[2010]Cross-media Retrieval Based on CSRN Clustering
基于十字注意力机制改进U-Transformer_的新冠肺炎影像分割
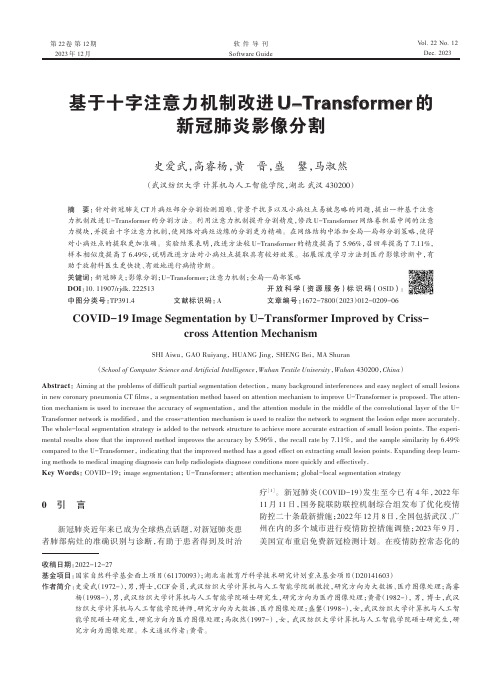
第 22卷第 12期2023年 12月Vol.22 No.12Dec.2023软件导刊Software Guide基于十字注意力机制改进U-Transformer的新冠肺炎影像分割史爱武,高睿杨,黄晋,盛鐾,马淑然(武汉纺织大学计算机与人工智能学院,湖北武汉 430200)摘要:针对新冠肺炎CT片病灶部分分割检测困难、背景干扰多以及小病灶点易被忽略的问题,提出一种基于注意力机制改进U-Transformer的分割方法。
利用注意力机制提升分割精度,修改U-Transformer网络卷积层中间的注意力模块,并提出十字注意力机制,使网络对病灶边缘的分割更为精确。
在网络结构中添加全局—局部分割策略,使得对小病灶点的提取更加准确。
实验结果表明,改进方法较U-Transformer的精度提高了5.96%,召回率提高了7.11%,样本相似度提高了6.49%,说明改进方法对小病灶点提取具有较好效果。
拓展深度学习方法到医疗影像诊断中,有助于放射科医生更快捷、有效地进行病情诊断。
关键词:新冠肺炎;影像分割;U-Transformer;注意力机制;全局—局部策略DOI:10.11907/rjdk.222513开放科学(资源服务)标识码(OSID):中图分类号:TP391.4 文献标识码:A文章编号:1672-7800(2023)012-0209-06COVID-19 Image Segmentation by U-Transformer Improved by Criss-cross Attention MechanismSHI Aiwu, GAO Ruiyang, HUANG Jing, SHENG Bei, MA Shuran(School of Computer Science and Artificial Intelligence,Wuhan Textile University,Wuhan 430200,China)Abstract:Aiming at the problems of difficult partial segmentation detection, many background interferences and easy neglect of small lesions in new coronary pneumonia CT films, a segmentation method based on attention mechanism to improve U-Transformer is proposed. The atten‐tion mechanism is used to increase the accuracy of segmentation, and the attention module in the middle of the convolutional layer of the U-Transformer network is modified, and the cross-attention mechanism is used to realize the network to segment the lesion edge more accurately. The whole-local segmentation strategy is added to the network structure to achieve more accurate extraction of small lesion points. The experi‐mental results show that the improved method improves the accuracy by 5.96%, the recall rate by 7.11%, and the sample similarity by 6.49% compared to the U-Transformer, indicating that the improved method has a good effect on extracting small lesion points. Expanding deep learn‐ing methods to medical imaging diagnosis can help radiologists diagnose conditions more quickly and effectively.Key Words:COVID-19; image segmentation; U-Transformer; attention mechanism; global-local segmentation strategy0 引 言新冠肺炎近年来已成为全球热点话题,对新冠肺炎患者肺部病灶的准确识别与诊断,有助于患者得到及时治疗[1]。
西氏公司丁基胶塞介绍材料
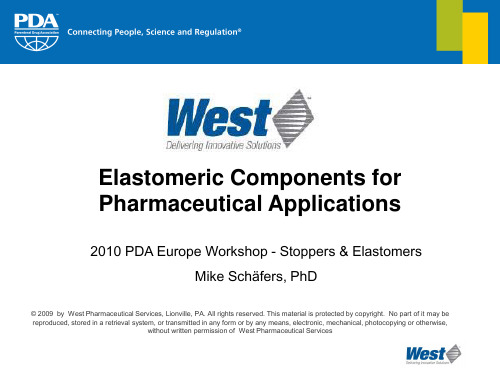
Final Inspection
Mixing control
Visual, dimensional Inspection
Compounding
Molding
Inspection Of Trimming Edge
Final Inspection List of Defects
Trimming
Final Treatment
Manufacturing Process
Raw Materials and Auxiliaries Weighing Mixing Dimensioning
Incoming Inspection
Overview of the rubber production process
Molding B2-Coating* Trimming Washing/Siliconization Automated Vision Inspection* Packaging Sterilization* Shipping
Lab Testing ( chemical)
Chemical/Physical Tests Acc. EP Other Pharmacopoeias Such As USP, JP Silicone Oil-Testing Functional Tests e.g. ISO 8536, Part 1, POF, etc. Pyrolysis - IR
Specification Limit: ≤ 5 CFUs per 100 cm2 of closure surface area
Proved Clean Index (PCI)
Rinse of ´Ready to Sterilize´ closures with appropriate solution, followed by filtration and counting of particles on filter Quantifies visible particulate into size ranges 25µ - 50µ; 51µ - 100µ; > 100µ Index calculated with larger particles having higher weight Does NOT count silicone particles
融合多尺度通道注意力的开放词汇语义分割模型SAN
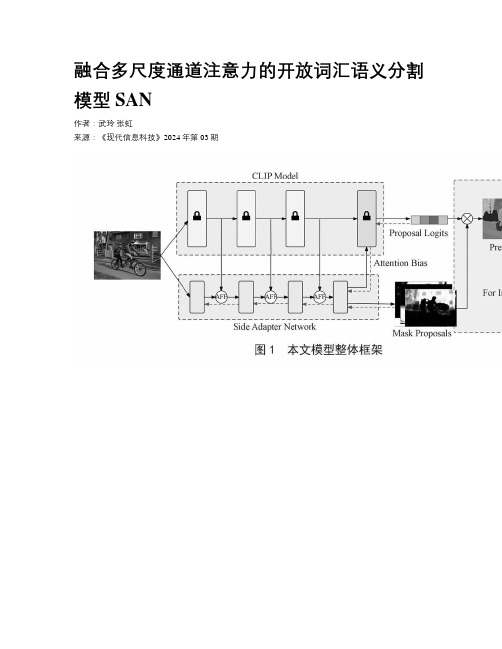
融合多尺度通道注意力的开放词汇语义分割模型SAN作者:武玲张虹来源:《现代信息科技》2024年第03期收稿日期:2023-11-29基金项目:太原师范学院研究生教育教学改革研究课题(SYYJSJG-2154)DOI:10.19850/ki.2096-4706.2024.03.035摘要:随着视觉语言模型的发展,开放词汇方法在识别带注释的标签空间之外的类别方面具有广泛应用。
相比于弱监督和零样本方法,开放词汇方法被证明更加通用和有效。
文章研究的目标是改进面向开放词汇分割的轻量化模型SAN,即引入基于多尺度通道注意力的特征融合机制AFF来改进该模型,并改进原始SAN结构中的双分支特征融合方法。
然后在多个语义分割基准上评估了该改进算法,结果显示在几乎不改变参数量的情况下,模型表现有所提升。
这一改进方案有助于简化未来开放词汇语义分割的研究。
关键词:开放词汇;语义分割;SAN;CLIP;多尺度通道注意力中图分类号:TP391.4;TP18 文献标识码:A 文章编号:2096-4706(2024)03-0164-06An Open Vocabulary Semantic Segmentation Model SAN Integrating Multi Scale Channel AttentionWU Ling, ZHANG Hong(Taiyuan Normal University, Jinzhong 030619, China)Abstract: With the development of visual language models, open vocabulary methods have been widely used in identifying categories outside the annotated label. Compared with the weakly supervised and zero sample method, the open vocabulary method is proved to be more versatile and effective. The goal of this study is to improve the lightweight model SAN for open vocabularysegmentation, which introduces a feature fusion mechanism AFF based on multi scale channel attention to improve the model, and improve the dual branch feature fusion method in the original SAN structure. Then, the improved algorithm is evaluated based on multiple semantic segmentation benchmarks, and the results show that the model performance has certain improvement with almost no change in the number of parameters. This improvement plan will help simplify future research on open vocabulary semantic segmentation.Keywords: open vocabulary; semantic segmentation; SAN; CLIP; multi scale channel attention 0 引言識别和分割任何类别的视觉元素是图像语义分割的追求。
海康威视产品说明书:无接触体温检测与戴口罩检测系统

Four adjustable lenses in one camera cover up to a 360° field of view, ensuring there are no monitoring blindspots. The monitoring tilt angle can also be adjusted.
Analysis
HikCentral
Trip & Fall Incidents
Loitering
Violent Motion
Guards check situation
Reception - Facial recognition & monitoring abnormal temperature.
NEED TO RECOGNIZE A VIP FROM YOUR BRANCH?
O ce & Customer Service Area - Indoor Panoramic Monitoring.
CAPTURE 360° IMAGES
MINI PANOVU CAMERAS
Hikvision’s PanoVu DS-2PT3326IZ-DE3 PanoVu Mini-Series Network PTZ camera, with integrated panoramic and PTZ cameras, is able to capture 360° images with its panoramic cameras, as well as detailed close-up images with the PTZ camera.
基于视觉特征的跨域图像检索算法的研究

摘要摘要随着成像传感器性能的不断发展和类型的不断丰富,同一事物来自不同成像载体、不同成像光谱、不同成像条件的跨域图像也日益增多。
为了高效地利用这些数字资源,人们往往需要综合多种不同的成像传感器以获得更加全面丰富的信息。
跨域图像检索就是研究如何在不同视觉域图像进行检索,相关课题已经成为当今计算机视觉领域的研究热点之一,并在很多领域都有广泛的应用,例如:异质图像配准与融合、视觉定位与导航、场景分类等。
因此,深入研究跨域图像之间的检索问题具有重要的理论意义和应用价值。
本文详细介绍了跨域图像检索的研究现状,深入分析了不同视觉域图像之间的内在联系,重点研究了跨域视觉显著性检测、跨域特征的提取与描述、跨域图像相似度度量这三个关键问题,并实现了基于显著性检测的跨域视觉检索方法、基于视觉词汇翻译器的跨域图像检索方法和基于共生特征的跨域图像检索方法。
论文的主要研究工作如下:(1)分析了跨域图像的视觉显著性,提出了一种基于显著性检测的跨域视觉检索方法。
该方法首先利用图像超像素区域的边界连接值,赋予各个区域不同的显著性值,获得主体目标区域;然后通过线性分类器进一步优化跨域特征,并对数据库图像进行多尺度处理;最后计算图像间的相似度,返回相似度得分最高的图像作为检索结果。
该方法有效地降低了背景等无关区域的干扰,提高了检索准确率。
(2)针对跨域图像特征差异较大无法直接进行匹配的问题,提出了一种基于视觉词汇翻译器的跨域图像检索方法。
该方法受语言翻译机制的启发,利用视觉词汇翻译器,建立了不同视觉域之间的联系。
该翻译器主要包含两部分:一是视觉词汇树,它可以看作是每个视觉域的字典;二是从属词汇树叶节点的索引文件,其中保存了不同视觉域间的翻译关系。
通过视觉词汇翻译器,跨域检索问题被转化为同域检索问题,从新的角度实现了跨视觉域间的图像检索。
实验验证了算法的性能。
(3)利用不同视觉域间的跨域共生相关性,提出了一种基于视觉共生特征的跨域图像检索方法。
移动互联网跨媒体信息检索技术
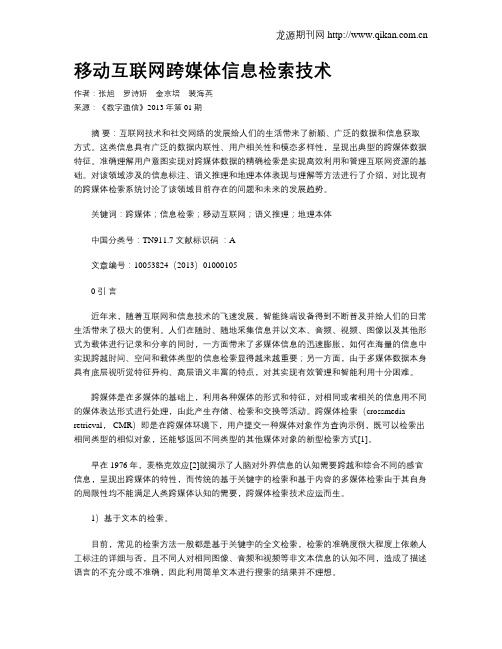
移动互联网跨媒体信息检索技术作者:张旭罗诗妍金京培裴海英来源:《数字通信》2013年第01期摘要:互联网技术和社交网络的发展给人们的生活带来了新颖、广泛的数据和信息获取方式。
这类信息具有广泛的数据内联性、用户相关性和模态多样性,呈现出典型的跨媒体数据特征。
准确理解用户意图实现对跨媒体数据的精确检索是实现高效利用和管理互联网资源的基础。
对该领域涉及的信息标注、语义推理和地理本体表现与理解等方法进行了介绍,对比现有的跨媒体检索系统讨论了该领域目前存在的问题和未来的发展趋势。
关键词:跨媒体;信息检索;移动互联网;语义推理;地理本体中国分类号:TN911.7 文献标识码:A文章编号:10053824(2013)010001050 引言近年来,随着互联网和信息技术的飞速发展,智能终端设备得到不断普及并给人们的日常生活带来了极大的便利。
人们在随时、随地采集信息并以文本、音频、视频、图像以及其他形式为载体进行记录和分享的同时,一方面带来了多媒体信息的迅速膨胀,如何在海量的信息中实现跨越时间、空间和载体类型的信息检索显得越来越重要;另一方面,由于多媒体数据本身具有底层视听觉特征异构、高层语义丰富的特点,对其实现有效管理和智能利用十分困难。
跨媒体是在多媒体的基础上,利用各种媒体的形式和特征,对相同或者相关的信息用不同的媒体表达形式进行处理,由此产生存储、检索和交换等活动。
跨媒体检索(crossmedia retrieval, CMR)即是在跨媒体环境下,用户提交一种媒体对象作为查询示例,既可以检索出相同类型的相似对象,还能够返回不同类型的其他媒体对象的新型检索方式[1]。
早在1976年,麦格克效应[2]就揭示了人脑对外界信息的认知需要跨越和综合不同的感官信息,呈现出跨媒体的特性,而传统的基于关键字的检索和基于内容的多媒体检索由于其自身的局限性均不能满足人类跨媒体认知的需要,跨媒体检索技术应运而生。
1)基于文本的检索。
cross-modal retrieval 指标

《探究跨模态检索指标》跨模态检索(cross-modal retrieval)是指在不同的数据模态之间进行相关内容的搜索和检索。
在信息检索领域,跨模态检索已经成为一个热门的话题,因为我们现在可以访问到各种类型的数据,比如文本、图像、视频和音频等。
针对这个主题,我们将首先从跨模态检索的定义开始,逐步深入探讨其相关指标及重要性。
1. 跨模态检索的定义跨模态检索是指在不同的模态之间进行信息检索和相似性匹配的过程。
在现实场景中,我们可能会面对不同类型的数据,比如从视觉图像到文本描述,或者从音频到视频内容。
跨模态检索的目标是在这些不同的数据模态之间建立联系,实现信息的交叉检索和匹配。
2. 跨模态检索指标的种类在跨模态检索任务中,有许多不同的指标可以用来评价检索结果的质量。
常见的指标包括但不限于以下几种:- 结构化相似度指标:用于衡量不同数据模态之间的结构化相似性,比如文本与图像之间的关联程度。
- 语义相关性指标:通过自然语言处理技术来度量文本和其他模态数据之间的语义相关性,比如使用词向量或者语义表示模型来衡量语义相似度。
- 图像相似度指标:针对图像模态数据,可以使用各种图像特征提取和相似度度量方法来评估图像之间的相似程度。
- 融合指标:结合多个模态数据的特征来计算综合的相似性指标,以更全面地评估跨模态检索结果的质量。
3. 指标选择的重要性在进行跨模态检索任务时,选择合适的指标非常重要。
不同的任务和应用场景可能需要不同的指标来评价检索结果。
比如在文本与图像的关联检索中,我们更关注语义相关性指标;而在音频与视频内容的匹配中,可能更关注结构化相似度指标。
在实际应用中,我们需要根据具体的任务需求来选择合适的指标进行评价。
4. 个人观点和理解在我看来,跨模态检索是一个非常具有挑战性和前沿的研究领域。
随着多模态数据的不断涌现,跨模态检索技术将对信息检索和智能推荐等领域产生重大影响。
在未来,我期待能够看到更多深度学习和多模态融合的技术应用于跨模态检索任务中,以提升检索结果的质量和效率。
参考文献

[1]卢汉清,刘静.基于图学习的自动图像标注[J]. 计算机学报,2008,31(9):1630-1645.[2]李志欣,施智平,李志清,史忠植.融合语义主题的图像自动标注[J].软件学报,2011,22(4):801-812.[3]Minyi Ke, Shuaihao Li, Yong Sun, Shengjun Cao . Research on similarity comparison by quantifying grey histogram based on multi-feature in CBIR [J]// Proceedings of the 3th International Conference on Education Technology and Training .IEEE Press.2010:422-424.[4] Wang Ke-Gang, Qi Li-Ying. Classification and Application of Images Based on Color Texture Feature[C]// Proceedings of 4th IEEE International Conference on Computer Science and Information Technology .IEEE Press. 2011:284-290.[5]Mohamed Maher Ben Ismail. Image Database Categorization based on a Novel Possibilistic Clustering and Feature Weighting Algorithm[C] // Proceedings of 2012 International Conference on Network and Computational Intelligence. 2012:122-127.[6]Du Gen-yuana, Miao Fang, Tian Sheng-li, Liu Ye.A modified fuzzy C-means algorithm in remote sensing image segmentation[C]// Proceedings of Environmental Science and Information Application Technology. 2009: 447-450.[7]Jeon J., Lavrenko V., Manmatha R. Automatic image annotation and retrieval using cross- media relevance models[C]// ACM SIGIR.ACM Press,2003:119- 126.[8]苗晓光,袁平波,何芳,俞能海. 一种新颖的自动图像标注方法[C].// 第十三届中国图象图形学术会议.2006:581-584参考文献正解[1] Smeulders A W M, Worring M, Santini S, et al. Content-based image retrieval at the end ofthe early years[J]. Pattern Analysis and Machine Intelligence, IEEE Transactions on,22(12),2000: 1349-1380.[2] Datta R, Joshi D, Li J, et al. Image retrieval: ideas, influences, and trends of the new age[J].ACM Computing Surveys (CSUR),40(2),2008: 5.[3] Mller H, Mller W, Squire D M G, et al. Performance evaluation in content-based imageretrieval: overview and proposals[J]. Pattern Recognition Letters,22(5),2001: 593-601.[4]Müller H, SO H E S. Text-based (image) retrieval[J]. HES SO//Valais, Sierre, Switzerland[Online] http://thomas. deselaers. de/teaching/files/tutorial_icpr08/03text Based Retrieval. pdf [Accessed 25 July 2010], 2007.[5]Zhao R, Grosky W I. Narrowing the semantic gap-improved text-based web document retrievalusing visual features[J]. Multimedia, IEEE Transactions on, 2002, 4(2): 189-200.[6]卢汉清,刘静.基于图学习的自动图像标注[J]. 计算机学报,2008,31(9):1630-1645.[7]李志欣,施智平,李志清.史忠植.融合语义主题的图像自动标注[J].软件学报,2011,22(4):801-812.[8] Li J, Wang JZ. Automatic linguistic indexing of pictures by a statistical modeling approach.IEEE Trans. on Pattern Analysis and Machine Intelligence, 2003,25(9):1075−1088. [doi:10.1109/TPAMI.2003.1227984][9] Chang E, Goh K, Sychay G, Wu G. CBSA: Content-Based soft annotation for multimodalimage retrieval using Bayes point machines. IEEE Trans. on Circuits and Systems for Video Technolo gy, 2003,13(1):26− 38. [doi: 10.1109/TCSVT.2002.808079][10]Carneiro G, Chan AB, Moreno PJ, Vasconcelos N. Supervised learning of semantic classes forimage annotation and retrieval. IEEE Trans. on Pattern Analysis and Machine Intelligence, 2007,29(3):394 − 410. [doi: 10.1109/TPAMI.2007.61][11]Blei DM, Jordan MI. Modeling annotated data. In: Proc. of the 26th Int’l ACM SIGIR Conf.on Research and Development in Information Retrieval. New York: ACM Press, 2003. 127− 134. [doi: 10.1145/860435.860460][12]Barnard K, Duygulu P, Forsyth D, de Freitas N, Blei DM, Jordan MI. Matching words andpictures. Journal of Machine Learning Research, 2003,3(2):1107 − 1135. [doi:10.1162/153244303322533214][13] LA VRENKO V, JEON J. Automatic image annotation and retrieval using cross-mediarelevance models. [C]//Proceeding of the 26th ACM SIGIR Conf. on Research and Development in Information Retrieval . New York: ACM, 2003: 119 − 126.[14]MINVI KE, SHUAIHAO LI, YONG SUN, SHENGJUN CAO. Research on similaritycomparison by quantifying grey histogram based on multi-feature in CBIR [C]//Proceeding of the 3rd International Conference on Education Technology and Training .IEEE,2010:422-424.[15] WANGKE GANG, QILI YING. Classification and application of images based on colortexture feature[C]// Proceedings of 4th IEEE International Conference on Computer Science and Information Technology .IEEE, 2011:284-290.[16]Mohamed Maher Ben Ismail. Image Database Categorization based on a Novel PossibilisticClustering and Feature Weighting Algorithm[C] // Proceedings of 2012 International Conference on Network and Computational Intelligence. 2012:122-127.[17]Du Gen-yuana, Miao Fang, Tian Sheng-li, Liu Ye.A modified fuzzy C-means algorithm inremote sensing image segmentation[C]// Proceedings of Environmental Science and Information Application Technology. 2009: 447-450.[18] Wang Ke-Gang, Qi Li-Ying. Classification and Application of Images Based on ColorTexture Feature[C]// Proceedings of 4th IEEE International Conference on Computer Science and Information Technology .IEEE Press. 2011:284-290.[19]Jeon J., Lavrenko V., Manmatha R. Automatic image annotation and retrieval using cross-media relevance models[C]// ACM SIGIR.ACM Press,2003:119- 126.[20]苗晓光,袁平波,何芳,俞能海. 一种新颖的自动图像标注方法[C].// 第十三届中国图象图形学术会议.2006:581-584.[21] Duygulu P, Barnard K, de Freitas J F G, et al. Object recognition as machine translation:Learning a lexicon for a fixed image vocabulary[M]//Computer Vision—ECCV 2002.Springer Berlin Heidelberg, 2002: 97-112.[22]王科平,王小捷,钟义信.加权特征自动图像标注方法[J].北京邮电大学学报,.2011:34(5):6-9.[23] Chen K, Li J, Ye L. Automatic Image Annotation Based on Region Feature[M]//Multimediaand Signal Processing. Springer Berlin Heidelberg, 2012: 137-145.[24]刘丽, 匡纲要. 图像纹理特征提取方法综述[J]. 中国图象图形学报, 2009, 14(4): 622-635.[25] 杨红菊, 张艳, 曹付元. 一种基于颜色矩和多尺度纹理特征的彩色图像检索方法[J]. 计算机科学, 2009, 36(9): 274-277.[26]Minyi Ke, Shuaihao Li, Yong Sun, Shengjun Cao . Research on similarity comparison byquantifying grey histogram based on multi-feature in CBIR [J]// Proceedings of the 3th International Conference on Education Technology and Training .IEEE Press.2010:422-424 [27] Mohamed Maher Ben Ismail. Image Database Categorization based on a Novel Possibilistic Clustering and Feature Weighting Algorithm[C] // Proceedings of 2012 International Conference on Network and Computational Intelligence. 2012:122-127.[28]Khalid Y I A, Noah S A. A framework for integrating DBpedia in a multi-modality ontologynews image retrieval system[C]//Semantic Technology and Information Retrieval (STAIR).IEEE, 2011: 144-149.[29]Celik T, Tjahjadi T. Bayesian texture classification and retrieval based on multiscale featurevector[J]. Pattern recognition letters, 2011, 32(2): 159-167.[30]Min R, Cheng H D. Effective image retrieval using dominant color descriptor and fuzzysupport vector machine[J]. Pattern Recognition, 2009, 42(1): 147-157.[31]Feng H, Shi R, Chua T S. A bootstrapping framework for annotating and retrieving WWWimages[C]//Proceedings of the 12th annual ACM international conference on Multimedia.ACM, 2004: 960-967.[22]Ke X, Chen G. Automatic Image Annotation Based on Multi-scale SalientRegion[M]//Unifying Electrical Engineering and Electronics Engineering.New York, 2014: 1265-1273.[33]Wartena C, Brussee R, Slakhorst W. Keyword extraction using wordco-occurrence[C]//Database and Expert Systems Applications (DEXA).IEEE, 2010: 54-58. [34]刘松涛, 殷福亮. 基于图割的图像分割方法及其新进展[J]. 自动化学报, 2012, 38(6):911-922.[35]陶文兵, 金海. 一种新的基于图谱理论的图像阈值分割方法[J]. 计算机学报, 2007, 30(1):110-119.[36]谭志明. 基于图论的图像分割及其嵌入式应用研究[D][J]. 博士学位论文) 上海交通大学,2007.[37] Shi J, Malik J. Normalized cuts and image segmentation[J]. Pattern Analysis and MachineIntelligence,2000, 22(8): 888-905.[38] Huang Z C, Chan P P K, Ng W W Y, et al. Content-based image retrieval using color momentand Gabor texture feature[C]//Machine Learning and Cybernetics (ICMLC), 2010 International Conference on. IEEE, 2010, 2: 719-724.[39]王涛, 胡事民, 孙家广. 基于颜色-空间特征的图像检索[J]. 软件学报, 2002, 13(10).[40] 朱兴全, 张宏江. iFind: 一个结合语义和视觉特征的图像相关反馈检索系统[J]. 计算机学报,2002, 25(7): 681-688.[41]Sural S, Qian G, Pramanik S. Segmentation and histogram generation using the HSV colorspace for image retrieval[C]//Image Processing. 2002. Proceedings. 2002 International Conference on. IEEE, 2002, 2: II-589-II-592 vol. 2.[42]Ojala T, Rautiainen M, Matinmikko E, et al. Semantic image retrieval with HSVcorrelograms[C]//Proceedings of the Scandinavian conference on Image Analysis. 2001: 621-627.[43]Yu H, Li M, Zhang H J, et al. Color texture moments for content-based imageretrieval[C]//Image Processing. 2002. Proceedings. 2002 International Conference on. IEEE, 2002, 3: 929-932.[44]Sun L, Ge H, Yoshida S, et al. Support vector description of clusters for content-based imageannotation[J]. Pattern Recognition, 2014, 47(3): 1361-1374.[45]Hiremath P S, Pujari J. Content based image retrieval using color, texture and shapefeatures[C]//Advanced Computing and Communications, 2007. ADCOM 2007.International Conference on. IEEE, 2007: 780-784.[46]Zhang D, Lu G. Generic Fourier descriptor for shape-based image retrieval[C]//Multimediaand Expo, 2002. ICME'02. Proceedings. 