The Value Grid for Semantic Technologies
【计算机应用与软件】_搜索技术_期刊发文热词逐年推荐_20140724
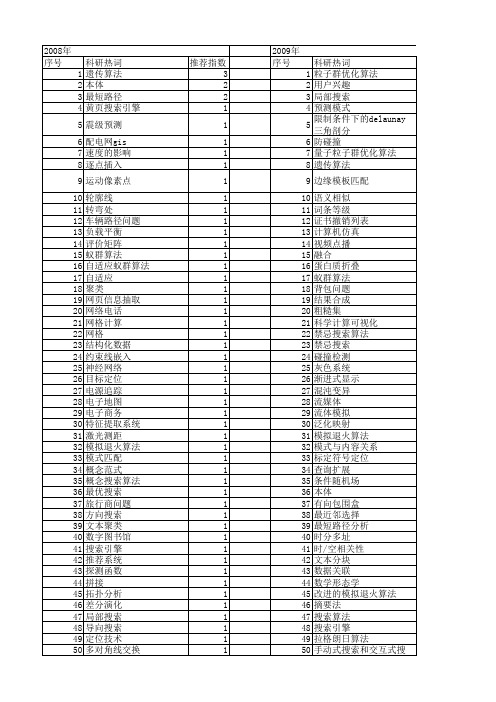
科研热词 搜索引擎 遗传算法 运动估计 自适应 垂直搜索 lucene 音乐数据库 遥测数据 迷宫搜索算法 运动拼接网格 路径规划 贝叶斯网络 调度 话题跟踪 话题检测 证书撤销 设计agent 认知无线电 蚂蚁算法 节点搜索 自动分流 聚类 网页清理 网络舆情 缺失数据 综合搜索 统计检验 统一数据接口 线性搜索 等高图 知识共享 直接解法 电脑鼠机器人 生长树 爬虫 熟收敛 灾变算子 混沌搜索 桌面搜索 标准遗传算法 栅格法 机器人 有解性 最优路径 时间步记忆搜索 无线传感器网络 族间交叉 旋律搜索 旅行商问题 数码问题 搜索算法 微粒群优化
推荐指数 2 2 2 1 1 1 1 1 1 1 1 1 1 1 1 1 1 1 1 1 1 1 1 1 1 1 1 1 1 1 1 1 1 1 1 1 1 1 1 1 1 1 1 1 1 1 1 1 1 1 1 1
2010年 序号 1 2 3 4 5 6 7 8 9 10 11 12 13 14 15 16 17 18 19 20 21 22 23 24 25 26 27 28 29 30 31 32 33 34 35 36 37 38 39 40 41 42 43 44 45 46 47 48 49 50 51 52
2009年 序号 1 2 3 4 5 6 7 8 9 10 11 12 13 14 15 16 17 18 19 20 21 22 23 24 25 26 27 28 29 30 31 32 33 34 35 36 37 38 39 40 41 42 43 44 45 46 47 48 49 50 51 52
1 1 1 1 1 1 1 1 1 1 1 1 1 1 1 1 1 1 1 1 1 1 1 1 1 1 1 1 1 1 1 1 1 1 1 1 1 1 1 1 1 1 1
梯度理论的发展及其意义
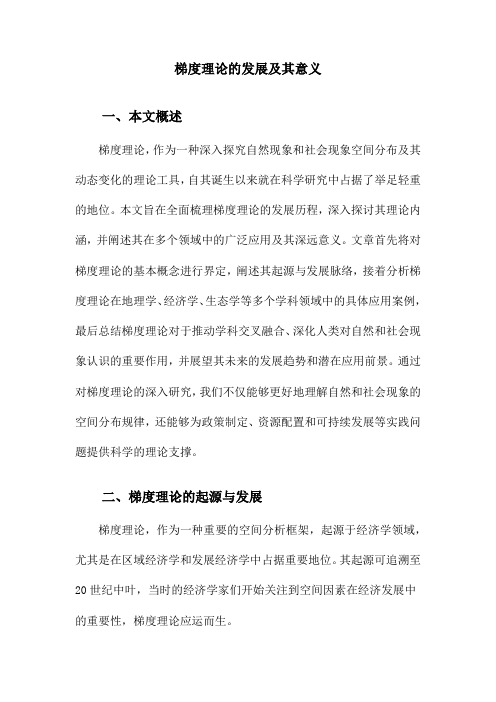
梯度理论的发展及其意义一、本文概述梯度理论,作为一种深入探究自然现象和社会现象空间分布及其动态变化的理论工具,自其诞生以来就在科学研究中占据了举足轻重的地位。
本文旨在全面梳理梯度理论的发展历程,深入探讨其理论内涵,并阐述其在多个领域中的广泛应用及其深远意义。
文章首先将对梯度理论的基本概念进行界定,阐述其起源与发展脉络,接着分析梯度理论在地理学、经济学、生态学等多个学科领域中的具体应用案例,最后总结梯度理论对于推动学科交叉融合、深化人类对自然和社会现象认识的重要作用,并展望其未来的发展趋势和潜在应用前景。
通过对梯度理论的深入研究,我们不仅能够更好地理解自然和社会现象的空间分布规律,还能够为政策制定、资源配置和可持续发展等实践问题提供科学的理论支撑。
二、梯度理论的起源与发展梯度理论,作为一种重要的空间分析框架,起源于经济学领域,尤其是在区域经济学和发展经济学中占据重要地位。
其起源可追溯至20世纪中叶,当时的经济学家们开始关注到空间因素在经济发展中的重要性,梯度理论应运而生。
梯度理论最初由美国经济学家埃德加·胡佛(Edgar Hoover)在20世纪50年代提出,他观察到在区域经济发展过程中,存在着一种由中心城市向外围地区扩散的经济活动梯度。
胡佛认为,这种梯度是由于资源、技术、信息等生产要素在空间中的不均匀分布所导致的。
随着研究的深入,梯度理论逐渐发展成为一种系统的空间经济理论。
其中,最具代表性的学者是瑞典经济学家冈纳·缪尔达尔(Gunnar Myrdal)。
他在其著作《经济理论与不发达地区》中,详细阐述了梯度理论的内涵和机制。
缪尔达尔认为,梯度现象是由经济发展的累积循环因果效应所导致的,富裕地区通过累积优势不断吸引资源和要素,形成经济发展的高地,而贫困地区则由于资源流失和要素不足而陷入低水平均衡陷阱。
此后,梯度理论不断得到完善和发展。
20世纪60年代,美国经济学家弗里德曼(John Friedmann)提出了著名的“核心-边缘”理论,进一步丰富了梯度理论的内容。
BAG a graph theoretic sequence clustering algorithm
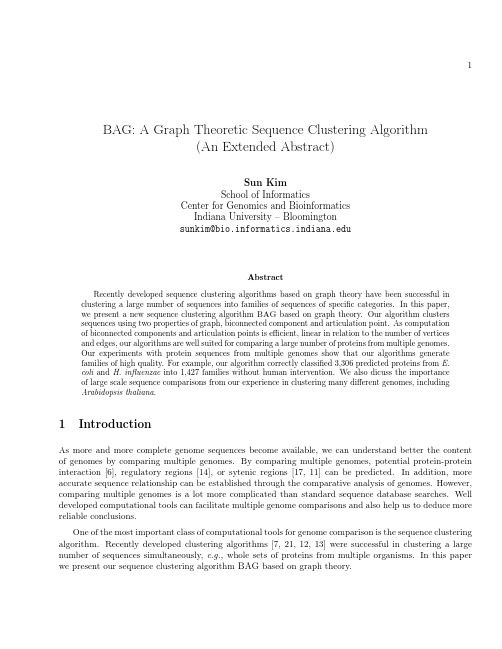
1 BAG:A Graph Theoretic Sequence Clustering Algorithm(An Extended Abstract)Sun KimSchool of InformaticsCenter for Genomics and BioinformaticsIndiana University–Bloomingtonsunkim@AbstractRecently developed sequence clustering algorithms based on graph theory have been successful in clustering a large number of sequences into families of sequences of specific categories.In this paper,we present a new sequence clustering algorithm BAG based on graph theory.Our algorithm clusterssequences using two properties of graph,biconnected component and articulation point.As computationof biconnected components and articulation points is efficient,linear in relation to the number of verticesand edges,our algorithms are well suited for comparing a large number of proteins from multiple genomes.Our experiments with protein sequences from multiple genomes show that our algorithms generatefamilies of high quality.For example,our algorithm correctly classified3,306predicted proteins from E.coli and H.influenzae into1,427families without human intervention.We also dicuss the importanceof large scale sequence comparisons from our experience in clustering many different genomes,includingArabidopsis thaliana.1IntroductionAs more and more complete genome sequences become available,we can understand better the content of genomes by comparing multiple genomes.By comparing multiple genomes,potential protein-protein interaction[6],regulatory regions[14],or sytenic regions[17,11]can be predicted.In addition,more accurate sequence relationship can be established through the comparative analysis of genomes.However, comparing multiple genomes is a lot more complicated than standard sequence database searches.Well developed computational tools can facilitate multiple genome comparisons and also help us to deduce more reliable conclusions.One of the most important class of computational tools for genome comparison is the sequence clustering algorithm.Recently developed clustering algorithms[7,21,12,13]were successful in clustering a large number of sequences simultaneously,e.g.,whole sets of proteins from multiple organisms.In this paper we present our sequence clustering algorithm BAG based on graph theory.2Pairwise Sequence Comparison to Genome ComparisonMost sequence clustering algorithms are based on the pairwise sequence comparison.There are many pairwise sequence alignment algorithms[1,19,20].These pairwise alignment algorithms are effective in detecting homology among sequences especially when similarity between sequences are relatively high.The most challenging task is to detect remotely related–distant in terms of sequence similarity–sequences. One effective method to detect remote homology is to use intermediate sequences[18].For example,a relationship between two sequences,s i and s j,may be detected via another sequence s k even when the sequence similarity between s i and s j cannot be detected by the pairwise alignment method.This can be seen as building a transitive relationship among the three sequences,i.e.,s i→s k→s j.Building the transitive relationships raises two important issues:what should be intermediate sequences?and how far can we build the trasitive relationships?Clustering algorithms use structures of sequence relationships to classify a set of sequences into families of sequences,F={F1,F2,...,F n}.While generating F,the remote homology detection issue and the transitivity bounding issue are systematically addressed with the structures of sequence relationships used by the clustering algorithm.Any two sequence,s i and s j in the same family F l={...,s i,s j,s k,..}are related by intermediate sequences,say s k,even when there is no observable relationship between s i and s j,thus remote homology detection issue is addressed.The sequences s i and s m in two different familiescould be related through intermediate sequences s m1,...,s mlbut such chaining of sequence relationshipsare blocked if the structure of sequence relationships used in the clustering algorithm classify s i and s m into two different families.Thus the transitivity issue is addressed.Recently developed sequence clustering algorithms were successful in clustering a large number of sequences into sequence families of highly specific categories[7,21,12,13].These clustering algorithms used graph theory explicitly or implicitly.In the following sections,we present our graph theoretic clustering sequence algorithm.3A New Graph Theoretic Sequence Clustering Algorithm3.1PreliminariesThe connected component of a graph is a subgraph where any two vertices in the subgraph are reachable from each other.An articulation point of G is a vertex whose removal disconnects G.For example,in Figure1the removal of a vertex s5disconnects G.A biconnected graph is a graph where there are at lest two disjoint paths for any pair of vertices.A biconnected component of G is a maximal biconnected subgraph.In Figure1,a subraph G1induced by vertices{s2,s3,s4}is a biconnected graph but it is not maximal since another subgraph G2induced by vertices{s1,s2,s3,s4,s5}is biconnected and G1is a subgraph of G2.There are two biconnected components,{s1,s2,s3,s4,s5}and{s5,s6,s7,s8,s9}of G in Figure1.3.2The basic algorithmWe present a new graph theoretic sequence clustering algorithm that explicitly uses two graph properties: biconnected components and articulation points(see Figure1).A biconnected component(BCC in short) corresponds to a family of sequences and an articulation point corresponds to a multidomian protein.As an articulation point is the only vertex that connects multiple biconnected components,i.e.,multiple families, it is intuitive to consider each articulation point as a candidate for multidomain sequence.Figure1:Biconnected components and articulation points.The vertex s5is an articulation point since removing the vertex results in separating the graph.A simple version of our algorithm works as follows.Given a set of sequences S={s1,s2,...,s n},pute similarities(s i,s j)for all1≤i,j≤n and i=j.2.Build a sequence graph G from the pairwise matches above a preset cutoffthreshold.3.Generate a set of subgraphs,{G1,G2,...,G m},each of which G i is a biconnected component.4.Then a set of vertices in each subgraph G i forms a family of sequences and each articulation pointbecomes a candidate for multidomain sequence.To reduce computation time in Step1,we can use well accepted approximation algorithms such as FASTA[19]or BLAST[1,2].We simply choose FASTA for the pairwise computation,and the computation is FASTA(s i,S)for all1≤i≤n.All pairwise comparisons can be computed once and saved for later use,especially for completely sequenced genomes.Indeed,there are several databases for precomputed all pairwise comparisons,e.g.,[16,5].From the precomputed pairwise comparison databases,we can retrieve pairwise comparisons for selected genomes for clustering analysis.Our algorithm is well suited for this type of comparative clustering analysis for an arbitrary set of genomes due to the computational efficiency,i.e,linear time complexity in relation to the number of edges.3.3Result from application of the basic algorithmWe performed a clustering analysis of all5,998predicted protein sequences from E.coli(GenBank ac-cession number U00096,4289proteins)and H.influenzae(GenBank accession number L42023,1709 proteins)with Zscore cutoffof400.1426families of3431sequences were clustered excluding families ofa single sequence(these families are uninformative).Most of the resulting families are clustered correctly with high precision according to the current annotation.However,there are cases where we cannot easily verify the correctness of the clustering result from the annotation.For example,it was not obvious that Family285is clustered correctly from the annotations in the heading as shown below.>gi|1573256|gb|AAC21953.1|L-serine deaminase(sdaA)[Haemophilus influenzae Rd]>gi|1788116|gb|AAC74884.1|L-serine deaminase[Escherichia coli K12]>gi|1789161|gb|AAC75839.1|L-serine dehydratase(deaminase),L-SD2[Escherichia coli K12] >gi|1789498|gb|AAC76146.1|putative L-serine dehydratase[Escherichia coli K12]>gi|1789500|gb|AAC76147.1|putative L-serine dehydratase[Escherichia coli K12](Hinf, SDH_A & B)1573256(Ecoli, SDH_B)1789500(Ecoli, SDH_A)1789498(Ecoli, SDH_A & B)(Ecoli, SDH_A & B)2552153857223752401166416275886101788116 1789161Figure 2:Sequence graphs with the Zscore cutoffthreshold of 400.The numbers on the edges denote the Zscores between two sequences.SDH αand SDH βand were detected by Pfam search.To verify the clustering result,we performed protein domain search using Pfam [3]7.0and found that there are two different domains,SDH αand SDH βin the family as shown in Figure 2.The family begin to separate into two families at a stricter cutoffvalue of 600as shown in Figure 3,i.e.,two BCCs,{1788116,1789161,1789498,1573256}and {1788116,1789500}1,and an articulation point,1788116.However,the question is how do we know the cutoffthreshold value 600apriori?.In addition,different families are characterized at different cutoffvalues in general.This fundamental issue will be effectively addressed in the extended version of our algorithm in the following section.4Bag :The Extended Clustering AlgorithmThe basic algorithm in Section 3.2is extended and called Biconnected components and Articulation points based Grouping of Sequences (Bag ).4.1Issues with the basic algorithmSeveral features of the basic algorithm presented in the previous section need attention:1.The cutoffthreshold setting issue :as shown with the example of SDH αand SDH βdomain proteins,we do not know the cutoffthreshold apriori ,Zscore 600for the example.2.Merging families :given a cutoffthreshold,several families may need to be tested for merging since the cutoffvalue may be too stringent so that sequences with the same domains are separated into multiple families.3.Spitting a family ;given a cutoffthreshold,a family may need to be tested for splitting into several ones.For example,sequences with SDH αand SDH βdomains in Figure 2.1The family {1788116,1789500}will be extended to include the two sequences,1573256and 1789161,that have the SDH βdomain,while the family is considered for merging through an articulation point,1788116(See the next section).(Hinf, SDH_A & B)1573256(Ecoli, SDH_A & B)(Ecoli, SDH_A & B)1788116 17891611789498(Ecoli, SDH_A)(Ecoli, SDH_B)1789500Figure 3:Sequence graphs with the Zscore cutoffthreshold of 600.The graph has two BCCs,{1788116,1789161,1789498,1573256}and {1788116,1789500},and an articulation point,1788116.4.Multidomain protein :how do we know an articulation point truly corresponds to a multidomain protein?Each of these features will be explored in the following subsections.4.2Setting the cutoffthresholdWe performed a series of clustering analyses with Zscore cutoffthresholds ranging from 100to 1000at 50increment intervals for the pairwise comparisons from E.coli and H.influenzae .The plot on the left side in Figure 4is the distribution of the number of biconnected components vs.the Zscore cutoffthreshold.We will call this plot the BCC plot .As we can observe in the figure,the number of biconnected components increases up to a certain value,150for Zscore,and then continue to decrease.The increase in the number of biconnected components is intuitive as a higher cutoffvalue will remove more false positives,thereby families of large size due to false positives being separated into several families.The decrease in the number of biconnected components is also intuitive as a higher cutoffvalue will remove more true positives,thereby more vertices become singletons,i.e.,vertices without incident edges;note that singletons are not counted.We would expect that there exists a peak in the BCC plot if the scoring method like Zscore effectively models the pairwise sequence relationship.This observation is consistent with non-statistical scores like Smith-Waterman score [10].Note that the basic clustering algorithm runs linear time in relation to the number of pairwise matches above a preset cutoffthreshold after computing pairwise matches from a set of sequences.The series of clustering analysis with Zscore in Figure 4took less than 6seconds on a Pentium IV 1.7GHz processor machine running Linux.This computational efficiency makes it possible to efficiently conduct the series of clustering analyses with varying cutoffthresholds to find the cutoffthreshold,C maxbiconn ,that generates the maximum number of biconnected components.However,we need to consider the number of articulation points since articulation points are candidates for multidomain proteins.The right plot in Figure 4shows the number of articulation points with respect to varying Zscore cutoffthresholds.The articulation points become candidates for multidomain proteins and need to be tested for having multidomain proteins:the0500100015002000020*******8001000N o o f b i c o n n e c t e d c o m p o n e n t s Zscore cutoff value E.coli + Hinf 05010015020025030035040002004006008001000N o o f a r t i c u l a t i o n p o i n t s Zscore cutoff value E.coli + Hinf Figure 4:The distribution of the number of biconnected components vs.the Zscore cutoffthreshold (left plot)and the distribution of the number of articulation points vs.the Zscore cutoffthreshold (right).test method will be briefly described in the following sections.We would avoid selecting the cutoffthreshold with too many articulation points.Let NAP C the number of articulation points at score C .One way to select the cutoffvalue is to use a ratio r =NAP C +I NAP C where I is the interval of the score for the series of clustering analysis.4.3The overview of the extended algorithmWe will describe the overview of the extended algorithm due to the space limitation of the paper.Details of the algorithm can be found in [10].Our algorithm BAG works as follows:1.Build a graph G from all pairwise comparisons result.2.Run the basic algorithm with cutoffscores ranging from C 1to C 2at each interval I and select a score,C maxbiconn ,where the number of biconnected components is the largest,and another score,C arti ,where the number of articulation points begin to decrease at a ratio r ≥∆.3.Select a cutoffscore C arti and generate biconnected components,G 1,G 2,...,G n with a set of articu-lation points {A 1,A 2,...,A m }.4.Iteratively split a biconnected components into several ones with more stringent cutoffscores until there is no candidate component for splitting.5.Iteratively merge a set of biconnected components into one with relaxing the cutoffscore to C maxbiconn until there is no candidate component for merging.When merging two biconnected components fails,consider a partial merging by relaxing cutoffscore to C maxbiconn (see the case in Figure 3).The overall procedure can be summarized in two steps:(1)generation of candidate families and (2)refinement of the families by merging and splitting.The fundamental question is which biconnected components need to be refined,i.e.,splitting (Step 3)and merging (Step 4).For this purpose,we propose two tests as below.: TonB_boxC domainFigure5:The region shared among gi1573631,gi1573714,gi3212198,and gi3212230can be computedby chaining pairwise overlaps.All four sequences share domains,that are not present in the Pfam database,and they are annotated to share the hemoglobin binding domain.The three sequences,gi1573631,gi1573714,and gi3212198,share the TonB boxC