Minimum Sample Size Proportion on the TI - GEOCITIESws对钛geocitiesws最小样本大小比例
一些常见的统计术语翻译
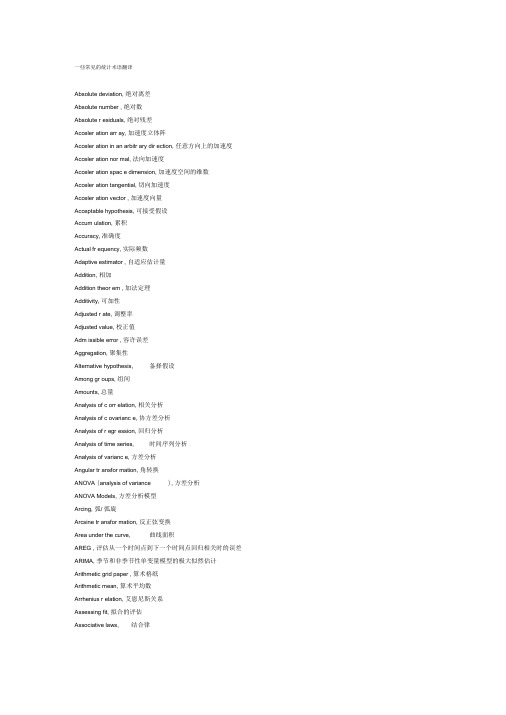
一些常见的统计术语翻译Absolute deviation, 绝对离差Absolute number , 绝对数Absolute r esiduals, 绝对残差Acceler ation arr ay, 加速度立体阵Acceler ation in an arbitr ary dir ection, 任意方向上的加速度Acceler ation nor mal, 法向加速度Acceler ation spac e dimension, 加速度空间的维数Acceler ation tangential, 切向加速度Acceler ation vector , 加速度向量Acceptable hypothesis, 可接受假设Accum ulation, 累积Accuracy, 准确度Actual fr equency, 实际频数Adaptive estimator , 自适应估计量Addition, 相加Addition theor em , 加法定理Additivity, 可加性Adjusted r ate, 调整率Adjusted value, 校正值Adm issible error , 容许误差Aggregation, 聚集性Alternative hypothesis, 备择假设Among gr oups, 组间Amounts, 总量Analysis of c orr elation, 相关分析Analysis of c ovarianc e, 协方差分析Analysis of r egr ession, 回归分析Analysis of time series, 时间序列分析Analysis of varianc e, 方差分析Angular tr ansfor mation, 角转换ANOVA (analysis of variance ), 方差分析ANOVA Models, 方差分析模型Arcing, 弧/ 弧旋Arcsine tr ansfor mation, 反正弦变换Area under the curve, 曲线面积AREG , 评估从一个时间点到下一个时间点回归相关时的误差ARIMA, 季节和非季节性单变量模型的极大似然估计Arithmetic grid paper , 算术格纸Arithmetic mean, 算术平均数Arrhenius r elation, 艾恩尼斯关系Assessing fit, 拟合的评估Associative laws, 结合律Asymmetric distribution, 非对称分布Asymptotic bias, 渐近偏倚Asymptotic efficiency, 渐近效率Asymptotic variance, 渐近方差Attributable risk, 归因危险度Attribute data, 属性资料Attribution, 属性Autoc orrelation, 自相关Autoc orrelation of residuals, 残差的自相关Aver age, 平均数Aver age c onfidenc e interval length, 平均置信区间长度Aver age growth r ate, 平均增长率Bar c hart, 条形图Bar gr aph, 条形图Base period, 基期Bayes' theorem , Bayes 定理Bell-shaped curve, 钟形曲线伯努力分布Ber noulli distribution,Best-trim estimator , 最好切尾估计量Bias, 偏性Binary logistic r egr ession, 二元逻辑斯蒂回归Binomial distribution, 二项分布Bisquare, 双平方Bivariate Corr elate, 二变量相关Bivariate nor mal distribution, 双变量正态分布Bivariate nor mal population, 双变量正态总体Biweight inter val, 双权区间Biweight M-estimator, 双权M 估计量Bloc k, 区组/ 配伍组BMDP(Biomedic al computer pr ograms), BMDP 统计软件包Boxplots, 箱线图/ 箱尾图Breakdown bound, 崩溃界/ 崩溃点Canonical c orrelation, 典型相关Caption, 纵标目Case-c ontrol study , 病例对照研究Categoric al variable, 分类变量Catenary, 悬链线Cauchy distribution, 柯西分布Cause-and-effect r elationship, 因果关系Cell, 单元Censoring, 终检Center of symmetry , 对称中心Centering and sc aling, 中心化和定标Centr al tendency, 集中趋势Centr al value, 中心值CHAID - x 2 Automatic Inter action Detector ,卡方自动交互检测Chanc e, 机遇Chanc e error , 随机误差Chanc e variable, 随机变量Char acteristic equation, 特征方程Char acteristic root, 特征根Char acteristic vector , 特征向量Chebshev criterion of fit, 拟合的切比雪夫准则Chernoff fac es, 切尔诺夫脸谱图Chi-square test, 卡方检验/咒2检验Choleskey dec omposition, 乔洛斯基分解Circle chart, 圆图Class interval, 组距Class mid-value, 组中值Class upper limit, 组上限Classified variable, 分类变量Cluster analysis, 聚类分析Cluster sampling, 整群抽样Code, 代码Coded data, 编码数据Coding, 编码Coefficient of c ontingency, 列联系数Coefficient of deter mination, 决定系数Coefficient of multiple c orr elation, 多重相关系数Coefficient of partial c orrelation, 偏相关系数Coefficient of pr oduction-moment c orrelation, 积差相关系数Coefficient of r ank corr elation, 等级相关系数Coefficient of r egr ession, 回归系数Coefficient of skewness, 偏度系数Coefficient of variation, 变异系数Cohort study, 队列研究Column, 列Column effect, 列效应Column factor , 列因素Combination pool, 合并Combinative table, 组合表Common factor , 共性因子Common regr ession coefficient, 公共回归系数Common value, 共同值Common varianc e, 公共方差Common variation, 公共变异Communality varianc e, 共性方差Compar ability, 可比性Comparison of bathes, 批比较Comparison value, 比较值Compartment model, 分部模型Compassion, 伸缩Complement of an event, 补事件Complete association, 完全正相关Complete dissociation, 完全不相关Complete statistic s, 完备统计量Completely r andomized design, 完全随机化设计Composite event, 联合事件Composite events, 复合事件Concavity, 凹性Conditional expectation, 条件期望Conditional likelihood, 条件似然Conditional pr obability, 条件概率Conditionally linear , 依条件线性Confidenc e interval, 置信区间Confidenc e lim it, 置信限Confidenc e lower lim it, 置信下限Confidenc e upper limit, 置信上限Confir matory Factor Analysis , 验证性因子分析Confir matory research, 证实性实验研究Confounding factor , 混杂因素Conjoint, 联合分析Consistency, 相合性Consistency chec k, 一致性检验Consistent asymptotic ally nor mal estimate, 相合渐近正态估计Consistent estimate, 相合估计Constr ained nonlinear r egr ession, 受约束非线性回归Constr aint, 约束Contam inated distribution, 污染分布Contam inated Gausssian, 污染高斯分布Contam inated nor mal distribution, 污染正态分布Contam ination, 污染Contam ination model, 污染模型Contingency table, 列联表Contour , 边界线Contribution r ate, 贡献率Control, 对照Controlled experiments, 对照实验Conventional depth, 常规深度Convolution, 卷积Corrected factor , 校正因子Corrected mean, 校正均值Correction coefficient, 校正系数Correctness, 正确性Correlation c oefficient, 相关系数Correlation index, 相关指数Correspondenc e, 对应Counting, 计数Counts, 计数/ 频数Covarianc e, 协方差Covariant, 共变Cox Regression, Cox 回归Criteria for fitting, 拟合准则Criteria of least squar es, 最小二乘准则Critic al r atio, 临界比Critic al r egion, 拒绝域Critic al value, 临界值Cr oss-over design, 交叉设计Cr oss-section analysis, 横断面分析Cr oss-section survey, 横断面调查Cr osstabs , 交叉表Cr oss-tabulation table, 复合表Cube r oot, 立方根Cumulative distribution function, 分布函数Cumulative probability, 累计概率Curvatur e, 曲率/ 弯曲Curvatur e, 曲率Curve fit , 曲线拟和Curve fitting, 曲线拟合Curvilinear r egression, 曲线回归Curvilinear r elation, 曲线关系Cut-and-try method, 尝试法Cycle, 周期Cyclist, 周期性D test, D 检验Data acquisition, 资料收集Data bank, 数据库Data c apacity, 数据容量Data deficiencies, 数据缺乏Data handling, 数据处理Data manipulation, 数据处理Data proc essing, 数据处理Data r eduction, 数据缩减Data set, 数据集Data sourc es, 数据来源Data tr ansfor mation, 数据变换Data validity, 数据有效性Data-in, 数据输入Data-out, 数据输出Dead time, 停滞期Degr ee of fr eedom, 自由度Degr ee of pr ecision, 精密度Degr ee of r eliability , 可靠性程度Degr ession, 递减Density function, 密度函数Density of data points,数据点的密度Dependent variable,应变量/ 依变量/ 因变量Dependent variable,因变量Depth, 深度Derivative matrix, 导数矩阵Derivative-fr ee methods, 无导数方法Design, 设计Deter minacy, 确定性Deter minant, 行列式Deter minant, 决定因素Deviation, 离差Deviation from aver age, 离均差Diagnostic plot, 诊断图Dichotomous variable, 二分变量Differential equation,微分方程Direct standardization, 直接标准化法Discr ete variable, 离散型变量DISCRIMINAN T, 判断Discriminant analysis, 判别分析Discriminant c oeffic ient, 判别系数Discriminant function, 判别值Disper sion, 散布/ 分散度Dispr oportional, 不成比例的Dispr oportionate sub-class numbers, 不成比例次级组含量Distribution free, 分布无关性/ 免分布Distribution shape, 分布形状Distribution-free method, 任意分布法Distributive laws, 分配律Distur banc e, 随机扰动项Dose response curve, 剂量反应曲线Double blind method, 双盲法Double blind trial, 双盲试验Double exponential distribution, 双指数分布Double logarithmic, 双对数Downward r ank, 降秩Dual-spac e plot, 对偶空间图DUD, 无导数方法新法Duncan's new multiple r ange method, 新复极差法/DuncanE-LEffect, 实验效应Eigenvalue, 特征值Eigenvector , 特征向量Ellipse, 椭圆Empiric al distribution, 经验分布Empiric al pr obability , 经验概率单位Enumer ation data, 计数资料Equal sun-class number , 相等次级组含量Equally likely , 等可能Equivarianc e, 同变性Error , 误差/ 错误Errorof estimate, 估计误差Error type I, 第一类错误Error type II, 第二类错误Estimand, 被估量Estimated err or mean squares, 估计误差均方Estimated err or sum of squar es, 估计误差平方和Euclidean distanc e,欧式距离Event, 事件Event, 事件Exc eptional data point, 异常数据点Expectation plane, 期望平面Expectation surfac e, 期望曲面Expected values, 期望值Experiment, 实验Experimental sampling, 试验抽样Experimental unit, 试验单位Explanatory variable, 说明变量Explor atory data analysis, 探索性数据分析Explore Summarize, 探索- 摘要Exponential curve, 指数曲线Exponential growth, 指数式增长EXSMOOTH, 指数平滑方法Extended fit, 扩充拟合Extr a par ameter ,附加参数Extr apolation, 外推法Extr eme observation, 末端观测值Extr emes, 极端值/ 极值F distribution, F分布 F test, F 检验Factor , 因素 / 因子Factor analysis, 因子分析Factor Analysis, 因子分析Factor scor e, 因子得分Factorial, 阶乘Factorial design, 析因试验设计False negative, 假阴性False negative error , 假阴性错误 Fam ily of distributions, 分布族 Fam ily of estimator s, 估计量族 Fanning, 扇面Fatality r ate, 病死率Field investigation, 现场调查Field survey , 现场调查Finite population, 有限总体 Finite-sample, 有限样本First derivative, 一阶导数First principal component,First quartile, 第一四分位数Fisher infor mation, 费雪信息量Fitted value, 拟合值Fourth, 四分点Frequency, 频率Frontier point, 界限点Function r elationship, 泛函关系Gaussian distribution, 高斯分布 / 正态分布Gini's mean difference,基尼均差 GLM (Gener al liner models), 通用线性模型Fitting a c urve, 曲线拟合 Fixed base,定基 Fluctuation, 随机起伏 For ec ast, 预测 Four fold table,四格表Fraction blow, 左侧比率Fractional error, 相对误差 Frequency polygon,频数多边图 Gamma distribution, 伽玛分布Gauss increment, 高斯增量Gauss-Newton incr ement, 高斯- 牛顿增量 Gener al census, 全面普查GENLOG (Gener alized liner models), 广义线性模型 Geometric mean,几何平均数 第一主成分Goodness of fit, 拟和优度/ 配合度Gradient of deter m inant, 行列式的梯度Graec o-Latin squar e, 希腊拉丁方Grand mean, 总均值Gross error s, 重大错误Gross-error sensitivity, 大错敏感度Group aver ages, 分组平均Grouped data, 分组资料Guessed mean, 假定平均数Half-life, 半衰期Hampel M-estimators, 汉佩尔M 估计量Happenstanc e, 偶然事件Har monic mean, 调和均数Hazar d function, 风险均数Hazar d r ate, 风险率Heading, 标目Heavy-tailed distribution, 重尾分布Hessian arr ay, 海森立体阵Heterogeneity , 不同质Heterogeneity of variance, 方差不齐Hier archic al classific ation, 组内分组Hier archic al clustering method, 系统聚类法High-lever age point, 高杠杆率点HILOGLINEAR, 多维列联表的层次对数线性模型Hinge, 折叶点Histogr am, 直方图Historical c ohort study, 历史性队列研究Holes, 空洞HOMALS, 多重响应分析Homogeneity of varianc e, 方差齐性Homogeneity test, 齐性检验Huber M-estimators, 休伯M 估计量Hyper bola, 双曲线Hypothesis testing, 假设检验Hypothetic al universe, 假设总体Impossible event, 不可能事件Independenc e, 独立性Independent variable, 自变量Index, 指标/ 指数Indir ect standardization, 间接标准化法Individual, 个体Infer enc e band, 推断带Infinite population, 无限总体Infinitely gr eat, 无穷大Infinitely small, 无穷小Influence curve, 影响曲线Intercept, 截距Interpolation, 内插法Invarianc e, 不变性Inverse matrix, 逆矩阵Inverse sine tr ansfor mation, 反正弦变换Iter ation, 迭代Jac obian deter m inant, 雅可比行列式Joint distribution function,分布函数 Joint probability, 联合概率Joint probability distribution,联合概率分布 K means method, 逐步聚类法Kaplan-Meier , 评估事件的时间长度Kaplan-Merier c hart, Kaplan-Merier图 Kendall's r ank c orrelation, Kendall等级相关 Kinetic, 动力学Kolmogor ov-Smirnove test, 柯尔莫哥洛夫 - 斯米尔诺夫检验Kruskal and Wallis test, Kr uskal 及 Wallis 检验 / 多样本的秩和检验 /H 检验 Kurtosis, 峰度Lac k of fit, 失拟Ladder of powers, 幂阶梯Lag, 滞后Lar ge sample, 大样本Lar ge sample test, 大样本检验Latin squar e, 拉丁方Latin squar e design, 拉丁方设计Leakage, 泄漏Least favor able c onfigur ation, 最不利构形Least favor able distribution, 最不利分布Least signific ant differ enc e, 最小显著差法Least squar e method, 最小二乘法Least-absolute-r esiduals estimates, Least-absolute-r esiduals fit, 最小绝对残差拟合 Least-absolute-r esiduals line, 最小绝对残差线 Legend, 图例L-estimator , L 估计量Infor mation capacity, 信息容量 Initial condition,初始条件 Initial estimate,初始估计值 Initial level,最初水平 Interaction,交互作用 Interaction terms, 交互作用项Interquartile range,四分位距 Interval estimation,区间估计 Intervals of equal probability, 等概率区间 Intrinsic c urvature,固有曲率Inverse probability,逆概率最小绝对残差估计L-estimator of loc ation, 位置L 估计量L-estimator of sc ale, 尺度L 估计量Level, 水平Life expectanc e, 预期期望寿命Life table, 寿命表Life table method, 生命表法Light-tailed distribution, 轻尾分布似然函数Likelihood function,似然比Likelihood r atio,line gr aph, 线图直线相关Linear corr elation,线性方程Linear equation,Linear pr ogr amm ing, 线性规划直线回归Linear regr ession,线性回归Linear Regression,Linear trend, 线性趋势Loading, 载荷Loc ation and sc ale equivarianc e, 位置尺度同变性Loc ation equivarianc e, 位置同变性Loc ation invarianc e, 位置不变性Loc ation sc ale family, 位置尺度族Log r ank test, 时序检验Logarithm ic curve, 对数曲线Logarithm ic nor mal distribution, 对数正态分布Logarithm ic sc ale, 对数尺度Logarithm ic tr ansfor mation, 对数变换Logic chec k, 逻辑检查Logistic distribution, 逻辑斯特分布Logit tr ansfor mation, Logit 转换LOGLINEAR, 多维列联表通用模型Lognor mal distribution, 对数正态分布Lost function, 损失函数Low corr elation, 低度相关Lower lim it, 下限Lowest-attained varianc e, 最小可达方差LSD, 最小显著差法的简称Lur king variable, 潜在变量M-RMain effect, 主效应Major heading, 主辞标目Marginal density function, 边缘密度函数Marginal pr obability, 边缘概率Marginal pr obability distribution, 边缘概率分布Matched data, 配对资料Matched distribution, 匹配过分布Matching of distribution, 分布的匹配Matching of tr ansfor mation, 变换的匹配Mathematic al expectation, 数学期望Mathematic al model, 数学模型Maximum L-estimator , 极大极小L 估计量Maximum likelihood method, 最大似然法Mean, 均数Mean squar es between groups, 组间均方Mean squar es within gr oup, 组内均方Means (Compar e means), 均值- 均值比较Median, 中位数Median effective dose, 半数效量Median lethal dose, 半数致死量Median polish, 中位数平滑Median test, 中位数检验Minimal sufficient statistic, 最小充分统计量Minimum distanc e estimation, 最小距离估计Minimum effective dose, 最小有效量Minimum lethal dose, 最小致死量Minimum varianc e estimator , 最小方差估计量MIN ITAB, 统计软件包Minor heading, 宾词标目Missing data, 缺失值Model specific ation, 模型的确定Modeling Statistic s , 模型统计Models for outliers, 离群值模型Modifying the model, 模型的修正Modulus of c ontinuity , 连续性模Mor bidity , 发病率Most favor able c onfigur ation, 最有利构形Multidimensional Sc aling (ASCAL), 多维尺度/ 多维标度Multinomial Logistic Regression , 多项逻辑斯蒂回归Multiple c omparison, 多重比较Multiple c orr elation , 复相关Multiple c ovarianc e, 多元协方差Multiple linear r egr ession, 多元线性回归Multiple r esponse , 多重选项Multiple solutions, 多解Multiplic ation theor em , 乘法定理Multir esponse, 多元响应Multi-stage sampling, 多阶段抽样Multivariate T distribution, 多元T 分布Mutual exclusive, 互不相容Mutual independenc e, 互相独立Natur al boundary, 自然边界Natur al dead, 自然死亡Natur al zer o, 自然零Negative c orr elation, 负相关Negative linear corr elation, 负线性相关Negatively skew ed, 负偏Newman-Keuls method, q 检验NK method, q 检验No statistic al signific ance, 无统计意义Nom inal variable, 名义变量Nonc onstancy of variability, 变异的非定常性Nonlinear regr ession, 非线性相关Nonpar ametric statistics, 非参数统计Nonpar ametric test, 非参数检验Nonpar ametric tests, 非参数检验Normal deviate, 正态离差Normal distribution, 正态分布Normal equation, 正规方程组Normal r anges, 正常范围Normal value, 正常值Nuisanc e par ameter , 多余参数/ 讨厌参数Null hypothesis, 无效假设Numeric al variable, 数值变量Objective function, 目标函数观察单位Observation unit,观察值Observed value,One sided test, 单侧检验One-way analysis of varianc e, 单因素方差分析Oneway ANOVA , 单因素方差分析Open sequential trial, 开放型序贯设计Optrim, 优切尾Optrim efficiency, 优切尾效率Order statistic s, 顺序统计量Or dered categories, 有序分类Or dinal logistic r egr ession , 序数逻辑斯蒂回归有序变量Or dinal variable,正交基Orthogonal basis,Orthogonal design, 正交试验设计Orthogonality c onditions, 正交条件ORTHOPLAN, 正交设计Outlier cutoffs, 离群值截断点Outlier s, 极端值OVE RALS , 多组变量的非线性正规相关Over shoot, 迭代过度Pair ed design, 配对设计Pair ed sample, 配对样本Pairwise slopes, 成对斜率Par abola, 抛物线Par allel tests, 平行试验Par ameter , 参数Par ametric statistic s, 参数统计Par ametric test, 参数检验Partial c orrelation, 偏相关Partial r egression, 偏回归Partial sorting, 偏排序Partials r esiduals, 偏残差Patter n, 模式Pear son curves, 皮尔逊曲线Peeling, 退层Perc ent bar gr aph, 百分条形图Perc entage, 百分比Perc entile, 百分位数Perc entile curves, 百分位曲线Periodicity , 周期性Per mutation, 排列P-estimator , P 估计量Pie graph, 饼图Pitman estimator , 皮特曼估计量Pivot, 枢轴量Planar , 平坦Planar assumption, 平面的假设PLANCARDS, 生成试验的计划卡Point estimation, 点估计Poisson distribution, 泊松分布Polishing, 平滑Polled standar d deviation, 合并标准差Polled varianc e, 合并方差Polygon, 多边图Polynomial, 多项式Polynomial c urve, 多项式曲线Population, 总体Population attributable risk,人群归因危险度Qualitative classific ation, 属性分类Qualitative method, 定性方法Quantile-quantile plot, Quantitative analysis, Quartile, 四分位数Quic k Cluster , 快速聚类Radix sort, 基数排序Random alloc ation, 随机化分组Random bloc ks design, 随机区组设计Random event, 随机事件Random ization, 随机化Range, 极差/ 全距Rank c orr elation, 等级相关Rank sum test, 秩和检验Rank test, 秩检验 Ranked data, 等级资料Rate, 比率Ratio, 比例 Positive c orrelation, 正相关Positively skewed, 正偏Posterior distribution, 后验分布Power of a test, 检验效能 Precision,精密度Predicted value, 预测值Preliminary analysis, 预备性分析Principal c omponent analysis, 主成分分析Prior distribution, 先验分布 Prior pr obability, Probabilistic model, probability, 概率Probability density Product moment, 先验概率概率模型, 概率密度 乘积矩 / 协方差Profile tr ace, 截面迹图Proportion, 比/ 构成比Proportion alloc ation in str atified random sampling, Proportionate, 成比例Proportionate sub-class numbers, 成比例次级组含量Prospective study , 前瞻性调查Proximities, 亲近性Pseudo F test, 近似 F 检验Pseudo model, 近似模型Pseudosigma, 伪标准差Purposive sampling, 有目的抽样QR dec omposition, QR 分解Quadratic approximation, 二次近似 按比例分层随机抽样分位数-分位数图 /Q-Q 图 定量分析Raw data, 原始资料Raw residual, 原始残差Rayleigh's test, 雷氏检验Rayleigh's Z, 雷氏Z 值Recipr ocal, 倒数Recipr ocal tr ansfor mation, 倒数变换Rec or ding, 记录Redesc ending estimators, 回降估计量Reducing dimensions, 降维Re-expression, 重新表达Refer enc e set, 标准组Region of acc eptanc e, 接受域Regr ession coefficient, 回归系数Regr ession sum of squar e, 回归平方和Rej ection point, 拒绝点Relative disper sion, 相对离散度Relative number , 相对数Reliability , 可靠性Repar ametrization, 重新设置参数Replication, 重复Report Summar ies, 报告摘要Residual sum of squar e, 剩余平方和Resistanc e, 耐抗性Resistant line, 耐抗线Resistant