Milagrito Detection of TeV Emission from Mrk 501
人工智能英文参考文献(最新120个)
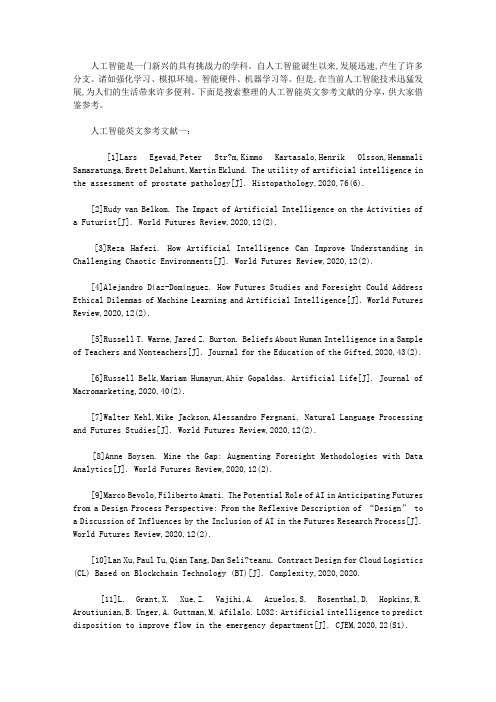
人工智能是一门新兴的具有挑战力的学科。
自人工智能诞生以来,发展迅速,产生了许多分支。
诸如强化学习、模拟环境、智能硬件、机器学习等。
但是,在当前人工智能技术迅猛发展,为人们的生活带来许多便利。
下面是搜索整理的人工智能英文参考文献的分享,供大家借鉴参考。
人工智能英文参考文献一:[1]Lars Egevad,Peter Str?m,Kimmo Kartasalo,Henrik Olsson,Hemamali Samaratunga,Brett Delahunt,Martin Eklund. The utility of artificial intelligence in the assessment of prostate pathology[J]. Histopathology,2020,76(6).[2]Rudy van Belkom. The Impact of Artificial Intelligence on the Activities ofa Futurist[J]. World Futures Review,2020,12(2).[3]Reza Hafezi. How Artificial Intelligence Can Improve Understanding in Challenging Chaotic Environments[J]. World Futures Review,2020,12(2).[4]Alejandro Díaz-Domínguez. How Futures Studies and Foresight Could Address Ethical Dilemmas of Machine Learning and Artificial Intelligence[J]. World Futures Review,2020,12(2).[5]Russell T. Warne,Jared Z. Burton. Beliefs About Human Intelligence in a Sample of Teachers and Nonteachers[J]. Journal for the Education of the Gifted,2020,43(2).[6]Russell Belk,Mariam Humayun,Ahir Gopaldas. Artificial Life[J]. Journal of Macromarketing,2020,40(2).[7]Walter Kehl,Mike Jackson,Alessandro Fergnani. Natural Language Processing and Futures Studies[J]. World Futures Review,2020,12(2).[8]Anne Boysen. Mine the Gap: Augmenting Foresight Methodologies with Data Analytics[J]. World Futures Review,2020,12(2).[9]Marco Bevolo,Filiberto Amati. The Potential Role of AI in Anticipating Futures from a Design Process Perspective: From the Reflexive Description of “Design” to a Discussion of Influences by the Inclusion of AI in the Futures Research Process[J]. World Futures Review,2020,12(2).[10]Lan Xu,Paul Tu,Qian Tang,Dan Seli?teanu. Contract Design for Cloud Logistics (CL) Based on Blockchain Technology (BT)[J]. Complexity,2020,2020.[11]L. Grant,X. Xue,Z. Vajihi,A. Azuelos,S. Rosenthal,D. Hopkins,R. Aroutiunian,B. Unger,A. Guttman,M. Afilalo. LO32: Artificial intelligence to predict disposition to improve flow in the emergency department[J]. CJEM,2020,22(S1).[12]A. Kirubarajan,A. Taher,S. Khan,S. Masood. P071: Artificial intelligence in emergency medicine: A scoping review[J]. CJEM,2020,22(S1).[13]L. Grant,P. Joo,B. Eng,A. Carrington,M. Nemnom,V. Thiruganasambandamoorthy. LO22: Risk-stratification of emergency department syncope by artificial intelligence using machine learning: human, statistics or machine[J]. CJEM,2020,22(S1).[14]Riva Giuseppe,Riva Eleonora. OS for Ind Robots: Manufacturing Robots Get Smarter Thanks to Artificial Intelligence.[J]. Cyberpsychology, behavior and social networking,2020,23(5).[15]Markus M. Obmann,Aurelio Cosentino,Joshy Cyriac,Verena Hofmann,Bram Stieltjes,Daniel T. Boll,Benjamin M. Yeh,Matthias R. Benz. Quantitative enhancement thresholds and machine learning algorithms for the evaluation of renal lesions using single-phase split-filter dual-energy CT[J]. Abdominal Radiology,2020,45(1).[16]Haytham H. Elmousalami,Mahmoud Elaskary. Drilling stuck pipe classification and mitigation in the Gulf of Suez oil fields using artificial intelligence[J]. Journal of Petroleum Exploration and Production Technology,2020,10(10).[17]Rüdiger Schulz-Wendtland,Karin Bock. Bildgebung in der Mammadiagnostik –Ein Ausblick <trans-title xml:lang="en">Imaging in breast diagnostics—an outlook [J]. Der Gyn?kologe,2020,53(6).</trans-title>[18]Nowakowski Piotr,Szwarc Krzysztof,Boryczka Urszula. Combining an artificial intelligence algorithm and a novel vehicle for sustainable e-waste collection[J]. Science of the Total Environment,2020,730.[19]Wang Huaizhi,Liu Yangyang,Zhou Bin,Li Canbing,Cao Guangzhong,Voropai Nikolai,Barakhtenko Evgeny. Taxonomy research of artificial intelligence for deterministic solar power forecasting[J]. Energy Conversion and Management,2020,214.[20]Kagemoto Hiroshi. Forecasting a water-surface wave train with artificial intelligence- A case study[J]. Ocean Engineering,2020,207.[21]Tomonori Aoki,Atsuo Yamada,Kazuharu Aoyama,Hiroaki Saito,Gota Fujisawa,Nariaki Odawara,Ryo Kondo,Akiyoshi Tsuboi,Rei Ishibashi,Ayako Nakada,Ryota Niikura,Mitsuhiro Fujishiro,Shiro Oka,Soichiro Ishihara,Tomoki Matsuda,Masato Nakahori,Shinji Tanaka,Kazuhiko Koike,Tomohiro Tada. Clinical usefulness of a deep learning‐based system as the first screening on small‐bowel capsule endoscopy reading[J]. Digestive Endoscopy,2020,32(4).[22]Masashi Fujii,Hajime Isomoto. Next generation of endoscopy: Harmony with artificial intelligence and robotic‐assisted devices[J]. Digestive Endoscopy,2020,32(4).[23]Roberto Verganti,Luca Vendraminelli,Marco Iansiti. Innovation and Design in the Age of Artificial Intelligence[J]. Journal of Product Innovation Management,2020,37(3).[24]Yuval Elbaz,David Furman,Maytal Caspary Toroker. Modeling Diffusion in Functional Materials: From Density Functional Theory to Artificial Intelligence[J]. Advanced Functional Materials,2020,30(18).[25]Dinesh Visva Gunasekeran,Tien Yin Wong. Artificial Intelligence in Ophthalmology in 2020: A Technology on the Cusp for Translation and Implementation[J]. Asia-Pacific Journal of Ophthalmology,2020,9(2).[26]Fu-Neng Jiang,Li-Jun Dai,Yong-Ding Wu,Sheng-Bang Yang,Yu-Xiang Liang,Xin Zhang,Cui-Yun Zou,Ren-Qiang He,Xiao-Ming Xu,Wei-De Zhong. The study of multiple diagnosis models of human prostate cancer based on Taylor database by artificial neural networks[J]. Journal of the Chinese Medical Association,2020,83(5).[27]Matheus Calil Faleiros,Marcello Henrique Nogueira-Barbosa,Vitor Faeda Dalto,JoséRaniery Ferreira Júnior,Ariane Priscilla Magalh?es Tenório,Rodrigo Luppino-Assad,Paulo Louzada-Junior,Rangaraj Mandayam Rangayyan,Paulo Mazzoncini de Azevedo-Marques. Machine learning techniques for computer-aided classification of active inflammatory sacroiliitis in magnetic resonance imaging[J]. Advances in Rheumatology,2020,60(1078).[28]Balamurugan Balakreshnan,Grant Richards,Gaurav Nanda,Huachao Mao,Ragu Athinarayanan,Joseph Zaccaria. PPE Compliance Detection using Artificial Intelligence in Learning Factories[J]. Procedia Manufacturing,2020,45.[29]M. Stévenin,V. Avisse,N. Ducarme,A. de Broca. Qui est responsable si un robot autonome vient à entra?ner un dommage ?[J]. Ethique et Santé,2020.[30]Fatemeh Barzegari Banadkooki,Mohammad Ehteram,Fatemeh Panahi,Saad Sh. Sammen,Faridah Binti Othman,Ahmed EL-Shafie. Estimation of Total Dissolved Solids (TDS) using New Hybrid Machine Learning Models[J]. Journal of Hydrology,2020.[31]Adam J. Schwartz,Henry D. Clarke,Mark J. Spangehl,Joshua S. Bingham,DavidA. Etzioni,Matthew R. Neville. Can a Convolutional Neural Network Classify Knee Osteoarthritis on Plain Radiographs as Accurately as Fellowship-Trained Knee Arthroplasty Surgeons?[J]. The Journal of Arthroplasty,2020.[32]Ivana Nizetic Kosovic,Toni Mastelic,Damir Ivankovic. Using Artificial Intelligence on environmental data from Internet of Things for estimating solar radiation: Comprehensive analysis[J]. Journal of Cleaner Production,2020.[33]Lauren Fried,Andrea Tan,Shirin Bajaj,Tracey N. Liebman,David Polsky,Jennifer A. Stein. Technological advances for the detection of melanoma: Part I. Advances in diagnostic techniques[J]. Journal of the American Academy of Dermatology,2020.[34]Mohammed Amoon,Torki Altameem,Ayman Altameem. Internet of things Sensor Assisted Security and Quality Analysis for Health Care Data Sets Using Artificial Intelligent Based Heuristic Health Management System[J]. Measurement,2020.[35]E. Lotan,C. Tschider,D.K. Sodickson,A. Caplan,M. Bruno,B. Zhang,Yvonne W. Lui. Medical Imaging and Privacy in the Era of Artificial Intelligence: Myth, Fallacy, and the Future[J]. Journal of the American College of Radiology,2020.[36]Fabien Lareyre,Cédric Adam,Marion Carrier,Juliette Raffort. Artificial Intelligence in Vascular Surgery: moving from Big Data to Smart Data[J]. Annals of Vascular Surgery,2020.[37]Ilesanmi Daniyan,Khumbulani Mpofu,Moses Oyesola,Boitumelo Ramatsetse,Adefemi Adeodu. Artificial intelligence for predictive maintenance in the railcar learning factories[J]. Procedia Manufacturing,2020,45.[38]Janet L. McCauley,Anthony E. Swartz. Reframing Telehealth[J]. Obstetrics and Gynecology Clinics of North America,2020.[39]Jean-Emmanuel Bibault,Lei Xing. Screening for chronic obstructive pulmonary disease with artificial intelligence[J]. The Lancet Digital Health,2020,2(5).[40]Andrea Laghi. Cautions about radiologic diagnosis of COVID-19 infection driven by artificial intelligence[J]. The Lancet Digital Health,2020,2(5).人工智能英文参考文献二:[41]K. Orhan,I. S. Bayrakdar,M. Ezhov,A. Kravtsov,T. ?zyürek. Evaluation of artificial intelligence for detecting periapical pathosis on cone‐beam computed tomography scans[J]. International Endodontic Journal,2020,53(5).[42]Avila A M,Mezi? I. Data-driven analysis and forecasting of highway traffic dynamics.[J]. Nature communications,2020,11(1).[43]Neri Emanuele,Miele Vittorio,Coppola Francesca,Grassi Roberto. Use of CT andartificial intelligence in suspected or COVID-19 positive patients: statement of the Italian Society of Medical and Interventional Radiology.[J]. La Radiologia medica,2020.[44]Tau Noam,Stundzia Audrius,Yasufuku Kazuhiro,Hussey Douglas,Metser Ur. Convolutional Neural Networks in Predicting Nodal and Distant Metastatic Potential of Newly Diagnosed Non-Small Cell Lung Cancer on FDG PET Images.[J]. AJR. American journal of roentgenology,2020.[45]Coppola Francesca,Faggioni Lorenzo,Regge Daniele,Giovagnoni Andrea,Golfieri Rita,Bibbolino Corrado,Miele Vittorio,Neri Emanuele,Grassi Roberto. Artificial intelligence: radiologists' expectations and opinions gleaned from a nationwide online survey.[J]. La Radiologia medica,2020.[46]?. ? ? ? ? [J]. ,2020,25(4).[47]Savage Rock H,van Assen Marly,Martin Simon S,Sahbaee Pooyan,Griffith Lewis P,Giovagnoli Dante,Sperl Jonathan I,Hopfgartner Christian,K?rgel Rainer,Schoepf U Joseph. Utilizing Artificial Intelligence to Determine Bone Mineral Density Via Chest Computed Tomography.[J]. Journal of thoracic imaging,2020,35 Suppl 1.[48]Brzezicki Maksymilian A,Bridger Nicholas E,Kobeti? Matthew D,Ostrowski Maciej,Grabowski Waldemar,Gill Simran S,Neumann Sandra. Artificial intelligence outperforms human students in conducting neurosurgical audits.[J]. Clinical neurology and neurosurgery,2020,192.[49]Lockhart Mark E,Smith Andrew D. Fatty Liver Disease: Artificial Intelligence Takes on the Challenge.[J]. Radiology,2020,295(2).[50]Wood Edward H,Korot Edward,Storey Philip P,Muscat Stephanie,Williams George A,Drenser Kimberly A. The retina revolution: signaling pathway therapies, genetic therapies, mitochondrial therapies, artificial intelligence.[J]. Current opinion in ophthalmology,2020,31(3).[51]Ho Dean,Quake Stephen R,McCabe Edward R B,Chng Wee Joo,Chow Edward K,Ding Xianting,Gelb Bruce D,Ginsburg Geoffrey S,Hassenstab Jason,Ho Chih-Ming,Mobley William C,Nolan Garry P,Rosen Steven T,Tan Patrick,Yen Yun,Zarrinpar Ali. Enabling Technologies for Personalized and Precision Medicine.[J]. Trends in biotechnology,2020,38(5).[52]Fischer Andreas M,Varga-Szemes Akos,van Assen Marly,Griffith L Parkwood,Sahbaee Pooyan,Sperl Jonathan I,Nance John W,Schoepf U Joseph. Comparison of Artificial Intelligence-Based Fully Automatic Chest CT Emphysema Quantification to Pulmonary Function Testing.[J]. AJR. American journal ofroentgenology,2020,214(5).[53]Moore William,Ko Jane,Gozansky Elliott. Artificial Intelligence Pertaining to Cardiothoracic Imaging and Patient Care: Beyond Image Interpretation.[J]. Journal of thoracic imaging,2020,35(3).[54]Hwang Eui Jin,Park Chang Min. Clinical Implementation of Deep Learning in Thoracic Radiology: Potential Applications and Challenges.[J]. Korean journal of radiology,2020,21(5).[55]Mateen Bilal A,David Anna L,Denaxas Spiros. Electronic Health Records to Predict Gestational Diabetes Risk.[J]. Trends in pharmacological sciences,2020,41(5).[56]Yao Xiang,Mao Ling,Lv Shunli,Ren Zhenghong,Li Wentao,Ren Ke. CT radiomics features as a diagnostic tool for classifying basal ganglia infarction onset time.[J]. Journal of the neurological sciences,2020,412.[57]van Assen Marly,Banerjee Imon,De Cecco Carlo N. Beyond the Artificial Intelligence Hype: What Lies Behind the Algorithms and What We Can Achieve.[J]. Journal of thoracic imaging,2020,35 Suppl 1.[58]Guzik Tomasz J,Fuster Valentin. Leaders in Cardiovascular Research: Valentin Fuster.[J]. Cardiovascular research,2020,116(6).[59]Fischer Andreas M,Eid Marwen,De Cecco Carlo N,Gulsun Mehmet A,van Assen Marly,Nance John W,Sahbaee Pooyan,De Santis Domenico,Bauer Maximilian J,Jacobs Brian E,Varga-Szemes Akos,Kabakus Ismail M,Sharma Puneet,Jackson Logan J,Schoepf U Joseph. Accuracy of an Artificial Intelligence Deep Learning Algorithm Implementing a Recurrent Neural Network With Long Short-term Memory for the Automated Detection of Calcified Plaques From Coronary Computed Tomography Angiography.[J]. Journal of thoracic imaging,2020,35 Suppl 1.[60]Ghosh Adarsh,Kandasamy Devasenathipathy. Interpretable Artificial Intelligence: Why and When.[J]. AJR. American journal of roentgenology,2020,214(5).[61]M.Rosario González-Rodríguez,M.Carmen Díaz-Fernández,Carmen Pacheco Gómez. Facial-expression recognition: An emergent approach to the measurement of tourist satisfaction through emotions[J]. Telematics and Informatics,2020,51.[62]Ru-Xi Ding,Iván Palomares,Xueqing Wang,Guo-Rui Yang,Bingsheng Liu,Yucheng Dong,Enrique Herrera-Viedma,Francisco Herrera. Large-Scale decision-making: Characterization, taxonomy, challenges and future directions from an Artificial Intelligence and applications perspective[J]. Information Fusion,2020,59.[63]Abdulrhman H. Al-Jebrni,Brendan Chwyl,Xiao Yu Wang,Alexander Wong,Bechara J. Saab. AI-enabled remote and objective quantification of stress at scale[J]. Biomedical Signal Processing and Control,2020,59.[64]Gillian Thomas,Elizabeth Eisenhauer,Robert G. Bristow,Cai Grau,Coen Hurkmans,Piet Ost,Matthias Guckenberger,Eric Deutsch,Denis Lacombe,Damien C. Weber. The European Organisation for Research and Treatment of Cancer, State of Science in radiation oncology and priorities for clinical trials meeting report[J]. European Journal of Cancer,2020,131.[65]Muhammad Asif. Are QM models aligned with Industry 4.0? A perspective on current practices[J]. Journal of Cleaner Production,2020,258.[66]Siva Teja Kakileti,Himanshu J. Madhu,Geetha Manjunath,Leonard Wee,Andre Dekker,Sudhakar Sampangi. Personalized risk prediction for breast cancer pre-screening using artificial intelligence and thermal radiomics[J]. Artificial Intelligence In Medicine,2020,105.[67]. Evaluation of Payer Budget Impact Associated with the Use of Artificial Intelligence in Vitro Diagnostic, Kidneyintelx, to Modify DKD Progression:[J]. American Journal of Kidney Diseases,2020,75(5).