2012年国际赛A题论文
2012全国竞赛论文评分建议2012全国赛A题:葡萄酒的评价

2012全国赛A题:葡萄酒的评价评阅要点解读与评分建议[广东赛区提供]总体评分分布:摘要10分●写作5分●总体评价5分●问题1 35分●问题2 15分●问题3 15分●问题4 10分●数据处理(缺失、异常)5分问题1:(35分)同一酒样评酒员之间差距小20分=原理5分+模型和方法 10分+结果与说明5分不同酒样之间区分度明显15分=原理5分+模型和方法 5分+结果与说明5分问题2:(15分)分级原则5分模型5分算法和结果5分问题3:(15分)分析关系的原理、结论评价5分模型和方法5分理化指标的分类和筛选5分问题4:(10分)建立模型5分结论及详细说明5分关于2012-A题评分要点的具体分析(至少17处)看到了网上几乎所有的参考答案,也听了一些老师的建议,现在结合评分要点来谈下自己的分析:在问题一中,答案是固定的,也就是显著和可信的问题,不管什么方法,但关键的还是说明假设检验的原理的依据,为什么要用这种方法,合适吗?所以,评分要点有以下几处:(1)数据的处理,包括缺失和异常数据,说明你处理的方法。
(2)检验模型的假设进行检验,例如样本的正态分布检验等(3)在前面的基础上,有些统计的方法不太适用,这时候要介绍你的方法原理和为什么要这么做。
(4)显著性分析和可信性分析。
这里面可能不同的方法结果不太一致,这时候要利用多个检验方法进行综合分析和考虑,给出较好的结果。
(5)由于评酒员都是感官评价的结果,前面是分两组样本分析的,这里可以针对每组评酒员的差异进行分析,即给出每个评酒员的品酒差异进行分析。
所以,第一问应该越详细越好。
这一问解决的感官评价的问题。
在问题二中,根据酿酒葡萄的理化指标和葡萄酒的质量对这些酿酒葡萄进行分级。
这里的方法较多,例如模糊综合评判,神经网络,聚类等。
每种方法都有自己的局限性,而且分级的个数也会有很大差异,这时候就要对自身的模型进行分析和判断。
关键点(1)无论哪种方法,酿酒葡萄的理化指标和葡萄酒的质量必须要说明清楚(2)模型本身的优缺点和适用范围进行分析明确(3)分级结果中应该对不同的酿酒葡萄进行明确的区分,例如哪种葡萄能造哪种级别的酒可以分析出来。
2012年全国大学生数学建模竞赛A题国一
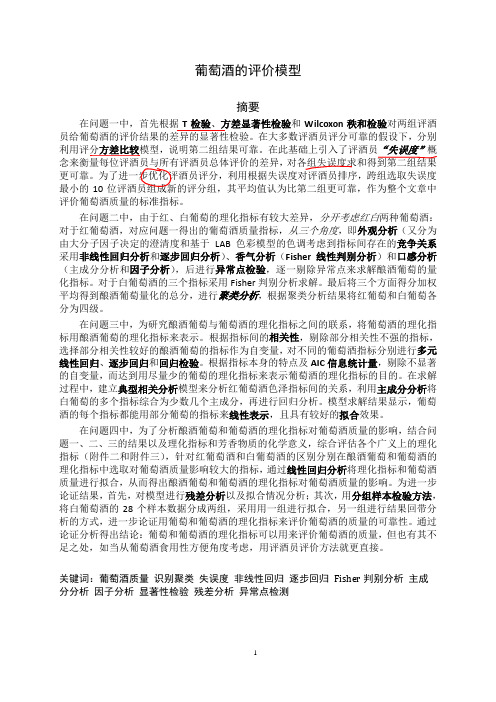
摘要
在问题一中,首先根据 T 检验、方差显著性检验和 Wilcoxon 秩和检验对两组评酒 员给葡萄酒的评价结果的差异的显著性检验。在大多数评酒员评分可靠的假设下,分别 利用评分方差比较模型,说明第二组结果可靠。在此基础上引入了评酒员“失误度”概 念来衡量每位评酒员与所有评酒员总体评价的差异, 对各组失误度求和得到第二组结果 更可靠。为了进一步优化评酒员评分,利用根据失误度对评酒员排序,跨组选取失误度 最小的 10 位评酒员组成新的评分组,其平均值认为比第二组更可靠,作为整个文章中 评价葡萄酒质量的标准指标。 在问题二中,由于红、白葡萄的理化指标有较大差异,分开考虑红白两种葡萄酒: 对于红葡萄酒,对应问题一得出的葡萄酒质量指标,从三个角度,即外观分析(又分为 由大分子因子决定的澄清度和基于 LAB 色彩模型的色调考虑到指标间存在的竞争关系 采用非线性回归分析和逐步回归分析) 、香气分析(Fisher 线性判别分析)和口感分析 (主成分分析和因子分析) ,后进行异常点检验,逐一剔除异常点来求解酿酒葡萄的量 化指标。对于白葡萄酒的三个指标采用 Fisher 判别分析求解。最后将三个方面得分加权 平均得到酿酒葡萄量化的总分,进行聚类分析,根据聚类分析结果将红葡萄和白葡萄各 分为四级。 在问题三中,为研究酿酒葡萄与葡萄酒的理化指标之间的联系,将葡萄酒的理化指 标用酿酒葡萄的理化指标来表示。根据指标间的相关性,剔除部分相关性不强的指标, 选择部分相关性较好的酿酒葡萄的指标作为自变量, 对不同的葡萄酒指标分别进行多元 线性回归、逐步回归和回归检验。根据指标本身的特点及 AIC 信息统计量,剔除不显著 的自变量,而达到用尽量少的葡萄的理化指标来表示葡萄酒的理化指标的目的。在求解 过程中,建立典型相关分析模型来分析红葡萄酒色泽指标间的关系,利用主成分分析将 白葡萄的多个指标综合为少数几个主成分,再进行回归分析。模型求解结果显示,葡萄 酒的每个指标都能用部分葡萄的指标来线性表示,且具有较好的拟合效果。 在问题四中,为了分析酿酒葡萄和葡萄酒的理化指标对葡萄酒质量的影响,结合问 题一、二、三的结果以及理化指标和芳香物质的化学意义,综合评估各个广义上的理化 指标(附件二和附件三) ,针对红葡萄酒和白葡萄酒的区别分别在酿酒葡萄和葡萄酒的 理化指标中选取对葡萄酒质量影响较大的指标, 通过线性回归分析将理化指标和葡萄酒 质量进行拟合,从而得出酿酒葡萄和葡萄酒的理化指标对葡萄酒质量的影响。为进一步 论证结果,首先,对模型进行残差分析以及拟合情况分析;其次,用分组样本检验方法, 将白葡萄酒的 28 个样本数据分成两组,采用用一组进行拟合,另一组进行结果回带分 析的方式,进一步论证用葡萄和葡萄酒的理化指标来评价葡萄酒的质量的可靠性。通过 论证分析得出结论:葡萄和葡萄酒的理化指标可以用来评价葡萄酒的质量,但也有其不 足之处,如当从葡萄酒食用性方便角度考虑,用评酒员评价方法就更直接。 关键词:葡萄酒质量 识别聚类 失误度 非线性回归 逐步回归 Fisher 判别分析 主成 分分析 因子分析 显著性检验 残差分析 异常点检测
2012数模国赛备赛优秀论文提取

问题1:汽车保险公司为了降低车辆出险率,鼓励保户续保,发展潜在保户,通常都会对满足一定要求的保户或者投保人给与一定比例的保费浮动优惠,就是通常所说的保费折扣。
请根据附件中的参考数据,以及第一阶段中对于影响续保率因素的分析,给出一套较为合理的保费浮动方案。
问题2:一些大型的保险公司要在全国很多地区设立分公司。
总公司每年要对分公司的业绩情况进行考核,考核结果直接影响分公司领导班子的去留。
传统的考核方法就是计算分公司的保费收入和理赔支出的差额。
一些分公司为了提高自己的考核成绩,会使用受理一些风险较大的投保或者故意拖延理赔的处理时间等方法。
因此,很多保险公司开始考虑引入风险评估机制来对分公司进行考核,潜在风险较低的分公司会得到较高的考核成绩,请建立合理的模型对参考数据中的汽车保险公司进行潜在风险的评估,并通过对模型的深入分析对该公司今后的风险控制提出建议。
1010车辆出现次数与续保率有着密切关系,通常出现次数越多续保费用也就越高,续保率也就越低。
平安保险公司:出险三次以上要按原价1.3倍购买商业险平安保险广西区南宁分公司表示:对在前一年未发生交通事故的车主第二年购买交强险时,可享受续保费率下浮10%的优惠,连续三年未发生交通事故的可下浮30%。
对于出险次数达到三次以上的车主,平安保险一般不会拒保,只会相应调高费率,第二年购买商业险的时候要按原价的1.3倍来购买。
华安保险公司:出险次数达到八次,不能购买其它车辆商业险能否购买到其他商业险,华安保险公司表示要根据车主的出险档次来定。
一年内出险次数达到八次,第二年在华安保险续保时只能买到交强险、第三者,其他商业保险就购买不到了。
所以说上面提到的那位网友也不能在华安保险买到其它商业险。
安邦保险公司:出险达到五次,第二年有有可能买不到其他商业险。
安邦保险公司:去年出险达到三次以上的,第二年交强险续保的时候公司要按车型来理算上浮费率。
如果出险次数达到五次,第二年只能买到交强险、第三者,其它商业保险可能买不到了。
2012国赛A题数学建模论文

R rij Ai Bj Yi Pi a pi Z
^
R 中第 i 行第 j 列中的元素
红葡萄第 i 个理化指标 白葡萄第 j 个理化指标 红葡萄第 i 个主成分 白葡萄第 i 主成分 主成分与原始数据的系数 得分估计值 第 i 个公因子的方差贡献率 对公因子逐步回归求得的函数 酿酒红葡萄的第 i 个典型变量 酿酒白葡萄的第 i 个典型变量 特征根
第一组得 分 82 74.2 85.3 79.4 69 68.4 77.5 71.4 72.9 74.3 72.3 63.3 65.9 69 72.4 74 78.8 73.1 72.2 77.8 76.4 71 75.9 73.3 77.1 81.3 64.8 81.3
第二组得分 77.9 75.8 75.6 76.9 81.5 75.5 74.2 72.3 80.4 79.8 71.4 72.4 73.9 77.1 78.4 67.3 80.3 76.7 76.4 76.6 79.2 79.4 77.4 76.1 79.5 74.3 77 79.6
我们参赛选择的题号是(从 A/B/C/D 中选择一项填写) : 我们的参赛报名号为(如果赛区设置报名号的话) : 所属学校(请填写完整的全名) : 参赛队员 (打印并签名) :1. 2. 3. 指导教师或指导教师组负责人 (打印并签名): 日期: 日
A
年
月
2012 高教社杯全国大学生数学建模竞赛
编 号 专 用 页
第一组得分 62.7 80.3 80.4 68.6 73.3 72.2 67.5 72.3 81.5 67.5 70.1 53.9 74.6 69.2 58.7 74.6 79.3 59.9 78.6 78.6 77.1 77.2 85.6 78 69.2 73.8 73
2012年 全国数学建模大赛A题获奖论文英文版English
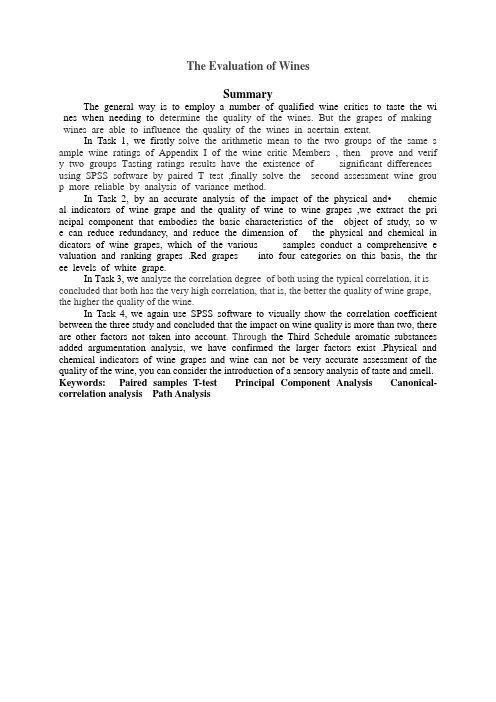
The Evaluation of WinesSummaryThe general way is to employ a number of qualified wine critics to taste the wi nes when needing to determine the quality of the wines. But the grapes of making wines are able to influence the quality of the wines in acertain extent.In Task 1, we firstly solve the arithmetic mean to the two groups of the same s ample wine ratings of Appendix I of the wine critic Members , then prove and verif y two groups Tasting ratings results have the existence of significant differences using SPSS software by paired T test ,finally solve the second assessment wine grou p more reliable by analysis of variance method.In Task 2, by an accurate analysis of the impact of the physical and•chemic al indicators of wine grape and the quality of wine to wine grapes ,we extract the pri ncipal component that embodies the basic characteristics of the object of study, so w e can reduce redundancy, and reduce the dimension of the physical and chemical in dicators of wine grapes, which of the various samples conduct a comprehensive e valuation and ranking grapes .Red grapes into four categories on this basis, the thr ee levels of white grape.In Task 3, we analyze the correlation degree of both using the typical correlation, it is concluded that both has the very high correlation, that is, the better the quality of wine grape, the higher the quality of the wine.In Task 4,we again use SPSS software to visually show the correlation coefficient between the three study and concluded that the impact on wine quality is more than two, there are other factors not