technique, 耐抗技术R-estimator of location, 位置R 估计量R-estimator of sc ale, 尺度R 估计量Retr ospective study, 回顾性调查Ridge tr ace, 岭迹Ridit analysis, Ridit 分析Rotation, 旋转Rounding, 舍入Row, 行Row effects, 行效应Row factor , 行因素RXC table, RXC 表S-ZSample, 样本Sample r egression c oefficient, 样本回归系数Sample size, 样本量Sample standar d deviation, 样本标准差Sampling error , 抽样误差SAS(Statistical analysis system ), SAS Scale, 尺度/ 量表Scatter diagr am, 散点图统计软件包Schematic plot, 示意图/ 简图Scor e test, 计分检验Screening, 筛检SEASON, 季节分析Sec ond derivative, 二阶导数Sec ond principal c omponent, 第二主成分SEM (Structur al equation modeling), 结构化方程模型Semi-logarithm ic gr aph, 半对数图Semi-logarithm ic paper , 半对数格纸Sensitivity c urve, 敏感度曲线Sequential analysis,贯序分析Sequential data set, 顺序数据集Sequential design, 贯序设计Sequential method, 贯序法Sequential test, 贯序检验法Serial tests, 系列试验Short-c ut method, 简捷法Sigmoid curve, S形曲线Sign function, 正负号函数Sign test, 符号检验Signed r ank, 符号秩Signific anc e test, 显著性检验Signific ant figur e, 有效数字Sim ple cluster sampling, 简单整群抽样Sim ple c orrelation, 简单相关Sim ple r andom sampling, 简单随机抽样Sim ple r egr ession, 简单回归simple table, 简单表Sine estimator , 正弦估计量Single-valued estimate, 单值估计Singular matrix, 奇异矩阵Skewed distribution, 偏斜分布Skewness, 偏度Slash distribution, 斜线分布Slope, 斜率Smirnov test, 斯米尔诺夫检验Source of variation, 变异来源Spear man r ank c orrelation, 斯皮尔曼等级相关Specific factor , 特殊因子Specific factor varianc e, 特殊因子方差Spectr a , 频谱Spherical distribution, 球型正态分布Spr ead, 展布SPSS(Statistical pac kage for the social scienc e), SPSS Spurious c orr elation, 假性相关Square root tr ansfor mation, 平方根变换Stabilizing variance, 稳定方差Standard deviation, 标准差Standard error , 标准误Standard error of differ ence, 差别的标准误Standard error of estimate, 标准估计误差Standard error of r ate, 率的标准误Standard nor mal distribution, 标准正态分布Standardization, 标准化Starting value, 起始值Statistic, 统计量Statistical c ontrol, 统计控制Statistical gr aph, 统计图Statistical inferenc e, 统计推断Statistical table, 统计表Steepest desc ent, 最速下降法Stem and leaf display, 茎叶图Step factor , 步长因子Stepwise r egr ession, 逐步回归Stor age, 存Strata, 层(复数)Stratified sampling, 分层抽样Stratified sampling, 分层抽样Strength, 强度Stringency , 严密性Structur al r elationship, 结构关系Studentized r esidual, 学生化残差/t 化残差Sub-class number s, 次级组含量Subdividing, 分割Sufficient statistic, 充分统计量Sum of pr oducts, 积和Sum of squares, 离差平方和Sur e event, 必然事件Survey, 调查Survival, 生存分析统计软件包Sum of squares about regr Sum of squares between gr Sum of squares of partial r ession, 回归平方和oups, 组间平方和egression, 偏回归平方和Survival r ate, 生存率Suspended r oot gr am, 悬吊根图Symmetry, 对称Systematic err or, 系统误差Systematic sampling, 系统抽样Tags, 标签Tail ar ea, 尾部面积Tail length, 尾长Tail weight, 尾重Tangent line, 切线Target distribution, 目标分布Taylor series, 泰勒级数Tendency of dispersion, 离散趋势Testing of hypotheses, 假设检验Theor etical frequency , 理论频数Time series, 时间序列Toler anc e interval, 容忍区间Toler anc e lower lim it, 容忍下限Toler anc e upper lim it, 容忍上限Torsion, 扰率Total sum of squar e, 总平方和Total variation, 总变异Transfor mation, 转换Treatment, 处理Trend, 趋势Trend of perc entage, 百分比趋势Trial, 试验Trial and err or method, 试错法Tuning c onstant, 细调常数Two sided test, 双向检验Two-stage least squar es, 二阶最小平方Two-stage sampling, 二阶段抽样Two-tailed test, 双侧检验Two-way analysis of varianc e, 双因素方差分析Two-way table, 双向表Type I err or, 一类错误/ a错误Type II err or,二类错误/ B错误UMVU, 方差一致最小无偏估计简称Unbiased estimate, 无偏估计Unc onstrained nonlinear r egr ession , 无约束非线性回归Unequal subclass number , 不等次级组含量Ungr ouped data, 不分组资料Unifor m coor dinate, 均匀坐标Unifor m distribution, 均匀分布Unifor m ly m inimum varianc e unbiased estimate, 方差一致最小无偏估计Unit, 单元Unor der ed categories, 无序分类Upper lim it, 上限Upwar d r ank, 升秩Vague conc ept, 模糊概念Validity , 有效性W test, W 检验W-estimation, W 估计量W-estimation of location,位置 W 估计量Width, 宽度 Wilcoxon paired test, 威斯康星配对法 / 配对符号秩和检验 Wild point, 野点 / 狂点Wild value, 野值 / 狂值Winsorized mean, 缩尾均值Withdr aw, 失访Youden's index, 尤登指数Z test, Z 检验Zer o corr elation, 零相关Z-tr ansfor mation, Z 变换 VARCOMP (Varianc e c omponent estimation), 方差元素估计 Variability , 变异性 Variable,变量 Varianc e,方差 Variation, 变异Varimax orthogonal rotation, 方差最大正交旋转 Volume of distribution,容积Weibull distribution, 威布尔分布 Weight, 权数Weighted Chi-squar e test, 加权卡方检验 /Coc hr an 检验 Weighted linear regression method, 加权直线回归 Weighted mean, 加权平均数Weighted mean squar Weighted sum of squarWeighting coefficient,Weighting method,e, 加权平均方差e, 加权平方和 权重系数 加权法。
Sample Size Determination
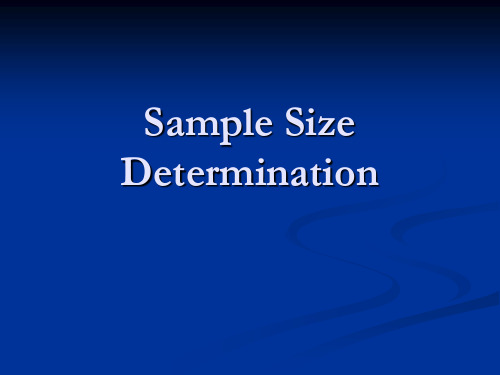
Iቤተ መጻሕፍቲ ባይዱtroduction
Freiman JA, NEJM, 1978;299:690-4
Reviewed the power of 71 published RCTs which had failed to detect a difference
Found that 67 could have missed a 25% therapeutic improvement
Factors That Influence Sample Size Calculations
Is the F/U long enough to be of any clinical relevance Desired level of significance Desired power One or two-tailed test Any explanation for the possible ranges or variations in outcome that is
Factors That Influence Sample Size Calculations
Research subjects
Target population Inclusion & exclusion criteria Baseline risk Pt. compliance rate Pt. drop-out rate
considerations) Adjustments (accounting for potential dropouts or
effect of covariates)
Introduction
Consequences of getting it wrong
非随机抽样和随机抽样的比较
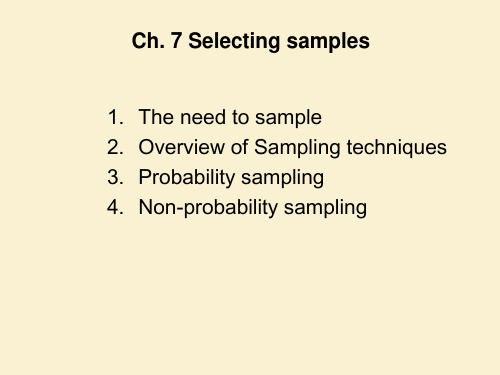
Population, sampling frame, samples
Select appropriate sampling technique
• Five main sampling techniques (1) simple random (2) systematic (3) stratified random (4) cluster (5) multi-stage
• Non-probability or judgemental sampling - Probability of each case from பைடு நூலகம்he population is unknown - Impossible to answer research questions or to address objectives that require you to make statistical inferences about the characteristics of the population - (case study strategy)
(1) identify a suitable sampling frame based on your research questions or objectives
(2) decide on a suitable sample size
(3) select the most appropriate sampling technique and select the sample
• Sample size the number of cases used for the research analysis
TI-83 84 Guide for Introductory Statistics说明书
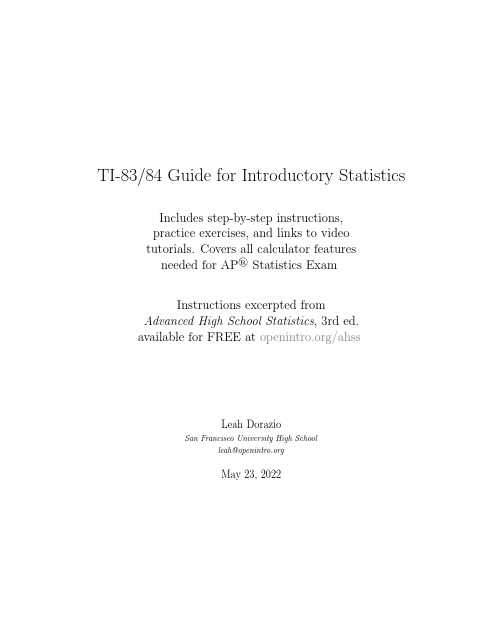
TI-83/84Guide for Introductory Statistics Includes step-by-step instructions,practice exercises,and links to videotutorials.Covers all calculator featuresneeded for AP®Statistics ExamInstructions excerpted fromAdvanced High School Statistics,3rd ed.available for FREE at /ahssLeah DorazioSan Francisco University High School******************May23,2022Copyright©2022OpenIntro,Inc.Third Edition.Updated:May23,2022.This guide is available under a Creative Commons license.Visit for a free PDF,to download the sourcefiles,or for more information about the license.AP®is a trademark registered and owned by the College Board,which was not involved in the production of, and does not endorse,this product.2ContentsSummarizing data5 Entering data (5)Calculating summary statistics (5)Drawing a box plot (6)What to do if you cannotfind L1or another list (6)Practice exercises (7)Finding area under the normal curve (7)Find a Z-score that corresponds to a percentile (8)Practice exercises (8)Probability10 Computing the binomial coefficient (10)Computing the binomial formula (11)Computing a cumulative binomial probability (11)Practice exercises (11)Inference for categorical data13 1-proportion Z-interval and Z-test (13)Practice exercises (14)2-proportion Z-interval and Z-test (15)Practice exercises (16)Finding area unders the Chi-square curve (17)Chi-square goodness offit test (17)Chi-square test for two-way tables (18)Practice exercises (19)Inference for numerical data20 1-sample t-test and t-interval (20)Practice exercises (21)1-sample t-test and t-interval with paired data (22)Practice exercises (23)2-sample t-test and t-interval (24)Practice exercises (26)Introduction to linear regression27 Finding a,b,R2,and r for a linear model (27)What to do if r2and r do not show up on a TI-83/84 (28)What to do if a TI-83/84returns:ERR:DIM MISMATCH (28)34CONTENTS Practice exercises (29)t-test and t-interval for the slope of a regression line (30)Summarizing dataEntering dataTI-83/84:ENTERING DATAThefirst step in summarizing data or making a graph is to enter the data set into a e STAT,Edit.1.Press STAT.2.Choose1:Edit.3.Enter data into L1or another list.Calculating summary statisticsTI-84:Usestandard1.2.3.4.5.6.7.TI-83:5Calculating the summary statistics will return the following information.It will be neces-sary to hit the down arrow to see all of the summary statistics.¯x Mean n Sample size or#of data points Σx Sum of all the data values minX MinimumΣx2Sum of all the squared data values Q1First quartileSx Sample standard deviation Med Medianσx Population standard deviation maxX MaximumDrawing a box plotTI-83/84:1.2.3.4.5.6.7.What to do if you cannotfind L1or another listTI-83/84:Restore1.2.3.Practice exercisesGUIDED PRACTICE0.1Enter the following10data points into a list on a calculator:5,8,1,19,3,1,11,18,20,5Find the summary statistics and make a box plot of the data.1Finding area under the normal curveTI-84:FINDING AREA UNDER THE NORMAL CURVEUse2ND VARS,normalcdf tofind an area/proportion/probability to the left or right of a Z-score or between two Z-scores.1.Choose2ND VARS(i.e.DISTR).2.Choose2:normalcdf.3.Enter the Z-scores that correspond to the lower(left)and upper(right)bounds.4.Leaveµas0andσas1.5.Down arrow,choose Paste,and hit ENTER.TI-83:Do steps1-2,then enter the lower bound and upper bound separated by a comma,e.g.normalcdf(2,5),and hit ENTER.1The summary statistics should be¯x=9.1,Sx=7.475,Q1=3,etc.The box plot should be as follows.Find a Z-score that corresponds to a percentileTI-84:FIND A Z-SCORE THAT CORRESPONDS TO A PERCENTILEUse2ND VARS,invNorm tofind the Z-score that corresponds to a given percentile.1.Choose2ND VARS(i.e.DISTR).2.Choose3:invNorm.3.Let Area be the percentile as a decimal(the area to the left of desired Z-score).4.Leaveµas0andσas1.5.Down arrow,choose Paste,and hit ENTER.TI-83:Do steps1-2,then enter the percentile as a decimal,e.g.invNorm(.40),then hit ENTER.Practice exercisesGUIDED PRACTICE0.3Find the area under the normal curve to right of Z=2.32normalcdf gives the result without drawing the graph.To draw the graph,do2nd VARS,DRAW, 1:ShadeNorm.However,beware of errors caused by other plots that might interfere with this plot.3Now we want to shade to the right.Therefore our lower bound will be2and the upper bound will be5(or a number bigger than5)to get P(Z>2)=0.023.GUIDED PRACTICE 0.4Find the area under the normal curve between -1.5and 1.5.4GUIDED PRACTICE 0.6Find the Z-score such that 20percent of the area is to the right of that Z-score.5GUIDED PRACTICE 0.8Approximately what percent of these babies weighed greater than 10pounds?74Herewe are given both the lower and the upper bound.Lower bound is -1.5and upper bound is 1.5.The area under the normal curve between -1.5and 1.5=P (−1.5<Z <1.5)=0.866.5If 20%of the area is the right,then 80%of the area is to the left.Letting area be 0.80,we get Z =0.841./1471-2393/8/57Z =10−7.441.33=ing a lower bound of 2and an upper bound of 5,we get P (Z >1.925)=0.027.Approximately 2.7%of the newborns weighed over 10pounds.Probability and probability distributionsComputing the binomial coefficientTI-83/84:Usesame1.2.3.4.5.6.Example:10Computing the binomial formulaTI-84:Useindependent1.2.3.4.5.6.TI-83:binompdf(n,Computing a cumulative binomial probabilityTI-84:Userences1.2.3.4.5.6.TI-83:follows:Practice exercisesGUIDED PRACTICE0.9Find the number of ways of arranging3blue marbles and2red marbles.8 8Here n=5and k=3.Doing5nCr3gives the number of combinations as10.GUIDED PRACTICE0.10There are13marbles in a bag.4are blue and9are red.Randomly draw5marbles with replacement.Find the probability you get exactly3blue marbles.9GUIDED PRACTICE0.11There are13marbles in a bag.4are blue and9are red.Randomly draw5marbles withreplacement.Find the probability you get at most3blue marbles(i.e.less than or equal to3blue marbles).109Here,n=5,p=4/13,and k=3,so set trials=5,p=4/13and x value=3.The probability is0.1396.10Similarly,set trials=5,p=4/13and x value=3.The cumulative probability is0.9662.Inference for categorical data 1-proportion Z-interval and Z-testTI-83/84:13TI-83/84:Use1.2.3.4.5.6.7.8.Practice exercisesGUIDED PRACTICE0.12Using a calculator,evaluate the confidence interval from the example on intelligent life.Recall that we wanted tofind a95%confidence interval for the proportion of U.S.adults who think there is intelligent life on other planets.The sample percent was68%and the sample size was1,033.11GUIDED PRACTICE0.13Using a calculator,find the test statistic and p-value for the example on nuclear energy.Recall that we were looking for evidence that more than half of U.S.adults oppose nuclear energy.The sample percent was54%,and the sample size was1019.1211Navigate to1-PropZInt on the calculator.Tofind x,the number of yes responses in the sample,we multiply the sample proportion by the sample size.Here0.68×1033=702.44.We must round this to an integer,so we use x=702.Also,n=1033and C-Level=0.95.The95%confidence interval is(0.651, 0.708).12Navigate to1-PropZTest on the calculator.Let p0=0.5.Tofind x,do0.54×1019=550.26.This needs to be an integer,so round to the closest integer.Here x=550.Also,n=1019.We are looking for evidence that greater than half oppose,so choose>p0.When we do Calculate,we get the test statistic: Z=2.64and the p-value:p=0.006.2-proportion Z-interval and Z-testTI-83/84:TI-83/84:Practice exercisesGUIDED PRACTICE0.14A quality control engineer collects a sample of gears,examining1000gears from eachcompany andfinds that879gears pass inspection from the current supplier and958pass inspection from the prospective e a calculator tofind a95%confidence interval for the difference(current−prospective)in the proportion that would pass inspection.1313Navigate to2-PropZInt on the calculator.Let x1=879,n1=1000,x2=958,and n2=1000.C-Level is.95.This should lead to an interval of(-0.1027,-0.0553),which matches what we found previously.14Navigate to2-PropZTest on the calculator.Correctly going through the calculator steps should lead to a solution with the test statistic z=-2.977and the p-value p=0.00145.These two values match our calculated values from the previous example to within rounding error.The pooled proportion is given as ^p=0.0133.Note:values for x1and x2were given in the table.If,instead,proportions are given,find x1 and x2by multiplying the proportions by the sample sizes and rounding the result to an integer.Finding areas under the chi-square curveTI-84:Chi-square goodness offit testTI-84:Use1.2.3.4.5.6.7.TI-83:manually,L3Chi-square test for two-way tablesTI-83/84:ENTERING DATA INTO A TWO-WAY TABLE1.Hit2ND x−1(i.e.MATRIX).2.Right arrow to EDIT.3.Hit1or ENTER to select matrix A.4.Enter the dimensions by typing#rows,ENTER,#columns,ENTER.5.Enter the data from the two-way table.TI-83/84:CHI-SQUARE TEST OF HOMOGENEITY AND INDEPENDENCE Use STAT,TESTS,χ2-Test.1.First enter two-way table data as described in the previous box.2.Choose STAT.3.Right arrow to TESTS.4.Down arrow and choose C:χ2-Test.5.Down arrow,choose Calculate,and hit ENTER,which returnsχ2chi-square test statisticp p-valuedf degrees of freedomPractice exercisesGUIDED PRACTICE0.16Use a calculator tofind the upper tail area for the the chi-square distribution with5degrees of freedom and aχ2=5.1.1515Use a lower bound of5.1,an upper bound of1000,and d f=5.The upper tail area is0.4038.16Enter the observed counts into L1and the expected counts into L2.the GOF test.Make sure that Observed:is L1and Expected:is L2.Let df:be5.You shouldfind thatχ2=17.36and p-value=0.004.17First create a2×3matrix with the data.Thefinal summaries should beχ2=106.4,p-value isp=8.06×10−24≈0,and df=2.Below is the matrix of expected values:Obama Congr.Dem.Congr.Rep.Approve731.59693.45693.96Disapprove726.41688.55689.04Inference for numerical data 1-sample t-test and t-intervalTI-83/84:20TI-83/84:Use1.2.3.4.5.6.Practice exercisesGUIDED PRACTICE0.19The average time for all runners whofinished the Cherry Blossom Run in2006was93.3 minutes.In2017,the average time for100randomly selected participants was97.3,witha standard deviation e a calculator tofind the T-statistic and p-value for the appropriate test to see if the average time for the participants in2017is different than it was in2006.18GUIDED PRACTICE0.20Use a calculator tofind a95%confidence interval for the mean mercury content in croakerwhitefish(Pacific).The sample size was15,and the sample mean and standard deviation were computed as0.287and0.069ppm(parts per million),respectively.1918Navigate to T-Test.Letµ0be93.3.