0+,was observed near 23746cm À1using Fourier transform emission spectroscopy as well as pulsed dye laser excitation spectroscopy.It was concluded that the X 0+state was the ground state of ReN since this transition was also observed in the laser excitation experiments.In subsequent studies atthe University of Victoria,additional transitions were observed using laser excitation of molecules produced in a laser-ablation source [7,8].A number of bands observed in the 375–542nm region were rotationally analyzed and classified into five electronic transitions [7].The lifetimes were also measured for the excited states.It was noted that a band observed at 540nm,labeled as [18.5]1–X 0+,showed an unusual profile due to extra branches and had an irreg-ular energy pattern because of strong perturbations in the excited state [8].A deperturbation analysis of this band was performed and dispersed fluorescence spectra were recorded for all previously observed excited states [8],which revealed the presence of a number of low-lying states below 12000cm À1.The X values for these states were established and are consistent with the electronic structure of ReN proposed in earlier studies.The approximate loca-tion of the expected low-lying states,X 3R À1;3D 3;3D 2;3D 1,and 1R þ0were determined from the dispersed fluorescence study.This work provided the location of an X =1state at 2630±100cm À1above the ground state,which is prob-ably the lower state of the two transitions observed in the present study.0022-2852/$-see front matter Ó2007Elsevier Inc.All rights reserved.doi:10.1016/j.jms.2007.09.013*Corresponding author.Fax:+15206218407.E-mail address:rram@ (R.S.Ram)./locate/jmsAvailable online at Journal of Molecular Spectroscopy 246(2007)192–197More recently ReN molecules were produced by Zhou and Andrews[9]by the reaction of laser ablated rhenium atoms with nitrogen and the fundamental band of ReN was measured in an N2matrix at10and20K providing a ground state vibrational interval close to the gas phase value [6].In another study the high-resolution laser inducedfluo-rescence spectra of ReN were recorded with a laser abla-tion/molecular beam spectrometer by Steimle and Virgo [10]and the(0,0)band of the[26.0]0+–X0+system of ReN was investigated in the presence of an electricfield.Ground and excited state electric dipole moments of1.96(8)and 3.53(4)D,respectively,were determined for187ReN[10].In the present work we report the observation of two new0–0bands near22110and22224cmÀ1having their lower state in common.The22110cmÀ1band also has its upper state in common with the[24.7]0+–X0+transition. This observation locates the lower X=1state at 2616.26cmÀ1above the ground state.2.ExperimentalThe experimental method and conditions for the obser-vation of ReN bands have been provided in our previous paper[6].Briefly,the molecules were produced in a rhe-nium hollow cathode lamp operated at300V and 457mA current.A slow and continuousflow of a mixture of about3Torr of Ne and5mTorr of N2was maintained through the lamp in order to observe the ReN bands.The spectra were recorded using the1-m Fourier transform spectrometer of the National Solar Observatory at Kitt Peak.The spectra in the10000–29000cmÀ1region were recorded in two parts.The10000–19500cmÀ1region was recorded using a UV beam splitter,Si-diode detectors and RG495red passfilters while the17000–29000cmÀ1 region was recorded using the same beam splitter,Si-diode detectors and CuSO4filters.In both the experiments the spectra were recorded at a resolution of0.02cmÀ1.The spectra were measured using a data reduction pro-gram called PC-DECOMP developed by J.Brault at the National Solar Observatory and calibrated using the mea-surements of the Ne atomic lines made by Palmer and Engleman[11].The new bands appear with very weak intensity(S/N ratio of$4)and are partly overlapped by the spectra of much stronger Nþ2lines.The precision of measurements of strong and unblended lines of ReN is expected to be of the order of±0.005cmÀ1.3.Results and discussionOur FTS spectrum consists of two new and very weak bands of ReN in the22000–22250cmÀ1region,in addition to the bands reported previously[6].A part of the spectrum with the R heads of the two0–0bands marked is presented in Fig.1.Although the band heads are weak,the branches were identified easily using a Loomis–Wood program.The structure of both these bands consists of P,Q and R branches with the Q branch being the most intense.The rotational assignment in the two bands was made by com-paring the combination differences.This analysis indicates that the two bands have a common lower state,which has been identified as an X=1state with small X-doubling constants.From this analysis we also conclude that the upper states of the two bands do not have any combination defects,consistent with an X=0assignment.Based on rotational analysis,the new bands at22110and 22224cmÀ1have been labeled as0+–A1and0À–A1transi-tions.A comparison of the rotational constants of the new states to the values for other states reported previously by Balfour and coworkers[7,8],suggests that22110and 24706cmÀ1bands probably also have a common upper state.It was noted that the0+–A1transition was also observed by Cao et al.