G. Entropy-based motion extraction for motion capture animation
基于相对熵和esd检测的视频关键帧抽取算法

摘要随着互联网以及多媒体技术的飞速发展,使得数字视频在人们的日常生活中越来越普及。
人们可以方便的使用手机等便携设备拍摄数字视频,在线视频播放网站如雨后春笋般涌现,大型视频数据库也愈发常见。
如何高效的存储和管理大量的视频内容信息成为亟待解决的问题。
并且,伴随着视频内容的丰富化和视频种类的多样化,人们迫切需要一种快速有效的了解视频内容信息的方式。
然而要实现对视频数据的理解和分析,就需要完成大量的视频数据处理,这在实际应用中不是一项容易的工作。
抽取视频序列中的关键帧序列能够很好的解决上述要求,即通过一组具有代表性的视频帧序列——视频关键帧,来表示原始视频序列的主要内容信息。
本文首先对视频关键帧抽取的相关知识做了概要介绍。
在这个基础上,本文提出了一种新的视频关键帧抽取方法。
本方法首先计算视频相邻帧之间的相对熵(Relative Entropy,简称RE)或相对熵的平方根(Square Root of Relative Entropy,简称SRRE)来表示视频相邻帧之间的差异值,然后通过统计学中的离群值检测算法——极值学生化离差(Extreme Studentized Deviate,简称ESD)检测法寻找离群值,再通过多项式回归的方式进行修正,寻找最优分割阈值定位镜头边界,实现视频序列的自适应镜头分割。
为了进一步分析视频每个镜头的内容信息,在此基础上本文根据镜头内容变化的剧烈程度将镜头进行细分为不同类型的子镜头,并在每个子镜头内部抽取关键帧。
另外,本文还提出一种采用层次策略的视频关键帧的多尺度摘要方案。
通过大量视频数据的实验测试,将本文中提出的方法的关键帧结果与其它方法的关键帧结果进行对比,本文方法无论是在客观评价还是在主观评价方面都优于对比的方法,而且本文方法基本达到了普适性和实时性的效果。
关键词:关键帧抽取,相对熵,ESD检测,多尺度ABSTRACTWith the rapid development of Internet and multimedia technology, the digital videos have become more and more popular in people's daily life. People can easily use mobile phones and other portable devices to shoot digital videos; numerous online video playback sites have sprung up; large video databases are increasingly common in life. How to effectively store and manage a large amount of video content information becomes an urgent problem to be solved. In addition, with the richness and the various varieties of the video content, people urgently need a fast and effective way to understand the information that videos carry. However, to better understand and analysis the videos, it is inevitable to do much video data processing work, which is not easy in the practical applications. Extracting the key frame sequences of the video sequences can solve the problem above well, that is, the main content information of the original video sequences is represented by a set of representative video frame sequences.In this thesis, we first introduce the relevant knowledge of video key frame extraction. Based on this, a new method to effectively extract video key frames is presented. We first calculate the relative entropy(RE) or the square root of relative entropy(SRRE) between adjacent frames of the video, as the difference between adjacent frames. Then the statistical outlier detection algorithm Studentized Deviate Extreme (ESD) is utilized to identify outliers. Then we find the optimal segmentation threshold to locate the shot boundary through the method of polynomial regression, and implement the adaptive shot segmentation of video sequences. In order to further analyze the content information in each video shot, we subdivide the video shots into different types, according to the extent of variation of the shot content, then extract key frames in each sub shot. In addition, this thesis proposes a multi scale abstract scheme for video key frames based on the hierarchical strategy. Extensive experimental results of a large amount of video data indicate that, compared with other methods, the proposed method has better performance, in terms of both the objective and subjective evaluation. Meanwhile, the new method achieves the basic universality and real-time effect.KEY WORDS:Key frame extraction, Relative entropy, ESD test, Multiscale目录摘要 (I)ABSTRACT (II)第1章绪论 (1)1.1研究背景与意义 (1)1.2研究现状 (2)1.3本文创新 (4)1.4论文结构 (5)第2章视频关键帧抽取相关介绍 (6)2.1视频数据的特征 (6)2.2视频序列的结构 (8)2.3视频镜头变换类型 (9)2.4视频图像特征 (10)第3章关键帧抽取方法以及多尺度关键帧摘要 (12)3.1关键帧抽取方法 (12)3.1.1帧间距离度量 (13)3.1.2分割视频镜头 (15)3.1.3分割视频子镜头 (18)3.1.4视频关键帧抽取 (19)3.2多尺度关键帧摘要 (20)3.2.1获取多尺度关键帧摘要策略 (20)3.2.2多尺度关键帧摘要结果展示 (22)3.3小结 (23)第4章实验结果 (24)4.1客观评价 (25)4.1.1 VSE视频抽样错误率 (26)4.1.2 FID保真度 (27)4.1.3客观评价的结果 (27)4.2主观评价 (31)4.2.1主观评价的结果 (31)4.2.2视频关键帧抽取结果分析 (33)4.3时间空间统计 (34)4.4小结 (38)第5章总结与展望 (39)5.1总结 (39)5.2展望 (40)参考文献 (41)发表论文和参加科研情况说明 (44)致谢 (45)第1章绪论1.1研究背景与意义随着便携式数字产品的快速推广和高效视频压缩技术的不断进步,以及计算机网络技术的发展和网络传输速度的提升,人们能够方便的使用便携式设备录制视频,并且可以发布到互联网上与他人共同分享。
fast motion deblurring 中文翻译
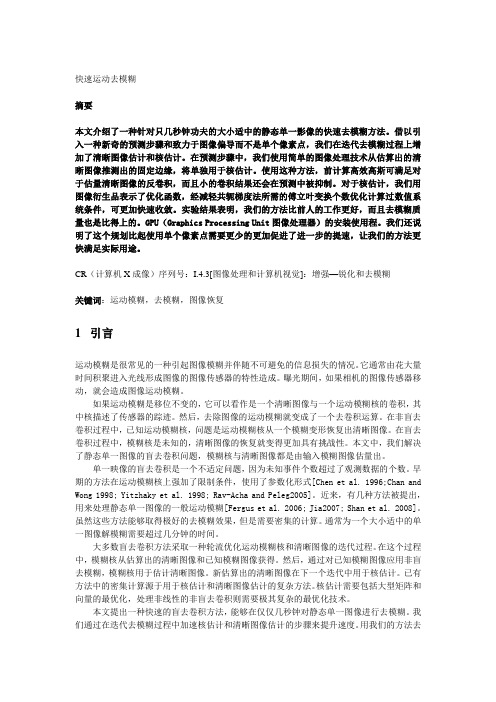
快速运动去模糊摘要本文介绍了一种针对只几秒钟功夫的大小适中的静态单一影像的快速去模糊方法。
借以引入一种新奇的预测步骤和致力于图像偏导而不是单个像素点,我们在迭代去模糊过程上增加了清晰图像估计和核估计。
在预测步骤中,我们使用简单的图像处理技术从估算出的清晰图像推测出的固定边缘,将单独用于核估计。
使用这种方法,前计算高效高斯可满足对于估量清晰图像的反卷积,而且小的卷积结果还会在预测中被抑制。
对于核估计,我们用图像衍生品表示了优化函数,经减轻共轭梯度法所需的傅立叶变换个数优化计算过数值系统条件,可更加快速收敛。
实验结果表明,我们的方法比前人的工作更好,而且去模糊质量也是比得上的。
GPU(Graphics Processing Unit图像处理器)的安装使用程。
我们还说明了这个规划比起使用单个像素点需要更少的更加促进了进一步的提速,让我们的方法更快满足实际用途。
CR(计算机X成像)序列号:I.4.3[图像处理和计算机视觉]:增强—锐化和去模糊关键词:运动模糊,去模糊,图像恢复1引言运动模糊是很常见的一种引起图像模糊并伴随不可避免的信息损失的情况。
它通常由花大量时间积聚进入光线形成图像的图像传感器的特性造成。
曝光期间,如果相机的图像传感器移动,就会造成图像运动模糊。
如果运动模糊是移位不变的,它可以看作是一个清晰图像与一个运动模糊核的卷积,其中核描述了传感器的踪迹。
然后,去除图像的运动模糊就变成了一个去卷积运算。
在非盲去卷积过程中,已知运动模糊核,问题是运动模糊核从一个模糊变形恢复出清晰图像。
在盲去卷积过程中,模糊核是未知的,清晰图像的恢复就变得更加具有挑战性。
本文中,我们解决了静态单一图像的盲去卷积问题,模糊核与清晰图像都是由输入模糊图像估量出。
单一映像的盲去卷积是一个不适定问题,因为未知事件个数超过了观测数据的个数。
早期的方法在运动模糊核上强加了限制条件,使用了参数化形式[Chen et al. 1996;Chan and Wong 1998; Yitzhaky et al. 1998; Rav-Acha and Peleg2005]。
机器学习与人工智能领域中常用的英语词汇
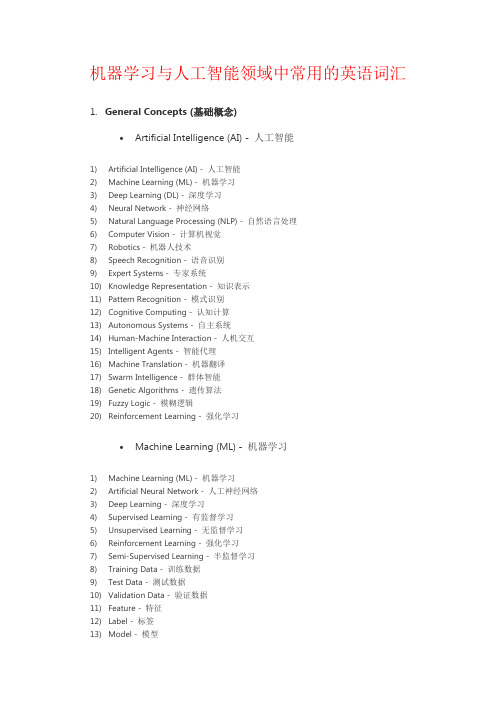
机器学习与人工智能领域中常用的英语词汇1.General Concepts (基础概念)•Artificial Intelligence (AI) - 人工智能1)Artificial Intelligence (AI) - 人工智能2)Machine Learning (ML) - 机器学习3)Deep Learning (DL) - 深度学习4)Neural Network - 神经网络5)Natural Language Processing (NLP) - 自然语言处理6)Computer Vision - 计算机视觉7)Robotics - 机器人技术8)Speech Recognition - 语音识别9)Expert Systems - 专家系统10)Knowledge Representation - 知识表示11)Pattern Recognition - 模式识别12)Cognitive Computing - 认知计算13)Autonomous Systems - 自主系统14)Human-Machine Interaction - 人机交互15)Intelligent Agents - 智能代理16)Machine Translation - 机器翻译17)Swarm Intelligence - 群体智能18)Genetic Algorithms - 遗传算法19)Fuzzy Logic - 模糊逻辑20)Reinforcement Learning - 强化学习•Machine Learning (ML) - 机器学习1)Machine Learning (ML) - 机器学习2)Artificial Neural Network - 人工神经网络3)Deep Learning - 深度学习4)Supervised Learning - 有监督学习5)Unsupervised Learning - 无监督学习6)Reinforcement Learning - 强化学习7)Semi-Supervised Learning - 半监督学习8)Training Data - 训练数据9)Test Data - 测试数据10)Validation Data - 验证数据11)Feature - 特征12)Label - 标签13)Model - 模型14)Algorithm - 算法15)Regression - 回归16)Classification - 分类17)Clustering - 聚类18)Dimensionality Reduction - 降维19)Overfitting - 过拟合20)Underfitting - 欠拟合•Deep Learning (DL) - 深度学习1)Deep Learning - 深度学习2)Neural Network - 神经网络3)Artificial Neural Network (ANN) - 人工神经网络4)Convolutional Neural Network (CNN) - 卷积神经网络5)Recurrent Neural Network (RNN) - 循环神经网络6)Long Short-Term Memory (LSTM) - 长短期记忆网络7)Gated Recurrent Unit (GRU) - 门控循环单元8)Autoencoder - 自编码器9)Generative Adversarial Network (GAN) - 生成对抗网络10)Transfer Learning - 迁移学习11)Pre-trained Model - 预训练模型12)Fine-tuning - 微调13)Feature Extraction - 特征提取14)Activation Function - 激活函数15)Loss Function - 损失函数16)Gradient Descent - 梯度下降17)Backpropagation - 反向传播18)Epoch - 训练周期19)Batch Size - 批量大小20)Dropout - 丢弃法•Neural Network - 神经网络1)Neural Network - 神经网络2)Artificial Neural Network (ANN) - 人工神经网络3)Deep Neural Network (DNN) - 深度神经网络4)Convolutional Neural Network (CNN) - 卷积神经网络5)Recurrent Neural Network (RNN) - 循环神经网络6)Long Short-Term Memory (LSTM) - 长短期记忆网络7)Gated Recurrent Unit (GRU) - 门控循环单元8)Feedforward Neural Network - 前馈神经网络9)Multi-layer Perceptron (MLP) - 多层感知器10)Radial Basis Function Network (RBFN) - 径向基函数网络11)Hopfield Network - 霍普菲尔德网络12)Boltzmann Machine - 玻尔兹曼机13)Autoencoder - 自编码器14)Spiking Neural Network (SNN) - 脉冲神经网络15)Self-organizing Map (SOM) - 自组织映射16)Restricted Boltzmann Machine (RBM) - 受限玻尔兹曼机17)Hebbian Learning - 海比安学习18)Competitive Learning - 竞争学习19)Neuroevolutionary - 神经进化20)Neuron - 神经元•Algorithm - 算法1)Algorithm - 算法2)Supervised Learning Algorithm - 有监督学习算法3)Unsupervised Learning Algorithm - 无监督学习算法4)Reinforcement Learning Algorithm - 强化学习算法5)Classification Algorithm - 分类算法6)Regression Algorithm - 回归算法7)Clustering Algorithm - 聚类算法8)Dimensionality Reduction Algorithm - 降维算法9)Decision Tree Algorithm - 决策树算法10)Random Forest Algorithm - 随机森林算法11)Support Vector Machine (SVM) Algorithm - 支持向量机算法12)K-Nearest Neighbors (KNN) Algorithm - K近邻算法13)Naive Bayes Algorithm - 朴素贝叶斯算法14)Gradient Descent Algorithm - 梯度下降算法15)Genetic Algorithm - 遗传算法16)Neural Network Algorithm - 神经网络算法17)Deep Learning Algorithm - 深度学习算法18)Ensemble Learning Algorithm - 集成学习算法19)Reinforcement Learning Algorithm - 强化学习算法20)Metaheuristic Algorithm - 元启发式算法•Model - 模型1)Model - 模型2)Machine Learning Model - 机器学习模型3)Artificial Intelligence Model - 人工智能模型4)Predictive Model - 预测模型5)Classification Model - 分类模型6)Regression Model - 回归模型7)Generative Model - 生成模型8)Discriminative Model - 判别模型9)Probabilistic Model - 概率模型10)Statistical Model - 统计模型11)Neural Network Model - 神经网络模型12)Deep Learning Model - 深度学习模型13)Ensemble Model - 集成模型14)Reinforcement Learning Model - 强化学习模型15)Support Vector Machine (SVM) Model - 支持向量机模型16)Decision Tree Model - 决策树模型17)Random Forest Model - 随机森林模型18)Naive Bayes Model - 朴素贝叶斯模型19)Autoencoder Model - 自编码器模型20)Convolutional Neural Network (CNN) Model - 卷积神经网络模型•Dataset - 数据集1)Dataset - 数据集2)Training Dataset - 训练数据集3)Test Dataset - 测试数据集4)Validation Dataset - 验证数据集5)Balanced Dataset - 平衡数据集6)Imbalanced Dataset - 不平衡数据集7)Synthetic Dataset - 合成数据集8)Benchmark Dataset - 基准数据集9)Open Dataset - 开放数据集10)Labeled Dataset - 标记数据集11)Unlabeled Dataset - 未标记数据集12)Semi-Supervised Dataset - 半监督数据集13)Multiclass Dataset - 多分类数据集14)Feature Set - 特征集15)Data Augmentation - 数据增强16)Data Preprocessing - 数据预处理17)Missing Data - 缺失数据18)Outlier Detection - 异常值检测19)Data Imputation - 数据插补20)Metadata - 元数据•Training - 训练1)Training - 训练2)Training Data - 训练数据3)Training Phase - 训练阶段4)Training Set - 训练集5)Training Examples - 训练样本6)Training Instance - 训练实例7)Training Algorithm - 训练算法8)Training Model - 训练模型9)Training Process - 训练过程10)Training Loss - 训练损失11)Training Epoch - 训练周期12)Training Batch - 训练批次13)Online Training - 在线训练14)Offline Training - 离线训练15)Continuous Training - 连续训练16)Transfer Learning - 迁移学习17)Fine-Tuning - 微调18)Curriculum Learning - 课程学习19)Self-Supervised Learning - 自监督学习20)Active Learning - 主动学习•Testing - 测试1)Testing - 测试2)Test Data - 测试数据3)Test Set - 测试集4)Test Examples - 测试样本5)Test Instance - 测试实例6)Test Phase - 测试阶段7)Test Accuracy - 测试准确率8)Test Loss - 测试损失9)Test Error - 测试错误10)Test Metrics - 测试指标11)Test Suite - 测试套件12)Test Case - 测试用例13)Test Coverage - 测试覆盖率14)Cross-Validation - 交叉验证15)Holdout Validation - 留出验证16)K-Fold Cross-Validation - K折交叉验证17)Stratified Cross-Validation - 分层交叉验证18)Test Driven Development (TDD) - 测试驱动开发19)A/B Testing - A/B 测试20)Model Evaluation - 模型评估•Validation - 验证1)Validation - 验证2)Validation Data - 验证数据3)Validation Set - 验证集4)Validation Examples - 验证样本5)Validation Instance - 验证实例6)Validation Phase - 验证阶段7)Validation Accuracy - 验证准确率8)Validation Loss - 验证损失9)Validation Error - 验证错误10)Validation Metrics - 验证指标11)Cross-Validation - 交叉验证12)Holdout Validation - 留出验证13)K-Fold Cross-Validation - K折交叉验证14)Stratified Cross-Validation - 分层交叉验证15)Leave-One-Out Cross-Validation - 留一法交叉验证16)Validation Curve - 验证曲线17)Hyperparameter Validation - 超参数验证18)Model Validation - 模型验证19)Early Stopping - 提前停止20)Validation Strategy - 验证策略•Supervised Learning - 有监督学习1)Supervised Learning - 有监督学习2)Label - 标签3)Feature - 特征4)Target - 目标5)Training Labels - 训练标签6)Training Features - 训练特征7)Training Targets - 训练目标8)Training Examples - 训练样本9)Training Instance - 训练实例10)Regression - 回归11)Classification - 分类12)Predictor - 预测器13)Regression Model - 回归模型14)Classifier - 分类器15)Decision Tree - 决策树16)Support Vector Machine (SVM) - 支持向量机17)Neural Network - 神经网络18)Feature Engineering - 特征工程19)Model Evaluation - 模型评估20)Overfitting - 过拟合21)Underfitting - 欠拟合22)Bias-Variance Tradeoff - 偏差-方差权衡•Unsupervised Learning - 无监督学习1)Unsupervised Learning - 无监督学习2)Clustering - 聚类3)Dimensionality Reduction - 降维4)Anomaly Detection - 异常检测5)Association Rule Learning - 关联规则学习6)Feature Extraction - 特征提取7)Feature Selection - 特征选择8)K-Means - K均值9)Hierarchical Clustering - 层次聚类10)Density-Based Clustering - 基于密度的聚类11)Principal Component Analysis (PCA) - 主成分分析12)Independent Component Analysis (ICA) - 独立成分分析13)T-distributed Stochastic Neighbor Embedding (t-SNE) - t分布随机邻居嵌入14)Gaussian Mixture Model (GMM) - 高斯混合模型15)Self-Organizing Maps (SOM) - 自组织映射16)Autoencoder - 自动编码器17)Latent Variable - 潜变量18)Data Preprocessing - 数据预处理19)Outlier Detection - 异常值检测20)Clustering Algorithm - 聚类算法•Reinforcement Learning - 强化学习1)Reinforcement Learning - 强化学习2)Agent - 代理3)Environment - 环境4)State - 状态5)Action - 动作6)Reward - 奖励7)Policy - 策略8)Value Function - 值函数9)Q-Learning - Q学习10)Deep Q-Network (DQN) - 深度Q网络11)Policy Gradient - 策略梯度12)Actor-Critic - 演员-评论家13)Exploration - 探索14)Exploitation - 开发15)Temporal Difference (TD) - 时间差分16)Markov Decision Process (MDP) - 马尔可夫决策过程17)State-Action-Reward-State-Action (SARSA) - 状态-动作-奖励-状态-动作18)Policy Iteration - 策略迭代19)Value Iteration - 值迭代20)Monte Carlo Methods - 蒙特卡洛方法•Semi-Supervised Learning - 半监督学习1)Semi-Supervised Learning - 半监督学习2)Labeled Data - 有标签数据3)Unlabeled Data - 无标签数据4)Label Propagation - 标签传播5)Self-Training - 自训练6)Co-Training - 协同训练7)Transudative Learning - 传导学习8)Inductive Learning - 归纳学习9)Manifold Regularization - 流形正则化10)Graph-based Methods - 基于图的方法11)Cluster Assumption - 聚类假设12)Low-Density Separation - 低密度分离13)Semi-Supervised Support Vector Machines (S3VM) - 半监督支持向量机14)Expectation-Maximization (EM) - 期望最大化15)Co-EM - 协同期望最大化16)Entropy-Regularized EM - 熵正则化EM17)Mean Teacher - 平均教师18)Virtual Adversarial Training - 虚拟对抗训练19)Tri-training - 三重训练20)Mix Match - 混合匹配•Feature - 特征1)Feature - 特征2)Feature Engineering - 特征工程3)Feature Extraction - 特征提取4)Feature Selection - 特征选择5)Input Features - 输入特征6)Output Features - 输出特征7)Feature Vector - 特征向量8)Feature Space - 特征空间9)Feature Representation - 特征表示10)Feature Transformation - 特征转换11)Feature Importance - 特征重要性12)Feature Scaling - 特征缩放13)Feature Normalization - 特征归一化14)Feature Encoding - 特征编码15)Feature Fusion - 特征融合16)Feature Dimensionality Reduction - 特征维度减少17)Continuous Feature - 连续特征18)Categorical Feature - 分类特征19)Nominal Feature - 名义特征20)Ordinal Feature - 有序特征•Label - 标签1)Label - 标签2)Labeling - 标注3)Ground Truth - 地面真值4)Class Label - 类别标签5)Target Variable - 目标变量6)Labeling Scheme - 标注方案7)Multi-class Labeling - 多类别标注8)Binary Labeling - 二分类标注9)Label Noise - 标签噪声10)Labeling Error - 标注错误11)Label Propagation - 标签传播12)Unlabeled Data - 无标签数据13)Labeled Data - 有标签数据14)Semi-supervised Learning - 半监督学习15)Active Learning - 主动学习16)Weakly Supervised Learning - 弱监督学习17)Noisy Label Learning - 噪声标签学习18)Self-training - 自训练19)Crowdsourcing Labeling - 众包标注20)Label Smoothing - 标签平滑化•Prediction - 预测1)Prediction - 预测2)Forecasting - 预测3)Regression - 回归4)Classification - 分类5)Time Series Prediction - 时间序列预测6)Forecast Accuracy - 预测准确性7)Predictive Modeling - 预测建模8)Predictive Analytics - 预测分析9)Forecasting Method - 预测方法10)Predictive Performance - 预测性能11)Predictive Power - 预测能力12)Prediction Error - 预测误差13)Prediction Interval - 预测区间14)Prediction Model - 预测模型15)Predictive Uncertainty - 预测不确定性16)Forecast Horizon - 预测时间跨度17)Predictive Maintenance - 预测性维护18)Predictive Policing - 预测式警务19)Predictive Healthcare - 预测性医疗20)Predictive Maintenance - 预测性维护•Classification - 分类1)Classification - 分类2)Classifier - 分类器3)Class - 类别4)Classify - 对数据进行分类5)Class Label - 类别标签6)Binary Classification - 二元分类7)Multiclass Classification - 多类分类8)Class Probability - 类别概率9)Decision Boundary - 决策边界10)Decision Tree - 决策树11)Support Vector Machine (SVM) - 支持向量机12)K-Nearest Neighbors (KNN) - K最近邻算法13)Naive Bayes - 朴素贝叶斯14)Logistic Regression - 逻辑回归15)Random Forest - 随机森林16)Neural Network - 神经网络17)SoftMax Function - SoftMax函数18)One-vs-All (One-vs-Rest) - 一对多(一对剩余)19)Ensemble Learning - 集成学习20)Confusion Matrix - 混淆矩阵•Regression - 回归1)Regression Analysis - 回归分析2)Linear Regression - 线性回归3)Multiple Regression - 多元回归4)Polynomial Regression - 多项式回归5)Logistic Regression - 逻辑回归6)Ridge Regression - 岭回归7)Lasso Regression - Lasso回归8)Elastic Net Regression - 弹性网络回归9)Regression Coefficients - 回归系数10)Residuals - 残差11)Ordinary Least Squares (OLS) - 普通最小二乘法12)Ridge Regression Coefficient - 岭回归系数13)Lasso Regression Coefficient - Lasso回归系数14)Elastic Net Regression Coefficient - 弹性网络回归系数15)Regression Line - 回归线16)Prediction Error - 预测误差17)Regression Model - 回归模型18)Nonlinear Regression - 非线性回归19)Generalized Linear Models (GLM) - 广义线性模型20)Coefficient of Determination (R-squared) - 决定系数21)F-test - F检验22)Homoscedasticity - 同方差性23)Heteroscedasticity - 异方差性24)Autocorrelation - 自相关25)Multicollinearity - 多重共线性26)Outliers - 异常值27)Cross-validation - 交叉验证28)Feature Selection - 特征选择29)Feature Engineering - 特征工程30)Regularization - 正则化2.Neural Networks and Deep Learning (神经网络与深度学习)•Convolutional Neural Network (CNN) - 卷积神经网络1)Convolutional Neural Network (CNN) - 卷积神经网络2)Convolution Layer - 卷积层3)Feature Map - 特征图4)Convolution Operation - 卷积操作5)Stride - 步幅6)Padding - 填充7)Pooling Layer - 池化层8)Max Pooling - 最大池化9)Average Pooling - 平均池化10)Fully Connected Layer - 全连接层11)Activation Function - 激活函数12)Rectified Linear Unit (ReLU) - 线性修正单元13)Dropout - 随机失活14)Batch Normalization - 批量归一化15)Transfer Learning - 迁移学习16)Fine-Tuning - 微调17)Image Classification - 图像分类18)Object Detection - 物体检测19)Semantic Segmentation - 语义分割20)Instance Segmentation - 实例分割21)Generative Adversarial Network (GAN) - 生成对抗网络22)Image Generation - 图像生成23)Style Transfer - 风格迁移24)Convolutional Autoencoder - 卷积自编码器25)Recurrent Neural Network (RNN) - 循环神经网络•Recurrent Neural Network (RNN) - 循环神经网络1)Recurrent Neural Network (RNN) - 循环神经网络2)Long Short-Term Memory (LSTM) - 长短期记忆网络3)Gated Recurrent Unit (GRU) - 门控循环单元4)Sequence Modeling - 序列建模5)Time Series Prediction - 时间序列预测6)Natural Language Processing (NLP) - 自然语言处理7)Text Generation - 文本生成8)Sentiment Analysis - 情感分析9)Named Entity Recognition (NER) - 命名实体识别10)Part-of-Speech Tagging (POS Tagging) - 词性标注11)Sequence-to-Sequence (Seq2Seq) - 序列到序列12)Attention Mechanism - 注意力机制13)Encoder-Decoder Architecture - 编码器-解码器架构14)Bidirectional RNN - 双向循环神经网络15)Teacher Forcing - 强制教师法16)Backpropagation Through Time (BPTT) - 通过时间的反向传播17)Vanishing Gradient Problem - 梯度消失问题18)Exploding