[68]Rohit Nishant,Mike Kennedy,Jacqueline Corbett. Artificial intelligence for sustainability: Challenges, opportunities, and a research agenda[J]. International Journal of Information Management,2020,53.[69]Hoang Nguyen,Xuan-Nam Bui. Soft computing models for predicting blast-induced air over-pressure: A novel artificial intelligence approach[J]. Applied Soft Computing Journal,2020,92.[70]Benjamin S. Hopkins,Aditya Mazmudar,Conor Driscoll,Mark Svet,Jack Goergen,Max Kelsten,Nathan A. Shlobin,Kartik Kesavabhotla,Zachary A Smith,Nader S Dahdaleh. Using artificial intelligence (AI) to predict postoperative surgical site infection: A retrospective cohort of 4046 posterior spinal fusions[J]. Clinical Neurology and Neurosurgery,2020,192.[71]Mei Yang,Runze Zhou,Xiangjun Qiu,Xiangfei Feng,Jian Sun,Qunshan Wang,Qiufen Lu,Pengpai Zhang,Bo Liu,Wei Li,Mu Chen,Yan Zhao,Binfeng Mo,Xin Zhou,Xi Zhang,Yingxue Hua,Jin Guo,Fangfang Bi,Yajun Cao,Feng Ling,Shengming Shi,Yi-Gang Li. Artificial intelligence-assisted analysis on the association between exposure to ambient fine particulate matter and incidence of arrhythmias in outpatients of Shanghai community hospitals[J]. Environment International,2020,139.[72]Fatemehalsadat Madaeni,Rachid Lhissou,Karem Chokmani,Sebastien Raymond,Yves Gauthier. Ice jam formation, breakup and prediction methods based on hydroclimatic data using artificial intelligence: A review[J]. Cold Regions Science and Technology,2020,174.[73]Steve Chukwuebuka Arum,David Grace,Paul Daniel Mitchell. A review of wireless communication using high-altitude platforms for extended coverage and capacity[J]. Computer Communications,2020,157.[74]Yong-Hong Kuo,Nicholas B. Chan,Janny M.Y. Leung,Helen Meng,Anthony Man-Cho So,Kelvin K.F. Tsoi,Colin A. Graham. An Integrated Approach of Machine Learning and Systems Thinking for Waiting Time Prediction in an Emergency Department[J]. International Journal of Medical Informatics,2020,139.[75]Matteo Terzi,Gian Antonio Susto,Pratik Chaudhari. Directional adversarial training for cost sensitive deep learning classification applications[J]. Engineering Applications of Artificial Intelligence,2020,91.[76]Arman Kilic. Artificial Intelligence and Machine Learning in Cardiovascular Health Care[J]. The Annals of Thoracic Surgery,2020,109(5).[77]Hossein Azarmdel,Ahmad Jahanbakhshi,Seyed Saeid Mohtasebi,Alfredo Rosado Mu?oz. Evaluation of image processing technique as an expert system in mulberry fruit grading based on ripeness level using artificial neural networks (ANNs) and support vector machine (SVM)[J]. Postharvest Biology and Technology,2020,166.[78]Wafaa Wardah,Abdollah Dehzangi,Ghazaleh Taherzadeh,Mahmood A. Rashid,M.G.M. Khan,Tatsuhiko Tsunoda,Alok Sharma. Predicting protein-peptide binding sites with a deep convolutional neural network[J]. Journal of Theoretical Biology,2020,496.[79]Francisco F.X. Vasconcelos,Róger M. Sarmento,Pedro P. Rebou?as Filho,Victor Hugo C. de Albuquerque. Artificial intelligence techniques empowered edge-cloud architecture for brain CT image analysis[J]. Engineering Applications of Artificial Intelligence,2020,91.[80]Masaaki Konishi. Bioethanol production estimated from volatile compositions in hydrolysates of lignocellulosic biomass by deep learning[J]. Journal of Bioscience and Bioengineering,2020,129(6).人工智能英文参考文献三:[81]J. Kwon,K. Kim. Artificial Intelligence for Early Prediction of Pulmonary Hypertension Using Electrocardiography[J]. Journal of Heart and Lung Transplantation,2020,39(4).[82]C. Maathuis,W. Pieters,J. van den Berg. Decision support model for effects estimation and proportionality assessment for targeting in cyber operations[J]. Defence Technology,2020.[83]Samer Ellahham. Artificial Intelligence in Diabetes Care[J]. The American Journal of Medicine,2020.[84]Yi-Ting Hsieh,Lee-Ming Chuang,Yi-Der Jiang,Tien-Jyun Chang,Chung-May Yang,Chang-Hao Yang,Li-Wei Chan,Tzu-Yun Kao,Ta-Ching Chen,Hsuan-Chieh Lin,Chin-Han Tsai,Mingke Chen. Application of deep learning image assessment software VeriSee? for diabetic retinopathy screening[J]. Journal of the Formosan Medical Association,2020.[85]Emre ARTUN,Burak KULGA. Selection of candidate wells for re-fracturing in tight gas sand reservoirs using fuzzy inference[J]. Petroleum Exploration and Development Online,2020,47(2).[86]Alberto Arenal,Cristina Armu?a,Claudio Feijoo,Sergio Ramos,Zimu Xu,Ana Moreno. Innovation ecosystems theory revisited: The case of artificial intelligence in China[J]. Telecommunications Policy,2020.[87]T. Som,M. Dwivedi,C. Dubey,A. Sharma. Parametric Studies on Artificial Intelligence Techniques for Battery SOC Management and Optimization of Renewable Power[J]. Procedia Computer Science,2020,167.[88]Bushra Kidwai,Nadesh RK. Design and Development of Diagnostic Chabot for supporting Primary Health Care Systems[J]. Procedia Computer Science,2020,167.[89]Asl? Bozda?,Ye?im Dokuz,?znur Begüm G?k?ek. Spatial prediction of PM 10 concentration using machine learning algorithms in Ankara, Turkey[J]. Environmental Pollution,2020.[90]K.P. Smith,J.E. Kirby. Image analysis and artificial intelligence in infectious disease diagnostics[J]. Clinical Microbiology and Infection,2020.[91]Alklih Mohamad YOUSEF,Ghahfarokhi Payam KAVOUSI,Marwan ALNUAIMI,Yara ALATRACH. Predictive data analytics application for enhanced oil recovery in a mature field in the Middle East[J]. Petroleum Exploration and Development Online,2020,47(2).[92]Omer F. Ahmad,Danail Stoyanov,Laurence B. Lovat. Barriers and pitfalls for artificial intelligence in gastroenterology: Ethical and regulatory issues[J]. Techniques and Innovations in Gastrointestinal Endoscopy,2020,22(2).[93]Sanne A. Hoogenboom,Ulas Bagci,Michael B. Wallace. Artificial intelligence in gastroenterology. The current state of play and the potential. How will it affect our practice and when?[J]. Techniques and Innovations in Gastrointestinal Endoscopy,2020,22(2).[94]Douglas K. Rex. Can we do resect and discard with artificial intelligence-assisted colon polyp “optical biopsy?”[J]. Techniques and Innovations in Gastrointestinal Endoscopy,2020,22(2).[95]Neal Shahidi,Michael J. Bourke. Can artificial intelligence accurately diagnose endoscopically curable gastrointestinal cancers?[J]. Techniques and Innovations in Gastrointestinal Endoscopy,2020,22(2).[96]Michael Byrne. Artificial intelligence in gastroenterology[J]. Techniques and Innovations in Gastrointestinal Endoscopy,2020,22(2).[97]Piet C. de Groen. Using artificial intelligence to improve adequacy of inspection in gastrointestinal endoscopy[J]. Techniques and Innovations in Gastrointestinal Endoscopy,2020,22(2).[98]Robin Zachariah,Andrew Ninh,William Karnes. Artificial intelligence for colon polyp detection: Why should we embrace this?[J]. Techniques and Innovations in Gastrointestinal Endoscopy,2020,22(2).[99]Alexandra T. Greenhill,Bethany R. Edmunds. A primer of artificial intelligence in medicine[J]. Techniques and Innovations in Gastrointestinal Endoscopy,2020,22(2).[100]Tomohiro Tada,Toshiaki Hirasawa,Toshiyuki Yoshio. The role for artificial intelligence in evaluation of upper GI cancer[J]. Techniques and Innovations in Gastrointestinal Endoscopy,2020,22(2).[101]Yahui Jiang,Meng Yang,Shuhao Wang,Xiangchun Li,Yan Sun. Emerging role of deep learning‐based artificial intelligence in tumor pathology[J]. Cancer Communications,2020,40(4).[102]Kristopher D. Knott,Andreas Seraphim,Joao B. Augusto,Hui Xue,Liza Chacko,Nay Aung,Steffen E. Petersen,Jackie A. Cooper,Charlotte Manisty,Anish N. Bhuva,Tushar Kotecha,Christos V. Bourantas,Rhodri H. Davies,Louise A.E. Brown,Sven Plein,Marianna Fontana,Peter Kellman,James C. Moon. The Prognostic Significance of Quantitative Myocardial Perfusion: An Artificial Intelligence–Based Approach Using Perfusion Mapping[J]. Circulation,2020,141(16).[103]Muhammad Asad,Ahmed Moustafa,Takayuki Ito. FedOpt: Towards Communication Efficiency and Privacy Preservation in Federated Learning[J]. Applied Sciences,2020,10(8).[104]Wu Wenzhi,Zhang Yan,Wang Pu,Zhang Li,Wang Guixiang,Lei Guanghui,Xiao Qiang,Cao Xiaochen,Bian Yueran,Xie Simiao,Huang Fei,Luo Na,Zhang Jingyuan,Luo Mingyan. Psychological stress of medical staffs during outbreak of COVID-19 and adjustment strategy.[J]. Journal of medical virology,2020.[105]. Eyenuk Fulfills Contract for Artificial Intelligence Grading of Retinal Images[J]. Telecomworldwire,2020.[106]Kim Tae Woo,Duhachek Adam. Artificial Intelligence and Persuasion: A Construal-Level Account.[J]. Psychological science,2020,31(4).[107]McCall Becky. COVID-19 and artificial intelligence: protecting health-care workers and curbing the spread.[J]. The Lancet. Digital health,2020,2(4).[108]Alca?iz Mariano,Chicchi Giglioli Irene A,Sirera Marian,Minissi Eleonora,Abad Luis. [Autism spectrum disorder biomarkers based on biosignals, virtual reality and artificial intelligence].[J]. Medicina,2020,80 Suppl 2.[109]Cong Lei,Feng Wanbing,Yao Zhigang,Zhou Xiaoming,Xiao Wei. Deep Learning Model as a New Trend in Computer-aided Diagnosis of Tumor Pathology for Lung Cancer.[J]. Journal of Cancer,2020,11(12).[110]Wang Fengdan,Gu Xiao,Chen Shi,Liu Yongliang,Shen Qing,Pan Hui,Shi Lei,Jin Zhengyu. Artificial intelligence system can achieve comparable results to experts for bone age assessment of Chinese children with abnormal growth and development.[J]. PeerJ,2020,8.[111]Hu Wenmo,Yang Huayu,Xu Haifeng,Mao Yilei. Radiomics based on artificial intelligence in liver diseases: where we are?[J]. Gastroenterology report,2020,8(2).[112]Batayneh Wafa,Abdulhay Enas,Alothman Mohammad. Prediction of the performance of artificial neural networks in mapping sEMG to finger joint angles via signal pre-investigation techniques.[J]. Heliyon,2020,6(4).[113]Aydin Emrah,Türkmen ?nan Utku,Namli G?zde,?ztürk ?i?dem,Esen Ay?e B,Eray Y Nur,Ero?lu Egemen,Akova Fatih. A novel and simple machine learning algorithm for preoperative diagnosis of acute appendicitis in children.[J]. Pediatric surgery international,2020.[114]Ellahham Samer. Artificial Intelligence in Diabetes Care.[J]. The Americanjournal of medicine,2020.[115]David J. Winkel,Thomas J. Weikert,Hanns-Christian Breit,Guillaume Chabin,Eli Gibson,Tobias J. Heye,Dorin Comaniciu,Daniel T. Boll. Validation of a fully automated liver segmentation algorithm using multi-scale deep reinforcement learning and comparison versus manual segmentation[J]. European Journal of Radiology,2020,126.[116]Binjie Fu,Guoshu Wang,Mingyue Wu,Wangjia Li,Yineng Zheng,Zhigang Chu,Fajin Lv. Influence of CT effective dose and convolution kernel on the detection of pulmonary nodules in different artificial intelligence software systems: A phantom study[J]. European Journal of Radiology,2020,126.[117]Georgios N. Kouziokas. A new W-SVM kernel combining PSO-neural network transformed vector and Bayesian optimized SVM in GDP forecasting[J]. Engineering Applications of Artificial Intelligence,2020,92.[118]Qingsong Ruan,Zilin Wang,Yaping Zhou,Dayong Lv. A new investor sentiment indicator ( ISI ) based on artificial intelligence: A powerful return predictor in China[J]. Economic Modelling,2020,88.[119]Mohamed Abdel-Basset,Weiping Ding,Laila Abdel-Fatah. The fusion of Internet of Intelligent Things (IoIT) in remote diagnosis of obstructive Sleep Apnea: A survey and a new model[J]. Information Fusion,2020,61.[120]Federico Caobelli. Artificial intelligence in medical imaging: Game over for radiologists?[J]. European Journal of Radiology,2020,126.以上就是关于人工智能参考文献的分享,希望对你有所帮助。
在海拔5000米以上地区利用单粒子方法探测γ暴实验构想--基于水切伦科夫技术
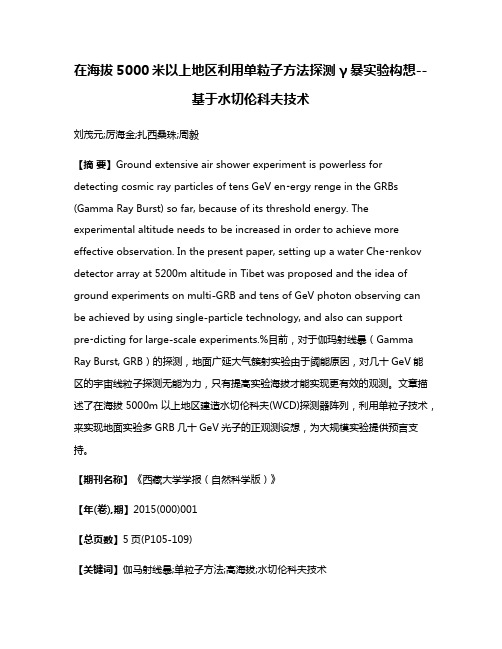
在海拔5000米以上地区利用单粒子方法探测γ暴实验构想--基于水切伦科夫技术刘茂元;厉海金;扎西桑珠;周毅【摘要】Ground extensive air shower experiment is powerless for detecting cosmic ray particles of tens GeV en⁃ergy renge in the GRBs (Gamma Ray Burst) so far, because of its threshold energy. The experimental altitude needs to be increased in order to achieve more effective observation. In the present paper, setting up a water Che⁃renkov detector array at 5200m altitude in Tibet was proposed and the idea of ground experiments on multi-GRB and tens of GeV photon observing can be achieved by using single-particle technology, and also can supportpre⁃dicting for large-scale experiments.%目前,对于伽玛射线暴(Gamma Ray Burst, GRB)的探测,地面广延大气簇射实验由于阈能原因,对几十GeV能区的宇宙线粒子探测无能为力,只有提高实验海拔才能实现更有效的观测。
文章描述了在海拔5000m以上地区建造水切伦科夫(WCD)探测器阵列,利用单粒子技术,来实现地面实验多GRB几十GeV光子的正观测设想,为大规模实验提供预言支持。
IEEEStdC57.124-1991
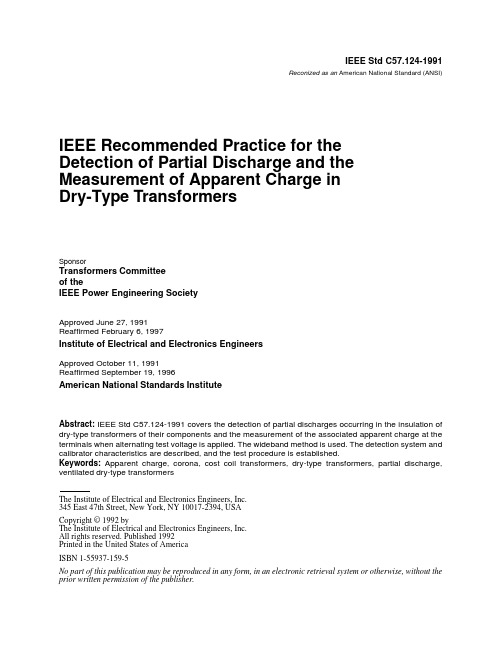
IEEE Std C57.124-1991Reconized as an American National Standard (ANSI)IEEE Recommended Practice for theDetection of Partial Discharge and the Measurement of Apparent Charge inDry-Type TransformersSponsorTransformers Committeeof theIEEE Power Engineering SocietyApproved June 27, 1991Reaffirmed February 6, 1997Institute of Electrical and Electronics EngineersApproved October 11, 1991Reaffirmed September 19, 1996American National Standards InstituteAbstract: IEEE Std C57.124-1991 covers the detection of partial discharges occurring in the insulation of dry-type transformers of their components and the measurement of the associated apparent charge at the terminals when alternating test voltage is applied. The wideband method is used. The detection system and calibrator characteristics are described, and the test procedure is established.Keywords: Apparent charge, corona, cost coil transformers, dry-type transformers, partial discharge, ventilated dry-type transformersThe Institute of Electrical and Electronics Engineers, Inc.345 East 47th Street, New York, NY 10017-2394, USACopyright © 1992 byThe Institute of Electrical and Electronics Engineers, Inc.All rights reserved. Published 1992Printed in the United States of AmericaISBN 1-55937-159-5No part of this publication may be reproduced in any form, in an electronic retrieval system or otherwise, without the prior written permission of the publisher.IEEE Standards documents are developed within the Technical Committees of the IEEE Societies and the Standards Coordinating Committees of the IEEE Standards Board. Members of the committees serve voluntarily and without compensation. They are not necessarily members of the Institute. The standards developed within IEEE represent a consensus of the broad expertise on the subject within the Institute as well as those activities outside of IEEE that have expressed an interest in participating in the development of the standard.Use of an IEEE Standard is wholly voluntary. The existence of an IEEE Standard does not imply that there are no other ways to produce, test, measure, purchase, market, or provide other goods and services related to the scope of the IEEE Standard. Furthermore, the viewpoint expressed