2002 IEEE International Conference on. IEEE, 2002, 1: 425-428.[47]Gevers T, Smeulders A W M. Pictoseek: Combining color and shape invariant features forimage retrieval[J]. Image Processing, IEEE Transactions on, 2000, 9(1): 102-119.[48] Bailloeul T, Zhu C, Xu Y. Automatic image tagging as a random walk with priors on thecanonical correlation subspace[C]//Proceedings of the 1st ACM international conference on Multimedia information retrieval. ACM, 2008: 75-82.[16]MOHAMED MAHER, BEN ISMAIL. Image database categorization based on a novelprobability clustering and feature weighting algorithm[C] // Proceedings of 2012 International Conference on Network and Computational Intelligence, 2012:122-127.[17]DU GENYUANA, TIAN SHENGLI, LIU YE.A modified fuzzy c-means algorithm in remotesensing image segmentation[C]// Proceedings of Environmental Science and Information Application Technology, 2009: 447-450.[18]SIVIC J, RUSSELL BC. Discovering objects and their location in images.[C] //Proceedingsof the 10th IEEE Int’l Conf. on Computer Vision .IEEE Computer Society, 2005:370 − 377.[19] DUYGULU P, BARNARD K, FORSYTH D. Object recognition as machine translation [J].//Learning a lexicon for a fixed image vocabulary In: HEYDEN A, NIELSEN M, JOHANSEN P, eds. Lecture Notes in Computer Science 2353,2002,45(1): 97−112.[20]JEON J, MANMA THA R. Automatic image annotation and retrieval using cross- mediarelevance models[C]// ACM SIGIR.ACM,2003:119- 126.[21]G K RAMANI and T ASSUDANI. Tag recommendation for photos[J].In Stanford CS229Class Project, 2009,23(1):130 − 145.[22] D. ZHANG and G. LU. A review on automatic image annotation techniques[J]. PatternRecognition, 2011,145(1):346–362.[23]K.BARNARD,P.DUGGULU,N FREITAS,D FORSYTH, D BLEI. Matching words andpictures [J].Journal of Machine Learning Research,2003,132(2):1107-1135.[24]苗晓光,袁平波,何芳,俞能海. 一种新颖的自动图像标注方法[C].// 第十三届中国图象图形学术会议.2006:581-584.[25] 王科平,王小捷, 钟义信.加权特征自动图像标注方法[J].北京邮电大学学报,2011,34(5):6−9.[26] JIN R, KANG F, SUKTHANKAR R. Correlated label propagation with application tomulti-label learning [C]. //Proceedings of the IEEE Computer Society Conference on Computer Vision and Pattern Recognition.2006:119-126.[27]YANG C.B, DONG, M, HUA J. Region-based image annotation using asymmetrical supportvector machine-based multiple-instance learning[C].// Proceeding of the CVPR.2006:2057–2063.[28] CARNEIRO G, V ASCONCELOS N. A database centric view of semantic image annotationand retrieval[C].// Proceeding of ACM SIGIR. 2005:559–566.[29] CUSANO C, CIOCCA G, SCHETTINI R. Image annotation using SVM[C].// Proceeding ofthe Internet Imaging, 2004: 330–338.[30]R Y AN, A HAUPTMANN, R JIN. Multimedia search with pseudo-relevancefeedback[C].//Proceeding of IEEE conference on Content-based Image and Video Retrieval.2007:238-247.[31] J W ANG, S KUMAR, S CHANG. Semi-supervised hashing for scalable imageretrieval[C].//Proceeding of IEEE conference on Computer Vision.2009:1-8.[32]M KOKARE, B CHATTERJI, P BISWAS. Comparison of similarity metrics for texture imageretrieval[C].//Proceeding of IEEE conference on Convergent Technologies for Asia-Pacific Region.2003:571-575.[33]EDWARD CHANG, KINGSHY GOH, GERARD SYCHAY, GANG WU. Content-base softannotation for multimodal image retrieval using bays point machines[J].CirSysVideo,2003,13(1):26-38.[34]SHI J, MALIK J. Normalized cuts and image segmentation[J].IEEE Transactions on PatternAnalysis and Machine Intelligence,2000,22(8):888-905.[35]ZHOU D, BOUSQUET O. Learning with local and global consistency[C].//Proceeding ofAdvances in Neural Information Proceeding Systems,2004:321-328.[36]Minyi Ke, Shuaihao Li, Yong Sun, Shengjun Cao . Research on similarity comparison byquantifying gray histogram based on multi-feature in CBIR [J]// Proceedings of the 3th International Conference on Education Technology and Training .IEEE Press.2010:422-424 [37] Wang Ke-Gang, Qi Li-Ying. Classification and Application of Images Based on ColorTexture Feature[C]// Proceedings of 4th IEEE International Conference on Computer Science and Information Technology .IEEE Press. PP:284-290 ,2011[38] Chen K, Li J, Ye L. Automatic Image Annotation Based on Region Feature[M]//Multimediaand Signal Processing. Springer Berlin Heidelberg, 2012: 137-145.[39]Lu Z, Ip H H S. Generalized relevance models for automatic image annotation [M]//Advancesin Multimedia Information Processing-PCM 2009. Springer Berlin Heidelberg, 2009: 245-255.[40]Fournier J, Cord M. A Flexible Search-by-Similarity Algorithm for Content-Based ImageRetrieval[C]//JCIS. 2002: 672-675.论文3[1]Zhang C, Chai J Y, Jin R. User term feedback in interactive text-based image retrieval[C]//Proceedings of the 28th annual international ACM SIGIR conference on Research and development in information retrieval. ACM, 2005: 51-58.[2]Akgül C B, Rubin D L, Napel S, et al. Content-based image retrieval in radiology: currentstatus and future directions[J]. Journal of Digital Imaging, 2011, 24(2): 208-222.[7]Zhang D, Islam M, Lu G. Structural image retrieval using automatic image annotation and region based inverted file[J]. Journal of Visual Communication and Image Representation, 2013, 24(7): 1087-1098.[8]Datta R, Joshi D, Li J, et al. Image retrieval: Ideas, influences, and trends of the new age[J]. ACM Computing Surveys (CSUR), 2008, 40(2): 111-115.[9]Li ZX, Shi ZP, Li ZQ, Shi ZZ. A survey of semantic mapping in image retrieval[J]. Journal of Computer-Aided Design and Computer Graphics, 2008,20(8):1085−1096 (in Chinese with English abstract).[10]Zhang D, Islam M M, Lu G. A review on automatic image annotation techniques[J]. Pattern Recognition, 2012, 45(1): 346-362.[11] Li J, Wang J Z. Automatic linguistic indexing of pictures by a statistical modeling approach[J]. Pattern Analysis and Machine Intelligence,2003, 25(9): 1075-1088.[12] Chang E, Goh K, Sychay G, et al. CBSA: content-based soft annotation for multimodal image retrieval using Bayes point machines[J]. Circuits and Systems for Video Technology,2003, 13(1): 26-38.[13]Jeon J, Lavrenko V, Manmatha R. Automatic image annotation and retrieval using cross-media relevance models[C]//Proceedings of the 26th annual international ACM SIGIR conference on Research and development in information retrieval. ACM,2003: 119−126.[14] Lavrenko V, Manmatha R, Jeon J. A Model for Learning the Semantics of Pictures[C]//NIPS. 2003: 11-18.[15]Feng S L, Manmatha R, Lavrenko V. Multiple bernoulli relevance models for image and video annotation[C]//Proceedings of the IEEE Computer Society conference onComputer Vision and Pattern Recognition.IEEE, 2005: 51-58.[16]Tian D, Zhao X, Shi Z. An Efficient Refining Image Annotation Technique by Combining Probabilistic Latent Semantic Analysis and Random Walk Model[J]. Intelligent Automation & Soft Computing, 2014 (ahead-of-print): 1-11.。
Peters (2010) Episodic Future Thinking Reduces Reward Delay Discounting
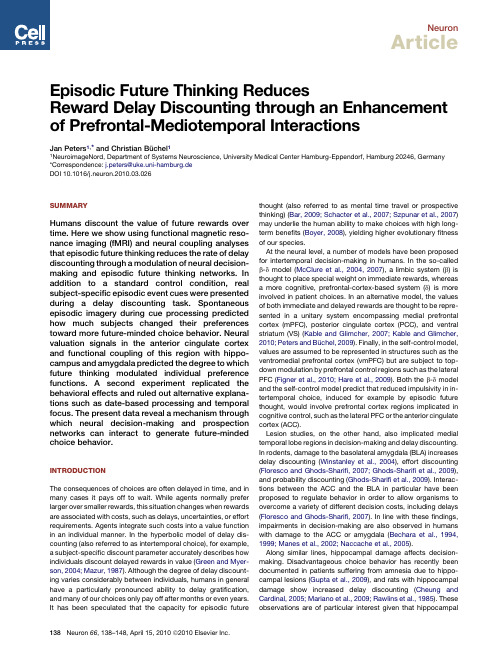
NeuronArticleEpisodic Future Thinking ReducesReward Delay Discounting through an Enhancement of Prefrontal-Mediotemporal InteractionsJan Peters1,*and Christian Bu¨chel11NeuroimageNord,Department of Systems Neuroscience,University Medical Center Hamburg-Eppendorf,Hamburg20246,Germany*Correspondence:j.peters@uke.uni-hamburg.deDOI10.1016/j.neuron.2010.03.026SUMMARYHumans discount the value of future rewards over time.Here we show using functional magnetic reso-nance imaging(fMRI)and neural coupling analyses that episodic future thinking reduces the rate of delay discounting through a modulation of neural decision-making and episodic future thinking networks.In addition to a standard control condition,real subject-specific episodic event cues were presented during a delay discounting task.Spontaneous episodic imagery during cue processing predicted how much subjects changed their preferences toward more future-minded choice behavior.Neural valuation signals in the anterior cingulate cortex and functional coupling of this region with hippo-campus and amygdala predicted the degree to which future thinking modulated individual preference functions.A second experiment replicated the behavioral effects and ruled out alternative explana-tions such as date-based processing and temporal focus.The present data reveal a mechanism through which neural decision-making and prospection networks can interact to generate future-minded choice behavior.INTRODUCTIONThe consequences of choices are often delayed in time,and in many cases it pays off to wait.While agents normally prefer larger over smaller rewards,this situation changes when rewards are associated with costs,such as delays,uncertainties,or effort requirements.Agents integrate such costs into a value function in an individual manner.In the hyperbolic model of delay dis-counting(also referred to as intertemporal choice),for example, a subject-specific discount parameter accurately describes how individuals discount delayed rewards in value(Green and Myer-son,2004;Mazur,1987).Although the degree of delay discount-ing varies considerably between individuals,humans in general have a particularly pronounced ability to delay gratification, and many of our choices only pay off after months or even years. It has been speculated that the capacity for episodic future thought(also referred to as mental time travel or prospective thinking)(Bar,2009;Schacter et al.,2007;Szpunar et al.,2007) may underlie the human ability to make choices with high long-term benefits(Boyer,2008),yielding higher evolutionaryfitness of our species.At the neural level,a number of models have been proposed for intertemporal decision-making in humans.In the so-called b-d model(McClure et al.,2004,2007),a limbic system(b)is thought to place special weight on immediate rewards,whereas a more cognitive,prefrontal-cortex-based system(d)is more involved in patient choices.In an alternative model,the values of both immediate and delayed rewards are thought to be repre-sented in a unitary system encompassing medial prefrontal cortex(mPFC),posterior cingulate cortex(PCC),and ventral striatum(VS)(Kable and Glimcher,2007;Kable and Glimcher, 2010;Peters and Bu¨chel,2009).Finally,in the self-control model, values are assumed to be represented in structures such as the ventromedial prefrontal cortex(vmPFC)but are subject to top-down modulation by prefrontal control regions such as the lateral PFC(Figner et al.,2010;Hare et al.,2009).Both the b-d model and the self-control model predict that reduced impulsivity in in-tertemporal choice,induced for example by episodic future thought,would involve prefrontal cortex regions implicated in cognitive control,such as the lateral PFC or the anterior cingulate cortex(ACC).Lesion studies,on the other hand,also implicated medial temporal lobe regions in decision-making and delay discounting. In rodents,damage to the basolateral amygdala(BLA)increases delay discounting(Winstanley et al.,2004),effort discounting (Floresco and Ghods-Sharifi,2007;Ghods-Sharifiet al.,2009), and probability discounting(Ghods-Sharifiet al.,2009).Interac-tions between the ACC and the BLA in particular have been proposed to regulate behavior in order to allow organisms to overcome a variety of different decision costs,including delays (Floresco and Ghods-Sharifi,2007).In line with thesefindings, impairments in decision-making are also observed in humans with damage to the ACC or amygdala(Bechara et al.,1994, 1999;Manes et al.,2002;Naccache et al.,2005).Along similar lines,hippocampal damage affects decision-making.Disadvantageous choice behavior has recently been documented in patients suffering from amnesia due to hippo-campal lesions(Gupta et al.,2009),and rats with hippocampal damage show increased delay discounting(Cheung and Cardinal,2005;Mariano et al.,2009;Rawlins et al.,1985).These observations are of particular interest given that hippocampal138Neuron66,138–148,April15,2010ª2010Elsevier Inc.damage impairs the ability to imagine novel experiences (Hassa-bis et al.,2007).Based on this and a range of other studies,it has recently been proposed that hippocampus and parahippocam-pal cortex play a crucial role in the formation of vivid event repre-sentations,regardless of whether they lie in the past,present,or future (Schacter and Addis,2009).The hippocampus may thus contribute to decision-making through its role in self-projection into the future (Bar,2009;Schacter et al.,2007),allowing an organism to evaluate future payoffs through mental simulation (Johnson and Redish,2007;Johnson et al.,2007).Future thinking may thus affect intertemporal choice through hippo-campal involvement.Here we used model-based fMRI,analyses of functional coupling,and extensive behavioral procedures to investigate how episodic future thinking affects delay discounting.In Exper-iment 1,subjects performed a classical delay discounting task(Kable and Glimcher,2007;Peters and Bu¨chel,2009)that involved a series of choices between smaller immediate and larger delayed rewards,while brain activity was measured using fMRI.Critically,we introduced a novel episodic condition that involved the presentation of episodic cue words (tags )obtained during an extensive prescan interview,referring to real,subject-specific future events planned for the respective day of reward delivery.This design allowed us to assess individual discount rates separately for the two experimental conditions,allowing us to investigate neural mechanisms mediating changes in delay discounting associated with episodic thinking.In a second behavioral study,we replicated the behavioral effects of Exper-iment 1and addressed a number of alternative explanations for the observed effects of episodic tags on discount rates.RESULTSExperiment 1:Prescan InterviewOn day 1,healthy young volunteers (n =30,mean age =25,15male)completed a computer-based delay discounting proce-dure to estimate their individual discount rate (Peters and Bu ¨-chel,2009).This discount rate was used solely for the purpose of constructing subject-specific trials for the fMRI session (see Experimental Procedures ).Furthermore,participants compiled a list of events that they had planned in the next 7months (e.g.,vacations,weddings,parties,courses,and so forth)andrated them on scales from 1to 6with respect to personal rele-vance,arousal,and valence.For each participant,seven subject-specific events were selected such that the spacing between events increased with increasing delay to the episode,and that events were roughly matched based on personal rele-vance,arousal,and valence.Multiple regression analysis of these ratings across the different delays showed no linear effects (relevance:p =0.867,arousal:p =0.120,valence:p =0.977,see Figure S1available online).For each subject,a separate set of seven delays was computed that was later used as delays in the control condition.Median and range for the delays used in each condition are listed in Table S1(available online).For each event,a label was selected that would serve as a verbal tag for the fMRI session.Experiment 1:fMRI Behavioral ResultsOn day 2,volunteers performed two sessions of a delay dis-counting procedure while fMRI was measured using a 3T Siemens Scanner with a 32-channel head-coil.In each session,subjects made a total of 118choices between 20V available immediately and larger but delayed amounts.Subjects were told that one of their choices would be randomly selected and paid out following scanning,with the respective delay.Critically,in half the trials,an additional subject-specific episodic tag (see above,e.g.,‘‘vacation paris’’or ‘‘birthday john’’)was displayed based on the prescan interview (see Figure 1)indicating which event they had planned on the particular day (episodic condi-tion),whereas in the remaining trials,no episodic tag was pre-sented (control condition).Amount and waiting time were thus displayed in both conditions,but only the episodic condition involved the presentation of an additional subject-specific event tag.Importantly,nonoverlapping sets of delays were used in the two conditions.Following scanning,subjects rated for each episodic tag how often it evoked episodic associations during scanning (frequency of associations:1,never;to 6,always)and how vivid these associations were (vividness of associa-tions:1,not vivid at all;to 6,highly vivid;see Figure S1).Addition-ally,written reports were obtained (see Supplemental Informa-tion ).Multiple regression revealed no significant linear effects of delay on postscan ratings (frequency:p =0.224,vividness:p =0.770).We averaged the postscan ratings acrosseventsFigure 1.Behavioral TaskDuring fMRI,subjects made repeated choices between a fixed immediate reward of 20V and larger but delayed amounts.In the control condi-tion,amounts were paired with a waiting time only,whereas in the episodic condition,amounts were paired with a waiting time and a subject-specific verbal episodic tag indicating to the subjects which event they had planned at the respective day of reward delivery.Events were real and collected in a separate testing session prior to the day of scanning.NeuronEpisodic Modulation of Delay DiscountingNeuron 