Measuring Data Believability a Provenance Approach
BELBIN English Self-Perception Inventory-A4

ANSWER SHEETSURNAME (PRINT):Sex: M /FFIRST NAME (PRINT):Organization:Department:Date:Directions for Self-Perception completion:The BELBIN® Self-Perception Inventory (SPI) should be completed, preferably when you can arrange a quiet period free from interruptions. It usually takes about 15 minutes to complete. You should answer the questions after some serious thought, whilst avoiding spending too long on any given section. There are no right or wrong answers.For each section distribute a total of exactly 10 points between the sentences that you think most accurately describe your behaviour. These points may be distributed between several sentences.Try to avoid both extremes of giving one sentence all ten points or allocating one point to every sentence in each section.Please allocate whole numbers only - no fractions or decimals. If you have no points to allocate to a sentence, please leave the box blank.SECTIONI SECTIONIISECTIONIIISECTIONIVSECTIONVSECTIONVISECTIONVIIMARK MARK MARK MARK MARK MARK MARK1.02.03.04.05.06.07.01.12.13.14.15.16.17.11.22.23.24.25.26.27.21.32.33.34.35.36.37.31.42.43.44.45.46.47.41.52.53.54.55.56.57.51.62.63.64.65.66.67.61.72.73.74.75.76.77.71.82.83.84.85.86.87.81.92.93.94.95.96.97.9TOTAL1010101010101070For each section distribute a total of ten marks among the sentences which you think most accurately describe your behaviour. These marks may be distributed among several sentences; in extreme cases they might be spread among all the sentences or 10 marks may be given to a single sentence. However try and avoid either extreme. Enter the points in the INTERPLACE answer sheet provided.I. WHAT I BELIEVE I CANCONTRIBUTE TO A TEAM:1.0I think I can quickly see and take advantage ofnew opportunities.1.1My comments both on general and specificpoints are well received.1.2I can work well with a very wide range ofpeople.1.3Producing ideas is one of my natural assets. 1.4My ability rests in being able to draw peopleout whenever I detect they have something ofvalue to contribute to group objectives.1.5I can be relied upon to finish any task Iundertake.1.6My technical knowledge and experience areusually my major assets.1.7I am prepared to be blunt and outspoken in thecause of making the right things happen.1.8I can usually tell whether a plan or idea will fit aparticular situation.1.9I can offer a reasoned and unbiased case foralternative courses of action.II. IF I HAVE A POSSIBLE SHORTCOMING IN TEAM WORK, IT COULD BE THAT:2.0I am not at ease unless meetings are wellstructured and controlled and generally wellconducted.2.1I am inclined to be too generous towardsothers who have a valid viewpoint that has notbeen given a proper airing.2.2I am reluctant to contribute unless the subjectcontains an area I know well.2.3I have a tendency to talk a lot once the groupgets on to a new topic.2.4I am inclined to undervalue the importance ofmy own contributions.2.5My objective outlook makes it difficult for me tojoin in readily and enthusiastically withcolleagues.2.6I am sometimes seen as forceful andauthoritarian when dealing with importantissues.2.7I find it difficult to lead from the front, perhapsbecause I am over-responsive to groupatmosphere.2.8I am apt to get too caught up in ideas thatoccur to me and so lose track of what ishappening.2.9I am reluctant to express my opinions onproposals or plans that are incomplete orinsufficiently detailed.III. WHEN INVOLVED IN A PROJECT WITH OTHER PEOPLE: 3.0I have an aptitude for influencing peoplewithout pressurising them.3.1I am generally effective in preventing carelessmistakes or omissions from spoiling thesuccess of an operation.3.2I like to press for action to make sure that themeeting does not lose sight of the mainobjective.3.3I can be counted on to contribute somethingoriginal.3.4I am always ready to back a good suggestionin the common interest.3.5One can be sure I will just be my natural self. 3.6I am quick to see the possibilities in new ideasand developments.3.7I try to maintain my sense of professionalism.3.8I believe my capacity for judgement can help tobring about the right decisions.3.9I can be relied on to bring an organisedapproach to the demands of a job.IV. MY CHARACTERISTICAPPROACH TO GROUP WORKIS THAT:4.0I maintain a quiet interest in getting to knowcolleagues better.4.1I contribute where I know what I am talkingabout.4.2I am not reluctant to challenge the view ofothers or to hold a minority view myself.4.3I can usually find an argument to refuteunsound propositions.4.4I think I have a talent for making things workonce a plan has been put into operation.4.5I prefer to avoid the obvious and to open uplines that have not been explored.4.6I bring a touch of perfectionism to any job Iundertake.4.7I like to be the one who makes contactsoutside the group or firm.4.8I enjoy the social side of working relationships.4.9While I am interested in hearing all views Ihave no hesitation in making up my mind oncea decision has to be made.V. I GAIN SATISFACTIONIN A JOB BECAUSE:5.0I enjoy analysing situations and weighing up allthe possible choices.5.1I am interested in finding practical solutions to problems.5.2I like to feel I am fostering good workingrelationships.5.3I can have a strong influence on decisions.5.4I have a chance of meeting new people withdifferent ideas.5.5I can get people to agree on priorities.5.6I feel I am in my element where I can give atask my full attention.5.7I can find an opportunity to stretch myimagination.5.8I feel that I am using my special qualificationsand training to advantage.5.9I usually find a job gives me the chance toexpress myself.VI. IF I AM SUDDENLY GIVEN A DIFFICULT TASK WITH LIMITED TIME and UNFAMILIAR PEOPLE: 6.0I usually succeed in spite of thecircumstances.6.1I like to read up as much as I conveniently canon a subject.6.2I would feel like devising a solution of my ownand then trying to sell it to the group.6.3I would be ready to work with the person whoshowed the most positive approach.6.4I would find some way of reducing the size ofthe task by establishing how differentindividuals can contribute.6.5My natural sense of urgency would help toensure that we did not fall behind schedule. 6.6I believe I would keep my cool and maintainmy capacity to think straight.6.7In spite of conflicting pressures I would pressahead with whatever needed to be done.6.8I would take the lead if the group was makingno progress.6.9I would open up discussions with the view tostimulating new thoughts and gettingsomething moving.VII. WITH REFERENCE TO THE PROBLEMS I EXPERIENCE WHEN WORKING IN GROUPS:7.0I am apt to overreact when people hold upprogress.7.1Some people criticise me for being tooanalytical.7.2My desire to check that we get the importantdetails right is not always welcome.7.3I tend to show boredom unless I am activelyengaged with stimulating people.7.4I find it difficult to get started unless the goalsare clear.7.5I am sometimes poor at putting acrosscomplex points that occur to me.7.6I am conscious of demanding from others thethings I cannot do myself.7.7I find others do not give me enoughopportunity to say all I want to say.7.8I am inclined to feel I am wasting my time andwould do better on my own.7.9I hesitate to express my personal views infront of difficult or powerful people.。
犬耳霜液浆产品说明书
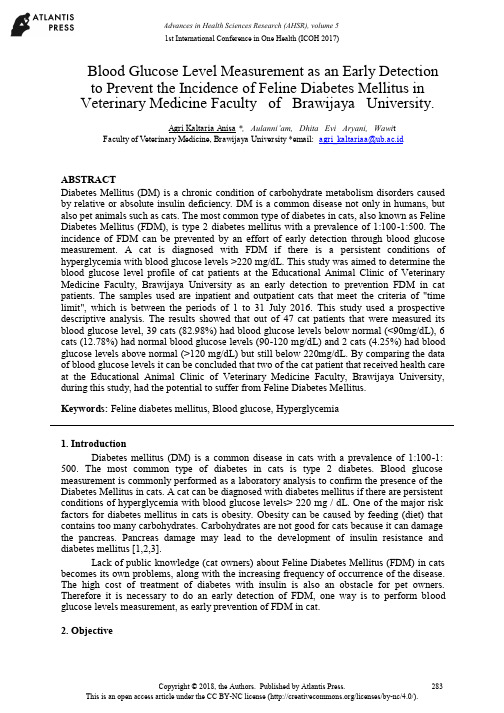
Blood Glucose Level Measurement as an Early Detection to Prevent the Incidence of Feline Diabetes Mellitus in Veterinary Medicine Faculty of Brawijaya University.Agri Kaltaria Anisa *, Aulanni’am, Dhita Evi Aryani, Wawi t Faculty of Veterinary Medicine, Brawijaya University *email: ********************.idABSTRACT Diabetes Mellitus (DM) is a chronic condition of carbohydrate metabolism disorders caused by relative or absolute insulin deficiency. DM is a common disease not only in humans, but also pet animals such as cats. The most common type of diabetes in cats, also known as Feline Diabetes Mellitus (FDM), is type 2 diabetes mellitus with a prevalence of 1:100-1:500. The incidence of FDM can be prevented by an effort of early detection through blood glucose measurement. A cat is diagnosed with FDM if there is a persistent conditions of hyperglycemia with blood glucose levels >220 mg/dL. This study was aimed to determine the blood glucose level profile of cat patients at the Educational Animal Clinic of Veterinary Medicine Faculty, Brawijaya University as an early detection to prevention FDM in cat patients. The samples used are inpatient and outpatient cats that meet the criteria of "time limit", which is between the periods of 1 to 31 July 2016. This study used a prospective descriptive analysis. The results showed that out of 47 cat patients that were measured its blood glucose level, 39 cats (82.98%) had blood glucose levels below normal (<90mg/dL), 6 cats (12.78%) had normal blood glucose levels (90-120 mg/dL) and 2 cats (4.25%) had blood glucose levels above normal (>120 mg/dL) but still below 220mg/dL. By comparing the data of blood glucose levels it can be concluded that two of the cat patient that received health care at the Educational Animal Clinic of Veterinary Medicine Faculty, Brawijaya University, during this study, had the potential to suffer from Feline Diabetes Mellitus.Keywords: Feline diabetes mellitus, Blood glucose, Hyperglycemia1. Introduction Diabetes mellitus (DM) is a common disease in cats with a prevalence of 1:100-1: 500. The most common type of diabetes in cats is type 2 diabetes. Blood glucose measurement is commonly performed as a laboratory analysis to confirm the presence of the Diabetes Mellitus in cats. A cat can be diagnosed with diabetes mellitus if there are persistent conditions of hyperglycemia with blood glucose levels> 220 mg / dL. One of the major risk factors for diabetes mellitus in cats is obesity. Obesity can be caused by feeding (diet) that contains too many carbohydrates. Carbohydrates are not good for cats because it can damage the pancreas. Pancreas damage may lead to the development of insulin resistance and diabetes mellitus [1,2,3]. Lack of public knowledge (cat owners) about Feline Diabetes Mellitus (FDM) in cats becomes its own problems, along with the increasing frequency of occurrence of the disease. The high cost of treatment of diabetes with insulin is also an obstacle for pet owners. Therefore it is necessary to do an early detection of FDM, one way is to perform blood glucose levels measurement, as early prevention of FDM in cat.2. Objective1st International Conference in One Health (ICOH 2017)The objective of this study was to determine the blood glucose profile from the results of blood glucose levels measurement in cat patients (in patients and out patients care) at Veterinary Medicine Faculty of Brawijaya University’s Education Animal Clinic. This was done as one of the early steps to prevent the incidence of Feline Diabetes Mellitus (FDM), especially in patients and out patients care at Veterinary Medicine Faculty of Brawijaya University’s Education Animal Clinic.3. MethodThis study was conducted as an observational study, where the researchers remotely measured the blood sugar levels of cat patients (in patients and out patients) at the Educational Animal Clinic of Veterinary Medicine Faculty, Brawijaya University. The populations used in this study were all patients’cats’ inpatient and outpatient care at the Educational Animal Clinic of Veterinary Medicine Faculty, Brawijaya University. The sample used were cat patients(inpatient and outpatient care) that meets the criteria of a time limit ("time limit"), which is between the period 1 to 31 July 2016. This study used a prospective descriptive analysis.4. Result and DiscussionThe results of the study, that was done during the period 1 to 31 July 2016 at the Educational Animal Clinic of Veterinary Medicine Faculty, Brawijaya University, prospectively obtained a sample of 47 cat patients (inpatients and outpatients care), which consisted of 20 male cats and 27 female cats. The age range of the sample taken was between 3 months to 5 years old, with the majority of the cats are from domestic breed. Blood glucose level were categorized into four groups, namely hypoglycemia (blood glucose <90 mg / dL), normal (90 mg / dL - 120 mg / dL, hyperglycemia (121 mg / dL - 219 mg / dL) and diabetes mellitus ( ≥ 220 mg / dL).The measurement of the blood sugar levels of 47 cat patients, obtained a total of 39 cats (82.98%) had blood glucose levels below normal (hypoglycemia) i.e. <90mg/dL, 6 cats (12.78%) had normal blood glucose levels between 90-120 mg/dL and 2 cats (4.25%) had blood glucose levels above normal (hyperglycemia) i.e. 121-219 mg/dL, but none of the measured cats had a blood glucose ≥ 220 such/dLasshown in table 1and figure 1. Blood glucose level has long been considered as a diagnostic tool for diabetes mellitus. It is commonly performed as a laboratory analysis to confirm the presence of the diabetes mellitus in cats. A cat can be diagnosed with diabetes mellitus if there are persistent conditions of hyperglycemia with blood glucose levels> 220 mg / dL. In this study none of the cat patients has blood glucose ≥ 220 mg/dL, so none of the cat patients are diagnosed with FDM. Although none of the cats are diagnosed to have FDM, the two cats with hyperglycemia are considered to be in risk if their blood glucose levels and living habits (diet, exercise etc.) are not monitored and controlled properly.Table 1 Distribution of Blood Glucose Level of Cat PatientsThe absence of cat patients that have blood glucose level in the range that is considered as diabetes was supported by supporting data, such as gender, age, body weight and breed of the cat patient samples.≥ 220121 mg/dL - 219 …90-120 mg/dL Blood Glucose Level≤ 900204060FIGURE 1. Distribution of Blood Glucose Level of the cat patientsGender, age, obesity and breed are risk factors of diabetes mellitus (DM). Male cats have higher risk to suffer diabetes mellitus compared to female cats. One of the reasons may be that male cats have lower sensitivity to insulin [2]. Meanwhile, the cat patient samples in this study consist of more female cats than male. Related to age, DM/FDM can happen to cats of all ages, but most of the cats that are diagnosed to suffer FDM are those with an age above 6 years old. Meanwhile in this study all of the cat patient samples aged less than 5 years old. Another risk factor of feline diabetes mellitus is obesity. Cats that are obese have a chance of about 4 times more likely to suffer from diabetes compared to cats with optimal weight. That is because obese cats have decreased insulin sensitivity [4].However, the results of this study note that all cat patient samples weight less than5 kg. In addition to gender, age, and obesity,a cat’s breed is also one of the risk factor was performed in Australia, New Zealand, and England showed that Burmese cats are fourtimes more at risk to suffer feline diabetes mellitus compared to domestic cat breed [2]. In this study, none of the cats are Burmese breed. Although none of the cat patients in this study are diagnosed to suffer FDM, a routine blood glucose level measurement is recommended to monitor the blood glucose level of the cats to prevent the cats from actually suffering feline diabetes mellitus.5. ConclusionsThe conclusion of this study is none of cat patients sampled at Veterinary Medicine Faculty of Brawijaya University’s Education Animal Clinic suffers from diabetes mellitus / Feline Diabetes Mellitus, but two cats are considered at risk because they have blood glucose level 121 mg/dL –219 mg/dL (hyperglycemia).AcknowledgmentThis project supported and fascilitated by Veterinary Medicine Faculty of Brawijaya University, and Biochemistry Department Team.ReferenceBirchard, S.J. and R.G. Sherding, 2006. Saunder Manual of Small Animal Practice. 3rd Edition. Saunder Elsevier. P.376-389.。
公允价值英文文献

Relative value relevance of historicalcost vs.fair value:Evidence frombank holding companiesInder K.Khurana *,Myung-Sun Kim 1School of Accountancy,College of Business,University of Missouri––Columbia,317Middlebush,Columbia,MO 65211,USAAbstractThis study complements the growing literature on the value relevance of fair value by examining the validity of the hypothesis that fair value is more informative than his-torical cost as a financial reporting standard for financial ing the fair value disclosures made under Statement of Financial Accounting Standards (SFAS)No.107and SFAS No.115by bank holding companies (BHCs)over the 1995–98period,we compare the relative explanatory power of fair value and historical cost in explaining equity values.For our entire sample,we are unable to detect a discernible difference in the informativeness of fair value measures collectively relative to historical cost mea-sures.However,for small BHCs and those with no analysts following,we find that his-torical cost measures of loans and deposits are more informative than fair values.Anecdotal evidence indicates that loans and deposits are not actively traded and often involve more subjectivity with respect to the methods and assumptions used in esti-mating their fair values.In contrast,fair value of available-for-sale securities,which are more actively traded in well-established markets,explains equity values more than historical cost.Taken together,our results are consistent with the notion that fair value is more (less)value relevant when objective market-determined fair value measures are (not)available.More importantly,our results suggest that simply requiring fair value as the reported measure for financial instruments may not improve the quality of *Corresponding author.Tel.:+1-573-882-3474;fax:+1-573-882-2437.E-mail addresses:khuranai@ (I.K.Khurana),sunkim@ (M.Kim).1Tel.:+1-573-882-1071.0278-4254/03/$-see front matter Ó2003Elsevier Science Inc.All rights reserved.doi:10.1016/S0278-4254(02)00084-4Journal of Accounting and Public Policy 22(2003)19–4220I.K.Khurana,M.Kim/Journal of Accounting and Public Policy22(2003)19–42 information for all BHCs unless appropriate estimation methods or guidance for financial instruments that are not traded in active markets can be established.Ó2003Elsevier Science Inc.All rights reserved.1.IntroductionRecently the Financial Accounting Standards Board(FASB)made a fun-damental decision that fair value is the most relevant attribute forfinancial instruments(FASB,2000,p.8).Although the quoted market value is the prescribed measure of fair value,the FASB adopted the term‘‘fair value’’instead of market value to encompass estimated values forfinancial instru-ments that are not traded in active markets.The decision to mandate fair value disclosures was made amidst a long-standing debate between the advocates of fair value accounting and advocates of historical cost accounting.The basic premise underlying the FASBÕs decision is that fair value offinancial assets and liabilities better enables investors,creditors and other users offinancial state-ments to assess the consequences of an entityÕs investment andfinancing strategies.2Advocates of historical cost,on the other hand,point to the re-duced reliability of fair value estimates relative to historical cost.Their argu-ments suggest that investors would be reluctant to base valuation decisions on the more subjective fair value estimates(Barth,1994,p.3).Given the FASBÕs stated long-term goal of having allfinancial assets and liabilities recognized in statements offinancial position at fair value rather than at amounts based on historical cost,the purpose of this study is to test claims that fair value is more informative relative to historical cost.Specifically,we examine whether fair value offinancial instruments is more informative than historical cost in explaining equity market values of bank holding companies (BHCs).The goal is to determine whether fair value has a higher association with equity market values of BHCs than historical cost.We focus on BHCs for several reasons.First,financial statements of BHCs are dominated by thefinancial instruments covered under the FASBÕs pro-nouncements on fair value disclosures.For our sample of BHCs,assets and liabilities subjected to fair value disclosures constitute,on average,87%and 88%of total book value of assets,respectively.Second,fair value disclosures are more comprehensive and standardized for BHCs than forfirms in other industries.Finally,BHCs enable us to evaluate whichfinancial instruments,if any,contribute to the higher association between fair value and equity values.2The FASBÕs Statement of Financial Accounting Standards(SFAS)No.133,Accounting for Derivative Instruments and Hedging Activities,explicitly states that fair values forfinancial assets and liabilities provide more relevant and understandable information than cost based measures (FASB,1998,–222).I.K.Khurana,M.Kim/Journal of Accounting and Public Policy22(2003)19–4221We use the fair value disclosures made under SFAS No.107,Disclosures about Fair Value of Financial Instruments,and SFAS No.115,Accounting for Certain Investments in Debt and Equity Securities,by302BHCs over the1995–98period.For our entire sample of BHCs,we are unable to detect a statistically significant difference between the explanatory power of historical cost and fair value measures offinancial instruments collectively in explaining equity values.We also provide evidence on whether the explanatory power of fair value relative to historical cost depends on additionalfirm characteristics.Fair value disclosures are likely to be more informative than historical cost for large BHCs,if they are more capable(than small BHCs)of precisely estimating fair value,and for BHCs operating in more transparent information environment, if this implies that information disclosed by thesefirms is more reliable.Our results