at the time a standard is approved and issued is subject to change brought about through developments in the state of the art and comments received from users of the standard. Every IEEE Standard is subjected to review at least every five years for revision or reaffirmation. When a document is more than five years old and has not been reaffirmed, it is reasonable to conclude that its contents, although still of some value, do not wholly reflect the present state of the art. Users are cautioned to check to determine that they have the latest edition of any IEEE Standard.Comments for revision of IEEE Standards are welcome from any interested party, regardless of membership affiliation with IEEE. Suggestions for changes in documents should be in the form of a proposed change of text, together with appropriate supporting comments.Interpretations: Occasionally questions may arise regarding the meaning of portions of standards as they relate to specific applications. When the need for interpretations is brought to the attention of IEEE, the Institute will initiate action to prepare appropriate responses. Since IEEE Standards represent a consensus of all concerned interests, it is important to ensure that any interpretation has also received the concurrence of a balance of interests. For this reason IEEE and the members of its technical committees are not able to provide an instant response to interpretation requests except in those cases where the matter has previously received formal consideration.Comments on standards and requests for interpretations should be addressed to:Secretary, IEEE Standards Board445 Hoes LaneP.O. Box 1331Piscataway, NJ 08855-1331USAIEEE Standards documents are adopted by the Institute of Electrical and Electronics Engineers without regard to whether their adoption may involve patents on articles, materials, or processes. Such adoption does not assume any liability to any patent owner, nor does it assume any obligation whatever to parties adopting the standards documents.Foreword(This foreword is not a part of IEEE Std C57.124-1991, IEEE Recommended Practice for the Detection of Partial Discharge and the Measurement of Apparent Charge in Dry-Type Transformers.)This recommended practice for measuring partial discharge of dry-type transformers was conceived for the purpose of establishing a standardized method for conducting partial discharge tests of dry-type transformers. The results of the tests may be compared with various transformer designs and manufacturers to establish a partial discharge limit for dry-type transformers.This recommended practice follows the format of IEEE Std 454, IEEE Recommended Practice for the Detection and Measurement of Partial Discharge (Corona) During Dielectric Tests, and IEEE Std C57.113, IEEE Guide for Partial Discharge Measurement in Liquid-Filled Power Transformers and Shunt Reactors. Sections on current detection were purposely omitted, as this technique is normally not used for dry-type transformers.There is no recognized definition of “partial discharge-free"”when referring to partial discharge inception voltage or extinction voltage. An arbitrary sensitivity of 10 pC is suggested until such time as a more definitive standard is established.Various specifications are already written specifying partial discharge-free transformers from 1.1 p.u. operating voltage to 2.0 p.u. operating voltage. It is the intent of this recommended practice to encourage manufacturers of dry-type transformers and users of dry-type transformers to investigate and report the results of factory tests and field experience of partial discharge in dry-type transformers. It is recognized that Paschen's Law applies to the partial discharge intensity and extinction voltage of dry-type transformers. It is conceivable that a dry-type transformer would test partial discharge-free at 1.65 p.u. voltage at room temperature and be barely partial discharge-free at operating temperature for a Class 220C. system. This correlation should be verified with field experience and reported.The guide specifies no particular discharge testing instruments and systems. Several commercially available units are being used. A measuring system of discreet components readily available has been used for measuring partial discharge. Most manufacturers' laboratories have partial discharge-free HV test sets and oscilloscopes. The only additional components required to complete the detection circuit are a partial discharge-free coupling capacitor composed of two 60 kV, .002 mfd capacitors in series, and an inductance composed of a coil of magnet wire. Calibration is accomplished using a calibrated square wave generator and a calibrated coupling capacitor of .0001 mfd.The following two test procedures are proposed:1)To test partial discharge between the coil and ground, normally accomplished during the applied voltage test.2)The test procedure takes place during the induced voltage test to detect partial discharge within a coil. It issuggested that partial discharge measurements be made in both modes. The partial discharge measurement may be made during the normal sequence of tests, while the applied and induced voltage tests are being made.An alternative sequence is to conduct the partial discharge test immediately following the applied voltage test and induced voltage test.The high-voltage bus bars of high-voltage transformers sometimes cause nondestructive partial discharge. This partial discharge in no way affects the reliability of the transformer coils. It may be necessary to disconnect the bus bar from the coils before conducting the partial discharge test on only the coils in order to test for partial discharge in the transformer coils. A note should be added to any test reports stating that the bus bar was removed for the test.At the time this document was submitted to the Standards Board, the Working Group on Recommended Practice for the Detection of Partial Discharges and the Measurement of Apparent Charge in Dry-Type Transformers had the following members:A.D. Kline, (Chairman)B. F. Allen Roy Bancroft D. A. Barnard A. Bimbiris M. Cambre O. R. Compton J. FrankE. Gearhart R. Hayes R. H. HollisterJ. W. HuppA.M. IversenA. J. JonnattiS. P. KennedyE. KoenigM. L. ManningR. A. MarekM. I. MitelmanJ. J. NayW. F. PattersonR. L. ProvostJ. RoddenV. ThenappanR. E. Uptegraff, Jr.G. H. VaillancourtH. J. WindischThe following persons were on the balloting committee that approved this document for submission to the IEEE Standards Board:E. J. Adolphsen L. C. Aicher D. J. AllanB. AllenR. Allustriarti M. S. Altman J. C. Arnold J. AubinR. Bancroft D. Barnard D. L. Basel P. L. Bellaschi S. Bennon W. B. Binder J. V. Bonucchi J. D. Borst C. V. Brown O. R. Compton F. W. Cook J. L. Corkran D. W. Crofts J. N. Davis D. J. Douglas R. F. Dudley J. C. Dutton J. K. Easley J. A. Ebert D. J. Fallon F. L. Foster M. Frydman H. E. Gabel R. E. Gearhart D. W. Gerlach D. A. Gillies R. S. Girgis R. L. GrubbF. J. GryszkiewiczG. HallJ. H. HarlowF. W. HeinrichsW. R. HenningD. R. HightonP. J. HoeflerC. HoeselR. H. HollisterC. C. HoneyE. HowellsC. HurryG. W. IliffY. P. IijimaR. G. JacobsenD.C. JohnsonD. L. JohnsonA. J. JonnattiC. P. KappelerR. B. KaufmanJ. J. KellyW. N. KennedyJ.P. KinneyB. KlaponskiA.D. KlineE. KoenigJ. G. LackeyR. E. LeeH.F. LightS.R. LindgrenL.W. LongL. A. LowdermilkR. I. LoweM. L. ManningH. B. MargolisT. MassoudaJ. W. MatthewsJ. McGillC. J. McMillenW. J. McNuttS. P. MehtaC. K. MillerC. H. MillianR. E. MinkwitzM. MitelmanH. R. MooreW. E. MorehartR. J. MuselW. H. MutschlerE. T. NortonR. A. OlssonB. K. PatelW. F. PattersonH. A. PearceD. PercoL. W. PierceJ. M. PollittC. P. RaymondC. A. RobbinsL. J. SavioW. E. SaxonD. N, SharmaV. ShenoyW. W. SteinL. R. StenslandD. SundinL. A. SwensonD. S. TakachV. ThenappanR. C. Thomas J. A. Thompson T. P. Traub D. E. Truax W. B. Uhl R. E. Uptegraff, Jr.G. H. VaillancourtA. VeitchL. B. WagenaarR. J. WheartyA. L. WilksW. E. WrennA. C. WurdackE. J. YasudaAt the time this recommended practice was published, it was under consideration for approval as an American National Standard. The Accredited Standards Committee on Transformers, Regulators, and Reactors, C57, had the following members at the time this document was sent to letter ballot:Leo J. Savio, ChairJohn A. Gauthier, SecretaryOrganization of Representative Electric Light and Power Group...............................................................................................P.E. OrehekS. M. A. RizviF. StevensJ. SullivanJ. C. ThompsonM.C. Mingoia (Alt.) Institute of Electrical and Electronics Engineers......................................................................J. D. BorstJ. DavisJ. H. HarlowL. SavioH. D. SmithR. A. VeitchNational Electrical Manufacturers Association........................................................................G. D. CoulterP. DeweverJ. D. DouglasA. A. GhafourianK. R. LinsleyR. L. PlasterH. RobinR. E. Uptegraff, Jr.P. J. Hopkinson (Alt.)J. Nay (Alt.) Tennessee Valley Authority.......................................................................................................F. A. Lewis Underwriters Laboratories, Inc.................................................................................................W. T. O'GradyUS Department of Agriculture, REA........................................................................................J. BohlkUS Department of Energy, Western Area Power Administration.............................................D. R. TorgersonUS Department of the Interior, Bureau of Reclamation............................................................F. W. Cook, Sr.US Department of the Navy, Civil Engineering Carps.............................................................H. P. StickleyWhen the IEEE Standards Board approved this standard on June 27, 1991, it had the following membership:Marco W. Migliaro, ChairDonald C. Loughry, Vice ChairAndrew G. Salem, SecretaryDennis BodsonPaul L. BorrillClyde CampJames M. Daly Donald C. Fleckenstein Jay Forster*David F. Franklin Ingrid Fromm Thomas L. HannanDonald N. HeirmanKenneth D. HendrixJohn W. HorchBen C. JohnsonIvor N. KnightJoseph Koepfinger*Irving KolodnyMichael A. LawlerJohn E. May, Jr.Lawrence V. McCAllT. Don Michael*Stig L. NilssonJohn L. RankineRonald H. ReimerGary S. RobinsonTerrance R. Whittemore*Member EmeritusAlso included are the following nonvoting IEEE Standards Board liaisons:Fernando Aldana Satish K. AggarwalJames Beall Richard B. EngelmanStanley Warshaw Deborah A. CzyzIEEE Standards Project EditorCLAUSE PAGE1. Scope (1)2. Purpose (1)3. References (1)4. Definitions (2)5. Partial Discharge Detection System (3)5.1High-Voltage Coupling Circuit (3)5.2Measuring Impedance Unit (Z m) (4)5.3Filter Characteristics (5)5.4Display Unit (5)5.5Discharge Meter (6)5.6Basic Sensitivity (6)5.7Partial Discharge Detector Basic Sensitivity Test (6)6. Calibrator Characteristics (6)6.1Calibrating Capacitor Value (C q) (7)6.2Pulse Generator Rise Time and Decay Time (7)6.3Pulse Generator Amplitude (U o) (7)6.4Pulse Generator Output Impedance (Z o) (7)6.5Calibrator Output Level Adjustment (7)6.6Pulse Generator Frequency (7)7. Tests (7)7.1General Requirements (7)7.2Conditioning (8)7.3Requirements for the Test Voltage (8)7.4Transformer Connections (8)7.5Significance of Various Test Connections (8)7.6Choice of Test Procedure (9)7.7Disturbances (10)8. Bibliography (18)Annex (informative) Partial Discharge Recognition (24)IEEE Recommended Practice for the Detection and the Measurement of Partial Discharge in Dry-Type Transformers1. ScopeThis recommended practice applies to the detection of partial discharges occurring in the insulation of dry-type transformers or their components, and to the measurement of the associated apparent charge at the terminals when an alternating test voltage is applied.2. PurposePartial discharge measurements in dry-type transformers may preferably be made on the basis of measurement of the apparent charge. Relevant measuring systems are classified as narrow-band or wide-band systems. Both systems are recognized and widely used. Without giving preference to one or the other, it is the object of this document to describe the wide-band method. General principles of partial discharge measurements, including the narrow-band method, are covered in IEEE Std 454-19731 [8]2, IEC 270 (1981) [6]3and IEC 76-3 (1980) [5].3. ReferencesThe following publications should be used in conjunction with this document. When the standards referred to in this guide are superseded by a new revision approved by the relevant standards authority, the latest revision should apply.[1] ANSI C68.1-1968, Standard for Measurement of Voltage In Dielectric Tests.41This standard has been withdrawn, however, copies are available from the Institute of Electrical and Electronics Engineers, Inc., Service Center, 445 Hoes Lane, Piscataway, N.J. 08855, U.S.A.2The numbers in brackets refer to those listed in Section 4.3IEC publications are available from IEC Sales Department, Case Postale 131, 3 rue de Varembé, CH 1211, Genève 20, Switzerland/Suisse. IEC publications are also available in the United States from the Sales Department, American National Standards Institute, 11 West 42nd Street, 13th Floor, New York, NY 10036, USA.4ANSI publications are available from the American National Standards Institute, 11 West 42nd Street, 13th Floor, New York, NY 10036.IEEE Std C57.124-1991IEEE RECOMMENDED PRACTICE FOR THE DETECTION OF PARTIAL DISCHARGE [2] ASTM D1868-81 (1990-E01), Method for Detection and Measurement of Partial Discharge (Corona) Pulses in Evaluation of Insulation Systems.5[3] ASTM STP-669, Engineering Dielectrics, Vol. 1, Corona Measurement and Interpretation.[4] CIGRE Working Group 21-03, "Recognition of Discharges," Electra, No. 11, pp. 61-98, Dec, 1969.6[5] IEC 76-3 (1980), Power Transformers, Part 3: Insulation Levels and Dielectric Tests.7[6] IEC 270 (1981), Partial Discharge Measurements.[7] IEEE Std 436-1991, IEEE Guide for Making Corona (Partial Discharge) Measurements of Electronics Transformers (ANSI).8[8] IEEE Std 454-1973, IEEE Recommended Practice for the Detection and Measurement of Partial Discharge (Corona) During Dielectric Tests.