Basic Reproduction number
新冠传播指数计算方法

新冠传播指数计算方法
计算新冠传播指数的方法有很多种,其中比较常见和常用的
包括基本传播数(BasicReproductionNumber,简称R0)、有效传播数(EffectiveReproductionNumber,简称Reff)和疫情传播力量指数(EpidemicForceIndex,简称EFI)等。
1.基本传播数(R0):R0是指在没有免疫力和防控措施的
情况下,一个感染者平均能够传染给多少个人。
计算R0的方法一般基于传染病传播过程的数学模型,结合流行病学调查数据
和传播特性等因素。
R0大于1表示疫情有扩散趋势,小于1表示疫情呈现下降态势。
2.有效传播数(Reff):Reff是指在实施了控制措施后,目前感染者平均能够传染给多少个人。
Reff的计算方法会考虑到
人口免疫率、感染者的防控措施遵守情况等因素。
Reff大于1,表示疫情仍然在扩散,小于1表示疫情得到了有效控制。
3.疫情传播力量指数(EFI):EFI是一个综合的指标,它综
合考虑了感染率、传播速度、传播路径、传播距离等多个因素,用来评估疫情的传播力量和风险。
EFI的计算方法一般结合了流行病学调查数据、统计学方法和地理信息系统(GIS)等技术。
这些指标的计算方法涉及到很多复杂的数学模型和统计方法,需要综合考虑病毒特性、疾病传播途径、人群行为等多个因素。
在实际应用中,通常需要借助专业的疫情数据分析和统计软件
来计算和预测传播指数,以便更好地制定疫情防控措施和应对策略。
传染病问题数学公式
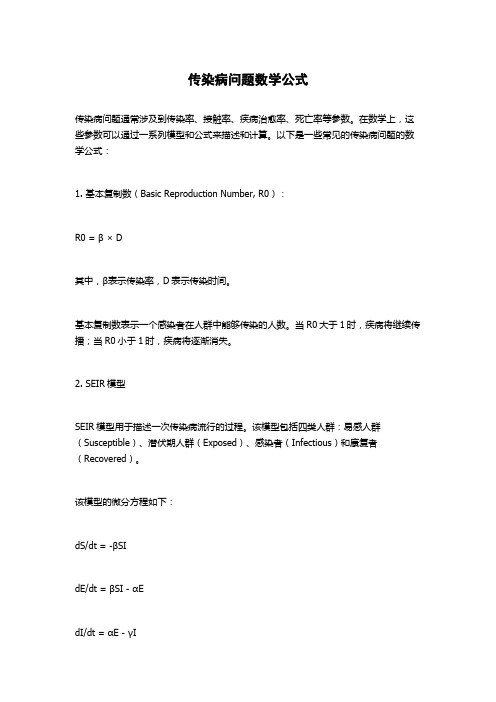
传染病问题数学公式
传染病问题通常涉及到传染率、接触率、疾病治愈率、死亡率等参数。
在数学上,这些参数可以通过一系列模型和公式来描述和计算。
以下是一些常见的传染病问题的数学公式:
1. 基本复制数(Basic Reproduction Number, R0):
R0 = β× D
其中,β表示传染率,D表示传染时间。
基本复制数表示一个感染者在人群中能够传染的人数。
当R0大于1时,疾病将继续传播;当R0小于1时,疾病将逐渐消失。
2. SEIR模型
SEIR模型用于描述一次传染病流行的过程。
该模型包括四类人群:易感人群(Susceptible)、潜伏期人群(Exposed)、感染者(Infectious)和康复者(Recovered)。
该模型的微分方程如下:
dS/dt = -βSI
dE/dt = βSI - αE
dI/dt = αE - γI
dR/dt = γI
其中,S、E、I、R分别表示四类人群的人数,β表示接触率,α表示潜伏期转化为感染期的概率,γ表示感染者恢复的概率。
3. SEIRD模型
SEIRD模型加入了死亡人群(Dead),用于描述一次传染病流行中的死亡情况。
该模型的微分方程如下:
dS/dt = -βSI
dE/dt = βSI - αE
dI/dt = αE - (γ+μ)I
dR/dt = γI
dD/dt = μI
其中,S、E、I、R、D分别表示五类人群的人数,β、α、γ、μ的含义与SEIR模型中相同,μ表示感染者死亡的概率。
什么是R0?
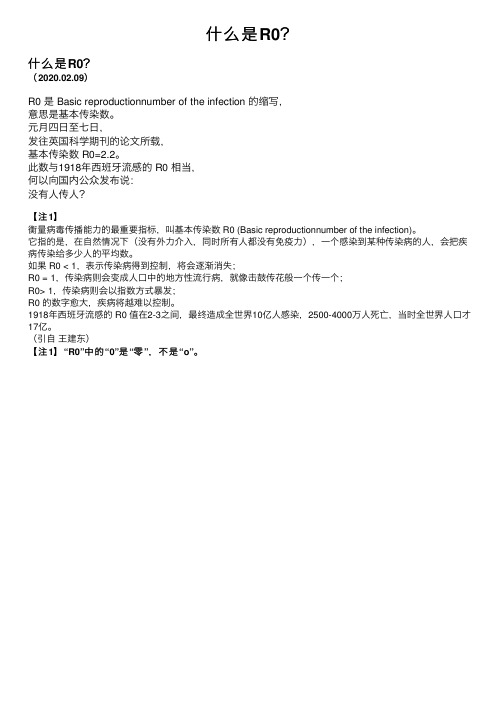
什么是R0?
什么是R0?
(2020.02.09)
R0 是 Basic reproductionnumber of the infection 的缩写,
意思是基本传染数。
元⽉四⽇⾄七⽇,
发往英国科学期刊的论⽂所载,
基本传染数 R0=2.2。
此数与1918年西班⽛流感的 R0 相当,
何以向国内公众发布说:
没有⼈传⼈?
【注1】
衡量病毒传播能⼒的最重要指标,叫基本传染数 R0 (Basic reproductionnumber of the infection)。
它指的是,在⾃然情况下(没有外⼒介⼊,同时所有⼈都没有免疫⼒),⼀个感染到某种传染病的⼈,会把疾病传染给多少⼈的平均数。
如果 R0 < 1,表⽰传染病得到控制,将会逐渐消失;
R0 = 1,传染病则会变成⼈⼝中的地⽅性流⾏病,就像击⿎传花般⼀个传⼀个;
R0> 1,传染病则会以指数⽅式暴发;
R0 的数字愈⼤,疾病将越难以控制。
1918年西班⽛流感的 R0 值在2-3之间,最终造成全世界10亿⼈感染,2500-4000万⼈死亡,当时全世界⼈⼝才17亿。
(引⾃王建东)
【注1】“R0”中的“0”是“零”,不是“o”。
流行病学专业英语词汇

流行病学专业英语词汇A安慰剂Placebo安慰剂效应placebo effectsB把握度study power半试验semi-trial保护率protective rate ,PR报告偏倚reporting bias暴露exposure暴露标志exposure marker暴露怀疑偏倚exposure suspicion bias爆发调查outbreak survey被动监测passive surveillance比例研究proportional study比值比Odds Ratio ,OR必需病因necessary factor of cause标点地图spot map标化比例死亡比standardized proportional mortality ratio,SPMR 标化比值比standardized odds ratio,SOR标化死亡比standardized mortality ratio,SMR标准化standardization标准化率比standardized rate ratio,SRR"冰山"现象ice-berg phenomenon丙型肝炎hepatitis C,HC并联试验parallel test病毒性肝炎viral hepatitis病例报告case report病例-病例研究case-case study病例调查case survey病例对照研究case-control study病例交叉设计case-crossover design病例交叉研究case-crossover study病例-系列研究case-series study病死率fatality rate病因causation of disease病因的异质性etiological heterogeneity病因分值etiologic fraction,EF病因链chains of causation病因网web of causation不合格ineligibility不依从noncomplianceC测量偏倚measurement bias长期变异secular change,trend variation超额死亡率excess mortality超额危险度excess risk巢式病例对照研究nested case-control study成本效果分析cost -effectiveness analysis成本效益分析cost-benefit analysis成本效用分析cost-utility成组匹配category matching抽样调查sampling survey抽样框架sampling frame抽样误差sampling error出生队列birth cohort出生队列分析法birth cohort analysis初级卫生保健primary health care ,PHC传播概率transmission probability传播机制mechanism of transmission传播途径path of transmission传播途径transmission route传播因素transmitting factor传染病communicable diseases传染过程infectious process传染性infectiousness串联试验serial test粗死亡率crude death rate改成crude mortality rate 较好粗一致性crude agreement促成病因contributory factor of cause错分偏倚misclassification bias错误分类misclassificationD大骨节病Kashin-Beck disease大流行pandemic代表性representativeness单倍型haplotype单纯随机抽样simple random sampling单盲single blind单项筛检single screening等位基因allele等位基因频率gene frequency地病endemic diseases地病学Endemiology地性endemicity地性氟中毒endemic fluorosis地性砷中毒endemic arsenism点突变point mutation点源流行point source epidemic碘缺乏病iodine deficiency disease调查随访研究survey follow-up study调整一致性adjusted agreement丁型肝炎hepatitis D,HD动态人群dynamic population毒力virulence短期波动irregular variation,rapid fluctuation队列cohort队列研究cohort study对象报告偏倚report bias多归因程序的法Multiple imputation procedure多国心血管病趋势和决定因素监测Multinational Monitoring of Trends and Determinants inardiovascular Diseases,MONICA多级抽样multistage sampling多群组比较研究multi-group comparison study多态性polymorphism多项筛检multiple screeningE恶性肿瘤流行病学cancer Epidemiology二代侵袭率,续发率secondary attack rate,SAR二级预防secondary preventionF发病率incidence rate,morbidity发病密度incidence density发病密度incidence density发生率研究incidence study飞沫droplet飞沫核droplet nuclei非即时性non-concurrent非均衡性错分differential misclassification非连续性状Discrete traits肺炎与流感死亡率pneumonia and influenza deaths,即P&I deaths分层stratification分层抽样stratified sampling分层随机分组stratified randomization分类变量categorical variable分离分析segregation analysis分子流行病学Molecular Epidemiology封闭性抗体blocking antibodies氟斑牙dental fluorosis氟骨症skeletal fluorosis负混杂negative confounding复等位基因位点multiple alles复杂性状complex traitG概念框架conceptual framework干预随访研究intervention follow-up study感染储主reservoir of infection感染来源source of infection感染力infectivity感染谱spectrum of infection感染梯度gradient of infection感染性腹泻infectious diarrhea感染性腹泻病infectious diarrheal disease感染症infections or infectious diseases高危人群策略high risk strategy个案case个案调查case study个案调查individual survey个体匹配individual matching工程干预engineering intervention共变法method of concomitant variation共显性codominance构成比proportion固定队列fixed cohort故意伤害intentional injuries关联的合理性plausibility of association关联的可重复性consistency of association关联的强度strength of association关联的时间性temporality of association关联的特异性specificity of association关联的一致性coherence of association观察偏倚observational bias观察性研究observational study观察一致率observation agreement广义遗传度broad heritability归因危险度attributable risk归因危险度百分比attributable risk percent,ARP,或AR%国际病毒分类委员会International Committee on Taxonomy of Viruses,ICTV 国际冠心病预防工作组The International Task Force for Prevention of Coronary Heart Disease国际疾病分类第十版International Classification of Diseases,ICD-10结核病防治项目National Tuberculosis Program,NTP卫生统计中心the National Center for Health Statistics,NCHSH合作率cooperation rate核心家系Nuclear families横断面研究cross sectional analysis横断面研究cross-sectional study患病率prevalence rate回顾性研究retrospective studies回忆偏倚recall bias混合型流行mixed epidemic混合研究mixed study混杂confounding混杂偏倚confounding bias混杂因素confounder,confounding factor活跃病区active endemic area获得性免疫缺陷综合症acquired immunodeficiency syndrome,AIDS 霍桑效应Howthorne effectJ机遇chance基本繁殖率basic reproduction rate基本繁殖数basic reproductive number,R0基本人群base population or population at risk基线信息baseline information基因gene基因毒性genotoxic基因型Genotyping基因型频率genotype frequency及时性timeliness疾病爆发disease outbreak疾病标志Markers of disease疾病的分布distribution of disease疾病的自然史natural history of disease疾病监测surveillance of disease疾病链the disease chain剂量反应关系dose-response relationship季节性seasonal variation,seasonality继发关联secondary association家庭簇研究family cluster study家庭聚集性Familial aggregation家庭聚集性研究familial aggregation study家庭相似性Family resemblance家系研究pedigree study甲型肝炎hepatitis A,HA假阳性率false positive rate假阴性率false negative rate间接传播indirect transmission间接关联indirect association间接接触indirect contact检出症侯偏倚detection signal bias简单随机分组simple randomization简明损伤定级法abbreviated injury scale,AIS健康促进health promotion健康工作者效应healthy worker effect健康监测系统health information system,HIS健康生命损失年years of life lived with disability,YLLD 教育干预educational intervention结局outcome结局变量outcome variable截断点cutoff金标准gold standard经济干预economic intervention经节肢动物媒介vector-borne经空气air-borne经食物food-borne经饮水drinking water-borne经载体vehicle-borne精确性precision聚合酶链式反应polymerase chain reaction,PCR均衡性错分non-differential misclassification均数回归趋势regression to the meanK抗原漂移antigenic drift抗原转变antigenic shift克山病Keshan disease空间、时间集研究space-time cluster study扩大免疫计划expanded program on immunization,EPI L来源同一identity by descent,IBD类试验,准试验quasi-trial,quasi-experiment类推法method of analogy累积发病率cumulative incidence罹患率attack rate礼貌偏倚politeness bias理论流行病学theoretical epidemiology历史性historical连锁linkage连锁不平衡linkage disequilibrium连锁分析Linkage analysis连锁平衡linkage equilibrium联合无应答偏倚combined nonrespondent bias联系率contact rate临床试验clinical trial灵敏度sensitivity领先时间偏倚lead time bias流感病毒influenza virus流行epidemic流行病模型epidemic model流行病学epidemiology流行病学监测epidemiological surveillance流行病学实验epidemiological experiment流行过程epidemic process流行曲线epidemic curve流行性出血热epidemic hemorrhagic fever,EHF流行性感冒Influenza流行性肾病nephropathia epidemica,NE率rate率比rate ratio率差rate difference,RD轮状模式wheel modeM蔓延流行propagated or progressive epidemic盲法blindness美国胆固醇教育规划National Cholesterol Education Program,NCEP 描述流行病学descriptive epidemiology描述性研究descriptive study目标人群target populationN暴露剂量Internal Dose部有效性internal validity剂量internal dose剂量的测定internal dose meters纳入/排除偏倚inclusion/exclusion bias奈曼偏倚Neyman biasP排除exclusions排除法method of exclusion排除偏倚exclusive bias配对pair matching"皮鞋"流行病学shoeleather epidemiology匹配matching匹配变量matching variable匹配过头overmatching匹配因素matching factors偏倚bias频数匹配frequency matching普查censusQ前瞻性prospective 8前瞻性研究prospective study潜伏期incubation period潜隐期latent period潜在工作损失年数Working Years of Potential Life Lost,WYPLL潜在价值损失年数Valued Years of Potential Life Lost,VYPLL潜在寿命损失年potential years of life lost,PYLL强制干预enforcement intervention求同法method of agreement求异法method of difference全人群策略population strategy缺课天数days lost from school缺勤天数days lost from work缺失数据missing data确定性检验explicit testing确定性模型deterministic modelR人传人person to person spread人类传染病anthroponosis人类基因组计划human genome project人类免疫缺陷病毒human immunodeficiency virus,HIV人群population人群病因分值population etiologic fraction,PEF人群的分层population stratification人群归因危险度population attributable risk,PAR人群归因危险度百分比population attributable risk percent,PARP 或PAR% 人群免疫性herd immunity人群易感性herd susceptibility人兽共患病,动物传染病zoonosis人畜共患病anthropozoonosis日期型date type入院率偏倚(伯克森偏倚)admission rate bias (Berkson's bias)。
一个传染病模型中的后向分支问题

一个传染病模型中的后向分支问题白婵;万辉【摘要】为了研究在考虑免疫接种情况下有限的医疗资源对疾病传播的影响,我们建立了一个带有特殊恢复率的SIVS传染病模型,研究了模型的基本动力学性质并对后向分支进行了详细的证明.结果表明,有限的医疗资源会导致重要的动力学性质,比如双稳现象等.后向分支意味着,即使基本再生数小于1模型依然可能会有稳定的地方病平衡点,基本再生数不能完全反映疾病流行与否.此时,人们应该注意疾病爆发时的初始状态.研究结果同时表明,充足的医疗资源和服务对于疾病的消除与控制非常重要.另外,文章也分析了免疫接种的影响.%In this paper,we formulate a SIVS epidemic model with special recovery rate to study the impact of limited medical resource on the transmission dynamics of diseases with vaccination.The basic investigation of the model has been finished. The backward bifurcation has been proved precisely. It is shown that limited medical resource leads to vital dynamics,such as bistability. Backward bifurcation implies that even if the basic reproduction number is smaller than unity,there may be a stable endemic equilibrium and the basic reproductive number itself is not enough to describe whether a disease will prevail or not and we should pay more attention to the initial conditions. It is also shown that suffi-cient medical services and medicines are very important for the disease control and eradication. Besides,the impact of vaccination has been explored too.【期刊名称】《南京师大学报(自然科学版)》【年(卷),期】2017(040)003【总页数】9页(P5-12,20)【关键词】免疫接种;传染病模型;医疗资源;平衡点;稳定性;后向分支【作者】白婵;万辉【作者单位】江苏省大规模复杂系统数值模拟重点实验室,南京师范大学数学科学学院,江苏南京210023;江苏省大规模复杂系统数值模拟重点实验室,南京师范大学数学科学学院,江苏南京210023【正文语种】中文Recently,attention has been given to vaccination and treatment policies in terms of the different vaccine classes,efficacy,treatment resource and associated costs([1-12],etc.).In[2],Kribs-Zaleta et al. introduced a vaccination compartment with temporarily immune state and set up a SIV model with general incidence rate. Their analysis indicated that when the vaccine for all population is not totally effective,the basic reproduction number R0 is no longer a threshold for the spread of diseases and the model will exhibit multiple endemic states. In[9],Shan and Zhu took the per capita recovery rate as a function of the number of hospital beds. Their analysis indicated that the system could undergo backward bifurcation,saddle-node bifurcation,Hopf bifurcation and cusp type of Bogdanov-Takens bifurcation within different conditions. In[11],Xiao and Tang analyzed a SIV epidemic model with nonlinear incidence rate. The main result shows that the system undergoesforward bifurcation with hysteresis except for the backward bifurcation. Besides,Erika et al.