taken into account.Through the Third Schedule aromatic substances added argumentation analysis, we have confirmed the larger factors exist .Physical and chemical indicators of wine grapes and wine can not be very accurate assessment of the quality of the wine, you can consider the introduction of a sensory analysis of taste and smell. Keywords:Paired samples T-test Principal Component Analysis Canonical- correlation analysis Path AnalysisIntroductionThe general way is to employ a number of qualified wine critics to tast the wines when needing to determine the quality of the wines. First,each tasting member in the taste of the wine samples give rates in accordance with the classification index, then sum the total scores to determine the quality of the wines. Quality of wine grape has a direct bearing with the quality of the wines. the physical and chemical indicators of wine grape and the wine can reflect the quality of the wine and grape to some extent. Following issues need to be addressed :1. Analysis in Annex 1 two groups of evaluation of wine member of the evaluation results whether there were significant differences of both, which a set of results more reliable.2. According to the physical and chemical indicators of the wine grape and wine quality, how about were these wine grape classified ?3. Analyze the link between the physical and chemical indicators of wine grapes and wine.4. Analyze the physical and chemical index of the wine grape and wine to the influence on the quality of wine, and demonstrate the ability to use the physical and chemical indicators of grape and wine to evaluate the quality of the wine.The analysis of issueBackgroundThe high-quality wines are popular in 2012. It ’s seems to be urgent to study that the main raw materials - whether the quality of the red grapes and white grapes of the wine is good or bad a decisive role. Therefore analyzed the relationship between wines ’ and grapes ’ quality and physical and chemical indicators over thirty kinds of physical and chemical indicators of grape and wine.Assumptions1 Each tasting wine samples from an approximate normal distribution of the distribution of the overall ;2 Tasting members are normal senses, there is not much difference ;3 Annex all the physical and chemical indicators can be representative of the nature of the study, omission of the object of study have a significant impact on physical and chemical indicators ;Symbolic representationα: Significant parameters ;W : Rejection region range ;m : The number of indicator variables ; 12,,...,,m x x x : Evaluation object ;i j a⋅ Standardized index value ; ,j j s μ: Sample mean and sample standard deviation of the j-th indicator ; R : Correlation coefficient matrix ; A : Standardized matrix; (1,2,,)i i m λ= Eigenvalue ;(1,2,,)i e i m = Eigenvectors ;1V Eigenvectors of the correlation coefficient matrix of red wine grapes; 1D Red wine grape correlation coefficient matrix eigenvalue;2V White wine grape correlation coefficient matrix of eigenvectors; 2D Eigenvalues of the correlation matrix of white wine grapes;12,,,p λλλ The characteristic value corresponding to the first, second ...... p maincomponen ;p The number of indicators in the wine ;q The number of indicators of the wine grape ;11R The coefficient matrix of the first set of variables ;22R The coefficient matrix of the second set of variables ;11'R 、22'R The correlation coefficient of the first set of variables and the second setof variables ;1Z Comprehensive evaluation function of the principal component of red grape wine grape ;2Z Comprehensive evaluation function of the principal components of the white grape wine grape ;,1,2,...,28j x j = Transverse section of physical and chemical indicators in accordance with Annex II to turn on behalf of the 27-level indicators of the total amino acids, proteins, VC, ......, as well as wine quality and wine quality ratings;,1,2,...,14,15;i y i = Wine quality, peel quality juice rate (%), respectively, in turn, said, stems ratio (%), one hundred quality / g, ear quality / g dry matter content g/100g solid acid than titratable acidity (g/l), PH value, soluble solids g/l, the reducing sugars g/L, total sugar g/L, and flavonols (mg/kg), resveratrol (mg/kg) and other physical and chemical indicators ;Model establishments and solutionsTask1:Analysis in Annex 1 two groups of evaluation of wine member of the evaluationresults whether there were significant differences of both, which a set of results more reliable.To review the wine member of the evaluation result, significant difference and credibility evaluation calculation methods are varied ,mainly including Sensory evaluation of significant differences, based on the evaluation of the credibility of the Analytic Hierarchy Process, discriminant analysis, T value analysis, F value analysis,etc.Firstly, in accordance with the principle of the score with the same sample that 10 Tasting 'average score obtained in Schedule I of the first and second sets each red and white wine sample tasting ratings. Are listed below:two tables of very difference.Establishments of Model 11-1 For the evaluation of red wine :First of all by the data observation, it is known that on the whole, in view of the same sample wine in the first group and the second group of score difference are more prominent,therefore the relationship between the two with a Broken line vividly expressed. From the sensory ,image display greater differences in two groups Tasting set of evaluation criteria, shown in the following figure line chart:Figure 1 the overall rating of FirstSet and SecondSet for