[9] IEEE Std C57.113-1991, IEEE Guide for Partial Discharge Measurement in Oil-Filled Power Transformers and Shunt Reactors (ANSI).4. Definitionspartial discharge: A partial discharge within the terms of this document is an electric discharge that only partially bridges the insulation between conductors. The term “corona ” has also been used frequently with this connotation. Such usage is imprecise and is gradually being discontinued in favor of the term “partial discharge.”apparent charge (terminal charge): The apparent charge (q) of a partial discharge is that charge which, if it could be injected instantaneously between the terminals of the test object, would momentarily change the voltage between its terminals by the same amount as the partial discharge itself. The apparent charge should not be confused with the charge transferred across the discharging cavity in the dielectric medium. Apparent charge within the terms of this document is expressed in coulombs, abbreviated C. One pC is equal to 10-12 coulombs.repetition rate(n).: The partial discharge pulse repetition rate (n) is the average number of partial discharge pulses per second measured over a selected period of time.acceptable terminal partial discharge level: The acceptable terminal partial discharge level is that specified maximum terminal partial discharge value for which measured terminal partial discharge values exceeding the said value are considered unacceptable. The method of measurement and the test voltage for a given test object should be specified with the acceptable terminal partial discharge level.voltage related to partial discharges: Voltage within the terms of this document is the phase-to-ground alternating voltage for applied tests (Fig 1) or terminal to terminal alternating voltage for induced voltage tests (Fig 8). Its value is expressed by its peak value divided by the square root of two.partial discharge inception voltage: The lowest voltage at which partial discharges exceeding a specified level are observed under specified conditions when the voltage applied to the test object is gradually increased from a lower value. This voltage is expressed as the peak value divided by the square root of two.5ASTM publications are available from the American Society for Testing and Materials, Customer Service Dept., 916 Race Street, Philadelphia, P.A. 19103, U.S. A.6CIGRE publications are available from the International Conference on Large-Voltage Electric Systems, 112 Boulevard Haussman, F-75008 Paris, France.7IEC publications are available from IEC Sales Department, Case Postale 131, 3 rue de Varembé, CH 1211, Genève 20, Switzerland/Suisse. IEC publications are also available in the United States from the Sales Department, American National Standards Institute, 11 West 42nd Street, 13th Floor, New York, NY 10036, USA.8IEEE publications are available from the Institute of Electrical and Electronics Engineers, Inc., Service Center, 445 Hoes Lane, Piscataway, N.J. 08855, U.S. A.partial discharge extinction voltage: The voltage at which partial discharges exceeding a specified level cease under specified conditions when the voltage is gradually decreased from a value exceeding the inception voltage. This voltage is expressed as the peak value divided by the square root of two.partial discharge-free test voltage: The partial discharge-free test voltage is a specified voltage, applied in accordance with a specified test procedure, at which the test object should not exhibit partial discharges above the acceptable energized background noise level.energized background noise level: The energized background noise level stated in pC is the residual response of the partial discharge measurement system to background noise of any nature after the test circuit has been calibrated and the test object is energized at a maximum of 50% of its nominal operating voltage.acceptable energized background noise level: The acceptable energized background noise level present during test should not exceed 50% of the acceptable terminal discharge level, and in any case, should be below 100 pC (5 pC if an acceptable terminal discharge level of 10 pC is required.)5. Partial Discharge Detection System(Figs 9 and 10 taken from ASTM STP669)The partial discharge detection system comprises the following components:1) a high-voltage coupling circuit (C1, C v)2) a measuring impedance unit (Z m consisting of R m, C2 and L)3)an amplifier and filter circuit4) a display unit5) a discharge meter6) a calibrator (C q, V l)7) a source filter (Z optional)5.1 High-Voltage Coupling CircuitThe purpose of the high-voltage coupling circuit is to allow the connection of the measuring impedance (Z m) to the high-voltage terminal of the transformer under test. In other types of high-voltage equipment, a single, low-capacitance high-voltage capacitor is usually used for this purpose, but in transformers, the equivalent terminal capacitance is usually very low, so a substantial amount of signal is normally produced by partial discharge of only a few pC, and measurement sensitivity is not a problem. At the same time, due to standing waves within the winding, a certain amount of signal cancellation may occur if the bandwidth is not sufficiently wide. Therefore, to insure that the input circuit of the partial discharge detection system does not act as a differentiator and reduce the total bandwidth of the system, it has been found that a high value for the coupling capacitor is necessary to produce a long-time constant of the input circuit. Even then, however, the low equivalent terminal capacitance of the transformer, usually less than 500 pF, will limit this time constant and it may not be possible to make it sufficiently long. Therefore, the use of a single coupling capacitor is not recommended. On the other hand, a satisfactory time constant can always be obtained by using a second capacitor (C2) as part of the measurement impedance, and this is the recommended method.As shown in Figs 9 and 10, high-voltage capacitor C1, and low-voltage capacitor C2, will form a voltage divider. This voltage divider will reduce the sensitivity of the measurement. To make sure that both the sensitivity is still sufficient and the time constant is sufficiently long, the values of C1, C2, and L should respect the conditions below:(1)≥C1100pF(2)where R m is the parallel resistive part of the measuring impedance unit (Z m ). The value of R m should be determined from the particular partial discharge instrument that is used.Example:For f L = 70kHz ,R m = 2.5k Ω and C 1 = 100pFA value of 1000 pF may be chosen for C 2 since 1000 pF ≥ 909 pF and (3)In cases where an RLC measurement impedance is used, the value of L should satisfy the equation below. This will ensure that the measurement bandwidth is unaffected by the presence of L .(4)If the transformer to be tested is fitted with a capacitive bushing tap, then this can be used directly as the high-voltage coupling circuit, and a separate coupling capacitor C 1 is not needed.5.2 Measuring Impedance Unit (Z m )The measuring impedance unit (Z m ) is located physically close to the high-voltage coupling circuit and serves two main purposes:1)It attenuates the test voltage present on the high-voltage coupling circuit to a safe value for measurement of partial discharge signals;2)It matches the amplifier and filter circuit to the high-voltage coupling circuit in insuring a fiat frequencyresponse across the full measurement bandwidth.The measuring impedance unit (Z m )should be configured in such a way as to permit test voltage level monitoring and to observe the phase relationship between the test voltage and the partial discharge pulses; this technique helps to identify the nature of the discharges.As shown in Figs 9 and 10, capacitor C v , whose capacitance value should be chosen to be at least 500 times that of C 1,may be placed in either one of the following two positions:1)In series with inductor L (Fig 9 or 10), or 2)In series with the low-voltage side of high-voltage capacitor C 1 (Fig 10.)C 212llf L R m -------------------≥C 216.28x 70000x 2500,----------------------------------------------909pF ==1000100-----------1015≤=L 25006.28x 70000,------------------------------- 5.7mH ==C 2C 1------15≤L R m 2llf L -----------≥Figure 9 shows the preferred position for C v since the input impedance of the display unit does not shunt the measurement impedance and can be neglected. However, some voltage will reach the input of the amplifier and filter circuit and may cause it to saturate. This voltage can be decreased by increasing the value of C v until it is less than 5V . If saturation occurs, a 20 nF low-voltage capacitor may be placed in series with the input of the amplifier and filter circuit as shown to decouple voltage at the excitation frequency.In the cases where one can not be absolutely certain that saturation of the amplifier will not occur, it is then advised to place C v in the position shown in Fig 10. The impedance of the display circuit will now shunt the measurement impedance and its input capacitance will need to be considered to calculate the value of C 2, as it will add to it.5.2.1 Lower and Upper Cut-Off Frequencies (f L and f H )The lower and upper cut-off frequencies f L and f H , respectively, are the frequencies at which the response to a constant sinesoidal input voltage has fallen by 6 db from the maximum value occurring inside the recommended bandwidth.f L should be located in the range from 70 to 120 kHz to minimize the effect of winding attenuation on partial discharge signals, and at the same time, to provide adequate rejection of SCR-generated noise present in manufacturing plants.An upper limit on f H of 300 kHz is usually necessary to prevent broadcast stations from interfering with the partial discharge measurement.5.3 Filter CharacteristicsThe filter characteristics of the partial discharge detection circuit should be such as to provide attenuation of at least 50db at 25 kHz, of at least 60 db at 15 kHz and below, and of at least 20 db at 500 kHz and above, with respect to the response at the geometric mean frequency (f C ) of the system pass bandwidth that is given by:The filter may be combined with an amplifier to form an active filter. Care should be taken to prevent the saturation of the filter input by the presence of the applied test voltage.5.3.1 Bandwidth ∆fThe bandwidth ∆f is defined as:∆f = f H − f LThe bandwidth should not be less than 100 kHz. A wider bandwidth provides a response whose level is less sensitive to the location of a partial discharge pulse along a transformer winding and is, therefore, more uniform. A bandwidth wider than 100 kHz is preferable, but may lead to background noise problems.5.3.2 LinearityThe instrument circuit, display unit, and discharge meter should be linear within plus or minus 10% of full scale in the range of 50 to 1000 pC.5.4 Display UnitThe display unit should be a cathode ray oscilloscope with a linear, rectangular, or an elliptical time-base. In all cases,the time-base should be synchronized to the test voltage, and at least 98% of a full cycle should be displayed. The phase relationship of the partial discharges to the test voltage should be easy to determine. A suitable graticule should be provided.f c f L f H •().5=。
无线电发射设备频谱异常检测与干扰定位研究
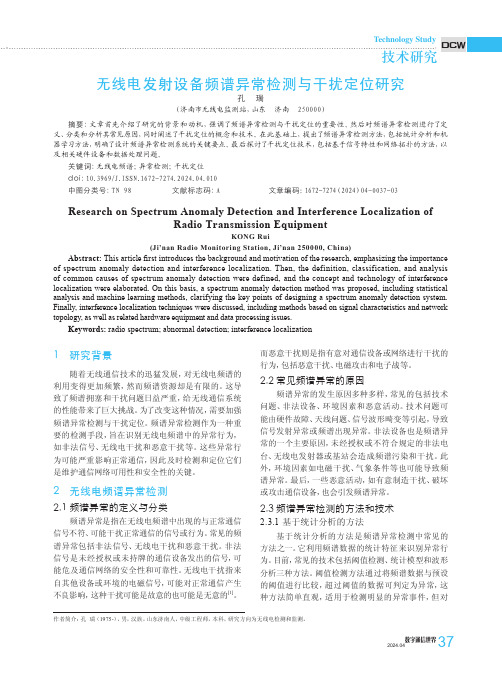
DCWTechnology Study技术研究37数字通信世界2024.041 研究背景随着无线通信技术的迅猛发展,对无线电频谱的利用变得更加频繁,然而频谱资源却是有限的。
这导致了频谱拥塞和干扰问题日益严重,给无线通信系统的性能带来了巨大挑战。
为了改变这种情况,需要加强频谱异常检测与干扰定位。
频谱异常检测作为一种重要的检测手段,旨在识别无线电频谱中的异常行为,如非法信号、无线电干扰和恶意干扰等。
这些异常行为可能严重影响正常通信,因此及时检测和定位它们是维护通信网络可用性和安全性的关键。
2 无线电频谱异常检测2.1 频谱异常的定义与分类频谱异常是指在无线电频谱中出现的与正常通信信号不符、可能干扰正常通信的信号或行为。
常见的频谱异常包括非法信号、无线电干扰和恶意干扰。
非法信号是未经授权或未持牌的通信设备发出的信号,可能危及通信网络的安全性和可靠性。
无线电干扰指来自其他设备或环境的电磁信号,可能对正常通信产生不良影响,这种干扰可能是故意的也可能是无意的[1]。
而恶意干扰则是指有意对通信设备或网络进行干扰的行为,包括恶意干扰、电磁攻击和电子战等。
2.2 常见频谱异常的原因频谱异常的发生原因多种多样,常见的包括技术问题、非法设备、环境因素和恶意活动。
技术问题可能由硬件故障、天线问题、信号波形畸变等引起,导致信号发射异常或频谱出现异常。
非法设备也是频谱异常的一个主要原因,未经授权或不符合规定的非法电台、无线电发射器或基站会造成频谱污染和干扰。
此外,环境因素如电磁干扰、气象条件等也可能导致频谱异常。
最后,一些恶意活动,如有意制造干扰、破坏或攻击通信设备,也会引发频谱异常。
2.3 频谱异常检测的方法和技术2.3.1 基于统计分析的方法基于统计分析的方法是频谱异常检测中常见的方法之一。
它利用频谱数据的统计特征来识别异常行为。
目前,常见的技术包括阈值检测、统计模型和波形分析三种方法。
阈值检测方法通过将频谱数据与预设的阈值进行比较,超过阈值的数据可判定为异常,这种方法简单直观,适用于检测明显的异常事件,但对无线电发射设备频谱异常检测与干扰定位研究孔 瑞(济南市无线电监测站,山东 济南 250000)摘要:文章首先介绍了研究的背景和动机,强调了频谱异常检测与干扰定位的重要性。
一种民用小型无人机的射频指纹识别方法
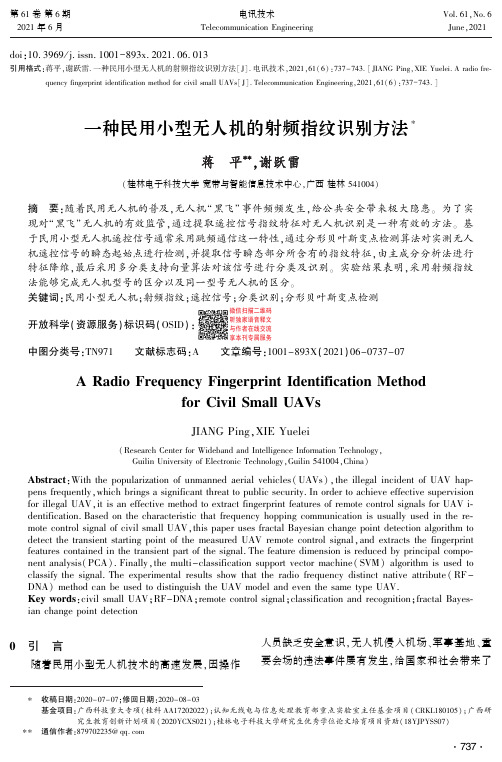
doi:10.3969/j.issn.1001-893x.2021.06.013引用格式:蒋平,谢跃雷.一种民用小型无人机的射频指纹识别方法[J].电讯技术,2021,61(6):737-743.[JIANG Ping,XIE Yuelei.A radio fre-quency fingerprint identification method for civil small UAVs[J].Telecommunication Engineering,2021,61(6):737-743.]一种民用小型无人机的射频指纹识别方法∗蒋㊀平∗∗,谢跃雷(桂林电子科技大学宽带与智能信息技术中心,广西桂林541004)摘㊀要:随着民用无人机的普及,无人机 黑飞 事件频频发生,给公共安全带来极大隐患㊂为了实现对 黑飞 无人机的有效监管,通过提取遥控信号指纹特征对无人机识别是一种有效的方法㊂基于民用小型无人机遥控信号通常采用跳频通信这一特性,通过分形贝叶斯变点检测算法对实测无人机遥控信号的瞬态起始点进行检测,并提取信号瞬态部分所含有的指纹特征,由主成分分析法进行特征降维,最后采用多分类支持向量算法对该信号进行分类及识别㊂实验结果表明,采用射频指纹法能够完成无人机型号的区分以及同一型号无人机的区分㊂关键词:民用小型无人机;射频指纹;遥控信号;分类识别;分形贝叶斯变点检测开放科学(资源服务)标识码(OSID):微信扫描二维码听独家语音释文与作者在线交流享本刊专属服务中图分类号:TN971㊀㊀文献标志码:A㊀㊀文章编号:1001-893X(2021)06-0737-07A Radio Frequency Fingerprint Identification Methodfor Civil Small UAVsJIANG Ping,XIE Yuelei(Research Center for Wideband and Intelligence Information Technology,Guilin University of Electronic Technology,Guilin541004,China) Abstract:With the popularization of unmanned aerial vehicles(UAVs),the illegal incident of UAV hap-pens frequently,which brings a significant threat to public security.In order to achieve effective supervision for illegal UAV,it is an effective method to extract fingerprint features of remote control signals for UAV i-dentification.Based on the characteristic that frequency hopping communication is usually used in the re-mote control signal of civil small UAV,this paper uses fractal Bayesian change point detection algorithm to detect the transient starting point of the measured UAV remote control signal,and extracts the fingerprint features contained in the transient part of the signal.The feature dimension is reduced by principal compo-nent analysis(PCA).Finally,the multi-classification support vector machine(SVM)algorithm is used to classify the signal.The experimental results show that the radio frequency distinct native attribute(RF-DNA)method can be used to distinguish the UAV model and even the same type UAV.Key words:civil small UAV;RF-DNA;remote control signal;classification and recognition;fractal Bayes-ian change point detection0㊀引㊀言随着民用小型无人机技术的高速发展,因操作人员缺乏安全意识,无人机侵入机场㊁军事基地㊁重要会场的违法事件屡有发生,给国家和社会带来了㊃737㊃第61卷第6期2021年6月电讯技术Telecommunication Engineering Vol.61,No.6 June,2021∗∗∗收稿日期:2020-07-07;修回日期:2020-08-03基金项目:广西科技重大专项(桂科AA17202022);认知无线电与信息处理教育部重点实验室主任基金项目(CRKL180105);广西研究生教育创新计划项目(2020YCXS021);桂林电子科技大学研究生优秀学位论文培育项目资助(18YJPYSS07)通信作者:879702235@严重的安全隐患[1]㊂因此,加强对无人机的管控势在必行,而如何探测和发现无人机则是实现管控的第一步[2-4]㊂探测和识别无人机的射频信号,是发现无人机的一种有效方法[5-7]㊂民用小型无人机的射频信号可分为遥控信号及图传信号,遥控信号用于无人机控制,通常采用跳频方式的扩频通信信号,而无人机图传信号则用于空中拍摄视频的传输,通常采用正交频分复用技术(Orthogonal Frequency Division Mul-tiplexing,OFDM)的调制信号㊂许多学者通过无人机遥控信号对无人机进行检测及识别,其中文献[5]给出了一种基于无线电信号特征识别的无人机监测算法设计,从跳频信号及图传信号方面对无人机进行探测,但未给出具体算法分析及更近一步的实现原理;文献[6]提出基于软件无线电平台的无人机入侵检测,通过无人机跳频信号特征对无人机进行检测与识别,能在15m内检测无人机的存在,但该方法无法完成对无人机具体型号的区分;文献[7]采用对跳频信号进行图像分类的方式完成无人机信号的检测与识别,并取得了较好的识别效果,但跳频信号易受噪声淹没造成信号丢失,导致其不能较好地进行参数估计,从而无法有效区分无人机型号,并且该方法不能区分个体㊂针对以上检测及识别所存在的缺陷,本文采用射频指纹提取法(Radio