[8]in their dispersedfluorescence study.The0À–A1transition at22224cmÀ1has been observed for thefirst time.The Re atom has two naturally occurring isotopes185Re(37.07%)and187Re(62.93%).The absence of any isotope splitting in the two bands indicates that both bands are0–0bands.A part of the spectrum of the22110cmÀ1band is shown in Fig.2where somelines Fig.1.A compressed portion of the emission spectrum of ReN marking the R heads of the0+–A1,0–0and0À–A1,0–0bands of ReN.Many of the strongest features are Re atomic lines.R.S.Ram et al./Journal of Molecular Spectroscopy246(2007)192–197193of the Q and P branches have been marked.The rotational lines were sorted out into branches using a color Loomis–Wood program running on a PC computer.The spectro-scopic constants were determined by fitting the observed line positions to the following customary energy level expression:(for the X =0+and 0Àstates)F v ðJ Þ¼T v þB v J ðJ þ1ÞÀD v ½J ðJ þ1Þ 2ð1Þ(for the X =1state)F v ðJ Þ¼T v þB v J ðJ þ1ÞÀD v ½J ðJ þ1Þ 2þH v ½J ðJþ1Þ 3Æ1=2f q v J ðJ þ1Þþq Dv ½J ðJ þ1Þ 2gð2ÞIn the final fit the rotational lines of the [23.8]1–X 0+,0–0band [6]and [24.7]0+–X 0+,0–0band [7]were also included with our data.The rotational lines of the [24.7]0+–X 0+transition were given slightly reduced weights due to a lar-ger uncertainty in measurements,compared to the FTS spectra.The current FTS measurements were given weights based on their signal-to-noise ratio and extent of blending.Lines affected by perturbations were given lower weights or were deweighted.Two sets of spectroscopic constants were obtained by fitting the combined data.In the first fit all the states were treated as case (c)states.The observed lines positions in the new bands are reported in Table 1,where O A C refers to the observed minus calculated differences based on constants obtained from the case (c)fit.In the second fit the X 0+and A 1states were treated as the com-ponents of the case (a),X 3R Àstate.An explicit listing of the matrix elements for the 3R ÀHamiltonian can be found in our previous paper on NH [12].The case (c)and case (a)constants obtained from our fits are provided in Tables 2and 3,respectively,where the spectroscopic constants for the [23.8]1state are also provided.The constants of the X 0+and [23.8]1states have changed slightly compared to those in reference 6,after combining with the data of other transitions.The electronic spectra of ReN have been studied in great detail by Balfour and coworkers [7,8]and an overview of the electronic structure of the low-lying states is already available.From these studies it was concluded that the ground state of ReN is an X =0+state,a spin component of the X 3R Àstate arising from the d 2r 2configuration.Here the d and r orbitals are essentially non-bonding correlating with 5d and 6s atomic orbitals,respectively,of the Re atom [7].From the dispersed fluorescence study,it was suggested that the X =1component of the X 3R Àstate is located at about 2600±100cm À1above the ground state.In addi-tion,the approximate location of the three components of the low-lying 3D state arising from the d 3r 1configuration and the 1R +state,most probably arising from the d 2r 2con-figuration,were also provided (Table 2of Ref.[8]).The current term value of 2616.26cm À1determined for the X =1state agrees well with the value determined in the dis-persed fluorescence study [8].The large splitting between the X =0+and X =1components is supported by large spin-orbit interaction of $2545cm À1for the rhenium atom [13].The large spin orbit splitting also suggests that the dif-ferent X states of ReN probably have a tendency towards Hund’s case (c)coupling.The rotational constants of 0.481100and 0.480888cm À1for the X0+and A 1states are very similar in magnitude,consistent with their assignment as X 3R 0+and X 3R 1com-ponents of the X 3R Àground state.The two excited X =0+and X =0Àstates also have similar rotational con-stants of 0.461679and 0.460407cm À1,respectively,and are separated by only $114cm À1.It is possible that the two states are also related.For example,an assignment as 3P 0+and 3P 0Àis a possibility for the two new states although it is difficult to draw any definite conclusion based only on the present observations.As pointed out above,the electronic states of ReN have a tendency towards Hund’s case (c)coupling,and the two states may very well be spin components of different states.There are notheoreticalFig.2.An expanded portion of the 0+–A 1,0–0band of ReN,marking some rotational lines in the Q and P branches.194R.S.Ram et al./Journal of Molecular Spectroscopy 246(2007)192–197R.S.Ram et al./Journal of Molecular Spectroscopy246(2007)192–197195 Table1Observed line positions(in cmÀ1)in the0+–A1,0–0and0À–A1,0–0bands of ReNJ0+–A10À–A1R(J)OÀC Q(J)OÀC P(J)OÀC R(J)OÀC Q(J)OÀC P(J)OÀC 422109.453À122223.939À9522109.260À3622109.006À2822223.477À18722108.757À922223.196À10822108.458À322222.855À21922108.116À122222.500À51022107.737222222.071À221122107.311À422221.610À291222106.848À822221.114À311322106.355À422094.293À2122220.562À471422105.817À722092.847À222219.954À781522105.245À522091.335À1122219.291À1221622089.813922218.421À3321722218.2552031822103.293À522086.592À1122217.407981922102.570022084.934À1122216.593682022101.8171322083.243À522215.746472122109.000022081.511022214.866342222100.162622079.735122213.952282322099.2871322077.918À422213.000252422076.071322212.0072422190.06311 2522097.397422210.9691922188.109À5 2622121.091À2822096.399522072.244À122209.8931622186.15016 2722120.974À1422095.357022070.284922208.764422184.1184 2822120.8281122094.2931222068.268322207.608522182.07825 2922120.613722093.173822066.212À322234.205322206.407322179.9586 3022120.3751922092.014322064.1451722205.165122177.8123 3122120.061À522090.811À722203.858À243222119.735022089.592722059.8481422233.1601022202.561322173.42322 3322057.6401322232.709À822201.186À622171.124À11 3422087.005422055.380À222232.240À122199.784À122168.84011 3522118.5141122085.650À122053.1091322231.727322198.333À322166.49614 3622117.991À2022084.270922050.7922122231.170422196.832À1322164.1028 3722117.475À422082.831022048.4161022230.574822195.307À522161.6726 3822116.912522081.369822045.999À322229.927422193.727À1022159.21319 3922116.293À122079.854222043.5711322229.246622192.110À1122156.6925 4022115.643322078.308522041.067À722228.524922190.456À622154.15727 4122114.950322076.7352122038.556622227.752422188.749À1322151.515À11 