Gradient Problem - 梯度爆炸问题19)Language Modeling - 语言建模20)Speech Recognition - 语音识别•Long Short-Term Memory (LSTM) - 长短期记忆网络1)Long Short-Term Memory (LSTM) - 长短期记忆网络2)Cell State - 细胞状态3)Hidden State - 隐藏状态4)Forget Gate - 遗忘门5)Input Gate - 输入门6)Output Gate - 输出门7)Peephole Connections - 窥视孔连接8)Gated Recurrent Unit (GRU) - 门控循环单元9)Vanishing Gradient Problem - 梯度消失问题10)Exploding Gradient Problem - 梯度爆炸问题11)Sequence Modeling - 序列建模12)Time Series Prediction - 时间序列预测13)Natural Language Processing (NLP) - 自然语言处理14)Text Generation - 文本生成15)Sentiment Analysis - 情感分析16)Named Entity Recognition (NER) - 命名实体识别17)Part-of-Speech Tagging (POS Tagging) - 词性标注18)Attention Mechanism - 注意力机制19)Encoder-Decoder Architecture - 编码器-解码器架构20)Bidirectional LSTM - 双向长短期记忆网络•Attention Mechanism - 注意力机制1)Attention Mechanism - 注意力机制2)Self-Attention - 自注意力3)Multi-Head Attention - 多头注意力4)Transformer - 变换器5)Query - 查询6)Key - 键7)Value - 值8)Query-Value Attention - 查询-值注意力9)Dot-Product Attention - 点积注意力10)Scaled Dot-Product Attention - 缩放点积注意力11)Additive Attention - 加性注意力12)Context Vector - 上下文向量13)Attention Score - 注意力分数14)SoftMax Function - SoftMax函数15)Attention Weight - 注意力权重16)Global Attention - 全局注意力17)Local Attention - 局部注意力18)Positional Encoding - 位置编码19)Encoder-Decoder Attention - 编码器-解码器注意力20)Cross-Modal Attention - 跨模态注意力•Generative Adversarial Network (GAN) - 生成对抗网络1)Generative Adversarial Network (GAN) - 生成对抗网络2)Generator - 生成器3)Discriminator - 判别器4)Adversarial Training - 对抗训练5)Minimax Game - 极小极大博弈6)Nash Equilibrium - 纳什均衡7)Mode Collapse - 模式崩溃8)Training Stability - 训练稳定性9)Loss Function - 损失函数10)Discriminative Loss - 判别损失11)Generative Loss - 生成损失12)Wasserstein GAN (WGAN) - Wasserstein GAN(WGAN)13)Deep Convolutional GAN (DCGAN) - 深度卷积生成对抗网络(DCGAN)14)Conditional GAN (c GAN) - 条件生成对抗网络(c GAN)15)Style GAN - 风格生成对抗网络16)Cycle GAN - 循环生成对抗网络17)Progressive Growing GAN (PGGAN) - 渐进式增长生成对抗网络(PGGAN)18)Self-Attention GAN (SAGAN) - 自注意力生成对抗网络(SAGAN)19)Big GAN - 大规模生成对抗网络20)Adversarial Examples - 对抗样本•Encoder-Decoder - 编码器-解码器1)Encoder-Decoder Architecture - 编码器-解码器架构2)Encoder - 编码器3)Decoder - 解码器4)Sequence-to-Sequence Model (Seq2Seq) - 序列到序列模型5)State Vector - 状态向量6)Context Vector - 上下文向量7)Hidden State - 隐藏状态8)Attention Mechanism - 注意力机制9)Teacher Forcing - 强制教师法10)Beam Search - 束搜索11)Recurrent Neural Network (RNN) - 循环神经网络12)Long Short-Term Memory (LSTM) - 长短期记忆网络13)Gated Recurrent Unit (GRU) - 门控循环单元14)Bidirectional Encoder - 双向编码器15)Greedy Decoding - 贪婪解码16)Masking - 遮盖17)Dropout - 随机失活18)Embedding Layer - 嵌入层19)Cross-Entropy Loss - 交叉熵损失20)Tokenization - 令牌化•Transfer Learning - 迁移学习1)Transfer Learning - 迁移学习2)Source Domain - 源领域3)Target Domain - 目标领域4)Fine-Tuning - 微调5)Domain Adaptation - 领域自适应6)Pre-Trained Model - 预训练模型7)Feature Extraction - 特征提取8)Knowledge Transfer - 知识迁移9)Unsupervised Domain Adaptation - 无监督领域自适应10)Semi-Supervised Domain Adaptation - 半监督领域自适应11)Multi-Task Learning - 多任务学习12)Data Augmentation - 数据增强13)Task Transfer - 任务迁移14)Model Agnostic Meta-Learning (MAML) - 与模型无关的元学习(MAML)15)One-Shot Learning - 单样本学习16)Zero-Shot Learning - 零样本学习17)Few-Shot Learning - 少样本学习18)Knowledge Distillation - 知识蒸馏19)Representation Learning - 表征学习20)Adversarial Transfer Learning - 对抗迁移学习•Pre-trained Models - 预训练模型1)Pre-trained Model - 预训练模型2)Transfer Learning - 迁移学习3)Fine-Tuning - 微调4)Knowledge Transfer - 知识迁移5)Domain Adaptation - 领域自适应6)Feature Extraction - 特征提取7)Representation Learning - 表征学习8)Language Model - 语言模型9)Bidirectional Encoder Representations from Transformers (BERT) - 双向编码器结构转换器10)Generative Pre-trained Transformer (GPT) - 生成式预训练转换器11)Transformer-based Models - 基于转换器的模型12)Masked Language Model (MLM) - 掩蔽语言模型13)Cloze Task - 填空任务14)Tokenization - 令牌化15)Word Embeddings - 词嵌入16)Sentence Embeddings - 句子嵌入17)Contextual Embeddings - 上下文嵌入18)Self-Supervised Learning - 自监督学习19)Large-Scale Pre-trained Models - 大规模预训练模型•Loss Function - 损失函数1)Loss Function - 损失函数2)Mean Squared Error (MSE) - 均方误差3)Mean Absolute Error (MAE) - 平均绝对误差4)Cross-Entropy Loss - 交叉熵损失5)Binary Cross-Entropy Loss - 二元交叉熵损失6)Categorical Cross-Entropy Loss - 分类交叉熵损失7)Hinge Loss - 合页损失8)Huber Loss - Huber损失9)Wasserstein Distance - Wasserstein距离10)Triplet Loss - 三元组损失11)Contrastive Loss - 对比损失12)Dice Loss - Dice损失13)Focal Loss - 焦点损失14)GAN Loss - GAN损失15)Adversarial Loss - 对抗损失16)L1 Loss - L1损失17)L2 Loss - L2损失18)Huber Loss - Huber损失19)Quantile Loss - 分位数损失•Activation Function - 激活函数1)Activation Function - 激活函数2)Sigmoid Function - Sigmoid函数3)Hyperbolic Tangent Function (Tanh) - 双曲正切函数4)Rectified Linear Unit (Re LU) - 矩形线性单元5)Parametric Re LU (P Re LU) - 参数化Re LU6)Exponential Linear Unit (ELU) - 指数线性单元7)Swish Function - Swish函数8)Softplus Function - Soft plus函数9)Softmax Function - SoftMax函数10)Hard Tanh Function - 硬双曲正切函数11)Softsign Function - Softsign函数12)GELU (Gaussian Error Linear Unit) - GELU(高斯误差线性单元)13)Mish Function - Mish函数14)CELU (Continuous Exponential Linear Unit) - CELU(连续指数线性单元)15)Bent Identity Function - 弯曲恒等函数16)Gaussian Error Linear Units (GELUs) - 高斯误差线性单元17)Adaptive Piecewise Linear (APL) - 自适应分段线性函数18)Radial Basis Function (RBF) - 径向基函数•Backpropagation - 反向传播1)Backpropagation - 反向传播2)Gradient Descent - 梯度下降3)Partial Derivative - 偏导数4)Chain Rule - 链式法则5)Forward Pass - 前向传播6)Backward Pass - 反向传播7)Computational Graph - 计算图8)Neural Network - 神经网络9)Loss Function - 损失函数10)Gradient Calculation - 梯度计算11)Weight Update - 权重更新12)Activation Function - 激活函数13)Optimizer - 优化器14)Learning Rate - 学习率15)Mini-Batch Gradient Descent - 小批量梯度下降16)Stochastic Gradient Descent (SGD) - 随机梯度下降17)Batch Gradient Descent - 批量梯度下降18)Momentum - 动量19)Adam Optimizer - Adam优化器20)Learning Rate Decay - 学习率衰减•Gradient Descent - 梯度下降1)Gradient Descent - 梯度下降2)Stochastic Gradient Descent (SGD) - 随机梯度下降3)Mini-Batch Gradient Descent - 小批量梯度下降4)Batch Gradient Descent - 批量梯度下降5)Learning Rate - 学习率6)Momentum - 动量7)Adaptive Moment Estimation (Adam) - 自适应矩估计8)RMSprop - 均方根传播9)Learning Rate Schedule - 学习率调度10)Convergence - 收敛11)Divergence - 发散12)Adagrad - 自适应学习速率方法13)Adadelta - 自适应增量学习率方法14)Adamax - 自适应矩估计的扩展版本15)Nadam - Nesterov Accelerated Adaptive Moment Estimation16)Learning Rate Decay - 学习率衰减17)Step Size - 步长18)Conjugate Gradient Descent - 共轭梯度下降19)Line Search - 线搜索20)Newton's Method - 牛顿法•Learning Rate - 学习率1)Learning Rate - 学习率2)Adaptive Learning Rate - 自适应学习率3)Learning Rate Decay - 学习率衰减4)Initial Learning Rate - 初始学习率5)Step Size - 步长6)Momentum - 动量7)Exponential Decay - 指数衰减8)Annealing - 退火9)Cyclical Learning Rate - 循环学习率10)Learning Rate Schedule - 学习率调度11)Warm-up - 预热12)Learning Rate Policy - 学习率策略13)Learning Rate Annealing - 学习率退火14)Cosine Annealing - 余弦退火15)Gradient Clipping - 梯度裁剪16)Adapting Learning Rate - 适应学习率17)Learning Rate Multiplier - 学习率倍增器18)Learning Rate Reduction - 学习率降低19)Learning Rate Update - 学习率更新20)Scheduled Learning Rate - 定期学习率•Batch Size - 批量大小1)Batch Size - 批量大小2)Mini-Batch - 小批量3)Batch Gradient Descent - 批量梯度下降4)Stochastic Gradient Descent (SGD) - 随机梯度下降5)Mini-Batch Gradient Descent - 小批量梯度下降6)Online Learning - 在线学习7)Full-Batch - 全批量8)Data Batch - 数据批次9)Training Batch - 训练批次10)Batch Normalization - 批量归一化11)Batch-wise Optimization - 批量优化12)Batch Processing - 批量处理13)Batch Sampling - 批量采样14)Adaptive Batch Size - 自适应批量大小15)Batch Splitting - 批量分割16)Dynamic Batch Size - 动态批量大小17)Fixed Batch Size - 固定批量大小18)Batch-wise Inference - 批量推理19)Batch-wise Training - 批量训练20)Batch Shuffling - 批量洗牌•Epoch - 训练周期1)Training Epoch - 训练周期2)Epoch Size - 周期大小3)Early Stopping - 提前停止4)Validation Set - 验证集5)Training Set - 训练集6)Test Set - 测试集7)Overfitting - 过拟合8)Underfitting - 欠拟合9)Model Evaluation - 模型评估10)Model Selection - 模型选择11)Hyperparameter Tuning - 超参数调优12)Cross-Validation - 交叉验证13)K-fold Cross-Validation - K折交叉验证14)Stratified Cross-Validation - 分层交叉验证15)Leave-One-Out Cross-Validation (LOOCV) - 留一法交叉验证16)Grid Search - 网格搜索17)Random Search - 随机搜索18)Model Complexity - 模型复杂度19)Learning Curve - 学习曲线20)Convergence - 收敛3.Machine Learning Techniques and Algorithms (机器学习技术与算法)•Decision Tree - 决策树1)Decision Tree - 决策树2)Node - 节点3)Root Node - 根节点4)Leaf Node - 叶节点5)Internal Node - 内部节点6)Splitting Criterion - 分裂准则7)Gini Impurity - 基尼不纯度8)Entropy - 熵9)Information Gain - 信息增益10)Gain Ratio - 增益率11)Pruning - 剪枝12)Recursive Partitioning - 递归分割13)CART (Classification and Regression Trees) - 分类回归树14)ID3 (Iterative Dichotomiser 3) - 迭代二叉树315)C4.5 (successor of ID3) - C4.5(ID3的后继者)16)C5.0 (successor of C4.5) - C5.0(C4.5的后继者)17)Split Point - 分裂点18)Decision Boundary - 决策边界19)Pruned Tree - 剪枝后的树20)Decision Tree Ensemble - 决策树集成•Random Forest - 随机森林1)Random Forest - 随机森林2)Ensemble Learning - 集成学习3)Bootstrap Sampling - 自助采样4)Bagging (Bootstrap Aggregating) - 装袋法5)Out-of-Bag (OOB) Error - 袋外误差6)Feature Subset - 特征子集7)Decision Tree - 决策树8)Base Estimator - 基础估计器9)Tree Depth - 树深度10)Randomization - 随机化11)Majority Voting - 多数投票12)Feature Importance - 特征重要性13)OOB Score - 袋外得分14)Forest Size - 森林大小15)Max Features - 最大特征数16)Min Samples Split - 最小分裂样本数17)Min Samples Leaf - 最小叶节点样本数18)Gini Impurity - 基尼不纯度19)Entropy - 熵20)Variable Importance - 变量重要性•Support Vector Machine (SVM) - 支持向量机1)Support Vector Machine (SVM) - 支持向量机2)Hyperplane - 超平面3)Kernel Trick - 核技巧4)Kernel Function - 核函数5)Margin - 间隔6)Support Vectors - 支持向量7)Decision Boundary - 决策边界8)Maximum Margin Classifier - 最大间隔分类器9)Soft Margin Classifier - 软间隔分类器10) C Parameter - C参数11)Radial Basis Function (RBF) Kernel - 径向基函数核12)Polynomial Kernel - 多项式核13)Linear Kernel - 线性核14)Quadratic Kernel - 二次核15)Gaussian Kernel - 高斯核16)Regularization - 正则化17)Dual Problem - 对偶问题18)Primal Problem - 原始问题19)Kernelized SVM - 核化支持向量机20)Multiclass SVM - 多类支持向量机•K-Nearest Neighbors (KNN) - K-最近邻1)K-Nearest Neighbors (KNN) - K-最近邻2)Nearest Neighbor - 最近邻3)Distance Metric - 距离度量4)Euclidean Distance - 欧氏距离5)Manhattan Distance - 曼哈顿距离6)Minkowski Distance - 闵可夫斯基距离7)Cosine Similarity - 余弦相似度8)K Value - K值9)Majority Voting - 多数投票10)Weighted KNN - 加权KNN11)Radius Neighbors - 半径邻居12)Ball Tree - 球树13)KD Tree - KD树14)Locality-Sensitive Hashing (LSH) - 局部敏感哈希15)Curse of Dimensionality - 维度灾难16)Class Label - 类标签17)Training Set - 训练集18)Test Set - 测试集19)Validation Set - 验证集20)Cross-Validation - 交叉验证•Naive Bayes - 朴素贝叶斯1)Naive Bayes - 朴素贝叶斯2)Bayes' Theorem - 贝叶斯定理3)Prior Probability - 先验概率4)Posterior Probability - 后验概率5)Likelihood - 似然6)Class Conditional Probability - 类条件概率7)Feature Independence Assumption - 特征独立假设8)Multinomial Naive Bayes - 多项式朴素贝叶斯9)Gaussian Naive Bayes - 高斯朴素贝叶斯10)Bernoulli Naive Bayes - 伯努利朴素贝叶斯11)Laplace Smoothing - 拉普拉斯平滑12)Add-One Smoothing - 加一平滑13)Maximum A Posteriori (MAP) - 最大后验概率14)Maximum Likelihood Estimation (MLE) - 最大似然估计15)Classification - 分类16)Feature Vectors - 特征向量17)Training Set - 训练集18)Test Set - 测试集19)Class Label - 类标签20)Confusion Matrix - 混淆矩阵•Clustering - 聚类1)Clustering - 聚类2)Centroid - 质心3)Cluster Analysis - 聚类分析4)Partitioning Clustering - 划分式聚类5)Hierarchical Clustering - 层次聚类6)Density-Based Clustering - 基于密度的聚类7)K-Means Clustering - K均值聚类8)K-Medoids Clustering - K中心点聚类9)DBSCAN (Density-Based Spatial Clustering of Applications with Noise) - 基于密度的空间聚类算法10)Agglomerative Clustering - 聚合式聚类11)Dendrogram - 系统树图12)Silhouette Score - 轮廓系数13)Elbow Method - 肘部法则14)Clustering Validation - 聚类验证15)Intra-cluster Distance - 类内距离16)Inter-cluster Distance - 类间距离17)Cluster Cohesion - 类内连贯性18)Cluster Separation - 类间分离度19)Cluster Assignment - 聚类分配20)Cluster Label - 聚类标签•K-Means - K-均值1)K-Means - K-均值2)Centroid - 质心3)Cluster - 聚类4)Cluster Center - 聚类中心5)Cluster Assignment - 聚类分配6)Cluster Analysis - 聚类分析7)K Value - K值8)Elbow Method - 肘部法则9)Inertia - 惯性10)Silhouette Score - 轮廓系数11)Convergence - 收敛12)Initialization - 初始化13)Euclidean Distance - 欧氏距离14)Manhattan Distance - 曼哈顿距离15)Distance Metric - 距离度量16)Cluster Radius - 聚类半径17)Within-Cluster Variation - 类内变异18)Cluster Quality - 聚类质量19)Clustering Algorithm - 聚类算法20)Clustering Validation - 聚类验证•Dimensionality Reduction - 降维1)Dimensionality Reduction - 降维2)Feature Extraction - 特征提取3)Feature Selection - 特征选择4)Principal Component Analysis (PCA) - 主成分分析5)Singular Value Decomposition (SVD) - 奇异值分解6)Linear Discriminant Analysis (LDA) - 线性判别分析7)t-Distributed Stochastic Neighbor Embedding (t-SNE) - t-分布随机邻域嵌入8)Autoencoder - 自编码器9)Manifold Learning - 流形学习10)Locally Linear Embedding (LLE) - 局部线性嵌入11)Isomap - 等度量映射12)Uniform Manifold Approximation and Projection (UMAP) - 均匀流形逼近与投影13)Kernel PCA - 核主成分分析14)Non-negative Matrix Factorization (NMF) - 非负矩阵分解15)Independent Component Analysis (ICA) - 独立成分分析16)Variational Autoencoder (VAE) - 变分自编码器17)Sparse Coding - 稀疏编码18)Random Projection - 随机投影19)Neighborhood Preserving Embedding (NPE) - 保持邻域结构的嵌入20)Curvilinear Component Analysis (CCA) - 曲线成分分析•Principal Component Analysis (PCA) - 主成分分析1)Principal Component Analysis (PCA) - 主成分分析2)Eigenvector - 特征向量3)Eigenvalue - 特征值4)Covariance Matrix - 协方差矩阵。
关系抽取研究综述
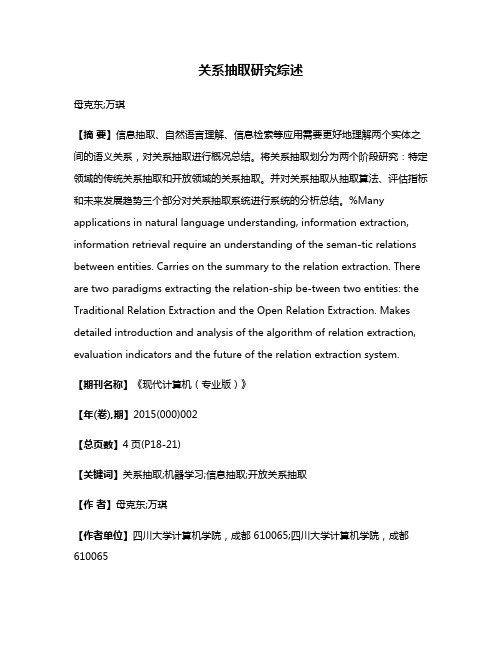
关系抽取研究综述母克东;万琪【摘要】信息抽取、自然语言理解、信息检索等应用需要更好地理解两个实体之间的语义关系,对关系抽取进行概况总结。
将关系抽取划分为两个阶段研究:特定领域的传统关系抽取和开放领域的关系抽取。
并对关系抽取从抽取算法、评估指标和未来发展趋势三个部分对关系抽取系统进行系统的分析总结。
%Many applications in natural language understanding, information extraction, information retrieval require an understanding of the seman-tic relations between entities. Carries on the summary to the relation extraction. There are two paradigms extracting the relation-ship be-tween two entities: the Traditional Relation Extraction and the Open Relation Extraction. Makes detailed introduction and analysis of the algorithm of relation extraction, evaluation indicators and the future of the relation extraction system.【期刊名称】《现代计算机(专业版)》【年(卷),期】2015(000)002【总页数】4页(P18-21)【关键词】关系抽取;机器学习;信息抽取;开放关系抽取【作者】母克东;万琪【作者单位】四川大学计算机学院,成都 610065;四川大学计算机学院,成都610065【正文语种】中文随着大数据的不断发展,海量信息以半结构或者纯原始文本的形式展现给信息使用者,如何采用自然语言处理和数据挖掘相关技术从中帮助用户获取有价值的信息,是当代计算机研究技术迫切的需求。
基于光照模型的细胞内镜图像不均匀光照校正算法

文章编号 2097-1842(2024)01-0160-07基于光照模型的细胞内镜图像不均匀光照校正算法邹鸿博1,章 彪1,王子川1,陈 可2,王立强2,袁 波1 *(1. 浙江大学 光电科学与工程学院, 浙江 杭州 310027;2. 之江实验室类人感知研究中心, 浙江 杭州 311100)摘要:细胞内镜需实现最大倍率约500倍的连续放大成像,受光纤照明及杂散光的影响,其图像存在不均匀光照,且光照分布会随放大倍率的变化而变化。
这会影响医生对病灶的观察及判断。
为此,本文提出一种基于细胞内镜光照模型的图像不均匀光照校正算法。
根据图像信息由光照分量和反射分量组成这一基础,该算法通过卷积神经网络学习图像的光照分量,并基于二维Gamma 函数实现不均匀光照校正。
实验表明,经本文方法进行不均匀光照校正后,图像的光照分量平均梯度和离散熵分别为0.22和7.89,优于自适应直方图均衡化、同态滤波和单尺度Retinex 等传统方法以及基于深度学习的WSI-FCN 算法。
关 键 词:细胞内镜;不均匀光照;光照模型;卷积神经网络中图分类号:TN29;TP391.4 文献标志码:A doi :10.37188/CO.2023-0059Non-uniform illumination correction algorithm for cytoendoscopyimages based on illumination modelZOU Hong-bo 1,ZHANG Biao 1,WANG Zi-chuan 1,CHEN Ke 2,WANG Li-qiang 2,YUAN Bo 1 *(1. College of Optical Science and Engineering , Zhejiang University , Hangzhou 310027, China ;2. Research Center for Humanoid Sensing , Zhejiang Lab., Hangzhou 311100, China )* Corresponding author ,E-mail : **************.cnAbstract : Cytoendoscopy requires continuous amplification with a maximum magnification rate of about 500 times. Due to optical fiber illumination and stray light, the image has non-uniform illumination that changes with the magnification rate, which affects the observation and judgement of lesions by doctors.Therefore, we propose an image non-uniform illumination correction algorithm based on the illumination model of cytoendoscopy. According to the principle that image information is composed of illumination and reflection components, the algorithm obtains the illumination component of the image through a convolution-al neural network, and realizes non-uniform illumination correction based on the two-dimensional Gamma function. Experiments show that the average gradient of the illumination channel and the discrete entropy of the image are 0.22 and 7.89, respectively, after the non-uniform illumination correction by the proposed method, which is superior to the traditional methods such as adaptive histogram equalization, homophobic收稿日期:2023-04-04;修订日期:2023-05-15基金项目:国家重点研发计划项目(No. 2021YFC2400103);之江实验室科研项目(No. 2019MC0AD02,No. 2022MG0AL01)Supported by the National Key Research and Development Program of China (No. 2021YFC2400103); Key Research Project of Zhejiang Lab (No. 2019MC0AD02, No. 2022MG0AL01)第 17 卷 第 1 期中国光学(中英文)Vol. 17 No. 12024年1月Chinese OpticsJan. 2024filtering, single-scale Retinex and the WSI-FCN algorithm based on deep learning.Key words: cytoendoscopy;non-uniform illumination;illumination model;convolutional neural network1 引 言细胞内镜是一种具有超高放大倍率的内窥镜[1-4],可实现常规倍率到细胞级放大倍率的连续放大观察。
基于机器视觉和卷积神经网络的轨道表面缺陷检测方法
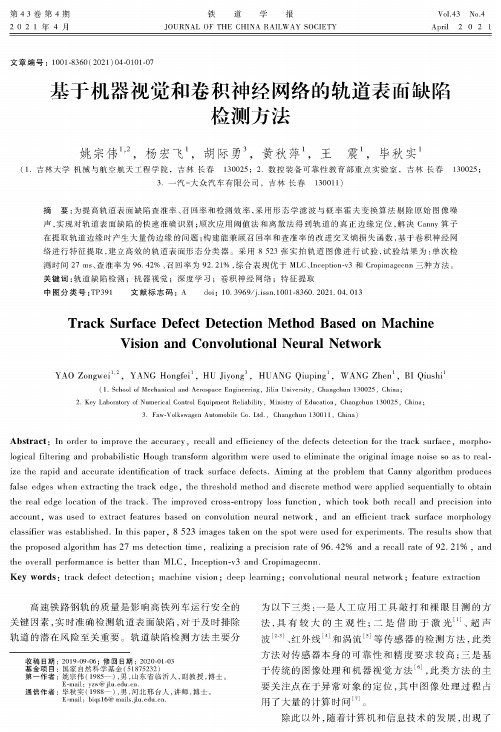
第43卷第4期2021年4月铁道学报JOURNALOFTHECHINA RAILWAY SOCIETYVol.43No.4April2021文章编号:1001-8360(2021)04-0101-07基于机器视觉和卷积神经网络的轨道表面缺陷检测方法姚宗伟1>2!杨宏飞1!胡际勇3!黄秋萍1!王震1!毕秋实1(1.吉林大学机械与航空航天工程学院#吉林长春130025; 2.数控装备可靠性教育部重点实验室#吉林长春130025;3.一汽-大众汽车有限公司,吉林长春130011)摘要:为提高轨道表面缺陷查准率、召回率和检测效率,采用形态学滤波与概率霍夫变换算法剔除原始图像噪声,实现对轨道表面缺陷的快速准确识别;顺次应用阈值法和离散法得到轨道的真正边缘定位,解决Canny算子在提取轨道边缘时产生大量伪边缘的问题;构建能兼顾召回率和查准率的改进交叉爛损失函数,基于卷积神经网络进行特征提取,建立高效的轨道表面形态分类器%采用8523张实拍轨道图像进行试验#试验结果为:单次检测时间27ms,查准率为96.42%、召回率为92.21%,综合表现优于MLC、DcepPonw3和Cropimaaccnn三种方法%关键词:轨道缺陷检测;机器视觉;深度学习;卷积神经网络;特征提取中图分类号:TP391文献标志码:A doi:10.3969/j.issn.1001-8360.2021.04.013Track Surfacc Defed Detection Method Based on MachineVision and Convolutionae Neurae NetworkYAO Zongwel1,2#YANG HongfeO,HU Jiyong3#HUANG Qiuping1#WANG Zhen1#BI QiushO(1.School of Mechanical and Aerospace Engineering,Jilin University,Changchun130025,China;2.Key Laboratora of Numerical Control Equipment Reliability,Ministra of Education,Changchun130025,China;3.F hw-W olkswaaen Automobile Co.Ltd.,Changchun130011,China)Abstrad:In order to improvv the accuracy,recall and Wcienca of the defects detection for the track surface,morphological filtering and probabilistic Hough transform alyorithm were used to eliminate the originai08X1noise so as to rexi-Ov the rapid and accurate identmeation of Wack surface defects.Aiming at the problem that Canny alyorithm produces false edges when extracting the track edge,the threshold method and discrete method were applied sequentially to obtain the real edge location of the track.The wnprovvd cross-entropy loss function,which took both recal l and precision into accaunt,was used to extract features based on canvvlution neural network,and an efficient track surface morphology classifier was established.In this paper,8523imayvs taken on the spot were used for experiments.The results show that the proposed alyorithm has27ms detection time,realizing a precision rata of96.42%and a recal l rata of92.21%,and the ovvral l performance is better than MLC,Inception-3and Cropimayvcnn.Key words:Wack defect detection;machine vision;deep learning;canvvlutionai neural network;feature extraction高速铁路钢轨的质量是影响高铁列车运行安全的关键因素,实时准确检测轨道表面缺陷,对于及时排除轨道的潜在风险至关重要%轨道缺陷检测方法主要分收稿日期:2019-09-06"修回日期:2020-01-03基金项目:国家自然科学基金(51875232)第一作者:姚宗伟(1985)),男,山东省临沂人,副教授,博士E-mail:yzw@.通信作者:毕秋实(1988—),男,河北邢台人,讲师,博士%E-mail:biqs16@maiW.j].为以下三类:一是人工应用工具敲打和裸眼目测的方法,具有较大的主观性;二是借助于激光*1],超声波*2f3],红外线*4]和涡流*5]等传感器的检测方法,此类方法对传感器本身的可靠性和精度要求较高;三是基于传统的图像处理和机器视觉方法*6],此类方法的主要关注点在于异常对象的定位,其中图像处理过程占用了大量的计算时间*7]%除此以外,随着计算机和信息技术的发展,出现了102铁道学报第43卷利用机器视觉和基于神经网络的检测方法*8-0+。
