Distribution - Wiley Home分布-威利家
维格纳—威利分布算法研究及应用
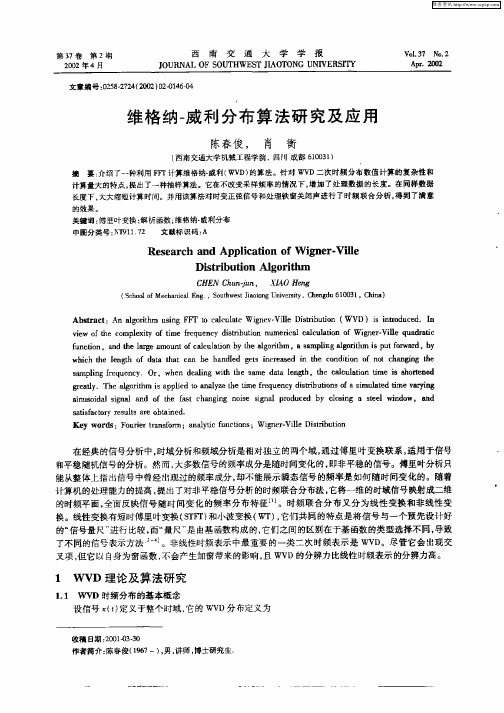
smpig f q e c Or a l eu n y n r ,w e el g wi e sle d t ln t h n d ai t t ai aa e gh,te c c lt n t s sotn n h h l h a uai me i h r e l o i ed
在经典的信号分析 中, 时域分析和频域分析是相对独立 的两个域 , 通过傅里叶变换联 系, 适用于信号 和平稳随机信号的分析 然而, 大多数信号 的频率成分是随时间变化的, 即非平稳 的信号。傅里叶分析只
能从整体上指 出信号中曾经出现过的频率成分 , 却不能展示 瞬态信号 的频率是如何随时 间变化 的。随着 计算机的处理能力的提高 , 出了对非平稳信号分析的时频联合分布法 , 提 它将一维的对域信号映射成二维 的时频平面 , 全面反映信号 随时间变化 的频率分布特征 …。时频联合分布又分为线性变换 和非线性变 换。线性变换有短时傅里叶变换(T T 和小渡变换 ( T , SF ) w ) 它们共同的特点是将信号与一个预先设计好 的“ 信号量尺” 进行 比较, 量尺” 而“ 是由基 函数构成 的, 它们之间的区别在于基函数 的类 型选择不同, 导致
中国分 类号 : T 1 2 N 9 1 7 文献标识码 : A
Re e r h a pl a in ofW in r Vi s a c nd Ap i to g e - le c Diti to g ih srbu i n Al ort m
C E h n , XA H n H NCu扣 I O eg
sn s ia in n o e fs h n ig n i s n l po u e b lsn te id w , a d iuod ls a a d f t at c a gn os i a rd c d y co ig a s l w n o gl h e g e n
经验模态分解和魏格纳-维利分布在往复泵泵阀振动信号特征提取中的应用

XU C a gh n I ifi h n .a g ,L U J— ,CHE Gu — ig ,XI ig e N or n u E Jn 。 (. ol efEe rm ca i l n zer gi hn nvrt o e o u D nyn 5 0 1 hn ; 1C lg l t eh n a gnei C i U i sy fP t l m, og i 2 76 ,C i e o co c E n n a e i re g a
t o f rfau e e t cin f m ir t n d t ftilx p mp v l e .F rt o l o e t r x r t o vb a i aa o p e u av s i l a o r o r s y,vb ain d t r e o o e n o s v rli — i r t aa a e d c mp s d i t e ea n o
tm e,fe e c nd a i r qu n y a mpltde d m an ft e o ii a ina a x rc e iu o is o h rg n lsg lc n bee ta t d. By c mb n n l W VDso MFs,t VD— o i i g al fI he W ba e i e・ e ue c itiut nso he o ii a i r to ina t n efc ie u r s in o h r s ・e m ntree c s d tm ・ q n y d srb i ft rg n lv b ai n sg lwih a fe tv s pp e so ft e c o str i e fr n e r f o ・
多分量线性调频信号时-频分析的交叉项抑制
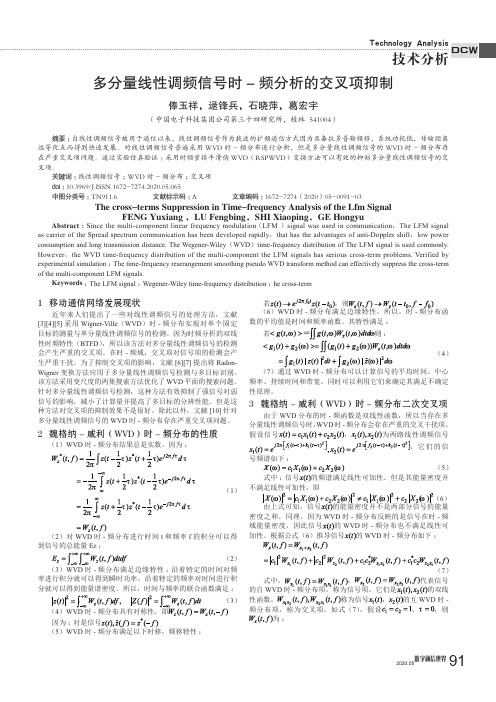
Technology Analysis技术分析DCW91数字通信世界2020.051 移动通信网络发展现状近年来人们提出了一些对线性调频信号的处理方法,文献[3][4][5]采用Wigner-Ville (WVD )时-频分布实现对单个固定目标的测量与单分量线性调频信号的检测。
因为时频分析的双线性时频特性(BTFD ),所以该方法对多分量线性调频信号的检测会产生严重的交叉项。
在时-频域,交叉项对信号项的检测会产生严重干扰。
为了抑制交叉项的影响,文献[6][7]提出将Radon-Wigner 变换方法应用于多分量线性调频信号检测与多目标识别。
该方法采用变尺度的两集搜索方法优化了WVD 平面的搜索问题。
针对多分量线性调频信号检测,这种方法有效抑制了强信号对弱信号的影响,减小了计算量并提高了多目标的分辨性能。
但是这种方法对交叉项的抑制效果不是很好。
除此以外,文献[10]针对多分量线性调频信号的WVD 时-频分布存在严重交叉项问题。
2 魏格纳-威利(WVD)时-频分布的性质(1)WVD 时-频分布结果总是实数。
因为:(1)(2)对WVD 时-频分布进行时间t 和频率f 的积分可以得到信号的总能量Ez :(2)(3)WVD 时-频分布满足边缘特性:沿着特定的时间对频率进行积分就可以得到瞬时功率,沿着特定的频率对时间进行积分就可以得到能量谱密度。
所以,时间与频率的联合函数满足:(3)频分布具有对称性,即因为:对是信号(5)WVD 时-频分布满足以下时移,频移特性:若,则(6)WVD 时-频分布满足边缘特性,所以,时-频分布函数的平均值是时间和频率函数。
其特性满足:若则:(4)(7)通过WVD 时-频分布可以计算信号的平均时间、中心频率、持续时间和带宽。
同时可以利用它们来确定其满足不确定性原理。
3 魏格纳-威利(WVD)时-频分布二次交叉项由于WVD 分布的时-频函数是双线性函数,所以当存在多分量线性调频信号时,WVD 时-频分布会存在严重的交叉干扰项。
威布尔概率分布及应用
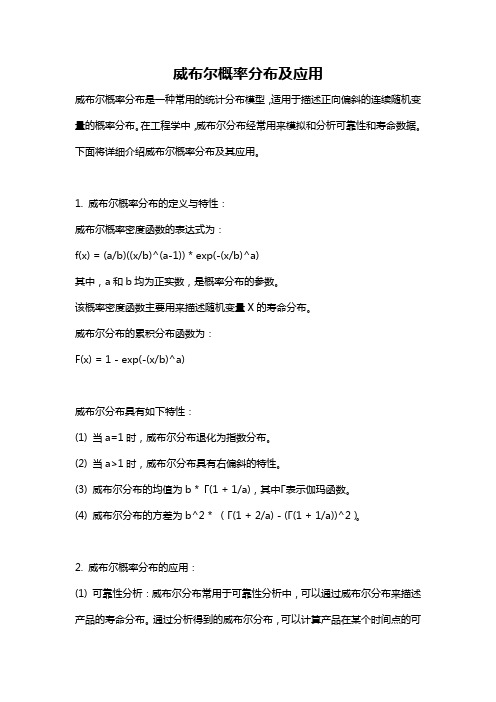
威布尔概率分布及应用威布尔概率分布是一种常用的统计分布模型,适用于描述正向偏斜的连续随机变量的概率分布。
在工程学中,威布尔分布经常用来模拟和分析可靠性和寿命数据。
下面将详细介绍威布尔概率分布及其应用。
1. 威布尔概率分布的定义与特性:威布尔概率密度函数的表达式为:f(x) = (a/b)((x/b)^(a-1)) * exp(-(x/b)^a)其中,a和b均为正实数,是概率分布的参数。
该概率密度函数主要用来描述随机变量X的寿命分布。
威布尔分布的累积分布函数为:F(x) = 1 - exp(-(x/b)^a)威布尔分布具有如下特性:(1) 当a=1时,威布尔分布退化为指数分布。
(2) 当a>1时,威布尔分布具有右偏斜的特性。
(3) 威布尔分布的均值为b * Γ(1 + 1/a),其中Γ表示伽玛函数。
(4) 威布尔分布的方差为b^2 * (Γ(1 + 2/a) - (Γ(1 + 1/a))^2)。
2. 威布尔概率分布的应用:(1) 可靠性分析:威布尔分布常用于可靠性分析中,可以通过威布尔分布来描述产品的寿命分布。
通过分析得到的威布尔分布,可以计算产品在某个时间点的可靠性,确定其在给定时间段内的失效概率,并进一步寻找改进措施,提高产品的可靠性。
(2) 寿命数据分析:威布尔分布也广泛应用于对某些机械设备、材料或系统的寿命数据进行建模与分析。
通过对实际寿命数据进行威布尔分布拟合,可以更准确地预测设备或系统在未来某个时间段内的失效概率,帮助制定相应的维修和更换计划。
(3) 临床试验:在医学和生物学中,临床试验数据经常具有右偏性,且描述的是某种事件或现象的寿命。
因此,威布尔分布在临床试验数据分析中的应用十分常见。
通过拟合试验数据得到的威布尔分布可以为研究人员提供反映疾病发展或治疗效果的信息,从而指导临床实践和决策。
(4) 金融风险管理:在金融领域,威布尔分布可以用来对风险事件的发生概率进行建模,如市场波动、信用违约等。
John Wiley全文电子期刊数据库
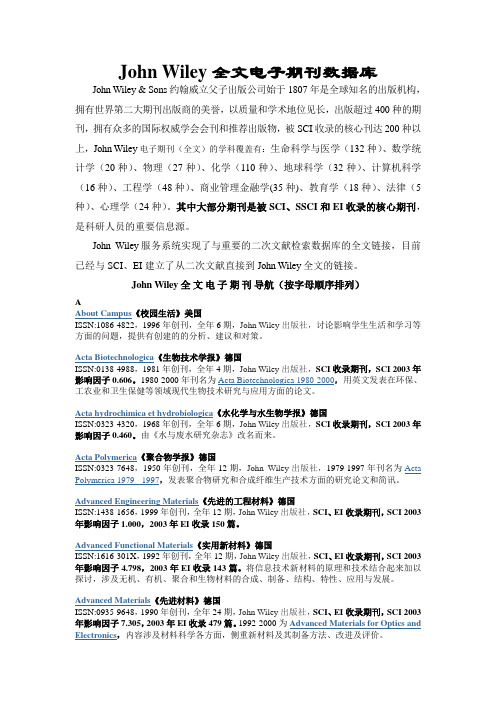
John Wiley全文电子期刊数据库John Wiley & Sons约翰威立父子出版公司始于1807年是全球知名的出版机构,拥有世界第二大期刊出版商的美誉,以质量和学术地位见长,出版超过400种的期刊,拥有众多的国际权威学会会刊和推荐出版物,被SCI收录的核心刊达200种以上,John Wiley电子期刊(全文)的学科覆盖有:生命科学与医学(132种)、数学统计学(20种)、物理(27种)、化学(110种)、地球科学(32种)、计算机科学(16种)、工程学(48种)、商业管理金融学(35种)、教育学(18种)、法律(5种)、心理学(24种)。
其中大部分期刊是被SCI、SSCI和EI收录的核心期刊,是科研人员的重要信息源。
John Wiley服务系统实现了与重要的二次文献检索数据库的全文链接,目前已经与SCI、EI建立了从二次文献直接到John Wiley全文的链接。
John Wiley全文电子期刊导航(按字母顺序排列)AAbout Campus《校园生活》美国ISSN:1086-4822,1996年创刊,全年6期,John Wiley出版社,讨论影响学生生活和学习等方面的问题,提供有创建的的分析、建议和对策。
Acta Biotechnologica《生物技术学报》德国ISSN:0138-4988,1981年创刊,全年4期,John Wiley出版社,SCI收录期刊,SCI 2003年影响因子0.606。
1980-2000年刊名为Acta Biotechnologica 1980-2000,用英文发表在环保、工农业和卫生保健等领域现代生物技术研究与应用方面的论文。
Acta hydrochimica et hydrobiologica《水化学与水生物学报》德国ISSN:0323-4320,1968年创刊,全年6期,John Wiley出版社,SCI收录期刊,SCI 2003年影响因子0.460。
John Wiley数据库的成立、发展历程以及涉及领域和访问方式
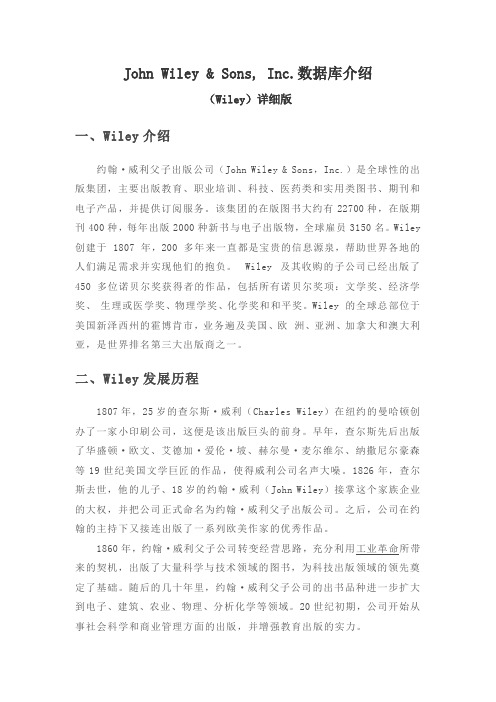
John Wiley & Sons, Inc.数据库介绍(Wiley)详细版一、Wiley介绍约翰·威利父子出版公司(John Wiley & Sons,Inc.)是全球性的出版集团,主要出版教育、职业培训、科技、医药类和实用类图书、期刊和电子产品,并提供订阅服务。
该集团的在版图书大约有22700种,在版期刊400种,每年出版2000种新书与电子出版物,全球雇员3150名。
Wiley 创建于 1807 年,200 多年来一直都是宝贵的信息源泉,帮助世界各地的人们满足需求并实现他们的抱负。
Wiley 及其收购的子公司已经出版了450 多位诺贝尔奖获得者的作品,包括所有诺贝尔奖项:文学奖、经济学奖、生理或医学奖、物理学奖、化学奖和和平奖。
Wiley 的全球总部位于美国新泽西州的霍博肯市,业务遍及美国、欧洲、亚洲、加拿大和澳大利亚,是世界排名第三大出版商之一。
二、Wiley发展历程1807年,25岁的查尔斯·威利(Charles Wiley)在纽约的曼哈顿创办了一家小印刷公司,这便是该出版巨头的前身。
早年,查尔斯先后出版了华盛顿·欧文、艾德加·爱伦·坡、赫尔曼·麦尔维尔、纳撒尼尔豪森等19世纪美国文学巨匠的作品,使得威利公司名声大噪。
1826年,查尔斯去世,他的儿子、18岁的约翰·威利(John Wiley)接掌这个家族企业的大权,并把公司正式命名为约翰·威利父子出版公司。
之后,公司在约翰的主持下又接连出版了一系列欧美作家的优秀作品。