66,138–148,April 15,2010ª2010Elsevier Inc.139and the frequency/vividness dimensions,yielding an‘‘imagery score’’for each subject.Individual participants’choice data from the fMRI session were then analyzed byfitting hyperbolic discount functions to subject-specific indifference points to obtain discount rates (k-parameters),separately for the episodic and control condi-tions(see Experimental Procedures).Subjective preferences were well-characterized by hyperbolic functions(median R2 episodic condition=0.81,control condition=0.85).Discount functions of four exemplary subjects are shown in Figure2A. For both conditions,considerable variability in the discount rate was observed(median[range]of discount rates:control condition=0.014[0.003–0.19],episodic condition=0.013 [0.002–0.18]).To account for the skewed distribution of discount rates,all further analyses were conducted on the log-trans-formed k-parameters.Across subjects,log-transformed discount rates were significantly lower in the episodic condition compared with the control condition(t(29)=2.27,p=0.016),indi-cating that participants’choice behavior was less impulsive in the episodic condition.The difference in log-discount rates between conditions is henceforth referred to as the episodic tag effect.Fitting hyperbolic functions to the median indifference points across subjects also showed reduced discounting in the episodic condition(discount rate control condition=0.0099, episodic condition=0.0077).The size of the tag effect was not related to the discount rate in the control condition(p=0.56). We next hypothesized that the tag effect would be positively correlated with postscan ratings of episodic thought(imagery scores,see above).Robust regression revealed an increase in the size of the tag effect with increasing imagery scores (t=2.08,p=0.023,see Figure2B),suggesting that the effect of the tags on preferences was stronger the more vividly subjects imagined the episodes.Examples of written postscan reports are provided in the Supplemental Results for participants from the entire range of imagination ratings.We also correlated the tag effect with standard neuropsychological measures,the Sensation Seeking Scale(SSS)V(Beauducel et al.,2003;Zuck-erman,1996)and the Behavioral Inhibition Scale/Behavioral Approach Scale(BIS/BAS)(Carver and White,1994).The tag effect was positively correlated with the experience-seeking subscale of the SSS(p=0.026)and inversely correlated with the reward-responsiveness subscale of the BIS/BAS scales (p<0.005).Repeated-measures ANOVA of reaction times(RTs)as a func-tion of option value(lower,similar,or higher relative to the refer-ence option;see Experimental Procedures and Figure2C)did not show a main effect of condition(p=0.712)or a condition 3value interaction(p=0.220),but revealed a main effect of value(F(1.8,53.9)=16.740,p<0.001).Post hoc comparisons revealed faster RTs for higher-valued options relative to similarly (p=0.002)or lower valued options(p<0.001)but no difference between lower and similarly valued options(p=0.081).FMRI DataFMRI data were modeled using the general linear model(GLM) as implemented in SPM5.Subjective value of each decision option was calculated by multiplying the objective amount of each delayed reward with the discount fraction estimated behaviorally based on the choices during scanning,and included as a parametric regressor in the GLM.Note that discount rates were estimated separately for the control and episodic conditions(see above and Figure2),and we thus used condition-specific k-parameters for calculation of the subjective value regressor.Additional parametric regressors for inverse delay-to-reward and absolute reward magnitude, orthogonalized with respect to subjective value,were included in theGLM.Figure2.Behavioral Data from Experiment1Shown are experimentally derived discount func-tions from the fMRI session for four exemplaryparticipants(A),correlation with imagery scores(B),and reaction times(RTs)(C).(A)Hyperbolicfunctions werefit to the indifference points sepa-rately for the control(dashed lines)and episodic(solid lines,filled circles)conditions,and thebest-fitting k-parameters(discount rates)and R2values are shown for each subject.The log-trans-formed difference between discount rates wastaken as a measure of the effect of the episodictags on choice preferences.(B)Robust regressionrevealed an association between log-differences indiscount rates and imagery scores obtained frompostscan ratings(see text).(C)RTs were signifi-cantly modulated by option value(main effectvalue p<0.001)with faster responses in trialswith a value of the delayed reward higher thanthe20V reference amount.Note that althoughseven delays were used for each condition,somedata points are missing,e.g.,onlyfive delay indif-ference points for the episodic condition areplotted for sub20.This indicates that,for the twolongest delays,this subject never chose the de-layed reward.***p<0.005.Error bars=SEM.Neuron Episodic Modulation of Delay Discounting140Neuron66,138–148,April15,2010ª2010Elsevier Inc.Episodic Tags Activate the Future Thinking NetworkWe first analyzed differences in the condition regressors without parametric pared to those of the control condi-tion,BOLD responses to the presentation of the delayed reward in the episodic condition yielded highly significant activations (corrected for whole-brain volume)in an extensive network of brain regions previously implicated in episodic future thinking (Addis et al.,2007;Schacter et al.,2007;Szpunar et al.,2007)(see Figure 3and Table S2),including retrosplenial cortex (RSC)/PCC (peak MNI coordinates:À6,À54,14,peak z value =6.26),left lateral parietal cortex (LPC,À44,À66,32,z value =5.35),and vmPFC (À8,34,À12,z value =5.50).Distributed Neural Coding of Subjective ValueWe then replicated previous findings (Kable and Glimcher,2007;Kable and Glimcher,2010;Peters and Bu¨chel,2009)using a conjunction analysis (Nichols et al.,2005)searching for regions showing a positive correlation between the height of the BOLD response and subjective value in the control and episodic condi-tions in a parametric analysis (Figure 4A and Table S3).Note that this is a conservative analysis that requires that a given voxel exceed the statistical threshold in both contrasts separately.This analysis revealed clusters in the lateral orbitofrontal cortex (OFC,À36,50,À10,z value =4.50)and central OFC (À18,12,À14,z value =4.05),bilateral VS (right:10,8,0,z value =4.22;left:À10,8,À6,z value =3.51),mPFC (6,26,16,z value =3.72),and PCC (À2,À28,24,z value =4.09),representing subjective (discounted)value in both conditions.We next analyzed the neural tag effect,i.e.,regions in which the subjective value correlation was greater for the episodic condi-tion as compared with the control condition (Figure 4B and Table S4).This analysis revealed clusters in the left LPC (À66,À42,32,z value =4.96,),ACC (À2,16,36,z value =4.76),left dorsolateral prefrontal cortex (DLPFC,À38,36,36,z value =4.81),and right amygdala (24,2,À24,z value =3.75).Finally,we performed a triple-conjunction analysis,testing for regions that were correlated with subjective value in both conditions,but in which the value correlation increased in the episodic condition.Only left LPC showed this pattern (À66,À42,30,z value =3.55,see Figure 4C and Table S5),the same region that we previously identified as delay-specific in valuation (Petersand Bu¨chel,2009).There were no regions in which the subjective value correlation was greater in the control condition when compared with the episodic condition at p <0.001uncorrected.ACC Valuation Signals and Functional Connectivity Predict Interindividual Differences in Discount Function ShiftsWe next correlated differences in the neural tag effect with inter-individual differences in the size of the behavioral tag effect.To this end,we performed a simple regression analysis in SPM5on the single-subject contrast images of the neural tag effect (i.e.,subjective value correlation episodic >control)using the behavioral tag effect [log(k control )–log(k episodic )]as an explana-tory variable.This analysis revealed clusters in the bilateral ACC (right:18,34,18,z value =3.95,p =0.021corrected,left:À20,34,20,z value =3.52,Figure 5,see Table S6for a complete list).Coronal sections (Figure 5C)clearly show that both ACC clusters are located in gray matter of the cingulate sulcus.Because ACC-limbic interactions have previously been impli-cated in the control of choice behavior (Floresco and Ghods-Sharifi,2007;Roiser et al.,2009),we next analyzed functional coupling with the right ACC from the above regression contrast (coordinates 18,34,18,see Figure 6A)using a psychophysiolog-ical interaction analysis (PPI)(Friston et al.,1997).Note that this analysis was conducted on a separate first-level GLM in which control and episodic trials were modeled as 10s miniblocks (see Experimental Procedures for details).We first identified regions in which coupling with the ACC changed in the episodic condition compared with the control condition (see Table S7)and then performed a simple regression analysis on these coupling parameters using the behavioral tag effect as an explanatory variable.The tag effect was associated with increased coupling between ACC and hippocampus (À32,À18,À16,z value =3.18,p =0.031corrected,Figure 6B)and ACC and left amygdala (À26,À4,À26,z value =2.95,p =0.051corrected,Figure 6B,see Table S8for a complete list of activa-tions).The same regression analysis in a second PPI with the seed voxel placed in the contralateral ACC region from the same regression contrast (À20,34,22,see above)yielded qual-itatively similar,though subthreshold,results in these same structures (hippocampus:À28,À32,À6,z value =1.96,amyg-dala:À28,À6,À16,z value =1.97).Experiment 2We conducted an additional behavioral experiment to address a number of alternative explanations for the observed effects of tags on choice behavior.First,it could be argued thatepisodicFigure 3.Categorical Effect of Episodic Tags on Brain ActivityGreater activity in lateral parietal cortex (left)and posterior cingulate/retrosplenial and ventro-medial prefrontal cortex (right)was observed in the episodic condition compared with the control condition.p <0.05,FWE-corrected for whole-brain volume.NeuronEpisodic Modulation of Delay DiscountingNeuron 66,138–148,April 15,2010ª2010Elsevier Inc.141tags increase subjective certainty that a reward would be forth-coming.In Experiment 2,we therefore collected postscan ratings of reward confidence.Second,it could be argued that events,always being associated with a particular date,may have shifted temporal focus from delay-based to more date-based processing.This would represent a potential confound,because date-associated rewards are discounted less than delay-associated rewards (Read et al.,2005).We therefore now collected postscan ratings of temporal focus (date-based versus delay-based).Finally,Experiment 1left open the question of whether the tag effect depends on the temporal specificity of the episodic cues.We therefore introduced an additional exper-imental condition that involved the presentation of subject-specific temporally unspecific future event cues.These tags (henceforth referred to as unspecific tags)were obtained by asking subjects to imagine events that could realistically happen to them in the next couple of months,but that were not directly tied to a particular point in time (see Experimental Procedures ).Episodic Imagery,Not Temporal Specificity,Reward Confidence,or Temporal Focus,Predicts the Size of the Tag EffectIn total,data from 16participants (9female)are included.Anal-ysis of pretest ratings confirmed that temporally unspecific and specific tags were matched in terms of personal relevance,arousal,valence,and preexisting associations (all p >0.15).Choice preferences were again well described by hyperbolic functions (median R 2control =0.84,unspecific =0.81,specific =0.80).We replicated the parametric tag effect (i.e.,increasing effect of tags on discount rates with increasing posttest imagery scores)in this independent sample for both temporally specific (p =0.047,Figure 7A)and temporally unspecific (p =0.022,Figure 7A)tags,showing that the effect depends on future thinking,rather than being specifically tied to the temporal spec-ificity of the event cues.Following testing,subjects rated how certain they were that a particular reward would actually be forth-coming.Overall,confidence in the payment procedure washighFigure 4.Neural Representation of Subjective Value (Parametric Analysis)(A)Regions in which the correlation with subjective value (parametric analysis)was significant in both the control and the episodic conditions (conjunction analysis)included central and lateral orbitofrontal cortex (OFC),bilateral ventral striatum (VS),medial prefrontal cortex (mPFC),and posterior cingulate cortex(PCC),replicating previous studies (Kable and Glimcher,2007;Peters and Bu¨chel,2009).(B)Regions in which the subjective value correlation was greater for the episodic compared with the control condition included lateral parietal cortex (LPC),ante-rior cingulate cortex (ACC),dorsolateral prefrontal cortex (DLPFC),and the right amygdala (Amy).(C)A conjunction analysis revealed that only LPC activity was positively correlated with subjective value in both conditions,but showed a greater regression slope in the episodic condition.No regions showed a better correlation with subjective value in the control condition.Error bars =SEM.All peaks are significant at p <0.001,uncorrected;(A)and (B)are thresholded at p <0.001uncorrected and (C)is thresholded at p <0.005,uncorrected for display purposes.NeuronEpisodic Modulation of Delay Discounting142Neuron 66,138–148,April 15,2010ª2010Elsevier Inc.(Figure 7B),and neither unspecific nor specific tags altered these subjective certainty estimates (one-way ANOVA:F (2,45)=0.113,p =0.894).Subjects also rated their temporal focus as either delay-based or date-based (see Experimental Procedures ),i.e.,whether they based their decisions on the delay-to-reward that was actually displayed,or whether they attempted to convert delays into the corresponding dates and then made their choices based on these dates.There was no overall significant effect of condition on temporal focus (one-way ANOVA:F (2,45)=1.485,p =0.237,Figure 7C),but a direct comparison between the control and the temporally specific condition showed a significant difference (t (15)=3.18,p =0.006).We there-fore correlated the differences in temporal focus ratings between conditions (control:unspecific and control:specific)with the respective tag effects (Figure 7D).There were no correlations (unspecific:p =0.71,specific:p =0.94),suggesting that the observed differences in discounting cannot be attributed to differences in temporal focus.High-Imagery,but Not Low-Imagery,Subjects Adjust Their Discount Function in an Episodic ContextFor a final analysis,we pooled the samples of Experiments 1and 2(n =46subjects in total),using only the temporally specific tag data from Experiment 2.We performed a median split into low-and high-imagery participants according to posttest imagery scores (low-imagery subjects:n =23[15/8Exp1/Exp2],imagery range =1.5–3.4,high-imagery subjects:n =23[15/8Exp1/Exp2],imagery range =3.5–5).The tag effect was significantly greater than 0in the high-imagery group (t (22)=2.6,p =0.0085,see Figure 7D),where subjects reduced their discount rate by onaverage 16%in the presence of episodic tags.In the low-imagery group,on the other hand,the tag effect was not different from zero (t (22)=0.573,p =0.286),yielding a significant group difference (t (44)=2.40,p =0.011).DISCUSSIONWe investigated the interactions between episodic future thought and intertemporal decision-making using behavioral testing and fMRI.Experiment 1shows that reward delay dis-counting is modulated by episodic future event cues,and the extent of this modulation is predicted by the degree of sponta-neous episodic imagery during decision-making,an effect that we replicated in Experiment 2(episodic tag effect).The neuroi-maging data (Experiment 1)highlight two mechanisms that support this effect:(1)valuation signals in the lateral ACC and (2)neural coupling between ACC and hippocampus/amygdala,both predicting the size of the tag effect.The size of the tag effect was directly related to posttest imagery scores,strongly suggesting that future thinking signifi-cantly contributed to this effect.Pooling subjects across both experiments revealed that high-imagery subjects reduced their discount rate by on average 16%in the episodic condition,whereas low-imagery subjects did not.Experiment 2addressed a number of alternative accounts for this effect.First,reward confidence was comparable for all conditions,arguing against the possibility that the tags may have somehow altered subjec-tive certainty that a reward would be forthcoming.Second,differences in temporal focus between conditions(date-basedFigure 5.Correlation between the Neural and Behavioral Tag Effect(A)Glass brain and (B and C)anatomical projection of the correlation between the neural tag effect (subjective value correlation episodic >control)and the behav-ioral tag effect (log difference between discount rates)in the bilateral ACC (p =0.021,FWE-corrected across an anatomical mask of bilateral ACC).(C)Coronal sections of the same contrast at a liberal threshold of p <0.01show that both left and right ACC clusters encompass gray matter of the cingulate gyrus.(D)Scatter-plot depicting the linear relationship between the neural and the behavioral tag effect in the right ACC.(A)and (B)are thresholded at p <0.001with 10contiguous voxels,whereas (C)is thresholded at p <0.01with 10contiguousvoxels.Figure 6.Results of the Psychophysiolog-ical Interaction Analysis(A)The seed for the psychophysiological interac-tion (PPI)analysis was placed in the right ACC (18,34,18).(B)The tag effect was associated with increased ACC-hippocampal coupling (p =0.031,corrected across bilateral hippocampus)and ACC-amyg-dala coupling (p =0.051,corrected across bilateral amygdala).Maps are thresholded at p <0.005,uncorrected for display purposes and projected onto the mean structural scan of all participants;HC,hippocampus;Amy,Amygdala;rACC,right anterior cingulate cortex.NeuronEpisodic Modulation of Delay DiscountingNeuron 66,138–148,April 15,2010ª2010Elsevier Inc.143。
