Application of Neural Networks and Statistical Pattern Recognition Algorithms Abstract to E
东北大学博士生发表学术论文量化标准(毕业标准)(2011版)
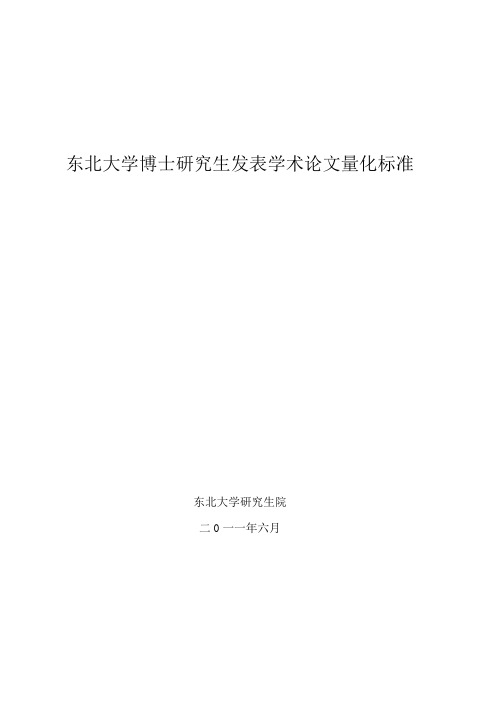
东北大学博士研究生发表学术论文量化标准东北大学研究生院二0一一年六月关于对博士生发表学术论文量化标准修订的说明博士研究生在读期间发表学术论文的质量是研究生培养质量和学位授予质量的重要标志之一。
根据东北大学学位评定委员会九届一次会议精神,决定以一级学科为单位修订博士研究生在读期间发表学术论文量化标准。
学院、学科点结合相关学科发展的新趋势和有关刊物、国际学术会议的规格水平,本着质量为重的原则,认真研究、确定了我校博士研究生在读期间发表学术论文的量化标准。
请有关学院、博士学位授权点和博士生指导教师依据该标准鼓励博士生在高水平的学术期刊上发表文章,从而不断提高博士生培养质量。
该量化标准从2012年10月及以后申请学位的博士生开始实施。
哲学学科1. 重点认定刊物凡被SCI、EI、ISTP 、SSCI、A&HCI检索的学术论文;被《新华文摘》、《新华月报》全文转载或者论点摘编;被《中国社会科学文摘》、《中国人民大学书刊复印资料》、《中国高等学校文科学术文摘》全文转载的学术论文;发表在《人民日报》、《光明日报》、《中国教育报》、《科技日报》、《法制日报》、《经济日报》理论版的学术论文;发表在当年CSSCI来源期刊上的学术论文。
2.普通认定刊物凡发表在当年CSSCI扩展版来源期刊上的学术论文;北京大学图书馆和北京高校图书馆期刊工作研究会合编的当年《中文核心期刊要目总览》中所列期刊发表的学术论文。
博士生答辩前必须在公开刊物(不含增刊和专辑)上发表至少3篇与博士学位论文内容相关的学术论文,其中至少有1篇发表在重点认定刊物上。
说明:(1)在国外哲学、人文社会科学学术期刊上发表与博士学位论文相关的外文文章,是否视为重点刊物论文,由本学科导师组认定;(2)申请博士学位的公开发表的学术论文,必须以东北大学为第一作者署名单位(包括博士生本人为第一作者和以导师为第一作者博士生为第二作者两种情况);(3)对于待发表的多篇论文按1篇计算,但必须有发表论文接收证明;(4)除被SCI、EI、ISTP 、SSCI、A&HCI检索的学术论文之外,所有发表在“增刊”上的学术论文一律视为一般公开发表的学术论文。
课文参考译文 (14)-信息科学与电子工程专业英语(第2版)-吴雅婷-清华大学出版社

Unit 14 计算机和网络Unit 14-1第一部分:计算机的进展计算机和信息技术的进展计算机和信息技术的诞生可以追溯到许多世纪以前。
数学的发展引起了计算工具的发展。
据说17世纪法国的Blaise Pascal构建了第一台计算机。
在19世纪,常被推崇为计算之父的英国人Charles Babbage设计了第一台“分析机”。
该机器有一个机械的计算“工厂”,类似于19世纪早期的提花织布机,采用穿孔卡片来存储数字和处理要求。
Ada Lovelace和他(Charles Babbage)致力于设计并提出了指令序列的概念——程序。
到1871年Babbage逝世,这台机器还没有完成。
将近一个世纪以后,随着电子机械计算机的发展(程序)这一概念再次出现。
1890年,Herman Hollerith采用穿孔卡片帮助美国人口普查局分类信息。
与此同时,电报电话的发明为通信和真空管的发展奠定了基础。
这一电子器件能够用于存储二进制形式的信息,即开或关,1或0。
第一台数字电子计算机ENIAC(电子计数积分计算机,见图14.1)是为美国军队开发的,并于1946年完成。
普林斯顿的数学教授V on Neumann对(程序)这一概念作了进一步深入的研究,加入了存储计算机程序的思想。
这就是存储在计算机内存中的指令序列,计算机执行这些指令完成程序控制的任务。
图14.1 ENIAC:第一台数字化电子计算机从这一阶段开始,计算机和计算机编程技术迅速发展。
从真空管发展到晶体管,大大减小了机器(计算机)的尺寸和成本,并提高了可靠性。
接着,集成电路技术的出现又减小了计算机的尺寸(和成本)。
20世纪60年代,典型的计算机是基于晶体管的机器,价值50万美金,并需要一个大空调房和一名现场工程师。
现在相同性能的计算机只要2000美元,并且放在桌上(就可使用了)。
随着计算机越来越小,越来越便宜,计算速度也更快——通过叫做芯片的单个集成电路来实现。
微处理器和微型计算机的发展微型计算机随着集成电路(或芯片)技术的发展而发展。
人工智能和机器学习的会议公告的英语作文

人工智能和机器学习的会议公告的英语作文全文共6篇示例,供读者参考篇1Conference Announcement: Future Robots & Smart Computers!Hey kids! Are you curious about robots, computers that can think like humans, and really cool technology? Then you won't want to miss the "Future Robotics and Machine Learning" conference happening next month at the Cityville Convention Center!What is Machine Learning?Machine learning is a way for computers to learn and get smarter without being directly programmed by humans. Instead of just following instructions, machine learning algorithms can look at data and learn patterns and rules on their own. It's like teaching a computer to think for itself!With machine learning, computers can do amazing things like:• Recognize objects, faces, and even emotions in photos and videos• Understand human speech and translate between languages• Make predictions about things like the weather or what movies you might like• Learn to play games better than any human• And much, much more!Machine learning is used in lots of really cool modern technologies like self-driving cars, smart home assistants like Alexa and Siri, and movie/TV show recommendations on streaming services. It helps make our lives easier and more convenient.What About Robots?While not exactly the human-like robots you see in movies, modern robots are still incredibly advanced machines that can perform complex tasks. Many robots use machine learning algorithms to operate. For example:• Factory robots can use machine vision to inspect products and identify defects• Surgical robots can assist do ctors with precise medical procedures• Exploratory robots can navigate across other planets like Mars• And home robot vacuums use sensors and mapping to clean your floors!Robots are great for automating dangerous, repetitive, or just plain boring jobs that humans shouldn't have to do. And with machine learning, they are only getting smarter and more capable.What Will Happen at the Conference?This conference is all about the latest developments in robotics, artificial intelligence (AI), and machine learning technology. Lots of cool demos, exhibits, and activities will be happening, like:• Getting to interact with real robots and see them in action• Watching experts give presentations about new AI breakthroughs• Learning how to code your own simple machine learning models• Checking out student projects that use AI and robotics• Talking to scientists and asking them your burning AI questions• Participating in fun, AI-themed games and contests• And more!You'll get to explore awesome new technologies that seem like science fiction, but are very much the science fact of the future. Who knows, you might be inspired to become an AI scientist or robotics engineer yourself someday!When and Where?The Future Robotics & Machine Learning conference will take place on June 15-17 at the Cityville Convention Center downtown. It runs from 10am to 6pm each day. Admission is 5 for students with plenty of fun activities and exhibits included.Parents, teachers, adults - you're welcome too! While targeted towards kids, this conference should be an engaging and educational experience for AI/tech enthusiasts of all ages.Don't miss out on this chance to get a glimpse of the mind-blowing and world-changing capabilities of artificial intelligence and robotics. Who knows what awesomeinnovations the machines of tomorrow will bring? Come find out by joining us at the Future Robotics & Machine Learning conference!See you there!篇2人工智能和机器学习大会公告亲爱的同学们,大家好!我是小班班,非常高兴地向大家宣布一个重要的消息。
Palo Alto Networks Cortex XSOAR Threat Intelligenc
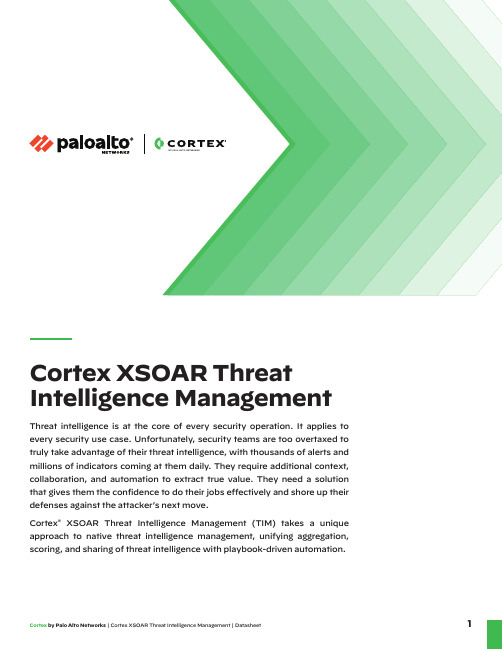
Cortex XSOAR ThreatI ntellig ence Manag ementThreat intelligence is at the core of every security operation. It applies to every security use case. Unfortunately, security teams are too overtaxed to truly take advantage of their threat intelligence, with thousands of alerts and millions of indicators coming at them daily. They require additional context, collaboration, and automation to extract true value. They need a solution that gives them the confidence to do their jobs effectively and shore up their defenses against the attacker’s next move.Cortex® X SOAR Threat Intelligence Management (TIM) takes a unique approach to native threat intelligence management, unifying aggregation, scoring, and sharing of threat intelligence with playbook-driven automation.Features and Capabilities Powerful, native centralized threat intel : Supercharge i nvestigations with instant access to the massive repository of built-in, high-fidelity Palo Alto Networks threat intelli -gence crowdsourced from the largest footprint of network, endpoint, and cloud intel sources (Tens of millions of mal -ware samples collected and firewall sessions analyzed daily).Indicator relationships : Indicator connections enable struc -tured relationships to be created between threat intelligence sources and incidents. These relationships surface importantcontext for security analysts on new threat actors and attack techniques.Hands-free automated playbooks with extensible integra-tions : Take automated action to shut down threats across more than 600 third-party products with purpose-built p laybooks based on proven SOAR capabilities.Granular indicator scoring and management : Take charge of your threat intel with playbook-based indicator lifecycle man -agement and transparent scoring that can be extended and customized with ease.Automated, multi-source feed aggregation : Eliminate manual tasks with automated playbooks to aggregate, parse, prioritize, and distribute relevant indicators in real time to security con -trols for continuous protection.Most comprehensive marketplace : The largest community of integrations with content packs that are prebuilt bundles of integrations, playbooks, dashboards, field subscription services, and all the dependencies needed to support specific security orchestration use cases. With 680+ prebuilt content packs of which 700+ are product integrations, you can buy i ntel on the go using Marketplace points.Business Value Figure 1:Control, enrich, and take actionwith playbook-driven automation Take Full Control Take complete control of your threat intelligence feeds Enrich Incident Response Make smarter incident response decisions by enriching every tool and process Actionable IntelClose the loop between intelligence and action withplaybook-driven automationFigure 2: Take control of your threat intel feedFigure 3:Make smarter decisions by enriching and prioritizing indicatorsFigure 4: Close the loop between intel and action with automationThreat Intelligence Combined with SOAR Security orchestration, automation, and response (SOAR) solutions have been developed to more seamlessly weave threat intelligence management into workflows by combin -ing TIM capabilities with incident management, orchestra -tion, and automation capabilities. Organizations looking for a threat intelligence platform often look for SOAR solutions that can weave threat intelligence into a more unified andautomated workflow—one that matches alerts both to their sources and to compiled threat intelligence data and that can automatically execute an appropriate response.As part of the extensible Cortex XSOAR platform, threat intel management unifies threat intelligence aggregation, scoring, and sharing with playbook-driven automation. It empowers security leaders with instant clarity into high-priority threats to drive the right response, in the right way, across the entire enterprise.way. Automated data enrichment of indicators provides ana-lysts with relevant threat data to make smarter decisions. Integrated case management allows for real-time collabora-tion, boosting operational efficiencies across teams, and auto-mated playbooks speed response across security use cases. Key Use CasesUse Case 1: Proactive Blocking of Known ThreatsChallengeThe security team needs to leverage threat intelligence to block or alert on known bad domains, IPs, hashes, etc. (indicators). The indicators are being collected from many different s ources, which need to be normalized, scored, and analyzed before the customer can push to security devices such as SIEM and firewall for alerting. Detection tools can only handle l imited amounts of threat intelligence data and need to constantly re-prioritize indicators.SolutionIndicator prioritization. Palo Alto Networks Threat Intelligence Management can ingest phishing alerts from email i nboxes through integrations. Once an alert is ingested, a playbook is Use Case 2: Dynamic Allow/Deny ListA dministratio nChallengeManual process for allow/deny lists. Managing a single allow list and updating across the enterprise can involve updating dozens of network devices. Security teams often have to liaise with firewall admins, IT teams, DevOps, and other teams to execute some parts of incident response.SolutionEliminate downtime by using automated playbooks to e xtract valid IP addresses and URLs to exclude from enforce-ment point EDLs, ensuring employees have access to these b usiness-critical applications at all times.Use Case 3: Cross-Functional IntelligenceS haringChallengeIntelligence sharing is unstructured. Most intelligence is still shared via unstructured formats such as email, PDF, blogs, etc. Sharing indicators of compromise is not enough. A dditional context is required for the shared intelligence to have value.Internal alerts3000 Tannery WaySanta Clara, CA 95054 Main: +1.408.753.4000 Sales: +1.866.320.4788 Support: +1.866.898.9087© 2021 Palo Alto Networks, Inc. Palo Alto Networks is a registeredt rademark of Palo Alto Networks. A list of our trademarks can be found at https:///company/trademarks.html. All other marks mentioned herein may be trademarks of their respective companies. cortex_ds_xsoar-threat-intelligence-management_062221SolutionIndicator connections enable structured relationships to be created between threat intelligence sources. These relation-ships surface important context for security analysts, threat analysts, and other incident response teams, who can collab-orate and resolve incidents via a single platform.Industry-Leading CustomerS uccessOur Customer Success team is dedicated to helping you get the best value from your Cortex XSOAR investments and giving you the utmost confidence that your business is safe. Here are our plans:• Standard Success, included with every Cortex XSOAR sub-scription, makes it easy for you to get started. You’ll have access to self-guided materials and online support tools to get you up and running quickly.• Premium Success, the recommended plan, includes every-thing in the Standard plan plus guided onboarding, custom workshops, 24/7 technical phone support, and access to the Customer Success team to give you a personalized ex-perience to help you realize optimal return on investment (ROI).Flexible DeploymentCortex XSOAR can be deployed on-premises, in a private cloud, or as a fully hosted solution. We offer the platform in multiple tiers to fit your needs.。
神经网络的研究和应用(英文文献)
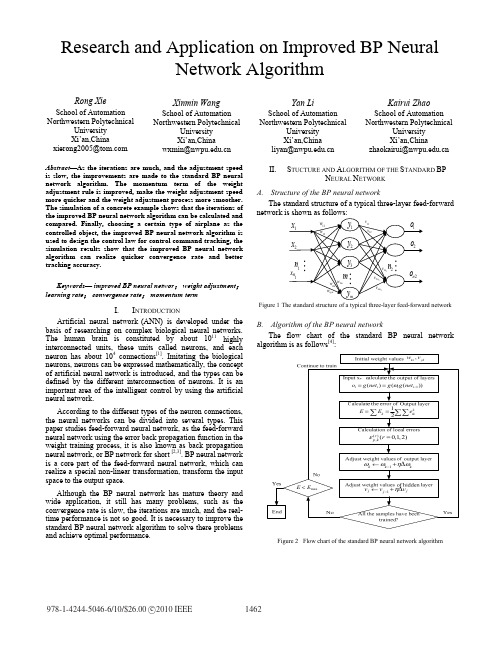
network is shown as follows:
x1
w11 w12
y1
v11 v12
o1
x2
y2
o2
# xn1n1
y3
m# w2m m
wn1m
ym
#n v1n2 2
v3n2
on2
vmn2
Figure 1 The standard structure of a typical three-layer feed-forward network
1462
III. IMPROVEMENT OF THE STANDARD BP NEURAL NETWORK ALGORITHM
The convergence rate of the standard BP algorithm is slow, and the iterations of the standard BP algorithm are much, they all have negative influences on the rapidity of the control system. In this paper, improvement has been made to the learning rate of the standard BP algorithm to accelerate the training speed of the neural network.