1860年,约翰·威利父子公司转变经营思路,充分利用工业革命所带来的契机,出版了大量科学与技术领域的图书,为科技出版领域的领先奠定了基础。
随后的几十年里,约翰·威利父子公司的出书品种进一步扩大到电子、建筑、农业、物理、分析化学等领域。
20世纪初期,公司开始从事社会科学和商业管理方面的出版,并增强教育出版的实力。
wiley数据库介绍
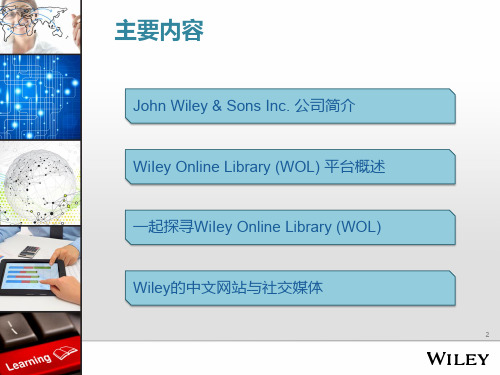
(部分期刊支持)
40
任一图书界面
图书内检索
所有范围 按出版名称 在这本图书内
41
参考书内检索 42
任一文章或章节下方
发现更多
查看更多相近研究 内容 查看更多该作者撰 写的文章
43
资源中心
培训/指南 研究人员 图书馆员
学协会 作者
开放获取
任何问题,您都可以与我们联系:china_marketing@
tobacco烟草environmental环境的34高级检索实例35高级检索实例36个人账户个人账户首页37个人账户激活停止邮件提醒已收藏的检索已收藏的出版物38个人账户邮件提醒管理邮件示例39期刊内检索所有范围按出版名称在本期刊内所有期次按引文部分期刊支持期刊界面40期刊内检索所有范围按出版名称在本期刊内所有期次在本期次内按引文部分期刊支持任一期次界面41图书内检索所有范围按出版名称在这本图书内任一图书界面42参考书内检索43发现更多查看更多相近研究内容查看更多该作者撰写的文章任一文章或章节下方44资源中心培训指南研究人员图书馆员学协会作者开放获取任何问题您都可以与我们联系
Wiley中国 微信
每天一篇 科研焦点
微信号:WileyChina
50
Wiley中国 博客
资讯热点 文章推荐 系列讲座 市场活动
/u/WileyChina
51
Wiley亚洲 博客
连接亚太 全球视野
Global Education
5
John Wiley & Sons Inc. 公司简介 WiIey Online Library (WOL) 平台概述 一起探寻WiIey Online Library (WOL) Wiley的中文网站与社交媒体
FAIRVALUEHIERARCHY-WileyHome:公允价值层级-威利家
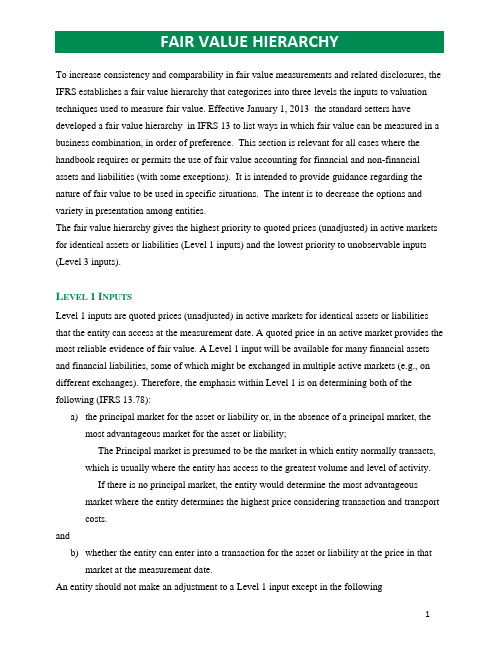
To increase consistency and comparability in fair value measurements and related disclosures, the IFRS establishes a fair value hierarchy that categorizes into three levels the inputs to valuation techniques used to measure fair value. Effective January 1, 2013 the standard setters have developed a fair value hierarchy in IFRS 13 to list ways in which fair value can be measured in a business combination, in order of preference. This section is relevant for all cases where the handbook requires or permits the use of fair value accounting for financial and non-financial assets and liabilities (with some exceptions). It is intended to provide guidance regarding the nature of fair value to be used in specific situations. The intent is to decrease the options and variety in presentation among entities.The fair value hierarchy gives the highest priority to quoted prices (unadjusted) in active markets for identical assets or liabilities (Level 1 inputs) and the lowest priority to unobservable inputs (Level 3 inputs).L EVEL 1I NPUTSLevel 1 inputs are quoted prices (unadjusted) in active markets for identical assets or liabilities that the entity can access at the measurement date. A quoted price in an active market provides the most reliable evidence of fair value. A Level 1 input will be available for many financial assets and financial liabilities, some of which might be exchanged in multiple active markets (e.g., on different exchanges). Therefore, the emphasis within Level 1 is on determining both of the following (IFRS 13.78):a)the principal market for the asset or liability or, in the absence of a principal market, themost advantageous market for the asset or liability;The Principal market is presumed to be the market in which entity normally transacts, which is usually where the entity has access to the greatest volume and level of activity.If there is no principal market, the entity would determine the most advantageous market where the entity determines the highest price considering transaction and transport costs.andb)whether the entity can enter into a transaction for the asset or liability at the price in thatmarket at the measurement date.An entity should not make an adjustment to a Level 1 input except in the followingcircumstances (IFRS 13.79):a)when an entity holds a large number of similar (but not identical) assets or liabilities (e.g.,debt securities) that are measured at fair value and a quoted price in an active market isavailable but not readily accessible for each of those assets or liabilities individually (i.e., given the large number of similar assets or liabilities held by the entity, it would bedifficult to obtain pricing information for each individual asset or liability at themeasurement date). In that case, as a practical expedient, an entity may measure fair value using an alternative pricing method that does not rely exclusively on quoted prices (e.g.,matrix pricing). However, the use of an alternative pricing method results in a fair valuemeasurement categorized within a lower level of the fair value hierarchy.b)when a quoted price in an active market does not represent fair value at the measurementdate. That might be the case if, for example, significant events (such as transactions in aprincipal-to-principal market, trades in a brokered market or announcements) take placeafter the close of a market but before the measurement date. An entity shall establish and consistently apply a policy for identifying those events that might affect fair valuemeasurements. However, if the quoted price is adjusted for new information, theadjustment results in a fair value measurement categorized within a lower level of the fair value hierarchy.c)when measuring the fair value of a liability or an entity’s own equity instrument using thequoted price for the identical item traded as an asset in an active market and that priceneeds to be adjusted for factors specific to the item or the asset (IFRS 13. 