indicate that historical cost is more informative than fair value for a subset of BHCs that are classified as small(based on market value of equity)and for a subset of BHCs with no analysts following.Additional analysis undertaken to identify the source of these results indicates that loans and deposits drive the higher informativeness of historical cost over fair value of the two subsets of BHCs.Anecdotal evidence indicates that loans and de-posits are not actively traded and often involve more subjectivity with respect to the methods and assumptions used in estimating fair value.In contrast, fair value of available-for-sale securities,which are more actively traded in well-established markets,explains equity values more than historical cost. Taken together,our results are consistent with the notion that fair value is more value relevant when objective market-determined fair value measures are available.For the remainingfinancial instruments(i.e.,held-to-maturity debt secu-rities andfinancial liabilities other than deposits),wefind neither fair value nor historical cost to provide greater information.Our inability to detect the dominance of one measure over the other may be due to a small difference between historical cost and fair value in our sample period(1995–98).33For example,if the difference between fair value and historical cost were recognized in the income statement,the average effect on earnings would be6%of income before extraordinary items for our sample,whereas the average effect(recalculated after adjusting for the differences in denominators)would be)11%and)26%for samples in Nelson(1996,p.168)and Park et al. (1999,p.357),respectively.To the extent that the differences between historical cost and fair value is due to changes in interest rates,the small differences(in absolute terms)between historical cost and fair value for our sample compared to those in prior studies can be due to the magnitude of changes in interest rates in our sample period.During the1995–98sample period covered by our study,the average annual unexpected interest rate changes in absolute terms(computed as the absolute value of the difference between actual annual three month Treasury bill rate less the prior year monthly average interest rate deflated by the prior interest rate)is11%.The corresponding numbers during1992–93(sample period covered by Nelson,1996,p.167)and1993–95(sample period covered by Park et al.,1999,p.353)are24%and28%,respectively.22I.K.Khurana,M.Kim/Journal of Accounting and Public Policy22(2003)19–42In light of the fact that our sample of BHCs exhibit small differences be-tween historical cost and fair value offinancial instruments,it is conceiv-able that fair value disclosures in an environment where fair value deviates dramatically from historical cost may have different implications for BHCsÕequity values than in the environment covered by this study.Our study differs from prior research on fair value disclosures in that we test for the relative information content of fair value and historical cost as opposed to incremental information content of fair value over and above historical cost.Incremental information content tests conducted in prior re-search assess whether fair value provides information content beyond his-torical costs(Biddle et al.,1995,p.3).Such incremental information tests ask whether the two measures(fair value and historical cost)together are more informative than one measure alone.On the other hand,relative information test conducted here ask whether fair value alone is more informative than historical cost alone and vice versa.Prior research on fair value disclosures has focused on incremental information content tests without explicitly test-ing whether fair value alone is more,equally,or less informative than his-torical cost.While results of incremental tests have been informative in the FASBÕs deliberations on fair value disclosure requirements(Barth et al.,2001,p.79), Ryan(1999,p.374)notes that the FASB has moved on to the next logical step of asking whether fair value as a basis is preferable to historical cost for balance sheet recognition.Our study is thefirst to conduct relative infor-mation content tests to assess whether fair value is more informative than historical cost and vice versa.In doing so,it complements the growing lit-erature on the value relevance of fair value.Empirical evidence(provided in our study)on the informativeness of fair value measures relative to historical cost measures should be useful to the FASB,which is interested in fair value as a replacement(or complement) to historical cost as a key measure forfinancial instruments.This knowl-edge can impart input into policy deliberations by allowing informed tradeoffs between benefits and costs of providing information about fair value.The remainder of this paper is organized as follows:Section2provides background information on fair value and discusses thefindings of prior re-search on fair value disclosures.Section3describes the motivation behind the empirical tests conducted in this study.Section4describes the methodology used to test the informativeness of fair value relative to historical cost using market value of equity.In Section5,we describe our data and sample selection procedure.Section6presents our empiricalfindings,and Section7concludes the paper.I.K.Khurana,M.Kim/Journal of Accounting and Public Policy22(2003)19–42232.Background and prior researchThe FASB(2000,p.8)has stated that its long-term goal is to have allfi-nancial assets and liabilities recognized in statements offinancial position at fair value rather than at amounts based on historical cost.It has also issued several significant pronouncements on fair value disclosures,SFAS No.107, Disclosures about Fair Value of Financial Instruments(FASB,1991),SFAS No.115,Accounting for Certain Investments in Debt and Equity Securities (FASB,1993),and SFAS No.133,Accounting for Derivative Instruments and Hedging Activities(FASB,1998).Underlying the issuance of these pro-nouncements is the belief that fair value provides information aboutfinancial assets and liabilities that is more relevant than amounts based on historical cost.The FASBÕs(2001,p.9)intermediate objective is to issue a statement that would describe more specifically how to determine fair value forfinancial in-struments and improve the form and content of the disclosures required by SFAS No.107.Fair value of afinancial instrument represents the amount at which afi-nancial instrument could be exchanged in a current transaction between willing parties,other than in a forced or liquidation sale(FASB,1991,–5).Although the market prices quoted in an active market provide the most reliable measure of fair value,market prices are often not available for manyfinancial instru-ments appearing on the balance sheets of BHCs.In such situations,BHCs must provide the best available estimate of a current market price by exercising judgments about the methods and assumptions to be used(FASB,1991,–22). As a result,fair value measures reported in thefinancial statements depict the managementÕs estimate of the present value of the net future cashflows em-bodied in an asset or liability,discounted to reflect both the current interest rate and the managementÕs assessment of the risk associated with those cash flows.Willis(1998,p.5)notes that fair values provide information about benefits expected from assets and burdens imposed by liabilities based on current economic conditions and expectations.The FASB(1991,–40)contends that periodic information about the fair value of an entityÕsfinancial instruments under current conditions and expectations should help users both in making their own predictions and in confirming or correcting their earlier expectations. Furthermore,the FASB maintains that fair values forfinancial assets and li-abilities provide more relevant and understandable information than historical cost measures:...fair value is more relevant tofinancial statement users than costfor assessing the liquidity or solvency of an entity because fair value24I.K.Khurana,M.Kim/Journal of Accounting and Public Policy22(2003)19–42 reflects the current cash equivalent of the entityÕsfinancial instru-ments rather than the price of a past transaction.With the passageof time,historical prices become irrelevant in assessing present li-quidity or solvency(FASB,1998,–222).Critics of fair value accounting point to the reduced reliability of fair value estimates relative to historical cost(Barth,1994,p.3).Historical cost infor-mation can be based on internally available information about prices in past transactions,without reference to outside market data.Fair value,in contrast, is based on current prices,which may require estimation and can lead to re-liability problems.Since fair values must be estimated for severalfinancial instruments that are not actively traded,estimation error could impair their value-relevance.2.1.Prior researchPrior research on value relevance(defined as the association between ac-counting numbers and security market values)has focused on whether fair value disclosures in the banking industry have incremental information content over and above historical cost.4Tests for incremental information content assess whether one measure provides information content in addition to that of another measure and are often used when one or more measures are given or required and another is supplemental(Biddle et al.,1995p.3;Jennings,1990 p.925).Biddle et al.(1995,p.3)point out that in the absence of an explicit test to examine whether one measure(e.g.,fair value)alone is equally,less,or more informative than another measure(e.g.,historical cost),incremental informa-tion content tests of fair value over historical cost measures can imply several different outcomes.Finding that fair value is incrementally informative can imply that fair value is as,more,or less informative than historical cost.Al-ternatively,finding that fair value is not incrementally informative can imply fair value is either equally or less informative than historical cost.Therefore, the mapping between an incremental and a relative information content test is not one-to-one.While incremental comparisons assess the incremental con-4Two notable exceptions of studies examining information content of fair value disclosures outside the banking industry are Simko(1999,p.247),who focuses on non-financialfirms,and Petroni and Wahlen(1995,p.719),who focus on property-liability insurance companies.Simko (1999,p.270)finds thatfinancial instrument liability fair value disclosures are generally value-relevant for non-financialfirms,while Petroni and Wahlen(1995,p.735)find that the fair values of only certain categories of investments(equity investments and USTreasury investments)are reflected in share prices and returns of property-liability insurers.For a review of the literature and the methodological issues(see Barth et al.,2001;Barth,2000;Holthausen and Watts,2001).I.K.Khurana,M.Kim/Journal of Accounting and Public Policy22(2003)19–4225 tribution of one measure over the other,relative comparisons reflect differences in incremental information content of the two measures.Prior studies examining incremental information content of fair value dis-closures by BHCs report mixedfindings regarding their ability to explain market value of equity or returns.Barth(1994,p.2),using the sample of BHCs over20years between1971and1990,finds that fair value of investment se-curities,which were disclosed even prior to SFAS107(FASB,1991),is sig-nificantly associated with market value of equity;and that historical cost provides no explanatory power incremental to fair value.Based on these two findings,Barth concludes that investment securitiesÕfair value(level variable) has more explanatory power than historical cost.5However,shefinds that unrealized gains and losses on investment securities as a group(change vari-able)do not possess explanatory power incremental to earnings in explaining returns.She attributed the observed lack of incremental information to pos-sible measurement error in the unrealized gains and losses on investment se-curities.During the time period covered by her study,the average annual unexpected interest rate change in absolute terms was21%.6 Three other studies examine the relation between BHC share prices and fair value disclosures forfinancial instruments provided under SFAS107for1992 and1993,and one other study focuses on the1993–95time period.7Using a market-to-book specification,Nelson(1996,p.173)finds that SFAS No.107 fair value disclosures have no incremental power to explain market values of equity relative to book values,with the exception of investment securities in 1992.Shefinds that none of the fair value measures are associated with stock returns.Eccher et al.(1996,p.114)find that fair value of investment securities has significant incremental explanatory power in explaining market value of equity,but that evidence on the other asset and liability variables examined is mixed and weak.In contrast,Barth et al.(1996,p.535)document that fair value estimates of loans during1992–93provide significant incremental ex-planatory power for BHCsÕmarket value of equity beyond that provided by related book values when additional variables(e.g.,non-performing loans and interest-sensitive assets and liabilities)are controlled for.Similarly,Park et al. (1999,p.368)find that for a pooled sample of BHCs during1993–95,unre-alized gains and losses on available-for-sale securities,held-to-maturity debt 5Her results apply only to investment securities and disclosures made before SFAS107and SFAS115.6We used the prior year monthly average interest rate of three month Treasury bills as a proxy for expected interest rate and deflated the unexpected interest rate change by the prior year interest rate.7The average annual unexpected interest rate changes(in absolute terms)during the sample periods covered in these studies were24%(1992–93)and28%(1993–95),respectively,whereas the corresponding interest rate change during our sample period is11%.26I.K.Khurana,M.Kim/Journal of Accounting and Public Policy22(2003)19–42 securities,and loans are incrementally value relevant in explaining annual re-turns when these variables are entered simultaneously into a regression model. Overall,the results of prior studies documenting the incremental informa-tiveness of fair value imply that fair value is either equally,less,or more in-formative than historical cost depending on the amount of unique information contained in fair value.3.Empirical testsOurfirst set of tests of relative information content is motivated by the mixedfindings on the incremental information content of fair value disclosures as well as the fact that there has been little empirical research testing the relative information content of fair value disclosures.Tests for relative information content assess whether one measure has greater information content than another and are often used when assessing mutually exclusive choices(e.g., Biddle et al.,1995,p.3).The FASB views fair value to be the most relevant attribute forfinancial instruments.If disclosed fair value estimates measure underlying fair values offinancial assets and liabilities reliably,then fair value measures are more likely to be related to market value of equity than historical cost measures.Therefore,relative information content comparisons between fair value and historical cost measures can provide useful input into the FASBÕs policy deliberations.Our second set of tests examines whether differentialfirm characteristics are related to differential reliability of fair value information and therefore,dif-ferential information content of fair value measures.Manyfinancial instru-ments appearing on the balance sheets of BHCs are not traded in established markets.As a result,obtaining a reliable fair value estimate could be prob-lematic.We consider twofirm characteristics that may affect the reliability of fair value measures,namely,size and information environment.Many contend that fair value of loans cannot be estimated reliably,espe-cially those subject to non-trivial default risk(Barth et al.,1996,p.514).If large banks have more resources(e.g.,more sophisticated investment depart-ments)available for estimation of fair value than small banks,then large banks are more likely to provide fair value estimates with less measurement error.8 We provide evidence on this issue by conducting relative information content tests for subsets of sample BHCs classified by size.Moreover,Ryan(1999,p.375)suggests that the richer the bankÕs infor-mation environment,the smaller the likelihood of reliability problems associ-8It is also possible that large banks could have lower risk because of greater opportunities for diversification.I.K.Khurana,M.Kim/Journal of Accounting and Public Policy22(2003)19–4227 ated with fair value estimates.There is considerable empirical evidence that suggests forecast dispersion is related to the quality offinancial disclosures. Swaminathan(1991,p.36)finds that forecast dispersion decreased following the release of newly mandated segment disclosures by the SEC.Dechow et al. (1996,p.26)find that forecast dispersion increased following alleged violations of generally accepted accounting principles.High forecast dispersion is also associated withfinancial disclosures that are given a low rating byfinancial analysts(Lang and Lundholm,1996,p.486).The implication is that the more reliable thefirmÕs overallfinancial reporting system,the less diverse should be the analystsÕopinion on thefirmÕs future prospects.We test whether fair value disclosures are more informative for BHCs operating in more transparent in-formation environment by usingfinancial analystsÕforecast dispersion to classify BHCs.9Our third set of tests examines the information content of an individual financial instrument.Certainfinancial instruments held by BHCs are likely to be actively traded in securities markets(e.g.,marketable securities).Fair value disclosures of suchfinancial instruments are based on readily observable market prices(Petroni and Wahlen,1995,p.725).However,loans are not actively traded and therefore involve more subjectivity with respect to the methods and assumptions used in estimating their fair values(Barth et al., 1996,p.530).Thus,fair value disclosures for certainfinancial instruments require more estimation than those for otherfinancial instruments.We provide evidence on the relative informativeness of historical cost and fair value measures of individualfinancial instruments.4.ModelWe utilize a cross-sectional valuation model based on the balance sheet identity that has been used extensively in the prior literature(Beaver et al., 1989,p.165;Barth,1991,p.438;and Barth et al.,1996,p.519).This model relates the market value of common equity to the historical cost and fair value measures of broad asset and liability categories offinancial institutions.The FASB(1993,–12)requires recognition of fair value for available-for-sale 9It is important to note thatfinancial analystsÕforecast dispersion measure should not be interpreted as implying that estimation quality of fair value per se is reflected in the analystsÕforecasts.Since earnings do not include unrealized gains or losses on securities other than trading securities,analystsÕforecast dispersion is not a direct indicator of the reliability of fair value estimates.Our empirical tests are based on the assumption that the measurement quality of earnings will be positively related to that of non-earnings information such as fair value measures. To the extent that this relation is weak,our empirical tests may be unable to detect the hypothesized effect.We thank an anonymous reviewer for this comment.28I.K.Khurana,M.Kim/Journal of Accounting and Public Policy22(2003)19–42 securities and does not alter the rules for marking trading securities to mar-ket.10Forfinancial instruments other than available-for-sale securities and trading securities,historical cost(fair value)is the amount recognized(dis-closed)in the balance sheet(footnotes).We estimate the following model with either historical cost or fair value:MV itþ3¼b0þb1AFS itþb2HTM itþb3LOAN itþb4DEPO itþb5OFINL itþb6OASSET itþb7OLIAB itþu itð1Þwhere i,t denotefirms andfiscal year-end,and MV is the market value of equity three months after thefiscal year-end,AFSis the available-for-sale se-curities atfiscal year-end,HTM is the held-to-maturity debt securities atfiscal year-end,LOAN is the loans atfiscal year-end,DEPO is the deposits atfiscal year-end,OFINL is thefinancial liabilities other than deposits atfiscal year-end,OASSET is the assets other than AFS,HTM,and LOAN atfiscal year-end,11OLIAB is the liabilities other than DEPO and OFINL atfiscal year-end.To mitigate the size or scale effect,we deflate all the variables with the market value of equity at the close of year tÀ1(see Brown et al.,1999,p.104).Consistent with prior research(Barth et al.,1996,p.519),non-financial assets(liabilities)are measured as the book value difference between total assets (liabilities)andfinancial assets(liabilities).12We test the relative informa-tiveness of fair value and historical cost measures by comparing the R2from each model using the Vuong(1989,p.307)test,a likelihood ratio test of model selection.The test compares the R2s of two non-nested regression models and selects the model with the higher explanatory power,consistent with assessing the relative informativeness of two mutually exclusive measures.A negative and significant Z-statistic would indicate that the residuals produced by the historical cost model are larger in magnitude than those produced by the fair 10Trading securities are marked to market both before and after SFAS No.115(FASB,1993,–12).11Because trading securities are recognized at fair value,BHCs do not provide historical cost information for trading securities.As a result,we do not examine trading securities separately. Instead,we include them as part of OASSET(defined as assets other than available-for-sale securities,held-to-maturity securities,and loans).12We exclude off-balance sheet items from our models because of limitations in interpreting many of the off-balance sheet fair value disclosures required under SFAS No.107.Consistent with the limitations outlined by Barth et al.(1996,p.523),wefind that a majority of our sample BHCs (1)fail to indicate clearly whether the net position with respect to off-balance sheet items is an asset or a liability;(2)net several off-balance sheet amounts,making it difficult to determine the asset and liability positions for the individual items;and(3)have inadequate disclosures making it impossible to compute an estimate of fair value for off-balance sheet items.。