From formula (1), the learning rate η influences the
weight adjustment value ǻW(n), and then influences the convergence rate of the network. If the learning rate η is too
基于属性增强的神经传感融合网络的人脸识别算法论文
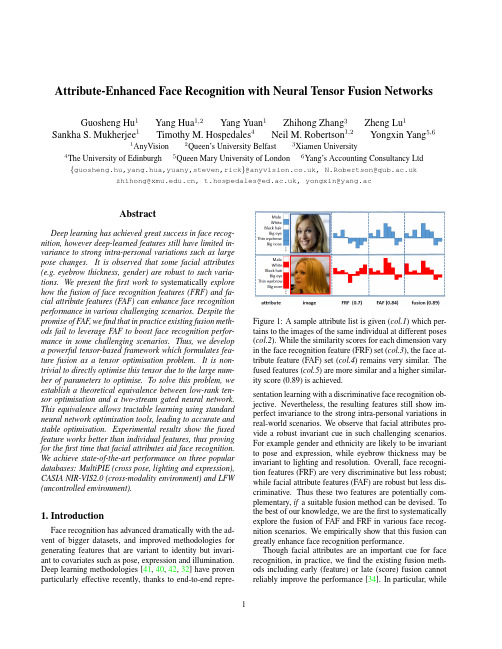
Attribute-Enhanced Face Recognition with Neural Tensor Fusion Networks Guosheng Hu1Yang Hua1,2Yang Yuan1Zhihong Zhang3Zheng Lu1 Sankha S.Mukherjee1Timothy M.Hospedales4Neil M.Robertson1,2Yongxin Yang5,61AnyVision2Queen’s University Belfast3Xiamen University 4The University of Edinburgh5Queen Mary University of London6Yang’s Accounting Consultancy Ltd {guosheng.hu,yang.hua,yuany,steven,rick}@,N.Robertson@ zhihong@,t.hospedales@,yongxin@yang.acAbstractDeep learning has achieved great success in face recog-nition,however deep-learned features still have limited in-variance to strong intra-personal variations such as large pose changes.It is observed that some facial attributes (e.g.eyebrow thickness,gender)are robust to such varia-tions.We present thefirst work to systematically explore how the fusion of face recognition features(FRF)and fa-cial attribute features(FAF)can enhance face recognition performance in various challenging scenarios.Despite the promise of FAF,wefind that in practice existing fusion meth-ods fail to leverage FAF to boost face recognition perfor-mance in some challenging scenarios.Thus,we develop a powerful tensor-based framework which formulates fea-ture fusion as a tensor optimisation problem.It is non-trivial to directly optimise this tensor due to the large num-ber of parameters to optimise.To solve this problem,we establish a theoretical equivalence between low-rank ten-sor optimisation and a two-stream gated neural network. This equivalence allows tractable learning using standard neural network optimisation tools,leading to accurate and stable optimisation.Experimental results show the fused feature works better than individual features,thus proving for thefirst time that facial attributes aid face recognition. We achieve state-of-the-art performance on three popular databases:MultiPIE(cross pose,lighting and expression), CASIA NIR-VIS2.0(cross-modality environment)and LFW (uncontrolled environment).1.IntroductionFace recognition has advanced dramatically with the ad-vent of bigger datasets,and improved methodologies for generating features that are variant to identity but invari-ant to covariates such as pose,expression and illumination. Deep learning methodologies[41,40,42,32]have proven particularly effective recently,thanks to end-to-endrepre-Figure1:A sample attribute list is given(col.1)which per-tains to the images of the same individual at different poses (col.2).While the similarity scores for each dimension vary in the face recognition feature(FRF)set(col.3),the face at-tribute feature(FAF)set(col.4)remains very similar.The fused features(col.5)are more similar and a higher similar-ity score(0.89)is achieved.sentation learning with a discriminative face recognition ob-jective.Nevertheless,the resulting features still show im-perfect invariance to the strong intra-personal variations in real-world scenarios.We observe that facial attributes pro-vide a robust invariant cue in such challenging scenarios.For example gender and ethnicity are likely to be invariant to pose and expression,while eyebrow thickness may be invariant to lighting and resolution.Overall,face recogni-tion features(FRF)are very discriminative but less robust;while facial attribute features(FAF)are robust but less dis-criminative.Thus these two features are potentially com-plementary,if a suitable fusion method can be devised.To the best of our knowledge,we are thefirst to systematically explore the fusion of FAF and FRF in various face recog-nition scenarios.We empirically show that this fusion can greatly enhance face recognition performance.Though facial attributes are an important cue for face recognition,in practice,wefind the existing fusion meth-ods including early(feature)or late(score)fusion cannot reliably improve the performance[34].In particular,while 1offering some robustness,FAF is generally less discrimina-tive than FRF.Existing methods cannot synergistically fuse such asymmetric features,and usually lead to worse perfor-mance than achieved by the stronger feature(FRF)only.In this work,we propose a novel tensor-based fusion frame-work that is uniquely capable of fusing the very asymmet-ric FAF and FRF.Our framework provides a more powerful and robust fusion approach than existing strategies by learn-ing from all interactions between the two feature views.To train the tensor in a tractable way given the large number of required parameters,we formulate the optimisation with an identity-supervised objective by constraining the tensor to have a low-rank form.We establish an equivalence be-tween this low-rank tensor and a two-stream gated neural network.Given this equivalence,the proposed tensor is eas-ily optimised with standard deep neural network toolboxes. Our technical contributions are:•It is thefirst work to systematically investigate and ver-ify that facial attributes are an important cue in various face recognition scenarios.In particular,we investi-gate face recognition with extreme pose variations,i.e.±90◦from frontal,showing that attributes are impor-tant for performance enhancement.•A rich tensor-based fusion framework is proposed.We show the low-rank Tucker-decomposition of this tensor-based fusion has an equivalent Gated Two-stream Neural Network(GTNN),allowing easy yet effective optimisation by neural network learning.In addition,we bring insights from neural networks into thefield of tensor optimisation.The code is available:https:///yanghuadr/ Neural-Tensor-Fusion-Network•We achieve state-of-the-art face recognition perfor-mance using the fusion of face(newly designed‘Lean-Face’deep learning feature)and attribute-based fea-tures on three popular databases:MultiPIE(controlled environment),CASIA NIR-VIS2.0(cross-modality environment)and LFW(uncontrolled environment).2.Related WorkFace Recognition.The face representation(feature)is the most important component in contemporary face recog-nition system.There are two types:hand-crafted and deep learning features.Widely used hand-crafted face descriptors include Local Binary Pattern(LBP)[26],Gaborfilters[23],-pared to pixel values,these features are variant to identity and relatively invariant to intra-personal variations,and thus they achieve promising performance in controlled environ-ments.However,they perform less well on face recognition in uncontrolled environments(FRUE).There are two main routes to improve FRUE performance with hand-crafted features,one is to use very high dimensional features(dense sampling features)[5]and the other is to enhance the fea-tures with downstream metric learning.Unlike hand-crafted features where(in)variances are en-gineered,deep learning features learn the(in)variances from data.Recently,convolutional neural networks(CNNs) achieved impressive results on FRUE.DeepFace[44],a carefully designed8-layer CNN,is an early landmark method.Another well-known line of work is DeepID[41] and its variants DeepID2[40],DeepID2+[42].The DeepID family uses an ensemble of many small CNNs trained in-dependently using different facial patches to improve the performance.In addition,some CNNs originally designed for object recognition,such as VGGNet[38]and Incep-tion[43],were also used for face recognition[29,32].Most recently,a center loss[47]is introduced to learn more dis-criminative features.Facial Attribute Recognition.Facial attribute recog-nition(FAR)is also well studied.A notable early study[21] extracted carefully designed hand-crafted features includ-ing aggregations of colour spaces and image gradients,be-fore training an independent SVM to detect each attribute. As for face recognition,deep learning features now outper-form hand-crafted features for FAR.In[24],face detection and attribute recognition CNNs are carefully designed,and the output of the face detection network is fed into the at-tribute network.An alternative to purpose designing CNNs for FAR is tofine-tune networks intended for object recog-nition[56,57].From a representation learning perspective, the features supporting different attribute detections may be shared,leading some studies to investigate multi-task learn-ing facial attributes[55,30].Since different facial attributes have different prevalence,the multi-label/multi-task learn-ing suffers from label-imbalance,which[30]addresses us-ing a mixed objective optimization network(MOON). Face Recognition using Facial Attributes.Detected facial attributes can be applied directly to authentication. Facial attributes have been applied to enhance face verifica-tion,primarily in the case of cross-modal matching,byfil-tering[19,54](requiring potential FRF matches to have the correct gender,for example),model switching[18],or ag-gregation with conventional features[27,17].[21]defines 65facial attributes and proposes binary attribute classifiers to predict their presence or absence.The vector of attribute classifier scores can be used for face recognition.There has been little work on attribute-enhanced face recognition in the context of deep learning.One of the few exploits CNN-based attribute features for authentication on mobile devices [31].Local facial patches are fed into carefully designed CNNs to predict different attributes.After CNN training, SVMs are trained for attribute recognition,and the vector of SVM scores provide the new feature for face verification.Fusion Methods.Existing fusion approaches can be classified into feature-level(early fusion)and score-level (late fusion).Score-level fusion is to fuse the similarity scores after computation based on each view either by sim-ple averaging[37]or stacking another classifier[48,37]. Feature-level fusion can be achieved by either simple fea-ture aggregation or subspace learning.For aggregation ap-proaches,fusion is usually performed by simply element wise averaging or product(the dimension of features have to be the same)or concatenation[28].For subspace learn-ing approaches,the features arefirst concatenated,then the concatenated feature is projected to a subspace,in which the features should better complement each other.These sub-space approaches can be unsupervised or supervised.Un-supervised fusion does not use the identity(label)informa-tion to learn the subspace,such as Canonical Correlational Analysis(CCA)[35]and Bilinear Models(BLM)[45].In comparison,supervised fusion uses the identity information such as Linear Discriminant Analysis(LDA)[3]and Local-ity Preserving Projections(LPP)[9].Neural Tensor Methods.Learning tensor-based compu-tations within neural networks has been studied for full[39] and decomposed[16,52,51]tensors.However,aside from differing applications and objectives,the key difference is that we establish a novel equivalence between a rich Tucker [46]decomposed low-rank fusion tensor,and a gated two-stream neural network.This allows us achieve expressive fusion,while maintaining tractable computation and a small number of parameters;and crucially permits easy optimisa-tion of the fusion tensor through standard toolboxes. Motivation.Facial attribute features(FAF)and face recognition features(FRF)are complementary.However in practice,wefind that existing fusion methods often can-not effectively combine these asymmetric features so as to improve performance.This motivates us to design a more powerful fusion method,as detailed in Section3.Based on our neural tensor fusion method,in Section5we system-atically explore the fusion of FAF and FRF in various face recognition environments,showing that FAF can greatly en-hance recognition performance.3.Fusing attribute and recognition featuresIn this section we present our strategy for fusing FAF and FRF.Our goal is to input FAF and FRF and output the fused discriminative feature.The proposed fusion method we present here performs significantly better than the exist-ing ones introduced in Section2.In this section,we detail our tensor-based fusion strategy.3.1.ModellingSingle Feature.We start from a standard multi-class clas-sification problem setting:assume we have M instances, and for each we extract a D-dimensional feature vector(the FRF)as{x(i)}M i=1.The label space contains C unique classes(person identities),so each instance is associated with a corresponding C-dimensional one-hot encoding la-bel vector{y(i)}M i=1.Assuming a linear model W the pre-dictionˆy(i)is produced by the dot-product of input x(i)and the model W,ˆy(i)=x(i)T W.(1) Multiple Feature.Suppose that apart from the D-dimensional FRF vector,we can also obtain an instance-wise B-dimensional facial attribute feature z(i).Then the input for the i th instance is a pair:{x(i),z(i)}.A simple ap-proach is to redefine x(i):=[x(i),z(i)],and directly apply Eq.(1),thus modelling weights for both FRF and FAF fea-tures.Here we propose instead a non-linear fusion method via the following formulationˆy(i)=W×1x(i)×3z(i)(2) where W is the fusion model parameters in the form of a third-order tensor of size D×C×B.Notation×is the tensor dot product(also known as tensor contraction)and the left-subscript of x and z indicates at which axis the ten-sor dot product operates.With Eq.(2),the optimisation problem is formulated as:minW1MMi=1W×1x(i)×3z(i),y(i)(3)where (·,·)is a loss function.This trains tensor W to fuse FRF and FAF features so that identity is correctly predicted.3.2.OptimisationThe proposed tensor W provides a rich fusion model. However,compared with W,W is B times larger(D×C vs D×C×B)because of the introduction of B-dimensional attribute vector.It is also almost B times larger than train-ing a matrix W on the concatenation[x(i),z(i)].It is there-fore problematic to directly optimise Eq.(3)because the large number of parameters of W makes training slow and leads to overfitting.To address this we propose a tensor de-composition technique and a neural network architecture to solve an equivalent optimisation problem in the following two subsections.3.2.1Tucker Decomposition for Feature FusionTo reduce the number of parameters of W,we place a struc-tural constraint on W.Motivated by the famous Tucker de-composition[46]for tensors,we assume that W is synthe-sised fromW=S×1U(D)×2U(C)×3U(B).(4) Here S is a third order tensor of size K D×K C×K B, U(D)is a matrix of size K D×D,U(C)is a matrix of sizeK C×C,and U(B)is a matrix of size K B×B.By restricting K D D,K C C,and K B B,we can effectively reduce the number of parameters from(D×C×B)to (K D×K C×K B+K D×D+K C×C+K B×B)if we learn{S,U(D),U(C),U(B)}instead of W.When W is needed for making the predictions,we can always synthesise it from those four small factors.In the context of tensor decomposition,(K D,K C,K B)is usually called the tensor’s rank,as an analogous concept to the rank of a matrix in matrix decomposition.Note that,despite of the existence of other tensor de-composition choices,Tucker decomposition offers a greater flexibility in terms of modelling because we have three hyper-parameters K D,K C,K B corresponding to the axes of the tensor.In contrast,the other famous decomposition, CP[10]has one hyper-parameter K for all axes of tensor.By substituting Eq.(4)into Eq.(2),we haveˆy(i)=W×1x(i)×3z(i)=S×1U(D)×2U(C)×3U(B)×1x(i)×3z(i)(5) Through some re-arrangement,Eq.(5)can be simplified as ˆy(i)=S×1(U(D)x(i))×2U(C)×3(U(B)z(i))(6) Furthermore,we can rewrite Eq.(6)as,ˆy(i)=((U(D)x(i))⊗(U(B)z(i)))S T(2)fused featureU(C)(7)where⊗is Kronecker product.Since U(D)x(i)and U(B)B(i)result in K D and K B dimensional vectors re-spectively,(U(D)x(i))⊗(U(B)z(i))produces a K D K B vector.S(2)is the mode-2unfolding of S which is aK C×K D K B matrix,and its transpose S T(2)is a matrix ofsize K D K B×K C.The Fused Feature.From Eq.(7),the explicit fused representation of face recognition(x(i))and facial at-tribute(z(i))features can be achieved.The fused feature ((U(D)x(i))⊗(U(B)z(i)))S T(2),is a vector of the dimen-sionality K C.And matrix U(C)has the role of“clas-sifier”given this fused feature.Given{x(i),z(i),y(i)}, the matrices{U(D),U(B),U(C)}and tensor S are com-puted(learned)during model optimisation(training).Dur-ing testing,the predictionˆy(i)is achieved with the learned {U(D),U(B),U(C),S}and two test features{x(i),z(i)} following Eq.(7).3.2.2Gated Two-stream Neural Network(GTNN)A key advantage of reformulating Eq.(5)into Eq.(7)is that we can nowfind a neural network architecture that does ex-actly the computation of Eq.(7),which would not be obvi-ous if we stopped at Eq.(5).Before presenting thisneural Figure2:Gated two-stream neural network to implement low-rank tensor-based fusion.The architecture computes Eq.(7),with the Tucker decomposition in Eq.(4).The network is identity-supervised at train time,and feature in the fusion layer used as representation for verification. network,we need to introduce a new deterministic layer(i.e. without any learnable parameters).Kronecker Product Layer takes two arbitrary-length in-put vectors{u,v}where u=[u1,u2,···,u P]and v=[v1,v2,···,v Q],then outputs a vector of length P Q as[u1v1,u1v2,···,u1v Q,u2v1,···,u P v Q].Using the introduced Kronecker layer,Fig.2shows the neural network that computes Eq.(7).That is,the neural network that performs recognition using tensor-based fu-sion of two features(such as FAF and FRF),based on the low-rank assumption in Eq.(4).We denote this architecture as a Gated Two-stream Neural Network(GTNN),because it takes two streams of inputs,and it performs gating[36] (multiplicative)operations on them.The GTNN is trained in a supervised fashion to predict identity.In this work,we use a multitask loss:softmax loss and center loss[47]for joint training.The fused feature in the viewpoint of GTNN is the output of penultimate layer, which is of dimensionality K c.So far,the advantage of using GTNN is obvious.Direct use of Eq.(5)or Eq.(7)requires manual derivation and im-plementation of an optimiser which is non-trivial even for decomposed matrices(2d-tensors)[20].In contrast,GTNN is easily implemented with modern deep learning packages where auto-differentiation and gradient-based optimisation is handled robustly and automatically.3.3.DiscussionCompared with the fusion methods introduced in Sec-tion2,we summarise the advantages of our tensor-based fusion method as follows:Figure3:LeanFace.‘C’is a group of convolutional layers.Stage1:64@5×5(64feature maps are sliced to two groups of32ones, which are fed into maxout function.);Stage2:64@3×3,64@3×3,128@3×3,128@3×3;Stage3:196@3×3,196@3×3, 256@3×3,256@3×3,320@3×3,320@3×3;Stage4:512@3×3,512@3×3,512@3×3,512@3×3;Stage5:640@ 5×5,640@5×5.‘P’stands for2×2max pooling.The strides for the convolutional and pooling layers are1and2,respectively.‘FC’is a fully-connected layer of256D.High Order Non-Linearity.Unlike linear methods based on averaging,concatenation,linear subspace learning [8,27],or LDA[3],our fusion method is non-linear,which is more powerful to model complex problems.Further-more,comparing with otherfirst-order non-linear methods based on element-wise combinations only[28],our method is higher order:it accounts for all interactions between each pair of feature channels in both views.Thanks to the low-rank modelling,our method achieves such powerful non-linear fusion with few parameters and thus it is robust to overfitting.Scalability.Big datasets are required for state-of-the-art face representation learning.Because we establish the equivalence between tensor factorisation and gated neural network architecture,our method is scalable to big-data through efficient mini-batch SGD-based learning.In con-trast,kernel-based non-linear methods,such as Kernel LDA [34]and multi-kernel SVM[17],are restricted to small data due to their O(N2)computation cost.At runtime,our method only requires a simple feed-forward pass and hence it is also favourable compared to kernel methods. Supervised method.GTNN isflexibly supervised by any desired neural network loss function.For example,the fusion method can be trained with losses known to be ef-fective for face representation learning:identity-supervised softmax,and centre-loss[47].Alternative methods are ei-ther unsupervised[8,27],constrained in the types of super-vision they can exploit[3,17],or only stack scores rather than improving a learned representation[48,37].There-fore,they are relatively ineffective at learning how to com-bine the two-source information in a task-specific way. Extensibility.Our GTNN naturally can be extended to deeper architectures.For example,the pre-extracted fea-tures,i.e.,x and z in Fig.2,can be replaced by two full-sized CNNs without any modification.Therefore,poten-tially,our methods can be integrated into an end-to-end framework.4.Integration with CNNs:architectureIn this section,we introduce the CNN architectures used for face recognition(LeanFace)designed by ourselves and facial attribute recognition(AttNet)introduced by[50,30]. LeanFace.Unlike general object recognition,face recognition has to capture very subtle difference between people.Motivated by thefine-grain object recognition in [4],we also use a large number of convolutional layers at early stage to capture the subtle low level and mid-level in-formation.Our activation function is maxout,which shows better performance than its competitors[50].Joint supervi-sion of softmax loss and center loss[47]is used for training. The architecture is summarised in Fig.3.AttNet.To detect facial attributes,our AttNet uses the ar-chitecture of Lighten CNN[50]to represent a face.Specifi-cally,AttNet consists of5conv-activation-pooling units fol-lowed by a256D fully connected layer.The number of con-volutional kernels is explained in[50].The activation func-tion is Max-Feature-Map[50]which is a variant of maxout. We use the loss function MOON[30],which is a multi-task loss for(1)attribute classification and(2)domain adaptive data balance.In[24],an ontology of40facial attributes are defined.We remove attributes which do not characterise a specific person,e.g.,‘wear glasses’and‘smiling’,leaving 17attributes in total.Once each network is trained,the features extracted from the penultimate fully-connected layers of LeanFace(256D) and AttNet(256D)are extracted as x and z,and input to GTNN for fusion and then face recognition.5.ExperimentsWefirst introduce the implementation details of our GTNN method.In Section5.1,we conduct experiments on MultiPIE[7]to show that facial attributes by means of our GTNN method can play an important role on improv-Table1:Network training detailsImage size BatchsizeLR1DF2EpochTraintimeLeanFace128x1282560.0010.15491hAttNet0.050.8993h1Learning rate(LR)2Learning rate drop factor(DF).ing face recognition performance in the presence of pose, illumination and expression,respectively.Then,we com-pare our GTNN method with other fusion methods on CA-SIA NIR-VIS2.0database[22]in Section5.2and LFW database[12]in Section5.3,respectively. Implementation Details.In this study,three networks (LeanFace,AttNet and GTNN)are discussed.LeanFace and AttNet are implemented using MXNet[6]and GTNN uses TensorFlow[1].We use around6M training face thumbnails covering62K different identities to train Lean-Face,which has no overlapping with all the test databases. AttNet is trained using CelebA[24]database.The input of GTNN is two256D features from bottleneck layers(i.e., fully connected layers before prediction layers)of LeanFace and AttNet.The setting of main parameters are shown in Table1.Note that the learning rates drop when the loss stops decreasing.Specifically,the learning rates change4 and2times for LeanFace and AttNet respectively.Dur-ing test,LeanFace and AttNet take around2.9ms and3.2ms to extract feature from one input image and GTNN takes around2.1ms to fuse one pair of LeanFace and AttNet fea-ture using a GTX1080Graphics Card.5.1.Multi-PIE DatabaseMulti-PIE database[7]contains more than750,000im-ages of337people recorded in4sessions under diverse pose,illumination and expression variations.It is an ideal testbed to investigate if facial attribute features(FAF) complement face recognition features(FRF)including tra-ditional hand-crafted(LBP)and deeply learned features (LeanFace)to improve the face recognition performance–particularly across extreme pose variation.Settings.We conduct three experiments to investigate pose-,illumination-and expression-invariant face recogni-tion.Pose:Uses images across4sessions with pose vari-ations only(i.e.,neutral lighting and expression).It covers pose with yaw ranging from left90◦to right90◦.In com-parison,most of the existing works only evaluate perfor-mance on poses with yaw range(-45◦,+45◦).Illumination: Uses images with20different illumination conditions(i.e., frontal pose and neutral expression).Expression:Uses im-ages with7different expression variations(i.e.,frontal pose and neutral illumination).The training sets of all settings consist of the images from thefirst200subjects and the re-maining137subjects for testing.Following[59,14],in the test set,frontal images with neural illumination and expres-sion from the earliest session work as gallery,and the others are probes.Pose.Table2shows the pose-robust face recognition (PRFR)performance.Clearly,the fusion of FRF and FAF, namely GTNN(LBP,AttNet)and GTNN(LeanFace,At-tNet),works much better than using FRF only,showing the complementary power of facial features to face recognition features.Not surprisingly,the performance of both LBP and LeanFace features drop greatly under extreme poses,as pose variation is a major factor challenging face recognition performance.In contrast,with GTNN-based fusion,FAF can be used to improve both classic(LBP)and deep(Lean-Face)FRF features effectively under this circumstance,for example,LBP(1.3%)vs GTNN(LBP,AttNet)(16.3%), LeanFace(72.0%)vs GTNN(LeanFace,AttNet)(78.3%) under yaw angel−90◦.It is noteworthy that despite their highly asymmetric strength,GTNN is able to effectively fuse FAF and FRF.This is elaborately studied in more detail in Sections5.2-5.3.Compared with state-of-the-art methods[14,59,11,58, 15]in terms of(-45◦,+45◦),LeanFace achieves better per-formance due to its big training data and the strong gener-alisation capacity of deep learning.In Table2,2D meth-ods[14,59,15]trained models using the MultiPIE images, therefore,they are difficult to generalise to images under poses which do not appear in MultiPIE database.3D meth-ods[11,58]highly depend on accurate2D landmarks for 3D-2D modellingfitting.However,it is hard to accurately detect such landmarks under larger poses,limiting the ap-plications of3D methods.Illumination and expression.Illumination-and expression-robust face recognition(IRFR and ERFR)are also challenging research topics.LBP is the most widely used handcrafted features for IRFR[2]and ERFR[33].To investigate the helpfulness of facial attributes,experiments of IRFR and ERFR are conducted using LBP and Lean-Face features.In Table3,GTNN(LBP,AttNet)signifi-cantly outperforms LBP,80.3%vs57.5%(IRFR),77.5% vs71.7%(ERFR),showing the great value of combining fa-cial attributes with hand-crafted features.Attributes such as the shape of eyebrows are illumination invariant and others, e.g.,gender,are expression invariant.In contrast,LeanFace feature is already very discriminative,saturating the perfor-mance on the test set.So there is little room for fusion of AttrNet to provide benefit.5.2.CASIA NIR-VIS2.0DatabaseThe CASIA NIR-VIS2.0face database[22]is the largest public face database across near-infrared(NIR)images and visible RGB(VIS)images.It is a typical cross-modality or heterogeneous face recognition problem because the gallery and probe images are from two different spectra.The。