殷保群教授个人简历范文
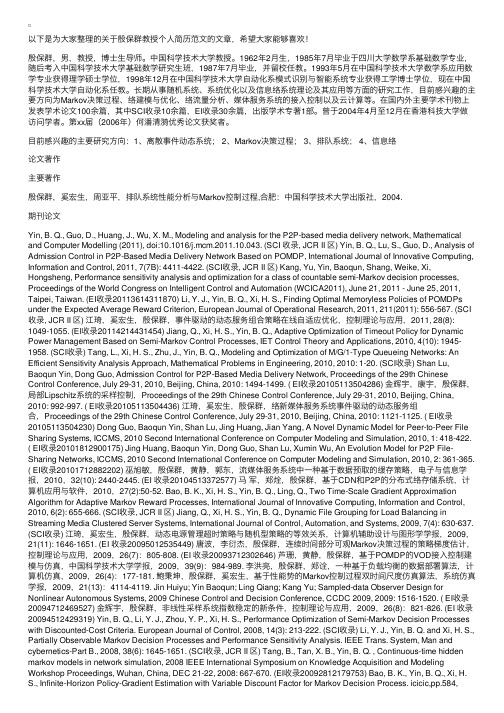
以下是为⼤家整理的关于殷保群教授个⼈简历范⽂的⽂章,希望⼤家能够喜欢!殷保群,男,教授,博⼠⽣导师。
中国科学技术⼤学教授。
1962年2⽉⽣,1985年7⽉毕业于四川⼤学数学系基础数学专业,随后考⼊中国科学技术⼤学基础数学研究⽣班,1987年7⽉毕业,并留校任教。
1993年5⽉在中国科学技术⼤学数学系应⽤数学专业获得理学硕⼠学位,1998年12⽉在中国科学技术⼤学⾃动化系模式识别与智能系统专业获得⼯学博⼠学位,现在中国科学技术⼤学⾃动化系任教。
长期从事随机系统、系统优化以及信息络系统理论及其应⽤等⽅⾯的研究⼯作,⽬前感兴趣的主要⽅向为Markov决策过程、络建模与优化、络流量分析、媒体服务系统的接⼊控制以及云计算等。
在国内外主要学术刊物上发表学术论⽂100余篇,其中SCI收录10余篇,EI收录30余篇,出版学术专著1部。
曾于2004年4⽉⾄12⽉在⾹港科技⼤学做访问学者。
第xx届(2006年)何潘清漪优秀论⽂获奖者。
⽬前感兴趣的主要研究⽅向:1、离散事件动态系统; 2、Markov决策过程; 3、排队系统; 4、信息络论⽂著作主要著作殷保群,奚宏⽣,周亚平,排队系统性能分析与Markov控制过程,合肥:中国科学技术⼤学出版社,2004.期刊论⽂Yin, B. Q., Guo, D., Huang, J., Wu, X. M., Modeling and analysis for the P2P-based media delivery network, Mathematical and Computer Modelling (2011), doi:10.1016/j.mcm.2011.10.043. (SCI 收录, JCR II 区) Yin, B. Q., Lu, S., Guo, D., Analysis of Admission Control in P2P-Based Media Delivery Network Based on POMDP, International Journal of Innovative Computing, Information and Control, 2011, 7(7B): 4411-4422. (SCI收录, JCR II 区) Kang, Yu, Yin, Baoqun, Shang, Weike, Xi, Hongsheng, Performance sensitivity analysis and optimization for a class of countable semi-Markov decision processes, Proceedings of the World Congress on Intelligent Control and Automation (WCICA2011), June 21, 2011 - June 25, 2011, Taipei, Taiwan. (EI收录20113614311870) Li, Y. J., Yin, B. Q., Xi, H. S., Finding Optimal Memoryless Policies of POMDPs under the Expected Average Reward Criterion, European Journal of Operational Research, 2011, 211(2011): 556-567. (SCI 收录, JCR II 区) 江琦,奚宏⽣,殷保群,事件驱动的动态服务组合策略在线⾃适应优化,控制理论与应⽤,2011, 28(8): 1049-1055. (EI收录20114214431454) Jiang, Q., Xi, H. S., Yin, B. Q., Adaptive Optimization of Timeout Policy for Dynamic Power Management Based on Semi-Markov Control Processes, IET Control Theory and Applications, 2010, 4(10): 1945-1958. (SCI收录) Tang, L., Xi, H. S., Zhu, J., Yin, B. Q., Modeling and Optimization of M/G/1-Type Queueing Networks: An Efficient Sensitivity Analysis Approach, Mathematical Problems in Engineering, 2010, 2010: 1-20. (SCI收录) Shan Lu, Baoqun Yin, Dong Guo, Admission Control for P2P-Based Media Delivery Network, Proceedings of the 29th Chinese Control Conference, July 29-31, 2010, Beijing, China, 2010: 1494-1499. ( EI收录20105113504286) ⾦辉宇,康宇,殷保群,局部Lipschitz系统的采样控制,Proceedings of the 29th Chinese Control Conference, July 29-31, 2010, Beijing, China, 2010: 992-997. ( EI收录20105113504436) 江琦,奚宏⽣,殷保群,络新媒体服务系统事件驱动的动态服务组合,Proceedings of the 29th Chinese Control Conference, July 29-31, 2010, Beijing, China, 2010: 1121-1125. ( EI收录20105113504230) Dong Guo, Baoqun Yin, Shan Lu, Jing Huang, Jian Yang, A Novel Dynamic Model for Peer-to-Peer File Sharing Systems, ICCMS, 2010 Second International Conference on Computer Modeling and Simulation, 2010, 1: 418-422. ( EI收录20101812900175) Jing Huang, Baoqun Yin, Dong Guo, Shan Lu, Xumin Wu, An Evolution Model for P2P File-Sharing Networks, ICCMS, 2010 Second International Conference on Computer Modeling and Simulation, 2010, 2: 361-365. ( EI收录20101712882202) 巫旭敏,殷保群,黄静,郭东,流媒体服务系统中⼀种基于数据预取的缓存策略,电⼦与信息学报,2010,32(10): 2440-2445. (EI 收录20104513372577) 马军,郑烇,殷保群,基于CDN和P2P的分布式络存储系统,计算机应⽤与软件,2010,27(2):50-52. Bao, B. K., Xi, H. S., Yin, B. Q., Ling, Q., Two Time-Scale Gradient Approximation Algorithm for Adaptive Markov Reward Processes, International Journal of Innovative Computing, Information and Control, 2010, 6(2): 655-666. (SCI收录, JCR II 区) Jiang, Q., Xi, H. S., Yin, B. Q., Dynamic File Grouping for Load Balancing in Streaming Media Clustered Server Systems, International Journal of Control, Automation, and Systems, 2009, 7(4): 630-637. (SCI收录) 江琦,奚宏⽣,殷保群,动态电源管理超时策略与随机型策略的等效关系,计算机辅助设计与图形学学报,2009, 21(11): 1646-1651. (EI 收录20095012535449) 唐波,李衍杰,殷保群,连续时间部分可观Markov决策过程的策略梯度估计,控制理论与应⽤,2009,26(7):805-808. (EI 收录20093712302646) 芦珊,黄静,殷保群,基于POMDP的VOD接⼊控制建模与仿真,中国科学技术⼤学学报,2009,39(9):984-989. 李洪亮,殷保群,郑诠,⼀种基于负载均衡的数据部署算法,计算机仿真,2009,26(4):177-181. 鲍秉坤,殷保群,奚宏⽣,基于性能势的Markov控制过程双时间尺度仿真算法,系统仿真学报,2009,21(13):4114-4119. Jin Huiyu; Yin Baoqun; Ling Qiang; Kang Yu; Sampled-data Observer Design for Nonlinear Autonomous Systems, 2009 Chinese Control and Decision Conference, CCDC 2009, 2009: 1516-1520. ( EI收录20094712469527) ⾦辉宇,殷保群,⾮线性采样系统指数稳定的新条件,控制理论与应⽤,2009,26(8):821-826. (EI 收录20094512429319) Yin, B. Q., Li, Y. J., Zhou, Y. P., Xi, H. S., Performance Optimization of Semi-Markov Decision Processes with Discounted-Cost Criteria. European Journal of Control, 2008, 14(3): 213-222. (SCI收录) Li, Y. J., Yin, B. Q. and Xi, H. S., Partially Observable Markov Decision Processes and Performance Sensitivity Analysis. IEEE Trans. System, Man and cybernetics-Part B., 2008, 38(6): 1645-1651. (SCI收录, JCR II 区) Tang, B., Tan, X. B., Yin, B. Q. , Continuous-time hidden markov models in network simulation, 2008 IEEE International Symposium on Knowledge Acquisition and Modeling Workshop Proceedings, Wuhan, China, DEC 21-22, 2008: 667-670. (EI收录20092812179753) Bao, B. K., Yin, B. Q., Xi, H. S., Infinite-Horizon Policy-Gradient Estimation with Variable Discount Factor for Markov Decision Process. icicic,pp.584,2008 3rd International Conference on Innovative Computing Information and Control, 2008. ( EI收录************) Chenfeng Xu, Jian Yang, Hongsheng Xi, Qi Jiang, Baoqun Yin, Event-related optimization for a class of resource location with admission control, 2008. IJCNN 2008. (IEEE World Congress on Computational Intelligence). IEEE International Joint Conference on Neural Networks, 1-8 June 2008: 1092 – 1097. ( EI收录************)JinHuiyu;KangYu;YinBaoqun; Synchronization of nonlinear systems with stair-step signal, 2008. CCC 2008. 27th Chinese Control Conference,16-18 July 2008: 459 – 463. ( EI收录************)JiangQi;XiHongsheng;YinBaoqun;XuChenfeng;Anevent-drivendynamicload balancing strategy for streaming media clustered server systems, 2008. CCC 2008. 27th Chinese Control Conference, 16-18 July 2008: 678 – 682. ( EI收录************)⾦辉宇,殷保群,唐波,⾮线性采样观测器的误差分析,中国科学技术⼤学学报,2008, 38(10): 1226-1231. 黄静,殷保群,李俊,基于观测的POMDP优化算法及其仿真,信息与控制,2008, 37(3): 346-351. 马军,殷保群,基于POMDP模型的机器⼈⾏动的仿真优化,系统仿真学报,2008, 20(21): 5903-5906. (EI 收录************)江琦,奚宏⽣,殷保群,动态电源管理超时策略⾃适应优化算法,控制与决策,2008, 23(4): 372-377. (EI 收录************)徐陈锋,奚宏⽣,江琦,殷保群,⼀类分层⾮结构化P2P系统的随机切换模型,控制与决策,2008, 23(3): 263-266. (EI 收录************)徐陈锋,奚宏⽣,殷保群,⼀类混合资源定位服务的优化模型,微计算机应⽤,2008,29(9):6-11. 郭东,郑烇,殷保群,王嵩,基于P2P媒体内容分发络中分布式节点的设计与实现,电信科学,2008,24(8): 45-49. Tang, H., Yin, B. Q., Xi, H. S., Error bounds of optimization algorithms for semi-Markov decision processes. International Journal of Systems Science, 2007, 38(9): 725-736. (SCI收录) 徐陈锋,奚宏⽣,江琦,殷保群,⼀类分层⾮结构化P2P系统的随机优化,系统科学与数学,2007, 27(3): 412-421. 蒋兆春,殷保群,李俊,基于耦合技术计算Markov链性能势的仿真算法,系统仿真学报,2007, 19(15): 3398-3401. (EI收录************)庞训磊,殷保群,奚宏⽣,⼀种使⽤TCP/ IP 协议实现⽆线传感器络互连的新型设计,传感技术学报,2007, 20(6): 1386-1390. Niu, L. M., Tan, X. B., Yin, B. Q. , Estimation of system power consumption on mobile computing devices, 2007. International Conference on Computational Intelligence and Security, Harbin, China, DEC 15-19, 2007: 1058-1061. (EI收录************)Jiang,Q.,Xi, H. S., Yin, B. Q., Dynamic file grouping for load balancing in streaming media clustered server systems. Proceedings of the 2007 International Conference on Information Acquisition, ICIA, Jeju City, South Korea, 2007:498-503. (EI收录************)徐陈锋, 奚宏⽣, 江琦, 殷保群,⼀类分层⾮结构化P2P系统的随机优化,第2xx届中国控制会议论⽂集,2007: 693-696. (EI收录************)Jiang,Q.,Xi,H.S.,Yin,B.Q.,OptimizationofSemi-MarkovSwitchingState-spaceControl Processes for Network Communication Systems. 第2xx届中国控制会议论⽂集,2007: 707-711. (EI收录************) Jiang, Q., Xi, H. S., Yin, B. Q., Adaptive Optimization of Time-out Policy for Dynamic Power Management Based on SMCP. Proc. of the 2007 IEEE Multi-conference on Systems and Control, Singapore, 2007: 319-324. (EI收录************)Jin,H. Y., Yin, B. Q., New Consistency Condition for Exponential Stabilization of Smapled-Data Nonlinear Systems. 第2xx届中国控制会议论⽂集,2007: 84-87. (EI收录************)江琦,奚宏⽣,殷保群,⽆线多媒体通信适应带宽配置在线优化算法,软件学报, 2007, 18(6): 1491-1500. (EI收录************)Ou,Q.,Jin,Y.D.,Zhou,T.,Wang,B.H.,Yin,B.Q.,Power-law strength-degree correlation from resource-allocation dynamics on weighted networks, Physical Review E, 2007, 75(2): 021102 (SCI收录) Yin, B. Q., Dai, G. P., Li, Y. J., Xi, H. S., Sensitivity analysis and estimates of the performance for M/G/1 queueing systems, Performance Evaluation, 2007, 64(4): 347-356. (SCI收录) 江琦,奚宏⽣,殷保群,动态电源管理的随机切换模型与在线优化,⾃动化学报,2007, 33(1): 66-71. (EI收录************)Zhang,D.L.,Yin,B.Q.,Xi,H.S.,Astate aggregation approach to singularly perturbed Markov reward processes. International Journal of Intelligent Technology, 2006, 2(4): 230-239. 欧晴,殷保群,奚宏⽣,基于动态平衡流的络赋权,中国科学技术⼤学学报,2006, 36(11): 1196-1201.殷保群,李衍杰,周亚平,奚宏⽣,可数半Markov控制过程折扣代价性能优化,控制与决策,2006, 21(8): 933-936. (EI收录************)江琦,奚宏⽣,殷保群,动态电源管理的随机切换模型与策略优化,计算机辅助设计与图形学学报,2006, 18(5): 680-686. (EI收录***********)代桂平,殷保群,李衍杰,奚宏⽣,半Markov控制过程基于性能势仿真的并⾏优化算法,中国科学技术⼤学学报,2006, 36(2): 183-186. 殷保群,李衍杰,唐昊,代桂平,奚宏⽣,半Markov决策过程折扣模型与平均模型之间的关系,控制理论与应⽤,2006, 23(1): 65-68. (EI收录***********)江琦,奚宏⽣,殷保群,半Markov控制过程在线⾃适应优化算法,第2xx届中国控制会议论⽂集,2006: 1066-1071. (ISTP收录BFQ63) Dai, G. P., Yin, B. Q., Li, Y. J., Xi, H. S., Performance Optimization Algorithms based on potential for Semi-Markov Control Processes. International Journal of Control, 2005, 78(11): 801-812. (SCI收录) Zhang, D. L., Xi, H. S., Yin, B. Q., Simulation-based optimization of singularly perturbed Markov reward processes with states aggregation. Lecture Notes in Computer Science, 2005, 3645: 129-138. (SCI 收录) Tang, H., Xi, H. S., Yin, B. Q., The optimal robust control policy for uncertain semi-Markov control processes. International Journal of System Science, 2005, 36(13): 791-800. (SCI收录) 张虎,殷保群,代桂平,奚宏⽣,G/M/1排队系统的性能灵敏度分析与仿真,系统仿真学报,2005, 17(5): 1084-1086. (EI收录***********)陈波,周亚平,殷保群,奚宏⽣,隐马⽒模型中的标量估计,系统⼯程与电⼦技术,2005, 27(6): 1083-1086. (EI收录***********)代桂平,殷保群,李衍杰,周亚平,奚宏⽣,半Markov控制过程在平均准则下的优化算法,中国科学技术⼤学学报,2005, 35(2): 202-207. 殷保群,李衍杰,奚宏⽣,周亚平,⼀类可数Markov控制过程的平稳策略,控制理论与应⽤,2005, 22(1): 43-46. (EI收录***********)Li,Y.J.,Yin,B.Q.,Xi,H.S.,Thepolicygradientestimationofcontinuous-timeHiddenMarkovDecision Processes. Proc. of IEEE ICIA, Hong Kong, 2005. (EI收录************)Sensitivity analysis and estimates of the performance for M/G/1 queueing systems, To Appear in Performance Evaluation, 2006.Performance optimization algorithms based on potential for semi-Markov control processes. International Journal of Control, Vol.78, No.11, 2005.The optimal robust control policy for uncertain semi-Markov control processes. International Journal of System Science, Vol.36, No.13, 2005.A state aggregation approach to singularly perturbed Markov reward processes. International Journal of Intelligent Technology, Vol.2, No.4, 2005.Simulation optimization algorithms for CTMDP based on randomized stationary policies, Acta Automatics Sinica, Vol. 30, No. 2, 2004.Performance optimization of continuous-time Markov control processes based on performance potentials, International Journal of System Science, Vol.34, No.1, 2003.Optimal Policies for a Continuous Time MCP with Compact Action set, Acta Automatics Sinica, Vol. 29, No. 2, 2003. Relations between Performance Potential and Infinitesimal Realization Factor in Closed Queueing Networks, Appl. Math. J. Chinese Univ. Ser. B, Vol. 17, No. 4, 2002.Sensitivity Analysis of Performance in Queueing Systems with Phase-Type Service Distribution, OR Transactions, Vol.4, No.4, 2000.Sensitivity Formulas of Performance in Two-Server Cyclic Queueing Networks with Phase-Type Distributed Service Times, International Transaction in Operational Research, Vol.6, No.6, 1999.Simulation-based optimization of singularly perturbed Markov reward processes with states aggregation. Lecture Notes in Computer Science, 2005.Markov decision problems with unbounded transition rates under discounted-cost performance criteria. Proceedings of WCICA, Vol.1, Hangzhou, China, 2004.排队系统性能分析与Markov控制过程,合肥:中国科学技术⼤学出版社,2004.可数半Markov控制过程折扣代价性能优化. 控制与决策,Vol.21, No.8, 2006.动态电源管理的随机切换模型与策略优化. 计算机辅助设计与图形学学报,Vol.18, No.5, 2006.半Markov决策过程折扣模型与平均模型之间的关系.控制理论与应⽤,Vol.23, No.1, 2006.⼀类可数Markov控制过程的平稳策略. 控制理论与应⽤,Vol.22, No.1, 2005.G/M/1排队系统的性能灵敏度分析与仿真.系统仿真学报,Vol.17, No.5, 2005.M/G/1排队系统的性能优化与算法,系统仿真学报,Vol.16, No.8, 2004.半Markov过程基于性能势的灵敏度分析和性能优化. 控制理论与应⽤,Vol.21, No.6, 2004.半Markov控制过程在折扣代价准则下的平稳策略. 控制与决策,Vol.19, No.6, 2004.Markov控制过程在紧致⾏动集上的迭代优化算法. 控制与决策,Vol.18, No.3, 2003.闭Jackson络的优化中减少仿真次数的算法研究,系统仿真学报,Vol.15, No.3, 2003.M/G/1排队系统的性能灵敏度估计与仿真,系统仿真学报,Vol.15, No.7, 2003.Markov控制过程基于性能势仿真的并⾏优化,系统仿真学报,Vol.15, No.11, 2003.Markov控制过程基于性能势的平均代价策略. ⾃动化学报,Vol.28, No.6, 2002.⼀类受控闭排队络基于性能势的性⽅程.控制理论与应⽤,Vol.19, No.4, 2002.Markov控制过程基于单个样本轨道的在线优化算法.控制理论与应⽤,Vol.19, No.6, 2002.闭排队络当性能函数与参数相关时的性能灵敏度分析,控制理论与应⽤,Vol.19, No.2, 2002.M/G/1 排队系统的性能灵敏度分析,⾼校应⽤数学学报,Vol.16, No.3, 2001.连续时间Markov决策过程在呼叫接⼊控制中的应⽤,控制与决策,Vol.19, 2001.具有不确定噪声的连续时间⼴义系统确保估计性能的鲁棒Kalman滤波器,控制理论与应⽤,Vol.18, No.5, 2001.状态相关闭排队络中的性能指标灵敏度公式,控制理论与应⽤,Vol.16, No.2, 1999.科研项⽬半Markov控制过程基于性能势的优化理论和并⾏算法,2003.1-2005.12,国家⾃然科学基⾦,60274012隐Markov过程的性能灵敏度分析与优化,2006.1-2008.12,国家⾃然科学基⾦, 60574065部分可观Markov系统的性能优化,2005.1-2006.12,安徽省⾃然科学基⾦, 050420301宽带信息运营⽀撑环境及接⼊系统的研制――⼦课题: 流媒体服务器研究及实现, 2005.1-2006.12, 国家863计划,2005AA103320离散复杂系统的控制与优化研究,2006.9-2008.8,中国科学院⾃动化研究所中国科学技术⼤学智能科学与技术联合实验室⾃主研究课题基⾦络新媒体服务系统的建模及其动⼒学⾏为分析研究,2012.01-2015.12,国家⾃然科学基⾦;⾯向服务任务的快速机器视觉与智能伺服控制,2010.01-2013.12,国家⾃然科学基⾦重点项⽬;新⼀代业务运⾏管控协同⽀撑环境的开发,2008.07-2011.06,国家863计划;多点协作的流媒体服务器集群系统及其性能优化,2006.12-2008.12,国家863计划;获奖情况第xx届何潘清漪优秀论⽂奖联系信息办公室地址:电⼆楼223 实验室地址:电⼆楼227 办公室电话:************。
[医药卫生]西氏公司丁基胶塞介绍材料
![[医药卫生]西氏公司丁基胶塞介绍材料](https://img.taocdn.com/s3/m/d99c3458777f5acfa1c7aa00b52acfc789eb9ff0.png)
Lab Testing ( particulates, microbiology)
Particle Counting Bioburden Endotoxins
Bioburden The level of microorganisms on the closure
Test for ´Ready to Sterilize´ Closures Based on EP Membrane Filtration Method Requires “extraction” from surface Appropriate rinse solutions and culture
Quantifies visible particulate into size ranges 25µ - 50µ; 51µ - 100µ; > 100µ
Index calculated with larger particles having higher weight Does NOT count silicone particles
In Process Control
Automated Vision Inspection* Final Inspection
Packaging
Sterilization* Shipping
* optional
Raw Materials and Auxiliaries
Mixing/Homogenising- Internal Mixer
Specific Gravity Of Test Sample - per Batch Shore A Of Vulcanized Sample - per Batch Dispersion Of Vulcanized Sample - per Batch Colour Of Vulcanized Sample - per Batch Ash - per 10 Batches Rheology Of The Compound - per 3 Batches
《MultimediaIEEETransactionson》期刊第47页50条数据
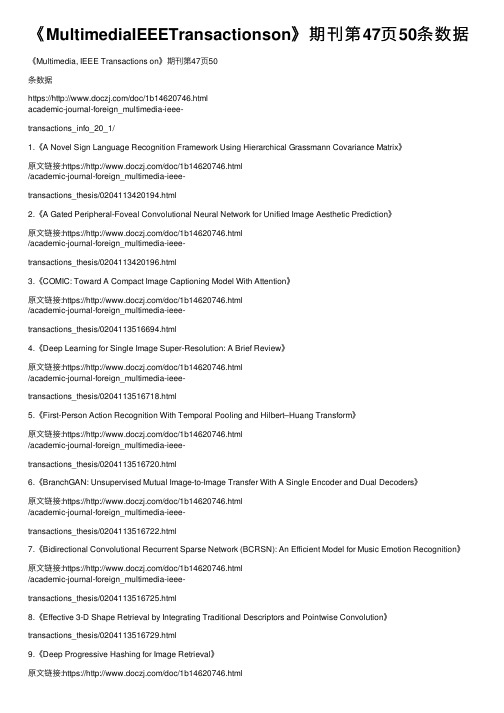