39). If noadjustment to the quoted price of the asset is required, the result is a fair valuemeasurement categorized within Level 1 of the fair value hierarchy. However, anyadjustment to the quoted price of the asset results in a fair value measurement categorized within a lower level of the fair value hierarchy.If an entity holds a position in a single asset or liability (including a position comprising a large number of identical assets or liabilities, such as a holding of financialinstruments) and the asset or liability is traded in an active market, the fair value of theasset or liability shall be measured within Level 1 as the product of the quoted price for the individual asset or liability and the quantity held by the entity (IFRS 13.80). That is thecase even if a market’s normal daily trading volume is not sufficient to absorb the quantityheld and placing orders to sell the position in a single transaction might affect the quoted price.For instance, if a company owns 16% of the shares of another entity do you take the fair value of each share regardless of the amount of shares owned or do you factor in avalue due to the large share ownership. This section now says that you value eachindividual share. You would not factor in any premium for the quantity held.L EVEL 2I NPUTSLevel 2 inputs are inputs other than quoted prices included within Level 1 that are observable for the asset or liability, either directly or indirectly (IFRS 13.81). A similar asset or liability is one that is reasonably comparable; for instance, one having similar patterns of cash flows that can be expected to respond to changes in economic conditions in the same way as those of the item being measured. Generally, when an asset or liability is sufficiently similar to an asset or liability being measured, adjustments for any difference are objectively determinable. For example, similar assets may be identical in all respects except for location. If the only difference between two assets is their location, the fair value will equal the observable price of an identical item in a different location plus costs to ship the item to the identical location as the asset being measured. If the asset or liability has a specified (contractual) term, a Level 2 input must be observable for substantially the full term of the asset or liability (IFRS 13.82). Level 2 inputs include the following:(a) quoted prices for similar assets or liabilities in active markets.(b) quoted prices for identical or similar assets or liabilities in markets that are not active.(c) inputs other than quoted prices that are observable for the asset or liability,for example:(i) interest rates and yield curves observable at commonly quoted intervals;(ii) implied volatilities; and(iii) credit spreads.(d) market-corroborated inputs.An adjustment to a Level 2 input that is significant to the entire measurement might result in a fair value measurement categorized within Level 3 of the fair value hierarchy if the adjustment uses significant unobservable inputs (IFRS 13.83).L EVEL 3I NPUTSLevel 3 inputs are unobservable inputs for the asset or liability. Unobservable inputs should be used to measure fair value to the extent that relevant observable inputs are unavailable, thereby allowing for situations in which there is little, if any, market activity for the asset or liability at the measurement date. However, the fair value measurement objective remains the same, e.g.,an exit price at the measurement date from the perspective of a market participant that holds the asset or owes the liability. Therefore, unobservable inputs should reflect the assumptions that market participants would use when pricing the asset or liability, including assumptions about risk (IFRS 13.87). Valuation techniques are to be consistent with the objective of estimating fair value, and should incorporate assumptions that marketplace participants would use whenever market-based information is available without undue cost or effort. If market-based information is not available without undue cost and effort, an entity may, as a practical expedient, use its own assumptions as inputs. However, for any valuation technique, market inputs should be maximized and use of internal estimates and assumptions minimized. For example, if an entity is aware of unique advantages or disadvantages that it possesses, such as favourable labour rates or superior processing or manufacturing technologies, it should adjust its entity-specific assumptions so that the inputs into the valuation process or model reflect those that marketplace participants would incorporate in an estimate of fair value (IFRS 13.89).Intangible assets often require the use of Level 3 criteria since one of the problems with intangible assets is that their unique nature in many cases precludes the use of information from other transactions. With the increasing importance of intangible assets, there has been a growing establishment of entities that specialize in measuring intangible assets, particularly brands. These valuation firms measure the worth of intangible assets by using variations of present value techniques, and multiples of variables such as royalty rates.Copyright © 2012 IFRS FoundationAll rights reserved. Reproduced by Wiley Canada with the permission of the IFRS Foundation ®.No permission granted to third parties to reproduce or distribute。