([7])studied the dynamics of a SIR epidemic model with nonlinear incidence rate,vertical transmission vaccination for the newborns and the capacity of treatment,that takes into account the limitedness of the medical resources and the efficiency of the supply of available medical resources. Under some conditions,they proved that the existence of backward bifurcation,the stability and the direction of Hopf bifurcation. They also explored how the mechanism of backward bifurcation affects the control of the infectious disease.In order to consider the impact of limited medical resources and vaccination on the transmission dynamics of infection diseases more precisely,we formulate a SIVS epidemic model with human population demography and vaccinated individuals.The organizations of this paper are as follow. Firstly,we will introduce our SIVS model in section 2. In section 3,we will analyze the existence of equilibria. Stability of equilibria and backward bifurcation analysis will be given in Section 4. Some discussion will be given in Section 5.We classify the population in a given region/area into three categories:susceptible,infective and vaccinated. Let S(t),I(t)and V(t)denote the number of susceptible,infective,vaccinated individuals at timet,respectively. Based on standard SIS model with the incidence of mass action,we can construct a modelwith initial data S(0)≥0,I(0)≥0,V(0)≥0,S(0)+I(0)+V(0)≤Λ/d,where all parameters listed in Table 1 are positive.In classical epidemic models,the per capita recovery rate is assumed to be a constant. Nevertheless,in general,the recovery rate depends on the resources of the health system available to the public,particularly the capacity of the hospital settings and efficiency of the treatment. There are many factors determining the recovery rate. The significant factor is the number of the hospital beds and medicines are another significant factors which are essential for safe and effective prevention,diagnosis and treatment of illness. We take the per capita recovery rate as μ=μ0+(μ1-μ0) which is used in[9]. Therefore,the recovery rate in a unit time μI is a that can describe the impact of limited medical resource.It is not difficult to prove the following theorem:Theorem 1 With an initial value condition in(1),there is a unique solution,and the solution remains positive and bounded for any finite time t≥0.Therefore,Model(1)is mathematically well-defined and biologically reasonable.Summing up the system(1),then =Λ-dN. So N(t)tends to Λ/d as t increases to infinity. Therefore,we can reduce the size of the model by letting S=Λ/d-I-V. Now the model becomesLet the right-hand side of(2)to be zero. One can verify that themodel(2)has one disease free equilibrium at E0=. The local stability of E0 can be obtained through a straightforward calculation for the eigenvalues. It follows from([13])that for the compartmental models,the local stability of the disease-free equilibrium is governed by the reproduction number ofthe model. If we use the notation in([13]),then we haveThe infected compartment is I,hence a straightforward calculation gives andHence the reproduction number is given by ρ(FV-1),andRemark 1 According to a straightforward calculation,R0 is a monotone decreasing function with respect to the vaccination rate φ.Let the right hand side of(2)be zero,then the endemic equilibriumE(I,V)satisfiesand I must satisfy the following equation:whereC=-β2Λσb+aβ+d(μ0+d)(φ+θ+d),a=-Λ(d+θ+σφ)+db(θ+d+σφ+μ0σ+dσ)+σbd(μ1-μ0),D=bd(d+μ1)(d+θ+φ)(1-R0).Because it is complicated to discuss the root of function F(I),we will study the number of the root from the geometry.Let the right-hand side of(2)be zero,thenwhereOne can easily verify thatandTherefore,f(I)is a monotonous decreasing concave function whereas g(I)is a convex function on the interval[0,Λ/d]. Besides,which implies thatNow we can discuss the number of the equilibrium points in three cases.(1)If R0>1,then f(0)<g(0),and g(Λ/d)<0 always exists. Thus there is only onepositive intersection which gives one endemic equilibrium(See Fig.1(a)). (2)If R0=1,then f(0)=g(0),and g(Λ/d)<0 always exists. Thus f(I)and g(I)will intersect one point if and only if f0(0)<g0(0)(See Fig.1(b)).(3)If R0<1,then f(0)>g(0),and g(Λ/d)0 always exists. Thus f(I)and g(I)will intersect with each other at two points which gives two endemic equilibrium if and only if f′(0)<g′(0)and there exists such that <g();The two points coalesce with each other if f()=and f′()=g′()(See Fig.1(c)(d)).As a result,we have the following theorem:Theorem 2 For the system(2).(1)The disease-free equilibrium E0 always exists.(2)If R0>1,there exists only one endemic equilibrium E1(I2,V2).(3)If R0=1,there exists one endemic equilibrium if and only if g0(0)>f0(0). Otherwise,there is no endemic equilibrium.(4)If R0<1,there exist two endemic equilibrium E1(I1,V1)and E2(I2,V2)if and only if f′(0)<g′(0)and there exists such that f()<g(),the two equilibria will coalesce if f()=g()and f′()=g′().Fix parametersΛ=1,d=0.1,β=0.2,θ=0.1,σ=0.4,b=0.1,μ0=0.2,φ=0.5,R0=2,1,0.2,0.047 respectively,we will get the Fig.1,respectively.Firstly,we will discuss stability of the disease-free equilibrium.The Jacobian matrix of system(2)So we will getwe have the following theorem:Theorem 3 When R0<1,E0 is locally asymptotically stable;when R0>1,E0 isunstable;when R0=1,E0 is a saddle-node.Proof One can verify that system at E0 has an eigenvalue,-(φ+θ+d)<0. The other eigenvalue is(μ1+d)(R0-1)<0. The result from the fact that(μ1+d)(R0-1)<0 is equivalent to R0<1 and(μ1+d)(R0-1)>0 is equivalent to R0>1. Hence,E0 is locally asymptotically stable if R0<1 and E0 is unstable if R0>1. Besides,obviously E0 is a Lyapunov singularity when R0=1. A straightforward calculation gives that one eigenvalue of Jacobian matrix of system at E0 is zero when R0=1,the other is -(φ+θ+d). The transformation I′=I,V′=V+ brings E0 to the origin. Then the system in a neighborhood of the origin becomesSimplifying the system,we will getLinearizing the above syst em and still substituting I=I′,V=V′. By straightforward calculating,we getwhereLet the right-hand side of the second equation be zero. According to implicit function,one can obtain a function for V in terms of I. SupposeV(I)=a1I+a2I2+O(I3)with V(0)=0. S ubstituting V(I)into ψ(I,V),Comparing the coefficients of the same powers gives that a1=-. Thus,andClearly,m=2 which implies that E0 is half saddle node. LetThen,E0 is left saddle and right node if C<0 while E0 is right saddle and left node if C>0.In the following,we will discuss the stability of the endemic equilibrium E-(I-,V-).The Jacobian matrix of the model(2)at E(I-,V-)givesand the corresponding characteristic equation is given bywhereObviously,F′(I)and H(I)determine the eigenvalues of the ma trix J(E-). It is easy to know F′(I1)>0,F′(I2)<0. So E2(I,V)is a hyperbolic saddle and is always unstable,and E1(I,V)is a anti-saddle. Furthermore,if H(I)>0,E1(I,V)is locally asymptotically stable;If H(I)=0,E2(I,V)is a weak focus or center;IfH(I)<0,E1(I,V)is a unstable node or focus. Thus we have the following theorem.Theorem 4 When R0>1,the system exists unique endemic equilibrium and it’s an anti-saddle;when R0<1,and the two endemic equilibriaexist,E1(I,V)is always unstable,E2(I,V)is locally asymptotically stable if H(I)>0 and unstable if H(I)<0.Lemma 1 (Theorem 3 in[14])AssumeA1:A=Dxf(0,0)=is the linearization matrix of system around the equilibrium 0 with φ evaluated at 0. Zero is a simple eigenvalue of A and all other eigenvalues of A have negative real parts;A2:Matrix A has a nonnegative right eigenvector w and a left eigenvector v corresponding to the zero eigenvalue.Let fk be the kth component of f andThe local dynamics of system around 0 are totally determined by a and b.(1)a>0,b>0. When φ<0with |φ|≪1. 0 is locally asymptotically stable. and there exists a positive unstable equilibrium;when 0<φ≪1,0 is unstable and there exists a negative and locally asymptotically stable equilibrium;(2)a<0,b<0. When φ<0 with |φ|≪1. 0 is unstable;When 0<φ≪1,0 is locally asymptotically stable,and there exists a positive unstable equilibrium. (3)a>0,b<0. When φ<0 with |φ|≪1. 0 is unstable;When 0<φ≪1,0 is stable,and there exists a locally asymptotically stable negative equilibrium,and a positive unstable equilibrium appears.(4)a<0,b>0. When φ<0 changes from negative to positive,0 changes its stability from stable to unstable. Correspondingly a negative unstable equilibrium becomes positive and locally asymptotically stable. Theorem 5 When R0=1,b<,the system will undergo backward bifurcation. Proof Let =-d. Then R0=1 if and only if μ1=.To apply Lemma 1,we essentially have to compute two quantities,labeled A and B,which depend on the higher order terms in the Taylor expansion of system,and require,for their computation,a change of coordinates involving the right and left eigenvectors of the Jacobian J(E0)associated with the eigenvalue λ=0. we will express A and B,in terms of parameters. The right and left eigenvectors of the Jacobian J(E0)arerespectively. In order to follow the notations introduced in Theorem 3of[18],we let x1=I,x2=V and φ=-μ1. Then the Taylor expansion system are represented by the fi(x,φ),(i=1,2),and we haveAll other derivatives equal to zero. Consequently,we can readily compute the following q uantity A:=∑ukwiwj=+2(-β+σβ)=2(-β+σβ)(f′(0)-g′(0)). Note that =1-R0+ and all other derivative equal to zero. so we can calculate B by substituting the vector v and w and the respective partial derivatives into the expression β= ∑ukwi=1-R0+.So we conclude that when R0=1 and f 0(0)<g0(0),A>0 and B>0,which is the defining condition for a backward bifurcation[14].In this paper,we formulate a SIVS epidemic model with special recovery rate to study the impact of limited medical resource on the transmission dynamics of diseases with vaccination.In Model(2),the disease-free equilibrium always exists which is locally asymptotically stable if R0<1 and unstable if R0>1. There is a unique endemic equilibrium if R0>1. Furthermore,on the one hand,if the medical resource in a given region is not sufficient enough,according to Theorem 5,backward bifurcation will occur and there are at most two endemic equilibria even if R0<1. One(E1)is a hyperbolic saddle and the other(E2)is a anti-saddle. Furthermore,E2 is a stable anti-saddle if H(I)>0. Backward bifurcation implies that the basic reproductive number itself is not enough to describe whether the disease will prevail or not and we should pay more attention to the initial value. On the other hand,if the medical resource is not less than the threshold value of b described in Theorem 5,R0 can act as a critical value and one just need to control R0 less than unity in order to control a disease. It is also shown that sufficient medical services and medicines are very important for the disease control and eradication. According to Remark 1,the basic reproduction number is monotone decreasing function with respect to the vaccination rate φ,which implies that vaccination may help to control a disease,especially if the medical resource is not sufficient. By vaccination,one can decrease the basic reproduction number to small enough value to guarantee there is noendemic equilibrium.[1] CUI J,MU X,WAN H. Saturation recovery leads to multiple endemic equilibria and backward bifurcation[J]. Journal of theoreticalbiology,2008,254:275-283.[2] KRIBS Z C,VELASCO H J. A simple vaccination model with multiple endemic states[J]. Math Biosci,2000,164:183-201.[3] LIU X,TAKEUCHI Y,IWAMI S. SVIR epidemic models with vaccination strategies[J]. Journal of theoretical biology,2008,253:1-11.[4] LI J,ZHAO Y,ZHU H. Bifurcation of an SIS model with nonlinear contact rate[J]. J Math Anal Appl,2015,432:1 119-1 138.[5] LI G,LI G F. Bifurcation analysis of an SIR epidemic model with the contact transmissions function[J]. Abstract and appliedanalysis,2014,Article ID 930541.[6] MAGPANTAY F M G,RIOLO M A,DOMENECH de CELLS M,et al. Epidemiological consequences of imperfect vaccines for immunizing Iinfection[J]. SIAM Journal on applied mathematics,2014,74:1 810-1 830.[7] ERIKA R,ERIC A,GERARDO G. Stability and bifurcation analysis of a SIR model with saturated incidence rate and saturated treatment[J]. Mathematics and computers in simulation,2016,121:109-132.[8] SHAN C,ZHU H. Bifurcations and complex dynamics of an SIR model with the impact of the number of hospital beds[J]. J differential equations,2014,257:1 662-1 688.[9] SHAN C,ZHU H. Nilpotent singularities and dynamics in an SIR type of compartmental model with hospital resources[J]. J differentialequations,2016,260:4 339-4 365.[10] WAN H,CUI J. Rich Dynamics of an epidemic model with saturation recovery[J]. Journal of applied mathematics,2013,Article ID 314958,9 pages.[11] XIAO Y,TANG S. Dynamics of infection with nonlinear incidence in a simple vaccination model[J]. Nonlinear analysis:real world applications,2010,11:4 154-4 163.[12] ZHOU T,ZHANG W,LU Q. Bifurcation analysis of an SIS epidemic model with saturated incidence rate and saturated treatment function[J]. J applied mathematics and computation,2014,226:288-305.[13] VAN DEN DRIESSCHE P,WATMOUGH J. Reproduction numbers and sub-threshold endemic equilibria for compartmental model of disease transmission[J]. J Math Biosci,2002,180:29-48.[14] CASTILLO C C,SONG B. Dynamical models of tuberculosis and their applications[J]. Mathematical biosciences and enginieering,2004(1):361-404.【相关文献】CLC number:175.12 Document codeA Article ID1001-4616(2017)03-0005-08Received data:2017-04-13.Foundation item:Supported by Jiangsu Overseas Research and Training Program for University Prominent Young & Middle-aged Tachers and Presidents;the NSF of the Jiangsu Higher Education Committee of China(15KJD110004);Project Founded by PAPD of Jiangsu Higher Education Institutions.Corresponding author:Wan Hui,associate professor,majored in mathematical biology. E-mail:****************doi:10.3969/j.issn.1001-4616.2017.03.002。