数据分析英语试题及答案

数据分析英语试题及答案一、选择题(每题2分,共10分)1. Which of the following is not a common data type in data analysis?A. NumericalB. CategoricalC. TextualD. Binary2. What is the process of transforming raw data into an understandable format called?A. Data cleaningB. Data transformationC. Data miningD. Data visualization3. In data analysis, what does the term "variance" refer to?A. The average of the data pointsB. The spread of the data points around the meanC. The sum of the data pointsD. The highest value in the data set4. Which statistical measure is used to determine the central tendency of a data set?A. ModeB. MedianC. MeanD. All of the above5. What is the purpose of using a correlation coefficient in data analysis?A. To measure the strength and direction of a linear relationship between two variablesB. To calculate the mean of the data pointsC. To identify outliers in the data setD. To predict future data points二、填空题(每题2分,共10分)6. The process of identifying and correcting (or removing) errors and inconsistencies in data is known as ________.7. A type of data that can be ordered or ranked is called________ data.8. The ________ is a statistical measure that shows the average of a data set.9. A ________ is a graphical representation of data that uses bars to show comparisons among categories.10. When two variables move in opposite directions, the correlation between them is ________.三、简答题(每题5分,共20分)11. Explain the difference between descriptive andinferential statistics.12. What is the significance of a p-value in hypothesis testing?13. Describe the concept of data normalization and its importance in data analysis.14. How can data visualization help in understanding complex data sets?四、计算题(每题10分,共20分)15. Given a data set with the following values: 10, 12, 15, 18, 20, calculate the mean and standard deviation.16. If a data analyst wants to compare the performance of two different marketing campaigns, what type of statistical test might they use and why?五、案例分析题(每题15分,共30分)17. A company wants to analyze the sales data of its products over the last year. What steps should the data analyst take to prepare the data for analysis?18. Discuss the ethical considerations a data analyst should keep in mind when handling sensitive customer data.答案:一、选择题1. D2. B3. B4. D5. A二、填空题6. Data cleaning7. Ordinal8. Mean9. Bar chart10. Negative三、简答题11. Descriptive statistics summarize and describe thefeatures of a data set, while inferential statistics make predictions or inferences about a population based on a sample.12. A p-value indicates the probability of observing the data, or something more extreme, if the null hypothesis is true. A small p-value suggests that the observed data is unlikely under the null hypothesis, leading to its rejection.13. Data normalization is the process of scaling data to a common scale. It is important because it allows formeaningful comparisons between variables and can improve the performance of certain algorithms.14. Data visualization can help in understanding complex data sets by providing a visual representation of the data, making it easier to identify patterns, trends, and outliers.四、计算题15. Mean = (10 + 12 + 15 + 18 + 20) / 5 = 14, Standard Deviation = √[(Σ(xi - mean)^2) / N] = √[(10 + 4 + 1 + 16 + 36) / 5] = √52 / 5 ≈ 3.816. A t-test or ANOVA might be used to compare the means ofthe two campaigns, as these tests can determine if there is a statistically significant difference between the groups.五、案例分析题17. The data analyst should first clean the data by removing any errors or inconsistencies. Then, they should transformthe data into a suitable format for analysis, such ascreating a time series for monthly sales. They might also normalize the data if necessary and perform exploratory data analysis to identify any patterns or trends.18. A data analyst should ensure the confidentiality andprivacy of customer data, comply with relevant data protection laws, and obtain consent where required. They should also be transparent about how the data will be used and take steps to prevent any potential misuse of the data.。
adsee
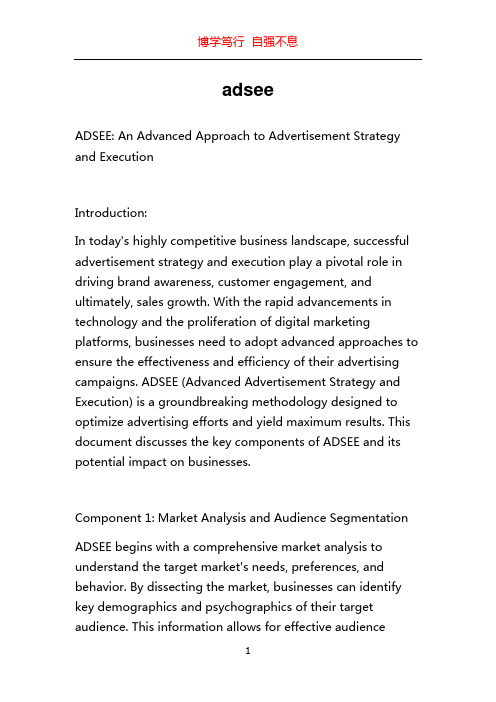
adseeADSEE: An Advanced Approach to Advertisement Strategy and ExecutionIntroduction:In today's highly competitive business landscape, successful advertisement strategy and execution play a pivotal role in driving brand awareness, customer engagement, and ultimately, sales growth. With the rapid advancements in technology and the proliferation of digital marketing platforms, businesses need to adopt advanced approaches to ensure the effectiveness and efficiency of their advertising campaigns. ADSEE (Advanced Advertisement Strategy and Execution) is a groundbreaking methodology designed to optimize advertising efforts and yield maximum results. This document discusses the key components of ADSEE and its potential impact on businesses.Component 1: Market Analysis and Audience SegmentationADSEE begins with a comprehensive market analysis to understand the target market's needs, preferences, and behavior. By dissecting the market, businesses can identify key demographics and psychographics of their target audience. This information allows for effective audiencesegmentation, ensuring that advertisements are tailored to specific consumer groups. By targeting specific segments, businesses can create highly personalized and relevant advertisements, heightening the chances of engagement and conversion.Component 2: Data-Driven InsightsADSEE leverages the power of data to gain valuable insights into consumer behavior and preferences. By analyzing data from various sources, such as customer interaction, social media engagement, and website analytics, businesses can better understand their audience. These insights enable businesses to make data-driven decisions when developing advertisement strategies. By continuously collecting and analyzing data, businesses can refine and optimize their advertisements based on real-time feedback and results.Component 3: Creative Content DevelopmentADSEE recognizes the importance of captivating and impactful advertising content. It emphasizes the creation of visually appealing and emotionally engaging advertisements. This component focuses on designing compelling visuals, crafting persuasive copy, and incorporating storytelling elements to drive brand affinity and audience connection. ADSEE encourages businesses to experiment with variouscreative formats and mediums to keep their advertisements fresh, relevant, and memorable.Component 4: Multi-Channel AdvertisingIn today's digital era, ADSEE emphasizes the power of multi-channel advertising. It recognizes that consumers interact with brands across multiple platforms, including social media, search engines, mobile apps, and websites. ADSEE advocates for businesses to have a strong presence on various platforms and develop advertisements tailored to each channel's unique requirements. By reaching consumers through their preferred channels, businesses can maximize the impact of their advertisement campaigns.Component 5: A/B Testing and Campaign OptimizationADSEE believes in continuous improvement through rigorous testing and optimization. It encourages businesses to conduct A/B testing, where two versions of an advertisement are compared to determine the most effective approach. By measuring key performance indicators such as click-through rates, conversion rates, and customer engagement, businesses can fine-tune their advertisements for optimal results. ADSEE stresses the importance of being agile and adaptable, making data-backed adjustments throughout the campaign duration.Conclusion:ADSEE offers businesses a comprehensive and advanced approach to advertisement strategy and execution. By conducting thorough market analysis, leveraging data-driven insights, creating compelling content, adopting multi-channel advertising, and continuously optimizing campaigns, businesses can maximize the impact of their advertisements. ADSEE empowers businesses to connect with their target audience in a more meaningful and effective way, driving brand awareness, customer engagement, and ultimately, business success. With ADSEE, businesses can stay ahead of the competition and create advertising campaigns that leave a lasting impression on their audience.。
Macrophages,Immunity,andMetabolicDisease

ImmunityReview Macrophages,Immunity,and Metabolic DiseaseJoanne C.McNelis1and Jerrold M.Olefsky1,*1Department of Medicine,University of California,San Diego,9500Gilman Drive,La Jolla,CA92093,USA*Correspondence:jolefsky@/10.1016/j.immuni.2014.05.010Chronic,low-grade adipose tissue inflammation is a key etiological mechanism linking the increasing inci-dence of type2diabetes(T2D)and obesity.It is well recognized that the immune system and metabolism are highly integrated,and macrophages,in particular,have been identified as critical effector cells in the initiation of inflammation and insulin resistance.Recent advances have been made in the understanding of macrophage recruitment and retention to adipose tissue and the participation of other immune cell pop-ulations in the regulation of this inflammatory process.Here we discuss the pathophysiological link between macrophages,obesity,and insulin resistance,highlighting the dynamic immune cell regulation of adipose tissue inflammation.We also describe the mechanisms by which inflammation causes insulin resistance and the new therapeutic targets that have emerged.IntroductionType2diabetes(T2D)has become a global epidemic,with huge social and economic costs.The World Health Organization esti-mates that3.4million deaths per year worldwide are attributable to T2D,a number that is predicted to increase in the next decade (http://www.who.int/mediacentre/factsheets/fs312/en/). Approximately$175billion is spent on diabetes-related health-care annually in the United States alone(Centers for Disease Control and Prevention,2011).The majority of cases of diabetes (80%)are attributable to the parallel increasing rates of obesity (/About_us/News_Landing_Page/ Diabetes-and-obesity-rates-soar/)and thus extensive research efforts have been made to elucidate the mechanistic links be-tween these two conditions.Nutrient excess and adiposity acti-vate several metabolic pathways implicated in the development of insulin resistance,including inflammatory signaling,lipotoxic-ity,aberrant adipokine secretion(Sartipy and Loskutoff,2003; Steppan et al.,2001;Yamauchi et al.,2001),adipose tissue hypoxia(Cramer et al.,2003),endoplasmic reticulum(ER)stress (Ozcan et al.,2004;Urano et al.,2000),and mitochondrial dysfunction(Furukawa et al.,2004).A detailed description of all of these processes is beyond the scope of this piece and there are excellent recent reviews on these subjects(Samuel and Shulman,2012;Hotamisligil,2010;Johnson and Olefsky,2013; Lee and Ozcan,2014).Here,we will focus on obesity-associated chronic inflammation,which we believe is a key,unifying com-ponent of insulin resistance.Indeed,several of the metabolic processes mentioned above,such as ER stress,hypoxia,and lipotoxicity,can all converge on the development of metabolic inflammation.Obesity-associated metabolic inflammation is unlike the paradigm of classical inflammation—an acute inflammatory response,defined by the characteristic signs of redness, swelling,and pain.Instead,it is a form of‘‘sterile inflammation’’produced in response to metabolic(rather than infectious)stimuli and is chronically sustained at a subacute level without adequate resolution.Thefirst evidence for a pathophysiological link between obesity,inflammation,and insulin resistance was provided more than a century ago,when it was observed that the anti-inflammatory drug salicylate,the principle metabolite in aspirin, had beneficial effects on glucose control in diabetic patients (Williamson,1901).This concept was revisited in1993when Hotamisligil et al.(1993)demonstrated that tumor necrosis factor-a(TNF-a)(a proinflammatory cytokine)is secreted,in increased amounts,from the adipose tissue of obese rodents and is a potent negative regulator of insulin signaling.The complexity of this inflammatory response was realized,some 10years later,when two groups independently demonstrated that obesity is associated with the accumulation of macro-phages in adipose tissue,which were found to be the principal source of inflammatory mediators,including TNF-a,expressed by this metabolic tissue(Weisberg et al.,2003;Xu et al.,2003).A number of reports have now demonstrated the key importance of macrophage-elicited metabolic inflammation in insulin resis-tance.During obesity this immune cell population differs,not only in number,but also in inflammatory phenotype and tissue localization.In this review we will focus on the pathophysiolog-ical connections between obesity,macrophages,and insulin resistance.In particular,we will describe the mechanisms by which macrophages are recruited to metabolic tissues,mediate inflammation,and impact insulin signaling.We will also discuss current anti-inflammatory therapeutic strategies for the treat-ment of type2diabetes(T2D).Inflammatory SignalingThe secretion of inflammatory cytokines and chemokines by adipose tissue macrophages(ATMs)extends beyond TNF-a and includes interleukin-6(IL-6),IL-1b,monocyte chemotactic protein1(MCP-1,CCL2),and macrophage inhibitory factor (MIF)(Olefsky and Glass,2010).Production of these inflam-matory factors is under the transcriptional control of two key intracellular inflammatory pathways,c-Jun N-terminal kinase (JNK)-activator protein1(AP1)and IKappa B kinase beta(IKK)-nuclear factor kappa-light-chain-enhancer of activated B cells (NF-k B),which differ in their upstream signaling components but converge on the induction of overlapping inflammatory genes.These two inflammatory pathways are initiated byalmost36Immunity41,July17,2014ª2014Elsevier Inc.all of the mediators implicated in the development of insulin resistance,including oxidative and ER stress,saturated fatty acids,and inflammatory cytokines,highlighting their importance in the pathogenesis of disease (Solinas and Karin,2010).IKK b -NF-k B signaling is initiated by activation of IKK b and sub-sequent phosphorylation of the inhibitor of NF-k B (I k B).In the noninflammatory state,I k B retains NF-k B in an inhibitory cyto-plasmic complex.After inflammatory stimuli,I k B is phospho-rylated,dissociates from NF-k B,and undergoes degradation.This permits the translocation of free NF-k B to the nucleus,where it binds to cognate DNA response elements,leading to transactivation of inflammatory genes.Similarly,activation of JNK-AP-1signaling by inflammatory mediators leads to phos-phorylation and activation of JNK,which then phosphorylates the N terminus of c-Jun.This initiates a switch of c-Jun dimers for c-Jun-c-Fos heterodimers,which ultimately stimulate tran-scription of inflammatory target genes.Both JNK1and IKK signaling are upregulated in adipose (Weisberg et al.,2003;Xu et al.,2003),skeletal muscle (Bandyopadhyay et al.,2005),and liver (Cai et al.,2005)from insulin-resistant rodents and humans.Obesity activates JNK and NF-k B signaling by several mecha-nisms.For example,IL-1and TNF-a instigate inflammatory signaling through classical activation of their cell surface recep-tors (Olefsky and Glass,2010).Alternatively,the inflammatory process can be initiated by activation of pattern recognition receptors (PRRs),which include Toll-like receptors (TLRs)and NOD-like receptors (NLRs).PRRs sense exogenous pathogen-associated molecular patterns (PAMPs),including microbial derived LPS,peptidoglycan,and bacterial DNA,as well as endo-degradationNF-κBAP-1Inflammatory gene expressionInsulin resistanceFigure 1.Inflammatory Signaling Pathways Implicated in the Development of Insulin ResistanceActivation of TLR2,TLR4,and/or tumor necrosis factor receptor (TNFR)leads to the activation of NF-k B and JNK signaling.The serine kinases IKK b and JNK phosphorylate IRS-1and IRS-2,inhibit-ing downstream insulin signaling.In addition,the activation of IKK b leads to the phosphorylation and degradation of the inhibitor of NF-k B,I k B,which permits the translocation of NF-k B to the nucleus.Similarly,the activation of JNK leads to the formation of the AP-1transcription factor.Nuclear NF-k B and AP-1transactivate inflamma-tory genes,which can contribute to insulin resis-tance in a paracrine manner.Abbreviations are as follows:PI3K,phosphoinositide 3-kinase;RIP,receptor interacting protein;Myd88,myeloid dif-ferentiation primary response gene-88;SFA,saturated fatty acid;TRADD,TNF receptor-asso-ciated death domain;TRAF2,TNF receptor-associated factor-2;TRIF,TIR domain containing adaptor protein inducing IFN-g .Adapted from Osborn and Olefsky (2012).genous damage-associated molecular patterns (DAMPs),such as saturated fatty acids (Nguyen et al.,2007),ATP,and heat shock proteins.Of the TLRs,TLR4has been shown to play a particularly impor-tant role in initiating saturated fatty acid-mediated macrophage inflammation.Indeed,hematopoietic cell-specific dele-tion of TLR4protects mice from high fat diet (HFD)-induced insu-lin resistance (Orr et al.,2012;Saberi et al.,2009).Obesity-associated PAMPs and DAMPs have also been shown to activate the nucleotide-binding domain and leucine-rich-repeat-containing (NLR)protein NLRP3inflammasome,a multiprotein complex comprised of a PRR (NLRP3),a protease (caspase 1),and an adaptor protein.Several studies have shown that obesity is associated with the activation of the inflamma-some in adipose tissue (Stienstra et al.,2012;Vandanmagsar et al.,2011).Upon activation of the inflammasome,caspase 1initiates the maturation of pro-IL-1b and pro-IL-18.Consistent with the proinflammatory effects of these cytokines,genetic ablation of components of the NLRP3inflammasome amelio-rates insulin resistance (Stienstra et al.,2011;Vandanmagsar et al.,2011).In addition to receptor-mediated pathways,inflam-matory signaling can be stimulated by cellular stresses such as reactive oxygen species (ROS),ER stress,hypoxia,and lipotox-icity,which can all be enhanced in the obese insulin-resistant state (Cramer et al.,2003;Furukawa et al.,2004;Samuel and Shulman,2012;Urano et al.,2000;Lee et al.,2014).Mechanisms of Insulin Resistance in InflammationNumerous studies have shown that metabolic inflammation mediates insulin resistance through the inhibition of insulin signaling.Insulin (I)binding to its receptor (R)initiates a compli-cated signaling cascade (Figure 1).In brief,IR activation stimulates the recruitment and phosphorylation of several IR substrates including insulin receptor substrate 1-4(IRS-1-4),src homology 2containing protein (SHC),and growth factorImmunity 41,July 17,2014ª2014Elsevier Inc.37ImmunityReviewreceptor-bound protein2(Grb-2),which leads to the activation of two downstream signaling pathways.The phosphatidylinosi-tol3-kinase pathway(PI3K)-protein kinase B(PKB)pathway plays a major role in eliciting the effects of insulin on metabolism, increasing skeletal muscle and adipocyte glucose uptake, glycogen synthesis,and lipogenesis,while suppressing hepatic glucose production.Activation of the Ras-mitogen activated protein kinase(MAPK)pathway mediates the effect of insulin on mitogenesis and cell growth.Inflammatory signaling can interfere with insulin action through several transcriptional and posttranscriptional mechanisms. First,stress-activated serine kinases,such as JNK and IKK b, phosphorylate IRs and IRS proteins at inhibitory sites,attenu-ating downstream insulin signaling(Gao et al.,2002;Ozes et al.,2001).Accordingly,abrogation of inflammatory signaling with salicylates,which inhibit IKK b,prevents inhibitory IRS-1 phosphorylation,restoring insulin sensitivity(Gao et al.,2003). Second,the transcription factors NF-k B and AP-1regulate the expression of several metabolic genes that influence insulin sensitivity.For example,inflammatory mediators induce the expression of suppressor of cytokine signaling(SOCS)proteins which bind to the insulin receptor and impair its ability to phos-phorylate IRS-1and IRS-2proteins(Emanuelli et al.,2000; Kawazoe et al.,2001;Ueki et al.,2004).Conversely,NF-k B represses the expression of several components of the insulin signaling pathway including glucose transporter type4 (GLUT4)(Stephens and Pekala,1991),IRS-1,and AKT(Ruan et al.,2002).Third,JNK signaling can regulate cytokine expres-sion posttranscriptionally by causing stabilization of mRNAs that encode inflammatory cytokines(Chen et al.,2000).Finally,a relatively recent discovery is that inflammatory signals may also influence insulin sensitivity by regulating microRNA(miRNA) expression.For example,TLR4signaling represses the expres-sion of miR-223,which negatively regulates inflammatory gene expression(Chen et al.,2012;Haneklaus et al.,2013).Several miRNAs are dysregulated in obesity and this topic has been the subject of several recent reviews(Haneklaus et al.,2013;Qu-iat and Olson,2013).Inflammation