domain in the Pfam database.This clustering result demonstrates that our algorithm correctly cluster sequences even when multiple domains are involved.1.AP-TEST tests an articulation point for having potential multidomains.2.RANGE-TEST tests each biconnected component for being a single family.These two tests are to see if there are common shared regions among the sequences.For example,Figure5shows that four sequences share common subsequence regions.Depending on the test result,splitting and merging operations are performed in a greedy fashion,i.e.,a resulting subgraph from a splitting or merging operation is not considered for further splitting or merging.5Clustering Two Bacterial GenomesIn this section,we will discuss the application of our clustering algorithm to clustering entire protein se-quences from complete genomes.With the current prototype implemented in C++with the LEDA[15] package,we were able to compare many different sets of genomes.An analysis of B.burgdorferi and T. pallidum can be found in[10].In this section,we describe a complete analysis of two bacterial genomes,E.coli and H.influenzae.Due to the space limitation,we cannot present a complete description.The more de-tailed clustering result will be available at /sunkim/BAG/ecoli.hinf/.As shown in Figure4,we picked150for C maxbiconn and400for C arti(see Section4.2)and the clustering analysis starts with Zscore400,i.e.,C arti,which clusters1,391families with103articulation points. Among103articulation points,18failed for AP-TEST,which implies families around these articulation points does share some domains in common,thus the families connected through the18articulation pointsare not considered for merging.Among1,391families,9failed for RANGE-TEST,which implies that each subgraph have multiple families and need to be split.We used I split=50for splitting families andI merge=50for merging families.Family Zscore Split families Sequences Common domains6145061.11573631157371432121983212230no domain detected61.215740243212230no domain detected181800181.1157308017892511790097Sulfate transp17901501790499xan ur permease181.217892501790499no domain detected 285600285.115732561788116SDH alpha17891611789498285.217891611789500SDH beta 11666001166.117863241788577178864317898161790796no domain detected1166.217886431790795no domain detected 11674501167.1178633217867441786937Usher17871721787782178842717886791789533179077223671881167.217896101788678no domain detectedTable1:The families failed for RANGE-TEST were separated into subfamilies of the same functional domains.Note that domains not common in the subfamilies are not shown.Thus,“no domain detected”means that there is no domain shared among all family members and each family member may have domains detected by Pfam search.Splitting families The list of5families among9families failed for RANGE-TEST is shown in Table??. These families are expected to have multiple domains that lead to the failure for RANGE-TEST.This can be verified either by Pfam search or by sequnece alignment with respect to the multidomain candidate(for example,aligning6sequences of the family61with repect to3212230in Table1).After splitting,there were1,427families.Among them,227families did not have any domains detected by the Pfam search. Merging families A hypergraph was formed for merging families:families from the previous step(Split-ting step)become nodes and two nodes(families)are connected if there is a sequence present in both families.All families in each biconnected component in the hypergraph are considered for merging.There were165cluster merging events.Table2shows examples of merging events.6The Importance of Large Scale Sequence ComparisonsWe performed another clustering analysis from25,545predicted protein sequences from Arabidopsis thaliana genome.4,614families with106articulation points were computed with C biconn=400and C arti=500. We are still in the process of verifying the clustering result.However,from the Pfam search result,we verified most of families with domains,that can be detected in the Pfam database,are clustered correctly.We found an interesting observation in the BCC plot of the Arabidopsis thaliana genome as shown in Figure6.Intuitively,the number of biconnected components increases as more proteins from genomes are compared since more genes(proteins)can be matched.However,what was really interesting was thatNew family Families Sequences Common domains 47-484717888541573186GATase(2),GMP synt C(2),tRNA Me trans4815731863212188GATase(2),GMP synt C,IMPDH C 77-78-797717862681790194lacI(2),Peripla BP like(2)7815744811789456lacI(2),Peripla BP like(2)79157348715738341574481lacI(2)17865401787580178790617879481788474178906817892021789846179019417903691790715111-112-113111178881117892531789606Amino oxidase,FAD binding3fer4pyr redox(2) 112178881117890672367245fer4(2)113157295015740801574621fer411317871221787749178787211317879601789370179032611323672452367345176-177-178176178651817907202-Hacid DH C,adh zinc(2) 17717877531790720adh zinc(2)178157300017877531787863adh zinc178807317884071788895179004517907181790819220-22122017892501790499xan ur permease 221157308017892511790097Sulfate transp,xan ur permease(2)17901501790499425-426-42742517893011790027no domain detected 42617884941790027PTS EIIA2(2)42715734241788494PTS EIIA2(2),PTS-HPr(2) 783-784-7857831787661178868217902813HCDH(2),3HCDH N(2),ECH 78417862201788682ECH(2)78517862201787659ECH(2)17876601789286Table2:Examples for merging events.The number in the parantheses at the end of a domain name denotes the number of occurrences of the domain.Even though several families can be merged into one, each family were initially grouped into ones of specific category.For example,families111,112,and113 can be merged into one that shares a single occurrence of the fer4domain.However,the three families were grouped correctly according to domains in the families;all members in the family111have Amino oxidase, FAD binding3,fer4,and pyr redox(2),and all members in the family112have two occurrences of the fer4 domain while all memerbs in the family113have only one occurrence of the fer4domain.The merging event also detects multidomain proteins.For example,1790027in the merging of425-426-427shares unknown domains with1789301,in addition to the PTS EIIA2domain.050010001500200025003000350040004500500001002003004005006007008009001000N o o f b i c o n n e c t e d c o m p o n e n t s Zscore cutoff value Arabidopsis 050010001500200002004006008001000N o o f b i c o n n e c t e d c o m p o n e n t s Zscore cutoff value E.coli + Hinf E.coli Hinf Figure 6:The plots of the number of biconnected components vs.Zscore cutoffthresholds:the left plot for Arabidopsis thaliana and the right plot for the bacterial genomes (E.coli and H.influenzae ).The number of BCCs does not decrease much as the cutoffincreases up to 1000in the clustering analysis of Arabidopsis thaliana ;the decreasing ratio can be measured in relation to the maximum number of BCCs.We call this the plataeu in the BCC plot,which begins to appear as a larger set of sequences are compared.the number of biconnected components did not decrease as fast as in the analysis of the two bacterial genomes.For example,the number of biconnected components at the Zscore cutoffof 1000was 3,587.What was happening was that many sequences in the same family have two or more separate paths –thus biconnected –through strong sequence similarities when many sequences are compared.In the Arabidopsis thaliana analysis,the numbers of BCCs vary less than 4%from the maximum BCC number at 400in the range of the cutoffvalues from 350to 650,which is almost flat.We call the observation the plateau in the BCC plot.The plataeu can be observed in the articulation plot as well.This observation provides more confidence in the clustering results since the numbers of candidates for families and multidomain proteins do not change much.The plataeu appears as larger sets of sequences are compared as shown in Figure 6:four BCC plots for two bacterial genomes (E.coli and H.influenzae )separately,two bacterial genomes combined,and Arabidopsis thaliana .The plataeu can be measured in terms of the decreasing ratio in relation to the maximum number of BCCs.This result supports the importance of large scale genome comparisons:the more genomes are compared the stronger sequence relationship,i.e.,similarity,can be used for clustering sequences,thus we can be more confident of the clustering result.More rigorous and detailed result will be reported in the forthcoming paper.7ConclusionAs more sequences become available in an exponential rate,sequence analysis on a large number of se-quences will be increasingly important.Sequence clustering algorithms are computational tools for that purpose.In this paper,we presented our clustering algorithm,BAG ,that used two graph properties,biconnected components and articulation points.Our algorithm were successful in clustering sequences into families of specific categories using the pairwise sequence comparison information only.For example,families in Figure5were clustered correctly to shares hemoglobin binding domains even when multiple domains are involved(a subset of the family share the TonB boxC domain),and families in Section3.3were separated into two families of SDHαand SDHβ.Note that these two family classifications cannot be achieved by either Pfam search(for families in Figure5)or by looking at annotations(for families in Section3.3).In addition,our algorithm can help to detect previously uncharacterized domains.For example,in the clustering analysis of E.coli and H. influenzae,227families did not have any domains detected by the Pfam search.Our algorithm utilizes the computational efficiency,i.e.,linear time complexity,to achieve clustering of families of very specific categories.In particular,our algorithm was successful in classifying families where the relationships among member sequences were defined at different scores;for example,Family181.1and 181.2can be separated at Zscore800but not at Zscore400where most of families were classified(see Table 1).As a result,families were clustered with highly specific precision.For example,families in Table2were separated even at the level of the number of domain occurrences;families111-112-113and176-177-178.Why can our algorithm cluster sequences into families of very specific categories?This can be explained in terms of previous work in the literature.First of all,use of intermediate sequences can detect remote homology[18].Grundy2demonstrated that a simple family pairwise search technique can classify sequences with high accuracy[9].Clustering from all pairwise comparisons incorporates these two techniques in a systematic way with structures of a graph.Thus,we would expect that clustering algorithms based on graph theory can cluster sequences into famlies of very specific categories.The future work for our algorithm includes applications of our algorithm to different types of sequences such as DNA and EST sequences.It would also be interesting to retain the hierarchical structure of the merging procedure so that sequence relationships can be seen at different levels.In addition,refining further each family in the context of genome,i.e.,orthologs as used in COG,is an interesting topic for further research.References[1]Altschul,S.F.,Gish,W.,Miller,W.,Myers,E.W.and Lipman,D.J.(1990)“Basic local alignmentsearch tool,”Journal of Molecular Biology215403-410.[2]Altschul,S.F.,Madden,T.L.,Schffer,A.A.,Zhang,J.,Zhang,Z.,Miller,W.and Lipman,D.J.(1997)“Gapped BLAST and PSI-BLAST:a new generation of protein database search programs,”Nucleic Acids Research,253389-3402.[3]Bateman,A.,Birney,E.,Durbin,R.,Eddy,S.R.,Howe,K.L.,and Sonnhammer,E.L.L.,(2000)“The Pfam Protein Families Database,”Nucleic Acids Research,28263-266.[4]G.Cannarozzi,M.T.Hallett,J.Norberg,and X.Zhou(2000)“A cross-comparison of a large datasetof genes,”Bioinformatics16654-655[5]Gilbert,D.G.(2002)“euGenes:a eukaryote genome information system,”Nucleic Acids Research,30,145-148[6]Enright A.J.,Iliopoulos I.,Krypides N.,Ouzounis C.A.,(1999)’Protein interaction maps for completegenomes based on gene fusion events’Nature402,86-902The author’s name changed to William Stafford Noble from William Noble Grundy.。
nature method points of view -回复
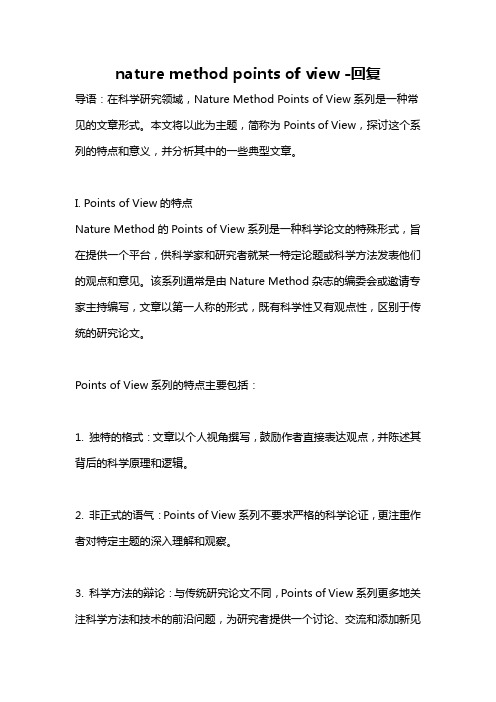
nature method points of view -回复导语:在科学研究领域,Nature Method Points of View系列是一种常见的文章形式。
本文将以此为主题,简称为Points of View,探讨这个系列的特点和意义,并分析其中的一些典型文章。
I. Points of View的特点Nature Method的Points of View系列是一种科学论文的特殊形式,旨在提供一个平台,供科学家和研究者就某一特定论题或科学方法发表他们的观点和意见。
该系列通常是由Nature Method杂志的编委会或邀请专家主持编写,文章以第一人称的形式,既有科学性又有观点性,区别于传统的研究论文。
Points of View系列的特点主要包括:1. 独特的格式:文章以个人视角撰写,鼓励作者直接表达观点,并陈述其背后的科学原理和逻辑。
2. 非正式的语气:Points of View系列不要求严格的科学论证,更注重作者对特定主题的深入理解和观察。
3. 科学方法的辩论:与传统研究论文不同,Points of View系列更多地关注科学方法和技术的前沿问题,为研究者提供一个讨论、交流和添加新见解的平台。
II. Points of View的意义1. 激发创新思考:Points of View系列提供了一个自由表达观点的机会,鼓励研究者在科学领域做出新的思考和突破。
通过这种非传统的论文形式,科学家们可以分享他们对科学问题的深入见解,推动科学研究的创新发展。
2. 促进科学交流:Points of View系列为科学社区提供一个开放的平台,使科学家们能够分享自己的观点和经验。
这种交流不仅促进科学界内部的合作与互动,还有助于学术界和产业界之间的对话与合作。
3. 引导科学发展方向:Points of View系列对于科学领域的发展具有引导作用。
通过探讨和评估新的科学方法和技术,文章可以对研究方向进行引导,推动科学研究从理论向实践的转变。
格洛弗算法

格洛弗算法格洛弗算法(GloVe)是一种用于词向量表示的算法,它结合了全局词汇共现统计和局部上下文窗口的信息,能够更好地捕捉词语之间的语义关系。
本文将详细介绍格洛弗算法的原理、应用以及一些相关的优化技巧。
1. 算法原理格洛弗算法的核心思想是通过对全局词汇共现矩阵进行分解,得到每个词语的向量表示。
具体而言,算法首先构建一个全局词汇共现矩阵,其中每个元素表示两个词语在上下文窗口中共同出现的次数。
然后,通过最小化目标函数来学习每个词语的向量表示。
目标函数包括两部分:全局共现统计和局部上下文窗口。
全局共现统计通过计算每对词语之间在整个语料库中共同出现的次数来衡量它们之间的关联程度。
而局部上下文窗口则考虑了每个词语与其周围邻居词语之间的关系。
具体来说,假设我们有一个大小为V×V(V为词汇表大小)的共现矩阵X,其中第i行第j列的元素表示词语i和词语j在上下文窗口中共同出现的次数。
那么,我们可以将每个词语的向量表示表示为两个向量的乘积:词语i的词向量和词语j的词向量的转置。
为了学习这些词向量,我们需要最小化以下目标函数:(w_i%5ETw_j-b_%7Bij%7D)%5E2)其中,wi和wj分别表示词语i和词语j的词向量,Xij表示共现矩阵中第i行第j列的元素,bij是一个偏差项(用于调整不同单词之间共现次数对目标函数的影响),f是一个权重函数。
通过最小化这个目标函数,我们可以得到每个词语对应的向量表示。
这些向量捕捉了单词之间丰富而复杂的关系,并可以用于各种自然语言处理任务。
2. 算法应用格洛弗算法在自然语言处理领域有广泛的应用。
以下是一些常见的应用场景:2.1 词语相似度计算通过学习到的词向量,我们可以计算两个词语之间的相似度。
一种常见的计算方法是使用余弦相似度,即计算两个词向量之间的夹角。
相似度较高的词语往往在语义上更接近。
2.2 词语聚类通过对学习到的词向量进行聚类,我们可以将具有相似语义特征的词语分组在一起。
一族多维Copula的理论介绍与实证分析的开题报告

一族多维Copula的理论介绍与实证分析的开题报告题目:一族多维Copula的理论介绍与实证分析研究背景:Copula理论是金融风险管理领域中经常使用的方法之一。
它是用来描述两个或多个变量之间的依赖关系的概率分布函数。
在实际应用中,经常需要使用多维的Copula分布来描述资产之间的相互依赖关系。
研究内容:1. Copula理论的基本原理和概念介绍。
包括Copula的定义、性质、多元分布函数、多维Copula的构造等基本概念。
2. 多维Copula的实证分析。
对多维Copula在金融领域的实际应用进行研究,例如在风险测度、投资组合优化、市场风险分析、债券定价等方面的应用。
3. 一族多维Copula的理论研究。
研究一族多维Copula的构造方法和性质,例如经典的Gumbel Copula、Clayton Copula、Frank Copula以及更多的扩展形式。
4. 实证分析和模拟研究。
通过实证分析和模拟研究,将新构造的多维Copula应用于金融风险管理领域中的实际问题,并与传统的多维Copula方法进行比较和验证。
研究方法:1. 文献综述:对Copula理论的相关文献进行综述和分析,总结Copula相关理论及其在金融领域的应用现状。
2. 实证分析:通过实际数据样本,应用多维Copula进行实证分析。
同时,应用Monte Carlo模拟方法对模拟数据进行分析。
3. 确定一族多维Copula模型:从传统Copula模型中发掘其不足之处, 提出一族多维Copula,探究其性质和构造方法。
研究意义:本研究将有助于深入了解Copula理论及在金融风险管理领域的应用。
对于构造新的多维Copula模型,对于改进目前金融风险管理中的实际问题将具有实际的帮助和应用意义。
同时也将拓展学术界对Copula理论相关的研究领域,扩大其应用范围。
预期结果:本研究将设计出一种更为实用的多维Copula模型,并将其应用于实际金融风险管理中,提高金融风险管理的可行性和更有效的风险控制。
基于方向可控金字塔二值图像投影的图像检索
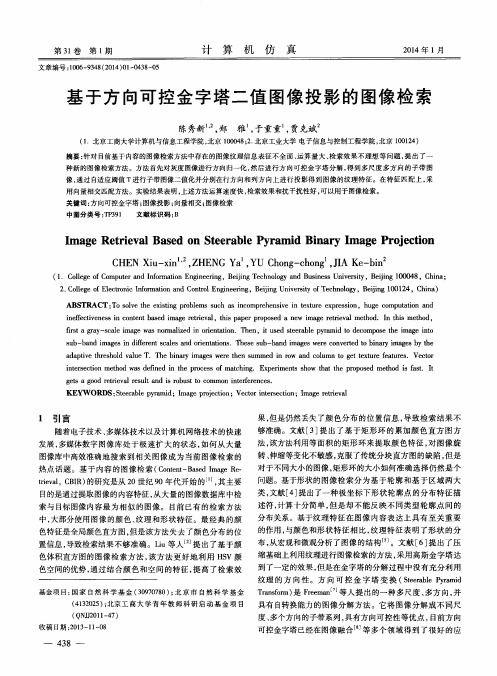
像, 通过自 适应 阈值 T 进行 子带 图像二值化并分别在行方向和列方 向上进行投影 得到图像 的纹 理特征。在特征 匹配上 , 采
用向量相交匹配方法 。实验结果表 明, 上述方法运算速度快 , 检索效果 和抗干扰性好 , 可 以用于 图像检索 。 关键词 : 方 向可控金字塔 ; 图像投影 ; 向量相交 ; 图像检索
i f st r a g r a y - s c a l e i ma g e w a s n o ma r li z e d i n o i r e n t a t i o n .T h e n,i t u s e d s t e e r a b l e p y r a mi d t o d e c o mp o s e t h e i ma g e i n t o s u b - b a n d i ma g e s i n d i f f e r e n t s c a l e s a n d o i r e n t a t i o n s .T h e s e s u b - b a n d i ma g e s we r e c o n v e r t e d t o b i n a r y i ma g e s b y t h e a d a p t i v e t h r e s h o l d v lu a e T .T h e b i n a r y i ma g e s w e r e t h e n s u mme d i n r o w a n d c o l u mn t o g e t t e x t u r e f e a t u r e s . Ve c t o r i n t e r s e c t i o n me t h o d w a s d e i f n e d i n t h e p r o c e s s o f ma t c h i n g .E x p e ime r n t s s h o w t h a t t h e p r o p o s e d me t h o d i s f a s t .I t
多层线性模型——原理与应用
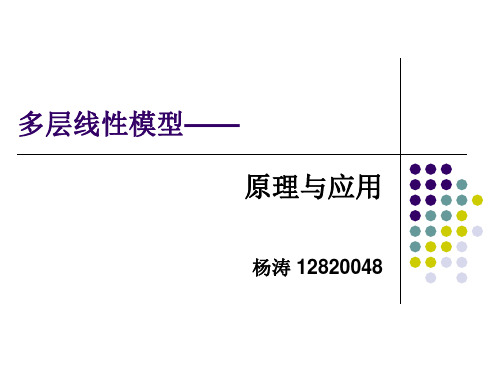
组织环境(W)
(1)求各个组织个体成员的成就目标导向对创造力的回
归
Yij 0 j 1 j X ij rij
(2)求组织环境对 0 j 和 1j 的回归方程
0 j 00 01W j 0 j
1 j 10 11W j 1 j
一、多层线性模型简介
✓ 4、多层线性模型的优点 (1)使用收缩估计的参数估计方法,使得估计结果更为
一、多层线性模型简介
✓ 5、多层线性模型的优点 (1) 用于类似组织管理、学校教育等具有多层数据结
构的领域研究。 ( 2) 用于个体重复测量数据的追踪研究。测量层面作
为第一水平,个体层面作为第二水平。 ( 3) 用于做文献综述,即对众多研究成果进行定量综
合。探讨不同研究中进行的处理、研究方法、被试特征和 背景上的差异与效应之间的关系。
组内分析组间分析的方法较前两种方法更多的考虑到了 第一层数据及第二层数据对变异产生的影响,但并无法对 组内效应和组间效应做出具体的解释,也就无法解释为什 么在不同的组变量间的关系存在差异。
一、多层线性模型简介
✓ 3、多层线性模型分析方法 回归的回归方法 Eg:个体成就目标导向(X)
个体创造力(Y)
Level-2: 0 j 00 u0 j var(u0 j ) 00 0 j 指第j个二层单位Y的平均值; eij 反应第j个二层单位对Y的随机效应; 00 指所有二层单位的Y的总体平均数; u0 j 指第二层方程的残差(随机误差项)。
二、多层线性模型基本原理
组内相关系数 ICC 00 / ( 00 2 )
二、多层线性模型基本原理
(4) 充分利用多层模型较为高级的统计估计方法来改 善单层回归的估计和分析。
二、多层线性模型基本原理
网格经济学模型
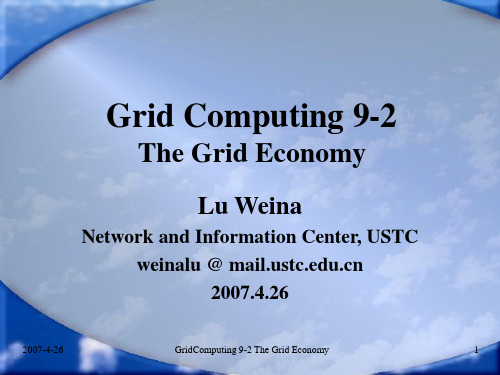
28/61
Outline
• • • • • INTRODUCTION 资源作为一种商品格经济学模型 定价、计费和付费机制 几个相关项目 博弈论
2007-4-26
GridComputing 9-2 The Grid Economy
29/61
Compute Power Market
• CPM 计算力市场是网格环境下基于市场机制的资源和 作业调度系统,它特别是针对低端个人计算设备设计 的。传送元计算环境到一个计算市场,通过闲置的资 源租用计算力,存贮,和特殊服务在计算市场中解决 问题。CPM主要由市场,资源消费者,资源提供者和 它们的相互作用组成。它支持商品市场模型、合约模 型、拍卖模型。
GridComputing 9-2 The Grid Economy
23/61
Outline
• • • • • OVERVIEW 网格经济学模型 定价、计费和付费机制 几个相关项目 博弈论
2007-4-26
GridComputing 9-2 The Grid Economy
24/61
定价、计费、付费
2007-4-26
GridComputing 9-2 The Grid Economy
20/61
拍卖模型
2007-4-26
GridComputing 9-2 The Grid Economy
21/61
拍卖模型
• 拍卖模型 – 上升拍卖(英式拍卖) – 下降拍卖(荷兰式拍卖) – 第一价格密封拍卖 – 第二价格密封拍卖(Vickrey拍卖) – ......