社团结构对一个SIR模型的基本再生数的影响

社团结构对一个SIR模型的基本再生数的影响基本再生数(Basic Reproduction Number,R0)是流行病学中一个经常用来衡量传染病传播能力的指标。
对于SIR模型来说,R0等于感染率(β)除以恢复率(γ)。
在传统的SIR模型中,人与人之间的接触是随机的,即任意一个易感者与患病者接触的概率是相等的。
但在现实生活中,人们的接触网络往往具有特定的结构,如社团结构。
社团结构指的是人们在某种联系下能够组成的小团体。
社团结构对SIR模型的传播动态和基本再生数有着深远的影响。
社团结构可以增加传播的速度。
在传统的SIR模型中,人与人之间的接触是随机的,患者与易感者的接触机会相等。
在社团结构中,患者与易感者之间的接触概率会增加。
因为社团结构中的人们更倾向于与同社团的人接触,从而增加了患者与易感者的接触机会,使得传播更为迅速。
这种增加传播速度的效应使得SIR模型中的基本再生数增大,从而增加了疫情的爆发风险。
社团结构可以增加疫情的规模。
在传统的SIR模型中,整个人群由一个大的网络所连接。
而在社团结构中,人们被分成了多个小的社团,社团内的人之间接触更频繁,而社团之间的接触相对较少。
这样的结构导致了疫情在社团内传播的机会更多,从而增加了疫情的规模。
SIR模型中的基本再生数在社团结构下更大,疫情的数量也更多。
社团结构还会影响疫情的传播路径。
在传统的SIR模型中,传播路径是随机的,任意一个易感者与患病者接触的概率是相等的。
但在社团结构中,易感者更容易接触到同社团的患者,从而顺着社团的内部连接路径传播。
这种传播路径的特点会导致疫情在社团内的传播更为迅速,同时也使得社团之间的传播较为缓慢。
在社团结构下,疫情的传播路径与传统的随机传播路径有所不同。
社团结构对一个SIR模型的基本再生数有着重要的影响。
社团结构不仅增加了传播速度和疫情规模,还对传播路径产生了一定的影响。
研究社团结构对传染病传播的影响,有助于制定更有效的防控措施,并提高对疫情的预测和应对能力。
R0是12说明
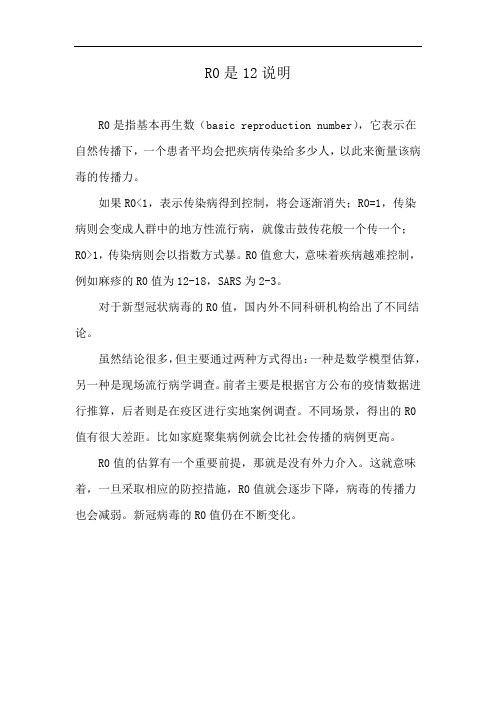
R0是12说明
R0是指基本再生数(basic reproduction number),它表示在自然传播下,一个患者平均会把疾病传染给多少人,以此来衡量该病毒的传播力。
如果R0<1,表示传染病得到控制,将会逐渐消失;R0=1,传染病则会变成人群中的地方性流行病,就像击鼓传花般一个传一个;
R0>1,传染病则会以指数方式暴。
R0值愈大,意味着疾病越难控制,例如麻疹的R0值为12-18,SARS为2-3。
对于新型冠状病毒的R0值,国内外不同科研机构给出了不同结论。
虽然结论很多,但主要通过两种方式得出:一种是数学模型估算,另一种是现场流行病学调查。
前者主要是根据官方公布的疫情数据进行推算,后者则是在疫区进行实地案例调查。
不同场景,得出的R0值有很大差距。
比如家庭聚集病例就会比社会传播的病例更高。
R0值的估算有一个重要前提,那就是没有外力介入。
这就意味着,一旦采取相应的防控措施,R0值就会逐步下降,病毒的传播力也会减弱。
新冠病毒的R0值仍在不断变化。
周期传染病模型的基本再生数

周期传染病模型的基本再生数∗白振国【摘要】本文是对周期传染病模型中基本再生数如何定义的一个简单综述。
基于常微分方程(ODE)、时滞微分方程(DDE)、偏微分方程(PDE)、差分方程和脉冲微分方程系统所描述的模型,我们利用积分算子谱半径的方法给出了这些不同类型模型的基本再生数的具体定义。
其结果有助于流行病学家们有效地预测具有季节性波动传染病的发展趋势。
%This paper is a brief survey on how to define the basic reproduction number in seasonally forced epidemiological models. Applying the method of the spectral radius of the integral operator, we present the definitions of the basic reproduction number in different types of models, which involve a variety of mathematical areas, such as ODE (ordinary differential equations), DDE (delay differential equations), PDE (partial differential equations), system of difference equations, system of impulsive differential equations. The results can help epi-demiologists effectively to predict the development trend of infectious diseases with seasonal fluctuation.【期刊名称】《工程数学学报》【年(卷),期】2013(000)002【总页数】9页(P175-183)【关键词】传染病模型;基本再生数;谱半径;季节性【作者】白振国【作者单位】西安电子科技大学理学院,西安 710071【正文语种】中文【中图分类】O175.1;O175.2;Q-3321 引言在传染病动力学的研究中,基本再生数(R0)是一个非常重要的概念.它表示在发病初期,当所有人均为易感者时,一个病人在其平均患病期内所传染的人数[1].通常,R0=1可作为决定疾病是否消亡的一个阈值,即:当R0<1时,疾病逐步消亡;而当R0>1时,疾病将始终存在而形成地方病.例如,对于如下一个经典的Kermack-McKendrick模型[2]其基本再生数的推导过程可借助于图1形象地描述.这表示:在一个所有人均为易感者的环境中,在t=0时刻引入的一个染病者到t时刻仍为病人的概率为e−γt,则在t时刻新感染的人数为βS0e−γt.因此就给出了一个染病者在其平均的患病期内所感染的人数,这与R0在医学中的解释也相符.然而,对于复杂的模型这样的推导并不总是成立.幸运的是,对于自治的传染病模型,Diekmann等,Driessche和Watmough引入了下一代方法(next generation method)来定义和计算R0[3,4].而对于非自治的人口和传染病模型,R0的定义和计算将更加复杂.图1: 感染的演变过程,其中(S0,0,0)为模型(1)的无病平衡点研究发现,对于一些具有周期参数的SIR或SIRS模型,决定疾病消亡与否的阈值与其参数平均化后的自治系统的基本再生数一致[5,6].然而,对于周期的SEIR或SEIRS模型,情况却并非如此.为了阐明这个缘由,我们考虑一个具有周期传染率的SEIR模型其中β(t)=β0[1+εcos(2πωt)],1/ω=365天.当β(t)=β0时,(2)的基本再生数为对于周期模型(2),这个平均的R0可解释为:在一个所有人均为易感者的环境中,如果疾病流行开始的时刻t满足cos(2πt/365)=0,那么在此时刻引入的一个病人在其患病期内所感染的平均人数.然而对于具有季节性波动的传染病模型,疾病的最终规模(the f i nal size)和传染病曲线的形状(如在一年中疾病的流行会出现多少次波峰等)强烈地依赖于一种病菌在人群中传染开始的时间t0,而自治的模型却不依赖于t0[7,8].因此,采用平均的R0并不能准确地判断出一种疾病是否会侵袭人类.事实上,对于周期的SEIR或SEIRS模型,一些研究已经表明平均意义下的R0有估高疾病发生的危险,见文献[6,9,10].那么,对于一般周期模型的R0,我们应该如何定义和计算它呢?许多年来,这是一个在人口和传染病动力学中非常难的问题.一些学者甚至认为:在周期环境中,基本再生数的概念可能已不再适用[11,12]或者即使能给出其定义但没有一般的数值方法来计算它[13].值得庆幸的是,这个问题终于在2006年得到解决.Baca¨er和Guernaoui在文献[14,15]中表明周期环境中的R0可定义为:在连续的T周期函数空间中一个线性积分算子的谱半径.也即,有一个唯一的实数R0,使得存在一个连续正的T周期向量值函数满足其中A(t,τ)为一n×n的非负连续矩阵函数且满足:对所有的τ,A(t,τ)=A(t+T,τ)及对所有的t,∫∞0A(t,τ)dτ有限.其中A(t,τ)中的每个元素Ai,j(t,τ)表示由一个在t−τ时刻类型为j的感染者在单位时刻t所感染的类型为i的人的数目.这个一般的定义适用于周期的ODE模型、DDE模型、PDE模型、脉冲微分方程模型等.特别地,对于ODE模型,王稳地和赵晓强[16]给出了一个更为具体的定义R0的方法.利用Floquet理论,他们也确立了R0的计算公式.这些先期的研究工作为我们研究具有季节性传染病的动力学行为提供了理论依据.2 “积分算子谱半径”方法的应用下面我们将针对几类不同的微分方程模型,给出这个方法具体应用的一些例子.2.1 ODE模型在文献[16]的例1中,王稳地和赵晓强考虑了一个具有两个菌株的周期的SIS模型其中S为易感者的数目,I1为菌株1的数目,I2为菌株2的数目.νI1I2表示菌株2可被菌株1再次感染,传染率β1(t)和β2(t)是两个连续非负的T周期函数.并假定模型(3)中的其它参数均为正.容易看出,模型(3)有一个无病稳定态x0(0,0,1).依据文献[16]中给出的定义R0的方法,我们有则模型(3)的基本再生数可定义为对于这个方法在其它ODE模型中的应用,我们可参见文献[16–19].2.2 差分方程模型考虑如下一个具有周期传染率的SIS模型其中β(t)=β(t+m)(m>2)为周期的接触率,µ>0为人口的出生率和死亡率,γ>0为感染者的恢复率.并假设0<µ+γ<1,0<µ+β(i)<1,i=0,1,···,m−1.系统(4)在无病平衡点E0(1,0)处线性化后染病者所对应的方程为其中F(t)=β(t),T(t)=1−(µ+γ).则依据文献[20],模型(4)的基本再生数可定义为其中r(A)表示矩阵A的谱半径.2.3 脉冲微分方程模型考虑一个具有脉冲接种和周期传染率的SIR模型模型(5)是文献[21]中所考虑模型的一种特殊形式.其中是饱和的发生率,µ为人口的出生率和死亡率,γ>0是感染者的康复率.T>0为对易感者进行两次脉冲接种的间隔时间,θk(06θk61)是在时刻t=kT对易感者的接种率.α(t)和β(t)为连续正的ω周期函数.并假定q=ω/T为一整数及θk=θk+q,k∈N.利用文献[21]中的引理2,系统(5)有一无病周期解(S∗(t),0,0),其中具有由文献[16]可得因此,模型(5)的基本再生数可定义为2.4 PDE和DDE模型Baca¨er在文献[22]中考虑了一个具有周期接触率和固定潜伏期的PDE模型其中E(t,x)是在t时刻具有染病年龄为x的潜伏者的数目,L表示固定的潜伏期,a为感染者的恢复率,c(t)=c0(1+εcos(wt))为周期的接触率.并假定总人口数为常数,则(6)可写为这是一个周期的时滞微分方程.在无病稳定态(N,0)线性化系统(7)可得染病者的方程令i∗(t)=c(t)I∗(t)为在t时刻处新感染的人数,则有其中则模型(7)的基本再生数可定义为积分算子在连续的2π/ω周期函数空间上的谱半径[14,15].尽管这样定义的R0没有显式表达式,但估计是可能的.Bacar基于文献[23]中的数值方法表明R0是下列具有连续分数方程的最大实根其中通过数值模拟,Baca¨er发现:1) 当ε=0时,R0不依赖于L;2) 当L=0或时,R0不依赖于ε;3) R0是关于L的2π/ω周期函数;4) 对于固定的L,R0是关于ε的增函数或减函数.此外,R0的估计表达式(9)也适用于方程(8)的积分核具有形式的一大类的ODE、DDE和PDE的传染病模型.上述例子表明,具有时滞的周期传染病模型的R0与季节性因素ε、周期2π/ω和潜伏期的长短L都有关系.这些参数的引入给数值估计R0带来了很大的困难.据作者所知,目前对于此类模型R0数值研究的文献非常少见,仅见文献[15].然而,时滞因素在许多具有周期波动发生率的媒介传播疾病中普遍存在,如疟疾、登革热、西尼罗河病毒等.因此,提出一些有效的数值估计此类模型R0的方法具有很大的应用价值,这将是我们今后进行研究的一个重要课题.3 在周期模型中R0的生物意义尽管周期模型中的R0可通过一个积分算子的谱半径来定义,但是它的生物意义并不明确.特别值得一提的是,这个R0的生物意义不能像自治的SIR或SEIR模型中一样解释为“在一个所有人均为易感者的环境中,由一个病人在其患病期内平均所感染的人数”.事实上,对于周期的传病模型,这是不合理的.例如考虑如下一个染病者所对应的方程其中a(t+T)=a(t),T>0,则一个在t0时刻引入的患者二次感染所产生的新的染病者的平均数目为称R0=/b为平均意义上的再生数实际上是指C(t0)在一个周期内的平均,即然而当b(t)也是一T周期函数时,利用文献[14,16]给出的方法可得R0=/.显然,在此情形下关于R0平均意义下的解释完全失效.Baca¨er在文献[24]中表明在周期模型中所定义的R0可解释为“渐近的每代增长率”.其它关于周期R0的研究工作,我们可参见文献[20,25–31].4 结论在本文,针对不同类型具有周期参数的微分方程模型,我们对它们所对应的基本再生数的定义方法给出了一个概括性的综述.这可为众多研究具有季节性波动传染病阈值动力学行为的学者提供一个重要的理论参考.参考文献:[1]Anderson R M,May R M.Infectious Diseases ofHumans[M].Oxford:Oxford University Press,1991[2]Kermack W O,McKendrick A G.A contribution to the mathematical theory of epidemics[J].Proceedings of the Royal Society of London Series A,1927,115(772):700-721[3]Diekmann O,Heesterbeek J A P,Metz J A J.On the def i nition and the computation of the basic reproduction ratio R0in models for infectious disease in heterogeneous populations[J].Journal of Mathematical Biology,1990,28(4):365-382[4]Driessche P van den,Watmough J.Reproduction numbers and sub-threshold endemic equilibria for compartmental models ofdisease[J].Mathematical Biosciences,2002,180(1-2):29-48[5]Thieme H R.Uniform persistence and permanence for nonautonomous semif l ows in population biology[J].Mathematical Biosciences,2000,166(2):173-201[6]Ma J L,Ma Z E.Epidemic threshold condition for seasonally forced SEIR models[J].Mathematical Biosciences and Engineering,2006,3(1):161-172 [7]Feng Z L,Towers S,Yang Y D.Modeling the ef f ects of vaccination and treatment on pandemic inf l uenza[J].The AAPS Journal,2011,13(3):427-437[8]Towers S,et al.Antiviral treatment for pandemic inf l uenza:assessing potential repercussions using a seasonally forced SIR model[J].Journal of Theoretical Biology,2011,289:259-268[9]Nakata Y,Kuniya T.Global dynamics of a class of SEIRS epidemic models in a periodic environment[J].Journal of Mathematical Analysis and Applications,2010,363(1):230-237[10]Bai Z G,Zhou Y C.Global dynamics of an SEIRS epidemic model with periodic vaccination and seasonal contact rate[J].Nonlinear Analysis:Real World Applications,2012,13(3):1060-1068[11]Heesterbeek J A P,Roberts M G.Threshold quantities for helminth infections[J].Journal of Mathematical Biology,1995,33(4):415-434 [12]Grassly N C,Fraser C.Seasonal infectious diseaseepidemiology[J].Proceedings of the Royal Society B,2006,273(1600):2541-2550[13]Wesley C L,Allen L J S.The basic reproduction number in epidemic models with periodic demographics[J].Journal of Biological Dynamics,2009,3(2-3):116-129[14]Baca¨er N,Guernaoui S.The epidemic threshold of vector-borne diseases with seasonality[J].Journal of Mathematical Biology,2006,53(3):421-436[15]Baca¨er N.Approximation of the basic reproduction number R0for vector-borne diseases with a periodic vector population[J].Bulletin of Mathematical Biology,2007,69(3):1067-1091[16]Wang W D,Zhao X Q.Threshold dynamics for compartmentalepidemic models in periodic environments[J].Journal of Dynamics and Dif f erential Equations,2008,20(3):699-717[17]Liu L J,Zhao X Q,Zhou Y C.A tuberculosis model withseasonality[J].Bulletin of Mathematical Biology,2010,72(4):931-952 [18]Bai Z G,Zhou Y C.Threshold dynamics of a bacillary dysentery model with seasonal f l uctuation[J].Discrete and Continuous Dynamical Systems-Series B,2011,15(1):1-14[19]Lou Y J,Zhao X Q.The periodic Ross-Macdonald model with dif f usion and advection[J].Applicable Analysis,2010,89(7):1067-1089[20]Baca¨er N.Periodic matrix population models:growth rate,basic reproduction number,and entropy[J].Bulletin of Mathematical Biology,2009,71(7):1781-1792[21]Gao S J,et al.Seasonality and mixed vaccination strategy in an epidemic model with vertical transmission[J].Mathematics and Computers in Simulation,2011,81(9):1855-1868[22]Baca¨er N,Ouifki R.Growth rate and basic reproduction number for population models with a simple periodic factor[J].Mathematical Biosciences,2007,210(2):647-658[23]Just W.On the eigenvalue spectrum for time-delayed Floquet problems[J].Physica D:Nonlinear Phenomena,2000,142(1-2):153-165 [24]Baca¨er N,Dads E A.Genealogy with seasonality,the basic reproduction number,and the inf l uenza pandemic[J].Journal of Mathematical Biology,2011,62(5):741-762[25]Baca¨er N,Gomes M G M.On the f i nal size of epidemics withseasonality[J].Bulletin of Mathematical Biology,2009,71(8):1954-1966 [26]Thieme H R.Spectral bound and reproduction number for inf i nite-dimensional population structure and time heterogeneity[J].SIAM Journal on Applied Mathematics,2009,70(1):188-211[27]Baca¨er N.The model of Kermack and McKendrick for the plague epidemic in Bombay and the type reproduction number with seasonality[J].Journal of Mathematical Biology,2012,64(3):403-422 [28]Cushing J M,Ackleh A S.A net reproductive number for periodic matrix models[J].Journal of Biological Dynamics,2012,6(2):166-188 [29]Allen L J S,Driessche P van den.The basic reproduction number in some discrete-time epidemic models[J].Journal of Dif f erence Equations and Applications,2008,14(10-11):1127-1147[30]Baca¨er N,Dads E A.On the biological interpretation of a def i nition for the parameter R0in periodic population models[J].Journal of Mathematical Biology,2012,65(4):601-621[31]Inaba H.On a new perspective of the basic reproduction number in heterogeneous environments[J].Journal of Mathematical Biology,2012,65(2):309-348。
传染病潜伏期的数学建模与预测
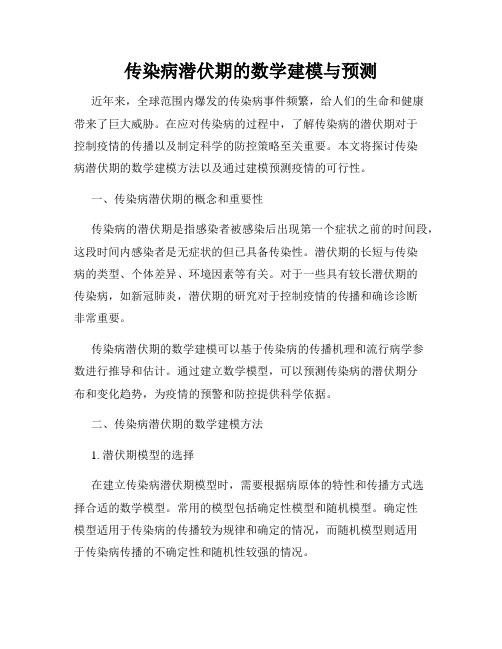
传染病潜伏期的数学建模与预测近年来,全球范围内爆发的传染病事件频繁,给人们的生命和健康带来了巨大威胁。
在应对传染病的过程中,了解传染病的潜伏期对于控制疫情的传播以及制定科学的防控策略至关重要。
本文将探讨传染病潜伏期的数学建模方法以及通过建模预测疫情的可行性。
一、传染病潜伏期的概念和重要性传染病的潜伏期是指感染者被感染后出现第一个症状之前的时间段,这段时间内感染者是无症状的但已具备传染性。
潜伏期的长短与传染病的类型、个体差异、环境因素等有关。
对于一些具有较长潜伏期的传染病,如新冠肺炎,潜伏期的研究对于控制疫情的传播和确诊诊断非常重要。
传染病潜伏期的数学建模可以基于传染病的传播机理和流行病学参数进行推导和估计。
通过建立数学模型,可以预测传染病的潜伏期分布和变化趋势,为疫情的预警和防控提供科学依据。
二、传染病潜伏期的数学建模方法1. 潜伏期模型的选择在建立传染病潜伏期模型时,需要根据病原体的特性和传播方式选择合适的数学模型。
常用的模型包括确定性模型和随机模型。
确定性模型适用于传染病的传播较为规律和确定的情况,而随机模型则适用于传染病传播的不确定性和随机性较强的情况。
2. 基本传播数的计算基本传播数(basic reproduction number,简称R0)是评估传染病传播强度的重要指标。
在传染病潜伏期模型中,可以通过计算R0来评估传染病的传播风险和潜在爆发的可能性。
3. 参数估计和模型验证传染病潜伏期模型的建立需要依靠传染病的实际数据进行参数估计和模型验证。
通过收集感染者的病程数据和接触者的流行病学调查数据,可以对潜伏期的分布和变化进行建模。
三、传染病潜伏期的预测和应用基于传染病潜伏期模型的建立和参数估计,可以进行传染病潜伏期的预测和应用。
具体包括以下几个方面:1. 疫情预警与监测通过传染病潜伏期模型,可以根据感染者的出现时间和疫情数据,实时监测和预测传染病的潜伏期分布和变化趋势。
这对于疫情的预警和监测提供重要依据,有助于及时采取控制措施并防止疫情扩散。
流行病学基本参数基本再生数
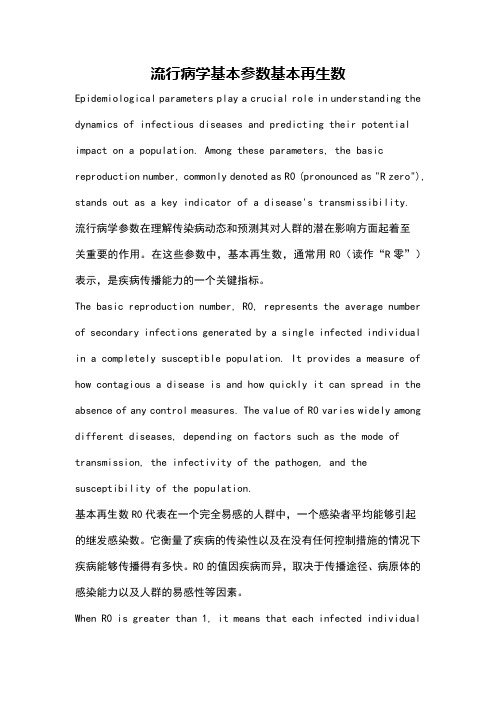
流行病学基本参数基本再生数Epidemiological parameters play a crucial role in understanding the dynamics of infectious diseases and predicting their potential impact on a population. Among these parameters, the basic reproduction number, commonly denoted as R0 (pronounced as "R zero"), stands out as a key indicator of a disease's transmissibility.流行病学参数在理解传染病动态和预测其对人群的潜在影响方面起着至关重要的作用。
在这些参数中,基本再生数,通常用R0(读作“R零”)表示,是疾病传播能力的一个关键指标。