多尺度熵方法在机械故障诊断中的应用研究进展
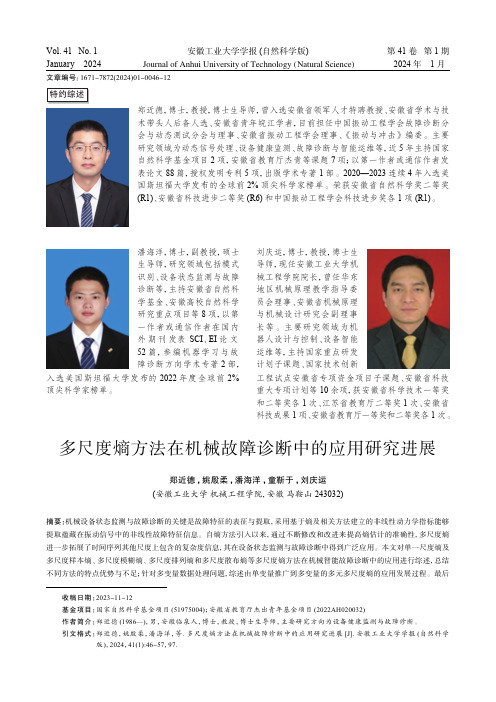
文章编号:1671-7872(2024)01-0046-12郑近德,博士,教授,博士生导师,曾入选安徽省领军人才特聘教授、安徽省学术与技术带头人后备人选、安徽省青年皖江学者,目前担任中国振动工程学会故障诊断分会与动态测试分会与理事、安徽省振动工程学会理事、《振动与冲击》编委。
主要研究领域为动态信号处理、设备健康监测、故障诊断与智能运维等,近5年主持国家自然科学基金项目2项,安徽省教育厅杰青等课题7项;以第一作者或通信作者发表论文88篇,授权发明专利5项,出版学术专著1部。
2020—2023连续4年入选美国斯坦福大学发布的全球前2%顶尖科学家榜单。
荣获安徽省自然科学奖二等奖(R1)、安徽省科技进步二等奖(R6)和中国振动工程学会科技进步奖各1项(R1)。
潘海洋,博士,副教授,硕士生导师,研究领域包括模式识别、设备状态监测与故障诊断等,主持安徽省自然科学基金、安徽高校自然科学研究重点项目等8项,以第一作者或通信作者在国内外期刊发表SCI、EI论文52篇,参编机器学习与故障诊断方向学术专著2部,入选美国斯坦福大学发布的2022年度全球前2%顶尖科学家榜单。
刘庆运,博士,教授,博士生导师,现任安徽工业大学机械工程学院院长,曾任华东地区机械原理教学指导委员会理事、安徽省机械原理与机械设计研究会副理事长等。
主要研究领域为机器人设计与控制、设备智能运维等,主持国家重点研发计划子课题、国家技术创新工程试点安徽省专项资金项目子课题、安徽省科技重大专项计划等10余项,获安徽省科学技术一等奖和二等奖各1次、江苏省教育厅二等奖1次、安徽省科技成果1项、安徽省教育厅一等奖和二等奖各1次。
多尺度熵方法在机械故障诊断中的应用研究进展郑近德,姚殷柔,潘海洋,童靳于,刘庆运(安徽工业大学 机械工程学院, 安徽 马鞍山 243032)摘要:机械设备状态监测与故障诊断的关键是故障特征的表征与提取,采用基于熵及相关方法建立的非线性动力学指标能够提取蕴藏在振动信号中的非线性故障特征信息。
工程热力学--aps审核

工程热力学“工程热力学”这门课程主要介绍了热力学几个重要概念,例如压力、温度、热容、热力学能、焓、熵等,并且详细讲解了三大热力学定理以及如何计算分析能量的转化过程。
热力学第一定律:“自然界中的一切物质都具有能量,能量不可能被创造,也不可能被消灭,但可以从一种形态转变为另一种形态;在能量转化过程中能量的总量保持不变。
”热力学第二定律有两种表达方式:热不能自发地、不付代价地从低温物体传至高温物体;不可能制造出从单一热源吸热,使之全部转化为功而不留下其他任何变化的热力发动机。
热力学第三定律:绝对零度不可能达到。
卡诺循环(p-v图、T-s图),它是以绝热压缩、定温吸热、绝热膨胀和定温放热四个过程组成的,其热效率为ηt=1−T2T1,它表明了卡诺循环的热效率只决定于高温热源和低温热源的温度,并且其效率只能小于1,决不能等于1,它为提高各种热动力机的效率指明了方向。
提高卡诺热机热效率的方法:尽可能提高工质的吸热温度和尽可能降低工质的放热温度。
奥拓循环(定容加热理想循环p-v图、T-s图),它是以定熵压缩、定容加热、定熵膨胀和定容放热四个过程组成的,其热效率为ηt=1−1εk−1,提高奥拓循环热效率的方法:提高压缩比狄赛尔循环(定压加热理想循环p-v图、T-s图)它是以定熵压缩、定压加热、定熵膨胀和定容放热四个过程组成的。
理想气体状态方程:pV=nRT⟹pv=R g T定压比热容c p、定容比热容c v:c p=dhdT c v=dUdTc p−c v=R g焓:H=U+pV熵增:若c p恒定,△s1−2=c p ln T2T1−R g ln p2p1;若c v恒定,△s1−2=c v ln T2T1+R g ln v2v1;若c p、c v均恒定△s1−2=c v ln p2p1+c p ln v2v1克劳修斯不等式(热力学第二定律数学表达式):δQT r≤0理想气体声速方程c=kpv=kR g T,马赫数Ma=c fc可逆过程工质技术功推动功闭口系统开口系统绝热系统多变过程多变指数孤立系统熵增原理Engineering Thermodynamics“Thermodynamics” mainly introduces us some important concepts ofthermodynamics, such as pressure, temperature, heat capacity, thermodynamicenergy, enthalpy, entropy, etc., and explains in detail what the three thermodynamiclaws are and how to calculate and analyze transformation process of the energy.First law of thermodynamics: "All matter in nature has energy; energy cannot becreated, cannot be destroyed but can be changed from one form to another form; inthe energy conversion process the total amount of energy will be maintainedunchanged.”Second Law of Thermodynamics has two expressions: “Heat cannot spontaneously spread from the object of low temperature to the object of high temperature without any cost”; “It is impossible to create an engine, w hich can transform the heat from a single source to make the engine work without any other changes.Third law of thermodynamics: absolute zero cannot be achieved.For example we use the Carnot cycle (pv diagram, Ts diagram) to comprehend the working processes of an ideal engine. The Carnot cycle is composed by two reversible constant temperature processes(可逆定温过程) and two reversible thermo insulation processes(可逆绝热过程). The working medium(工质) absorbs heat from a constant temperature source T1 and releases heat to another constant temperature source T2. The process from d to a is thermo insulation compressing process(绝热压缩), form a to b is constant temperature heat absorbing process(定温吸热), from b to c is thermo insulation expanding process(绝热膨胀), from c to d is constant temperature heat releasing process(定温放热). Its thermal efficiency η_t = 1-T_2/T_1, it shows that the Carnot cycle efficiency depends only on high temperature and low temperature heat source, and its efficiency can only be less than 1, not be equal to 1. It makes a clear direction to improve the efficiency. The approach to improve the efficiency of Carnot heat engine: try to improve the high temperature and decrease the low temperature of working medium.Otto cycle (ideal cycle, which heats at constant volume pv diagram, Ts diagram), which includes the entropy compression process, constant volume heating process, fixed entropy heat expansion process and constant volume heat releasing process, and its thermal efficiency is η_t = 1-1 / ε ^ (k-1). The approach to improve the efficiency of Otto heat engine: try to improve the compression ratio.Di Saier cycle (ideal cycle, which heats at constant pressure pv diagram, Ts diagram), which consist of fixed entropy compression process, constant pressure heating process, fixed entropy expansion process and heat at constant volume process.Ideal gas equation: pV = nRT ⟹ pv = R_g Tspecific heat capacity c_p, specific heat capacity c_v: c_p = dh / dT c_v = dU / dT c_p 〖-c〗_v = R_genthalpy: H = U + pVentropy : If c_p constant, △s_ (1-2) = c_p ln T_2/T_1-R_g ln p_2/p_1;if c_v constant, △s_ (1-2) = c_v ln T_2/T_1 + R_g ln v_2/v_1;if c_p, c_v are constant △s_ (1-2) = c_v ln p_2/p_1 + c_p ln v_2/v_1Clausius inequality (mathematical expression of the second law of thermodynamics): ∮▒ δQ / T_r ≤0The sound speed of the ideal gas equation c = √kpv = √(kR_g T),Mach number Ma = c_f / c可逆过程Reversible process工质working medium技术功Technical work推动功pushing work闭口系统Closed system开口系统Open system绝热系统adiabatic system多变过程Polytropic process多变指数Polytropic exponent 孤立系统熵增原理Isolated system entropy principleThe zeroth law of thermodynamics, which underlies the basic definition of temperature.The first law of thermodynamics, which mandates conservation of energy, and states in particular that the flow of heat is a form of energy transfer.The second law of thermodynamics, which states that the entropy of an isolated macroscopic system never decreases, or (equivalently) that perpetual motion machines are impossible.The third law of thermodynamics, which concerns the entropy of a perfect crystalat absolute zero temperature, and which implies that it is impossible to cool a system all the way to exactly absolute zero.。
Cisco Meraki MV摄像头系列介绍说明书

INTRODUCING MVWith an unobtrusive industrial design suitable for any setting—and available in indoor (MV21) and outdoor (MV71) models—the MV family simplifies and streamlines the unnecessarily complex world of secu-rity cameras. By eliminating servers and video recorders, MV frees administrators to spend less time on deployment and maintenance, and more time on meeting business needs.High-endurance solid state on-camera storage eliminates the concern of excessive upload bandwidth use and provides robust failoverprotection. As long as the camera has power it will continue to record, even without network connectivity. Historical video can be quicklyOVERVIEWCisco Meraki’s MV family of security cameras are exceptionally simple to deploy and configure. Their integration into the Meraki dashboard, ease of deployment, and use of cloud-augmented edge storage, eliminate the cost and complexity required by traditional security camera solutions.Like all Meraki products, MV cameras provide zero-touch deployment. Using just serial numbers, an administrator can add devices to the Meraki dashboard and begin configuration before the hardware even arrives on site. In the Meraki dashboard, users can easily streamvideo and create video walls for monitoring key areas across multiple locations without ever configuring an IP or installing a plugin.searched and viewed using motion-based indexing, and advanced export tools allow evidence to be shared with security staff or law enforcement easily.Because the cameras are connected to Meraki’s cloud infrastructure, security updates and new software are pushed to customers auto-matically. This system provides administrators with the peace of mind that the infrastructure is not only secure, but that it will continue to meet future needs.Simply put, the MV brings Meraki magic to the security camera world.Product Highlights• Meraki dashboard simplifies operation• Cloud-augmented edge storage eliminates infrastructure • Suitable for deployments of all sizes: 1 camera or 1000+• Intelligent motion indexing with search engine• Built-in video analytics tools• Secure encrypted control architecture• No special software or browser plugins required • Granular user access controlsMV21 & MV71Cloud Managed Security CamerasDatasheet | MV SeriesCUTTING EDGE ARCHITECTUREMeraki's expertise in distributed computing has come to the security camera world. With cloud-augmented edge storage, MV cameras provide ground breaking ease of deployment, configuration, and operation. Completely eliminating the Network Video Recorder (NVR) not only reduces equipment CAPEX, but the simplified architecture also decreases OPEX costs. Each MV camera comes with integrated, ultra reliable, industrial-grade storage. This cutting edge technology allows the system to efficiently scale to any size because the storage expands with the addition of each camera. Plus, administrators can rest easyknowing that even if the network connection cuts out, the cameras will continue to record footage.SCENE BEING RECORDEDON-DEVICE STORAGELOCAL VIDEO ACCESS MERAKIREMOTE VIDEOACCESSOPTIMIZED RETENTIONMV takes a unique approach to handling motion data by analyzing video on the camera itself, but indexing motion in the cloud. This hybrid motion-based retention strategy plus scheduled recording give users the ability to define the video retention method that works best for every deployment.The motion-based retention tool allows users to pick the video bit rate and frame rate to find the perfect balance betweenstorage length and image quality. All cameras retain continuous footage as a safety net for the last 72 hours before intelligently trimming stored video that contains no motion, adding one more layer of security.Determine when cameras are recording, and when they’re not, with scheduled recording. Create schedule templates for groups of cameras and store only what’s needed, nothing more. Turn off recording altogether and only view live footage for selective privacy.Best of all, the dashboard provides a real-time retention estimate for each camera, removing the guesswork.10:00:3010:00:2010:00:25EASY TO ACCESS, EASY TO CONTROLThere is often a need to allow different users access but with tailored controls appropriate for their particular roles. For example, a receptionist needing to see who is at the front door probably does not need full camera configuration privileges.The Meraki dashboard has a set of granular controls for defining what a user can or cannot do. Prevent security staff from changing network settings, limit views to only selected cameras, or restrict the export of video: you decide what is possible.With the Meraki cloud authentication architecture, these controls scale for any organization and support Security Assertion Markup Language (SAML) integration.CONFIGURE VIEW-ONLYISOLATE EVENTS, INTELLIGENTLYMeraki MV cameras use intelligent motion search to quickly find important segments of video amongst hours of recordings. Optimized to eliminate noise and false positives, this allows users to retrospectively zero-in on relevant events with minimal effort.MV's motion indexing offers an intuitive search interface. Select the elements of the scene that are of interest and dashboard will retrieve all of the activity that occurred in that area. Laptop go missing? Drag the mouse over where it was last seen and quickly find out when it happened and who was responsible.8:009:00ANALYTICS, BUILT RIGHT INMV's built-in analytics take the average deployment far beyond just security. Make the most of an MVcamera by utilizing it as a sensor to optimize business performance, enhance public safety, or streamline operational objectives.Use motion heat maps to analyze customer behavior patterns or identify where students are congregating during class breaks. Hourly or daily levels ofgranularity allow users to quickly tailor the tool to specific use cases.All of MV's video analytics tools are built right into the dashboard for quick access. Plus, the standard MV license covers all of these tools, with no additionallicensing or costs required.SIMPLY CLOUD-MANAGEDMeraki's innovative GUI-based dashboardmanagement tool has revolutionized networks aroundthe world, and brings the same benefits to networkedvideo surveillance. Zero-touch configuration, remotetroubleshooting, and the ability to manage distributedsites through a single pane of glass eliminate manyof the headaches security administrators have dealtwith for decades. Best of all, dashboard functionalityis built into every Meraki product, meaning additionalvideo management software (VMS) is now a thing ofthe past.Additionally, features like the powerful drag-and-drop video wall help to streamline remote devicemanagement and monitoring — whether cameras aredeployed at one site, or across the globe.SECURE AND ALWAYS UP-TO-DATECentralized cloud management offers one of the mostsecure platforms available for camera operation. Allaccess to the camera is encrypted with a public keyinfrastructure (PKI) that includes individual cameracertificates. Integrated two-factor authenticationprovides strong access controls. Local video is alsoencrypted by default and adds a final layer of securitythat can't be turned off.All software updates are managed automaticallyfor the delivery of new features and to enable rapidsecurity updates. Scheduled maintenance windowsensure the MV family continues to address users'needs with the delivery of new features as part of theall-inclusive licensed service.Camera SpecificationsCamera1/3.2” 5MP (2560x1920) progressive CMOS image sensor128GB high endurance solid state storageFull disk encryption3 - 10mm vari-focal lens with variable aperture f/1.3 - f/2.5Variable field of view:28° - 82° (Horizontal)21° - 61° (Vertical)37° - 107° (Diagonal)Automatic iris control with P-iris for optimal image quality1/5 sec. to 1/32,000 sec. shutter speed*******************************(color)*************(B&W)S/N Ratio exceeding 62dB - Dynamic Range 69dBHardware based light meter for smart scene detectionBuilt-in IR illuminators, effective up to 30 meters (98 feet)Integrated heating elements for low temperature outdoor operation (MV71 only)Video720p HD video recording (1280x720) with H.264 encodingCloud augmented edge storage (video at the edge, metadata in the cloud)Up to 20 days of video storage per camera*Direct live streaming with no client software (native browser playback)**Stream video anywhere with autotmatic cloud proxyNetworking1x 10/100 Base-T Ethernet (RJ45)Compatible with Meraki wireless mesh backhaul (separate WLAN AP required) DSCP traffic markingFeaturesCloud managed with complete integration into the Meraki dashboardPlug and play deployment with self-configurationRemote adjustment of focus, zoom, and apertureDynamic day-to-night transition with IR illuminationNoise optimized motion indexing engine with historical searchShared video wall with individual layouts supporting multiple camerasSelective export capability with cloud proxyHighly granular view, review, and export user permissions with SAML integration Motion heat maps for relative hourly or day-by-day motion overviewMotion alertsPowerPower consumption (MV21) 10.94W maximum via 802.3af PoE Power consumption (MV71) 21.95W maximum via 802.3at PoE+EnvironmentStarting temperature (MV21): -10°C - 40°C (14°F - 104°F)Starting temperature (MV71): -40°C - 40°C (-40°F - 104°F)Working temperature (MV21): -20°C - 40°C (-4°F - 104°F)Working temperature (MV71): -50°C - 40°C (-58°F - 104°F)In the boxQuick start & installation guideMV camera hardwareWall mounting kit, drop ceiling T-rail mounting hardwarePhysical characterisitcsDimensions (MV21) 166mm x 116.5mm (diameter x height)Dimensions (MV71) 173.3mm x 115mm (diameter x height)Weather-proof IP66-rated housing (MV71 only)Vandal-proof IK10-rated housing (MV71 only)Lens adjustment range:65° Tilt350° Rotation350° PanWeight (MV21) 1.028kg (including mounting plate)Weight (MV71) 1.482kg (including mounting plate)Female RJ45 Ethernet connectorSupports Ethernet cable diameters between 5-8mm in diameterStatus LEDReset buttonWarrantyWarranty (MV21) 3 year hardware warranty with advanced replacementWarranty (MV71) 3 year hardware warranty with advanced replacementOrdering InformationMV21-HW: Meraki MV21 Cloud Managed Indoor CameraMV71-HW: Meraki MV71 Cloud Managed Outdoor CameraLIC-MV-XYR: Meraki MV Enterprise License (X = 1, 3, 5, 7, 10 years)MA-INJ-4-XX: Meraki 802.3at Power over Ethernet injector (XX = US, EU, UK, or AU) Note: Each Meraki camera requires a license to operate* Storage duration dependent on encoding settings.** Browser support for H.264 decoding required.Mounting Accessories Specifications Meraki Wall Mount ArmWall mount for attaching camera perpendicular to mounting surfaceIncludes pendant capSupported Models: MV21, MV71Dimensions (Wall Arm) 140mm x 244mm x 225.4mmDimensions (Pendant Cap) 179.9mm x 49.9mm (Diameter x Height)Combined Weight 1.64kgMeraki Pole MountPole mount for poles with diameter between 40mm - 145mm (1.57in - 5.71in)Can be combined with MA-MNT-MV-1: Meraki Wall Mount ArmSupported Models: MV71Dimensions 156.7mm x 240mm x 68.9mmWeight 1.106kgMeraki L-Shape Wall Mount BracketCompact wall mount for attaching camera perpendicular to mounting surfaceSupported Models: MV21, MV71Dimensions 206mm x 182mm x 110mmWeight 0.917kgOrdering InformationMA-MNT-MV-1: Meraki Wall Mount Arm for MV21 and MV71MA-MNT-MV-2: Meraki Pole Mount for MV71MA-MNT-MV-3: Meraki L-Shape Wall Mount Bracket for MV21 and MV71。