2007-4-26
GridComputing 9-2 The Grid Economy
2007-4-26
the rising sea 代数几何 发表
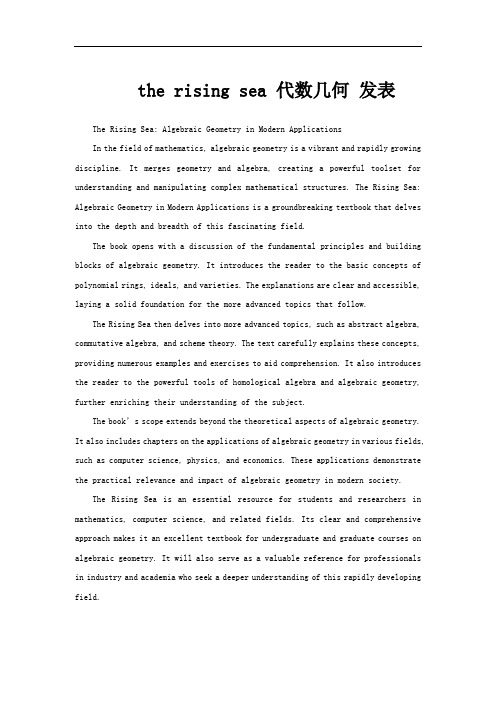
the rising sea 代数几何发表The Rising Sea: Algebraic Geometry in Modern ApplicationsIn the field of mathematics, algebraic geometry is a vibrant and rapidly growing discipline. It merges geometry and algebra, creating a powerful toolset for understanding and manipulating complex mathematical structures. The Rising Sea: Algebraic Geometry in Modern Applications is a groundbreaking textbook that delves into the depth and breadth of this fascinating field.The book opens with a discussion of the fundamental principles and building blocks of algebraic geometry. It introduces the reader to the basic concepts of polynomial rings, ideals, and varieties. The explanations are clear and accessible, laying a solid foundation for the more advanced topics that follow.The Rising Sea then delves into more advanced topics, such as abstract algebra, commutative algebra, and scheme theory. The text carefully explains these concepts, providing numerous examples and exercises to aid comprehension. It also introduces the reader to the powerful tools of homological algebra and algebraic geometry, further enriching their understanding of the subject.The book’s scope extends beyond the theoretical aspects of algebraic geometry. It also includes chapters on the applications of algebraic geometry in various fields, such as computer science, physics, and economics. These applications demonstrate the practical relevance and impact of algebraic geometry in modern society.The Rising Sea is an essential resource for students and researchers in mathematics, computer science, and related fields. Its clear and comprehensive approach makes it an excellent textbook for undergraduate and graduate courses on algebraic geometry. It will also serve as a valuable reference for professionals in industry and academia who seek a deeper understanding of this rapidly developing field.。
A Tutorial on Support Vector Machines for Pattern Recognition

burges@
Bell Laboratories, Lucent Technologies Editor: Usama Fayyad Abstract. The tutorial starts with an overview of the concepts of VC dimension and structural risk minimization. We then describe linear Support Vector Machines (SVMs) for separable and non-separable data, working through a non-trivial example in detail. We describe a mechanical analogy, and discuss when SVM solutions are unique and when they are global. We describe how support vector training can be practically implemented, and discuss in detail the kernel mapping technique which is used to construct SVM solutions which are nonlinear in the data. We show how Support Vector machines can have very large (even infinite) VC dimension by computing the VC dimension for homogeneous polynomial and Gaussian radial basis function kernels. While very high VC dimension would normally bode ill for generalization performance, and while at present there exists no theory which shows that good generalization performance is guaranteed for SVMs, there are several arguments which support the observed high accuracy of SVMs, which we review. Results of some experiments which were inspired by these arguments are also presented. We give numerous examples and proofs of most of the key theorems. There is new material, and I hope that the reader will find that even old material is cast in a fresh light. Keywords: support vector machines, statistical learning theory, VC dimension, pattern recognition
多维空间一般Benjamin-Bona-Mahony方程解的逐点估计

u 一 △O t u
叩是正常数, 是实常向量. f ( u ) ( f l ( u ) , …, ( u ) ) 给定初值为
u I : 0 =U 0 .
( 2 )
这里拉普拉斯算子 △ = ∑ , n是空间维数.
j =l
方程 ( 1 ) 叫做一般 B e n j a mi n - B o n a - Ma h o n y 方程. 著名的 B B M方程是由 B e n j a m i n , B o n a 和 Ma h o n y在文献 『 1 1 中提 出的,该 方程是 作 为研 究非 线性色散 媒 介里 的长波 传播 的模 型方 程.在文献 [ 2 ] 中,郭提出并研究了多维空间里 的一般 B B M 方程 ( G B B M) . C B B M 方程解 的存在性和唯一性已经被多名作者证 明 ( 文献 [ 1 — 3 ] ) . 解的衰减性也在文献 [ 4 — 7 ] 中被研究. 但是他们所有的结果都是在 范数意义下.本文 中,我们给 出了这个方程解的更为精确的 逐点衰减估计, 且这个估计是最优的.由于我们的估计首先要基于解的整体存在性, 所以先 把整体存在性结果列 出来. 定理 1 . 1 令 球体
在本文中, 我们用 C表示一般常数. f=f e - i x .  ̄ f ( x ) d x表示函数 ,的傅里叶变换.
F ( , ) 表 示 函数 l 厂的逆傅 里 叶变换 . 示 通 常的 S o b o l e v空 间,范 数形 式 为 是 一个 实 向量 . , ( ) , m∈ , P E[ 1 , +。 。 ] 表
= v ∈H ( ) n L 1 ( ” ) : m a x ( 1 l v l l L , 【 l u l 1 日 ) R )
【国家自然科学基金】_嵌入关系_基金支持热词逐年推荐_【万方软件创新助手】_20140802
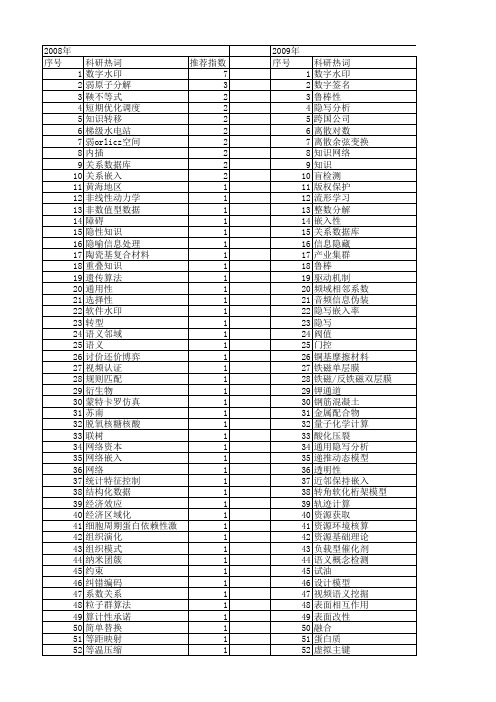
2009年 序号 1 2 3 4 5 6 7 8 9 10 11 12 13 14 15 16 17 18 19 20 21 22 23 24 25 26 27 28 29 30 31 32 33 34 35 36 37 38 39 40 41 42 43 44 45 46 47 48 49 50 51 52
竞争神经网络 空间聚类 租金 神经网络 社会资本 社会网络 社会嵌入 碳纳米管负载铂 砂质海床 相空间重构 相变 盲水印 盲检测 特征点 熔化 温度场 温州 渗流场 混淆交叉 混淆 混沌时间序列 混沌序列 混合粒子群算法 海底管道 水印容量 水印 水下机器人 正交实验法 模糊逻辑 模式"趋同" 模型编辑 概率分析 树匹配 查询扩展 机械扩径 机会约束规划 机会主义行为 有限元模拟 有监督局部线性嵌入 智能推理 数据降维 数字水印算法 数值模拟 支持向量机树 支持向量机 描述器 损伤 指令特征 拉格郎日插值公式 拉伸性能 技术创新 忠诚性承诺 形状记忆合金 弱原子
节能方案 能量节省率 网络嵌入性 网格 综合业务系统 维数约减 绩效 结构嵌入 结构健康监测 经典核化理论 织物仿真 组织间关系 线性近邻传递算法 线性分类方法 纺织cad 纱线建模 纤维丛 簇绒地毯 第三体 竞争情报 空闲模式 秘密通信 离散补充变量方法 离散时间排队系统 社会资本 社会网络 磁电阻效应 碳酸盐岩储层 硬化参量 砂土 石油工程 短期预测 知识转移 相互作用 盲信号分离 留职意图 电磁悬浮 电力负荷 理论体系 环zn上圆锥曲线 状态相似矩阵 特征提取 物料清单 演进路径 源汇分析 混沌动力系统 液态合金 浸入子丛 测地线距离 治理过程 治理结构 水解层 水印提取 水印嵌入
Survey of clustering data mining techniques
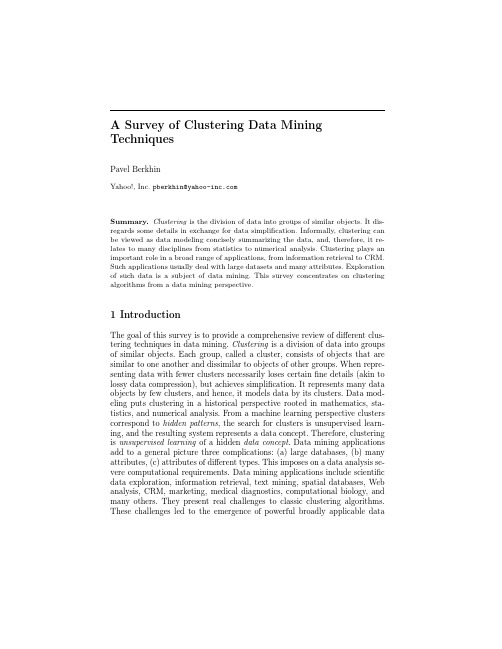
A Survey of Clustering Data Mining TechniquesPavel BerkhinYahoo!,Inc.pberkhin@Summary.Clustering is the division of data into groups of similar objects.It dis-regards some details in exchange for data simplifirmally,clustering can be viewed as data modeling concisely summarizing the data,and,therefore,it re-lates to many disciplines from statistics to numerical analysis.Clustering plays an important role in a broad range of applications,from information retrieval to CRM. Such applications usually deal with large datasets and many attributes.Exploration of such data is a subject of data mining.This survey concentrates on clustering algorithms from a data mining perspective.1IntroductionThe goal of this survey is to provide a comprehensive review of different clus-tering techniques in data mining.Clustering is a division of data into groups of similar objects.Each group,called a cluster,consists of objects that are similar to one another and dissimilar to objects of other groups.When repre-senting data with fewer clusters necessarily loses certainfine details(akin to lossy data compression),but achieves simplification.It represents many data objects by few clusters,and hence,it models data by its clusters.Data mod-eling puts clustering in a historical perspective rooted in mathematics,sta-tistics,and numerical analysis.From a machine learning perspective clusters correspond to hidden patterns,the search for clusters is unsupervised learn-ing,and the resulting system represents a data concept.Therefore,clustering is unsupervised learning of a hidden data concept.Data mining applications add to a general picture three complications:(a)large databases,(b)many attributes,(c)attributes of different types.This imposes on a data analysis se-vere computational requirements.Data mining applications include scientific data exploration,information retrieval,text mining,spatial databases,Web analysis,CRM,marketing,medical diagnostics,computational biology,and many others.They present real challenges to classic clustering algorithms. These challenges led to the emergence of powerful broadly applicable data2Pavel Berkhinmining clustering methods developed on the foundation of classic techniques.They are subject of this survey.1.1NotationsTo fix the context and clarify terminology,consider a dataset X consisting of data points (i.e.,objects ,instances ,cases ,patterns ,tuples ,transactions )x i =(x i 1,···,x id ),i =1:N ,in attribute space A ,where each component x il ∈A l ,l =1:d ,is a numerical or nominal categorical attribute (i.e.,feature ,variable ,dimension ,component ,field ).For a discussion of attribute data types see [106].Such point-by-attribute data format conceptually corresponds to a N ×d matrix and is used by a majority of algorithms reviewed below.However,data of other formats,such as variable length sequences and heterogeneous data,are not uncommon.The simplest subset in an attribute space is a direct Cartesian product of sub-ranges C = C l ⊂A ,C l ⊂A l ,called a segment (i.e.,cube ,cell ,region ).A unit is an elementary segment whose sub-ranges consist of a single category value,or of a small numerical bin.Describing the numbers of data points per every unit represents an extreme case of clustering,a histogram .This is a very expensive representation,and not a very revealing er driven segmentation is another commonly used practice in data exploration that utilizes expert knowledge regarding the importance of certain sub-domains.Unlike segmentation,clustering is assumed to be automatic,and so it is a machine learning technique.The ultimate goal of clustering is to assign points to a finite system of k subsets (clusters).Usually (but not always)subsets do not intersect,and their union is equal to a full dataset with the possible exception of outliersX =C 1 ··· C k C outliers ,C i C j =0,i =j.1.2Clustering Bibliography at GlanceGeneral references regarding clustering include [110],[205],[116],[131],[63],[72],[165],[119],[75],[141],[107],[91].A very good introduction to contem-porary data mining clustering techniques can be found in the textbook [106].There is a close relationship between clustering and many other fields.Clustering has always been used in statistics [10]and science [158].The clas-sic introduction into pattern recognition framework is given in [64].Typical applications include speech and character recognition.Machine learning clus-tering algorithms were applied to image segmentation and computer vision[117].For statistical approaches to pattern recognition see [56]and [85].Clus-tering can be viewed as a density estimation problem.This is the subject of traditional multivariate statistical estimation [197].Clustering is also widelyA Survey of Clustering Data Mining Techniques3 used for data compression in image processing,which is also known as vec-tor quantization[89].Datafitting in numerical analysis provides still another venue in data modeling[53].This survey’s emphasis is on clustering in data mining.Such clustering is characterized by large datasets with many attributes of different types. Though we do not even try to review particular applications,many important ideas are related to the specificfields.Clustering in data mining was brought to life by intense developments in information retrieval and text mining[52], [206],[58],spatial database applications,for example,GIS or astronomical data,[223],[189],[68],sequence and heterogeneous data analysis[43],Web applications[48],[111],[81],DNA analysis in computational biology[23],and many others.They resulted in a large amount of application-specific devel-opments,but also in some general techniques.These techniques and classic clustering algorithms that relate to them are surveyed below.1.3Plan of Further PresentationClassification of clustering algorithms is neither straightforward,nor canoni-cal.In reality,different classes of algorithms overlap.Traditionally clustering techniques are broadly divided in hierarchical and partitioning.Hierarchical clustering is further subdivided into agglomerative and divisive.The basics of hierarchical clustering include Lance-Williams formula,idea of conceptual clustering,now classic algorithms SLINK,COBWEB,as well as newer algo-rithms CURE and CHAMELEON.We survey these algorithms in the section Hierarchical Clustering.While hierarchical algorithms gradually(dis)assemble points into clusters (as crystals grow),partitioning algorithms learn clusters directly.In doing so they try to discover clusters either by iteratively relocating points between subsets,or by identifying areas heavily populated with data.Algorithms of thefirst kind are called Partitioning Relocation Clustering. They are further classified into probabilistic clustering(EM framework,al-gorithms SNOB,AUTOCLASS,MCLUST),k-medoids methods(algorithms PAM,CLARA,CLARANS,and its extension),and k-means methods(differ-ent schemes,initialization,optimization,harmonic means,extensions).Such methods concentrate on how well pointsfit into their clusters and tend to build clusters of proper convex shapes.Partitioning algorithms of the second type are surveyed in the section Density-Based Partitioning.They attempt to discover dense connected com-ponents of data,which areflexible in terms of their shape.Density-based connectivity is used in the algorithms DBSCAN,OPTICS,DBCLASD,while the algorithm DENCLUE exploits space density functions.These algorithms are less sensitive to outliers and can discover clusters of irregular shape.They usually work with low-dimensional numerical data,known as spatial data. Spatial objects could include not only points,but also geometrically extended objects(algorithm GDBSCAN).4Pavel BerkhinSome algorithms work with data indirectly by constructing summaries of data over the attribute space subsets.They perform space segmentation and then aggregate appropriate segments.We discuss them in the section Grid-Based Methods.They frequently use hierarchical agglomeration as one phase of processing.Algorithms BANG,STING,WaveCluster,and FC are discussed in this section.Grid-based methods are fast and handle outliers well.Grid-based methodology is also used as an intermediate step in many other algorithms (for example,CLIQUE,MAFIA).Categorical data is intimately connected with transactional databases.The concept of a similarity alone is not sufficient for clustering such data.The idea of categorical data co-occurrence comes to the rescue.The algorithms ROCK,SNN,and CACTUS are surveyed in the section Co-Occurrence of Categorical Data.The situation gets even more aggravated with the growth of the number of items involved.To help with this problem the effort is shifted from data clustering to pre-clustering of items or categorical attribute values. Development based on hyper-graph partitioning and the algorithm STIRR exemplify this approach.Many other clustering techniques are developed,primarily in machine learning,that either have theoretical significance,are used traditionally out-side the data mining community,or do notfit in previously outlined categories. The boundary is blurred.In the section Other Developments we discuss the emerging direction of constraint-based clustering,the important researchfield of graph partitioning,and the relationship of clustering to supervised learning, gradient descent,artificial neural networks,and evolutionary methods.Data Mining primarily works with large databases.Clustering large datasets presents scalability problems reviewed in the section Scalability and VLDB Extensions.Here we talk about algorithms like DIGNET,about BIRCH and other data squashing techniques,and about Hoffding or Chernoffbounds.Another trait of real-life data is high dimensionality.Corresponding de-velopments are surveyed in the section Clustering High Dimensional Data. The trouble comes from a decrease in metric separation when the dimension grows.One approach to dimensionality reduction uses attributes transforma-tions(DFT,PCA,wavelets).Another way to address the problem is through subspace clustering(algorithms CLIQUE,MAFIA,ENCLUS,OPTIGRID, PROCLUS,ORCLUS).Still another approach clusters attributes in groups and uses their derived proxies to cluster objects.This double clustering is known as co-clustering.Issues common to different clustering methods are overviewed in the sec-tion General Algorithmic Issues.We talk about assessment of results,de-termination of appropriate number of clusters to build,data preprocessing, proximity measures,and handling of outliers.For reader’s convenience we provide a classification of clustering algorithms closely followed by this survey:•Hierarchical MethodsA Survey of Clustering Data Mining Techniques5Agglomerative AlgorithmsDivisive Algorithms•Partitioning Relocation MethodsProbabilistic ClusteringK-medoids MethodsK-means Methods•Density-Based Partitioning MethodsDensity-Based Connectivity ClusteringDensity Functions Clustering•Grid-Based Methods•Methods Based on Co-Occurrence of Categorical Data•Other Clustering TechniquesConstraint-Based ClusteringGraph PartitioningClustering Algorithms and Supervised LearningClustering Algorithms in Machine Learning•Scalable Clustering Algorithms•Algorithms For High Dimensional DataSubspace ClusteringCo-Clustering Techniques1.4Important IssuesThe properties of clustering algorithms we are primarily concerned with in data mining include:•Type of attributes algorithm can handle•Scalability to large datasets•Ability to work with high dimensional data•Ability tofind clusters of irregular shape•Handling outliers•Time complexity(we frequently simply use the term complexity)•Data order dependency•Labeling or assignment(hard or strict vs.soft or fuzzy)•Reliance on a priori knowledge and user defined parameters •Interpretability of resultsRealistically,with every algorithm we discuss only some of these properties. The list is in no way exhaustive.For example,as appropriate,we also discuss algorithms ability to work in pre-defined memory buffer,to restart,and to provide an intermediate solution.6Pavel Berkhin2Hierarchical ClusteringHierarchical clustering builds a cluster hierarchy or a tree of clusters,also known as a dendrogram.Every cluster node contains child clusters;sibling clusters partition the points covered by their common parent.Such an ap-proach allows exploring data on different levels of granularity.Hierarchical clustering methods are categorized into agglomerative(bottom-up)and divi-sive(top-down)[116],[131].An agglomerative clustering starts with one-point (singleton)clusters and recursively merges two or more of the most similar clusters.A divisive clustering starts with a single cluster containing all data points and recursively splits the most appropriate cluster.The process contin-ues until a stopping criterion(frequently,the requested number k of clusters) is achieved.Advantages of hierarchical clustering include:•Flexibility regarding the level of granularity•Ease of handling any form of similarity or distance•Applicability to any attribute typesDisadvantages of hierarchical clustering are related to:•Vagueness of termination criteria•Most hierarchical algorithms do not revisit(intermediate)clusters once constructed.The classic approaches to hierarchical clustering are presented in the sub-section Linkage Metrics.Hierarchical clustering based on linkage metrics re-sults in clusters of proper(convex)shapes.Active contemporary efforts to build cluster systems that incorporate our intuitive concept of clusters as con-nected components of arbitrary shape,including the algorithms CURE and CHAMELEON,are surveyed in the subsection Hierarchical Clusters of Arbi-trary Shapes.Divisive techniques based on binary taxonomies are presented in the subsection Binary Divisive Partitioning.The subsection Other Devel-opments contains information related to incremental learning,model-based clustering,and cluster refinement.In hierarchical clustering our regular point-by-attribute data representa-tion frequently is of secondary importance.Instead,hierarchical clustering frequently deals with the N×N matrix of distances(dissimilarities)or sim-ilarities between training points sometimes called a connectivity matrix.So-called linkage metrics are constructed from elements of this matrix.The re-quirement of keeping a connectivity matrix in memory is unrealistic.To relax this limitation different techniques are used to sparsify(introduce zeros into) the connectivity matrix.This can be done by omitting entries smaller than a certain threshold,by using only a certain subset of data representatives,or by keeping with each point only a certain number of its nearest neighbors(for nearest neighbor chains see[177]).Notice that the way we process the original (dis)similarity matrix and construct a linkage metric reflects our a priori ideas about the data model.A Survey of Clustering Data