英文文献小短文(原文加汉语翻译)

A fern that hyperaccumulates arsenic(这是题目,百度一下就能找到原文好,原文还有表格,我没有翻译)A hardy, versatile, fast-growing plant helps to remove arsenic from contaminated soilsContamination of soils with arsenic,which is both toxic and carcinogenic, is widespread1. We have discovered that the fern Pteris vittata (brake fern) is extremely efficient in extracting arsenic from soils and translocating it into its above-ground biomass. This plant —which, to our knowledge, is the first known arsenic hyperaccumulator as well as the first fern found to function as a hyperaccumulator— has many attributes that recommend it for use in the remediation of arsenic-contaminated soils.We found brake fern growing on a site in Central Florida contaminated with chromated copper arsenate (Fig. 1a). We analysed the fronds of plants growing at the site for total arsenic by graphite furnace atomic absorption spectroscopy. Of 14 plant species studied, only brake fern contained large amounts of arsenic (As;3,280–4,980 p.p.m.). We collected additional samples of the plant and soil from the contaminated site (18.8–1,603 p.p.m. As) and from an uncontaminated site (0.47–7.56 p.p.m. As). Brake fern extracted arsenic efficiently from these soils into its fronds: plants growing in the contaminated site contained 1,442–7,526p.p.m. Arsenic and those from the uncontaminated site contained 11.8–64.0 p.p.m. These values are much higher than those typical for plants growing in normal soil, which contain less than 3.6 p.p.m. of arsenic3.As well as being tolerant of soils containing as much as 1,500 p.p.m. arsenic, brake fern can take up large amounts of arsenic into its fronds in a short time (Table 1). Arsenic concentration in fern fronds growing in soil spiked with 1,500 p.p.m. Arsenic increased from 29.4 to 15,861 p.p.m. in two weeks. Furthermore, in the same period, ferns growing in soil containing just 6 p.p.m. arsenic accumulated 755 p.p.m. Of arsenic in their fronds, a 126-fold enrichment. Arsenic concentrations in brake fern roots were less than 303 p.p.m., whereas those in the fronds reached 7,234 p.p.m.Addition of 100 p.p.m. Arsenic significantly stimulated fern growth, resulting in a 40% increase in biomass compared with the control (data not shown).After 20 weeks of growth, the plant was extracted using a solution of 1:1 methanol:water to speciate arsenic with high-performance liquid chromatography–inductively coupled plasma mass spectrometry. Almostall arsenic was present as relatively toxic inorganic forms, with little detectable organoarsenic species4. The concentration of As(III) was greater in the fronds (47–80%) than in the roots (8.3%), indicating that As(V) was converted to As(III) during translocation from roots to fronds.As well as removing arsenic from soils containing different concentrations of arsenic (Table 1), brake fern also removed arsenic from soils containing different arsenic species (Fig. 1c). Again, up to 93% of the arsenic was concentrated in the fronds. Although both FeAsO4 and AlAsO4 are relatively insoluble in soils1, brake fern hyperaccumulated arsenic derived from these compounds into its fronds (136–315 p.p.m.)at levels 3–6 times greater than soil arsenic.Brake fern is mesophytic and is widely cultivated and naturalized in many areas with a mild climate. In the United States, it grows in the southeast and in southern California5. The fern is versatile and hardy, and prefers sunny (unusual for a fern) and alkaline environments (where arsenic is more available). It has considerable biomass, and is fast growing, easy to propagate,and perennial.We believe this is the first report of significant arsenic hyperaccumulationby an unmanipulated plant. Brake fern has great potential to remediate arsenic-contaminated soils cheaply and could also aid studies of arsenic uptake, translocation, speciation, distribution and detoxification in plants. *Soil and Water Science Department, University ofFlorida, Gainesville, Florida 32611-0290, USAe-mail: lqma@†Cooperative Extension Service, University ofGeorgia, Terrell County, PO Box 271, Dawson,Georgia 31742, USA‡Department of Chemistry & SoutheastEnvironmental Research Center, FloridaInternational University, Miami, Florida 33199,1. Nriagu, J. O. (ed.) Arsenic in the Environment Part 1: Cyclingand Characterization (Wiley, New York, 1994).2. Brooks, R. R. (ed.) Plants that Hyperaccumulate Heavy Metals (Cambridge Univ. Press, 1998).3. Kabata-Pendias, A. & Pendias, H. in Trace Elements in Soils and Plants 203–209 (CRC, Boca Raton, 1991).4. Koch, I., Wang, L., Ollson, C. A., Cullen, W. R. & Reimer, K. J. Envir. Sci. Technol. 34, 22–26 (2000).5. Jones, D. L. Encyclopaedia of Ferns (Lothian, Melbourne, 1987).积累砷的蕨类植物耐寒,多功能,生长快速的植物,有助于从污染土壤去除砷有毒和致癌的土壤砷污染是非常广泛的。
威布尔分布假设检验方法
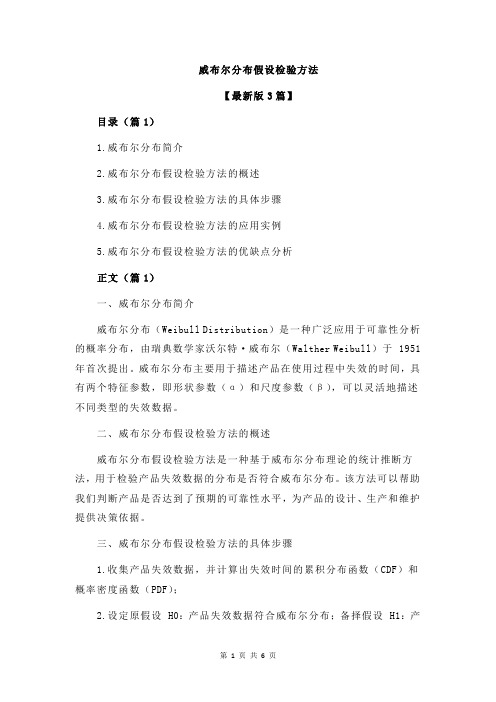