The basic reproduction number, R0, represents the average number of secondary infections generated by a single infected individual in a completely susceptible population. It provides a measure of how contagious a disease is and how quickly it can spread in the absence of any control measures. The value of R0 varies widely among different diseases, depending on factors such as the mode of transmission, the infectivity of the pathogen, and the susceptibility of the population.基本再生数R0代表在一个完全易感的人群中,一个感染者平均能够引起的继发感染数。
基本再生数的计算公式
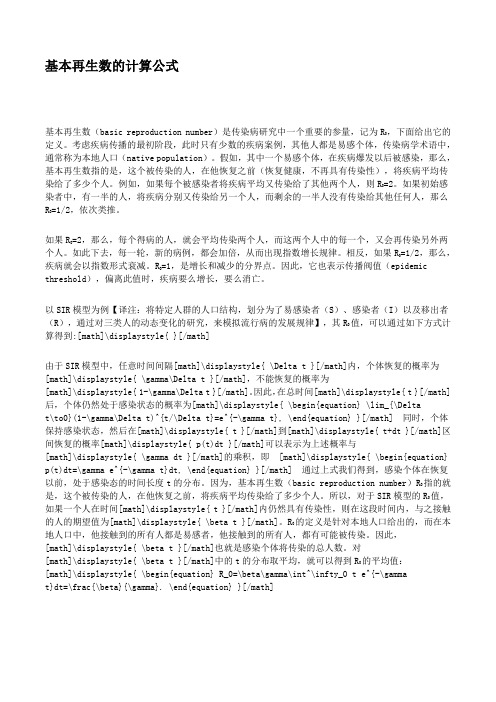
基本再生数的计算公式基本再生数(basic reproduction number)是传染病研究中一个重要的参量,记为R0,下面给出它的定义。
考虑疾病传播的最初阶段,此时只有少数的疾病案例,其他人都是易感个体,传染病学术语中,通常称为本地人口(native population)。
假如,其中一个易感个体,在疾病爆发以后被感染,那么,基本再生数指的是,这个被传染的人,在他恢复之前(恢复健康,不再具有传染性),将疾病平均传染给了多少个人。
例如,如果每个被感染者将疾病平均又传染给了其他两个人,则R0=2。
如果初始感染者中,有一半的人,将疾病分别又传染给另一个人,而剩余的一半人没有传染给其他任何人,那么R0=1/2,依次类推。
如果R0=2,那么,每个得病的人,就会平均传染两个人,而这两个人中的每一个,又会再传染另外两个人。
如此下去,每一轮,新的病例,都会加倍,从而出现指数增长规律。
相反,如果R0=1/2,那么,疾病就会以指数形式衰减。
R0=1,是增长和减少的分界点。
因此,它也表示传播阀值(epidemic threshold),偏离此值时,疾病要么增长,要么消亡。
以SIR模型为例【译注:将特定人群的人口结构,划分为了易感染者(S)、感染者(I)以及移出者(R),通过对三类人的动态变化的研究,来模拟流行病的发展规律】,其R0值,可以通过如下方式计算得到:[math]\displaystyle{ }[/math]由于SIR模型中,任意时间间隔[math]\displaystyle{ \Delta t }[/math]内,个体恢复的概率为[math]\displaystyle{ \gamma\Delta t }[/math],不能恢复的概率为[math]\displaystyle{ 1-\gamma\Delta t }[/math]。
因此,在总时间[math]\displaystyle{ t }[/math]后,个体仍然处于感染状态的概率为[math]\displaystyle{ \begin{equation} \lim_{\Deltat\to0}(1-\gamma\Delta t)^{t/\Delta t}=e^{-\gamma t}, \end{equation} }[/math] 同时,个体保持感染状态,然后在[math]\displaystyle{ t }[/math]到[math]\displaystyle{ t+dt }[/math]区间恢复的概率[math]\displaystyle{ p(t)dt }[/math]可以表示为上述概率与[math]\displaystyle{ \gamma dt }[/math]的乘积,即[math]\displaystyle{ \begin{equation} p(t)dt=\gamma e^{-\gamma t}dt, \end{equation} }[/math] 通过上式我们得到,感染个体在恢复以前,处于感染态的时间长度t的分布。
Reproduction numbers and sub-threshold endemic equilibria for compartmental models of disease trans
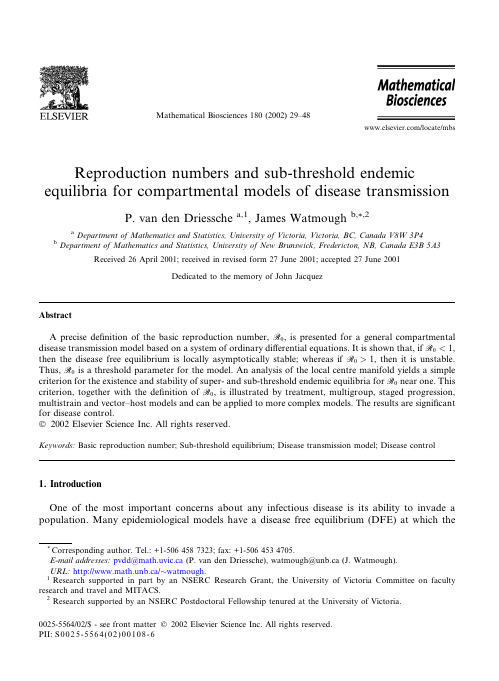
Reproduction numbers and sub-threshold endemicequilibria for compartmental models of disease transmissionP.van den Driesschea,1,James Watmough b,*,2aDepartment of Mathematics and Statistics,University of Victoria,Victoria,BC,Canada V8W 3P4b Department of Mathematics and Statistics,University of New Brunswick,Fredericton,NB,Canada E3B 5A3Received 26April 2001;received in revised form 27June 2001;accepted 27June 2001Dedicated to the memory of John JacquezAbstractA precise definition of the basic reproduction number,R 0,is presented for a general compartmental disease transmission model based on a system of ordinary differential equations.It is shown that,if R 0<1,then the disease free equilibrium is locally asymptotically stable;whereas if R 0>1,then it is unstable.Thus,R 0is a threshold parameter for the model.An analysis of the local centre manifold yields a simple criterion for the existence and stability of super-and sub-threshold endemic equilibria for R 0near one.This criterion,together with the definition of R 0,is illustrated by treatment,multigroup,staged progression,multistrain and vector–host models and can be applied to more complex models.The results are significant for disease control.Ó2002Elsevier Science Inc.All rights reserved.Keywords:Basic reproduction number;Sub-threshold equilibrium;Disease transmission model;Disease control1.IntroductionOne of the most important concerns about any infectious disease is its ability to invade a population.Many epidemiological models have a disease free equilibrium (DFE)at whichtheMathematical Biosciences 180(2002)29–48/locate/mbs*Corresponding author.Tel.:+1-5064587323;fax:+1-5064534705.E-mail addresses:pvdd@math.uvic.ca (P.van den Driessche),watmough@unb.ca (J.Watmough).URL:http://www.math.unb.ca/$watmough.1Research supported in part by an NSERC Research Grant,the University of Victoria Committee on faculty research and travel and MITACS.2Research supported by an NSERC Postdoctoral Fellowship tenured at the University of Victoria.0025-5564/02/$-see front matter Ó2002Elsevier Science Inc.All rights reserved.PII:S0025-5564(02)00108-630P.van den Driessche,J.Watmough/Mathematical Biosciences180(2002)29–48population remains in the absence of disease.These models usually have a threshold parameter, known as the basic reproduction number,R0,such that if R0<1,then the DFE is locally as-ymptotically stable,and the disease cannot invade the population,but if R0>1,then the DFE is unstable and invasion is always possible(see the survey paper by Hethcote[1]).Diekmann et al.[2]define R0as the spectral radius of the next generation matrix.We write down in detail a general compartmental disease transmission model suited to heterogeneous populations that can be modelled by a system of ordinary differential equations.We derive an expression for the next generation matrix for this model and examine the threshold R0¼1in detail.The model is suited to a heterogeneous population in which the vital and epidemiological parameters for an individual may depend on such factors as the stage of the disease,spatial position,age or behaviour.However,we assume that the population can be broken into homo-geneous subpopulations,or compartments,such that individuals in a given compartment are indistinguishable from one another.That is,the parameters may vary from compartment to compartment,but are identical for all individuals within a given compartment.We also assume that the parameters do not depend on the length of time an individual has spent in a compart-ment.The model is based on a system of ordinary equations describing the evolution of the number of individuals in each compartment.In addition to showing that R0is a threshold parameter for the local stability of the DFE, we apply centre manifold theory to determine the existence and stability of endemic equilib-ria near the threshold.We show that some models may have unstable endemic equilibria near the DFE for R0<1.This suggests that even though the DFE is locally stable,the disease may persist.The model is developed in Section2.The basic reproduction number is defined and shown to bea threshold parameter in Section3,and the definition is illustrated by several examples in Section4.The analysis of the centre manifold is presented in Section5.The epidemiological ramifications of the results are presented in Section6.2.A general compartmental epidemic model for a heterogeneous populationConsider a heterogeneous population whose individuals are distinguishable by age,behaviour, spatial position and/or stage of disease,but can be grouped into n homogeneous compartments.A general epidemic model for such a population is developed in this section.Let x¼ðx1;...;x nÞt, with each x i P0,be the number of individuals in each compartment.For clarity we sort the compartments so that thefirst m compartments correspond to infected individuals.The distinc-tion between infected and uninfected compartments must be determined from the epidemiological interpretation of the model and cannot be deduced from the structure of the equations alone,as we shall discuss below.It is plausible that more than one interpretation is possible for some models.A simple epidemic model illustrating this is given in Section4.1.The basic reproduction number can not be determined from the structure of the mathematical model alone,but depends on the definition of infected and uninfected compartments.We define X s to be the set of all disease free states.That isX s¼f x P0j x i¼0;i¼1;...;m g:In order to compute R0,it is important to distinguish new infections from all other changes inpopulation.Let F iðxÞbe the rate of appearance of new infections in compartment i,Vþi ðxÞbe therate of transfer of individuals into compartment i by all other means,and VÀi ðxÞbe the rate oftransfer of individuals out of compartment i.It is assumed that each function is continuously differentiable at least twice in each variable.The disease transmission model consists of non-negative initial conditions together with the following system of equations:_x i¼f iðxÞ¼F iðxÞÀV iðxÞ;i¼1;...;n;ð1Þwhere V i¼VÀi ÀVþiand the functions satisfy assumptions(A1)–(A5)described below.Sinceeach function represents a directed transfer of individuals,they are all non-negative.Thus,(A1)if x P0,then F i;Vþi ;VÀiP0for i¼1;...;n.If a compartment is empty,then there can be no transfer of individuals out of the compartment by death,infection,nor any other means.Thus,(A2)if x i¼0then VÀi ¼0.In particular,if x2X s then VÀi¼0for i¼1;...;m.Consider the disease transmission model given by(1)with f iðxÞ,i¼1;...;n,satisfying con-ditions(A1)and(A2).If x i¼0,then f iðxÞP0and hence,the non-negative cone(x i P0, i¼1;...;n)is forward invariant.By Theorems1.1.8and1.1.9of Wiggins[3,p.37]for each non-negative initial condition there is a unique,non-negative solution.The next condition arises from the simple fact that the incidence of infection for uninfected compartments is zero.(A3)F i¼0if i>m.To ensure that the disease free subspace is invariant,we assume that if the population is free of disease then the population will remain free of disease.That is,there is no(density independent) immigration of infectives.This condition is stated as follows:(A4)if x2X s then F iðxÞ¼0and VþiðxÞ¼0for i¼1;...;m.The remaining condition is based on the derivatives of f near a DFE.For our purposes,we define a DFE of(1)to be a(locally asymptotically)stable equilibrium solution of the disease free model,i.e.,(1)restricted to X s.Note that we need not assume that the model has a unique DFE. Consider a population near the DFE x0.If the population remains near the DFE(i.e.,if the introduction of a few infective individuals does not result in an epidemic)then the population will return to the DFE according to the linearized system_x¼Dfðx0ÞðxÀx0Þ;ð2Þwhere Dfðx0Þis the derivative½o f i=o x j evaluated at the DFE,x0(i.e.,the Jacobian matrix).Here, and in what follows,some derivatives are one sided,since x0is on the domain boundary.We restrict our attention to systems in which the DFE is stable in the absence of new infection.That is, (A5)If FðxÞis set to zero,then all eigenvalues of Dfðx0Þhave negative real parts.P.van den Driessche,J.Watmough/Mathematical Biosciences180(2002)29–4831The conditions listed above allow us to partition the matrix Df ðx 0Þas shown by the following lemma.Lemma 1.If x 0is a DFE of (1)and f i ðx Þsatisfies (A1)–(A5),then the derivatives D F ðx 0Þand D V ðx 0Þare partitioned asD F ðx 0Þ¼F 000 ;D V ðx 0Þ¼V 0J 3J 4;where F and V are the m Âm matrices defined byF ¼o F i o x j ðx 0Þ !and V ¼o V i o x jðx 0Þ !with 16i ;j 6m :Further ,F is non-negative ,V is a non-singular M-matrix and all eigenvalues of J 4have positive real part .Proof.Let x 02X s be a DFE.By (A3)and (A4),ðo F i =o x j Þðx 0Þ¼0if either i >m or j >m .Similarly,by (A2)and (A4),if x 2X s then V i ðx Þ¼0for i 6m .Hence,ðo V i =o x j Þðx 0Þ¼0for i 6m and j >m .This shows the stated partition and zero blocks.The non-negativity of F follows from (A1)and (A4).Let f e j g be the Euclidean basis vectors.That is,e j is the j th column of the n Ân identity matrix.Then,for j ¼1;...;m ,o V i o x jðx 0Þ¼lim h !0þV i ðx 0þhe j ÞÀV i ðx 0Þh :To show that V is a non-singular M-matrix,note that if x 0is a DFE,then by (A2)and (A4),V i ðx 0Þ¼0for i ¼1;...;m ,and if i ¼j ,then the i th component of x 0þhe j ¼0and V i ðx 0þhe j Þ60,by (A1)and (A2).Hence,o V i =o x j 0for i m and j ¼i and V has the Z sign pattern (see Appendix A).Additionally,by (A5),all eigenvalues of V have positive real parts.These two conditions imply that V is a non-singular M-matrix [4,p.135(G 20)].Condition (A5)also implies that the eigenvalues of J 4have positive real part.