each of the red wine samplesThen, the overall rating results of the two rating wine group in a significant level 0.05α= are made a significant difference test. Firstly, each wine sample is selected from the large number of the same kind of samples wine from testing samples ,sample population can be approximated as a normal distribution . Secondly, Of all samples tested constitute 27 paired samples tested overall. Therefore we paired samples T-test two samples. The results are as follows:Table 3 The paired samples T-test the first and second Sets(The red)Inspection objectthe difference with 95% confidence interval tNPLowerlimitCeilingFirst and Second0.41569 4.66579 2.458 26 0.021In fact, P<0.05α=and 1/2,0.975,262.458 2.0555n t t α->==, the result falls into Rejection region {}1/2W t t α-=≥.Therefore the overall evaluation criteria of the members of the twogroups of wine critic has a significant difference.Which is trustworthier : The smaller the v ariance,the trustworthier the group’s evaluate ,when we study a single kind of wine sample. In that ,we compare the variance of these two groups to define the trustworthier group which have a smaller variance .Table 4 The credibility test for red wineWine sample sFirstSet ’s Variance Second’sVarianceThetrustworthiergroupWine sample sFirstSet ’s Variance Second’sVarianceThetrustworthiergroup1 236.1 736.9 一 15 770.1 372.1 二2 358.1 146 二 16 112.9 180.9 一3 412.4 276.4 二 17 792.1 82.5 二 4 972.4 371.6 二 18 424.9 452.4 一which is the better,the ratio of the two groups for 8:19;therefore the Second is trustworthier.1 -1 For the evaluation of white wine :Firstly,in a word,only studying the same sample wine,two groups are in small diference., therefore the relationship between the two with a Broken line vividly expressed.Said out as follows:Figure 2 the overall rating of FirstSet and SecondSet for each of the white wine samples Because wo use the same way to answer about the white wines’ question , There is no longer the detailed solution process .In summary,the evaluation results of both show significant differences,and the Second is trustworthier wherever in the red wines or the white wines. At the same time ,we find a standard to measure the quality of wine—the tasing ratings of the trustworthier sommeliers. Task2: According to the physical and chemical indicators of the wine grape and wine quality, how about were these wine grape classified ?Analysis of Model 2:There are a lot of the physical and chemical indicators of wine grapes that include more than 30 level indicators and some secondary indicators in Annex 2.we hope that many high correlation variables in wine grape physicochemical indicators are converted into mutually independent or uncorrelated variables,to choose only a small amount of indicators that can reflect most of the nature of the object of study. There are so-called Principal component which can be used to explain the research object indicators.2-1 The Step of using Principal Component Analisis way:(1) Standardize raw dataThere are m indicator variables that can be used to Principal Component Analisis way,namedas 12,,...,.m x x x There are all (1n or )2n evaluation objects.In there ,128,27m n ==,and 228n =( 1n represents the red wine as a research object, 2nrepresents the white wine as aresearch object ). The value of the j th indicator of the ith evaluation object is i j a ⋅,thereforewe gain the initial matrix of the object of study:1111m n n m a a A a a ⋅⋅⋅⋅⎛⎫ ⎪= ⎪ ⎪⎝⎭ ,The value of each indicator is converted to a standardized indicator value.As follows:,1,2,...,;1,2,...,,i j i i j ja ai n j m s μ⋅⋅-=== Among :11,1,2,...,,n i i j j i a s j m n μ⋅====∑At the same time,j and j srespectively is the sample’s mean and standard deviation Correspondingly ,1,2,...,j j j jx xj m s μ-==It ’s a standardized indicator variable.(2)Calculting the Correlation coefficient matrix R: the Correlation coefficient matrix()i j m n R r ⋅⨯=11122122212m m m m m m m r r r r r r R r r r ⋅⋅⋅⋅⋅⋅⋅⋅⋅⎡⎤⎢⎥⎢⎥=⎢⎥⎢⎥⎣⎦ TR A A =*,among : A represents A’s standardized matrix .By nature that R is a realsymmetric matrix (j i i j r r ⋅⋅=r ij =r ji ),therefore we only need to calculate on the upper triangle element or lower triangular elements to seek R . The Correlation coefficient matrix of the red wine grapes is R1,and the Correlation coefficient matrix of the white is R2. Specific data, see Annex 1.0 and Annex 3.0.(3)Calculating eigenvalues and eigenvectorsFirstly , we have solved the characteristic equation 0=-R I λ. We usually obtain eigenvalues (1,2,,)i i m λ= by Jacobi method , then arrange its in order of size 12,0m λλλ≥≥≥≥Secondly ,we respectively obtain the eigenvector (1,2,,)i e i m = corresponding to theeigenvalue i λ. Here is a requirement ,means i e =1,211mijj e ==∑,of which ij e represents the j-th component of the vector i e .The eigenvector V1 and eigenvalue D1 of the red wine grapes’ Correlation coefficient matrix , see Annex 2.0.The Characteristic roots of red wine grapes D1 Sequence :-0.0000 0.0000 0.0016 0.0058 0.0112 0.0156 0.0299 0.0504 0.0652 0.0826 0.1764 0.2025 0.2275 0.2350 0.3019 0.3712 0.5499 0.6844 0.7307 0.8076 0.9969 1.2228 1.5217 1.9934 2.8682 3.2615 4.7702 6.8158The eigenvector V2 and eigenvalue D2 of the white wine grapes’ Correlation coefficient matrix , see Annex 4.0.The Characteristic roots of white wine grapes D2 Sequence :-0.0000 0.0008 0.0026 0.0060 0.0250 0.0441 0.0634 0.0790 0.0953 0.1352 0.2719 0.3142 0.3156 0.3798 0.4458 0.6326 0.7267 0.8919 0.9663 1.0753 1.2937 1.4996 1.6175 1.7869 2.0894 2.9810 4.6623 5.5981(4)Calculation of the contribution rate and cumulative