威布尔分布假设检验方法【最新版3篇】目录(篇1)1.威布尔分布简介2.威布尔分布假设检验方法的概述3.威布尔分布假设检验方法的具体步骤4.威布尔分布假设检验方法的应用实例5.威布尔分布假设检验方法的优缺点分析正文(篇1)一、威布尔分布简介威布尔分布(Weibull Distribution)是一种广泛应用于可靠性分析的概率分布,由瑞典数学家沃尔特·威布尔(Walther Weibull)于 1951 年首次提出。
威布尔分布主要用于描述产品在使用过程中失效的时间,具有两个特征参数,即形状参数(α)和尺度参数(β),可以灵活地描述不同类型的失效数据。
二、威布尔分布假设检验方法的概述威布尔分布假设检验方法是一种基于威布尔分布理论的统计推断方法,用于检验产品失效数据的分布是否符合威布尔分布。
该方法可以帮助我们判断产品是否达到了预期的可靠性水平,为产品的设计、生产和维护提供决策依据。
三、威布尔分布假设检验方法的具体步骤1.收集产品失效数据,并计算出失效时间的累积分布函数(CDF)和概率密度函数(PDF);2.设定原假设 H0:产品失效数据符合威布尔分布;备择假设 H1:产品失效数据不符合威布尔分布;3.选择适当的统计检验方法,如 Kolmogorov-Smirnov 检验、Shapiro-Wilk 检验等,对原假设进行检验;4.根据检验结果判断是否拒绝原假设,若拒绝原假设,则认为产品失效数据不符合威布尔分布,反之则认为符合。
四、威布尔分布假设检验方法的应用实例假设我们有一组电子产品的失效数据,我们需要判断这组数据是否符合威布尔分布。
首先,我们计算出失效数据的 CDF 和 PDF;然后,选择Kolmogorov-Smirnov 检验进行假设检验;最后,根据检验结果判断失效数据是否符合威布尔分布。
五、威布尔分布假设检验方法的优缺点分析优点:1.威布尔分布具有较强的理论基础,可以较好地描述失效数据的分布特征;2.威布尔分布假设检验方法具有较高的灵敏度和特异性,可以有效地检验产品失效数据的分布;3.该方法适用于不同类型的失效数据,具有较强的通用性。
维格纳-威利分布(WVD)平滑方法的研究
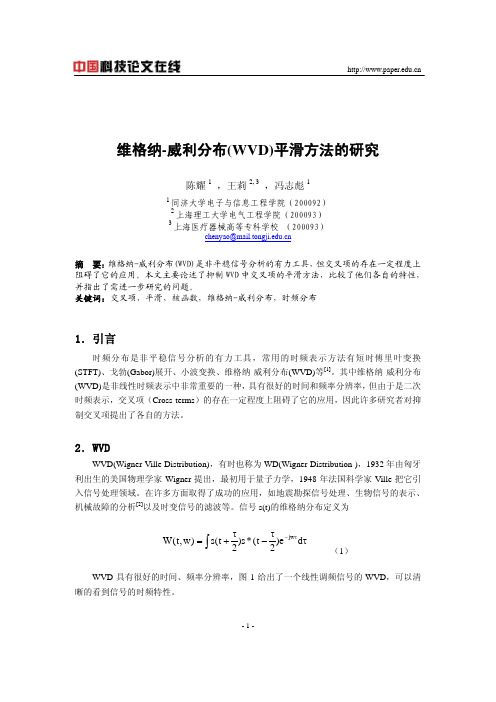
- 1 -维格纳-威利分布(WVD)平滑方法的研究陈耀1 ,王莉2, 3 ,冯志彪11同济大学电子与信息工程学院(200092)2上海理工大学电气工程学院(200093)3上海医疗器械高等专科学校 (200093) chenyao@摘 要:维格纳-威利分布(WVD)是非平稳信号分析的有力工具,但交叉项的存在一定程度上阻碍了它的应用。
本文主要论述了抑制WVD 中交叉项的平滑方法,比较了他们各自的特性,并指出了需进一步研究的问题。
关键词:交叉项,平滑,核函数,维格纳-威利分布,时频分布1.引言时频分布是非平稳信号分析的有力工具,常用的时频表示方法有短时傅里叶变换(STFT)、戈勃(Gabor)展开、小波变换、维格纳-威利分布(WVD)等[1]。
其中维格纳-威利分布(WVD)是非线性时频表示中非常重要的一种,具有很好的时间和频率分辨率,但由于是二次时频表示,交叉项(Cross-terms )的存在一定程度上阻碍了它的应用,因此许多研究者对抑制交叉项提出了各自的方法。
2.WVDWVD(Wigner-Ville Distribution),有时也称为WD(Wigner Distribution ),1932年由匈牙利出生的美国物理学家Wigner 提出,最初用于量子力学,1948年法国科学家Ville 把它引入信号处理领域。
在许多方面取得了成功的应用,如地震勘探信号处理、生物信号的表示、机械故障的分析[2]以及时变信号的滤波等。
信号s(t)的维格纳分布定义为ττ−τ+=τ−∫d e )2t (*s )2t (s )w ,t (W jw (1)WVD 具有很好的时间、频率分辨率,图1给出了一个线性调频信号的WVD ,可以清晰的看到信号的时频特性。
- 2 -(a) 原始信号 (b) WVD图1 线性调频信号由于是WVD 属于二次时频表示,对有些信号不可避免会产生交叉项,交叉项的存在对它的应用造成了不小的障碍,图2给出了两个线性调频信号的叠加WVD ,可以明显看到两个信号之间的交叉项。
Discrete Weibull分布类说明书
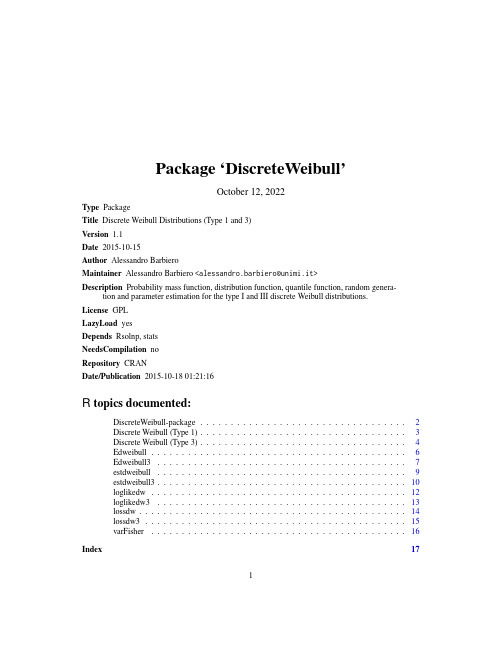
Package‘DiscreteWeibull’October12,2022Type PackageTitle Discrete Weibull Distributions(Type1and3)Version1.1Date2015-10-15Author Alessandro BarbieroMaintainer Alessandro Barbiero<****************************>Description Probability mass function,distribution function,quantile function,random genera-tion and parameter estimation for the type I and III discrete Weibull distributions.License GPLLazyLoad yesDepends Rsolnp,statsNeedsCompilation noRepository CRANDate/Publication2015-10-1801:21:16R topics documented:DiscreteWeibull-package (2)Discrete Weibull(Type1) (3)Discrete Weibull(Type3) (4)Edweibull (6)Edweibull3 (7)estdweibull (9)estdweibull3 (10)loglikedw (12)loglikedw3 (13)lossdw (14)lossdw3 (15)varFisher (16)Index1712DiscreteWeibull-packageDiscreteWeibull-packageDiscrete Weibull Distributions(Type1and3)DescriptionProbability mass function,distribution function,quantile function,random generation and param-eter estimation for the type I and III discrete Weibull distributions.In the present version,some modifications have been made on the functions in Edweibull for the computation of the moments of the type I discrete Weibull distribution.DetailsPackage:DiscreteWeibullType:PackageVersion: 1.1Date:2015-10-17License:GPLLazyLoad:yesDepends:RsolnpAuthor(s)Alessandro Barbiero<****************************>ReferencesT.Nakagawa and S.Osaki(1975)The discrete Weibull distribution,IEEE Transactions on Relia-bility,24(5),pp.300-301D.N.P.Murthy,M.Xie,R Jiang(2004)Weibull models,John Wiley&Sons:Hoboken,New JerseyW.J.Padgett and J.D.Spurrier(1985)Discrete failure models,IEEE Transactions on Reliability, 34(3),pp.253-256H.Rinne(2008)The Weibull Distribution:A Handbook.CRC Press:Boca Raton,FloridaM.S.A.Khan,A.Khalique,and A.M.Abouammoh(1989)On estimating parameters in a discrete Weibull distribution,IEEE Transactions on Reliability,38(3),pp.348-350See Alsoddweibull,estdweibull,Edweibull,ddweibull3,estdweibull3,Edweibull3Discrete Weibull(Type1)The type1discrete Weibull distributionDescriptionProbability mass function,distribution function,quantile function and random generation for the discrete Weibull distribution with parameters q andβUsageddweibull(x,q,beta,zero=FALSE)pdweibull(x,q,beta,zero=FALSE)qdweibull(p,q,beta,zero=FALSE)rdweibull(n,q,beta,zero=FALSE)Argumentsx vector of quantilesp vector of probabilitiesqfirst parameterbeta second parameterzero TRUE,if the support contains0;FALSE otherwisen sample sizeDetailsThe discrete Weibull distribution has probability mass function given by P(X=x;q,β)=q(x−1)β−q xβ,x=1,2,3,...,if zero=FALSE;or P(X=x;q,β)=q xβ−q(x+1)β,x=0,1,2, (i)zero=TRUE.The cumulative distribution function is F(x;q,β)=1−q xβif zero=FALSE;F(x;q,β)= 1−q(x+1)βotherwiseValueddweibull gives the probability function,pdweibull gives the distribution function,qdweibull gives the quantile function,and rdweibull generates random values.Author(s)Alessandro BarbieroExamples#Ex.1x<-1:10q<-0.6beta<-0.8ddweibull(x,q,beta)t<-qdweibull(0.99,q,beta)tpdweibull(t,q,beta)#x<-0:10ddweibull(x,q,beta,zero=TRUE)t<-qdweibull(0.99,q,beta,zero=TRUE)tpdweibull(t,q,beta,zero=TRUE)#Ex.2q<-0.4beta<-0.7n<-100x<-rdweibull(n,q,beta)tabulate(x)/sum(tabulate(x))y<-1:round(max(x))#compare withddweibull(y,q,beta)Discrete