Ã3.The basic reproduction numberThe basic reproduction number,denoted R 0,is ‘the expected number of secondary cases produced,in a completely susceptible population,by a typical infective individual’[2];see also [5,p.17].If R 0<1,then on average an infected individual produces less than one new infected individual over the course of its infectious period,and the infection cannot grow.Conversely,if R 0>1,then each infected individual produces,on average,more than one new infection,and the disease can invade the population.For the case of a single infected compartment,R 0is simply the product of the infection rate and the mean duration of the infection.However,for more complicated models with several infected compartments this simple heuristic definition of R 0is32P.van den Driessche,J.Watmough /Mathematical Biosciences 180(2002)29–48insufficient.A more general basic reproduction number can be defined as the number of new infections produced by a typical infective individual in a population at a DFE.To determine the fate of a‘typical’infective individual introduced into the population,we consider the dynamics of the linearized system(2)with reinfection turned off.That is,the system _x¼ÀD Vðx0ÞðxÀx0Þ:ð3ÞBy(A5),the DFE is locally asymptotically stable in this system.Thus,(3)can be used to de-termine the fate of a small number of infected individuals introduced to a disease free population.Let wi ð0Þbe the number of infected individuals initially in compartment i and letwðtÞ¼w1ðtÞ;...;w mðtÞðÞt be the number of these initially infected individuals remaining in the infected compartments after t time units.That is the vector w is thefirst m components of x.The partitioning of D Vðx0Þimplies that wðtÞsatisfies w0ðtÞ¼ÀV wðtÞ,which has the unique solution wðtÞ¼eÀVt wð0Þ.By Lemma1,V is a non-singular M-matrix and is,therefore,invertible and all of its eigenvalues have positive real parts.Thus,integrating F wðtÞfrom zero to infinity gives the expected number of new infections produced by the initially infected individuals as the vector FVÀ1wð0Þ.Since F is non-negative and V is a non-singular M-matrix,VÀ1is non-negative[4,p.137 (N38)],as is FVÀ1.To interpret the entries of FVÀ1and develop a meaningful definition of R0,consider the fate of an infected individual introduced into compartment k of a disease free population.The(j;k)entry of VÀ1is the average length of time this individual spends in compartment j during its lifetime, assuming that the population remains near the DFE and barring reinfection.The(i;j)entry of F is the rate at which infected individuals in compartment j produce new infections in compartment i. Hence,the(i;k)entry of the product FVÀ1is the expected number of new infections in com-partment i produced by the infected individual originally introduced into compartment k.Fol-lowing Diekmann et al.[2],we call FVÀ1the next generation matrix for the model and set R0¼qðFVÀ1Þ;ð4Þwhere qðAÞdenotes the spectral radius of a matrix A.The DFE,x0,is locally asymptotically stable if all the eigenvalues of the matrix Dfðx0Þhave negative real parts and unstable if any eigenvalue of Dfðx0Þhas a positive real part.By Lemma1, the eigenvalues of Dfðx0Þcan be partitioned into two sets corresponding to the infected and uninfected compartments.These two sets are the eigenvalues of FÀV and those ofÀJ4.Again by Lemma1,the eigenvalues ofÀJ4all have negative real part,thus the stability of the DFE is determined by the eigenvalues of FÀV.The following theorem states that R0is a threshold parameter for the stability of the DFE.Theorem2.Consider the disease transmission model given by(1)with fðxÞsatisfying conditions (A1)–(A5).If x0is a DFE of the model,then x0is locally asymptotically stable if R0<1,but un-stable if R0>1,where R0is defined by(4).Proof.Let J1¼FÀV.Since V is a non-singular M-matrix and F is non-negative,ÀJ1¼VÀF has the Z sign pattern(see Appendix A).Thus,sðJ1Þ<0()ÀJ1is a non-singular M-matrix;P.van den Driessche,J.Watmough/Mathematical Biosciences180(2002)29–483334P.van den Driessche,J.Watmough/Mathematical Biosciences180(2002)29–48where sðJ1Þdenotes the maximum real part of all the eigenvalues of the matrix J1(the spectral abscissa of J1).Since FVÀ1is non-negative,ÀJ1VÀ1¼IÀFVÀ1also has the Z sign pattern.Ap-plying Lemma5of Appendix A,with H¼V and B¼ÀJ1¼VÀF,we have ÀJ1is a non-singular M-matrix()IÀFVÀ1is a non-singular M-matrix:Finally,since FVÀ1is non-negative,all eigenvalues of FVÀ1have magnitude less than or equal to qðFVÀ1Þ.Thus,IÀFVÀ1is a non-singular M-matrix;()qðFVÀ1Þ<1:Hence,sðJ1Þ<0if and only if R0<1.Similarly,it follows thatsðJ1Þ¼0()ÀJ1is a singular M-matrix;()IÀFVÀ1is a singular M-matrix;()qðFVÀ1Þ¼1:The second equivalence follows from Lemma6of Appendix A,with H¼V and K¼F.The remainder of the equivalences follow as with the non-singular case.Hence,sðJ1Þ¼0if and only if R0¼1.It follows that sðJ1Þ>0if and only if R0>1.ÃA similar result can be found in the recent book by Diekmann and Heesterbeek[6,Theorem6.13].This result is known for the special case in which J1is irreducible and V is a positive di-agonal matrix[7–10].The special case in which V has positive diagonal and negative subdiagonal elements is proven in Hyman et al.[11,Appendix B];however,our approach is much simpler(see Section4.3).4.Examples4.1.Treatment modelThe decomposition of fðxÞinto the components F and V is illustrated using a simple treat-ment model.The model is based on the tuberculosis model of Castillo-Chavez and Feng[12,Eq.(1.1)],but also includes treatment failure used in their more elaborate two-strain model[12,Eq.(2.1)].A similar tuberculosis model with two treated compartments is proposed by Blower et al.[13].The population is divided into four compartments,namely,individuals susceptible to tu-berculosis(S),exposed individuals(E),infectious individuals(I)and treated individuals(T).The dynamics are illustrated in Fig.1.Susceptible and treated individuals enter the exposed com-partment at rates b1I=N and b2I=N,respectively,where N¼EþIþSþT.Exposed individuals progress to the infectious compartment at the rate m.All newborns are susceptible,and all indi-viduals die at the rate d>0.Thus,the core of the model is an SEI model using standard inci-dence.The treatment rates are r1for exposed individuals and r2for infectious individuals. However,only a fraction q of the treatments of infectious individuals are successful.Unsuc-cessfully treated infectious individuals re-enter the exposed compartment(p¼1Àq).The diseasetransmission model consists of the following differential equations together with non-negative initial conditions:_E¼b1SI=Nþb2TI=NÀðdþmþr1ÞEþpr2I;ð5aÞ_I¼m EÀðdþr2ÞI;ð5bÞ_S¼bðNÞÀdSÀb1SI=N;ð5cÞ_T¼ÀdTþr1Eþqr2IÀb2TI=N:ð5dÞProgression from E to I and failure of treatment are not considered to be new infections,but rather the progression of an infected individual through the various compartments.Hence,F¼b1SI=Nþb2TI=NB B@1C CA and V¼ðdþmþr1ÞEÀpr2IÀm Eþðdþr2ÞIÀbðNÞþdSþb1SI=NdTÀr1EÀqr2Iþb2TI=NB B@1C CA:ð6ÞThe infected compartments are E and I,giving m¼2.An equilibrium solution with E¼I¼0has the form x0¼ð0;0;S0;0Þt,where S0is any positive solution of bðS0Þ¼dS0.This will be a DFE if and only if b0ðS0Þ<d.Without loss of generality,assume S0¼1is a DFE.Then,F¼0b100;V¼dþmþr1Àpr2Àm dþr2;givingVÀ1¼1ðdþmþr1Þðdþr2ÞÀm pr2dþr2pr2m dþmþr1and R0¼b1m=ððdþmþr1Þðdþr2ÞÀm pr2Þ.A heuristic derivation of the(2;1)entry of VÀ1and R0are as follows:a fraction h1¼m=ðdþmþr1Þof exposed individuals progress to compartment I,a fraction h2¼pr2=ðdþr2Þof infectious individuals re-enter compartment E.Hence,a fractionh1of exposed individuals pass through compartment I at least once,a fraction h21h2passthroughat least twice,and a fraction h k 1h k À12pass through at least k times,spending an average of s ¼1=ðd þr 2Þtime units in compartment I on each pass.Thus,an individual introduced into com-partment E spends,on average,s ðh 1þh 21h 2þÁÁÁÞ¼s h 1=ð1Àh 1h 2Þ¼m =ððd þm þr 1Þðd þr 2ÞÀm pr 2Þtime units in compartment I over its expected lifetime.Multiplying this by b 1gives R 0.The model without treatment (r 1¼r 2¼0)is an SEI model with R 0¼b 1m =ðd ðd þm ÞÞ.The interpretation of R 0for this case is simpler.Only a fraction m =ðd þm Þof exposed individuals progress from compartment E to compartment I ,and individuals entering compartment I spend,on average,1=d time units there.Although conditions (A1)–(A5)do not restrict the decomposition of f i ðx Þto a single choice for F i ,only one such choice is epidemiologically correct.Different choices for the function F lead to different values for the spectral radius of FV À1,as shown in Table 1.In column (a),treatment failure is considered to be a new infection and in column (b),both treatment failure and pro-gression to infectiousness are considered new infections.In each case the condition q ðFV À1Þ<1yields the same portion of parameter space.Thus,q ðFV À1Þis a threshold parameter in both cases.The difference between the numbers lies in the epidemiological interpretation rather than the mathematical analysis.For example,in column (a),the infection rate is b 1þpr 2and an exposed individual is expected to spend m =ððd þm þr 1Þðd þr 2ÞÞtime units in compartment I .However,this reasoning is biologically flawed since treatment failure does not give rise to a newly infected individual.Table 1Decomposition of f leading to alternative thresholds(a)(b)Fb 1SI =N þb 2TI =N þpr 2I 0000B B @1C C A b 1SI =N þb 2TI =N þpr 2I m E 000B B @1C C A Vðd þm þr 1ÞE Àm E þðd þr 2ÞI Àb ðN ÞþdS þb 1SI =N dT Àr 1E Àqr 2I þb 2TI =N 0B B @1C C A ðd þm þr 1ÞE ðd þr 2ÞI Àb ðN ÞþdS þb 1SI =N dT Àr 1E Àqr 2I þb 2TI =N 0B B @1C C A F0b 1þpr 200 0b 1þpr 2m 0 V d þm þr 10Àm d þr 2d þm þr 100d þr 2 q (FV À1)b 1m þpr 2mðd þm þr 1Þðd þr 2Þffiffiffiffiffiffiffiffiffiffiffiffiffiffiffiffiffiffiffiffiffiffiffiffiffiffiffiffiffiffiffiffiffiffiffiffiffiffiffiffib 1m þpr 2mðd þm þr 1Þðd þr 2Þs 36P.van den Driessche,J.Watmough /Mathematical Biosciences 180(2002)29–484.2.Multigroup modelIn the epidemiological literature,the term‘multigroup’usually refers to the division of a het-erogeneous population into several homogeneous groups based on individual behaviour(e.g., [14]).Each group is then subdivided into epidemiological compartments.The majority of mul-tigroup models in the literature are used for sexually transmitted diseases,such as HIV/AIDS or gonorrhea,where behaviour is an important factor in the probability of contracting the disease [7,8,14,15].As an example,we use an m-group SIRS-vaccination model of Hethcote[7,14]with a generalized incidence term.The sample model includes several SI multigroup models of HIV/ AIDS as special cases[8,15].The model equations are as follows:_I i ¼X mj¼1b ijðxÞS i I jÀðd iþc iþ iÞI i;ð7aÞ_S i ¼ð1Àp iÞb iÀðd iþh iÞS iþr i R iÀX mj¼1b ijðxÞS i I j;ð7bÞ_Ri¼p i b iþc i I iþh i S iÀðd iþr iÞR i;ð7cÞfor i¼1;...;m,where x¼ðI1;...;I m;S1;...;S m;R1;...;R mÞt.Susceptible and removed individu-als die at the rate d i>0,whereas infected individuals die at the faster rate d iþ i.Infected in-dividuals recover with temporary immunity from re-infection at the rate c i,and immunity lasts an expected1=r i time units.All newborns are susceptible,and a constant fraction b i are born into each group.A fraction p i of newborns are vaccinated at birth.Thereafter,susceptible individuals are vaccinated at the rate h i.The incidence,b ijðxÞdepends on individual behaviour,which determines the amount of mixing between the different groups(see,e.g.,Jacquez et al.[16]). The DFE for this model isx0¼ð0;...;0;S01;...;S0m;R01;...;R0mÞt;whereS0 i ¼b i d ið1Àp iÞþr iðÞd iðd iþh iþr iÞ;R0 i ¼b iðh iþd i p iÞd iðd iþh iþr iÞ:Linearizing(7a)about x¼x0givesF¼S0i b ijðx0ÞÂÃandV¼½ðd iþc iþ iÞd ij ;where d ij is one if i¼j,but zero otherwise.Thus,FVÀ1¼S0i b ijðx0Þ=ðd iÂþc iþ iÞÃ:P.van den Driessche,J.Watmough/Mathematical Biosciences180(2002)29–4837For the special case with b ij separable,that is,b ijðxÞ¼a iðxÞk jðxÞ,F has rank one,and the basic reproduction number isR0¼X mi¼1S0ia iðx0Þk iðx0Þd iþc iþ i:ð8ÞThat is,the basic reproduction number of the disease is the sum of the‘reproduction numbers’for each group.4.3.Staged progression modelThe staged progression model[11,Section3and Appendix B]has a single uninfected com-partment,and infected individuals progress through several stages of the disease with changing infectivity.The model is applicable to many diseases,particularly HIV/AIDS,where transmission probabilities vary as the viral load in an infected individual changes.The model equations are as follows(see Fig.2):_I 1¼X mÀ1k¼1b k SI k=NÀðm1þd1ÞI1;ð9aÞ_Ii¼m iÀ1I iÀ1Àðm iþd iÞI i;i¼2;...;mÀ1;ð9bÞ_Im¼m mÀ1I mÀ1Àd m I m;ð9cÞ_S¼bÀbSÀX mÀ1k¼1b k SI k=N:ð9dÞThe model assumes standard incidence,death rates d i>0in each infectious stage,and thefinal stage has a zero infectivity due to morbidity.Infected individuals spend,on average,1=m i time units in stage i.The unique DFE has I i¼0,i¼1;...;m and S¼1.For simplicity,define m m¼0. Then F¼½F ij and V¼½V ij ,whereF ij¼b j i¼1;j6mÀ1;0otherwise;&ð10ÞV ij¼m iþd i j¼i;Àm j i¼1þj;0otherwise:8<:ð11ÞLet a ij be the(i;j)entry of VÀ1.Thena ij¼0i<j;1=ðm iþd iÞi¼j;Q iÀ1k¼jm kQ ik¼jðm kþd kÞj<i:8>>><>>>:ð12ÞThus,R0¼b1m1þd1þb2m1ðm1þd1Þðm2þd2Þþb3m1m2ðm1þd1Þðm2þd2Þðm3þd3ÞþÁÁÁþb mÀ1m1...m mÀ2ðm1þd1Þ...ðm mÀ1þd mÀ1Þ:ð13ÞThe i th term in R0represents the number of new infections produced by a typical individual during the time it spends in the i th infectious stage.More specifically,m iÀ1=ðm iÀ1þd iÀ1Þis the fraction of individuals reaching stage iÀ1that progress to stage i,and1=ðm iþd iÞis the average time an individual entering stage i spends in stage i.Hence,the i th term in R0is the product of the infectivity of individuals in stage i,the fraction of initially infected individuals surviving at least to stage i,and the average infectious period of an individual in stage i.4.4.Multistrain modelThe recent emergence of resistant viral and bacterial strains,and the effect of treatment on their proliferation is becoming increasingly important[12,13].One framework for studying such sys-tems is the multistrain model shown in Fig.3,which is a caricature of the more detailed treatment model of Castillo-Chavez and Feng[12,Section2]for tuberculosis and the coupled two-strain vector–host model of Feng and Velasco-Hern a ndez[17]for Dengue fever.The model has only a single susceptible compartment,but has two infectious compartments corresponding to the two infectious agents.Each strain is modelled as a simple SIS system.However,strain one may ‘super-infect’an individual infected with strain two,giving rise to a new infection incompartment。