4222114.203À722075.1082322035.988222226.927À1122187.027722148.896À5 4322113.421À1322073.4271022033.378À422226.078À922185.229À622146.23914 4422112.612À522071.7201222030.735À322225.182À1222183.406À222143.506À2 4522111.753À622069.964522028.041À1222224.255À322140.743À6 4622110.8751622068.169022025.306À2322223.276À522179.628022137.9566 4722109.907À1122066.345522022.561À222222.257À422177.665À1022135.097À11 4822108.916À1922019.752À622221.198À222175.673À622132.2293 4922107.893À1922062.554À422016.894À1722220.071À2422173.645422129.3043 5022106.848322060.598À822014.007À1722218.950222171.561022126.329À7 5122105.714À2422058.616222011.096022217.757À222169.440222123.3366 5222056.572À922008.109À1922216.521À722167.2831022120.270À11 5322103.379À1722054.494À1222005.109À822215.254À122165.072722117.1910 5422102.122À4022052.382À922002.037À2922213.9521422162.820522114.051À8 5522100.847À3922050.211À2321998.940À3522212.583422160.520À222110.875À11 5622099.525À4322048.000À3621995.786À5522211.179222158.193722107.6798 5722045.745À5121992.613À5322209.735222155.80805822043.439À7621989.380À7022208.246122153.395822101.101À15 5922041.067À12521986.111À8122206.724922097.768À8 6022205.1652322148.425922094.388À5 6122203.513À1322145.862À422090.9733 6222034.0719922201.870322143.282922087.497À6 6322031.5426122200.165122140.641422083.993À3 6422028.9934522198.405À1322137.956À222080.4505 6522026.4053322196.633322135.240422076.842À11(continued on next page)predictions available for ReN to help in the electronic assignment of the observed X states.Our analysis indicates that excited states of both transi-tions are affected by local perturbations.The0+–A1,0–0 band at22110cmÀ1is perturbed near J=60while the 0À–A1,0–0band at22224cmÀ1is affected by perturba-tions near J=16.Several X=0and1states which have been observed[7,8]in the vicinity of the two states may be responsible for these perturbations.4.ConclusionTwo new emission bands of ReN,having a common X=1lower state,have been observed in the21000–Table1(continued)J0+–A10À–A1R(J)OÀC Q(J)OÀC P(J)OÀC R(J)OÀC Q(J)OÀC P(J)OÀC 6622023.7893622194.769À2722132.473322073.2267 6722021.1111922192.9412022129.66436822018.4092022190.968À3422126.807À26922015.6521122189.014À2522123.915222062.047À15 7022012.8691722187.027À622120.974022058.255À3 7122010.0331522184.979À322117.99107222007.147622182.886À222114.950À1522050.53713 7322004.223322180.756622111.871À2422046.591À1 7422001.258222042.6246 7521998.251422038.62422 7622034.5431 7721992.100022030.4488 7821988.966822026.284À10 7921985.767À522022.091À15 8021982.532À1022017.8815 8121979.27478221975.95148321972.568À158421969.17428521965.714À18621962.2162Note.OÀC are observed minus calculated values in the units of10À3cmÀ1.Table2Case(c)spectroscopic constants(in cmÀ1)for the X0+,A1,[23.8]1,0+and0Àstates of ReNConstants a X0+A1[23.8]10+0ÀT000.02616.263(11)23746.4178(12)24726.098(11)24840.623(11) B00.481100(25)0.480888(14)0.439780(25)0.461679(14)0.460407(14) 107·D0 3.922(82) 3.384(20) 4.704(80) 4.245(19) 3.783(20) 1012·H0 3.76(83)— 3.11(80)——104·q0— 2.4589(38)À4.738(12)——109·q D0—— 5.194(26)——a Values in parentheses are one standard deviation in the last digits quoted.Table3Case(a)spectroscopic constants(in cmÀ1)for the X3RÀ,[23.8]1,0+and0Àstates of ReNConstants a X3RÀ[23.8]10+0ÀT000.022002.3830(74)b22982.0294(39)b23096.5547(44)b B00.4809566(75)0.439641(11)0.4617418(71)0.4604696(71) 107·D0 3.499(13) 4.313(12) 4.340(12) 3.880(13) 1013·H0 2.20(63)———104·q0—À4.742(12)——109·q D0— 5.33(25)——101·c0 1.5314(71)———k01308.5154(54)———104·k D0À1.862(44)———a Values in parentheses are one standard deviation in the last digits quoted.b The T00values are different from those in Table2since the zero energy is different for the case(a)fit.196R.S.Ram et al./Journal of Molecular Spectroscopy246(2007)192–19722500cmÀ1region using a Fourier transform spectrometer. These bands have been assigned as the0–0bands of the0+–A1and0À–A1transitions of ReN.The22110cmÀ1band also has its upper state in common with the[24.7]0+–X0+ transition observed previously by Balfour et al.[7,8].This observation places the A1state at2616.26cmÀ1above the ground X0+state.The common lower state,A1,is most probably the X=1spin component of the X3RÀground state of ReN.This work provides improved spectroscopic constants for thefirst excited state,A1,of ReN. AcknowledgmentWe thank M.Dulick of the National Solar Observatory for assistance in obtaining the spectra.The National Solar Observatory is operated by the association of Universities for research in Astronomy,Inc.,under contract with the National Science Foundation.The research described here was supported by funds from the NASA laboratory astro-physics program.Some support was also provided by the Natural Sciences and Engineering Research Council of Canada.References[1]F.A.Cotton,G.Wilkinson,C.A.Murillo,M.Bochmann,AdvancedInorganic Chemistry,A Comprehensive Text,Wiley,New York, 1999.[2]N.N.Greenwood,A.Earnshaw,The Chemistry of the Elements,Pergamon Press,Oxford,1990.[3]D.R.Lide,CRC Handbook of Chemistry and Physics,73rd ed.,CRCPress,Boca Raton,1992.[4]F.Gassner,E.Dinjus,H.Gorls,W.Leitner,Organometallics15(1996)2078–2082.[5]M.A.Casado,J.J.Perez-Torrente,M.A.Ciriano,L.A.Oro, A.Orejon,C.Claver,Organometallics18(1999)3035–3044.[6]R.S.Ram,P.F.Bernath,W.J.Balfour,J.Cao,C.X.W.Qian,S.J.Rixon,J.Mol.Spectrosc.168(1994)350–362.[7]W.J.Balfour,J.Cao,C.X.W.Qian,S.J.Rixon,J.Mol.Spectrosc.183(1997)113–118.[8]J.Cao,W.J.Balfour,C.X.W.Qian,J.Phys.Chem.A101(1997)6741–6745.[9]M.Zhou,L.Andrews,J.Phys.Chem.A102(1998)9061–9071.[10]T.C.Steimle,W.L.Virgo,J.Chem.Phys.121(2004)12411–12420.[11]B.A.Palmer,R.Engleman,Atlas of the Thorium Spectrum,LosAlamos National Laboratory,Los Alamos,1983.[12]C.R.Brazier,R.S.Ram,P.F.Bernath,J.Mol.Spectrosc.120(1986)381–402.[13]H.Lefebvre-Brion,R.W.Field,Perturbations in the Spectra ofDiatomic Molecules,Academic Press,Inc.,Orlando,1986.R.S.Ram et al./Journal of Molecular Spectroscopy246(2007)192–197197。