contribution rate of the main componentsThe contribution rate of main component i z :1(1,2,,)imkk i m λλ==∑The cumulative contribution rate :11(1,2,,)ikk mkk i m λλ===∑∑We generally take the characteristic values of 85-95% of the cumulatiive contribution rate corresponding to 1-st,2-nd,…,p-th(p≤m) main cpmponent.2-2 The results of principal component analysis :The eigenvalues 123,,,.....p λλλλwhose accumulative contribution rate of 85-95% can be generally selected as principal component parameters . According to theconclusion of the Principal Component Analysis , whenever in red wine or the white wine, the quality of wine is regarded as the first principal component. This shows that the quality of the evaluation of the grade of wine grapes wine share an important role .In addition ,we also gain:1. In the system which the red wine grapes act as study ,the effect of the principal component analysis of the first eleven characteristic roots whose cumulative contribution rate achieves 90% above is very great . Therefore we choose the first fourteen principal components []121314,,...,,y y y yto run a comprehensive evaluation. The contributions of fourteen principal component variables are the weight tobuild principal component comprehensive evaluation model of the red wine grapes , namly:345678910111213112140.116482559y +0.10243608y 0.0711931110.054346623+0.043671585y +0.035603699y +0.02884296y +0.026096522y +0.024442944y +0.019639356y +0.0.243422980.17036401325719y 894y 0.010782181y Z y y y ++++=+Therefore, we put all the objects corresponding to factors of the various principal components into the model ,to gain the comprehensive evaluation and sort results of the red wine grapes.2-3 ConclutionFor the red wine grapes , there are quite difference to various samples of grapes , therefore the red wine grape samples are divided into four grades: "Premium", "Great", "Qualified" and "Bad" .No. 26 sample is classified as "Premium" level by visible quality and particularly high comprehensive evaluation ;No. 17,24,5, and 20 are classified as "Great" level by great quality and high comprehensive evaluation ;No. 23,25,10,12,18,27,6,8,14,9,19,and sample is classified as "Qualified" level ;And , No. 15,3,13,4,21,7,11,2,22,and 16 sample are only classified as "Bad" , because their evaluateons are less than 60.For the white wine grapes,…Task3:Analyze the link between the physical and chemical indicators of wine grapes and wine.3-1 Model preparation :Introduced the idea of canonical correlation analysis In task 3 , we study the correlation relationship between 27 physical and chemical indicators of wine grapes ,10 of the red wines and 9 of the white wines ; the method which is similar to the main component is used, to respectively find the linear combination of the two sets of variables.Can make the number of variables to simplify, and can achieve the purpose of analysis correlation.3-2 Modeling steps :一、According to the purpose of the analysis to establish the original matrixAmong: p is the number of indicators in the wines, q is the wine grape number of indicators11111111p q n np n nq x x y y x x y y ⎡⎤⎢⎥⎢⎥⎢⎥⎣⎦;二、Standardization of the original data , and calculating a correlation coefficient matrix11212122R R R R R ⎡⎤=⎢⎥⎣⎦Among: 11R ,22R respectively is the correlation coefficient matrix of the first set ofvariables and a second set of variables .三、Seeking canonical correlation coefficient and canonical variablesCalculate the eigenvalues and eigenvectors of the matrix A and B ,to gain the canonical correlation coefficient and canonical variables.Among:1111122221A R R R R --= ,1122211112B R R R R --=.四、Making canonical aorrelation analysis by using SPSS,to analysis of the link between the physical and chemical indicators of the wine grape and wine.The first step, the original data entry wine grapes and wines .As follows : X1,X2, X3 ,X4 ,X5 respectively represents Anthocyanin ,Tannin, Total phenolic , Wine total flavonoids , DPPH half inhibition volume ; Y1 ,Y2 ,Y3 …Y16 respectively represents the original data of the wine grapes of Annex II (Vertical indicators from the total amino acids ). Due to the limited space here is only part of the data is given. Detailed data see Annex Table 5.0(redpu.xls).Run in SPSS results are as follows:between the various indicators are small.If the correlation coefficient of the two indicators, two indicators may reflect the same ,and you can consider the merger.Analyze the physical and chemical index of the wine grape and wine to the influence on the quality of wine, and demonstrate the ability to use the physical and chemical indicators of grape and wine to evaluate the quality of the wine.4-1 The idea of Model 4:In order to analysis of wine grape and wine physical and chemical index to the influence on the quality of wine , we introduce linear regression theory to realize size analysis method . Using the Path Analysis on the basis of multiple regression , correlation coefficient iy r is decomposed into the direct path coefficients and indirect path coefficients .Path Analysis of the theory has been proved that any simple Correlation coefficient (iy r)between an independent variable i x and dependent variable y= Direct path (iy P)coefficient between x and y+ Indirect path coefficients of all the i x and y;Indirect path coefficients ibetween an independent variable any i x and dependent variable any y=Correlation coefficient (iy r)×Path coefficients (jy p) .Making Path Analysis process, it is generally believed that the most difficult part to calculate is the path coefficients. In fact , the path