社团结构对一个SIR模型的基本再生数的影响
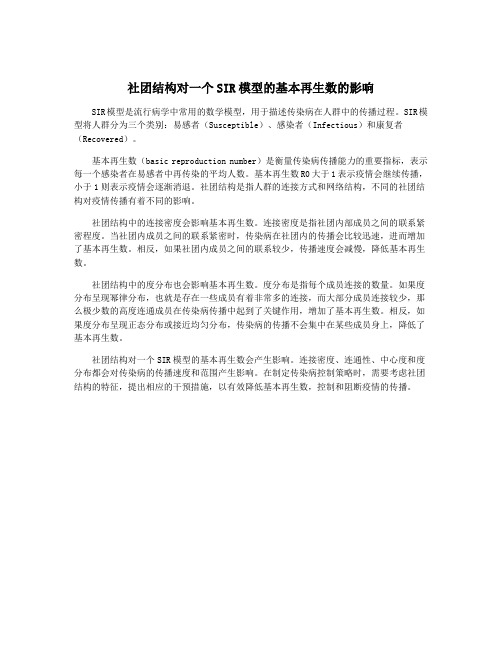
社团结构对一个SIR模型的基本再生数的影响
SIR模型是流行病学中常用的数学模型,用于描述传染病在人群中的传播过程。
SIR模型将人群分为三个类别:易感者(Susceptible)、感染者(Infectious)和康复者(Recovered)。
基本再生数(basic reproduction number)是衡量传染病传播能力的重要指标,表示每一个感染者在易感者中再传染的平均人数。
基本再生数R0大于1表示疫情会继续传播,小于1则表示疫情会逐渐消退。
社团结构是指人群的连接方式和网络结构,不同的社团结构对疫情传播有着不同的影响。
社团结构中的连接密度会影响基本再生数。
连接密度是指社团内部成员之间的联系紧密程度。
当社团内成员之间的联系紧密时,传染病在社团内的传播会比较迅速,进而增加了基本再生数。
相反,如果社团内成员之间的联系较少,传播速度会减慢,降低基本再生数。
社团结构中的度分布也会影响基本再生数。
度分布是指每个成员连接的数量。
如果度分布呈现幂律分布,也就是存在一些成员有着非常多的连接,而大部分成员连接较少,那么极少数的高度连通成员在传染病传播中起到了关键作用,增加了基本再生数。
相反,如果度分布呈现正态分布或接近均匀分布,传染病的传播不会集中在某些成员身上,降低了基本再生数。
社团结构对一个SIR模型的基本再生数会产生影响。
连接密度、连通性、中心度和度分布都会对传染病的传播速度和范围产生影响。
在制定传染病控制策略时,需要考虑社团结构的特征,提出相应的干预措施,以有效降低基本再生数,控制和阻断疫情的传播。
2021-2022学年湖北省武汉市黄陂区九年级(上)期中数学试卷(解析版)

2021-2022学年湖北省武汉市黄陂区九年级第一学期期中数学试卷一、选择题(共10小题,每小题3分,满分30分)1.将方程3x2+2x=5化成一元二次方程的一般形式,若二次项系数为3,则一次项系数和常数项分别是()A.2,5B.2,﹣5C.﹣2,5D.﹣2,﹣52.下列各图案中,属于中心对称图形的是()A.B.C.D.3.方程x2﹣6x=0两个根分别为x1,x2,则x1+x2的值是()A.﹣3B.0C.3D.64.点(1,﹣2)在抛物线y=x2﹣4x+n上,则n的值为()A.﹣2B.﹣1C.1D.25.如图,点A,B分别是两个半圆的圆心,则该图案的对称中心是()A.点A B.点BC.线段AB的中点D.无法确定6.关于x的方程x2﹣3x+n=0有两个不相等的实数根,则n的取值范围是()A.n<B.n≤C.n>﹣D.n>7.抛物线y=(x+1)2﹣2向右平移2个单位再向上平移1个单位后所得抛物线的顶点是()A.(1,1)B.(1,﹣1)C.(﹣1,﹣2)D.(1,﹣2)8.如图,在一块长30m,宽20m的矩形苗圃基地上修建两横一纵三条等宽的道路,剩余空地种植花苗,设道路的宽为xm,若种植花苗的面积为522m2,依题意列方程()A.20x+30×2x=600﹣522B.20x+30×2x﹣x2=600﹣522C.(20﹣2x)(30﹣x)=522D.(20﹣x)(30﹣2x)=5229.如图,抛物线C1:y=x2﹣2x(0≤x≤2)交x轴于O,A两点;将C1绕点A旋转180°得到抛物线C2,交x轴于A1;将C2绕点A1旋转180°得到抛物线C3,交x轴于A2,…,如此进行下去,则抛物线C10的解析式是()A.y=﹣x2+38x﹣360B.y=﹣x2+34x﹣288C.y=x2﹣36x+288D.y=﹣x2+38x+36010.如图,在Rt△ABC中,∠ACB=90°,∠BAC=30°,BC=2,线段BC绕点B旋转到BD,连AD,E为AD的中点,连CE,则CE的长不可能是()A.1.2B.2.05C.2.7D.3.1二、填空题(共6小题,每小题3分,共18分)11.若2是方程x2﹣c=0的一个根,则c的值为.12.x2﹣6x+()=(x﹣)213.如图,在△ABC中,∠BAC=80°,将△ABC绕点A逆时针旋转110°得到△ADE,点B的对应点D恰好落在BC的延长线上,则∠E的度数为°.14.某高档游泳健身馆每人每次游泳健身的票价为80元,每日平均客流量为136人,为了促进全民健身运动,游泳馆决定降价促销,经市场调查发现,票价每下降1元,每日游泳健身的人数平均增加2人.当每日销售收入最大时,票价下调元.15.二次函数y=ax2+2ax+c(a,c为常数且a<0)经过(1,m),且mc<0,下列结论:①c>0;②a<﹣;③若关于x的方程ax2+2ax=p﹣c(p>0)有整数解,则符合条件的p的值有3个;④当a≤x≤a+2时,二次函数的最大值为c,则a=﹣4.其中一定正确的有.(填序号即可)16.如图,菱形ABCD中,AB=12,∠BAD=60°,E为线段BC的中点.若点P是线段AB上一动点,Q为线段AD上一点,则△PQE的周长的最小值是.三、解答题(共8小题,共72分)17.解方程:x2﹣x﹣1=0.18.已知二次函数y=x2﹣4x+3.(1)填表:x…01234…y……(2)在平面直角坐标系中画出函数y=x2﹣4x+3的图象;(3)由图象可知,当y>0时,x的取值范围是.(直接写出结果)19.用一条长40cm的绳子围成一个矩形,设矩形的一边长为xcm.(1)若围成的矩形面积为75cm2,求x的值;(2)当x为何值时围成的矩形面积最大,最大面积是多少?20.如图,在△ABC中,点A(﹣3,﹣1),B(1,1),C(0,3).(1)将△ABC绕点O顺时针旋转90°,点A,B,C的对应点A1,B1,C1均落在格点上,画出旋转后的△A1B1C1,并直接写出点A1,B1,C1的坐标;(2)将△ABC绕点A旋转后,B,C对应点B2,C2均落在格点上,画出旋转后的△AB2C2,并直接写出点B2,C2的坐标;(3)若线段B1C1绕某点旋转后恰好与线段B2C2重合,直接写该点的坐标为.21.已知抛物线y=x2﹣2(m﹣1)x+m2与x轴分别交于(x1,0),(x2,0)两点.(1)求m的取值范围.(2)若x1,x2满足(x1+2)(x2+2)=5,求m的值.(3)点(a,y1),(b,y2),(﹣,y3)均在抛物线上,若﹣<a<b,请直接写出y1,y2,y3的大小关系(用“<”连接).22.R0,也叫基本传染数,或者基本再生数,英文为Basicreproductionnumber.更确切的定义是:在没有外力介入,所有人都没有免疫力的情况下,一个感染某种传染病的人,总共会传染给其他多少个人的平均数.例如:有1人感染新型冠状病毒,若R0=3.50,则经两轮传染后感染新型冠状病毒的人数为:1+1×3.50+1×3.50×3.50≈17(人).时下人心惶惶的新型冠状病毒的基本传染数据估计为3.30到5.40之间.请解答下列问题:(1)若现有10人感染新型冠状病毒,则经历两轮传染后,感染新型冠状病毒的人数大约在什么范围内(直接写出结果,结果保留整数)?(2)最近,新型冠状病毒变异出德尔塔毒株,德尔塔变异病毒的R0值极高.若1人患病,在无任何外力影响下经历两轮传染后共有73人感染.①求德尔塔变异病毒的R0值;②国家研制出新冠疫苗后发现,通过接种疫苗可以使得R0值随接种人数比例的增高同步降低.例如,当疫苗全民接种率达到40%时,此时的R0值为:R0(1﹣40%)=0.6R0.若有1人感染德尔塔变异病毒,要在两轮内将总感染人数控制在7人以内,再加以隔离等措施的干涉,就可控制住疫情,则全民接种率至少应该达到多少?23.在Rt△ABC中,∠ABC=90°,∠A=α,O为AC的中点,将点O沿BC翻折得到点O′,将△ABC绕点O′顺时针旋转,使点B与C重合,旋转后得到△ECF.(1)如图1,旋转角为.(用含α的式子表示)(2)如图2,连BE,BF,点M为BE的中点,连接OM,①∠BFC的度数为.(用含α的式子表示)②试探究OM与BF之间的关系.(3)如图3,若α=30°,请直接写出的值为.24.抛物线C1:y=ax2+bx+3交x轴于A(﹣1,0),B(3,0),交y轴于C.(1)求抛物线的解析式.(2)如图1,抛物线的对称轴l交BC于M,交OB于N,点I为MN的中点.若抛物线上一点P关于点I的中心对称点Q正好落在坐标轴上,求点P的坐标;(3)如图2,点G(﹣3,0),将抛物线C1平移得到抛物线C2,C2的顶点D始终在线段CG上,抛物线C2与x轴交于EF两点,过点D作DH垂直于x轴于点H,线段DH 和EF之间存在怎样的数量关系?判断并说明理由.参考答案一、选择题(共10小题,每小题3分,满分30分)1.将方程3x2+2x=5化成一元二次方程的一般形式,若二次项系数为3,则一次项系数和常数项分别是()A.2,5B.2,﹣5C.﹣2,5D.﹣2,﹣5【分析】首先移项把5移到等号左边,然后再确定一次项系数和常数项.解:3x2+2x=5,3x2+2x﹣5=0,一次项系数是2、常数项是﹣5,故选:B.2.下列各图案中,属于中心对称图形的是()A.B.C.D.【分析】根据中心对称图形定义:把一个图形绕某一点旋转180°,如果旋转后的图形能够与原来的图形重合,那么这个图形就叫做中心对称图形,这个点叫做对称中心进行分析即可.解:选项A、B、C均不能找到这样的一个点,使图形绕某一点旋转180°后与原来的图形重合,所以不是中心对称图形,选项D能找到这样的一个点,使图形绕某一点旋转180°后与原来的图形重合,所以是中心对称图形,故选:D.3.方程x2﹣6x=0两个根分别为x1,x2,则x1+x2的值是()A.﹣3B.0C.3D.6【分析】根据根与系数的关系直接求解.解:∵方程x2﹣6x=0两个根分别为x1,x2,∴x1+x2=6,故选:D.4.点(1,﹣2)在抛物线y=x2﹣4x+n上,则n的值为()A.﹣2B.﹣1C.1D.2【分析】把点(1,﹣2)代入抛物线y=x2﹣4x+n,即可解得n.解:∵点(1,﹣2)在抛物线y=x2﹣4x+n上,∴﹣2=1﹣4+n,解得n=1.故选:C.5.如图,点A,B分别是两个半圆的圆心,则该图案的对称中心是()A.点A B.点BC.线段AB的中点D.无法确定【分析】由已知两个图形的位置,判断它们是否中心对称,可以把各对应点连线,看所有连线是否交于同一点.解:如图对称中心是AB的中点,故选:C.6.关于x的方程x2﹣3x+n=0有两个不相等的实数根,则n的取值范围是()A.n<B.n≤C.n>﹣D.n>【分析】根据方程有两个不相等的实数根,则Δ>0,即Δ=(﹣3)2﹣4n>0,求出n 的取值范围即可.解:∵关于x的方程x2﹣3x+n=0有两个不相等的实数根,∴Δ>0,即Δ=(﹣3)2﹣4n>0,∴n<,故选:A.7.抛物线y=(x+1)2﹣2向右平移2个单位再向上平移1个单位后所得抛物线的顶点是()A.(1,1)B.(1,﹣1)C.(﹣1,﹣2)D.(1,﹣2)【分析】直接根据二次函数图象平移的规律即可得出平移后的抛物线的解析式,进而即可得到结论.解:抛物线y=(x+1)2﹣2向右平移2个单位再向上平移1个单位后所得抛物线是:y =(x+1﹣2)2﹣2+1,即y=(x﹣1)2﹣1,所以顶点为(1,﹣1).故选:B.8.如图,在一块长30m,宽20m的矩形苗圃基地上修建两横一纵三条等宽的道路,剩余空地种植花苗,设道路的宽为xm,若种植花苗的面积为522m2,依题意列方程()A.20x+30×2x=600﹣522B.20x+30×2x﹣x2=600﹣522C.(20﹣2x)(30﹣x)=522D.(20﹣x)(30﹣2x)=522【分析】设道路的宽为xm,则种植花苗的部分可合成长(30﹣x)m,宽(20﹣2x)m的矩形,根据种植花苗的面积为522m2,即可得出关于x的一元二次方程,此题得解.解:设道路的宽为xm,则种植花苗的部分可合成长(30﹣x)m,宽(20﹣2x)m的矩形,依题意得:(30﹣x)(20﹣2x)=522,故选:C.9.如图,抛物线C1:y=x2﹣2x(0≤x≤2)交x轴于O,A两点;将C1绕点A旋转180°得到抛物线C2,交x轴于A1;将C2绕点A1旋转180°得到抛物线C3,交x轴于A2,…,如此进行下去,则抛物线C10的解析式是()A.y=﹣x2+38x﹣360B.y=﹣x2+34x﹣288C.y=x2﹣36x+288D.y=﹣x2+38x+360【分析】根据图象的旋转变化规律,可得出抛物线C10的开口方向及与x轴两个交点坐标,从而可求出其解析式.解:∵抛物线C1:y=x(x﹣2)(0≤x≤2)与x轴交于点O,A;∴抛物线C1开口向上(a=1),且经过O(0,0),A(2,0),∵将C1绕点A旋转180°得C2,交x轴于A1;∴抛物线C2开口向下(a=﹣1),且经过A(2,0),A1(4,0),∵将C2绕点A1旋转180°得到抛物线C3,交x轴于A2,∴抛物线C3开口向上(a=1),且经过A1(4,0),A2(6,0),…,如此进行下去,直至得C10,∴抛物线C10开口向下(a=﹣1),且经过A8(18,0),A9(20,0),∴C10的解析式为:y10=﹣(x﹣18)(x﹣20)=﹣x2+38x﹣360,故选:A.10.如图,在Rt△ABC中,∠ACB=90°,∠BAC=30°,BC=2,线段BC绕点B旋转到BD,连AD,E为AD的中点,连CE,则CE的长不可能是()A.1.2B.2.05C.2.7D.3.1【分析】作AB的中点O,连接OE,根据E为AD的中点,O为AB中点,可得OE=BD =1,从而知点E的轨迹是以点O为圆心,1为半径的圆,可求得CE最大值为3,即可得到答案.解:作AB的中点O,连接OE,如图:由题意知:BD=BC=2,∵点E为AD的中点,点O为AB中点,∴OE=BD=1,∴点E的轨迹是以O为圆心,1为半径的圆,∴当点E在CO延长线上时,CE最大,而由∠ACB=90°,∠BAC=30°,BC=2可得AB=4,∵点O为AB中点,∴OC=AB=2,∴CE最大为OC+OE=2+1=3,∴CE的长度不能是3.1,故选:D.二、填空题(共6小题,每小题3分,共18分)11.若2是方程x2﹣c=0的一个根,则c的值为4.【分析】根据方程的解的概念将x=2代入方程x2﹣c=0,据此可得关于c的方程,解之可得答案.解:根据题意,将x=2代入方程x2﹣c=0,得:4﹣c=0,解得c=4,故答案为:4.12.x2﹣6x+(9)=(x﹣3)2【分析】先根据乘积二倍项确定出后一个数为3,再根据完全平方公式a2±2ab+b2=(a ±b)2即可解答.解:∵(x﹣3)2=x2﹣6x+32=x2﹣6x+9,故答案为:9,3.13.如图,在△ABC中,∠BAC=80°,将△ABC绕点A逆时针旋转110°得到△ADE,点B的对应点D恰好落在BC的延长线上,则∠E的度数为65°.【分析】由旋转的性质可得AB=AD,∠BAD=110°,∠ACB=∠E,由等腰三角形的性质可求∠ABC=35°,由三角形内角和定理可求解.解:∵将△ABC绕点A逆时针旋转110°得到△ADE,∴AB=AD,∠BAD=110°,∠ACB=∠E,∴∠ABC=35°,∵∠BAC=80°,∴∠ACB=65°=∠E,故答案为:65.14.某高档游泳健身馆每人每次游泳健身的票价为80元,每日平均客流量为136人,为了促进全民健身运动,游泳馆决定降价促销,经市场调查发现,票价每下降1元,每日游泳健身的人数平均增加2人.当每日销售收入最大时,票价下调6元.【分析】根据票价为80元,每日平均客流量为136人,当票价每下降1元,每日游泳健身的人数平均增加2人,列出函数解析式,并根据函数的性质求函数的最大值.解:设票价下调x元,每日销售收入为w元,由题意得:w=(2x+136)(80﹣x)=﹣2x2+24x+10880=﹣2(x﹣6)2+10952.∵﹣2<0,∴当x=6时,w最大,∴当每日销售收入最大时,票价下调6元,故答案为:6.15.二次函数y=ax2+2ax+c(a,c为常数且a<0)经过(1,m),且mc<0,下列结论:①c>0;②a<﹣;③若关于x的方程ax2+2ax=p﹣c(p>0)有整数解,则符合条件的p的值有3个;④当a≤x≤a+2时,二次函数的最大值为c,则a=﹣4.其中一定正确的有①②③④.(填序号即可)【分析】根据题目中的二次函数的图象和性质,可以判断各个小题中的结论是否正确,从而可以解答本题.解:∵二次函数y=ax2+2ax+c(a,c为常数且a<0)经过(1,m),∴a+2a+c=m,即3a+c=m,∴3ac+c2=cm,∵mc<0,∴3ac+c2<0,∴0≤c2<﹣3ac,∵a<0,∴c>0,故①正确;∴c<﹣3a,∴a<﹣,故②正确;∵c>0,mc<0,∴m<0,∴点(1,m)在x轴的下方,∵抛物线的对称轴为直线x=﹣=﹣1,a<0,c>0,∴抛物线与直线y=p(p>0)交点的横坐标为整数的有﹣2,﹣1,0三个,∴若关于x的方程ax2+2ax=p﹣c(p>0)有整数解,则符合条件的p的值有3个,故③正确;∵抛物线对称轴为直线x=﹣1,与y轴的交点为(0,c),∴抛物线过(﹣2,c),∵a≤x≤a+2时,二次函数的最大值为c,∴a+2=﹣2,∴a=﹣4,故④正确;故答案为:①②③④.16.如图,菱形ABCD中,AB=12,∠BAD=60°,E为线段BC的中点.若点P是线段AB上一动点,Q为线段AD上一点,则△PQE的周长的最小值是6.【分析】作E点关于AB的对称点G,作E点关于AD的对称点F,连结FG交AD于点Q,交AB于点P,此时△PQE的周长最小,连结DE;先求出FE是BC的垂直平分线,再由DE是BC的垂直平分线,可知D点在EF上,利用直角三角形的勾股定理分别求出FH=18,GH=12,在Rt△FHG中,FG==6.解:作E点关于AB的对称点G,作E点关于AD的对称点F,连结FG交AD于点Q,交AB于点P,∴FQ=EQ,PE=PG,∴PQ+QE+PE=FQ+PQ+GP=FG,此时△PQE的周长最小,由对称性可得,FE⊥AD,GE⊥AB,∵E是BC的中点,∴FE是BC的垂直平分线,连结DE,∵菱形ABCD中,∠BAD=60°,∴△BCD是等边三角形,∵E是BC的中点,∴DE是BC的垂直平分线,∴D点在EF上,∴DF=DE,在Rt△BCD中,BC=12,∠BCD=60°,∴DE=6,∴EF=12,∵AB⊥GH,FH⊥GH,∴FH∥AB∥CD,∴∠HFE=∠CDE=30°,∴HE=EF=6,在Rt△EFH中,FH==18,∵∠ABC=180°﹣∠BAD=120°,∴∠EBM=60°,∴∠BEM=30°,在Rt△BEM中,BM=BE=3,∴ME=3,∴GM=ME=3,∴GE=6,∴GH=GE+EH=6+6=12,在Rt△FHG中,FG==6,∴△PQE的周长的最小值是6,故答案为:6.三、解答题(共8小题,共72分)17.解方程:x2﹣x﹣1=0.【分析】此题考查了公式法解一元二次方程,解题时要注意将方程化为一般形式.确定a,b,c的值,然后检验方程是否有解,若有解,代入公式即可求解.解:∵a=1,b=﹣1,c=﹣1,∴△=(﹣1)2﹣4×1×(﹣1)=5>0,则∴,.18.已知二次函数y=x2﹣4x+3.(1)填表:x…01234…y…30﹣103…(2)在平面直角坐标系中画出函数y=x2﹣4x+3的图象;(3)由图象可知,当y>0时,x的取值范围是x<1或x>3.(直接写出结果)【分析】(1)将x的值逐个代入y=x2﹣4x+3计算即可得对应的y值;(2)描点,连线即可画出函数图象;(3)观察图象在x轴上方的部分,对应x的范围即为所求.解:(1)x=0时,y=x2﹣4x+3=3;x=1时,y=x2﹣4x+3=0;x=2时,y=x2﹣4x+3=﹣1;x=3时,y=x2﹣4x+3=0;x=4时,y=x2﹣4x+3=3;故答案为:3,0,﹣1,0,3;(2)画出函数y=x2﹣4x+3的图象如下:(3)由图象可知,当y>0时,x的取值范围是x<1或x>3,故答案为:x<1或x>3.19.用一条长40cm的绳子围成一个矩形,设矩形的一边长为xcm.(1)若围成的矩形面积为75cm2,求x的值;(2)当x为何值时围成的矩形面积最大,最大面积是多少?【分析】(1)设矩形的一边长为xcm,则矩形的另一边长为(20﹣x)cm,根据矩形的面积为75cm2,由矩形的面积公式列出一元二次方程,解方程即可;(2)设矩形的面积为ycm2,由矩形的面积公式写出函数解析式,并根据函数的性质求最值即可.解:(1)由已知,矩形的另一边长为(20﹣x)cm,由题意得:x(20﹣x)=75,整理得:x2﹣20x+75=0,解得:x1=5,x2=15,答:x的值为5cm或15cm;(2)设矩形的面积为ycm2,由题意得:y=x(20﹣x)=﹣x2+20x=﹣(x﹣10)2+100,∵﹣1<0,∴当x=10时,y有最大值,最大值为100,答:当x为10cm时围成的矩形面积最大,最大面积是100cm2.20.如图,在△ABC中,点A(﹣3,﹣1),B(1,1),C(0,3).(1)将△ABC绕点O顺时针旋转90°,点A,B,C的对应点A1,B1,C1均落在格点上,画出旋转后的△A1B1C1,并直接写出点A1,B1,C1的坐标;(2)将△ABC绕点A旋转后,B,C对应点B2,C2均落在格点上,画出旋转后的△AB2C2,并直接写出点B2,C2的坐标;(3)若线段B1C1绕某点旋转后恰好与线段B2C2重合,直接写该点的坐标为(4,﹣2).【分析】(1)利用旋转变换的在分别作出A,B,C的对应点A1,B1,C1即可;(2)利用旋转变换的性质分别作出B,C的对应点B2,C2即可;(3)线段B1B2,C1C2的垂直平分线的交点即为所求.解:(1)如图,△A1B1C1即为所求,点A1(﹣1,3),B1(1,﹣1),C1(3,0);(2)如图,△AB2C2即为所求,点B2(1,﹣3),C2(2,﹣1);(3)若线段B1C1绕某点旋转后恰好与线段B2C2重合,该点Q的坐标为(4,﹣2).故答案为:(4,﹣2).21.已知抛物线y=x2﹣2(m﹣1)x+m2与x轴分别交于(x1,0),(x2,0)两点.(1)求m的取值范围.(2)若x1,x2满足(x1+2)(x2+2)=5,求m的值.(3)点(a,y1),(b,y2),(﹣,y3)均在抛物线上,若﹣<a<b,请直接写出y1,y2,y3的大小关系(用“<”连接).【分析】(1)先计算判别式的值,然后根据判别式的意义进行证明;(2)根据根与系数的关系x1+x2=2(m﹣1),x1x2=m2,然后利用x1+2)(x2+2)=5,得到关于m的方程,解方程即可;(3)求得抛物线开口向上,对称轴为直线x=m﹣1,由m<得出m﹣1<﹣,然后根据二次函数的性质即可得到结论.解:(1)根据题意得:△=4(m﹣1)2﹣4m2=﹣8m+4>0,解得m<;(2)根据题意得x1+x2=2(m﹣1),x1x2=m2,∵(x1+2)(x2+2)=5,∴x1x2+2(x1+x2)+4=5,∴m2+4m﹣4+4=5,整理得m2+4m﹣5=0,解得m1=﹣5,m2=1,而m<;∴m的值为﹣5;(3)∵y=x2﹣2(m﹣1)x+m2,∴抛物线开口向上,对称轴为直线x=﹣=m﹣1,∴m<,∴m﹣1<﹣∵﹣<a<b,∴﹣<a<b,∴y3<y1<y2.22.R0,也叫基本传染数,或者基本再生数,英文为Basicreproductionnumber.更确切的定义是:在没有外力介入,所有人都没有免疫力的情况下,一个感染某种传染病的人,总共会传染给其他多少个人的平均数.例如:有1人感染新型冠状病毒,若R0=3.50,则经两轮传染后感染新型冠状病毒的人数为:1+1×3.50+1×3.50×3.50≈17(人).时下人心惶惶的新型冠状病毒的基本传染数据估计为3.30到5.40之间.请解答下列问题:(1)若现有10人感染新型冠状病毒,则经历两轮传染后,感染新型冠状病毒的人数大约在什么范围内(直接写出结果,结果保留整数)?(2)最近,新型冠状病毒变异出德尔塔毒株,德尔塔变异病毒的R0值极高.若1人患病,在无任何外力影响下经历两轮传染后共有73人感染.①求德尔塔变异病毒的R0值;②国家研制出新冠疫苗后发现,通过接种疫苗可以使得R0值随接种人数比例的增高同步降低.例如,当疫苗全民接种率达到40%时,此时的R0值为:R0(1﹣40%)=0.6R0.若有1人感染德尔塔变异病毒,要在两轮内将总感染人数控制在7人以内,再加以隔离等措施的干涉,就可控制住疫情,则全民接种率至少应该达到多少?【分析】(1)根据题意,分别计算R0=3.30和R0=5.40时,经历两轮传染后,感染新型冠状病毒的人数,即可得到答案;(2)①由已知列出方程,即可解得德尔塔变异病毒的R0值;②根据已知列出不等式,即可解得答案.解:(1)当R0=3.30时,经历两轮传染后,感染新型冠状病毒的人数为:10+10×3.30+10×3.30×3.30≈152(人),当R0=5.40时,经历两轮传染后,感染新型冠状病毒的人数为:10+10×5.40+10×5.40×5.40≈356(人),∴现有10人感染新型冠状病毒,则经历两轮传染后,感染新型冠状病毒的人数大约在152人至356人;(2)①根据题意得:1+1×R0+1×R0×R0=73,即R02+R0﹣72=0,解得R0=﹣9(舍去)或R0=8,答:德尔塔变异病毒的R0值为8;②设全民接种率至少应该达到x%,根据题意得:1+1×8(1﹣x%)+1×8(1﹣x%)×8(1﹣x%)≤7,令8(1﹣x%)=y,则1+y+y2≤7,∴y2+y﹣6≤0,解得﹣3≤y≤2,即8(1﹣x%)≤2,∴x%≥75%,答:全民接种率至少应该达到75%.23.在Rt△ABC中,∠ABC=90°,∠A=α,O为AC的中点,将点O沿BC翻折得到点O′,将△ABC绕点O′顺时针旋转,使点B与C重合,旋转后得到△ECF.(1)如图1,旋转角为2α.(用含α的式子表示)(2)如图2,连BE,BF,点M为BE的中点,连接OM,①∠BFC的度数为α.(用含α的式子表示)②试探究OM与BF之间的关系.(3)如图3,若α=30°,请直接写出的值为.【分析】(1)根据旋转的性质和轴对称的性质即可得出结论;(2)①连接BO、EO,延长OM交EF于N,结合(1)求出∠BCF=180°﹣2α,再由BC=CF即可得出结论;②先证出△OBM≌△NEM,进而证出四边形OBFN是矩形可得结论;(3)连接CO'交BF于H,由α=30°以及(2)的结论可得HF=CF,进而得到CF =,求出EF=,在Rt△BEF中用勾股定理可得BE OM,可得答案.解:如图1,连接OB、O′B、O′C,∵∠ABC=90°,O为AC的中点,∴OB=OA=OC=AC,∴∠OBA=∠A=α,∴∠CBO=∠ABC﹣∠OBA=90°﹣α,∵将点O沿BC翻折得到点O',∴∠CBO'=∠CBO=90°﹣α,由旋转可知,O'B=O'C,∠FCO'=∠CBO',BC=CF,∴∠BCO'=∠CBO'=90°﹣α,∴∠BO'C=180°﹣2∠CBO'=180°﹣2(90°﹣α)=2α,故答案为:2α;(2)①如图2,连接BO、EO,延长OM交EF于N,由(1)和图1知:∠FCO'=∠CBO'=90°﹣α,BC=CF,∴∠BCF=2∠CBO'=2×(90°﹣α)=180°﹣2α,∵BC=CF,∴∠BFC=∠FBC==α,故答案为:α;②如图2,由①得:∠CBF=∠BFC=∠A=α,由旋转可知:∠CFE=∠BCA,AC=EF,∵∠ABC=90°,∴∠A+∠BCA=90°,∴∠BFC+∠CFE=90°,∴BF⊥EF,∵OC=OB,∴∠OBC=∠BCA,∴∠A+∠BCA=90°,∴∠CBF+∠OBC=90°,∴OB⊥BF,∴OB∥EF,∴∠OBM=∠NEM,∵M为BE的中点,∴BM=EM,在△OBM和△NEM中,,∴△OBM≌△NEM(ASA),∴EN=BO,OM=MN=ON,∴EN=AC=EF,∴N为EF的中点,∴ON∥BF,∵BF⊥EF,∴ON⊥EF,∴四边形OBFN是矩形,∴ON=BF,又∵OM=ON,∴BF=2OM;(3)如图3,连接CO'交BF于H,∵∠BCO'=∠FCO',BC=CF,∴CH⊥BF,BF=2HF,∵BF=2OM,∴OM=HF,由(2)①知:∠BFC=α,∵α=30°,∴∠BFC=30°,∴HF=CF,∵OM=HF,∴CF=,又∵∠ECF=∠ABC=90°,∠FEC=∠A=α=30°,∴EF=2CF=,∴BE===OM,∴=,故答案为:.24.抛物线C1:y=ax2+bx+3交x轴于A(﹣1,0),B(3,0),交y轴于C.(1)求抛物线的解析式.(2)如图1,抛物线的对称轴l交BC于M,交OB于N,点I为MN的中点.若抛物线上一点P关于点I的中心对称点Q正好落在坐标轴上,求点P的坐标;(3)如图2,点G(﹣3,0),将抛物线C1平移得到抛物线C2,C2的顶点D始终在线段CG上,抛物线C2与x轴交于EF两点,过点D作DH垂直于x轴于点H,线段DH 和EF之间存在怎样的数量关系?判断并说明理由.【分析】(1)将点A和点B代入函数解析式求得a与b的大小,从而得到抛物线的解析式;(2)先令x=0求得点C的坐标,然后求得直线BC的解析式,从而求出点M与点N的坐标,进而得到点I的坐标,再利用中心对称的性质求得点P的对称点Q,最后利用坐标轴上点的坐标特征得到点P的坐标;(3)先通过点G与点C的坐标求出直线GC的解析式,然后表示出顶点D的坐标,进而通过顶点式得到抛物线C2的解析式,再令y=0求得点E与点F的横坐标,最后求得DH与EF的长度得到结果.解:(1)将A(﹣1,0),B(3,0)代入y=ax2+bx+3,得,解得:,∴抛物线的解析式为y=﹣x2+2x+3.(2)当x=0时,y=3,则点C(0,3),设直线BC的解析式为y=kx+b,则,解得:,∴直线BC的解析式为y=﹣x+3,∵抛物线y=﹣x2+2x+3的对称轴为直线x=1,∴N(1,0),当x=1时,y=2,即M(1,2),∴MN的中点I的坐标为(1,1),设P(x,﹣x2+2x+3),则点Q的坐标为(2﹣x,x2﹣2x﹣1),当点Q在x轴上时,x2﹣2x﹣1=0,解得:x=1+,或x=1﹣,∴点P为(1+,2)或(1﹣,2),当点Q在y轴上时,2﹣x=0,∴x=2,∴点P为(2,3),综上所述,点P的坐标为(1+,2)或(1﹣,2)或(2,3).(3)EF2=4DH,理由如下,设直线GC的解析式为y=mx+n,则,解得:,∴直线GC的解析式为y=x+3,设点D的坐标为(a,a+3)(﹣3≤a≤0),则DH=a+3,∴抛物线C2的解析式为y=﹣(x﹣a)2+a+3,当y=0时,﹣(x﹣a)2+a+3=0,解得:x=a+或x=a﹣,∴EF=|a+﹣(a﹣)|=2,∴EF2=4(a+3)=4DH.。