Weibull(Type3)The type3discrete Weibull distributionDescriptionProbability mass function,distribution function,quantile function,random generation,and hazard function for the type3discrete Weibull distribution with parameters c andβUsageddweibull3(x,c,beta)pdweibull3(x,c,beta)qdweibull3(p,c,beta)rdweibull3(n,c,beta)hdweibull3(x,c,beta)Argumentsx vector of values/quantilesp vector of probabilitiescfirst parameterbeta second parameter n sample sizeDetailsThe type 3discrete Weibull distribution is characterized by the following cumulative distribution function:F (x ;c,β)=1−exp(−c x +1j =0j β),for x =0,1,2,...,with c >0and β>=−1.Valueddweibull3gives the probability function,pdweibull3gives the distribution function,qdweibull3gives the quantile function,hdweibull3gives the hazard function,and rdweibull generates ran-dom values.Author(s)Alessandro Barbiero Examples#ddweibull3x <-0:10c <-0.3beta <-0.75p <-ddweibull3(x,c,beta)pplot(x,p,type="b",ylab=expression(P(X)==x))#pdweibull3x <-0:10c <-0.5beta <-0.5p <-pdweibull3(x,c,beta)pcumsum(ddweibull3(x,c,beta))plot(x,p,type="s",ylab=expression(P(X<=x)))#qdweibull3p <-c(1:9)/10pc <-0.1beta <-0.5qdweibull3(p,c,beta)pdweibull3(10,c,beta)pdweibull3(9,c,beta)#rdweibull3n <-20c <-0.25beta <--0.25x <-rdweibull3(n,c,beta)xbeta <-0x <-rdweibull3(n,c,beta)xbeta<-0.25x<-rdweibull3(n,c,beta)xn<-1000x<-rdweibull3(n,c,beta)obs<-c(sum(x==0),tabulate(x))obs<-obs/sum(obs)theo<-ddweibull3(min(x):max(x),c,beta)barplot(rbind(obs,theo),beside=TRUE,names.arg=min(x):max(x),ylab="relative frequency/probability",col=1:2)legend(24,0.1,c("observed","theoretical"),pch=15,col=1:2)#hdweibull3x<-0:15c<-0.5hn<-hdweibull3(x,c,beta=-0.5)h0<-hdweibull3(x,c,beta=0)hp<-hdweibull3(x,c,beta=0.5)plot(x,hn,type="b",ylim=c(0,1),ylab="hazard rate")points(x,h0,type="b",col=2)points(x,hp,type="b",col=3)legend(11,0.5,c("beta<0","beta=0","beta>0"),col=1:3,pch=21)Edweibull Expected valuesDescriptionFirst and second order moments,variance and expected value of the reciprocal for the type1discrete Weibull distributionUsageEdweibull(q,beta,eps=1e-04,nmax=1000,zero=FALSE)E2dweibull(q,beta,eps=1e-04,nmax=1000,zero=FALSE)Vdweibull(q,beta,eps=1e-04,nmax=1000,zero=FALSE)ERdweibull(q,beta,eps=1e-04,nmax=1000)Argumentsqfirst parameterbeta second parametereps error threshold for the numerical computation of the expected valuenmax maximum value considered for the numerical approximate computation of the expected value;zero TRUE,if the support contains0;FALSE otherwiseDetailsThe expected value is numerically computed considering a truncated support:integer values smaller than or equal to2F−1(1−eps;q,β)are considered,where F−1is the inverse of the cumulative distribution function(implemented by the function qdweibull).However,if such value is greater than nmax,the expected value is computed recalling the formula of the expected value of the corre-sponding continuous Weibull distribution(see the reference),adding0.5.Similar arguments apply to the other moments.Valuethe(approximate)expected values of the discrete Weibull distribution:Edweibull gives thefirst order moment,E2dweibull the second order moment,Vdweibull the variance,ERdweibull the expected value of the reciprocal(only if zero is FALSE)Author(s)Alessandro BarbieroReferencesM.S.A.Khan,A.Khalique,and A.M.Abouammoh(1989)On estimating parameters in a discrete Weibull distribution,IEEE Transactions on Reliability,38(3),pp.348-350Examplesq<-0.75beta<-1.25Edweibull(q,beta)E2dweibull(q,beta)Vdweibull(q,beta)ERdweibull(q,beta)#if beta=0.75...beta<-0.75Edweibull(q,beta)Edweibull(q,beta,nmax=100)#here above,the approximation through the continuous model intervenes#if beta=1...beta<-1Edweibull(q,beta)#which equals...1/(1-q)Edweibull3Expected valuesDescriptionFirst and second order moments for the type3discrete Weibull distributionUsageEdweibull3(c,beta,eps=1e-04)E2dweibull3(c,beta,eps=1e-04)Argumentscfirst parameterbeta second parametereps error threshold for the numerical computation of the expected valueDetailsThe expected values are numerically computed considering a truncated support:integer values smaller than or equal to2F−1(1−eps;c,β)),where F−1is the inverse of the cumulative distribu-tion function(implemented by the function qdweibull3)Valuethe(approximate)expected values of the discrete Weibull distribution:Edweibull3gives thefirst order moment,E2dweibull3the second order momentAuthor(s)Alessandro BarbieroExamplesc<-0.4beta<-0.25Edweibull3(c,beta)c<-0.4beta<--0.75Edweibull3(c,beta)#may require too much timeEdweibull3(c,beta,eps=0.001)#try with a smaller eps->worse approximationc<-rep(0.1,11)beta<-(0:10)/10Edweibull3(c,beta)c<-rep(0.5,11)beta<-(-5:5)/10Edweibull3(c,beta)#E2dweibull3c<-0.4beta<-0.25E2dweibull3(c,beta)c<-rep(0.1,11)beta<-(0:10)/10Edweibull3(c,beta)c<-rep(0.8,11)beta<-(-5:5)/11E2dweibull3(c,beta)estdweibull Estimation of parametersDescriptionEstimation of the parameters of the type1discrete Weibull distributionUsageestdweibull(x,method="ML",zero=FALSE,eps=1e-04,nmax=1000)Argumentsx the vector of sample valuesmethod"ML"for the