coefficients that we need to can be obtained by liner regression calculation . Then Indirect path coefficients can be multiplied by the correlation coefficient .4-2Problem-solving steps :4.2-1Data entryStart SPSS program, data input SPSS,name of each variable, andset the variable label.Wine quality is acted as the dependent variable y, and the physical and chemical indicators of the wine grape and wine including Anthocyanins, Tannins, Total Phenols, Wine Total Flavonoids, Resveratrol,Trans polydatin,Cis polydatin,Trans-resveratrol,Cis-resveratrol,DPPH half inhibition volume,and Color were the independent variables x1, x2, x3,x4,x5,x6,x7,x8,x9,x10.4.2-2Normality test on the dependent variable yFigure 3y s Standard Q-Q diagramStrengths and Weaknesses1.The strengths of model(1) The model established by the questions one to four are in strict theory based on analysis derived,comparing theoretical calculations with actual background, we have amended ,therefore it makes model more reasonable.(2) The use of paired samples T-test makes the results more reliable . This paper originality is very strong,because of the most of the models in the article derived and established strictly.(3) All calculations are used to specialized mathematical software and processing large amounts of data, such conclusions credibility is higher.We quantitative analysis many influencing factors that model involves, to make the paper more persuasive.2.The weaknesses of modelIn questionI and II ,due to the choice of the numerical inevitably produce a slight error and the complexity of factors affect ,so the calculation results inevitably produce certain error. References[1] Dongyan Chen , Dongmei Li , Shuzhong Wang , Mathematical Model [M].Beijing:Science Press,2007[2] Fengqiu Liu , Shanqiang Li , Zuobao Cao, Mathematical experiment [M].Harbin: HarbinInstitute of Technology Press,2010[3] Shisong Mao,Yiming Cheng,Xiaolong Pu, Probability theory and mathematical statisticstutorial [M].Beijing: Higher Education Press,2004[4] Shoukui Si,Xi Sun, Algorithm and application of mathematical modeling [M].Beijing:National Defence Industry Press,2011[5] Zhixing Zhang, Design and Application of the MATLAB program [M].Beijing: TsinghuaUniversity Press,2002。
2012年数学建模A题优秀论文
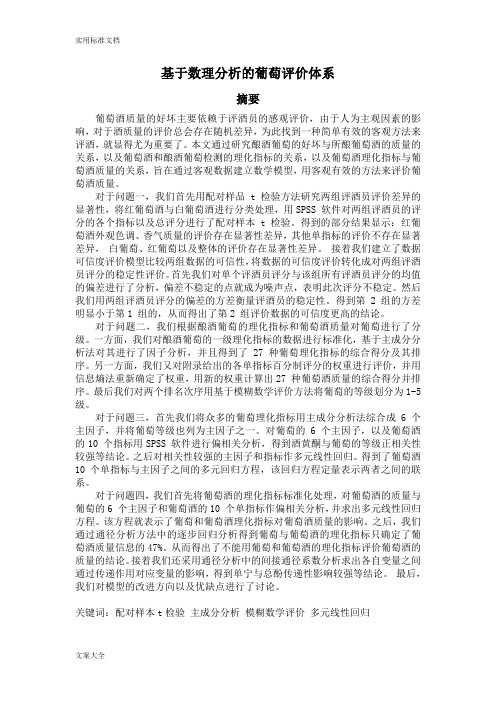
基于数理分析的葡萄评价体系摘要葡萄酒质量的好坏主要依赖于评酒员的感观评价,由于人为主观因素的影响,对于酒质量的评价总会存在随机差异,为此找到一种简单有效的客观方法来评酒,就显得尤为重要了。
本文通过研究酿酒葡萄的好坏与所酿葡萄酒的质量的关系,以及葡萄酒和酿酒葡萄检测的理化指标的关系,以及葡萄酒理化指标与葡萄酒质量的关系,旨在通过客观数据建立数学模型,用客观有效的方法来评价葡萄酒质量。
对于问题一,我们首先用配对样品t 检验方法研究两组评酒员评价差异的显著性,将红葡萄酒与白葡萄酒进行分类处理,用SPSS 软件对两组评酒员的评分的各个指标以及总评分进行了配对样本t 检验。
得到的部分结果显示:红葡萄酒外观色调、香气质量的评价存在显著性差异,其他单指标的评价不存在显著差异,白葡萄、红葡萄以及整体的评价存在显著性差异。
接着我们建立了数据可信度评价模型比较两组数据的可信性,将数据的可信度评价转化成对两组评酒员评分的稳定性评价。
首先我们对单个评酒员评分与该组所有评酒员评分的均值的偏差进行了分析,偏差不稳定的点就成为噪声点,表明此次评分不稳定。
然后我们用两组评酒员评分的偏差的方差衡量评酒员的稳定性。
得到第 2 组的方差明显小于第1 组的,从而得出了第2 组评价数据的可信度更高的结论。
对于问题二,我们根据酿酒葡萄的理化指标和葡萄酒质量对葡萄进行了分级。
一方面,我们对酿酒葡萄的一级理化指标的数据进行标准化,基于主成分分析法对其进行了因子分析,并且得到了27 种葡萄理化指标的综合得分及其排序。
另一方面,我们又对附录给出的各单指标百分制评分的权重进行评价,并用信息熵法重新确定了权重,用新的权重计算出27 种葡萄酒质量的综合得分并排序。
最后我们对两个排名次序用基于模糊数学评价方法将葡萄的等级划分为1-5 级。
对于问题三,首先我们将众多的葡萄理化指标用主成分分析法综合成 6 个主因子,并将葡萄等级也列为主因子之一。
对葡萄的 6 个主因子,以及葡萄酒的10 个指标用SPSS 软件进行偏相关分析,得到酒黄酮与葡萄的等级正相关性较强等结论。
2012国赛A题-葡萄酒

4.1.1 置信区间法 为了降低各评酒员之间的异质性, 先分别计算每一组中所有评酒员对同一酒样的平 均值( s j )和标准差( j ),评酒员 i 对酒样品 j 评价的置信区间为 s j j[1] 。 如果评酒员 i 对酒品 j 的评分( sij )在其置信区间内则保留;如果评酒员 i 对酒品 j 的评分( sij ) 不在其置信区间内则逐步调整,使评分都处于置信区间 s j j 内,具体为: 若 sij <j , 则 Sij =sij + j ; 若 sij >j ,则 Sij =sij - j 。 直接使用 matlab (附录一) 通过置信区间法对两组评酒员对红葡萄酒和白葡萄酒的 评分进行修正, 此时的数据更加可信, 同时对每一个酒样的得分求均值, 结果见表 4.1.2 (只给出第一组的红葡萄酒数据) 。 表 2 红葡萄酒样品经置信区间检验转 zg jx Fjx Yj
四、模型建立及求解
说明:限于篇幅因素,本文说明模型原理时一律用红葡萄(酒)数据说明,白葡萄(酒) 只给出最终结果。 4.1 问题一的求解 首先,将每个评酒员对葡萄酒样品的分类指标打分求和,用得到的总分代表该评酒 员对葡萄酒样品质量的评价结果。然而,由于每个评酒员的评价尺度、评价位置以及评 价方向的差异,在对评价结果进行统计分析时,必须对评酒员的原始数据进行相应的处 理,以降低评酒员的系统误差(即异质性) ,真实反映样品间的差异。 表 1 红葡萄酒样品的原始数据
葡萄酒质量评价方法的研究
摘要
本文给出了判别评价结果显著性差异以及可信度的方法, 建立了模糊综合评价模型 用熵权法对酿酒葡萄进行分级, 根据多元回归分析拟合出了酿酒葡萄与葡萄酒理化指标 间的关系, 问题一:用评酒员对葡萄酒各类指标打分的总分衡量葡萄酒的质量,并利用置信区 间法降低评酒员的异质性,使数据更真实的反映酒样间的差异。再将这些数据进行方差 分析可知两组评酒员评价对红葡萄酒的评价没有显著差异, 对白葡萄酒的评价有显著性 差异。最后根据信度分析可知第一组评酒员更可信。 问题二:首先将酿酒葡萄的理化指标进行主成分分析,将葡萄酒质量和提取的主成 分一起作为衡量酿酒葡萄质量的指标,利用模糊综合评价法的原理及其评价方法,同时 将信息论中的熵值引入模糊综合评价隶属矩阵的确定过程, 利用熵权法构造隶属函数矩 阵, 尽量消除传统权重确定中主观因素的影响, 从而对酿酒葡萄进行了良好的质量评价。 问题三:首先借上问主成分分析法,将葡萄和葡萄酒的理化指标进行处理。然后, 在假设条件下,建立多元线性回归模型,运用多元线性回归分析法分析葡萄和葡萄酒理 化指标,做近似拟合,得出相应的拟合度值。对相关且拟合度高的自、因变量之间进行 分析,最终得到结论。 问题四:先建立因果关系模型,分别对葡萄芳香物质与葡萄酒芳香物质,葡萄酒理 化指标与葡萄酒评价分数进行如上问的分析,综合两者,并在给出的关系模型的基础上 对能否用葡萄及葡萄酒理化指标作为判断方法给予阐述。
2012年国赛A葡萄酒获奖论文带附录(完整版)

2012年国赛A葡萄酒获奖论文带附录(完整版)2012高教社杯全国大学生数学建模竞赛编号专用页赛区评阅编号(由赛区组委会评阅前进行编号):赛区评阅记录(可供赛区评阅时使用):评阅人评分备注全国统一编号(由赛区组委会送交全国前编号):全国评阅编号(由全国组委会评阅前进行编号):A 题葡萄酒的评价摘要:确定葡萄酒质量时一般是通过聘请一批有资质的评酒员进行品评。
一方面由于每个品酒员间存在评价尺度、评价位置和评价方向等方面的差异,导致不同品酒员对同一酒样的评价存在差异,从而不能真实地反映不同酒样间的差异。
另一方面葡萄酒的质量和酿酒葡萄的好坏又有直接的关系,于是根据题中所给的条件和问题提出相关的约束条件和目标函数,建立合理的数学模型。
对于问题一,在分析附件1中所给的数据后,首先根据每组的10名评酒员对其中的一种酒进行品尝后确定葡萄的质量,然后在进行分析评酒员评27种红葡萄酒的差异,最后运用方差分析对两组评酒员的评价结果进行测定,得出两组评酒员存在是否有显著性差异的结果,看其哪组评酒员的技术水平更高些。
问题二是为了对酿酒葡萄进行分级,要从酿酒葡萄的理化指标和葡萄酒的质量进行分级,在附件2、3中,发现酿酒葡萄的成分数据中有很多因素,首先对酿酒葡萄的理化指标经过查找资料、专家咨询进行了较为有效的分类,我们从中选取一些有效因素,例如:氨基酸总量、糖、单宁、色差值、酸、芳香物质等。
然后再采取系统聚类分析法对酿酒葡萄进行分级。
等级大致分为优、良、中、差四个级别。
在解决问题三时,不仅要考虑酿酒葡萄还要考虑葡萄酒的理化指标,因而采用多元回归模型,模型如下:其中,b0为常数项,为回归系数,错误!未找到引用源。
是随机误差。
2012美赛A题分析

1.单纯的称量叶子的质量是没有研究价值的,因为不同的植株,不同的叶子的其质量不会相同,这样不同植被的数据无任何联系,与后面的分类要求矛盾。
2.考虑到植物的呼吸作用与光合作用,可以从此处下手:
利用有关实验仪器(空气中氧气,二氧化碳的浓度测量以及湿度的测量),建立数学模型,分析植株一昼夜的呼吸量与光合作用量(生物书上有详细介绍),这是完全可以实现的。其呼吸作用量,为植株的不同部分提供能量(可以考虑不同的部分建立不同的权重),在这里不但有叶子自身的需要,还有通过树干的韧皮部运输到植被其他地方以作用,用作诸如树干的形成层分生细胞以及其他作用(好好研究树干的作用)。而光合作用于呼吸作用的差值便是植株一昼夜的糖分积累,则积累到植株的各个部分(叶子、树干、根等)。
5.关于叶子的分类,也是建立在地理环境对植株生理作用的影响,虽然是建立在叶子质量上的分类,我的理解是对有机物积累能力的分类:可以考虑在主体上按热带、亚热带、温带、寒带等划分(叶的形状),在同一纬度上,通过其积累有机物能力划分(质量的不同)。