具有变化潜伏期的结核病模型稳定性分析

具有变化潜伏期的结核病模型稳定性分析霍海峰;党帅军【摘要】A tuberculosis model with variable latent period was studied and the basic reproduction number R0 was obtained by using regeneration matrix. There would be a disease-free equilibrium and it was globally asymptotically stable when R0≤1. Then, if R0>1, there would be only a unique endemic equilibrium,which was also globally, asymptotically stable.%研究一类具有变化潜伏期的结核病模型,利用再生矩阵方法,得到基本再生数R0,进一步得到当R0≤1时,系统存在无病平衡点,且是全局渐近稳定的,当R0>1时,系统存在唯一的地方病平衡点,且是全局渐近稳定的.【期刊名称】《兰州理工大学学报》【年(卷),期】2011(037)003【总页数】5页(P133-137)【关键词】结核病;变化潜伏期;平衡点;全局渐近稳定【作者】霍海峰;党帅军【作者单位】兰州理工大学理学院,甘肃兰州,730050;兰州理工大学理学院,甘肃兰州,730050【正文语种】中文【中图分类】O175.1结核病是通过空气传播的一种慢性疾病[1],它由结核杆菌感染引起,又称为“痨病”.结核病一般通过空气传播,当患者咳嗽、打喷嚏、说话或吐痰时,他们把结核杆菌排放到空气中,只需要吸入少量杆菌就可造成感染,有些感染结核杆菌的人马上患病,也就是说这些人不经过潜伏期直接患结核病,成为结核病病人[1],但是有的人由于免疫系统可杀死或者“隔离”结核杆菌,就进入了潜伏期.潜伏期也是因人而异,有些感染者潜伏期较短,大概在两年之内,有些感染者潜伏期长达几十年,还有一部分感染者甚至一生都处在潜伏期,而且不发病.文献[2]研究具有阶段结构的结核病模型,其中将潜伏期分成前期和后期两个阶段进行研究.文献[3]将潜伏期分成短潜伏期和长潜伏期两类,与文献[2]不同的是这里的两个潜伏期之间没有联系,感染者只能进入短潜伏期或者长潜伏期.本文在文献[3]中模型的基础上进行改进,考虑感染者不经过潜伏期直接患病的情况,并研究改进模型中无病平衡点和地方病平衡点的全局渐近稳定性,其他相关文献可参见文献[4,5].1 模型建立根据流行病动力学仓室建模思想,本文把总人群分成5类:S(t)表示t时刻易感者的数量,E 1(t)表示t时刻短潜伏期感染者的数量,E 2(t)表示t时刻长潜伏期感染者的数量,I(t)表示t时刻染病者的数量,R(t)表示t时刻治愈者的数量.设N(t)表示t时刻的人口总数,所以N(t)=S(t)+E 1(t)+E 2(t)+I(t)+R(t).假设所有易感者与染病者进行有效接触后成为短潜伏期感染者,长潜伏期感染者或者直接成为染病者,可得具有变化潜伏期的结核病传播机制如图1所示.相应的结核病动力学模型为式中:Λ表示人口的自然补充率,μ表示人口的自然死亡率,d表示人口的因病死亡率,β表示感染率,k1表示短潜伏期感染者转化成染病者的速度,k2表示长潜伏期感染者转化成染病者的速度,r表示染病者的治愈率,p1,p2,p3表示易感者和染病者有效接触后分别转化成短潜伏期感染者,长潜伏期感染者,染病者的比例,p1+p2+p3=1,并且所有参数非负.图1 具有变化潜伏期的结核病传播机制图Fig.1 Transfer mechanism diagramof tuberculosis with variable latent period2 无病平衡点的全局渐近稳定性3 地方病平衡点的全局渐近稳定性4 数值模拟根据国家统计局发布的《中华人民共和国2009年国民经济和社会发展统计公报》,卫生部公布的《中国结核病防治社会评价结果》,以及参考文献[9]中的相关数据,可以确定表1中部分参数的参数值.当β=0.001时,R0<1,图2给出此时具有不同初值的结核病患者的时间曲线,当β=1时,R0>1,图3给出此时具有不同初值的结核病患者的时间曲线.表1 系统(5)中参数的含义和参数值Tab.1 Implication and magnitude of parameters in system (5)参数含义参数值数据来源Λ人口自然补充率 2/年参考文献[9]μ人口自然死亡率 0.007 08 《中华人民共和国2009年国民经济和社会发展统计公报》d结核病死亡率 0.022 7 参考文献[9]r结核病治愈率0.916 《中国结核病防治社会评价结果》β感染率可变估计k1 短潜伏期感染者转化成结核病患者的速度 0.5 估计k2 长潜伏期感染者转化成结核病患者的速度 0.5 估计p1 易感者感染结核杆菌后进入短潜伏期的比例 0.3 估计p2 易感者感染结核杆菌后进入长潜伏期的比例 0.5 估计p3 易感者感染结核杆菌后直接患病的比例 0.2估计图2 当β=0.001时,R0<1,患病人数I(t)随时间的变化Fig.2 Variation of I (t)with time forβ=0.001,or R0<1图3 当β=1时,R0>1,患病人数I(t)随时间的变化Fig.3 Variation of I(t)with time forβ=1,or R0>1从图2和图3的数值模拟结果可以得出:无论初值取多少,随着时间的增加,患病人数I(t)分别趋向于0和一个固定值,这就说明当R 0≤1时,无病平衡点p 0在Ω内是全局渐近稳定的,当R 0>1时,地方病平衡点p*在Ω内是全局渐近稳定的.5 结论本文将所有易感者与染病者进行有效接触后,分成短潜伏期感染者,长潜伏期感染者或者直接成为染病者三类,研究具有变化潜伏期的结核病模型,通过构造Liapunov函数,利用LaSalle不变集原理,证明平衡点的全局稳定性.当R 0≤1时,无病平衡点p 0全局渐近稳定,这就意味着结核病被根除了,当R0>1时,地方病平衡点p*全局渐近稳定,这意味着结核病将成为地方病而一直存在.致谢:本文得到教育部科学技术研究重点项目(209131)、教育部留学回国人员科研启动基金、甘肃省高校研究生导师基金(0803-01)、人力资源和社会保障部留学人员科技活动项目择优资助、兰州理工大学优秀青年教师培养计划(Q200703)及博士启动基金的资助,在此表示感谢.参考文献:[1] CASTILL CHAVEZ C,SONG B J.Dynamical models of tuber-culosis and their applications[J].Mathematical Biosciences and Engineering,2004,1(2):361-404.[2] ZIV E,DALEY C L,BLOWER S M.Early therapy for latent tuberculosis infection[J].American Journal of Epidemiology,2001,153:381-385. [3] FENG W Y,GAO F.Proceedings of the SNPD 2007 [C].Qingdao:IEEE Computer Society,2007:422-425.[4]霍海峰,佘玉星,孟新友.一类公共健康教育影响下的戒烟模型的稳定性[J].兰州理工大学学报,2010,36(3):135-138.[5]霍海峰,付强,孙小科,等.一类时滞周期捕食-食饵模型的持久性[J].兰州理工大学学报,2009,35(3):134-138.[6] VAN DEN DRIESSCHE P,WATMOUGH J.Reproduction numbers and sub-threshold endemic equilibria for compartmental models of disease transmission[J].Mathematical Biosciences,2002,180:29-48.[7] LASALLE J P.The stability of dynamical systems[M].Philadelphia:Society for Industrial and Applied Mathematics,1976.[8] LASALLE J P.Stability theory for ordinary differential equations [J].Differential Equations,1968,41:57-65.[9] BOWONG S,TEWA J J.Mathematical analysis of a tuberculosis model with differential infectivity [J].Commun Nonlinear Sci Numer Simulat,2009,14:4010-4021.。
流行病学专业英语词汇

流行病学专业英语词汇A安慰剂Placebo安慰剂效应placebo effectsB把握度study power半试验semi-trial保护率protective rate ,PR报告偏倚reporting bias暴露exposure暴露标志exposure marker暴露怀疑偏倚exposure suspicion bias爆发调查outbreak survey被动监测passive surveillance比例研究proportional study比值比Odds Ratio ,OR必需病因necessary factor of cause标点地图spot map标化比例死亡比standardized proportional mortality ratio,SPMR 标化比值比standardized odds ratio,SOR标化死亡比standardized mortality ratio,SMR标准化standardization标准化率比standardized rate ratio,SRR"冰山"现象ice-berg phenomenon丙型肝炎hepatitis C,HC并联试验parallel test病毒性肝炎viral hepatitis病例报告case report病例-病例研究case-case study病例调查case survey病例对照研究case-control study病例交叉设计case-crossover design病例交叉研究case-crossover study病例-系列研究case-series study病死率fatality rate病因causation of disease病因的异质性etiological heterogeneity病因分值etiologic fraction,EF病因链chains of causation病因网web of causation不合格ineligibility不依从noncomplianceC测量偏倚measurement bias长期变异secular change,trend variation超额死亡率excess mortality超额危险度excess risk巢式病例对照研究nested case-control study成本效果分析cost -effectiveness analysis成本效益分析cost-benefit analysis成本效用分析cost-utility成组匹配category matching抽样调查sampling survey抽样框架sampling frame抽样误差sampling error出生队列birth cohort出生队列分析法birth cohort analysis初级卫生保健primary health care ,PHC传播概率transmission probability传播机制mechanism of transmission传播途径path of transmission传播途径transmission route传播因素transmitting factor传染病communicable diseases传染过程infectious process传染性infectiousness串联试验serial test粗死亡率crude death rate改成crude mortality rate 较好粗一致性crude agreement促成病因contributory factor of cause错分偏倚misclassification bias错误分类misclassificationD大骨节病Kashin-Beck disease大流行pandemic代表性representativeness单倍型haplotype单纯随机抽样simple random sampling单盲single blind单项筛检single screening等位基因allele等位基因频率gene frequency地方病endemic diseases地方病学Endemiology地方性endemicity地方性氟中毒endemic fluorosis地方性砷中毒endemic arsenism点突变point mutation点源流行point source epidemic碘缺乏病iodine deficiency disease调查随访研究survey follow-up study调整一致性adjusted agreement丁型肝炎hepatitis D,HD动态人群dynamic population毒力virulence短期波动irregular variation,rapid fluctuation队列cohort队列研究cohort study对象报告偏倚report bias多归因程序的方法Multiple imputation procedure多国心血管病趋势和决定因素监测Multinational Monitoring of Trends and Determinants inardiovascular Diseases,MONICA 多级抽样multistage sampling多群组比较研究multi-group comparison study多态性polymorphism多项筛检multiple screeningE恶性肿瘤流行病学cancer Epidemiology二代侵袭率,续发率secondary attack rate,SAR二级预防secondary preventionF发病率incidence rate,morbidity发病密度incidence density发病密度incidence density发生率研究incidence study飞沫droplet飞沫核droplet nuclei非即时性non-concurrent非均衡性错分differential misclassification非连续性状Discrete traits肺炎与流感死亡率pneumonia and influenza deaths,即P&I deaths分层stratification分层抽样stratified sampling分层随机分组stratified randomization分类变量categorical variable分离分析segregation analysis分子流行病学Molecular Epidemiology封闭性抗体blocking antibodies氟斑牙dental fluorosis氟骨症skeletal fluorosis负混杂negative confounding复等位基因位点multiple alles复杂性状complex traitG概念框架conceptual framework干预随访研究intervention follow-up study感染储主reservoir of infection感染来源source of infection感染力infectivity感染谱spectrum of infection感染梯度gradient of infection感染性腹泻infectious diarrhea感染性腹泻病infectious diarrheal disease感染症infections or infectious diseases高危人群策略high risk strategy个案case个案调查case study个案调查individual survey个体匹配individual matching工程干预engineering intervention共变法method of concomitant variation共显性codominance构成比proportion固定队列fixed cohort故意伤害intentional injuries关联的合理性plausibility of association关联的可重复性consistency of association关联的强度strength of association关联的时间性temporality of association关联的特异性specificity of association关联的一致性coherence of association观察偏倚observational bias观察性研究observational study观察一致率observation agreement广义遗传度broad heritability归因危险度attributable risk归因危险度百分比attributable risk percent,ARP,或AR%国际病毒分类委员会International Committee on Taxonomy of Viruses,ICTV 国际冠心病预防工作组The International Task Force for Prevention of Coronary Heart Disease国际疾病分类第十版International Classification of Diseases,ICD-10国家结核病防治项目National Tuberculosis Program,NTP国家卫生统计中心the National Center for Health Statistics,NCHSH合作率cooperation rate核心家系Nuclear families横断面研究cross sectional analysis横断面研究cross-sectional study患病率prevalence rate回顾性研究retrospective studies回忆偏倚recall bias混合型流行mixed epidemic混合研究mixed study混杂confounding混杂偏倚confounding bias混杂因素confounder,confounding factor活跃病区active endemic area获得性免疫缺陷综合症acquired immunodeficiency syndrome,AIDS 霍桑效应Howthorne effectJ机遇chance基本繁殖率basic reproduction rate基本繁殖数basic reproductive number,R0基本人群base population or population at risk基线信息baseline information基因gene基因毒性genotoxic基因型Genotyping基因型频率genotype frequency及时性timeliness疾病爆发disease outbreak疾病标志Markers of disease疾病的分布distribution of disease疾病的自然史natural history of disease疾病监测surveillance of disease疾病链the disease chain剂量反应关系dose-response relationship季节性seasonal variation,seasonality继发关联secondary association家庭簇研究family cluster study家庭聚集性Familial aggregation家庭聚集性研究familial aggregation study家庭相似性Family resemblance家系研究pedigree study甲型肝炎hepatitis A,HA假阳性率false positive rate假阴性率false negative rate间接传播indirect transmission间接关联indirect association间接接触indirect contact检出症侯偏倚detection signal bias简单随机分组simple randomization简明损伤定级法abbreviated injury scale,AIS健康促进health promotion健康工作者效应healthy worker effect健康监测系统health information system,HIS健康生命损失年years of life lived with disability,YLLD 教育干预educational intervention结局outcome结局变量outcome variable截断点cutoff金标准gold standard经济干预economic intervention经节肢动物媒介vector-borne经空气air-borne经食物food-borne经饮水drinking water-borne经载体vehicle-borne精确性precision聚合酶链式反应polymerase chain reaction,PCR均衡性错分non-differential misclassification均数回归趋势regression to the meanK抗原漂移antigenic drift抗原转变antigenic shift克山病Keshan disease空间、时间集研究space-time cluster study扩大免疫计划expanded program on immunization,EPIL来源同一identity by descent,IBD类试验,准试验quasi-trial,quasi-experiment类推法method of analogy累积发病率cumulative incidence罹患率attack rate礼貌偏倚politeness bias理论流行病学theoretical epidemiology历史性historical连锁linkage连锁不平衡linkage disequilibrium连锁分析Linkage analysis连锁平衡linkage equilibrium联合无应答偏倚combined nonrespondent bias联系率contact rate临床试验clinical trial灵敏度sensitivity领先时间偏倚lead time bias流感病毒influenza virus流行epidemic流行病模型epidemic model流行病学epidemiology流行病学监测epidemiological surveillance流行病学实验epidemiological experiment流行过程epidemic process流行曲线epidemic curve流行性出血热epidemic hemorrhagic fever,EHF流行性感冒Influenza流行性肾病nephropathia epidemica,NE率rate率比rate ratio率差rate difference,RD轮状模式wheel modeM蔓延流行propagated or progressive epidemic盲法blindness美国国家胆固醇教育规划National Cholesterol Education Program,NCEP 描述流行病学descriptive epidemiology描述性研究descriptive study目标人群target populationN内暴露剂量Internal Dose内部有效性internal validity内剂量internal dose内剂量的测定internal dose meters纳入/排除偏倚inclusion/exclusion bias奈曼偏倚Neyman biasP排除exclusions排除法method of exclusion排除偏倚exclusive bias配对pair matching"皮鞋"流行病学shoeleather epidemiology匹配matching匹配变量matching variable匹配过头overmatching匹配因素matching factors偏倚bias频数匹配frequency matching普查censusQ前瞻性prospective 8前瞻性研究prospective study潜伏期incubation period潜隐期latent period潜在工作损失年数Working Years of Potential Life Lost,WYPLL潜在价值损失年数Valued Years of Potential Life Lost,VYPLL潜在寿命损失年potential years of life lost,PYLL强制干预enforcement intervention求同法method of agreement求异法method of difference全人群策略population strategy缺课天数days lost from school缺勤天数days lost from work缺失数据missing data确定性检验explicit testing确定性模型deterministic modelR人传人person to person spread人类传染病anthroponosis人类基因组计划human genome project人类免疫缺陷病毒human immunodeficiency virus,HIV人群population人群病因分值population etiologic fraction,PEF人群的分层population stratification人群归因危险度population attributable risk,PAR人群归因危险度百分比population attributable risk percent,PARP 或PAR% 人群免疫性herd immunity人群易感性herd susceptibility人兽共患病,动物传染病zoonosis人畜共患病anthropozoonosis日期型date type入院率偏倚(伯克森偏倚)admission rate bias (Berkson's bias)。
两个流行病模型的定性分析
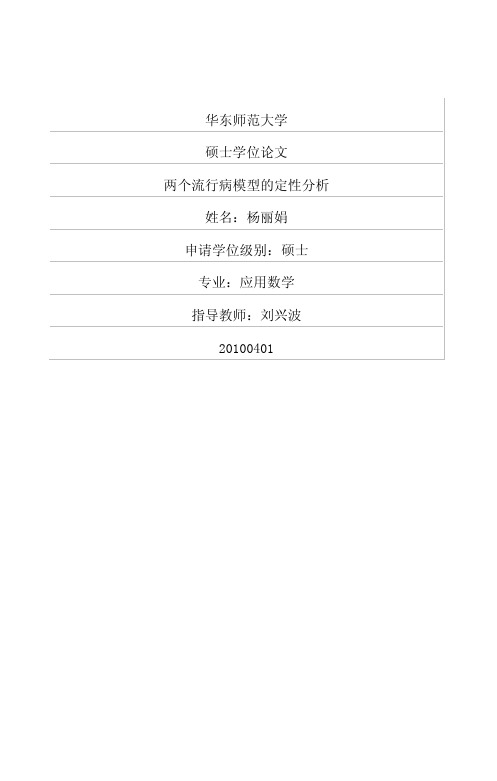
拟传染病传播规律的数学模型,将疾病传播规律与特点公式化。分析公式来探索疾病传播与 人类利用医学上的各种手段进行治疗与防控之间的关系,努力寻求它们遵循的规律,试图预
测疾病将要发展的趋势,希望通过人为因素的干预能够在节约资源的情况下有效控制疾病 的传播和大范围爆发. 流行病学是研究疾病在时间和空间上的传播规律,目的就是找出疾病产生,持久,爆发 和能否根除的决定因素.从而为人类如何更有效的与流行疾病作斗争服务. 自Kermack和Mckendrick在1926年构造了著名的SIR仓室模型.后来又在所建的模 型的基础上,提出了区分疾病流行与否的”阀值理论”.这种仓室模型及研究模型的”阀值理 论”一直沿用至今并且得到很大发展.根据现实流行病的特点如疾病的周期性,潜伏期和不 同的发生率函数等特点以及人类对疾病采取的干涉措施如接种预苗,对患者的隔离,医学
掣=A—pN(f)-郴(D吨fit)咱∞)
≤A—pN(t), 所以由比较原理有 limⅣ(f)s—A.