英文教材《Environmental Hydraulics for Open Channel Flows》List-of-Symbols_

∫
0
design upstream head (m) residual head (m) upstream total head (m) downstream total head (m) local total head (m) defined as:
Hϭ
2 P V ϩzϩ g 2g
h
specific enthalpy (i.e. enthalpy per unit mass) (J/kg): h ϭ e ϩ
List of symbols
A As B Bmax Bmin C Ca CChézy CD CL Cd Cdes Co Cp Cs (Cs)mean Csound Cv D DH
Cs
ቤተ መጻሕፍቲ ባይዱ
Ds Dt D1, D2 d dab db dc dcharac dconj do
flow cross-sectional area (m2) particle cross-sectional area (m2) open channel free-surface width (m) inlet lip width (m) of MEL culvert (1) minimum channel width (m) for onset of choking flow (2) barrel width (m) of a culvert (1) celerity (m/s): e.g. celerity of sound in a medium, celerity of a small disturbance at a free surface (2) dimensional discharge coefficient Cauchy number Chézy coefficient (m1/2/s) dimensionless discharge coefficient (SI units) lift coefficient (1) skin friction coefficient (also called drag coefficient) (2) drag coefficient design discharge coefficient (SI units) initial celerity (m/s) of a small disturbance ∂h specific heat at constant pressure (J/kg/K): C p ϭ ∂T P mean volumetric sediment concentration mean sediment suspension concentration sound celerity (m/s) specific heat at constant volume (J/kg/K) sediment concentration circular pipe diameter (m) hydraulic diameter (m), or equivalent pipe diameter, defined as: cross -sectional area 4A DH ϭ 4 ϭ wetted perimeter Pw 2 sediment diffusivity (m /s) diffusion coefficient (m2/s) characteristics of velocity distribution in turbulent boundary layer flow depth (m) measured perpendicular to the channel bed air bubble diameter (m) brink depth (m) critical flow depth (m) characteristic geometric length (m) conjugate flow depth (m) (1) uniform equilibrium flow depth (m): i.e. normal depth (2) initial flow depth (m)
A survey of content based 3d shape retrieval methods

A Survey of Content Based3D Shape Retrieval MethodsJohan W.H.Tangelder and Remco C.VeltkampInstitute of Information and Computing Sciences,Utrecht University hanst@cs.uu.nl,Remco.Veltkamp@cs.uu.nlAbstractRecent developments in techniques for modeling,digitiz-ing and visualizing3D shapes has led to an explosion in the number of available3D models on the Internet and in domain-specific databases.This has led to the development of3D shape retrieval systems that,given a query object, retrieve similar3D objects.For visualization,3D shapes are often represented as a surface,in particular polygo-nal meshes,for example in VRML format.Often these mod-els contain holes,intersecting polygons,are not manifold, and do not enclose a volume unambiguously.On the con-trary,3D volume models,such as solid models produced by CAD systems,or voxels models,enclose a volume prop-erly.This paper surveys the literature on methods for con-tent based3D retrieval,taking into account the applicabil-ity to surface models as well as to volume models.The meth-ods are evaluated with respect to several requirements of content based3D shape retrieval,such as:(1)shape repre-sentation requirements,(2)properties of dissimilarity mea-sures,(3)efficiency,(4)discrimination abilities,(5)ability to perform partial matching,(6)robustness,and(7)neces-sity of pose normalization.Finally,the advantages and lim-its of the several approaches in content based3D shape re-trieval are discussed.1.IntroductionThe advancement of modeling,digitizing and visualizing techniques for3D shapes has led to an increasing amount of3D models,both on the Internet and in domain-specific databases.This has led to the development of thefirst exper-imental search engines for3D shapes,such as the3D model search engine at Princeton university[2,57],the3D model retrieval system at the National Taiwan University[1,17], the Ogden IV system at the National Institute of Multimedia Education,Japan[62,77],the3D retrieval engine at Utrecht University[4,78],and the3D model similarity search en-gine at the University of Konstanz[3,84].Laser scanning has been applied to obtain archives recording cultural heritage like the Digital Michelan-gelo Project[25,48],and the Stanford Digital Formae Urbis Romae Project[75].Furthermore,archives contain-ing domain-specific shape models are now accessible by the Internet.Examples are the National Design Repos-itory,an online repository of CAD models[59,68], and the Protein Data Bank,an online archive of struc-tural data of biological macromolecules[10,80].Unlike text documents,3D models are not easily re-trieved.Attempting tofind a3D model using textual an-notation and a conventional text-based search engine would not work in many cases.The annotations added by human beings depend on language,culture,age,sex,and other fac-tors.They may be too limited or ambiguous.In contrast, content based3D shape retrieval methods,that use shape properties of the3D models to search for similar models, work better than text based methods[58].Matching is the process of determining how similar two shapes are.This is often done by computing a distance.A complementary process is indexing.In this paper,indexing is understood as the process of building a datastructure to speed up the search.Note that the term indexing is also of-ten used for the identification of features in models,or mul-timedia documents in general.Retrieval is the process of searching and delivering the query results.Matching and in-dexing are often part of the retrieval process.Recently,a lot of researchers have investigated the spe-cific problem of content based3D shape retrieval.Also,an extensive amount of literature can be found in the related fields of computer vision,object recognition and geomet-ric modelling.Survey papers to this literature have been provided by Besl and Jain[11],Loncaric[50]and Camp-bell and Flynn[16].For an overview of2D shape match-ing methods we refer the reader to the paper by Veltkamp [82].Unfortunately,most2D methods do not generalize di-rectly to3D model matching.Work in progress by Iyer et al.[40]provides an extensive overview of3D shape search-ing techniques.Atmosukarto and Naval[6]describe a num-ber of3D model retrieval systems and methods,but do not provide a categorization and evaluation.In contrast,this paper evaluates3D shape retrieval meth-ods with respect to several requirements on content based 3D shape retrieval,such as:(1)shape representation re-quirements,(2)properties of dissimilarity measures,(3)ef-ficiency,(4)discrimination abilities,(5)ability to perform partial matching,(6)robustness,and(7)necessity of posenormalization.In section2we discuss several aspects of3D shape retrieval.The literature on3D shape matching meth-ods is discussed in section3and evaluated in section4. 2.3D shape retrieval aspectsIn this section we discuss several issues related to3D shape retrieval.2.1.3D shape retrieval frameworkAt a conceptual level,a typical3D shape retrieval frame-work as illustrated byfig.1consists of a database with an index structure created offline and an online query engine. Each3D model has to be identified with a shape descrip-tor,providing a compact overall description of the shape. To efficiently search a large collection online,an indexing data structure and searching algorithm should be available. The online query engine computes the query descriptor,and models similar to the query model are retrieved by match-ing descriptors to the query descriptor from the index struc-ture of the database.The similarity between two descriptors is quantified by a dissimilarity measure.Three approaches can be distinguished to provide a query object:(1)browsing to select a new query object from the obtained results,(2) a direct query by providing a query descriptor,(3)query by example by providing an existing3D model or by creating a3D shape query from scratch using a3D tool or sketch-ing2D projections of the3D model.Finally,the retrieved models can be visualized.2.2.Shape representationsAn important issue is the type of shape representation(s) that a shape retrieval system accepts.Most of the3D models found on the World Wide Web are meshes defined in afile format supporting visual appearance.Currently,the most common format used for this purpose is the Virtual Real-ity Modeling Language(VRML)format.Since these mod-els have been designed for visualization,they often contain only geometry and appearance attributes.In particular,they are represented by“polygon soups”,consisting of unorga-nized sets of polygons.Also,in general these models are not“watertight”meshes,i.e.they do not enclose a volume. By contrast,for volume models retrieval methods depend-ing on a properly defined volume can be applied.2.3.Measuring similarityIn order to measure how similar two objects are,it is nec-essary to compute distances between pairs of descriptors us-ing a dissimilarity measure.Although the term similarity is often used,dissimilarity corresponds to the notion of dis-tance:small distances means small dissimilarity,and large similarity.A dissimilarity measure can be formalized by a func-tion defined on pairs of descriptors indicating the degree of their resemblance.Formally speaking,a dissimilarity measure d on a set S is a non-negative valued function d:S×S→R+∪{0}.Function d may have some of the following properties:i.Identity:For all x∈S,d(x,x)=0.ii.Positivity:For all x=y in S,d(x,y)>0.iii.Symmetry:For all x,y∈S,d(x,y)=d(y,x).iv.Triangle inequality:For all x,y,z∈S,d(x,z)≤d(x,y)+d(y,z).v.Transformation invariance:For a chosen transforma-tion group G,for all x,y∈S,g∈G,d(g(x),g(y))= d(x,y).The identity property says that a shape is completely similar to itself,while the positivity property claims that dif-ferent shapes are never completely similar.This property is very strong for a high-level shape descriptor,and is often not satisfied.However,this is not a severe drawback,if the loss of uniqueness depends on negligible details.Symmetry is not always wanted.Indeed,human percep-tion does not alwaysfind that shape x is equally similar to shape y,as y is to x.In particular,a variant x of prototype y,is often found more similar to y then vice versa[81].Dissimilarity measures for partial matching,giving a small distance d(x,y)if a part of x matches a part of y, do not obey the triangle inequality.Transformation invariance has to be satisfied,if the com-parison and the extraction process of shape descriptors have to be independent of the place,orientation and scale of the object in its Cartesian coordinate system.If we want that a dissimilarity measure is not affected by any transforma-tion on x,then we may use as alternative formulation for (v):Transformation invariance:For a chosen transforma-tion group G,for all x,y∈S,g∈G,d(g(x),y)=d(x,y).When all the properties(i)-(iv)hold,the dissimilarity measure is called a metric.Other combinations are possi-ble:a pseudo-metric is a dissimilarity measure that obeys (i),(iii)and(iv)while a semi-metric obeys only(i),(ii)and(iii).If a dissimilarity measure is a pseudo-metric,the tri-angle inequality can be applied to make retrieval more effi-cient[7,83].2.4.EfficiencyFor large shape collections,it is inefficient to sequen-tially match all objects in the database with the query object. Because retrieval should be fast,efficient indexing search structures are needed to support efficient retrieval.Since for query by example the shape descriptor is computed online, it is reasonable to require that the shape descriptor compu-tation is fast enough for interactive querying.2.5.Discriminative powerA shape descriptor should capture properties that dis-criminate objects well.However,the judgement of the sim-ilarity of the shapes of two3D objects is somewhat sub-jective,depending on the user preference or the application at hand.E.g.for solid modeling applications often topol-ogy properties such as the numbers of holes in a model are more important than minor differences in shapes.On the contrary,if a user searches for models looking visually sim-ilar the existence of a small hole in the model,may be of no importance to the user.2.6.Partial matchingIn contrast to global shape matching,partial matching finds a shape of which a part is similar to a part of another shape.Partial matching can be applied if3D shape mod-els are not complete,e.g.for objects obtained by laser scan-ning from one or two directions only.Another application is the search for“3D scenes”containing an instance of the query object.Also,this feature can potentially give the user flexibility towards the matching problem,if parts of inter-est of an object can be selected or weighted by the user. 2.7.RobustnessIt is often desirable that a shape descriptor is insensitive to noise and small extra features,and robust against arbi-trary topological degeneracies,e.g.if it is obtained by laser scanning.Also,if a model is given in multiple levels-of-detail,representations of different levels should not differ significantly from the original model.2.8.Pose normalizationIn the absence of prior knowledge,3D models have ar-bitrary scale,orientation and position in the3D space.Be-cause not all dissimilarity measures are invariant under ro-tation and translation,it may be necessary to place the3D models into a canonical coordinate system.This should be the same for a translated,rotated or scaled copy of the model.A natural choice is tofirst translate the center to the ori-gin.For volume models it is natural to translate the cen-ter of mass to the origin.But for meshes this is in gen-eral not possible,because they have not to enclose a vol-ume.For meshes it is an alternative to translate the cen-ter of mass of all the faces to the origin.For example the Principal Component Analysis(PCA)method computes for each model the principal axes of inertia e1,e2and e3 and their eigenvaluesλ1,λ2andλ3,and make the nec-essary conditions to get right-handed coordinate systems. These principal axes define an orthogonal coordinate sys-tem(e1,e2,e3),withλ1≥λ2≥λ3.Next,the polyhe-dral model is rotated around the origin such that the co-ordinate system(e x,e y,e z)coincides with the coordinatesystem(e1,e2,e3).The PCA algorithm for pose estimation is fairly simple and efficient.However,if the eigenvalues are equal,prin-cipal axes may switch,without affecting the eigenvalues. Similar eigenvalues may imply an almost symmetrical mass distribution around an axis(e.g.nearly cylindrical shapes) or around the center of mass(e.g.nearly spherical shapes). Fig.2illustrates the problem.3.Shape matching methodsIn this section we discuss3D shape matching methods. We divide shape matching methods in three broad cate-gories:(1)feature based methods,(2)graph based meth-ods and(3)other methods.Fig.3illustrates a more detailed categorization of shape matching methods.Note,that the classes of these methods are not completely disjoined.For instance,a graph-based shape descriptor,in some way,de-scribes also the global feature distribution.By this point of view the taxonomy should be a graph.3.1.Feature based methodsIn the context of3D shape matching,features denote ge-ometric and topological properties of3D shapes.So3D shapes can be discriminated by measuring and comparing their features.Feature based methods can be divided into four categories according to the type of shape features used: (1)global features,(2)global feature distributions,(3)spa-tial maps,and(4)local features.Feature based methods from thefirst three categories represent features of a shape using a single descriptor consisting of a d-dimensional vec-tor of values,where the dimension d isfixed for all shapes.The value of d can easily be a few hundred.The descriptor of a shape is a point in a high dimensional space,and two shapes are considered to be similar if they are close in this space.Retrieving the k best matches for a3D query model is equivalent to solving the k nearest neighbors -ing the Euclidean distance,matching feature descriptors can be done efficiently in practice by searching in multiple1D spaces to solve the approximate k nearest neighbor prob-lem as shown by Indyk and Motwani[36].In contrast with the feature based methods from thefirst three categories,lo-cal feature based methods describe for a number of surface points the3D shape around the point.For this purpose,for each surface point a descriptor is used instead of a single de-scriptor.3.1.1.Global feature based similarityGlobal features characterize the global shape of a3D model. Examples of these features are the statistical moments of the boundary or the volume of the model,volume-to-surface ra-tio,or the Fourier transform of the volume or the boundary of the shape.Zhang and Chen[88]describe methods to com-pute global features such as volume,area,statistical mo-ments,and Fourier transform coefficients efficiently.Paquet et al.[67]apply bounding boxes,cords-based, moments-based and wavelets-based descriptors for3D shape matching.Corney et al.