《MultimediaIEEETransactionson》期刊第47页50条数据《Multimedia, IEEE Transactions on》期刊第47页50条数据https:///doc/1b14620746.htmlacademic-journal-foreign_multimedia-ieee-transactions_info_20_1/1.《A Novel Sign Language Recognition Framework Using Hierarchical Grassmann Covariance Matrix》原⽂链接:https:///doc/1b14620746.html/academic-journal-foreign_multimedia-ieee-transactions_thesis/0204113420194.html2.《A Gated Peripheral-Foveal Convolutional Neural Network for Unified Image Aesthetic Prediction》原⽂链接:https:///doc/1b14620746.html/academic-journal-foreign_multimedia-ieee-transactions_thesis/0204113420196.html3.《COMIC: Toward A Compact Image Captioning Model With Attention》原⽂链接:https:///doc/1b14620746.html/academic-journal-foreign_multimedia-ieee-transactions_thesis/0204113516694.html4.《Deep Learning for Single Image Super-Resolution: A Brief Review》原⽂链接:https:///doc/1b14620746.html/academic-journal-foreign_multimedia-ieee-transactions_thesis/0204113516718.html5.《First-Person Action Recognition With Temporal Pooling and Hilbert–Huang Transform》原⽂链接:https:///doc/1b14620746.html/academic-journal-foreign_multimedia-ieee-transactions_thesis/0204113516720.html6.《BranchGAN: Unsupervised Mutual Image-to-Image Transfer With A Single Encoder and Dual Decoders》原⽂链接:https:///doc/1b14620746.html/academic-journal-foreign_multimedia-ieee-transactions_thesis/0204113516722.html7.《Bidirectional Convolutional Recurrent Sparse Network (BCRSN): An Efficient Model for Music Emotion Recognition》原⽂链接:https:///doc/1b14620746.html/academic-journal-foreign_multimedia-ieee-transactions_thesis/0204113516725.html8.《Effective 3-D Shape Retrieval by Integrating Traditional Descriptors and Pointwise Convolution》transactions_thesis/0204113516729.html9.《Deep Progressive Hashing for Image Retrieval》原⽂链接:https:///doc/1b14620746.html/academic-journal-foreign_multimedia-ieee-transactions_thesis/0204113516733.html10.《Can Categories and Attributes Be Learned in a Multi-Task Way?》原⽂链接:https:///doc/1b14620746.html/academic-journal-foreign_multimedia-ieee-transactions_thesis/0204113516737.html11.《Adaptive Convolution for Object Detection》原⽂链接:https:///doc/1b14620746.html/academic-journal-foreign_multimedia-ieee-transactions_thesis/0204113516740.html12.《A Novel Projective-Consistent Plane Based Image Stitching Method》原⽂链接:https:///doc/1b14620746.html/academic-journal-foreign_multimedia-ieee-transactions_thesis/0204113516743.html13.《Weakly Supervised Dual Learning for Facial Action Unit Recognition》原⽂链接:https:///doc/1b14620746.html/academic-journal-foreign_multimedia-ieee-transactions_thesis/0204113516744.html14.《Multi-Speaker Tracking From an Audio–Visual Sensing Device》原⽂链接:https:///doc/1b14620746.html/academic-journal-foreign_multimedia-ieee-transactions_thesis/0204113516747.html15.《AccAnn: A New Subjective Assessment Methodology for Measuring Acceptability and Annoyance of Quality of Experience》原⽂链接:https:///doc/1b14620746.html/academic-journal-foreign_multimedia-ieee-transactions_thesis/0204113516750.html16.《Naturalness-Aware Deep No-Reference Image Quality Assessment》原⽂链接:https:///doc/1b14620746.html/academic-journal-foreign_multimedia-ieee-transactions_thesis/0204113516753.html17.《Adaptive Cyclopean Image-Based Stereoscopic Image-Quality Assessment Using Ensemble Learning》原⽂链接:https:///doc/1b14620746.html/academic-journal-foreign_multimedia-ieee-transactions_thesis/0204113516756.html18.《Superpixel Segmentation Based on Square-Wise Asymmetric Partition and Structural Approximation》原⽂链接:https:///doc/1b14620746.html/academic-journal-foreign_multimedia-ieee-transactions_thesis/0204113516758.html19.《FIVR: Fine-Grained Incident Video Retrieval》transactions_thesis/0204113516759.html20.《Multi-Person Pose Estimation Using Bounding Box Constraint and LSTM》原⽂链接:https:///doc/1b14620746.html/academic-journal-foreign_multimedia-ieee-transactions_thesis/0204113516760.html21.《Quality-Aware Unpaired Image-to-Image Translation》原⽂链接:https:///doc/1b14620746.html/academic-journal-foreign_multimedia-ieee-transactions_thesis/0204113516761.html22.《Cross-Modality Bridging and Knowledge Transferring for Image Understanding》原⽂链接:https:///doc/1b14620746.html/academic-journal-foreign_multimedia-ieee-transactions_thesis/0204113516763.html23.《Deep Objective Quality Assessment Driven Single Image Super-Resolution》原⽂链接:https:///doc/1b14620746.html/academic-journal-foreign_multimedia-ieee-transactions_thesis/0204113516784.html24.《Real-Time Visual–Inertial SLAM Based on Adaptive Keyframe Selection for Mobile AR Applications》原⽂链接:https:///doc/1b14620746.html/academic-journal-foreign_multimedia-ieee-transactions_thesis/0204113516873.html25.《Adaptive Hypergraph Embedded Semi-Supervised Multi-Label Image Annotation》原⽂链接:https:///doc/1b14620746.html/academic-journal-foreign_multimedia-ieee-transactions_thesis/0204113516874.html26.《TPCKT: Two-Level Progressive Cross-Media Knowledge Transfer》原⽂链接:https:///doc/1b14620746.html/academic-journal-foreign_multimedia-ieee-transactions_thesis/0204113516875.html27.《Supervised Robust Discrete Multimodal Hashing for Cross-Media Retrieval》原⽂链接:https:///doc/1b14620746.html/academic-journal-foreign_multimedia-ieee-transactions_thesis/0204113516876.html28.《Effective Image Retrieval via Multilinear Multi-Index Fusion》原⽂链接:https:///doc/1b14620746.html/academic-journal-foreign_multimedia-ieee-transactions_thesis/0204113516877.html29.《Feature Affinity-Based Pseudo Labeling for Semi-Supervised Person Re-Identification》原⽂链接:https:///doc/1b14620746.html/academic-journal-foreign_multimedia-ieee-transactions_thesis/0204113516878.html30.《Distributed and Efficient Object Detection via Interactions Among Devices, Edge, and Cloud》原⽂链接:https:///doc/1b14620746.html/academic-journal-foreign_multimedia-ieee-transactions_thesis/0204113516879.html31.《SkeletonNet: A Hybrid Network With a Skeleton-Embedding Process for Multi-View Image Representation Learning》原⽂链接:https:///doc/1b14620746.html/academic-journal-foreign_multimedia-ieee-transactions_thesis/0204113516880.html32.《Decoupled Spatial Neural Attention for Weakly Supervised Semantic Segmentation》原⽂链接:https:///doc/1b14620746.html/academic-journal-foreign_multimedia-ieee-transactions_thesis/0204113516881.html33.《Deep Hierarchical Encoder–Decoder Network for Image Captioning》原⽂链接:https:///doc/1b14620746.html/academic-journal-foreign_multimedia-ieee-transactions_thesis/0204113516882.html34.《Message From the Outgoing Editor-in-Chief》原⽂链接:https:///doc/1b14620746.html/academic-journal-foreign_multimedia-ieee-transactions_thesis/0204113754116.html35.《Generative Model Driven Representation Learning in a Hybrid Framework for Environmental Audio Scene and Sound Event Recognition》原⽂链接:https:///doc/1b14620746.html/academic-journal-foreign_multimedia-ieee-transactions_thesis/0204113754118.html36.《Image Vectorization With Real-Time Thin-Plate Spline》原⽂链接:https:///doc/1b14620746.html/academic-journal-foreign_multimedia-ieee-transactions_thesis/0204113754120.html37.《Radiance–Reflectance Combined Optimization and Structure-Guided ?0-Norm for Single Image Dehazing》原⽂链接:https:///doc/1b14620746.html/academic-journal-foreign_multimedia-ieee-transactions_thesis/0204113754121.html38.《Generative Adversarial Network-Based Intra Prediction for Video Coding》原⽂链接:https:///doc/1b14620746.html/academic-journal-foreign_multimedia-ieee-transactions_thesis/0204113754122.html39.《Online Robust Principal Component Analysis With Change Point Detection》原⽂链接:https:///doc/1b14620746.html/academic-journal-foreign_multimedia-ieee-40.《QoE Analysis of Dense Multiview Video With Head-Mounted Devices》原⽂链接:https:///doc/1b14620746.html/academic-journal-foreign_multimedia-ieee-transactions_thesis/0204113754129.html41.《Efficient and Secure Image Communication System Based on Compressed Sensing for IoT Monitoring Applications》原⽂链接:https:///doc/1b14620746.html/academic-journal-foreign_multimedia-ieee-transactions_thesis/0204113754135.html42.《Deep Position-Sensitive Tracking》原⽂链接:https:///doc/1b14620746.html/academic-journal-foreign_multimedia-ieee-transactions_thesis/0204113754138.html43.《Using Blockchain for Improved Video Integrity Verification》原⽂链接:https:///doc/1b14620746.html/academic-journal-foreign_multimedia-ieee-transactions_thesis/0204113754140.html44.《Multi-Task Learning for Acoustic Event Detection Using Event and Frame Position Information》原⽂链接:https:///doc/1b14620746.html/academic-journal-foreign_multimedia-ieee-transactions_thesis/0204113754182.html45.《Steered Mixture-of-Experts for Light Field Images and Video: Representation and Coding》原⽂链接:https:///doc/1b14620746.html/academic-journal-foreign_multimedia-ieee-transactions_thesis/0204113754183.html46.《Design of Compressed Sensing System With Probability-Based Prior Information》原⽂链接:https:///doc/1b14620746.html/academic-journal-foreign_multimedia-ieee-transactions_thesis/0204113754184.html47.《Rate-Distortion Optimal Joint Texture and Depth Map Coding for 3-D Video Streaming》原⽂链接:https:///doc/1b14620746.html/academic-journal-foreign_multimedia-ieee-transactions_thesis/0204113754185.html48.《Spatiotemporal Recurrent Convolutional Networks for Recognizing Spontaneous Micro-Expressions》原⽂链接:https:///doc/1b14620746.html/academic-journal-foreign_multimedia-ieee-transactions_thesis/0204113754186.html49.《Image Retargetability》原⽂链接:https:///doc/1b14620746.html/academic-journal-foreign_multimedia-ieee-50.《Stereoscopic Image Stitching via Disparity-Constrained Warping and Blending》原⽂链接:https:///doc/1b14620746.html/academic-journal-foreign_multimedia-ieee-transactions_thesis/0204113754188.html。
IBM Cognos Transformer V11.0 用户指南说明书
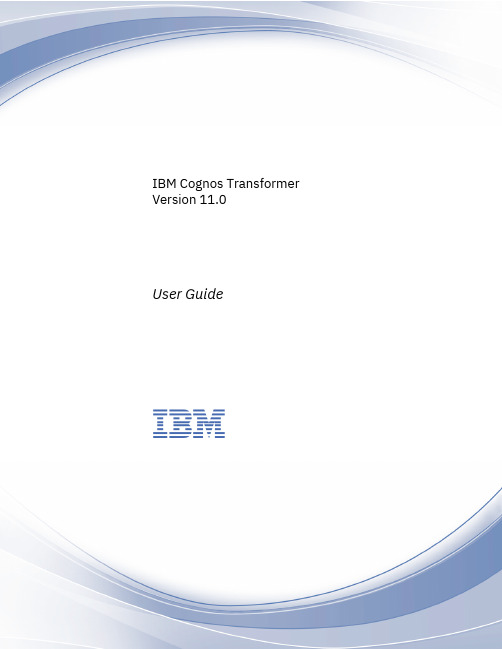
浙江拟提名度国家自然科学奖项目公示内容
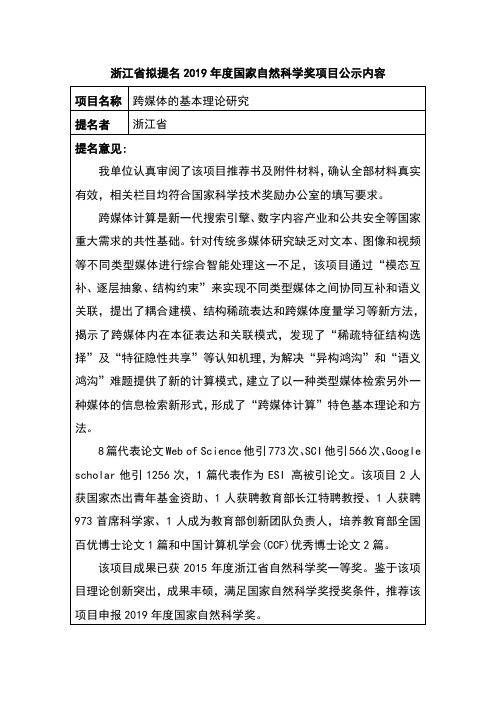
浙江省拟提名2019年度国家自然科学奖项目公示内容项目名称 跨媒体的基本理论研究提名者 浙江省提名意见:我单位认真审阅了该项目推荐书及附件材料,确认全部材料真实有效,相关栏目均符合国家科学技术奖励办公室的填写要求。
跨媒体计算是新一代搜索引擎、数字内容产业和公共安全等国家重大需求的共性基础。
针对传统多媒体研究缺乏对文本、图像和视频等不同类型媒体进行综合智能处理这一不足,该项目通过“模态互补、逐层抽象、结构约束”来实现不同类型媒体之间协同互补和语义关联,提出了耦合建模、结构稀疏表达和跨媒体度量学习等新方法,揭示了跨媒体内在本征表达和关联模式,发现了“稀疏特征结构选择”及“特征隐性共享”等认知机理,为解决“异构鸿沟”和“语义鸿沟”难题提供了新的计算模式,建立了以一种类型媒体检索另外一种媒体的信息检索新形式,形成了“跨媒体计算”特色基本理论和方法。
8篇代表论文Web of Science他引773次、SCI他引566次、Google scholar他引1256次,1篇代表作为ESI 高被引论文。
该项目2人获国家杰出青年基金资助、1人获聘教育部长江特聘教授、1人获聘973首席科学家、1人成为教育部创新团队负责人,培养教育部全国百优博士论文1篇和中国计算机学会(CCF)优秀博士论文2篇。
该项目成果已获2015年度浙江省自然科学奖一等奖。
鉴于该项目理论创新突出,成果丰硕,满足国家自然科学奖授奖条件,推荐该项目申报2019年度国家自然科学奖。
提名该项目为国家自然科学奖二等奖。
项目简介:多媒体信息理解是计算机学科的重要研究领域,迄今,已积累了对图像、音频、视频、图形等类型媒体进行处理的较成熟的理论和技术,然而在多媒体语义理解上,底层特征和高层语义之间的“鸿沟”一直是难以逾越的障碍。
究其因,传统的多媒体语义理解研究方法关注单一媒体自身的特征,而缺乏对图像、音频、视频等不同类型媒体进行交叉关联与综合处理的能力。
该项目提出了“跨媒体计算”基本理论,突出媒体之间的“跨越”这一科学问题,创造性提出了耦合关联建模、结构稀疏表达和跨媒体度量学习等一系列新方法,揭示了跨媒体内在本征表达和关联模式,发现了“结构稀疏选择”及“模态特征-隐性结构-高层语义”级联共享等认知机理,为解决“异构鸿沟”和“语义鸿沟”难题提供了新的计算模式,建立了以一种类型媒体检索另外一种媒体的信息检索新形式。
博世汽车SPC
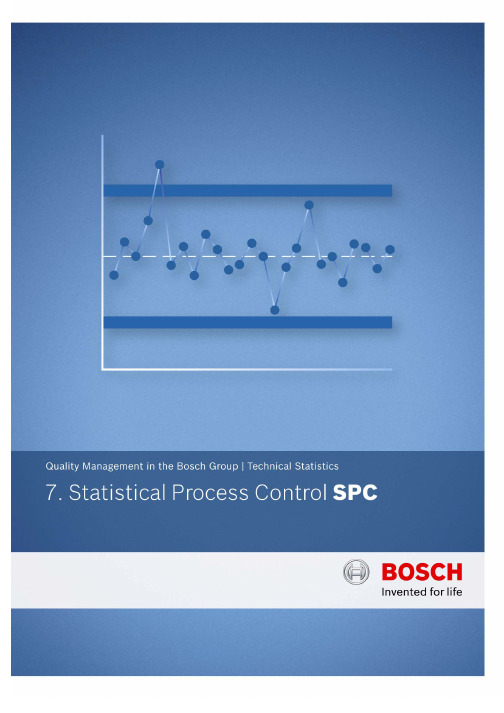
4th Edition, 07.20053rd Edition dated 06.19942nd Edition dated 05.19901st Edition dated 09.19872005 Robert Bosch GmbHTable of ContentsIntroduction (5)1. Terms for Statistical Process Control (6)2. Planning .........................................................................................................................................................8 2.1 Selection of Product Characteristics .................................................................................................8 2.1.1 Test Variable ........................................................................................................................8 2.1.2 Controllability ......................................................................................................................9 2.2 Measuring Equipment .......................................................................................................................9 2.3 Machinery .........................................................................................................................................9 2.4 Types of Characteristics and Quality Control Charts ......................................................................10 2.5 Random Sample Size ......................................................................................................................11 2.6 Defining the Interval for Taking Random Samples (11)3. Determining Statistical Process Parameters ................................................................................................12 3.1 Trial Run .........................................................................................................................................12 3.2 Disturbances ....................................................................................................................................12 3.3 General Comments on Statistical Calculation Methods ..................................................................12 3.4 Process Average ..............................................................................................................................13 3.5 Process Variation . (14)4. Calculation of Control Limits ......................................................................................................................15 4.1 Process-Related Control Limits ......................................................................................................15 4.1.1 Natural Control Limits for Stable Processes ......................................................................16 4.1.1.1 Control Limits for Location Control Charts .........................................................16 4.1.1.2 Control Limits for Variation Control Charts ........................................................18 4.1.2 Calculating Control Limits for Processes with Systematic Changes in the Average .........19 4.2 Acceptance Control Chart (Tolerance-Related Control Limits) .....................................................20 4.3 Selection of the Control Chart .........................................................................................................21 4.4 Characteristics of the Different Types of Control Charts . (22)5. Preparation and Use of Control Charts ........................................................................................................23 5.1 Reaction Plan (Action Catalog) .......................................................................................................23 5.2 Preparation of the Control Chart .....................................................................................................23 5.3 Use of the Control Chart .................................................................................................................23 5.4 Evaluation and Control Criteria ......................................................................................................24 5.5 Which Comparisons Can be Made? (25)6. Quality Control, Documentation .................................................................................................................26 6.1 Evaluation .......................................................................................................................................26 6.2 Documentation .. (26)7. SPC with Discrete Characteristics ...............................................................................................................27 7.1 General ............................................................................................................................................27 7.2 Defect Tally Chart for 100% Testing . (27)8. Tables (28)9. Example of an Event Code for Mechanically Processed Parts ....................................................................29 9.1 Causes .............................................................................................................................................29 9.2 Action ..............................................................................................................................................29 9.3 Handling of the Parts/Goods ...........................................................................................................30 9.4 Action Catalog .. (30)10. Example of an x -s Control Chart (32)11. Literature (33)12. Symbols (34)Index (35)IntroductionStatistical Process Control (SPC) is a procedure for open or closed loop control of manufacturing processes, based on statistical methods.Random samples of parts are taken from the manufacturing process according to process-specific sampling rules. Their characteristics are measured and entered in control charts. This can be done with computer support. Statistical indicators are calculated from the measurements and used to assess the current status of the process. If necessary, the process is corrected with suitable actions.Statistical principles must be observed when taking random samples.The control chart method was developed by Walter Andrew Shewhart (1891-1967) in the 1920´s and described in detail in his book “Economic Control of Quality of Manufactured Product”, published in 1931.There are many publications and self-study programs on SPC. The procedures described in various publications sometimes differ significant-ly from RB procedures.SPC is used at RB in a common manner in all divisions. The procedure is defined in QSP0402 [1] in agreement with all business divisions and can be presented to customers as the Bosch approach.Current questions on use of SPC and related topics are discussed in the SPC work group. Results that are helpful for daily work and of general interest can be summarized and published as QA Information sheets. SPC is an application of inductive statistics. Not all parts have been measured, as would be the case for 100% testing. A small set of data, the random sample measurements, is used to estimate parameters of the entire population.In order to correctly interpret results, we have to know which mathematical model to use, where its limits are and to what extent it can be used for practical reasons, even if it differs from the real situation.We differentiate between discrete (countable) and continuous (measurable) characteristics. Control charts can be used for both types of characteristics.Statistical process control is based on the concept that many inputs can influence a process.The “5 M´s” – man, machine, material, milieu, method – are the primary groups of inputs. Each “M” can be subdivided, e.g. milieu in temperature, humidity, vibration, contamination, lighting, ....Despite careful process