Frequency Distinct Native At-tribute,RF-DNA)[8-9]对遥控信号进行检测及识别㊂首先零中频接收机对无人机遥控信号进行侦收,随后检测遥控信号瞬态部分起始点并进行统计特征提取,构造RF-DNA指纹特征并对其进行特征降维,最后由多支持向量机(Support Vector Machine, SVM)分类器对无人机型号以及同一型号的个体进行区分㊂1 无人机遥控信号模型对于无人机的检测与识别,需从信号方面进行分析㊂民用无人机遥控信号通常采用跳频方式进行扩频通信[10-11],因此遥控信号即为用于无人机控制的跳频信号㊂跳频信号因其具有较好的抗干扰能力,广泛用于通信对抗方面,而民用无人机的控制也在其列㊂信息数据m(t)通过信号调制器得到d(t),发射的跳频信号为S(t)=d(t)S FH(t)㊂(1)式中:S FH(t)是跳频信号,表达式为S FH(t)=AðN-1k=0w T(t-kT h)cos[2πf k(t-kT h)+φn]㊂(2)式中:N为频点个数;A为振幅;w T为宽度为T h的矩形窗,T h为跳频信号的跳频周期;f0,f1,f2, ,f k为调频频率集;φn为初始相位,n=0,1,2, ,N-1㊂实测无人机遥控信号离散数据由Cool Edit Pro 软件打开,如图1所示㊂图1㊀无人机遥控信号瞬态及稳态图㊃837㊃电讯技术㊀㊀㊀㊀2021年㊀㊀从图1可知,不同厂商无人机机型具有不同瞬态部分,但同一无人机型号的瞬态部分不易区分㊂本文主要基于民用无人机遥控信号瞬态部分进行研究㊂对于无人遥控信号瞬态部分,因无人机发射设备硬件特性不同,导致瞬态部分出现细微差异,这些差异主要由无人机发射设备系统中的分立器件㊁信号混频器㊁功率放大器㊁数模转换器㊁滤波器㊁锁相环等多种硬件设备产生㊂瞬态部分不携带数据信息,只与硬件设备本身的特性有关,具有唯一性,所以常对瞬态部分进行分析㊂瞬态部分存在于信号功率由零变为额定功率之间,所以一般存在发射设备开关机时刻㊂因此采集发射设备的瞬态部分具有一定难度,尤其体现在硬件接收设备[12]㊂由于无人机遥控信号采用跳频通信方式,在操控无人机期间,信号会不停经历由功率零到额定功率的变化过程,所以采用RF -DNA 方法对无人机遥控信号进行检测及识别是一个有效的方法㊂2㊀基于RF -DNA 的无人机识别2.1㊀RF -DNA 特征提取RF -DNA 方法是近年来较为关注方法之一,最早由美国空军技术学院Temple 等人提出㊂该方法是一种采用统计方法生成射频指纹(Radio Frequen-cy Fingerprinting,RFF)特征的计算框架,可分成瞬态信号子区域划分㊁瞬态信号基础特征生成和瞬态信号统计特征生成㊂对于该算法,对其分步骤描述㊂Step 1㊀对接收信号X (n )进行希尔伯特变换,得其解析式:X (n )=I (n )+j Q (n )㊂(3)式中:I (n )㊁Q (n )为正交信号㊂Step 2㊀求信号瞬时幅度a (n )㊁瞬时相位p (n )和瞬时频率f (n ):a (n )=I (n )2+Q (n )2,(4)p (n )=arctan Q (n )I (n )éëêêùûúú,(5)f (n )=12πp (n )-p (n -1)Δn㊂(6)Step 3㊀为了消除零中频接收机偏差对瞬时信号影响,对瞬时信号进行中心化处理:a c (n )=a (n )-u a ,(7)f c (n )=f (n )-u f ㊂(8)对于瞬时相位,需在中心化处理之前对瞬时相位中的非线性分量进行逐个滤除,以保证特征提取质量:p nl =p (n )-2πu f (n )Δt ,(9)p c (n )=p nl (n )-u p nl ㊂(10)式中:u a ㊁u f 表示瞬时幅度与瞬时频率的均值,Δt 表示采样时间间隔,u p nl 为消除非线性分量后瞬时相位平均值,p nl 表示非线性相位响应,a c (n )㊁f c (n )㊁p c (n )分别为中心化处理后的瞬时幅度㊁瞬时频率㊁瞬时相位值㊂Step 4㊀将以上所求三个瞬时特征a c (n )㊁f c (n )㊁p c (n )进行分区,并对其求特征值㊂这里特征值有两种方式,第一种为求三个时域瞬时信号的方差㊁偏度和峰度,第二种为求三个时域瞬时信号的标准差㊁方差㊁偏度和峰度㊂标准差:σ=1N x ðN xn =1(x c (n )-u )2㊂(11)方差:σ2=1N x ðN xn =1(x c (n )-u )2㊂(12)偏度:r =1N x σ3ðN xn =1(x c (n )-u )3㊂(13)峰度:k =1N x σ4ðN xn =1(x c (n )-u )4㊂(14)式中:N x 表示中心化数据x c (n )的长度,u 表示x c (n )的均值㊂Step 5㊀求其特征向量,因其具有三种特征,方法一为标准RF -DNA 法,只求方差㊁偏度㊁峰度,则特征具有3ˑ3维,而方法二添加标准差这一特征,则特征具有3ˑ4维㊂每一架无人机的每一个跳频信号瞬时幅度㊁瞬时频率㊁瞬时相位特征所求标准差㊁方差㊁偏度㊁峰度的集如下:F a =[σσ2r k ]a ,(15)F p =σσ2r k []p ,(16)F f =σσ2r k []f ,(17)㊃937㊃第61卷蒋平,谢跃雷:一种民用小型无人机的射频指纹识别方法第6期F i=[F a F p F f]㊂(18)式中:F i为每一架无人机每一跳信号的特征集合㊂一架无人机所有跳频信号瞬态特征集如下:F R=F1,F2,F3, ,F N[]㊂(19)式中:N为每一架无人机跳频信号总共个数㊂所有无人机的无人机跳频信号瞬态特征集合表达式如下:F C=F R1,F R2,F R3, ,F Rj[]㊂(20)式中:j为无人机个数㊂取一组各个无人机瞬时幅度的标准差㊁方差㊁峰度㊁偏度特征进行特征统计,统计值如表1所示㊂表1㊀遥控信号瞬时幅度统计特征表特征标准差方差偏度峰度大疆精灵4pro1号8.611274.15310.0282 1.8725大疆精灵4pro2号7.633258.2650-0.6562 2.2574司马航模x8hw404.37152 1.6379ˑ105-0.2373 2.1364HM 6.021632.2594-7.231976.5866大疆悟252.0166 2.7057ˑ103 1.3153 3.8405司马航模x25pro233.0327 5.4304ˑ104-0.3815 1.6164 2.2㊀识别算法本文主要目的是从信号角度对无人机进行识别,其中识别的具体步骤如下:Step1㊀采集无人机实测数据㊂Step2㊀采用分形贝叶斯变点检测算法对无人机遥控信号瞬态部分进行提取㊂Step3㊀采用RF-DNA统计特征法进行特征提取,提取采用两种方式,第一种含有标准差,第二种不含有标准差㊂Step4㊀对Step3所提取的特征集采用主成分分析(Principal Component Analysis,PCA)算法进行特征降维,特征降维可将维数降维为二维㊁三维㊁四维等,不同维数对识别率有一定影响㊂Step5㊀通过SVM[13]分类器对降维后的数据进行分类识别㊂这里分类器采用Libsvm进行分类,该分类器具有多分类特点,采用的是一对一法完成多分类操作㊂3㊀实验分析本次实验主要采用自制硬件设备对大疆精灵4pro1号及2号㊁司马航模x8hw㊁HM㊁大疆悟2㊁司马航模x25pro无人机信号进行采集,完成相应信号预处理及分类识别,采集系统如图2所示㊂图2㊀无人机遥控信号采集系统实物图通过对5架无人机共225组信号数据段进行实验,其中每个无人机训练数据30组,测试数据15组㊂实验中,因含有三个瞬时特征且每一瞬时特征含有多种特征信息,且含有标准差的特征维数为12维,不含有标准差的为9维㊂采用PCA算法将特征集降维到二维㊁三维,其中二维散点图坐标轴F1㊁F2分别代表二维中维数特征,三维散点图中坐标轴F1㊁F2㊁F3分别代表三维中维数的特征㊂实验1:采用不含有标准差㊁特征降维维数为二维的方式进行分类识别,实测数据二维散点图如图3所示㊂图3㊀无人机遥控信号不含标准差二维特征散点图㊃047㊃电讯技术㊀㊀㊀㊀2021年该图共10类散点数据,主要是5类无人机训练数据和5类无人机测试数据,不同颜色及形状表示不同无人机㊂无人机训练数据用于建立数据单元库,无人机测试数据用于测试无人机识别率㊂从图中可知,不同无人机训练数据散点图分布区域不同,测试数据同样,但部分测试数据存在于其他组训练数据中,故该部分数据为错误识别组㊂无人机遥控信号不含标准差且二维特征识别率表如表2所示,其中无人机总识别率为80%㊂表2㊀无人机遥控信号不含标准差二维特征识别率无人机型号无人机识别率/%无人机总识别率/%大疆精灵4pro 80.000司马航模x8hw 73.333HM100.000大疆悟2无人机73.333司马航模x25pro73.33380实验2:采用不含标准差且特征维数为三维的方式进行分类识别,其散点图㊁识别率如图4及表3所示㊂图4㊀无人机遥控信号不含标准差三维特征散点图表3㊀无人机遥控信号不含标准差三维特征识别率无人机型号无人机识别率/%无人机总识别率/%大疆精灵4pro 100.000司马航模x8hw 86.666HM93.333大疆悟2无人机100.000司马航模x25pro93.33394.666通过图4及表3可知,相对于二维而言,在同样不含有标准差时,三维识别效果更佳,识别率达到94.666%㊂实验3:采用标准差且特征降维维数为二维的方式进行分类识别,其散点图及识别率如图5及表4所示㊂从图和表可知,相对于不含有标准差的二维散点图,含有标准差性能更好,且识别率达到97.333%㊂图5㊀无人机遥控信号含标准差二维特征散点图表4㊀无人机遥控信号含标准差二维特征识别率无人机型号无人机识别率/%无人机总识别率/%大疆精灵4pro 93.333司马航模x8hw100.000HM 100.000大疆悟2无人机100.000司马航模x25pro93.33397.333实验4:采用标准差,特征降维维数为三维方式进行分类识别,散点图及识别率如图6和表5所示,其中含有标准差且三维特征时,其识别率与二维特征相同㊂图6㊀无人机遥控信号含标准差三维特征散点图表5㊀无人机遥控信号含标准差三维特征识别率无人机型号无人机识别率/%无人机总识别率/%大疆精灵4pro 93.333司马航模x8hw 100.000HM100.000大疆悟2无人机100.000司马航模x25pro93.33397.333为更进一步测试识别性能,在实测数据中叠加㊃147㊃第61卷蒋平,谢跃雷:一种民用小型无人机的射频指纹识别方法第6期高斯白噪声,具体方法及步骤如下:Step 1㊀对实测训练数据建立数据单元库及训练数据特征集㊂Step 2㊀对实测测试数据叠加高斯白噪声㊂Step 3㊀通过分形贝叶斯变点检测㊁RF -DNA 统计特征提取已加高斯白噪声后数据特征集㊂Step 4㊀Step 3中已加高斯白噪声后数据特征集与Step 1中训练数据特征集进行均值中心化,产生新特征集,取新特征集中已加高斯白噪声部分特征数据组作为测试特征集㊂Step 5㊀对Step 4所得测试特征集进行PCA 降维,其中为验证维数影响,选择一维㊁二维㊁三维㊁四维作为测试变量㊂Step 6㊀采用SVM 多分类器进行分类,绘出两种识别率曲线图,一种为含有标准差二维特征㊁含有标准差三维特征㊁不含有标准差二维特征㊁不含有标准差三维特征在不同信噪比下识别率对比图,命名为无人机遥控信号不同标准差及不同维数特征识别率图;另一种为含有标准差下一维㊁二维㊁三维㊁四维特征在不同信噪比的识别率对比图,命名为无人机遥控信号含有标准差下不同维数特征识别率图㊂图7为无人机遥控信号不同标准差及不同维数特征识别率图㊂从图中可知,含有标准差识别率优于不含标准差识别率,且三维总体高于二维㊂含有标准差时,信噪比大于15dB 时,其二维及三维识别率大于70%,而不含有标准差时,信噪比大于20dB时,其识别率大于70%㊂总体而言,随着高斯白噪声的增加,识别率逐渐下降,但因对其中心化处理㊁散点图较为集中等原因,其识别率在低于40%以下呈现低识别率随机起伏等混乱状态㊂图7㊀无人机遥控信号不同标准差及不同维数特征识别率图图8是在不同信噪比且含有标准差这一特征下不同维数识别率,总体来说,四维优于三维,三维优于二维及一维㊂在信噪比小于-5dB 时,各维识别率皆低于60%;在信噪比大于20dB 时,各维数识别率且大于90%,且四维最高㊂从识别曲线总体来看,维数越高其识别率更高㊂图8㊀无人机遥控信号含有标准差下不同维数特征识别率图通过以上四个实验得出采用RF -DNA 法对实测无人机遥控信号可以完成其型号的区分,其中三维识别率最高,为97.33%㊂为了更好地验证射频指纹方法的优点,取同一型号的两架大疆精灵4pro 无人机进行个体区分实验,并得出散点图及不同维数识别曲线图㊂由图9(a)可知,同一型号无人机散点图较为紧密,区分难度较大,对分类器有一定要求㊂由图9(b)可知,一维与二维曲线相同,但整体维数对识别率无较大影响,主要受分析数量所限从而无法凸显维数优势㊂总体来说随着信噪比增加,识别率逐渐升高,当信噪比在17dB 以上时各维数识别率达到80%,因此可证明射频指纹识别法可对无人机个体进行区分㊂(a)同一型号无人机遥控信号二维散点图(b)同一型号无人机遥控信号含有标准差下不同维数特征识别率图图9㊀同一型号无人机遥控信号散点图及识别率图㊃247㊃ 电讯技术㊀㊀㊀㊀2021年4 结束语本文针对无人机 黑飞 问题,采用RF-DNA方法完成了无人机具体型号及其个体的识别,可为无人机有效监管提供帮助㊂采用是否含有标准差以及不同维数作为测试条件,验证了在含有标准差且维数为四维时对无人机的型号区分效果最好㊂而通过对两架大疆精灵4pro无人机进行同一型号个体区分实验,得出RF-DNA能够区分同一型号无人机,但是无人机型号的区分抗噪性能高于同一型号的个体区分㊂此外,由于本实验目前只做了两架无人机的同一型号区分,后面应考虑增加更多同一型号无人机,以便于验证一定数量无人机同时存在对个体区别所带来的影响㊂并且,下一步应寻求更好的特征及分类方式从而更有效地对同一型号无人机进行个体区分,增加其实用价值㊂参考文献:[1]㊀赵时轮.无人机危害及恐怖行为反制对策研究[J].中国军转民,2019(6):15-20.[2]㊀李晓文.小型无人机在战术空中控制中的应用分析[J].飞航导弹,2020(5):49-53.[3]㊀张嘉,李润文,崔铠韬.浅析无人机管控手段及无人机无线电反制设备对民航空管运行的影响[J].中国无线电,2019(8):16-18.[4]㊀罗淮鸿,卢盈齐.国外反 低慢小 无人机能力现状与发展趋势[J].飞航导弹,2019(6):32-36. [5]㊀何小勇,韩兵,张笑语,等.一种基于无线电信号特征识别的无人机监测算法设计[J].中国无线电,2019(11):72-74.[6]㊀徐淑正,孙忆南,皇甫丽英,等.基于软件无线电平台的无人机入侵检测[J].实验室研究与探索,2018,37(12):64-67.[7]㊀刘丽.民用无人机跳频信号分析与识别技术研究[D].北京:北京邮电大学,2019.[8]㊀季澈,彭林宁,胡爱群,等.基于射频信号特征的Air-max设备指纹提取方法[J].数据采集与处理,2020,35(2):331-343.[9]㊀曾勇虎,陈翔,林云,等.射频指纹识别的研究现状及趋势[J].电波科学学报,2020,35(3):305-315. [10]㊀张宝林,吕军,李彤,等.一种改进的跳频信号参数估计方法[J].电讯技术,2018,58(11):1310-1316. [11]㊀耿健,杨威.一种同步组网跳频信号的盲分离方法[J].电讯技术,2018,58(12):1464-1469. [12]㊀魏兰兰.基于设备指纹的无线设备识别研究[D].北京:北京交通大学,2019.[13]㊀梁楠,邹志红.结合新型模糊支持向量机和证据理论的多传感器水质数据融合[J].电讯技术,2020,60(3):331-337.作者简介:蒋㊀平㊀男,1994年生于四川成都,2017年于成都工业学院获工学学士学位,现为桂林电子科技大学硕士研究生,主要研究方向为无人机信号侦测与识别㊁FPGA的数字系统设计㊂谢跃雷㊀男,1975年生于河北邯郸,1997年和2003年于桂林电子科技大学分别获工学学士学位和硕士学位,现为副教授㊁硕士生导师,主要研究方向为通信信号处理㊁阵列信号处理及信号处理的VLSI设计实现㊂㊃347㊃第61卷蒋平,谢跃雷:一种民用小型无人机的射频指纹识别方法第6期。
分子生物学英文文献6

Chapter19Detection and Quantitative Analysis of Small RNAs by PCR Seungil Ro and Wei YanAbstractIncreasing lines of evidence indicate that small non-coding RNAs including miRNAs,piRNAs,rasiRNAs, 21U endo-siRNAs,and snoRNAs are involved in many critical biological processes.Functional studies of these small RNAs require a simple,sensitive,and reliable method for detecting and quantifying levels of small RNAs.Here,we describe such a method that has been widely used for the validation of cloned small RNAs and also for quantitative analyses of small RNAs in both tissues and cells.Key words:Small RNAs,miRNAs,piRNAs,expression,PCR.1.IntroductionThe past several years have witnessed the surprising discovery ofnumerous non-coding small RNAs species encoded by genomesof virtually all species(1–6),which include microRNAs(miR-NAs)(7–10),piwi-interacting RNAs(piRNAs)(11–14),repeat-associated siRNAs(rasiRNAs)(15–18),21U endo-siRNAs(19),and small nucleolar RNAs(snoRNAs)(20).These small RNAsare involved in all aspects of cellular functions through direct orindirect interactions with genomic DNAs,RNAs,and proteins.Functional studies on these small RNAs are just beginning,andsome preliminaryfindings have suggested that they are involvedin regulating genome stability,epigenetic marking,transcription,translation,and protein functions(5,21–23).An easy and sensi-tive method to detect and quantify levels of these small RNAs inorgans or cells during developmental courses,or under different M.Sioud(ed.),RNA Therapeutics,Methods in Molecular Biology629,DOI10.1007/978-1-60761-657-3_19,©Springer Science+Business Media,LLC2010295296Ro and Yanphysiological and pathophysiological conditions,is essential forfunctional studies.Quantitative analyses of small RNAs appear tobe challenging because of their small sizes[∼20nucleotides(nt)for miRNAs,∼30nt for piRNAs,and60–200nt for snoRNAs].Northern blot analysis has been the standard method for detec-tion and quantitative analyses of RNAs.But it requires a relativelylarge amount of starting material(10–20μg of total RNA or>5μg of small RNA fraction).It is also a labor-intensive pro-cedure involving the use of polyacrylamide gel electrophoresis,electrotransfer,radioisotope-labeled probes,and autoradiogra-phy.We have developed a simple and reliable PCR-based methodfor detection and quantification of all types of small non-codingRNAs.In this method,small RNA fractions are isolated and polyAtails are added to the3 ends by polyadenylation(Fig.19.1).Small RNA cDNAs(srcDNAs)are then generated by reverseFig.19.1.Overview of small RNA complementary DNA(srcDNA)library construction forPCR or qPCR analysis.Small RNAs are polyadenylated using a polyA polymerase.ThepolyA-tailed RNAs are reverse-transcribed using a primer miRTQ containing oligo dTsflanked by an adaptor sequence.RNAs are removed by RNase H from the srcDNA.ThesrcDNA is ready for PCR or qPCR to be carried out using a small RNA-specific primer(srSP)and a universal reverse primer,RTQ-UNIr.Quantitative Analysis of Small RNAs297transcription using a primer consisting of adaptor sequences atthe5 end and polyT at the3 end(miRTQ).Using the srcD-NAs,non-quantitative or quantitative PCR can then be per-formed using a small RNA-specific primer and the RTQ-UNIrprimer.This method has been utilized by investigators in numer-ous studies(18,24–38).Two recent technologies,454sequenc-ing and microarray(39,40)for high-throughput analyses of miR-NAs and other small RNAs,also need an independent method forvalidation.454sequencing,the next-generation sequencing tech-nology,allows virtually exhaustive sequencing of all small RNAspecies within a small RNA library.However,each of the clonednovel small RNAs needs to be validated by examining its expres-sion in organs or in cells.Microarray assays of miRNAs have beenavailable but only known or bioinformatically predicted miR-NAs are covered.Similar to mRNA microarray analyses,the up-or down-regulation of miRNA levels under different conditionsneeds to be further validated using conventional Northern blotanalyses or PCR-based methods like the one that we are describ-ing here.2.Materials2.1.Isolation of Small RNAs, Polyadenylation,and Purification 1.mirVana miRNA Isolation Kit(Ambion).2.Phosphate-buffered saline(PBS)buffer.3.Poly(A)polymerase.4.mirVana Probe and Marker Kit(Ambion).2.2.Reverse Transcription,PCR, and Quantitative PCR 1.Superscript III First-Strand Synthesis System for RT-PCR(Invitrogen).2.miRTQ primers(Table19.1).3.AmpliTaq Gold PCR Master Mix for PCR.4.SYBR Green PCR Master Mix for qPCR.5.A miRNA-specific primer(e.g.,let-7a)and RTQ-UNIr(Table19.1).6.Agarose and100bp DNA ladder.3.Methods3.1.Isolation of Small RNAs 1.Harvest tissue(≤250mg)or cells in a1.7-mL tube with500μL of cold PBS.T a b l e 19.1O l i g o n u c l e o t i d e s u s e dN a m eS e q u e n c e (5 –3 )N o t eU s a g em i R T QC G A A T T C T A G A G C T C G A G G C A G G C G A C A T G G C T G G C T A G T T A A G C T T G G T A C C G A G C T A G T C C T T T T T T T T T T T T T T T T T T T T T T T T T V N ∗R N a s e f r e e ,H P L CR e v e r s e t r a n s c r i p t i o nR T Q -U N I r C G A A T T C T A G A G C T C G A G G C A G GR e g u l a r d e s a l t i n gP C R /q P C Rl e t -7a T G A G G T A G T A G G T T G T A T A G R e g u l a r d e s a l t i n gP C R /q P C R∗V =A ,C ,o r G ;N =A ,C ,G ,o r TQuantitative Analysis of Small RNAs299 2.Centrifuge at∼5,000rpm for2min at room temperature(RT).3.Remove PBS as much as possible.For cells,remove PBScarefully without breaking the pellet,leave∼100μL of PBS,and resuspend cells by tapping gently.4.Add300–600μL of lysis/binding buffer(10volumes pertissue mass)on ice.When you start with frozen tissue or cells,immediately add lysis/binding buffer(10volumes per tissue mass)on ice.5.Cut tissue into small pieces using scissors and grind it usinga homogenizer.For cells,skip this step.6.Vortex for40s to mix.7.Add one-tenth volume of miRNA homogenate additive onice and mix well by vortexing.8.Leave the mixture on ice for10min.For tissue,mix it every2min.9.Add an equal volume(330–660μL)of acid-phenol:chloroform.Be sure to withdraw from the bottom phase(the upper phase is an aqueous buffer).10.Mix thoroughly by inverting the tubes several times.11.Centrifuge at10,000rpm for5min at RT.12.Recover the aqueous phase carefully without disrupting thelower phase and transfer it to a fresh tube.13.Measure the volume using a scale(1g=∼1mL)andnote it.14.Add one-third volume of100%ethanol at RT to the recov-ered aqueous phase.15.Mix thoroughly by inverting the tubes several times.16.Transfer up to700μL of the mixture into afilter cartridgewithin a collection bel thefilter as total RNA.When you have>700μL of the mixture,apply it in suc-cessive application to the samefilter.17.Centrifuge at10,000rpm for15s at RT.18.Collect thefiltrate(theflow-through).Save the cartridgefor total RNA isolation(go to Step24).19.Add two-third volume of100%ethanol at RT to theflow-through.20.Mix thoroughly by inverting the tubes several times.21.Transfer up to700μL of the mixture into a newfilterbel thefilter as small RNA.When you have >700μL of thefiltrate mixture,apply it in successive appli-cation to the samefilter.300Ro and Yan22.Centrifuge at10,000rpm for15s at RT.23.Discard theflow-through and repeat until all of thefiltratemixture is passed through thefilter.Reuse the collectiontube for the following washing steps.24.Apply700μL of miRNA wash solution1(working solu-tion mixed with ethanol)to thefilter.25.Centrifuge at10,000rpm for15s at RT.26.Discard theflow-through.27.Apply500μL of