数字与模拟通信系统英文版第八版教学设计

Digital and Analog Communications Systems Teaching Design forEighth EditionIntroductionDigital and analog communication systems are imperative in today’s world as they allow people to exchange information and ideas over long distances. The communication systems range from simple computer networks to advanced satellite and radio communication systems. The eighth edition of theDigital and Analog Communications Systems book provides students with an in-depth understanding of digital and analog communication systems. This teaching design will outline the different topics covered in the book and how instructors should approach teaching them.Course ObjectivesInstructors should m to achieve the following objectivesat the end of the course:•Understanding the fundamentals of digital and analog communication systems.•Developing the knowledge and skills required to design and analyze communication systems.•Understanding the design principles of carrier modulated communication systems, spectral analysis, andnoise in communication systems.•Developing the ability to solve practical communication system problems.•Understanding the implementation, performance analysis, and design of digital communications systems.Course Content and TopicsThe eighth edition of Digital and Analog Communication Systems covers a wide range of topics that are crucial to understanding digital and analog communication systems. Here is an overview of the topics covered in the book: Chapter 1: Introduction to Communication Systems•Overview of communication systems•Types of communication systems•Elements of Communication systemsChapter 2: Fourier Analysis of Signals and Systems•Introduction to Fourier series and Fourier transform•Properties of Fourier transform•Fourier spectrum analysis for periodic signals•Fourier transform analysis for non-periodic signalsChapter 3: Amplitude Modulation•Amplitude modulation and demodulation•DSB modulators•Single sideband (SSB) modulation•Vestigial sideband (VSB)Chapter 4: Angle Modulation•Phase Modulation•Frequency Modulation•Frequency DiscriminatorsChapter 5: Probability and Random Variables •Probability Theory•Uniform Distribution•Gaussian Distribution•Chi-Square Distribution•Properties of Random Variables Chapter 6: Random Processes•Stationary Random Processes•Gaussian Random Process•Narrowband Random Process•Power Spectral DensityChapter 7: Noise in Analog Communication Systems•Introduction to Thermal Noise•Signal-To-Noise Ratio•Noise in Amplitude Modulation•Noise in Angle ModulationChapter 8: Digital Communications Principles•Introduction to Digital Communications•Source Coding Principles•Channel Coding Principles•Error Detection and Correction Codes•Digital Modulation PrinciplesChapter 9: Information Theory and Coding•Entropy and Information rate•Noiseless Coding and Huffman Codes•Shannon’s Source and Channel Coding Theorems•Error-Correcting CodesTeaching Methods and EvaluationThe teaching of digital and analog communication systems requires a mix of theoretical and practical knowledge. Instructors can adopt various teaching methods, including:•Lectures, which provide students with theoretical knowledge and principles of communication systems.•Tutorials, which involve solving practical communication system problems.•Assignments, which can be given to evaluate the students’ knowledge of different communication systemtopics.•Projects, which provide students with the opportunity to design and implement a communicationsystem of their choice.Students’ progress can be evaluated thr ough continuous assessment via assignments, projects, oral presentations, and exams. Instructors can use various evaluation criteria, such as the ability to solve communication system problems, the ability to identify and analyze communication system faults, and knowledge of communication system design principles.ConclusionThe eighth edition of Digital and Analog Communication Systems provides a comprehensive overview of digital and analog communication systems. Instructors can use this teaching design to guide students in understanding the various concepts covered in the book. The course contentcovers the essential topics that students need to develop the skills and knowledge required to design and analyze communication systems. With the knowledge gned from this course, students can effectively design and implement digital and analog communication systems that meet various communication needs.。
基于NHHT的小电流接地系统单相接地故障特性研究(IJISA-V8-N12-6)
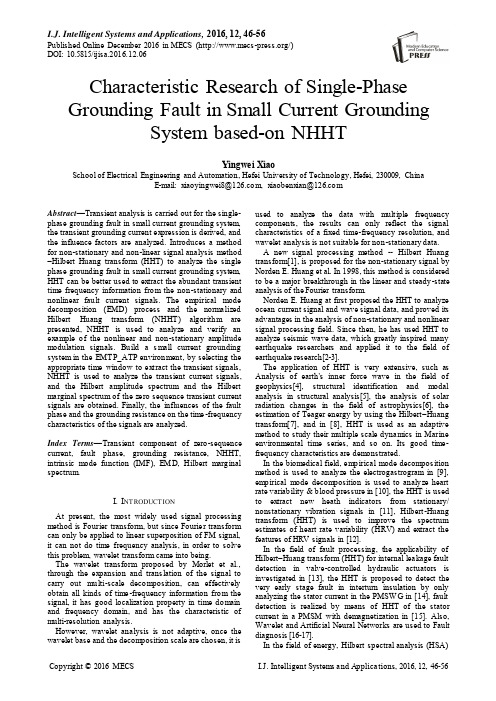
I. INT RODUCT ION At present, the most widely used signal processing method is Fourier transform, but since Fourie r transform can only be applied to linear superposition of FM signal, it can not do time frequency analysis, in order to solve this problem, wavelet transform came into being. The wavelet transform proposed by Morlet et al., through the expansion and trans lation of the signal to carry out mu lti-scale deco mposition, can effectively obtain all kinds of time -frequency information fro m the signal, it has good localizat ion property in time do main and frequency domain, and has the characteristic of multi-resolution analysis. However, wavelet analysis is not adaptive, once the wavelet base and the decomposition scale are chosen, it is Copyright © 2016 MECS
心脏超声中英文对照词汇

Left atrium
左心左心室长轴切面
Left ventricle, LV
左心室发育不全综合征
Hypoplastic left heart syndrome
左心室收缩末期内径
Left ventricle end systolic dimension, LVEDD
左心室流出道梗阻
Left ventricle outflow obstruction
取样容积
Sample volume
图像分辨率
Image resolution
实时频谱分析
Real-time spectral analysis
房间隔缺损
Atrial septal defect, ASD
房间隔脂肪瘤样肥厚
Lipomatous hypertrophy of the atrial septum
胸骨旁短轴切面
能量对比成像
脂肪瘤
欢迎您的下载,
资料仅供参考!