¯x is97.3,S x is17.0,and n=100.Choose=to correspond to H A.We get t=2.353and the p-value p=0.021.The d f=100−1=99.19Navigate to TInterval.We do not have all the data,so choose Stats.Enter¯x and Sx.Note:Sx is the sample standard deviation(0.069),not the SE of the sample mean.Let n=15and C-Level=0.95. This should give the interval(0.249,0.325).The d f=15−1=141-sample t-test and t-interval with paired dataTI-83/84:1-SAMPLE T-TEST WITH PAIRED DATAUse STAT,TESTS,T-Test.1.Choose STAT.2.Right arrow to TESTS.3.Down arrow and choose2:T-Test.4.Choose Data if you have all the data or Stats if you have the mean and standarddeviation.5.Letµ0be the null or hypothesized value ofµdiff.•If you choose Data,let List be L3or the list in which you entered thedifferences(don’t forget to enter the differences!)and let Freq be1.•If you choose Stats,enter the mean,SD,and sample size of the differences.6.Choose=,<,or>to correspond to H A.7.Choose Calculate and hit ENTER,which returns:t t statisticp p-value¯x the sample mean of the differencesSx the sample SD of the differencesn the sample size of the differencesPractice exercisesGUIDED PRACTICE0.22Use the data in the table above tofind the test statistic and p-value for a test of H0:µdiff=0versus H A:µdiff=0.2120Navigate to TInterval.We do not have all the data,so choose Stats.Enter¯x=3.58and Sx=13.42. Let n=68and C-Level=0.95.This should give the interval(0.332,6.828).The intervals are equivalent when rounded to two decimal places.21Navigate to T-Test.We do not have all the data,so choose Stats.Enterµ0=0,¯x=3.58,Sx= 13.42,n=68,and choose=µ0since the alternative hypothesis is two-sided.This should give the interval t=2.2and p=0.031.2-sample t-test and t-intervalTI-83/84:2-SAMPLE T-TESTUse STAT,TESTS,2-SampTTest.1.Choose STAT.2.Right arrow to TESTS.3.Choose4:2-SampTTest.4.Choose Data if you have all the data or Stats if you have the means and standarddeviations.•If you choose Data,let List1be L1or the list that contains sample1andlet List2be L2or the list that contains sample2(don’t forget to enter thedata!).Let Freq1and Freq2be1.•If you choose Stats,enter the mean,SD,and sample size for sample1andfor sample25.Choose=,<,or>to correspond to H A.6.Let Pooled be NO.7.Choose Calculate and hit ENTER,which returns:t t statistic Sx1SD of sample1p p-value Sx2SD of sample2df degrees of freedom n1size of sample1¯x1mean of sample1n2size of sample2¯x2mean of sample2TI-83/84: Use1.2.3.4.5.6.Practice exercises22Navigate to2-SampTTest.Because we have the summary statistics rather than all of the data,choose Stats.Let¯x1=3.50,Sx1=5.17,n1=9,¯x2=-4.33,Sx2=2.76,and n2=9.We get t=4.01,and the p-value p=8.4×10−4=0.00084.The degrees of freedom for the test is df=12.2.23Navigate to2-SampTInt.Because we have the summary statistics rather than all of the data,choose Stats.Let¯x1=79.41,Sx1=14,n1=30,¯x2=74.1,Sx2=20,and n2=30.The interval is(−3.6,14.2)with d f=51.9.Introduction to linear regression Finding a,b,R2,and r for a linear modelR,AND r FOR A LINEAR MODELTI-84:FINDING a,b,2Use STAT,CALC,LinReg(a+bx).1.Choose STAT.2.Right arrow to CALC.3.Down arrow and choose8:LinReg(a+bx).•Caution:choosing4:LinReg(ax+b)will reverse a and b.4.Let Xlist be L1and Ylist be L2(don’t forget to enter the x and y values in L1and L2before doing this calculation).5.Leave FreqList blank.6.Leave Store RegEQ blank.7.Choose Calculate and hit ENTER,which returns:a a,the y-intercept of the bestfit lineb b,the slope of the bestfit liner2R2,the explained variancer r,the correlation coefficientTI-83:Do steps1-3,then enter the x list and y list separated by a comma,e.g. LinReg(a+bx)L1,L2,then hit ENTER.27What to do if r2and r do not show up on a TI-83/84WHAT TO DO IF2r AND r DO NOT SHOW UP ON A TI-83/84If r2and r do now show up when doing STAT,CALC,LinReg,the diagnostics must be turned on.This only needs to be once and the diagnostics will remain on.1.Hit2ND0(i.e.CATALOG).2.Scroll down until the arrow points at DiagnosticOn.3.Hit ENTER and ENTER again.The screen should now say:DiagnosticOnDoneWhat to do if a TI-83/84returns:ERR:DIM MIS-MATCHPractice exercisesGUIDED PRACTICE0.25The data set loan50,introduced in Chapter1,contains information on randomly sampledloans offered through the Lending Club.A subset of the data matrix is shown in Figure1. Use a calculator tofind the equation of the least squares regression line for predicting loan amount from total income.24total income loan amount159********260000600037500025000475000600052540002500066700064007288003000Figure1:Sample of data from loan50.24a=11121and b=0.0043,thereforeˆy=11121+0.0043x.t-test and t-interval for the slope of a regression lineTI-83/84:TI-84:。
常用统计学英文名词
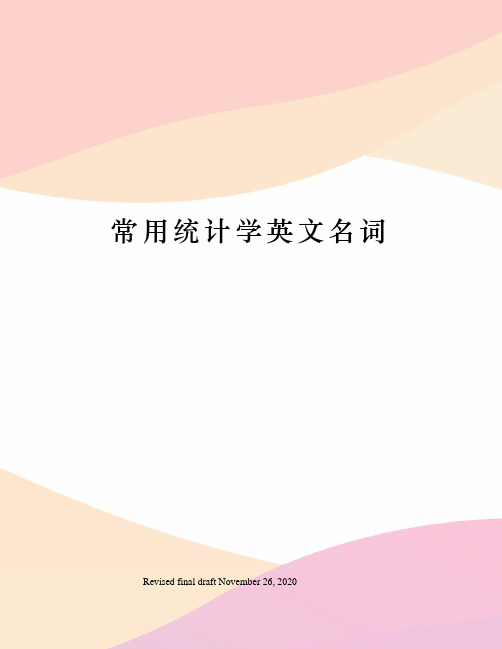
常用统计学英文名词 Revised final draft November 26, 2020常用统计学英文名词(英汉对照)Absolute deviation?绝对离差Absolute number 绝对数Absolute residuals 绝对残差Acceleration normal 法向加速度Acceleration space dimension 加速度空间的维数Acceleration tangential 切向加速度Acceleration vector 加速度向量Acceptable hypothesis 可接受假设Accumulation累积Accuracy 准确度Actual frequency实际频数Actual value 实际数Adaptive estimator自适应估计量Addition 相加Addition theorem 加法定理Additivity 可加性Adjusted rate 调整率Adjusted value 校正值Admissible error 容许误差Alternative hypothesis 备择假设Among groups 组间Amounts总量Analysis of correlation相关分析Analysis of covariance协方差分析Analysis of regression 回归分析Analysis of time series 时间序列分析Analysis of variance方差分析Angular transformation 角转换ANOVA(analysis of variance)方差分析ANOVA Models 方差分析模型Arcing弧/弧旋Arcsine transformation 反正弦变换Area under the curve曲线面积Arithmetic grid paper 算术格纸Arithmetic mean 算术平均数Assessing fit拟合的评估Associative laws 结合律Asymmetric distribution非对称分布Asymptotic bias渐近偏倚Asymptotic efficiency渐近效率Asymptotic variance 渐近方差Attributable risk 归因危险度Attribute data 属性资料Attribution属性Autocorrelation 自相关Autocorrelation of residuals 残差的自相关Average平均数Average confidence interval length平均置信区间长度Average growth rate 平均增长率Bar chart 条形图Bar graph 条形图Base period基期Bayes' theorem Bayes定理Bell-shaped curve 钟形曲线Bernoulli distribution 伯努力分布Best-trim estimator 最好切尾估计量Bias 偏性Binary logistic regression 二元逻辑斯蒂回归Binomial distribution 二项分布Bisquare 双平方Bivariate Correlate二变量相关Bivariate normal distribution 双变量正态分布Bivariate normal population 双变量正态总体Biweight interval 双权区间Biweight M-estimator 双权M估计量Block 区组/配伍组BMDP(Biomedical computer programs) BMDP统计软件包Boxplots 箱线图/箱尾图Breakdown bound 崩溃界/崩溃点Canonical correlation典型相关Caption 纵标目Case-control study 病例对照研究Categorical variable 分类变量Catenary 悬链线Cauchy distribution 柯西分布Cause-and-effect relationship因果关系Cell 单元Censoring 终检Center of symmetry 对称中心Centering and scaling 中心化和定标Central tendency集中趋势Central value 中心值CHAID -χ2 Automatic Interaction Detector 卡方自动交互检测Chance机遇Chance error 随机误差Chance variable随机变量Characteristic equation 特征方程Characteristic root 特征根Characteristic vector 特征向量Chebshev criterion of fit 拟合的切比雪夫准则Chernoff faces 切尔诺夫脸谱图Chi-square test 卡方检验/χ2检验Choleskey decomposition 乔洛斯基分解Circle chart 圆图Class interval组距Class mid-value 组中值Class upper limit 组上限Classified variable 分类变量Cluster analysis 聚类分析Cluster sampling 整群抽样Code 代码Coded data 编码数据Coding 编码Coefficient of contingency 列联系数Coefficient of determination 决定系数Coefficient of multiple correlation 多重相关系数Coefficient of partial correlation 偏相关系数Coefficient of production-moment correlation 积差相关系数Coefficient of rank correlation 等级相关系数Coefficient of regression 回归系数Coefficient of skewness 偏度系数Coefficient of variation变异系数Cohort study 队列研究Column列Column effect 列效应Column factor 列因素Combination pool合并Combinative table 组合表Common factor共性因子Common regression coefficient 公共回归系数Common value 共同值Common variance 公共方差Common variation 公共变异Communality variance 共性方差Comparability 可比性Comparison of bathes 批比较Comparison value比较值Compartment model 分部模型Compassion 伸缩Complement of an event 补事件Complete association完全正相关Complete dissociation完全不相关Complete statistics完备统计量Completely randomized design 完全随机化设计Composite event 联合事件Composite events 复合事件Concavity 凹性Conditional expectation 条件期望Conditional likelihood 条件似然Conditional probability 条件概率Conditionally linear 依条件线性Confidence interval 置信区间Confidence limit 置信限Confidence lower limit 置信下限Confidence upper limit 置信上限Confirmatory Factor Analysis 验证性因子分析Confirmatory research 证实性实验研究Confounding factor 混杂因素Conjoint 联合分析Consistency相合性Consistency check 一致性检验Consistent asymptotically normal estimate 相合渐近正态估计Consistent estimate 相合估计Constrained nonlinear regression 受约束非线性回归Constraint约束Contaminated distribution 污染分布Contaminated Gausssian 污染高斯分布Contaminated normal distribution 污染正态分布Contamination污染Contamination model 污染模型Contingency table 列联表Contour 边界线Contribution rate 贡献率Control 对照Controlled experiments 对照实验Conventional depth 常规深度Convolution 卷积Corrected factor 校正因子Corrected mean 校正均值Correction coefficient 校正系数Correctness?正确性Correlation coefficient 相关系数Correlation index 相关指数Correspondence 对应Counting 计数Counts 计数/频数Covariance 协方差Covariant 共变Cox Regression Cox回归Criteria for fitting 拟合准则Criteria of least squares 最小二乘准则Critical ratio 临界比Critical region 拒绝域Critical value 临界值Cross-over design 交叉设计Cross-section analysis 横断面分析Cross-section survey 横断面调查Crosstabs 交叉表Cross-tabulation table 复合表Cube root 立方根Cumulative distribution function 分布函数Cumulative probability 累计概率Curvature 曲率/弯曲Curvature 曲率Curve fit 曲线拟和Curve fitting 曲线拟合Curvilinear regression 曲线回归Curvilinear relation 曲线关系Cut-and-try method尝试(方)法Cycle 周期Cyclist 周期性D test D检验Data acquisition 资料收集Data bank 数据库Data capacity 数据容量Data deficiencies 数据缺乏Data handling 数据处理Data manipulation 数据处理Data processing 数据处理Data reduction 数据缩减Data set 数据集Data sources 数据来源Data transformation 数据变换Data validity 数据有效性Data-in 数据输入Data-out 数据输出Dead time 停滞期Degree of freedom自由度(程度)Degree of precision 精密度Degree of reliability可靠性程度Degression 递减Density function 密度函数Density of data points 数据点的密度Dependent variable 应变量/依变量/因变量Dependent variable 因变量Depth 深度Derivative matrix 导数矩阵Derivative-free methods 无导数方法Design 设计Determinacy确定性Determinant 行列式Determinant 决定因素Deviation 离差Deviation from average 离均差Diagnostic plot 诊断图Dichotomous variable 二分变量Differential equation 微分方程Direct standardization 直接标准化法Discrete variable 离散型变量DISCRIMINANT 判断Discriminant analysis判别分析Discriminant coefficient 判别系数Discriminant function 判别值Dispersion 散布/分散度Disproportional 不成比例的Disproportionate sub-class numbers 不成比例次级组含量Distribution free 分布无关性/免分布Distribution shape 分布形状Distribution-free method 任意分布法Distributive laws 分配律Disturbance 随机扰动项Dose response curve 剂量反应曲线Double blind method 双盲法Double blind trial 双盲试验Double exponential distribution 双指数分布Double logarithmic 双对数Downward rank 降秩Dual-space plot 对偶空间图DUD 无导数方法Duncan's new multiple range method 新复极差法/Duncan新法Effect 实验效应Eigenvalue 特征值Eigenvector 特征向量Ellipse 椭圆Empirical distribution 经验分布Empirical probability 经验概率单位Enumeration data 计数资料Equal sun-class number 相等次级组含量Equally likely 等可能Equivariance 同变性Error 误差/错误Error of estimate 估计误差Error type I 第一类错误Error type II 第二类错误Estimand 被估量Estimated error mean squares 估计误差均方Estimated error sum of squares 估计误差平方和Euclidean distance 欧式距离Event 事件Event 事件Exceptional data point 异常数据点Expectation plane 期望平面Expectation surface 期望曲面Expected values 期望值Experiment 实验Experimental sampling 试验抽样Experimental unit 试验单位Explanatory variable 说明变量Exploratory data analysis 探索性数据分析Explore Summarize 探索-摘要Exponential curve 指数曲线Exponential growth 指数式增长EXSMOOTH 指数平滑方法Extended fit 扩充拟合Extra parameter附加参数Extrapolation 外推法Extreme observation 末端观测值Extremes 极端值/极值F distribution F分布F test F检验Factor 因素/因子Factor analysis 因子分析Factor Analysis 因子分析Factor score 因子得分Factorial 阶乘Factorial design 析因试验设计False negative 假阴性False negative error 假阴性错误Family of distributions 分布族Family of estimators 估计量族Fanning扇面Fatality rate 病死率Field investigation 现场调查Field survey 现场调查Finite population 有限总体Finite-sample 有限样本First derivative 一阶导数First principal component 第一主成分First quartile 第一四分位数Fisher information 费雪信息量Fitted value 拟合值Fitting a curve 曲线拟合Fixed base 定基Fluctuation 随机起伏Forecast预测Four fold table 四格表Fourth 四分点Fraction blow 左侧比率Fractional error 相对误差Frequency 频率Frequency polygon 频数多边图Frontier point 界限点Function relationship 泛函关系Gamma distribution 伽玛分布Gauss increment 高斯增量Gaussian distribution 高斯分布/正态分布Gauss-Newton increment 高斯-牛顿增量General census 全面普查GENLOG (Generalized liner models) 广义线性模型Geometric mean几何平均数Gini's mean difference 基尼均差GLM (General liner models) 通用线性模型Goodness of fit 拟和优度/配合度Gradient of determinant 行列式的梯度Graeco-Latin square 希腊拉丁方Grand mean 总均值Gross errors 重大错误Gross-error sensitivity 大错敏感度Group averages 分组平均Grouped data 分组资料Guessed mean 假定平均数Half-life 半衰期Hampel M-estimators 汉佩尔M估计量Happenstance 偶然事件Harmonic mean 调和均数Hazard function 风险均数Hazard rate 风险率Heading 标目Heavy-tailed distribution 重尾分布Hessian array 海森立体阵Heterogeneity不同质Heterogeneity of variance 方差不齐Hierarchical classification 组内分组Hierarchical clustering method 系统聚类法High-leverage point 高杠杆率点HILOGLINEAR 多维列联表的层次对数线性模型Hinge 折叶点Histogram 直方图Historical cohort study 历史性队列研究Holes 空洞HOMALS 多重响应分析Homogeneity of variance 方差齐性Homogeneity test 齐性检验Huber M-estimators 休伯M估计量Hyperbola 双曲线Hypothesis testing 假设检验Hypothetical universe 假设总体Impossible event 不可能事件Independence 独立性Independent variable 自变量Index 指标/指数Indirect standardization 间接标准化法Individual 个体Inference band 推断带Infinite population 无限总体Infinitely great 无穷大Infinitely small 无穷小Influence curve 影响曲线Information capacity 信息容量Initial condition 初始条件Initial estimate 初始估计值Initial level 最初水平Interaction 交互作用Interaction terms 交互作用项Intercept 截距Interpolation 内插法Interquartile range 四分位距Interval estimation 区间估计Intervals of equal probability 等概率区间Intrinsic curvature 固有曲率Invariance 不变性Inverse matrix 逆矩阵Inverse probability 逆概率Inverse sine transformation 反正弦变换Iteration 迭代Jacobian determinant 雅可比行列式Joint distribution function 分布函数Joint probability 联合概率Joint probability distribution 联合概率分布K means method 逐步聚类法Kaplan-Meier 评估事件的时间长度Kaplan-Merier chart Kaplan-Merier图Kendall's rank correlation Kendall等级相关Kinetic 动力学Kolmogorov-Smirnove test 柯尔莫哥洛夫-斯米尔诺夫检验Kruskal and Wallis test Kruskal及Wallis检验/多样本的秩和检验/H检验Kurtosis 峰度Lack of fit 失拟Ladder of powers 幂阶梯Lag 滞后Large sample 大样本Large sample test 大样本检验Latin square 拉丁方Latin square design 拉丁方设计Leakage 泄漏Least favorable configuration 最不利构形Least favorable distribution 最不利分布Least significant difference 最小显着差法Least square method 最小二乘法Least-absolute-residuals estimates 最小绝对残差估计Least-absolute-residuals fit 最小绝对残差拟合Least-absolute-residuals line 最小绝对残差线Legend 图例L-estimator L估计量L-estimator of location 位置L估计量L-estimator of scale 尺度L估计量Level 水平Life expectance 预期期望寿命Life table 寿命表Life table method 生命表法Light-tailed distribution 轻尾分布Likelihood function 似然函数Likelihood ratio 似然比line graph 线图Linear correlation 直线相关Linear equation 线性方程Linear programming 线性规划Linear regression 直线回归Linear Regression 线性回归Linear trend 线性趋势Loading 载荷Location and scale equivariance 位置尺度同变性Location equivariance 位置同变性Location invariance 位置不变性Location scale family 位置尺度族Log rank test 时序检验Logarithmic curve 对数曲线Logarithmic normal distribution 对数正态分布Logarithmic scale 对数尺度Logarithmic transformation 对数变换Logic check 逻辑检查Logistic distribution 逻辑斯特分布Logit transformation Logit转换LOGLINEAR 多维列联表通用模型Lognormal distribution 对数正态分布Lost function 损失函数Low correlation 低度相关Lower limit 下限Lowest-attained variance 最小可达方差LSD 最小显着差法的简称Lurking variable 潜在变量Main effect 主效应Major heading 主辞标目Marginal density function 边缘密度函数Marginal probability 边缘概率Marginal probability distribution 边缘概率分布Matched data 配对资料Matched distribution 匹配过分布Matching of distribution 分布的匹配Matching of transformation 变换的匹配Mathematical expectation 数学期望Mathematical model 数学模型Maximum L-estimator 极大极小L 估计量Maximum likelihood method 最大似然法Mean 均数Mean squares between groups 组间均方Mean squares within group 组内均方Means (Compare means) 均值-均值比较Median 中位数Median effective dose 半数效量Median lethal dose 半数致死量Median polish 中位数平滑Median test 中位数检验Minimal sufficient statistic 最小充分统计量Minimum distance estimation 最小距离估计Minimum effective dose 最小有效量Minimum lethal dose 最小致死量Minimum variance estimator 最小方差估计量MINITAB 统计软件包Minor heading 宾词标目Missing data 缺失值Model specification 模型的确定Modeling Statistics 模型统计Models for outliers 离群值模型Modifying the model 模型的修正Modulus of continuity 连续性模Morbidity 发病率Most favorable configuration 最有利构形Multidimensional Scaling (ASCAL) 多维尺度/多维标度Multinomial Logistic