6.关于“最小面积的”的遮蔽以及“最大范围的扩展”,可以从不同的地理环境对植物的光合作用、呼吸作用以及蒸腾作用的角度分析。
注:之所以落叶是因为在秋末时,树停止吸收水分无机物等,以减少呼吸、蒸腾作用,3.叶子的输送量与树干的韧皮部等密切相关,这就跟我们的网速一样,另一个方面,也就是说叶子的质量与树干密切相关,可以找一下有关文献。
4.据此,想一下,如果把一天内植株的能量积累看做A,叶子积累b,树干积累c,其他组织积累d,公式为A=b+c+d,在一年的不同时期有不同的权重,然后 ,就可以得到叶子以及其他组织在不同时期重量的范围值。(还可以更忽悠一些)
7.植株的叶的形状与树干的结构也是从最大光合作用的角度考虑。
2012年数学建模A题一等奖获奖论文
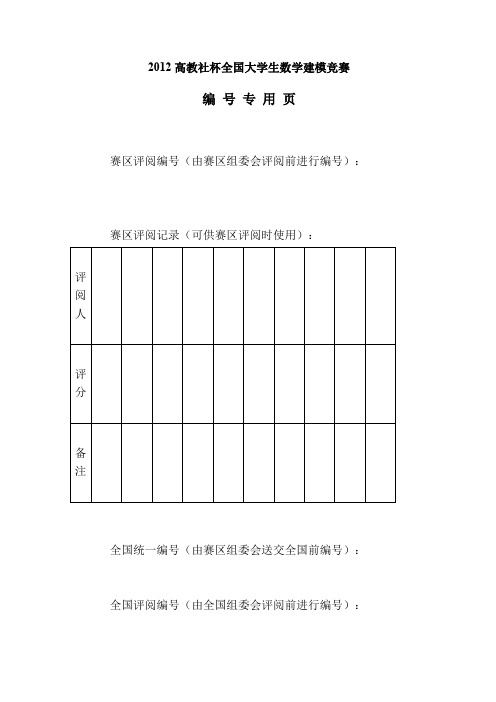
分的差异是否在一定的置信区间内,若不在,则认为评分差异性显著。 考虑到本题的背景,两组评分的差异可体现在对样本酒的排名差异上。由于 该问属于食品评价中的感官评价问题,因此,可结合感官评价中的排序检验与非 参数检验中的符号秩检验,对两组评分的显著性进行评价。 1.1.1 样品秩次和秩和的求解 评酒员对每一个酒样均从四大方面进行了评分。根据题意,葡萄酒的质量由 总分所确定。 因此, 我们将每一个方面的评分加和, 得到 i 品酒员对葡萄酒样品 j 的总评分。 以红葡萄酒的评价为例,对于品酒员 i ,将其对 27 种样品的评分进行排序, 评分最高的酒样秩次为 1,当多个样品有相同秩次时,则取平均秩次。记在 i 品 酒员的评价排序中, j 酒样的秩次为 xij ,可得到秩次矩阵为:
6
秩和得到一个新的排序。由于此排序综合了 20 个评酒员的结果,因此,更能反 应酒样的排序真实性,即认为该综合排序为理想排序。记样品 j 在第一组、第二 组排序内的秩次为 X j (1) , X j (2) ,综合之后排序秩次为 X j 。红葡萄酒三种排序的 比较图如下:
二、问题分析
问题一的分析 问题一中,每个品酒员都要从外观、香气、口感、整体四个大方面对每个酒 样进行评分,可将每个方面的评分相加作为总分确定葡萄酒的质量。问题一涉及 的是葡萄酒感官评价结果的统计检验问题,由于样本量偏小,且葡萄酒质量评分 的分布难以确定,因此,可考虑采取非参数检验的办法。 结合本题的背景,对于问题一中感官评价的问题,可选用排列试验中的排序 检验法对总分进行排序。对于 10 种排序结果,根据每一个排序的秩次求得每个 样品的秩和。最后通过秩和的非参数检验的方法评价有无显著性差异。 要评价哪组的评价结果更可信,主要是检验组内品酒员的评分是否集中,即 比较哪组的方差更小, 亦可以通过该组内所有品酒员与最终得分的差异来确定谁 的可信度更改。 问题二的分析 问题二中,对酿酒葡萄进行分级时,根据题意要将葡萄的理化指标与葡萄酒 的质量统一结合作为参考。 而葡萄酒的质量则是通过问题一中感官评价的得分反 应的。由于理化指标过多,因此在解决本问时,首先应该完成对指标的处理,尤 其是怎样将附表三的芳香物质与附表二中的理化指标结合起来。 由于指标的繁杂,且难以确定指标是偏大型还是偏小型,因此,可考虑将众 多指标数据经过转换,统一成与感官排序一样的排序类型数据,这样,转换后的 指标即可直接用来对葡萄进行分级。 本问的整体思想还是可运用排序检验中的求 秩和的方法。
国赛A题优秀论文
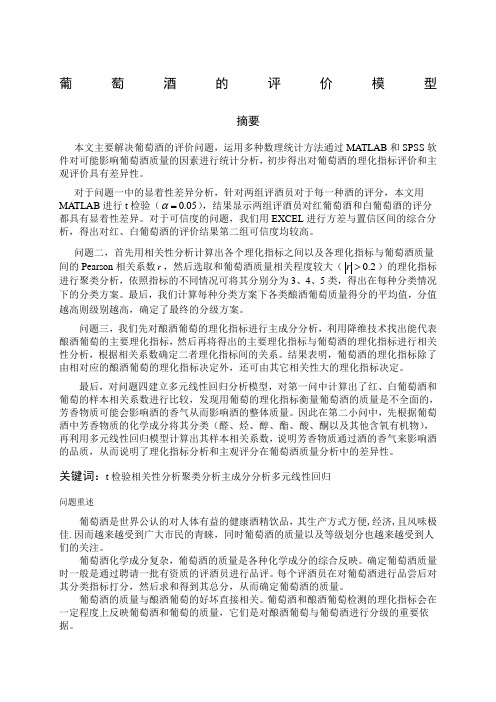
葡萄酒的评价模型摘要本文主要解决葡萄酒的评价问题,运用多种数理统计方法通过MATLAB和SPSS软件对可能影响葡萄酒质量的因素进行统计分析,初步得出对葡萄酒的理化指标评价和主观评价具有差异性。
对于问题一中的显着性差异分析,针对两组评酒员对于每一种酒的评分,本文用α=),结果显示两组评酒员对红葡萄酒和白葡萄酒的评分MATLAB进行t检验(0.05都具有显着性差异。
对于可信度的问题,我们用EXCEL进行方差与置信区间的综合分析,得出对红、白葡萄酒的评价结果第二组可信度均较高。
问题二,首先用相关性分析计算出各个理化指标之间以及各理化指标与葡萄酒质量间的Pearson相关系数r,然后选取和葡萄酒质量相关程度较大(0.2r>)的理化指标进行聚类分析,依照指标的不同情况可将其分别分为3、4、5类,得出在每种分类情况下的分类方案。
最后,我们计算每种分类方案下各类酿酒葡萄质量得分的平均值,分值越高则级别越高,确定了最终的分级方案。
问题三,我们先对酿酒葡萄的理化指标进行主成分分析,利用降维技术找出能代表酿酒葡萄的主要理化指标,然后再将得出的主要理化指标与葡萄酒的理化指标进行相关性分析,根据相关系数确定二者理化指标间的关系。
结果表明,葡萄酒的理化指标除了由相对应的酿酒葡萄的理化指标决定外,还可由其它相关性大的理化指标决定。
最后,对问题四建立多元线性回归分析模型,对第一问中计算出了红、白葡萄酒和葡萄的样本相关系数进行比较,发现用葡萄的理化指标衡量葡萄酒的质量是不全面的,芳香物质可能会影响酒的香气从而影响酒的整体质量。
因此在第二小问中,先根据葡萄酒中芳香物质的化学成分将其分类(醛、烃、醇、酯、酸、酮以及其他含氧有机物),再利用多元线性回归模型计算出其样本相关系数,说明芳香物质通过酒的香气来影响酒的品质,从而说明了理化指标分析和主观评分在葡萄酒质量分析中的差异性。
关键词:t检验相关性分析聚类分析主成分分析多元线性回归问题重述葡萄酒是世界公认的对人体有益的健康酒精饮品,其生产方式方便,经济,且风味极佳.因而越来越受到广大市民的青睐,同时葡萄酒的质量以及等级划分也越来越受到人们的关注。
2012年美国大学生数学建模竞赛MCM-A题-树与树叶-一等奖论文

2012年美国大学生数学建模竞赛MCM-A题-树与树叶-一等奖论文Leaf-Mass & Leaves ClassificationFebruary 14, 2012AbstractIn this paper, we present a statistical model to estimate the leaf mass and classify various leaf shapes based on the data obtained through tree harvest and leaf removal of 21 urban trees. We first explain the diversification of leaf shapes and describe the relationship between leaf shapes and tree profile in order to define the correlation between tree profile and leaf shapes. In order to estimate leaf mass, we apply the regression analysis with four observed independent variables, which allows us to estimate the leaf-mass of a whole-tree. In order to classify leaves, we use hierarchical clustering method according to the dry leaf-mass by Ward's Method. In this method we use the standard Euclidean distance as our principle of classification. Finally we obtain a regression model with vary high coefficient of determination and a good way to classify leaves.For office use onlyT1 ________________T2 ________________T3 ________________T4 ________________ Team Control NumberProblem Chosen AFor office use only F1 ________________ F2 ________________ F3 ________________ F4 ________________2012 Mathematical Contest in Modeling (MCM) Summary Sheet(Attach a copy of this page to each copy of your solution paper.)Type a summary of your results on this page. Do not include the name of your school, advisor, or team members on this page.Dear Editors,By operating and analyzing the experimental data, we have set up an optimizedregression model to estimate leaf mass, and have classified leaves into several specificgroups depending on a reasonable criterion. More importantly, we have noticed somereasonable results.To begin with, a classic regression model is cited to uncover the ambiguousrelationship between tree forms and leaf shapes. As a matter of fact, the leaf shapesare negatively correlated to the tree forms, and the result enables to expose the truthwhy narrower leaves on lower-crown trees are comparatively prolific.Moreover, compared with other regression models, an optimal regression modelestimating leaf mass was proposed. Under the specific significance level, tree mass isclosely relevant to some tree characteristics including crown height (m), crown radius(m) and leaf mass per area of crown projection (2/m g ). Therefore, if theseparameters are measured, the actual tree mass can beestimated.In addition, hierarchical clustering method is applied in leaf classification. In this way,leaves can be reasonably classified relying on the different dry mass.Contents1 Introduction (3)1.1 Outline of Our Approach (3)2 Leaves and the Leaf Shapes (4)2.1 The Diversification of Leaf Shapes (4)2.2 The Relationship between Leaf Shapes and Tree Profile (5)2.3 Leaf Mosaic (7)3 Estimating Leaf Mass (8)3.1 Assumptions of Regression Analysis (8)3.2 Basic Regression Model (8)3.2.1 Independent and Dependent Variables (9)3.2.2 Regression Equation (9)3.2.3 Correlation Matrix and Multicollinearity (9)3.2.4 A Global Test on the Set of Independent Variables (10)3.2.5 Checking the Significance of Each Independent Variable andANOVA Table (11)3.2.6 Diagnostic Plots for the Regression Model (12)3.3 Improved Regression Model (16)3.3.1 Solution and Results Analysis (17)4 Hierarchical Clustering in Leaves (19)4.1 Data Description (19)4.2 Methodology (20)4.3 Results of Clustering (21)4.4 Conclusion (21)5 References (22)。