致力为企业和个人提供合同协议,策划案计划书,学习资料等等
打造全网一站式需求
三尖瓣前瓣
Anterior tricuspid valve, ATV
三尖瓣狭窄
Tricuspid valve stenosis
三尖瓣疾病
Tricuspid disease
三尖瓣隔瓣
Septal tricuspid valve, STV
三维超声心动图
Three-dimensional echocardiography
折射
Refraction
时间分辨率
Temporal resolution
时间增益补偿
Time gain compensation
脉冲序列的傅里叶变换计算
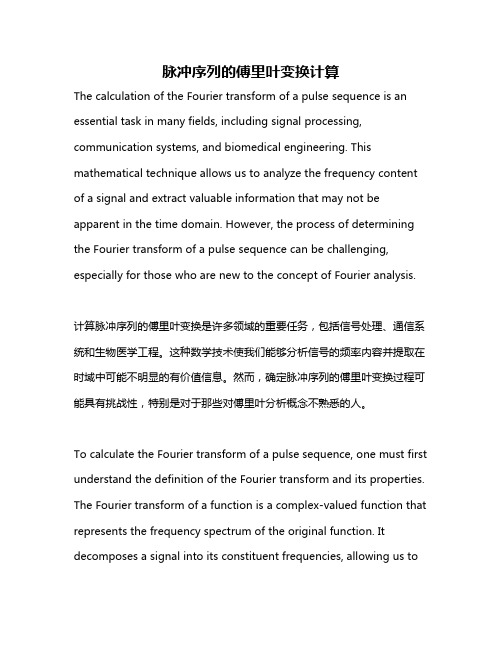
脉冲序列的傅里叶变换计算The calculation of the Fourier transform of a pulse sequence is an essential task in many fields, including signal processing, communication systems, and biomedical engineering. This mathematical technique allows us to analyze the frequency content of a signal and extract valuable information that may not be apparent in the time domain. However, the process of determining the Fourier transform of a pulse sequence can be challenging, especially for those who are new to the concept of Fourier analysis.计算脉冲序列的傅里叶变换是许多领域的重要任务,包括信号处理、通信系统和生物医学工程。
这种数学技术使我们能够分析信号的频率内容并提取在时域中可能不明显的有价值信息。
然而,确定脉冲序列的傅里叶变换过程可能具有挑战性,特别是对于那些对傅里叶分析概念不熟悉的人。
To calculate the Fourier transform of a pulse sequence, one must first understand the definition of the Fourier transform and its properties. The Fourier transform of a function is a complex-valued function that represents the frequency spectrum of the original function. It decomposes a signal into its constituent frequencies, allowing us toanalyze its frequency content. The properties of the Fourier transform, such as linearity, time shifting, and frequency shifting, are crucial for simplifying the calculation process and understanding the relationship between the time and frequency domains.要计算脉冲序列的傅里叶变换,首先必须了解傅里叶变换的定义及其性质。
稀疏恢复和傅里叶采样
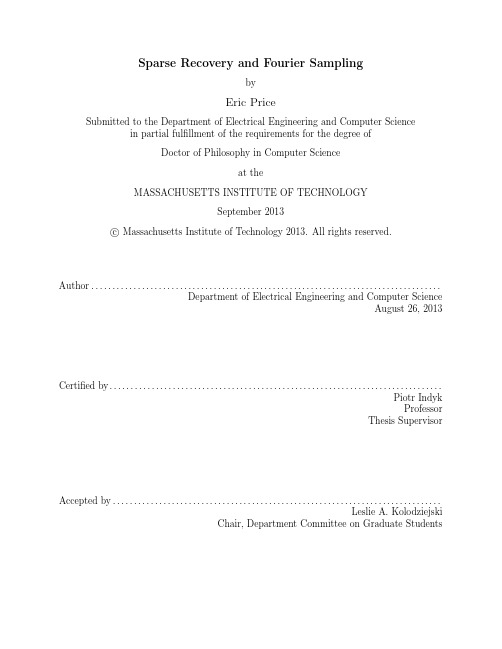
Accepted by . . . . . . . . . . . . . . . . . . . . . . . . . . . . . . . . . . . . . . . . . . . . . . . . . . . . . . . . . . . . . . . . . . . . . . . . . . . . . . Leslie A. Kolodziejski Chair, Department Committee on Graduate Students
2
Sparse Recovery and Fourier Sampling by Eric Price
Submitted to the Department of Electrical Engineering and Computer Science on August 26, 2013, in partial fulfillment of the requirements for the degree of Doctor of Philosophy in Computer Science
Author . . . . . . . . . . . . . . . . . . . . . . . . . . . . . . . . . . . . . . . . . . . . . . . . . . . . . . . . . . . . . . . . . . . . . . . . . . . . . . . . . . . Department of Electrical Engineering and Computer Science August 26, 2013
Certified by . . . . . . . . . . . . . . . . . . . . . . . . . . . . . . . . . . . . . . . . . . . . . . . . . . . . . . . . . . . . . . . . . . . . . . . . . . . . . . . Piotr Indyk Professor Thesis Supervisor
美国国家自然科学基金
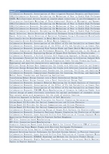
窗口傅里叶变换
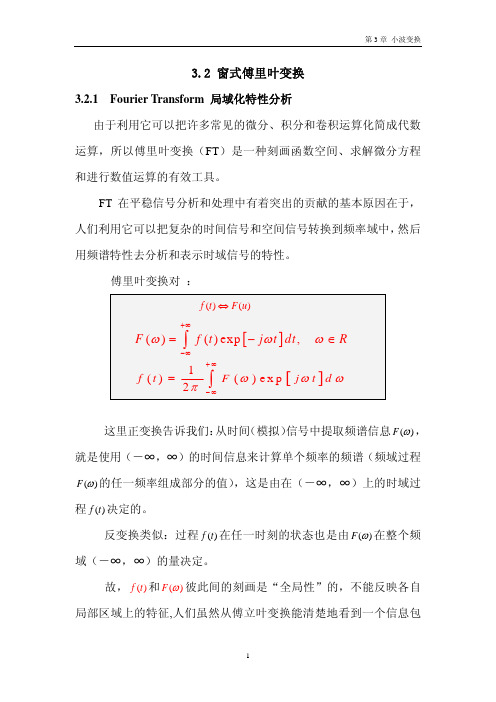
∫ Gf (ξ ,u) = f (t)g (t − u) e− jξtdt R
( ) 其中积分核 g t − u e− jξt : The windowed Fourier transform
family of atoms is obtained by time translations and frequency modulations of the original window. This atom has a frequency center
Fig. 1 a stationary signal
2
And the following is its FT:
第 3 章 小波变换
Fig.2
The next figure shows A signal with four different frequency components at four different time intervals, hence a non-stationary signal. The interval 0 to 300 ms has a 100 Hz sinusoid, the interval 300 to 600 ms has a 50 Hz sinusoid, the interval 600 to 800 ms has a 25 Hz sinusoid, and finally the interval 800 to 1000 ms has a 10 Hz sinusoid.