Mining Techniques7With the(sparsified)connectivity matrix we can associate the weighted connectivity graph G(X,E)whose vertices X are data points,and edges E and their weights are defined by the connectivity matrix.This establishes a connection between hierarchical clustering and graph partitioning.One of the most striking developments in hierarchical clustering is the algorithm BIRCH.It is discussed in the section Scalable VLDB Extensions.Hierarchical clustering initializes a cluster system as a set of singleton clusters(agglomerative case)or a single cluster of all points(divisive case) and proceeds iteratively merging or splitting the most appropriate cluster(s) until the stopping criterion is achieved.The appropriateness of a cluster(s) for merging or splitting depends on the(dis)similarity of cluster(s)elements. This reflects a general presumption that clusters consist of similar points.An important example of dissimilarity between two points is the distance between them.To merge or split subsets of points rather than individual points,the dis-tance between individual points has to be generalized to the distance between subsets.Such a derived proximity measure is called a linkage metric.The type of a linkage metric significantly affects hierarchical algorithms,because it re-flects a particular concept of closeness and connectivity.Major inter-cluster linkage metrics[171],[177]include single link,average link,and complete link. The underlying dissimilarity measure(usually,distance)is computed for every pair of nodes with one node in thefirst set and another node in the second set.A specific operation such as minimum(single link),average(average link),or maximum(complete link)is applied to pair-wise dissimilarity measures:d(C1,C2)=Op{d(x,y),x∈C1,y∈C2}Early examples include the algorithm SLINK[199],which implements single link(Op=min),Voorhees’method[215],which implements average link (Op=Avr),and the algorithm CLINK[55],which implements complete link (Op=max).It is related to the problem offinding the Euclidean minimal spanning tree[224]and has O(N2)complexity.The methods using inter-cluster distances defined in terms of pairs of nodes(one in each respective cluster)are called graph methods.They do not use any cluster representation other than a set of points.This name naturally relates to the connectivity graph G(X,E)introduced above,because every data partition corresponds to a graph partition.Such methods can be augmented by so-called geometric methods in which a cluster is represented by its central point.Under the assumption of numerical attributes,the center point is defined as a centroid or an average of two cluster centroids subject to agglomeration.It results in centroid,median,and minimum variance linkage metrics.All of the above linkage metrics can be derived from the Lance-Williams updating formula[145],d(C iC j,C k)=a(i)d(C i,C k)+a(j)d(C j,C k)+b·d(C i,C j)+c|d(C i,C k)−d(C j,C k)|.8Pavel BerkhinHere a,b,c are coefficients corresponding to a particular linkage.This formula expresses a linkage metric between a union of the two clusters and the third cluster in terms of underlying nodes.The Lance-Williams formula is crucial to making the dis(similarity)computations feasible.Surveys of linkage metrics can be found in [170][54].When distance is used as a base measure,linkage metrics capture inter-cluster proximity.However,a similarity-based view that results in intra-cluster connectivity considerations is also used,for example,in the original average link agglomeration (Group-Average Method)[116].Under reasonable assumptions,such as reducibility condition (graph meth-ods satisfy this condition),linkage metrics methods suffer from O N 2 time complexity [177].Despite the unfavorable time complexity,these algorithms are widely used.As an example,the algorithm AGNES (AGlomerative NESt-ing)[131]is used in S-Plus.When the connectivity N ×N matrix is sparsified,graph methods directly dealing with the connectivity graph G can be used.In particular,hierarchical divisive MST (Minimum Spanning Tree)algorithm is based on graph parti-tioning [116].2.1Hierarchical Clusters of Arbitrary ShapesFor spatial data,linkage metrics based on Euclidean distance naturally gener-ate clusters of convex shapes.Meanwhile,visual inspection of spatial images frequently discovers clusters with curvy appearance.Guha et al.[99]introduced the hierarchical agglomerative clustering algo-rithm CURE (Clustering Using REpresentatives).This algorithm has a num-ber of novel features of general importance.It takes special steps to handle outliers and to provide labeling in assignment stage.It also uses two techniques to achieve scalability:data sampling (section 8),and data partitioning.CURE creates p partitions,so that fine granularity clusters are constructed in parti-tions first.A major feature of CURE is that it represents a cluster by a fixed number,c ,of points scattered around it.The distance between two clusters used in the agglomerative process is the minimum of distances between two scattered representatives.Therefore,CURE takes a middle approach between the graph (all-points)methods and the geometric (one centroid)methods.Single and average link closeness are replaced by representatives’aggregate closeness.Selecting representatives scattered around a cluster makes it pos-sible to cover non-spherical shapes.As before,agglomeration continues until the requested number k of clusters is achieved.CURE employs one additional trick:originally selected scattered points are shrunk to the geometric centroid of the cluster by a user-specified factor α.Shrinkage suppresses the affect of outliers;outliers happen to be located further from the cluster centroid than the other scattered representatives.CURE is capable of finding clusters of different shapes and sizes,and it is insensitive to outliers.Because CURE uses sampling,estimation of its complexity is not straightforward.For low-dimensional data authors provide a complexity estimate of O (N 2sample )definedA Survey of Clustering Data Mining Techniques9 in terms of a sample size.More exact bounds depend on input parameters: shrink factorα,number of representative points c,number of partitions p,and a sample size.Figure1(a)illustrates agglomeration in CURE.Three clusters, each with three representatives,are shown before and after the merge and shrinkage.Two closest representatives are connected.While the algorithm CURE works with numerical attributes(particularly low dimensional spatial data),the algorithm ROCK developed by the same researchers[100]targets hierarchical agglomerative clustering for categorical attributes.It is reviewed in the section Co-Occurrence of Categorical Data.The hierarchical agglomerative algorithm CHAMELEON[127]uses the connectivity graph G corresponding to the K-nearest neighbor model spar-sification of the connectivity matrix:the edges of K most similar points to any given point are preserved,the rest are pruned.CHAMELEON has two stages.In thefirst stage small tight clusters are built to ignite the second stage.This involves a graph partitioning[129].In the second stage agglomer-ative process is performed.It utilizes measures of relative inter-connectivity RI(C i,C j)and relative closeness RC(C i,C j);both are locally normalized by internal interconnectivity and closeness of clusters C i and C j.In this sense the modeling is dynamic:it depends on data locally.Normalization involves certain non-obvious graph operations[129].CHAMELEON relies heavily on graph partitioning implemented in the library HMETIS(see the section6). Agglomerative process depends on user provided thresholds.A decision to merge is made based on the combinationRI(C i,C j)·RC(C i,C j)αof local measures.The algorithm does not depend on assumptions about the data model.It has been proven tofind clusters of different shapes,densities, and sizes in2D(two-dimensional)space.It has a complexity of O(Nm+ Nlog(N)+m2log(m),where m is the number of sub-clusters built during the first initialization phase.Figure1(b)(analogous to the one in[127])clarifies the difference with CURE.It presents a choice of four clusters(a)-(d)for a merge.While CURE would merge clusters(a)and(b),CHAMELEON makes intuitively better choice of merging(c)and(d).2.2Binary Divisive PartitioningIn linguistics,information retrieval,and document clustering applications bi-nary taxonomies are very useful.Linear algebra methods,based on singular value decomposition(SVD)are used for this purpose in collaborativefilter-ing and information retrieval[26].Application of SVD to hierarchical divisive clustering of document collections resulted in the PDDP(Principal Direction Divisive Partitioning)algorithm[31].In our notations,object x is a docu-ment,l th attribute corresponds to a word(index term),and a matrix X entry x il is a measure(e.g.TF-IDF)of l-term frequency in a document x.PDDP constructs SVD decomposition of the matrix10Pavel Berkhin(a)Algorithm CURE (b)Algorithm CHAMELEONFig.1.Agglomeration in Clusters of Arbitrary Shapes(X −e ¯x ),¯x =1Ni =1:N x i ,e =(1,...,1)T .This algorithm bisects data in Euclidean space by a hyperplane that passes through data centroid orthogonal to the eigenvector with the largest singular value.A k -way split is also possible if the k largest singular values are consid-ered.Bisecting is a good way to categorize documents and it yields a binary tree.When k -means (2-means)is used for bisecting,the dividing hyperplane is orthogonal to the line connecting the two centroids.The comparative study of SVD vs.k -means approaches [191]can be used for further references.Hier-archical divisive bisecting k -means was proven [206]to be preferable to PDDP for document clustering.While PDDP or 2-means are concerned with how to split a cluster,the problem of which cluster to split is also important.Simple strategies are:(1)split each node at a given level,(2)split the cluster with highest cardinality,and,(3)split the cluster with the largest intra-cluster variance.All three strategies have problems.For a more detailed analysis of this subject and better strategies,see [192].2.3Other DevelopmentsOne of early agglomerative clustering algorithms,Ward’s method [222],is based not on linkage metric,but on an objective function used in k -means.The merger decision is viewed in terms of its effect on the objective function.The popular hierarchical clustering algorithm for categorical data COB-WEB [77]has two very important qualities.First,it utilizes incremental learn-ing.Instead of following divisive or agglomerative approaches,it dynamically builds a dendrogram by processing one data point at a time.Second,COB-WEB is an example of conceptual or model-based learning.This means that each cluster is considered as a model that can be described intrinsically,rather than as a collection of points assigned to it.COBWEB’s dendrogram is calleda classification tree.Each tree node(cluster)C is associated with the condi-tional probabilities for categorical attribute-values pairs,P r(x l=νlp|C),l=1:d,p=1:|A l|.This easily can be recognized as a C-specific Na¨ıve Bayes classifier.During the classification tree construction,every new point is descended along the tree and the tree is potentially updated(by an insert/split/merge/create op-eration).Decisions are based on the category utility[49]CU{C1,...,C k}=1j=1:kCU(C j)CU(C j)=l,p(P r(x l=νlp|C j)2−(P r(x l=νlp)2.Category utility is similar to the GINI index.It rewards clusters C j for in-creases in predictability of the categorical attribute valuesνlp.Being incre-mental,COBWEB is fast with a complexity of O(tN),though it depends non-linearly on tree characteristics packed into a constant t.There is a similar incremental hierarchical algorithm for all numerical attributes called CLAS-SIT[88].CLASSIT associates normal distributions with cluster nodes.Both algorithms can result in highly unbalanced trees.Chiu et al.[47]proposed another conceptual or model-based approach to hierarchical clustering.This development contains several different use-ful features,such as the extension of scalability preprocessing to categori-cal attributes,outliers handling,and a two-step strategy for monitoring the number of clusters including BIC(defined below).A model associated with a cluster covers both numerical and categorical attributes and constitutes a blend of Gaussian and multinomial models.Denote corresponding multivari-ate parameters byθ.With every cluster C we associate a logarithm of its (classification)likelihoodl C=x i∈Clog(p(x i|θ))The algorithm uses maximum likelihood estimates for parameterθ.The dis-tance between two clusters is defined(instead of linkage metric)as a decrease in log-likelihoodd(C1,C2)=l C1+l C2−l C1∪C2caused by merging of the two clusters under consideration.The agglomerative process continues until the stopping criterion is satisfied.As such,determina-tion of the best k is automatic.This algorithm has the commercial implemen-tation(in SPSS Clementine).The complexity of the algorithm is linear in N for the summarization phase.Traditional hierarchical clustering does not change points membership in once assigned clusters due to its greedy approach:after a merge or a split is selected it is not refined.Though COBWEB does reconsider its decisions,its。
时标上一类非线性中立型微分方程的振动性
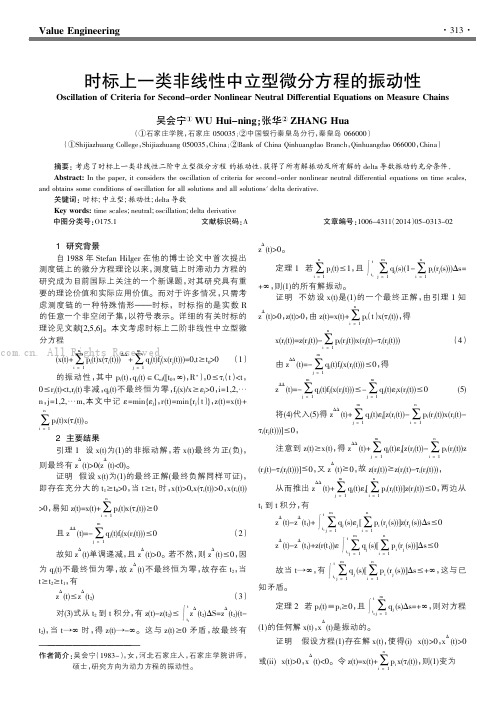
Value Engineering1研究背景自1988年Stefan Hilger 在他的博士论文中首次提出测度链上的微分方程理论以来,测度链上时滞动力方程的研究成为目前国际上关注的一个新课题,对其研究具有重要的理论价值和实际应用价值。
而对于许多情况,只需考虑测度链的一种特殊情形———时标,时标指的是实数R 的任意一个非空闭子集,以符号表示。
详细的有关时标的理论见文献[2,5,6]。
本文考虑时标上二阶非线性中立型微分方程(x(t)+n i =1∑p i (t)x(τi (t)))ΔΔ+mj =1∑q j (t)f j (x(r j (t)))=0,t ⩾t 0>0(1)的振动性,其中p i (t),q j (t)∈C rd ([t 0,∞),R +),0⩽τi (t)<t ,0⩽r j (t)<t,r j (t)非减,q j (t)不最终恒为零,f j (x)/x ⩾εj >0,i=1,2,…n ,j=1,2,…m,本文中记ε=min{εj },r(t)=min{r j (t)},z(t)=x(t)+ni =1∑p i(t)x(τi (t))。
2主要结果引理1设x(t)为(1)的非振动解,若x(t)最终为正(负),则最终有z Δ(t)>0(z Δ(t)<0)。
证明假设x(t)为(1)的最终正解(最终负解同样可证),即存在充分大的t 1⩾t 0>0,当t ⩾t 1时,x(t)>0,x(τi (t))>0,x(r i (t))>0,易知z(t)=x(t)+ni =1∑p i (t)x(τi (t))⩾0且z ΔΔ(t)=-m j =1∑q j (t)f j (x(r i (t)))⩽0(2)故知z Δ(t)单调递减,且z Δ(t)>0。
若不然,则z Δ(t)⩽0,因为q j (t)不最终恒为零,故z Δ(t)不最终恒为零,故存在t 2,当t ⩾t 2⩾t 1,有z Δ(t)⩽z Δ(t 2)(3)对(3)式从t 2到t 积分,有z(t)-z(t 2)⩽t t ∫z Δ(t 2)ΔS=z Δ(t 2)(t-t 2),当t→∞时,得z(t)→-∞。
Cheng Hao,2009,Lithos
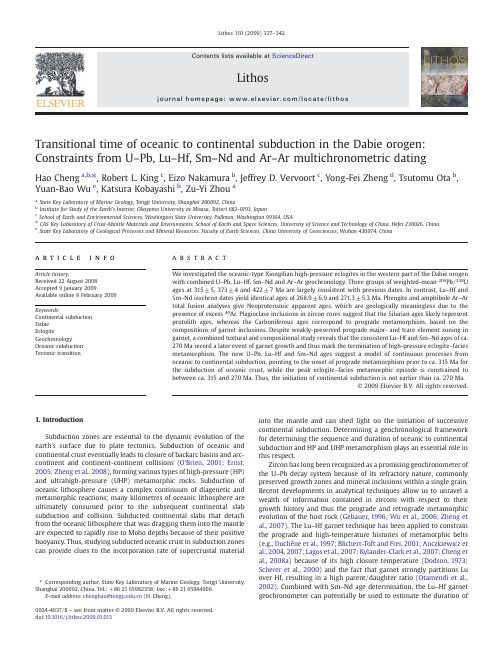
Transitional time of oceanic to continental subduction in the Dabie orogen:Constraints from U –Pb,Lu –Hf,Sm –Nd and Ar –Ar multichronometric datingHao Cheng a ,b ,⁎,Robert L.King c ,Eizo Nakamura b ,Jeffrey D.Vervoort c ,Yong-Fei Zheng d ,Tsutomu Ota b ,Yuan-Bao Wu e ,Katsura Kobayashi b ,Zu-Yi Zhou aaState Key Laboratory of Marine Geology,Tongji University,Shanghai 200092,ChinabInstitute for Study of the Earth's Interior,Okayama University at Misasa,Tottori 682-0193,Japan cSchool of Earth and Environmental Sciences,Washington State University,Pullman,Washington 99164,USA dCAS Key Laboratory of Crust-Mantle Materials and Environments,School of Earth and Space Sciences,University of Science and Technology of China,Hefei 230026,China eState Key Laboratory of Geological Processes and Mineral Resources,Faculty of Earth Sciences,China University of Geosciences,Wuhan 430074,Chinaa b s t r a c ta r t i c l e i n f o Article history:Received 22August 2008Accepted 9January 2009Available online 8February 2009Keywords:Continental subduction Dabie EclogiteGeochronologyOceanic subduction Tectonic transitionWe investigated the oceanic-type Xiongdian high-pressure eclogites in the western part of the Dabie orogen with combined U –Pb,Lu –Hf,Sm –Nd and Ar –Ar geochronology.Three groups of weighted-mean 206Pb/238U ages at 315±5,373±4and 422±7Ma are largely consistent with previous dates.In contrast,Lu –Hf and Sm –Nd isochron dates yield identical ages of 268.9±6.9and 271.3±5.3Ma.Phengite and amphibole Ar –Ar total fusion analyses give Neoproterozoic apparent ages,which are geologically meaningless due to the presence of excess 40Ar.Plagioclase inclusions in zircon cores suggest that the Silurian ages likely represent protolith ages,whereas the Carboniferous ages correspond to prograde metamorphism,based on the compositions of garnet inclusions.Despite weakly-preserved prograde major-and trace element zoning in garnet,a combined textural and compositional study reveals that the consistent Lu –Hf and Sm –Nd ages of ca.270Ma record a later event of garnet growth and thus mark the termination of high-pressure eclogite –facies metamorphism.The new U –Pb,Lu –Hf and Sm –Nd ages suggest a model of continuous processes from oceanic to continental subduction,pointing to the onset of prograde metamorphism prior to ca.315Ma for the subduction of oceanic crust,while the peak eclogite –facies metamorphic episode is constrained to between ca.315and 270Ma.Thus,the initiation of continental subduction is not earlier than ca.270Ma.©2009Elsevier B.V.All rights reserved.1.IntroductionSubduction zones are essential to the dynamic evolution of the earth's surface due to plate tectonics.Subduction of oceanic and continental crust eventually leads to closure of backarc basins and arc-continent and continent-continent collisions (O'Brien,2001;Ernst,2005;Zheng et al.,2008),forming various types of high-pressure (HP)and ultrahigh-pressure (UHP)metamorphic rocks.Subduction of oceanic lithosphere causes a complex continuum of diagenetic and metamorphic reactions;many kilometres of oceanic lithosphere are ultimately consumed prior to the subsequent continental slab subduction and collision.Subducted continental slabs that detach from the oceanic lithosphere that was dragging them into the mantle are expected to rapidly rise to Moho depths because of their positive buoyancy.Thus,studying subducted oceanic crust in subduction zones can provide clues to the incorporation rate of supercrustal materialinto the mantle and can shed light on the initiation of successive continental subduction.Determining a geochronological framework for determining the sequence and duration of oceanic to continental subduction and HP and UHP metamorphism plays an essential role in this respect.Zircon has long been recognized as a promising geochronometer of the U –Pb decay system because of its refractory nature,commonly preserved growth zones and mineral inclusions within a single grain.Recent developments in analytical techniques allow us to unravel a wealth of information contained in zircons with respect to their growth history and thus the prograde and retrograde metamorphic evolution of the host rock (Gebauer,1996;Wu et al.,2006;Zheng et al.,2007).The Lu –Hf garnet technique has been applied to constrain the prograde and high-temperature histories of metamorphic belts (e.g.,Duchêne et al.,1997;Blichert-Toft and Frei,2001;Anczkiewicz et al.,2004,2007;Lagos et al.,2007;Kylander-Clark et al.,2007;Cheng et al.,2008a )because of its high closure temperature (Dodson,1973;Scherer et al.,2000)and the fact that garnet strongly partitions Lu over Hf,resulting in a high parent/daughter ratio (Otamendi et al.,2002).Combined with Sm –Nd age determination,the Lu –Hf garnet geochronometer can potentially be used to estimate the duration ofLithos 110(2009)327–342⁎Corresponding author.State Key Laboratory of Marine Geology,Tongji University,Shanghai 200092,China.Tel.:+862165982358;fax:+862165984906.E-mail address:chenghao@ (H.Cheng).0024-4937/$–see front matter ©2009Elsevier B.V.All rights reserved.doi:10.1016/j.lithos.2009.01.013Contents lists available at ScienceDirectLithosj ou r n a l h o m e pa g e :ww w.e l s ev i e r.c o m/l o c a t e /l i t h o sFig.1.Simpli fied geologic map of the Huwan mélange area (b)in southern Dabie orogen (a),modi fied after Ye et al.(1993)and Liu et al.(2004b),showing the sample localities for the Xiongdian eclogite.References:asterisk,this study;[1],Ratschbacher et al.(2006);[2],Jahn et al.(2005);[3],Liu et al.(2004a);[4],Eide et al.(1994);[5],Webb et al.(1999);[6],Xu et al.(2000);[7],Ye et al.(1993);[8],Sun et al.(2002);[9],Jian et al.(1997);[10],Jian et al.(2000);[11],Gao et al.(2002);[12],Li et al.