maximum likelihood method;"M"for the method of moments;"P"for the method of proportionszero TRUE,if the support contains0;FALSE otherwiseeps error threshold for the computation of the moments of the distributionnmax maximum value considered for the numerical computation of the expected valueValuethe vector of the estimates of q andβAuthor(s)Alessandro BarbieroSee AlsoddweibullExamples#Ex1n<-10q<-0.5beta<-0.8x<-rdweibull(n,q,beta)estdweibull(x,"ML")#maximum likelihood method#it may return some harmless warnings#that depend on the optimization function used in the maximization routineestdweibull(x,"M")#method of momentsestdweibull(x,"P")#method of proportion#the estimates provided by the three methods may be quite different#from the true values...and to each other#change the sample sizen<-50q<-0.5beta<-0.8x<-rdweibull(n,q,beta)estdweibull(x,"ML")#maximum likelihood methodestdweibull(x,"M")#method of momentsestdweibull(x,"P")#method of proportion#the estimates should be(on average)closer to the true values#...and to each other#When the estimation methods fail...#Ex2#only1s and2sx<-c(1,1,1,1,1,1,2,2,2,2)estdweibull(x,"ML")#fails!estdweibull(x,"M")#fails!estdweibull(x,"P")#fails!#Ex3#no1sx<-c(2,2,3,4,5,5,5,6,6,8,10)estdweibull(x,"ML")#worksestdweibull(x,"M")#worksestdweibull(x,"P")#fails!estdweibull3Estimation of parametersDescriptionEstimation of the parameters of the type3discrete Weibull distributionUsageestdweibull3(x,method="P",eps=1e-04)Argumentsx the vector of sample valuesmethod"ML"for the maximum likelihood method;"M"for the method of moments;"P"for the method of proportionseps error threshold for the computation of the moments of the distributionValuethe vector of the estimates of c andβAuthor(s)Alessandro Barbieroestdweibull311 See Alsoddweibull3Examples#Ex1x<-c(0,0,0,0,0,1,1,1,1,1,1,1,2,2,2,2,3,3,4,6)estdweibull3(x,"P")estdweibull3(x,"ML")estdweibull3(x,"M")#Ex2n<-20c<-1/3beta<-2/3x<-rdweibull3(n,c,beta)estdweibull3(x,"P")par<-estdweibull3(x,"ML")par-loglikedw3(par,x)par<-estdweibull3(x,"M")parlossdw3(par,x)n<-50x<-rdweibull3(n,c,beta)estdweibull3(x,"P")estdweibull3(x,"ML")estdweibull3(x,"M")n<-100x<-rdweibull3(n,c,beta)estdweibull3(x,"P")estdweibull3(x,"ML")estdweibull3(x,"M")#Ex3:a piece of simulation studynSim<-50n<-50c<-0.2beta<-0.7par<-matrix(0,nSim,2)for(i in1:nSim){x<-rdweibull3(n,c,beta)par[i,]<-estdweibull3(x,"ML")}op<-par(mfrow=c(1,2))boxplot(par[,1],xlab=expression(hat(c)[ML]))abline(h=c)boxplot(par[,2],xlab=expression(hat(beta)[ML]))abline(h=beta)op<-par()loglikedw Loglikelihood functionDescriptionLoglikelihood function(changed in sign)for the type1discrete Weibull distributionUsageloglikedw(par,x,zero=FALSE)Argumentspar the vector of parameters,q andβx the vector of sample valueszero TRUE,if the support contains0;FALSE otherwiseValuethe value of the loglikelihood function(changed in sign)for the observed sample x under the pa-rameters parAuthor(s)Alessandro BarbieroSee AlsoestdweibullExamplesx<-c(1,1,1,2,2,2,2,2,2,3,4,4,5,6,8)-loglikedw(c(0.8,1),x)#loglikelihood function for q=0.8and beta=1-loglikedw(c(0.4,2),x)#loglikelihood function for q=0.4and beta=2par<-estdweibull(x,"ML")#parameter estimates derived by the ML methodpar-loglikedw(par,x)#the maximum value of the loglikelihood functionloglikedw3Loglikelihood functionDescriptionLoglikelihood function(changed in sign)for the type3discrete Weibull distributionUsageloglikedw3(par,x)Argumentspar the vector of parameters,c andβx the vector of sample valuesValuethe value of the loglikelihood function(changed in sign)for the observed sample x under the pa-rameters parAuthor(s)Alessandro BarbieroSee Alsoestdweibull3Examplesn<-20c<-1/3beta<-2/3x<-rdweibull3(n,c,beta)par<-estdweibull3(x,"ML")par-loglikedw3(par,x)14lossdw lossdw Loss functionDescriptionLoss function for the method of moments(type1discrete Weibull)Usagelossdw(par,x,zero=FALSE,eps=1e-04,nmax=1000)Argumentspar vector of parameters q andβx the vector of sample valueszero TRUE,if the support contains0;FALSE otherwiseeps error threshold for the numerical computation of the expected valuenmax maximum value considered for the numerical computation of the expected valueDetailsThe loss function is given by L(x;q,β)=[m1−E(X;q,β)]2+[m2−E(X2;q,β)]2,where E(·) denotes the expected value,m1and m2are thefirst and second order sample moments respectively. Valuethe value of the quadratic loss functionAuthor(s)Alessandro BarbieroSee AlsoEdweibullExamplesx<-c(1,1,1,1,1,2,2,2,3,4)lossdw(c(0.5,1),x)par<-estdweibull(x,"M")#parameter estimates derived by the method of momentsparlossdw(par,x)#the loss is zero using these estimateslossdw315 lossdw3Loss functionDescriptionLoss function for the method of moments(type3discrete Weibull)Usagelossdw3(par,x,eps=1e-04)Argumentspar vector of parameters q andβx the vector of sample valueseps error threshold for the numerical computation of the expected valueDetailsThe loss function is given by L(x;c,β)=[m1−E(X;c,β)]2+[m2−E(X2;c,β)]2,where E(·) denotes the expected value,m1and m2are thefirst and second order sample moments respectively. Valuethe