(2)
也就是说,若要一个函数 g (t) 作为时窗,其谱函数 G(ω) 作为频窗,
则 g(t) 和 G(ω) 同时具有较强的衰减性,应同时满足以上两式。
例 取时窗函数
傅里叶变换红外光谱和漫反射傅里叶变换红外光谱
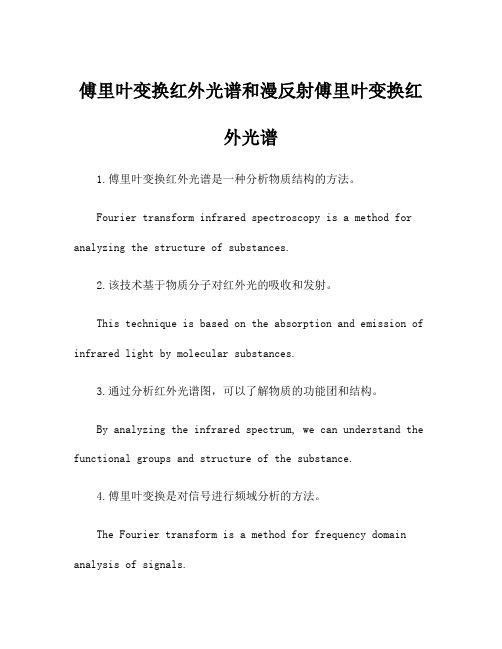
傅里叶变换红外光谱和漫反射傅里叶变换红外光谱1.傅里叶变换红外光谱是一种分析物质结构的方法。
Fourier transform infrared spectroscopy is a method for analyzing the structure of substances.2.该技术基于物质分子对红外光的吸收和发射。
This technique is based on the absorption and emission of infrared light by molecular substances.3.通过分析红外光谱图,可以了解物质的功能团和结构。
By analyzing the infrared spectrum, we can understand the functional groups and structure of the substance.4.傅里叶变换是对信号进行频域分析的方法。
The Fourier transform is a method for frequency domain analysis of signals.5.通过傅里叶变换,可以将时域信号转化为频域信号。
Through Fourier transform, the time domain signal can be converted into frequency domain signal.6.在红外光谱分析中,傅里叶变换可以提取出样品的结构信息。
In infrared spectroscopy analysis, Fourier transform can extract structural information from the sample.7.这种分析方法对于化学品的鉴别和质量控制非常重要。
This analysis method is very important for theidentification and quality control of chemicals.8.傅里叶变换红外光谱还可以用于药物分析和环境监测。
超导脉冲傅里叶变换核磁共振波谱测试方法通则

超导脉冲傅里叶变换核磁共振波谱测试方法通则1.超导脉冲傅里叶变换核磁共振波谱检测方法是一种常用的分析化学方法。
The superconducting pulse Fourier transform nuclear magnetic resonance spectroscopy detection method is a commonly used analytical chemistry method.2.该方法可用于分析样品中的核磁共振信号,并提供有关化合物结构和组成的信息。
This method can be used to analyze nuclear magnetic resonance signals in samples and provide information about the structure and composition of compounds.3.通过将样品置于强磁场中,并施加脉冲序列,可以激发核磁共振现象。
By placing the sample in a strong magnetic field and applying a pulse sequence, nuclear magnetic resonance phenomena can be excited.4.随后,通过傅里叶变换处理,可以将获得的时间域数据转换为频谱图。
Subsequently, the obtained time-domain data can be transformed into a spectrum by Fourier transformation processing.5.该频谱图可以显示样品中的不同核的化学环境以及它们之间的相互作用。
The spectrum can show the chemical environments of different nuclei in the sample and their interactions.6.超导脉冲傅里叶变换核磁共振波谱检测方法需要仪器设备的支持。
乙酸丙酯红外光谱的操作流程
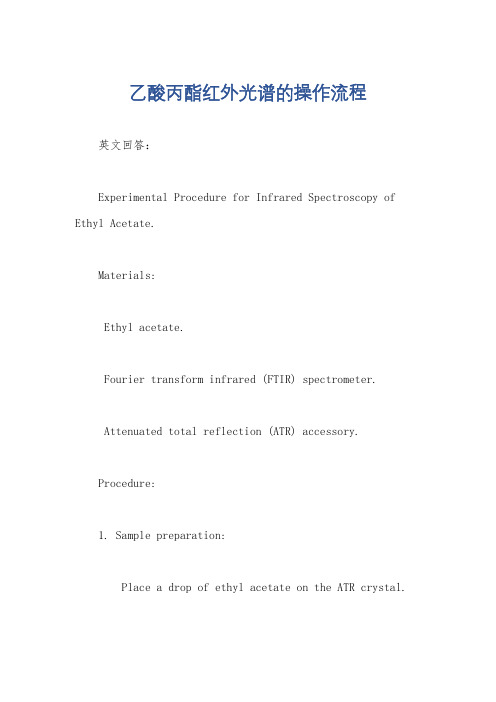
乙酸丙酯红外光谱的操作流程英文回答:Experimental Procedure for Infrared Spectroscopy of Ethyl Acetate.Materials:Ethyl acetate.Fourier transform infrared (FTIR) spectrometer.Attenuated total reflection (ATR) accessory.Procedure:1. Sample preparation:Place a drop of ethyl acetate on the ATR crystal.2. Spectrometer setup:Ensure the FTIR spectrometer is calibrated and the ATR accessory is securely attached.Set the scan range to cover the region of interest (typically 4000-400 cm^-1).3. Data acquisition:Collect a background spectrum without the sample on the ATR crystal.Place the ethyl acetate sample on the ATR crystal and collect a sample spectrum.4. Data analysis:Identify the characteristic absorption bands of ethyl acetate in the sample spectrum.Compare the sample spectrum to a reference spectrumor literature data to confirm the identity of the sample.中文回答:乙酸丙酯红外光谱操作流程。
Fourier Transform Infrared spectrometry傅里叶变换红外光谱法
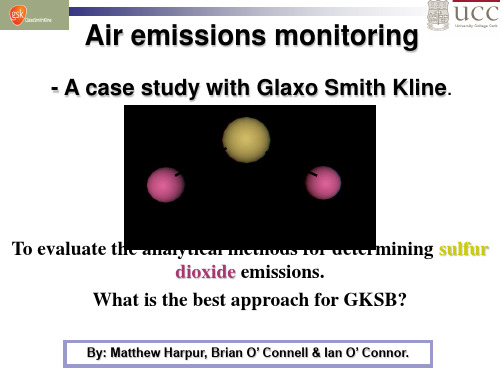
The EPA has a team that carry out spot checks on flue gas
monitoring, occasionally throughout the year to validate results.
To date GSK have been in full compliance with EPA regulations
This requires all industrial operations covered by the directive
to obtain a permit from authorities within the country.
The Permit is based on the concept of Best Available
The units operate at a
temperature of 1100 0C and there is 99.99% removal of the toxic waste.