Control Systems Engineering
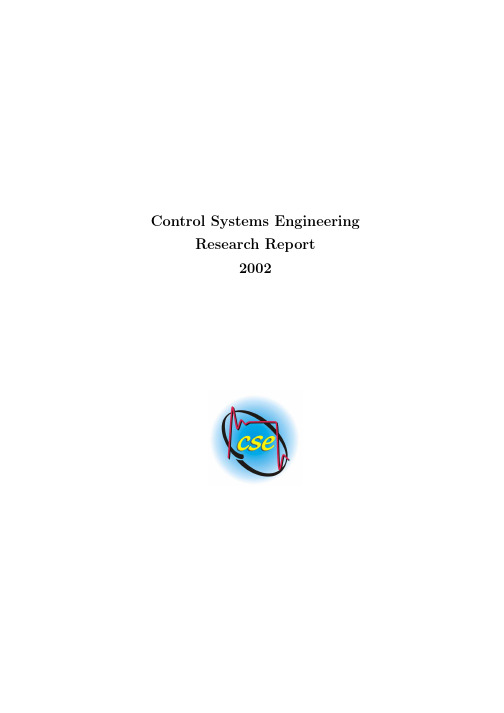
Control Systems Engineering Research Report2002Control Systems EngineeringSection CROSS(Control,Risk,Optimization,Stochastics and Systems)Faculty of Information Technology and SystemsDelft University of TechnologyPostal address:Visiting addressP.O.Box5031Mekelweg42600GA Delft2628CD DelftThe Netherlands The NetherlandsPhone:+31-15-2785119Fax:+31-15-2786679Email:control@its.tudelft.nlc 2002Control Systems Engineering,rmation Technology and Systems,Delft University ofTechnologyAll rights reserved.No part of the publication may be reproduced in any form by print,photoprint, microfilm or any other means without written permission from the publisher.Contents1Introduction11.1Overview (1)1.2Address and location (3)1.3Staffin2002 (4)2Intelligent modeling,control&decision making52.1Affordable digitalfly-by-wireflight control systems for small commercial aircraft52.2Intelligent adaptive control of bioreactors (6)2.3Fuzzy control of multivariable processes (7)2.4Neuro-fuzzy modeling in model-based fault detection,fault isolation and con-troller reconfiguration (7)2.5Intelligent molecular diagnostic systems (7)2.6Model based optimization of fed-batch bioprocesses (9)2.7Estimation of respiratory parameters via fuzzy clustering (10)2.8Fuzzy model based control with use of a priori knowledge (10)3Distributed and hybrid systems123.1Modeling and analysis of hybrid systems (12)3.2Model predictive control for discrete-event systems (13)3.3Model predictive control for piece-wise affine systems (13)3.4Model predictive control for hybrid systems (14)3.5Optimal traffic control (14)3.6Advanced control techniques for optimal adaptive traffic control (15)3.7Optimal transfer coordination for railway systems (16)3.8Real-time control of smart structures (17)4Fault-tolerant control194.1Model-based fault detection and controller reconfiguration for wind turbines.194.2Model-based fault detection and identification of sensor and actuator faults forsmall commercial aircraft (20)5Nonlinear analysis,control and identification215.1System identification of bio-technological processes (21)5.2Classification of buried objects based on ground penetrating radar signals..215.3Control of a jumbo container crane(JCC project) (22)5.4X-by-wire (23)5.5Analysis and design of nonlinear control systems for switching networks (24)5.6Bounding uncertainty in subspace identification (25)5.7New passivity properties for nonlinear electro-mechanical systems (26)5.8Relating Lagrangian and Hamiltonian descriptions of electrical circuits (27)5.9Discrete-time sliding mode control (27)5.10Nonlinear control systems analysis (28)5.11Model and controller reduction for nonlinear systems (28)5.12Robust and predictive control using neural networks (29)5.13The standard predictive control problem (30)5.14Predictive control of nonlinear systems in the process industry (30)5.15Identification of nonlinear state-space systems (31)5.16Development of computationally efficient and numerically robust system iden-tification software (32)1Introduction1.1OverviewThis report presents an overview of the ongoing research projects during2002at the Control Systems Engineering(CSE)group of the Faculty of Information Technology and Systems of Delft University of Technology.As revealed by the new logo of the group,a number of major changes have taken place. Three of these major events will be briefly discussed.First,the stronger emphasis on a systems oriented research approach has motivated a change of the name from Control Laboratory into Control Systems Engineering group.Second,in September2001Prof.dr.ir.M.Verhaegen was appointed as the new chairman of the CSE group.With his arrival an impulse was given to strengthen the development of new methods and techniques for identification and fault-tolerant control design.The primary focus of the programme development is to formulate new research initiatives and to initiate research alliances with established Dutch and European research-oriented laboratories and industry.New research proposals will be formulated within the four main themes:intelligent modeling,control and decision making;distributed and hybrid systems;fault-tolerant control; and analysis,control and identification of nonlinear systems—as depicted by the vertical columns in Figure1.The overall focus will remain on complex nonlinear systems,new application directions,however,may be included,such as adaptive optics which more and more rely on advanced control techniques.The CSE group is also taking part in new research programme definitions of the Faculty of Information Technology and Systems,such as the Intelligent Systems Consortium(iSc)chaired by Prof.P.Dewilde.Third,the CSE group strives to strengthen the research and teaching cooperation in the area of control systems engineering with other leading Systems and Control Engineering groups in Delft.To accomplish this goal,the CSE actively supports the creation of a joint Delft Center on Systems and Control Engineering.The research interests of the CSE group are focused on the following areas:•Intelligent modeling,control and decision making:black-box and gray-box modeling of dynamic systems with fuzzy logic and neural net-works,and design of controllers using fuzzy set techniques.•Distributed and hybrid systems:analysis and control methods,multi-agent control,hierarchical control,and model pre-dictive control of hybrid systems.•Fault-tolerant control:fault detection and isolation with system identification and extended Kalmanfiltering, probabilistic robust control.•Nonlinear analysis,control and identification:nonlinear predictive control,sliding mode control,iterative learning control,nonlinear dynamic model inversion,Lagrangian and Hamiltonian modeling and control frame-works(energy based),identification of a composite of numerical local linear state space models to approximate nonlinear dynamics.The goal of the CSE group is to develop innovative methodologies in thefields indicated above.An important motive in demonstrating their relevance is to cooperate with nationalFigure1:Overview of the research topics of the Control System Engineering group. and international research organizations and industry to validate the real-life potential of the new methodologies.The main applicationfields are:•Smart structures:X-by-wire,road traffic sensors,high performance control using smart materials,adaptive optics,laboratory-on-a-chip,micro robotics.•Power engineering:switching networks,power distribution and conversion,condition monitoring in off-shore wind turbines.•Telecommunication•Motion control:autonomous and intelligent mobile systems,mobile robots,container transport,aircraft and satellite control,traffic control.•Bioprocess technology:fermentation processes,waste-water treatment.The CSE group currently consists of27scientific and support staff:8permanent scientific staff,10PhD students,2postdoctoral researchers,and7support personnel.The research activities are for a large partfinanced from external sources including the Dutch National Science Foundation(STW),Delft University of Technology,the European Union,and indus-try.Additional information can be found at http://lcewww.et.tudelft.nl/.1.2Address and locationControl Systems EngineeringFaculty of Information Technology&SystemsDelft University of TechnologyPostal address:P.O.Box50312600GA DelftThe NetherlandsVisiting address:Mekelweg42628CD DelftThe NetherlandsPhone:+31-15-2785119Fax:+31-15-27866791.3Staffin2002Scientific staffProf.dr.ir.M.H.G.VerhaegenProf.dr.ir.J.HellendoornProf.dr.ir.R.Babuˇs kaDr.ir.T.J.J.van den BoomDr.ir.B.De SchutterDr.ir.J.B.KlaassensDr.ir.J.M.A.ScherpenDr.ir.V.VerdultPhD students&postdoctoral researchers Dr.J.Clemente GallardoIr.P.R.FraanjeIr.A.HegyiIr.K.J.G.HinnenIr.D.JeltsemaR.Lopez Lena,MScIr.S.Meˇs i´cIr.M.L.J.OosteromIr.G.PastoreNon-scientific staffC.J.M.DukkerIng.P.M.EmonsP.MakkesIng.W.J.M.van GeestD.NoteboomG.J.M.van der WindtIng.R.M.A.van PuffelenAdvisorsProf.ir.G.Honderd,em.Prof.ir.H.R.van Nauta Lemke,em. Prof.ir.H.B.Verbruggen,em.2Intelligent modeling,control&decision makingThis research theme focuses on the use of fuzzy logic,neural networks and evolutionary al-gorithms in the analysis and design of models and controllers for nonlinear dynamic systems. Fuzzy logic systems offer a suitable framework for combining knowledge of human experts with partly known mathematical models and data,while artificial neural networks are effec-tive black-box function approximators with learning and adaptation capabilities.Evolution-ary algorithms are randomized optimization techniques useful in searching high-dimensional spaces and tuning of parameters in fuzzy and neural systems.These techniques provide tools for solving complex design problems under uncertainty by providing the ability to learn from past experience,perform complex pattern recognition tasks and fuse information from various sources.Application domains include fault-tolerant control,nonlinear system identification, autonomous and adaptive control,among others.2.1Affordable digitalfly-by-wireflight control systems for small commer-cial aircraftProject members:M.L.J.Oosterom,R.Babuˇs ka,H.B.VerbruggenSponsored by:European Community GROWTH project ADFCS–IIThe objective of this project is to apply thefly-by-wire(FBW)technology inflight control systems of a smaller category of aircraft(see Figure2).In FBW digitalflight control systems, there is no direct link between the control stick and pedals,which are operated by the pilot, and the control surfaces.All measured signals,including the pilot inputs,are processed by the flight control computer that computes the desired control surface deflections.This scheme enables theflight control engineer to alter the dynamic characteristics of the bare aircraft through an appropriate design of theflight control laws.Moreover,important safety features can be included in the control system,such asflight envelope protection.This increases the safety level compared to aircraft with mechanical control systems.Our task in the project is to assess the benefits and to verify the validity of the soft-computing techniques in the FBW control system design and sensor management.These novel techniques are combined with standard,well-proven methods of the aircraft industry.Figure2:The Galaxy business jet(left)and validation of the control system through pilot-in-the-loop simulations at the Research Flight Simulator of the NLR(right).Figure3:The experimental laboratory setup(left)and the basic model-based adaptive control scheme(right).The research topics are the design of gain-scheduled control laws,fault detection,isolation and reconfiguration,and an expert system monitoring of the overall operational status of both the pilot and the aircraft.For control design,fault detection and identification system,fuzzy logic approaches are adopted in order to extend linear design techniques to nonlinear systems. Moreover,a neuro-fuzzy virtual sensor will be developed in close cooperation with Alenia to replace hardware sensors.For the pilot-aircraft status monitor a fuzzy expert system will be developed that has the functionality of a warning and advisory/decision aiding system.2.2Intelligent adaptive control of bioreactorsProject members:R.Babuˇs ka,M.Damen,S.Meˇs i´cSponsored by:SenterThe goal of this research is the development and implementation of a robust self-tuning con-troller for fermentation processes.To ensure an optimal operating conditions,the pH value, the temperature and the dissolved oxygen concentration in the fermenter must be controlled within tight bounds.Ideally,the same control unit should be able to ensure the required performance for a whole variety of fermentation processes(different microorganisms),differ-ent scales(volume of1liter to10000liters)and throughout the entire process run.Figure3 shows an experimental laboratory setup used in this project.The main control challenge is the fact that the dynamics of the system depend on the particular process type and scale and moreover are strongly time-varying,due to gradual changes in the process operating conditions.Controllers withfixed parameters cannot fulfill these requirements.Self-tuning(adaptive) control is applied to address the time-varying nature of the process.Among the different types of adaptive controllers(model-free,model-based,gain-scheduled,etc.),the model-based approach is pursued.The model is obtained through a carefully designed local identification experiment.Special attentions is paid to the robustness of the entire system in order to ensure safe and stable operation under all circumstances.The main contribution of this research is the development,implementation and experimental validation of a complete self-tuning control system.The robustness of the system is achieved by combining well-proven identification and control design methods with a supervisory fuzzy expert system.This research is being done a cooperation between Applikon Dependable Instruments B.V.,Schiedam,Faculty of Electrical Engineering,Eindhoven University of Technology and Faculty of Information Technology and Systems and Kluyver Laboratory for Biotechnology, both at Delft University of Technology.2.3Fuzzy control of multivariable processesProject members:R.Babuˇs ka,S.Mollov,H.B.VerbruggenFuzzy control provides effective solutions for nonlinear and partially unknown processes, mainly because of its ability to combine information form different sources,such as avail-able mathematical models,experience of operators,process measurements,etc.Extensive research has been devoted to single-input single-output fuzzy control systems,including mod-eling and control design aspects,analysis of stability and robustness,adaptive control.Mul-tivariable fuzzy control,however,have received considerably less attention,despite strong practical needs for multivariable control solutions,indicated among otherfields from process industry,(waste)water treatment,or aerospace engineering.Yet,theoretical foundations and methodological aspects of multivariable control are not well developed.This research project focuses on the use of fuzzy logic in model-based control of multiple-input,multiple-output(MIMO)systems.Recent developments include effective optimization techniques and robust stability constraints for nonlinear model predictive control.The devel-oped predictive control methods have been applied to the design of an Engine Management System for the gasoline direct injection engine benchmark,developed as a case study within the European research project FAMIMO(see Figure4).An extension of the Relative Gain Array approach has been proposed that facilitates the analysis of interactions in MIMO fuzzy models.2.4Neuro-fuzzy modeling in model-based fault detection,fault isolationand controller reconfigurationProject members:M.H.G.Verhaegen,J.Hellendoorn,R.Babuˇs ka,S.Kanev,A.Ichtev Sponsored by:STWMost fault tolerant control systems rely on two modules:(model-based)fault detection and isolation module and controller reconfiguration module.The two key elements in designing these two systems are the development of a mathematical model and a suitable decision mechanism to localize the failure and to select a new controller configuration.This project focuses on the development of a design framework in which the mathematical model and the corresponding observer are represented as a composition of local models,each describing the system in a particular operating regime or failure mode.The use of fuzzy Takagi-Sugeno models for residual generation has been investigated.On the basis of residuals soft fault detection and isolation and controller reconfiguration are performed.2.5Intelligent molecular diagnostic systemsProject members:L.Wessels,P.J.van der Veen,J.HellendoornAir BurngasesFigure4:Fuzzy predictive control of a gasoline directinjection engine. Sponsored by:DIOC-5:Intelligent Molecular Diagnostic SystemsIt is the goal of the DIOC-5(DIOC:Delft Interfaculty Research Center)program to produce an Intelligent Molecular Diagnostic System(IMDS).The IMDS will consist of two basic com-ponents:a measurement device and an information processing unit(IPU).The measurement device is a chemical sensor on a chip,which will be capable of rapidly performing vast num-bers of measurements simultaneously,consuming a minimal amount of chemical reagents and sample(see Figure5).Figure5:A prototype IMDS chip containing a matrix of25pico-liter wells.The IPU transforms the complex,raw measurements obtained from the sensor into output that can be employed as high-level decision support in various application domains.See[41]for a possible realization of the IPU.Members of the Control Systems Engineering group and the Information and Communica-tion Theory group are responsible for the realization of the Information Processing Unit.Un-raveling the metabolic processes and the associated regulatory mechanisms of yeast is a very interesting application area for the DIOC-5technology.We are focusing on problems associ-ated with gene and protein levels,and will integrate this information with existing knowledge about metabolic processes developed at the Kluyver Laboratory(One of the DIOC-5part-ners).More specifically,gene expression data and protein concentration measurements are employed to model the genetic networks,i.e.,to postulate possible‘genetic wiring diagrams’based on the expression data(See[40]for some preliminary results in this area.) It is envisaged that at the end of this project,genetic network information,protein func-tional knowledge and metabolic models can be integrated into a single hierarchical model, capable of providing metabolic engineers with greater insight into the yeast metabolism.For additional information see the IMDS Web page.12.6Model based optimization of fed-batch bioprocessesProject members:J.A.Roubos,P.Krabben,R.Babuˇs ka,J.J.Heijnen,H.B.Verbruggen Sponsored by:DIOC-6:Mastering the Molecules in Manufacturing,DSM Anti Infectives Many biotechnological production systems are based on batch and fed-batch processes.Op-timization of the product formation currently requires a very expensive and time consuming experimental program to determine the optima by trial and error.The aim of this project is to find a more efficient development path for fed-batch bioprocesses by an optimal combination of experiments and process models.The two main research topics of this project are:•Development of a user friendly modeling environment for fed-batch processes.The soft-ware tool must be able to use different types of knowledge coming from experts,experi-ments andfirst-principles,i.e.,conservation laws.New modeling methods such as fuzzy logic,neural networks and hybrid models will be used.•Iterative optimal experiment design.First some basic experiments can be done to esti-mate some preliminary parameters for the system.The idea is to make a rough model to design the next experiment.First,a stoichiometric model is made and thereafter a structured biochemical model that will be gradually improved according to the fermen-tation data.The main objective is to predict the right trends.The actual values are less important at the initial stages.Once the model is sufficient in terms of quantitative prediction of the production process for a variable external environment,it will be used to determine optimal feeding strategies for the reactor in order to improve product quality and/or quantity.These feeding strategies will be applied in an on-line process control environment.Recent developments and publications can be found at the project Web page2.1http://www.ph.tn.tudelft.nl/Projects/DIOC/Progress.html2http://lcewww.et.tudelft.nl/˜roubos/02401020Time [s]p h a s e 1p h a s e 2p h a s e 3phase 4P r e s s u r e [h P a ]Figure 6:Partitioning of the respiratory cycle is obtained automatically by fuzzy clustering.Each segment represents a characteristic phase of the respiratory cycle.2.7Estimation of respiratory parameters via fuzzy clusteringProject members:R.Babuˇs ka,M.S.Lourens,A.F.M.Verbraak and J.Bogaard (University Hospital Rotterdam)The monitoring of respiratory parameters estimated from flow-pressure-volume measurements can be used to assess patients’pulmonary condition,to detect poor patient-ventilator interac-tion and consequently to optimize the ventilator settings.A new method has been investigated to obtain detailed information about respiratory parameters without interfering with the ven-tilation.By means of fuzzy clustering,the available data set is partitioned into fuzzy subsets that can be well approximated by linear regression models locally.Parameters of these models are then estimated by least-squares techniques.By analyzing the dependence of these local parameters on the location of the model in the flow-volume-pressure space,information on the patients’pulmonary condition can be gained.The effectiveness of the proposed approaches has been studied by analyzing the dependence of the expiratory time constant on the volume in patients with chronic obstructive pulmonary disease (COPD)and patients without COPD.2.8Fuzzy model based control with use of a priori knowledgeProject members:R.Babuˇs ka,J.Abonyi (University of Veszpr´e m,Hungary)Effective development of nonlinear dynamic process models is of great importance in the application of model-based control.Typically,one needs to blend information from different sources:experience of operators and designers,process data and first principle knowledge formulated by mathematical equations.To incorporate a priori knowledge into data-driven identification of dynamic fuzzy models of the Takagi-Sugeno type a constrained identification algorithm has been developed,where the constrains on the model parameters are based on the knowledge about the process stability,minimal or maximal gain,and the settling time.The algorithm has been successfully applied to off-line and on-line adaptation of fuzzy models.When no a priori knowledge about the local dynamic behavior of the process is available, information about the steady-state characteristic could be extremely useful.Because of the difficult analysis of the steady-state behavior of dynamic fuzzy models of the Takagi-Sugeno type,block-oriented fuzzy models have been developed.In the Fuzzy Hammerstein(FH) model,a static fuzzy model is connected in series with a linear dynamic model.The obtained FH model is incorporated in a model-based predictive control scheme.Results show that the proposed FH modeling approach is useful for modular parsimonious modeling and model-based control of nonlinear systems.3Distributed and hybrid systemsHybrid systems typically arise when a continuous-time system is coupled with a logic con-troller,or when we have a system in which external inputs or internal events may cause a sudden change in the dynamics of the system.So hybrid systems exhibit both continuous-variable and discrete-event behavior.Due to the intrinsic complexity of hybrid systems control design techniques for hybrid systems we could either focus on special subclasses of hybrid sys-tems,or use a distributed or hierarchical approach to decompose the controller design problem into smaller subproblems that are easier to solve.In our research we use both approaches.3.1Modeling and analysis of hybrid systemsProject members:B.De Schutter,W.M.P.H.Heemels(Eindhoven University of Technology), A.Bemporad(ETH Z¨u rich)Hybrid systems arise from the interaction between continuous-variable systems(i.e.,systems that can be described by a system of difference or differential equations)and discrete-event systems(i.e.,asynchronous systems where the state transitions are initiated by events;in general the time instants at which these events occur are not equidistant).In general we could say that a hybrid system can be in one of several modes whereby in each mode the behavior of the system can be described by a system of difference or differential equations, and that the system switches from one mode to another due to the occurrence of an event (see Figure7).We have shown that several classes of hybrid systems:piecewise-affine systems,mixed logical dynamical systems,complementarity systems and max-min-plus-scaling systems are equivalent[6,7,24,25].Some of the equivalences are established under(rather mild)addi-tional assumptions.These results are of paramount importance for transferring theoreticalFigure7:Schematic representation of a hybrid system.properties and tools from one class to another,with the consequence that for the study of a particular hybrid system that belongs to any of these classes,one can choose the most convenient hybrid modeling framework.Related research is described under Project3.3.In addition,we have also shown an equivalence between two type of mathematical pro-gramming problems:the linear complementarity problem(LCP)and the extended linear complementarity problem(ELCP)[17].More specifically,we have shown that an ELCP with a bounded feasible set can be recast as an LCP.This result allows us to apply existing LCP algorithms to solve ELCPs[16].3.2Model predictive control for discrete-event systemsProject members:B.De Schutter,T.J.J.van den BoomModel predictive control(MPC)is a very popular controller design method in the process industry.An important advantage of MPC is that it allows the inclusion of constraints on the inputs and ually MPC uses linear discrete-time models.In this project we extend MPC to a class of discrete-event systems.Typical examples of discrete-event systems are:flexible manufacturing systems,telecommunication networks,traffic control systems, multiprocessor operating systems,and logistic systems.In general models that describe the behavior of a discrete-event system are nonlinear in conventional algebra.However,there is a class of discrete-event systems–the max-plus-linear discrete-event systems–that can be described by a model that is“linear”in the max-plus algebra.We have further developed our MPC framework for max-plus-linear discrete-event systems and included the influences of noise and disturbances[33,34,35,36,37].In addition,we have also extended our results to discrete-event systems that can be described by models in which the operations maximization,minimization,addition and scalar multiplication appear[22], and to discrete-event systems with both hard and soft synchronization constraints[19](see also Project3.7).3.3Model predictive control for piece-wise affine systemsProject members:B.De Schutter,T.J.J.van den BoomWe have extended our results on model predictive control(MPC)for discrete event systems (see Project3.2)to a class of hybrid systems that can be described by a continuous piecewise-affine state space model.More specifically,we have considered systems of the formx(k)=P x(x(k−1),u(k))y(k)=P y(x(k),u(k)),where x,u and y are respectively,the state,the input and the output vector of the system,and where the components of P x and P y are continuous piecewise-affine(PWA)scalar functions,i.e.,functions that satisfy the following conditions:1.The domain space of f is divided into afinite number of polyhedral regions;2.In each region f can be expressed as an affine function;3.f is continuous on any boundary between two regions.。