can also affect insulin action indirectly by modu-lating various metabolic pathways,resulting in the production of ‘‘second messengers,’’such as fatty acids,that promote insulin resistance.For example,TNF-a stimulates adipocyte lipolysis contributing to elevated serum free fatty acid(FFA)concentra-tions,which can lead to decreased insulin sensitivity.Addition-ally,inflammatory signaling induces the expression of genes involved in lipid processing,including the enzymes that synthe-size ceramide,a sphingolipid that inhibits insulin activation of AKT(Holland et al.,2011;Schubert et al.,2000).Indeed,mice lacking TLR4are protected from ceramide accumulation and in-sulin resistance after the infusion of saturated fatty acids (Holland et al.,2011),and treating HFD mice with myriocin,an in-hibitor of ceramide production,improves glucose tolerance (Ussher et al.,2010).Inflammatory mediators also stimulate de novo hepatic lipogenesis,contributing to steatosis and elevated serum lipid levels.Treatment of mice with TNF-a or IL-1b in-creases the activity of acetyl-CoA carboxylase,the rate-limiting step in lipid synthesis(Feingold and Grunfeld,1992).Similarly, transgenic overexpression of IKK-b in hepatocytes stimulates de novo hepatic lipogenesis(van Diepen et al.,2011).NF-k B and AP-1also induce the expression of inflammatory cytokines,which can then act in an autocrine or paracrine manner,initiating a feed-forward loop to exacerbate insulin resistance.In addition,it is thought that if the magnitude of cyto-kine production is great enough,they can‘‘leak’’out of the adi-pose tissue and potentiate insulin resistance in an endocrine fashion in peripheral tissues such as muscle and liver(Osborn and Olefsky,2012).In line with this concept,elevated concentra-tions of TNF-a,IL-6,and MCP-1have been observed in the serum of individuals with diabetes and prospective studies have shown that circulating inflammatory markers are indicative of future disease risk.However,further studies are required to determine whether circulating cytokines are sufficient to induce insulin resistance or whether they are merely a marker of tissue inflammation.Obesity and Adipose Tissue MacrophagesAdipose tissue macrophages(ATMs)can span the spectrum from an anti-inflammatory to a proinflammatory phenotype. The nomenclature to define different macrophage populations is variable and somewhat confusing,as described in the accom-panying review(Murray et al.,2014,this issue).Here we refer to anti-inflammatory macrophages as M2-like or alternatively acti-vated macrophages(AAMs),and proinflammatory macrophages as M1-like or classically activated macrophages(CAMs)(Olefsky and Glass,2010).AAMs predominantly make up the tissue-resident macrophages dispersed throughout lean adipose and support adipose homeostasis(Odegaard et al.,2007). Conversely,during obesity,the balance is tilted toward the recruitment of CAMs,which are primarily found in a ring-like configuration around large dying adipocytes,termed crown-like structures(CLSs)(Lumeng et al.,2008).These two macro-phage populations are phenotypically and functionally distinct. M2macrophages express CD11b,F4/80,CD301,and CD206 and promote local insulin sensitivity through production of anti-inflammatory cytokines,such as IL-10(Olefsky and Glass, 2010).In contrast,M1macrophages express CD11c in addition to CD11b and F4/80and secrete inflammatory factors including TNF-a,IL-1b,IL-6,leukotriene B4(LTB4),and nitric oxide(NO) (Lumeng et al.,2007).The recruitment,differentiation,and/or survival of these macrophage subpopulations are contingent on the local signals produced within adipose tissue.The alternative activation of tissue-resident macrophages is mediated by the type2cytokine IL-4,which is expressed at high amounts in lean adipose tissue (Wu et al.,2011).IL-4induces the expression of peroxisome pro-liferator activated receptor gamma(PPAR g)(Huang et al.,1999) and peroxisome proliferator activated receptor delta(PPAR d) (Kang et al.,2008),which are required for maintenance of the alternatively activated state(Desvergne,2008;Odegaard et al., 2007).Conversely,in the obese state,inflammatory mediators released from adipose tissue,such as saturated fatty acids,cy-tokines,LTB4,and interferon-g(IFN-g),induce the recruitment of monocytes and/or their differentiation into M1-like macro-phages.Macrophage polarization states are also associated with dif-ferential activation of intrinsic biochemical pathways,including those of glucose,lipid,amino acid,and iron metabolism.For example,M1macrophages rely on glycolysis and oxidative38Immunity41,July17,2014ª2014Elsevier Inc.Immunity Reviewphosphorylation of pyruvate,whereas M2macrophages exhibit high rates of fatty acid oxidation(Biswas and Mantovani, 2012).Modifications to macrophage metabolic homeostasis result in altered energy supply and the production of lipid-and amino acid-derived mediators,which enable the macrophage to promote or resolve inflammation and contribute to the mainte-nance of the polarization state.Excellent reviews on this topic have recently been published(Biswas and Mantovani,2012;Re-calcati et al.,2012).Although the classification of these two distinct ATM popula-tions is useful for experimental purposes,it is important to appre-ciate that it is an oversimplification.In vivo,macrophages are a heterogeneous population and can display phenotypes across the spectrum from anti-to proinflammatory.Furthermore, ATMs display plasticity and can alter or‘‘switch’’phenotypes in response to changes in the local microenvironment(Li et al., 2010).Mechanisms of Inflammation-Induced Insulin Resistance:Lessons from Animal ModelsThe most compelling evidence for a mechanistic link between inflammation and insulin resistance has been provided by mu-rine studies that,by a variety of models,have repeatedly demonstrated the etiological role of M1macrophages in insulin resistance(see Table S1available online).Although murine models strongly suggest a role for inflammation in the patho-genesis of insulin resistance in human obesity,thefidelity with which these mouse models translate to man is not proven and there are several differences in immune response mechanisms between mice and men.Definitive anti-inflammatory pharmaco-logical studies will be needed to solidify the applicability of mouse to human disease and this is described in more detail later in this review(see Anti-inflammatory Therapeutic Strate-gies).Nevertheless,several lines of evidence indicate that inflammation is causally linked to insulin resistance in mice. First,the ablation of inflammatory CD11c+myeloid cells(Pat-souris et al.,2008)or depletion of ATMs by intraperitoneal administration of clodronate liposomes(Bu et al.,2013;Feng et al.,2011)improves glucose tolerance in obese insulin-resis-tant mice,confirming the requirement of this immune cell popu-lation in the etiology of insulin resistance.In addition,studies have shown that the polarization state of ATMs is a key determi-nant of the adipose tissue inflammatory milieu and insulin sensi-tivity.Accordingly,mice with a myeloid-specific deletion of the transcriptional regulators PPAR g(Hevener et al.,2007;Ode-gaard et al.,2007)and PPAR d(Desvergne,2008;Kang et al., 2008;Odegaard et al.,2008),which are critical for the mainte-nance of the AAM state,display reduced adipose AAMs and are predisposed to HFD-induced adipose tissue inflammation, glucose intolerance,and insulin resistance.Finally,ablation of JNK(Han et al.,2013;Sabio et al.,2008;Solinas et al.,2007; Vallerie et al.,2008;Zhang et al.,2011)or IKK b(Arkan et al., 2005)protects mice from HFD-induced adipose tissue inflam-mation,confirming the importance of these inflammatory signaling pathways.In these studies,the gene-targeted mice re-tained systemic insulin sensitivity,demonstrating that inhibition of inflammatory signals in macrophages is sufficient to mitigate obesity-induced insulin resistance not only in adipose tissue, but also in muscle and liver.ATM RecruitmentAlthough macrophages are a key effector cell in the propagation of inflammation,it is clear that adipocytes are an important initi-ator of the inflammatory response.Adipocytes are not simply a storage depot for excess energy but are dynamic endocrine cells that produce and secrete both proinflammatory and anti-inflam-matory bioactive molecules,depending on microenvironmental cues.Secretion of these factors can regulate the recruitment and activation of immune cell populations.During the develop-ment of obesity,nutrient excess tips the balance toward the development of a more inflammatory adipocyte state,including the secretion of potent chemoattractants such as MCP-1and LTB4.These chemoattractants provide a chemotactic gradient for the recruitment of monocytes to adipose tissue,where they subsequently mature into ATMs.In addition,once recruited, proinflammatory macrophages themselves secrete additional chemokines,initiating a feed-forward loop and potentiating the inflammatory response.Of the known adipocyte-derived chemokines,MCP-1and its receptor chemokine(C-C motif)receptor2(CCR2)have been intensively studied.Several reports have shown that MCP-1is secreted in parallel with increasing adiposity in both mice and humans(Chen et al.,2005;Christiansen et al.,2005;Kim et al., 2006).In murine models of obesity,adipose tissue expression of MCP-1is rapidly induced after the initiation of HFD feeding and serum MCP-1concentrations are significantly elevated after4weeks of this regime(Chen et al.,2005).In support of the MCP-1-CCR2system playing a role in ATM recruitment, CCR2-and MCP-1-deficient mice exhibit reduced ATM content, insulin resistance,and hyperinsulinemia(Gutierrez et al.,2011; Weisberg et al.,2006),and overexpression of adipocyte MCP-1was sufficient to induce adipose inflammation and insulin resistance in lean mice(Kamei et al.,2006).Furthermore, treatment of mice with a pharmacological antagonist of CCR2 lowered ATM content and improved insulin sensitivity without altering body mass(Sullivan et al.,2013;Tamura et al.,2010). However,other studies have shown that CCR2-deficient mice are not protected from HFD-induced insulin resistance and macrophage accumulation(Chen et al.,2005;Gutierrez et al., 2011).The reasons for these discordantfindings are unclear, but the complexity and redundancy of chemokine signaling in different genetic backgrounds may play a role.The chemoattractant LTB4and its specific receptor BLT1 have also been implicated in macrophage recruitment to in-flamed adipose tissue.LTB4is synthesized from arachadonic acid by the5-lipoxygenase(5-LOX)pathway(Spite et al., 2011).The expression and activity of key components of this pathway are increased in adipocytes and M1macrophages in obesity(Mothe-Satney et al.,2012).Consistent with this,LTB4 concentration is elevated in the adipose tissue and serum of murine models of obesity,in correlation with adipocyte size (Mothe-Satney et al.,2012).Supporting a pathological role for this increase,genetic deletion or pharmacological inhibition of 5-LOX(Mothe-Satney et al.,2012)or5-LO activating protein (FLAP)(Horrillo et al.,2010)protects mice from HFD-induced macrophage accumulation and associated insulin resistance. Targeting the LTB4-BLT1axis more specifically,recent studies show that genetic depletion of BLT1protects mice from obesity-induced inflammation and insulin resistance(Spite Immunity41,July17,2014ª2014Elsevier Inc.39Immunity Reviewet al.,2011),making this receptor an attractive potential target for drug discovery.Neuronal guidance molecules,factors typically studied for their role in embryonic axon development,were recently found to participate in the regulation of immune cell function.So far, four families of neural guidance cues have been implicated in the regulation of immune cell migration:the netrins,slits, ephrins,and semaphorins(Funk and Orr,2013;Wanschel et al.,2013).One such molecule,Semaphorin3E(Sema3E), can act as an adipocyte-derived chemokine to induce macro-phage recruitment to adipose tissue via its receptor PlexinD1, expressed on ATMs.Shimizu et al.(2013)observed that HFD feeding selectively increased Sema3E expression in visceral adipose tissue,accompanied by a parallel increase in serum Sema3E levels.Overexpression of Sema3E in adipocytes induced adipose tissue inflammation and insulin resistance in chow-fed mice,whereas genetic deletion of Sema3E or the sequestration of serum Sema3E with a soluble form of PlexinD1markedly improved these parameters.Sema3E is also elevated in the serum of diabetic humans,suggesting that this pathway may play a role in human disease(Schmidt and Moore,2013).ATM RetentionThe majority of studies on ATM accumulation have focused on the recruitment of monocytes to inflamed adipocytes,but macrophage emigration from adipose tissue might also be impaired in the obese state.The resolution of inflammation is a highly orchestrated process involving several cell types and mediators.The egress of macrophages out of inflamed tissue to local lymphoid tissues is an integral part of this process and is due to the concerted effect of chemo-repulsive forces from inflamed tissue and chemo-attractive signals from local lymph nodes(Bellingan et al.,1996;Randolph,2008).In addition to classical chemokines,neural guidance molecules also regulate this process(van Gils et al.,2012).The concept that macrophage emigration might be impaired in obese adipose tissue stems from the study of macrophage retention in atherosclerotic plaques.In murine models of athero-sclerosis,lowering of serum cholesterol concentrations or trans-plantation of the aortic arch from atherosclerotic LDL receptor KO mice to WT mice reestablishes macrophage egress to lymph nodes,reducing artery wall inflammation and plaque instability (Feig et al.,2011).These studies have led to the identification of key pathways that regulate this process.For example,the chemokine receptor CCR7,which is expressed on macro-phages,promotes the recruitment of inflammatory macro-phages toward chemokine(C-C motif)ligand19(CCL19)and CCL21,secreted from lymphoid tissues.Upregulation of CCR7 by atheroma macrophages is necessary for the resolution of inflammation induced by the correction of dyslipidemia(Wan et al.,2013).There may also be signals that emanate from adipose tissue that prevent macrophage egress.For example,Netrin-1, secreted by macrophages in mouse atheroma,acts in an auto-crine/paracrine manner to retard the egress of macrophages that express the Netrin-1receptor rin-1is particularly interesting because,unlike other chemokines,it blocks macro-phage movement by inhibiting actin reorganization,making cells refractory to further chemokine stimuli.It is likely that expression of Netrin-1by adipocytes or ATMs potentiates the inflammatory phenotype of obese adipose tissue by inhibiting the process of resolution.Inflammation in Other Tissue TypesGiven the obvious connection between obesity and adiposity, studies have naturally focused on obesity-driven inflammation in adipose tissue.However,obesity can also causes inflamma-tion in other metabolic tissues such as liver,pancreatic islets, and perhaps also muscle.The liver is the major source of endogenous glucose produc-tion,which in the normal state is inhibited by the postprandial rise in insulin elevations.When the liver is insulin resistant, this inhibitory effect is impaired while the stimulatory effect of in-sulin on lipogenesis remains intact,contributing to the develop-ment of hyperglycemia and hepatic steatosis.Many studies have shown that obesity induces hepatic inflammation(Lanthier et al., 2011;Osborn and Olefsky,2012)associated with a substantial increase in liver macrophages(Johnson and Olefsky,2013;Ob-stfeld et al.,2010).As in adipose,liver macrophages comprise two populations-resident macrophages,termed Kupffer cells (KCs)and recruited hepatic macrophages(RHMs).KCs are long lived and relatively abundant in the liver,representing about 20%–25%of nonparenchymal cell population,in the nonin-flamed state(Tang et al.,2013).KCs play an important role in tissue homeostasis,clearing foreign and harmful particles,for which their location in the liver sinusoids makes them well posi-tioned.In contrast,recruited macrophages are short lived and enter the liver in increased numbers during obesity,due to the secretion of chemokines,particularly MCP-1(Obstfeld et al., 2010;Oh et al.,2012).Chemical ablation of phagocytic cells in the liver(including KCs and RHMs)protects mice from HFD-induced insulin resistance,demonstrating the importance of these cells in the development of metabolic dysfunction(Lanthier et al.,2011;Neyrinck et al.,2009).In addition,genetic models have been used to establish a role for hepatic inflammation in insulin sensitivity.Depletion or overexpression of IKK b,specif-ically within hepatocytes,has shown that hepatic inflammation can regulate local insulin sensitivity,but not peripheral insulin sensitivity,in muscle and fat(Arkan et al.,2005;Cai et al., 2005).In obesity,the situation in liver is similar to that in adipose tissue with increased recruitment and activation of liver macro-phages,increased inflammatory signaling,and local production of inflammatory cytokines and chemokines.It is likely that the inflammatory cytokines exert paracrine effects to cause hepatic insulin resistance,similar to the situation in adipose tissue(see Figure2).Skeletal muscle is the primary site of glucose uptake,account-ing for around80%of insulin-stimulated glucose disposal(Os-born and Olefsky,2012).Therefore,decreased muscle insulin sensitivity in obesity has a profound effect on hyperglycemia in insulin-resistant individuals.Several studies have shown that obesity is associated with increased muscle inflammatory gene expression,along with macrophage infiltration in both mice and humans(Fink et al.,2013,2014;Hevener et al.,2007; Nguyen et al.,2007).These macrophages are largely localized to the small intermuscular adipose depots(termed marbling) that arise within skeletal muscle in obesity(Fink et al.,2014).40Immunity41,July17,2014ª2014Elsevier Inc.Immunity Review。
LCAV,

1
LCAV, 3 IOA, Ecole Polytechnique F´ ed´ erale de Lausanne, Switzerland 2 EECS Dept., University of California at Berkeley, USA email: [martin.vetterli,pina.marziliano,thierry.blu]@epfl.chK −1 Nhomakorabea0
100
−0.5
50
−1
XD [m]
−100 −50 0 50 100 150
=
k=0
nk m ck WN ,
m = 0, . . . , N − 1 (5)
0
−1.5 −32 0 32 64 96 128 160 192 224 256 288
−150
(c)
Piecewise bandlimited signal 2
1
0.5
150
where δ [n] is the Kronecker delta and equal to 1 if n = 0 and 0 if n = 0. The corresponding discrete-time Fourier series (DTFS) coefficients are defined by
Piecewise bandlimited signal
0.25
We consider sampling discrete-time periodic signals which are piecewise bandlimited, that is, a signal that is the sum of a bandlimited signal with a piecewise polynomial signal containing a finite number of transitions. These signals are not bandlimited and thus the Shannon1 sampling theorem for bandlimited signals can not be applied. In this paper, we derive sampling and reconstruction schemes based on those developed in [1, 6, 7] for piecewise polynomial signals which take into account the extra degrees of freedom due to the bandlimitedness. 1. INTRODUCTION Sampling of bandlimited signals has been a subject of interest to the sampling community for more than half a century [4]. The well-known sampling theorem [2] states that a continuous-time signal x(t) bandlimited to [−ωm , ωm ] is uniquely represented by a uniform set of samples x[n] = x(nT ) taken T seconds apart, if the sampling rate is greater or equal to the bandwidth of the signal, that is, 2π/T ≥ 2ωm . But not all signals are bandlimited. Recall the definition of bandlimited. Definition 1 B −bandlimited signal. A discrete-time periodic signal x[n] with period N is B −bandlimited if the discrete-time Fourier series coefficients X [k ] are nonzero inside the band [−B, B ] and zero outside the band [−B, B ], that is, N −1 x[n] e−i2πnk/N k ∈ [−B, B ] (1) X [k ] = n =0 0 k ∈ [−B, B ] with k ∈ Z, B ∈ N In [1, 6, 7] sampling theorems for particular nonbandlimited signals namely streams of Diracs and piecewise polynomial signals were given. These signals
统计数据英文作文
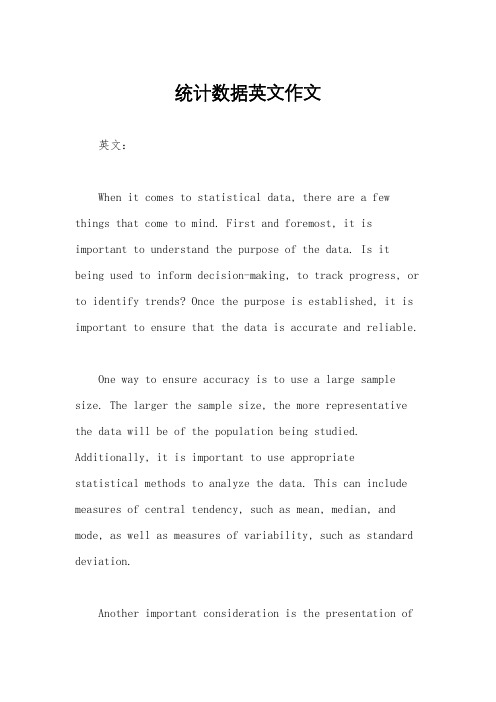
统计数据英文作文英文:When it comes to statistical data, there are a few things that come to mind. First and foremost, it is important to understand the purpose of the data. Is it being used to inform decision-making, to track progress, or to identify trends? Once the purpose is established, it is important to ensure that the data is accurate and reliable.One way to ensure accuracy is to use a large sample size. The larger the sample size, the more representative the data will be of the population being studied. Additionally, it is important to use appropriatestatistical methods to analyze the data. This can include measures of central tendency, such as mean, median, and mode, as well as measures of variability, such as standard deviation.Another important consideration is the presentation ofthe data. Data can be presented in a variety of ways, including tables, graphs, and charts. The choice of presentation method will depend on the purpose of the data and the audience it is intended for. For example, a graph may be more effective for presenting trends over time, while a table may be more appropriate for comparing data across different categories.Overall, statistical data can be a powerful tool for informing decision-making and identifying trends. However, it is important to ensure that the data is accurate, reliable, and presented in a way that is appropriate for the intended audience.中文:谈到统计数据,有几个重要的方面需要考虑。
市场调查方法(英文版)第十四章
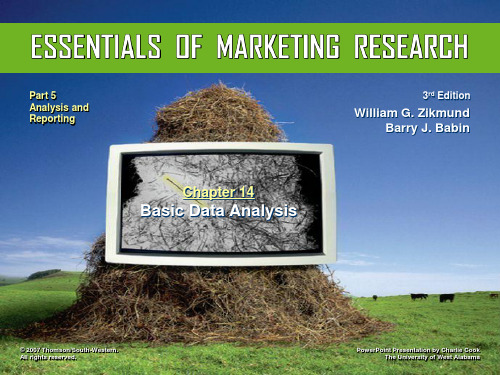
➢ Elaboration analysis: an analysis of the basic crosstabulation for each level of a variable not previously considered, such as subgroups of the sample.