By products of the incineration
红外光谱技术在淀粉粒有序结构分析中的应用
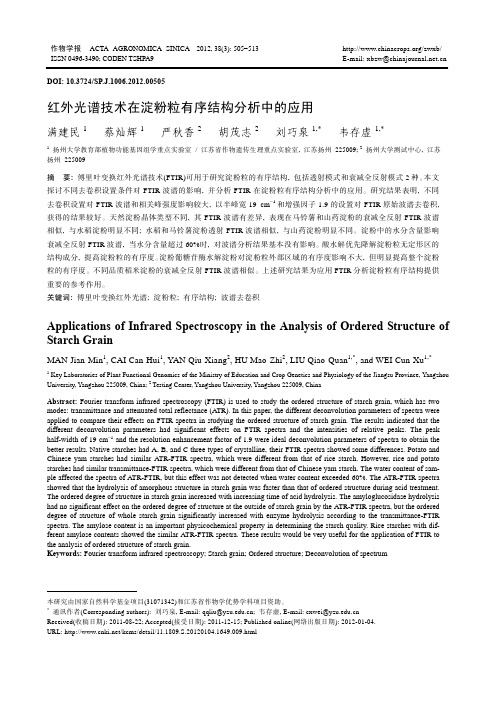
作物学报ACTA AGRONOMICA SINICA 2012, 38(3): 505-513 http:///zwxb/ ISSN 0496-3490; CODEN TSHPA9E-mail: xbzw@DOI: 10.3724/SP.J.1006.2012.00505红外光谱技术在淀粉粒有序结构分析中的应用满建民1蔡灿辉1严秋香2胡茂志2刘巧泉1,*韦存虚1,*1扬州大学教育部植物功能基因组学重点实验室 / 江苏省作物遗传生理重点实验室, 江苏扬州 225009; 2扬州大学测试中心, 江苏扬州 225009摘要: 傅里叶变换红外光谱技术(FTIR)可用于研究淀粉粒的有序结构, 包括透射模式和衰减全反射模式2种。
本文探讨不同去卷积设置条件对FTIR波谱的影响, 并分析FTIR在淀粉粒有序结构分析中的应用。
研究结果表明, 不同去卷积设置对FTIR波谱和相关峰强度影响较大, 以半峰宽19 cm-1和增强因子1.9的设置对FTIR原始波谱去卷积,获得的结果较好。
天然淀粉晶体类型不同, 其FTIR波谱有差异, 表现在马铃薯和山药淀粉的衰减全反射FTIR波谱相似, 与水稻淀粉明显不同; 水稻和马铃薯淀粉透射FTIR波谱相似, 与山药淀粉明显不同。
淀粉中的水分含量影响衰减全反射FTIR波谱, 当水分含量超过60%时, 对波谱分析结果基本没有影响。
酸水解优先降解淀粉粒无定形区的结构成分, 提高淀粉粒的有序度。
淀粉葡糖苷酶水解淀粉对淀粉粒外部区域的有序度影响不大, 但明显提高整个淀粉粒的有序度。
不同品质稻米淀粉的衰减全反射FTIR波谱相似。
上述研究结果为应用FTIR分析淀粉粒有序结构提供重要的参考作用。
关键词:傅里叶变换红外光谱; 淀粉粒; 有序结构; 波谱去卷积Applications of Infrared Spectroscopy in the Analysis of Ordered Structure of Starch GrainMAN Jian-Min1, CAI Can-Hui1, YAN Qiu-Xiang2, HU Mao-Zhi2, LIU Qiao-Quan1,*, and WEI Cun-Xu1,*1 Key Laboratories of Plant Functional Genomics of the Ministry of Education and Crop Genetics and Physiology of the Jiangsu Province, Yangzhou University, Yangzhou 225009, China;2 Testing Center, Yangzhou University, Yangzhou 225009, ChinaAbstract: Fourier transform infrared spectroscopy (FTIR) is used to study the ordered structure of starch grain, which has two modes: transmittance and attenuated total reflectance (ATR). In this paper, the different deconvolution parameters of spectra were applied to compare their effects on FTIR spectra in studying the ordered structure of starch grain. The results indicated that the different deconvolution parameters had significant effects on FTIR spectra and the intensities of relative peaks. The peak half-width of 19 cm-1 and the resolution enhancement factor of 1.9 were ideal deconvolution parameters of spectra to obtain the better results. Native starches had A, B, and C three types of crystalline, their FTIR spectra showed some differences. Potato and Chinese yam starches had similar ATR-FTIR spectra, which were different from that of rice starch. However, rice and potato starches had similar transmittance-FTIR spectra, which were different from that of Chinese yam starch. The water content of sam-ple affected the spectra of ATR-FTIR, but this effect was not detected when water content exceeded 60%. The ATR-FTIR spectra showed that the hydrolysis of amorphous structure in starch grain was faster than that of ordered structure during acid treatment. The ordered degree of structure in starch grain increased with increasing time of acid hydrolysis. The amyloglucosidase hydrolysis had no significant effect on the ordered degree of structure at the outside of starch grain by the ATR-FTIR spectra, but the ordered degree of structure of whole starch grain significantly increased with enzyme hydrolysis according to the transmittance-FTIR spectra. The amylose content is an important physicochemical property in determining the starch quality. Rice starches with dif-ferent amylose contents showed the similar ATR-FTIR spectra. These results would be very useful for the application of FTIR to the analysis of ordered structure of starch grain.Keywords: Fourier transform infrared spectroscopy; Starch grain; Ordered structure; Deconvolution of spectrum本研究由国家自然科学基金项目(31071342)和江苏省作物学优势学科项目资助。
- 1、下载文档前请自行甄别文档内容的完整性,平台不提供额外的编辑、内容补充、找答案等附加服务。
- 2、"仅部分预览"的文档,不可在线预览部分如存在完整性等问题,可反馈申请退款(可完整预览的文档不适用该条件!)。
- 3、如文档侵犯您的权益,请联系客服反馈,我们会尽快为您处理(人工客服工作时间:9:00-18:30)。
a r X i v :a s t r o -p h /9612076v 1 7 D e c 19961Fourier spectrum analysis of the new solar neutrino capture rate data for the Homestake experimentH.J.Haubold aaOuter Space Office,United Nations,Vienna International Centre,P.O.Box 500,1400Vienna,AustriaThe paper provides results of the Fourier spectrum analysis of the new Ar-37production rate data of the Homestake solar neutrino experiment and compares them with results for earlier data,revealing the harmonic content in the Ar-37production in the Homestake experiment.1.THE SOLAR NEUTRINO PROBLEMAll four solar neutrino experiments (Homestake,Kamiokande,GALLEX,SAGE)ob-serve a deficit of solar neutrinos compared to the predictions of the Standard Solar Model.Additionally,any two of the three classes of solar neutrino experiments indicate that the largest suppression is in the middle of the solar neutrino spectrum (the Be-7line and the lower part of the B-8spectrum)[1].Among these four experiments the Homestake experi-ment is taking data for almost 25years [2,3].The reliability of the radiochemical method for detecting solar neutrinos has been tested recently by the GALLEX experiment [1].2.IS THE SOLAR NEUTRINO FLUX CONSTANT?All efforts to resolve the solar neutrino problem by improving solar,nuclear,and neutrino physics have not proven successful so far[4].This may also mean that the average solar neutrino flux extracted from the four experiments may not be the proper quantity to explain the production of neutrinos in the gravitationally stabilized solar fusion reactor [5,6].This conjecture is supported by the fact that the pattern of the detected solar neutrino flux in the four solar neutrino experiments hints at possible variation of the solar neutrino flux over time,which has been generated interest to look at time dependent phenomena [4,7–10]related to solar physics and neutrino