t--*oa
卢
根据系统(1.2)的生物学意义,我们只需在闭集F=I(S,E,,,Q,尺)∈避oIO≤S+E+,+Q+
R≤三}内研究系统(1.2),F为系统(1.2)的最大正向不变集.其中R%={ol,jr2,勋,目,玛):
between the
basic reproduction number
R0.We
show that the disease・free
equilibrium is globally asymptotically stable when Ro S
1,and
if Ro>1,the disease free
其中S(D,E(唬J(r),Q(f),R(t)分别表示在时刻t易感染人口,潜伏期人口,未隔离的感染人
具有一般死亡率的SIRS模型研究
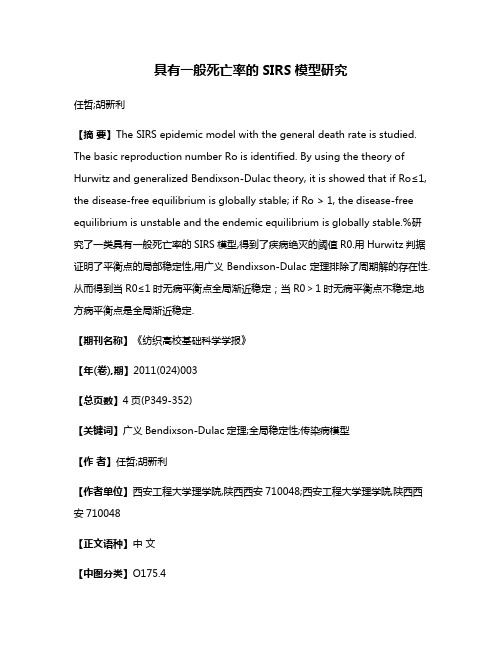
具有一般死亡率的SIRS模型研究任哲;胡新利【摘要】The SIRS epidemic model with the general death rate is studied. The basic reproduction number Ro is identified. By using the theory of Hurwitz and generalized Bendixson-Dulac theory, it is showed that if Ro≤1, the disease-free equilibrium is globally stable; if Ro > 1, the disease-free equilibrium is unstable and the endemic equilibrium is globally stable.%研究了一类具有一般死亡率的SIRS模型,得到了疾病绝灭的阈值R0.用Hurwitz判据证明了平衡点的局部稳定性,用广义Bendixson-Dulac定理排除了周期解的存在性.从而得到当R0≤1时无病平衡点全局渐近稳定;当R0>1时无病平衡点不稳定,地方病平衡点是全局渐近稳定.【期刊名称】《纺织高校基础科学学报》【年(卷),期】2011(024)003【总页数】4页(P349-352)【关键词】广义Bendixson-Dulac定理;全局稳定性;传染病模型【作者】任哲;胡新利【作者单位】西安工程大学理学院,陕西西安710048;西安工程大学理学院,陕西西安710048【正文语种】中文【中图分类】O175.41 引言与预备知识传染病是危害人类健康的大敌,长期以来人类与传染病做了不屈不挠的斗争.随着近年来新型传染病的出现(如SARS、禽流感等)及老传染病的重新抬头(如百日咳等疾病),研究传染病的传播规律已经成为应用数学的一个热点问题.传染病通常由病毒或细菌引起.由细菌引起的传染病的传播规律往往符合SIS,SIRS,SIR等传染病模型,如鼠疫、白喉、炭疽、霍乱等疾病;由病毒引起的传染病的传播规律往往符合SIS,SIRS等传染病模型,如病毒性肝炎、水痘、麻疹、艾滋病等疾病[1].关于此类模型研究的已经很多,有些模型涉及到接触传染、垂直传染和媒介传染等不同的传染方式,有些涉及到因病死亡、预防接种等因素,有些考虑周期环境下疾病的传播,构成了丰富多彩的传染病动力学模型[2-11].然而,在以往的模型中都是考虑常数死亡率或者是Logistic死亡率,而F.E.Smith在1963年研究一种水蚤时发现种群的相对增长率不符合线性增长规律[12].基于这样的事实,本文考虑具有一般死亡率的 SIRS模型,并用广义的Bendixson-Dulac定理排除了周期解的存在性,从而得到平衡点的全局稳定性.本文研究模型S(t)、I(t)、R(t)分别表示易感者、染病者、恢复者在t时刻的数量,N(t)为总人口数量.其中r是自然出生率;β是传染率系数;f(N)是种群自然死亡率且满足f(N)>0,f'(N)>0,f(0)<r<f(∞);α为因病死亡率;ε为免疫丧失率;γ为恢复率.因为N=S+I+R,所以总人口满足方程N'=N(r-f(N))-αI.首先对模型做归一化处理.令x=S/N,y=I/N,z=R/N,则x,y,z分别表示易感染类(S)、感染类(I)、移出类(R)在种群中所占的比率.系统(1)可化为因为x+y+z=1,故系统(2)第3个方程可由前两个方程得到,从而系统(2)等价于显然D={(x,y)|x>0,y≥0,x+y<1}是系统(3)的正向不变集.由文献[13]可得基本再生数为R02 平衡点的存在性定理1 系统(3)总存在无病平衡点P0(x0,y0),其中x0=1,y0=0.当R0>1时,系统(3)存在惟一地方病平衡点P*(x*,y*),其中x*满足α(β-α)y2-[β(γ+α+r+ε)-α(ε+γ+α+2r)]y+(r+ε)(γ+α+r)(R0-1)=0.证明显然,系统(3)存在无病平衡点P0(x0,y0),x0=1,y0=0.解方程由方程(4)中的第2个方程有将式(5)代入方程(4)中第1个方程,有若R0≤1,直线l1:βx+αy-(γ+α+r)=0与x、y轴的交点分别为因+1>1,所以直线l1在区域D之外,方程(4)无解.若R0>1时,则β>α.直线l1:βx+αy-(γ+α+r)=0与直线x+y=1在第一象限内相交与点M.解方程得到M的纵坐标,因此,系统(3)地方病平衡点P*(x*,y*)存在性等价于方程F(y)=0在区间(0,¯y)内解存在.由式(6)得F(¯y)<0,又F(0)=(r+ε)(γ+α+r)(R0-1)>0.由根的存在性定理及F(y)的凹性可知,方程F(y)=0在区间(0,¯y)内存在惟一解.综合上述,若R0≤1时,无病平衡点P0(x0,y0),其中x0=1,y0=0是系统(3)在区域D上的惟一平衡点.若R0>1时,系统(3)除无病平衡点P0(x0,y0)外,还有惟一的地方病平衡点P*(x*,y*).3 平衡点的稳定性定理2 对于系统(3),当R0≤1时,无病平衡点P0(x0,y0),x0=1,y0=0是局部渐近稳定;当R0>1时,无病平衡点P0(x0,y0)不稳定,但地方病平衡点P*(x*,y*)是局部渐稳定.证明系统(3)在平衡点P0(x0,y0),x0=1,y0=0处的Jacobin矩阵为J(P0)的2个特征根分别为λ1=-(r+ε),λ2=(r+α+γ)(R0-1),从而由Hurwitz 判据知当R0>1时,无病平衡点P0(x0,y0)不稳定;当R0≤1时,无病平衡点P0(x0,y0)局部渐近稳定.系统(3)在平衡点P*(x*,y*)处的Jacobin矩阵为计算可得 det J(P*)=-αy*[(β-α)y*+(r+ε)]-βy*[-(β-α)x* -ε]=-y*F'(y),tr J(P*)=-(β -α)y*+由定理1的证明过程可知y* <(r+ε)/α.所以det J(P*)>0,tr J(P*)<0,即J(P*)的特征根具有负实部.根据Hurwitz判据知,地方病平衡点P*(x*,y*)是局部渐近稳定.为了排除系统的周期解,将要用到引理1,即广义Bendixson-Dulac定理.引理1[1]设S⊂R3是有向光滑的曲面.Γ(t)⊂S是任意一条光滑闭曲线,且Γ(t)是曲面S'⊂S的边界.若f:Γ(t)→R3是Lipschitz的,且f和g满足g(Γ(t))·f(Γ(t))=0,(Curl g)·n >0(或者<0)在S上.这里n是曲面S上的单位法向量,则Γ(t)不可能是系统˙x=f(x)的异宿环.定理3 对于系统(3),当R0≤1时,无病平衡点P0(x0,y0)是全局渐近稳定的;当R0>1时,地方病平衡点P*(x*,y*)是全局渐近稳定的.证明首先证明系统没有周期解.由于区域Ω ={(x,y,z)|x>0,y≥0,z>0,x+y+z=1}是系统(3)的正向不变集,又易知系统区域Ω的边界线不可能是系统(3)的周期解.所以仅在区域Ω内讨论.如果系统(3)在Ω内有周期解φ(t)={x(t),y(t),z(t)},并且φ(t)的轨线Γ所围的平面区域Ⅱ位于Ω的内部.记 f1,f2,f3,分别为系统(3)右端的表达式,则则显然g·f=0.记 g=(g1,g2,g3)和 Curl g=,其中在区域Ω 内Curl g·n=Curl g·(1,1,1)T=设平面区域Ⅱ的方向是向上,轨线Γ的方向与Ⅱ的方向成右手法则.由于向量(1,1,1)T是平面区域Ⅱ的法向量,由引理1知,系统(3)没有周期解.结合定理2可以得到当R0≤1时,无病平衡点P0(x0,y0)是全局渐近稳定的;当R0>1,时,地方病平衡点P*(x*,y*)是全局渐近稳定的.4 结束语讨论了具有一般死亡率的SIRS模型,证明了当R0≤1时,无病平衡点P0(x0,y0)是全局渐近稳定的,疾病绝灭;当R0>1时,地方病平衡点P*(x*,y*)是全局渐近稳定的,此时,疾病持续.参考文献:【相关文献】[1]马知恩,周义仓,王稳地,等.传染病动力学的数学建模与研究[M].北京:科学出版社,2004.[2] KERMARK M D,MCKENDRICK A G.Contributions to the mathematical theory of epidemics[J].Proc Roy Soc:A.PartⅠ,1927,115(5):700-721.[3]赵文才,孟新柱.具有垂直传染的SIR脉冲预防接种模型[J].应用数学,2009,22(3):676-682.[4]辛京奇,王文娟.一类具有阶段结构和接种的SIR传染病模型[J].数学的实践与认识,2009,39(12):103-108.[5]李录苹,贾建文.具有周期传染率和垂直传染的SIR传染病模型的周期解[J].数学的实践与认识,2009,39(12):127-135.[6]赵文才,孟新柱.一类具有Logistic死亡率的脉冲免疫接种SIRS传染病模型[J].吉林大学学报:理学版,2009,47(6):1 165-1 171.[7]胡新利,周义仓.具有周期传染率的SIR传染病模型的周期解[J].生物数学学报,2008,23(1):91-100.[8]付景超,井元伟,张中华,等.具垂直传染和连续预防接种的SIRS传染病模型的研究[J].生物数学学报,2008,23(2):273-278.[9]胡新利,孙法国,王彩霞.具有饱和接触率和垂直感染的SIR模型全局分析[J].纺织高校基础科学学报,2010,23(1):120-122.[10]周玲丽,胡新利,孙传姣.具有脉冲接种的传染病模型的渐近分析[J].纺织高校基础学科学报,2003,16(2):105-110.[11]胡新利,王凯明,金上海.一类SIR流行病模型的周期解的全局存在性[J].纺织高校基础学科学报,2004,17(3):194-197.[12]马知恩.种群生态学的数学建模与研究[M].合肥:安徽教育出版社,1996.[13] Van den DRIESSCHE P,WATMOUGH J.Reproduction numbers and sub-threshold endemic equilibria for compartmental models of disease transmission [J].Math Biosci,2002,180:29-48.。
2020年我国儿童百日咳免疫预防策略(全文)
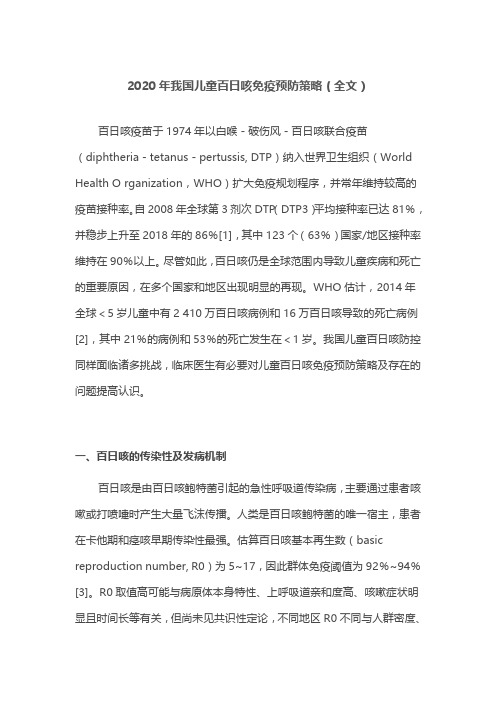
2020年我国儿童百日咳免疫预防策略(全文)百日咳疫苗于1974年以白喉-破伤风-百日咳联合疫苗(diphtheria-tetanus-pertussis, DTP)纳入世界卫生组织(World Health O rganization,WHO)扩大免疫规划程序,并常年维持较高的疫苗接种率。
自2008年全球第3剂次DTP(DTP3)平均接种率已达81%,并稳步上升至2018年的86%[1],其中123个(63%)国家/地区接种率维持在90%以上。
尽管如此,百日咳仍是全球范围内导致儿童疾病和死亡的重要原因,在多个国家和地区出现明显的再现。
WHO估计,2014年全球<5岁儿童中有2 410万百日咳病例和16万百日咳导致的死亡病例[2],其中21%的病例和53%的死亡发生在<1岁。
我国儿童百日咳防控同样面临诸多挑战,临床医生有必要对儿童百日咳免疫预防策略及存在的问题提高认识。
一、百日咳的传染性及发病机制百日咳是由百日咳鲍特菌引起的急性呼吸道传染病,主要通过患者咳嗽或打喷嚏时产生大量飞沫传播。
人类是百日咳鲍特菌的唯一宿主,患者在卡他期和痉咳早期传染性最强。
估算百日咳基本再生数(basic reproduction number, R0)为5~17,因此群体免疫阈值为92%~94%[3]。
R0取值高可能与病原体本身特性、上呼吸道亲和度高、咳嗽症状明显且时间长等有关,但尚未见共识性定论,不同地区R0不同与人群密度、接触频次或人口规模有关。
家庭传播中易感者感染率约90%,学校传播感染率为50%~80%。
隐性感染者和症状不典型的青少年和成人也具有传染性。
百日咳的发病机制尚不完全清楚,其毒素是重要的致病因素,如百日咳毒素(pertussis toxin,PT)、腺苷酸环化酶可导致黏膜纤毛上皮细胞变性、纤毛麻痹、蛋白合成减少,细胞器破坏;丝状血凝素(filamentous hemagglutinin,FHA)、黏附素和菌毛蛋白可促进附着上皮细胞等。