[21]introduce convex-hull based indices like hull crumpliness(the ratio of the object surface area and the surface area of its convex hull),hull packing(the percentage of the convex hull volume not occupied by the object),and hull compactness(the ratio of the cubed sur-face area of the hull and the squared volume of the convex hull).Kazhdan et al.[42]describe a reflective symmetry de-scriptor as a2D function associating a measure of reflec-tive symmetry to every plane(specified by2parameters) through the model’s centroid.Every function value provides a measure of global shape,where peaks correspond to the planes near reflective symmetry,and valleys correspond to the planes of near anti-symmetry.Their experimental results show that the combination of the reflective symmetry de-scriptor with existing methods provides better results.Since only global features are used to characterize the overall shape of the objects,these methods are not very dis-criminative about object details,but their implementation is straightforward.Therefore,these methods can be used as an activefilter,after which more detailed comparisons can be made,or they can be used in combination with other meth-ods to improve results.Global feature methods are able to support user feed-back as illustrated by the following research.Zhang and Chen[89]applied features such as volume-surface ratio, moment invariants and Fourier transform coefficients for 3D shape retrieval.They improve the retrieval performance by an active learning phase in which a human annotator as-signs attributes such as airplane,car,body,and so on to a number of sample models.Elad et al.[28]use a moments-based classifier and a weighted Euclidean distance measure. Their method supports iterative and interactive database searching where the user can improve the weights of the distance measure by marking relevant search results.3.1.2.Global feature distribution based similarityThe concept of global feature based similarity has been re-fined recently by comparing distributions of global features instead of the global features directly.Osada et al.[66]introduce and compare shape distribu-tions,which measure properties based on distance,angle, area and volume measurements between random surface points.They evaluate the similarity between the objects us-ing a pseudo-metric that measures distances between distri-butions.In their experiments the D2shape distribution mea-suring distances between random surface points is most ef-fective.Ohbuchi et al.[64]investigate shape histograms that are discretely parameterized along the principal axes of inertia of the model.The shape descriptor consists of three shape histograms:(1)the moment of inertia about the axis,(2) the average distance from the surface to the axis,and(3) the variance of the distance from the surface to the axis. Their experiments show that the axis-parameterized shape features work only well for shapes having some form of ro-tational symmetry.Ip et al.[37]investigate the application of shape distri-butions in the context of CAD and solid modeling.They re-fined Osada’s D2shape distribution function by classifying2random points as1)IN distances if the line segment con-necting the points lies complete inside the model,2)OUT distances if the line segment connecting the points lies com-plete outside the model,3)MIXED distances if the line seg-ment connecting the points lies passes both inside and out-side the model.Their dissimilarity measure is a weighted distance measure comparing D2,IN,OUT and MIXED dis-tributions.Since their method requires that a line segment can be classified as lying inside or outside the model it is required that the model defines a volume properly.There-fore it can be applied to volume models,but not to polyg-onal soups.Recently,Ip et al.[38]extend this approach with a technique to automatically categorize a large model database,given a categorization on a number of training ex-amples from the database.Ohbuchi et al.[63],investigate another extension of the D2shape distribution function,called the Absolute Angle-Distance histogram,parameterized by a parameter denot-ing the distance between two random points and by a pa-rameter denoting the angle between the surfaces on which two random points are located.The latter parameter is ac-tually computed as an inner product of the surface normal vectors.In their evaluation experiment this shape distribu-tion function outperformed the D2distribution function at about1.5times higher computational costs.Ohbuchi et al.[65]improved this method further by a multi-resolution ap-proach computing a number of alpha-shapes at different scales,and computing for each alpha-shape their Absolute Angle-Distance descriptor.Their experimental results show that this approach outperforms the Angle-Distance descrip-tor at the cost of high processing time needed to compute the alpha-shapes.Shape distributions distinguish models in broad cate-gories very well:aircraft,boats,people,animals,etc.How-ever,they perform often poorly when having to discrimi-nate between shapes that have similar gross shape proper-ties but vastly different detailed shape properties.3.1.3.Spatial map based similaritySpatial maps are representations that capture the spatial lo-cation of an object.The map entries correspond to physi-cal locations or sections of the object,and are arranged in a manner that preserves the relative positions of the features in an object.Spatial maps are in general not invariant to ro-tations,except for specially designed maps.Therefore,typ-ically a pose normalization is donefirst.Ankerst et al.[5]use shape histograms as a means of an-alyzing the similarity of3D molecular surfaces.The his-tograms are not built from volume elements but from uni-formly distributed surface points taken from the molecular surfaces.The shape histograms are defined on concentric shells and sectors around a model’s centroid and compare shapes using a quadratic form distance measure to compare the histograms taking into account the distances between the shape histogram bins.Vrani´c et al.[85]describe a surface by associating to each ray from the origin,the value equal to the distance to the last point of intersection of the model with the ray and compute spherical harmonics for this spherical extent func-tion.Spherical harmonics form a Fourier basis on a sphere much like the familiar sine and cosine do on a line or a cir-cle.Their method requires pose normalization to provide rotational invariance.Also,Yu et al.[86]propose a descrip-tor similar to a spherical extent function and a descriptor counting the number of intersections of a ray from the ori-gin with the model.In both cases the dissimilarity between two shapes is computed by the Euclidean distance of the Fourier transforms of the descriptors of the shapes.Their method requires pose normalization to provide rotational in-variance.Kazhdan et al.[43]present a general approach based on spherical harmonics to transform rotation dependent shape descriptors into rotation independent ones.Their method is applicable to a shape descriptor which is defined as either a collection of spherical functions or as a function on a voxel grid.In the latter case a collection of spherical functions is obtained from the function on the voxel grid by restricting the grid to concentric spheres.From the collection of spher-ical functions they compute a rotation invariant descriptor by(1)decomposing the function into its spherical harmon-ics,(2)summing the harmonics within each frequency,and computing the L2-norm for each frequency component.The resulting shape descriptor is a2D histogram indexed by ra-dius and frequency,which is invariant to rotations about the center of the mass.This approach offers an alternative for pose normalization,because their method obtains rotation invariant shape descriptors.Their experimental results show indeed that in general the performance of the obtained ro-tation independent shape descriptors is better than the cor-responding normalized descriptors.Their experiments in-clude the ray-based spherical harmonic descriptor proposed by Vrani´c et al.[85].Finally,note that their approach gen-eralizes the method to compute voxel-based spherical har-monics shape descriptor,described by Funkhouser et al.[30],which is defined as a binary function on the voxel grid, where the value at each voxel is given by the negatively ex-ponentiated Euclidean Distance Transform of the surface of a3D model.Novotni and Klein[61]present a method to compute 3D Zernike descriptors from voxelized models as natural extensions of spherical harmonics based descriptors.3D Zernike descriptors capture object coherence in the radial direction as well as in the direction along a sphere.Both 3D Zernike descriptors and spherical harmonics based de-scriptors achieve rotation invariance.However,by sampling the space only in radial direction the latter descriptors donot capture object coherence in the radial direction,as illus-trated byfig.4.The limited experiments comparing spherical harmonics and3D Zernike moments performed by Novotni and Klein show similar results for a class of planes,but better results for the3D Zernike descriptor for a class of chairs.Vrani´c[84]expects that voxelization is not a good idea, because manyfine details are lost in the voxel grid.There-fore,he compares his ray-based spherical harmonic method [85]and a variation of it using functions defined on concen-tric shells with the voxel-based spherical harmonics shape descriptor proposed by Funkhouser et al.[30].Also,Vrani´c et al.[85]accomplish pose normalization using the so-called continuous PCA algorithm.In the paper it is claimed that the continuous PCA is better as the conventional PCA and better as the weighted PCA,which takes into account the differing sizes of the triangles of a mesh.In contrast with Kazhdan’s experiments[43]the experiments by Vrani´c show that for ray-based spherical harmonics using the con-tinuous PCA without voxelization is better than using rota-tion invariant shape descriptors obtained using voxelization. Perhaps,these results are opposite to Kazhdan results,be-cause of the use of different methods to compute the PCA or the use of different databases or both.Kriegel et al.[46,47]investigate similarity for voxelized models.They obtain a spatial map by partitioning a voxel grid into disjoint cells which correspond to the histograms bins.They investigate three different spatial features asso-ciated with the grid cells:(1)volume features recording the fraction of voxels from the volume in each cell,(2) solid-angle features measuring the convexity of the volume boundary in each cell,(3)eigenvalue features estimating the eigenvalues obtained by the PCA applied to the voxels of the model in each cell[47],and a fourth method,using in-stead of grid cells,a moreflexible partition of the voxels by cover sequence features,which approximate the model by unions and differences of cuboids,each containing a number of voxels[46].Their experimental results show that the eigenvalue method and the cover sequence method out-perform the volume and solid-angle feature method.Their method requires pose normalization to provide rotational in-variance.Instead of representing a cover sequence with a single feature vector,Kriegel et al.[46]represent a cover sequence by a set of feature vectors.This approach allows an efficient comparison of two cover sequences,by compar-ing the two sets of feature vectors using a minimal match-ing distance.The spatial map based approaches show good retrieval results.But a drawback of these methods is that partial matching is not supported,because they do not encode the relation between the features and parts of an object.Fur-ther,these methods provide no feedback to the user about why shapes match.3.1.4.Local feature based similarityLocal feature based methods provide various approaches to take into account the surface shape in the neighbourhood of points on the boundary of the shape.Shum et al.[74]use a spherical coordinate system to map the surface curvature of3D objects to the unit sphere. By searching over a spherical rotation space a distance be-tween two curvature distributions is computed and used as a measure for the similarity of two objects.Unfortunately, the method is limited to objects which contain no holes, i.e.have genus zero.Zaharia and Prˆe teux[87]describe the 3D Shape Spectrum Descriptor,which is defined as the histogram of shape index values,calculated over an en-tire mesh.The shape index,first introduced by Koenderink [44],is defined as a function of the two principal curvatures on continuous surfaces.They present a method to compute these shape indices for meshes,byfitting a quadric surface through the centroids of the faces of a mesh.Unfortunately, their method requires a non-trivial preprocessing phase for meshes that are not topologically correct or not orientable.Chua and Jarvis[18]compute point signatures that accu-mulate surface information along a3D curve in the neigh-bourhood of a point.Johnson and Herbert[41]apply spin images that are2D histograms of the surface locations around a point.They apply spin images to recognize models in a cluttered3D scene.Due to the complexity of their rep-resentation[18,41]these methods are very difficult to ap-ply to3D shape matching.Also,it is not clear how to define a dissimilarity function that satisfies the triangle inequality.K¨o rtgen et al.[45]apply3D shape contexts for3D shape retrieval and matching.3D shape contexts are semi-local descriptions of object shape centered at points on the sur-face of the object,and are a natural extension of2D shape contexts introduced by Belongie et al.[9]for recognition in2D images.The shape context of a point p,is defined as a coarse histogram of the relative coordinates of the re-maining surface points.The bins of the histogram are de-。
基于机器学习的旋转机械故障诊断方法的研究
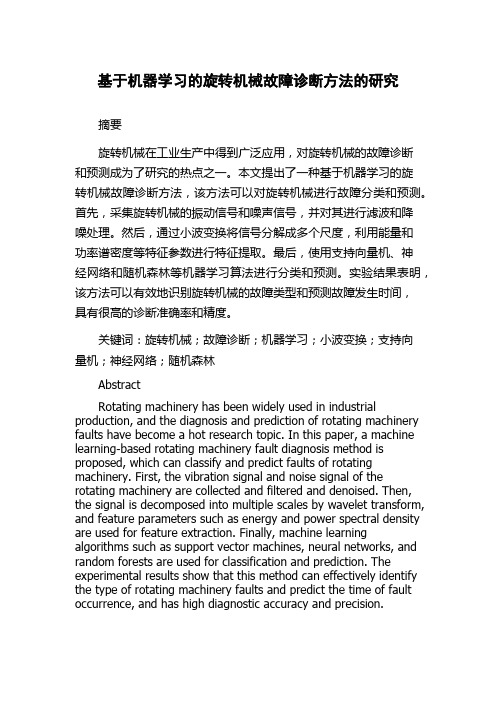
基于机器学习的旋转机械故障诊断方法的研究摘要旋转机械在工业生产中得到广泛应用,对旋转机械的故障诊断和预测成为了研究的热点之一。
本文提出了一种基于机器学习的旋转机械故障诊断方法,该方法可以对旋转机械进行故障分类和预测。
首先,采集旋转机械的振动信号和噪声信号,并对其进行滤波和降噪处理。
然后,通过小波变换将信号分解成多个尺度,利用能量和功率谱密度等特征参数进行特征提取。
最后,使用支持向量机、神经网络和随机森林等机器学习算法进行分类和预测。
实验结果表明,该方法可以有效地识别旋转机械的故障类型和预测故障发生时间,具有很高的诊断准确率和精度。
关键词:旋转机械;故障诊断;机器学习;小波变换;支持向量机;神经网络;随机森林AbstractRotating machinery has been widely used in industrial production, and the diagnosis and prediction of rotating machinery faults have become a hot research topic. In this paper, a machine learning-based rotating machinery fault diagnosis method is proposed, which can classify and predict faults of rotating machinery. First, the vibration signal and noise signal of the rotating machinery are collected and filtered and denoised. Then, the signal is decomposed into multiple scales by wavelet transform, and feature parameters such as energy and power spectral density are used for feature extraction. Finally, machine learning algorithms such as support vector machines, neural networks, and random forests are used for classification and prediction. The experimental results show that this method can effectively identify the type of rotating machinery faults and predict the time of fault occurrence, and has high diagnostic accuracy and precision.Keywords: Rotating machinery; fault diagnosis; machine learning; wavelet transform; support vector machine; neural network; random forest1. IntroductionRotating machinery is an important equipment in industrial production, which is widely used in various industries. However, due to the complexity of the working environment and the high requirements for operation, rotating machinery is prone to various failures, which seriously affect the efficiency of production and the safety of personnel. Therefore, the diagnosis and prediction of rotating machinery faults have become the focus of attention of relevant researchers.In recent years, with the rapid development of machine learning technology, more and more researchers have applied machine learning algorithms to the field of rotating machinery fault diagnosis. Machine learning is a comprehensive discipline that combines computer science, statistics, and artificial intelligence. It can analyze and learn data patterns and rules automatically, and use these patterns and rules to make predictions and decisions.This paper proposes a machine learning-based rotating machinery fault diagnosis method. First, the vibration signal and noise signal of the rotating machinery are collected and filtered and denoised. Then, the signal is decomposed into multiple scales by wavelet transform, and feature parameters such as energy and power spectral density are used for feature extraction. Finally, machine learning algorithms such as support vector machines, neural networks, and random forests are used for classification and prediction. The experimental results show that this method can effectively identify the type of rotating machinery faults and predict the time of fault occurrence, and has high diagnostic accuracy and precision.2. Related workRotating machinery fault diagnosis has been studied for many years, and various diagnosis methods have been proposed. Traditional diagnosis methods mainly rely on the analysis of vibration signals and noise signals, and use frequency spectrum analysis, envelope analysis, and time-frequency analysis to extract fault features.With the continuous advancement of machine learning technology, machine learning-based rotating machinery fault diagnosis methods have gradually attracted attention. For example, Bai et al. [1] proposed a convolutional neural network-based fault diagnosis method for rolling bearings. The method uses a data augmentation strategy to improve the performance of the model, and achieves a high diagnostic accuracy of 