Regression 多项逻辑斯蒂回归Multiple comparison 多重比较Multiple correlation 复相关Multiple covariance 多元协方差Multiple linear regression 多元线性回归Multiple response 多重选项Multiple solutions 多解Multiplication theorem 乘法定理Multiresponse 多元响应Multi-stage sampling 多阶段抽样Multivariate T distribution 多元T分布Mutual exclusive 互不相容Mutual independence 互相独立Natural boundary 自然边界Natural dead 自然死亡Natural zero 自然零Negative correlation 负相关Negative linear correlation 负线性相关Negatively skewed 负偏Newman-Keuls method q检验NK method q检验No statistical significance 无统计意义Nominal variable 名义变量Nonconstancy of variability 变异的非定常性Nonlinear regression 非线性相关Nonparametric statistics 非参数统计Nonparametric test 非参数检验Nonparametric tests 非参数检验Normal deviate 正态离差Normal distribution 正态分布Normal equation 正规方程组Normal ranges 正常范围Normal value 正常值Nuisance parameter 多余参数/讨厌参数Null hypothesis 无效假设Numerical variable 数值变量Objective function 目标函数Observation unit 观察单位Observed value 观察值One sided test 单侧检验One-way analysis of variance 单因素方差分析Oneway ANOVA 单因素方差分析Open sequential trial 开放型序贯设计Optrim 优切尾Optrim efficiency 优切尾效率Order statistics 顺序统计量Ordered categories 有序分类Ordinal logistic regression 序数逻辑斯蒂回归Ordinal variable 有序变量Orthogonal basis 正交基Orthogonal design 正交试验设计Orthogonality conditions 正交条件ORTHOPLAN 正交设计Outlier cutoffs 离群值截断点Outliers 极端值OVERALS 多组变量的非线性正规相关Overshoot 迭代过度Paired design 配对设计Paired sample 配对样本Pairwise slopes 成对斜率Parabola 抛物线Parallel tests 平行试验Parameter 参数Parametric statistics 参数统计Parametric test 参数检验Partial correlation 偏相关Partial regression 偏回归Partial sorting 偏排序Partials residuals 偏残差Pattern 模式Pearson curves 皮尔逊曲线Peeling 退层Percent bar graph 百分条形图Percentage 百分比Percentile 百分位数Percentile curves 百分位曲线Periodicity 周期性Permutation 排列P-estimator P估计量Pie graph 饼图Pitman estimator 皮特曼估计量Pivot 枢轴量Planar 平坦Planar assumption 平面的假设PLANCARDS 生成试验的计划卡Point estimation 点估计Poisson distribution 泊松分布Polishing 平滑Polled standard deviation 合并标准差Polled variance 合并方差Polygon 多边图Polynomial 多项式Polynomial curve 多项式曲线Population 总体Population attributable risk 人群归因危险度Positive correlation 正相关Positively skewed 正偏Posterior distribution 后验分布Power of a test 检验效能Precision 精密度Predicted value 预测值Preliminary analysis 预备性分析Principal component analysis 主成分分析Prior distribution 先验分布Prior probability 先验概率Probabilistic model 概率模型probability 概率Probability density 概率密度Product moment 乘积矩/协方差Profile trace 截面迹图Proportion 比/构成比Proportion allocation in stratified random sampling 按比例分层随机抽样Proportionate 成比例Proportionate sub-class numbers 成比例次级组含量Prospective study 前瞻性调查Proximities 亲近性Pseudo F test 近似F检验Pseudo model 近似模型Pseudosigma 伪标准差Purposive sampling 有目的抽样QR decomposition QR分解Quadratic approximation 二次近似Qualitative classification 属性分类Qualitative method 定性方法Quantile-quantile plot 分位数-分位数图/Q-Q图Quantitative analysis 定量分析Quartile 四分位数Quick Cluster 快速聚类Radix sort 基数排序Random allocation 随机化分组Random blocks design 随机区组设计Random event 随机事件Randomization 随机化Range 极差/全距Rank correlation 等级相关Rank sum test 秩和检验Rank test 秩检验Ranked data 等级资料Rate 比率Ratio 比例Raw data 原始资料Raw residual 原始残差Rayleigh's test 雷氏检验Rayleigh's Z 雷氏Z值Reciprocal 倒数Reciprocal transformation 倒数变换Recording 记录Redescending estimators 回降估计量Reducing dimensions 降维Re-expression 重新表达Reference set 标准组Region of acceptance 接受域Regression coefficient 回归系数Regression sum of square 回归平方和Rejection point 拒绝点Relative dispersion 相对离散度Relative number 相对数Reliability 可靠性Reparametrization 重新设置参数Replication 重复Report Summaries 报告摘要Residual sum of square 剩余平方和Resistance 耐抗性Resistant line 耐抗线Resistant technique 耐抗技术R-estimator of location 位置R估计量R-estimator of scale 尺度R估计量Retrospective study 回顾性调查Ridge trace 岭迹Ridit analysis Ridit分析Rotation 旋转Rounding 舍入Row 行Row effects 行效应Row factor 行因素RXC table RXC表Sample 样本Sample regression coefficient 样本回归系数Sample size 样本量Sample standard deviation 样本标准差Sampling error 抽样误差SAS(Statistical analysis system ) SAS统计软件包Scale 尺度/量表Scatter diagram 散点图Schematic plot 示意图/简图Score test 计分检验Screening 筛检SEASON 季节分析Second derivative 二阶导数Second principal component 第二主成分SEM (Structural equation modeling) 结构化方程模型Semi-logarithmic graph 半对数图Semi-logarithmic paper 半对数格纸Sensitivity curve 敏感度曲线Sequential analysis 贯序分析Sequential data set 顺序数据集Sequential design 贯序设计Sequential method 贯序法Sequential test 贯序检验法Serial tests 系列试验Short-cut method 简捷法Sigmoid curve S形曲线Sign function 正负号函数Sign test 符号检验Signed rank 符号秩Significance test 显着性检验Significant figure 有效数字Simple cluster sampling 简单整群抽样Simple correlation 简单相关Simple random sampling 简单随机抽样Simple regression 简单回归simple table 简单表Sine estimator 正弦估计量Single-valued estimate 单值估计Singular matrix 奇异矩阵Skewed distribution 偏斜分布Skewness 偏度Slash distribution 斜线分布Slope 斜率Smirnov test 斯米尔诺夫检验Source of variation 变异来源Spearman rank correlation 斯皮尔曼等级相关Specific factor 特殊因子Specific factor variance 特殊因子方差Spectra 频谱Spherical distribution 球型正态分布Spread 展布SPSS(Statistical package for the social science) SPSS统计软件包Spurious correlation 假性相关Square root transformation 平方根变换Stabilizing variance 稳定方差Standard deviation 标准差Standard error 标准误Standard error of difference 差别的标准误Standard error of estimate 标准估计误差Standard error of rate 率的标准误Standard normal distribution 标准正态分布Standardization 标准化Starting value 起始值Statistic 统计量Statistical control 统计控制Statistical graph 统计图Statistical inference 统计推断Statistical table 统计表Steepest descent 最速下降法Stem and leaf display 茎叶图Step factor 步长因子Stepwise regression 逐步回归Storage 存Strata 层(复数)Stratified sampling 分层抽样Stratified sampling 分层抽样Strength 强度Stringency 严密性Structural relationship 结构关系Studentized residual 学生化残差/t化残差Sub-class numbers 次级组含量Subdividing 分割Sufficient statistic 充分统计量Sum of products 积和Sum of squares 离差平方和Sum of squares about regression 回归平方和Sum of squares between groups 组间平方和Sum of squares of partial regression 偏回归平方和Sure event 必然事件Survey 调查Survival 生存分析Survival rate 生存率Suspended root gram 悬吊根图Symmetry 对称Systematic error 系统误差Systematic sampling 系统抽样Tags 标签Tail area 尾部面积Tail length 尾长Tail weight 尾重Tangent line 切线Target distribution 目标分布Taylor series 泰勒级数Tendency of dispersion 离散趋势Testing of hypotheses 假设检验Theoretical frequency 理论频数Time series 时间序列Tolerance interval 容忍区间Tolerance lower limit 容忍下限Tolerance upper limit 容忍上限Total sum of square 总平方和Total variation 总变异Transformation 转换Treatment 处理Trend 趋势Trend of percentage 百分比趋势Trial 试验Trial and error method 试错法Tuning constant 细调常数Two sided test 双向检验Two-stage least squares 二阶最小平方Two-stage sampling 二阶段抽样Two-tailed test 双侧检验Two-way analysis of variance 双因素方差分析Two-way table 双向表Type I error 一类错误/α错误Type II error 二类错误/β错误UMVU 方差一致最小无偏估计简称Unbiased estimate 无偏估计Unconstrained nonlinear regression 无约束非线性回归Unequal subclass number 不等次级组含量Ungrouped data 不分组资料Uniform coordinate 均匀坐标Uniform distribution 均匀分布Uniformly minimum variance unbiased estimate 方差一致最小无偏估计Unit 单元Unordered categories 无序分类Upper limit 上限Upward rank 升秩Vague concept 模糊概念Validity 有效性VARCOMP (Variance component estimation) 方差元素估计Variability 变异性Variable 变量Variance 方差Variation 变异Varimax orthogonal rotation 方差最大正交旋转Volume of distribution 容积W test W检验Waiting time 等待时间Wald's test 正态近似检验,U检验Waston-William's test Waston-William检验Weibull distribution 威布尔分布Weight 权数Weighted Chi-square test 加权卡方检验/Cochran检验Weighted linear regression method 加权直线回归Weighted mean 加权平均数Weighted mean square 加权平均方差Weighted sum of square 加权平方和Weighting coefficient 权重系数Weighting method 加权法W-estimation W估计量W-estimation of location 位置W估计量Width 宽度Wilcoxon paired test 威斯康星配对法/配对符号秩和检验Wild point 野点/狂点Wild value 野值/狂值Winsorized mean 缩尾均值Withdraw 失访Youden's index 尤登指数Z test Z检验Zero correlation 零相关Z-transformation Z变换常用统计学英文名词(汉英对照)Bayes定理 Bayes' theoremBMDP统计软件包 BMDP(Biomedical computer programs) Cox回归 Cox RegressionD检验 D testF分布 F distributionF检验 F testKaplan-Merier图 Kaplan-Merier chartKendall等级相关 Kendall's rank correlationKruskal及Wallis检验/多样本的秩和检验/H检验 Kruskal and Wallis test Logit转换 Logit transformationL估计量 L-estimatorP估计量 P-estimatorQR分解 QR decompositionq检验 Newman-Keuls methodq检验 NK methodRidit分析 Ridit analysisRXC表 RXC tableSAS统计软件包 SAS(Statistical analysis system )SPSS统计软件包 SPSS(Statistical package for the social science)S形曲线 Sigmoid curveWaston-William检验 Waston-William's testW估计量 W-estimationW检验 W testZ变换 Z-transformationZ检验 Z test按比例分层随机抽样 Proportion allocation in stratified random sampling凹性 Concavity百分比 Percentage百分比趋势 Trend of percentage百分条形图 Percent bar graph百分位曲线 Percentile curves百分位数 Percentile半对数格纸 Semi-logarithmic paper半对数图 Semi-logarithmic graph半数效量 Median effective dose半数致死量 Median lethal dose半衰期 Half-life报告摘要 Report Summaries备择假设 Alternative hypothesis被估量 Estimand崩溃界/崩溃点 Breakdown bound比/构成比 Proportion比较值 Comparison value比例 Ratio比率 Rate必然事件 Sure event边界线 Contour边缘概率 Marginal probability边缘概率分布 Marginal probability distribution边缘密度函数 Marginal density function编码 Coding编码数据 Coded data变换的匹配 Matching of transformation变量 Variable变异 Variation变异的非定常性 Nonconstancy of variability变异来源 Source of variation变异系数 Coefficient of variation变异性 Variability标目 Heading标签 Tags标准差 Standard deviation标准估计误差 Standard error of estimate标准化 Standardization标准误 Standard error标准正态分布 Standard normal distribution标准组 Reference set宾词标目 Minor heading饼图 Pie graph病例对照研究 Case-control study病死率 Fatality rate伯努力分布 Bernoulli distribution泊松分布 Poisson distribution补事件 Complement of an event不变性 Invariance不成比例次级组含量 Disproportionate sub-class numbers 不成比例的 Disproportional不等次级组含量 Unequal subclass number不分组资料 Ungrouped data不可能事件 Impossible event不同质 Heterogeneity步长因子 Step factor参数 Parameter参数检验 Parametric test参数统计 Parametric statistics残差的自相关 Autocorrelation of residuals层(复数) Strata差别的标准误 Standard error of difference尝试法 Cut-and-try method常规深度 Conventional depth成比例 Proportionate成比例次级组含量 Proportionate sub-class numbers成对斜率 Pairwise slopes乘法定理 Multiplication theorem乘积矩/协方差 Product moment尺度/量表 Scale尺度L估计量 L-estimator of scale尺度R估计量 R-estimator of scale充分统计量 Sufficient statistic抽样误差 Sampling error初始估计值 Initial estimate初始条件 Initial condition处理 Treatment次级组含量 Sub-class numbers存 Storage大错敏感度 Gross-error sensitivity大样本 Large sample大样本检验 Large sample test代码 Code单侧检验 One sided test单因素方差分析 One-way analysis of variance 单因素方差分析 Oneway ANOVA单元 Cell单元 Unit单值估计 Single-valued estimate导数矩阵 Derivative matrix倒数 Reciprocal倒数变换 Reciprocal transformation等待时间 Waiting time等概率区间 Intervals of equal probability等级相关 Rank correlation等级相关系数 Coefficient of rank correlation 等级资料 Ranked data等可能 Equally likely低度相关 Low correlation递减 Degression第二类错误 Error type II第二主成分 Second principal component第一类错误 Error type I第一四分位数 First quartile第一主成分 First principal component典型相关 Canonical correlation点估计 Point estimation调查 Survey调和均数 Harmonic mean调整率 Adjusted rate迭代 Iteration迭代过度 Overshoot定基 Fixed base定量分析 Quantitative analysis定性方法 Qualitative method动力学 Kinetic独立性 Independence队列研究 Cohort study对称 Symmetry对称中心 Center of symmetry对偶空间图 Dual-space plot对数变换 Logarithmic transformation对数尺度 Logarithmic scale对数曲线 Logarithmic curve对数正态分布 Logarithmic normal distribution对数正态分布 Lognormal distribution对应 Correspondence对照 Control对照实验 Controlled experiments多边图 Polygon多阶段抽样 Multi-stage sampling多解 Multiple solutions多维尺度/多维标度 Multidimensional Scaling (ASCAL) 多维列联表的层次对数线性模型 HILOGLINEAR多维列联表通用模型 LOGLINEAR多项逻辑斯蒂回归 Multinomial Logistic Regression 多项式 Polynomial多项式曲线 Polynomial curve多余参数/讨厌参数 Nuisance parameter多元T分布 Multivariate T distribution多元线性回归 Multiple linear regression多元响应 Multiresponse多元协方差 Multiple covariance多重比较 Multiple comparison多重相关系数 Coefficient of multiple correlation 多重响应分析 HOMALS多重选项 Multiple response多组变量的非线性正规相关 OVERALS二变量相关 Bivariate Correlate二次近似 Quadratic approximation二分变量 Dichotomous variable二阶导数 Second derivative二阶段抽样 Two-stage sampling二阶最小平方 Two-stage least squares二类错误/β错误 Type II error二项分布 Binomial distribution二元逻辑斯蒂回归 Binary logistic regression发病率 Morbidity法向加速度 Acceleration normal反正弦变换 Arcsine transformation反正弦变换 Inverse sine transformation泛函关系 Function relationship方差 Variance方差不齐 Heterogeneity of variance方差分析 Analysis of variance方差分析 ANOVA (analysis of variance)方差分析模型 ANOVA Models方差齐性 Homogeneity of variance方差一致最小无偏估计 Uniformly minimum variance unbiased estimate 方差一致最小无偏估计简称 UMVU方差元素估计 VARCOMP (Variance component estimation)方差最大正交旋转 Varimax orthogonal rotation非参数检验 Nonparametric test非参数检验 Nonparametric tests非参数统计 Nonparametric statistics非对称分布 Asymmetric distribution非线性相关 Nonlinear regression费雪信息量 Fisher information分布的匹配 Matching of distribution分布函数 Cumulative distribution function分布函数 Joint distribution function分布无关性/免分布 Distribution free分布形状 Distribution shape分布族 Family of distributions分部模型 Compartment model分层抽样 Stratified sampling分层抽样 Stratified sampling分割 Subdividing分类变量 Categorical variable分类变量 Classified variable分配律 Distributive laws分位数-分位数图/Q-Q图 Quantile-quantile plot分组平均 Group averages分组资料 Grouped data风险均数 Hazard function风险率 Hazard rate峰度 Kurtosis符号检验 Sign test符号秩 Signed rank负偏 Negatively skewed负线性相关 Negative linear correlation。
管理统计学之megastat应用举例
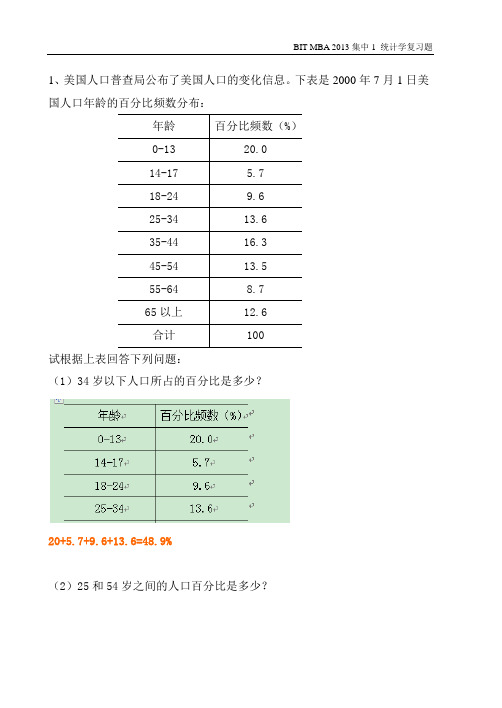
1、美国人口普查局公布了美国人口的变化信息。
下表是2000年7月1日美国人口年龄的百分比频数分布:试根据上表回答下列问题:(1)34岁以下人口所占的百分比是多少?20+5.7+9.6+13.6=48.9%(2)25和54岁之间的人口百分比是多少?13.6+16.3+13.5=43.4%(3)超过34岁的人口百分比是多少?16.3+13.5+8.7+12.6=51.1%(4)已知总人口为2.75亿,有多少人年龄低于25岁?2.75亿×(20.0+5.7+9.6)%=0.97亿2、为考察大学生的月消费情况,调查者选取了一个班级作为样本,表1是该班学生月消费额的频数分布情况,根据此资料回答下列问题:解题过程请看《Megastat应用根据样本数据算均值、方差、直方图等等》表1 学生月消费额的频数分布(1)计算此班级的平均月消费额以及月消费额的标准差;Descriptive statistics(1)计算此班级的平均月消费额以及月消费额的标准差;count 53mean 483.96sample variance 14,209.00sample standard deviation 119.20minimum 350maximum 750range 400此班级的平均月消费额:483.96月消费额的标准差:14209(2)试画出频数分布图,并据此判断数据的偏态分布类型;Frequency Distribution - Quantitative(2)试画出频数分布图,并据此判断数据的偏态分布类型;cumulativelower upper midpoint width frequency percent frequency percent 350 < 400 375 50 15 28.8 15 28.8 400 < 450 425 50 0 0.0 15 28.8 450 < 500 475 50 18 34.6 33 63.5 500 < 550 525 50 0 0.0 33 63.5 550 < 600 575 50 10 19.2 43 82.7 600 < 650 625 50 0 0.0 43 82.7 650 < 700 675 50 7 13.5 50 96.2 700 < 750 725 50 0 0.0 50 96.2 750 < 800 775 50 2 3.8 52 100.052 100.0数据右偏分布(3)试分析众数、中位数分别在哪一组。
Samplingandsamplesizecalculation样本量计算方法大全
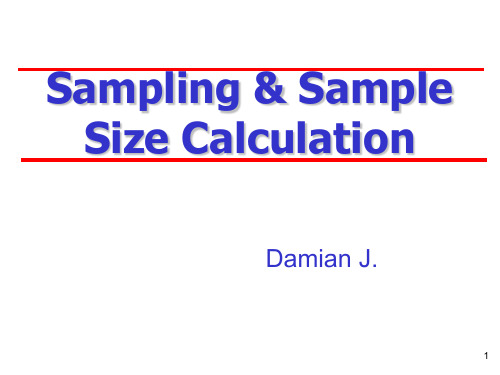
11
Sampling methods in qualitative studies: Non-probability samples (1)
• Qualitative research methods are typically used when focus is on a limited number of informants
• Key respondents should never be chosen at random, but purposively from among those who have the best possible knowledge, experience or overview with respect to topic of your study.
Target Population Sampled Population Sample 4
What makes a "good" sample?
A RUSsIaN sample:
– Representative – Unbiased – Sampling Error can be quantified – Maximum Information for minimum cost – Non-sampling error is corrected for as
• Examples: – Stigma (e.g. of leprosy, TB, HIV, epilepsy) is considered a complicating factor in the control of diseases: to obtain insight in how stigma manifests itself in different characteristics (cultures, sex, area: rural/urban, in well-to-do and poor patients), a researcher has to take care that all these groups are included in the sample. – To assess whether social distance influences stigma, one could also interview blood relatives (parents or children), spouses, friends, neighbours of patients.
Determining the sample size
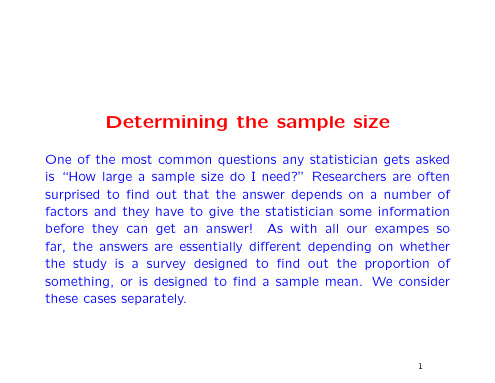
6
A different type of variation is “What if we have no initial estimate of p ˆ?” In this case, the convention is to assume p ˆ = 0.5.
ˆ(1−p ˆ) The reason is that the standard error formula, p n , is largest when p ˆ = 0.5, so this is a conservative assumption that allows for p ˆ being unknown a priori.
7
Sample size to estimate a population mean The issues are similar if we are designing a survey or an experiment to estimate a population mean. In this case, the formula is s ME = t √ ( ‡) n where • ME is the desired margin of error • t is the t-score that we use to calculate the confidence interval, that depends on both the degrees of freedom and the desired confidence level, • s is the standard deviation, • n is the sample size we want to find.
2
Possible answers might be: 1. “We need a margin of error less than 2.5%”. Typical surveys have margins of error ranging from less than 1% to something of the order of 4% — we can choose any margin of error we like but need to specify it. 2. 95% confidence intervals are typical but not in any way mandatory — we could do 90%, 99% or something else entirely. For this example, we assume 95%. 3. May be guided by past surveys or general knowledge of public opinion. Let’s suppose answer is 30%.