control, uncontrolled, random effects of several inputs cause deviation of actual characteristic values from their targets (usually the middle of the tolerance range).The random effects of several inputs ideally result in a normal distribution for the characteristic.Many situations can be well described with a normal distribution for SPC.A normal distribution is characterized with two parameters, the mean µ and the standard deviation σ.The graph of the density function of a normal distribution is the typical bell curve, with inflection points at σµ− and σµ+.In SPC, the parameters µ and σ of the population are estimated based on random sample measurements and these estimates are used to assess the current status of the process.1. Terms for Statistical Process ControlProcessA process is a series of activities and/or procedures that transform raw materials or pre-processed parts/components into an output product.The definition from the standard [3] is: “Set of interrelated or interacting activities which trans-forms inputs into outputs.”This booklet only refers to manufacturing or assembly processes.Stable processA stable process (process in a state of statistical control) is only subject to random influences (causes). Especially the location and variation of the process characteristic are stable over time (refer to [4])Capable processA process is capable when it is able to completely fulfill the specified requirements. Refer to [11] for determining capability indices. Shewhart quality control chartQuality control chart for monitoring a parameter of the probability distribution of a characteristic, in order to determine whether the parameter varies from a specified value.SPCSPC is a standard method for visualizing and controlling (open or closed loop) processes, based on measurements of random samples.The goal of SPC is to ensure that the planned process output is achieved and that corresponding customer requirements are fulfilled.SPC is always linked to (manual or software supported) use of a quality control chart (QCC). QCC´s are filled out with the goal of achieving, maintaining and improving stable and capable processes. This is done by recording process or product data, drawing conclusions from this data and reacting to undesirable data with appropriate actions.The following definitions are the same as or at least equivalent to those in [6].Limiting valueLower or upper limiting valueLower limiting valueLowest permissible value of a characteristic (lower specification limit LSL)Upper limiting valueHighest permissible value of a characteristic (upper specification limit USL)ToleranceUpper limiting value minus lower limiting value:LSLUSLT−=Tolerance rangeRange of permissible characteristic values between the lower and upper limiting valuesCenter point C of the tolerance rangeThe average of the lower and upper limiting values:2LSLUSL C +=Note: For characteristics with one-sided limits (only USL is specified), such as roughness (Rz), form and position (e.g. roundness, perpen-dicularity), it is not appropriate to assume 0=LSL and thus to set 2/USLC= (also refer to the first comment in Section 4.1.1.1).PopulationThe total of all units taken into considerationRandom sampleOne or more units taken from the population or from a sub-population (part of a population)Random sample size nThe number of units taken for the random sample Mean (arithmetic)The sum of theix measurements divided by the number of measurements n:∑=⋅=niixnx11Median of a sampleFor an odd number of samples put in order from the lowest to highest value, the value of the sample number (n+1)/2. For an even number of samples put in order from the lowest to highest value, normally the average of the two samples numbered n/2 and (n/2)+1. (also refer to [13])Example: For a sample of 5 parts put in order from the lowest to the highest value, the median is the middle value of the 5 values.Variance of a sampleThe sum of the squared deviations of the measurements from their arithmetic mean, divided by the number of samples minus 1:()∑=−⋅−=niixxns12211Standard deviation of a sampleThe square root of the variance:2ss=RangeThe largest individual value minus the smallest individual value:minmaxxxR−=2. PlanningPlanning according to the current edition of QSP 0402 “SPC”, which defines responsibilities. SPC control of a characteristic is one possibility for quality assurance during manufacturing and test engineering.2.1 Selection of Product CharacteristicsSpecification of SPC characteristics and their processes should be done as early as possible (e.g. by the simultaneous engineering team). They can also, for example, be an output of the FMEA.This should take• Function,• Reliability,• Safety,•Consequential costs of defects,•The degree of difficulty of the process,• Customer requests, and•Customer connection interfaces, etc.into account.The 7 W-questions can be helpful in specifying SPC characteristics (refer to “data collection” in “Elementary Quality Assurance Tools” [8]): Example of a simple procedure for inspection planning:Why do I need to know what, when, where and how exactly?How large is the risk if I don’t know this? Note: It may be necessary to add new SPC characteristics to a process already in operation. On the other hand, there can be reasons (e.g. change of a manufacturing method or intro-duction of 100% testing) for replacing existing SPC control with other actions.SPC characteristics can be product or process characteristics.Why?Which or what? Which number or how many?Where? Who?When?With what or how exactly?2.1.1 Test VariableDefinition of the “SPC characteristic”, direct or indirect test variable. Note: If a characteristic cannot be measured directly, then a substitute characteristic must be found that has a known relationship to it.2.1.2 ControllabilityThe process must be able to be influenced (controlled) with respect to the test variable. Normally manufacturing equipment can be directly controlled in a manner that changes the test variable in the desired way (small control loop). According to Section 1, “control” in the broadest sense can also be a change of tooling, machine repair or a quality meeting with a supplier to discuss quality assurance activities (large control loop).2.2 Measuring EquipmentDefinition and procurement or check of the measuring equipment for the test variable.Pay attention to:• Capability of measuring and test processes, • Objectiveness,• Display system (digital),• Handling. The suitability of a measurement process for the tested characteristic must be proven with a capability study per [12].In special cases, a measurement process with known uncertainty can be used (pay attention to [10] and [12]).Note: The units and reference value must correspond to the variables selected for the measurement process.2.3 MachineryBefore new or modified machinery is used, a machine capability study must be performed (refer to QSP0402 [1] and [11]). This also applies after major repairs.Short-term studies (e.g. machine capability studies) register and evaluate characteristics of products that were manufactured in one continuous production run. Long-term studies use product measurements from a longer period of time, representative of mass production. Note: The general definition of SPC (Section 1) does not presume capable machines. However, if the machines are not capable, then additional actions are necessary to ensure that the quality requirements for manufactured products are fulfilled.2.4 Types of Characteristics and Control Charts This booklet only deals with continuous anddiscrete characteristics. Refer to [6] for these andother types of characteristics.In measurement technology, physical variables are defined as continuous characteristics. Counted characteristics are special discrete characteristics. The value of the characteristic is called a “counted value”. For example, the number of “bad” parts (defective parts) resulting from testing with a limit gage is a counted value. The value of the characteristic (e.g. the number 17, if 17 defective parts were found) is called a “counted value”.SPC is performed with manually filled out form sheets (quality control charts) or on a computer.A control chart consists of a chart-like grid for entering numerical data from measured samples and a diagram to visualize the statistical indices for the process location and variation calculated from the data.If a characteristic can be measured, then a control chart for continuous characteristics must be used. Normally the sx− chart with sample size 5=n is used.2.5 Random Sample SizeThe appropriate random sample size is a compromise between process performance, desired accuracy of the selected control chart (type I and type II errors, operation characteristic) and the need for an acceptable amount of testing. Normally 5=n is selected. Smaller random samples should only be selected if absolutely necessary.2.6 Defining the Interval for Taking Random SamplesWhen a control chart triggers action, i.e. when the control limits are exceeded, the root cause must be determined as described in Section 5.4, reaction to the disturbance initiated with suitable actions (refer to the action catalog) and a decision made on what to do with the parts produced since the last random sample was taken. In order to limit the financial “damage” caused by potentially necessary sorting or rework, the random sample interval – the time between taking two random samples – should not be too long.The sampling interval must be individually determined for each process and must be modified if the process performance has permanently changed.It is not possible to derive or justify the sampling interval from the percentage of defects. A defect level well below 1% cannot be detected on a practical basis with random samples. A 100% test would be necessary, but this is not the goal of SPC. SPC is used to detect process changes.The following text lists a few examples of SPC criteria to be followed.1. After setup, elimination of disturbances orafter tooling changes or readjustment, measure continuously (100% or with randomsamples) until the process is correctly centered (the average of several measure-ments/medians!). The last measurements canbe used as the first random sample for furtherprocess monitoring (and entered in the control chart). 2. Random sample intervals for ongoingprocess control can be defined in the following manner, selecting the shortest interval appropriate for the process.Definition corresponding to the expected average frequency of disturbances (as determined in the trial run or as is knownfrom previous process experience).Approximately 10 random samples within this time period.Definition depending on specified preventivetooling changes or readjustment intervals.Approximately 3 random samples within thistime period.Specification of tooling changes or readjust-ment depending on SPC random samples.Approximately 5 random samples within theaverage tooling life or readjustment interval.But at least once for the production quantitythat can still be contained (e.g. delivery lot,transfer to the next process, defined lots forconnected production lines)!3. Take a final random sample at the end of aseries, before switching to a different producttype, in order to confirm process capabilityuntil the end of the series.Note: The test interval is defined based on quantities (or time periods) in a manner that detects process changes before defects are produced. More frequent testing is necessary for unstable processes.3. Determining Statistical Process Parameters3.1 Trial RunDefinition of control limits requires knowledge or estimation of process parameters. This is determined with a trial run with sampling size and interval as specified in Sections 2.5 and 2.6. For an adequate number of parts for initial calculations, take a representative number of unsorted parts, at least 25=m samples (with n = 5, for example), yielding no fewer than 125 measured values. It is important to assess the graphs of the measured values themselves, the means and the standard deviations. Their curves can often deliver information on process performance characteristics (e.g. trends, cyclical variations).3.2 DisturbancesIf non-random influences (disturbances) occur frequently during the trial run, then the process is not stable (not in control). The causes of the disturbances must be determined and elimi-nated before process control is implemented (repeat the trial run).3.3 General Comments on Statistical Calculation MethodsComplicated mathematical procedures are no longer a problem due to currently available statistics software, and use of these programs is of course allowed and widespread (also refer to QSP0402 [1]).The following procedures were originally developed for use with pocket calculators. They are typically included in statistics programs.Note: Currently available software programs allow use of methods for preparing, using and evaluation control charts that are better adapted to process-specific circumstances (e.g. process models) than is possible with manual calculation methods. However, this unavoidably requires better knowledge of statistical methods and use of statistics software. Personnel and training requirements must take this into account.Each business division and each plant should have a comprehensively trained SPC specialist as a contact person.Parameter µ is estimated by:Example (Section 10): samplesof number valuesx the of total mxx mj j===∑=1ˆµ3622562862662.......x ˆ=+++==µor:samplesof number mediansthe of total mxx m j j===∑=1~~ˆµ46225626363....x ~ˆ=+++==µIf µˆ significantly deviates from the center point C for a characteristic with two-sided limits, then this deviation should be corrected by adjusting the machine.Parameter σ is estimated by:Example (Section 10):a) ∑=⋅=m j j s m 121ˆσ41125552450550222.......ˆ=+++=σsamplesof number variancesthe of total =σˆNote: s =σˆ is calculated directly from 25 individual measurements taken from sequential random samples (pocket calculator).or b) na s=σˆ, where27125552450550.......s =+++=samplesof number deviationsdard tan s the of total ms s mj j==∑=1351940271...a s ˆn ===σnn a3 0.89 5 0.94 See Section 8, Table 1 7 0.96 for additional valuesor c) ndR =σˆ, with96225611....R =+++= samplesof number rangesthe of total mR R mj j==∑=1271332962...d R ˆn ===σn n d3 1.69 5 2.33 See Section 8, Table 1 7 2.70 for additional values Note: The use of table values n a and n d pre-supposes a normal distribution!Some of these calculation methods were originally developed to enable manual calculation using a pocket calculator. Formula a) is normally used in currently available statistics software.4. Calculation of Control Limits4.1 Process-Related Control LimitsThe control limits (lower control limit LCL andupper control limit UCL) are set such that 99% of all the values lie within the control limits in the case of a process which is only affected by random influences (random causes).If the control limits are exceeded, it must there-fore be assumed that systematic, non-random influences (non-random causes) are affecting the process.These effects must be corrected or eliminated by taking suitable action (e.g. adjustment).Relation between the variance (standard deviation x σ) of the single values (original values, individuals) and the variance (standard deviation x σ) of the mean values: nxx σσ=.4.1.1 Natural Control Limits for Stable Processes4.1.1.1 Control Limits for Location Control Charts (Shewhart Charts)For two-sided tolerances, the limits for controlling the mean must always be based on the center point C. Note: C is replaced by the process mean x =µˆ for processes where the center point C cannot be achieved or for characteristics with one-sided limits.* Do not use for moving calculation of indices!Note: Use of the median-R chart is onlyappropriate when charts are manually filled out, without computer support.n*A E C n c'E EE E3 1.68 1.02 1.16 2.93 1.73 5 1.230.59 1.20 3.09 1.337 1.020.44 1.21 3.19 1.18Estimated values µˆ and σˆ are calculated per Sections 3.4 and 3.5.Refer to Section 8 Table 2 for additional values.Comments on the average chart: For characteristics with one-sided limits (or in general for skewed distributions) and small n , the random sample averages are not necessarily normally distributed. It could be appropriate to use a Pearson chart in this case. This chart has the advantage compared to the Shewhart chart that the control limits are somewhat wider apart. However, it has the disadvantage that calculation of the control limits is more complicated, in actual practice only possible on the computer.Control charts with moving averagesThe x chart with a moving average is a special case of the x chart. For this chart, only single random samples are taken.n sample measurements are formally grouped as a random sample and the average of these n measurements is calculated as the mean.For each new measurement from a single random sample that is added to the group, the first measurement of the last group is deleted, yielding a new group of size n , for which the new average is calculated.Of course, moving averages calculated in this manner are not mutually independent. That is why this chart has a delayed reaction to sudden process changes. The control limits correspond to those for “normal” average charts:σˆn .C LCL ⋅−=582 σˆn.C UCL ⋅+=582Calculate σˆ according to Section 3.5 a)Control limits for )3(1=n :σˆ.C LCL ⋅−=51 σˆ.C UCL ⋅+=51Example for )3(1=n :3 74 741.x = 3 7 4 9 762.x = 3 7 4 9 2 053.x = 3 7 4 9 2 8 364.x =This approach for moving sample measurements can also be applied to the variation, so that an s x − chart with a moving average and moving standard deviation can be used.After intervention in the process or process changes, previously obtained measurements may no longer be used to calculate moving indices.4.1.1.2 Control Limits for Variation Control ChartsThe control limits to monitor the variation (depending on n ) relate to σˆ and s and like-wise R (= “Central line”).s charta) generally applicable formula(also for the moving s x − chart)Example (Section 10):σˆB UCL 'Eob⋅= 62351931...UCL =⋅=σˆB LCL 'Eun ⋅= 30351230...LCL =⋅=b) for standard s x − chartNote: Formula a) must be used in the case ofmoving s calculation. Calculation of σˆ per Section 3.5 a).s B UCL *Eob ⋅= 62271052...UCL =⋅=s B LCL *Eun ⋅=30271240...LCL =⋅=R chartR D UCL Eob ⋅=2696212...UCL =⋅=R D LCL Eun ⋅=70962240...LCL =⋅=Tablen 'Eun B 'Eob B *Eun B *Eob B Eun D Eob D3 5 70.07 0.23 0.34 2.30 1.93 1.76 0.08 0.24 0.35 2.60 2.05 1.88 0.08 0.24 0.34 2.61 2.10 1.91See Section 8, Table 2 for further values4.1.2 Calculating Control Limits for Processes with Systematic Changes in the AverageIf changes of the mean need to be considered as a process-specific feature (trend, lot steps, etc.) and it is not economical to prevent such changes of the mean, then it is necessary to extend the “natural control limits”. The procedure for calculating an average chart with extended control limits is shown below.The overall variation consists of both the “inner” variation (refer to Section 3.5) of the random samples and of the “outer” variation between the random samples.Calculation procedure Control limits for the mean。