Hale Waihona Puke © 2007 Thomson/South-Western. All rights reserved.
14–6
EXHIBIT 14.2 Cross-Tabulation Tables from a Survey on Ethics in America
From Roger Ricklefs, “Ethics in America,” The Wall Street Journal, October 31, 1983, p. 33, 42; November 1, 1983, p. 33; November 2, 1983, p. 33; and November 3, 1983, pp. 33, 37.
the reader to view several cross-tabulations at once
• Charts and Graphs
➢ Translate information into visual forms so that relationships may be easily grasped
Part 5 Analysis and Reporting
Chapter 14
Basic Data Analysis
3rd Edition
William G. Zikmund Barry J. Babin
Has privatization of state-owned enterprises in Iran led to improved performance
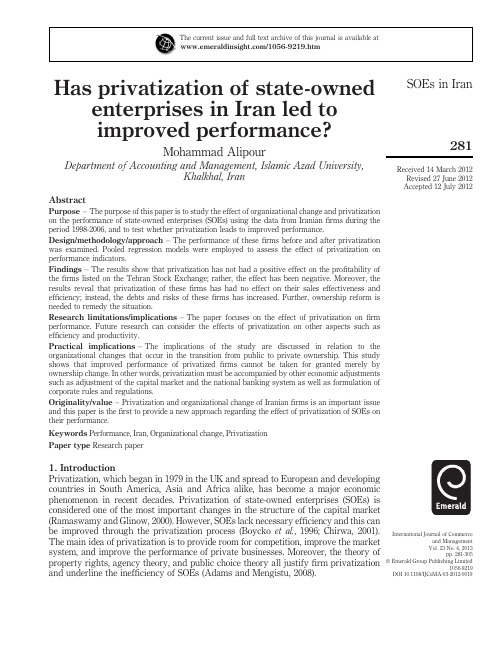
Has privatization of state-ownedenterprises in Iran led to improved performance?Mohammad AlipourDepartment of Accounting and Management,Islamic Azad University,Khalkhal,IranAbstractPurpose –The purpose of this paper is to study the effect of organizational change and privatization on the performance of state-owned enterprises (SOEs)using the data from Iranian firms during the period 1998-2006,and to test whether privatization leads to improved performance.Design/methodology/approach –The performance of these firms before and after privatization was examined.Pooled regression models were employed to assess the effect of privatization on performance indicators.Findings –The results show that privatization has not had a positive effect on the profitability of the firms listed on the Tehran Stock Exchange;rather,the effect has been negative.Moreover,the results reveal that privatization of these firms has had no effect on their sales effectiveness and efficiency;instead,the debts and risks of these firms has increased.Further,ownership reform is needed to remedy the situation.Research limitations/implications –The paper focuses on the effect of privatization on firm performance.Future research can consider the effects of privatization on other aspects such as efficiency and productivity.Practical implications –The implications of the study are discussed in relation to the organizational changes that occur in the transition from public to private ownership.This study shows that improved performance of privatized firms cannot be taken for granted merely by ownership change.In other words,privatization must be accompanied by other economic adjustments such as adjustment of the capital market and the national banking system as well as formulation of corporate rules and regulations.Originality/value –Privatization and organizational change of Iranian firms is an important issue and this paper is the first to provide a new approach regarding the effect of privatization of SOEs on their performance.Keywords Performance,Iran,Organizational change,Privatization Paper type Research paper1.IntroductionPrivatization,which began in 1979in the UK and spread to European and developing countries in South America,Asia and Africa alike,has become a major economic phenomenon in recent decades.Privatization of state-owned enterprises (SOEs)is considered one of the most important changes in the structure of the capital market (Ramaswamy and Glinow,2000).However,SOEs lack necessary efficiency and this can be improved through the privatization process (Boycko et al.,1996;Chirwa,2001).The main idea of privatization is to provide room for competition,improve the market system,and improve the performance of private businesses.Moreover,the theory of property rights,agency theory,and public choice theory all justify firm privatization and underline the inefficiency of SOEs (Adams and Mengistu,2008).The current issue and full text archive of this journal is available at /1056-9219.htmReceived 14March 2012Revised 27June 2012Accepted 12July 2012International Journal of Commerceand Management Vol.23No.4,2013pp.281-305q Emerald Group Publishing Limited1056-9219DOI 10.1108/IJCoMA-03-2012-0019SOEs in Iran281Privatization is a means for improving firm performance through organizational change and increasing the role of the private sector,providing that at least 51percent of the shares of SOEs are transferred to the private sector.Thus,we can argue that privatization is the transfer of shares from SOEs to the private sector (Bortolotti et al.,2003).Ramamurti (2000)defines privatization as selling out the SOEs to the private sector.The profitability of the private sector is expected to increase following the transfer of ownership from the public to the private sector;privatization makes managers more focused on increasing profitability,since in the private sector managers must directly respond to shareholders (Yarrow,1986).Moreover,poor economic and financial performance of most SOEs necessitates their privatization (Lee,2006).According to Tian (2001)and Boycko et al.(1996),privately owned enterprises and privatized firms have a better performance record in comparison with SOEs,and other studies have shown that privatization improves firm performance (Boardman et al.,2002;Chang,2007).In Iran,public firms have been transferred to the private sector since 1991.Taking into consideration the more than 20years’experience of privatization in this country,the purpose of the present paper is to study the effect of privatization on the performance of the firms listed on the Tehran Stock Exchange (TSE)whose shares have been transferred to the private sector by at least 50percent.Indeed,it is expected that privatization will have a positive effect on the performance of these firms.Among the developing and developed countries,more than 100countries have implemented the privatization initiative and a large body of research has been dedicated to studying the effect of privatization on the performance of firms.Some researchers state that this process motivates managers and makes them reconsider their goals,thus leading to improved performance of firms.Most studies have found little or no improvement or have even found a decline in performance following an IPO as a result of government control (Chen et al.,2006,2008;Sun and Tong,2003;Wang,2005;Garcia and Anson,2007;Al-Jazzaf,1999;Omran,2002).Limi (2003)argues that privatization may or may not have a positive effect on financial performance.The analysis of the impact of privatization on financial performance of enterprises in Iran is important since:.There are high expectations placed upon privatization,by policy makers and the public at large,to improve enterprise performance and achieve macroeconomic stabilization..Many earlier studies have focused on firms in developed markets and there is an increasing amount of literature on firms in developing markets,overlooking countries in the Middle East.Moreover,this study contributes to the literature on privatization because it adds new empirical evidence about Iran,questioning whether privatization programs have helped to improve the performance of the companies.Our analysis of a sample of Iranians SOEs privatized during the period 1998-2006shows that privatization is associated with a significant decline in firm performance.This is consistent with the findings of Chen et al.(2006,2008),Sun and Tong (2003),Wang (2005),Garcia and Anson (2007),Al-Jazzaf (1999),Omran (2002)and Li et al.(2007).Privatizations in Iran have had a less favorable outcome relative to the experience of other countries.IJCOMA 23,4282The remainder of the paper is organized as follows:in Section2,the literature on the effect of privatization on performance is provided;Section3deals with the process of privatization in Iran;and Section4presents research methodology,variables,and the samples.Finally,Section5involves data analysis and the conclusions are presented in Section6.2.A review of the literatureIt is expected that privatization will change the mechanisms and structures offirms, change the motives of business managers and will consequently improvefirm performance(Laffont and Tirole,1991).The empirical literature on privatization has found significant performance gains from privatizing former state-ownedfirms. Megginson et al.(1994)compared the average operating performance of61firms from 18different countries and32industries three years before and after privatization and reported economically and statistically significant post-privatization increases in output(real sales),operating efficiency,profitability,capital investment spending,and dividend payments,as well as significant decreases in leverage.There was no evidence of employment declines after privatization,but significant changes infirm directors. They thus concluded that privatization improvesfirm performance.D’Souza and Megginson(1999)studied78firms from ten developing countries and 15developed countries over the period1990-1994and reported significant post-privatization improvement in performance indicators(e.g.output(real sales), operating efficiency and profitability),as well as significant decreases in leverage. Capital investment spending increases,but insignificantly,while employment declines significantly.Dewenter and Malatesta(2001)compared pre-vs post-privatization performance of 63large,high information companies divested during1981-1994over both short-and long-term horizons.They also examined the long-run stock return performance of privatizedfirms and compared the relative performance of a large sample(1,500firm-year observations)of state and privately ownedfirms during1975, 1985and1995.They documented significant increases in profitability(using net income)and significant decreases in leverage and labor intensity(employees/sales) over both short-and long-term comparison horizons.They reported operating profits increase prior to privatization,but not after it.Their results also strongly indicated that privatefirms outperform state-ownedfirms.Macquieira and Zurita(1996)studied the performance of22privatizedfirms in Chile before and after privatization.Theyfirst tested for performance changes without adjusting for overall improvements in the Chilean economy,then with an adjustment for changes experienced by other Chileanfirms over the study period.They also documented significant increases in output,profitability,employment,investment and dividend payments.After adjusting for market movements,however,the changes in output,employment,and liquidity were no longer significant,and they found that averagefirm leverage increases significantly.Boubakri and Cosset(1998)compared the pre-and post-privatization performance of79firms from21developing countries and32industries over the period1980-1992 and they also reported significant post-privatization increases in output(real sales), operating efficiency,profitability,capital investment spending,dividend payments, and employment,as well as significant decreases in leverage.Omran(2001)studied the SOEs in Iran283change in performance of 69privatized firms between 1994and 1998in Egypt and found that profitability,operating efficiency,capital spending,dividends,and liquidity increase significantly after privatization,while leverage,employment,and financial risk (measured as the inverse of times interest earned)decline significantly.Boubakri and Cosset (2002)examined pre-vs post-privatization performance of 16African firms privatized through public share offering during the period 1989-1996and documented significant increase in capital spending by privatized firms,but found only insignificant changes in profitability,efficiency,output,and leverage.Sun and Tong (2002)compared the pre-vs post-privatization performance of 24firms in Malaysia and showed that privatization has led to an increase in profitability and dividends of these firms,as well as reduced leverage.Bortolotti et al.(2002)examined the financial and operating performance of 31telecommunication companies around the world which were either completely or partially privatized.They found that profitability,output,operating efficiency,and capital investment spending increase significantly after privatization,while employment and leverage decline significantly.Claessens and Djankov (2002)studied the advantages of privatization in seven Eastern European countries and concluded that privatization greatly increases sales,efficiency,and labor productivity.Viani (2004)studied telecommunication companies in developing countries.He selected 23companies as a sample from 1986to 2001and came to the conclusion that the state-owned companies had less efficiency in comparison with privatized companies,yet their profitability was higher.Omran (2004)examined the pre-vs post-privatization performance of 69firms in Egypt which were privatized between 1994and 1998and found significant increases in profitability,operating efficiency,capital expenditures,and dividends.Conversely,significant decreases in employment,leverage,and risk were found,although output showed an insignificant decrease following privatization.Megginson (2005)provided an excellent survey of the empirical literature on bank privatization.Based on a review of a large number of studies,he concluded that private banks are usually more efficient than state-owned banks.However,in the case of partial privatization,the effects on performance depend on institutional and regulatory environments.Gupta (2005)studied partial privatization in 38Indians firms between 1990and 1998and found that partial privatization has positive and highly significant impact on firm sales,profits,and labor productivity.Cheelo and Munalula (2005)examined the effect of privatization on 48banks in Zambia from 1997to 1999and reported the great positive effect of privatization on the profitability and output of these banks.Jia et al.(2005)studied 53semi-privatized firms in China from 1993to 2002that were listed on the Hong Kong Stock Exchange and found that real net profit significantly increased for the majority of firms,return on sales significantly declined on average,and real output increased.They also found that these firms underperformed the Hong Kong market over the long run.They finally concluded that listing on an established stock exchange and allowing foreign investors to purchase shares improves the performance of SOEs.Boubakri et al.(2005)analyzed the post-privatization performance of 81banks in 22developing countries.Analysis of accounting indicators of performance revealed that the poor performers were selected for privatization and the impact of privatization on performance was ambiguous.While profitability increased,the impact on efficiency,risk exposure,and capitalization largely depended on whether the control of theIJCOMA 23,4284privatized bank rested with the government,foreign investors,local industrial groups, or individuals.Berger et al.(2005)examined the performance of privatized banks in Argentina during the1990s.They found that privatized banks strongly outperformed state-owned banks.They also reported that moving from state-owned to private improves the performance of individual banks.Beck et al.(2005)studied the Nigerian banking system using data on accounting measures of performance for69banks.They concluded that privatization leads to improved performance and those banks that continued to have minority government ownership performed worse than fully privatized banks.Loc et al.(2006)studied the effect of privatization onfirm performance in Vietnam.They examined thefinancial and operating performance of121firms from1993to2004before and after privatization and found that profitability,sales revenue,employee income,and efficiency of thesefirms increased after privatization.Mathur and Banchuenvijit(2007)examined the changes in thefinancial and operating performance of103firms worldwide that were privatized through public offering during1993-2003in both emerging markets and developed countries.The empirical results from the Wilcoxon and proportion tests showed increases in profitability,operating efficiency,capital spending,output,and dividend payments,as well as decreases in leverage and total employment.Otchere(2007)studied56privatized banks in various developed countries and reported that privatization has improved the operating performance and stock market performance of these banks.Kerr et al.(2008)examined the long-term performance of private IPO companies in New Zealand and Australia and concluded that privatization has a great effect on investment and market liquidity and that,generally,those who invest in these companies gain higher yields.Astami et al.(2010)studied157companies in Indonesia for the year2006and showed that privately owned enterprises have higher levels of performance than those fully owned by the government.There were also significant differences infinancial leverage,firm size,assets-in-place,financial statement reliability,and industry variances between fully privatized and partially privatized SOEs.Sarkar and Sensarma(2010)examined the impact of partial privatization on performance of state-owned banks using data from the Indian banking industry during the period1986-2003.Choosing26partially-private banks,they showed that partial privatization results in significant improvement in performance of state-owned banks.Thisfinding was robust to alternative model specifications and different techniques for controlling potential selection ing data on Indian Government-ownedfirms,Dinc and Gupta(2011)investigated the influence of political andfinancial factors on the decision to privatize government-ownedfiing political variables as an instrument for the privatization decision,they found that privatization has a positive impact onfirm performance.However,reviewing the literature reveals that privatization does not always lead to improvement in the performance offirms.Vining and Boardman(1992)examined 87research studies and concluded that only28articles had reported improvement of performance after privatization.Aivazian et al.(2005)argue that even without privatization,corporate governance reform is potentially an effective way of improving the performance of SOEs.Chen et al.(2009)argue that the operating efficiency of Chinese listed companies varies across the type of controlling shareholders,SOE controlledfirms perform best,and state asset management bureaus and private controlledfirms perform worst.SOEs in Iran285Al-Jazzaf (1999)examined the impact of privatization on airline performance in ten countries and found that sales grow rapidly and net income,total assets,capital expenditures,and dividends increase moderately after privatization.Furthermore,efficiency and yield improve after privatization.However,profitability slightly declines due to increases in capital investment spending and airlines financial and administrative restructuring costs.Omran (2002)compared the performance of privatized firms to a matched set of 54firms that remained state owned and found that SOEs’performance also improves significantly during the post-privatization period and that privatized companies do not perform any better than SOEs.Feng et al.(2002)studied the effect of privatization on the financial and operating performance of 31firms in Singapore and reported insignificant improvement in the post-privatization performance of these firms.They found that output and leverage improve but efficiency deteriorates after privatization.Sun and Tong (2003)used a relatively small 1994-1998panel data set of 634listed firms to examine the effect of ownership on three indicators of profitability.They found that privatization was effective in improving the SOEs’earnings ability and real sales,as well as labor productivity,but was not so effective in improving profits and leverage.Fong and Lam (2004)studied the performance of 132firms in China and found that privatization only boosts performance of firms in the competitive manufacturing industry,not in the industry closely guarded by the government.They also found that privatization to institutions harms the firms in the more competitive industry,but it does not affect the performance of firms in the closely guarded industry.Otchere (2005)examined operating and stock price performance of 18privatized banks and their 28rivals in low-and middle-income countries and found that privatized banks underperform the market in the long run.He also found that the operating performance of privatized banks increases slightly.Chen et al.(2006)investigated the operating performance of 1,078privatized firms in China.They found that there is a decline in profitability and asset utilization in the five years after privatization and this contrasts with the results of privatizations in other countries,which show improvements in financial performance.However,they also found that performance is a function of who controls the firm after its listing.In particular,the decline in performance is much less when private investors control the firm.Garcia and Anson (2007)studied the effect of the Spanish privatization process on the performance and corporate governance of the firms that were privatized through public offerings over the period ing conventional pre-vs post-privatization comparisons,they did not find significant improvements in privatized firms’profitability and efficiency.Chen et al.