miRNA wash solution2/3(working solu-tion mixed with ethanol)to thefilter.28.Centrifuge at10,000rpm for15s at RT.29.Discard theflow-through and repeat Step27.30.Centrifuge at12,000rpm for1min at RT.31.Transfer thefilter cartridge to a new collection tube.32.Apply100μL of pre-heated(95◦C)elution solution orRNase-free water to the center of thefilter and close thecap.Aliquot a desired amount of elution solution intoa1.7-mL tube and heat it on a heat block at95◦C for∼15min.Open the cap carefully because it might splashdue to pressure buildup.33.Leave thefilter tube alone for1min at RT.34.Centrifuge at12,000rpm for1min at RT.35.Measure total RNA and small RNA concentrations usingNanoDrop or another spectrophotometer.36.Store it at–80◦C until used.3.2.Polyadenylation1.Set up a reaction mixture with a total volume of50μL in a0.5-mL tube containing0.1–2μg of small RNAs,10μL of5×E-PAP buffer,5μL of25mM MnCl2,5μL of10mMATP,1μL(2U)of Escherichia coli poly(A)polymerase I,and RNase-free water(up to50μL).When you have a lowconcentration of small RNAs,increase the total volume;5×E-PAP buffer,25mM MnCl2,and10mM ATP should beincreased accordingly.2.Mix well and spin the tube briefly.3.Incubate for1h at37◦C.3.3.Purification 1.Add an equal volume(50μL)of acid-phenol:chloroformto the polyadenylation reaction mixture.When you have>50μL of the mixture,increase acid-phenol:chloroformaccordingly.2.Mix thoroughly by tapping the tube.Quantitative Analysis of Small RNAs3013.Centrifuge at10,000rpm for5min at RT.4.Recover the aqueous phase carefully without disrupting thelower phase and transfer it to a fresh tube.5.Add12volumes(600μL)of binding/washing buffer tothe aqueous phase.When you have>50μL of the aqueous phase,increase binding/washing buffer accordingly.6.Transfer up to460μL of the mixture into a purificationcartridge within a collection tube.7.Centrifuge at10,000rpm for15s at RT.8.Discard thefiltrate(theflow-through)and repeat until allof the mixture is passed through the cartridge.Reuse the collection tube.9.Apply300μL of binding/washing buffer to the cartridge.10.Centrifuge at12,000rpm for1min at RT.11.Transfer the cartridge to a new collection tube.12.Apply25μL of pre-heated(95◦C)elution solution to thecenter of thefilter and close the cap.Aliquot a desired amount of elution solution into a1.7-mL tube and heat it on a heat block at95◦C for∼15min.Open the cap care-fully because it might be splash due to pressure buildup.13.Let thefilter tube stand for1min at RT.14.Centrifuge at12,000rpm for1min at RT.15.Repeat Steps12–14with a second aliquot of25μL ofpre-heated(95◦C)elution solution.16.Measure polyadenylated(tailed)RNA concentration usingNanoDrop or another spectrophotometer.17.Store it at–80◦C until used.After polyadenylation,RNAconcentration should increase up to5–10times of the start-ing concentration.3.4.Reverse Transcription 1.Mix2μg of tailed RNAs,1μL(1μg)of miRTQ,andRNase-free water(up to21μL)in a PCR tube.2.Incubate for10min at65◦C and for5min at4◦C.3.Add1μL of10mM dNTP mix,1μL of RNaseOUT,4μLof10×RT buffer,4μL of0.1M DTT,8μL of25mM MgCl2,and1μL of SuperScript III reverse transcriptase to the mixture.When you have a low concentration of lig-ated RNAs,increase the total volume;10×RT buffer,0.1M DTT,and25mM MgCl2should be increased accordingly.4.Mix well and spin the tube briefly.5.Incubate for60min at50◦C and for5min at85◦C toinactivate the reaction.302Ro and Yan6.Add1μL of RNase H to the mixture.7.Incubate for20min at37◦C.8.Add60μL of nuclease-free water.3.5.PCR and qPCR 1.Set up a reaction mixture with a total volume of25μL ina PCR tube containing1μL of small RNA cDNAs(srcD-NAs),1μL(5pmol of a miRNA-specific primer(srSP),1μL(5pmol)of RTQ-UNIr,12.5μL of AmpliTaq GoldPCR Master Mix,and9.5μL of nuclease-free water.ForqPCR,use SYBR Green PCR Master Mix instead of Ampli-Taq Gold PCR Master Mix.2.Mix well and spin the tube briefly.3.Start PCR or qPCR with the conditions:95◦C for10minand then40cycles at95◦C for15s,at48◦C for30s and at60◦C for1min.4.Adjust annealing Tm according to the Tm of your primer5.Run2μL of the PCR or qPCR products along with a100bpDNA ladder on a2%agarose gel.∼PCR products should be∼120–200bp depending on the small RNA species(e.g.,∼120–130bp for miRNAs and piRNAs).4.Notes1.This PCR method can be used for quantitative PCR(qPCR)or semi-quantitative PCR(semi-qPCR)on small RNAs suchas miRNAs,piRNAs,snoRNAs,small interfering RNAs(siRNAs),transfer RNAs(tRNAs),and ribosomal RNAs(rRNAs)(18,24–38).2.Design miRNA-specific primers to contain only the“coresequence”since our cloning method uses two degeneratenucleotides(VN)at the3 end to make small RNA cDNAs(srcDNAs)(see let-7a,Table19.1).3.For qPCR analysis,two miRNAs and a piRNA were quan-titated using the SYBR Green PCR Master Mix(41).Cyclethreshold(Ct)is the cycle number at which thefluorescencesignal reaches the threshold level above the background.ACt value for each miRNA tested was automatically calculatedby setting the threshold level to be0.1–0.3with auto base-line.All Ct values depend on the abundance of target miR-NAs.For example,average Ct values for let-7isoforms rangefrom17to20when25ng of each srcDNA sample from themultiple tissues was used(see(41).Quantitative Analysis of Small RNAs3034.This method amplifies over a broad dynamic range up to10orders of magnitude and has excellent sensitivity capable ofdetecting as little as0.001ng of the srcDNA in qPCR assays.5.For qPCR,each small RNA-specific primer should be testedalong with a known control primer(e.g.,let-7a)for PCRefficiency.Good efficiencies range from90%to110%calcu-lated from slopes between–3.1and–3.6.6.On an agarose gel,mature miRNAs and precursor miRNAs(pre-miRNAs)can be differentiated by their size.PCR prod-ucts containing miRNAs will be∼120bp long in size whileproducts containing pre-miRNAs will be∼170bp long.However,our PCR method preferentially amplifies maturemiRNAs(see Results and Discussion in(41)).We testedour PCR method to quantify over100miRNAs,but neverdetected pre-miRNAs(18,29–31,38). AcknowledgmentsThe authors would like to thank Jonathan Cho for reading andediting the text.This work was supported by grants from theNational Institute of Health(HD048855and HD050281)toW.Y.References1.Ambros,V.(2004)The functions of animalmicroRNAs.Nature,431,350–355.2.Bartel,D.P.(2004)MicroRNAs:genomics,biogenesis,mechanism,and function.Cell, 116,281–297.3.Chang,T.C.and Mendell,J.T.(2007)Theroles of microRNAs in vertebrate physiol-ogy and human disease.Annu Rev Genomics Hum Genet.4.Kim,V.N.(2005)MicroRNA biogenesis:coordinated cropping and dicing.Nat Rev Mol Cell Biol,6,376–385.5.Kim,V.N.(2006)Small RNAs just gotbigger:Piwi-interacting RNAs(piRNAs) in mammalian testes.Genes Dev,20, 1993–1997.6.Kotaja,N.,Bhattacharyya,S.N.,Jaskiewicz,L.,Kimmins,S.,Parvinen,M.,Filipowicz, W.,and Sassone-Corsi,P.(2006)The chro-matoid body of male germ cells:similarity with processing bodies and presence of Dicer and microRNA pathway components.Proc Natl Acad Sci U S A,103,2647–2652.7.Aravin,A.A.,Lagos-Quintana,M.,Yalcin,A.,Zavolan,M.,Marks,D.,Snyder,B.,Gaaster-land,T.,Meyer,J.,and Tuschl,T.(2003) The small RNA profile during Drosophilamelanogaster development.Dev Cell,5, 337–350.8.Lee,R.C.and Ambros,V.(2001)An exten-sive class of small RNAs in Caenorhabditis ele-gans.Science,294,862–864.u,N.C.,Lim,L.P.,Weinstein, E.G.,and Bartel,D.P.(2001)An abundant class of tiny RNAs with probable regulatory roles in Caenorhabditis elegans.Science,294, 858–862.gos-Quintana,M.,Rauhut,R.,Lendeckel,W.,and Tuschl,T.(2001)Identification of novel genes coding for small expressed RNAs.Science,294,853–858.u,N.C.,Seto,A.G.,Kim,J.,Kuramochi-Miyagawa,S.,Nakano,T.,Bartel,D.P.,and Kingston,R.E.(2006)Characterization of the piRNA complex from rat testes.Science, 313,363–367.12.Grivna,S.T.,Beyret,E.,Wang,Z.,and Lin,H.(2006)A novel class of small RNAs inmouse spermatogenic cells.Genes Dev,20, 1709–1714.13.Girard, A.,Sachidanandam,R.,Hannon,G.J.,and Carmell,M.A.(2006)A germline-specific class of small RNAs binds mammalian Piwi proteins.Nature,442,199–202.304Ro and Yan14.Aravin,A.,Gaidatzis,D.,Pfeffer,S.,Lagos-Quintana,M.,Landgraf,P.,Iovino,N., Morris,P.,Brownstein,M.J.,Kuramochi-Miyagawa,S.,Nakano,T.,Chien,M.,Russo, J.J.,Ju,J.,Sheridan,R.,Sander,C.,Zavolan, M.,and Tuschl,T.(2006)A novel class of small RNAs bind to MILI protein in mouse testes.Nature,442,203–207.15.Watanabe,T.,Takeda, A.,Tsukiyama,T.,Mise,K.,Okuno,T.,Sasaki,H.,Minami, N.,and Imai,H.(2006)Identification and characterization of two novel classes of small RNAs in the mouse germline: retrotransposon-derived siRNAs in oocytes and germline small RNAs in testes.Genes Dev,20,1732–1743.16.Vagin,V.V.,Sigova,A.,Li,C.,Seitz,H.,Gvozdev,V.,and Zamore,P.D.(2006)A distinct small RNA pathway silences selfish genetic elements in the germline.Science, 313,320–324.17.Saito,K.,Nishida,K.M.,Mori,T.,Kawa-mura,Y.,Miyoshi,K.,Nagami,T.,Siomi,H.,and Siomi,M.C.(2006)Specific asso-ciation of Piwi with rasiRNAs derived from retrotransposon and heterochromatic regions in the Drosophila genome.Genes Dev,20, 2214–2222.18.Ro,S.,Song,R.,Park, C.,Zheng,H.,Sanders,K.M.,and Yan,W.(2007)Cloning and expression profiling of small RNAs expressed in the mouse ovary.RNA,13, 2366–2380.19.Ruby,J.G.,Jan,C.,Player,C.,Axtell,M.J.,Lee,W.,Nusbaum,C.,Ge,H.,and Bartel,D.P.(2006)Large-scale sequencing reveals21U-RNAs and additional microRNAs and endogenous siRNAs in C.elegans.Cell,127, 1193–1207.20.Terns,M.P.and Terns,R.M.(2002)Small nucleolar RNAs:versatile trans-acting molecules of ancient evolutionary origin.Gene Expr,10,17–39.21.Ouellet,D.L.,Perron,M.P.,Gobeil,L.A.,Plante,P.,and Provost,P.(2006)MicroR-NAs in gene regulation:when the smallest governs it all.J Biomed Biotechnol,2006, 69616.22.Maatouk,D.and Harfe,B.(2006)MicroR-NAs in development.ScientificWorldJournal, 6,1828–1840.23.Kim,V.N.and Nam,J.W.(2006)Genomics of microRNA.Trends Genet,22, 165–173.24.Bohnsack,M.T.,Kos,M.,and Tollervey,D.(2008)Quantitative analysis of snoRNAassociation with pre-ribosomes and release of snR30by Rok1helicase.EMBO Rep,9, 1230–1236.25.Hertel,J.,de Jong, D.,Marz,M.,Rose,D.,Tafer,H.,Tanzer, A.,Schierwater,B.,and Stadler,P.F.(2009)Non-codingRNA annotation of the genome of Tri-choplax adhaerens.Nucleic Acids Res,37, 1602–1615.26.Kim,M.,Patel,B.,Schroeder,K.E.,Raza,A.,and Dejong,J.(2008)Organization andtranscriptional output of a novel mRNA-like piRNA gene(mpiR)located on mouse chro-mosome10.RNA,14,1005–1011.27.Mishima,T.,Takizawa,T.,Luo,S.S.,Ishibashi,O.,Kawahigashi,Y.,Mizuguchi, Y.,Ishikawa,T.,Mori,M.,Kanda,T., and Goto,T.(2008)MicroRNA(miRNA) cloning analysis reveals sex differences in miRNA expression profiles between adult mouse testis and ovary.Reproduction,136, 811–822.28.Papaioannou,M.D.,Pitetti,J.L.,Ro,S.,Park, C.,Aubry, F.,Schaad,O.,Vejnar,C.E.,Kuhne, F.,Descombes,P.,Zdob-nov, E.M.,McManus,M.T.,Guillou, F., Harfe,B.D.,Yan,W.,Jegou,B.,and Nef, S.(2009)Sertoli cell Dicer is essential for spermatogenesis in mice.Dev Biol,326, 250–259.29.Ro,S.,Park,C.,Sanders,K.M.,McCarrey,J.R.,and Yan,W.(2007)Cloning and expres-sion profiling of testis-expressed microRNAs.Dev Biol,311,592–602.30.Ro,S.,Park,C.,Song,R.,Nguyen,D.,Jin,J.,Sanders,K.M.,McCarrey,J.R.,and Yan, W.(2007)Cloning and expression profiling of testis-expressed piRNA-like RNAs.RNA, 13,1693–1702.31.Ro,S.,Park,C.,Young,D.,Sanders,K.M.,and Yan,W.(2007)Tissue-dependent paired expression of miRNAs.Nucleic Acids Res, 35,5944–5953.32.Siebolts,U.,Varnholt,H.,Drebber,U.,Dienes,H.P.,Wickenhauser,C.,and Oden-thal,M.(2009)Tissues from routine pathol-ogy archives are suitable for microRNA anal-yses by quantitative PCR.J Clin Pathol,62, 84–88.33.Smits,G.,Mungall,A.J.,Griffiths-Jones,S.,Smith,P.,Beury,D.,Matthews,L.,Rogers, J.,Pask, A.J.,Shaw,G.,VandeBerg,J.L., McCarrey,J.R.,Renfree,M.B.,Reik,W.,and Dunham,I.(2008)Conservation of the H19 noncoding RNA and H19-IGF2imprint-ing mechanism in therians.Nat Genet,40, 971–976.34.Song,R.,Ro,S.,Michaels,J.D.,Park,C.,McCarrey,J.R.,and Yan,W.(2009)Many X-linked microRNAs escape meiotic sex chromosome inactivation.Nat Genet,41, 488–493.Quantitative Analysis of Small RNAs30535.Wang,W.X.,Wilfred,B.R.,Baldwin,D.A.,Isett,R.B.,Ren,N.,Stromberg, A.,and Nelson,P.T.(2008)Focus on RNA iso-lation:obtaining RNA for microRNA (miRNA)expression profiling analyses of neural tissue.Biochim Biophys Acta,1779, 749–757.36.Wu,F.,Zikusoka,M.,Trindade,A.,Das-sopoulos,T.,Harris,M.L.,Bayless,T.M., Brant,S.R.,Chakravarti,S.,and Kwon, J.H.(2008)MicroRNAs are differen-tially expressed in ulcerative colitis and alter expression of macrophage inflam-matory peptide-2alpha.Gastroenterology, 135(1624–1635),e24.37.Wu,H.,Neilson,J.R.,Kumar,P.,Manocha,M.,Shankar,P.,Sharp,P.A.,and Manjunath, N.(2007)miRNA profiling of naive,effec-tor and memory CD8T cells.PLoS ONE,2, e1020.38.Yan,W.,Morozumi,K.,Zhang,J.,Ro,S.,Park, C.,and Yanagimachi,R.(2008) Birth of mice after intracytoplasmic injec-tion of single purified sperm nuclei and detection of messenger RNAs and microR-NAs in the sperm nuclei.Biol Reprod,78, 896–902.39.Guryev,V.and Cuppen,E.(2009)Next-generation sequencing approaches in genetic rodent model systems to study func-tional effects of human genetic variation.FEBS Lett.40.Li,W.and Ruan,K.(2009)MicroRNAdetection by microarray.Anal Bioanal Chem.41.Ro,S.,Park,C.,Jin,JL.,Sanders,KM.,andYan,W.(2006)A PCR-based method for detection and quantification of small RNAs.Biochem and Biophys Res Commun,351, 756–763.。
外文资料翻译---具有高灵敏度的甲醛气体传感器的制备及其气敏特性
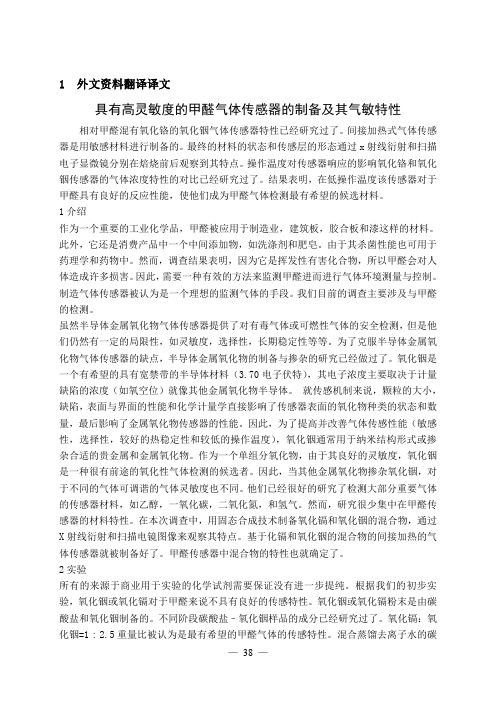
1 外文资料翻译译文具有高灵敏度的甲醛气体传感器的制备及其气敏特性相对甲醛混有氧化铬的氧化铟气体传感器特性已经研究过了。
间接加热式气体传感器是用敏感材料进行制备的。
最终的材料的状态和传感层的形态通过x射线衍射和扫描电子显微镜分别在焙烧前后观察到其特点。
操作温度对传感器响应的影响氧化铬和氧化铟传感器的气体浓度特性的对比已经研究过了。
结果表明,在低操作温度该传感器对于甲醛具有良好的反应性能,使他们成为甲醛气体检测最有希望的候选材料。
1介绍作为一个重要的工业化学品,甲醛被应用于制造业,建筑板,胶合板和漆这样的材料。
此外,它还是消费产品中一个中间添加物,如洗涤剂和肥皂。
由于其杀菌性能也可用于药理学和药物中。
然而,调查结果表明,因为它是挥发性有害化合物,所以甲醛会对人体造成许多损害。
因此,需要一种有效的方法来监测甲醛进而进行气体环境测量与控制。
制造气体传感器被认为是一个理想的监测气体的手段。
我们目前的调查主要涉及与甲醛的检测。
虽然半导体金属氧化物气体传感器提供了对有毒气体或可燃性气体的安全检测,但是他们仍然有一定的局限性,如灵敏度,选择性,长期稳定性等等。
为了克服半导体金属氧化物气体传感器的缺点,半导体金属氧化物的制备与掺杂的研究已经做过了。
氧化铟是一个有希望的具有宽禁带的半导体材料(3.70电子伏特),其电子浓度主要取决于计量缺陷的浓度(如氧空位)就像其他金属氧化物半导体。
就传感机制来说,颗粒的大小,缺陷,表面与界面的性能和化学计量学直接影响了传感器表面的氧化物种类的状态和数量,最后影响了金属氧化物传感器的性能。
因此,为了提高并改善气体传感性能(敏感性,选择性,较好的热稳定性和较低的操作温度),氧化铟通常用于纳米结构形式或掺杂合适的贵金属和金属氧化物。
作为一个单组分氧化物,由于其良好的灵敏度,氧化铟是一种很有前途的氧化性气体检测的候选者。
因此,当其他金属氧化物掺杂氧化铟,对于不同的气体可调谐的气体灵敏度也不同。
Back_Focal_Plane
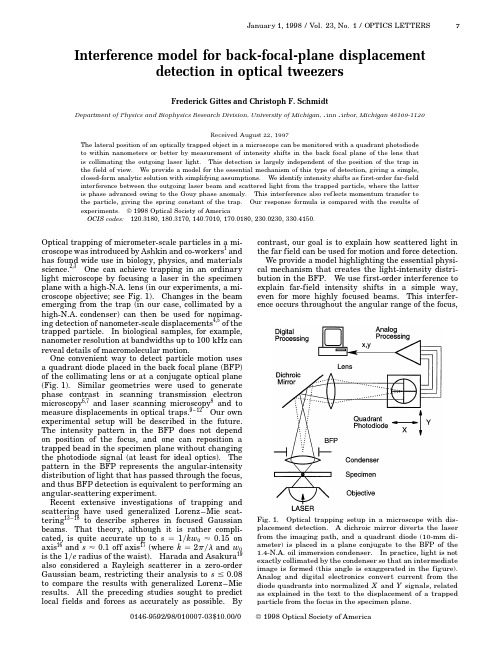