99.8%.Liu et al. [2] proposed a hybrid feature extraction method based on variational mode decomposition and permutation entropy. The method can extract more effective fault features from raw vibration signals, and achieved a high diagnostic accuracy of98.5%.Zheng et al. [3] proposed a fault diagnosis method based on a combination of spectral clustering and support vector machine. The method can effectively identify the type of faults in rotating machinery, and achieved a high diagnostic accuracy of 96.3%.3. Methodology3.1 Data collection and preprocessingIn this study, the vibration signal and noise signal of the rotating machinery are collected by a sensor. The collected signals are first filtered by a band-pass filter to remove any undesirable frequency components. Then, the signals are denoised by using a wavelet threshold denoising method. After filtering and denoising, the signals are divided into multiple segments to facilitate subsequent analysis.3.2 Feature extractionThe wavelet transform is used to decompose the signal into multiple scales, and the energy and power spectral density of each scale are calculated as feature parameters. Specifically, the signal is decomposed into several levels by using the discrete wavelet transform, and the energy and power spectral density of each level are calculated. Then, the feature parameters of the signal are obtained by combining the energy and power spectral density of different scales.3.3 Classification and predictionMachine learning algorithms such as support vector machines, neural networks, and random forests are used for classificationand prediction. Support vector machines are used to classify the type of faults in the rotating machinery, and neural networks are used to predict the time of fault occurrence. Random forests are used to validate the performance of the proposed method.4. ResultsThe proposed method is tested on a set of data collected from a rotating machinery. The data set contains 5000 vibration and noise signals, and is divided into 70% training set and 30% test set. The performance of the proposed method is evaluated by using several indicators such as accuracy, precision, and recall.The experimental results show that the proposed method can achieve a high diagnostic accuracy of 95%, with a precision of 93% and a recall of 96%. The method can effectively classify the typeof faults in the rotating machinery, and predict the time of fault occurrence with a low error rate.5. ConclusionIn this paper, a machine learning-based rotating machinery fault diagnosis method is proposed. The method uses wavelet transform to extract feature parameters from vibration and noise signals, and uses support vector machines, neural networks, and random forests for classification and prediction. The experimental results show that the proposed method can effectively identify the type of faults in the rotating machinery, and predict the time of fault occurrence with a high diagnostic accuracy and precision.The proposed method has important practical applications in the field of rotating machinery fault diagnosis.。
HSfMHybridStructure-from-Motion《学习笔记》
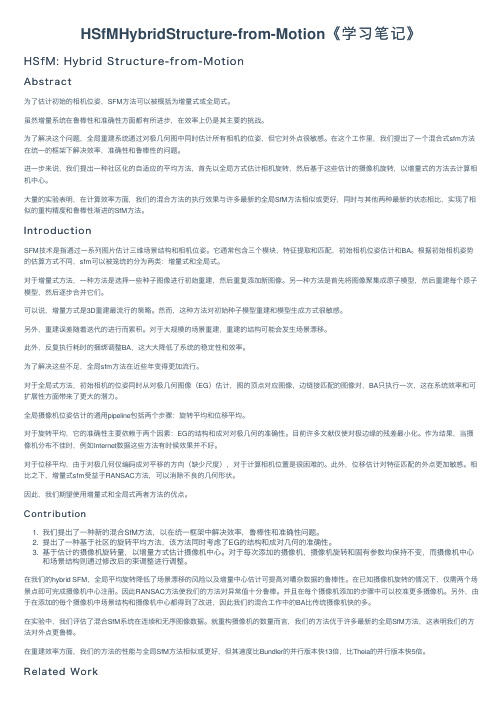
HSfMHybridStructure-from-Motion《学习笔记》HSfM: Hybrid Structure-from-MotionAbstr a c t为了估计初始的相机位姿,SFM⽅法可以被概括为增量式或全局式。
虽然增量系统在鲁棒性和准确性⽅⾯都有所进步,在效率上仍是其主要的挑战。
为了解决这个问题,全局重建系统通过对极⼏何图中同时估计所有相机的位姿,但它对外点很敏感。
在这个⼯作⾥,我们提出了⼀个混合式sfm⽅法在统⼀的框架下解决效率,准确性和鲁棒性的问题。
进⼀步来说,我们提出⼀种社区化的⾃适应的平均⽅法,⾸先以全局⽅式估计相机旋转,然后基于这些估计的摄像机旋转,以增量式的⽅法去计算相机中⼼。
⼤量的实验表明,在计算效率⽅⾯,我们的混合⽅法的执⾏效果与许多最新的全局SfM⽅法相似或更好,同时与其他两种最新的状态相⽐,实现了相似的重构精度和鲁棒性渐进的SfM⽅法。
Intro duc tio nSFM技术是指通过⼀系列图⽚估计三维场景结构和相机位姿。
它通常包含三个模块,特征提取和匹配,初始相机位姿估计和BA。
根据初始相机姿势的估算⽅式不同,sfm可以被笼统的分为两类:增量式和全局式。
对于增量式⽅法,⼀种⽅法是选择⼀些种⼦图像进⾏初始重建,然后重复添加新图像。
另⼀种⽅法是⾸先将图像聚集成原⼦模型,然后重建每个原⼦模型,然后逐步合并它们。
可以说,增量⽅式是3D重建最流⾏的策略。
然⽽,这种⽅法对初始种⼦模型重建和模型⽣成⽅式很敏感。
另外,重建误差随着迭代的进⾏⽽累积。
对于⼤规模的场景重建,重建的结构可能会发⽣场景漂移。
此外,反复执⾏耗时的捆绑调整BA,这⼤⼤降低了系统的稳定性和效率。
为了解决这些不⾜,全局sfm⽅法在近些年变得更加流⾏。
对于全局式⽅法,初始相机的位姿同时从对极⼏何图像(EG)估计,图的顶点对应图像,边链接匹配的图像对,BA只执⾏⼀次,这在系统效率和可扩展性⽅⾯带来了更⼤的潜⼒。
全局摄像机位姿估计的通⽤pipeline包括两个步骤:旋转平均和位移平均。
基于gg模糊聚类的滚动轴承退化阶段划分研究
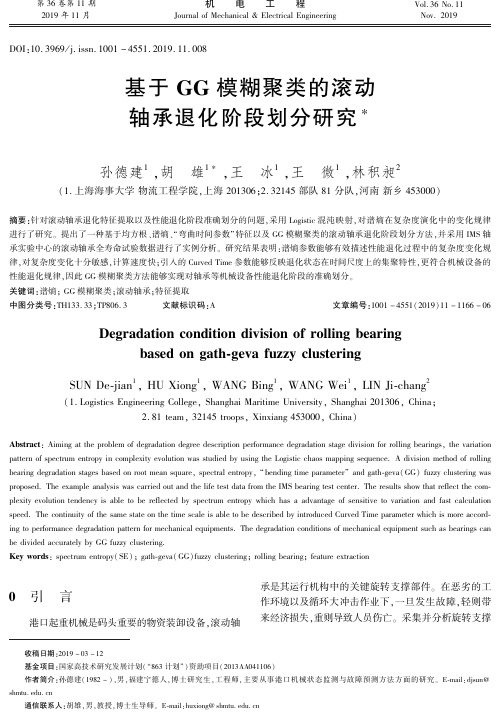
第36卷第11期2019年11月机㊀㊀电㊀㊀工㊀㊀程JournalofMechanical&ElectricalEngineeringVol.36No.11Nov.2019收稿日期:2019-03-12基金项目:国家高技术研究发展计划( 863计划 )资助项目(2013AA041106)作者简介:孙德建(1982-)ꎬ男ꎬ福建宁德人ꎬ博士研究生ꎬ工程师ꎬ主要从事港口机械状态监测与故障预测方法方面的研究ꎮE ̄mail:djsun@shmtu.edu.cn通信联系人:胡雄ꎬ男ꎬ教授ꎬ博士生导师ꎮE ̄mail:huxiong@shmtu.edu.cnDOI:10.3969/j.issn.1001-4551.2019.11.008基于GG模糊聚类的滚动轴承退化阶段划分研究∗孙德建1ꎬ胡㊀雄1∗ꎬ王㊀冰1ꎬ王㊀微1ꎬ林积昶2(1.上海海事大学物流工程学院ꎬ上海201306ꎻ2.32145部队81分队ꎬ河南新乡453000)摘要:针对滚动轴承退化特征提取以及性能退化阶段准确划分的问题ꎬ采用Logistic混沌映射ꎬ对谱熵在复杂度演化中的变化规律进行了研究ꎮ提出了一种基于均方根㊁谱熵㊁ 弯曲时间参数 特征以及GG模糊聚类的滚动轴承退化阶段划分方法ꎬ并采用IMS轴承实验中心的滚动轴承全寿命试验数据进行了实例分析ꎮ研究结果表明:谱熵参数能够有效描述性能退化过程中的复杂度变化规律ꎬ对复杂度变化十分敏感ꎬ计算速度快ꎻ引入的CurvedTime参数能够反映退化状态在时间尺度上的集聚特性ꎬ更符合机械设备的性能退化规律ꎬ因此GG模糊聚类方法能够实现对轴承等机械设备性能退化阶段的准确划分ꎮ关键词:谱熵ꎻGG模糊聚类ꎻ滚动轴承ꎻ特征提取中图分类号:TH133.33ꎻTP806.3㊀㊀㊀㊀文献标识码:A文章编号:1001-4551(2019)11-1166-06Degradationconditiondivisionofrollingbearingbasedongath ̄gevafuzzyclusteringSUNDe ̄jian1ꎬHUXiong1ꎬWANGBing1ꎬWANGWei1ꎬLINJi ̄chang2(1.LogisticsEngineeringCollegeꎬShanghaiMaritimeUniversityꎬShanghai201306ꎬChinaꎻ2.81teamꎬ32145troopsꎬXinxiang453000ꎬChina)Abstract:AimingattheproblemofdegradationdegreedescriptionperformancedegradationstagedivisionforrollingbearingsꎬthevariationpatternofspectrumentropyincomplexityevolutionwasstudiedbyusingtheLogisticchaosmappingsequence.Adivisionmethodofrollingbearingdegradationstagesbasedonrootmeansquareꎬspectralentropyꎬ bendingtimeparameter andgath ̄geva(GG)fuzzyclusteringwasproposed.TheexampleanalysiswascarriedoutandthelifetestdatafromtheIMSbearingtestcenter.Theresultsshowthatreflectthecom ̄plexityevolutiontendencyisabletobereflectedbyspectrumentropywhichhasaadvantageofsensitivetovariationandfastcalculationspeed.ThecontinuityofthesamestateonthetimescaleisabletobedescribedbyintroducedCurvedTimeparameterwhichismoreaccord ̄ingtoperformancedegradationpatternformechanicalequipments.ThedegradationconditionsofmechanicalequipmentsuchasbearingscanbedividedaccuratelybyGGfuzzyclustering.Keywords:spectrumentropy(SE)ꎻgath ̄geva(GG)fuzzyclusteringꎻrollingbearingꎻfeatureextraction0㊀引㊀言港口起重机械是码头重要的物资装卸设备ꎬ滚动轴承是其运行机构中的关键旋转支撑部件ꎮ在恶劣的工作环境以及循环大冲击作业下ꎬ一旦发生故障ꎬ轻则带来经济损失ꎬ重则导致人员伤亡ꎮ采集并分析旋转支撑部件的运行监测信号ꎬ准确识别其性能退化阶段ꎬ能够有效降低故障发生概率ꎮ在实际工况下ꎬ滚动轴承振动信号一般表现出非线性㊁非平稳㊁非周期的特点ꎬ需要针对性地分析其中蕴含的退化规律以及状态演化规律ꎮ退化特征提取是准确识别健康状态的基础[1]ꎮ目前该领域主要基于时域㊁频域以及时频域分析方法而展开研究ꎮ其中ꎬ时域和频域统计特征因计算简便快速而得到一定的应用ꎬ如信号的有效值㊁方差㊁平均频率等[2 ̄3]ꎬ但该类方法本质上基于线性信号分析方法ꎬ无法全面反映性能退化过程中的非线性规律ꎮ而针对工程振动信号非线性㊁非平稳性的特点ꎬ以信息熵为基础的复杂度分析方法为非线性退化规律挖掘提供了一条有效的思路ꎮ包括以模糊熵[4]㊁样本熵[5]㊁近似熵[6]为代表的行为复杂度和以谱熵㊁C0复杂度为代表的结构复杂度ꎮ研究表明:行为复杂度一般涉及嵌入维数的选取ꎬ结果稳定性不足ꎬ运算速度较慢ꎻ结构复杂度方法参数少ꎬ计算速度快ꎮ其中的谱熵以FFT变换为基础ꎬ不涉及中间参数ꎬ结果稳定ꎬ能够有效地分析短时㊁非平稳㊁有噪声干扰的数据[7]ꎮ文献[8]证明了SE和C0复杂度曲线能够正确有效地描述连续混沌系统的动力学特征ꎮ当前ꎬ谱熵算法主要应用在混沌系统的复杂性分析上ꎬ利用该算法分析机械设备性能退化规律的研究很少ꎬ开展基于谱熵的退化特征分析具有一定的研究空间ꎮ退化阶段的划分是健康状态评估中的难点问题ꎮ采用主观划分方法缺乏一定的科学性ꎮ而实际的特征数据演化进程中ꎬ同类状态的数据中蕴含着一定的集聚性ꎮ挖掘特征数据的内禀的关联性和集聚性ꎬ能够提高退化阶段划分的科学性ꎮ为促进现场在线状态评估奠定基础ꎬ以GG(gustafaon ̄kesselclustering)为代表的无监督聚类引入了模糊最大似然估计距离范数[9]ꎬ能够更精确地挖掘数据集中的聚类特性ꎮ目前关于GG算法的研究集中在故障模式的诊断ꎬ对性能退化状态的聚类则相对较少[10 ̄12]ꎮ综上ꎬ本文提出一种基于GG模糊聚类的滚动轴承退化阶段划分方法ꎬ提取振动信号的均方根㊁谱熵以及 弯曲时间参数 作为三维特征向量ꎬ以模糊聚类方法对性能退化状态进行无监督聚类ꎬ采用IMS的轴承全寿命试验数据对方法进行验证ꎮ1㊀基于谱熵的退化特征分析1.1㊀谱熵定义谱熵的主要思想是以傅里叶变换为基础ꎬ分析傅里叶变换得到的频域内的能量分布ꎬ并基于香农熵理论而得到描述信号复杂度的指标ꎮ该算法的基本流程如下[13]:(1)直流部分去除ꎮ假设x(n)为长度N的时间序列ꎬ利用下式去除掉信号的直流成分ꎬ从而可使频谱更准确地表征信号的能量ꎬ即:x(n)=x(n)-x(1)式中:x 信号的均值ꎬx=1NðN-1n=0x(n)ꎮ(2)对去除直流分量的信号进行离散傅里叶变换ꎬ即:X(k)=ðN-1n=0x(n)e-j2πNnk=ðN-1n=0x(n)WnkN(2) (3)相对功率谱计算ꎮ对经过离散处理后的频谱序列ꎬ取其前半部分进行计算ꎬ并利用Paserval算法ꎬ得到其中一个特定频率的功率谱大小ꎬ即:p(k)=1N|X(k)|2ꎬk=0ꎬ1ꎬ2ꎬ ꎬN/2-1(3)信号的总功率可定义为:Ptot=1NðN/2-1k=0|X(k)|2(4)相对功率谱的概率可表示为:Pk=P(k)Ptot=1N|X(k)|21NðN/2-1k=0|X(k)|2=|X(k)|2ðN/2-1k=0|X(k)|2(5)其中ꎬðN/2-1k=0Pk=1ꎮ(4)以香农熵理论为基础ꎬ计算信号的谱熵seꎬ即:se=-ðN/2-1k=0PklnPk(6)一般情况下ꎬ由于se的最大值为ln(N/2)ꎬ一般会对谱熵进行归一化操作ꎬ得到归一化的谱熵ꎬ即:SE(N)=selnN2()(7)通过以上定义可以看出ꎬ谱熵能够描述信号的结构稳定性:功率谱变化情况越不稳定ꎬ则信号的结构组成越简单ꎬ其序列振幅越不明显ꎬ相应的得到的测量值也较小ꎻ反之ꎬ信号的结构组成越复杂ꎬ参数的取值越大ꎮ本文以谱熵对滚动轴承的复杂性退化趋势进行描述ꎮ1.2㊀退化特征性能分析笔者以Logistic混沌映射序列作为仿真信号ꎬ分析7611第11期孙德建ꎬ等:基于GG模糊聚类的滚动轴承退化阶段划分研究谱熵的演化规律ꎬ信号表达式如下[14]:x(t+1)=λx(t)(1-x(t))(8)式中:x(t)ɪ(0ꎬ1)ꎻt 迭代次数ꎻλ 非线性参数(不同的参数取值使信号呈现不同的动力学行为)ꎮ设置初始值为x=0.4ꎬ分别计算每个λ取值下的谱熵ꎮLogistic混沌映射谱熵参数演化曲线如图1所示ꎮ图1㊀Logistic混沌映射谱熵参数演化曲线由图1可以看出:当λɪ[3ꎬ3.57]时ꎬ系统处于周期变化的状态ꎬSE取值维持在0.2附近ꎬ并随着周期个数的增加在3.45处出现谱熵取值的增大ꎻ当λɪ[3.57ꎬ4.0]时ꎬ系统处于混沌状态ꎬ同时一些狭小的区间混杂有周期性循环ꎬ使序列的复杂性下降ꎮ谱熵参数能够清晰反映混沌态复杂度不断增大的趋势ꎬ并在其中混杂的周期状态时出现取值的下降ꎮ与此同时ꎬ谱熵的运算过程中不涉及中间参数ꎬ运算速度快ꎬ适合在线退化特征分析ꎮ2㊀退化阶段划分流程与评价2.1㊀退化特征选取为了提高特征向量的全面性ꎬ本研究提取信号的三维特征向量[RMSꎬSEꎬCurvedtime]ꎮ其中ꎬ均方根RMS能够表征信号的能量累积变化ꎻSE复杂度能够反映性能退化进程中的复杂度变化ꎻCurvedtime(CT)能够反映性能退化进程中的时间集聚特性ꎮ该指标的提出是考虑到同一种运行状态在退化时间上具有内在的集聚特性ꎬ并且机械设备在性能退化初期的变化平缓ꎬ在退化进程中后期的变化会比较剧烈ꎮ结合指数函数的特性ꎬ本文将全寿命时间参数T归一化并映射到函数CT=eT-1中获得初期平缓㊁后期剧烈的 弯曲时间维度 ꎬ从而更准确地反映机械设备性能退化的时间分布特性ꎮ2.2㊀退化阶段划分效果评价本文采用GG模糊聚类[15]对退化阶段进行划分ꎮ模糊聚类的效果采用基于隶属度矩阵U的分类系数(classificationcoefficientꎬCC)和平均模糊熵(averagefuzzyentropyꎬAFE)[16]ꎮCC指标越接近1ꎬAFE指标越接近0ꎬ模糊聚类的效果越好ꎮ此外ꎬ本文提出并采用序列离散度指标(sequencedispersionꎬSD)ꎬ对同一退化状态在时间尺度的集聚性进行评价ꎮ具体的计算方法如下:对于某个聚类ꎬ假设I为该集合的样本标签序列ꎬn为该聚类的样本个数ꎬm-1为该标签序列最大值与最小值之差ꎬ定义该聚类的序列离散度如下:b=(m-n)m(9)显然ꎬ如果I为连续序列ꎬ则b=0ꎻI越不连续ꎬ序列中存在 空位 越多ꎬ序列离散度越大ꎮ假设整个样本集合被划分为c类ꎬ则此次聚类的时间序列离散度计算如下:γ=ðci=1bi(10)由式(10)可知:该指标越接近于0ꎬ代表时间集聚度越高ꎬ退化状态聚类效果越好ꎻ取值越大ꎬ时间集聚度越低ꎬ聚类效果越差ꎮ2.3㊀特征向量选取本文提出一种基于GG模糊聚类的滚动轴承退化阶段划分方法ꎮ该退化状态划分流程如图2所示ꎮ图2㊀退化状态划分流程8611 机㊀㊀电㊀㊀工㊀㊀程第36卷由图2可知:在获得滚动轴承全寿命数据之后ꎬ按照退化特征提取㊁GG模糊聚类㊁聚类效果评价3个阶段ꎬ可以实现轴承全寿命退化阶段的无监督划分ꎮ3㊀实例分析本节采用的全寿命数据集来自辛辛那提大学IMS中心[17]ꎮ实验中采用的轴承类型为RexnordZA ̄2115双列滚子轴承ꎬ滚子数量为16ꎬ滚子组节圆直径为75.501mmꎬ滚子直径为8.4074mmꎬ接触角为15.17ʎꎮ本研究选取其中一组数据集进行分析ꎮ该组试验的负载为5000N㊁转速1500r/min㊁采样频率20kHzꎬ每组采样时间为1sꎬ组间采样间隔为10minꎮ数据集采样组数为984ꎮ试验台停机时ꎬ检查发现1#轴承出现故障ꎬ失效形式为外圈故障ꎮ其余ꎬ2#~4#轴承完好ꎮ3.1㊀退化特征提取本文分别对每组采样数据进行退化特征分析ꎬ计算谱熵㊁RMS以及CurvedTimeꎮ轴承全寿命数据集下的性能退化特征趋势如图3所示ꎮ图3㊀轴承全寿命数据集下的性能退化特征趋势图由图3可以看出:整体趋势上ꎬ信号的复杂程度随着性能退化程度加深而逐渐降低ꎬ有效值RMS的趋势则与之相反ꎮ这说明随着性能退化程度的增加ꎬ信号中的随机成分逐渐减少ꎬ信号的复杂度随之降低ꎻ从能量累积观点上看ꎬ信号的能量随着退化程度的增加而不断增大ꎬ有效值RMS也随之增大ꎮ从细节上看ꎬ两个特征参数均呈现出一定的阶段性ꎬ反映了轴承性能退化的不同状态ꎮ另外ꎬCurvedTime参数的变化率在一定程度上反映了退化状态的演化速率ꎮ3.2㊀退化阶段划分参考文献研究中常用的4种不同退化阶段的划分方法[18 ̄19]将全寿命退化过程划分为:正常状态(Nor ̄mal)㊁轻微退化状态(Slight)㊁严重退化状态(Severe)㊁失效状态(failure)ꎮ本文采用GG模糊聚类算法对4种退化阶段进行无监督聚类ꎮ设置参数为c=4ꎬm=2ꎬ容差为ε=0.00001ꎮ滚动轴承的状态退化阶段划分结果如图4所示ꎮ图4㊀退化状态划分结果图由图4可以看出:在约第520组采样点之前ꎬ轴承处于正常状态ꎬ谱熵取值维持在0.7附近ꎮ当轴承性能轻微退化时ꎬ谱熵曲线敏感度快速下降ꎬ并出现明显的波动现象ꎬ直到第820组采样点左右ꎮ该阶段自集聚为轻微退化阶段ꎮ之后认为轴承性能严重退化ꎬ谱熵曲线基本维持在0.4左右ꎬ数值反弹不大ꎻ当进入到失效状态时ꎬ退化特征参数总体较低ꎬ且出现一些数值异常的离散点ꎬ此时认为轴承已经完全失效ꎮ按照聚类的评价方法ꎬ4个分组的序列离散度分别为0.0019㊁0.0651㊁0.0500㊁0.4103ꎬ本次分类的总的序列离散度为0.5272ꎮ3.3㊀对比分析本文设计并采用了CurvedTime参数作为时间集聚度的特征指标ꎬ为了分析弯曲时间特征参数对于状态划分的影响ꎬ分别采用二维特征[SEꎬRMS]和三维特征[SEꎬRMSꎬT]进行对比分析ꎬ其中ꎬT为未经映射的时间特征参数ꎮ不同特征参数下的聚类效果如图5所示ꎮ不同参数下的聚类定量评价结果如表1所示ꎮ9611 第11期孙德建ꎬ等:基于GG模糊聚类的滚动轴承退化阶段划分研究图5㊀不同特征参数的聚类效果图表1㊀不同特征参数的定量评价结果特征选取分类系数平均模糊熵序列离散度[SEꎬRMS]0.98100.02303.5959[SEꎬRMSꎬT]0.98390.02510.9577[SEꎬRMSꎬCT]0.98260.01360.5272㊀㊀对比表1可以看出:3种方法在分类系数和平均模糊熵参数上取值相近ꎬ但序列离散度取值较大ꎬ说明不同退化阶段的时间集聚性较差ꎮ图5(a)由于未采用时间特征维度ꎬ使得同一状态的样本的评判标准缺乏时间上的考量ꎬ导致轻微退化状态出现不连续性ꎻ图5(b)尽管引入了时间T参数ꎬ但由于未做映射处理ꎬ使得性能退化在时间尺度上的 变化速率 相同ꎬ进而导致了状态分类的 过早集聚 ꎬ出现一定程度上的状态边界误判ꎮ本研究保持三维特征向量[SEꎬRMSꎬCT]不变ꎬ分别采用GK聚类㊁FCM聚类进行对比分析ꎮ定量对比结果如表2所示ꎮ表2㊀不同聚类算法的定量评价结果聚类算法分类系数平均模糊熵序列离散度GK聚类算法0.87190.26660.0161FCM聚类算法0.84550.30120.0256GG聚类算法0.98260.01360.5272㊀㊀对比表2可以看出:由于引入了CurvedTime参数ꎬ3种方法的序列离散度SD均较低ꎬ说明分类的时间集聚度较高ꎮ但GK和FCM两种聚类算法的平均模糊熵较高ꎬ说明模糊矩阵U中的隶属度取值相近ꎬ容易造成状态误判ꎮ而GG聚类算法由于引入了模糊最大似然估计距离范数ꎬ在分类效果上较优ꎮ4㊀结束语本文提出了一种GG模糊聚类的滚动轴承退化阶段划分方法ꎬ并采用仿真和实例数据进行了分析验证ꎬ得到了以下结论:(1)谱熵参数能够反映信号中不规则成分的比例ꎬ有效描述性能退化过程中的规律性ꎬ并且对复杂度变化十分敏感ꎬ计算速度快ꎬ对Logistics方程和实例信号的分析验证了该方法的有效性ꎻ(2)引入的CurvedTime参数能够反映退化状态在时间尺度上的集聚特性ꎬ更符合机械设备的性能退化规律ꎻ(3)GG聚类方法能够对任意形状的数据进行聚类ꎬ将时间约束加入到特征向量中ꎬ能够在保持聚类精度的同时ꎬ提高退化状态的时间聚集度ꎮ以该结论为基础ꎬ下一步有必要深入研究基于GG聚类的在线状态评估方法ꎮ参考文献(References):[1]㊀年夫顺.关于故障预测与健康管理技术的几点认识[J].仪器仪表学报ꎬ2018ꎬ39(8):1 ̄14.[2]㊀ARANEORꎬATTOLINIGꎬCELOZZISꎬetal.Time ̄do ̄mainshieldingperformanceofenclosures:acomparisonofdifferentglobalapproaches[J].IEEETransactionsonE ̄lectromagneticCompatibilityꎬ2016ꎬ58(2):434 ̄441. [3]㊀肖顺根ꎬ马善红ꎬ宋萌萌ꎬ等.基于EEMD和PCA滚动轴承性能退化指标的提取方法[J].江南大学学报:自然科学版ꎬ2015ꎬ14(5):572 ̄579.[4]㊀王付广ꎬ李㊀伟ꎬ郑近德ꎬ等.基于多频率尺度模糊熵和ELM的滚动轴承剩余寿命预测[J].噪声与振动控制ꎬ2018ꎬ38(1):188 ̄192.[5]㊀杨大为ꎬ赵永东ꎬ冯辅周ꎬ等.基于参数优化变分模态分解和多尺度熵偏均值的行星变速箱故障特征提取[J].兵工学报ꎬ2018ꎬ39(9):1683 ̄1691.[6]㊀李学军ꎬ何能胜ꎬ何宽芳ꎬ等.基于小波包近似熵和SVM的圆柱滚子轴承诊断[J].振动㊁测试与诊断ꎬ2015(6):1031 ̄1036.[7]㊀冉㊀杰ꎬ刘衍民ꎬ王常春ꎬ等.二维离散Lorenz混沌系统的复0711 机㊀㊀电㊀㊀工㊀㊀程第36卷杂度分析[J].遵义师范学院学报ꎬ2018ꎬ20(4):81 ̄82ꎬ99.[8]㊀叶晓林ꎬ牟㊀俊ꎬ王智森ꎬ等.基于SE和C_0算法的连续混沌系统复杂度分析[J].大连工业大学学报ꎬ2018ꎬ37(1):67 ̄72.[9]㊀王㊀冰ꎬ王㊀微ꎬ胡㊀雄ꎬ等.基于GG模糊聚类的退化状态识别方法[J].仪器仪表学报ꎬ2018ꎬ39(3):21 ̄28.[10]㊀张立国ꎬ李㊀盼ꎬ李梅梅ꎬ等.基于ITD模糊熵和GG聚类的滚动轴承故障诊断[J].仪器仪表学报ꎬ2014ꎬ35(11):2624 ̄2632.[11]㊀WANGNꎬLIUXꎬYINJ.ImprovedGath ̄Gevacluste ̄ringforfuzzysegmentationofhydrometeorologicaltimese ̄ries[J].StochasticEnvironmentalResearchandRiskAssessmentꎬ2012ꎬ26(1):139 ̄155.[12]㊀YUKꎬLINTRꎬTANJW.Abearingfaultdiagnosistech ̄niquebasedonsingularvaluesofEEMDspatialconditionmatrixandGath ̄Gevaclustering[J].AppliedAcousticsꎬ2017(121):33 ̄45.[13]㊀孙克辉ꎬ贺少波ꎬ何㊀毅ꎬ等.混沌伪随机序列的谱熵复杂性分析[J].物理学报ꎬ2013ꎬ62(1):35 ̄42.[14]㊀王㊀涛ꎬ杨㊀越ꎬ顾雪平ꎬ等.基于小波模糊熵GG聚类的同调机群识别[J].电力自动化设备ꎬ2018ꎬ38(7):140 ̄147.[15]㊀张淑清ꎬ包红燕ꎬ李㊀盼ꎬ等.基于RQA与GG聚类的滚动轴承故障识别[J].中国机械工程ꎬ2015ꎬ26(10):1385 ̄1390.[16]㊀陈东宁ꎬ张运东ꎬ姚成玉ꎬ等.基于FVMD多尺度排列熵和GK模糊聚类的故障诊断[J].机械工程学报ꎬ2018ꎬ54(14):16 ̄27.[17]㊀LEEJꎬQIUHꎬYUGꎬetal.BearingdatasetfromIMSofuniversityofCincinnatiandNASAamesprognosticsdatarepository[EB/OL].[2010 ̄12 ̄10].http://ti.arc.nasa.gov/tech/dashpcoe/prognostic ̄data ̄repository.[18]㊀田再克ꎬ李洪儒ꎬ孙㊀健ꎬ等.基于改进MF ̄DFA和SSM ̄FCM的液压泵退化状态识别方法[J].仪器仪表学报ꎬ2016ꎬ37(8):1851 ̄1860.[19]㊀谭晓栋ꎬ邱㊀静ꎬ罗建禄ꎬ等.基于HSGT的装备健康状态评估技术[J].振动㊁测试与诊断ꎬ2017ꎬ37(5):886 ̄891.[编辑:方越婷]本文引用格式:孙德建ꎬ胡㊀雄ꎬ王㊀冰ꎬ等.基于GG模糊聚类的滚动轴承退化阶段划分研究[J].机电工程ꎬ2019ꎬ36(11):1166-1171.SUNDe ̄jianꎬHUXiongꎬWANGBingꎬetal.Degradationconditiondivisionofrollingbearingbasedongath ̄gevafuzzyclustering[J].JournalofMechanical&ElectricalEngineeringꎬ2019ꎬ36(11):1166-1171.«机电工程»杂志:http://www.meem.com.cn1711 第11期孙德建ꎬ等:基于GG模糊聚类的滚动轴承退化阶段划分研究。
基于G1-熵权法的正交实验设计对比BP神经网络优化香芩解热颗粒水提工艺

基于G 1-熵权法的正交实验设计对比BP 神经网络优化香芩解热颗粒水提工艺Δ程炳铎 1, 2, 3*,罗丽琴 1, 2,李元增 1, 2, 3,姜婕 1, 2, 3,陈怡莹 1, 2, 3,赵济 1, 2,薛蕊 1, 2,马云淑 1, 2, 3 #(1.云南中医药大学中药学院,昆明 650500;2.云南省傣医药与彝医药重点实验室,昆明 650500;3.云南省高校外用给药系统与制剂技术研究重点实验室/云南省南药可持续利用研究重点实验室/云南省药食同源饮品工程中心,昆明 650500)中图分类号 R 917 文献标志码 A 文章编号 1001-0408(2024)01-0027-06DOI 10.6039/j.issn.1001-0408.2024.01.05摘要 目的 优化香芩解热颗粒的水提工艺。
方法 以加水倍数、提取时间、提取次数为考察因素,以连翘酯苷A 、黄芩苷、连翘苷、千层纸素A-7-O -β-D-葡萄糖醛酸苷、汉黄芩苷、黄芩素、汉黄芩素含量和出膏率为评价指标,设计3因素3水平的正交实验,并利用G 1-熵权法对上述指标进行综合评分,得正交实验优化的水提工艺。
以9组正交实验结果为测试和训练数据,以加水倍数、提取时间、提取次数为输入节点,以综合评分为输出节点,利用BP 神经网络建模进行网络模型优化和水提工艺寻优。
验证并比较两种方法所得水提工艺参数,确定香芩解热颗粒的最佳水提工艺。
结果 香芩解热颗粒经正交实验优化后的水提工艺为加水倍数8倍、提取次数3次、提取时间1 h ,综合评分为96.84分(RSD 为0.90%)。
BP 神经网络建模优化后的水提工艺为加水倍数12倍、提取次数4次、提取时间0.5 h ,综合评分为92.72分(RSD 为0.77%),略低于正交实验所得工艺。
结论 本研究成功优化了香芩解热颗粒的最佳水提工艺,具体为加水倍数8倍、提取次数3次、提取时间1 h 。
关键词 香芩解热颗粒;水提工艺;G 1-熵权法;正交实验;BP 神经网络Optimization of water extraction technology of Xiangqin jiere granules by orthogonal design based on G 1-entropy weight compared with BP neural networkCHENG Bingduo 1, 2, 3,LUO Liqin 1, 2,LI Yuanzeng 1, 2, 3,JIANG Jie 1, 2, 3,CHEN Yiying 1, 2, 3,ZHAO Ji 1, 2,XUE Rui 1, 2,MA Yunshu 1, 2, 3(1. School of TCM , Yunnan University of Chinese Medicine , Kunming 650500, China ;2. Yunnan Key Lab of Dai and Yi Medicine , Kunming 650500, China ;3. Key Laboratory of External Drug Delivery System and Preparation Technology Research in Universities of Yunnan Province/Yunnan Provincial Key Laboratory of Sustainable Utilization of Southern Medicine/Engineering Research Center for Medicine and Food Homologous Beverage of Yunnan Province , Kunming 650500, China )ABSTRACTOBJECTIVE Optimizing the water extraction technology of Xiangqin jiere granules. METHODS The orthogonaltest of 3 factors and 3 levels was designed , and comprehensive scoring was conducted for the above indexes by using G 1-entropy weight to obtain the optimized water extraction technology of Xiangqin jiere granules with water addition ratio , extraction time and extraction times as factors , using the contents of forsythoside A , baicalin , phillyrin , oroxylin A-7-O -β-D-glycoside , wogonoside , baicalein and wogonin , and extraction rate as evaluation indexes. BP neural network modeling was used to optimize the network model and water extraction process using the results of 9 groups of orthogonal tests as test and training data , the water addition multiple , decocting time and extraction times as input nodes , and the comprehensive score as output nodes. Then the two analysis methods were compared by verification test to find the best water extraction process parameters. RESULTS The water extraction technology optimized by the orthogonal test was 8-fold water , extracting 3 times , extracting for 1 h each time. Comprehensivescore was 96.84 (RSD =0.90%). The optimal water extractiontechnology obtained by BP neural network modeling included 12-fold water , extracting 4 times , extracting for 0.5 h each time. The comprehensive score was 92.72 (RSD =0.77%), which was slightly lower than that of the orthogonal test. CONCLUSIONS The water extraction technology of Xiangqin jiere granules is optimized successfully in the study , which includes adding 8-fold water , extracting 3 times ,andΔ 基金项目国家中医药管理局高水平中医药重点学科建设项目(No.国中医药人教函〔2023〕85号);云南省科技厅重点研发计划项目(No.202103AC 100005);云南省傣医药与彝医药重点实验室开放课题(No.202210SS 2204)*第一作者硕士研究生。
融合空洞空间金字塔池化和注意力的轻量化遥感影像道路提取

第 45 卷 第 1 期航天返回与遥感2024 年 2 月SPACECRAFT RECOVERY & REMOTE SENSING111融合空洞空间金字塔池化和注意力的轻量化遥感影像道路提取刘志恒 1 岳子腾 2,* 周绥平 1 江澄 3 节永师 3 陈雪梅 4(1 西安电子科技大学空间科学与技术学院,西安 710126)(2 北京航空航天大学电子信息工程学院,北京 100191)(3 北京空间机电研究所先进光学遥感技术北京市重点实验室,北京 100094)(4 西安航天天绘数据技术有限公司,西安 710100)摘 要 针对高分辨率遥感影像中道路形状结构错综复杂,出现窄小型道路提取错误或漏分的问题,提出一种基于空洞空间金字塔池化和注意力机制的轻量化遥感影像道路提取方法。
首先,在原始高分辨率网络(HRNet)基础上,通过引入空洞空间金字塔池化模块,实现多尺度道路信息融合;再引入挤压激励通道注意力机制,增强网络特征表征质量;最后使用深度可分离卷积方法改进网络残差模块实现模型轻量化,以降低模型计算复杂度。
在公开数据集上进行了模型性能测试,实验结果表明,文章所提算法的准确率、精确率、召回率、F1分数和平均交并比,相比原始HRNet分别提升了5.35 %、2.15 %、4.1 %、3.15 %和14.34 %,且减少了36.1 %的参数数量;相比其他网络,该算法突出了细小道路的特征,道路预测结果连续性、完整性好,并且模型小易于部署在实时检测设备中,有效改善了道路提取任务中错分和缺失的情况,是一种适应性更强、分割精度更高、更轻量化的多尺度道路提取算法。