(2008)examined the effect of restructuring on the performance of 79SOEs in China and found that there is no improvement in efficiency and profitability after the restructuring.Goldeng et al.(2008)compared privately-owned and state-owned firms during the 1990s in Norway and showed that POEs have a better performance in comparison with SOEs.Bachiller (2009)studied the effect of ownership on efficiency in Spanish companies and showed that the improvements in efficiency are not related to privatization.The results of the research that have reported improved or declined performance as a result of privatization are presented in Tables I and II,respectively.we propose the following as our main testable hypothesis:H1.A firm’s operating profitability increases after privatization.IJCOMA 23,4286S t u d yS a m p l e a n d s t u d y p e r i o d S u m m a r y o f e m p i r i c a l fin d i n g s a n d c o n c l u s i o n sM e g g i n s o n e t a l.(1994)C o m p a r e d t h e fin a n c i a l a n d o p e r a t i n g p e r f o r m a n c e o f 61fir m s f r o m 18d i f f e r e n t c o u n t r i e s a n d 32i n d u s t r i e s t h r e e y e a r s b e f o r e a n d a f t e r p r i v a t i z a t i o n f o r t h e p e r i o d 1961a n d 1989.E x a m i n e d fin a n c i a l r a t i o s b e f o r e a n d a f t e r p r i v a t i z a t i o nR e p o r t e d e c o n o m i c a l l y a n d s t a t i s t i c a l l y s i g n i fic a n t p o s t -p r i v a t i z a t i o n i n c r e a s e s i n o u t p u t (r e a l s a l e s ),o p e r a t i n g e f fic i e n c y ,p r o fit a b i l i t y ,c a p i t a l i n v e s t m e n t s p e n d i n g ,a n d d i v i d e n d p a y m e n t s ,a s w e l l a s s i g n i fic a n t d e c r e a s e s i n l e v e r a g e .T h e r e w a s n o e v i d e n c e o f e m p l o y m e n t d e c l i n e s a f t e r p r i v a t i z a t i o n ,b u t s i g n i fic a n t c h a n g e s i n fir m d i r e c t o r s .T h e y t h u s c o n c l u d e d t h a t p r i v a t i z a t i o n i m p r o v e s fir m p e r f o r m a n c e D e w e n t e r a n d M a l a t e s t a (2001)C o m p a r e d p r e -v s p o s t -p r i v a t i z a t i o n p e r f o r m a n c e o f 63l a r g e ,h i g h i n f o r m a t i o n c o m p a n i e s d i v e s t e d d u r i n g 1981-1994o v e r b o t h s h o r t -a n d l o n g -t e r m h o r i z o n s .T h e y a l s o e x a m i n e d t h e l o n g -r u n s t o c k r e t u r n p e r f o r m a n c e o f p r i v a t i z e d fir m s a n d c o m p a r e d t h e r e l a t i v e p e r f o r m a n c e o f a l a r g e s a m p l e (1,500fir m -y e a r s o b s e r v a t i o n )o f s t a t e a n d p r i v a t e l y o w n e d fir m s d u r i n g 1975,1985a n d 1995D o c u m e n t e d s i g n i fic a n t i n c r e a s e s i n p r o fit a b i l i t y (u s i n g n e t i n c o m e )a n d s i g n i fic a n t d e c r e a s e s i n l e v e r a g e a n d l a b o r i n t e n s i t y (e m p l o y e e s /s a l e s )o v e r b o t h s h o r t -a n d l o n g -t e r m c o m p a r i s o n h o r i z o n s .T h e y r e p o r t e d o p e r a t i n g p r o fit s i n c r e a s e p r i o r t o p r i v a t i z a t i o n ,b u t n o t a f t e r i t .T h e i r r e s u l t s a l s o s t r o n g l y i n d i c a t e d t h a t p r i v a t e fir m s o u t -p e r f o r m s t a t e -o w n e d fir m s M a c q u i e i r a a n d Z u r i t a (1996)S t u d i e d t h e p e r f o r m a n c e o f 22p r i v a t i z e d fir m s i n C h i l e b e f o r e a n d a f t e r p r i v a t i z a t i o nU n a d j u s t e d r e s u l t s v i r t u a l l y i d e n t i c a l t o M N R o v e r t h e s t u d y p e r i o d .T h e y d o c u m e n t e d s i g n i fic a n t i n c r e a s e s i n o u t p u t ,p r o fit a b i l i t y ,e m p l o y m e n t ,i n v e s t m e n t a n d d i v i d e n d p a y m e n t s .A f t e r a d j u s t i n g f o r m a r k e t m o v e m e n t s ,h o w e v e r ,t h e c h a n g e s i n o u t p u t ,e m p l o y m e n t ,a n d l i q u i d i t y w e r e n o l o n g e r s i g n i fic a n t ,a n d t h e y f o u n d t h a t a v e r a g e fir m l e v e r a g e i n c r e a s e s s i g n i fic a n t l y B o u b a k r i a n d C o s s e t (1998)C o m p a r e d t h e p r e -a n d p o s t -p r i v a t i z a t i o n p e r f o r m a n c e o f 79fir m s f r o m 21d e v e l o p i n g c o u n t r i e s a n d 32i n d u s t r i e s o v e r t h e p e r i o d 1980-1992R e p o r t e d s i g n i fic a n t p o s t -p r i v a t i z a t i o n i n c r e a s e s i n o u t p u t (r e a l s a l e s ),o p e r a t i n g e f fic i e n c y ,p r o fit a b i l i t y ,c a p i t a l i n v e s t m e n t s p e n d i n g ,d i v i d e n d p a y m e n t s a n d e m p l o y m e n t ,a s w e l l a s s i g n i fic a n t d e c r e a s e s i n l e v e r a g e (c o n t i n u e d )Table I.Studies that have reported improved performance as a result ofprivatizationSOEs in Iran287S t u d yS a m p l e a n d s t u d y p e r i o d S u m m a r y o f e m p i r i c a l fin d i n g s a n d c o n c l u s i o n sD ’S o u z a a n d M e g g i n s o n (1999)S t u d i e d 78fir m s f r o m t e n d e v e l o p i n g c o u n t r i e s a n d 15d e v e l o p e d c o u n t r i e s o v e r t h e p e r i o d 1990-1994R e p o r t e d s i g n i fic a n t p o s t -p r i v a t i z a t i o n i m p r o v e m e n t i n p e r f o r m a n c e i n d i c a t o r s (e .g .o u t p u t (r e a l s a l e s ),o p e r a t i n g e f fic i e n c y a n d p r o fit a b i l i t y ),a s w e l l a s s i g n i fic a n t d e c r e a s e s i n l e v e r a g e .C a p i t a l i n v e s t m e n t s p e n d i n g i n c r e a s e s ,b u t i n s i g n i fic a n t l y ,w h i l e e m p l o y m e n t d e c l i n e s s i g n i fic a n t l y O m r a n (2001)S t u d i e d t h e c h a n g e i n p e r f o r m a n c e o f 69p r i v a t i z e d fir m s b e t w e e n 1994a n d 1998i n E g y p tF o u n d t h a t p r o fit a b i l i t y ,o p e r a t i n g e f fic i e n c y ,c a p i t a l s p e n d i n g ,d i v i d e n d s a n d l i q u i d i t y i n c r e a s e s i g n i fic a n t l y a f t e r p r i v a t i z a t i o n ,w h i l e l e v e r a g e ,e m p l o y m e n t ,a n d fin a n c i a l r i s k d e c l i n e s i g n i fic a n t l y B o u b a k r i a n d C o s s e t (2002)E x a m i n e d p r e -v s p o s t -p r i v a t i z a t i o n p e r f o r m a n c e o f 16A f r i c a n fir m s p r i v a t i z e d t h r o u g h p u b l i c s h a r e o f f e r i n g d u r i n g t h e p e r i o d 1989-1996D o c u m e n t e d s i g n i fic a n t i n c r e a s e i n c a p i t a l s p e n d i n g b y p r i v a t i z e d fir m s ,b u t f o u n d o n l y i n s i g n i fic a n t c h a n g e s i n p r o fit a b i l i t y ,e f fic i e n c y ,o u t p u t a n d l e v e r a g e S u n a n d T o n g (2002)C o m p a r e d t h e p r e -v s p o s t -p r i v a t i z a t i o n p e r f o r m a n c e o f 24fir m s i n M a l a y s i aS h o w e d t h a t p r i v a t i z a t i o n h a s l e d t o a n i n c r e a s e i n p r o fit a b i l i t y o f t h e s e fir m s a n d d i v i d e n d s a n d r e d u c e d l e v e r a g e B o r t o l o t t i e t a l.(2002)E x a m i n e d t h e fin a n c i a l a n d o p e r a t i n g p e r f o r m a n c e o f 31t e l e c o m m u n i c a t i o n c o m p a n i e s a r o u n d t h e w o r l d o v e r t h e p e r i o d N o v e m b e r 1981t o N o v e m b e r 1998,w h i c h w e r e e i t h e r c o m p l e t e l y o r p a r t i a l l y p r i v a t i z e dF o u n d t h a t p r o fit a b i l i t y ,o u t p u t ,o p e r a t i n g e f fic i e n c y ,a n d c a p i t a l i n v e s t m e n t s p e n d i n g i n c r e a s e d s i g n i fic a n t l y a f t e r p r i v a t i z a t i o n ,w h i l e e m p l o y m e n t a n d l e v e r a g e d e c l i n e d s i g n i fic a n t l y C l a e s s e n s a n d D j a n k o v (2002)S t u d i e d t h e a d v a n t a g e s o f p r i v a t i z a t i o n i n s e v e n E a s t e r n -E u r o p e a n c o u n t r i e sF o u n d t h a t p r i v a t i z a t i o n i s a s s o c i a t e d w i t h s i g n i fic a n t i n c r e a s e s i n s a l e s r e v e n u e s a n d l a b o r p r o d u c t i v i t y ,a n d ,t o a l e s s e r e x t e n t ,w i t h f e w e r j o b l o s s e s V i a n i (2004)S t u d i e d t h e t e l e c o m m u n i c a t i o n c o m p a n i e s i n d e v e l o p i n g c o u n t r i e s .H e s e l e c t e d 23c o m p a n i e s a s s a m p l e f r o m 1986t o 2001F o u n d t h a t t h e s t a t e -o w n e d c o m p a n i e s h a d l e s s e f fic i e n c y i n c o m p a r i s o n w i t h p r i v a t i z e d c o m p a n i e s ,y e t t h e i r p r o fit a b i l i t y w a s h i g h e r O m r a n (2004)E x a m i n e d t h e p r e -v s p o s t -p r i v a t i z a t i o n p e r f o r m a n c e o f 69fir m s i n E g y p t w h i c h w e r e p r i v a t i z e d b e t w e e n 1994a n d 1998D o c u m e n t e d s i g n i fic a n t i n c r e a s e s i n p r o fit a b i l i t y ,o p e r a t i n g e f fic i e n c y ,c a p i t a l e x p e n d i t u r e s ,a n d d i v i d e n d s .C o n v e r s e l y ,s i g n i fic a n t d e c r e a s e s i n e m p l o y m e n t ,l e v e r a g e ,a n d r i s k a r e f o u n d ,a l t h o u g h o u t p u t s h o w s a n i n s i g n i fic a n t d e c r e a s e f o l l o w i n g p r i v a t i z a t i o n (c o n t i n u e d )Table I.IJCOMA 23,4288。
【高考真题】2024年高考英语真题试卷(新高考Ⅰ卷)
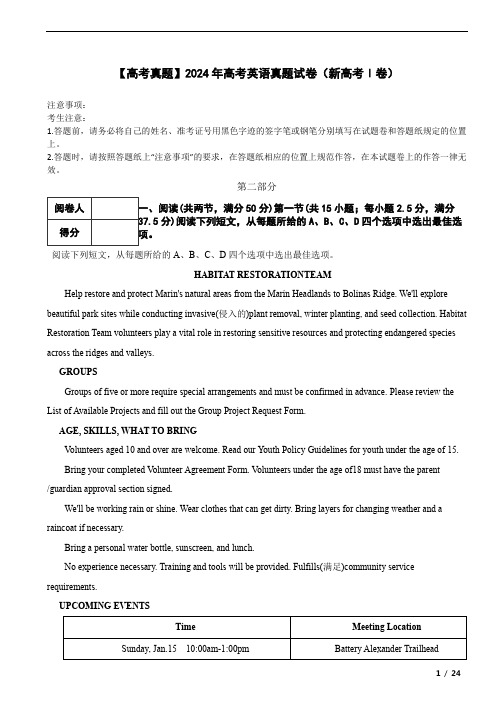
【高考真题】2024年高考英语真题试卷(新高考Ⅰ卷)注意事项:考生注意:1.答题前,请务必将自己的姓名、准考证号用黑色字迹的签字笔或钢笔分别填写在试题卷和答题纸规定的位置上。
2.答题时,请按照答题纸上“注意事项”的要求,在答题纸相应的位置上规范作答,在本试题卷上的作答一律无效。
第二部分(共两节,满分50分)第一节(共15小题;每小题2.5分,满分分)阅读下列短文,从每题所给的A、B、C、D四个选项中选出最佳选阅读下列短文,从每题所给的A、B、C、D四个选项中选出最佳选项。
HABITAT RESTORATIONTEAMHelp restore and protect Marin's natural areas from the Marin Headlands to Bolinas Ridge. We'll explore beautiful park sites while conducting invasive(侵入的)plant removal, winter planting, and seed collection. Habitat Restoration Team volunteers play a vital role in restoring sensitive resources and protecting endangered species across the ridges and valleys.GROUPSGroups of five or more require special arrangements and must be confirmed in advance. Please review the List of Available Projects and fill out the Group Project Request Form.AGE, SKILLS, WHAT TO BRINGV olunteers aged 10 and over are welcome. Read our Youth Policy Guidelines for youth under the age of 15.Bring your completed V olunteer Agreement Form. V olunteers under the age of18 must have the parent/guardian approval section signed.We'll be working rain or shine. Wear clothes that can get dirty. Bring layers for changing weather and a raincoat if necessary.Bring a personal water bottle, sunscreen, and lunch.No experience necessary. Training and tools will be provided. Fulfills(满足)community service requirements.UPCOMING EVENTS1.What is the aim of the Habitat Restoration Team?A.To discover mineral resources.B.To develop new wildlife parks.C.To protect the local ecosystemD.To conduct biological research.2.What is the lower age limit for joining the Habitat Restoration Team?A.5.B.10.C.15.D.18.3.What are the volunteers expected to do?A.Bring their own tools.B.Work even in bad weather.C.Wear a team uniform D.Do at least three projects.阅读下列短文,从每题所给的A、B、C、D四个选项中选出最佳选项。
【供应链金融风险研究国内外文献综述2300字】

供应链金融风险研究国内外文献综述1 国外研究现状从上世纪八十年代开始供应链金融的定义逐步被人们关注,国外涉及到供应链金融的思想观点与实践的应用相对成熟,对其定义的内涵外延比国内更为广泛,包括基于债券、股票等金融衍生商品这类动产质押业务风险研究、供应链金融的契约设计等方面。
M.Theodore,Paul D.Hutchison(2002)提出了供应链风险及其管理的相关概念,现金流管控是供应链金融领域十分关键的内容,供应链风控中的核心就是成功的现金流管控。
Cossin and Hricko(2003)基于企业违约概率与质押物价值,研究了具有价格风险商品作为质押的风险工具,质押物有助于进一步缓释银行信贷风险的作用。
Jimenez and Saurina(2004)研究了资产支持信贷中风险的影响因素包括质押物、银行(借款人)类型以及银行企业的关系等,合理的质押率有效缓释风险暴露,减少银行信贷损失。
Menkhoff,Neuberger and Suwanaporn(2006)的研究表明在不同国家,质押物对风险缓释的作用不同,质押物缓释风险的作用在发展中国家比发达国家显得更为重要。
Martin R(2007)系统分析了供应链资金流管控成本和危机、提升资金流效益的具体情况,指出根据供应链金融可让资金管理更加高效,但要严苛控制相应风险。
Lai and Debo(2009)对有资金局限的供应链存货中的相应问题进行了分析,通过库存契约设计能有效识别供应链上下游风险因子,从而提高供应链库存风险评价的准确性。
Hamadi和Matoussi(2010)根据剖析Logistic模型BP技术评估供应链金融风险的具体状况,表面三层BP神经网络模型在对上市房地产公司风险评价方面具有更好的准确性。
Qin and Ding(2011)分析了供应链金融领域里的风险变化现象,根据相应的风险迁徙模型,基于符合供应链金融条件,降低了借贷与信贷的风险。
directions of precision aerial application for site-specific crop management in the USA

ReviewCurrent status and future directions of precision aerial application forsite-specific crop management in the USAYubin Lan a,∗,Steven J.Thomson b,Yanbo Huang b,W.Clint Hoffmann a,Huihui Zhang ca USDA,ARS,Areawide Pest Management Research Unit(APMRU),2771F&B Road,College Station,TX77845,USAb United States Department of Agriculture(USDA),Agricultural Research Service(ARS),Crop Production Systems Research Unit(CPSRU),Stoneville,MS,USAc Department of Bio.and Agric.Engineering,Texas A&M University,College Station,TX,USAa r t i c l e i n f oArticle history:Received17November2009Received in revised form4June2010Accepted6July2010Keywords:Precision agricultureSite-specific managementRemote sensingGPS/GISVariable-rate aerial applicationa b s t r a c tThefirst variable-rate aerial application system was developed about a decade ago in the USA and sincethen,aerial application has benefitted from these technologies.Many areas of the United States relyon readily available agricultural airplanes or helicopters for pest management,and variable-rate aerialapplication provides a solution for applyingfield inputs such as cotton growth regulators,defoliants,andinsecticides.In the context of aerial application,variable-rate control can simply mean terminating sprayoverfield areas that do not require inputs,terminating spray near pre-defined buffer areas determined byGlobal Positioning,or applying multiple rates to meet the variable needs of the crop.Prescription mapsfor aerial application are developed using remote sensing,Global Positioning,and Geographic Informa-tion System technologies.Precision agriculture technology has the potential to benefit the agriculturalaviation industry by saving operators and farmers time and money.Published by Elsevier B.V. Contents1.Introduction (34)2.Current status (35)2.1.Remote sensing (35)2.1.1.ADC camera (35)2.1.2.Geospatial systems MS4100camera (35)2.2.Spatial statistics (36)2.3.Variable-rate aerial application (36)3.Future directions (37)3.1.Real time image processing (37)3.2.VRT system (37)3.3.Multisensor data fusion technology (37)4.Summary (37)References (37)1.Introduction1Aerial application,commonly called crop dusting,involvesspraying crops with fertilizers,pesticides,fungicides,and othercrop protection materials from agricultural aircraft.Precision∗Corresponding author.Tel.:+19792603759.E-mail address:n@(n).1Mention of trademark,vendor,or proprietary product does not constitute a guar-antee or warranty of the product by the USDA and does not imply its approval tothe exclusion of other products that may also be suitable.agriculture includes various technologies that allow agriculturalprofessionals to use information management tools to optimizeagriculture production.The new technologies allow aerial appli-cators to improve application accuracy and efficiency.It has beenabout a decade since development of thefirst variable-rate aerialapplication system.Many areas of the United States rely on readilyavailable agricultural airplanes or helicopters for pest management.Several types of precision agriculture technologies that assist aerialapplicators include global positioning system(GPS),geographicinformation system(GIS),soil mapping,yield monitoring,nutri-ent managementfield mapping,aerial photography,variable-ratecontrollers,and new types of nozzles such as pulse width mod-0168-1699/$–see front matter.Published by Elsevier B.V.doi:10.1016/pag.2010.07.001n et al./Computers and Electronics in Agriculture74 (2010) 34–3835ulation and variable-rate nozzles.Variable-rate aerial application provides a solution for applyingfield inputs such as cotton growth regulators,defoliants,and insecticides.Prescription maps for aerial application have been developed using remote sensing and GPS/GIS technologies.Precision agriculture technology has the potential to benefit the agricultural aviation industry by saving operators and farmers time and money.Airborne remote sensing may also benefit aerial applicators by creating a new revenue source because agricultural aircraft are eas-ier to schedule for frequent remote sensing missions that would coincide with aerial spray applications.An airborne remote sens-ing system produces precise images for spatial analyses of plant stress due to water or nutrient status in thefield,disease,and pest infestations.However,natural variations in biological characteris-tics,presence of disease and insects,and the interactions among these factors combine to influence crop quality and yield.Spatial statistics can often increase understanding of thefield and plant conditions.Through image processing,remote sensing data are converted into prescription maps for variable-rate aerial applica-tion.Therefore,remote sensing,spatial statistics,and variable-rate control technologies are all necessary ingredients for a precision aerial application system.This paper will discuss the current state of the above three areas,examine several current trends,and conclude with suggestions for future development.2.Current status2.1.Remote sensingWith an increasing population and a commensurate need for increasing agricultural production,there is an urgent need to improve management of agricultural resources.Satellite and aerial remote sensing technologies have advanced rapidly in recent years and have become effective tools for site-specific management in crop protection and production.Many satellite companies provide satellite imagery data at different spatial,spectral and temporal res-olutions for use in precision agriculture.Repeated satellite imagery allows for dynamic crop development monitoring and yield fore-casting.Earth-observing satellite systems,such as Landsat systems (NASA–National Aeronautics and Space Administration,Wash-ington,DC),have an advantage for large-scale analysis at regional levels but are limited in spatial resolution.High-resolution satellite systems,such as IKONOS(GeoEye,Dulles,Virginia)and QuickBird (DigitalGlobe,Longmont,Colorado),have been available in recent years,but scheduling these systems for appropriate bands,loca-tion offlight,proper altitude,and time of acquisition is difficult. Compared with satellite-based systems,airborne remote sensing systems offer aflexible,do-it-yourself platform for acquiring high quality and high-spatial resolution imagery when atmospheric, environmental and solar conditions are acceptable.One platform that carries remote sensing instruments is the air-borne remote sensing system.There are many usable platforms ranging from helicopters,Unmanned Airborne Vehicles(UAVs)to fixed-wing aircraft.Remote sensing instruments include digital cameras,CCD cameras,video cameras,hyperspectral cameras,mul-tispectral cameras and thermal-imaging cameras.Hyperspectral imaging is part of a class of techniques commonly referred to as spectral imaging or spectral analysis.Hyperspectral imaging and multispectral imaging are related but are usually distinguished by the number of spectral bands.Multispectral data contains from sev-eral to tens of spectral bands.Hyperspectral data contains dozens to hundreds of bands.However,hyperspectral imaging may be best defined by the manner in which the data are collected.Hyperspec-tral data cover a set of contiguous spectral bands(usually by one sensor).Multispectral data comprise a set of optimally chosen spec-tral bands that are typically not contiguous and can be collected from multiple e of aerial hyperspectral remote sensing in agriculture has been steadily increasing during the past decade (Goel et al.,2003;Yang et al.,2004,2009;Jang et al.,2005;Uno et al., 2005;Zarco-Tejada et al.,2005).Compared with hyperspectral sys-tems,multispectral systems are much less expensive and are less data-intensive.Airborne multispectral systems are cost-effective and a good source of crop,soil,weed or ground cover informa-tion for agricultural application and production(Moran et al.,1997; Senay et al.,1998;GopalaPillai and Tian,1999;Yang and Anderson, 1999;Yang and Everitt,2002;Pinter et al.,2003;Dobermann and Ping,2004;Huang et al.,2008;Inman et al.,2008;Yang et al.,2009; Lan et al.,2009;Zhang et al.,2009).In practical applications of airborne remote sensing,different types of multispectral imaging systems have been adopted based on economic and technical feasibilities.Here,we limit our discussion to an aircraft platform with two camera systems,which include a low-cost ADC(Agricultural Digital Camera)and a relatively expen-sive and high-performance