2023国际贸易专业英语考点
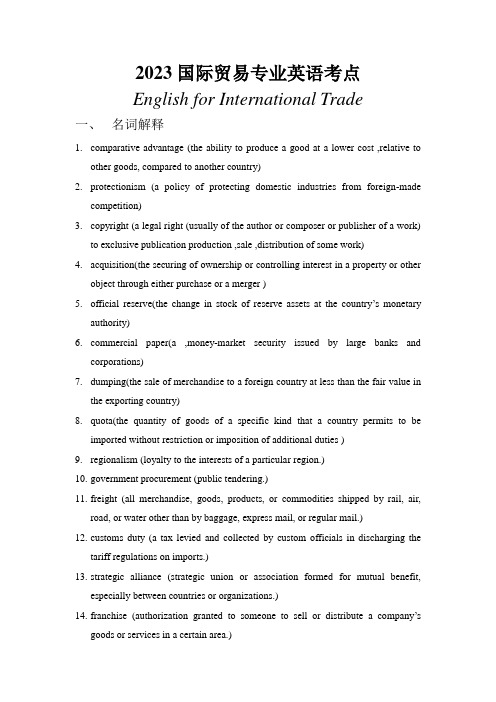
2023国际贸易专业英语考点English for International Trade一、名词解释parative advantage (the ability to produce a good at a lower cost ,relative toother goods, compared to another country)2.protectionism (a policy of protecting domestic industries from foreign-madecompetition)3.copyright (a legal right (usually of the author or composer or publisher of a work)to exclusive publication production ,sale ,distribution of some work)4.acquisition(the securing of ownership or controlling interest in a property or otherobject through either purchase or a merger )5.official reserve(the change in stock of reserve assets at the country’s monetaryauthority)mercial paper(a ,money-market security issued by large banks andcorporations)7.dumping(the sale of merchandise to a foreign country at less than the fair value inthe exporting country)8.quota(the quantity of goods of a specific kind that a country permits to beimported without restriction or imposition of additional duties )9.regionalism (loyalty to the interests of a particular region.)ernment procurement (public tendering.)11.freight (all merchandise, goods, products, or commodities shipped by rail, air,road, or water other than by baggage, express mail, or regular mail.)12.customs duty (a tax levied and collected by custom officials in discharging thetariff regulations on imports.)13.strategic alliance (strategic union or association formed for mutual benefit,especially between countries or organizations.)14.franchise (authorization granted to someone to sell or distribute a company’sgoods or services in a certain area.)15.clearance (the completion of customs entry formalities resulting in the release ofgoods from customs custody to the importer.)16.open account (credit extended that is not supported by a note, mortgage, or otherformal written evidence of indebtedness.)17.honour (accept as pay.)18.default (failure to meet legal obligations in contract, such as the failure to makethe monthly mortgage payment.)19.cumulative (accumulative; increasing by successive addition.)20.Freight All Kinds (FAK) (This kind of freight is based on the volume or weightonly, and does not consider the attribute, type and other factors.)二、选词填空1.The theory of comparative costs suggested that country will specialize inproducing goods and services in which they have a comparative advantage2.The domestic consumers benefit from international trade because it lowers theprices of goods and makes available goods that cannot be produced at home.3.Licensing involve granting a license to a foreign firm to produce and market aproduct for which the foreign firm pays royalties to the parent firm4.This may draw down gold reserves5.The most notable drawback of protective tariffs is that they increase the price ofthe good subject to the tariff, disadvantaging consumers of that good.6.Many observers argue that the purpose of agricultural subsidies is in the end toprotect farmers so that they will continue to vote for the politicians that vote for these subsidies7.For the WTO”s procedure to succeed, countries must (adhere to) its decisions,even when they disagree with them.ernment procurement (accounts for) a substantial part of the global economy.9.If the seller is to pay the cost and bear the risk of unloading and clearing customsand (make delivery) on the dock thereafter, the term is DEQ or delivered ex-quay, duty paid.10.Once delivery is made to the carrier, the buyer is in essentially the same positionas the buyer who (takes delivery) ex works.11.Joint ventures frequently provide a means to avoid restrictions on foreignownership that many countries (have incorporated into) their legal systems.12.The L/C must (comply with) the terms and conditions in the contract.13.Whether we place a large order with you will depend on (subsequent) marketcondition.14.Revolving credits is rather a credit of fixed amount, which has to be increased (bymeans of) amendment instructions after each drawing or, alternatively, a credit of fixed total amount is payable by specific installments.15.It is(in the company’s best interest)to make effective use of the process and toensure that costs are kept to a minimum wherever possible.三、判断题1.According absolute model , each country should specialized in and supply theproducts where it has an absolute advantage.(T)2.Most of the nations in the world can not afford to adopt an autarky policy inforeign trade (T)3.Regarding joint ventures ,the host country usually imposes some restriction on theproportion of foreign investment in certain industries (T)4.Every complete economic transaction is recorded twice(T)5.Ad valorem duties are more effective than specific duties in protecting domesticfirms from foreign competition(F)6.Persistent dumping does no harm to the importing country, so it should not berestricted .(F)petition policy is not a hard issue at WTO panels. (F)8.At the Kennedy Round the low-income countries were controlled by thehigh-income countries. (T)9.Under DES, The buyer is responsible for all expenses after the ship’s arrival,including unloading, clearing customs, and inland freight. (T)10.The only difference between CFR and CIF is that under a CFR contract the buyermist purchase a marine insurance contract to cover the goods while they are on the voyage. (T)11.It is critically important for the franchisor to maintain quality controls over thefranchisees. (T)12.The “battle of the forms ”means the two parties negotiate to agree commonground and the exact terms that will constitute the contract. (T)13.Both the revocable L/C and irrevocable L/C offer importers and exporters samesecuring payment. (F)14.There are various cost involved in the international transaction, including banks’charges for opening and confirming L/Cs and subsequent charges whenever discrepancies occur. (T)15.The last thing that an exporter wants to deal with is a bulk consignment that isloaded onto a vessel that is patently not built for the purpose. (T)四、汉译英1.通过国际贸易,可以使消费者和贸易国获取本国没有的商品和服务。
pmsampsize 1.1.3 包说明说明书

Package‘pmsampsize’December6,2023Version1.1.3Date2023-12-05Title Sample Size for Development of a Prediction ModelMaintainer Joie Ensor<***************.uk>Depends R(>=2.1)Suggests statsDescription Computes the minimum sample size required for the development of a new multivari-able prediction model using the criteria proposed by Ri-ley et al.(2018)<doi:10.1002/sim.7992>.pmsampsize can be used to calculate the mini-mum sample size for the development of models with continuous,binary or survival(time-to-event)outcomes.Riley et al.(2018)<doi:10.1002/sim.7992>lay out a series of criteria the sam-ple size should meet.These aim to minimise the overfitting and to ensure precise estima-tion of key parameters in the prediction model.License GPL(>=3)RoxygenNote7.2.3Encoding UTF-8NeedsCompilation noAuthor Joie Ensor[aut,cre]Repository CRANDate/Publication2023-12-0609:10:02UTCR topics documented:pmsampsize (2)Index71pmsampsize pmsampsize-Sample Size for Development of a Prediction ModelDescriptionpmsampsize computes the minimum sample size required for the development of a new multivari-able prediction model using the criteria proposed by Riley et al.2018.Usagepmsampsize(type,nagrsquared=NA,csrsquared=NA,rsquared=NA,parameters,shrinkage=0.9,prevalence=NA,cstatistic=NA,seed=123456,rate=NA,timepoint=NA,meanfup=NA,intercept=NA,sd=NA,mmoe=1.1)Argumentstype specifies the type of analysis for which sample size is being calculated•"c"specifies sample size calculation for a prediction model with a contin-uous outcome•"b"specifies sample size calculation for a prediction model with a binaryoutcome•"s"specifies sample size calculation for a prediction model with a survival(time-to-event)outcomenagrsquared for type="b"or type="s"this specifies the expected value of the Nagelkerke’s R-squared of the new model,which is the Cox-Snell R-squared scaled to lie inthe[0,1]range.It is interpretable in the same way as the standard R-squared,i.e.the percentage of variation in outcome values explained by the model.Pleaseread the description of rsquared for additional details about specifying the ex-pected R-squared performancecsrsquared for type="b"or type="s"this specifies the expected value of the Cox-Snell R-squared of the new model.The Cox-Snell R-squared is the generalised versionof the well-known R-squared for continuous outcomes,based on the likelihood.Please read the description of rsquared for additional details about specifyingthe expected R-squared performance.The papers by Riley et al.(see references)outline how to obtain the Cox-Snell R-squared value from published studiesif they are not reported,using other information(such as the C-statistic[seecstatistic()option below]).rsquared for type="c"this specifies the expected value of the R-squared of the new model,where R-squared is the percentage of variation in outcome values ex-plained by the model.For example,the user may input the value of the R-squared reported for a previous prediction model study in the samefield.If tak-ing a value from a previous prediction model development study,users shouldinput the model’s adjusted R-squared value,not the apparent R-squared value,as the latter is optimistic(biased).However,if taking the R-squared value froman external validation of a previous model,the apparent R-squared can be used(as the validation data was not used for development,and so R-squared appar-ent is then unbiased).Users should be conservative with their chosen R-squaredvalue;for example,by taking the R-squared value from a previous model,evenif they hope their new model will improve performance.parameters specifies the number of candidate predictor parameters for potential inclusion in the new prediction model.Note that this may be larger than the number ofcandidate predictors,as categorical and continuous predictors often require twoor more parameters to be estimated.shrinkage specifies the level of shrinkage desired at internal validation after developing the new model.Shrinkage is a measure of overfitting,and can range from0to1,with higher values denoting less overfitting.We recommend a shrinkage=0.9(the default in pmsampsize),which indicates that the predictor effect(beta coef-ficients)in the model would need to be shrunk by10%to adjust for overfitting.See references below for further information.prevalence(type="b"option)specifies the overall outcome proportion(for a prognostic model)or overall prevalence(for a diagnostic model)expected within the modeldevelopment dataset.This should be derived based on previous studies in thesame population.cstatistic(type="b"option)specifies the C-statistic reported in an existing prediction model study to be used in conjunction with the expected prevalence to approxi-mate the Cox-Snell R-squared using the approach of Riley et al.2020.Ideally,this should be an optimism-adjusted C-statistic.The approximate Cox-Snell R-squared value is used as described above for the csrsquared()option,and sois treated as a baseline for the expected performance of the new model.seed(type="b"option)specifies the initial value of the random-number seed used by the random-number functions when simulating data to approximate the Cox-Snell R-squared based on reported C-statistic and expect prevalence as describedby Riley et al.2020rate(type="s"option)specifies the overall event rate in the population of interest, for example as obtained from a previous study,for the survival outcome of in-terest.NB:rate must be given in time units used for meanfup and timepointoptions.timepoint(type="s"option)specifies the timepoint of interest for prediction.NB:time units must be the same as given for meanfup option(e.g.years,months).meanfup(type="s"option)specifies the average(mean)follow-up time anticipated for individuals in the model development dataset,for example as taken from a pre-vious study in the population of interest.NB:time units must be the same asgiven for timepoint option.intercept(type="c"options)specifies the average outcome value in the population of interest e.g.the average blood pressure,or average pain score.This could bebased on a previous study,or on clinical knowledge.sd(type="c"options)specifies the standard deviation(SD)of outcome values in the population e.g.the SD for blood pressure in patients with all other predictorsset to the average.This could again be based on a previous study,or on clinicalknowledge.mmoe(type="c"options)multiplicative margin of error(MMOE)acceptable for cal-culation of the intercept.The default is a MMOE of10%.Confidence intervalfor the intercept will be displayed in the output for reference.See referencesbelow for further information.Detailspmsampsize can be used to calculate the minimum sample size for the development of models with continuous,binary or survival(time-to-event)outcomes.Riley et y out a series of criteria the sample size should meet.These aim to minimise the overfitting and to ensure precise estimation of key parameters in the prediction model.For continuous outcomes,there are four criteria:i)small overfitting defined by an expected shrinkage of predictor effects by10%or less,ii)small absolute difference of0.05in the model’s apparent and adjusted R-squared value,iii)precise estimation of the residual standard deviation,andiv)precise estimation of the average outcome value.The sample size calculation requires the user to pre-specify(e.g.based on previous evidence) the anticipated R-squared of the model,and the average outcome value and standard deviation of outcome values in the population of interest.For binary or survival(time-to-event)outcomes,there are three criteria:i)small overfitting defined by an expected shrinkage of predictor effects by10%or less,ii)small absolute difference of0.05in the model’s apparent and adjusted Nagelkerke’s R-squared value,andiii)precise estimation(within+/-0.05)of the average outcome risk in the population for a key timepoint of interest for prediction.With thanks to Richard D.Riley,Emma C Martin,Gary Collins,Glen Martin&Kym Snell for helpful input&feedbackValueA list including a matrix of calculated sample size requirements for each criteria defined under’De-tails’,and a series of vectors of parameters used in the calculations as well as thefinal recommended minimum sample size and number of events required for model development.Author(s)Joie Ensor(University of Birmingham,***************.uk),ReferencesRiley RD,Ensor J,Snell KIE,Harrell FE,Martin GP,Reitsma JB,et al.Calculating the sample size required for developing a clinical prediction model.BMJ(Clinical research ed).2020Riley RD,Snell KIE,Ensor J,Burke DL,Harrell FE,Jr.,Moons KG,Collins GS.Minimum sample size required for developing a multivariable prediction model:Part I continuous outcomes.Statistics in Medicine.2018(in-press).doi:10.1002/sim.7993Riley RD,Snell KIE,Ensor J,Burke DL,Harrell FE,Jr.,Moons KG,Collins GS.Minimum sample size required for developing a multivariable prediction model:Part II binary and time-to-event outcomes.Statistics in Medicine.2018(in-press).doi:10.1002/sim.7992Riley,RD,Van Calster,B,Collins,GS.A note on estimating the Cox-Snell R2from a reportedC statistic(AUROC)to inform sample size calculations for developing a prediction model with abinary outcome.Statistics in Medicine.2020Examples##Examples based on those included in two papers by Riley et al.##published in Statistics in Medicine(2018).##NB:Survival example based on Riley et al.BMJ paper(2020).##Binary outcomes(Logistic prediction models)#Use pmsampsize to calculate the minimum sample size required to develop a#multivariable prediction model for a binary outcome using24candidate#predictor parameters.Based on previous evidence,the outcome prevalence is#anticipated to be0.174(17.4%)and a lower bound(taken from the adjusted#Cox-Snell R-squared of an existing prediction model)for the new model s#R-squared value is0.288pmsampsize(type="b",csrsquared=0.288,parameters=24,prevalence=0.174)#Now lets assume we could not obtain a Cox-Snell R-squared estimate from an existing #prediction model,but instead had a C-statistic(0.89)reported for the existing prediction #model.We can use this C-statistic along with the prevalence to approximate the Cox-Snell #R-squared using the approach of Riley et al.(2020).Use pmsampsize with the cstatistic() #option instead of rsquared()option.pmsampsize(type="b",cstatistic=0.89,parameters=24,prevalence=0.174)##Survial outcomes(Cox prediction models)#Use pmsampsize to calculate the minimum sample size required for developing#a multivariable prediction model with a survival outcome using30candidate#predictors.We know an existing prediction model in the same field has an#R-squared adjusted of0.051.Further,in the previous study the mean#follow-up was2.07years,and overall event rate was0.065.We select a#timepoint of interest for prediction using the newly developed model of2#yearspmsampsize(type="s",csrsquared=0.051,parameters=30,rate=0.065, timepoint=2,meanfup=2.07)##Continuous outcomes(Linear prediction models)#Use pmsampsize to calculate the minimum sample size required for developing#a multivariable prediction model for a continuous outcome(here,FEV1say),#using25candidate predictors.We know an existing prediction model in the#same field has an R-squared adjusted of0.2,and that FEV1values in the#population have a mean of1.9and SD of0.6pmsampsize(type="c",rsquared=0.2,parameters=25,intercept=1.9,sd=0.6)Indexpmsampsize,27。
民航国际结算专业术语

专业术语DEFINITIONS OF MPA-P AND EXTRACTS FROM THE RAMAmount to be Prorated (ATBP)被分摊额The through fare or excess baggage charge for which Proration is required irrespective of the directionality of the fareBase Amount基础额The amount (expressed in local currency or as otherwise agreed) published in Part 2 of the Prorate Manual to be applied when establishing the value of a ProvisoCircle Trip环程travel from a point and return thereto by a continuous, circuitous air route, including travel comprising two fare components but which do not meet the conditions of the round trip definitionDifferential等级差额an amount shown separately on a ticket for travel in a higher class of service within a fare componentCode-share Arrangement代码共享协议An arrangement wherebya) an airline who cannot operate a sector due to the absence of full local traffic rights shows its designator on a flight coupon(s) although the operation for such sector(s) is by another airline.b) an airline who can operate a sector with full local traffic rights but has decided not to do so and instead shows its designator on a flight coupon(s) although the operation for such sector(s) is by another airlineFare Calculation Point票价计算点a point at which a fare has been broken for fare construction purposesIssuing Airline出票航空公司The airline whose documents are issued.Journey (New)航程the entire itinerary shown on the ticketNormal Fare普通票价A fare for which the following conditions have been met:1. published as a one way and/or round trip fare2. having a one year validity3. having no restrictions in respect of:3.1 advance purchase3.2 minimum stay3.3 reservations/payment/ticketing3.4 time of day travel3.5 specified flights3.6 eligibilityPoint of Turnaround回折点A point on a round trip fare where the outbound portion ends and the inbound portion commencesProration比例分摊The division of the through fare or excess baggage charge, Including the deduction of Provisos, where applicableProviso固定收费An exception to the proration rules of the MPA filed with the Prorate Agency and published in the Prorate ManualReissued Ticket换票A ticket issued in exchange for totally unused or partially used ticket(s)Round Trip来回程travel entirely by air from a point to another point and return to the original point comprising two half round trip fare components only, for which the applicable normal half round trip fare for each component, measured from the point of unit origin, is the same for the routing travelled; provided that this definition shall not apply to round the world travelif the fares to be used differ through class of service/seasonality/midweek-weekend/carrier variations, the outbound fare shall be used also for the inbound fare component for the purpose of determining if the pricing unit is a round tripSignatory签约航空公司An airline which has signed the MPASpecial Fare特殊票价Any fare other than a Normal FareStopover Charge中途分程附加费A charge for stopover(s) paid as a separate amount in addition to the through fareStraight Rate Prorate直线比例分摊The proportional division of the through fare or excess baggage charge, disregarding Provisos Surcharge附加额An additional charge shown separately in the fare calculation e.g. weekend, stopover, supersonic, fuel, security, stretcher, etc.Through Fare直通票价The total fare from the first point covered by the ATBP to the last point covered by the ATBPDEFINITION OF PRORATION TERMS USED IN MPA-CAir Waybill Issuing Airline航空货运单的销售航空公司The airline in whose name the air waybill for carriage of a cargo shipment which is to be prorated under this agreement has been issued.Bulk Unitization Charges集装箱运费Special charges for shipments carried in unit load devices pursuant to a bulk unitization programme, unitization incentive programme and/or unitization discount programme.Charge运费a) the amount resulting from multiplication of a rate by the chargeable weightb) a flat amount applicable to a shipment (minimum charge or charge for a shipment carried in unit load devices) which is currently published in TACT as beingapplicable for carriage between the city of origin and city of destination,Factor Based Proration以系数为基础的比例分摊The principle of sharing joint revenue in proportion to the applicable factors for carriage over the section as published in the Prorate Manual – Cargo (PMC). Flagged Rate or ChargeThe Rate or Charge individually established by an airline and currently published in TACT as being applicable for carriage of goods between the city of origin and city of destination. It shall comprise either a rate for a unit of weight (or volume) and/or a flat amount applicable to a shipment (minimum charge or charge for a shipment carried in unit load devices).This Rate or Charge shall be identified in TACT by the appropriate Airline Designator. General Cargo Rate (GCR)普货运价A cargo rate established for the carriage of general cargo.Interpretation – Formal官方解释An informal interpretation subsequently adopted by a General Prorate Meeting. Interpretation – Informal非官方解释An interpretation made by the Prorate Agent at the request of one or more signatories. Such informal interpretation to be placed on the Agenda of the next Prorate Meeting for adoption.Interline Carriage联运Carriage over the section of two or more airlines.Joint Revenue联运收入The revenue for interline carriage between city of origin and city of destination shall be comprised of the Weight Charge and Valuation Charge only. All other charges, such as fuel surcharges, security surcharges, Transhipment and terminal charges, customs clearance, COD fees, etc. are excluded.Mixed Consignments混运货物A consignment of different commodities, articles or goods, whether packed or tied together or contained in separate packages: provided that a consignment consisting wholly of different articles or goods qualifying for the same rate and conditions need not be considered to be a mixed consignment.Multilateral Rate or ChargeThe Rate or Charge currently published in TACT as being applicable for carriage of goods between the city of origin and city of destination. It shall comprise either a rate for a unit of weight (or volume) and/or a flat amount applicable to a shipment (minimum charge or charge for a shipment carried in unit load devices).This Rate or Charge shall not be identified in TACT by an airline designator.Non-Signatory非签约航空公司An airline who has not signed the Multilateral Proration Agreement - Cargo.Portion部分航段Carriage by air or surface between any two consecutive points within a section. Prorate Manual - Cargo (PMC)货运分摊手册The manual produced by the Prorate Working Group - Cargo and the Prorate Agency containing Prorate Factors, Provisos/Requirements, Prorate Agreements and relevant information pertaining thereto.Proviso固定收费A provision made and filed by a signatory for a share of joint revenue not in accordance with the proration rules of the Multilateral Proration Agreement - Cargo. Rate运价The amount charged for the carriage of a unit of goods which is currently published in TACT as being applicable for carriage of a unit of weight (or volume) between the city of origin and city of destination,Rate, Class等级货物运价A rate applicable to a specifically designated class of goods.Rate, Full Applicable Local适用的当地运价The rate or charge which would have been charged for the shipment over the section concerned in the direction of carriage had such section been the complete carriage. Rate, Normal N运价The under 45 kilogramme rate established for General Cargo.Note: In certain areas under 45 kilogramme rates do not exist.In such cases the Normal Rate will be the highest rate established for General Cargo.Rate, Specific Commodity指定商品运价A rate applicable to carriage of specifically designated commodities.Requirement非签约航空公司的固定收费A notification by a non-signatory for a share of joint revenue not in accordance with the proration rules of the Multilateral Proration Agreement - Cargo.Section连续航段Carriage by air and/or surface between two or more points performed consecutively by one airline.Shortest Operated Mileage (SOM)直飞里程The Shortest Operated Mileage is that which is operated by one consecutive carrier. This will be either a non-stop sector, or a combination of sectors on the same carrier, provided there is no direct service between the points concerned, by the same carrier.Note for information- The carriers used will be those contained in the OAG schedule database excluding those passenger only airlines as designated as such in theIATA Airline Coding Directory as at the cut-off date for submissions to the subsequent issue of the PMC.Signatory签约航空公司An airline which has signed the Multilateral Proration Agreement - Cargo.TACT航空货运手册The Air Cargo Tariff published by IATA.Transhipment转运The unloading of cargo from one flight and loading to another for onward carriage. Valuation Charge声明价值附加费The amount resulting from the application of a percentage based on a declared value for carriage of goods (valuation charge).Weight Charges航空运费The amount resulting from multiplication of a rate by the chargeable weight, or a flat amount applicable to a shipment (minimum charge or charge for a shipment carried in unit load devices).。
Sampling and sample size calculation 样本量计算方法大全
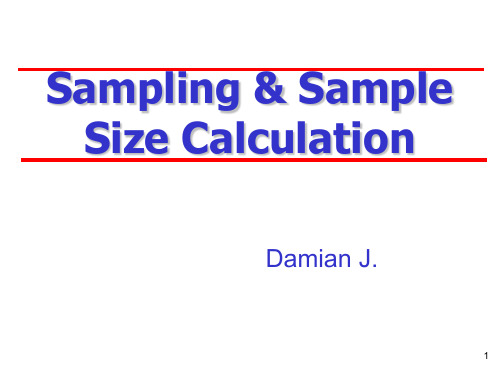
Non-probability samples (3):
Homogeneous sampling
• Sometimes a researcher would like to have specific information about one particular group only (e.g. a group that, for unclear reasons, is more at risk than others) • Example:
13
Maximum variation sampling
• If a researcher wants to obtain as complete as possible insight in a certain issue in all its variations, maximum variation sampling will be used • Examples: – Stigma (e.g. of leprosy, TB, HIV, epilepsy) is considered a complicating factor in the control of diseases: to obtain insight in how stigma manifests itself in different characteristics (cultures, sex, area: rural/urban, in well-to-do and poor patients), a researcher has to take care that all these groups are included in the sample. – To assess whether social distance influences stigma, one could also interview blood relatives (parents or children), spouses, friends, neighbours of patients. • If a researcher is interested in specific groups and interviews a fixed number per group, this type of sampling is also called QUOTA sampling.