value of the quadratic loss functionAuthor(s)Alessandro BarbieroSee AlsoEdweibull3Examplesn<-25c<-1/3beta<-2/3x<-rdweibull3(n,c,beta)par<-estdweibull3(x,"M")parlossdw3(par,x)16varFisher varFisher Observed Fisher information matrixDescriptionObserved Fisher information matrix on a sample from the type1discrete Weibull distribution UsagevarFisher(x,zero=FALSE)Argumentsx a vector of sample valueszero TRUE,if the support contains0;FALSE otherwiseValuea list of two matrices:the observed Fisher information matrix,and its inverse,which containsasymptotic variances and covariances of the maximum likelihood estimators of q andβAuthor(s)Alessandro BarbieroSee AlsoestdweibullExamplesx<-rdweibull(100,2/3,3/2)estdweibull(x,"ML")IF<-varFisher(x)[[2]]diag(IF)diag(IF)/length(x)#asymptotic variances of the ML estimators directly estimated from the sampleIndex∗distributionDiscrete Weibull(Type1),3Discrete Weibull(Type3),4Edweibull,6Edweibull3,7estdweibull,9estdweibull3,10loglikedw,12loglikedw3,13lossdw,14lossdw3,15varFisher,16∗htestestdweibull,9estdweibull3,10loglikedw,12loglikedw3,13varFisher,16∗packageDiscreteWeibull-package,2 ddweibull,2,9ddweibull(Discrete Weibull(Type1)),3 ddweibull3,2,11ddweibull3(Discrete Weibull(Type3)), 4Discrete Weibull(Type1),3Discrete Weibull(Type3),4 DiscreteWeibull(DiscreteWeibull-package),2 DiscreteWeibull-package,2E2dweibull(Edweibull),6E2dweibull3(Edweibull3),7Edweibull,2,6,14Edweibull3,2,7,15ERdweibull(Edweibull),6estdweibull,2,9,12,16estdweibull3,2,10,13hdweibull3(Discrete Weibull(Type3)),4loglikedw,12loglikedw3,13lossdw,14lossdw3,15pdweibull(Discrete Weibull(Type1)),3pdweibull3(Discrete Weibull(Type3)),4qdweibull,7qdweibull(Discrete Weibull(Type1)),3qdweibull3,8qdweibull3(Discrete Weibull(Type3)),4rdweibull(Discrete Weibull(Type1)),3rdweibull3(Discrete Weibull(Type3)),4varFisher,16Vdweibull(Edweibull),617。
分布模型

其他分布函数
均匀分布:
u (a, b)
1 , a xb ba fu ( x) 0, x a, x b
fu ( x)
a
b x
瑞利分布:
R ( )
fR (x)
x
2
x
2 2
fR (x)
e
2
3 贝塔分布:
( , )
f (x) x
1
正态分布的失效密度函数为:
1 t 2
2
f (t )
1 2
e
2 失效分布函数为
F (t )
t
1 2
e
1 x 2
dx
0
3 失效率函数
(t )
f (t ) R (t )
4 正态分布的可靠度函数
R (t )
通过李老师深入细致的讲解我对现代设计理论及方法有了较深刻的认识, 通过系统设计优化方法、优化设计方法、可靠性设计、 反求工程设计、绿色 制造等知识的学习。培养了自己运用现代先进的设计理论和方法解决工程问 题的能力,自己受益匪浅。
谢 谢
一威布尔分布二指数分布三正态分布四对数正态分布五均匀分布六贝塔分布七瑞利分布八伽马分布一威布尔分布威布尔分布是近年来在可靠性分析中使用最为广泛的模型它能全面地描述浴盆失效概率曲线的各个阶段
可靠性设计概率分布模型
姓 学
名: 号:
可靠性分析中经常采用的 概率分布类型有
一、威布尔分布 二、指数分布 三、正态分布 四、对数正态分布 五、均匀分布 六、贝塔分布 七、瑞利分布 八、伽马分布
1 失效分布函数为:
t r m
Wiley InterScience数据库使用指南

Wiley InterScience 数据库使用指南一、数据库简介John Wiley & Sons Inc.是一个有近200年历史的国际知名专业出版机构,在化学、生命科学和医学以及工程技术等领域学术文献出版方面颇具权威性。
Wiley InterScience 是John Wiely & Sons 创建的综合性网络出版及服务平台,收录了360多种科学、工程技术、医疗领域及相关专业期刊、30多种大型专业参考书、13种实验室手册的全文和500多个题目的Wiley 学术图书的全文,内容涉及商业、金融和管理、化学、计算机科学、地球科学、教育学、工程学、法律、生命科学与医学、数学统计学、物理等十四个学科。
请注意:我局用户使用Wiley InterScience 数据库是按下载(包括打开、浏览、打印、下载)的全文单篇计费。
因此如果您发现对您审查有用的文献,请及时打印或下载到本地硬盘,尽量避免重复下载!检索学科分类浏览基本检索高级检索CrossRef 检索 二、浏览方法在Wiley InterScience 数据库的主界面上,点击“Browse by subject area ”,可以按14个学科主题来浏览相关的文献,每个主题又细分了下级类目,同时列出该主题相关的文献类型,如:Journals 、Online books 、Reference works 等。
您也可以选择点击“Browse by product type ”,按Jornals 、Online books 、Reference works 、Datebases 、Current Protocols 、Collections 等类型来浏览。
1.期刊 Wiley InterScience 上的期刊内容根据对应的刊号,按期编排,呈现给读者。
最近的一期往往出现在最前面。
保存刊名到我的文档建立邮件通知文点击文献题录下的“Abstract ”,可以查看文献的摘要。
威布尔分析方法
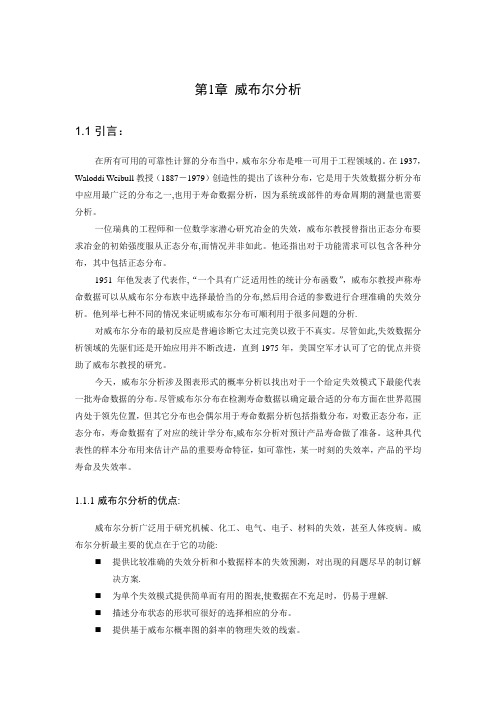
第1章威布尔分析1.1 引言:在所有可用的可靠性计算的分布当中,威布尔分布是唯一可用于工程领域的。
在1937,Waloddi Weibull教授(1887-1979)创造性的提出了该种分布,它是用于失效数据分析分布中应用最广泛的分布之一,也用于寿命数据分析,因为系统或部件的寿命周期的测量也需要分析。
一位瑞典的工程师和一位数学家潜心研究冶金的失效,威布尔教授曾指出正态分布要求冶金的初始强度服从正态分布,而情况并非如此。
他还指出对于功能需求可以包含各种分布,其中包括正态分布。
1951年他发表了代表作,“一个具有广泛适用性的统计分布函数”,威布尔教授声称寿命数据可以从威布尔分布族中选择最恰当的分布,然后用合适的参数进行合理准确的失效分析。
他列举七种不同的情况来证明威布尔分布可顺利用于很多问题的分析.对威布尔分布的最初反应是普遍诊断它太过完美以致于不真实。
尽管如此,失效数据分析领域的先驱们还是开始应用并不断改进,直到1975年,美国空军才认可了它的优点并资助了威布尔教授的研究。
今天,威布尔分析涉及图表形式的概率分析以找出对于一个给定失效模式下最能代表一批寿命数据的分布。
尽管威布尔分布在检测寿命数据以确定最合适的分布方面在世界范围内处于领先位置,但其它分布也会偶尔用于寿命数据分析包括指数分布,对数正态分布,正态分布,寿命数据有了对应的统计学分布,威布尔分析对预计产品寿命做了准备。
这种具代表性的样本分布用来估计产品的重要寿命特征,如可靠性,某一时刻的失效率,产品的平均寿命及失效率。
1.1.1威布尔分析的优点:威布尔分析广泛用于研究机械、化工、电气、电子、材料的失效,甚至人体疫病。
威布尔分析最主要的优点在于它的功能:⏹提供比较准确的失效分析和小数据样本的失效预测,对出现的问题尽早的制订解决方案.⏹为单个失效模式提供简单而有用的图表,使数据在不充足时,仍易于理解.⏹描述分布状态的形状可很好的选择相应的分布。
⏹提供基于威布尔概率图的斜率的物理失效的线索。
四大分布简述-心理统计
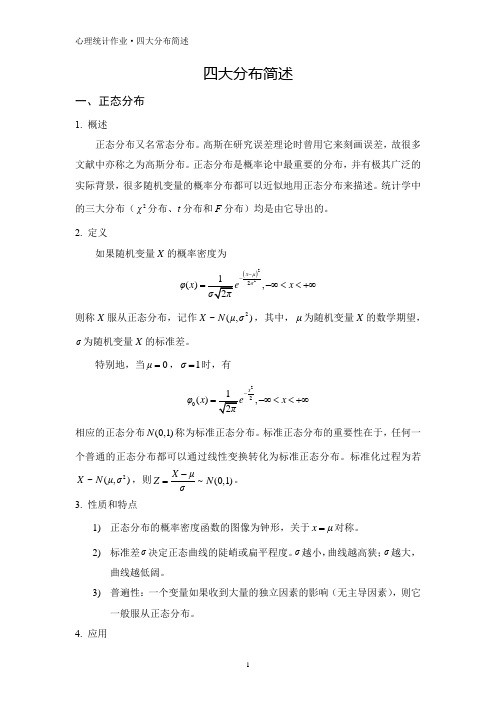
四大分布简述一、正态分布1. 概述正态分布又名常态分布。
高斯在研究误差理论时曾用它来刻画误差,故很多文献中亦称之为高斯分布。
正态分布是概率论中最重要的分布,并有极其广泛的实际背景,很多随机变量的概率分布都可以近似地用正态分布来描述。
统计学中的三大分布(2χ分布、t分布和F分布)均是由它导出的。
2. 定义如果随机变量X的概率密度为()222(),xμσφx x--=-∞<<+∞则称X服从正态分布,记作2~(,)X Nμσ,其中,μ为随机变量X的数学期望,σ为随机变量X的标准差。
特别地,当0μ=,1σ=时,有22(),xφx x-=-∞<<+∞相应的正态分布(0,1)N称为标准正态分布。
标准正态分布的重要性在于,任何一个普通的正态分布都可以通过线性变换转化为标准正态分布。
标准化过程为若2~(,)X Nμσ,则(0,1)XμZ~Nσ-=。
3. 性质和特点1)正态分布的概率密度函数的图像为钟形,关于xμ=对称。
2)标准差σ决定正态曲线的陡峭或扁平程度。
σ越小,曲线越高狭;σ越大,曲线越低阔。
3)普遍性:一个变量如果收到大量的独立因素的影响(无主导因素),则它一般服从正态分布。
4. 应用1) 估计频数分布。
2) 制定参考值范围。
3) 质量控制:3σ准则。
4) 二项分布、t 分布等的正态近似计算。
5) 正态分布是许多统计方法的理论基础。
检验、方差分析、相关和回归分析等多种统计方法均要求分析的指标服从正态分布。
二、2χ分布1. 概述2χ分布是由海尔默特(Hermert )和皮尔逊(Pearson )分别于1875年和1900年推导出来的。
2. 定义设随机变量12,,,n X X X 相互独立,且()1,2,,=i X i n 服从标准正态分布(0,1)N ,则它们的平方和21=∑n i i X 服从自由度为n 的2χ分布,记作2()χn 。
3. 性质和特点1) 2χ分布的密度函数在第一象限内呈正偏态(右偏态)。