机器翻译中的常用神经网络模型
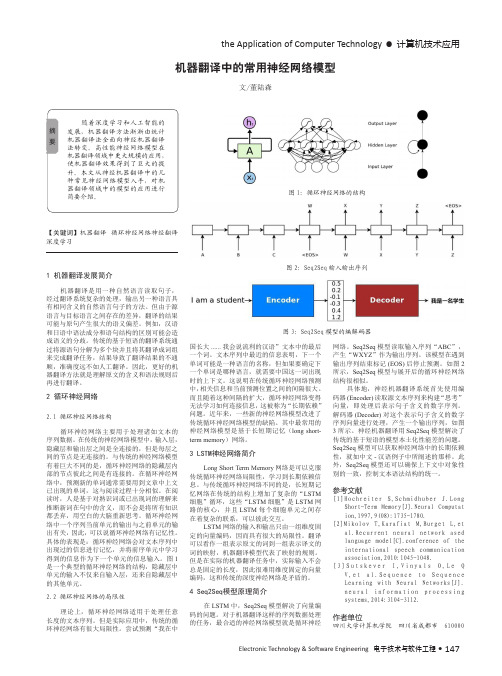
the Application of Computer Technology •计算机技术应用Electronic Technology & Software Engineering 电子技术与软件工程• 147【关键词】机器翻译 循环神经网络神经翻译 深度学习1 机器翻译发展简介机器翻译是用一种自然语言读取句子,经过翻译系统复杂的处理,输出另一种语言具有相同含义的自然语言句子的方法。
但由于源语言与目标语言之间存在的差异,翻译的结果可能与原句产生很大的语义偏差。
例如,汉语和日语中语法成分和语句结构的区别可能会造成语义的分歧。
传统的基于短语的翻译系统通过将源语句分解为多个块并且将其翻译成词组来完成翻译任务,结果导致了翻译结果的不通顺,准确度远不如人工翻译。
因此,更好的机器翻译方法就是理解原文的含义和语法规则后再进行翻译。
2 循环神经网络2.1 循环神经网络结构循环神经网络主要用于处理诸如文本的序列数据。
在传统的神经网络模型中,输入层,隐藏层和输出层之间是全连接的,但是每层之间的节点是无连接的。
与传统的神经网络模型有着巨大不同的是,循环神经网络的隐藏层内部的节点彼此之间是有连接的。
在循环神经网络中,预测新的单词通常需要用到文章中上文已出现的单词,这与阅读过程十分相似。
在阅读时,人是基于对熟识词或已出现词的理解来推断新词在句中的含义,而不会是将所有知识都丢弃,用空白的大脑重新思考。
循环神经网络中一个序列当前单元的输出与之前单元的输出有关,因此,可以说循环神经网络有记忆性。
具体的表现是:循环神经网络会对文本序列中出现过的信息进行记忆,并将前序单元中学习得到的信息作为下一个单元的信息输入。
图1是一个典型的循环神经网络的结构,隐藏层中单元的输入不仅来自输入层,还来自隐藏层中的其他单元。
2.2 循环神经网络的局限性理论上,循环神经网络适用于处理任意长度的文本序列。
但是实际应用中,传统的循环神经网络有很大局限性。
卷积神经网络机器学习外文文献翻译中英文2020
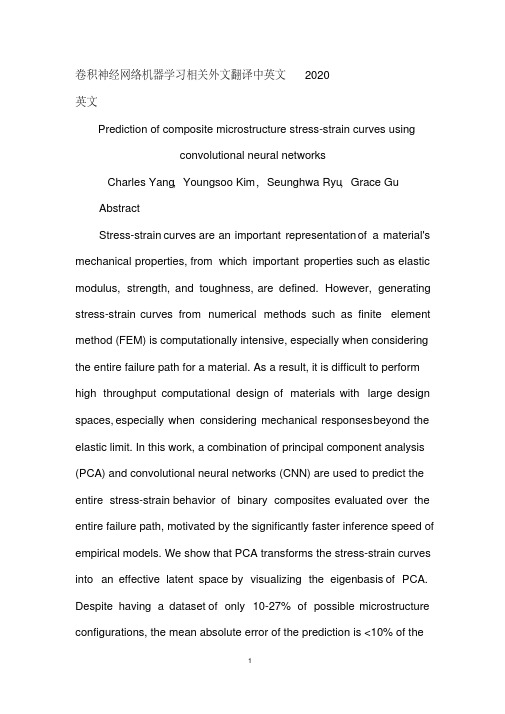
卷积神经网络机器学习相关外文翻译中英文2020英文Prediction of composite microstructure stress-strain curves usingconvolutional neural networksCharles Yang,Youngsoo Kim,Seunghwa Ryu,Grace GuAbstractStress-strain curves are an important representation of a material's mechanical properties, from which important properties such as elastic modulus, strength, and toughness, are defined. However, generating stress-strain curves from numerical methods such as finite element method (FEM) is computationally intensive, especially when considering the entire failure path for a material. As a result, it is difficult to perform high throughput computational design of materials with large design spaces, especially when considering mechanical responses beyond the elastic limit. In this work, a combination of principal component analysis (PCA) and convolutional neural networks (CNN) are used to predict the entire stress-strain behavior of binary composites evaluated over the entire failure path, motivated by the significantly faster inference speed of empirical models. We show that PCA transforms the stress-strain curves into an effective latent space by visualizing the eigenbasis of PCA. Despite having a dataset of only 10-27% of possible microstructure configurations, the mean absolute error of the prediction is <10% of therange of values in the dataset, when measuring model performance based on derived material descriptors, such as modulus, strength, and toughness. Our study demonstrates the potential to use machine learning to accelerate material design, characterization, and optimization.Keywords:Machine learning,Convolutional neural networks,Mechanical properties,Microstructure,Computational mechanics IntroductionUnderstanding the relationship between structure and property for materials is a seminal problem in material science, with significant applications for designing next-generation materials. A primary motivating example is designing composite microstructures for load-bearing applications, as composites offer advantageously high specific strength and specific toughness. Recent advancements in additive manufacturing have facilitated the fabrication of complex composite structures, and as a result, a variety of complex designs have been fabricated and tested via 3D-printing methods. While more advanced manufacturing techniques are opening up unprecedented opportunities for advanced materials and novel functionalities, identifying microstructures with desirable properties is a difficult optimization problem.One method of identifying optimal composite designs is by constructing analytical theories. For conventional particulate/fiber-reinforced composites, a variety of homogenizationtheories have been developed to predict the mechanical properties of composites as a function of volume fraction, aspect ratio, and orientation distribution of reinforcements. Because many natural composites, synthesized via self-assembly processes, have relatively periodic and regular structures, their mechanical properties can be predicted if the load transfer mechanism of a representative unit cell and the role of the self-similar hierarchical structure are understood. However, the applicability of analytical theories is limited in quantitatively predicting composite properties beyond the elastic limit in the presence of defects, because such theories rely on the concept of representative volume element (RVE), a statistical representation of material properties, whereas the strength and failure is determined by the weakest defect in the entire sample domain. Numerical modeling based on finite element methods (FEM) can complement analytical methods for predicting inelastic properties such as strength and toughness modulus (referred to as toughness, hereafter) which can only be obtained from full stress-strain curves.However, numerical schemes capable of modeling the initiation and propagation of the curvilinear cracks, such as the crack phase field model, are computationally expensive and time-consuming because a very fine mesh is required to accommodate highly concentrated stress field near crack tip and the rapid variation of damage parameter near diffusive cracksurface. Meanwhile, analytical models require significant human effort and domain expertise and fail to generalize to similar domain problems.In order to identify high-performing composites in the midst of large design spaces within realistic time-frames, we need models that can rapidly describe the mechanical properties of complex systems and be generalized easily to analogous systems. Machine learning offers the benefit of extremely fast inference times and requires only training data to learn relationships between inputs and outputs e.g., composite microstructures and their mechanical properties. Machine learning has already been applied to speed up the optimization of several different physical systems, including graphene kirigami cuts, fine-tuning spin qubit parameters, and probe microscopy tuning. Such models do not require significant human intervention or knowledge, learn relationships efficiently relative to the input design space, and can be generalized to different systems.In this paper, we utilize a combination of principal component analysis (PCA) and convolutional neural networks (CNN) to predict the entire stress-strain c urve of composite failures beyond the elastic limit. Stress-strain curves are chosen as the model's target because t hey are difficult to predict given their high dimensionality. In addition, stress-strain curves are used to derive important material descriptors such as modulus, strength, and toughness. In this sense, predicting stress-straincurves is a more general description of composites properties than any combination of scaler material descriptors. A dataset of 100,000 different composite microstructures and their corresponding stress-strain curves are used to train and evaluate model performance. Due to the high dimensionality of the stress-strain dataset, several dimensionality reduction methods are used, including PCA, featuring a blend of domain understanding and traditional machine learning, to simplify the problem without loss of generality for the model.We will first describe our modeling methodology and the parameters of our finite-element method (FEM) used to generate data. Visualizations of the learned PCA latent space are then presented, a long with model performance results.CNN implementation and trainingA convolutional neural network was trained to predict this lower dimensional representation of the stress vector. The input to the CNN was a binary matrix representing the composite design, with 0's corresponding to soft blocks and 1's corresponding to stiff blocks. PCA was implemented with the open-source Python package scikit-learn, using the default hyperparameters. CNN was implemented using Keras with a TensorFlow backend. The batch size for all experiments was set to 16 and the number of epochs to 30; the Adam optimizer was used to update the CNN weights during backpropagation.A train/test split ratio of 95:5 is used –we justify using a smaller ratio than the standard 80:20 because of a relatively large dataset. With a ratio of 95:5 and a dataset with 100,000 instances, the test set size still has enough data points, roughly several thousands, for its results to generalize. Each column of the target PCA-representation was normalized to have a mean of 0 and a standard deviation of 1 to prevent instable training.Finite element method data generationFEM was used to generate training data for the CNN model. Although initially obtained training data is compute-intensive, it takes much less time to train the CNN model and even less time to make high-throughput inferences over thousands of new, randomly generated composites. The crack phase field solver was based on the hybrid formulation for the quasi-static fracture of elastic solids and implementedin the commercial FEM software ABAQUS with a user-element subroutine (UEL).Visualizing PCAIn order to better understand the role PCA plays in effectively capturing the information contained in stress-strain curves, the principal component representation of stress-strain curves is plotted in 3 dimensions. Specifically, we take the first three principal components, which have a cumulative explained variance ~85%, and plot stress-strain curves in that basis and provide several different angles from which toview the 3D plot. Each point represents a stress-strain curve in the PCA latent space and is colored based on the associated modulus value. it seems that the PCA is able to spread out the curves in the latent space based on modulus values, which suggests that this is a useful latent space for CNN to make predictions in.CNN model design and performanceOur CNN was a fully convolutional neural network i.e. the only dense layer was the output layer. All convolution layers used 16 filters with a stride of 1, with a LeakyReLU activation followed by BatchNormalization. The first 3 Conv blocks did not have 2D MaxPooling, followed by 9 conv blocks which did have a 2D MaxPooling layer, placed after the BatchNormalization layer. A GlobalAveragePooling was used to reduce the dimensionality of the output tensor from the sequential convolution blocks and the final output layer was a Dense layer with 15 nodes, where each node corresponded to a principal component. In total, our model had 26,319 trainable weights.Our architecture was motivated by the recent development and convergence onto fully-convolutional architectures for traditional computer vision applications, where convolutions are empirically observed to be more efficient and stable for learning as opposed to dense layers. In addition, in our previous work, we had shown that CNN's werea capable architecture for learning to predict mechanical properties of 2Dcomposites [30]. The convolution operation is an intuitively good fit forpredicting crack propagation because it is a local operation, allowing it toimplicitly featurize and learn the local spatial effects of crack propagation.After applying PCA transformation to reduce the dimensionality ofthe target variable, CNN is used to predict the PCA representation of thestress-strain curve of a given binary composite design. After training theCNN on a training set, its ability to generalize to composite designs it hasnot seen is evaluated by comparing its predictions on an unseen test set.However, a natural question that emerges i s how to evaluate a model's performance at predicting stress-strain curves in a real-world engineeringcontext. While simple scaler metrics such as mean squared error (MSE)and mean absolute error (MAE) generalize easily to vector targets, it isnot clear how to interpret these aggregate summaries of performance. It isdifficult to use such metrics to ask questions such as “Is this modeand “On average, how poorly will aenough to use in the real world” given prediction be incorrect relative to some given specification”. Although being able to predict stress-strain curves is an importantapplication of FEM and a highly desirable property for any machinelearning model to learn, it does not easily lend itself to interpretation. Specifically, there is no simple quantitative way to define whether two-world units.stress-s train curves are “close” or “similar” with real Given that stress-strain curves are oftentimes intermediary representations of a composite property that are used to derive more meaningful descriptors such as modulus, strength, and toughness, we decided to evaluate the model in an analogous fashion. The CNN prediction in the PCA latent space representation is transformed back to a stress-strain curve using PCA, and used to derive the predicted modulus, strength, and toughness of the composite. The predicted material descriptors are then compared with the actual material descriptors. In this way, MSE and MAE now have clearly interpretable units and meanings. The average performance of the model with respect to the error between the actual and predicted material descriptor values derived from stress-strain curves are presented in Table. The MAE for material descriptors provides an easily interpretable metric of model performance and can easily be used in any design specification to provide confidence estimates of a model prediction. When comparing the mean absolute error (MAE) to the range of values taken on by the distribution of material descriptors, we can see that the MAE is relatively small compared to the range. The MAE compared to the range is <10% for all material descriptors. Relatively tight confidence intervals on the error indicate that this model architecture is stable, the model performance is not heavily dependent on initialization, and that our results are robust to differenttrain-test splits of the data.Future workFuture work includes combining empirical models with optimization algorithms, such as gradient-based methods, to identify composite designs that yield complementary mechanical properties. The ability of a trained empirical model to make high-throughput predictions over designs it has never seen before allows for large parameter space optimization that would be computationally infeasible for FEM. In addition, we plan to explore different visualizations of empirical models-box” of such models. Applying machine in an effort to “open up the blacklearning to finite-element methods is a rapidly growing field with the potential to discover novel next-generation materials tailored for a variety of applications. We also note that the proposed method can be readily applied to predict other physical properties represented in a similar vectorized format, such as electron/phonon density of states, and sound/light absorption spectrum.ConclusionIn conclusion, we applied PCA and CNN to rapidly and accurately predict the stress-strain curves of composites beyond the elastic limit. In doing so, several novel methodological approaches were developed, including using the derived material descriptors from the stress-strain curves as interpretable metrics for model performance and dimensionalityreduction techniques to stress-strain curves. This method has the potential to enable composite design with respect to mechanical response beyond the elastic limit, which was previously computationally infeasible, and can generalize easily to related problems outside of microstructural design for enhancing mechanical properties.中文基于卷积神经网络的复合材料微结构应力-应变曲线预测查尔斯,吉姆,瑞恩,格瑞斯摘要应力-应变曲线是材料机械性能的重要代表,从中可以定义重要的性能,例如弹性模量,强度和韧性。
机器学习讲座

The learning target is ……
Input:
y1 has the maximum value
“3”
The learning target is defined on the training data.
Learning Target
x1
……
x2
……
y1 is 1 y2 is 2
……
Softmax
…… ……
16 x 16 = 256
Ink → 1 No ink → 0
x256
……
y10 is 0
Output Layer (Option)
• Softmax layer as the output layer
Softmax Layer
Probability: 1 > ������������ > 0 ������������������ = 1
3
z1
e
z2 1
e
z3 -3 e
e z1 20
Step 3: pick the best function
Human Brains PlayGround的网址是: /
Neural Network
Neuron
z a1w1akwk aKwK b
a1 w1
A simple function
Step 3: pick the best function
Three Steps for Deep Learning
Step 1: deNfineeuraalset of fNuentcwtioornk
Step 2: goodness of
function
Art+实验室共识刘平

共识刘平在学术界获得了广泛的认可 和赞誉,其研究成果多次获得国内外 学术奖项的肯定。
THANK YOU
强调技术创新的适度与审慎
共识刘平同时强调在技术创新的运用中要保持适度与审慎。技术只是手段,不应过分追求技术的炫酷而牺牲艺术 的本质。
对未来发展的展望
倡导跨学科合作与交流
共识刘平认为,未来的艺术发展需要打破学科界限,加强与其他领域的合作与交流。通过跨学科的碰 撞与融合,可以产生更多创新的艺术观念和形式。
计算机视觉
实验室在计算机视觉领域开展深 入研究,涉及目标检测、图像识 别、图像生成等方面的研究。
数据挖掘与机器学
习
实验室关注数据挖掘和机器学习 算法的研究,探索如何从大量数 据中提取有价值的信息和知识。
实验室研究成果
发表高水平论文
01
实验室成员在人工智能领域的国际顶级会议和期刊上发表多篇
高水平的学术论文。
丰富艺术表现形式
共识刘平在艺术领域的研究与实践,为艺术表现形式的探索提供了 新的可能性,为观众带来了更加丰富的艺术体验。
提高艺术地位
共识刘平在学术界的贡献和影响力,提高了艺术领域在社会中的地 位和认知度。
对学术界的贡献与评价
学术贡献
共识刘平在学术界的研究成果丰硕, 为相关领域的发展做出了重要贡献。
去中心化金融领域的发展具有重要影响。
02
区块链技术创新
共识刘平在区块链技术领域做出了多项创新,包括共识机制、智能合约
、去中心化应用等方面。他的研究成果推动了区块链技术的实际应用和
发展。
03
金融科技研究
共识刘平在金融科技领域也有深入研究,致力于将区块链技术和金融业
务相结合,为金融行业带来更多创新和价值。
基于FE-YOLOv5s的变电所安全帽佩戴检测

数字技术与应用 Digital Technology &Application
Vol.42 No.1 Jan 2024
中图分类号:TP391.41
文献标识码:A
DOI:10.19695/12-1369.2024.01.19
2.3.2 注意力机制实验 为了验证网络加入 ECA 注意力机制的有效性,并分 析引入 ECA 注意力机制相较其他注意力机制的优越性,本 文选取了目前主流的注意力机制 CA、CBAM、SimAM、 SGE 等 4 种加入对比实验中。为了保证实验的变量唯一, 我们将上述的注意力机制加入到 F-YOLOv5s 相同网络
收稿日期 :2023-10-18 作者简介 :马三保(1976—),男,安徽界首人,本科,高级工程师,从事矿井提升系统研究工作。
60
马三保 王鹏彬 程磊等:基于 FE-YOLOv5s 的变电所安全帽佩戴检测
2024 年 第 1 期
特征增强操作。其中传统的 SPPF 模块采用的是 CBS 模 块进行特征提取,但是在视觉领域当中我们不能忽视卷 积层在空间层面的敏感度问题,所以我们采用 FReLU 激活函数 [8] 替换原模块中的 SiLU 激活函数,构成新模 块 FSPPF 模块,FSPPF 模块结构图如图 2 所示。
2
CA
0.911 0.919 0.925 0.14
3
CBAM
0.89 0.898 0.917 0.893
4
SimAM 0.903 0.898 0.927 0.900
5
SGE
0.903 0.906 0.929 0.904
实验结果表明,在引入 ECA 注意力机制以后,虽然 在召回率方面提升只有 0.2%,但是在精准率方面提升较 为明显,提升了 4%。在 5 组实验中,引入 ECA 注意力 机制的实验 1,在精准率、mAP、F1 分数三项中均为最 高值。实验证明引入 ECA 注意力机制可以极大增强网络 对小尺度目标的数字技术与应用
一种新的部分神经进化网络的股票预测(英文)
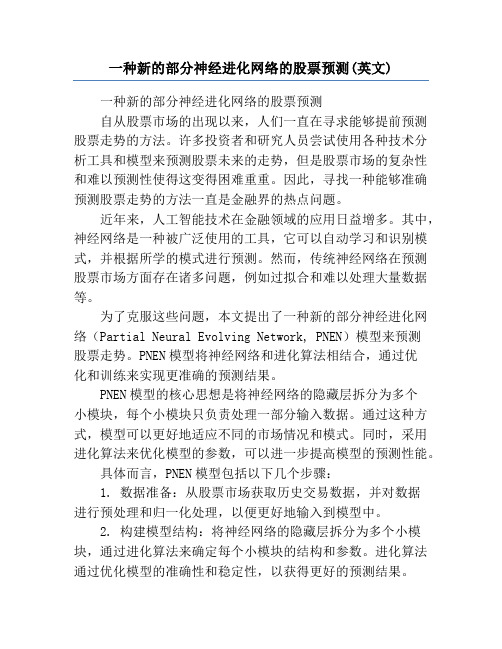
一种新的部分神经进化网络的股票预测(英文)一种新的部分神经进化网络的股票预测自从股票市场的出现以来,人们一直在寻求能够提前预测股票走势的方法。
许多投资者和研究人员尝试使用各种技术分析工具和模型来预测股票未来的走势,但是股票市场的复杂性和难以预测性使得这变得困难重重。
因此,寻找一种能够准确预测股票走势的方法一直是金融界的热点问题。
近年来,人工智能技术在金融领域的应用日益增多。
其中,神经网络是一种被广泛使用的工具,它可以自动学习和识别模式,并根据所学的模式进行预测。
然而,传统神经网络在预测股票市场方面存在诸多问题,例如过拟合和难以处理大量数据等。
为了克服这些问题,本文提出了一种新的部分神经进化网络(Partial Neural Evolving Network, PNEN)模型来预测股票走势。
PNEN模型将神经网络和进化算法相结合,通过优化和训练来实现更准确的预测结果。
PNEN模型的核心思想是将神经网络的隐藏层拆分为多个小模块,每个小模块只负责处理一部分输入数据。
通过这种方式,模型可以更好地适应不同的市场情况和模式。
同时,采用进化算法来优化模型的参数,可以进一步提高模型的预测性能。
具体而言,PNEN模型包括以下几个步骤:1. 数据准备:从股票市场获取历史交易数据,并对数据进行预处理和归一化处理,以便更好地输入到模型中。
2. 构建模型结构:将神经网络的隐藏层拆分为多个小模块,通过进化算法来确定每个小模块的结构和参数。
进化算法通过优化模型的准确性和稳定性,以获得更好的预测结果。
3. 训练模型:使用历史数据集对模型进行训练,并通过反向传播算法来更新模型的权重和偏置。
同时,通过与进化算法的交互,不断调整模型结构和参数。
4. 预测结果:使用训练好的模型对未来的股票走势进行预测。
通过模型对市场的分析和判断,可以为投资者提供决策参考。
为了验证PNEN模型的效果,我们在实际的股票市场数据上进行了实验。
结果表明,与传统神经网络模型相比,PNEN 模型在预测股票走势方面具有更好的准确性和稳定性。
Real-time Interaction in VR with a Distributed Multi-Agent System
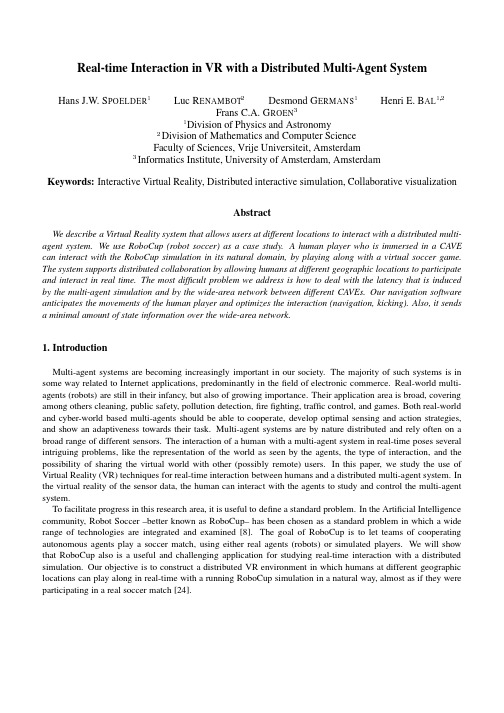
Real-time Interaction in VR with a Distributed Multi-Agent System Hans J.W.S POELDER Luc R ENAMBOT Desmond G ERMANS Henri E.B ALFrans C.A.G ROENDivision of Physics and AstronomyDivision of Mathematics and Computer ScienceFaculty of Sciences,Vrije Universiteit,AmsterdamInformatics Institute,University of Amsterdam,AmsterdamKeywords:Interactive Virtual Reality,Distributed interactive simulation,Collaborative visualizationAbstractWe describe a Virtual Reality system that allows users at different locations to interact with a distributed multi-agent system.We use RoboCup(robot soccer)as a case study.A human player who is immersed in a CAVE can interact with the RoboCup simulation in its natural domain,by playing along with a virtual soccer game. The system supports distributed collaboration by allowing humans at different geographic locations to participate and interact in real time.The most difficult problem we address is how to deal with the latency that is induced by the multi-agent simulation and by the wide-area network between different CAVEs.Our navigation software anticipates the movements of the human player and optimizes the interaction(navigation,kicking).Also,it sendsa minimal amount of state information over the wide-area network.1.IntroductionMulti-agent systems are becoming increasingly important in our society.The majority of such systems is in some way related to Internet applications,predominantly in thefield of electronic commerce.Real-world multi-agents(robots)are still in their infancy,but also of growing importance.Their application area is broad,covering among others cleaning,public safety,pollution detection,firefighting,traffic control,and games.Both real-world and cyber-world based multi-agents should be able to cooperate,develop optimal sensing and action strategies, and show an adaptiveness towards their task.Multi-agent systems are by nature distributed and rely often on a broad range of different sensors.The interaction of a human with a multi-agent system in real-time poses several intriguing problems,like the representation of the world as seen by the agents,the type of interaction,and the possibility of sharing the virtual world with other(possibly remote)users.In this paper,we study the use of Virtual Reality(VR)techniques for real-time interaction between humans and a distributed multi-agent system.In the virtual reality of the sensor data,the human can interact with the agents to study and control the multi-agent system.To facilitate progress in this research area,it is useful to define a standard problem.In the Artificial Intelligence community,Robot Soccer–better known as RoboCup–has been chosen as a standard problem in which a wide range of technologies are integrated and examined[8].The goal of RoboCup is to let teams of cooperating autonomous agents play a soccer match,using either real agents(robots)or simulated players.We will show that RoboCup also is a useful and challenging application for studying real-time interaction with a distributed simulation.Our objective is to construct a distributed VR environment in which humans at different geographic locations can play along in real-time with a running RoboCup simulation in a natural way,almost as if they were participating in a real soccer match[24].Our work on RoboCup differs from earlier case studies like virtual tennis[14]in that humans interact with a running distributed simulation program and not just with other humans.A human can take over the role of any of the simulated soccer players,which is useful for testing strategies that have not been coded in the simulation program yet.Interacting with a simulation program,however,is even harder to implement than interaction between humans.A simulation program will unavoidably introduce delays,which make a realistic real-time visualization and interaction a challenging problem,as we will discuss in depth in the paper.The paper is organized as follows.In Section2,we discuss related work.Section3introduces some basic concepts of RoboCup.In Section4,we present an overview of our virtual RoboCup environment.Some difficult implementation issues on real-time navigation,interaction,and remote collaboration are discussed in depth in Section5.Finally,we draw conclusions and discuss future work in Section6.The main contributions of the paper are as follows:1.We propose RoboCup as a useful case study for VR research on real-time interactions between humans andmulti-agent systems(or distributed simulation programs in general).2.We describe a prototype distributed implementation that allows humans at different geographic locationsto participate in the same virtual soccer match.This prototype has been demonstrated at the RoboCup’99 tournament in Stockholm,by playing a distributed virtual match using CA VEs[2]in Amsterdam and Stock-holm.3.We discuss one difficult and important problem in detail:how to achieve a natural interaction in the presenceof latencies.The latency problem is caused by the delay that the simulation introduces in generating the virtual reality.Also,when multiple CA VEs are used,the wide-area interconnection network increases the latency even further.The latency problem is general and also appears in other VR applications in which humans interact with simulations[22].The paper describes several experiments that give more insight into this problem.2.Related workInteractive and collaborative visualization radically change the way scientists use computer systems[4].With interactive visualization,a user can interact with a program in its visual domain.Distributed collaboration al-lows multiple users at different geographic locations to cooperate,by interacting in real time through a shared application[11,22].Many existing applications restrict the interaction to the visualization process(e.g.,the direction of view,the zoom factor).A more advanced form of interaction(i.e.,steering)allows the user to interact with the simulation process.Several systems exist that support steering[15,17],but they typically provide low-level interactions and require users to monitor or change the application program’s internal variables.In[21],the authors describe how robots can be steered from a Virtual Reality environment.Our RoboCup application allows the user to interact with the simulation program in a high-level,natural way.We think that this capability is beneficial to many scientific applications.Examples of such high-level interaction are reported in the literature for simulation of molecular docking and molecular dynamics[10,12].Although RoboCup may seem very different from scientific applications,it in fact can be used to study many issues:distributed multi-agent systems,teleimmersion,remote collaboration and man-multi-agent interaction.Our application is collaborative in that it allows real-time