physics.3.FOURIER SPECTRUM OF THE HOMESTAKE DATAFigs.1and 2show the Ar-37production rate data which Davis and collaborators [2,3]have acquired and analyzed in more than 25years.For the analysis of the radioactive decay of argon with a small number of counts the method of maximum likelihood was employed which brings in a wide range of error.These error bars are omitted in Figs.1and 2and will not be taken into account in the following analysis.Thus,Figs.1and 2show2only the mean values for the Ar-37production rate for the various individual experiments. The data are unevenly spaced and the two data sets contain only low numbers of data. Recently,the Ar-37production rate data,shown in Fig.1,have been reviewed,taking into account counter efficiencies,chemical yields,and background effects[3].The new analysis has yielded an overall rate approximately ten percent higher than the earlier analysis.The time distribution of the data in Figs.1and2is essentially unchanged.The combined maximum likelihood rate for the99experiments in Fig.2is0.480±0.032Ar-37 atoms per day in615tons of perchlorethylen or2.55SNU(1SNU=10−36captures/target atom/sec).This experimental result,compared with the theoretical predictions of8SNU for the Standard Solar Model,has become known as the solar neutrino problem[4].The following provides the Fourier spectrum analysis of the earlier Ar-37production data[9] and,for comparison,of the new data.The analysis shows that the review of the earlier data did not change essentially the resulting power spectral density that reveals a strong harmonic content in the data.To remove some of the high frequency noise from the data in Figs.1and2,thefive-point moving average is calculated,which adjusts each point to be the average value of thefive points around it.The results in Figs.3and4are longer than original by one point.This causes a slight phase shift in the display.The fast Fourier transform of a real series results in a complex series where the second half of the result is the mirror image of thefirst half.The power spectral density is the magnitude squared of thefirst half of the fast Fourier transform and factors out the length of the original series.The power spectral density in Figs.5and6is useful for comparing the frequency spectrum of different series and can be thought of as the power of a series at a particular frequency[9].4.CONCLUSIONThe investigation of the variation of the Ar-37production rate over time in the Homes-take solar neutrino experiment reveals several periodicities shorter than or almost equal to 11years.A number of harmonics contained in the Ar-37data set may have a non-random origin.It is a striking fact that the power spectrum density did not change significantly in the various analysis undertaken over the years while gradually accumulating data in the Homestake experiment.It can not be excluded at this point of time that the discovered harmonic content of the data reflects solar activity through a not yet known physical phenomena,seated in the deep interior of the sun.Attention is drawn to the existence of distinct peaks in the power spectrum density of the Ar-37production rate.Extending and improving of the data could be achieved through taking into account the results of the other three solar neutrino experiments(Kamiokande,GALLEX,SAGE)if they continue operating over a sufficient period of time.In conclusion,the present results confirm the existence of periodicities in the new set of Homestake data,previously reported for the earlier data.3Figure1.The observed Ar-37produc-tion rate in the Homestake experiment (”earlier”data)for runs18(1970.78)-109 (1990.04)[abscissa:time(years),ordinate: Ar-37production rate(atoms/day)].Figure2.The observed Ar-37produc-tion rate in the Homestake experiment (”new data”)for runs18(1970.78)-124 (1992.38)[abscissa:time(years),ordinate: Ar-37production rate(atoms/day)].Figure3.Five-point moving average of the Ar-37production rate shown in Fig.1.Figure4.Five-point moving average of the Ar-37production rate shown in Fig.2.4Figure5.The power spectral density for the Ar-37record(abscissa:frequency(cy-cles/year),ordinate:power)shown in Fig-ure1.Figure6.The power spectral density for the Ar-37record(abscissa:frequency(cy-cles/year),ordinate:power)shown in Fig-ure2.Fig.1and2exhibit periods of high and low Ar-37production rates indicating that there is an apparent change in the signal.In Figs.3and4one notes that thefive-point moving average in the periods1977to1980and1987to1990shows a strongly suppressed signal. The highest peakes in Figs.5and6appear at about10.0yr and0.5yr periodicities. Shorter periods of1.66yr and0.71yr can also be observed.REFERENCES1.T.A.Kirsten,Ann.N.Y.Acad.Sci.759(1995)1.2.R.Davis Jr.,Ann.N.Y.Acad.Sci.655(1992)209.3.R.Davis Jr.,presentation made at the188th meeting of the American AstronomicalSociety,9-13June1996,University of Wisconsin,Madison,Wisconsin,USA.4.J.N.Bahcall,Neutrino Astrophysics,Cambridge University Press,Cambridge,1989.5.H.J.Haubold and A.M.Mathai,Astrophys.Sp.Sci.228(1995)113.6. A.A.Grandpierre,Astr.Astrophys.308(1996)199.7.R.H.Dicke,Nature276(1978)676.8.G.F.Rabey and H.A.Hill,Ann.N.Y.Acad.Sci.571(1989)594.9.H.J.Haubold and J.Beer,in Solar-Terrestrial Variability and Global Change(Eds.W.Schroeder and J.P.Legrand),Proceedings of the IUGG/IAGA General Assembly, Vienna,1991.10.R.L.McNutt Jr.,Science270(1995)1635.。