SAMPLESIZEANDPOW...
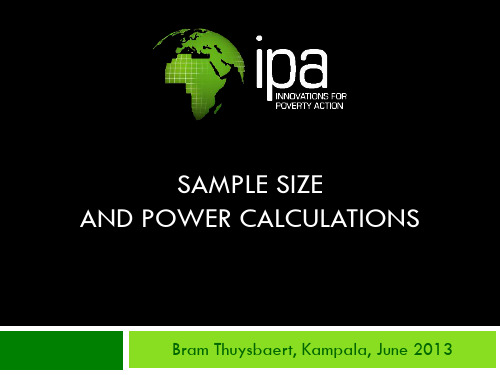
0.35
0.3
H0
0.25 0.2
Hβ
0.15
0.1
0.05
0
-4
-3
-2
-1
0
1
2
3
4
5
6
Power of the test
0.5
0.45
0.4
0.35
0.3
H0
0.25 0.2
Hβ
0.15
0.1
0.05
0
-4
-3
-2
-1
0
1
2
3
4
5
6
Power: main ingredients
1. Effect Size 2. Sample Size 3. Variance 4. Clustering 5. Non-Compliance
160 140 120 100
80 60 40 20
0
0 5 10 15 20 25 30 35 40 45 50 55 60 65 70 75 80 85 90 95 100
test scores
control treatment
Two key statistical principles
1. THE LAW OF LARGE NUMBERS The more observations you have, the closer most averages are to the true average
0.5
0.45
0.4
0.35
0.3
0.25
0.2
0.15
0.1
0.05
0
-4
-3
-2
商务统计习题

Practice Test 1 Business Statistics:Multiple Choice: Each is worth two pointsIdentify the letter of the choice that best completes the statement or answers the question.**Skip 15-17,18,20 Ch10-12,13 Material____ 1. The sum of the relative frequencies 频率for all classes will always equala. the sample sizeb. the number of classesc. oned. any value larger than one____ 2. The interquartile range四分位差 isa. the 50th percentileb. another name for the variancec. the difference between the largest and smallest valuesd. the difference between the third quartile and the first quartile____ 3. The standard deviation 标准偏差of a sample of 100 observations观察值 equals 64. The variance of the sample equalsa. 8b. 10c. 6400d. 4,096____ 4. The numerical value数值 of the standard deviation can never bea. larger than the varianceb. Zeroc. Negatived. smaller than the variance____ 5. The set of all possible sample points (experimental outcomes) is calleda. a sampleb. an eventc. the sample spaced. a population____ 6. A random variable 随机变量that can assume假设 only a finite 有限number of values is referred to as a(n)a. infinite sequenceb. finite sequencec. discrete random variabled. discrete probability functionExhibit展示 5-11A local bottling 瓶装company has determined the number of machine breakdowns per month and their respective probabilities asshown below:Number ofBreakdowns Probability0 0.121 0.382 0.253 0.184 0.07____ 7. Refer to Exhibit 5-11. The probability of at least 3 breakdowns in a month is????a. 0.5b. 0.10c. 0.30d. 0.90____ 8. A normal probability distribution概率分布a. is a continuous probability distributionb. is a discrete离散 probability distributionc. can be either continuous or discreted. must have a standard deviation of 1Exhibit 6-6The starting salaries of individuals 个体with an MBA degree are normally distributed with a mean of $40,000 and a standarddeviation of $5,000.____ 9. Refer to Exhibit 6-6. What percentage of MBA's will have starting salaries of $34,000 to $46,000?a. 38.49%b. 38.59%c. 50%d. 76.98%____ 10. Given that Z is a standard normal random variable, what is the value of Z if the area between -Z and Z is 0.901?a. 1.96b. -1.96c. 0.4505d. ±1.65____ 11. Which of the following is not a measure 估计of central location中位值?a. meanb. Median中间值c. varianced. Mode众数____ 12. The descriptive measure of dispersion离差量度 that is based on the concept of a deviation about the mean isa. the rangeb. the interquartile rangec. the absolute 绝对value of the ranged. the standard deviation____ 13. Which of the following symbols represents the mean of the population?a. σ2b. σc. μd.____ 14. Which of the following symbols represents the size of the samplea. σ2b. σc. Nd. n____ 15. If two events are independent, thena. they must be mutually exclusiveb. the sum of their probabilities must be equal to onec. their intersection交集 must be zerod. None of these alternatives is correct..____ 16. Which of the following statements is(are) always true???????a. -1 ≤ P(E i) ≤1b. P(A) = 1 - P(A c)c. P(A) + P(B) = 1d. ∑P ≥ 1____ 17. A measure of the average value of a random variable is called a(n)a. varianceb. standard deviationc. expected valued. coefficient of variation____ 18. Four percent of the customers of a mortgage company default on their payments. A sample of five customers is selected. What is the probability that exactly two customers in the sample will default on their payments?a. 0.2592b. 0.0142c. 0.9588d. 0.7408____ 19. The expected value of a discrete random variable????a. is the most likely or highest probability value for the random variableb. will always be one of the values x can take on, although it may not be the highest probability value forthe random variablec. is the average value for the random variable over many repeats of the experimentd. None of these alternatives is correct.____ 20. Which of the following is not a property of a binomial 二项式experiment?a. the experiment consists of a sequence of n identical trialsb. each outcome can be referred to as a success or a failurec. the probabilities of the two outcomes can change from one trial to the nextd. the trials are independentExhibit 5-9The probability distribution for the daily sales at Michael's Co. is given below.Daily Sales(In $1,000s) Probability40 0.150 0.460 0.370 0.2____ 21. Refer to Exhibit 5-9. The expected daily sales area. $55,000b. $56,000c. $50,000d. $70,000____ 22. For a standard normal distribution, the probability of z 0 isa. zerob. -0.5c. 0.5d. oneExhibit 6-2The weight of football players is normally distributed with a mean of 200 pounds and a standard deviation of 25 pounds.____ 23. Refer to Exhibit 6-2. What percent of players weigh between 180 and 220 pounds?a. 28.81%b. 0.5762%c. 0.281%d. 57.62%Exhibit 6-6The starting salaries of individuals with an MBA degree are normally distributed with a mean of $40,000 and a standard deviationof $5,000.____ 24. Refer to Exhibit 6-6. What is the probability that a randomly selected individual with an MBA degree will get a starting salary of at least $47,500?a. 0.4332b. 0.9332c. 0.0668d. 0.5000Short Answer/Problems1.The following data represent the daily demand (y in thousands of units) and the unit price (x in dollars) for a product.Daily Demand (y) Unit Price (x)47 139 335 544 334 620 815 1630 6a. Compute and interpret the sample covariance协方差 for the above data.b. Compute and interpret the sample correlation coefficient.相关系数2.The daily dinner bills in a local restaurant are normally distributed with a mean of $28 and a standard deviation of $6.a. What is the probability that a randomly selected bill will be at least $39.10?b. What percentage of the bills will be less than $16.90?c. What are the minimum and maximum of the middle 95% of the bills?d. If twelve of one day's bills had a value of at least $43.06, how many bills did the restaurant collect on that day?3.Below you are given a partial computer output based on a sample of 7 observations, relating an independent variable (x) and a dependentvariable (y).Predictor Coefficient Standard ErrorConstant 24.112 8.376x -0.252 0.253Analysis of VarianceSOURCE SSRegression 196.893Error 94.822a. Develop the estimated regression line.b. If you are given that x = 50, find the estimate of y based on your regression equation.c. Determine the coefficient of determination and interpret your answer.Solutions to MC1. ANS:C2.ANS:D3.ANS:D4.ANS:C5.ANS:C6.ANS:C7.ANS:D8.ANS:A9. ANS:D10.ANS:D11. ANS: C12.ANS:D13.ANS: C14.ANS:D15.ANS: D16.ANS:B17.ANS: C18. ANS: B19. ANS: C20.ANS:C21.ANS: B22.ANS:C23.ANS: D24.ANS:C25.ANS: C26. ANS: A27. ANS: B28. ANS: D29. ANS: CSolutions to Short Answer1. ANS:a. -47 (rounded). Since the covariance is negative, it indicates a negative relationship between x and y.b. -0.922. There is a strong negative relationship between x and y.2. ANS:a. 0.0322b. 0.0322c. minimum = $16.24 maximum = $39.06d. 2,0003. ANS:a.= 24.112 + 0.816xb.If x = 50 then = 24.112 + 0.816x 24.1 + 0.82 ( 50 ) = 65.1c. 0.675 So there is a fairly strong positive relationship between x and y.Practice Test 2 Business Statistics:Multiple Choice: Each is worth two pointsIdentify the letter of the choice that best completes the statement or answers the question.____ 1. The sample statistic s is the point estimator ofa. μb. σc.d.____ 2. A sample statistic is an unbiased estimator of the population parameter ifa. the expected value of the sample statistic is equal to zerob. the expected value of the sample statistic is equal to onec. the expected value of the sample statistic is equal to the population parameterd. it is equal to zero____ 3. A property of a point estimator that occurs whenever larger sample sizes tend to provide point estimates closer to the population parameter is known asb. unbiased samplingc. consistencyd. relative estimation____ 4. A random sample of 121 bottles of cologne showed an average content of 4 ounces. It is known that the standard deviation标准差of the contents (i.e., of the population) is 0.22 ounces. In this problem the 0.22 isa. a parameter参数b. a statisticc. the standard error标准误差 of the meand. the average content of colognes in the long run____ 5. A sample of 92 observations is taken from an infinite population. The sampling distribution of is approximately大约a. normal because is always approximately normally distributedb. normal because the sample size is small in comparison to the population sizec. normal because of the central limit theorem中心极限定理d. None of these alternatives is correct.____ 6. As the number of degrees of freedom for a t distribution increases, the difference between the t distribution and the standard normal distributiona. becomes largerb. becomes smallerc. stays the samed. None of these alternatives is correct.____ 7. From a population of 200 elements, a sample of 49 elements is selected. It is determined that the sample mean is 56 and the sample standard deviation is 14. The standard error of the mean isa. 3b. 2c. greater than 2d. less than 2____ 8. Which of the following is(are) point estimator(s)?a. σb. μc. sd. α____B 9. A population characteristic, such as a population mean总体平均值, is calleda. a statisticb. a parameterc. a sampled. the mean deviation___A_ 10. The sample statistic, such as , s, or , that provides the point estimate of the population parameter is known asa. a point estimatorb. a parameterc. a population parameterd. a population statistic____A 11. The fact that the sampling distribution of sample means can be approximated by a normal probability distribution whenever the sample size is large is based on thea. central limit theoremb. fact that we have tables of areas for the normal distributionc. assumption that the population has a normal distributiond. None of these alternatives is correct.____C 12. Random samples of size 17 are taken from a population that has 200 elements, a mean of 36, and a standard deviation of 8. The mean and the standard deviation of the sampling distribution of the sample means area. 8.7 and 1.94c. 36 and 1.86d. 36 and 8____A 13. When constructing a confidence interval for the population mean and a small sample is used, the degrees of freedom for the t distribution equalsa. n-1b. nc. 29d. 30____A_ 14. The collection of all possible sample points in an experiment isa. the sample spaceb. a sample pointc.an experimentd. the population_____D 15. Of five letters (A, B, C, D, and E), two letters are to be selected at random. How many possible selections are there?a. 20b. 7c. 5!d. 10_____B 16. The “Top Three” at a racetrack consists of picking the correct order of the first three horses in a race. If there are 10 horses in a particular race, how many “Top Three” outcomes are there?a. 302,400b. 720c. 1,814,400d. 10_____ C17. Given that event E has a probability of 0.25, the probability of the complement 补足of event Ea. cannot be determined with the above informationb. can have any value between zero and onec. must be 0.75d. is 0.25_____A 18. The symbol ⋃ shows thea.union of eventsb. intersection of eventsc.sum of the probabilities of eventsd. sample space_____B19. If P(A) = 0.38, P(B) = 0.83, and P(A ⋂ B) = 0.57; then P(A ⋃ B) =a. 1.21b. 0.64c. 0.78d. 1.78_____ B20. If P(A) = 0.62, P(B) = 0.47, and P(A ⋃ B) = 0.88; then P(A ⋂ B) =a. 0.2914b. 1.9700c. 0.6700d. 0.2100_____D 21. If P(A) = 0.85, P(A ⋃ B) = 0.72, and P(A ⋂ B) = 0.66, then P(B) =a. 0.15b. 0.53c. 0.28d. 0.15_____D 22. Two events are mutually exclusive ifa. the probability of their intersection is 1b. they have no sample points in commonc. the probability of their intersection is 0.5d. the probability of their intersection is 1 and they have no sample points in commonC 23. If A and B are mutually exclusive events with P(A) = 0.3 and P(B) = 0.5, thenP(A ⋂ B) =a. 0.30b. 0.15c. 0.00d. 0.20_____ C24. If A and B are mutually exclusive events with P(A) = 0.3 and P(B) = 0.5, thenP(A ⋃ B) =a. 0.00b. 0.15c. 0.8d. 0.2____B_ 25. A subset of a population selected to represent the population is aa.subsetb.samplec.small populationd. None of the alternative answers is correct._____A 26. A simple random sample of size n from an infinite population of size N is to be selected. Each possible sample should havea. the same probability of being selectedb. a probability of 1/n of being selectedc. a probability of 1/N of being selectedd. a probability of N/n of being selected_____D 27. A probability distribution for all possible values of a sample statistic is known as aa.sample statisticb.parameterc.simple random sampled.sampling distribution_____D 28. From a population of 200 elements, the standard deviation is known to be 14. A sample of 49 elements is selected. It is determined that the sample mean is 56. The standard error of the mean isa. 3b. 2c. greater than 2d. less than 2_____ D29. From a population of 500 elements, a sample of 225 elements is selected. It is known that the variance of the population is 900. The standard error of the mean is approximatelya. 1.1022b. 2c. 30d. 1.4847Short Answer/ProblemsDirections: Clearly designate your solution to each portion of the questions asked and show yourentire work and method for arriving at the solution.1. The sales records of a real estate agency show the following sales over the past 200 days:b. Assign probabilities to the sample points and show their values.c. What is the probability that the agency will not sell any houses in a given day?d. What is the probability of selling at least 2 houses?e. What is the probability of selling 1 or 2 houses?f. What is the probability of selling less than 3 houses?2. Assume two events A and B are mutually exclusive and, furthermore, P(A) = 0.2 and P(B) = 0.4.a. Find P(A ⋂ B).b. Find P(A ⋃ B).c. Find P(A⎜B).3.You are given the following information on Events A, B, C, and D. P(A) = .4, P(B) = .2, P(C) = .1,P(A ⋃ D) = .6, P(A⎜B) = .3, P(A ⋂ C) = .04, P(A ⋂ D) = .03a. Compute P(D).b. Compute P(A ⋂ B).c. Compute P(A⎜C).d. Compute the probability of the complement of C.e. Are A and B mutually exclusive? Explain your answer.f. Are A and B independent? Explain your answer.g. Are A and C mutually exclusive? Explain your answer.h. Are A and C independent? Explain your answer.4. Consider a population of five weights identical in appearance but weighing 1, 3, 5, 7, and 9ounces.a. Determine the mean and the variance of the population.b. Sampling without replacement from the above population with a sample size of 2 produces ten possiblesamples. Using the ten sample mean values, determine the mean of the population and the variance of .c. Compute the standard error of the mean.5. A population of 1,000 students spends an average of $10.50 a day on dinner. The standarddeviation of the expenditure is $3. A simple random sample of 64 students is taken.a. What are the expected value, standard deviation, and shape of the sampling distribution of the sample mean?b. What is the probability that these 64 students will spend a combined total of more than $715.21?c. What is the probability that these 64 students will spend a combined total between $703.59 and $728.45? Solutions to MC Problems1. ANS: B2ANS:C3.ANS:C4. ANS:A5. ANS:C6. ANS:B7.ANS:D 8.ANS:C9.ANS:B10. ANS:A11. ANS: A12.ANS:C13.ANS: A14.ANS:A15.ANS: D16.ANS:B17.ANS: C18.ANS:A19. ANS: B20.ANS:D21.ANS: B22.ANS:B23.ANS:C24.ANS:C25. ANS: B26. ANS: A27. ANS: D28.ANS: D29. ANS: DShort Answer/ProblemsDirections: Clearly designate your solution to each portion of the questions asked and show yourentire work and method for arriving at the solution.1. ANSWERS:2. ANSWERS:a. 0.0 b. 0.6 c. 0.03ANSWERS:a. 0.23b. 0.06c. 0.4d. 0.9e. No, P(A⎜B) ≠ 0f. No, P(A⎜B) ≠ P(A)g. No, P(A ⋂ C) ≠ 0h. Y es, P(A⎜C) = P(A)4. ANSWERS:a. 5 and 8b. 5 and 3c. 1.7325. ANSWERS:a. 10.5 0.363 normalb. 0.0314c. 0.0794。
DYNAMICLIGHTSCATTERING-BiophysicsFacility动态光散射..