January1,1998/Vol.23,No.1/OPTICS LETTERS7 Interference model for back-focal-plane displacementdetection in optical tweezersFrederick Gittes and Christoph F.SchmidtDepartment of Physics and Biophysics Research Division,University of Michigan,Ann Arbor,Michigan48109-1120Received August22,1997The lateral position of an optically trapped object in a microscope can be monitored with a quadrant photodiodeto within nanometers or better by measurement of intensity shifts in the back focal plane of the lens thatis collimating the outgoing laser light.This detection is largely independent of the position of the trap inthe field of view.We provide a model for the essential mechanism of this type of detection,giving a simple,closed-form analytic solution with simplifying assumptions.We identify intensity shifts as first-order far-fieldinterference between the outgoing laser beam and scattered light from the trapped particle,where the latteris phase advanced owing to the Gouy phase anomaly.This interference also ref lects momentum transfer tothe particle,giving the spring constant of the trap.Our response formula is compared with the results of experiments.©1998Optical Society of AmericaOCIS codes:120.3180,180.3170,140.7010,170.0180,230.0230,330.4150.Optical trapping of micrometer-scale particles in a mi-croscope was introduced by Ashkin and co-workers1andhas found wide use in biology,physics,and materialsscience.2,3One can achieve trapping in an ordinary light microscope by focusing a laser in the specimenplane with a high-N.A.lens(in our experiments,a mi-croscope objective;see Fig.1).Changes in the beam emerging from the trap(in our case,collimated by ahigh-N.A.condenser)can then be used for nonimag-ing detection of nanometer-scale displacements4,5of the trapped particle.In biological samples,for example,nanometer resolution at bandwidths up to100kHz canreveal details of macromolecular motion.One convenient way to detect particle motion usesa quadrant diode placed in the back focal plane(BFP)of the collimating lens or at a conjugate optical plane (Fig.1).Similar geometries were used to generatephase contrast in scanning transmission electronmicroscopy6,7and laser scanning microscopy8and to measure displacements in optical traps.9–12Our ownexperimental setup will be described in the future.The intensity pattern in the BFP does not depend on position of the focus,and one can reposition atrapped bead in the specimen plane without changingthe photodiode signal(at least for ideal optics).The pattern in the BFP represents the angular-intensitydistribution of light that has passed through the focus,and thus BFP detection is equivalent to performing an angular-scattering experiment.Recent extensive investigations of trapping andscattering have used generalized Lorenz–Mie scat-tering13–18to describe spheres in focused Gaussianbeams.That theory,although it is rather compli-cated,is quite accurate up to s1͞kw0ഠ0.15on axis16and sഠ0.1off axis17(where k2p͞l and w0is the1͞e radius of the waist).Harada and Asakura19also considered a Rayleigh scatterer in a zero-order Gaussian beam,restricting their analysis to s#0.08 to compare the results with generalized Lorenz–Mie results.All the preceding studies sought to predict local fields and forces as accurately as possible.By contrast,our goal is to explain how scattered light in the far field can be used for motion and force detection. We provide a model highlighting the essential physi-cal mechanism that creates the light-intensity distri-bution in the BFP.We use first-order interference to explain far-field intensity shifts in a simple way, even for more highly focused beams.This interfer-ence occurs throughout the angular range of thefocus, Fig.1.Optical trapping setup in a microscope with dis-placement detection.A dichroic mirror diverts the laser from the imaging path,and a quadrant diode(10-mm di-ameter)is placed in a plane conjugate to the BFP of the 1.4-N.A.oil immersion condenser.In practice,light is not exactly collimated by the condenser so that an intermediate image is formed(this angle is exaggerated in the figure). Analog and digital electronics convert current from the diode quadrants into normalized X and Y signals,related as explained in the text to the displacement of a trapped particle from the focus in the specimen plane.0146-9592/98/010007-03$10.00/0©1998Optical Society of America8OPTICS LETTERS/Vol.23,No.1/January1,1998 unlike in more-familiar plane-wave scattering prob-lems.13The momentum transfer implicit in the an-gular shift of intensity also allows us to estimate the lateral constant for a trapped particle.To emphasize the interference mechanism,we simplify the actual ex-perimental situation by considering Rayleigh scatter-ing within a zero-order Gaussian beam.The latter is only approximate for our focus,which has sഠ0.19, whereas a zero-order Gaussian beam has been shown even at s0.1to contain an average error of4.4%,14 and the Rayleigh approximation is not highly accurate for spheres as large as ours͑d0.5m m͒.Our cal-culated detector response profile is nevertheless very similar to the experimental response.Light emerging from the trapping focus at anglesu and f(Fig.2)will be detected in the BFP at a ra-dius f sin u(by the sine condition20),where f is the focal length of the lens.We consider angular-intensity changes caused by first-order interference for a given lateral displacement of a bead away from the focus in the specimen plane.Our approximations are as fol-lows:(1)We are concerned with small beads,andso we assume a Rayleigh scatterer(a delta-function polarizability),but we use the uniform-field polariz-ability(at optical frequencies)that corresponds to our actual sphere volume[Eq.(3),below].(2)The focus is described in a paraxial scalar Gaussian beam approxi-mation.21In our experiment the focus is roughly30±in angular radius;the input has w inഠ1.0mm,with the objective f2.1mm.A small-angle approxima-tion will not correctly predict axial trapping forces,but we are concerned with lateral displacement detection and trapping force.We also assume that the trapped particles lie in the focal plane;in reality there is some axial displacement.Defining r0to be at the focus,we observe diverging light at an angle u from the optical axis. The outgoing unscattered electric field21isE͑r͒ഠ2ikw0I1/2totr͑pe s c s1/2exp͑ikr2k2w20u2͞4͒.(1)A factor exp͑2i v t͒is implicit.Here k2p n s͞l0(in our experiment l01.064m m);e s,n s,and c s are the permittivity,the refractive index,and the speed of light in the solvent,respectively;w0is the1͞e radius of the focus,and I tot is the total power in the beam.We use the SI system.The factor2i in Eq.(1)represents the phase anomaly of the focus(the Gouy phase jump)20 and introduces an important90±phase shift between scattered and unscattered light in the far field.A small spherical particle of diameter d2a is located at r s in the focal plane,taken for simplicity to be a lateral displacement by x.The unscattered field at this point isE͑r s͒E͑x͒2I1/2totw0͑pe s c s͒1/2exp͑2x2͞w20͒.(2)The particle will have an induced dipole moment p4pe s a E.For an index of refraction n rn͞n s relative to the solvent,the uniform-field susceptibility is22aa3n2r21n2r120.0074d3,(3)assuming that n1.45for silica beads and n s1.33in aqueous solution(at l01.064m m).In a Rayleigh approximation the scattered field at large r isE0͑r͒ഠk2arE͑x͒exp͑ik j r2r s j͒,ഠk2arE͑x͒exp͓ik͑r2x sin u cos f͔͒(4)at observation angle u and f(f0along the x axis).We consider only first-order interference,which is justified since intensity modulation owing to the bead is small throughout the BFP.Discarding an j E0j2yields the average change in intensity I:d Ie s c s2͑j E1E0j22j E j2͒ഠe s c s Re͑EE0ء͒.(5)Substituting expressions(1),(2),and(4)into Eq.(5) gives usd I͑x͒I tot2k3ap r2exp͑2x2͞w20͒3sin͑kx sin u cos f͒exp͑2k2w20u2͞4͒.(6) Equation(6)describes the angular-interference pat-tern caused by a particle displaced by x from the optical axis in the focal plane,observed in a direction͑u,f͒.A split diode monitoring the BFP of the collimating lens(Fig.1)is oriented for detection along the6x axis; intensity changes on the͑1͒and͑2͒halves are equal and opposite.Integrating Eq.(6)over angles u and f with2p͞2,f,p͞2and with sin uഠ0givesI12I2I11I2ഠ16ppk aw0G͑x͞w0͒,G͑u͒exp͑22u2͒Z uexp͑y2͒d y.(7)Expression(7)predicts absolute detector response. For x,,w0,the response is proportional to d3͞w30, showing a sensitive dependence on particle and focus size.G͑u͒is calculable with Dawson’s inte-gral,23and in Fig.3expression(7)is compared with the experimental data.In Fig.3we apply expres-sion(7)(dashed curve),using thenominal sphere sizeFig.2.Focus geometry as discussed in the text.A refrac-tive particle,located in the focal plane of a Gaussian beam, is displaced laterally by x.Interference of scattered and unscattered light is considered at a large distance j r j and is observed in the BFP at a radius f sin u(the collimating lens,of focal length f,obeys the sine condition).The an-gular radius of the Gaussian focus(ϳ30±)is exaggerated.January1,1998/Vol.23,No.1/OPTICS LETTERS9Fig.3.Experimental calibration data compared with model response.Solid curve,a silica bead fixed upon a cover slip was moved by a piezoelectric stage through the sensitive range of the detector.Dashed curve,expres-sion(7)with no adjustable parameters besides the nominal sphere size͑0.5m m͒and a focus size͑w0ഠ0.53m m͒estimated from the input beam width.The function G͑x͞w0͒in expression(7)has a slope of w021at x0andextrema G͑x͞w0͒60.334at x60.552w0.͑d0.5m m͒and the focus size estimated roughly21 from the input Gaussian beam width͑w inഠ1.0mm͒and obtain kw0ഠ2f͞w in,implying that w0ഠ0.53m m. This ab initio model response,with no adjustable parameters besides w0and d,is quite similar to the measured response.The closeness of the agreement may perhaps be partly fortuitous,given the strong dependence of expression(7)on w20.The d3dependence of the linear response in ex-pression(7)is intrinsic for detection based on small-particle scattering.Our model works for spheres with d0.5m mഠl͞2.For beads much larger than l,ray optics applies and l drops out of the problem.Then d is the only length scale,and the response can depend only on x͞d;consequently the large-sphere linear re-sponse must decrease as1͞d.Our model provides a simple picture not only of detection but also of lateral trapping.The rate of light momentum transfer implied by the interference modulation,Eq.(6),can be shown to equal,as it must,the lateral trapping force of the laser focus. The Minkowski form of the stress tensor22for the momentum f lux of outgoing light has T rrI͞c s. Using I from Eq.(6)and integrating the x projection ͑T rr sin u cos f͒over u and f in the paraxial case gives the spring constant for the trapped particle:F x͞xഠ16a I totc s w40,(8)which agrees with the transverse gradient force F gpe s a=j E j2evaluated at the waist.Thus one can also use BFP detection to track directly the time-dependent force being exerted on the particle(which was done in a counterpropagating-beam trap11).In the past,laser trapping of spheres was mod-eled quantitatively for particles large enough for a geometric-optics treatment.24For smaller particles, for which ray optics is inappropriate,intuitive un-derstanding of trapping and detection has been rela-tively inaccessible,although rigorous electromagnetic expansions describing spheres near foci have been pre-sented and used.14–18Here we have found that a simple model based on pure interference throughout the numerical aperture of the focus evidently captures the physical mechanism of lateral trapping and detec-tion for smaller particles.This study was supported in part by the Whitaker Foundation,the National Science Foundation(grant BIR95-12699),and donors to the Petroleum Research Fund,which is administered by the American Chemical Society.We thank Winfield Hill and the Rowland Institute for Science for generous technical support. References1.A.Ashkin,J.M.Dziedzic,J.C.Bjorkholm,and S.Chu,Opt.Lett.11,288(1986).2.A.Ashkin,A94,4853(1997).3.K.Svoboda and S.M.Block,Annu.Rev.Biophys.Biomol.Struct.23,247(1994).4.W.Denk and W.W.Webb,Appl.Opt.29,2382(1990).5.K.Svoboda,C.F.Schmidt,B.J.Schnapp,and S.M.Block,Nature(London)365,721(1993).6.N.H.Dekkers and H.de Lang,Philips Tech.Rev.37,1(1977).7.N.H.Dekkers and H.de Lang,Optik41,452(1974).8.T.Wilson,Optik80,167(1988).9.L.P.Ghislain,N.A.Switz,and W.W.Webb,Rev.Sci.Instrum.65,2762(1994).10.L.P.Ghislain and W.W.Webb,Opt.Lett.18,1678(1993).11.S.B.Smith,Y.J.Cui,and C.Bustamante,Science271,795(1996).12.K.Visscher,S.P.Gross,and S.M.Block,IEEE J.Select.Topics Quantum Electron.2,1066(1996).13.H.C.van de Hulst,Light Scattering by Small Particles(Wiley,New Y ork,1957).14.J.P.Barton and D.R.Alexander,J.Appl.Phys.66,2800(1989).15.J.P.Barton,D.R.Alexander,and S.A.Schaub,J.Appl.Phys.66,4594(1989).16.J.A.Lock and G.Gouesbet,J.Opt.Soc.Am.A11,2503(1994).17.G.Gouesbet and J.A.Lock,J.Opt.Soc.Am.A11,2516(1994).18.K.F.Ren,G.Gr´e han,and G.Gouesbet,Appl.Opt.35,2702(1996).19.Y.Harada and T.Asakura,mun.124,529(1996).20.M.Born and E.Wolf,Principles of Optics,6th ed.(Pergamon,London,1989).21.A.E.Siegman,Lasers(University Science,Mill Valley,Calif.,1986).22.J. D.Jackson,Classical Electrodynamics,2nd ed.(Wiley,New Y ork,1975).23.W.H.Press,B.P.Flannery,S.A.Teukolsky,and W.T.Vetterling,Numerical Recipes in C,2nd ed.(Cambridge U.Press,Cambridge,1992).24.R.C.Gauthier and S.W allace,J.Opt.Soc.Am.B12,1680(1995).。
绝缘阻抗测试,维护超级对撞机的正常运转
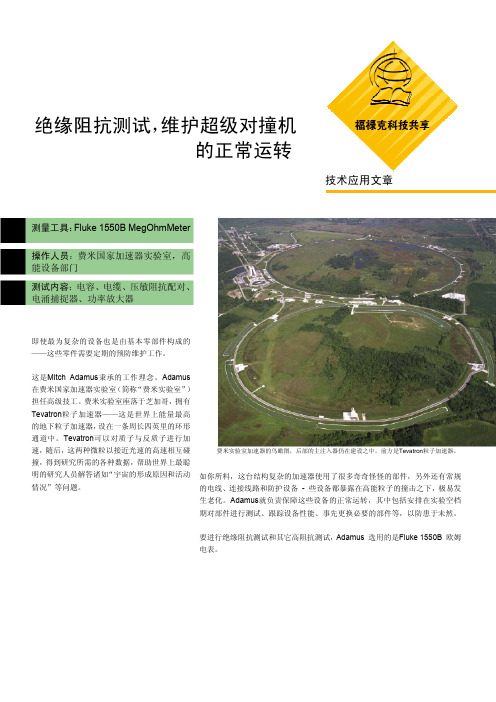
绝缘阻抗测试,维护超级对撞机的正常运转技术应用文章测量工具:Fluke 1550B MegOhmMeter操作人员:费米国家加速器实验室,高能设备部门测试内容:电容、电缆、压敏阻抗配对、电涌捕捉器、功率放大器即使最为复杂的设备也是由基本零部件构成的——这些零件需要定期的预防维护工作。
这是Mitch Adamus秉承的工作理念。
Adamus在费米国家加速器实验室(简称“费米实验室”)担任高级技工。
费米实验室座落于芝加哥,拥有Tevatron粒子加速器——这是世界上能量最高的地下粒子加速器,设在一条周长四英里的环形通道中。
Tevatron可以对质子与反质子进行加速,随后,这两种微粒以接近光速的高速相互碰撞,得到研究所需的各种数据,帮助世界上最聪明的研究人员解答诸如“宇宙的形成原因和活动情况”等问题。
费米实验室加速器的鸟瞰图,后部的主注入器仍在建设之中,前方是Tevatron粒子加速器。
如你所料,这台结构复杂的加速器使用了很多奇奇怪怪的部件,另外还有常规的电线、连接线路和防护设备 - 些设备都暴露在高能粒子的撞击之下,极易发生老化。
Adamus就负责保障这些设备的正常运转,其中包括安排在实验空档期对部件进行测试、跟踪设备性能、事先更换必要的部件等,以防患于未然。
要进行绝缘阻抗测试和其它高阻抗测试,Adamus 选用的是Fluke 1550B 欧姆电表。
标准测试电缆测试要确保各种系统中大型同轴电缆和高压电缆的性能良好,技术人员使用Hi -pot在5 kV电压下进行测试。
“高压电缆是典型的故障点,”Adamus说道,“一旦发生问题,电压迅速下降。
”问题的根源通常显而易见——由于周边环境恶劣,这些电缆常常在发生老化之前就直接报废了。
“只要如果能通过5 kV的测试,我们就认为该电缆状态良好,”Adamus 介绍说,“如果5 kV 测试不过,说明存在问题。
”测试方法使用Hi-pot对电缆进行测试,费米实验室公司的技术人员将1550B 设置为5 kV。
Confocal 介绍
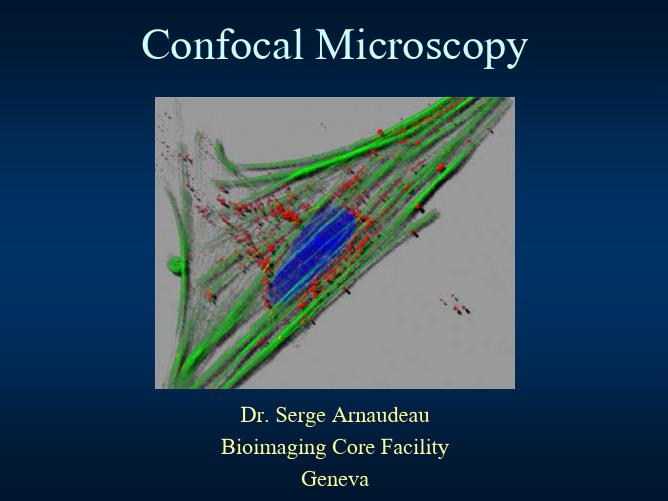
Dynodes
Side on design
Gain varies with the voltage across the dynodes and the total number of dynodes With typically 9 dynodes, gain of 4x106 can be achieved
Confocal microscope principle
x pinhole Light source detector Transmissive design Sample objective (object plane) y pinhole
objective
• “conjugate focal planes” • illumination and detection of the same focal point • need to displace the sample in x and y to construct an image
A section of mouse intestine imaged with both confocal and non-confocal microscopy
How big is a Laser Scanning Confocal Microscope ?