interaction between humans at different geographic locations,which is a challenge for the implementation.The system-induced latency and the network latency between the different sites become a difficult problem.Some applications address the network latency problem by using dedicated ATM links[6,9].In[16],the authors compare the performance of the users in achieving different tasks under several network conditions(varying latency and jitter)in a collaborative virtual environment.They2show that high latency reduces performance;the variability of the latency makes the use of prediction difficult and introduces a lack of hand-eye coordination.An interesting performance metric is the complete time(lag)in system response,including the simulation,tracking,rendering,network,and g over200msec makes interactivity difficult[25].We therefore try to minimize the amount of communication between the user and the simulation program.In particular,we couple remote users at the level of the simulation program and not at the visual level,allowing us to transfer the(small)simulation state instead of(large)images.Similar to our problem is the problem of remote modeling of objects in a large distributed system(large-scale military simulations or networked games).However,in RoboSoccer,there is only one centralized world state(at the Soccer Server),so techniques like Dead Reckoning or Position-History Based Dead Reckoning[23]cannot be applied.Our research also is related to other work on virtual games.Molet et al.implemented a collaborative environ-ment that is used for a virtual tennis game between humans at different locations[14].We do not study interaction between humans alone.Rather,we study interaction between humans and simulation programs.Also,we put much less emphasis on realistic modeling of human bodies.The RoboCup application raises interesting problems for the human-computer interaction,for example which input and output devices to use and how to manipulate objects in virtual environments.Another issue is the movement of the user in a limited area,as a soccerfield is much larger than the CA VE.We do not investigate this problem in our research and simply use the wand(i.e.,a3D mouse)to navigate,although this is not a natural way to navigate.One approach is to scale the user movements to the size of thefield when he goes out of a delimited zone[14].Other approaches have been proposed such as neural networks to detect walking movement[26]or hardware devices to allow locomotion[5].3.RoboCupRoboCup(or The Robot World Cup)attempts to promote intelligent robotics research by providing a common task for evaluating various theories,algorithms,and agent architectures.RoboCup comprises two sub-fields:real robots and simulations.This paper deals with the simulation part of RoboCup.Figure1.Software architecture of the RoboCup application The software architecture of the RoboCup application is shown in Figure1.The Translator and CAVE monitor are developed by us and are described later in the paper;the remaining components are part of existing RoboCup software and are described in[8].3Camera Viewevent driven(coupled to ball)2D Workbench point and selecthuman3D immersive1The original RoboCup simulation software can be found at 4Workbench (Delft)Figure2.Interactive and collaborative visualization of a soccer matchbetween camera positions according to a user-definable algorithm.This mode thus is an approximation of the standard TV coverage[3,7].The second mode is implemented on a workbench2and provides a3D overview of the soccer match.Its main feature is that visualization is miniaturized to allow a human to overview the state (without a predefined viewing angle).The goal here is to allow a coach to steer the game and give instructions to the players.We have implemented the2D camera system and we have a partial implementation of the workbench software.In the remainder of the paper,however,we focus on the third mode,the CA VE mode.The CA VE mode allows the user to be immersed in the game and to interact with it.We have implemented a new RoboCup monitor,the CAVE monitor,which uses the same information and communication as the original2D monitor(see Figure1),but now visualizes the state of the game in a CA VE.As a starting point in the visualization, we have built a virtual stadium and a parameterized soccer player.Animation of the players is based on the ‘walker’motion data included in the GLUT(OpenGL Utility Toolkit)distribution.The state of this walker is characterized byfive degrees of freedom.Its movement is governed by a set of basic points that are interpolated by splines.The movement as a whole is periodical.We discriminate three different modes of movement:standing still,walking,and running.The visualization of the movement is adjusted by linearly interpolating between the three modes.The visualization system has to compute several quantities from successive states of the game.The reason is that the soccer server tries to reduce the required communication bandwidth by sending a minimal amount of information to the visualization system.For example,the direction of movement and velocity of the players and events like kicking the ball have to be determined from two successive states of the game.Likewise,determining the acceleration requires three successive states.In addition to this visualization software,we have developed software to track the behavior of a human in the CA VE.We use three trackers to handle the interaction of the human with the VR software.One tracker is connected to the viewing glasses and is used to monitor positional changes of the human player inside the CA VE. The second tracker is connected to the wand,which can be used for global movements over the soccerfield.The third tracker is attached to the foot of the human player and is used to recognize a kick.The most difficult problem in realizing a virtual RoboCup system is caused by the latency of the simulation program(the Soccer Server).If the human player moves over the virtual soccerfield,these moves happen almost instantaneously for the human.In contrast,the Soccer Server will require some time to process the change of position.As a consequence,the position of the human in the CA VE may be different from the position stored inthe server.Such a difference will especially affect the kick command,since the RoboCup rules require the player to be within a certain distance from the ball to be able to kick it.The human player may thus think he is close enough to the virtual ball,but the server (the simulation)may have different information and ignore the kick.This problem is a typical example of how a delay introduced by a simulation program can harm a natural and real-time interaction[16].Direction of the kickFigure 3.Interaction radius and human position according to Soccer ServerTo study this problem,we introduce a visual cue for the human,called a blue disk .The center of the disk corresponds to the human position as currently stored by the server,and the radius corresponds with the interaction area (i.e.,the area in which the human is allowed to kick).This is illustrated in Figure ually,the blue disk will stay close to the human player.If not,the lack of tracking makes the user aware that,according to the server,he is making an illegal move.In practical experiments,we have determined that the human user can easily recognize the disk and does not experience its presence as distracting.More implementation details on this problem will be given in Section 5.Our virtual RoboCup environment also allows visualization front ends at different geographic locations to be coupled to the same simulation.In this respect,the environment is an example of a distributed collaborative application,which is becoming an important class of scientific applications [4].We have explored this possibility in a virtual soccer match played on August 3,1999using the CAVEs in Amsterdam and Stockholm.For this match,human players in both cities joined the team of simulated players.The large distance (1300kilometers)between the two CA VEs introduces a similar latency problem as described above:the actual position of the human in the remote CA VE may be different from the position currently stored at the Soccer Server.This holds especially for the human player in Stockholm,because the server and the simulated players were run in Amsterdam.We will describe and study this latency effect in Section 5.In the visualization,the remote human position is shown by a pyramid and the corresponding player is shown by an ordinary player.Arrows denote the viewing direction of the human in the remote CA VE.5.Implementation and Performance IssuesOur RoboCup system uses the standard Soccer Server that is part of the existing RoboCup software.We use player processes that were developed at the University of Amsterdam.We extended this software in several ways.First,we have implemented a CA VE monitor,which visualizes the soccer game in a CA VE,as discussed in the previous section.In addition,we adapted the virtual player process to allow a human to take over the identity of6one of the players,participate in the game,and interact with the simulation.For this purpose,we use a Translator process(see Figure1)that translates tracker changes into soccer commands and transmits these to the Soccer Server(just like the normal virtual players do).Finally,we coupled two CA VEs at different locations.The two CA VEs are connected to the same Soccer Server,so the two humans participate in the same game.The two CA VEs exchange tracking data to achieve this coupling,using the network functionalities of the CA VE library.3. TranslationPlayer1. Visualizationdraw stadiumanimate player 1animate ...draw ballFigure4.Temporal differenceThe major problem in implementing the system is its inherent delay,as illustrated in Figure4.At each cycle, the CA VE monitor receives state information from the Soccer Server.This data is expanded from2D to3D and visualized in the CA VE.The user then reacts to the world he is immersed in.These movements are converted by the translator process into commands,which are sent back to the Soccer Server.All these steps are processed in a pipelined manner,so the user reacts on the previous state of the Soccer Server,and his movements are processed during the next simulation step.This inherent lag of the system significantly influences the navigation, the interaction,and the collaboration,as we describe below.To study and optimize our system,we have done several experiments,using the CA VE located at SARA Am-sterdam[20].This CA VE is connected to an IBM SP/2,which runs the RoboCup simulation(using multiple processors for the players and other processes).For the wide-area experiments,we use a second CA VE,located at Stockholm[18],which also is connected to a local SP/2.Our goal is to let the human player in the CA VE be able to kick the ball when he wants.This is only possible if,according to the Soccer Server,the human is close enough to the ball.Thus,we have to reduce the distance between the position of the user in the CA VE and the corresponding position in the simulated world as much as possible,to offer natural interaction.One obvious solution could have been to sample a joystick(mounted on the wand)and to emit the directly corresponding commands(forward motion means dash,sideways motion means turn).The visualization is then updated for the next cycle with the monitor data.When using such an approach, however,the user always reacts on a past view.Also,his movements are limited,so he experiences non-natural interaction.Moreover,the refresh rate of the monitor data from the Soccer Server is only10Hz,which is too slow to provide smooth visualization.Below,we explain our solution to this problem in more detail.We have tested our system with two’benchmark’trajectories.One is the path of a simulated player of the ’CMUnited98’-team(CMU-track),taken from thefinal of the1998RoboCup Simulator Tournament.This trajec-tory is originally built out of simulation-native turn and dash-commands.It is ideally suited to test the algorithm. The second benchmark is the path of a human in the CA VE that uses a joystick(mounted on the wand)to steer the player around the soccerfield(Joystick-track).Because this trajectory is not native to the Soccer Server,we expect it to be harder to follow.We replayed these two trajectories,varied the dash-factor parameter of our algorithm(see7below),and measured the average distance between the user and the corresponding simulated player.5.1.Navigationin the simulationin the virtual worldSoccer fieldFigure5.Difference between human and represented player As described above,the translator process receives changes in the position and orientation of the human player in the CA VE.It compares this information with the position and orientation stored in the simulation(see Figure5). It then tries to emit a soccer command that reduces the difference between the two(i.e.,which will bring the simulated position and orientation close to those of the human).Since the Soccer Server will accept only one command per100msec,the translator waits until the Soccer Server has acknowledged the previous command before sending a new command.An interesting problem is which command should be emitted by the translator.Both the position and orientation may differ between the virtual world and the simulation.Ideally,the translator thus should emit a turn-command to cover the difference in orientation(the angle)and at the same time a dash command to cover the distance, as illustrated in Figure5.Unfortunately,the Soccer Server allows only one command per cycle,so we need a way to serialize turn and dash-commands.We tried several strategies and found that the most accurate strategy is simply to alternate the turn and dash-commands.Unlike other strategies,this gives two steady streams of commands(with afixed time between each consecutive command)for both the angle and the distance.This property allows us to optimize the turn and dash-parameters by using simple extrapolations and by partially modeling the inverse of the Soccer Server.Taken this into account,the algorithm is left with one variable parameter,the dash-factor.The algorithm is constructed in such a way that this parameter should have a value of100.0momentum units to keep the distance minimal.Figure6shows the results for both trajectories in local and wide-area setups.The shaded area indicates the interaction range,the distance at which the player is allowed to kick the ball.It has been set to one meter by the Soccer Server.As expected,the wide-area results show a larger average distance.In this case,the algorithm needs to recover from network latency-effects and glitches common to the Internet.Furthermore,the algorithm clearly prefers motions that are native to the Soccer Server.The average distances for the CMU-track are smaller than those of the joystick-track.Due to the large amount of noise in the system,even when using average distances,it is not clear which dash-factor is optimal for the tested trajectories.The results show roughly that a dash-factor below80increases the81101009080706050012dash-factor (momentum units)d i s t a n c e (m e t e r s )Figure 6.Dash-factor influence on average distanceaverage distance.For these low-strength cases,the algorithm can not keep up with the speed of the human in the CA VE.5.2.InteractionFull interaction with a RoboCup soccer game consists furthermore of being able to kick the virtual ball.For this,we added a third tracker to the CA VE setup at SARA.The VR software recognizes a kick-command using this tracker,attached to the preferred foot of the human.A kick-command is issued when three conditions are met.Firstly,the tangential speed of the foot must be above a set threshold.Furthermore,the human must be within kicking-range of the ball,and finally,the foot has to move towards the ball.The force-parameter of the kick-command is derived from the instantaneous speed of the foot,and the angle-parameter is derived from the instantaneous direction of the foot.Both speed and direction are sampled at the point where the speed reaches a local ing this approach it is possible to give both hard and soft (dribbling)kicks.Since the accuracy of the trackers we use (Ascension Flock-of-Birds magnetic trackers)is limited,especially when the tracker is at a highly asymmetric position as is the case for the foot tracker,the detection of the kick from this data is rather crude.Figure 7shows the distribution of distances measured for the CMU-track both locally and in a wide-area setup.It shows that 50%of the time (indicated by the line marked ’50%’in Figure 7),the distance is below 1meter and 90%of the time (indicated by the line marked ’90%’in Figure 7),it is below 1.6meters making natural interaction possible in most but not all cases.The tail (indicated as ’large latency errors’in Figure 7)is larger for the wide-area case.This is expected,because the algorithm recovers more from latency problems in the wide-area setup.Currently we are preparing experiments to test the ability of experienced and inexperienced users to play soc-cer in our virtual environment.It should consist of some local exercises (dribbling,following a path,moving around objects,etc.)and some global tasks (running across the field with the ball,kicking accurately over long distances,etc.).The experiments should give more insight on the effectivity of natural interaction in the RoboCup environment.5.3.CollaborationDuring the RoboCup’99event,we played a soccer match between two human players in two CA VES (in Am-sterdam and in Stockholm).The Soccer Server was located in Amsterdam,so the Amsterdam-player experienced900.51 1.52 2.53 3.54o c c u p a t i o n (r e l. #)distance (meters)Figure 7.Distance-histogram for CMU-tracklocal latency effects,whereas the Stockholm-player experienced wide-area latency effects.Even though this dif-ference was noticeable in the soccer experience on both sides,the game was a success.6.Conclusion and Future workWe have described a Virtual Reality system that allows users at different locations to interact with a distributed multi-agent system.For our case study,RoboCup,the interaction is natural and is similar to that of a human soccer player:the user in a CA VE can kick a virtual ball.The most difficult problem we addressed is how to deal with the latency that is induced by the multi-agent simulation and by the wide-area network that connects different CA VEs.This latency causes a difference between the real position of the human in the virtual space and the position stored by the tency is unavoidable,so to some extent it is a problem one has to live with.We reduce the impact of the latency problem in several ways.Our navigation software anticipates the movements of the human player and tries to reduce the difference in the positions.Also,we provide visual feedback to the user,showing the current position according to the simulation.Finally,we exploit the principle of compression and send a minimal amount of state information over the network.Rather than sending complete images,we transmit the state of the soccer game (which is much smaller)and expand this state locally to 3D images.This is especially important for communication over (slow)wide-area networks.In our current and future work,we also study other scientific applications that can exploit interactive and collaborative visualization [19].Example applications we intend to investigate are simulation of nonlinear systems (e.g.,lasers),based on our earlier work described in [13],visualization of molecular dynamics,and interactive visualization of the cornea of the human eye [27,28].Such scientific applications will exhibit similar problems as those identified for RoboCup.For example,visualization of the cornea would be very useful during eye surgery,but the modeling of the cornea is a computation-intensive process,resulting in latency problems.We are currently studying how parallel cluster computing [1]may reduce the latency and allow the visualization to be done in real-time.References[1]H.Bal,R.Bhoedjang,R.Hofman,C.Jacobs,ngendoen,T.R¨u hl,and F.Kaashoek.Performance Evaluation ofthe Orca Shared Object System.ACM Transactions on Computer Systems ,16(1):1–40,Feb.1998.10[2] C.Cruz-Neira,D.J.Sandin,T.A.DeFanti,R.V.Kenyon,and J.C.Hart.The CA VE:audio visual experience automaticvirtual munications of the ACM,35(6):64–72,June1992.[3]S.M.Drucker and D.Zeltzer.CamDroid:A system for implementing intelligent camera control.In1995Symposiumon Interactive3D Graphics,pages139–144,Apr.1995.[4] A.Foster and C.Kesselman.The Grid:Blueprint for a New Computer Infrastructure.Morgan Kaufman,1998.[5]H.Iwata.Walking About Virtual Environments on an Infinite Floor.In IEEE Virtual Reality’99,pages286–293,1999.[6] A.Johnson,J.Leigh,and J.Costigan.Projects in VR:Multiway tele-immersion at Supercomputing97.IEEE ComputerGraphics and Applications,18(4):6–9,July/Aug.1998.[7]P.Karp and S.Feiner.Automated presentation planning of animation using task decomposition with heuristic reasoning.In Graphics Interface’93,pages118–127,May1993.[8]H.Kitano,M.Veloso,P.Stone,M.Tambe,S.Coradeschi,E.Osawa,I.Noda,H.Matsubara,and M.Asada.TheRoboCup Synthetic Agents Challenge97.In M.Pollack,editor,15th International Joint Conference on Artificial Intelligence,pages24–29,1997.[9]motte,E.Flerackers,F.Van Reeth,R.Earnshaw,and J.De Matos.Visinet:Collaborative3D Visualization andVR over ATM Networks.IEEE Computer Graphics&Applications,17(2):66–75,Mar.-Apr.1997.[10]J.Leech,J.Prins,and J.Hermans.SMD:Visual Steering of Molecular Dynamics for Protein Design.IEEE Computa-tional Science&Engineering,3(4):38–45,Winter1996.[11]J.Leigh,A.Johnson,T.DeFanti,and M.Brown.A Review of Tele-Immersive Applications in the CA VE ResearchNetwork.In IEEE Virtual Reality’99,pages180–187,1999.[12]D.Levine,M.Facello,P.Hallstrom,G.Reeder,B.Walenz,and F.Stevens.Stalk:An Interactive System for VirtualMolecular Docking.IEEE Computational Science,4(2):55–65,April-June1997.[13]C.Mirasso,M.Mulder,H.Spoelder,and D.Lenstra.Visualization of the Sisyphus puters in Physics,11(3):282–286,May/June1997.[14]T.Molet,A.Aubel,T.Gapin,S.Carion,E.Lee,N.Magnenat-Thalmann,H.Noser,I.Pandzic,G.Sannier,andD.Thalmann.Anyone for Tennis?Presence,8(2):140–156,Apr.1999.[15]J.Mulder,J.van Wijk,and R.van Liere.A Survey of Computational Steering Environments.Future GenerationComputer Systems,13(6),1998.[16]K.S.Park and R.Kenyon.Effects of Network Characteristics on Human Performance in a Collaborative VirtualEnvironment.In IEEE Virtual Reality’99,pages104–111,1999.[17]S.Parker,ler,C.Hansen,and C.Johnson.An Integrated Problem Solving Environment:the SCIrun Computa-tional Steering System.In Hawaii International Conference of System Sciences,pages147–156,Jan.1998.[18]Center for Parallel Computers,Royal Institute of Technology,Stockholm.http://www.pdc.kth.se.[19]L.Renambot,H.Bal,D.Germans,and H.Spoelder.CA VEStudy:an Infrastructure for Computational Steering inVirtual Reality Environments.Technical report,Vrije Universiteit Amsterdam,Faculty of Sciences,Mar.2000.[20]SARA,Academic Computing Services Amsterdam.http://www.sara.nl.[21]K.Simsarian,L.Fahlen,and E.Frecon.Virtually Telling Robots What To Do.In Informatique Montpellier1995,Interface to Real and Virtual worlds.,1995.[22]S.Singhal and worked Virtual Environments:Design and Implementation.Addison-Wesley,1999.[23]S.K.Singhal.Effective remote modeling in large-scale distributed simulation and visualization environments.Ph.D.Thesis CS-TR-96-1574,Stanford University,Department of Computer Science,Sept.1996.[24]H.Spoelder,L.Renambot,D.Germans,H.Bal,and F.Groen.Man Multi-Agent Interaction in VR:a Case Study withRoboCup.In IEEE Virtual Reality2000(poster),New Brunswick,NJ,Mar.2000.[25]V.E.Taylor,J.Chen,T.L.Disz,M.E.Papka,and R.Stevens.Interactive Virtual Reality in Simulations:ExploringLag Time.IEEE Computational Science&Engineering,3(4):46–54,1996.[26]oh,K.Arthur,M.Whitton,R.Bastos,A.Steed,M.Slater,and F.Brooks.Walking Walking-in-Place Flying,in Virtual Environments.In Computer Graphics,ACM SIGGRAPH’99Proceedings,1999.[27]F.V os and H.Spoelder.Visualization in Corneal Topography.In IEEE Visualization’98,pages427–430,Oct.1998.[28]F.V os,G.van der Heijde,H.Spoelder,I.van Stokkum,and F.Groen.A new PRBA-based Instrument to Measure theShape of the Cornea.IEEE Trans.on Instrum.Meas.,46(4):794–797,1997.11。
复杂动态网络的合作控制
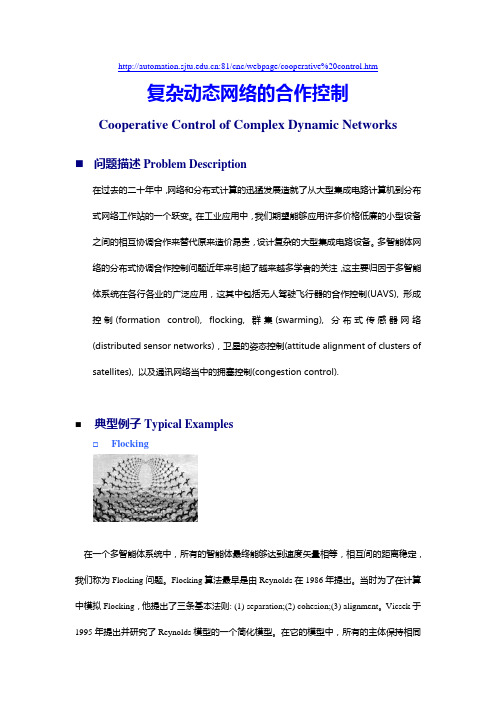
:81/cnc/webpage/cooperative%20control.htm复杂动态网络的合作控制Cooperative Control of Complex Dynamic Networks⏹问题描述 Problem Description在过去的二十年中,网络和分布式计算的迅猛发展造就了从大型集成电路计算机到分布式网络工作站的一个跃变。
在工业应用中,我们期望能够应用许多价格低廉的小型设备之间的相互协调合作来替代原来造价昂贵,设计复杂的大型集成电路设备。
多智能体网络的分布式协调合作控制问题近年来引起了越来越多学者的关注,这主要归因于多智能体系统在各行各业的广泛应用,这其中包括无人驾驶飞行器的合作控制(UAVS), 形成控制(formation control), flocking, 群集(swarming), 分布式传感器网络(distributed sensor networks),卫星的姿态控制(attitude alignment of clusters of satellites), 以及通讯网络当中的拥塞控制(congestion control).⏹典型例子 Typical Examples☐Flocking在一个多智能体系统中,所有的智能体最终能够达到速度矢量相等,相互间的距离稳定,我们称为Flocking问题。
Flocking算法最早是由Reynolds在1986年提出。
当时为了在计算中模拟Flocking,他提出了三条基本法则: (1) separation;(2) cohesion;(3) alignment。
Vicsek于1995年提出并研究了Reynolds模型的一个简化模型。
在它的模型中,所有的主体保持相同的速度运行,这个仅仅体现了Reynolds算法中的alignment。
近年来,许多控制学者也在研究Flocking问题,他们通过构建微分方程组将Flocking问题进行抽象化,利用人工势能结合速度一致(consensus)的方法来实现Flocking算法。
Consensus and Cooperation in Networked Multi-Agent Systems
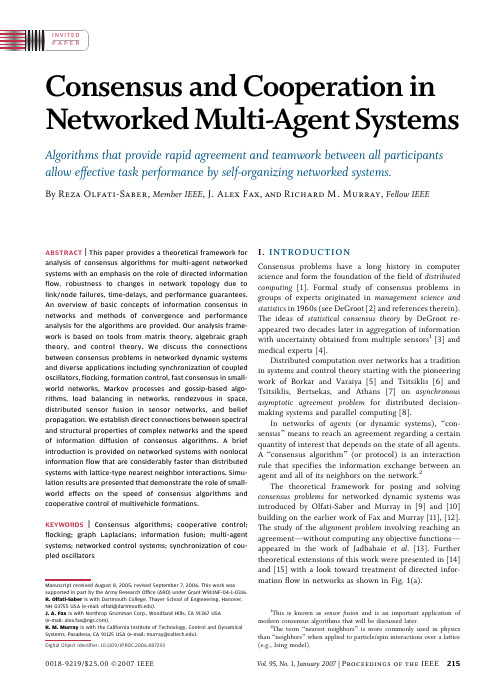