关键词 道路提取 空间金字塔池化 通道注意力机制 可分离卷积 高分辨率网络 遥感影像中图分类号:TP751 文献标志码:A 文章编号:1009-8518(2024)01-0111-12DOI:10.3969/j.issn.1009-8518.2024.01.010Lightweight Remote Sensing Image Road Extraction Combing Atrous Spatial Pyramid Pooling and Attention Mechanism LIU Zhiheng1 YUE Ziteng2,* ZHOU Suiping1 JIANG Cheng3 JIE Yongshi3 CHEN Xuemei4( 1 School of Aerospace Science and Technology, Xidian University, Xi’an 710126, China )( 2 School of Electronics and Information Engineering, Beihang University, Beijing 100191, China )( 3 Beijing Key Laboratory of Advanced Optical Remote Sensing Technology,Beijing Institute of Space Mechanics & Electricity, Beijing 100094, China )( 4 Xi’an Aerospace Remote Sensing Data Technology Co., Ltd., Xi’an 710100, China )收稿日期:2023-08-08基金项目:陕西省自然科学基础研究计划资助项目(2023-JC-QN-0299);先进光学遥感技术北京市重点实验室开放基金项目(AORS20238);自然资源部矿山地质灾害成灾机理与防控重点实验室项目(2022-08);中央高校基本科研业务费专项资金资助项目(300102353502);自然资源部国土卫星遥感应用重点实验室开放基金项目(KLSMNR-G202303)引用格式:刘志恒, 岳子腾, 周绥平, 等. 融合空洞空间金字塔池化和注意力的轻量化遥感影像道路提取[J]. 航天返回与遥感, 2024, 45(1): 111-122.LIU Zhiheng, YUE Ziteng, ZHOU Suiping, et al. Lightweight Remote Sensing Image Road Extraction Combing AtrousSpatial Pyramid Pooling and Attention Mechanism[J]. Spacecraft Recovery & Remote Sensing, 2024, 45(1): 111-122.(in Chinese)112航 天 返 回 与 遥 感2024 年第 45 卷Abstract Aiming at the problem of intricate road shape and structure in high-resolution remote sensing images, where narrow and small roads are extracted incorrectly or omitted, a lightweight remote sensing image road extraction method based on Atrous Space Pyramid Pooling and Attention Mechanism is proposed. Firstly, based on the original HRNet network, multi-scale road information fusion is realized by introducing the ASPP. Secondly, the Squeeze and Excitation channel attention mechanism (SE-networks) is introduced to enhance the quality of network feature representation. Finally, using deep separable convolution to improve the network residual module to realize the model lightweight and reduce the complexity of model calculation. Experimental results on the publicly available dataset show that the accuracy, precision, recall, F1 score and the MIoU of the proposed algorithm was improved respectively by 5.35%, 2.15%, 4.1%, 3.15% and 14.34%, compared with the original HRNet network, and reduce the number of parameters by 35.6%. Compared with other networks, the algorithm highlights the characteristics of small roads, and the prediction results have good continuity and integrity. As the small size, the proposed model is easier to deploy in real-time detection equipment. The proposed model effectively reduces the road extraction fault and missing, implements a stronger adaptability, higher segmentation accuracy, more lightweight multi-scale road semantic segmentation algorithm.Keywords road extraction; ASPP; channel attention mechanism; separable convolution; High-Resolution Network; remote sensing images0 引言在城市发展和规划过程中,道路是不可或缺的元素之一。
薄壁件铣削颤振特征提取的GA-PE-VMD和MSE方法
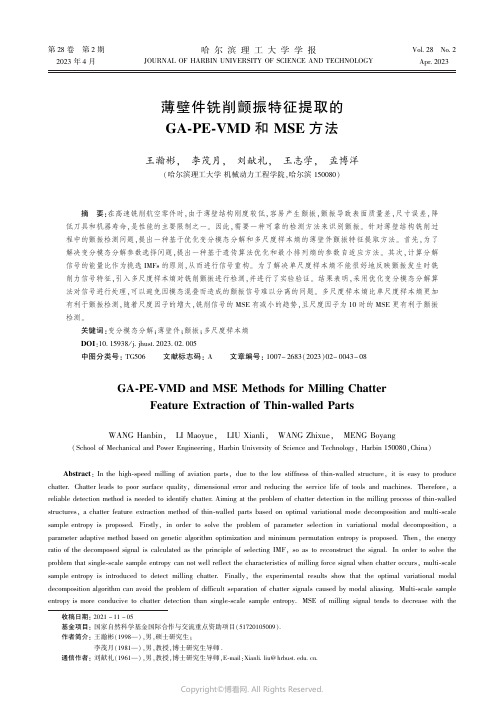
四年级下册语文四单元作文450字写景全文共9篇示例,供读者参考四年级下册语文四单元作文450字写景篇1秋天来了,秋姑娘迈着轻盈的脚步向我们走来,天高云淡,秋高气爽。
快看,那柿子树上的一粒粒柿子像一把把小灯笼,你挤我碰,好象争着要人们去摘呢!秋风轻轻吹过,银杏树、枫树的叶子,飘落了下来,有的像黄蝴蝶、红蝴蝶在空中翩翩起舞,有的像一把把小扇子飘下来,还有的像舞蹈演员在表演空旋……好看得很!还有那菊花仙子的颜色,五彩斑斓、个性鲜明;有紫的、有金黄的、有粉红的、还有天蓝的……美丽的菊花在秋天里频频点头,好象在说:“秋天来了,秋天来了!我们换上新衣服啦!”风儿轻轻吹,小草轻轻摇。
秋天的小草,没有春天那么嫩绿,没有夏天那么茂盛,也没有冬天那么光秃,秋天的小草是那么金黄,也有几处的小草是绿的,真像一条黄绿相间的地毯。
说到小松鼠,真是可爱极了!它知道秋天来了,寒冷的冬天也即将来临,松鼠们正忙着搬运粮食,准备过冬呢!秋天真美啊!像一幅多姿多彩的画!四年级下册语文四单元作文450字写景篇2以前,从没有这么仔细地欣赏天空,原来,天空原来那么蓝,映着洁白无暇的白云,远处还有一条叮咚叮咚的小溪,水很浅,只要你把脚放在里面轻轻一荡,就会有许许多多的水痕,久久不肯离去,仿佛那恋恋不舍的小黄鹂。
旁边开着几朵五彩缤纷的小花,有杜鹃花,鸡冠花,梨花、迎春花,不久,天空飘来刺骨的寒风,我没防备打了个抖,瞧,那几朵生长在荒郊野外、无人栽培的花,正坚强地怒放着,不怕风,不怕雨。
我想,这些小花那么娇小,却那么坚强。
它们不顾风,保护着后面的花朵,仿佛妈妈保护自己的儿女一样,顿时,我深深地感动了。
看着这么痛苦的花,我不禁想把它们摘下放进手心里,不让它们被刺骨的寒风吹倒。
最终,我没有这样做,为什么?因为如果我摘下来,它们会枯萎。
我就不能继续欣赏了,我要把这些花儿献给以后来这里游玩的人,沁人心脾的香味,要与大家分享!四年级下册语文四单元作文450字写景篇3淅淅沥沥的春雨缠缠绵绵地下着。
学术不端行为之一:不当署名
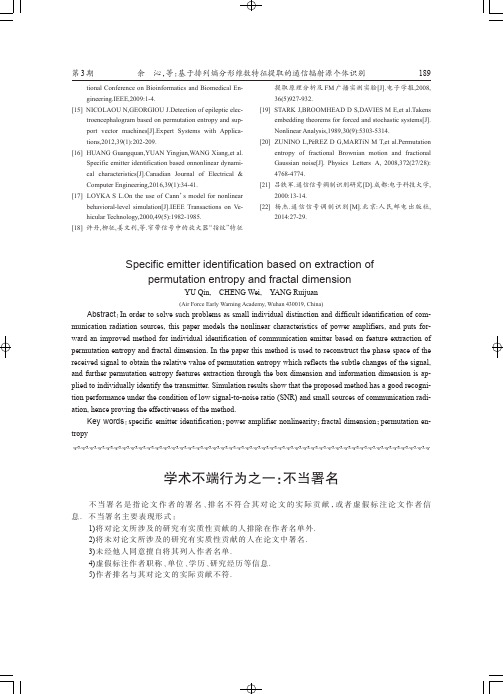
第3期余沁,等:基于排列熵分形维数特征提取的通信辐射源个体识别189tional Conference on Bioinformatics and Biomedical En-gineering.IEEE,2009:1-4.[15]NICOLAOU N,GEORGIOU J.Detection of epileptic elec-troencephalogram based on permutation entropy and sup-port vector machines[J].Expert Systems with Applica-tions,2012,39(1):202-209.[16]HUANG Guangquan,YUAN Yingjun,WANG Xiang,et al.Specific emitter identification based onnonlinear dynami-cal characteristics[J].Canadian Journal of Electrical &Computer Engineering,2016,39(1):34-41.[17]LOYKA S L.On the use of Cann ’s model for nonlinear behavioral-level simulation[J].IEEE Transactions on Ve-hicular Technology,2000,49(5):1982-1985.[18]许丹,柳征,姜文利,等.窄带信号中的放大器“指纹”特征提取原理分析及FM 广播实测实验[J].电子学报,2008,36(5)927-932.[19]STARK J,BROOMHEAD D S,DA VIES M E,et al.Takensembedding theorems for forced and stochastic systems[J].Nonlinear Analysis,1989,30(9):5303-5314.[20]ZUNINO L,PéREZ D G,MARTíN M T,et al.Permutationentropy of fractional Brownian motion and fractionalGaussian noise[J].Physics Letters A,2008,372(27/28):4768-4774.[21]吕铁军.通信信号调制识别研究[D].成都:电子科技大学,2000:13-14.[22]杨杰.通信信号调制识别[M].北京:人民邮电出版社,2014:27-29.Specific emitter identification based on extraction ofpermutation entropy and fractal dimensionYU Qin,CHENG Wei,YANG Ruijuan(Air Force Early Warning Academy,Wuhan 430019,China)Abstract :In order to solve such problems as small individual distinction and difficult identification of com-munication radiation sources,this paper models the nonlinear characteristics of power amplifiers,and puts for-ward an improved method for individual identification of communication emitter based on feature extraction of permutation entropy and fractal dimension.In the paper this method is used to reconstruct the phase space of the received signal to obtain the relative value of permutation entropy which reflects the subtle changes of the signal,and further permutation entropy features extraction through the box dimension and information dimension is ap-plied to individually identify the transmitter.Simulation results show that the proposed method has a good recogni-tion performance under the condition of low signal-to-noise ratio (SNR)and small sources of communication radi-ation,hence proving the effectiveness of the method.Key words :specific emitter identification ;power amplifier nonlinearity ;fractal dimension ;permutation en-tropy学术不端行为之一:不当署名不当署名是指论文作者的署名、排名不符合其对论文的实际贡献,或者虚假标注论文作者信息.不当署名主要表现形式:1)将对论文所涉及的研究有实质性贡献的人排除在作者名单外.2)将未对论文所涉及的研究有实质性贡献的人在论文中署名.3)未经他人同意擅自将其列入作者名单.4)虚假标注作者职称、单位、学历、研究经历等信息.5)作者排名与其对论文的实际贡献不符.。
基于CYCBD 和麻雀搜索算法的滚动轴承故障特征提取方法
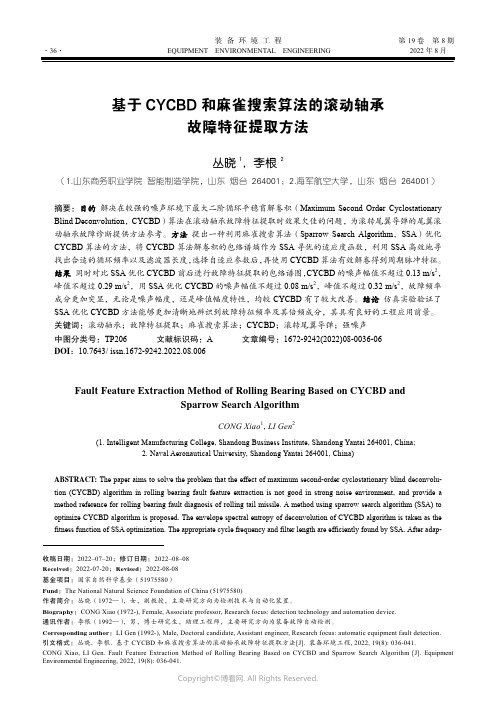
装备环境工程第19卷第8期·36·EQUIPMENT ENVIRONMENTAL ENGINEERING2022年8月基于CYCBD和麻雀搜索算法的滚动轴承故障特征提取方法丛晓1,李根2(1.山东商务职业学院 智能制造学院,山东 烟台 264001;2.海军航空大学,山东 烟台 264001)摘要:目的解决在较强的噪声环境下最大二阶循环平稳盲解卷积(Maximum Second Order Cyclostationary Blind Deconvolution,CYCBD)算法在滚动轴承故障特征提取时效果欠佳的问题,为滚转尾翼导弹的尾翼滚动轴承故障诊断提供方法参考。
方法提出一种利用麻雀搜索算法(Sparrow Search Algorithm,SSA)优化CYCBD算法的方法,将CYCBD算法解卷积的包络谱熵作为SSA寻优的适应度函数,利用SSA高效地寻找出合适的循环频率以及滤波器长度,选择自适应参数后,再使用CYCBD算法有效解卷得到周期脉冲特征。
结果同时对比SSA优化CYCBD前后进行故障特征提取的包络谱图,CYCBD的噪声幅值不超过0.13 m/s2,峰值不超过0.29 m/s2,用SSA优化CYCBD的噪声幅值不超过0.08 m/s2,峰值不超过0.32 m/s2,故障频率成分更加突显,无论是噪声幅度,还是峰值幅度特性,均较CYCBD有了较大改善。
结论仿真实验验证了SSA优化CYCBD方法能够更加清晰地辨识到故障特征频率及其倍频成分,其具有良好的工程应用前景。
关键词:滚动轴承;故障特征提取;麻雀搜索算法;CYCBD;滚转尾翼导弹;强噪声中图分类号:TP206 文献标识码:A 文章编号:1672-9242(2022)08-0036-06DOI:10.7643/ issn.1672-9242.2022.08.006Fault Feature Extraction Method of Rolling Bearing Based on CYCBD andSparrow Search AlgorithmCONG Xiao1, LI Gen2(1. Intelligent Manufacturing College, Shandong Business Institute, Shandong Yantai 264001, China;2. Naval Aeronautical University, Shandong Yantai 264001, China)ABSTRACT: The paper aims to solve the problem that the effect of maximum second-order cyclostationary blind deconvolu-tion (CYCBD) algorithm in rolling bearing fault feature extraction is not good in strong noise environment,and provide a method reference for rolling bearing fault diagnosis of rolling tail missile. A method using sparrow search algorithm (SSA) to optimize CYCBD algorithm is proposed. The envelope spectral entropy of deconvolution of CYCBD algorithm is taken as the fitness function of SSA optimization. The appropriate cycle frequency and filter length are efficiently found by SSA. After adap-收稿日期:2022–07–20;修订日期:2022–08–08Received:2022-07-20;Revised:2022-08-08基金项目:国家自然科学基金(51975580)Fund:The National Natural Science Foundation of China (51975580)作者简介:丛晓(1972—),女,副教授,主要研究方向为检测技术与自动化装置。
- 1、下载文档前请自行甄别文档内容的完整性,平台不提供额外的编辑、内容补充、找答案等附加服务。
- 2、"仅部分预览"的文档,不可在线预览部分如存在完整性等问题,可反馈申请退款(可完整预览的文档不适用该条件!)。
- 3、如文档侵犯您的权益,请联系客服反馈,我们会尽快为您处理(人工客服工作时间:9:00-18:30)。
Entropy-Based Motion Extraction for Motion Capture AnimationClifford K. F. So and George BaciuDepartment of Computing - The Hong Kong Polytechnic UniversityEmail: {cskfso, csgeroge}@.hkAbstractIn this paper we present a new segmentation solution for extracting motion patterns frommotion capture data by searching for critical keyposes in the motion sequence. A rank isestablished for critical keyposes that identifies the significance of the directional change inmotion data. The method is based on entropy metrics, specifically the mutual informationmeasure. Displacement histograms between frames are evaluated and the mutualinformation metric is employed in order to calculate the inter-frame dependency. The mostsignificant keypose identifies the largest directional change in the motion data. This willhave the lowest mutual information level from all the candidate keyposes. Less significantkeyposes are then listed with higher mutual information levels. The results show that themethod has higher sensitivity in the directional change than methods based on themagnitude of the velocity alone. This method is intended to provide a summary of a motionclip by ranked keyposes, which is highly useful in motion browsing and motion retrievedatabase system.Keywords: motion capture, animation, entropy, mutual information, motion database.IntroductionThe advent of motion capture systems has had a significant impact on the speedy generation of motion for 3D characters for animation and clinical studies in gate analysis. Current motion capture devices capture human motion at high frequency [1] and the motion can be retargeted to computer characters for realistic movements. Applications of motion capture are continuously growing in number and range from crowd generation and controlling the motion of synthetic characters in large scale movie productions to short TV commercials, 3D computer games, bio-kinematics analysis in sports and clinical studies, simulations and preservation of style in specific motions that can be generated by unique individuals, such as for example variations of Kong-Fu in martial arts, dancing and acrobatics. However, motion generation often involves long motion capture sessions and careful editing in order to adapt and fulfill a particular behavior of a character. The captured motion is often archived and annotated for future use. The motion database is probably the most important asset in a production studio. Recorded motions are frequently recalled from the database and new motions are reconstructed from the given clips.The work of this paper is motivated by the need to work with larger motion databases that require frequent motion storage and retrieval operations. The fundamental questions that we attempt to answer are:How can we efficiently store all the captured motion into a motion database?What is the most meaningful way to summarize a motion clip other than text annotation?How can we retrieve motion segments in a more intuitive way than simply using text annotation?What features should be extracted from the motion clips for an efficient indexing strategy?We attempt to address these questions by proposing a new method that first identifies significant changes in the motion patterns and establishing a entropy based ranking in order to extract meaningful motion segments. We define a keypose to represent a time sample of the 3D motion such that the motion that occurs prior to the keypose is significantly different from the motion that occurs after the keypose. A keypose gives a visual summary of the motion and identifies a unique posture during the change between two continuous motion changes. A keypose contains more information than text annotation. The use of keyposes is similar to a “keyframe” in the traditional animation, however a keypose is different from a keyframe in that it is the representation of an “extreme” pose at the time when the motion has to pass through from one continuous pattern to another and, therefore, it has a substantial directional change. Thus, a keypose serves as the signature of the motion like the poses featured in dancing motion, kung-fu motion and simple walking or running cycles.We first solve the keypose extraction problem by using a mutual information method to identify critical keyposes from a given motion sequence. The result of this extraction is a list of ranked critical keyposes that have been detected in a large motion segment. Keyposes determine a substantial directional change in the motion pattern. We use an entropy measure, specifically the mutual information measure, to quantify the amountof information that is passed from one frame to another. A large difference in the directional movement between two frames leads to a weak inter-frame dependency and hence a lower level of mutual information. Our algorithm ranks the keyposes based on their corresponding mutual information levels. These levels are related to the significance in the directional change of the motion. The rank of the keyposes can then be used further in the indexing of the motion retrieval system.We organize the paper as follows: We first present some background and related work. We give the entropy metric and the details of our work in the next section. We will present the result and compare to the previous work, and gives other possible applications. The final section concludes the paper and suggests future directions.Related workRecently, Li et al. [2] presented a motion retrieval system based on a hierarchical motion index tree. The similarity between the sample and the motion in the sub-library is calculated through an elastic match. In order to improve the efficiency of the similarity calculation, an adaptive clustering-based keypose extraction algorithm is adopted. The keyposes are used in the calculation instead of the whole motion sequence. Our work differs from Li et al by searching and identifying the “extreme” poses rather than the most similar poses at the centroids.Some recent research work also suggests the concept of a motion database. These techniques are mainly motion re-synthesis techniques that automatically generate new motions from existing motion clips. Some algorithms re-synthesize a new motion basedon non-annotated motion database with user-specified constrains [3-8]. These algorithms synthesize a new motion that follows a path, go to a particular position, or perform certain activity at a specified time. The motion databases referred to, however, are limited to a set of motion samples and targeted to specific motion re-synthesis needs. We address the broader notion of a motion database and retrieval environment for practical motion editing sessions.To concatenate the motion corpus efficiently together, Gleicher et al. [9] proposes a Snap-Together Motion system that processes a set of motion corpus to a set of short clips that can be concatenate to a continuous stream. The system builds a graph structure that facilities efficient planning of character motion. Kovar et al. [10] constructs a motion graph that consists of both original motion and automatically generated transitions to facilitate synthesizing walks on the ground. Both works involve retrieving similar character poses from the set of motion corpus. An efficient motion retrieval system will be beneficial to synthesizing more complex motions by maintaining a larger set of motion corpus.Recently, some motion synthesis techniques are based on a manually annotated motion database [11,12]. A new motion is synthesized based on basic vocabulary of terms specified by the animator such as “walk”, “run” or “jump.” Thus, motion clips are hand-annotated before the synthesis. The new motion is reassembled by blending the annotated motion clips. Automatic annotation using Support Vector Machine (SVM) classifiers has also been investigated by Arikan et al. [12]. However, human intervention is necessary in the classification in order to guide the system to learncertain actions. Such a classifier can lead to the success of building a text-based motion retrieval database.Other work involves the idea of “keyframe” or “keypose”. Pullen et al. [13] propose motion “texturing” based on the animation keyframes. Keyframes and curves are outlined by the animator and the motion capture data are mapped to the curve based on a matching process. Bevilacqua et al. [14,15] propose to extract keypose from motion capture data based on the magnitude of velocity. We provide better solution by exploring the information content present the transition between motion patterns. We then compare their results in the result section. Kim et al. [16] propose extracting motion rhythms based on the zero-crossings of the second derivative (acceleration) of each motion signal. A zero-crossing of the acceleration of motion signal could potentially mean a keypose. But their work addresses a very different problem. Their emphasis is on finding the motion beat. Potential keyposes outside the beat are not considered. This can pose problems in more generic types of motion sequences. For this it is important to rank the keyposes and attempt to find a measure of the significance in the change in motion pattern. Our work is based on using the mutual information metric in order to find all the potential keyposes in the motion with more emphasis on determining the significant keyposes.The problem we are dealing with is analogous to the keyframe extraction in video indexing and retrieval system. Certain color histogram-based algorithms are developed for detecting video cuts [17-20] and fades [21-25]. Our method is similar to a recentvideo cut and fade detection algorithm by Z. Černekov á et al. [26], which is based on entropy metrics of mutual information and joint entropy.Entropy metric-based keypose extractionMutual informationThe entropy of a discrete random variable X measures the information content or “uncertainty” of X . Let A x = {a 1, a 2,…, a N } be the outcome of X having probabilities {p 1, p 2,…, p N } with p X = (x = a i ) = p i , p i ≥ 0 and 1)(=!"x p X A x X . The entropy is defined as:!"