Laser module 405, 458, 477, 488, 514, 561, 633 nm Scanning head
System electronic rack
LASER
Light Amplification by Stimulated Emission of Radiation
• High intensity • Spatial and temporal coherence • Monochromatic • Focused
浅析近红外技术在进口塑料粒子海关查验中的应用
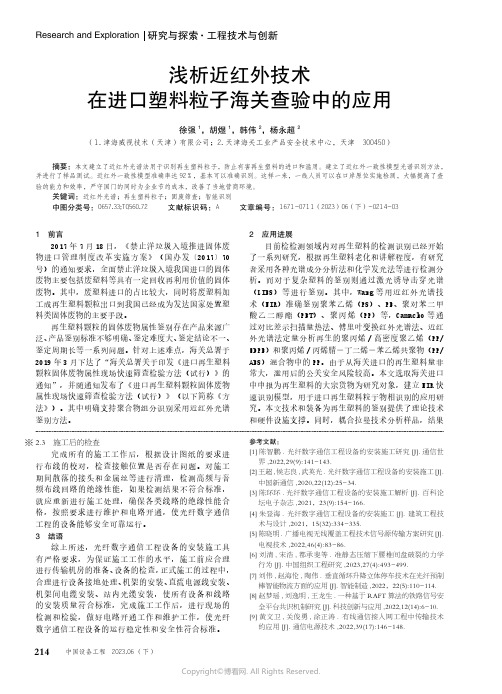
浅析近红外技术 在进口塑料粒子海关查验中的应用
徐强 1,胡煜 1,韩伟 2,杨永超 2 (1. 津海威视技术(天津)有限公司;2. 天津海关工业产品安全技术中心,天津 300450)
摘要:本文建立了近红外光谱法用于识别再生塑料粒子,防止有害再生塑料的进口和滥用。建立了近红外一致性模型光谱识别方法, 并进行了样品测试。近红外一致性模型准确率达 92%,基本可以准确识别。这样一来,一线人员可以在口岸原位实施检测,大幅提高了查 验的能力和效率,严守国门的同时为企业节约成本,改善了当地营商环境。
炼铁高炉机械设备的技术性分析
李虎,王建新 (山东省冶金设计院股份有限公司,山东 济南 250101)
摘要:高炉的设备是影响炼铁生产的一个关键因素,本文就高炉设备的各个方面进行了详细的论述,其中包括高炉的进料设备技术、 高炉设备技术等,以期为冶金行业的发展提供有力的支撑。
关键词:炼铁高炉;机械设备;技术性分析 中图分类号:TF54 文献标识码:A 文章编号:1671-0711(2023)06(下)-0216-03
2 应用进展 目前检检测领域内对再生塑料的检测识别已经开始
了一系列研究,根据再生塑料老化和讲解程度,有研究 者采用各种光谱成分分析法和化学发光法等进行检测分 析。而对于复杂塑料的鉴别则通过激光诱导击穿光谱 (LIBS)等进行鉴别。其中,Wang 等用近红外光谱技 术(NIR)准确鉴别聚苯乙烯(PS)、PE、聚对苯二甲 酸 乙 二 醇 酯(PET)、 聚 丙 烯(PP) 等,Camacho 等 通 过对比差示扫描量热法、傅里叶变换红外光谱法、近红 外光谱法定量分析再生的聚丙烯 / 高密度聚乙烯(PP/ HDPE)和聚丙烯 / 丙烯腈-丁二烯-苯乙烯共聚物(PP/ ABS)混合物中的 PP。由于从海关进口的再生塑料量非 常大,滥用后的公关安全风险较高。本文选取海关进口 中申报为再生塑料的大宗货物为研究对象,建立 NIR 快 速识别模型,用于进口再生塑料粒子物相识别的应用研 究。本文技术和装备为再生塑料的鉴别提供了理论技术 和硬件设施支撑。同时,耦合拉曼技术分析样品,结果
ICP-AES法测定石灰石中二氧化硅量
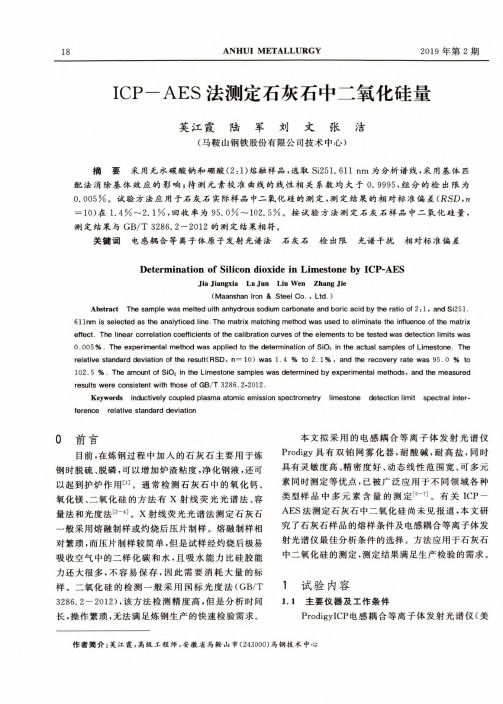
ICP-AES法测定石灰石中二氧化硅量荚江霞陆军刘文张洁(马鞍山钢铁股份有限公司技术中心)摘要采用无水碳酸钠和硼酸(2:1)熔融样品,选取Si251.611nm为分析谱线,采用基体匹配法消除基体效应的影响;待测元素校准曲线的线性相关系数均大于0.9995,组分的检出限为0.005%。
试验方法应用于石灰石实际样品中二氧化硅的测定,测定结果的相对标准偏差(RSD,n =10)在1.4%〜2.1%,回收率为95.0%〜102.5%。
按试验方法测定石灰石样品中二氧化硅量,测定结果与GB/T3286.2-2012的测定结果相符。
关键词电感耦合等离子体原子发射光谱法石灰石检出限光谱干扰相对标准偏差Determination of Silicon dioxide in Limestone by ICP-AESJia Jiangxia Lu Jun Liu Wen Zhang Jie(Maanshan Iron&Steel Co.,Ltd.)Abstract The sample was melted uith an h ydrous sodium carbonate and boric acid by the ratio of2:1,and Si251. 611nm is selected as the analyticed line.The matrix matching method was used to eliminate the influence of the matrix effect.The linear correlation coefficients of the calibration curves of the elements to be tested was detection limits was 0.005%.The experimental method was applied to the determination of SiO2in the actual samples of Limestone.The relative standard deviation of the result(RSD,n=10)was1.4%to2.1%,and the recovery rate was95.0%to 102.5%.The amount of SiO2in the Limestone samples was determined by experimental methods,and the measured results were consistent with those of GB/T3286.2-2012.Keywords inductively coupled plasma atomic emission spectrometry limestone detection limit spectral interference relative standard deviation0前言目前,在炼钢过程中加入的石灰石主要用于炼钢时脱硫、脱磷,可以增加炉渣粘度,净化钢液,还可以起到护炉作用⑴。
DETECTION DEVICE AND DETECTION METHOD FOR INTERMOL
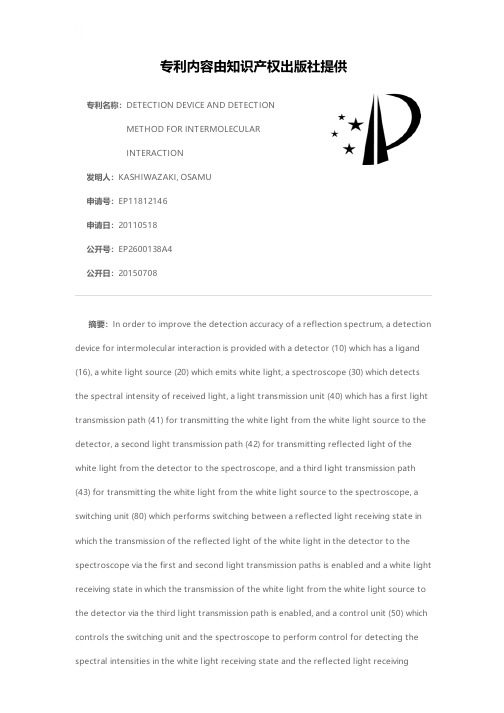
专利名称:DETECTION DEVICE AND DETECTION METHOD FOR INTERMOLECULARINTERACTION发明人:KASHIWAZAKI, OSAMU申请号:EP11812146申请日:20110518公开号:EP2600138A4公开日:20150708专利内容由知识产权出版社提供摘要:In order to improve the detection accuracy of a reflection spectrum, a detection device for intermolecular interaction is provided with a detector (10) which has a ligand (16), a white light source (20) which emits white light, a spectroscope (30) which detects the spectral intensity of received light, a light transmission unit (40) which has a first light transmission path (41) for transmitting the white light from the white light source to the detector, a second light transmission path (42) for transmitting reflected light of the white light from the detector to the spectroscope, and a third light transmission path (43) for transmitting the white light from the white light source to the spectroscope, a switching unit (80) which performs switching between a reflected light receiving state in which the transmission of the reflected light of the white light in the detector to the spectroscope via the first and second light transmission paths is enabled and a white light receiving state in which the transmission of the white light from the white light source to the detector via the third light transmission path is enabled, and a control unit (50) which controls the switching unit and the spectroscope to perform control for detecting the spectral intensities in the white light receiving state and the reflected light receivingstate, respectively.申请人:KONICA MINOLTA ADVANCED LAYERS, INC.更多信息请下载全文后查看。
基于高精度光学腔测量气体中痕微量NO_(2)的方法
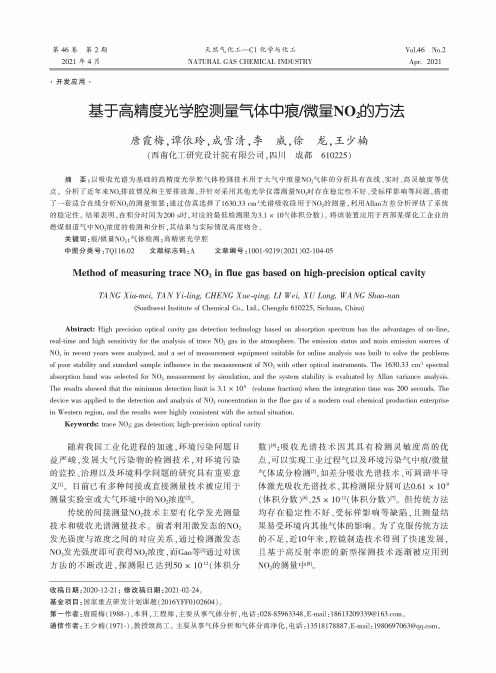
第46卷第2期 2021年4月天然气化工一C1化学与化工NATURAL GAS CHEMICAL INDUSTRYVol.46 No.2Apr. 2021•开发应用•基于高精度光学腔测量气体中痕/微量NO2的方法唐霞梅,谭依玲,成雪清,李威,徐龙,王少楠(西南化工研究设计院有限公司,四川成都610225)摘要:以吸收光谱为基础的高精度光学腔气体检测技术用于大气中痕量NO。
气体的分析具有在线、实时、高灵敏度等优 点。
分析了近年来NOx排放情况和主要排放源,并针对采用其他光学仪器测量NO2时存在稳定性不好、受标样影响等问题,搭建 了一套适合在线分析N〇2的测量装置;通过仿真选择了 1630.33 cm-1光谱吸收段用于N〇2的测量,利用Allan方差分析评估了系统 的稳定性。
结果表明,在积分时间为200 s时,对应的最低检测限为3.1 x 10鄄9(体积分数)。
将该装置应用于西部某煤化工企业的 燃煤烟道气中N〇2浓度的检测和分析,其结果与实际情况高度吻合。
关键词:痕/微量N〇2;气体检测;高精密光学腔中图分类号:TQ116.02 文献标志码:A 文章编号:1001-9219(2021)02-104-05Method of measuring trace N〇2 in flue gas based on high-precision optical cavity TANG Xia-mei, TAN Yi-ling, CHENG Xue-qing, LI Wei, XU Long, WANG Shao-nan(Southwest Institute of Chemical Co., Ltd., Chengdu 610225, Sichuan, China)Abstract: High precision optical cavity gas detection technology based on absorption spectrum has the advantages of on-line, real-time and high sensitivity for the analysis of trace N〇2 gas in the atmosphere. The emission status and main emission sources of N〇x in recent years were analyzed, and a set of measurement equipment suitable for online analysis was built to solve the problems of poor stability and standard sample influence in the measurement of N〇2 with other optical instruments. The 1630.33 cm-1spectral absorption band was selected for N〇2 measurement by simulation, and the system stability is evaluated by Allan variance analysis. The results showed that the minimum detection limit is 3.1 x 109(volume fraction) when the integration time was 200 seconds. The device was applied to the detection and analysis of N〇2 concentration in the flue gas of a modern coal chemical production enterprise in Western region, and the results were highly consistent with the actual situation.Keywords: trace N〇2; gas detection; high-precision optical cavity随着我国工业化进程的加速,环境污染问题日 益严峻,发展大气污染物的检测技术,对环境污染 的监控、治理以及环境科学问题的研究具有重要意 义[1]。
- 1、下载文档前请自行甄别文档内容的完整性,平台不提供额外的编辑、内容补充、找答案等附加服务。
- 2、"仅部分预览"的文档,不可在线预览部分如存在完整性等问题,可反馈申请退款(可完整预览的文档不适用该条件!)。
- 3、如文档侵犯您的权益,请联系客服反馈,我们会尽快为您处理(人工客服工作时间:9:00-18:30)。
OG2.1.111 Milagrito Detection of TeV Emission from Mrk501Stefan Westerhoff for the Milagro CollaborationUniversity of California,Santa Cruz,CA95064,USAAbstractThe Milagro water Cherenkov detector near Los Alamos,New Mexico,has been operated as a sky monitor at energies of a few TeV between February1997and April1998.Serving as a test run for the full Milagro detector,Milagrito has taken data during the strong and long-lasting1997flare of Mrk501.We present results from the analysis of Mrk501and compare excess and background rate with expectations from the detector simulation.1Introduction:With the detection of4Galactic and3extragalatic sources,Very High Energy(VHE)-ray astronomy, studying the sky at energies above100GeV,has become one of the most interesting frontiers in astronomy. Source detections and analyses in thisfield are still dominated by the highly successful atmospheric Cherenkov technique.Cherenkov telescopes and telescope arrays are optimal tools for the detailed study of established sources and their energy spectra and the theory-guided search for yet unknown sources.There is,however, also a strong case for instruments able to perform an unbiased,systematic and continuous search for TeV sources,thus overcoming the limitations imposed by the low duty cycle and smallfield of view of Cherenkov telecopes.Consequently,the observation technique must exploit the particle content of air showers rather than the Cherenkov light.Afirst-generation all-sky monitor operating at energies below1TeV,the Milagro detector(McCullough et al.,1999)located2650m above sea level near Los Alamos,New Mexico,at latitude N,started data taking in agro is a water Cherenkov detector of size.Two layers of photomultiplier tubes detect the Cherenkov light produced by secondary particles entering the water.The first layer,with450tubes on a grid at a depth of1.4m,allows the shower direction and thus the direction of the primary particle to be reconstructed,while the second layer with273tubes at a depth of 7.0m primarily detects the penetrating component of air showers,i.e.muons,hadrons,and highly energetic electromagnetic particles.A smaller,less sensitive prototype,Milagrito(Atkins et al.,1999),has taken data between February1997 and agrito,a one-layer detector of size with228photomultiplier tubes on a grid at a rather shallow depth of0.9m,served mainly as a test run for this relatively new detection technique.This prototype has,however,taken data during a very intense and long-lastingflare of Mrk501in 1997(Samuelson et al.,1998).For the evaluation of the performance of VHE instruments,the Crab nebula is usually used as a“standard candle”.It is a well-studied steady source with aflux of(1) (Hillas et al.,1998).Simulations indicate that the expected significance from Milagrito for the Crab nebula is less than,ruling out the possibility of using a Crab signal to test Milagrito’s performance.A detection of Mrk501with a sufficiently high significance can be expected had the averageflux been in excess of the Crab flux.During itsflare in1997,Mrk501has been intensively studied with several air Cherenkov telescopes. Although not covering the same observation times,the averagefluxes measured by Whipple(Samuelson et al.,1998)and the HEGRA stereo system of air Cherenkov telescopes(Aharonian et al.,1999)agree extremely well both in shape and magnitude,and they both indicate a significant deviation of the energy spectrum fromFigure1:(a)Effective area of Milagrito for reconstructed-and cosmic ray showers,averaged over a zenith angle range from,as a function of the primary energy.(b)Effective area for-showers for various zenith angle ranges.a simple power ing an averageflux as measured by Whipple,(2) simulations of the Milagrito detector response predict the expected integral-rate from Mrk501to be3.6times the Crab rate.Although highly variable sources like Mrk501are not well-suited for checking the sensitivity of detectors integrating over long time periods,the observation of an excess from Mrk501still provides a test for the sensitivity of Milagrito and reliability of the detector simulation.In addition,observations with Cherenkov telescopes cover only the time from February to October,while Milagrito continued to monitor Mrk501in late1997and early1998.2Milagrito Performance:Sensitivity predictions for Milagrito are based on a detector simulation using the CORSIKA5.61air shower simulation code for the development of the shower in the Earth’s atmosphere,and the GEANT3.21package for the simulation of the detector.The simulation is described in detail elsewhere(Atkins et al.,1999).The Milagrito detector operated with a minimum requirement of100hit tubes per event.Figure1(a)shows the effective area of Milagrito for-showers and cosmic ray background showers induced by protons, helium,and nitrogen,the latter used for representing the combined CNOflux,as a function of the energy of the primary particle.Figure1(b)shows how the efficiency depends on the zenith angle.At energies,the effective area for proton-induced showers is larger than for-showers.This is related to the fact that-induced(thus almost purely electromagnetic)showers are usually more laterally confined so the area covered by the particles reaching detector altitude is smaller than for hadron-induced showers,which tend to have“hot spots”with high particle density at large distances from the shower core. At energies above5TeV,the larger effective area for-induced showers provides an intrinsic cosmic ray background rejection.Figure2:(a)Angular resolution and(b)energy distribution of-showers triggering Milagrito.As a large fraction of showers fulfilling the trigger condition have their core outside the sensitive detector area,the effective area is larger than the geometrical area above3TeV.In fact,only of the proton showers and of the-showers triggering the Milagrito detector have their core on the pond.This leads to a rather broad energy distribution starting at energies as low as100GeV,with no well defined threshold energy(Figure2(b)).The median energy varies slightly with the source declination,ranging from3TeV for sources at to7TeV for sources with.The water Cherenkov technique uses water both as the converter and the detector medium.Consequently, the efficiency for detecting low energy air shower particles is very high,leading to a good sensitivity even for showers with primary energy below1TeV.The anglefitting,however,has to deal with a considerable amount of light late as compared to the shower front reaching the detector.The“late light”is partly produced by low energy particles which tend to trail the shower front.More important,however,is the horizontal light component resulting from the large Cherenkov angle in water(),multiple scattering,-rays,and scattering and reflection of Cherenkov light.The expected angular resolution for cosmic rays agrees with our observations of the cosmic ray shadow of the moon(Wascko et al.,1999).Milagrito’s angular resolution is a strong function of the number of the tubes in thefit to the arrival times of the tubes(Figure2(a)).For the initial source search,a minimum number of40tubes used in the shower planefit is required.This leads to a measured rate of reconstructed events per day from cosmic ray showers in a typical source bin with radius at the declination of Mrk501.This is in good agreement with the predicted rate of events per day from protons,Helium,and CNO nuclei.In the simulation,the contribution of He and CNO to the total trigger rate turns out to be and,respectively.3Results:A straight-forward analysis with a source bin of radius centered on Mrk501leads to an excess. According to simulations this bin size contains of the source events and is optimal for an analysis treating all events equally.The corresponding excess rate averaged over the lifetime of Milagrito(370equivalent source days for Mrk501)is.Figure3:Excess/background for Mrk501as a function of time.At the current sensitivity level the data is consistent with a constantflux.Figure3shows how the excess is accumulated over Milagrito’s lifetime.At our present level of sensitivity, the data is consistent with aflux constant in time.Using the averageflux as measured by air Cherenkov telescopes between February and October1997, simulations predict a-rate of for Milagrito and are thus consistent with the measured excess during this period,.An analysis that takes account of the strong dependence of the resolution on the number of photomultipliers in thefit should be more sensitive to emission from a point source.The results of such an analysis will be presented at the conference.The analysis was extended to10other nearby blazars()in Milagrito’sfield of view,but Mrk501 remains the only analyzed source with a significance in excess of.Results from this blazar sample are reported elsewhere(Westerhoff et al.,1999).This research was supported in part by the National Science Foundation,the U.S.Department of Energy Office of High Energy Physics,the U.S.Department of Energy Office of Nuclear Physics,Los Alamos National Laboratory,the University of California,the Institute of Geophysics and Planetary Physics,The Research Corporation,and the California Space Institute.ReferencesAharonian,F.et al.1999,Astron.&Astrophys.,in press.Atkins et al.1999,Nucl.Instr.Meth.A,in preparation.McCullough,J.F.et al.,these ICRC proceedings(HE6.1.02).Hillas,A.M.et al.1998,ApJ503,744.Samuelson,F.W.et al.,1998,ApJ501,L17.Wascko,M.O.et al.,these ICRC proceedings(SH3.2.39).Westerhoff,S.et al.1999,Proc.Texas Symposium on Relativistic Astrophys.,Paris(France),1998.。