multispectral camera.2.1.1.ADC cameraThe Tetracam ADC camera(Tetracam,Inc.,Gainesville,FL) is equipped with a 3.2-megapixel CMOS(Complementary Metal–Oxide–Semiconductor)sensor(2048×1536pixels)or a5.0-megapixel CMOS sensor(2560×1920pixels).It has green,red and near-infrared(NIR)sensitivity with bands approximately equal to Landsat Thematic Mapper2,Thematic Mapper3and Thematic Mapper4bands,which fall in the520–600nm,630–690nm,and 760–900nm wavelengths.Band information provide data needed for extraction of vegetation indices such as NDVI,SAVI,canopy segmentation and NIR/Green ratios.Standard global positioning system(GPS)data capture from an external receiver adds position-ing data to the images.The camera weighs640grams with8AA alkaline batteries.The3.2-megapixel ADCfitted with an8.5mm lens is able to achieve a0.5m/pixel ground resolution at1340m (4400ft)AGL(Above Ground Level).The current cost of the Tetra-cam ADC camera in2009is about$5000.Currently a proprietary software package,PixelWrench2,is used to work with the Tetracam ADC camera to manage and process ADC images.Another proprietary software package,SensorLink, provides a GPS waypoint triggering application enabling camera triggering at pre-defined waypoints.The ADC cameras are portable and can be used onfixed-wing aircraft such as the single-engine Cessna210(Cessna Aircraft Com-pany,Wichita,Kansas),the Air Tractor402B(Air Tractor,Inc.,Olney, Texas),and the UAV(Unmanned Autonomous Vehicle)helicopter such as Rotomotion SR20(Rotomotion,LLC,Charleston,South Car-olina)with limited payload.2.1.2.Geospatial systems MS4100cameraThe MS4100camera(Geospatial Systems,Inc.,West Henri-etta,New York)is a multispectral3-CCD(Charge-Coupled Device) Color/CIR(Color Infrared)digital camera.It provides a digital imag-ing quality with a1920(horizontal)×1080(vertical)pixel array per sensor and60◦widefield of view with a14-mm,f/2.8lens. Color-separating optics work in concert with a large-format pro-gressive scan CCD sensors to maximize resolution,dynamic range, andfield of view.The MS4100camera is available in two spec-tral configurations:RGB(Red Green Blue)for high quality color imaging and CIR for multispectral applications.The camera images the four spectral bands from400to1000nm,and acquires sepa-rate red(660–40nm bandwidth),green(540–40nm bandwidth), and blue(460–45nm bandwidth)image planes.The camera pro-vides composite color images and individual color plane images.It is also able to acquire and provide composite and individual plane images from red,green,and NIR(800–65nm bandwidth)bandsn et al./Computers and Electronics in Agriculture74 (2010) 34–38that approximate Landsat satellite thematic mapper bands(NASA, Washington,DC;USGS,Reston,VA).The MS4100is able to fur-ther provide RGB and CIR images concurrently and has the option for other custom spectral configurations.When running the RGB or CIR configuration individually,a base configuration will sup-port any three-tap configuration running at8bits per color plane (i.e.24-bit RGB).Adding a fourth8-bit tap or outputting10bits per color plane requires an additional port with a second cable. The MS4100camera configures the digital output of image data with CameraLink standard or parallel digital data in either EIA-644or RS-422differential format.The camera works with the NI IMAQ PCI-1424/1428framegrabber(National Instruments,Austin, Texas).With the software DTControl-FG(Geospatial Systems,Inc) and the CameraLink configuration,the camera system acquires images from the framegrabber directly from within the DTControl program.The current cost of the MS4100camera is about$20,000.In practical use of the camera on aircraft,operation of the camera would require a technician to control imaging and any ancillary control functions.This is somewhat impractical for small agricultural airplanes as the pilot cannot operate the camera effec-tively andfly the airplane simultaneously.Control automation is necessary of the multispectral camera is necessary in order to reduce labor required and maintain consistency of camera oper-ation.Based on the needs in agricultural research and applications, the TerraHawk camera control system(TerraVerde Technologies, Inc.,Stillwater,Oklahoma)is commercially available and is being integrated to automate the operation of the MS4100camera with: (1)Dragonfly TM software to control the operation of the camera, especially to trigger the camera based on thefield shapefile polygon with GPS receiver;(2)a gimbal controller to stabilize and control the camera for roll,pitch,and yaw aircraft rotations duringflight.Huang et al.(2009)concluded that the Tetracam camera in its present state is more suitable for slower moving platforms that canfly close to the ground,such as the UAV;the MS4100imaging system worked very well being mounted on an agricultural aircraft like the Air Tractor402B.Huang et al.(2008)and Lan et al.(2009) have demonstrated the capability and performance of the MS4100 airborne imaging system for crop pest management.Such multispectral instruments typically capture imagery that can be related to relative radiance in the visible and near infrared regions.However,all remote sensing measurements can be affected by variable ground conditions,such as plant architecture,canopy characteristics,crop row orientation and coverage,and background soil properties.All of these ground conditions can contribute towards spatial variability within thefield and betweenfields. Sometimes aerial remotely sensed data alone cannot capture all the information required.Data from imagery,ground-truth mea-surements,and spatial analysis together allow for a more complete understanding of afield’s spatial complexity.2.2.Spatial statisticsThe techniques of spatial statistics werefirst developed and for-malized in the1950s.Recently,with the development of GIS,spatial statistics have drawn considerable attention and have been widely applied in spatial data modeling and analysis for natural sciences such as geophysics,biology,epidemiology and agriculture.There have been numerous studies demonstrating the benefits of spatial analysis to agricultural management.Stein et al.(1997)empha-sized the use of spatial analysis in reducing production risks and in formulating variable resource allocation.In a case study to model spatially varied yield monitor data for corn nitrogen response, Bongiovanni and Lowenberg-DeBoer(2000,2002)determined that spatial regression analysis of yield monitor data could be used to estimate the site-specific crop nitrogen response needed tofine tune variable-rate fertilization strategies for maize and mbert and Lowenberg-DeBoer(2003)demonstrated that the spa-tial econometric,geostatistical approach and spatial trend analysis offered stronger statistical evidence of spatial heterogeneity of nitrogen response than the ordinary least squares or nearest neigh-bor analysis.Yao et al.(2003)investigated soil nutrient mapping by a co-located co-kriging estimator using soil sampling data and aerial hyperspectral image.Misaghi et al.(2004)developed a model to predict strawberry yield using aerial images,soil parameters, and plant parameters.Bajwa and Mozaffari(2007)tested various spatial models in analysis of the variations in GNDVI(Green Nor-malized Difference Vegetative Index),a vegetative index derived from aerial remote sensing data in the Visible and NIR(VNIR) regions,in response to nitrogen treatments and petiole nitrate content.Overall,remote sensing imagery data and spatial statistical methods can provide valuable and complete information in the site-specific management.This information can be used to produce an application map and support aerial variable-rate application.2.3.Variable-rate aerial applicationIf agricultural aircraft offer a different view of remote sens-ing,then precision agriculture takes aerial application to new heights.Several types of precision agriculture technologies that assist aerial applicators include GPS,GIS,soil sampling,yield mon-itoring,nutrient managementfield mapping,aerial photography, and variable-rate application technology.Moran et al.(1997)presented an infrastructure that holds promise for incorporating aircraft remote sensing technology into precision crop management.In thefirst stage,images are acquired and processed to values of surface reflectance and registered to field coordinates.In the second stage,these images are converted to physical crop and soil information.In the third stage,this dis-tributed information about crop and soil conditions is interpreted to produce maps of management units for variable-rate material application.Variable-rate technology is focused on applying pes-ticides,herbicides,soil amendments,plant harvesting aids and fertilizers at various rates and at specific locations.Aerial appli-cation maps are created with variable rates customarily in GIS and then converted to prescription format by software supplied by the airplane’s guidance system manufacturer.The variable-rate system uses preloaded“prescription maps”to changeflow rates through thefield depending on where the application is needed most,least, or where it should not be used at all.There are two components in a variable-rate aerial application system:GPS and Variable Flow control system.In today’s market, one manufacturer,Hemisphere GPS of Calgary,AB,Canada,has developed the Satloc M3and acquired the Del Norte Flying Flagman. For liquid applications,the Aerial Ace and IntelliFlow(compatible with both Hemisphere Air guidance systems)automatically apply the proper rate of spray at the proper time using a variable or con-stant rate.Theflow system varies the setting of aflow control valve by responding to changes in ground speed.The Fire/Dry Gate Con-troller(FDGC)is also a new technology that has been added to the list of products to interface with both the M3and Flying Flagman. Another new technology that Hemisphere GPS has worked on is the Crescent Receiver.The new receiver gives20Hz capability,which means that a pilots’Lightbar will update20times/pared with most technologies that offer5or10Hz updating,20Hz updating has the potential for better application accuracy because of the smaller time window within which to make rate changes.There are also other companies that offer similar technologies.AG-NAV Inc.’s popular technology is the AG-NAV2,which provides the pilot with swath,directional guidance and other navigation information. ADAPCO,Inc.,Sanford,FL offers the Wingman TM GX and NextStar TM flow control systems technologies to aerial applicators.Wingmann et al./Computers and Electronics in Agriculture74 (2010) 34–3837GX is a highly advanced precision guidance and recording system that was developed to improve aerial pesticide application accuracy and efficiency by processing real time meteorology onboard the air-craft and providing instantaneous optimization offset distance.The AutoCal IIflow controller(Houma Avionics,Houma,LA)can inter-face with all swath guidance systems.The AutoCal II controls boom flow rate by controlling the spray pump output.Only recently have agricultural aircraft been equipped to imple-ment variable-rate application to match site-specific needs of the crop.Variable-rate aerial application systems have seen limited use only within the past six years or so,and very little infor-mation has been presented on the accuracy of these systems for placement of chemical and response of these systems to changing rate requirements.In addition to variable-rate application,aerial flow control systems must adjustflow properly to accommodate changes in ground speed.Smith and Thomson(2005)evaluated position latency of the GPS receiver used in the Satloc Airstar M3 swath guidance against a known ground position.Theflow con-trol portion of the system has been tested for positioning accuracy (Thomson et al.,2009)and improved by comparing measuredflow rates and step-change responses to desiredflow rate response curves and modifying the control program accordingly(Thomson et al.,2010).3.Future directionsThe USDA,ARS conducts research involving GPS-based real time guidance and GIS systems for agricultural aircraft to conserve mate-rial and provide a commensurate reduction in deleterious pesticide loading to the environment.As precision agriculture continues to grow,more operators are becoming familiar with these tech-nologies because the demand is growing from farmers.Research continues to be conducted to enhance these technologies and create new technologies for accuracy and efficiency.3.1.Real time image processingReal time processing of imagery is needed to bridge the gap between remote sensing and variable-rate aerial application. Data analysis and interpretation is one of the most important parts of precision aerial application.Whether collected from air-borne images,ground-based sensors and instrumentation systems, human observations,or laboratory samples,data must be analyzed properly to understand cause-and-effect relationships.To develop appropriate prescription maps for variable-rate aerial application, thefindings from multispectral aerial images in near real time has been a challenge.The ultimate goal is to develop a user-friendly image processing software system,aiming to analyze the data rapidly from aerial images so that variable-rate spraying can occur immediately after data acquisition.3.2.VRT systemThere is limited application for turn-key commercial VRT devices due to their perceived high cost and operational difficulty. An economical and user-oriented system is needed that could pro-cess spatially distributed information,and apply only the necessary amounts of pesticide to the infested area efficiently and to minimize environmental damage.Additionally,nozzles are designed to pro-duce optimal droplet size spectra for mitigation of off-target drift and to provide maximum application efficacy.These desired size ranges require the nozzles to operate within proper boundaries of their design pressure.Variable rates called for by the aerial appli-cation system might operate these nozzles outside their optimal pressure ranges making their valid use questionable if a wide range offlowrates is required.This would not be a problem for“on-off”variable control.3.3.Multisensor data fusion technologyA key step in successful precision system development is cre-ation of accurate prescription maps for aerial application.Creation of these maps can be assisted by multisensor,multispectral,mul-titemporal and even multi-resolution data fusion utilizing GIS techniques.The data fusion will be based on new methods for the fusion of heterogeneous data:numerical or measurable(radiomet-ric,multispectral,and spatial information)and symbolic(thematic, human interpretation and ground truth)data.The multisensor data fusion scheme needs to be fully integrated into the system through GIS.4.SummaryPrecision aerial application will result in more judicious use of pesticides,thereby satisfying environmentalists,legislators and rge farms typical of the US and parts of China will benefit greatly though the use of these technologies.Small farmers could use precision agriculture technologies by a cooperative system to urgently deal with some areawide pest management issues.Pre-cision aerial application will allow for the targeting of inputs to specific areas offields,enabling farmers to remain successful in an increasingly competitive industry.ReferencesBajwa,S.G.,Mozaffari,M.,2007.Effect of N availability on vegetative index of cotton canopy:a spatial regression approach.Trans.ASABE50(5),1883–1892. Bongiovanni,R.G.,Lowenberg-DeBoer,J.M.,2000.Nitrogen Management in Corn Using Site-Specific Crop Response Estimates from a Spatial Regression Model.In:Proceedings of the5th International Conference on Precision Agriculture, Bloomington,MN.Center for Precision Agriculture,University of Minnesota,St.Paul,MN,16–19July2000.Bongiovanni,R.G.,Lowenberg-DeBoer,J.M.,2002.Economic of nitrogen response variability over space and time:results from the1999–2001field trials in Argentina.In:Proceedings of the6th International Conference on Precision Agriculture,Bloomington,MN,ASA-CSSA-SSSA,Madison,WI,14–17July,2002. Dobermann,A.,Ping,J.L.,2004.Geostatistical integration of yield monitor data and remote sensing improves yield maps.Agron.J.96(1),285–297.Goel,P.K.,Prasher,S.O.,Landry,J.A.,Patel,R.M.,Viau,A.A.,Miller,J.R.,2003.Estima-tion of crop biophysical parameters through airborne andfield hyperspectral remote sensing.Trans.ASAE46(4),1235–1246.GopalaPillai,S.,Tian,L.,1999.In-field variability detection and spatial yield modeling for corn using digital aerial imaging.Trans.ASAE42(6),1911–1920.Huang,Y.,Lan,Y.,Hoffmann,W.C.,e of airborne multi-spectral imagery in pest management systems.Agr.Eng.Int.X,Manuscript IT07010.Huang,Y.,Thomson,S.J.,Lan,Y.,Maas,S.J.,2009.Multispectral imaging system for Airborne remote sensing to support site-specific agricultural management.In:Proceedings of the3rd Asian Conference on Precision Agriculture(ACPA), Beijing,China,14–17October,2009.Inman,D.,Khosla,R.,Reich,R.,Westfall,D.G.,2008.Normalized difference vegetation index and soil color-based management zones in irrigated maize.Agron.J.100(1),60–66.Jang,G.S.,Sudduth,K.A.,Hong,S.Y.,Kitchen,N.R.,Palm,H.L.,2005.Relating image-derived vegetation indices to crop yield.In:Proceedings of the20th Biennial Workshop on Aerial Photography,Videography,and High-Resolution Digital Imagery for Resource Assessment,CD-ROM.Lambert,D.M.,Lowenberg-DeBoer,J.M.,2003.Spatial regression models for yield monitor data:a case study from Argentina.In:American Agricultural Economics Association Annual Meeting,Montreal,Canada,27–30July,2003.Lan,L.,Huang,Y.,Martin,D.E.,Hoffmann,W.C.,2009.Development of an airborne remote sensing system for crop pest management:system integration and ver-ification.Trans.ASABE25(4),607–615.Misaghi,F.,Dayyanidardashti,S.,Mohammadi,K.,Ehsani,M.R.,2004.Application of Artificial Neural Network and Geostatistical Methods in Analyzing Strawberry Yield Data.ASAE Paper Number:041147.ASAE,St.Joseph,MI.Moran,M.S.,Inoue,Y.,Barnes,E.M.,1997.Opportunities and limitations for image-based remote sensing in precision crop management.Remote Sens.Environ.61(3),319–346.Pinter Jr.,P.J.,Hatfield,J.L.,Schepers,J.S.,Barnes,E.M.,Moran,M.S.,Daughtry,C.S.T., Upchurch,D.R.,2003.Remote sensing for crop management.Photogram.Eng.Remote Sens.69(6),647–664.n et al./Computers and Electronics in Agriculture74 (2010) 34–38Senay,G.B.,Ward,A.D.,Lyon,J.G.,Fausey,N.R.,Nokes,S.E.,1998.Manipulation of high spatial resolution aircraft remote sensing data for use in site-specific farming.Trans.ASAE41(2),489–495.Smith,L.A.,Thomson,S.J.,2005.GPS position latency determination and ground speed calibration for the Satloc Airstar M3.Appl.Eng.Agric.21(5),769–776. Stein,A.,Brouwer,J.,Bouma,J.,1997.Methods for comparing spatial variability patterns of millet yield and soil data.Soil Sci.Soc.Am.J.61,861–870. Thomson,S.J.,Smith,L.A.,Hanks,J.E.,2009.Evaluation of application accuracy and performance of a hydraulically operated variable-rate aerial application system.Trans.ASABE52(3),715–722.Thomson,S.J.,Huang,Y.,Hanks,J.E.,Martin,D.E.,Smith,L.A.,2010.Improvingflow response of a variable-rate aerial application system by interactive refinement.Comput.Electron.Agr.73(1),99–104.Uno,Y.,Prasher,S.O.,Lacroix,R.,Goel,R.K.,Karimi,Y.,Viau,A.,Patel,R.M.,2005.Artificial neural networks to predict corn yield from compact airborne spectro-graphic imager put.Electron.Agr.47(2),149–161.Yang,C.,Anderson,G.L.,1999.Airborne videography to identify spatial plant growth variability for grain sorghum.Precision Agric.1(1),67–79.Yang,C.,Everitt,J.H.,2002.Relationships between yield monitor data and airborne multispectral multidate digital imagery for grain sorghum.Precision Agric.3(4), 373–388.Yang,C.,Everitt,J.H.,Bradford,J.M.,Murden,D.,2004.Airborne hyperspectral imagery and yield monitor data for mapping cotton yield variability.Precision Agric.5(5),445–461.Yang,C.,Everitt,J.H.,Bradford,J.M.,Murden,D.,parison of airborne mul-tispectral and hyperspectral imagery for estimating grain sorghum yield.Trans.ASABE52(2),641–649.Yao,H.,Tian,L.,Wang,G.,Colonna,I.A.,2003.Soil Nutrient Mapping Using Aerial Hypersepctral Imaging and Soil Sampling Data–A Geostatistical Approach.ASAE Paper Number:031046.ASAE,St.Joseph,MI.Zarco-Tejada,P.J.,Ustin,S.L.,Whiting,M.L.,2005.Temporal and spatial relationships between within-field yield variability in cotton and high-spatial hyperspectral remote sensing imagery.Agron.J.97(3),641–653.Zhang,H.,Lan,Y.,Lacey,R.,Hoffmann,W.C.,Huang,Y.,2009.Analysis of vegetation indices derived from aerial multispectral and ground hyperspectral data.Int.J.Agric.Biol.Eng.2(3),33–40.。
外文文献及翻译
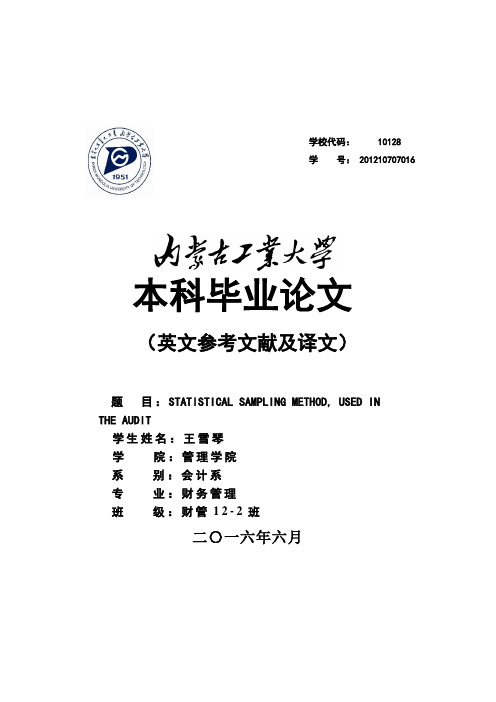