(2001);[13],Wu et al.(2008).amp —amphibole;brs —barroisite;phen —phengite;zrn —zircon.328H.Cheng et al./Lithos 110(2009)327–342garnet growth,which either reflects early prograde metamorphism (Lapen et al.,2003),exhumation(Cheng et al.,2009)or a particular garnet growth stage(Skora et al.,2006).Dating the exhumation of high-pressure(HP)and ultra-high-pressure(UHP)metamorphic rocks by conventional step-heating Ar–Ar technique was largely hampered and discredited due to the presence of excess/inherited argon(Li et al.,1994;Kelley,2002).However,the Ar–Ar geochron-ometer remains irreplaceable in constraining the exhumation of HP/ UHP metamorphic rocks because of its intermediate closure tempera-ture.Nevertheless,timing must be integrated with textures and petrology in order to quantify the dynamics of geological processes, whichever geochronological method is used.During the past two decades,considerable progress has been made in constraining the prograde metamorphism and exhumation of HP/ UHP metamorphism of the Dabie–Sulu orogen by a variety of geochronological methods,indicating a Triassic collision between the South China and North China Blocks(e.g.,Eide et al.,1994;Ames et al., 1996;Rowley et al.,1997;Hacker et al.,1998;Li et al.,2000,2004; Zheng et al.,2004).The initiation of continental subduction is pinned to ca.245Ma(Hacker et al.,2006;Liu et al.,2006a;Wu et al.,2006; Cheng et al.,2008a),but the exact time is poorly constrained.On the other hand,thefingerprints of early continental subduction may not be preserved in continental-type metamorphic rocks due to the succes-sive high-temperature prograde and retrograde overprints.Alterna-tively,the timing of initiation of continental subduction subsequent to the termination of oceanic subduction may be registered in the HP/ UHP eclogites,whose protoliths are of oceanic origin.Currently,the only outcropping candidate is the Xiongdian HP eclogite in the western part of the Dabie orogen(Li et al.,2001;Fu et al.,2002).However,U–Pb zircon ages ranging from216±4to449±14Ma have been obtained for the Xiongdian eclogite(Jian et al.,1997;Sun et al.,2002;Gao et al., 2002);the geological significance of this age spread is controversial. Efforts to clarify the geochronological evolution of the Xiongdian eclogite were hampered by a much older Sm–Nd garnet-whole-rock isochron of533±13Ma(Ye et al.,1993)and the fact that further Sm–Nd and Rb–Sr analyses failed to produce mineral isochrons(Li et al., 2001;Jahn et al.,2005),although oxygen isotopic equilibrium was largely attained(Jahn et al.,2005).Here,we present a combined U–Pb,Lu–Hf,Sm–Nd,Ar–Ar and oxygen multi-isotopic and mineral chemical study of the Xiongdian eclogite.The differences in these systems,in conjunction with chemical profiles in garnet porphyroblasts and zircons,provide a window into the time-scales of the oceanic subduction and sub-sequent exhumation.2.Geochronological background and sample descriptionsThe Qinling–Dabie–Sulu orogen in east-central China marks the junction between the North and South China Blocks(Cong,1996; Zheng et al.,2005).The western part of the Dabie orogen,usually termed the West Dabie and sometimes the Hong'an terrane,is separated from the Tongbaishan in the west by the Dawu Fault and from the East Dabie by the Shangma fault in the east(Fig.1a).It contains a progressive sequence of metamorphic zones characterized by increasing metamorphic grade,from transitional blueschist–greenschist in the south,through epidote–amphibolite and quartz eclogite,to coesite eclogite in the north(e.g.,Zhou et al.,1993;Hacker et al.,1998;Liu et al.,2004b,2006b).The Xiongdian eclogites crop out in the northwestern corner of the West Dabie,in the Xiongdian mélange within the Huwan mélange after the definition of Ratschba-cher et al.(2006),in analogy to the terms of the Sujiahe mélange(Jian et al.,1997)and Huwan shear zone(Sun et al.,2002).The Huwan mélange consists of eclogite,gabbro,amphibolite,marble,and quartzite.The eclogitic metamorphic peak for the Xiongdian eclogite is estimated at600–730°C,1.4–1.9GPa(Fu et al.,2002),550–570°C,∼2.1GPa(Liu et al.,2004b)and540–600°C,∼2.0GPa(Ratschbacher et al.,2006),followed by retrogression at530–685°C and∼0.6GPa (Fu et al.,2002).Except for the Xiongdian eclogite,consistent Triassic metamorphic ages have been obtained for other eclogites across the West Dabie (Webb et al.,1999;Sun et al.,2002;Liu et al.,2004a;Wu et al.,2008). This indicates that West Dabie is largely a coherent part of an HP–UHP belt elsewhere in the Dabie–Sulu orogenic belt.Geochronological debate is limited to the Xiongdian eclogite(Fig.1b).U–Pb zircon ages ranging from ca.216to ca.449Ma have been obtained for the Xiongdian eclogite.Jian et al.(1997)reported ca.400,ca.373and 301±0.6Ma ages by ID–TIMS method.Weighted-mean SHRIMP ages range from335±2to424±5Ma(Jian et al.,2000).The Silurian U–Pb zircon ages were interpreted as the age of the protolith,while the Carboniferous ages mark high-pressure metamorphism(Jian et al., 1997,2000).Weighted-mean206Pb/238U SHRIMP U–Pb zircons ages of 433±9,367±10and398±5Ma were interpreted as the protolith age,while323±7and312±5Ma likely date the high-pressure metamorphism(Sun et al.,2002).A Triassic age of216±4Ma together with449±14and307±14Ma weighted-mean206Pb/238U SHRIMP U–Pb zircon ages appear to argue for the involvement of the Triassic subduction in the Xiongdian eclogite(Gao et al.,2002).A garnet-whole-rock Sm–Nd isochron of533±13Ma(Ye et al.,1993)was interpreted to reflect the high-pressure metamorphism age.Several Table1Chemical compositions of the Xiongdian eclogite from the western Dabie.Sample number DB17DB18(Major oxides in%)SiO254.5452.45 TiO20.370.43 Al2O314.6212.35 Fe2O38.7710.15 MnO0.150.16 MgO 6.669.91 CaO10.3510.26 Na2O 2.88 2.65 K2O0.600.28 P2O50.060.05 Cr2O3⁎6601118 NiO⁎137247 L.O.I0.87 1.28 Total99.95100.11 (Trace elements in ppm)Li27.627.0 Be0.560.47 Rb9.7813.8 Sr178130Y12.612.7 Cs0.89 3.67 Ba86552.4 La 2.21 1.77 Ce 5.97 5.12 Pr0.880.80 Nd 4.35 4.10 Sm 1.25 1.26 Eu0.470.39 Gd 1.53 1.52 Tb0.280.29 Dy 1.83 1.91 Ho0.410.42 Er 1.14 1.19 Tm0.190.19 Yb 1.31 1.34 Lu0.200.20 Pb 6.44 1.85 Th0.050.07 U0.110.06 Zr28.828.2 Nb 1.19 1.77 Hf0.870.88 Ta0.050.08⁎In ppm.329H.Cheng et al./Lithos110(2009)327–342Sm –Nd and Rb –Sr analyses failed to produces isochrons (Li et al.,2001;Jahn et al.,2005),which was believed to be due to unequilibrated isotopic systems despite the fact that oxygen isotopic equilibrium was largely attained (Jahn et al.,2005).Phengite 40Ar/39Ar ages of ca.430–350Ma have been explained as the retrograde metamorphic age (Xu et al.,2000).The 310±3Ma phengite 40Ar/39Ar age (Webb et al.,1999)is likely geologically meaningless due to the concave-upward age spectrum,indicating the presence of excess argon.Collectively,existing geochronology provides an apparently con flicting picture for the Xiongdian eclogites.The timing of the oceanic crust subduction and exhumation essentially remains to be resolved.The two eclogites examined in this study were selected based on their mineral assemblages,inclusion types and geological context (Fig.1).The one (DB17)from the east bank of the river to the east of Xiongdian village is a coarse-grained and strongly foliated banded eclogite,composed mainly of garnet,omphacite and phengite.A second (DB18)eclogite was sampled about 50m to the north of DB17and is strongly foliated with a similar mineralogy assemblage but smaller garnet grains.3.MethodsSample preparation,mineral separation and chemical procedures for isotope analysis,instrumentation and standard reference materials used to determine whole rock and bulk mineral compositions,in situ major and trace element analyses (Institute for Study of the Earth's Interior,Okayama University at Misasa,Japan),zircon U –Pb isotope and trace element analyses (China University of Geosciences in Wuhan),Lu –Hf and Sm –Nd isotope analyses (Washington State University),Ar –Ar isotope analyses (Guangzhou Institute of Geo-chemistry,Chinese Academy of Sciences)and oxygen isotope analyses (University of Science and Technology of China)are described in the Appendix .4.Results4.1.Bulk chemical compositionThe Xiongdian eclogites are mainly of basaltic composition,but they show a wide range of major and trace element abundances.Despite the high SiO 2(52–58%)and low TiO 2(0.32–0.43%)contents,Fig.2.Whole rock chemical analysis data.(a)Chondrite-normalized REE distribution patterns of the Xiongdian eclogites.(b)Primitive-mantle-normalized spidergrams of the Xiongdianeclogites.Fig.3.Backscattered-electron images and rim-to-rim major-element compositional zoning pro files of representative garnets in the matrix and as inclusions in zircon.Amp —amphibole;Ap —apatite;Cal —calcite;Cpx —clinopyroxene;Zo —zoisite;Phen —phengite;Omp —omphacite;Qtz —quartz;Zrn —zircon.330H.Cheng et al./Lithos 110(2009)327–342they have MgO=5.1–9.9%,Cr=430–1118ppm,Ni=88–247ppm (Table 1;Li et al.,2001;Fu et al.,2002;Jahn et al.,2005).In contrast to existing LREE-enriched chondritic REE patterns,our samples have rather flat REE patterns around ten times more chondritic abundances with small,both negative and positive Eu anomalies (Fig.2a).Rubidium is depleted and Sr displays enrichment with respect to Ce.Both negative and no Nb anomalies relative to La were observed (Fig.2b).The N-MORB-normalized value of Th is around 0.5,lower than previous reported values of up to 25(Li et al.,2001).4.2.Petrography and mineral compositionThe Xiongdian eclogites occur as thin layers intercalated with dolomite –plagioclase gneiss and phengite –quartz schist (Fu et al.,2002),mainly consisting of garnet,omphacite,epidote (clinozoisite),phengite and minor amphibole,quartz and kyanite (Fig.3).Zircons were observed both as inclusions in garnet porphyroblasts and in the matrix.The samples have similar mineral assemblages,but differ in modal compositions.Omphacite (X Jd =0.46–0.48)is unzoned.Phengite has 3.30–3.32Si apfu and ∼0.4wt.%TiO 2.Garnets range in size from 0.5to 5mm in diameter,either as porphyroblasts or as coalesced polycrystals,mostly with idioblastic shapes with inclusions of quartz,calcite,apatite and omphacite (Fig.3).Garnet is largely homogeneous (Prp 24–25Alm 49–50Grs 24–25Sps 1.5–1.9),but shows a slightly Mn-enriched core (Fig.3d;Table 2).HREEs in large garnet porphyroblasts,such as Yb and Lu,display weak but continuous decreases in concentration from core to rim (Fig.4a),mimicking the MnO zoning pattern,which could be explained by their high af finity for garnet and likely arises from an overall Rayleigh distillation process during early garnet growth (Hollister,1966;Otamendi et al.,2002).However,the limited variation in MREE concentrations,such as Sm and Nd,in garnet with respect to the weak zoning in HREE (Fig.4a)might be explained by growth in an environment where MREEs are not limited and continuously supplied by the breakdown of other phases.Hafnium has a fairly flat pro file (Table 3),re flecting its incompatible character in garnet and absence of Hf-competing reactions involved in garnet growth.Two distinct domains can be de fined in the large garnet porphyroblasts based on the chemical zoning and the abundance of inclusions.These zones are an inclusion-rich core with richer Mn and HREE and an inclusion-free rim with poorer Mn and HREE (Fig.3d).The inclusion-free rim for individual garnet has a rather similar width of 200–250μm (Fig.3).Although concentrations of Nd (0.22–0.41ppm)and Sm (0.33–0.48ppm)vary within single garnet grains,the Sm/Nd ratios (0.8–2.2)are consistentTable 2Representative major-element data of the garnets,omphacites,phengites,amphiboles and zoisites.(wt.%)Grt Omp RimCore Inclusions-in-zircon Rim Core SiO 238.6838.6438.6638.5338.6538.6637.8637.7555.9356.1256.1356.20TiO 20.050.060.050.050.050.050.050.080.120.110.110.11Al 2O 321.9221.9422.0721.9921.9921.8421.6821.8611.2611.2211.3311.26FeO ⁎22.9823.0523.0623.1623.0523.1124.4224.33 4.25 4.23 4.32 4.27MnO 0.680.720.790.880.750.680.990.930.030.020.030.02MgO 6.37 6.38 6.28 6.31 6.36 6.35 4.23 4.748.158.027.968.13CaO 9.108.949.028.929.038.9910.579.5013.2213.3613.3213.34Na 2O 0.030.030.030.030.030.030.020.01 6.65 6.41 6.39 6.42K 2O 0.000.000.000.000.000.000.000.000.000.000.000.00Total 99.8099.7799.9699.8799.9199.7199.8299.2199.6099.6099.7099.87O.N.12121212121212126666Si 2.986 2.984 2.981 2.975 2.980 2.988 2.958 2.962 1.996 2.010 2.010 2.007Al 1.994 1.997 2.006 2.001 1.999 1.990 1.997 2.0210.4740.4730.4780.474Ti 0.0030.0030.0030.0030.0030.0030.0030.0050.0030.0030.0030.003Fe 2+ 1.486 1.491 1.489 1.499 1.489 1.496 1.596 1.5990.1270.1270.1290.128Mn 0.0440.0470.0520.0580.0490.0440.0660.0620.0010.0010.0010.001Mg 0.7330.7350.7220.7260.7310.7320.4930.5540.4340.4280.4250.433Ca 0.7530.7400.7450.7380.7460.7440.8850.7980.5060.5130.5110.511Na 0.0040.0050.0050.0050.0050.0050.0030.0020.4600.4450.4430.445K0.0000.0000.0000.0000.0000.0000.0000.0000.0000.0000.0000.000Phn Amp Zo RimCore Rim Core Mantle Core SiO 248.8649.0949.3349.0147.0847.0746.7246.7539.0538.9239.0239.02TiO 20.400.410.410.400.220.220.220.220.130.130.130.12Al 2O 329.0328.6829.0129.1912.6612.8112.5812.6228.5528.2128.7328.62FeO ⁎ 1.99 1.99 2.00 1.9711.6011.4811.4611.36 6.01 6.01 6.03 6.07MnO 0.000.000.000.010.100.090.090.090.050.050.060.05MgO 2.79 2.77 2.78 2.8012.2012.4712.4412.300.070.060.070.07CaO 0.010.010.010.019.9710.0910.0710.1024.1023.8624.1324.14Na 2O 0.930.920.920.91 2.79 2.77 2.82 2.830.000.000.000.00K 2O 10.009.919.819.780.480.470.470.470.000.000.000.00Total 94.0293.7894.2894.0997.0997.4996.8896.7697.9697.2498.1698.09O.N.111111112323232312.512.512.512.5Si 3.302 3.323 3.318 3.304 6.831 6.800 6.799 6.809 3.008 3.019 3.000 3.003Al 2.313 2.288 2.300 2.319 2.164 2.182 2.158 2.167 2.592 2.579 2.603 2.596Ti 0.0200.0210.0210.0200.0240.0240.0240.0240.0070.0070.0070.007Fe 2+0.1120.1130.1130.111 1.407 1.387 1.394 1.3830.3870.3900.3880.390Mn 0.0000.0000.0000.0000.0120.0120.0120.0120.0040.0040.0040.004Mg 0.2820.2800.2790.282 2.639 2.686 2.699 2.6700.0070.0070.0070.008Ca 0.0010.0010.0010.001 1.550 1.562 1.570 1.577 1.989 1.983 1.988 1.990Na 0.1220.1210.1200.1190.7840.7770.7950.7980.0000.0000.0000.000K0.8620.8550.8420.8410.0890.0870.0880.0880.0000.0000.0000.000⁎Total iron;concentrations reported as wt.%.331H.Cheng et al./Lithos 110(2009)327–342with those obtained by ID-MC-ICPMS (1.9–2.4)within error (Fig.5a),indicating that the Nd isotopic analyses in this study are essentially unaffected by MREE-rich inclusions,likely due to ef ficient mineral picking and/or concentrated H 2SO 4pre-leaching.The consistent Hf concentrations of 0.10–0.13ppm within single grains with those (0.11–0.13ppm)by ID-MC-ICPMS indicates the Hf-rich phases were essentially removed during digestion (Fig.5b).The overall Lu concentration slightly skews towards the garnet rim because of the weak zoning pattern and the spherical geometry effect,i.e.,the outershells dominate the volume of Lu (Cheng et al.,2008a ).The 0.90–0.93ppm Lu contents by ID-MC-ICPMS apparently resemble those of the garnet rim,which could be readily explained by the spherical geometry effect.However,we interpret this with caution because individual garnet porphyroblasts could have different zoning patterns and the individual Lu pro file might not be representative of the population of garnet grains,although the chemical zoning center (nucleation site)coincides with the geometric center (Fig.3d),suggesting asymmetric garnet growth.In addition,biased mineral hand-picking should be considered (Cheng et al.,2008a,b ).Moreover,since the thin-section preparation method for this study cannot ensure that the real center of the garnet was exposed,the observed zoning here likely only represents a minimum zoning of particular garnet porphyroblasts.4.3.Estimation of P –T conditionsMetamorphic peak P –T conditions of 2.2GPa and 620°C for the DB17Xiongdian eclogite (Fig.6)are evaluated on the basis of recent cali-brations of the assemblage garnet+omphacite+phengite+kyanite+quartz,according to the dataset of Holland and Powell (1998).Higher P –T values of 2.4GPa and 650°C are calculated with the calibrations of Krogh Ravna and Terry (2004).While a temperature of 620±29°C is estimated by quartz –garnet O isotope thermometer (Zheng,1993),Ti-in-zircon thermometer (Watson et al.,2006;Ferry and Watson,2007)gives similar value of 695±22°C.Zr-in-rutile thermometer (Watson et al.,2006;Ferry and Watson,2007)yields a lower value of 634–652°C and a similar temperature of 683–701°C (Fig.6)when using the pressure-dependent calibration of Tomkins et al.(2007)at 2.2GPa.Calibration 1uses updated versions of the thermodynamic dataset and activity models in the programs THERMOCALC3.26and AX (Holland,Powell,1998;latest updated dataset;Powell et al.,1998)by using an avPT calculation in the simpli fied model system NCKFMASH with excess SiO 2and H 2O.Calibration 2uses thermobarometry based on the database of Holland and Powell (1998)and activity models for garnet (Ganguly et al.,1996),clinopyroxene (Holland and Powell,1990)and phengite (Holland and Powell,1998).Analyses of garnet,omphacite and phengite (Table 2)were processed according to the two calibrations.Calibration 3uses mineral O isotope compositions (Table 4)to estimate temperature based on the quartz –garnet O isotope thermometer (Zheng,1993).Calibrations 4and 5use Ti contents in zircon by LA-ICPMS and Zr concentration of rutile by SIMS (Table 5)to temperature estimations based on the Ti-in-zircon and Zr-in-rutile thermometers,respectively (Watson et al.,2006;Ferry and Watson,2007;Tomkins et al.,2007).The assemblage of garnet –omphacite –kyanite –phengite –quartz is representative of metamorphic peak conditions of theXiongdianFig.4.Chondrite-normalized REE patterns (Sun and McDonough,1989)of zircons,garnets and omphacite from Xiongdian eclogite (a)and REE distribution patterns between zircon and garnet (b).The equilibrium D REE(Zrn/Grt)values of Rubatto (2002),Whitehouse and Platt (2003)and Rubatto and Hermann (2007)are presented for comparison.Table 3SIMS Sm,Nd,Hf and Lu concentration pro files of the garnets in Figs.4and 5.