2012国赛A题优秀论文

葡萄酒的评价模型摘要本文主要解决葡萄酒的评价问题,运用多种数理统计方法通过MATLAB和SPSS软件对可能影响葡萄酒质量的因素进行统计分析,初步得出对葡萄酒的理化指标评价和主观评价具有差异性。
对于问题一中的显著性差异分析,针对两组评酒员对于每一种酒的评分,本文用α=),结果显示两组评酒员对红葡萄酒和白葡萄酒的评分MATLAB进行t检验(0.05都具有显著性差异。
对于可信度的问题,我们用EXCEL进行方差与置信区间的综合分析,得出对红、白葡萄酒的评价结果第二组可信度均较高。
问题二,首先用相关性分析计算出各个理化指标之间以及各理化指标与葡萄酒质量间的Pearson相关系数r,然后选取和葡萄酒质量相关程度较大(0.2r>)的理化指标进行聚类分析,依照指标的不同情况可将其分别分为3、4、5类,得出在每种分类情况下的分类方案。
最后,我们计算每种分类方案下各类酿酒葡萄质量得分的平均值,分值越高则级别越高,确定了最终的分级方案。
问题三,我们先对酿酒葡萄的理化指标进行主成分分析,利用降维技术找出能代表酿酒葡萄的主要理化指标,然后再将得出的主要理化指标与葡萄酒的理化指标进行相关性分析,根据相关系数确定二者理化指标间的关系。
结果表明,葡萄酒的理化指标除了由相对应的酿酒葡萄的理化指标决定外,还可由其它相关性大的理化指标决定。
最后,对问题四建立多元线性回归分析模型,对第一问中计算出了红、白葡萄酒和葡萄的样本相关系数进行比较,发现用葡萄的理化指标衡量葡萄酒的质量是不全面的,芳香物质可能会影响酒的香气从而影响酒的整体质量。
因此在第二小问中,先根据葡萄酒中芳香物质的化学成分将其分类(醛、烃、醇、酯、酸、酮以及其他含氧有机物),再利用多元线性回归模型计算出其样本相关系数,说明芳香物质通过酒的香气来影响酒的品质,从而说明了理化指标分析和主观评分在葡萄酒质量分析中的差异性。
关键词:t检验相关性分析聚类分析主成分分析多元线性回归一、问题重述葡萄酒是世界公认的对人体有益的健康酒精饮品,其生产方式方便, 经济, 且风味极佳. 因而越来越受到广大市民的青睐,同时葡萄酒的质量以及等级划分也越来越受到人们的关注。
2012年国赛数学建模A题优秀论文
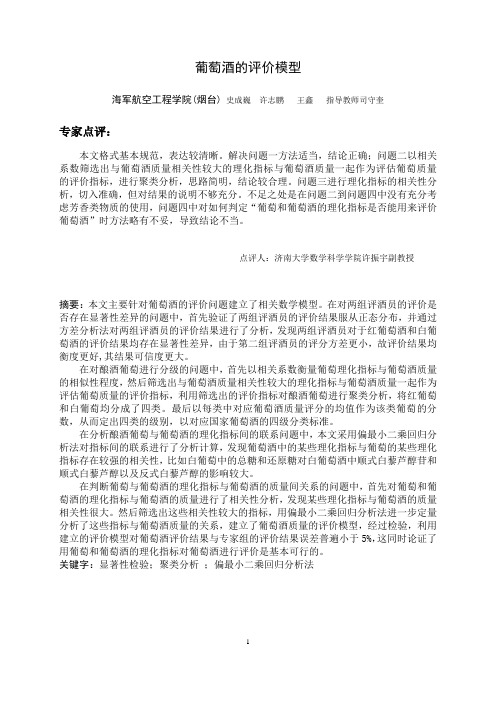
葡萄酒的评价模型海军航空工程学院(烟台)史成巍许志鹏王鑫指导教师司守奎专家点评:本文格式基本规范,表达较清晰。
解决问题一方法适当,结论正确;问题二以相关系数筛选出与葡萄酒质量相关性较大的理化指标与葡萄酒质量一起作为评估葡萄质量的评价指标,进行聚类分析,思路简明,结论较合理。
问题三进行理化指标的相关性分析,切入准确,但对结果的说明不够充分。
不足之处是在问题二到问题四中没有充分考虑芳香类物质的使用,问题四中对如何判定“葡萄和葡萄酒的理化指标是否能用来评价葡萄酒”时方法略有不妥,导致结论不当。
点评人:济南大学数学科学学院许振宇副教授摘要:本文主要针对葡萄酒的评价问题建立了相关数学模型。
在对两组评酒员的评价是否存在显著性差异的问题中,首先验证了两组评酒员的评价结果服从正态分布,并通过方差分析法对两组评酒员的评价结果进行了分析,发现两组评酒员对于红葡萄酒和白葡萄酒的评价结果均存在显著性差异,由于第二组评酒员的评分方差更小,故评价结果均衡度更好,其结果可信度更大。
在对酿酒葡萄进行分级的问题中,首先以相关系数衡量葡萄理化指标与葡萄酒质量的相似性程度,然后筛选出与葡萄酒质量相关性较大的理化指标与葡萄酒质量一起作为评估葡萄质量的评价指标,利用筛选出的评价指标对酿酒葡萄进行聚类分析,将红葡萄和白葡萄均分成了四类。
最后以每类中对应葡萄酒质量评分的均值作为该类葡萄的分数,从而定出四类的级别,以对应国家葡萄酒的四级分类标准。
在分析酿酒葡萄与葡萄酒的理化指标间的联系问题中,本文采用偏最小二乘回归分析法对指标间的联系进行了分析计算,发现葡萄酒中的某些理化指标与葡萄的某些理化指标存在较强的相关性,比如白葡萄中的总糖和还原糖对白葡萄酒中顺式白藜芦醇苷和顺式白藜芦醇以及反式白藜芦醇的影响较大。
在判断葡萄与葡萄酒的理化指标与葡萄酒的质量间关系的问题中,首先对葡萄和葡萄酒的理化指标与葡萄酒的质量进行了相关性分析,发现某些理化指标与葡萄酒的质量相关性很大。
2012数学建模优秀论文A题(借鉴着去写摘要).

基于系统综合评价的城市表层土壤重金属污染分析摘要本文针对城市表层土壤重金属污染问题,首先对各重金属元素进行分析,然后对各种重金属元素的基本数据进行统计分析及无量纲化处理,再对各金属元素进行相关性分析,最后针对各个问题建立模型并求解。
针对问题一,我们首先利用EXCEL 和 SPSS 统计软件对各金属元素的数据进行处理,再利用Matlab 软件绘制出该城区内8种重金属元素的空间分布图最后通过内梅罗污染模型:2/12max22⎪⎪⎭⎫ ⎝⎛+=P P P 平均综,其中平均P 为所有单项污染指数的平均值,m ax P 为土壤环境中针对问题二,我们首先利用EXCELL 软件画出8种元素在各个区内相对含量的柱状图,由图可以明显地看出各个区内各种元素的污染情况,然后再根据重金属元素污染来源及传播特征进行分析,可以得出工业区及生活区重金属的堆积和迁移是造成污染的主要原因,Cu 、Hg 、Zn 主要在工业区和交通区如公路、铁路等交通设施的两侧富集,随时间的推移,工业区、交通区的土壤重金属具有很强的叠加性,受人类活动的影响较大。
同时城市人口密度,土地利用率,机动车密度也是造成重金属污染的原因。
针对问题三,我们从两个方面考虑建模即以点为传染源和以线为传染源。
针对以点为传染源我们建立了两个模型:无约束优化模型()[]()[]()22y i y x i x m D -+-=,得到污染源的位置坐标()6782,5567;有衰减的扩散过程模型得位置坐标(8500,5500),模型为:u k zu c y u b x u a h u 2222222222-∂∂+∂∂+∂∂=∂∂, 针对以线为传染源我们建立了l c be u Y ∆-+=0模型,并通过线性拟合分析线性污染源的位置。
针对问题四,我们在已有信息的基础上,还应收集不同时间内的样点对应的浓度以及各污染源重金属的产生率。
根据高斯浓度模型建立高斯修正模型,得到浓度关于时间和空间的表达式ut e C C -⋅=0。
2012大学生英语竞赛A类决赛真题及详解
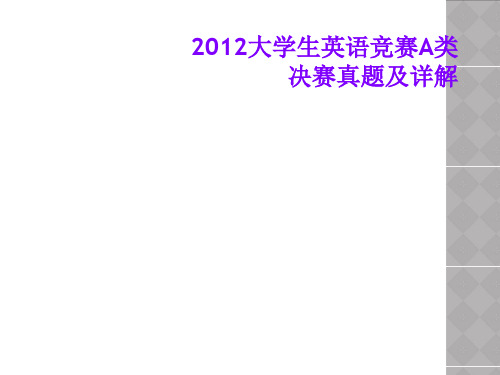
What does the man think of David? A. He thinks David is the best candidate. B. He doesn’t think David has a strong personality. C. He doesn’t think David is ideal for the project.
choosing actors, writing scripts to designing the costumes and even producing the brochure. M: Sounds like a recipe for chaos to me!
Where does the conversation probably take place? A. In a hospital. B. In a spa. C. In a gym.
Where can the woman get the details about the application? A. From the university newspaper. B. From the booklet the man offers. C. From the email the man will send her.
What does the man think of the drama club? A. It helps students in many ways. B. It needs proper management. C. It should be run by students.
【听力原文】 M: Are the teachers involved in your drama club? W: Not at all. Our club is in charge of everything, from
2012年数模国赛A题葡萄酒

承诺书我们仔细阅读了中国大学生数学建模竞赛的竞赛规则.我们完全明白,在竞赛开始后参赛队员不能以任何方式(包括电话、电子邮件、网上咨询等)与队外的任何人(包括指导教师)研究、讨论与赛题有关的问题。
我们知道,抄袭别人的成果是违反竞赛规则的, 如果引用别人的成果或其他公开的资料(包括网上查到的资料),必须按照规定的参考文献的表述方式在正文引用处和参考文献中明确列出。
我们郑重承诺,严格遵守竞赛规则,以保证竞赛的公正、公平性。
如有违反竞赛规则的行为,我们将受到严肃处理。
我们授权全国大学生数学建模竞赛组委会,可将我们的论文以任何形式进行公开展示(包括进行网上公示,在书籍、期刊和其他媒体进行正式或非正式发表等)。
我们参赛选择的题号是(从A/B/C/D中选择一项填写): A我们的参赛报名号为(如果赛区设置报名号的话):所属学校(请填写完整的全名):参赛队员(打印并签名) :1.2.3.指导教师或指导教师组负责人(打印并签名):日期:年月日赛区评阅编号(由赛区组委会评阅前进行编号):编号专用页赛区评阅编号(由赛区组委会评阅前进行编号):赛区评阅记录(可供赛区评阅时使用):评阅人评分备注全国统一编号(由赛区组委会送交全国前编号):全国评阅编号(由全国组委会评阅前进行编号):A 题 葡萄酒的评价摘要本文探讨的是判定两组评价结果有无显著性差异,研究葡萄分级,葡萄与葡萄酒理化指标间联系,酿酒葡萄和葡萄酒的理化指标对葡萄酒质量的影响的问题。
首先,不同的两组的评酒员对一种葡萄酒进行品评打分时,打分的高低不同既可能源于不同组的评酒员的差别,也可能源于组内评酒员的差别。
这种特征符合单因素方差分析法的适用问题。
因此,可以利用统计学中单因素方差分析的方法,对每一种酒以组别为控制变量,打分值为观测变量,利用EXCEL2007自带的单因素方差分析功能求解出F 检验值和P 值,查表得F 临界值,在给定05.0=α的检验水平下,若临界值F F >,P<0.05则判断控制变量给观测变量带来了显著影响。
- 1、下载文档前请自行甄别文档内容的完整性,平台不提供额外的编辑、内容补充、找答案等附加服务。
- 2、"仅部分预览"的文档,不可在线预览部分如存在完整性等问题,可反馈申请退款(可完整预览的文档不适用该条件!)。
- 3、如文档侵犯您的权益,请联系客服反馈,我们会尽快为您处理(人工客服工作时间:9:00-18:30)。
1)控制树叶的形状是多基因决定的;
2)正常情况下,树叶是由主基因完全达;
3)主基因的贡献率达到70%到80%左右;
4)每一片叶上的微效基因都不少于20种 ;
5)树叶存在微效基因,单个微效基因部分 表达;
6)大量的微效基因会积累导致叶子形状不 一样。
微效基因的贡献率相同的概率大约为:
p 10%10%L 10% ( 1 )20 10
的轮廓的一ቤተ መጻሕፍቲ ባይዱ比较
为了判断我们的猜想是正确的,我们的模 型的总体想法是比较一些特定参数的树 之间剖面和树叶的形状,从而确定是否存 在关系。
5.3 此时我们把树也看成二维的图, 把模型一的方法简化,只考虑其中权 值大的三个因素
1)Rectangularity 2)Aspect ratio 3)Circularity
因为p趋于0,所以微效基因的表达是不可 能相同的,也就是说一棵树上没有相同的 两片树叶。并且往往每片树叶上的微效基 因都是大于20种,这就使得相同的概率P 更小。综上述,世界上是没有两片完全相 同的树叶。
2、决定树叶不同形状的外部因素分析:
查找资料显示,树叶形状和结构,与制造 叶子所需的碳量,叶子的寿命和叶子进行 光合作用的速率,这三个基本要素有关 。
Task2: Do the shapes “minimize” overlapping individual shadows that are cast, so as to maximize exposure? Does the distribution of leaves within the “volume” of the tree and its branches effect the shape? 问题二:是不是树叶之间减少遮挡,来获 得最大的光照,叶子的形状和树的体积及 枝干分布有关系吗?