Consensus and Cooperation in Networked Multi-Agent SystemsAlgorithms that provide rapid agreement and teamwork between all participants allow effective task performance by self-organizing networked systems.By Reza Olfati-Saber,Member IEEE,J.Alex Fax,and Richard M.Murray,Fellow IEEEABSTRACT|This paper provides a theoretical framework for analysis of consensus algorithms for multi-agent networked systems with an emphasis on the role of directed information flow,robustness to changes in network topology due to link/node failures,time-delays,and performance guarantees. An overview of basic concepts of information consensus in networks and methods of convergence and performance analysis for the algorithms are provided.Our analysis frame-work is based on tools from matrix theory,algebraic graph theory,and control theory.We discuss the connections between consensus problems in networked dynamic systems and diverse applications including synchronization of coupled oscillators,flocking,formation control,fast consensus in small-world networks,Markov processes and gossip-based algo-rithms,load balancing in networks,rendezvous in space, distributed sensor fusion in sensor networks,and belief propagation.We establish direct connections between spectral and structural properties of complex networks and the speed of information diffusion of consensus algorithms.A brief introduction is provided on networked systems with nonlocal information flow that are considerably faster than distributed systems with lattice-type nearest neighbor interactions.Simu-lation results are presented that demonstrate the role of small-world effects on the speed of consensus algorithms and cooperative control of multivehicle formations.KEYWORDS|Consensus algorithms;cooperative control; flocking;graph Laplacians;information fusion;multi-agent systems;networked control systems;synchronization of cou-pled oscillators I.INTRODUCTIONConsensus problems have a long history in computer science and form the foundation of the field of distributed computing[1].Formal study of consensus problems in groups of experts originated in management science and statistics in1960s(see DeGroot[2]and references therein). The ideas of statistical consensus theory by DeGroot re-appeared two decades later in aggregation of information with uncertainty obtained from multiple sensors1[3]and medical experts[4].Distributed computation over networks has a tradition in systems and control theory starting with the pioneering work of Borkar and Varaiya[5]and Tsitsiklis[6]and Tsitsiklis,Bertsekas,and Athans[7]on asynchronous asymptotic agreement problem for distributed decision-making systems and parallel computing[8].In networks of agents(or dynamic systems),B con-sensus[means to reach an agreement regarding a certain quantity of interest that depends on the state of all agents.A B consensus algorithm[(or protocol)is an interaction rule that specifies the information exchange between an agent and all of its neighbors on the network.2 The theoretical framework for posing and solving consensus problems for networked dynamic systems was introduced by Olfati-Saber and Murray in[9]and[10] building on the earlier work of Fax and Murray[11],[12]. The study of the alignment problem involving reaching an agreement V without computing any objective functions V appeared in the work of Jadbabaie et al.[13].Further theoretical extensions of this work were presented in[14] and[15]with a look toward treatment of directed infor-mation flow in networks as shown in Fig.1(a).Manuscript received August8,2005;revised September7,2006.This work was supported in part by the Army Research Office(ARO)under Grant W911NF-04-1-0316. R.Olfati-Saber is with Dartmouth College,Thayer School of Engineering,Hanover,NH03755USA(e-mail:olfati@).J.A.Fax is with Northrop Grumman Corp.,Woodland Hills,CA91367USA(e-mail:alex.fax@).R.M.Murray is with the California Institute of Technology,Control and Dynamical Systems,Pasadena,CA91125USA(e-mail:murray@).Digital Object Identifier:10.1109/JPROC.2006.8872931This is known as sensor fusion and is an important application of modern consensus algorithms that will be discussed later.2The term B nearest neighbors[is more commonly used in physics than B neighbors[when applied to particle/spin interactions over a lattice (e.g.,Ising model).Vol.95,No.1,January2007|Proceedings of the IEEE2150018-9219/$25.00Ó2007IEEEThe common motivation behind the work in [5],[6],and [10]is the rich history of consensus protocols in com-puter science [1],whereas Jadbabaie et al.[13]attempted to provide a formal analysis of emergence of alignment in the simplified model of flocking by Vicsek et al.[16].The setup in [10]was originally created with the vision of de-signing agent-based amorphous computers [17],[18]for collaborative information processing in ter,[10]was used in development of flocking algorithms with guaranteed convergence and the capability to deal with obstacles and adversarial agents [19].Graph Laplacians and their spectral properties [20]–[23]are important graph-related matrices that play a crucial role in convergence analysis of consensus and alignment algo-rithms.Graph Laplacians are an important point of focus of this paper.It is worth mentioning that the second smallest eigenvalue of graph Laplacians called algebraic connectivity quantifies the speed of convergence of consensus algo-rithms.The notion of algebraic connectivity of graphs has appeared in a variety of other areas including low-density parity-check codes (LDPC)in information theory and com-munications [24],Ramanujan graphs [25]in number theory and quantum chaos,and combinatorial optimization prob-lems such as the max-cut problem [21].More recently,there has been a tremendous surge of interest V among researchers from various disciplines of engineering and science V in problems related to multia-gent networked systems with close ties to consensus prob-lems.This includes subjects such as consensus [26]–[32],collective behavior of flocks and swarms [19],[33]–[37],sensor fusion [38]–[40],random networks [41],[42],syn-chronization of coupled oscillators [42]–[46],algebraic connectivity 3of complex networks [47]–[49],asynchro-nous distributed algorithms [30],[50],formation control for multirobot systems [51]–[59],optimization-based co-operative control [60]–[63],dynamic graphs [64]–[67],complexity of coordinated tasks [68]–[71],and consensus-based belief propagation in Bayesian networks [72],[73].A detailed discussion of selected applications will be pre-sented shortly.In this paper,we focus on the work described in five key papers V namely,Jadbabaie,Lin,and Morse [13],Olfati-Saber and Murray [10],Fax and Murray [12],Moreau [14],and Ren and Beard [15]V that have been instrumental in paving the way for more recent advances in study of self-organizing networked systems ,or swarms .These networked systems are comprised of locally interacting mobile/static agents equipped with dedicated sensing,computing,and communication devices.As a result,we now have a better understanding of complex phenomena such as flocking [19],or design of novel information fusion algorithms for sensor networks that are robust to node and link failures [38],[72]–[76].Gossip-based algorithms such as the push-sum protocol [77]are important alternatives in computer science to Laplacian-based consensus algorithms in this paper.Markov processes establish an interesting connection between the information propagation speed in these two categories of algorithms proposed by computer scientists and control theorists [78].The contribution of this paper is to present a cohesive overview of the key results on theory and applications of consensus problems in networked systems in a unified framework.This includes basic notions in information consensus and control theoretic methods for convergence and performance analysis of consensus protocols that heavily rely on matrix theory and spectral graph theory.A byproduct of this framework is to demonstrate that seem-ingly different consensus algorithms in the literature [10],[12]–[15]are closely related.Applications of consensus problems in areas of interest to researchers in computer science,physics,biology,mathematics,robotics,and con-trol theory are discussed in this introduction.A.Consensus in NetworksThe interaction topology of a network of agents is rep-resented using a directed graph G ¼ðV ;E Þwith the set of nodes V ¼f 1;2;...;n g and edges E V ÂV .TheFig.1.Two equivalent forms of consensus algorithms:(a)a networkof integrator agents in which agent i receives the state x j of its neighbor,agent j ,if there is a link ði ;j Þconnecting the two nodes;and (b)the block diagram for a network of interconnecteddynamic systems all with identical transfer functions P ðs Þ¼1=s .The collective networked system has a diagonal transfer function and is a multiple-input multiple-output (MIMO)linear system.3To be defined in Section II-A.Olfati-Saber et al.:Consensus and Cooperation in Networked Multi-Agent Systems216Proceedings of the IEEE |Vol.95,No.1,January 2007neighbors of agent i are denoted by N i ¼f j 2V :ði ;j Þ2E g .According to [10],a simple consensus algorithm to reach an agreement regarding the state of n integrator agents with dynamics _x i ¼u i can be expressed as an n th-order linear system on a graph_x i ðt Þ¼X j 2N ix j ðt ÞÀx i ðt ÞÀÁþb i ðt Þ;x i ð0Þ¼z i2R ;b i ðt Þ¼0:(1)The collective dynamics of the group of agents following protocol (1)can be written as_x ¼ÀLx(2)where L ¼½l ij is the graph Laplacian of the network and itselements are defined as follows:l ij ¼À1;j 2N i j N i j ;j ¼i :&(3)Here,j N i j denotes the number of neighbors of node i (or out-degree of node i ).Fig.1shows two equivalent forms of the consensus algorithm in (1)and (2)for agents with a scalar state.The role of the input bias b in Fig.1(b)is defined later.According to the definition of graph Laplacian in (3),all row-sums of L are zero because of P j l ij ¼0.Therefore,L always has a zero eigenvalue 1¼0.This zero eigenvalues corresponds to the eigenvector 1¼ð1;...;1ÞT because 1belongs to the null-space of L ðL 1¼0Þ.In other words,an equilibrium of system (2)is a state in the form x üð ;...; ÞT ¼ 1where all nodes agree.Based on ana-lytical tools from algebraic graph theory [23],we later show that x Ãis a unique equilibrium of (2)(up to a constant multiplicative factor)for connected graphs.One can show that for a connected network,the equilibrium x üð ;...; ÞT is globally exponentially stable.Moreover,the consensus value is ¼1=n P i z i that is equal to the average of the initial values.This im-plies that irrespective of the initial value of the state of each agent,all agents reach an asymptotic consensus regarding the value of the function f ðz Þ¼1=n P i z i .While the calculation of f ðz Þis simple for small net-works,its implications for very large networks is more interesting.For example,if a network has n ¼106nodes and each node can only talk to log 10ðn Þ¼6neighbors,finding the average value of the initial conditions of the nodes is more complicated.The role of protocol (1)is to provide a systematic consensus mechanism in such a largenetwork to compute the average.There are a variety of functions that can be computed in a similar fashion using synchronous or asynchronous distributed algorithms (see [10],[28],[30],[73],and [76]).B.The f -Consensus Problem and Meaning of CooperationTo understand the role of cooperation in performing coordinated tasks,we need to distinguish between un-constrained and constrained consensus problems.An unconstrained consensus problem is simply the alignment problem in which it suffices that the state of all agents asymptotically be the same.In contrast,in distributed computation of a function f ðz Þ,the state of all agents has to asymptotically become equal to f ðz Þ,meaning that the consensus problem is constrained.We refer to this con-strained consensus problem as the f -consensus problem .Solving the f -consensus problem is a cooperative task and requires willing participation of all the agents.To demonstrate this fact,suppose a single agent decides not to cooperate with the rest of the agents and keep its state unchanged.Then,the overall task cannot be performed despite the fact that the rest of the agents reach an agree-ment.Furthermore,there could be scenarios in which multiple agents that form a coalition do not cooperate with the rest and removal of this coalition of agents and their links might render the network disconnected.In a dis-connected network,it is impossible for all nodes to reach an agreement (unless all nodes initially agree which is a trivial case).From the above discussion,cooperation can be infor-mally interpreted as B giving consent to providing one’s state and following a common protocol that serves the group objective.[One might think that solving the alignment problem is not a cooperative task.The justification is that if a single agent (called a leader)leaves its value unchanged,all others will asymptotically agree with the leader according to the consensus protocol and an alignment is reached.However,if there are multiple leaders where two of whom are in disagreement,then no consensus can be asymptot-ically reached.Therefore,alignment is in general a coop-erative task as well.Formal analysis of the behavior of systems that involve more than one type of agent is more complicated,partic-ularly,in presence of adversarial agents in noncooperative games [79],[80].The focus of this paper is on cooperative multi-agent systems.C.Iterative Consensus and Markov ChainsIn Section II,we show how an iterative consensus algorithm that corresponds to the discrete-time version of system (1)is a Markov chainðk þ1Þ¼ ðk ÞP(4)Olfati-Saber et al.:Consensus and Cooperation in Networked Multi-Agent SystemsVol.95,No.1,January 2007|Proceedings of the IEEE217with P ¼I À L and a small 90.Here,the i th element of the row vector ðk Þdenotes the probability of being in state i at iteration k .It turns out that for any arbitrary graph G with Laplacian L and a sufficiently small ,the matrix P satisfies the property Pj p ij ¼1with p ij !0;8i ;j .Hence,P is a valid transition probability matrix for the Markov chain in (4).The reason matrix theory [81]is so widely used in analysis of consensus algorithms [10],[12]–[15],[64]is primarily due to the structure of P in (4)and its connection to graphs.4There are interesting connections between this Markov chain and the speed of information diffusion in gossip-based averaging algorithms [77],[78].One of the early applications of consensus problems was dynamic load balancing [82]for parallel processors with the same structure as system (4).To this date,load balancing in networks proves to be an active area of research in computer science.D.ApplicationsMany seemingly different problems that involve inter-connection of dynamic systems in various areas of science and engineering happen to be closely related to consensus problems for multi-agent systems.In this section,we pro-vide an account of the existing connections.1)Synchronization of Coupled Oscillators:The problem of synchronization of coupled oscillators has attracted numer-ous scientists from diverse fields including physics,biology,neuroscience,and mathematics [83]–[86].This is partly due to the emergence of synchronous oscillations in coupled neural oscillators.Let us consider the generalized Kuramoto model of coupled oscillators on a graph with dynamics_i ¼ Xj 2N isin ð j À i Þþ!i (5)where i and !i are the phase and frequency of the i thoscillator.This model is the natural nonlinear extension of the consensus algorithm in (1)and its linearization around the aligned state 1¼...¼ n is identical to system (2)plus a nonzero input bias b i ¼ð!i À"!Þ= with "!¼1=n P i !i after a change of variables x i ¼ð i À"!t Þ= .In [43],Sepulchre et al.show that if is sufficiently large,then for a network with all-to-all links,synchroni-zation to the aligned state is globally achieved for all ini-tial states.Recently,synchronization of networked oscillators under variable time-delays was studied in [45].We believe that the use of convergence analysis methods that utilize the spectral properties of graph Laplacians willshed light on performance and convergence analysis of self-synchrony in oscillator networks [42].2)Flocking Theory:Flocks of mobile agents equipped with sensing and communication devices can serve as mobile sensor networks for massive distributed sensing in an environment [87].A theoretical framework for design and analysis of flocking algorithms for mobile agents with obstacle-avoidance capabilities is developed by Olfati-Saber [19].The role of consensus algorithms in particle-based flocking is for an agent to achieve velocity matching with respect to its neighbors.In [19],it is demonstrated that flocks are networks of dynamic systems with a dynamic topology.This topology is a proximity graph that depends on the state of all agents and is determined locally for each agent,i.e.,the topology of flocks is a state-dependent graph.The notion of state-dependent graphs was introduced by Mesbahi [64]in a context that is independent of flocking.3)Fast Consensus in Small-Worlds:In recent years,network design problems for achieving faster consensus algorithms has attracted considerable attention from a number of researchers.In Xiao and Boyd [88],design of the weights of a network is considered and solved using semi-definite convex programming.This leads to a slight increase in algebraic connectivity of a network that is a measure of speed of convergence of consensus algorithms.An alternative approach is to keep the weights fixed and design the topology of the network to achieve a relatively high algebraic connectivity.A randomized algorithm for network design is proposed by Olfati-Saber [47]based on random rewiring idea of Watts and Strogatz [89]that led to creation of their celebrated small-world model .The random rewiring of existing links of a network gives rise to considerably faster consensus algorithms.This is due to multiple orders of magnitude increase in algebraic connectivity of the network in comparison to a lattice-type nearest-neighbort graph.4)Rendezvous in Space:Another common form of consensus problems is rendezvous in space [90],[91].This is equivalent to reaching a consensus in position by a num-ber of agents with an interaction topology that is position induced (i.e.,a proximity graph).We refer the reader to [92]and references therein for a detailed discussion.This type of rendezvous is an unconstrained consensus problem that becomes challenging under variations in the network topology.Flocking is somewhat more challenging than rendezvous in space because it requires both interagent and agent-to-obstacle collision avoidance.5)Distributed Sensor Fusion in Sensor Networks:The most recent application of consensus problems is distrib-uted sensor fusion in sensor networks.This is done by posing various distributed averaging problems require to4In honor of the pioneering contributions of Oscar Perron (1907)to the theory of nonnegative matrices,were refer to P as the Perron Matrix of graph G (See Section II-C for details).Olfati-Saber et al.:Consensus and Cooperation in Networked Multi-Agent Systems218Proceedings of the IEEE |Vol.95,No.1,January 2007implement a Kalman filter [38],[39],approximate Kalman filter [74],or linear least-squares estimator [75]as average-consensus problems .Novel low-pass and high-pass consensus filters are also developed that dynamically calculate the average of their inputs in sensor networks [39],[93].6)Distributed Formation Control:Multivehicle systems are an important category of networked systems due to their commercial and military applications.There are two broad approaches to distributed formation control:i)rep-resentation of formations as rigid structures [53],[94]and the use of gradient-based controls obtained from their structural potentials [52]and ii)representation of form-ations using the vectors of relative positions of neighboring vehicles and the use of consensus-based controllers with input bias.We discuss the later approach here.A theoretical framework for design and analysis of distributed controllers for multivehicle formations of type ii)was developed by Fax and Murray [12].Moving in formation is a cooperative task and requires consent and collaboration of every agent in the formation.In [12],graph Laplacians and matrix theory were extensively used which makes one wonder whether relative-position-based formation control is a consensus problem.The answer is yes.To see this,consider a network of self-interested agents whose individual desire is to minimize their local cost U i ðx Þ¼Pj 2N i k x j Àx i Àr ij k 2via a distributed algorithm (x i is the position of vehicle i with dynamics _x i ¼u i and r ij is a desired intervehicle relative-position vector).Instead,if the agents use gradient-descent algorithm on the collective cost P n i ¼1U i ðx Þusing the following protocol:_x i ¼Xj 2N iðx j Àx i Àr ij Þ¼Xj 2N iðx j Àx i Þþb i (6)with input bias b i ¼Pj 2N i r ji [see Fig.1(b)],the objective of every agent will be achieved.This is the same as the consensus algorithm in (1)up to the nonzero bias terms b i .This nonzero bias plays no role in stability analysis of sys-tem (6).Thus,distributed formation control for integrator agents is a consensus problem.The main contribution of the work by Fax and Murray is to extend this scenario to the case where all agents are multiinput multioutput linear systems _x i ¼Ax i þBu i .Stability analysis of relative-position-based formation control for multivehicle systems is extensively covered in Section IV.E.OutlineThe outline of the paper is as follows.Basic concepts and theoretical results in information consensus are presented in Section II.Convergence and performance analysis of consensus on networks with switching topology are given in Section III.A theoretical framework for cooperative control of formations of networked multi-vehicle systems is provided in Section IV.Some simulationresults related to consensus in complex networks including small-worlds are presented in Section V.Finally,some concluding remarks are stated in Section VI.RMATION CONSENSUSConsider a network of decision-making agents with dynamics _x i ¼u i interested in reaching a consensus via local communication with their neighbors on a graph G ¼ðV ;E Þ.By reaching a consensus,we mean asymptot-ically converging to a one-dimensional agreement space characterized by the following equation:x 1¼x 2¼...¼x n :This agreement space can be expressed as x ¼ 1where 1¼ð1;...;1ÞT and 2R is the collective decision of the group of agents.Let A ¼½a ij be the adjacency matrix of graph G .The set of neighbors of a agent i is N i and defined byN i ¼f j 2V :a ij ¼0g ;V ¼f 1;...;n g :Agent i communicates with agent j if j is a neighbor of i (or a ij ¼0).The set of all nodes and their neighbors defines the edge set of the graph as E ¼fði ;j Þ2V ÂV :a ij ¼0g .A dynamic graph G ðt Þ¼ðV ;E ðt ÞÞis a graph in which the set of edges E ðt Þand the adjacency matrix A ðt Þare time-varying.Clearly,the set of neighbors N i ðt Þof every agent in a dynamic graph is a time-varying set as well.Dynamic graphs are useful for describing the network topology of mobile sensor networks and flocks [19].It is shown in [10]that the linear system_x i ðt Þ¼Xj 2N ia ij x j ðt ÞÀx i ðt ÞÀÁ(7)is a distributed consensus algorithm ,i.e.,guarantees con-vergence to a collective decision via local interagent interactions.Assuming that the graph is undirected (a ij ¼a ji for all i ;j ),it follows that the sum of the state of all nodes is an invariant quantity,or P i _xi ¼0.In particular,applying this condition twice at times t ¼0and t ¼1gives the following result¼1n Xix i ð0Þ:In other words,if a consensus is asymptotically reached,then necessarily the collective decision is equal to theOlfati-Saber et al.:Consensus and Cooperation in Networked Multi-Agent SystemsVol.95,No.1,January 2007|Proceedings of the IEEE219average of the initial state of all nodes.A consensus algo-rithm with this specific invariance property is called an average-consensus algorithm [9]and has broad applications in distributed computing on networks (e.g.,sensor fusion in sensor networks).The dynamics of system (7)can be expressed in a compact form as_x ¼ÀLx(8)where L is known as the graph Laplacian of G .The graph Laplacian is defined asL ¼D ÀA(9)where D ¼diag ðd 1;...;d n Þis the degree matrix of G with elements d i ¼Pj ¼i a ij and zero off-diagonal elements.By definition,L has a right eigenvector of 1associated with the zero eigenvalue 5because of the identity L 1¼0.For the case of undirected graphs,graph Laplacian satisfies the following sum-of-squares (SOS)property:x T Lx ¼12Xði ;j Þ2Ea ij ðx j Àx i Þ2:(10)By defining a quadratic disagreement function as’ðx Þ¼12x T Lx(11)it becomes apparent that algorithm (7)is the same as_x ¼Àr ’ðx Þor the gradient-descent algorithm.This algorithm globallyasymptotically converges to the agreement space provided that two conditions hold:1)L is a positive semidefinite matrix;2)the only equilibrium of (7)is 1for some .Both of these conditions hold for a connected graph and follow from the SOS property of graph Laplacian in (10).Therefore,an average-consensus is asymptotically reached for all initial states.This fact is summarized in the following lemma.Lemma 1:Let G be a connected undirected graph.Then,the algorithm in (7)asymptotically solves an average-consensus problem for all initial states.A.Algebraic Connectivity and Spectral Propertiesof GraphsSpectral properties of Laplacian matrix are instrumen-tal in analysis of convergence of the class of linear consensus algorithms in (7).According to Gershgorin theorem [81],all eigenvalues of L in the complex plane are located in a closed disk centered at Áþ0j with a radius of Á¼max i d i ,i.e.,the maximum degree of a graph.For undirected graphs,L is a symmetric matrix with real eigenvalues and,therefore,the set of eigenvalues of L can be ordered sequentially in an ascending order as0¼ 1 2 ÁÁÁ n 2Á:(12)The zero eigenvalue is known as the trivial eigenvalue of L .For a connected graph G , 290(i.e.,the zero eigenvalue is isolated).The second smallest eigenvalue of Laplacian 2is called algebraic connectivity of a graph [20].Algebraic connectivity of the network topology is a measure of performance/speed of consensus algorithms [10].Example 1:Fig.2shows two examples of networks of integrator agents with different topologies.Both graphs are undirected and have 0–1weights.Every node of the graph in Fig.2(a)is connected to its 4nearest neighbors on a ring.The other graph is a proximity graph of points that are distributed uniformly at random in a square.Every node is connected to all of its spatial neighbors within a closed ball of radius r 90.Here are the important degree information and Laplacian eigenvalues of these graphsa Þ 1¼0; 2¼0:48; n ¼6:24;Á¼4b Þ 1¼0; 2¼0:25; n ¼9:37;Á¼8:(13)In both cases, i G 2Áfor all i .B.Convergence Analysis for Directed Networks The convergence analysis of the consensus algorithm in (7)is equivalent to proving that the agreement space characterized by x ¼ 1; 2R is an asymptotically stable equilibrium of system (7).The stability properties of system (7)is completely determined by the location of the Laplacian eigenvalues of the network.The eigenvalues of the adjacency matrix are irrelevant to the stability analysis of system (7),unless the network is k -regular (all of its nodes have the same degree k ).The following lemma combines a well-known rank property of graph Laplacians with Gershgorin theorem to provide spectral characterization of Laplacian of a fixed directed network G .Before stating the lemma,we need to define the notion of strong connectivity of graphs.A graph5These properties were discussed earlier in the introduction for graphs with 0–1weights.Olfati-Saber et al.:Consensus and Cooperation in Networked Multi-Agent Systems220Proceedings of the IEEE |Vol.95,No.1,January 2007。
The UMAP Journal 14(1) Neural Networks
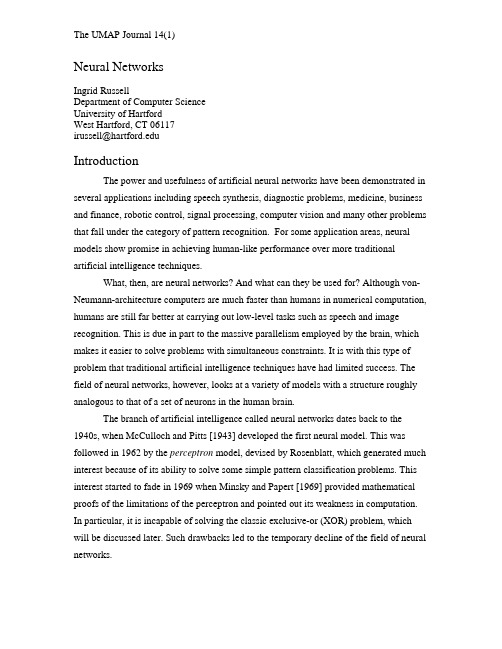