(ppm)RimCore Cpx Li 0.93 1.140.880.840.890.980.750.520.990.580.690.870.670.7522.1Sr 0.100.130.120.120.100.100.100.120.110.120.130.100.110.1033.5Y 45.646.846.647.346.447.148.350.052.053.553.155.354.657.80.92Hf 0.110.130.120.120.110.110.120.120.120.100.110.100.100.100.41La 0.010.020.020.010.000.000.010.010.010.010.010.010.020.010.02Ce 0.040.050.050.060.050.040.040.040.050.030.040.040.050.030.12Pr 0.010.020.030.020.020.020.020.020.020.020.030.020.020.020.03Nd 0.390.330.280.380.350.270.220.280.340.310.270.410.280.260.36Sm 0.450.360.380.440.470.410.480.450.450.410.340.330.420.410.31Eu 0.270.270.270.280.300.240.280.280.250.300.290.240.250.220.22Gd 1.85 1.96 1.75 1.80 1.85 1.78 1.85 1.84 1.93 1.82 1.57 1.92 1.69 1.530.65Dy 5.68 5.86 5.58 6.18 5.87 5.84 5.79 6.19 6.46 6.40 5.50 6.91 6.09 6.400.26Er 3.74 4.13 4.04 4.25 4.23 4.16 3.76 4.15 4.65 4.99 4.53 4.98 4.63 5.200.06Yb 4.10 4.18 4.01 3.86 4.23 4.11 4.49 4.34 4.97 5.19 5.19 5.65 5.10 5.690.12Lu0.900.910.880.840.840.891.131.151.281.261.261.331.321.420.01332H.Cheng et al./Lithos 110(2009)327–342eclogite.A partly-calibrated thermobarometer is de fined by the three reactions of 3Celadonite +1Pyrope +2Grossular =3Muscovite +6Diopside,2Kyanite+3Diopside =1Pyrope +1Grossular +2Quartz,and 3Celadonite +4Kyanite=3Muscovite +1Pyrope +4Quartz.An intersection point of 2.2GPa and 620°C is de fined and therefore independent of commonly-used Fe –Mg exchange thermometers.This offers an advantage with regards to garnet –clinopyroxene,which is prone to retrograde reactions and problems stemming from ferric iron estimation of omphacite (Li et al.,2005).Results are plotted according to the calibrations mentioned above.The three reactions and intersection points are shown according to programs of calibrations 1–5in Fig.6.4.4.Oxygen isotopic dataThe O isotope compositions of minerals for the two eclogites are presented in Table 4.When paired with quartz for isotope geothermo-metry,garnet,omphacite,phengite,kyanite,zoisite and amphibole yield temperatures of 620±29,563±35,567±43,508±31,404±28and 685±39°C for eclogite DB17,respectively.Because these temperatures are concordant with rates of O diffusion and thus closure temperatures in the mineral assemblage garnet +omphacite +kyanite+phengite+quartz (Zheng and Fu,1998),representative of metamorphic peak conditions,a continuous resetting of O isotopes in the different mineral-pair systems is evident during cooling (Giletti,1986;Eiler et al.,1993;Chen et al.,2007).Quartz –garnet pairs from eclogite DB17give temperatures of 620±29°C,which are consistent with those calibrated by the THERMOCALCmethod,indicating that O isotope equilibrium was achieved and preserved during eclogite –facies recrystallization (Fig.7a).This is also evidenced by the apparent equilibrium fractionation between garnet and omphacite (Fig.7b).In contrast,equilibrium fractionation was not attained between garnets and omphacites in eclogite DB18.The calculated quartz –amphibole pair temperature of 685±39°C is distinctly higher than the 508±31°C from the quartz –zoisite pair.Because oxygen diffusion in amphibole is faster than in zoisite and kyanite (Zheng and Fu,1998),amphibole exchanges oxygen isotopes with water faster than zoisite during retrogression.Consequently,the O isotope temperature increases for the quartz –amphibole pair,whereas the quartz –zoisite temperature decreases relative to the formation temperature.In this regard,the retrograde metamorphism of amphibolite –facies should take place at a temperature between ∼685and ∼508°C.On the other hand,the low quartz –kyanite pair temperature (404±28°C)could be interpreted as a result of in fluence by retrogressive metamorphism without a clear geologicalmeaning.Fig.5.Sm/Nd versus Nd and Lu/Hf versus Hf plots for garnet and whole rock.ID:data obtained by the isotope dilution method using MC-ICPMS.IMS:data obtained by ion microprobe.bombWR —whole rock by bomb-digestion,savWR —whole rock by Savillex-digestion.Error bars for both IMS and ID methods are signi ficantly smaller than thesymbols.Fig.6.Peak P –T estimates of the Xiongdian eclogite.Reactions of py +2gr +3cel =6di +3mu;3di+2ky =py+gr +2q;and 3cel +4ky =py +3mu +4q and intersection points are plotted according to the calibrations of Holland and Powell (1998,latest updated dataset)in solid lines and Krogh Ravna and Terry (2004)in dashed lines.Coesite quartz equilibrium is also shown (Holland and Powell,1998).Abbreviations:alm —almandine,gr —grossular,py —pyrope,cel —celadonite,mu —muscovite,di —diopside,jd —jadeite,coe —coesite.Temperatures estimated by quartz –garnet oxygen isotope thermometry (Zheng,1993),Ti-in-zircon and Zr-in-rutile thermometries (Watson et al.,2006;Tomkins et al.,2007)are also shown.Table 4Oxygen isotope data of minerals for the Xiongdian eclogite.Sample number Mineral δ18O (‰)Pair Δ18O (‰)T 1(°C)T 2(°C)DB17Quartz 12.86,12.66Phengite 10.26,10.14Qtz –Phn 2.57567±43Garnet 8.83,8.85Qtz –Grt 3.93620±29605±22Omphacite 9.64,9.56Qtz –Omp 3.17563±35574±28Zoisite 9.31,9.43Qtz –Zo 3.40508±31494±21Amphibole 9.83,9.60Qtz –Amp 3.06685±39Kyanite 9.36,–Qtz –Ky3.41404±28WR 9.85,9.91DB18Garnet 9.74,9.59Omphacite 8.58,8.48Omp –Grt −1.14WR10.15,9.99T 1and T 2were calculated based on the theoretical calibrations of Zheng (1993)and Matthews (1994),respectively,with omphacite (Jd 45Di 55).Uncertainty on the temperature is derived from error propagation of the average reproducibility of ±15‰for δ18O (‰)values in the fractionation equations.333H.Cheng et al./Lithos 110(2009)327–342。
基于MSPA和电路理论的珠三角核心城市通风廊道构建
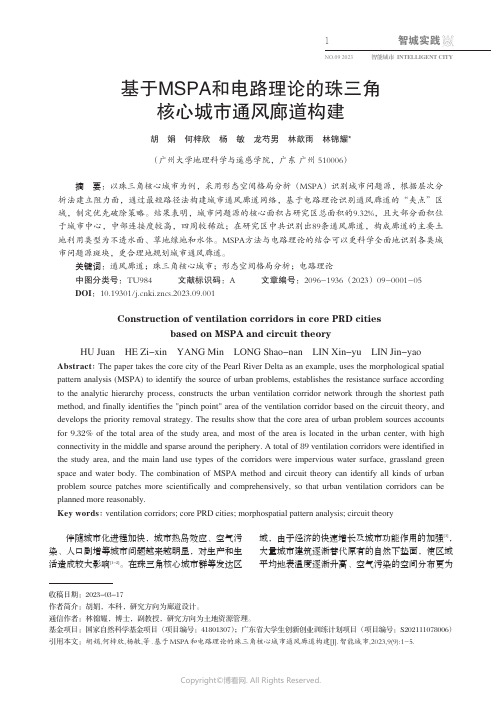
基于MSPA和电路理论的珠三角核心城市通风廊道构建胡娟 何梓欣 杨敏 龙芍男 林歆雨 林锦耀*(广州大学地理科学与遥感学院,广东 广州 510006)摘要:以珠三角核心城市为例,采用形态空间格局分析(MSPA)识别城市问题源,根据层次分析法建立阻力面,通过最短路径法构建城市通风廊道网络,基于电路理论识别通风廊道的“夹点”区域,制定优先破除策略。
结果表明,城市问题源的核心面积占研究区总面积的9.32%,且大部分面积位于城市中心,中部连接度较高,四周较稀疏;在研究区中共识别出89条通风廊道,构成廊道的主要土地利用类型为不透水面、草地绿地和水体。
MSPA方法与电路理论的结合可以更科学全面地识别各类城市问题源斑块,更合理地规划城市通风廊道。
关键词:通风廊道;珠三角核心城市;形态空间格局分析;电路理论中图分类号:TU984 文献标识码:A 文章编号:2096-1936(2023)09-0001-05DOI:10.19301/ki.zncs.2023.09.001Construction of ventilation corridors in core PRD citiesbased on MSPA and circuit theoryHU Juan HE Zi-xin YANG Min LONG Shao-nan LIN Xin-yu LIN Jin-yao Abstract:The paper takes the core city of the Pearl River Delta as an example, uses the morphological spatial pattern analysis (MSPA) to identify the source of urban problems, establishes the resistance surface according to the analytic hierarchy process, constructs the urban ventilation corridor network through the shortest path method, and finally identifies the "pinch point" area of the ventilation corridor based on the circuit theory, and develops the priority removal strategy. The results show that the core area of urban problem sources accounts for 9.32% of the total area of the study area, and most of the area is located in the urban center, with high connectivity in the middle and sparse around the periphery. A total of 89 ventilation corridors were identified in the study area, and the main land use types of the corridors were impervious water surface, grassland green space and water body. The combination of MSPA method and circuit theory can identify all kinds of urban problem source patches more scientifically and comprehensively, so that urban ventilation corridors can be planned more reasonably.Key words:ventilation corridors; core PRD cities; morphospatial pattern analysis; circuit theory伴随城市化进程加快,城市热岛效应、空气污染、人口剧增等城市问题越来越明显,对生产和生活造成较大影响[1-2]。
基于高斯混合模型的生物医学领域双语句子对齐
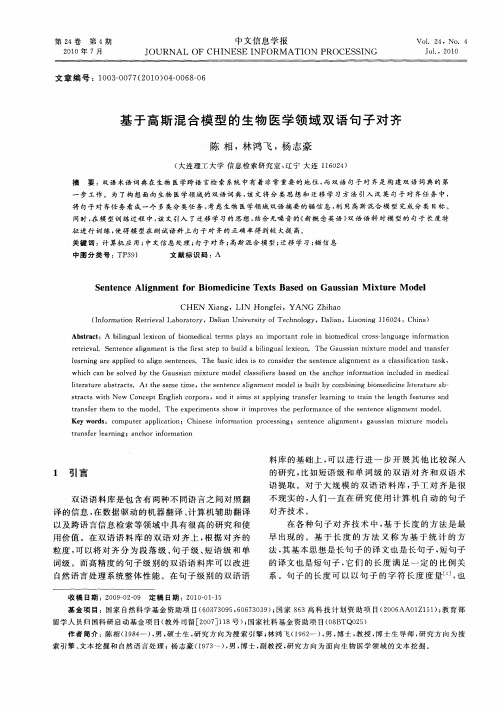
(no mainRer v l b r tr If r t ti a La oao y,Dain Unv riyo c n lg o e l iest f a Teh oo y,Dain,Lio ig 1 6 2 l a a nn 1 0 4,Chn ) ia
A b ta t A ii ua e c n ofbim e c lt r s l y n m p t nt o e i o e c l r s —a sr c : blng llxio o dia e m p a s a i ora r l n bim dia c o s lngu ge i o m a in a nf r to r t iv 1 Se t nc i m e s t e fr tsep t uid a biigua e ion The Ga sa i ur o la r s e e re a. n e eal gn nti h is t O b l ln ll x c . us in m xt e m de nd tan f r
基 于高 斯 混合 模 型 的生 物 医学领 域 双语 句 子 对 齐
陈 相 ,林 鸿 飞 ,杨 志 豪
( 连 理 工 大 学 信 息 检 索 研 究 室 , 宁 大连 l6 2 ) 大 辽 1 0 4 摘
一
要 :双语 术语 词 典 在 生 物 医学跨 语 言 检 索 系统 中有 着 非 常 重 要 的 地 位 , 而双 语 句子 对 齐 是 构 建 双 语 词 典 的 第
征 进 行 训 练 , 得 模 型在 测 试语 料 上 句 子 对 齐 的 正 确 率得 到较 大ห้องสมุดไป่ตู้ 高 。 使
关 键 词 :计 算机 应 用 ; 中文 信 息 处 理 ; 句子 对 齐 ; 高斯 混 合 模 型 ; 迁移 学 习 ; 信 息 锚
Advances in
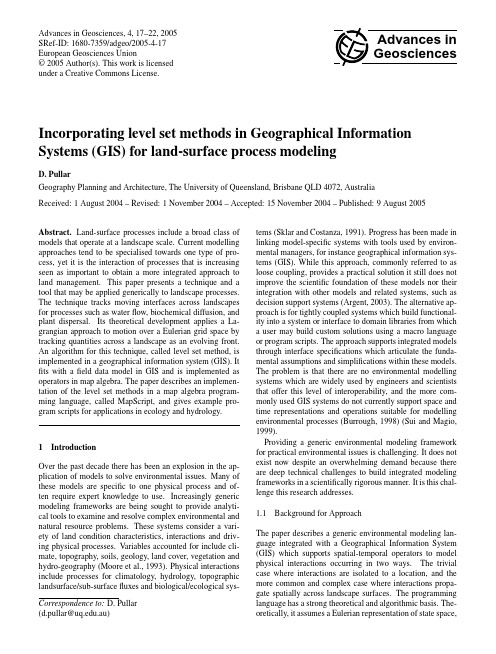
Advances in Geosciences,4,17–22,2005 SRef-ID:1680-7359/adgeo/2005-4-17 European Geosciences Union©2005Author(s).This work is licensed under a Creative CommonsLicense.Advances in GeosciencesIncorporating level set methods in Geographical Information Systems(GIS)for land-surface process modelingD.PullarGeography Planning and Architecture,The University of Queensland,Brisbane QLD4072,Australia Received:1August2004–Revised:1November2004–Accepted:15November2004–Published:9August2005nd-surface processes include a broad class of models that operate at a landscape scale.Current modelling approaches tend to be specialised towards one type of pro-cess,yet it is the interaction of processes that is increasing seen as important to obtain a more integrated approach to land management.This paper presents a technique and a tool that may be applied generically to landscape processes. The technique tracks moving interfaces across landscapes for processes such as waterflow,biochemical diffusion,and plant dispersal.Its theoretical development applies a La-grangian approach to motion over a Eulerian grid space by tracking quantities across a landscape as an evolving front. An algorithm for this technique,called level set method,is implemented in a geographical information system(GIS).It fits with afield data model in GIS and is implemented as operators in map algebra.The paper describes an implemen-tation of the level set methods in a map algebra program-ming language,called MapScript,and gives example pro-gram scripts for applications in ecology and hydrology.1IntroductionOver the past decade there has been an explosion in the ap-plication of models to solve environmental issues.Many of these models are specific to one physical process and of-ten require expert knowledge to use.Increasingly generic modeling frameworks are being sought to provide analyti-cal tools to examine and resolve complex environmental and natural resource problems.These systems consider a vari-ety of land condition characteristics,interactions and driv-ing physical processes.Variables accounted for include cli-mate,topography,soils,geology,land cover,vegetation and hydro-geography(Moore et al.,1993).Physical interactions include processes for climatology,hydrology,topographic landsurface/sub-surfacefluxes and biological/ecological sys-Correspondence to:D.Pullar(d.pullar@.au)tems(Sklar and Costanza,1991).Progress has been made in linking model-specific systems with tools used by environ-mental managers,for instance geographical information sys-tems(GIS).While this approach,commonly referred to as loose coupling,provides a practical solution it still does not improve the scientific foundation of these models nor their integration with other models and related systems,such as decision support systems(Argent,2003).The alternative ap-proach is for tightly coupled systems which build functional-ity into a system or interface to domain libraries from which a user may build custom solutions using a macro language or program scripts.The approach supports integrated models through interface specifications which articulate the funda-mental assumptions and simplifications within these models. The problem is that there are no environmental modelling systems which are widely used by engineers and scientists that offer this level of interoperability,and the more com-monly used GIS systems do not currently support space and time representations and operations suitable for modelling environmental processes(Burrough,1998)(Sui and Magio, 1999).Providing a generic environmental modeling framework for practical environmental issues is challenging.It does not exist now despite an overwhelming demand because there are deep technical challenges to build integrated modeling frameworks in a scientifically rigorous manner.It is this chal-lenge this research addresses.1.1Background for ApproachThe paper describes a generic environmental modeling lan-guage integrated with a Geographical Information System (GIS)which supports spatial-temporal operators to model physical interactions occurring in two ways.The trivial case where interactions are isolated to a location,and the more common and complex case where interactions propa-gate spatially across landscape surfaces.The programming language has a strong theoretical and algorithmic basis.The-oretically,it assumes a Eulerian representation of state space,Fig.1.Shows a)a propagating interface parameterised by differ-ential equations,b)interface fronts have variable intensity and may expand or contract based onfield gradients and driving process. but propagates quantities across landscapes using Lagrangian equations of motion.In physics,a Lagrangian view focuses on how a quantity(water volume or particle)moves through space,whereas an Eulerian view focuses on a localfixed area of space and accounts for quantities moving through it.The benefit of this approach is that an Eulerian perspective is em-inently suited to representing the variation of environmen-tal phenomena across space,but it is difficult to conceptu-alise solutions for the equations of motion and has compu-tational drawbacks(Press et al.,1992).On the other hand, the Lagrangian view is often not favoured because it requires a global solution that makes it difficult to account for local variations,but has the advantage of solving equations of mo-tion in an intuitive and numerically direct way.The research will address this dilemma by adopting a novel approach from the image processing discipline that uses a Lagrangian ap-proach over an Eulerian grid.The approach,called level set methods,provides an efficient algorithm for modeling a natural advancing front in a host of settings(Sethian,1999). The reason the method works well over other approaches is that the advancing front is described by equations of motion (Lagrangian view),but computationally the front propagates over a vectorfield(Eulerian view).Hence,we have a very generic way to describe the motion of quantities,but can ex-plicitly solve their advancing properties locally as propagat-ing zones.The research work will adapt this technique for modeling the motion of environmental variables across time and space.Specifically,it will add new data models and op-erators to a geographical information system(GIS)for envi-ronmental modeling.This is considered to be a significant research imperative in spatial information science and tech-nology(Goodchild,2001).The main focus of this paper is to evaluate if the level set method(Sethian,1999)can:–provide a theoretically and empirically supportable methodology for modeling a range of integral landscape processes,–provide an algorithmic solution that is not sensitive to process timing,is computationally stable and efficient as compared to conventional explicit solutions to diffu-sive processes models,–be developed as part of a generic modelling language in GIS to express integrated models for natural resource and environmental problems?The outline for the paper is as follow.The next section will describe the theory for spatial-temporal processing us-ing level sets.Section3describes how this is implemented in a map algebra programming language.Two application examples are given–an ecological and a hydrological ex-ample–to demonstrate the use of operators for computing reactive-diffusive interactions in landscapes.Section4sum-marises the contribution of this research.2Theory2.1IntroductionLevel set methods(Sethian,1999)have been applied in a large collection of applications including,physics,chemistry,fluid dynamics,combustion,material science,fabrication of microelectronics,and computer vision.Level set methods compute an advancing interface using an Eulerian grid and the Lagrangian equations of motion.They are similar to cost distance modeling used in GIS(Burroughs and McDonnell, 1998)in that they compute the spread of a variable across space,but the motion is based upon partial differential equa-tions related to the physical process.The advancement of the interface is computed through time along a spatial gradient, and it may expand or contract in its extent.See Fig.1.2.2TheoryThe advantage of the level set method is that it models mo-tion along a state-space gradient.Level set methods start with the equation of motion,i.e.an advancing front with velocity F is characterised by an arrival surface T(x,y).Note that F is a velocityfield in a spatial sense.If F was constant this would result in an expanding series of circular fronts,but for different values in a velocityfield the front will have a more contorted appearance as shown in Fig.1b.The motion of thisinterface is always normal to the interface boundary,and its progress is regulated by several factors:F=f(L,G,I)(1)where L=local properties that determine the shape of advanc-ing front,G=global properties related to governing forces for its motion,I=independent properties that regulate and influ-ence the motion.If the advancing front is modeled strictly in terms of the movement of entity particles,then a straightfor-ward velocity equation describes its motion:|∇T|F=1given T0=0(2) where the arrival function T(x,y)is a travel cost surface,and T0is the initial position of the interface.Instead we use level sets to describe the interface as a complex function.The level set functionφis an evolving front consistent with the under-lying viscosity solution defined by partial differential equa-tions.This is expressed by the equation:φt+F|∇φ|=0givenφ(x,y,t=0)(3)whereφt is a complex interface function over time period 0..n,i.e.φ(x,y,t)=t0..tn,∇φis the spatial and temporal derivatives for viscosity equations.The Eulerian view over a spatial domain imposes a discretisation of space,i.e.the raster grid,which records changes in value z.Hence,the level set function becomesφ(x,y,z,t)to describe an evolv-ing surface over time.Further details are given in Sethian (1999)along with efficient algorithms.The next section de-scribes the integration of the level set methods with GIS.3Map algebra modelling3.1Map algebraSpatial models are written in a map algebra programming language.Map algebra is a function-oriented language that operates on four implicit spatial data types:point,neighbour-hood,zonal and whole landscape surfaces.Surfaces are typ-ically represented as a discrete raster where a point is a cell, a neighbourhood is a kernel centred on a cell,and zones are groups of mon examples of raster data include ter-rain models,categorical land cover maps,and scalar temper-ature surfaces.Map algebra is used to program many types of landscape models ranging from land suitability models to mineral exploration in the geosciences(Burrough and Mc-Donnell,1998;Bonham-Carter,1994).The syntax for map algebra follows a mathematical style with statements expressed as equations.These equations use operators to manipulate spatial data types for point and neighbourhoods.Expressions that manipulate a raster sur-face may use a global operation or alternatively iterate over the cells in a raster.For instance the GRID map algebra (Gao et al.,1993)defines an iteration construct,called do-cell,to apply equations on a cell-by-cell basis.This is triv-ially performed on columns and rows in a clockwork manner. However,for environmental phenomena there aresituations Fig.2.Spatial processing orders for raster.where the order of computations has a special significance. For instance,processes that involve spreading or transport acting along environmental gradients within the landscape. Therefore special control needs to be exercised on the order of execution.Burrough(1998)describes two extra control mechanisms for diffusion and directed topology.Figure2 shows the three principle types of processing orders,and they are:–row scan order governed by the clockwork lattice struc-ture,–spread order governed by the spreading or scattering ofa material from a more concentrated region,–flow order governed by advection which is the transport of a material due to velocity.Our implementation of map algebra,called MapScript (Pullar,2001),includes a special iteration construct that sup-ports these processing orders.MapScript is a lightweight lan-guage for processing raster-based GIS data using map alge-bra.The language parser and engine are built as a software component to interoperate with the IDRISI GIS(Eastman, 1997).MapScript is built in C++with a class hierarchy based upon a value type.Variants for value types include numeri-cal,boolean,template,cells,or a grid.MapScript supports combinations of these data types within equations with basic arithmetic and relational comparison operators.Algebra op-erations on templates typically result in an aggregate value assigned to a cell(Pullar,2001);this is similar to the con-volution integral in image algebras(Ritter et al.,1990).The language supports iteration to execute a block of statements in three ways:a)docell construct to process raster in a row scan order,b)dospread construct to process raster in a spreadwhile(time<100)dospreadpop=pop+(diffuse(kernel*pop))pop=pop+(r*pop*dt*(1-(pop/K)) enddoendwhere the diffusive constant is stored in thekernel:Fig.3.Map algebra script and convolution kernel for population dispersion.The variable pop is a raster,r,K and D are constants, dt is the model time step,and the kernel is a3×3template.It is assumed a time step is defined and the script is run in a simulation. Thefirst line contained in the nested cell processing construct(i.e. dospread)is the diffusive term and the second line is the population growth term.order,c)doflow to process raster byflow order.Examples are given in subsequent sections.Process models will also involve a timing loop which may be handled as a general while(<condition>)..end construct in MapScript where the condition expression includes a system time variable.This time variable is used in a specific fashion along with a system time step by certain operators,namely diffuse()andfluxflow() described in the next section,to model diffusion and advec-tion as a time evolving front.The evolving front represents quantities such as vegetation growth or surface runoff.3.2Ecological exampleThis section presents an ecological example based upon plant dispersal in a landscape.The population of a species follows a controlled growth rate and at the same time spreads across landscapes.The theory of the rate of spread of an organism is given in Tilman and Kareiva(1997).The area occupied by a species grows log-linear with time.This may be modelled by coupling a spatial diffusion term with an exponential pop-ulation growth term;the combination produces the familiar reaction-diffusion model.A simple growth population model is used where the reac-tion term considers one population controlled by births and mortalities is:dN dt =r·N1−NK(4)where N is the size of the population,r is the rate of change of population given in terms of the difference between birth and mortality rates,and K is the carrying capacity.Further dis-cussion of population models can be found in Jrgensen and Bendoricchio(2001).The diffusive term spreads a quantity through space at a specified rate:dudt=Dd2udx2(5) where u is the quantity which in our case is population size, and D is the diffusive coefficient.The model is operated as a coupled computation.Over a discretized space,or raster,the diffusive term is estimated using a numerical scheme(Press et al.,1992).The distance over which diffusion takes place in time step dt is minimally constrained by the raster resolution.For a stable computa-tional process the following condition must be satisfied:2Ddtdx2≤1(6) This basically states that to account for the diffusive pro-cess,the term2D·dx is less than the velocity of the advancing front.This would not be difficult to compute if D is constant, but is problematic if D is variable with respect to landscape conditions.This problem may be overcome by progressing along a diffusive front over the discrete raster based upon distance rather than being constrained by the cell resolution.The pro-cessing and diffusive operator is implemented in a map al-gebra programming language.The code fragment in Fig.3 shows a map algebra script for a single time step for the cou-pled reactive-diffusion model for population growth.The operator of interest in the script shown in Fig.3is the diffuse operator.It is assumed that the script is run with a given time step.The operator uses a system time step which is computed to balance the effect of process errors with effi-cient computation.With knowledge of the time step the it-erative construct applies an appropriate distance propagation such that the condition in Eq.