问题四:怎么估计一棵树上树叶的质量?
二、赏析Outstanding论文
1、问题的分解
1)Classification the different types of leaves 各类树叶的分类
2)Relationship between the leaf distribution and leaf shap 叶子的分布和叶子的形状的关系
4.2为了简化模型,我们使用菱形,主轴的 长度来标示Lmajorand长度的短轴指示 为Lminor,来取代树叶的形状。
4.3当遮挡比E为0的时候
4.4模型的检验
5.模型三:树的轮廓和树叶的关系
5.1我们有一个原始的猜想,树叶的形状是 一个二维模拟树的轮廓。
5.2 Comparison of Leaf Shape and Tree Contour,叶子的形状和树
6、模型四:一棵树上树叶的质量
6.1 Leaf Mass and Tree Age 1)Leaf Mass and CO2 Sequestration 2)CO2 Sequestration and Tree Age
6.2 Leaf Mass and Tree Size
6.2 Tree Age and Tree Size
3、从叶脉分析
叶脉决定着树叶的一切,为树叶提供结 构性的支撑,抵御侵害,,传输养分,甚 至将化学信号传给植物。一些科学家们所 建立的模型正是通对综合上述的决定性因 素——光合作用速率、树叶寿命、碳消耗 甚至氮消耗之间的关系。直接引用参考文 献的内容。
模型二: 1、叶子获得最大光照的分析
由于叶子具有叶镶嵌状态,叶镶嵌是同一 个枝上的叶不论是那一种叶序,叶总是不 相重叠而成镶嵌状态进行排列的现象
3)Relationship between the tree profile and leaf shape 树的轮廓和叶子的形状的关系
4)Calculation of the total leaf mass on a leaf 计算树叶的质量
2、问题的假设
2.1假设所有的树都开放生长,不密集生长和孤立生长
模型三: 叶子的分布对叶子的形状的影响模型
在一棵树上 ,决定叶子的分布的因素有, 叶子的疏密程度,叶子的空间置,叶龄。
叶子的疏密程度影响叶子周围的二氧化 碳的浓度,叶子的空间位置决定了叶子接 收阳光的光照强度及生长速率,同时也影 响叶子周围的二氧化碳浓度,叶子的年龄 决定了叶子生长的时间,叶子的生长时间 影响叶子的大小形状。
那么我们可以得到:
n
S0
d
2 j
(i
0,1,
3,
4...)
j 1
S0 D2
由于树上同一级的树叶的叶柄半径近似 相等,并且树叶一般都在树枝的末端, 那么树叶的数量近似为:
n D d
2、求解单片树叶的质量 (1)我们可以用天枰测出任意数q片树叶的质 量m1,然后计算出每片的质量m2
m2=m1/ q (2)我们可以测出每片树叶的宽和长,根据计 算公式: s 1.2(s sW) 用叶柄直径近似认为是叶片的厚度,就可以 得到体积:
2012年国际赛A题论 文
对比赏析
主要内容:
一、2012年国际赛赛题讲解 二、赏析Outstanding论文 三、我们组论文的阅读 四、总结
一、2012年国际赛赛题讲解
Task1 :explain why do leaves have the various shapes that they have? 问题一:解释为什么树叶有那么多的形状?
3.12 权重的求解
求得的权重: CR=0.0064<0.001
3.13 Model Testing ,模型的检验
检验的结果: 检验结果表明,该树叶属于第四类
4、模型二:叶子的分布和形状的关系
4.1Idealized Leaf Distribution Model,理想化叶分布
4.1.1我们假设树垂直地面,直指天空; 4.1.2我们假设这棵树是放置在纬度的L(北方)。 因此最大的一年平均太阳能的高度,这是获得 在中午春分,被定义为α。
6) Edge regularity perimeter index
7) Proportional index
3.2 Rectangularity,树叶的面 积和树叶边框矩形的面积之比
3.3 Aspect ratio,树叶边 框矩形的宽长比
3.4 Circularity,树叶内切 圆和外截圆的半径之比
3.5 Form factor,形状因子,一个著名 的形状描述参数,是另一个基本指标的 叶分类。最大可能值是1的参数。叶子 面积和叶子周长的比值乘以常数。
3.6 Edge regularity area index, 叶子的面积和轮廓多边形周长比值
3.7Edge regularity perimeter index,轮廓的多边形周长和树叶 周长的比,最大值为1
P is the general profile of a tree(volume, height, mass, diameter).
A is the age of the tree.
k1, k2, k3, k4, k5 and k6 are constants.
三、我们组论文的阅读
模型一: 1、树叶不同形状的分析模型
2.2假设树叶不反应树的特殊功能,仅仅是为了树进行光 合作用和呼吸作用
2.3假设树叶的分布仅仅是植物向光性的一种自然表现。
2.4假设树的轮廓只包含树在地面上的部分
2.5假设比较树叶的不同,忽略其表面的凸凹不平的叶脉 2.6假设的叶子是的树唯一反应光合作用和呼吸作用,储 存二氧化碳的部分 2.7假设一棵树储存碳量是一片叶子净吸收的二氧化碳量 2.8假设树木在健康、成熟和稳定的条件生长。同一物种 的树木有相似的特点
V=d*s 不同的树的树叶有不同的密度p,p也与叶子 的含水量有关系,但p一般稍小于水密度, 近似值的取为0.9.
m2=p*v
3、一棵树上的树叶的总质量求解: 我们通过以上计算算出了每棵树上的树叶个数, 也算出了一片树叶的质量,所以总的质量M
3.8 Proportional index,将 叶子在垂直叶子主脉四等分
3.9 现在,我们可以开发一个数据库的六个 最常见的叶子上面讨论包含了七参数。
3.10 6种北美常见的树叶的10个数据
3.11 当给一个特定的叶,我们可以计算出它 的七个特征,并与我们的数据库通过计算的 平方偏差的每个参数的给定的叶子从相应的 标准参数的每个类别。考虑到有些参数分辨 树叶的能力不一样,所以加入权重。
我们设定树叶形状复杂度的目标函数为y, 碳含量的变化度为a,叶子寿命的变化度为 b,光合作用速率的变化度为c。a为叶子 中碳含量在50.0%到52%的可能的取值的 个数,b为叶子寿命在几月到几十年可能的 取值的个数,c为叶子光合作用速率可能取 值的个数,也就是说a,b,c是很大的值。 那么
y=abc y就更大了,也就是说树叶的形状复杂度很 高,也就是说几乎没有两片树的叶子是一 样的。
模型四: 测定一个树上树叶的质量
假设树木自然生长,没有受到人为修剪 和破坏; 假设树木的生长过程,没有受到重大灾 难,如:干旱,洪涝,病虫等 。
达芬奇树干算法公式告诉我们: 大树主干的横截面积=下级分支树干截面积之和
n
Si S(i1) j (i 0,1, 3, 4...) j 1
1、如果小树的每一个分支都是叶子的叶柄,
Task3: Speaking of profiles, is leaf shape related to tree (branching profile structure)?
问题三:简而言之,树叶的形状和树的轮廓 (枝干分布)有关系吗?
Task4:How would you estimate the leaf mass of a tree ?
5、当阳光强度一定和树木的树叶数目相 同的时候,倒锥型轮廓的树,阳光损失量 最大,正锥型的阳光损失量较小,球形和 椭球形的损失介于二者之间,圆柱形损失 最小。那么一般来说圆柱的树叶平均生长 速率大于真锥形球形和椭球型大于倒锥形, 叶子的形状肯定就不一样了。