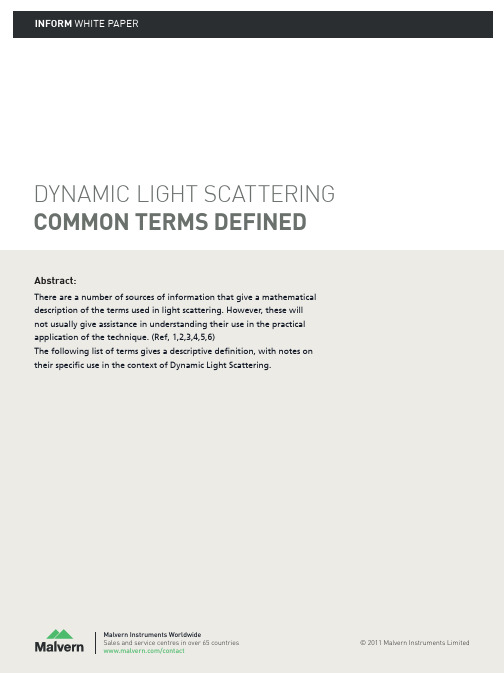
DYNAMIC LIGHT SCATTERING COMMON TERMS DEFINEDZ-Average sizeThe Z-Average size or Z-Average mean used in dynamic light scatteringis a parameter also known as the cumulants mean. It is the primary and most stable parameter produced by the technique. The Z-Average mean is the best value to report when used in a quality control setting as it is defi ned in ISO 13321 and more recently ISO 22412 which defi nes this mean as the ‘harmonic intensity averaged particle diameter’.The Z-average size will only be comparable with the size measuredby other techniques if the sample is monomodal (i.e. only one peak), spherical or near-spherical in shape, monodisperse (i.e. very narrow width of distribution), and the sample is prepared in a suitable dispersant, as the Z-Average mean size can be sensitive to even small changes in the sample, e.g. the presence of a small proportion of aggregates. It should be noted that the Z-average is a hydrodynamic parameter and is therefore only applicable to particles in a dispersion or molecules in solution. Cumulants analysisThis is a simple method of analysing the autocorrelation function generated by a DLS experiment. The calculation is defi ned in ISO 13321 and ISO 22412. As it is a moments expansion it can produce a numberof values, however only the fi rst two terms are used in practice, a mean value for the size (Z-Average), and a width parameter known as the Polydispersity Index (PdI). The Z-Average is an Intensity-based calculated value and should never be confused with or directly compared to a Mass or Number mean value produced by other methods. The calculation isdefi ned in the ISO standards, so all systems that use this calculation as recommended should give comparable results if the same scattering angle is used.Polydispersity IndexThis index is a number calculated from a simple 2 parameter fi t to the correlation data (the cumulants analysis). The Polydispersity Index is dimensionless and scaled such that values smaller than 0.05 are rarely seen other than with highly monodisperse standards. Values greaterthan 0.7 indicate that the sample has a very broad size distribution andis probably not suitable for the dynamic light scattering (DLS) technique. The various size distribution algorithms work with data that falls between these two extremes. The calculations for these parameters are defi ned in the ISO standard document 13321:1996 E and ISO 22412:2008. PolydispersityIn light scattering, the term polydispersity and % polydispersity are derived from the Polydispersity Index, a parameter calculated froma Cumulants analysis of the DLS-measured intensity autocorrelation function. In the Cumulants analysis, a single particle size mode is assumed and a single exponential fi t is applied to the autocorrelation functionand the polydispersity describes the width of the assumed Gaussian distribution. In terms of a protein analysis, a % polydispersity less than 20% indicates that the sample is monodisperse.Diffusion Coeffi cientParticles and molecules in suspension/solution undergo Brownian motion. This is the motion induced by the bombardment by solvent molecules that themselves are moving due to their thermal energy. If the particles or molecules are illuminated with a laser, the intensity of the scattered light fl uctuates at a rate that is dependent upon the size of the particles as smaller particles are “kicked” further by the solvent molecules and move more rapidly. Analysis of these intensity fl uctuations yields the velocity of the Brownian motion and hence the particle size using the Stokes-Einstein relationship. The diffusion coeffi cient, therefore, defi nes this Brownian motion of the analyte or particle in that particular solvent environment. The translational diffusion coeffi cient will depend not only on the size of the particle “core” but also on any surface structure, as well as the concentration and type of ions in the medium. Hydrodynamic diameterThe hydrodynamic size measured by Dynamic Light Scattering (DLS) isdefi ned as “the size of a hypothetical hard sphere that diffuses in the same fashion as that of the particle being measured”. In practice though, particles or macromolecules in solution are non-spherical, dynamic (tumbling), and solvated. Because of this, the diameter calculated from the diffusional properties of the particle will be indicative of the apparent size of the dynamic hydrated/solvated particle. Hence the terminology, Hydrodynamic diameter. The hydrodynamic diameter, or Stokes diameter, therefore is that of a sphere that has the same translational diffusion coeffi cient as the particle being measured, assuming a hydration layer surrounding the particle or molecule.Correlation Curve – or correlation functionThe measured data in a dynamic light scattering (DLS) experiment isthe correlation curve which should be a smooth, single exponential decay function for a mono-size particle dispersion. Embodied withinthe correlation curve is all of the information regarding the diffusion of particles within the sample being measured. By fi tting the correlation curve to an exponential function, the diffusion coeffi cient (D) can be calculated (D is proportional to the lifetime of the exponential decay). With the diffusion coeffi cient (D) now known, the hydrodynamic diameter can be calculated by using a variation of the Stokes-Einstein equation. For a polydisperse sample this curve is a sum of exponential decays.Y-Intercept or InterceptIn DLS the Y-Intercept, or more simply Intercept, refers to the intersection of the correlation curve on the y-axis of the correlogram. The y-intercept can be used to evaluate the signal-to-noise ratio from a measured sample and thus is often used to judge data quality. It is usually scaled suchthat an ideal signal will give a value of 1, and a good system will give intercepts in excess of 0.6, and greater than 0.9 for the best systems.Deconvolution or Deconvolution algorithmAn algorithm-based approach to resolving a mixture of exponentials derived from a polydisperse sample into a number of intensity values each associated with a discrete size band. The particle size distribution from dynamic light scattering (DLS) is derived from a deconvolution of the measured intensity autocorrelation function of the sample. Generally, this is accomplished using a non-negatively constrained least squares (NNLS) fi tting algorithm, a common examples being CONTIN.Count Rate or Photon Count RateIn DLS this is simply the number of photons detected and is usually stated in a “per second” basis. This is useful for determining the sample quality, by monitoring its stability as a function of time, and is alsoused to set instrument parameters such as the attenuator setting and sometimes analysis duration. The count rate needs to be above some minimum value in order to have enough signal for analysis, however all detectors have a maximum count rate where the response remains linear,and if the count rate is not adjusted automatically, the manufacturer recommendations for adjusting the count rate must be observed.Intensity DistributionThe fi rst order result from a DLS experiment is an intensity distribution of particle sizes. The intensity distribution is naturally weighted according to the scattering intensity of each particle fraction or family. For biological materials or polymers the particle scattering intensity is proportional to the square of the molecular weight. As such, the intensity distribution can be somewhat misleading, in that a small amount of aggregation/ agglomeration or presence or a larger particle species can dominate the distribution. However this distribution can be used as a sensitive detector for the presence of large material in the sample.Volume DistributionAlthough the fundamental size distribution generated by DLS is an intensity distribution, this can be converted, using Mie theory, to a volume distribution or a distribution describing the relative proportion of multiple components in the sample based on their mass or volume rather than based on their scattering (Intensity.)When transforming an intensity distribution to a volume/mass distribution, there are 4 assumptions that must be accepted.• All particles are spherical• All particles are homogeneous• The optical properties of the particles are known, i.e. the real & imaginary components of the refractive index• There is no error in the intensity distributionAn understanding of these assumptions is particularly important since the DLS technique itself produces distributions with inherent peak broadening, so there will always be some error in the representation of the intensity distribution. As such, volume and number distributions derived from these intensity distribution are best used for comparative purposes, or for estimating the relative proportions where there are multiple modes, or peaks, and should never be considered absolute. Itis therefore good practice to report the size of the peak based on an intensity analysis and report the relative percentages only (not size) from a Volume distribution analysis.References:[1] International Standard ISO13321 Methods for Determination of Particle Size Distribution Part 8: Photon Correlation Spectroscopy, International Organisation for Standardisation (ISO) 1996.[2] ] International Standard ISO22412 Particle Size Analysis – DynamicLight Scattering, International Organisation for Standardisation (ISO) 2008.[3] Dahneke, B.E. (ed) Measurement of Suspended Particles by Quasi-elastic Light Scattering, Wiley, 1983.[4] Pecora, R. Dynamic Light Scattering: Applications of Photon Correlation Spectroscopy, Plenum Press, 1985.[5] Washington, C. Particle Size Analysis In Pharmaceutics And Other Industries: Theory And Practice, Ellis Horwood, England, 1992.[6] Johnson, C.S. Jr. and Gabriel, D.A. Laser Light Scattering, Dover Publications, Inc., New York 1981Malvern Instruments WorldwideSales and service centres in over 65 countries /contact All information supplied within is correct at time of publication.Malvern Instruments pursues a policy of continual improvement due to technical development. We therefore reserve the right to deviate from information, descriptions, and speci fi cations in this publication without notice. Malvern Instruments shall not be liable for errors contained herein or for incidental or consequential damages in connection with the furnishing, performance or use of this material.Malvern and the ‘hills’ logo, Bohlin, Gemini, Insitec, ISys, Kinexus, Mastersizer, Morphologi,Rosand, SyNIRgi, Viscotek and Zetasizer, are International Trade Marks owned by Malvern Instruments Ltd.Malvern Instruments LimitedGrovewood Road, Malvern,Worcestershire, UK. WR14 1XZTelephone: +44 (0)1684 892456Facsimile: +44 (0)1684 892789Malvern Instruments is partof Spectris plc, the PrecisionInstrumentation and ControlsCompany.Spectris and the Spectris logo areTrade Marks of Spectris plc.© 2011 Malvern Instruments Limited MRK1764-01。
博世汽车SPC
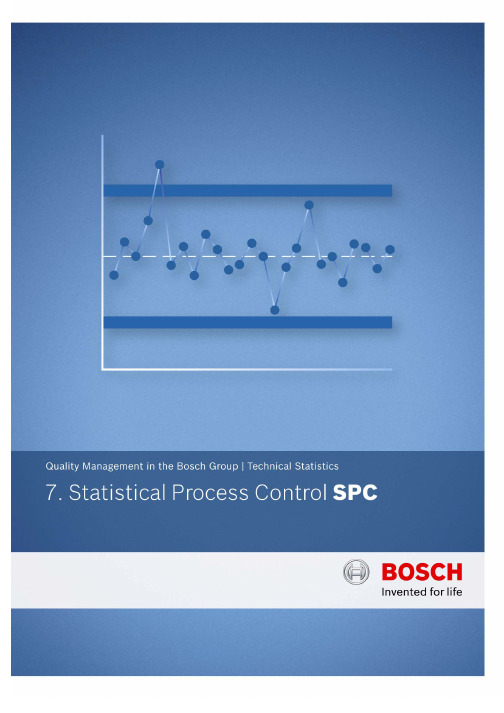
4th Edition, 07.20053rd Edition dated 06.19942nd Edition dated 05.19901st Edition dated 09.19872005 Robert Bosch GmbHTable of ContentsIntroduction (5)1. Terms for Statistical Process Control (6)2. Planning .........................................................................................................................................................8 2.1 Selection of Product Characteristics .................................................................................................8 2.1.1 Test Variable ........................................................................................................................8 2.1.2 Controllability ......................................................................................................................9 2.2 Measuring Equipment .......................................................................................................................9 2.3 Machinery .........................................................................................................................................9 2.4 Types of Characteristics and Quality Control Charts ......................................................................10 2.5 Random Sample Size ......................................................................................................................11 2.6 Defining the Interval for Taking Random Samples (11)3. Determining Statistical Process Parameters ................................................................................................12 3.1 Trial Run .........................................................................................................................................12 3.2 Disturbances ....................................................................................................................................12 3.3 General Comments on Statistical Calculation Methods ..................................................................12 3.4 Process Average ..............................................................................................................................13 3.5 Process Variation . (14)4. Calculation of Control Limits ......................................................................................................................15 4.1 Process-Related Control Limits ......................................................................................................15 4.1.1 Natural Control Limits for Stable Processes ......................................................................16 4.1.1.1 Control Limits for Location Control Charts .........................................................16 4.1.1.2 Control Limits for Variation Control Charts ........................................................18 4.1.2 Calculating Control Limits for Processes with Systematic Changes in the Average .........19 4.2 Acceptance Control Chart (Tolerance-Related Control Limits) .....................................................20 4.3 Selection of the Control Chart .........................................................................................................21 4.4 Characteristics of the Different Types of Control Charts . (22)5. Preparation and Use of Control Charts ........................................................................................................23 5.1 Reaction Plan (Action Catalog) .......................................................................................................23 5.2 Preparation of the Control Chart .....................................................................................................23 5.3 Use of the Control Chart .................................................................................................................23 5.4 Evaluation and Control Criteria ......................................................................................................24 5.5 Which Comparisons Can be Made? (25)6. Quality Control, Documentation .................................................................................................................26 6.1 Evaluation .......................................................................................................................................26 6.2 Documentation .. (26)7. SPC with Discrete Characteristics ...............................................................................................................27 7.1 General ............................................................................................................................................27 7.2 Defect Tally Chart for 100% Testing . (27)8. Tables (28)9. Example of an Event Code for Mechanically Processed Parts ....................................................................29 9.1 Causes .............................................................................................................................................29 9.2 Action ..............................................................................................................................................29 9.3 Handling of the Parts/Goods ...........................................................................................................30 9.4 Action Catalog .. (30)10. Example of an x -s Control Chart (32)11. Literature (33)12. Symbols (34)Index (35)IntroductionStatistical Process Control (SPC) is a procedure for open or closed loop control of manufacturing processes, based on statistical methods.Random samples of parts are taken from the manufacturing process according to process-specific sampling rules. Their characteristics are measured and entered in control charts. This can be done with computer support. Statistical indicators are calculated from the measurements and used to assess the current status of the process. If necessary, the process is corrected with suitable actions.Statistical principles must be observed when taking random samples.The control chart method was developed by Walter Andrew Shewhart (1891-1967) in the 1920´s and described in detail in his book “Economic Control of Quality of Manufactured Product”, published in 1931.There are many publications and self-study programs on SPC. The procedures described in various publications sometimes differ significant-ly from RB procedures.SPC is used at RB in a common manner in all divisions. The procedure is defined in QSP0402 [1] in agreement with all business divisions and can be presented to customers as the Bosch approach.Current questions on use of SPC and related topics are discussed in the SPC work group. Results that are helpful for daily work and of general interest can be summarized and published as QA Information sheets. SPC is an application of inductive statistics. Not all parts have been measured, as would be the case for 100% testing. A small set of data, the random sample measurements, is used to estimate parameters of the entire population.In order to correctly interpret results, we have to know which mathematical model to use, where its limits are and to what extent it can be used for practical reasons, even if it differs from the real situation.We differentiate between discrete (countable) and continuous (measurable) characteristics. Control charts can be used for both types of characteristics.Statistical process control is based on the concept that many inputs can influence a process.The “5 M´s” – man, machine, material, milieu, method – are the primary groups of inputs. Each “M” can be subdivided, e.g. milieu in temperature, humidity, vibration, contamination, lighting, ....Despite careful process control, uncontrolled, random effects of several inputs cause deviation of actual characteristic values from their targets (usually the middle of the tolerance range).The random effects of several inputs ideally result in a normal distribution for the characteristic.Many situations can be well described with a normal distribution for SPC.A normal distribution is characterized with two parameters, the mean µ and the standard deviation σ.The graph of the density function of a normal distribution is the typical bell curve, with inflection points at σµ− and σµ+.In SPC, the parameters µ and σ of the population are estimated based on random sample measurements and these estimates are used to assess the current status of the process.1. Terms for Statistical Process ControlProcessA process is a series of activities and/or procedures that transform raw materials or pre-processed parts/components into an output product.The definition from the standard [3] is: “Set of interrelated or interacting activities which trans-forms inputs into outputs.”This booklet only refers to manufacturing or assembly processes.Stable processA stable process (process in a state of statistical control) is only subject to random influences (causes). Especially the location and variation of the process characteristic are stable over time (refer to [4])Capable processA process is capable when it is able to completely fulfill the specified requirements. Refer to [11] for determining capability indices. Shewhart quality control chartQuality control chart for monitoring a parameter of the probability distribution of a characteristic, in order to determine whether the parameter varies from a specified value.SPCSPC is a standard method for visualizing and controlling (open or closed loop) processes, based on measurements of random samples.The goal of SPC is to ensure that the planned process output is achieved and that corresponding customer requirements are fulfilled.SPC is always linked to (manual or software supported) use of a quality control chart (QCC). QCC´s are filled out with the goal of achieving, maintaining and improving stable and capable processes. This is done by recording process or product data, drawing conclusions from this data and reacting to undesirable data with appropriate actions.The following definitions are the same as or at least equivalent to those in [6].Limiting valueLower or upper limiting valueLower limiting valueLowest permissible value of a characteristic (lower specification limit LSL)Upper limiting valueHighest permissible value of a characteristic (upper specification limit USL)ToleranceUpper limiting value minus lower limiting value:LSLUSLT−=Tolerance rangeRange of permissible characteristic values between the lower and upper limiting valuesCenter point C of the tolerance rangeThe average of the lower and upper limiting values:2LSLUSL C +=Note: For characteristics with one-sided limits (only USL is specified), such as roughness (Rz), form and position (e.g. roundness, perpen-dicularity), it is not appropriate to assume 0=LSL and thus to set 2/USLC= (also refer to the first comment in Section 4.1.1.1).PopulationThe total of all units taken into considerationRandom sampleOne or more units taken from the population or from a sub-population (part of a population)Random sample size nThe number of units taken for the random sample Mean (arithmetic)The sum of theix measurements divided by the number of measurements n:∑=⋅=niixnx11Median of a sampleFor an odd number of samples put in order from the lowest to highest value, the value of the sample number (n+1)/2. For an even number of samples put in order from the lowest to highest value, normally the average of the two samples numbered n/2 and (n/2)+1. (also refer to [13])Example: For a sample of 5 parts put in order from the lowest to the highest value, the median is the middle value of the 5 values.Variance of a sampleThe sum of the squared deviations of the measurements from their arithmetic mean, divided by the number of samples minus 1:()∑=−⋅−=niixxns12211Standard deviation of a sampleThe square root of the variance:2ss=RangeThe largest individual value minus the smallest individual value:minmaxxxR−=2. PlanningPlanning according to the current edition of QSP 0402 “SPC”, which defines responsibilities. SPC control of a characteristic is one possibility for quality assurance during manufacturing and test engineering.2.1 Selection of Product CharacteristicsSpecification of SPC characteristics and their processes should be done as early as possible (e.g. by the simultaneous engineering team). They can also, for example, be an output of the FMEA.This should take• Function,• Reliability,• Safety,•Consequential costs of defects,•The degree of difficulty of the process,• Customer requests, and•Customer connection interfaces, etc.into account.The 7 W-questions can be helpful in specifying SPC characteristics (refer to “data collection” in “Elementary Quality Assurance Tools” [8]): Example of a simple procedure for inspection planning:Why do I need to know what, when, where and how exactly?How large is the risk if I don’t know this? Note: It may be necessary to add new SPC characteristics to a process already in operation. On the other hand, there can be reasons (e.g. change of a manufacturing method or intro-duction of 100% testing) for replacing existing SPC control with other actions.SPC characteristics can be product or process characteristics.Why?Which or what? Which number or how many?Where? Who?When?With what or how exactly?2.1.1 Test VariableDefinition of the “SPC characteristic”, direct or indirect test variable. Note: If a characteristic cannot be measured directly, then a substitute characteristic must be found that has a known relationship to it.2.1.2 ControllabilityThe process must be able to be influenced (controlled) with respect to the test variable. Normally manufacturing equipment can be directly controlled in a manner that changes the test variable in the desired way (small control loop). According to Section 1, “control” in the broadest sense can also be a change of tooling, machine repair or a quality meeting with a supplier to discuss quality assurance activities (large control loop).2.2 Measuring EquipmentDefinition and procurement or check of the measuring equipment for the test variable.Pay attention to:• Capability of measuring and test processes, • Objectiveness,• Display system (digital),• Handling. The suitability of a measurement process for the tested characteristic must be proven with a capability study per [12].In special cases, a measurement process with known uncertainty can be used (pay attention to [10] and [12]).Note: The units and reference value must correspond to the variables selected for the measurement process.2.3 MachineryBefore new or modified machinery is used, a machine capability study must be performed (refer to QSP0402 [1] and [11]). This also applies after major repairs.Short-term studies (e.g. machine capability studies) register and evaluate characteristics of products that were manufactured in one continuous production run. Long-term studies use product measurements from a longer period of time, representative of mass production. Note: The general definition of SPC (Section 1) does not presume capable machines. However, if the machines are not capable, then additional actions are necessary to ensure that the quality requirements for manufactured products are fulfilled.2.4 Types of Characteristics and Control Charts This booklet only deals with continuous anddiscrete characteristics. Refer to [6] for these andother types of characteristics.In measurement technology, physical variables are defined as continuous characteristics. Counted characteristics are special discrete characteristics. The value of the characteristic is called a “counted value”. For example, the number of “bad” parts (defective parts) resulting from testing with a limit gage is a counted value. The value of the characteristic (e.g. the number 17, if 17 defective parts were found) is called a “counted value”.SPC is performed with manually filled out form sheets (quality control charts) or on a computer.A control chart consists of a chart-like grid for entering numerical data from measured samples and a diagram to visualize the statistical indices for the process location and variation calculated from the data.If a characteristic can be measured, then a control chart for continuous characteristics must be used. Normally the sx− chart with sample size 5=n is used.2.5 Random Sample SizeThe appropriate random sample size is a compromise between process performance, desired accuracy of the selected control chart (type I and type II errors, operation characteristic) and the need for an acceptable amount of testing. Normally 5=n is selected. Smaller random samples should only be selected if absolutely necessary.2.6 Defining the Interval for Taking Random SamplesWhen a control chart triggers action, i.e. when the control limits are exceeded, the root cause must be determined as described in Section 5.4, reaction to the disturbance initiated with suitable actions (refer to the action catalog) and a decision made on what to do with the parts produced since the last random sample was taken. In order to limit the financial “damage” caused by potentially necessary sorting or rework, the random sample interval – the time between taking two random samples – should not be too long.The sampling interval must be individually determined for each process and must be modified if the process performance has permanently changed.It is not possible to derive or justify the sampling interval from the percentage of defects. A defect level well below 1% cannot be detected on a practical basis with random samples. A 100% test would be necessary, but this is not the goal of SPC. SPC is used to detect process changes.The following text lists a few examples of SPC criteria to be followed.1. After setup, elimination of disturbances orafter tooling changes or readjustment, measure continuously (100% or with randomsamples) until the process is correctly centered (the average of several measure-ments/medians!). The last measurements canbe used as the first random sample for furtherprocess monitoring (and entered in the control chart). 