(3)is not violated.The level set algorithm(Sethian,1999)is used to do this in a stable and accurate way.As a diffusive front propagates through the raster,a cost distance kernel assigns the proper time to each raster cell.The time assigned to the cell corresponds to the minimal cost it takes to reach that cell.Hence cell pro-cessing is controlled by propagating the kernel outward at a speed adaptive to the local context rather than meeting an arbitrary global constraint.3.3Hydrological exampleThis section presents a hydrological example based upon sur-face dispersal of excess rainfall across the terrain.The move-ment of water is described by the continuity equation:∂h∂t=e t−∇·q t(7) where h is the water depth(m),e t is the rainfall excess(m/s), q is the discharge(m/hr)at time t.Discharge is assumed to have steady uniformflow conditions,and is determined by Manning’s equation:q t=v t h t=1nh5/3ts1/2(8)putation of current cell(x+ x,t,t+ ).where q t is theflow velocity(m/s),h t is water depth,and s is the surface slope(m/m).An explicit method of calcula-tion is used to compute velocity and depth over raster cells, and equations are solved at each time step.A conservative form of afinite difference method solves for q t in Eq.(5). To simplify discussions we describe quasi-one-dimensional equations for theflow problem.The actual numerical com-putations are normally performed on an Eulerian grid(Julien et al.,1995).Finite-element approximations are made to solve the above partial differential equations for the one-dimensional case offlow along a strip of unit width.This leads to a cou-pled model with one term to maintain the continuity offlow and another term to compute theflow.In addition,all calcu-lations must progress from an uphill cell to the down slope cell.This is implemented in map algebra by a iteration con-struct,called doflow,which processes a raster byflow order. Flow distance is measured in cell size x per unit length. One strip is processed during a time interval t(Fig.4).The conservative solution for the continuity term using afirst or-der approximation for Eq.(5)is derived as:h x+ x,t+ t=h x+ x,t−q x+ x,t−q x,txt(9)where the inflow q x,t and outflow q x+x,t are calculated in the second term using Equation6as:q x,t=v x,t·h t(10) The calculations approximate discharge from previous time interval.Discharge is dynamically determined within the continuity equation by water depth.The rate of change in state variables for Equation6needs to satisfy a stability condition where v· t/ x≤1to maintain numerical stabil-ity.The physical interpretation of this is that afinite volume of water wouldflow across and out of a cell within the time step t.Typically the cell resolution isfixed for the raster, and adjusting the time step requires restarting the simulation while(time<120)doflow(dem)fvel=1/n*pow(depth,m)*sqrt(grade)depth=depth+(depth*fluxflow(fvel)) enddoendFig.5.Map algebra script for excess rainfallflow computed over a 120minute event.The variables depth and grade are rasters,fvel is theflow velocity,n and m are constants in Manning’s equation.It is assumed a time step is defined and the script is run in a simulation. Thefirst line in the nested cell processing(i.e.doflow)computes theflow velocity and the second line computes the change in depth from the previous value plus any net change(inflow–outflow)due to velocityflux across the cell.cycle.Flow velocities change dramatically over the course of a storm event,and it is problematic to set an appropriate time step which is efficient and yields a stable result.The hydrological model has been implemented in a map algebra programming language Pullar(2003).To overcome the problem mentioned above we have added high level oper-ators to compute theflow as an advancing front over a land-scape.The time step advances this front adaptively across the landscape based upon theflow velocity.The level set algorithm(Sethian,1999)is used to do this in a stable and accurate way.The map algebra script is given in Fig.5.The important operator is thefluxflow operator.It computes the advancing front for waterflow across a DEM by hydrologi-cal principles,and computes the local drainageflux rate for each cell.Theflux rate is used to compute the net change in a cell in terms offlow depth over an adaptive time step.4ConclusionsThe paper has described an approach to extend the function-ality of tightly coupled environmental models in GIS(Ar-gent,2004).A long standing criticism of GIS has been its in-ability to handle dynamic spatial models.Other researchers have also addressed this issue(Burrough,1998).The con-tribution of this paper is to describe how level set methods are:i)an appropriate scientific basis,and ii)able to perform stable time-space computations for modelling landscape pro-cesses.The level set method provides the following benefits:–it more directly models motion of spatial phenomena and may handle both expanding and contracting inter-faces,–is based upon differential equations related to the spatial dynamics of physical processes.Despite the potential for using level set methods in GIS and land-surface process modeling,there are no commercial or research systems that use this mercial sys-tems such as GRID(Gao et al.,1993),and research systems such as PCRaster(Wesseling et al.,1996)offerflexible andpowerful map algebra programming languages.But opera-tions that involve reaction-diffusive processing are specific to one context,such as groundwaterflow.We believe the level set method offers a more generic approach that allows a user to programflow and diffusive landscape processes for a variety of application contexts.We have shown that it pro-vides an appropriate theoretical underpinning and may be ef-ficiently implemented in a GIS.We have demonstrated its application for two landscape processes–albeit relatively simple examples–but these may be extended to deal with more complex and dynamic circumstances.The validation for improved environmental modeling tools ultimately rests in their uptake and usage by scientists and engineers.The tool may be accessed from the web site .au/projects/mapscript/(version with enhancements available April2005)for use with IDRSIS GIS(Eastman,1997)and in the future with ArcGIS. It is hoped that a larger community of users will make use of the methodology and implementation for a variety of environmental modeling applications.Edited by:P.Krause,S.Kralisch,and W.Fl¨u gelReviewed by:anonymous refereesReferencesArgent,R.:An Overview of Model Integration for Environmental Applications,Environmental Modelling and Software,19,219–234,2004.Bonham-Carter,G.F.:Geographic Information Systems for Geo-scientists,Elsevier Science Inc.,New York,1994. Burrough,P.A.:Dynamic Modelling and Geocomputation,in: Geocomputation:A Primer,edited by:Longley,P.A.,et al., Wiley,England,165-191,1998.Burrough,P.A.and McDonnell,R.:Principles of Geographic In-formation Systems,Oxford University Press,New York,1998. Gao,P.,Zhan,C.,and Menon,S.:An Overview of Cell-Based Mod-eling with GIS,in:Environmental Modeling with GIS,edited by: Goodchild,M.F.,et al.,Oxford University Press,325–331,1993.Goodchild,M.:A Geographer Looks at Spatial Information Theory, in:COSIT–Spatial Information Theory,edited by:Goos,G., Hertmanis,J.,and van Leeuwen,J.,LNCS2205,1–13,2001.Jørgensen,S.and Bendoricchio,G.:Fundamentals of Ecological Modelling,Elsevier,New York,2001.Julien,P.Y.,Saghafian,B.,and Ogden,F.:Raster-Based Hydro-logic Modelling of Spatially-Varied Surface Runoff,Water Re-sources Bulletin,31(3),523–536,1995.Moore,I.D.,Turner,A.,Wilson,J.,Jenson,S.,and Band,L.:GIS and Land-Surface-Subsurface Process Modeling,in:Environ-mental Modeling with GIS,edited by:Goodchild,M.F.,et al., Oxford University Press,New York,1993.Press,W.,Flannery,B.,Teukolsky,S.,and Vetterling,W.:Numeri-cal Recipes in C:The Art of Scientific Computing,2nd Ed.Cam-bridge University Press,Cambridge,1992.Pullar,D.:MapScript:A Map Algebra Programming Language Incorporating Neighborhood Analysis,GeoInformatica,5(2), 145–163,2001.Pullar,D.:Simulation Modelling Applied To Runoff Modelling Us-ing MapScript,Transactions in GIS,7(2),267–283,2003. Ritter,G.,Wilson,J.,and Davidson,J.:Image Algebra:An Overview,Computer Vision,Graphics,and Image Processing, 4,297–331,1990.Sethian,J.A.:Level Set Methods and Fast Marching Methods, Cambridge University Press,Cambridge,1999.Sklar,F.H.and Costanza,R.:The Development of Dynamic Spa-tial Models for Landscape Ecology:A Review and Progress,in: Quantitative Methods in Ecology,Springer-Verlag,New York, 239–288,1991.Sui,D.and R.Maggio:Integrating GIS with Hydrological Mod-eling:Practices,Problems,and Prospects,Computers,Environ-ment and Urban Systems,23(1),33–51,1999.Tilman,D.and P.Kareiva:Spatial Ecology:The Role of Space in Population Dynamics and Interspecific Interactions.Princeton University Press,Princeton,New Jersey,USA,1997. Wesseling C.G.,Karssenberg, D.,Burrough,P. A.,and van Deursen,W.P.:Integrating Dynamic Environmental Models in GIS:The Development of a Dynamic Modelling Language, Transactions in GIS,1(1),40–48,1996.。
- 1、下载文档前请自行甄别文档内容的完整性,平台不提供额外的编辑、内容补充、找答案等附加服务。
- 2、"仅部分预览"的文档,不可在线预览部分如存在完整性等问题,可反馈申请退款(可完整预览的文档不适用该条件!)。
- 3、如文档侵犯您的权益,请联系客服反馈,我们会尽快为您处理(人工客服工作时间:9:00-18:30)。
The Value Grid for Semantic TechnologiesAlistair MilesSTFC e-Science Centre, STFC Rutherford Appleton Laboratory, Chilton,OX11 0QX, UKes@AbstractThis paper situates formal ontologies as one of many products in a multi-tier value grid of semantic technologies.Incremental strategies for the exploitation of intermediate products in the value grid are discussed, as a possible step towards cost-effective, low-risk and scalable business models for the exploitation of semantic technologies. A case study is presented, illustrating a hypothetical value grid for the management of scientific data from a large-scale experimental facility. Suggestions are made for the design of predictable,repeatable collaborative processes for adding value in semantic technology value grids.1 IntroductionAn ontology is the product of a group of people, collaborating to articulate their commonly held conceptualisation of a domain, through the use of a formal logical language such as KIF or OWL [1, 2]. Typically, an ontology is intended for deployment in software systems which leverage the shared conceptualisation to provide unique,high-value services,such as data integration or information retrieval. Ontologies are not the only means of articulating a shared conceptualisation, however. Controlled vocabularies, taxonomies, thesauri, classification schemes, topic maps, subject heading systems, semantic networks – to name a selection – are all specifications of a shared conceptualisation, albeit“informal”or“semi-formal”. These and many other types of product have to be considered,in order to design solutions to specific problems at reasonable cost;solutions that are feasible,scalable and part of a sustainable business model. This begs a number of questions.What possible paths exist from knowledge expressed informally(unstructured information)to formal ontologies?How can these paths be broken down into stages, and what does each stage produce? In what ways can these different products be exploited?What are the likely costs, benefits and risks associated with different paths and different stages? How much human effort will be required, and how can this effort be reduced by computation? How can the necessary human effort be organised into efficient work flows that enable collaboration? Does economic and practical scalability vary with different paths and different products? Value chains and value gridsThis paper works towards answers to these questions, by viewing ontologies as products in a value grid of semantic technologies. The notion of a “value grid” has evolved from the original conception of a“value chain”,which is a sequence of value-enhancing activities,where raw materials are formed into components that are assembled into final products,distributed, sold and serviced [3]. The “value grid” extends this view,to see value creation as multi-dimensional rather than linear. In a value grid, the vertical dimension describes multiple tiers from primary inputs (raw materials) to end users; the horizontal dimension describes opportunities at the same tier across parallel value chains; and the diagonal dimension describes opportunities for integration between value chains [3].Value grids, rather than value chains, are used here, because the extra degrees of freedom allow for a subtle analysis of relationships between products such as thesauri and ontologies, where several possible configurations can be discussed.By exploring and “mapping-out” the value grid in which ontologies are situated, we may begin to define profit-maximising strategies for the exploitation of semantic technologies.Such strategies might,for example,identify and incrementally exploit a fine-grained sequence of products leading from unstructured information to formal ontologies. By taking this incremental approach,a project would not“overshoot”itsrequirements and formalise beyond what is necessary or worthwhile. A project would also be able to return value to its customers early and often.If there is a tight cycle whereby stakeholders perceive early returns on small levels of investment,they may be inspired to deepen their initial commitment.A heavyweight“all-or-nothing”approach to ontology engineering demands high levels of stakeholder commitment, both initially and on an ongoing basis, with delayed returns. Frustration can ensue because stakeholders cannot be persuaded to make the commitment required by such an approach, nor can they be persuaded to take ownership of the product. In some situations a formal ontology is the only product that can support the desired functionality, and therefore an“all-or-nothing”approach is appropriate. However,there are many other situations in which other products in the value grid may be exploited, and therefore other strategies become available.Section 2 begins an exploration of the value grid by re-analysing a case study involving the management of scientific data from a large scale facility.The case study explores options for adding value to a catalogue of experimental data, for which uncontrolled keywords are already present.Collaboration engineeringAlmost all value-adding activities in the exploitation of semantic technologies require at least some human intellectual input. Moreover, because semantic technologies demand shared conceptualisations,these activities are necessarily collaborative.This dependence on collaboration is a major source of both cost and risk throughout the value grid, because designing and managing predictable,repeatable collaborative processes is a significant challenge. The successful execution of a collaborative process traditionally depends on the involvement of a professional facilitator – someone who can design and enact a dynamic process, structuring tasks and managing relationships between people, tasks and technologies [4]. However, the continuous involvement of a professional facilitator is expensive.To reduce this dependence on professional facilitators, the field of collaboration engineering has sought to codify and package key“facilitation interventions” in forms that can be executed by team members themselves [4]. A “collaboration engineer” designs a group process in a way that can be transferred to a “practitioner” – a domain expert who can execute a single team process as a team leader in their particular domain.The collaboration process is broken down into a set of atomic collaborative activities(“thinklets”). Each of these basic units comprises a named, packaged,thinking activity that creates a predictable,repeatable pattern of collaboration among people working towards a goal. I.e. each unit provides a concrete group-dynamics intervention,complete with instructions for implementation as part of some group process [4].Collaborative activities may be characterised in a variety of ways [4]. For example, an activity can be classified according to the pattern of collaboration:divergent activities move from fewer to more concepts;convergent activities focus attention from many to fewer concepts; organising activities increase understanding of relationships between concepts;evaluating activities assess concepts relative to some criteria.An activity can also be classified according to its outcome: whether the product is an unstructured collection of concepts;an overview;a structure such as a list,tree or directed graph; and whether the output has been “judged” and/or “cleaned” [4]. These and other tools from collaboration engineering are used in section3to analyse traditional assumptions about collaborative ontology engineering, and to begin a detailed analysis of collaborative activities involved in the construction of a shared conceptualisation.Section 4 discusses a selection of relevant work, and section 5 presents conclusions and further work.2 Value Grid Case StudyThis section describes a case study, illustrating a