Neural NetworksIngrid RussellDepartment of Computer ScienceUniversity of HartfordWest Hartford, CT 06117irussell@IntroductionThe power and usefulness of artificial neural networks have been demonstrated in several applications including speech synthesis, diagnostic problems, medicine, business and finance, robotic control, signal processing, computer vision and many other problems that fall under the category of pattern recognition. For some application areas, neural models show promise in achieving human-like performance over more traditional artificial intelligence techniques.What, then, are neural networks? And what can they be used for? Although von-Neumann-architecture computers are much faster than humans in numerical computation, humans are still far better at carrying out low-level tasks such as speech and image recognition. This is due in part to the massive parallelism employed by the brain, which makes it easier to solve problems with simultaneous constraints. It is with this type of problem that traditional artificial intelligence techniques have had limited success. The field of neural networks, however, looks at a variety of models with a structure roughly analogous to that of a set of neurons in the human brain.The branch of artificial intelligence called neural networks dates back to the 1940s, when McCulloch and Pitts [1943] developed the first neural model. This was followed in 1962 by the perceptron model, devised by Rosenblatt, which generated much interest because of its ability to solve some simple pattern classification problems. This interest started to fade in 1969 when Minsky and Papert [1969] provided mathematical proofs of the limitations of the perceptron and pointed out its weakness in computation. In particular, it is incapable of solving the classic exclusive-or (XOR) problem, which will be discussed later. Such drawbacks led to the temporary decline of the field of neural networks.The last decade, however, has seen renewed interest in neural networks, both among researchers and in areas of application. The development of more-powerful networks, better training algorithms, and improved hardware have all contributed to the revival of the field. Neural-network paradigms in recent years include the Boltzmann machine, Hopfield’s network, Kohonen’s network, Rumelhart’s competitive learning model, Fukushima’s model, and Carpenter and Grossberg’s Adaptive Resonance Theory model [Wasserman 1989; Freeman and Skapura 1991]. The field has generated interest from researchers in such diverse areas as engineering, computer science, psychology, neuroscience, physics, and mathematics. We describe several of the more important neural models, followed by a discussion of some of the available hardware and software used to implement these models, and a sampling of applications.DefinitionInspired by the structure of the brain, a neural network consists of a set of highly interconnected entities, called nodes or units . Each unit is designed to mimic itsbiological counterpart, the neuron. Each accepts a weighted set of inputs and responds with an output. Figure 1 presents a picture of one unit in a neural network.1x 3x()A f S = 2xFigure 1. A single unit in a neural network. Let ),...,,(21n x x x X = , where the are real numbers, represent the set of inputs presented to the unit U . Each input has an associated weight that represents the strength i xof that particular connection. Let ),...,,(21n w w w W = , with real, represent the weightvector corresponding to the input vector i w X . Applied to U , these weighted inputs producea net sum at U given by ∑⋅==V W x w S i i .Learning rules, which we will discuss later, will allow the weights to be modified dynamically.The state of a unit U is represented by a numerical value A , the activation value of U . An activation function f determines the new activation value of a unit from the net sum to the unit and the current activation value. In the simplest case, f is a function of only the net sum, so ()A f S =. The output at unit U is in turn a function of A , usually taken to be just A .A neural network is composed of such units and weighted unidirectional connections between them. In some neural nets, the number of units may be in thethousands. The output of one unit typically becomes an input for another. There may also be units with external inputs and/or outputs. Figure 2 shows one example of a possible neural network structure.Figure 2. An example of a neural network structure.For a simple linear network , the activation function is a linear function, so thatf(cS)=cf(S),f(S 1+S 2)=f(S 1)+f(S 2)Another common form for an activation function is a threshold function : the activation value is 1 if the net sum S is greater than a given constant T , and is 0 otherwise.Single-Layer Linear NetworksA single –layer neural network consists of a set of units organized in a layer. Each unit U i receives a weighted input x j with weight w ji . Figure 3 shows a single-layer linear model with m inputs and n outputs.Figure 3. A single-layer linear model. Let be the input vector and let the activation function f be simply, so that the activation value is just the net sum to a unit. The (m x x x X ,,,21… =)n m × weight matrix is111212122212n n m m mn w w w w w w W w w w ⎛⎞⎜⎟⎜⎟=⎜⎟⎜⎟⎝⎠…… … Thus the output y k at unit U k is x 1x 2x my 1 y 2 y n()1212,,,k k k mk m x x y w w w x ⎛⎞⎜⎟⎜⎟=⎜⎟⎜⎟⎝⎠…So the output vector is given by(12,,,T n Y y y y = …)111T nx mx mx Y W X =Learning RulesA simple linear network, with its fixed weights, is limited in the range of output vectors it can associate with input vectors. For example, consider the set of input vectors (x 1,x 2), where each x i is either 0 or 1. No simple linear network can produce outputs as shown in Table 1, for which the output is the boolean exclusive-or (XOR) of the inputs. (You can easily show that the two weights w l and w 2 would have to satisfy threeinconsistent linear equations.) Implementing the XOR function is a classic problem in neural networks, as it is a subproblem of other more complicated problems.Table 1.Inputs and outputs for a neural net that implements the boolean exclusives (XOR) function.Hence, in addition to the network topology, an important component of mostneural networks is a learning rule . A learning rule allows the network to adjust its connection weights in order to associate given input vectors with corresponding output vectors. During training periods, the input vectors are repeatedly presented, and the weights are adjusted according to the learning rule, until the network learns the desiredassociations, i.e., until . It is this ability to learn that is one of the mostattractive features of neural networks.T Y W X = A single-layer model usually uses either the Hebb rule or the delta rule .In the Hebb rule, the change ij w δin the weights is calculated as follows. Let()(11,,,,,T m )n X x x Y y y ……be the input and output vectors that we wish to associate. Ineach training iteration, the weights are adjusted by,ij i j w ex y δ=where e is a constant called the learning rate , usually taken to be the reciprocal of the number of training vectors presented. During the training period, a number of suchiterations can be made, letting the (),X Y pairs vary over the associations to be learned. A network using the Hebb rule is guaranteed (by mathematical proof) to be able to learn associations for which the set of input vectors are orthogonal. [McClelland andRumelhart et al. 1986]. A disadvantage of the Hebb rule is that if the input vectors are not mutually orthogonal, interference may occur and the network may not be able to learn the associations.The delta rule was developed to address the deficiencies of the Hebb rule. Under the delta rule, the change in weight is()ij i j j w rx t y δ=−Wherer is the learning rate,t j is the target output, andy j is the actual output at unit U j .The delta rule changes the weight vector in a way that minimizes the error , the difference between the target output and the actual output. It can be shownmathematically that the delta rule provides a very efficient way to modify .the initial weight vector toward the optimal one (the one that corresponds to minimum error)[McClelland and Rumelhart et al. 1986]. It is possible for a network to learn moreassociations with the delta rule than with the Hebb rule. McClelland and Rumelhart et al.prove that a network using the delta rule can learn associations whenever the inputs are linearly independent [1986].Threshold NetworksMuch early work in neural networks involved the perceptron . Devised byRosenblatt, a perceptron is a single-layer network with an activation function given by1()0if S T f S otherwise >⎧=⎨⎩where T is some constant. Because it uses a threshold function, such a network is called a threshold network .But even though it uses a nonlinear activation function, the perceptron still cannot implement the XOR function. That is, a perceptron is not capable of responding with an output of 1 whenever it is presented with input vectors (0,l) or (1,0), and responding with output 0 otherwise.The impossibility proof is easy. There would have to be a weight vector for which for which the scalar product net sum(1112,W w w = )111212S W X w x w x =⋅=+leads to an output of 1 for input (0,l) or (1,0), and 0 otherwise (see Table 2).Table 2.Inputs, net sum, and desired output for a perceptmn that implementsthe boolean exclusives (XOR) function.Now, the line with equation w ll x l + w 21x 2 = T divides the x l x 2-plane into tworegions, as illustrated in Figure 4. Input vectors that produce a net sum S greater than T lie on one side of the line, while those with net sum less than T lie on the other side. For the network to represent the XOR function, the inputs (1,l) and (0, 0), with sums (w l +w 2)w11x1 + w21x2 = T(0,1) (1,1)(0,0) (1,0)Figure 4. The graph of w11x1+w21x2=T.and 0, must produce outputs on one side, while the inputs (1, 0) and (0, 1), with sums w l and w2, must produce outputs on the other side. But if w l> T and w2> T, then w l+ w2> T; and similarly for <. So a perceptron cannot represent the XOR function.In fact, there are many other functions that cannot be represented by a single-layer network with fixed weights. While such limitations were the cause of a temporary decline of interest in the perceptron and in neural networks in general, the perceptron laid foundations for much of the later work in neural networks. The limitations of single-layer networks can, in fact, be overcome by adding more layers; as we will see in the following section, there is a multilayer threshold system that can represent the XOR function.Multilayer NetworksA multilayer network has two or more layers of units, with the output from one layer serving as input to the next. The layers with no external output connections are referred to as hidden layers (Figure 5).However, any multilayer system with fixed weights that has a linear activation function is equivalent to a single-layer linear system. Take, for example, the case of atwo-layer linear system. The input vector to the first layer is X , the output ofthe first layer is given as input to the second layer, and the second layer produces output 1Y W X = 2Z W Y = .hidden layerFigure 5. A multilayer network.Hence()()2121Z W W X W W X == Consequently, the system is equivalent to a single-layer network with weight matrix W = W 2W 1. By induction, a linear system with any number n of layers is equivalent to a single-layer linear system whose weight matrix is the product of the n intermediate weight matrices.On the other hand, a multilayer system that is not linear can provide morecomputational capability than a single-layer system. For instance, the problems encountered by the perceptron can be overcome with the addition of hidden layers; Figure 6 demonstrates how a multilayer system can represent the XOR function. The threshold is set to zero, and consequently a unit responds if its activation is greater than zero.The weight matrices for the two layers are12111,.111W W −⎛⎞⎛==⎜⎟⎜−⎝⎠⎝⎞⎟⎠, We thus get 12111,1000T T W W ⎛⎞⎛⎞⎛⎞==⎜⎟⎜⎟⎜⎟⎝⎠⎝⎠⎝⎠12000,1111T T W W ⎛⎞⎛⎞⎛⎞,==⎜⎟⎜⎟⎜⎟⎝⎠⎝⎠⎝⎠12100,0100T T W W ⎛⎞⎛⎞⎛⎞,==⎜⎟⎜⎟⎜⎟⎝⎠⎝⎠⎝⎠12000,0000T T W W ⎛⎞⎛⎞⎛⎞.==⎜⎟⎜⎟⎜⎟⎝⎠⎝⎠⎝⎠With input vector (1,0) or (0,l), the output produced at the outer layer is 1; otherwise it is 0.Multilayer networks have proven to be very powerful. In fact, any booleanfunction can be implemented by such a network [McClelland and Rumelhart 1988].Figure 6. A multilayer system representation of the XOR function.Multilayer networks have proven to be very powerful. In fact, any boolean function can be implemented by such a network [McClelland and Rumelhart 1988].Multilayer Networks with LearningNo learning algorithm had been available for multilayer networks until Rumelhart, Hinton, and Williams introduced the backpropagation training algorithm, also referred to as the generalized delta rule [1988]. At the output layer, the output vector is compared to the expected output. If the difference is zero, no changes are made to the weights of the connections. If the difference is not zero, the error is calculated from the delta rule and is propagated back through the network. The idea, similar to that of the delta rule, is to adjust the weights to minimize the difference between the real output and the expected output. Such networks can learn arbitrary associations by using differentiable activation functions. A theoretical foundation of backpropagation can be found in McClelland and Rumelhart et al. [1986] and in Rumelhart et al. [1988].One drawback of backpropagation is its slow rate of learning, making it less than ideal for real-time use. In spite of some drawbacks, backpropagation has been a widely used algorithm, particularly in pattern recognition problems.All the models discussed so far use supervised learning, i.e., the network is provided the expected output and trained to respond correctly. Other neural network models employ unsupervised learning schemes. Unsupervised learning implies the absence of a trainer and no knowledge beforehand of what the output should be for any given input. The network acts as a regularity detector and tries to discover structure in the patterns presented to it. Such networks include competitive learning, for which there are four major models [Wasserman 1989; Freeman and Skapura 1991; McClelland and Rumelhart et al. 1986].Software and Hardware ImplementationIt is relatively easy to write a program to simulate one of the networks described in the preceding sections (see, e.g., Dewdney [1992]); and a number of commercial software packages are available, including some for microcomputers. Many programs feature a neural-network development system that supports several different neural types, to allow the user to build, train, and test networks for different applications. Reid and Zeichick provide a description of 50 commercial neural-network products, as well as pricing information and the addresses of suppliers [1992].The training of a neural network through software simulation demands intensive mathematical computation, often leading to excessive training times on ordinary general-purpose processors. A neural network accelerator board, such as the NeuroBoard developed to support the NeuroShell package, can provide high-speed performance. NeuroBoard’s speed is up to 100 times that of a 20 MHz 30386 chip with a math co-processor.Another alternative is a chip that implements neural networks in hardware;both analog and digital implementations are available. Carver Mead at UCLA, a leading researcher in analog neural-net chips, has developed an artificial retina [1989]. Two companies lead in commercialized neural network chip development: Intel, with its 80170 ETANN (Electronically Trainable Artificial Neural Network) chip, and NeuralSemiconductor, with its DNNA (Digital Neural Network Architecture) chip. These chips, however, do not have the capabilities of on-chip learning. In both cases, the chip is interfaced with a software simulation package, based on backpropagation, which is used for training and adjustment of weights; the adjusted weights are then transferred to the chip [Caudill 1991]. The first chips with on-chip training capability should be available soon.ApplicationsNeural networks have been applied to a wide variety of different areas including speech synthesis, pattern recognition, diagnostic problems, medical illnesses, robotic control and computer vision.Neural networks have been shown to be particularly useful in solving problems where traditional artificial intelligence techniques involving symbolic methods have failed or proved inefficient. Such networks have shown promise in problems involving low-level tasks that are computationally intensive, including vision, speech recognition, and many other problems that fall under the category of pattern recognition. Neural networks, with their massive parallelism, can provide the computing power needed for these problems. A major shortcoming of neural networks lies in the long training times that they require, particularly when many layers are used. Hardware advances should diminish these limitations, and neural-network-based systems will become greater complements to conventional computing systems.Researchers at Ford Motor Company are developing a neural-network system that diagnoses engine malfunctions. While an experienced technician can analyze engine malfunction given a set of data, it is extremely complicated to design a rule-based expert system to do the same diagnosis. Marko et al. [I990] trained a neural net to diagnose engine malfunction, given a number of different faulty states of an engine such as open plug, broken manifold, etc. The trained network had a high rate of correct diagnoses. Neural nets have also been used in the banking industry, for example, in the evaluation of credit card applications.Most neural network applications, however, have been concentrated in the area of pattern recognition, where traditional algorithmic approaches have been ineffective. Suchnets have been used for classifying a given input into one of a number of categories and have demonstrated success, even with noisy input, when compared to other more conventional techniques.Since the 1970s, work has been done on monitoring the Space ShuttleMain Engine (SSME), involving the development of an Integrated Diagnostic System (IDS). The IDS is a hierarchical multilevel system, which integrates various fault detection algorithms to provide a monitoring system that works for all stages of operation of the SSME. Three fault-detection algorithms have been used, depending on the SSME sensor data. These employ statistical methods that have a high computational complexity and a low degree of reliability, particularly in the presence of noise. Systems based on neural networks offer promise for a fast and reliable real-time system to help overcome these difficulties, as is seen in the work of Dietz et al. [1989]. This work involves the development of a fault diagnostic system for the SSME that is based on three-layer backpropagation networks. Neural networks in this application allow for better performance and for the diagnosis to be accomplished in real time. Furthermore, because of the parallel structure of neural networks, better performance is realized by parallel algorithms running on parallel architectures.At Boeing Aircraft Company, researchers have been developing a neural network to identify aircraft parts that have already been designed and manufactured,in efforts to help them with the production of new parts. Given a new design, the system attempts to identify a previously designed part that resembles the new one. If one is found, it may be able to be modified to conform to the new specifications, thus saving time and money in the manufacturing process.Neural networks have also been used in biomedical research, which often involves the analysis and classification of an experiment's outcomes. Traditional techniques include the linear discriminant function and the analysis of covariance. The outcome of the experiment is in some cases dependent on a number of variables, with the dependence usually a nonlinear function that is not known. Such problems can, in many cases, be managed by neural networks.Stubbs [I990] presents three biomedical applications in which neural networks have been used, one of which involves drug design. Non-steroidal anti-inflammatorydrugs (NOSAIDs) are a commonly prescribed class of drugs, which in some cases may cause adverse reactions. The rate of adverse reactions (ADR) is about l0%, with 1% of these involving serious cases and 0.1% being fatal [Stubbs 1990]. A three-layer backpropagation neural network was developed to predict the frequency of serious ADR cases for 17 particular NOSAIDs, using four inputs, each representing a particular property of the drugs. The predicted rates given by the model matched within 5% the observed rates, a much better performance than by other techniques. Such a neural network might be used to predict the ADR rate for new drugs, as well as to determine the properties that tend to make for "safe" drugs.ConclusionIn the early days of neural networks, some overly optimistic hopes for success were not always realized, causing a temporary setback to research. Today, though, a solid basis of theory and applications is being formed; and the field has begun to flourish. For some tasks, neural networks will never replace conventional methods; but for a growing list of applications, the neural architecture will provide either an alternative or a complement to these other techniques.ReferencesCarpenter, G., and S. Grossberg. 1988. The ART of Adaptive Pattern Recognition by a Self-organizing Neural Network. IEEE Computer 21: 77-88.Caudill, M. 1990. Using neural nets: Diagnostic expert nets. A1Expert 5(9) (September 1990): 43-47.-------. 1991. Embedded neural networks. AI Expert 6 (4) (April 1991): 40-45. Denning, Peter J. 1992. The science of computing: Neural networks. American Scientist 80: 426-429.Dewdney, A.K. 1992. Computer recreations: Programming a neural net. Algorithm: Recreational Computing 3 (4) (October-December 1992): 11-15.Dietz, W., E. Kiech, and M. Ali. 1989. Jet and rocket engine fault diagnosis in real time. Journal of Neural Network Computing (Summer 1989): 5-18.Freeman, J., and D. Skapura. 1991. Neural Networks. Reading MA: Addison-Wesley.Fukushima, K. 1988. A neural network for visual pattern recognition. IEEE Computer 21 (3) (March 1988): 65-75.Kohonen, T. 1988. Self-Organization and Associative Memory. New York: Springer-Verlag.Marko, K., J. Dosdall, and J. Murphy. 1990. Automotive control system diagnosis using neural nets for rapid pattern classification of large data sets. In Proceedings of the International Joint Conference on Neural Networks I-33-I-38. Piscataway, NJ: IEEE Service Center.McClelland, J., D. Rumelhart, and the PDP Research Group. 1986. ParallelDistributed Processing: Explorations in the Microstructure of Cognition. Vol. 1: Foundations. Cambridge, MA: MIT Press.McClelland, J., and D. Rumelhart. 1988. Explorations in Parallel Distributed Processing. Cambridge, MA: MIT Press.McCulloch, W., and W. Pitts. 1943. A logical calculus of the ideas imminent in nervous activity. Bulletin of Mathematical Biophysics 5: 115-33.Mead, C. 1989. Analog VLSI and Neural Systems. Reading MA: Addison-Wesley. Minsky, M., and S. Papert. 1969. Perceptrons. Cambridge, MA: MIT Press.Reid, K., and A. Zeichick. 1992. Neural network products resource guide. AI Expert 7 (6) (June 1992): 50-56.Rumelhart, D., G. Hinton, and R. Williams. 1988. Learning internal representations by error propagation. In Neurocornputing, edited by J. Anderson and E. Rosenfeld, 675-695. Cambridge, MA: MIT Press.Russell, I. 1991. Self-organization and adaptive resonance theory networks. In Proceedings of the Fourth Annual Neural Networks and Parallel Processing Systems Conference, edited by Samir I. Sayegh, 227-234. Indianapolis, IN: Indiana University-Purdue University.Shea, P., and V. Lin. 1989. Detection of explosives in checked airline baggage using an artificial neural system. International Journal of Neural Networks 1 (4) (October 1989): 249-253.Stubbs, D. 1990. Three applications of neurocomputing in biomedical research. Neurocomputing 2: 61-66.Wasserman, P. 1989. Neural Network Computing. New York: Van Nostrand Reinhold.。
Neural Network Architectures
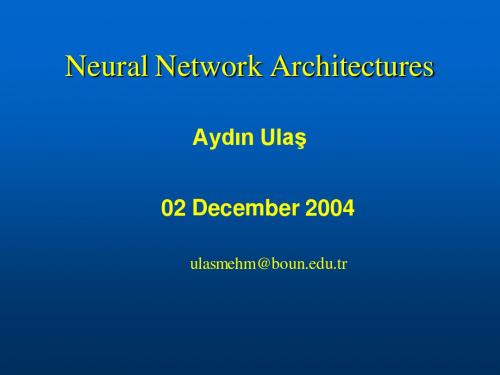
The use of NN needs a good comprehension of the problem
Implementation of Neural Networks
Generic architectures (PC’s etc) Specific Neuro-Hardware Dedicated circuits
Introduction
Some numbers…
– The human brain contains about 10 billion nerve cells (neurons) – Each neuron is connected to the others through 10000 synapses
Neural Network Architectures
Aydın Ulaş 02 December 2004
ulasmehm@.tr
Outline Of Presentation
Introduction Neural Networks Neural Network Architectures Conclusions
An Example Regression
4 2
0
-2
-4
-6
-8
-10 -2
-1.5
-1
-0.5
0
0.5
1
1.5
2
Example Classification
Handwritten digit recognition 16x16 bitmap representation
– Converted to 1x256 bit vector
1 1+ex −x) p(
fundamentals_of_brain_network_analysis_概述及解释说明
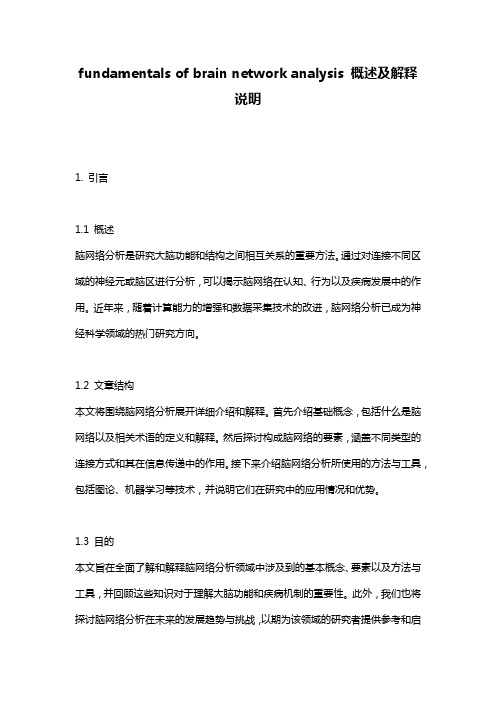
fundamentals of brain network analysis 概述及解释说明1. 引言1.1 概述脑网络分析是研究大脑功能和结构之间相互关系的重要方法。
通过对连接不同区域的神经元或脑区进行分析,可以揭示脑网络在认知、行为以及疾病发展中的作用。
近年来,随着计算能力的增强和数据采集技术的改进,脑网络分析已成为神经科学领域的热门研究方向。
1.2 文章结构本文将围绕脑网络分析展开详细介绍和解释。
首先介绍基础概念,包括什么是脑网络以及相关术语的定义和解释。
然后探讨构成脑网络的要素,涵盖不同类型的连接方式和其在信息传递中的作用。
接下来介绍脑网络分析所使用的方法与工具,包括图论、机器学习等技术,并说明它们在研究中的应用情况和优势。
1.3 目的本文旨在全面了解和解释脑网络分析领域中涉及到的基本概念、要素以及方法与工具,并回顾这些知识对于理解大脑功能和疾病机制的重要性。
此外,我们也将探讨脑网络分析在未来的发展趋势与挑战,以期为该领域的研究者提供参考和启示。
请确保文章内容的流畅性,并尽量使用清晰易懂的语言解释相关概念。
2. 正文:2.1 脑网络分析的基础概念脑网络分析是一种研究大脑连接模式和功能的方法。
它基于图论原理,将大脑视为一个复杂网络,通过分析节点(代表大脑区域)之间的连接以及连接强度来揭示大脑活动的本质。
脑网络分析被广泛应用于神经科学领域,对理解大脑的结构和功能具有重要意义。
2.2 脑网络的构成要素大脑网络主要由两个构成要素组成:节点和边。
在这里,节点代表特定的大脑区域或神经元群落,而边则表示这些节点之间存在的连接关系。
网络中的每个节点都具有一定的功能或特性,并且节点之间的连接可以根据不同的标准进行定义,如结构连接、功能连接等。
2.3 脑网络分析方法与工具在进行脑网络分析时,常用的方法包括静态方法和动态方法。
静态方法侧重于揭示大脑静息状态下的结构和功能连接模式,如静态脑网络分析、全局效能指数等;而动态方法则关注于研究大脑在时间和空间上的变化,如时序网络分析、突触可塑性等。
- 1、下载文档前请自行甄别文档内容的完整性,平台不提供额外的编辑、内容补充、找答案等附加服务。
- 2、"仅部分预览"的文档,不可在线预览部分如存在完整性等问题,可反馈申请退款(可完整预览的文档不适用该条件!)。
- 3、如文档侵犯您的权益,请联系客服反馈,我们会尽快为您处理(人工客服工作时间:9:00-18:30)。