2. Random sample intervals for ongoingprocess control can be defined in the following manner, selecting the shortest interval appropriate for the process.Definition corresponding to the expected average frequency of disturbances (as determined in the trial run or as is knownfrom previous process experience).Approximately 10 random samples within this time period.Definition depending on specified preventivetooling changes or readjustment intervals.Approximately 3 random samples within thistime period.Specification of tooling changes or readjust-ment depending on SPC random samples.Approximately 5 random samples within theaverage tooling life or readjustment interval.But at least once for the production quantitythat can still be contained (e.g. delivery lot,transfer to the next process, defined lots forconnected production lines)!3. Take a final random sample at the end of aseries, before switching to a different producttype, in order to confirm process capabilityuntil the end of the series.Note: The test interval is defined based on quantities (or time periods) in a manner that detects process changes before defects are produced. More frequent testing is necessary for unstable processes.3. Determining Statistical Process Parameters3.1 Trial RunDefinition of control limits requires knowledge or estimation of process parameters. This is determined with a trial run with sampling size and interval as specified in Sections 2.5 and 2.6. For an adequate number of parts for initial calculations, take a representative number of unsorted parts, at least 25=m samples (with n = 5, for example), yielding no fewer than 125 measured values. It is important to assess the graphs of the measured values themselves, the means and the standard deviations. Their curves can often deliver information on process performance characteristics (e.g. trends, cyclical variations).3.2 DisturbancesIf non-random influences (disturbances) occur frequently during the trial run, then the process is not stable (not in control). The causes of the disturbances must be determined and elimi-nated before process control is implemented (repeat the trial run).3.3 General Comments on Statistical Calculation MethodsComplicated mathematical procedures are no longer a problem due to currently available statistics software, and use of these programs is of course allowed and widespread (also refer to QSP0402 [1]).The following procedures were originally developed for use with pocket calculators. They are typically included in statistics programs.Note: Currently available software programs allow use of methods for preparing, using and evaluation control charts that are better adapted to process-specific circumstances (e.g. process models) than is possible with manual calculation methods. However, this unavoidably requires better knowledge of statistical methods and use of statistics software. Personnel and training requirements must take this into account.Each business division and each plant should have a comprehensively trained SPC specialist as a contact person.Parameter µ is estimated by:Example (Section 10): samplesof number valuesx the of total mxx mj j===∑=1ˆµ3622562862662.......x ˆ=+++==µor:samplesof number mediansthe of total mxx m j j===∑=1~~ˆµ46225626363....x ~ˆ=+++==µIf µˆ significantly deviates from the center point C for a characteristic with two-sided limits, then this deviation should be corrected by adjusting the machine.Parameter σ is estimated by:Example (Section 10):a) ∑=⋅=m j j s m 121ˆσ41125552450550222.......ˆ=+++=σsamplesof number variancesthe of total =σˆNote: s =σˆ is calculated directly from 25 individual measurements taken from sequential random samples (pocket calculator).or b) na s=σˆ, where27125552450550.......s =+++=samplesof number deviationsdard tan s the of total ms s mj j==∑=1351940271...a s ˆn ===σnn a3 0.89 5 0.94 See Section 8, Table 1 7 0.96 for additional valuesor c) ndR =σˆ, with96225611....R =+++= samplesof number rangesthe of total mR R mj j==∑=1271332962...d R ˆn ===σn n d3 1.69 5 2.33 See Section 8, Table 1 7 2.70 for additional values Note: The use of table values n a and n d pre-supposes a normal distribution!Some of these calculation methods were originally developed to enable manual calculation using a pocket calculator. Formula a) is normally used in currently available statistics software.4. Calculation of Control Limits4.1 Process-Related Control LimitsThe control limits (lower control limit LCL andupper control limit UCL) are set such that 99% of all the values lie within the control limits in the case of a process which is only affected by random influences (random causes).If the control limits are exceeded, it must there-fore be assumed that systematic, non-random influences (non-random causes) are affecting the process.These effects must be corrected or eliminated by taking suitable action (e.g. adjustment).Relation between the variance (standard deviation x σ) of the single values (original values, individuals) and the variance (standard deviation x σ) of the mean values: nxx σσ=.4.1.1 Natural Control Limits for Stable Processes4.1.1.1 Control Limits for Location Control Charts (Shewhart Charts)For two-sided tolerances, the limits for controlling the mean must always be based on the center point C. Note: C is replaced by the process mean x =µˆ for processes where the center point C cannot be achieved or for characteristics with one-sided limits.* Do not use for moving calculation of indices!Note: Use of the median-R chart is onlyappropriate when charts are manually filled out, without computer support.n*A E C n c'E EE E3 1.68 1.02 1.16 2.93 1.73 5 1.230.59 1.20 3.09 1.337 1.020.44 1.21 3.19 1.18Estimated values µˆ and σˆ are calculated per Sections 3.4 and 3.5.Refer to Section 8 Table 2 for additional values.Comments on the average chart: For characteristics with one-sided limits (or in general for skewed distributions) and small n , the random sample averages are not necessarily normally distributed. It could be appropriate to use a Pearson chart in this case. This chart has the advantage compared to the Shewhart chart that the control limits are somewhat wider apart. However, it has the disadvantage that calculation of the control limits is more complicated, in actual practice only possible on the computer.Control charts with moving averagesThe x chart with a moving average is a special case of the x chart. For this chart, only single random samples are taken.n sample measurements are formally grouped as a random sample and the average of these n measurements is calculated as the mean.For each new measurement from a single random sample that is added to the group, the first measurement of the last group is deleted, yielding a new group of size n , for which the new average is calculated.Of course, moving averages calculated in this manner are not mutually independent. That is why this chart has a delayed reaction to sudden process changes. The control limits correspond to those for “normal” average charts:σˆn .C LCL ⋅−=582 σˆn.C UCL ⋅+=582Calculate σˆ according to Section 3.5 a)Control limits for )3(1=n :σˆ.C LCL ⋅−=51 σˆ.C UCL ⋅+=51Example for )3(1=n :3 74 741.x = 3 7 4 9 762.x = 3 7 4 9 2 053.x = 3 7 4 9 2 8 364.x =This approach for moving sample measurements can also be applied to the variation, so that an s x − chart with a moving average and moving standard deviation can be used.After intervention in the process or process changes, previously obtained measurements may no longer be used to calculate moving indices.4.1.1.2 Control Limits for Variation Control ChartsThe control limits to monitor the variation (depending on n ) relate to σˆ and s and like-wise R (= “Central line”).s charta) generally applicable formula(also for the moving s x − chart)Example (Section 10):σˆB UCL 'Eob⋅= 62351931...UCL =⋅=σˆB LCL 'Eun ⋅= 30351230...LCL =⋅=b) for standard s x − chartNote: Formula a) must be used in the case ofmoving s calculation. Calculation of σˆ per Section 3.5 a).s B UCL *Eob ⋅= 62271052...UCL =⋅=s B LCL *Eun ⋅=30271240...LCL =⋅=R chartR D UCL Eob ⋅=2696212...UCL =⋅=R D LCL Eun ⋅=70962240...LCL =⋅=Tablen 'Eun B 'Eob B *Eun B *Eob B Eun D Eob D3 5 70.07 0.23 0.34 2.30 1.93 1.76 0.08 0.24 0.35 2.60 2.05 1.88 0.08 0.24 0.34 2.61 2.10 1.91See Section 8, Table 2 for further values4.1.2 Calculating Control Limits for Processes with Systematic Changes in the AverageIf changes of the mean need to be considered as a process-specific feature (trend, lot steps, etc.) and it is not economical to prevent such changes of the mean, then it is necessary to extend the “natural control limits”. The procedure for calculating an average chart with extended control limits is shown below.The overall variation consists of both the “inner” variation (refer to Section 3.5) of the random samples and of the “outer” variation between the random samples.Calculation procedure Control limits for the mean。
英汉对照计量经济学术语
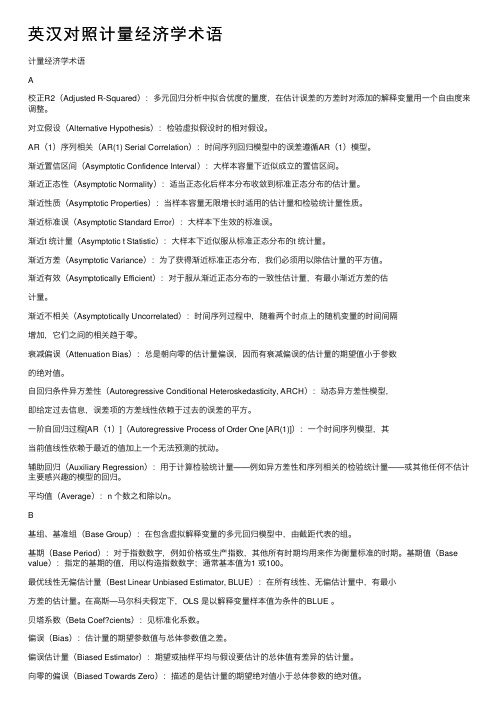
英汉对照计量经济学术语计量经济学术语A校正R2(Adjusted R-Squared):多元回归分析中拟合优度的量度,在估计误差的⽅差时对添加的解释变量⽤⼀个⾃由度来调整。
对⽴假设(Alternative Hypothesis):检验虚拟假设时的相对假设。
AR(1)序列相关(AR(1) Serial Correlation):时间序列回归模型中的误差遵循AR(1)模型。
渐近置信区间(Asymptotic Confidence Interval):⼤样本容量下近似成⽴的置信区间。
渐近正态性(Asymptotic Normality):适当正态化后样本分布收敛到标准正态分布的估计量。
渐近性质(Asymptotic Properties):当样本容量⽆限增长时适⽤的估计量和检验统计量性质。
渐近标准误(Asymptotic Standard Error):⼤样本下⽣效的标准误。
渐近t 统计量(Asymptotic t Statistic):⼤样本下近似服从标准正态分布的t 统计量。
渐近⽅差(Asymptotic Variance):为了获得渐近标准正态分布,我们必须⽤以除估计量的平⽅值。
渐近有效(Asymptotically Efficient):对于服从渐近正态分布的⼀致性估计量,有最⼩渐近⽅差的估计量。
渐近不相关(Asymptotically Uncorrelated):时间序列过程中,随着两个时点上的随机变量的时间间隔增加,它们之间的相关趋于零。
衰减偏误(Attenuation Bias):总是朝向零的估计量偏误,因⽽有衰减偏误的估计量的期望值⼩于参数的绝对值。
⾃回归条件异⽅差性(Autoregressive Conditional Heteroskedasticity, ARCH):动态异⽅差性模型,即给定过去信息,误差项的⽅差线性依赖于过去的误差的平⽅。
⼀阶⾃回归过程[AR(1)](Autoregressive Process of Order One [AR(1)]):⼀个时间序列模型,其当前值线性依赖于最近的值加上⼀个⽆法预测的扰动。
Samplingdistributionofsampleproportions

Sampling distribution of sample proportionsIn these notes you will see how to apply the result known as the Central Limit Theorem to sample proportions. We first review the result. If a random sample is selected and the sample proportion ˆpof a particular outcome (which we call Success) is computed, then, provided the sample size n is large enough (usually above 30), and both , the distribution of sample proportions can be approximately modeled by a Normal model with mean 10,(1)10np n p ≥−≥pand standard deviation , where p is the true proportion of “successes” in the population.Let us look at some examples. Exercise 5 chapter 18. We are going to approach the problem using the Binomial model first. If the student obtained 42% heads in 200 tosses of a coin, it means the number of heads was actually 84. Assuming the tosses were independent, and the coin is fair, so that it has equal chance of landing heads or tails, the number of heads in 200 tosses follows a Binomial model with 200,0.5n p ==. Then in 200 tosses we expect to obtain about 100 heads. Now we know that due to variability the actual number may not be exactly 100. The question we need to answer is whether 84 heads is unusually small so that what we are witnessing is an unlikely event. In order to answer the question we will compute the probability of obtaining 84 or even fewer heads in 200 tosses. So if X is the random variable counting the number of heads we compute: (84)(200,0.5,84)0.0141P X binomcdf ≤==. This result can be interpreted like this: 84 heads or fewer can be observed in about 1.4% of such experiments. Since the probability of the event is so small (much below 0.05) we may conclude either of the following: (a) We are witnessing a very unusual event; (b) The coin may not be fair, so in reality the true proportion of heads may be below0.5, or (c) That student may have made a mistake in counting the number of heads.Now we approach the same problem using the sampling distribution of the sample proportion of the number of heads. Assuming again that the coin is fair, is the true proportion of heads. In 200 tosses we expect about 100 heads and 100 tails, so the conditions are satisfied and we can use the normal model for the sampleproportion. Again, we would like to assess whether 0.5p =ˆ.42p=is unusually small. According to the Central Limit Theorem, the sample proportions follow a Normal modelwith mean and standard deviation 0.5p=0.035σ==. Just for a rough estimate, we can see that .42 is more than two standard deviations below the mean, so this is enough to tell us this is an unusual event to observe. We will be more precise and compute the probability of observing such a low proportion of heads or even lowerthan that, so we will compute (0.42)P X ≤ for a normal model with mean and standard deviation 0.5p=0.035==(0,0.42,0.5,0.42,0.5,0.035)σ. We have . ˆ(0.42)0.035)0.0111P pnormalcdf ≤==Notice that even if the probability we computed is not the same as the one we obtained using the Binomial model, the two values lead to the same conclusion: the probability is very small, so this is a very unlikely event. Note : Review the TI83 command for computing probabilities in the Normal model. Remember that in Chapter 6 when we computed normal probabilities, the probability of the event above was computed as:ˆ(0.42)(99,0.0111P pnormalcdf E ≤=−= Notice that the value is identical to the one computed above, and the reason is that 0 is sufficiently far (more than 3 standard deviations) from the mean 0.5 so that any area to the left of zero is practically zero. Also, it makes more sense from the point of view of computations to look at values between 0 and 0.42 rather than −∞ and 0.42 since the sample proportion can only take values between 0 and 1.Exercise 14. Let us compute the sample proportion of students engaged in binge drinking at that particular college. We have 96ˆ0.393244p ==. Compared to 44% it falls below that value. The question we would like to answer is whereas this proportion is much smaller, or unusually smaller compared to 44%.We first check that the conditions of using the normal model apply. We have a large sample, n=244, and both , and 2440.44=⋅=107.3610np ≥(1)136.64n p 10−=≥.So ˆpfollows a Normal model with mean 0.44 and standard deviation 0.031σ==. We have: ˆ(0.393P p )(0,0.393,0.44,0.031normalcdf )0.0647≤==. So, the probability of having a sample in which he proportion of binge drinking students is 0.393 or even lower is 0.0647. This is not a large probability, but according to our rule for unusual events, the probability is above 0.05, so we cannot classify the event as an unlikely one.Therefore, we should not be very surprised at this result. Again, let us approach the same problem using the Binomial model. We can assume that the number of students who binge drink follows a Binomial model with n=244 and probability of “success” p=0.44. Then, to answer the question in the problem we compute . The probability is not equal to the one obtained using the Normal model, however, since the value is 0.08>0.05, again we conclude the event is not unusual, so we should not be surprised.(96)(0.0802P X binomcdf ≤==244,0.44,96) Exercise 20. First of all, in this problem the answers may vary quite a lot based on everybody’ s understanding of the word “very sure”. So, if we look at the proportionˆp of people who will order the chef’s steak special, it follows a model which is approximately Normal with mean 0.20 and standard deviationσ==0.0298~0.03Arguing that values that are farther than two standard deviation above the mean are unusual, it means it would be unusual to have more than 0.20+0.06=0.26=26% orders for the chef’s special.So, if I were to make a recommendation, I would tell the chef to prepare chef’s special steaks.⋅≈1800.2647Now, to play it even safer, we may prepare the chef’s special for 27.5% (i.e. two and a half standard deviations above the mean) of the expected number of customers, this leading to preparing 50 chef’s special steaks.Now, if you open this document and read it, work problems 18 and 19 following the examples I provided. You need not give two solutions as I gave for exercises 5 and 14, but I would like to see complete solutions with explanations.Write the solutions nicely on paper and bring them on Monday to class for extra credit. You are allowed to work together and discuss the problems but make sure to show your individual work and explanations.。
BayesianPower 软件包说明说明书

Package‘BayesianPower’October12,2022Type PackageTitle Sample Size and Power for Comparing Inequality ConstrainedHypothesesVersion0.2.3Author Fayette KlaassenMaintainer Fayette Klaassen<**************************>Description A collection of methods to determine the required sample size forthe evaluation of inequality constrained hypotheses by means of a Bayesfactor.Alternatively,for a given sample size,the unconditional errorprobabilities or the expected conditional error probabilities can bedetermined.Additional material on the methods in this package isavailable in Klaassen,F.,Hoijtink,H.&Gu,X.(2019)<doi:10.31219/osf.io/d5kf3>.License LGPL-3Encoding UTF-8LazyData trueRoxygenNote7.1.0Suggests knitr,rmarkdown,testthatVignetteBuilder knitrNeedsCompilation noRepository CRANDate/Publication2020-06-2208:40:16UTCR topics documented:bayes_error (2)bayes_power (2)bayes_sampsize (4)calc_bf (5)calc_fc (6)eval_const (6)samp_bf (7)samp_dist (7)1Index9 bayes_error Determine the unconditional error probabilities for a set of simulatedBayes factors.DescriptionDetermine the unconditional error probabilities for a set of simulated Bayes factors.Usagebayes_error(BFs1,BFs2,bound1=1,bound2=1/bound1)ArgumentsBFs1A vector.Simulated BF12under H1for a given nBFs2A vector.Simulated BF12under H2for a given nbound1A number.The boundary above which BF12favors H1bound2A number.The boundary below which BF12favors H2ValueA named vector.The Type1,Type2,Decision error and Area of Indecision probabilities and themedian Bayes factors under H1and H2bayes_power Determine the’power’for a Bayesian hypothesis testDescriptionDetermine the’power’for a Bayesian hypothesis testUsagebayes_power(n,h1,h2,m1,m2,sd1=1,sd2=1,scale=1000,bound1=1,bound2=1/bound1,datasets=1000,nsamp=1000,seed=31)Argumentsn A number.The sample sizeh1A constraint matrix defining H1h2A constraint matrix defining H2m1A vector of expected population means under H1m2A vector of expected populations means under H2m2must be of same length as m1sd1A vector of standard deviations under H1.Must be a single number(equal standard deviation under all populations),or a vector of the same length as m1 sd2A vector of standard deviations under H2.Must be a single number(equal standard deviation under all populations),or a vector of the same length as m2 scale A number specifying the prior scalebound1A number.The boundary above which BF12favors H1bound2A number.The boundary below which BF12favors H2datasets A number.The number of datasets to compute the error probabilitiesnsamp A number.The number of prior or posterior samples to determine thefit and complexityseed A number.The random seed to be setValueThe Type1,Type2,Decision error and Area of Indecision probability and the median BF12s under H1and H2Examples#Short example WITH SMALL AMOUNT OF SAMPLESh1<-matrix(c(1,-1,0,0,1,-1),nrow=2,byrow=TRUE)h2<-"c"m1<-c(.4,.2,0)m2<-c(.2,0,.1)bayes_power(40,h1,h2,m1,m2,datasets=50,nsamp=50)4bayes_sampsize bayes_sampsize Determine the required sample size for a Bayesian hypothesis testDescriptionDetermine the required sample size for a Bayesian hypothesis testUsagebayes_sampsize(h1,h2,m1,m2,sd1=1,sd2=1,scale=1000,type=1,cutoff,bound1=1,bound2=1/bound1,datasets=1000,nsamp=1000,minss=2,maxss=1000,seed=31)Argumentsh1A constraint matrix defining H1.h2A constraint matrix defining H2.m1A vector of expected population means under H1(standardized).m2A vector of expected populations means under H2(standardized).m2must be of same length as m1sd1A vector of standard deviations under H1.Must be a single number(equal standard deviation under all populations),or a vector of the same length as m1 sd2A vector of standard deviations under H2.Must be a single number(equal standard deviation under all populations),or a vector of the same length as m2 scale A number specifying the prior scaletype A character.The type of error to be controlled options are:"1","2","de", "aoi","med.1","med.2"cutoff A number.The cutoff criterion for type.If type is"1","2","de","aoi", cutoff must be between0and1If type is"med.1"or"med.2",cutoff mustbe larger than1calc_bf5 bound1A number.The boundary above which BF12favors H1bound2A number.The boundary below which BF12favors H2datasets A number.The number of datasets to compute the error probabilitiesnsamp A number.The number of prior or posterior samples to determine thefit and complexityminss A number.The minimum sample size to considermaxss A number.The maximum sample size to considerseed A number.The random seed to be setValueThe sample size for which the chosen type of error probability is at the set cutoff,and the according error probabilities and median Bayes factorsExamples#Short computation example NOT SUFFICIENT SAMPLESh1<-matrix(c(1,-1),nrow=1,byrow=TRUE)h2<- cm1<-c(.4,0)m2<-c(0,.1)bayes_sampsize(h1,h2,m1,m2,sd1=1,sd2=1,scale=1000,type="de",cutoff=.125,nsamp=50,datasets=50,minss=40,maxss=70)calc_bf Compute a Bayes factorDescriptionCompute a Bayes factorUsagecalc_bf(data,h1,h2,scale,nsamp=1000)Argumentsdata A matrix.The dataset for which the BF must be computedh1A constraint matrix defining H1.h2A constraint matrix defining H2.scale A number specifying the prior scale.nsamp A number.The number of prior or posterior samples to determine theValueBF12,that is,the evidence for H1relative to H26eval_const calc_fc Compute the complexity orfit for two hypotheses.DescriptionCompute the complexity orfit for two hypotheses.Usagecalc_fc(hyp,hyp2,means,sds,nsamp=1000)Argumentshyp A constraint matrix defining H1.hyp2A constraint matrix defining H2OR a character u or c specifying an un-constrained or complement hypothesismeans A vector of posterior or prior meanssds A vector or posterior or prior standard deviationnsamp A number.The number of prior or posterior samples to determine thefit and complexityValueA vector.The proportion of posterior samples in agreement with H1and with H2eval_const Evaluate a constraint matrix for a set of prior/posterior samplesDescriptionEvaluate a constraint matrix for a set of prior/posterior samplesUsageeval_const(hyp,samples)Argumentshyp A constraint matrix defining a hypothesis.samples A matrix.Prior or posterior samples,the number of columns corresponds to the number of groups,the number of rows the number of samplesValueA number between0and1.The proportion of samples in which the constraints are met.samp_bf7samp_bf Sample multiple datasets and compute the Bayes factor in eachDescriptionSample multiple datasets and compute the Bayes factor in eachUsagesamp_bf(datasets,n,ngroup,means,sds,h1,h2,scale,nsamp)Argumentsdatasets A number.The number of datasets to simulate for each sample size nn A number.The group sample size to be used in data simulationngroup A number.The number of groups.means A vector of expected population means.sds A vector of expected population standard deviations Note,when standardized,this is a vector of1sh1A constraint matrix defining H1.h2A constraint matrix defining H2.scale A number specifying the prior scale.nsamp A number.The number of samples for thefit and complexity See?BayesianPower::calc_fc ValueA vector of Bayes factors BF12for each of the simulated datasetssamp_dist Sample from prior or posterior distributionDescriptionSample from prior or posterior distributionUsagesamp_dist(nsamp,means,sds)8samp_distArgumentsnsamp A number.The number of prior or posterior samples to determine thefit and complexitymeans A vector.The prior or posterior means for each groupsds A number or a vector.The standard deviations for each group If a number is used,the same prior or posterior standard deviation is used for each group.ValueA matrix of nsamp rows and as many columns as the length of means.Indexbayes_error,2bayes_power,2bayes_sampsize,4calc_bf,5calc_fc,6eval_const,6samp_bf,7samp_dist,79。
- 1、下载文档前请自行甄别文档内容的完整性,平台不提供额外的编辑、内容补充、找答案等附加服务。
- 2、"仅部分预览"的文档,不可在线预览部分如存在完整性等问题,可反馈申请退款(可完整预览的文档不适用该条件!)。
- 3、如文档侵犯您的权益,请联系客服反馈,我们会尽快为您处理(人工客服工作时间:9:00-18:30)。
Example Solution
z * p*q* 0.03 n
1.960 (.5)(.5) 0.03 n
1.9600.5 n .03
Choosing the sample size
• Calculate margin of error for proportions just like margin of error for means
• m = z*(S.E.) = z * p q
n
• But S.E. depends on p-hat which you don’t know yet
– You should get n = 1025, so sample size decreased. – If we let p* = .6 instead of .4, how does the answer
change? – What conclusion do you draw?
Today’s Objectives
– Note: p = probability, p-hat = sample proportion
• Write a sentence that states your conclusion
Example
A coin is flipped 4040 times. Heads comes up 2048 times. Is the coin fair?
Ha: p ≠ .5
• TI gives z = .88, p = .38
• Conclusion: There is not enough evidence (p = .38) to conclude that the coin is unfair.
– Note that the calculator multiplied the tail area by 2 automatically, so we get the same result as yesterday.
• Students will use TI to form a confidence interval for proportions.
• Students will calculate the minimum sample size needed to achieve a confidence interval with desired margin of error.
• TI gives (.49151, .52235) • Conclusion: We are 95% confident that
the true proportion of heads is between 49% and 52%
– Note that this INCLUDES 50%, our definition of fair
California Standards: • 18.0 Students determine the P- value for a statistic for a
simple random sample from a normal distribution. • 20.0 Students are familiar with the t distributions and t
State hypotheses, carry out the test on the TI, and write a sentence that states your conclusion about the fairness of the coin.
Example Solution
• Ho: p = .5
Example continued
• For the same coin flip problem (2048 heads in 4040 tosses), state a 95% confidence interval for the true proportion of heads on this coin.
and fail to reject the null.
Confidence Intervals for proportion on the TI
• Select Stat : Tests / 1-PropZInt • Enter the number of success as x • Enter the sample size as n • Choose the appropriate C-Level • Calculate to find the interval • Write a sentence that states your conclusion
• Replace m with desired margin of error
– For example m = .03 for plus/minus 3%
• Solve for n
Example
You want to conduct a poll to estimate support for a candidate with 95% confidence. You want results accurate to within 3%.
• Students will use TI to perform a hypothesis test for proportions.
• Students will use TI to form a confidence interval for proportions.
• Students will calculate the minimum sample size needed to achieve a confidence interval with desired margin of error.
Write hypotheses, calculate the sample proportion, perform a test, and write your conclusion.
Today’s Objectives
• Students will use TI to perform a hypothesis test for proportions.
test and understand their uses.
Homework
• Read pages 669 – 674 • Do problems 10, 11, 13, 14, 15
ห้องสมุดไป่ตู้
Minimum Sample Size -----------
Proportions on the TI
Section 12.1.2
Starter 12.1.2
Some people think that chemists are more likely than others to have female children. Perhaps they are exposed to chemicals that cause this. Between 1980 and 1990 in Washington state, 555 children were born to chemists. Of these births, 273 were girls. During this period, 48.8% of all births in Washington were girls. Is there evidence that the proportion of girls born to chemists is higher than normal?
California Standards: • 18.0 Students determine the P- value for a statistic for a
simple random sample from a normal distribution. • 20.0 Students are familiar with the t distributions and t
test and understand their uses.
Hypothesis tests for proportion on the TI
• Select Stat : Tests / 1-PropZTest • Enter the null hypothesis as po • Enter the number of success as x • Enter the sample size as n • Choose the appropriate alternative hypothesis • Calculate to find z and p
– The z* came from the 95% confidence level. – The p* and q* came from an assumption.
• What if p* ≠ .5? How does that change sample size needed?
– Try a new assumption of p* = .4 and see what that does to the previous calculation.
n 1067.1 So use n = 1068
Follow-up
• And that’s what they really do on large national polls
– They only need a little over 1000 in the sample if valid sampling methods are used.