基于对象特征的深度哈希跨模态检索

基于对象特征的深度哈希跨模态检索朱杰1,白弘煜1,张仲羽1,谢博鋆2,张俊三3+1.中央司法警官学院信息管理系,河北保定0710002.河北大学数学与信息科学学院,河北保定0710023.中国石油大学(华东)计算机科学与技术学院,山东青岛266580+通信作者E-mail:*******************.cn 摘要:随着不同模态的数据在互联网中的飞速增长,跨模态检索逐渐成为了当今的一个热点研究问题。
哈希检索因其快速、有效的特点,成为了大规模数据跨模态检索的主要方法之一。
在众多图像-文本的深度跨模态检索算法中,设计的准则多为尽量使得图像的深度特征与对应文本的深度特征相似。
但是此类方法将图像中的背景信息融入到特征学习中,降低了检索性能。
为了解决此问题,提出了一种基于对象特征的深度哈希(OFBDH )跨模态检索方法。
此方法从特征映射中学习到优化的、有判别力的极大激活特征作为对象特征,并将其融入到图像与文本的跨模态网络学习中。
实验结果表明,OFBDH 能够在MIRFLICKR-25K 、IAPR TC-12和NUS-WIDE 三个数据集上获得良好的跨模态检索结果。
关键词:对象特征;跨模态损失;网络参数学习;检索文献标志码:A中图分类号:TP391Object Feature Based Deep Hashing for Cross-Modal RetrievalZHU Jie 1,BAI Hongyu 1,ZHANG Zhongyu 1,XIE Bojun 2,ZHANG Junsan 3+1.Department of Information Management,The National Police University for Criminal Justice,Baoding,Hebei 071000,China2.College of Mathematics and Information Science,Hebei University,Baoding,Hebei 071002,China3.College of Computer Science and Technology,China University of Petroleum,Qingdao,Shandong 266580,China Abstract:With the rapid growth of data with different modalities on the Internet,cross-modal retrieval has gradually become a hot research topic.Due to its efficiency and effectiveness,Hashing based methods have become one of the most popular large-scale cross-modal retrieval strategies.In most of the image-text cross-modal retrieval methods,the goal is to make the deep features of the images similar to the corresponding deep text features.However,these methods incorporate background information of the images into the feature learning,as a result,the retrieval performance is decreased.To solve this problem,OFBDH (object feature based deep Hashing)is proposed to learn计算机科学与探索1673-9418/2021/15(05)-0922-09doi:10.3778/j.issn.1673-9418.2006062基金项目:国家自然科学基金(61802269);河北省自然科学基金青年基金项目(F2018511002);河北省高等学校科学技术研究项目(Z2019037,QN2018251);河北大学高层次创新人才科研启动经费项目;2019年中央司法警官学院省级大学生创新创业训练计划项目(S201911903004)。
产品处理流程和方法

产品处理流程和方法英文回答:Product Handling Processes and Methodologies.Product handling involves a wide range of processes and methodologies that ensure the safe, efficient, andeffective movement of products throughout the supply chain. These processes can vary depending on the specific industry, product characteristics, and the scale of operations. The primary objectives of product handling are to:Maintain product quality and prevent damage.Optimize workflow and reduce lead times.Control inventory and minimize waste.Ensure compliance with regulations and industry standards.Enhance safety and minimize accidents.Common product handling processes include:Receiving and Inspection: Products are received from suppliers and inspected for quality, quantity, and compliance with purchase orders.Storage and Warehousing: Products are stored in warehouses or distribution centers under appropriate conditions to maintain their integrity.Order Picking and Fulfillment: Customer orders are picked from inventory and packaged for shipment.Shipping and Transportation: Products are transportedto customers using various modes of transport (e.g., trucking, rail, air).Returns and Claims Processing: Returned products are managed, inspected, and processed for credit or replacement.Product handling methodologies focus on improving efficiency and reducing costs. These methodologies include:First-In, First-Out (FIFO): Products are stored and retrieved based on their arrival date, ensuring that older products are shipped first.Last-In, First-Out (LIFO): Products are stored and retrieved in the reverse order of their arrival, meaning that newer products are shipped first.Cross-docking: Products are unloaded from incoming trucks and directly loaded onto outbound trucks, minimizing storage time.Automated Storage and Retrieval Systems (ASRS): Computer-controlled systems automate the storage and retrieval of products, reducing labor costs and improving accuracy.中文回答:产品处理流程和方法。
抗超氧化物歧化酶1抗体说明书
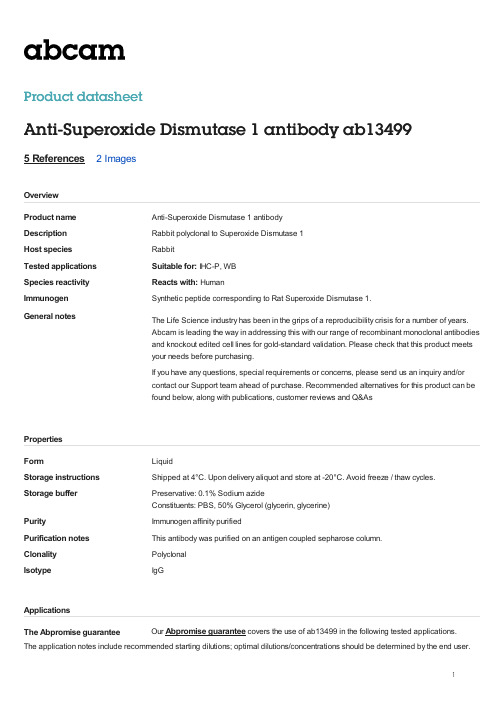
Product nameAnti-Superoxide Dismutase 1 antibody DescriptionRabbit polyclonal to Superoxide Dismutase 1Host speciesRabbit Tested applicationsSuitable for: IHC-P, WB Species reactivityReacts with: Human ImmunogenSynthetic peptide corresponding to Rat Superoxide Dismutase 1.General notes The Life Science industry has been in the grips of a reproducibility crisis for a number of years.Abcam is leading the way in addressing this with our range of recombinant monoclonal antibodiesand knockout edited cell lines for gold-standard validation. Please check that this product meetsyour needs before purchasing.If you have any questions, special requirements or concerns, please send us an inquiry and/orcontact our Support team ahead of purchase. Recommended alternatives for this product can befound below, along with publications, customer reviews and Q&AsFormLiquid Storage instructionsShipped at 4°C. Upon delivery aliquot and store at -20°C. Avoid freeze / thaw cycles.Storage bufferPreservative: 0.1% Sodium azide Constituents: PBS, 50% Glycerol (glycerin, glycerine)PurityImmunogen affinity purified Purification notesThis antibody was purified on an antigen coupled sepharose column.ClonalityPolyclonal Isotype IgGThe Abpromise guarantee Product datasheetAnti-Superoxide Dismutase 1 antibody ab134995 References 2 ImagesOverviewPropertiesApplicationsOur Abpromise guarantee covers the use of ab13499 in the following tested applications.The application notes include recommended starting dilutions; optimal dilutions/concentrations should be determined by the end user.Function Destroys radicals which are normally produced within the cells and which are toxic to biologicalsystems.Involvement in disease Defects in SOD1 are the cause of amyotrophic lateral sclerosis type 1 (ALS1) [MIM:105400].ALS1 is a familial form of amyotrophic lateral sclerosis, a neurodegenerative disorder affectingupper and lower motor neurons and resulting in fatal paralysis. Sensory abnormalities are absent.Death usually occurs within 2 to 5 years. The etiology of amyotrophic lateral sclerosis is likely tobe multifactorial, involving both genetic and environmental factors. The disease is inherited in 5-10% of cases leading to familial forms.Sequence similarities Belongs to the Cu-Zn superoxide dismutase family.Post-translational modifications Unlike wild-type protein, the pathogenic variants ALS1 Arg-38, Arg-47, Arg-86 and Ala-94 are polyubiquitinated by RNF19A leading to their proteasomal degradation. The pathogenic variants ALS1 Arg-86 and Ala-94 are ubiquitinated by MARCH5 leading to their proteasomal degradation.The ditryptophan cross-link at Trp-33 is reponsible for the non-disulfide-linked homodimerization. Such modification might only occur in extreme conditions and additional experimental evidence is required.Cellular localization Cytoplasm. The pathogenic variants ALS1 Arg-86 and Ala-94 gradually aggregates andaccumulates in mitochondria.Western blot - Anti-Superoxide Dismutase 1 antibody (ab13499)Anti-Superoxide Dismutase 1 antibody (ab13499) at 1 µg/ml + Cell lysates prepared from mixed human cell lines (A431, A549,HCT116, HeLa, HEK293, HepG2, HL-60, HUVEC, Jurkat, MCF7, PC3 and T98G)Predicted band size: 18 kDaApplication Abreviews NotesIHC-P Use a concentration of 2 µg/ml. Perform heat mediated antigenretrieval before commencing with IHC staining protocol.WB Use a concentration of 1 µg/ml. Detects a band of approximately19, 23 kDa (predicted molecular weight: 18 kDa).TargetImagesImmunohistochemistry (Formalin/PFA-fixed paraffin-embedded sections) - Anti-Superoxide Dismutase 1 antibody (ab13499)Ab13499 staining Human normal colon. Staining is localized to the nucleus.Left panel: with primary antibody at 2 ug/ml. Right panel: isotype control.Sections were stained using an automated system DAKO Autostainer Plus , at room temperature. Sections were rehydrated and antigen retrieved with the Dako 3-in-1 AR buffer citrate pH 6.0 in a DAKO PT Link. Slides were peroxidase blocked in 3% H2O2 in methanol for 10 minutes. They were then blocked with Dako Protein block for 10 minutes (containing casein 0.25% in PBS) then incubated with primary antibody for 20 minutes and detected with Dako Envision Flex amplification kit for 30 minutes. Colorimetric detection was completed with Diaminobenzidine for 5 minutes. Slides were counterstained with Haematoxylin and coverslipped under DePeX. Please note that for manual staining we recommend to optimize the primary antibody concentration and incubation time (overnight incubation), and amplification may be required.Please note: A ll products are "FOR RESEA RCH USE ONLY. NOT FOR USE IN DIA GNOSTIC PROCEDURES"Our Abpromise to you: Quality guaranteed and expert technical supportReplacement or refund for products not performing as stated on the datasheetValid for 12 months from date of deliveryResponse to your inquiry within 24 hoursWe provide support in Chinese, English, French, German, Japanese and SpanishExtensive multi-media technical resources to help youWe investigate all quality concerns to ensure our products perform to the highest standardsIf the product does not perform as described on this datasheet, we will offer a refund or replacement. For full details of the Abpromise, please visit https:///abpromise or contact our technical team.Terms and conditionsGuarantee only valid for products bought direct from Abcam or one of our authorized distributors。
英语整理文献阅读
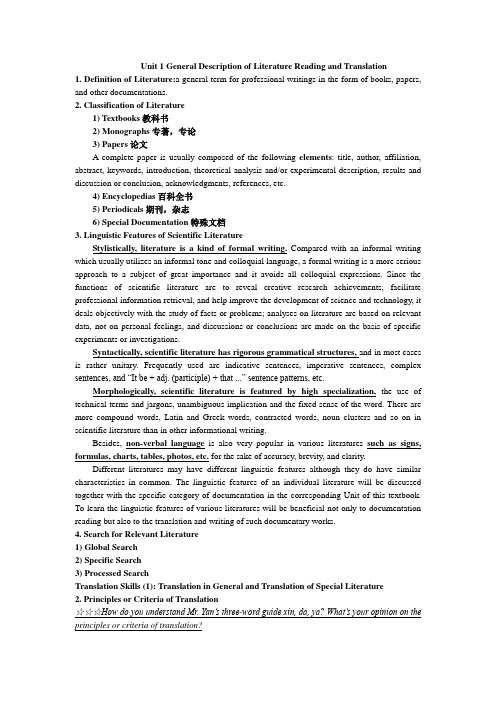
Unit 1 General Description of Literature Reading and Translation1. Definition of Literature:a general term for professional writings in the form of books, papers, and other documentations.2. Classification of Literature1) Textbooks教科书2) Monographs专著,专论3) Papers论文A complete paper is usually composed of the following elements: title, author, affiliation, abstract, keywords, introduction, theoretical analysis and/or experimental description, results and discussion or conclusion, acknowledgments, references, etc.4) Encyclopedias百科全书5) Periodicals期刊,杂志6) Special Documentation特殊文档3. Linguistic Features of Scientific LiteratureStylistically, literature is a kind of formal writing. Compared with an informal writing which usually utilizes an informal tone and colloquial language, a formal writing is a more serious approach to a subject of great importance and it avoids all colloquial expressions. Since the functions of scientific literature are to reveal creative research achievements, facilitate professional information retrieval, and help improve the development of science and technology, it deals objectively with the study of facts or problems; analyses on literature are based on relevant data, not on personal feelings, and discussions or conclusions are made on the basis of specific experiments or investigations.Syntactically, scientific literature has rigorous grammatical structures, and in most cases is rather unitary. Frequently used are indicative sentences, imperative sentences, complex sentences, and “It be + adj. (participle) + that ...” sentence patterns, etc.Morphologically, scientific literature is featured by high specialization,the use of technical terms and jargons, unambiguous implication and the fixed sense of the word. There are more compound words, Latin and Greek words, contracted words, noun clusters and so on in scientific literature than in other informational writing.Besides, non-verbal language is also very popular in various literatures such as signs, formulas, charts, tables, photos, etc. for the sake of accuracy, brevity, and clarity.Different literatures may have different linguistic features although they do have similar characteristics in common. The linguistic features of an individual literature will be discussed together with the specific category of documentation in the corresponding Unit of this textbook. To learn the linguistic features of various literatures will be beneficial not only to documentation reading but also to the translation and writing of such documentary works.4. Search for Relevant Literature1) Global Search2) Specific Search3) Processed SearchTranslation Skills (1): Translation in General and Translation of Special Literature2. Principles or Criteria of Translation☆☆☆How do you understand Mr. Yan’s three-word guide xin, da, ya? What’s your opinion on the principles or criteria of translation?答:Despite a variety of opinions, two criteria are almost unanimously accepted by all, namely, the criterion of faithfulness/accuracy (忠实/准确) and that of smoothness (流畅). We may also take these two criteria as the principles of scientific literature translation. By faithfulness/accuracy, we mean to be faithful not only to the original contents, to the original meaning and views, but also to the original form and style. By smoothness, we mean not only easy and readable rendering, but also idiomatic expression in the target language, free from stiff formula and mechanical copying from dictionaries.3. Literal Translation and Free TranslationWhat are literal translation and free translation? And what principles should a translator abide by in applying them?答:Literal translation (直译) and free translation (意译) are two dynamic approaches in dealing with such awkward situations.The so-called literal translation, superficially speaking, means “not to alter the original words and sentences”; strictly speaking, it strives “to keep the sentiments and style of the original.” It takes sentences as its basic units and takes the whole text (discourse) into consideration at the same time in the course of translation. Furthermore, it strives to reproduce both the ideological content and the style of the original works and retains as much as possible the figures of speech. There are quite a lot of examples of successful literal translation that have been adopted as idiomatic Chinese expressions. For example, crocodile‟s tears (鳄鱼的眼泪), armed to the teeth (武装到牙齿), chain reaction (连锁反应), gentlemen‟s agreement (君子协定), and so on. Similarly, some Chinese idioms also find their English counterparts through literal translation. For example, 纸老虎(paper tiger),一国两制(one country, two systems ), and so on.Free translation is an alternative approach which is used mainly to convey the meaning and spirit of the original without trying to reproduce its sentence patterns or figures of speech. This approach is most frequently adopted when it is really impossible for the translator to do literal translation. For example:Adam‟s Apple 喉结at sixes and sevens 乱七八槽It rains cats and dogs. 大雨滂沱Don‟t cross the bridge till you get to it. 不必担心过早。
逆向文件频率(inverse
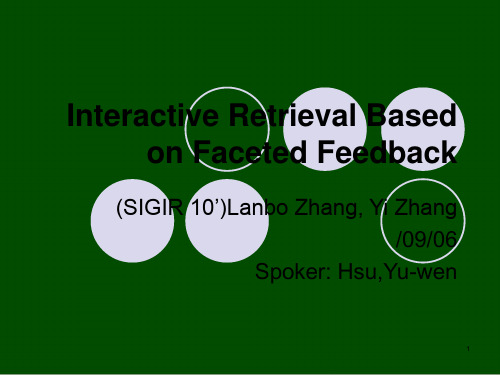
Facet-value pair recommendation
in the form of facet-value pairs *Experimental settings
Recall@N (R@N) (the recall of top N documents)
7
*Top Document Frequency (TDF)
initial query
baseline retrieval algorithm
ranked document
Document 1 Document 2 Document 3
Document N
candidate 1 2 3 4
K
assumption: the more frequently a facet-value pair appears in the top ranked documents, the more likely the user will like it.