Application of Neural Networks and Statistical Pattern Recognition Algorithmsto Earthquake Risk EvaluationG.Giacinto (*), R.Paolucci (+), and F.Roli (*)*Dept. of Electrical and Electronic Eng., University of Cagliari, ITALYPiazza D'Armi, 09123, Cagliari, ITALY e-mail {giacinto, roli}@diee.unica.it+Dept. of Structural Eng., Technical University of Milan, ITALY Piazza Leonardo da Vinci 32, 20133, Milan, ITALY e-mail paolucci@esdra.stru.polimi.itAbstractThis paper reports the experimental results on the application of different pattern recognition algorithms to the evaluation of earthquake risk for real geological structures. The study area used for the experiments is related to a well-known geological structure representing a “triangular valley over bedrock”. Performances obtained by two neural networks and two statistical classifiers are reported and compared. The advantages provided by the use of methods for combining multiple classifiers are also discussed and the related results reported.Keywords: Earthquake risk evaluation; Statistical and neural classifiers; Combination of multiple classifiers.1. IntroductionThe ability to realistically predict “ground shaking” at a given location during an earthquake is crucial for seismic risk prevention strategies in urban systems, as well as for the safe design of major structures. However, the largest seismic events of the last decade have demonstrated that the observed ground shaking can be much more severe than expected and its spatial distribution poorly related to the "earthquake risk maps" previously prepared by seismologists or earthquake engineers (Faccioli, 1996). Therefore, a major improvement of the present ability to compile earthquake risk maps is required to mitigate the impact of earthquakes on urban areas, to plan land use and to prepare effective emergency plans.In the fields of seismology and structural engineering, risk maps are obtained by “combining”data related to factors that mainly affect earthquake risk. The main “data sources” currently used are:• data on regional seismicity, typically based on historical or seismotectonic observations;• data on the “geological setting” of the study area;• data on the “vulnerability” of the human and natural environment;• data on the effects of the so-called “local soil conditions” (e.g., topographic and geological irregularities of the soil profile) on the spatial variation of ground motion during an earthquake (Sanchez-Sesma, 1987).The latter data source allows earthquake engineers to predict risk degrees at locations characterized by different soil conditions.In this paper, we focus on the development of pattern recognition techniques for the automatic evaluation of the effects of local soil conditions. It has been pointed out that such “site effects” were one of the main causes of concentrated damage during some of the largest earthquakes of the last decades (e.g., the earthquake that struck Mexico City in September 1985). The classical algorithms for the evaluation of the seismic site effects are briefly reviewed in Section 2, where the advantages and the potentialities of the use of pattern recognition techniques are also discussed. The formulation of earthquake risk evaluation as a pattern recognition problem is described in Section 3. Section 4 gives a brief description of neural networks and statistical pattern recognition algorithms used in the experiments. Methods used for “combining” the results provided by these algorithms are also briefly described. Section 5 describes the “study case” used for experiments. Performances obtained by different pattern recognition algorithms and by their “combination” are also reported and compared. Conclusions are drawn in Section 6.2. Earthquake risk evaluationFirst of all, it should be pointed out that the evaluation of site effects is not the only information commonly used by earthquake engineers to compile risk maps. As pointed out in the Introduction, local soil conditions strongly affect earthquake risk but additionalinformation should be used to completely evaluate earthquake risk for a study area. However, in the following, we will refer to site effects evaluation as the “earthquake risk evaluation problem”.The problem considered can be defined as follows. Given the local site conditions (e.g., topographic profile, geological layering and soil mechanical properties) and given the “input earthquake” (e.g., a plane wave of given amplitude and shape propagating towards the earth’s surface), find the ground motion at different locations (“sites”) of the study area.The approach that has been generally used so far by earthquake engineers to solve the above problem is mainly based on different techniques for the numerical integration of the elasto-dynamics equations of motion, with the proper boundary and initial conditions (Aki and Richards, 1980). These numerical tools for the simulation of seismic wave propagation provide “solutions” that engineers usually summarize in a few parameters, such as the peak ground acceleration, the duration of motion, or other measures deemed adequate to represent the severity of ground shaking at different sites. Subsequently, according to the values of the above parameters, a risk map is compiled by assigning a certain degree of risk (e.g., low, medium or high risk) to each site.There are three main limitations in using classical numerical tools for earthquake risk evaluation:• the poor knowledge of the geological setting of the study area that prevents, in many cases, the creation of an accurate numerical model of the study area;• the uncertainties in the values of local soil conditions;• the huge computational burden required by numerical procedures to perform fully three-dimensional (3D) dynamic wave propagation analyses on realistic geologic configurations. In terms of pattern recognition, it is worth noting that the above-mentioned numerical tools follow the classical “model-based” approach to engineering problem solving that demands a detailed and precise model of the physical phenomenon to be investigated (Haykin, 1996). The model of the study area allows earthquake engineers to develop a numerical “transfer function” that uses the seismic wave as input and provides the severities of ground shakings at the different locations as outputs. (From a pattern recognition point of view, the definition ofthe above transfer function can be regarded as a problem of estimating an “input-output function”).On the basis of the above considerations, the pattern recognition approach seems to exhibit several features that could help to overcome the above limitations of classical numerical tools:• pattern recognition provides a “non-parametric” approach to the solution of problems that involve the estimation of input-output functions. Pattern recognition algorithms like the k-nearest neighbor classifier or the multi-layer perceptron neural network can be used to estimate an input-output function without needing a model of the physical mechanism underlying the function;• pattern recognition provides algorithms that are able to “learn” the desired input-output function by “examples”;• pattern recognition algorithms based on neural network models have proved they can effectively handle uncertainties in input data;• pattern recognition algorithms exhibit reasonable computational complexities with respect to those of numerical procedures currently used for wave propagation simulation. Therefore, the pattern recognition approach could be successfully used to overcome the lack of “models” for real study areas, to handle uncertainties in local site conditions, and to provide earthquake engineers with fast computational tools.3. Formulation of the earthquake risk evaluation as a pattern recognition problemAs pointed out in the previous Section, the earthquake risk evaluation problem basically involves the “assignment” of “risk degrees” to different locations of a given study area. Therefore, it can be naturally formulated as a pattern recognition problem. The formulation requires the pattern recognition concepts of “patterns”, “features”, and “data classes” to be expressed in terms of the “elements” and “data” involved in earthquake risk evaluation. To this regard, let us use an example of a specific risk evaluation problem. Figure 1 illustrates a study area characterized by a geological structure representing a “triangular valley over bedrock”. In the earthquake engineering field, this is an interesting study case, as it constitutesa reasonable approximation of many real geological structures, such as sediment-filled alluvial valleys. The main elements and related data involved in the risk evaluation for a triangular valley are the following:•the “shape” of the valley that can be characterized by geometrical features;•the “sediment basin” (i.e., the soil underlying the valley) and the “bedrock” that can be characterized by their mechanical properties;•the seismic wave that can be described by features commonly used for signal characterization (e.g., peak amplitude and fundamental frequency of the wave);•the so-called “receivers” that are related to the locations of the study area for which risk degrees are to be evaluated.From the above definitions, it is easy to see that “receivers” can be regarded as “patterns” for any earthquake risk evaluation problem. In order to characterize such patterns, “features”related to the position of the receivers, the “shape” of the geological structure, the mechanical properties of the soil underlying the receivers, and measures characterizing the “input”seismic wave can be used. With regard to the definition of “data classes”, data classes can be easily associated to the considered risk degrees (e.g., three data classes related to “low”,“medium” and “high” risk). If we assume to use “supervised” pattern recognition algorithms,“training sets” must also be created. Unfortunately, as pointed out in Section 2, poor and rough data are usually available for real geological structures. Typically, a few “accelerograph stations” record seismic motions for the locations of a large area and earthquake engineers are unable to “infer” ground shakings for the remaining locations. Consequently, the most practical way to build up training sets is to use numerical procedures for the simulation of wave propagation. For complex geological structures, “approximate”simulations could be carried out (e.g., “local” 2D simulations could be used for the simulation of complex 3D structures). Typically, due to the computational load and to the above-discussed limitations of present numerical codes, small and “noisy” training sets should be expected.4. Pattern recognition algorithms and combination methodsDifferent neural networks and statistical classification algorithms were applied to the evaluation of earthquake risk. Among neural network classifiers, the multilayer perceptron (MLP) and the probabilistic neural network (PNN) were used. (A brief description of such neural networks is given in Sections 4.1 and 4.2). The well-known k-nearest neighbor (k-NN) and Gaussian classifiers were adopted to evaluate performances of classical statistical algorithms. For a description of such statistical classifiers, the reader should refer to Fukunaga (1990). To utilize the complementary characteristics of the above classification algorithms, methods for combining the results provided by multiple classifiers were also applied (Section 4.3)4.1 Multilayer perceptron neural networkMultilayer perceptrons are artificial neural network models whose architecture consists of multiple layers of neurons with connections only between neurons in neighboring layers. A numerical value called “weight” is attached to every connection in the network. Information is processed starting from one side of the network called the “input layer” and moving through successive “hidden layers” to the “output layer”. As an example, Figure 2a shows the topology of an MLP neural network with only one hidden layer. Each neuron computes a so-called “net input” from the outputs of previous neurons and from the weights of the connections. Typically, such a net input is a weighted sum, and a numerical value, called “bias”, is added to the net input (Figure 2b). In MLPs, a function called “activation function”, is applied to the net input. In our experiments, we used a sigmoid function. One of the most commonly used training schemes for MLPs is the error back-propagation (EBP) learning algorithm. This is a learning algorithm by “examples” based on a “gradient descent”technique. Typically, there is an output neuron for each data class and an input pattern is classified as belonging to a given class if the related output neuron has the highest activation among all the output neurons. Therefore, for each input pattern, the EBP algorithm adjusts the values of the network connections in order to maximize the activation value of the neuronrelated to the correct class and to minimize the activation values of all the other output neurons.The reader interested in a detailed description of MLPs can refer to Hertz et al. (1991).4.2 Probabilistic neural networkProbabilistic Neural Networks (PNNs) are a model for supervised classification based on multivariate probability estimation (Specht, 1990). They are based on an extension of the Parzen approach to univariate probability estimation (Fukunaga, 1990). Given a set of N samples X i drawn from a statistical distribution p( X ), the Parzen approach provides an asymptotic, unbiased and consistent estimate ˆ()pX of the related probability density function by using an appropriate “kernel function” k ⋅() which is applied to each sample considered,i.e.:ˆp X N k X X i i N ()=−()=∑11 .(1)PNNs are based on an extension of such an approach to the multivariate case (Cacoullos,1966), utilizing the Gaussian kernel function. The typical architecture of a PNN is shown in Figure 3. The network consists of an input layer, one hidden layer and an output layer. The hidden layer has as many neurons as the number of training patterns; as a kernel function,each neuron has a Gaussian type of activation function, and is centered on the feature vector of the corresponding training pattern. The output layer has as many neurons as the number of data classes considered; the activation function of each output neuron computes the sum of the inputs to the neuron. The neurons of the hidden layer propagate their outputs only to the neuron of the output layer corresponding to the class the training pattern belongs to. Given the feature vector of an unknown pattern as input to the net, the neurons of the output layer provide the estimates of the probability that the unknown pattern belongs to each of the data classes. The classification is carried out by using the “Winner Takes All” decision rule to identify the most probable class. Training PNNs consists in the optimization of the Gaussiankernel by trials with different values of the “smoothing parameter” (Specht, 1990) which tunes the width of the Gaussian function.4.3 Methods for combining multiple classifiersSome methods to combine results provided by multiple classifiers have been proposed in the literature (Suen, 1992). Let us assume a pattern recognition problem with M "data classes".Each class represents a set of specific patterns. Each pattern is characterized by a feature vector X . In addition, let us assume that K different classification algorithms are available to solve the classification problem at hand. Therefore, we can consider “ensembles” formed by "k" different classifiers (k=1..K). In order to exploit the complementary characteristics of available classifiers, the combination methods described in the following can be used.4.3.1 Combination by Voting PrincipleLet us assume that each classifier contained in the given ensemble performs a "hard"classification assigning each input pattern to one of the M data classes. A simple method to combine results provided by different classifiers is to interpret each classification result as a "vote" for one of the M data classes. Consequently, the data class that receives a number of votes higher than a prefixed threshold is taken as the "final" classification. Typically, the threshold is half the number of the considered classifiers ("majority rule"). More conservative rules can be adopted (e.g., the "unison" rule).4.3.2 Combination by Belief FunctionsIt is well known that some classification algorithms can provide an estimate of the posterior probability that an input pattern X belongs to the data class ωi :ˆ(/),,,p X X i M i ∈=ω 1K (2)For example, estimates of the post-probabilities are provided by multilayer perceptrons (Serpico and Roli, 1995). Post-probabilities can be computed in a straightforward manner for the k-NN classifier. This combination method utilizes the prior knowledge available on each classifier. In particular, this method utilizes knowledge about the "errors" made by each classifier on the training set patterns. Such prior knowledge is contained in the so-called"confusion matrices". For the z th classifier C z , it is quite simple to see that the confusion matrix can provide estimates of the following probabilities: ˆ(/()),,,,,,p X C X j i M j M z K i z ∈====ω 111K K K (3)On the basis of the above probabilities, the combination can be carried out by the following "belief" functions: bel i pX C X j i M i k k k K ()ˆ(/()),,=∈===∏ηω 11K (4)The final classification is taken by assigning the input pattern X to the data class for which bel(i) is maximum.5. Experimental results5.1 The study caseThe considered study case was a triangular valley over bedrock (Figure 1). In order to apply our pattern recognition algorithms, twenty-one “receivers” were used and a numerical procedure designed for fast analyses of 2D wave propagation within triangular valleys was applied to “predict” ground shakings for different receiver locations (Paolucci et al., 1992).For simulation purposes, we assumed an input earthquake wave represented by a “plane shear” wave propagating towards the earth surface. A Ricker type of time-dependence was implemented for this wave, since “Ricker waves” are widely used in seismic wave propagation analyses (Ricker, 1953). From an earthquake engineering viewpoint, the parameters used to characterize the valley were the following:• the “length” of the valley (L) and the two dipping angles π/2N 1 and π/2N 2;•the mechanical properties of the soil inside the valley (ρv = material density, βv = shear wave propagation velocity, Q v = quality factor, describing the internal dissipation of thematerial due to its non-elastic behavior) and of the “bedrock”, that is, the rigid basementunderlying the valley (ρr , βr). We assumed that no dissipation occurred inside the bedrock,and that ρr βr>>ρvβv ;• the positions of the locations (“receivers”) where ground motion is measured;• the fundamental frequency (f p) of the input seismic wave.The main objective of the simulations carried out was to create a “data set” containing examples of the degrees of ground shaking for receivers (i.e., “patterns”) characterized by different soil conditions and different “wavelengths” of the input earthquake wave. We performed many runs of our simulator using different values of the two dipping angles and different values of the wavelength. The other measures related to the mechanical properties of the valley and the bedrock were kept constant throughout all simulations. For each run, our simulator provided the ground motion as output in terms of acceleration time histories for the twenty-one receivers at the surface of the valley. The whole simulation phase produced a data set consisting of 6300 patterns. Each pattern was related to a receiver and, in terms of pattern recognition, it was characterized by the following four features: the receiver position x/L, the two parameters defining the angles N1 and N2, and the “normalized wavelength” λ/L of theinput wave (where λ = βv /fpis the fundamental wavelength calculated inside the valley). Inorder to apply our supervised pattern recognition algorithms, each pattern was assigned to one of three “risk classes” (low, medium, and high risk) on the basis of the ground shaking values predicted by our simulator. In particular, the severity of ground shaking was computed by the peak acceleration and by the “intensity of motion” (the latter calculated as an integral measure of ground motion over its whole duration).The obtained data set was randomly subdivided into a training and a test set of different sizes.5.2 ResultsFor each kind of classification algorithm, a long “design phase” involving “trials” with different classifier architectures and learning parameters was carried out. The main objectiveof these experiments was to assess the best performances provided by “single” classifiers after long design phases and to compare such performances with the ones obtained by combining the results provided by multiple classifiers.In addition, experiments with training sets of different sizes (i.e., 10%, 20%, 30%, 40%, and 50% of the data set) were carried out in order to evaluate the effect of training set size on the performances of the different classifiers.For the k-nearest neighbor classifier, we carried out different trials with twenty-five values of the "k" parameter ranging from 1 up to 49. For the multilayer perceptron neural network, five different architectures with one or two hidden layers and various numbers of hidden neurons (4-4-3, 4-6-3, 4-8-3, 4-6-4-3, 4-8-4-3) were considered. For all architectures, one input neuron for each feature and one output neuron for each data class was used. We trained the networks using two different values of the learning rate (i.e., 0.01, and 0.04). For each architecture and for each value of the learning rate, ten trials with different random initial-weights ("multi-start" learning strategy) were carried out. Therefore, a set of 100 MLPs was obtained. The Gaussian classifier and the Probabilistic Neural Networks needed no design phases.At the end of the above-mentioned long design phase, a set of 127 classifiers was trained and tested on the selected data set.The performances obtained by the above classifiers on the test set are summarized in Table 1. Table 1 refers to classifiers trained by the “10% training set” (i.e., the training set containing 10% of the patterns forming the data set). For the k-NN and the MLP classifiers, the lower, the mean, and the higher classification accuracies obtained in the aforementioned design phase are shown. The “design complexity” column gives the number of “trials” carried out for each classifier (using different architectures and learning parameters). It is worth noticing that the MLP provided the best classification accuracy and it outperformed the k-NN classifier (92.95% vs. 85.84%). This result seems to indicate that the k-NN classifier suffered from the small training set size (10% of the data set). This conclusion is confirmed by results obtained using larger training sets (Figure 4). For each kind of classifier, Figure 4 shows the trend of the classification accuracy as a function of the training set size. The difference in accuracy between the k-NN and the MLP classifiers is reduced as the size is increased.In order to prove that the combination of different classifiers generates satisfactory classification accuracies with "reduced" design phases, we combined the results provided by different ensembles formed by just three classifiers obtained without any design phase (i.e., an a priori fixed “k” value was used for the k-NN and a single random weight trial was performed for each MLP architecture considered). Table 2 shows the results provided by three different classifier ensembles. For each ensemble, the classifiers results were combined by the majority rule and by the belief function method. It is worth noting that the design phase necessary to produce these classifier ensembles involves the training and the testing of just three classifiers (i.e., design complexity=3). This fast design phase can be used to obtain satisfactory performances that are close to the ones provided by the best single classifier obtained after a design phase involving 127 classifiers (MLP with 92.95% accuracy; see Table 1).Other similar experiments, that are not reported for the sake of brevity, confirmed the conclusion that the combination of different classification algorithms can be used to obtain satisfactory classification accuracies with reduced design phases.6. ConclusionsThe potentials of the use of pattern recognition techniques to evaluate earthquake risk for real geological structures have been evaluated in this paper. The reported results point out that pattern recognition techniques allow earthquake engineers to classify the risk degrees of different sites with satisfactory accuracy. In particular, they can be used to overcome the limitations of numerical procedures currently used for risk evaluation. On the other hand, such procedures can be effectively used for the risk evaluation of small parts of a large study area in order to create training sets required by supervised algorithms. Finally, from the pattern recognition viewpoint, the reported results point out that the combination of different classification algorithms can be used to obtain satisfactory classification accuracies with very short design phases.AcknowledgmentsThis research was partially supported by the EC Environment Research Programme (TRISEE Project, 3D Site Effects and Soil Foundation Interaction in Earthquake and Vibration Risk Evaluation, Contract:ENV4-CT96, Climatology and Natural Hazards). The authors wish to thank Prof. E. Faccioli for his helpful comments and suggestions.ReferencesAki K. and P. Richards (1980). Quantitative Sesimology, Theory and Methods, W.H. Freeman and Co., San Francisco.Cacoullos, T. (1966). Estimation of multivariate density. Ann. Inst. Stat. Math. 18, pp. 179-189.Faccioli E. (1996). On the use of engineering seismology tools in ground shaking scenarios. State of the art paper, 11th World Conf. on Earthquake Engineering, Paper n. 2007, Acapulco, Mexico.Fukunaga, K. (1990). Introduction to Statistical Pattern Recognition. Academic Press, Inc., New York, 2nd edition.S.Haykin (1996). Neural Networks expand SP's Horizons. IEEE Signal Processing Magazine, March 1996, pp. 24-49Hertz, J., A. Krogh and R.G. Palmer (1991). Introduction to the Theory of Neural Computation. Addison Wesley Pub. Co., The Advance Book Program.Paolucci R., Suarez M. and F.J. Sanchez-Sesma (1992). Fast computation of SH seismic response for a class of alluvial valleys. Bulletin of the Seismological Society of America, Vol. 82, pp. 2075-2086.Ricker N. (1953). The form and the laws of propagation of seismic wavelets. Geophysics, Vol. 18, pp. 10-40.Sanchez-Sesma F.J. (1987). Site effects on strong ground motion. Soil Dynamics and Earthquake Engineering, Vol. 6, pp. 124-132.Serpico, S.B. and F. Roli (1995). Classification of multisensor remote-sensing images by structured neural networks. IEEE Transactions on Geoscience and Remote Sensing 33, 562-578.Specht, D.F. (1990). Probabilistic neural networks. Neural Networks 3, 109-118.Suen et al. (1992). Methods for combining multiple classifiers and their applications to handwriting recognition, IEEE Trans. on Systems, Man, and Cyb., Vol. 22, No. 3, May/June 1992, pp. 418-435.。