possible value grid for semantic technologies to improve the management of experimental data from the ISIS facility [5]. Currently, metadata describing experimental outputs is managed via a metadata catalogue in which one or more uncontrolled keywords may be associated with each experiment.However,many of the keywords are synonyms,and the keywords constitute a local dialect that can be hard to penetrate for users of other, similar facilities [6] – therefore current retrieval services are limited. This case study was the subject of a recent project,exploring the feasibility of using a formal ontology for enhanced retrieval services [6]. An ontology was developed to replace the keyword indexing system, in collaboration withISIS scientists. However, a number of difficulties were encountered, not least the lack of consensus on a best-practice methodology for ontology engineering,and the difficulty of facilitating collaboration between ontology engineers and domain experts – in part due to the inability of domain experts to comprehend and use ontology engineering tools [6].What products, other than an ontology, might be exploited to improve retrieval services for ISIS experimental data,and how might these be developed and deployed as part of an incremental strategy?If an incremental strategy is followed,all possible value will be extracted from currently held assets, before any investment of effort is made in the development of further products. An uncontrolled keyword vocabulary is in itself an important product in the value grid. To maximise the value being obtained from this asset,the keyword index could be exploited to provide a number of additional services,both to users searching for experimental data (searchers) and to users entering a description of their own experiments (submitters).For the searcher,the process of entering and refining/modifying search queries could be supported in several ways.Query hints and suggestions could be provided, which could be either passive or active.A passive example would be to present a visualisation of the keyword index to the searcher(e.g.as a“tag cloud”). An active example would be to perform sub-string matching on queries as they are being typed, and suggest completions from the current keyword vocabulary. The keyword index could also be used as the subject of a statistical analysis to crudely identify clusters of related experiments.Clusters could be used to group results within large result sets, and to provide “see also” links between individual results.For the submitter,an“auto-completion” suggestion feature could be provided when entering keywords.Keywords could also be suggested prior to entry,based on a direct analysis of the text of the experiment title and abstract,or perhaps based on a more sophisticated statistical comparison of the title and abstract with other titles and abstracts already indexed with keywords.These additional services might also lead to a marginal increase in the quality of the keyword index, by providing paths for feedback between keyword entry and keyword use. If users are able to perceive the impact of their keyword assignments and those of their peers,and can adapt their usage depending on the behaviour of others in the community, this could, at least in principle, lead to communities and patterns of use emerging – this is the theoretical basis for “social tagging”.A second product in the value grid might be a set of synonym(equivalence)links between currently used keywords. Synonym links could be immediately exploited in a number of ways. For the searcher,synonyms could be used to provide suggestions for alternative queries. Synonyms could also be used“behind the scenes”to expand queries,increasing recall without demanding any additional action from the searcher. Synonyms could also improve the performance of natural language processing techniques, because they remove the necessity to perform co-reference resolution [7]. This in turn could improve the analysis of available text in titles and abstracts, leading to better suggestions for keyword entry and better clustering of related experiments.If a preference is expressed for one keyword in each synonym set,then a primitive controlled vocabulary is produced. This preference could be indicated to the submitter during keyword entry, which could influence convergence in keyword usage,without restricting the freedom of the keyword system. Note that vocabulary control is also a prerequisite for most products in the value grid involving formalisation of syntax and/or semantics.Once keyword synonym sets have been identified, various products can be created which organise these sets in different ways.One such product involves a high level categorisation,such that all synonym sets are placed into one of a small number of categories (a.k.a. “facets”). Casely-Hayford & Sufi found several high-level categories,including the experimental instrument,the subject of the investigation, the investigating body/group and the year of the experiment [6]. By producing a high-level categorisation of all synonym sets, various options immediately become available for provision of services to both searcher and submitter.For the searcher,“faceted” search/browse interfaces can be constructed, allowing users to build composite queries involving multiple categories, such as searching for experiments on a particular instrument by a particular group in a particular year.For the submitter,the submission form could be structured, and a smaller number of suggestions could be given for keyword values specific toeach category.Another,complementary,option would be to organise synonym sets into hierarchies(trees) and/or to find associative links between sets. Hierarchies can be exploited in a number of ways.For example,suggestions could be provided to the searcher for making their current query either more general or more specific. Hierarchical relationships could also be used behind the scenes to expand keyword queries, further improving recall. Another possibility is to offer hierarchies as a means of browsing a set of results. Associative links could be exploited to provide additional “see also” links for browsing result sets.Another value-adding activity would be to annotate synonym sets with small amounts of explanatory text. An annotated vocabulary could be exploited in a non-intrusive way to assist both searcher and submitter, e.g. by using annotations as the content of“tool tips”associated with keywords displayed in user interfaces.In sum,there are many ways in which value could be added to the current keyword system, without going as far as the development of a formal ontology. There are also ways of adding value,without enforcing strict vocabulary control. A range of products can be identified, including an uncontrolled keyword vocabulary; synonym sets; primitive controlled vocabulary; categorised(faceted)vocabulary;structured vocabulary (primitive thesaurus); and annotated vocabulary.Each of these products could be exploited to provide new features. Each of these products also, to a certain extent, could provide input(“raw materials”)to the development of other products, including those at higher levels of formalisation.A viable incremental strategy might be to develop and exploit products in the order introduced above.3 CollaborationAll semantic technologies work from the assumption that a shared conceptualisation is captured in some sort of specification,from which various useful and unique functionalities are then derived.Under this assumption,an information system will only be useful to those people who actually share the conceptualisation which is deployed therein. Of course, a single person could be employed to attempt to capture, integrate and articulate a conceptualisation held by others.However,it is generally assumed necessary for knowledgeable members of the application domain(“domain experts”)to be involved in the conceptualisation process, so that their views may be represented directly.To achieve some assurance of “sharedness”, more than one person must be involved, and therefore the conceptualisation process demands collaboration. Effective collaboration is a critical factor in the successful application of any semantic technology.This section examines the nature of collaboration in the application of semantic technologies, beginning with assumptions about collaboration in ontology engineering.Collaborative ontology engineering Methodologies for ontology engineering typically assume that participants in the process play one of two roles: either ontology engineer or domain expert(see e.g.[8]).The conceptualisation which is shared by the domain experts is to be captured in and expressed by the ontology. The ontology engineer is responsible for implementation of the ontology in an ontology language. Beyond these two statements, little is said about how the domain expert and the ontology engineer should interact during the conceptualisation process, or indeed what their specific goals are.Is an ontology engineer supposed to translate informal statements made by the domain experts into formal statements in terms of an ontology language? Is the ontology engineer supposed to educate the domain experts in ontology modelling, and encourage them to structure their thoughts in terms of classes, properties, individuals, axioms etc.? Should the ontology engineer broker agreements between domain experts in an attempt to reach consensus? Is it realistic to expect domain experts to carry out discussions using a formal ontology language?Three distinct collaborative relationships can be identified:relationships between domain experts; relationships between ontology engineers;and relationships between domain experts and ontology engineers.What are the natures and goals of these different collaborations? How may they be facilitated and supported by software tools?Many, if not all, of the currently available tools intended to support“collaborative”ontology development are unclear as to who they are intended to support collaboration between (although cf. [9]). Some projects have found that domain experts lack both the experience and the willingness to engage with ontology development tools.This means that,if a commitment is made to formal ontologyengineering, other tools have to be built in order to enable communication between ontology engineers and domain experts(e.g.the “OntoMaintainer” [6]). What of tools to enable direct collaboration between domain experts? Without these, how are domain experts supposed to “share” their conceptualisation?As implied in the previous section, some value chains – paths through the value grid – might employ logical formalism only during the later stages of production, if at all. Activities such as finding synonym links between keywords, organising synonym sets into categories, hierarchies and networks, may be quite intuitive, and certainly won't require any experience of ontology engineering.Who,then,should be involved during different stages of the conceptualisation process? What is their role, and what skills do they require? Conceptualisation processesThe study of collaboration must be the first consideration in the design of new software tools to support the conceptualisation process;tools specifically designed to support direct facilitated collaboration between domain experts.It is beyond the scope of the current paper to undertake such a study in any depth. However, below families of collaborative activities are sketched in outline; activities which could form the basis for the design of predictable, repeatable processes for the construction of a shared conceptualisation. The aim is to suggest ways of breaking the conceptualisation process down into a set of composable activities – building blocks which could be arranged into collaborative processes ad hoc in order to construct different products and traverse different paths in the semantic technology value grid. Possibilities for supporting these activities with “computer aid” are also discussed.In the case study given above, an uncontrolled keyword vocabulary was already present. However, many projects will have to start from scratch. Therefore, a first family of collaborative activities are those directed towards the collection of“raw materials”for the conceptualisation process–objects which convey informal expressions of meaning, without any context or structure,such as keywords, fragments of text, images, audio or video clips etc. All activities in this family are divergent – the aim is to obtain a comprehensive collection of everything that might be relevant.One concrete example of an activity in this family is“word association”– members of a group are asked to propose words or phrases in association with prompted suggestions.The group could continuously prompt each other, or could be prompted from a number of predefined starting points.A second concrete example is “literature scanning”,where individuals read documents and extract important words or phrases [10]. Both of these activities benefit from rich interaction between participants, and may be “computer aided” e.g. via statistical analyses of textual material.Another,quite different, concrete example of an activity in this family is “social tagging”, where individuals use their own keywords to describe objects of interest, generating an “folksonomy”.In the ISIS case study, the first proposed activity was the establishment of synonym links between keywords. We can generalise this to identify a family of activities, whose goal is to organise objects collected as“raw materials”into “synonym sets”,where each set of objects provides at least a partial indication or perception of a distinct “concept” to one or more persons in the collaboration(although “synonym” is used loosely here,especially if“synonyms”can include multimedia objects).Note that this activity is also divergent – the goal is to find all reasonable sets for all members of the collaboration,so that all views are initially represented.Variant concrete activities within this family might involve people working on an individual “work space”, seeing other colleagues' sets only when made,or might involve all members of the collaboration working simultaneously in a shared work space, seeing and influencing each others' actions in real time. Because the number of collected objects may initially be large, this second family of activities is also a candidate for “computer aid”. Synonyms might be suggested via background sources, such as general thesauri or word nets;or from mathematical analysis of usage graphs in social tagging networks [11].Another family of activities involves judging synonym sets – rating and voting on sets so that the “best” are kept and the “worst” are discarded. Sets are “better” if they provide a clear indication of a distinct “concept” that is recognised to some extent across the collaboration.This is a convergent activity,resulting in a“cleaner” collection [4]. However, activities in this family do not have to have absolute agreement – the aim could simply be to provide a candidate collection, deemed worthy of further evaluation and elaboration.Once candidate synonym sets have been judged,for those that remain it is likely that further modifications will need to be made.Two families of activities for adding/removing objects from synonym sets can be identified:those making proposed changes (divergent) and those judging/voting and choosing alternative propositions(convergent).Adding textual annotations – complete or partial definitions – to synonym sets could be identified as a separate activity, carried out before and/or after judging of synonym sets. Effort could be prioritised in a number of ways, e.g. by targeting those accepted candidates for which there was the least consensus during judging.Adding annotations might also be carried out as part of judging, where individuals add short annotations in order to clarify meaning and “improve” or “promote” a set.Several families of activities for organising synonym sets into higher-level structures can be identified,according to structures being produced.Structures include high-level grouping/categorisation;hierarchies(trees); association networks (graphs). Activities can also be characterised according to divergent or convergent aims;for example,some activities will collect proposals for structuring in different ways, whereas others will evaluate and choose between alternatives.Of course, the process will have to reach a point where the conceptualisation is deemed “complete”, i.e. sufficient to generate the desired product and achieve chosen quality criteria. This suggests various activities for the evaluation of a conceptualisation as a whole,and for collaborative decisions to“publish”(i.e.to release a new “edition”).4 DiscussionThesauri and other types of KOSThe study of “knowledge organisation systems” (KOS)is highly relevant to an exploration of products in the semantic technology value grid. There are many different types of KOS, including thesauri,taxonomies,classification schemes and subject heading systems (although the distinctions are sometimes blurred, see [12] and [13]). Nevertheless, KOS generally provide a controlled vocabulary,may provide synonym links,and may organise their conceptual units into hierarchies and/or networks of association. Ontologies are sometimes viewed as a type of KOS,although ontologies are fundamentally different due to their formal semantics.It is possible to use thesauri as input to a formal ontology engineering process (see e.g. [14] and [15]). However, a thesaurus or taxonomy is not necessarily an appropriate precursor to the development of a formal ontology. A thesaurus developed for one application (e.g. information retrieval) might be quite useless as input to the development of an ontology for another(e.g. database schema integration).Nevertheless, activities and techniques used in the development of thesauri, taxonomies etc.might be applicable to early stages in the development of a shared conceptualisation,depending on the ways in which products of the conceptualisation process are to be exploited.Aitchison et al.[10]divide the process of constructing a thesaurus into two major phases: term selection and finding structure. Techniques for the collection of terms include selection from existing terminological sources(other thesauri, classification schemes,glossaries etc.),and manual scanning and/or automatic analysis of relevant literature. Finding structure begins with a preliminary organisation of terms into a few broad subject categories (e.g. “catering”). Within each category, basic facets are then recognised and stated(e.g.“equipment”,“operations”). Within basic facets, terms are then arranged into hierarchies.Finally,scope notes,equivalence relationships,associative relationships and additional(poly-)hierarchical relationships are added. In the case study described in section 2, it was suggested that finding synonym links between keywords be done prior to finding other structures,because the synonym links can be immediately exploited for query expansion, natural language processing and other tasks. In contrast,Aitchison et al.find equivalence relationships after finding hierarchical and categorical structures.In 10 two roles are identified:“thesaurus compiler” and “subject specialist” (cf. “ontology engineer” and “domain expert”). Although it is recommended these two roles should interact during construction of a thesaurus, it is clear that most of the actual work of selecting terms and, in particular, finding structures, is to be done by the thesaurus compiler.Little,if any,attention is paid to different ways of structuring collaborations within and between subject specialists and thesaurus compilers, in order to produce successful,repeatable,outcomes.This suggests that collaboration engineering has much to offer the study of collaborative processes in the construction of both thesauri and formal ontologies.。