each metadata field is called a facet, and a facet with a specific value is called a facet-value pair
language: Chinese format: ppt subject: IR genre: comedy
逆向文件频率inverse逆向文件
Interactive Retrieval Based on Faceted Feedback
(SIGIR 10’)Lanbo Zhang, Yi Zhang /09/06
- 1、下载文档前请自行甄别文档内容的完整性,平台不提供额外的编辑、内容补充、找答案等附加服务。
- 2、"仅部分预览"的文档,不可在线预览部分如存在完整性等问题,可反馈申请退款(可完整预览的文档不适用该条件!)。
- 3、如文档侵犯您的权益,请联系客服反馈,我们会尽快为您处理(人工客服工作时间:9:00-18:30)。
Journal of Computational Information Systems 6:9(2010) 2821-2830Available at Cross-media Retrieval Based on CSRN ClusteringCheng ZENG1,†, Zhenzhen WANG1, Gang DU21State Key Lab of Software Engineering, Wuhan University, Wuhan, China, 4300722School of Computer, Wuhan University, Wuhan, China, 430072AbstractA novel cross-media retrieval methodology is proposed to help user more accurately and naturally describe theirrequirement and gain response. The first problem to be solved in this paper is how to build the bridge among heterogeneous information. An efficient approach is adopted to mine the semantic relationship among different multimedia data. We propose the scheme, called cross-media semantic relationship network (CSRN), to store the cross-media relationship knowledge, which is constructed by mining various potential relation information in the Internet. By hierarchical fuzzy clustering based on semantic relationship against CSRN, we obtain semantic vector bundle which could gather different modalities but same semantic of diverse media feature vectors. Furthermore, dynamic linear hash index is used in these vectors to adapt to the flexible expanding or updating of CSRN and quickly retrieval based on multiple examples without the limit of media modality by hash intersection. We show through experiment based on different modalities or different numbers of examples that our approach can provide more flexible retrieval mode and more accurate retrieval results.Keywords:Cross Media; Semantic Relationship; Semantic Vector Bundle; Hierarchical Fuzzy Clustering1. IntroductionCommercial multimedia search engines consider more on efficiency and realize search based on relevant technique of keywords or low-level features matching, such as Google.image, Bing.image and Like, GazoPa, etc. However, these systems provide only simple requirement expressing way to user so that user’s intention is difficult to be understood and the results are always poor amd single modality. On the contrary, academic circles are exploring better man-machine information exchanging technology, where the machine would adapt to the human’s habit of expressing and receiving information as much as possible, but not that the human have to adapt to the understanding ability of machine. Lots of new research topics emerge which generally refer to the concept “semantic”, such as semantic search, semantic multimedia, semantic computing and so on. Their common ground is to attempt to create, process or display information with the thinking way as human, e.g. IGroup[1] provides the semantic classifications of results. Hakia, Cuil, Kosmix and PlayAudioVideo support multimodal of results displaying, and Powerset focuses on natural language search. In addition, there are so many techniques[2-5] which realize semantic or personalized retrieval based on ontology.Many earlier cross-media studies [6,7] focused on integrating or managing heterogeneous multimedia data. Henceforth, cross-media technology is applied into diverse multimedia retrieval domain. Liu [8] proposes a cross-media manifold co-training mechanism between keyword-based metric space and vision-based metric space and deduce a best dual-space fusion by minimizing manifold-based visual & textual energy criterion. At last, he realizes image annotation and retrieval. In [9], a dual cross-media †Corresponding author.Email addresses: zengc@ (Cheng ZENG), zhenzhen_1987cn@ (Zhenzhen WANG),melephas@ (Gang DU)1553-9105/ Copyright © 2010 Binary Information PressSeptember, 20102822 C. Zeng et al. /Journal of Computational Information Systems 6:9(2010) 2821-2830 relevance model which considers both word-to-image and word-to-word relations is proposed to automatically annotate and retrieval images. Furthermore, there are lots of researches on video retrieval based on cross-media. Bruno et al. [10] designs a multimodal dissimilar space which is constructed based on positive and negative examples distribution and realizes video retrieval by space clustering and modalities fusing. Meanwhile, many researchers explore cross-media comprehensive retrieval way, such as a typical application about E-learning [11]. [12] utilizes the complementarity among different modalities of media data to mine co-occurrence relationships of news items, and then provides cross-media content complementation. Zhuang et al. [13,14] integrate the webpage structure analyzing and low-level features correlation mining of multimedia data to construct cross-media relationship graph UCCG, and short-term and long-term feedbacks play vital role in the method.Cross-media technology aims at making maximum use of relevance, cooperation, complementarity among diverse modalities of media to fuse all sorts of information. As a result, user can gain the desired information with higher accuracy, more quickly speed and lower cost. The kernel of realizing cross-media retrieval is to discover the semantic relationship among different multimedia data. However, the semantic “gap” and the feature diversity among various modalities of media make the development of this domain difficult in taking a step. Furthermore, the common character of high information density of multimedia data lead to that the relevant processes generally require a large amount of computing time and resources. It is very difficult to develop a universal cross-media retrieval engine at present. However, the technology has good application prospect in some certain domain, such as E-learning, E-business, tourism and entertainment, etc.2. Framework of Cross-media Retrieval SystemThis paper gains semantic relationships among diverse data from internet where multitudinous knowledge is contributed by various persons or software systems every moment. Initially, we don’t concern about the modality of data, but pay attention on the channels where we can get the relationship. All media objects, which are the results by processing multimedia document and semantic relationship among them will be stored into a unified model, called cross-media semantic relationship network (CSRN). For multimodal, time-based and text types of multimedia document, we segment them into the smaller granularity of unit. The kernel of our approach is how to construct and utilize CSRN. We give the definition of CSRN below: Definition 1. Let each node represent a modality of media object, the metadata structure of is as follows:, , , , , , , , , (1) Each edge , denotes semantic relationship between different media objects, which comes from 4 aspects:processes space dependency of web data based on visual structure and hyperlink in the webpage, e.g. adjacent text, image or other modality of media objects in a webpage have more similar semantic;analyzes the labels and ranking of search results coming from multiple multimedia search engines based on the same search condition;mines the inherent relevancy among different modalities of data or same modality of segmented data in a multimedia document, e.g. visual, audio and textual data in a news video, or all video scene clips in a sport video have certain relevancy each other;accumulates potential user feedback during the process of user browsing. E.g. those results clicked one after another generally have approximative topic or similar semantic.We call the the integration of these nodes and edges as CSRN.In formula (1), each media object has a globally unique identity SID and GID corresponds to the document ID. Moreover, not each media object corresponds to an example. Time-based media such as video, audio possibly contain too much semantic in a same document, which possibly lead to most media objects having semantic relaionship each other. Overly abundant semantic relationship in CSRN is equivalent to not be able to gain any valuable information. Moreover, a multimedia document includingC. Zeng et al. /Journal of Computational Information Systems 6:9(2010) 2821-2830 2823multiple modalities of data will also affect the analysis. Thus, it’s necessary to previously split or segment these multimedia documents before they are regarded as media objects. Likewise, text is segmented based on paragraph to reduce the semantic complexity of each media object.After CSRN is constructed, the hierarchical fuzzy clustering will be used on CSRN. The purpose is to put all media objects with similar semantic into same cluster, called semantic category (SC). It is possible that a media object belongs to multiple SC, and even several media objects belong to distinct or same SC in different conditions. Thus, the hierarchical fuzzy clustering method will be suitable and essential to these statuses. All media objects will be substituted for their feature vectors. Because there are a large number of different modalities of media object in same SC and many of them possess similar low-level features which are actually redundant, we quantify all feature vectors in a SC by feature vectors clustering against different modalities of media objects, respectively. Then the typical feature vectors(TV), called semantic vector bundle (SVB), will be selected to represent the SC. It means that we obtain the mapping among all possible feature vectors of different modalities but similar semantic. At last, hash indexs will be respectively created on these TV sets corresponding to different modalities of media objects. Thus, if a user submits several media examples with same or different modalities, they will be preprocessed and transformed into feature vectors, and then respectively locate their own SC sets. The architecture of our cross-media retrieval method is shown in Fig.1.3. Cross-media Semantic Relationship NetworkA great deal of researchers improve the system performance by mining implicit or explicit knowledge in the internet. Furthermore, most of commercial search engines use keywords and hyperlink extracted from Webpages to index the images, videos or other data. In our approach, all initial cross-media semanticrelationship knowledge comes from internet and these knowledge will be stored in CSRN.Fig.1 Architecture of Cross-media Retrieval System2824 C. Zeng et al. /Journal of Computational Information Systems 6:9(2010) 2821-28303.1. Webpage Visual Structures and Hyperlink AnalysisSome privious methods regard webpages as information units. A webpage generally contains multiple and different granularities of semantic so that these methods will confuse the semantic correlation among media objects. VIPS algorithm [15] is proposed to segment webpage by analyzing DOM tree based on visual structure. The highest sensitive granularity of VIPS is <TD> level because its purpose is to segment webpage block. But in our work, extracting the media objects and the relationship among them are the final purpose. Hence we improve VIPS algorithm so that it is sensitive to URL address, <BR> in continuous text and hyperlink etc.. Thus, each leaf node will actually correspond to a media object and the semantic relationship only exist between any two leaf nodes. Fig.2 shows an example of webpage visual segmentation and the final visual structure tree constructed by improved VIPS is shown in Fig.3.Fig.2 An Example of Webpage Visual Segmentation Fig.3 An Example of Webpage Visual Structure TreeThe similarity between any two nodes can be calculated based on the shortest path in the tree. Becausesemantic generalization will lose some semantic information while semantic specialization narrows therange of semantic description, we give different semantic attenuation constants α and βrespectivelycorresponding to upward and downward section in the path. The formula of relationship calculatingbetween any two media object and is as follows:, 1 0 1 0 (2) where n and m denote the numbers of upward and downward sections, respectively.3.2. Results Analysis of Multimedia Search EnginesMultimedia search engines return lots of related multimedia documents based on keywords, such asgoogle.image, Youtube, Flickr etc.. Hakia, Cuil even simultaneously returns different types of multimedia documents. A concept set is extracted from LSCOM, and then the set is extended with WordNet. These concepts will be selected or combined according to some certain rules in turn and be simultaneouslysubmited to all search engines. For each search, all returned results are expected to have similar semantic, and a sub-CSRN could be constructed. But polysemy confuses the results and affects the ranking.Contextual concepts in result labels are used to filter most confusing results. Thus, we syntheticallyconsider the result label which are preprocessed to only reserve noun and verb keywords, and result rankingof search engine to measure the semantic relationship among returned results.At first, , which denotes semantic similarity between search keywords set and concepts set corresponding to v th result label will be calculated:, , , ,,, , (3)In the above formula, KM denotes classical Kuhn-Munkres algorithm, and , denotes the semantic similarity between any two concepts , in and , respectively, and 0 , 0 where , are represented as the amount of retained search keywords and correspondingreturned results, respectively., represents the number of same concept between and , while,is the amount of all different concepts in and . is constant between 0 and 1 when theC. Zeng et al. /Journal of Computational Information Systems 6:9(2010) 2821-2830 2825 intersection between and is null after deleting same concepts from them. Semantic similarity function [16] based on WordNet is utilized to calculate the similarity between different concepts.,1 2 , ,, / , , ,(4) Detailed mathematical introduction of formula (4) can be found in [16]. We previously construct a concept similarity map (CSM) for avoiding repeatedly computing , .We define semantic relationship, of any two results (media objects) with same search keywords in formula (5). It is measured based on the cosine of angles between two result vectors constructed in the results semantic similarity space (R3S) where horizontal ordinate denotes the similarity degree between result label and search keywords, and vertical ordinate denotes ranking value., , ,, ,0 , (5)where , are decimal value which denote the results of ranking number i and j divided by , respectively.3.3. Other Aspects of Semantic Relationship and Their SynthesisFor mining aspect of relationship, we require to adjust the size of each time-based or multimodal media object in initial CSRN or even decompose it based on modality so as to simplify its semantic. Currently, there are lots of mature technique to support splitting diverse modalities of data from multimodal document, such as splitting audio from video. Then different modalities of time-based data contained in the same document will be jointly segmented with same boundary, where audio data are segmented referring to the results of video segmentation based on scene. Each video or audio segment would eventually correspond to a node (media object) in CSRN. The semantic relationship between different modalities of data segment within same time range are always 1, namely, 1. While semantic relationship between different data segments with same modality is defined based on adjacency distance:,1 01√ 1⁄ 1 (6)where x denotes the interval number of segment between and , and is a constant representing degeneration degree of semantic relationship.aspect will be able to optimize the relationship knowledge in CSRN after each time of cross-media search feedback, such as browsing, printing, downloading, etc., where the system will save operating results with same search condition. These are regarded as implicit user contributing. The relationship among the results will be used to update CSRN:, ∑ · 2, 0 (7)where denotes the amount that and have same operation in same search condition and represents the influence degree of each kind of operation. If the result of updated, is greater than 1, it will be directly replaced by 1.4. CSRN Index for Efficiently Retrieval and ExpandingYou could submit an image example and find a most similar image in CSRN with traditional feature matching technique, and then gain related diverse modalities of multimedia documents based on cross-media semantic relationship. However, when user submits several examples which are heterogeneous/homogeneous, the above way is possible to return several irrelative results sets. Furthermore, the retrieval efficiency will be low and CSRN expanding is difficult when new multimedia documents are inserted. Thus, we will introduce an efficient cross-media index mechanism in this section.2826 C. Zeng et al. /Journal of Computational Information Systems 6:9(2010) 2821-28304.1. Hierarchical Fuzzy ClusteringBy clustering on CSRN, all media objects with similar semantic can be congregated into the same semantic category (SC). However, most media objects possess complex semantic, and they are impossible to simply belong to a certain SC. Moreover, the clustering granularity is difficult to make certain. If the granularity is fine, we can obtain relatively accurate results but lose the recall ratio. Thus, it’s necessary to use hierarchical fuzzy clustering (HFC) algorithm against CSRN.The whole process of HFC includes two phases. In the first phase, K-distance neighbor is used to construct the initial status of HFC. Each media object and its K-distanced neighbors set can be picked out easily from CSRN which is stored with symmetric matrix. The average distance between and any object in can be calculated and denoted as . Then the relative density of K-distance neighbor of object is defined as follows:,…, , ,…,,…, , ,…, (8)Given the threshold value 0, is considered as a core object when 1 . For with , core objects set is defined as | where O is the list of entire core objects } which is the initial status of any cluster. In other words, a core object with current global minimum and without class mark will be picked out, and is obtained to form a new marked cluster . Then all objects in of cluster will be gradually extended by adding the K-distance neighbors of each core object in turn. Assume that is one core object in cluster , which has not been extended, and its K-distance neighbors can be obtained, denoted as 1,…, . If is a core object of other cluster, it will be ignored. Otherwise, it will be added into cluster . The cluster can be extended until no more objects in it can be extended. The process continues until all core objects have been extended. Here we can find that core objects are pivotal for each cluster and it is impossible that a core object belongs to more than one cluster, while other objects can exist in multiple clusters in the same time. During the course of extension, two clusters will be marked as adjacent clusters when part of objects they include are the same. Thus, we obtain the highest granularity of fuzzy clustering result.In the second phase, the clusters obtained in the first phase will be fuzzily clustered further. First, fuzzy similarity , of any two adjacent clusters and is calculated as:, ,, (9)Then descending threshold value set 1,…, is selected for measuring similariy from fine granularity to coarse granularity. For each cluster , similar cluster set under certain granularity level ∆ is defined as | , . The clusters in can be united into one cluster on level ∆. As the value of descend, we can obtain hierarchical clustering of objects and the granularities of clustering are from fine to coarse, until they are united into one cluster.4.2. Index on CSRNMedia feature vector quantification is the first process step which does not reduce the dimension number of vector but the amount of vectors. However, the dimension and implication of heterogeneous media feature vectors are different. Thus, we firstly distinguish feature vectors according to media modality, and then cluster these vectors with FEC algorithm [17], and the average vector (called typical vector, TV) is regarded as the representative of a cluster, shown in Fig.4. All TVs in same SC make up a semantic vector bundle (SVB). Each SVB enumerates various feature vectors which come from different modalities of media objects but represent similar semantic. We build the mapping relation , between TV and feature vectors in corrrespondng cluster and , between TV and SC where , σ respectively denotes the granularity level in HFC and media modality.For flexibly expanding or updating CSRN, and efficiently searching feature vector, we create dynamic linear hash index on which computes the hash value of TV only one times, stores hash values usingC. Zeng et al. /Journal of Computational Information Systems 6:9(2010) 2821-2830 2827as few buckets as possible and linearly increases buckets. Several vector hash functions are respectively defined for different dimension of vector corresponding to different media modality . The actual active bit number of could be calculated as follows, which always use the low order bits:(10)Where is represented as the amount of TV of modality σ.If a user submits an example , the system will extract its feature vector at first, and then invoke the corresponding . The returned result determines a unique hash bucket which stores a TV set having same hash value. The media object whose feature vector is most similar with must also exist in this bucket. Then, we use vector matching technique to locate the media object. Suppose Top( ) denoted the most similar TV with in hash bucket . We can get many SC sets of different granularity levels based on , .∑, (11) By now, user actually gains a result set containing multiple modalities of media objects which have been classified through SC in different granularity levels. is the highest granularity. If a user pays attention on precision, he can select fine granularity. When user submits multiple examples which are even different modalities, we use hash intersection to realize search. The search examples set is represented as . The system returns a corresponding to each example so that we can calculate the intersection among these SC sets of different examples in each granularity level.∑, (12)5. Experiments5.1. Data sources and CSRNOur experiments data all come from internet through webpage analysis which includes two aspects of pages. The first is the common pages of Web encyclopedia 1, Yahoo!news, etc.. We manually select the initial pages as entrance, and then the related webpages will be automatically crawled based on hyperlinks. The another is results page of multimedia search engines such as Google.image, Hakia, Flickr, etc. We extract 118 concepts with distinct visual or audio characteristic from LSCOM and expand them with synonym and cognate words in WordNet. At last, 203 concepts are used and they compose into 1065 keywords set, each set of which includes 1 to 3 concepts. These keywords set are simultaneously submited to all search engines in turn and are projected into the same R3S to calculate results similarity each other for same search condition. For ensuring relatively high accuracy and avoiding overfull results set, we only analyze and store 1; ; .Fig.4 The Schematic Diagram of CSRN Index2828 the top from 3some a minute For (mean,contras and th feature 5.2. Th Fig.5(1multip text by as lowest quantit contain semant video a and ge ways t higher filters reduce examp For by mu previou multip examp modali audio m precisi consid of com search 2http://w C p 15 results fo 352 video, 134audio material e data for all ti image, we ex , variance, ske st, Orientation he motion fea es slope, roll-o he Performan 1)-(3) show th le same moda y image as ex spect where te precision due ty in asp ns the feature tic relationshi and audio hav enerate relation to get audio d average searc the semantic e the deviatio les is similar a (1) Cross-media Image ExaFig.better reflecti ultiple modalit us dataset, sh le modalities les and more ities of media media data as ion of image+erable role du mbinational ex examples as/sm C. Zeng et al. /or each search 4,798 text par l websites suc ime-based me xtract 3 types o ewness), and 3n). For video ature histogra off, centroid an nce of Cross-m he experimen ality of media xample has the ext and imag e to less relati ect. Although e in key fram ip informatio ve better searc nship in data. It can be ch accuracies distinction am n between se as Zhuang’s a Retrieval by ample 5 Comparison of ng the charac ties of media hown in Fig.6s of example e modalities o a data as exam s examples ha +audio and v uring the proce xamples. Thes many as posmzy/dongwu-yx-7/Journal of Co h. We totally c ragraphs and 2ch as smzy 2 an edia.of color featur 3 types of text segment, we am in whole nd OER are u media Retrieva nt results of q a data as exam e highest prec e are in the m ionship which h video has sam me which is th n about imag h accuracies e aspect. But th e seen that m than single d mong differen earch example approach [14], b (2) f Cross-media Ret teristic of ind a data combin 6. We can ob es generally r of examples c mples, we can ave higher ac video+audio ess of cross-m se phenomeno sible will mak711-6.htmlomputational Icollect 40,610 2,809 audios nd so on. We re including c ture features i mainly reserv segment. For used.alquerying diffe mples which a cision because majority. But h are mainly l me situation a he same as an ge and enhan each other bec his situation w multiple docum document in a nt examples an es and user r ut we do not r Cross-media Ret Video Example trieval among Di ex intersection nation as exam bserve severa receives high can receive hi still get good ccuracy than p are higher th media retrieval on demonstratke the system Information S images and 3which are ext filter voice in color histogram including MR ve the feature r audio, cepst erent modaliti are all out of e we extract a querying aud limited in as audio, the fe n image. So a nce relational cause most of will be possibl ments as exam ll three figure nd receives th requirement. rely on iterativ trieval by e fferent Modalitie n in our appro mples where al interesting her search ac igher accuracy d results. Seco pure visual m han that of im l which signif te the principlm more accura Systems 6:9(203,379 video se tracted from v n audio and on m, color cohe RSAR, Gabor, e of key fram tral features ies of media our dataset. I a mass of sem dio by image and asp feature vector actually, video search accur f audio data ar ly changed if mples together es owing to SC heir semantic The search a ve short-term (3) Cross- Au es of Search Exam oach, we test c all examples phenomenon ccuracy than y. Even thoug ond, the comb media combina mage+video. ficantly raises le that using vately understa 010) 2821-283egments which videos or com nly analyze the rence, color m Tamura (coar me regarded as MFCC and s objects by sin In Fig.5(1), qu mantic relation as example h pects and has extracted from o indirectly u racy. In Fig.5e splitted from f we have mor r receive rema C intersection in common s accuracy of m feedback.-media Retrieval udio Example mples cross-media re are also out n. First, query single moda gh we only u ination of visu ation, e.g. the Third, text p s the search pr various modaland user requi 30h come me frome first 1moment rseness, s image spectral ngle or uerying nship in has the a small m video uses the (2)-(3), m video re other arkably n which so as to multiplebyetrieval t of the ying by ality of use two ual and search plays a recision lities ofirement。