#=X A x X X x p x p X H )(log )()( (1)The joint entropy of two discrete random variables X and Y is given by:!"#=Y X A A y x XY XY y x p y x p Y X H ,,),(log ),(),( (2)where p XY (x,y ) is the joint probability density function. The mutual information measures the reduction in the uncertainty of X given the knowledge of Y :!"#=Y X A A y x Y X XY XY y p x p y x p y x p Y X I ,,)()(),(log ),(),( (3)The mutual information can also be calculated by relating to the joint entropy of X and Y :I (X , Y ) = H (X ) + H (Y ) − H (X , Y ) (4)The mutual information gives us a measure of association between X and Y . It measures the overlap information carried in X and Y . This is the overlap region of H(X ) and H(Y ). On the other hand, the joint entropy measures the unified information carried in X and Y , which is the union region of H(X ) and H(Y ).Displacement Histogram and mutual information of displacementThe data we consider is the set of the global XYZ coordinates of the markers captured by a motion capture system. This format is currently generated by modern motion capture systems such as VICON. Let (x i,f , y i,f , z i,f ) be the global XYZ coordinates of marker i in frame f . We assume that the data does not contain missing samples. Missing marker data due to occlusion has to be fully reconstructed before the calculation and it can be performed as in [27].We define vector d i,f be the displacement vector of marker i in frame f to the next frame, which is!!!"#$$$%&'''=!!!"#$$$%&=+++f i f i f i f i f i f i f i f i f i f i z z y y x x dz dy dx d ,1,,1,,1,,,,, (5)where dx i,f , dy i,f and dz i,f correspond to the displacements in XYZ axis. d i,f forms an instantaneous velocity of marker i in frame f to the next frame.The next step is to calculate the displacement histogram according to n number of discretization levels. Consider only the X coordinates. We choose the global maximum and minimum values of dx i,f as the range of the discretization. Let the displacementhistogram },,,{,2,1,X n f X f Xf X f a a a A K = be the n discretized outcome of dx i,f in frame fhaving corresponding probabilities },,,{,2,1,X n f X f Xf X f p p p B K =, where X i f X i f a p ,,= / totalnumber of markers. Similarity, the joint probability of the discretized dx i,f between frame f and f +1 can be expressed as a n × n matrix ),(s r C X f , with 1 ≤ r ≤ n and 1 ≤ s ≤n . Element (r , s ) in ),(s r C X f represents the probability of a marker having discretization level r in frame f and discretization level s in frame f + 1. Let X f I be the mutual information measure of the displacement from frame f to f + 1 for the X coordinates. We construct the mutual information measure X f I by applying equation (3) to the elements of vector X f B and matrix ),(s r C X f :!!=="=n r n s X f Xf X f X fXf s B r B s r C s r C I 11)()(),(log ),( (6)We do the same formulation for the Y and Z coordinates in order to obtain the Yf I andZf I . Hence, the total mutual information I f of the displacement of frame f to f + 1 isZ f Yf X f f I I I I ++= (7)Keypose detectionA keypose involves a change of velocity (or a change of displacement vectors between frames) in the motion. A low mutual information I f indicates a high probability of dissimilar velocity between frames, which indicates a high probability of occurrence of keypose. Figure 1 shows an example of mutual information plot of a motion sampled in 120Hz with n = 256 discretization levels. Lower I f level indicates a relatively significant change of velocity and higher I f level indicate a relatively similar movement. We propose to localize the mutual information in order to emphasize the local changes. We plot ][ˆf w f f I E I I = (8)where E w [I f ] = the mean of I f-w to I f+w-1. E w [I f ] forms a window always centered on frame f . We restrict the left boundary of the window to 1 when f -w < 1 and the right boundary to the maximum number of frames when f +w-1 exceeds the maximum number. Figure 2 shows the fI ˆ plot of Figure 1 with w = 60 (total window size = 1 second). The vertical lines show the keyposes extracted as the low minima of fI ˆ.There are several ways to pick the low minima of f I ˆ. The fI ˆ curve can be trimmed horizontally with a threshold ∈. Several local troughs will be isolated and the minimumvalue of each trough can be picked. Another way is to sort the fI ˆ in an increasing order. We adaptively increase the threshold ∈ until a particular number or density of troughsare reached. Only the lowest fI ˆ point of each trough will be output as keypose. We may also consider to smooth the signal but this may potentially incur inaccurate detection of sharp mutual information changes. The example shown in Figure 2 is extracted with threshold ∈ = 0.9.ResultsKeypose extractionFigure 3 shows the result of a dance motion. The motion is sampled at 120Hz. Thelocalized mutual information fI ˆ is calculated with 1 second windows size and 256 discretization levels. The keyposes are extracted with increasing threshold ∈. The motion starts with a lean to the left and a lean to the right followed by a leap of the right leg and the left leg. The number of each snapshot in Figure 3 indicates the order of theextracted keyposes. In the beginning, the 2 most significant keyposes (largest change of motion) are extracted with low ∈(0.75). Two more keyposes (3 and 4) then appear when ∈ increases to 0.90. The less significant keyposes (5, 6 and 7) are extracted with higher ∈ = 0.95. They are mainly the transit poses in between the significant poses. The significance of the keyposes decreases with increasing threshold level. The process stops upon a particular number or density of keyposes is reached, or a pre-defined maximum threshold is reached (1.0 is a reasonable number as we are interested in poses below average). Such snapshots of the keyposes give a brief summary of the motion events along the time.Comparison with magnitude-velocity methodWe try to compare our method with a magnitude-velocity method proposed by Bevilacqua [10]. The magnitude-velocity method calculates the magnitudes of the velocity of the markers at each frame to the next frame. All the magnitudes are summed up to form a 1-D signal. The local minima of the plot will be extracted as keyposes. In order to compare this method with our method, we try to localize the signal by the same 1-second window.Our result shows that our mutual information method is more sensitive to directional changes than the magnitude-velocity method. Figure 4 shows the plot of our localized mutual information curve and the magnitude-velocity curve of another dance motion. Under the same threshold ∈ = 0.90, 22 keyposes are picked by our method (They are indicated by the vertical lines in the figure), and 15 keyposes are picked by the magnitude-velocity method. The figure shows that nearly all the keyposes picked by themagnitude-velocity are also picked by our method. We can name the extra keyposes detected by our method as transition keyposesWe further investigate the property of the transition keyposes in Figure 4. Figure 5 (upper) shows the zoomed view of the region X and Y. In region X, three keyposes are picked by the mutual information method. The keypose in interest is the circled one. The character rises his arms from the starting frame to the circled keypose and pulls back in the third keypose. The magnitude-velocity method fails to detect this change of direction since the motion is in continuous magnitude-deceleration. In region Y, the character is stretching his right leg. The uppermost point of where the character lifts his leg is extracted as keypose (circled in the figure). The leg is putting down after this pose. The magnitude-velocity method fails to detect this post again as the local maximum of the magnitude-velocity does not necessarily imply a change of direction.In general, the transition keyposes detected by our method have no direct association to the shape of the magnitude-velocity curve nor its first derivative. Though some keyposes picked by our method meet the local maxima of the magnitude-velocity curve (the last keypose in region X and the circled keypose in region Y), not all the local maxima of the speed can simply imply changes of direction (as shown in the rest of Figure 4). The first keypose in Figure 5 also gives us as a good example that the transition keypose can be under a continuous speed deceleration.Our method can also used to detect static part of the body. We can calculate the mutual information of the individual parts (2 limbs, 2 legs) of the body. The lower partof Figure 5 shows the result of the region X and Y. Two legs are relatively more static in region X and the left leg is relatively more static in region Y.Motion database retrievalKeypose extraction is a pre-processing step of motion database retrieval. It improves the matching efficiency by eliminating the similar poses near the keyposes. Liu et. al. [2] employs a clustering method to pick the keypose in the centroid for further elastic matching between two sequences. Our method can also be an alternative to the clustering method. Figure 6 shows the keyframe extraction with our method for an original motion and three other motions. The threshold ∈ of the localized mutual information used is 1.00. 5 keyframes are extracted in each of the motion. Motion A andB are shown to be similar to the original motion. MotionC is shown to be dissimilar to the others. We can measure the similarity of keypose v and w in terms of their vector dot-products:!="##$%&&'()=m i i i i i w v w v m w v 11cos 1),(* (9)where v i is the i -th vector defined between the markers of keypose v , and m is the total number of vectors available, which is the total number of markers minus 1. The vectors are expressed in terms of the local frame defined in the hip joint of the character in order to preserve the local orientation of the character. Equation (9) measures the average angle difference of the vectors between the two keyframes.Figure 7 shows the average angle difference between the keyposes of the original motion and motion A, B, and C. Motion A is found to be very similar to the originalmotion since it has an overall angle error of π/16. Motion B is different at the beginning (about π/8) but very similar at the end of the motion sequence (about π/16). Motion C is found to be different overall as it has an error above π/4. The result shows that the method provides an efficient motion pattern comparison method independent of absolute time. For more precise motion comparison, the time gap between each keypose can be included in the distance function. We can add a penalty function for larger time gaps.In summary, we have shown a practical method for our keypose extraction method for motion comparison. An open problem is to compare the motion with different number of keyposes in a motion retrieval system. The elastic matching proposed by Liu et. al. [2] can be a possible starting point for solving this problem since it considers the time-warping between keyposes.ConclusionWe have presented a new keypose extraction method from motion captured data based on the mutual information metric. The method produces a list of keyposes ranked by their significance of directional change. The metric of the method is based on the mutual information of the displacements of the markers between frames. A lower mutual information level indicates a high probability of dissimilar displacements between frames. The main feature of our method is that it has a higher sensitivity to directional change than the magnitude-velocity method. This work provides the basis for another application such as music beat synchronization, motion browsing and amotion retrieval system. Our method is shown to be useful in extracting the “extreme” keyposes used in the motion similarity computations for a motion retrieval database.References[1] VICON 8i, VICON Motion Systems, Oxford Metrics Limited.[2] F. Liu, Y. Zheung, F. Wu, and Y. Pan. 3D motion retrieval with motion index tree.In Computer Vision and Image Understanding, 92:265-284, 2003.[3] Y. Li, T. Wang, and H. Y. Shum. Motion texture: A two-level statistical model forcharacter motion synthesis. In Proceedings of ACM SIGGGRAPH 2002, Annual Conference Series, ACM SIGGRAPH, 465-472, 2002.[4] A. Bruderlin and T. Calvert. Knowledge-driven, interactive animation of humanrunning. In Graphics Interface, Canadian Human-Computer Communicates Society, 213-221, May 1996.[5] L. Molina-Tanco and A. Hilton 2000. Realistic synthesis of novel humanmovements from a database of motion capture examples. In Workshop on Human Motion 2000 (HUMO’00), 137-142, 2000.[6] O. Arikan and D. A. Forsyth. Interactive motion generation from examples. InProceedings of ACM SIGGGRAPH 2002, Annual Conference Series, ACM SIGGRAPH, 483-490, 2002.[7] J. Lee, J. Chai, P. Reitsma, J. Hodgins, and N. Pollard. Interactive control ofavatars animated with human motion data. In Proceedings of ACM SIGGGRAPH 2002, Annual Conference Series, ACM SIGGRAPH, 491-500, 2002.[8] M. Gleicher. Comparing Constraint-Based Motion Editing Methods. In GraphicalModels, 63(2):107-134, 2001.[9] M. Gleicher, H. J. Shin, L. Kovar, and A. Jepsen. Snap Together Motion:Assembling Run-Time Animation. In Symposium on Interactive 3D Graphics 2003, 181-188, April 2003.[10] L. Kovar, M. Gleicher and F. Pighin. Motion Graphs. In Proceedings of ACMSIGGGRAPH 2002, Annual Conference Series, ACM SIGGRAPH, 473-482, 2002.[11] C. Rose, M. F. Cohen, and B. Bodenheimer. Verbs and adverbs: Multi-dimensional motion interpolation. In IEEE Computer Graphics and Applications, 18(5):32-41, 1998.[12] O. Arikan, D. A. Forsyth, and J. F. O’Brien. Motion Synthesis from Annotations.In ACM Transactions on Graphics (TOG), 22(3):402-408, July 2003.[13] K. Pullen and C. Bregler. Motion capture assisted animation: Texturing andsynthesis. In Proceedings of ACM SIGGGRAPH 2002, Annual Conference Series, ACM SIGGRAPH, 501-508, 2002.[14] F. Bevilacqua, J. Ridenour, and D. J. Cuccia. 3D motion capture data: motionanalysis and mapping to music. In Proceedings of the Workshop/Symposium on Sensing and Input for Media-centric Systems 2002, Santa Barbara CA, 2002. [15] F. Bevilacqua, L. Naugle and I. Valverde. Virtual dance and music environmentusing motion capture. In Proceeding of IEEE Multimedia Technology And Applications Conference, Irvine CA, 2001.[16] T. Kim, S. I. Park, and S. Y. Shin. Rhythmic-motion synthesis based on motion-beat analysis. In ACM Transactions on Graphics (TOG), 22(3):392-401, July 2003.[17] G. Ahanger and T. D. C. Little. A survey of technologies for parsing and indexingdigital video. In Journal of Visual Communication and Image Representation, 7(1):28-43, 1996.[18] A. Dailianas, R. B. Allen, and P. England. Comparison of automatic videosegmentation algorithms. In Proceedings, SPIE Photonics East’95: Integration Issues in Large Commercial Media Delivery Systems, Philadelphia, 2615:2-16, Oct. 1995.[19] N. V. Patel and I. K. Sethi. Video shot detection and characterization for videodatabases. In Pattern Recognition, 30(4):583-592, April 1997.[20] S. Tsekeridou and I. Pitas. Content-based video parsing and indexing based onaudio-visual interaction. In IEEE Transactions on Circuits and Systems for Video Technology, 11(4):522-535, 2001.[21] M. S. Drew, Z.-N. Li, and X. Zhong. Video dissolve and wipe detection viaspatio-temporal images of chromatic histogram differences. In Proceeding of IEEE International Conference on Image Processing (ICIP 2000), 3:909-932, 2000.[22] R. Lienhart. Reliable dissolve detection. In Proceeding of SPIE Storage andRetrieval for Media Databases 2001, 4315:219-230, Jan. 2001.[23] R. Lienhart and A. Zaccarin. A system for reliable dissolve detection in video. InProceeding of IEEE International Conference on Image Processing (ICIP 2001), Thessaloniki, Greece, Oct. 2001.[24] Y. Wang, Z. Liu, and J.-Ch. Huang. Multimedia content analysis using both audioand visual clues. In IEEE Signal Processing Magazine, 17(6):12-36, Nov. 2000.[25] R. Zabih, J. Miller, and K. Mai. A feature-based algorithm for detecting andclassifying production effects. In ACM Journal of Multimedia Systems, 7:119-128, 1999.[26] Z. Černeková, C. Nikou, and I. Pitas, Entropy metrics used for videosummarization. In Proceedings of the 18th Spring Conference on Computer Graphics, Buderice, Slovakia, 73-82, 2002.[27] K. Kurihara, S. Hoshino, K. Yamane and Y. Nakamura. Optical motion capturesystem with pan-tilt camera tracking and realtime data processing. In Processings of 2002 IEEE International Conference on Robotics and Automation, 1241-1248, May 2002.2468101121241361frame number m u t u a l i n f o r m a t i o nFigure 1: The mutual information I f of an example motion sampled at 120 Hz. The displacement discretization level n = 256.dfd0.00.51.01.52.0localizedmutualinformation size is1 21 32 4 07 1 3 6 2 5 4。