((英文参考文献及译文)二〇一六年六月本科毕业论文 题 目:STATISTICAL SAMPLING METHOD, USED INTHE AUDIT学生姓名:王雪琴学 院:管理学院系 别:会计系专 业:财务管理班 级:财管12-2班 学校代码: 10128 学 号: 201210707016Statistics and AuditRomanian Statistical Review nr. 5 / 2010STATISTICAL SAMPLING METHOD, USED IN THE AUDIT - views, recommendations, fi ndingsPhD Candidate Gabriela-Felicia UNGUREANUAbstractThe rapid increase in the size of U.S. companies from the earlytwentieth century created the need for audit procedures based on the selectionof a part of the total population audited to obtain reliable audit evidence, tocharacterize the entire population consists of account balances or classes oftransactions. Sampling is not used only in audit – is used in sampling surveys,market analysis and medical research in which someone wants to reach aconclusion about a large number of data by examining only a part of thesedata. The difference is the “population” from which the sample is selected, iethat set of data which is intended to draw a conclusion. Audit sampling appliesonly to certain types of audit procedures.Key words: sampling, sample risk, population, sampling unit, tests ofcontrols, substantive procedures.Statistical samplingCommittee statistical sampling of American Institute of CertifiedPublic Accountants of (AICPA) issued in 1962 a special report, titled“Statistical sampling and independent auditors’ which allowed the use ofstatistical sampling method, in accordance with Generally Accepted AuditingStandards (GAAS). During 1962-1974, the AICPA published a series of paperson statistical sampling, “Auditor’s Approach to Statistical Sampling”, foruse in continuing professional education of accountants. During 1962-1974,the AICPA published a series of papers on statistical sampling, “Auditor’sApproach to Statistical Sampling”, for use in continuing professional educationof accountants. In 1981, AICPA issued the professional standard, “AuditSampling”, which provides general guidelines for both sampling methods,statistical and non-statistical.Earlier audits included checks of all transactions in the period coveredby the audited financial statements. At that time, the literature has not givenparticular attention to this subject. Only in 1971, an audit procedures programprinted in the “Federal Reserve Bulletin (Federal Bulletin Stocks)” includedseveral references to sampling such as selecting the “few items” of inventory.Statistics and Audit The program was developed by a special committee, which later became the AICPA, that of Certified Public Accountants American Institute.In the first decades of last century, the auditors often applied sampling, but sample size was not in related to the efficiency of internal control of the entity. In 1955, American Institute of Accountants has published a study case of extending the audit sampling, summarizing audit program developed by certified public accountants, to show why sampling is necessary to extend the audit. The study was important because is one of the leading journal on sampling which recognize a relationship of dependency between detail and reliability testing of internal control.In 1964, the AICPA’s Auditing Standards Board has issued a report entitled “The relationship between statistical sampling and Generally Accepted Auditing Standards (GAAS)” which illustrated the relationship between the accuracy and reliability in sampling and provisions of GAAS.In 1978, the AICPA published the work of Donald M. Roberts,“Statistical Auditing”which explains the underlying theory of statistical sampling in auditing.In 1981, AICPA issued the professional standard, named “Audit Sampling”, which provides guidelines for both sampling methods, statistical and non-statistical.An auditor does not rely solely on the results of a single procedure to reach a conclusion on an account balance, class of transactions or operational effectiveness of the controls. Rather, the audit findings are based on combined evidence from several sources, as a consequence of a number of different audit procedures. When an auditor selects a sample of a population, his objective is to obtain a representative sample, ie sample whose characteristics are identical with the population’s characteristics. This means that selected items are identical with those remaining outside the sample.In practice, auditors do not know for sure if a sample is representative, even after completion the test, but they “may increase the probability that a sample is representative by accuracy of activities made related to design, sample selection and evaluation” [1]. Lack of specificity of the sample results may be given by observation errors and sampling errors. Risks to produce these errors can be controlled.Observation error (risk of observation) appears when the audit test did not identify existing deviations in the sample or using an inadequate audit technique or by negligence of the auditor.Sampling error (sampling risk) is an inherent characteristic of the survey, which results from the fact that they tested only a fraction of the total population. Sampling error occurs due to the fact that it is possible for Revista Română de Statistică nr. 5 / 2010Statistics and Auditthe auditor to reach a conclusion, based on a sample that is different from the conclusion which would be reached if the entire population would have been subject to audit procedures identical. Sampling risk can be reduced by adjusting the sample size, depending on the size and population characteristics and using an appropriate method of selection. Increasing sample size will reduce the risk of sampling; a sample of the all population will present a null risk of sampling.Audit Sampling is a method of testing for gather sufficient and appropriate audit evidence, for the purposes of audit. The auditor may decide to apply audit sampling on an account balance or class of transactions. Sampling audit includes audit procedures to less than 100% of the items within an account balance or class of transactions, so all the sample able to be selected. Auditor is required to determine appropriate ways of selecting items for testing. Audit sampling can be used as a statistical approach and a non- statistical.Statistical sampling is a method by which the sample is made so that each unit consists of the total population has an equal probability of being included in the sample, method of sample selection is random, allowed to assess the results based on probability theory and risk quantification of sampling. Choosing the appropriate population make that auditor’ findings can be extended to the entire population.Non-statistical sampling is a method of sampling, when the auditor uses professional judgment to select elements of a sample. Since the purpose of sampling is to draw conclusions about the entire population, the auditor should select a representative sample by choosing sample units which have characteristics typical of that population. Results will not extrapolate the entire population as the sample selected is representative.Audit tests can be applied on the all elements of the population, where is a small population or on an unrepresentative sample, where the auditor knows the particularities of the population to be tested and is able to identify a small number of items of interest to audit. If the sample has not similar characteristics for the elements of the entire population, the errors found in the tested sample can not extrapolate.Decision of statistical or non-statistical approach depends on the auditor’s professional judgment which seeking sufficient appropriate audits evidence on which to completion its findings about the audit opinion.As a statistical sampling method refer to the random selection that any possible combination of elements of the community is equally likely to enter the sample. Simple random sampling is used when stratification was not to audit. Using random selection involves using random numbers generated byRomanian Statistical Review nr. 5 / 2010Statistics and Audit a computer. After selecting a random starting point, the auditor found the first random number that falls within the test document numbers. Only when the approach has the characteristics of statistical sampling, statistical assessments of risk are valid sampling.In another variant of the sampling probability, namely the systematic selection (also called random mechanical) elements naturally succeed in office space or time; the auditor has a preliminary listing of the population and made the decision on sample size. “The auditor calculated a counting step, and selects the sample element method based on step size. Step counting is determined by dividing the volume of the community to sample the number of units desired. Advantages of systematic screening are its usability. In most cases, a systematic sample can be extracted quickly and method automatically arranges numbers in successive series.”[2].Selection by probability proportional to size - is a method which emphasizes those population units’recorded higher values. The sample is constituted so that the probability of selecting any given element of the population is equal to the recorded value of the item;Stratifi ed selection - is a method of emphasis of units with higher values and is registered in the stratification of the population in subpopulations. Stratification provides a complete picture of the auditor, when population (data table to be analyzed) is not homogeneous. In this case, the auditor stratifies a population by dividing them into distinct subpopulations, which have common characteristics, pre-defined. “The objective of stratification is to reduce the variability of elements in each layer and therefore allow a reduction in sample size without a proportionate increase in the risk of sampling.” [3] If population stratification is done properly, the amount of sample size to come layers will be less than the sample size that would be obtained at the same level of risk given sample with a sample extracted from the entire population. Audit results applied to a layer can be designed only on items that are part of that layer.I appreciated as useful some views on non-statistical sampling methods, which implies that guided the selection of the sample selecting each element according to certain criteria determined by the auditor. The method is subjective; because the auditor selects intentionally items containing set features him.The selection of the series is done by selecting multiple elements series (successive). Using sampling the series is recommended only if a reasonable number of sets used. Using just a few series there is a risk that the sample is not representative. This type of sampling can be used in addition to other samples, where there is a high probability of occurrence of errors. At the arbitrary selection, no items are selected preferably from the auditor, Revista Română de Statistică nr. 5 / 2010Statistics and Auditthat regardless of size or source or characteristics. Is not the recommended method, because is not objective.That sampling is based on the auditor’s professional judgment, which may decide which items can be part or not sampled. Because is not a statistical method, it can not calculate the standard error. Although the sample structure can be constructed to reproduce the population, there is no guarantee that the sample is representative. If omitted a feature that would be relevant in a particular situation, the sample is not representative.Sampling applies when the auditor plans to make conclusions about population, based on a selection. The auditor considers the audit program and determines audit procedures which may apply random research. Sampling is used by auditors an internal control systems testing, and substantive testing of operations. The general objectives of tests of control system and operations substantive tests are to verify the application of pre-defined control procedures, and to determine whether operations contain material errors.Control tests are intended to provide evidence of operational efficiency and controls design or operation of a control system to prevent or detect material misstatements in financial statements. Control tests are necessary if the auditor plans to assess control risk for assertions of management.Controls are generally expected to be similarly applied to all transactions covered by the records, regardless of transaction value. Therefore, if the auditor uses sampling, it is not advisable to select only high value transactions. Samples must be chosen so as to be representative population sample.An auditor must be aware that an entity may change a special control during the course of the audit. If the control is replaced by another, which is designed to achieve the same specific objective, the auditor must decide whether to design a sample of all transactions made during or just a sample of transactions controlled again. Appropriate decision depends on the overall objective of the audit test.Verification of internal control system of an entity is intended to provide guidance on the identification of relevant controls and design evaluation tests of controls.Other tests:In testing internal control system and testing operations, audit sample is used to estimate the proportion of elements of a population containing a characteristic or attribute analysis. This proportion is called the frequency of occurrence or percentage of deviation and is equal to the ratio of elements containing attribute specific and total number of population elements. WeightRomanian Statistical Review nr. 5 / 2010Statistics and Audit deviations in a sample are determined to calculate an estimate of the proportion of the total population deviations.Risk associated with sampling - refers to a sample selection which can not be representative of the population tested. In other words, the sample itself may contain material errors or deviations from the line. However, issuing a conclusion based on a sample may be different from the conclusion which would be reached if the entire population would be subject to audit.Types of risk associated with sampling:Controls are more effective than they actually are or that there are not significant errors when they exist - which means an inappropriate audit opinion. Controls are less effective than they actually are that there are significant errors when in fact they are not - this calls for additional activities to establish that initial conclusions were incorrect.Attributes testing - the auditor should be defining the characteristics to test and conditions for misconduct. Attributes testing will make when required objective statistical projections on various characteristics of the population. The auditor may decide to select items from a population based on its knowledge about the entity and its environment control based on risk analysis and the specific characteristics of the population to be tested.Population is the mass of data on which the auditor wishes to generalize the findings obtained on a sample. Population will be defined compliance audit objectives and will be complete and consistent, because results of the sample can be designed only for the population from which the sample was selected.Sampling unit - a unit of sampling may be, for example, an invoice, an entry or a line item. Each sample unit is an element of the population. The auditor will define the sampling unit based on its compliance with the objectives of audit tests.Sample size - to determine the sample size should be considered whether sampling risk is reduced to an acceptable minimum level. Sample size is affected by the risk associated with sampling that the auditor is willing to accept it. The risk that the auditor is willing to accept lower, the sample will be higher.Error - for detailed testing, the auditor should project monetary errors found in the sample population and should take into account the projected error on the specific objective of the audit and other audit areas. The auditor projects the total error on the population to get a broad perspective on the size of the error and comparing it with tolerable error.For detailed testing, tolerable error is tolerable and misrepresentations Revista Română de Statistică nr. 5 / 2010Statistics and Auditwill be a value less than or equal to materiality used by the auditor for the individual classes of transactions or balances audited. If a class of transactions or account balances has been divided into layers error is designed separately for each layer. Design errors and inconsistent errors for each stratum are then combined when considering the possible effect on the total classes of transactions and account balances.Evaluation of sample results - the auditor should evaluate the sample results to determine whether assessing relevant characteristics of the population is confirmed or needs to be revised.When testing controls, an unexpectedly high rate of sample error may lead to an increase in the risk assessment of significant misrepresentation unless it obtained additional audit evidence to support the initial assessment. For control tests, an error is a deviation from the performance of control procedures prescribed. The auditor should obtain evidence about the nature and extent of any significant changes in internal control system, including the staff establishment.If significant changes occur, the auditor should review the understanding of internal control environment and consider testing the controls changed. Alternatively, the auditor may consider performing substantive analytical procedures or tests of details covering the audit period.In some cases, the auditor might not need to wait until the end audit to form a conclusion about the effectiveness of operational control, to support the control risk assessment. In this case, the auditor might decide to modify the planned substantive tests accordingly.If testing details, an unexpectedly large amount of error in a sample may cause the auditor to believe that a class of transactions or account balances is given significantly wrong in the absence of additional audit evidence to show that there are not material misrepresentations.When the best estimate of error is very close to the tolerable error, the auditor recognizes the risk that another sample have different best estimate that could exceed the tolerable error.ConclusionsFollowing analysis of sampling methods conclude that all methods have advantages and disadvantages. But the auditor is important in choosing the sampling method is based on professional judgment and take into account the cost / benefit ratio. Thus, if a sampling method proves to be costly auditor should seek the most efficient method in view of the main and specific objectives of the audit.Romanian Statistical Review nr. 5 / 2010Statistics and Audit The auditor should evaluate the sample results to determine whether the preliminary assessment of relevant characteristics of the population must be confirmed or revised. If the evaluation sample results indicate that the relevant characteristics of the population needs assessment review, the auditor may: require management to investigate identified errors and likelihood of future errors and make necessary adjustments to change the nature, timing and extent of further procedures to take into account the effect on the audit report.Selective bibliography:[1] Law no. 672/2002 updated, on public internal audit[2] Arens, A şi Loebbecke J - Controve …Audit– An integrate approach”, 8th edition, Arc Publishing House[3] ISA 530 - Financial Audit 2008 - International Standards on Auditing, IRECSON Publishing House, 2009- Dictionary of macroeconomics, Ed C.H. Beck, Bucharest, 2008Revista Română de Statistică nr. 5 / 2010Statistics and Audit摘要美国公司的规模迅速增加,从第二十世纪初创造了必要的审计程序,根据选定的部分总人口的审计,以获得可靠的审计证据,以描述整个人口组成的帐户余额或类别的交易。
2024年新课标Ⅰ卷英语真题(含听力)(原卷版)
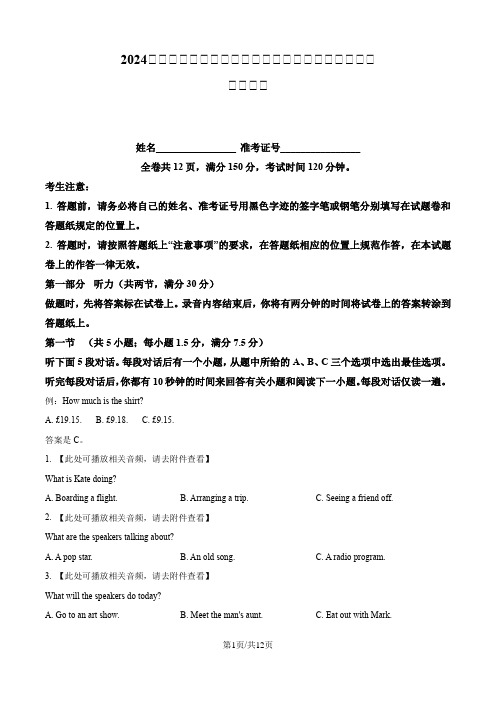
11 How did Jack go to school when he was a child?
A.By bike.B.On foot.C.By bus.
12.What is Jack's attitude toward parents driving their kids to school?
We'll be working rain or shine. Wear clothes hat can get dirty. Bring layers for changing weather and a raincoat if necessary.
Bring a personal water bottle, sunscreen, and lunch.
B.They can be used in cooking.
C.They bear a lot of fruit soon.
16.What is difficult for Marie to grow?
A.Herbs.B.Carrots.C.Pears.
17.What is Marie's advice to those interested in kitchen gardening?
Battery Alexander Trailhead
Sunday, Jan. 22 10:00 am — 2:30 pm
Stinson Beach Parking Lot
Sunday, Jan. 29 9:30 am — 2:30 pm
Coyote Ridge Trailhead
21.What is the aim of the Habitat Restoration Team?
利用Fishbein模型进行市场定位分析

利用Fishbein模型进行市场定位分析市场定位是指在竞争激烈的市场环境中,通过确定目标市场和市场定位战略,将产品或服务与目标市场需求相匹配,以达到竞争优势和市场份额的目标。
Fishbein模型是用于市场定位分析的一种重要工具,它是由美国社会心理学家Martin Fishbein于1967年推出的,主要用于分析消费者对产品或服务的态度形成和购买决策过程。
Fishbein模型是基于消费者的评价和态度,通过对产品和服务的不同属性进行评估,从而确定其满意度和购买意愿。
该模型主要包括两个关键要素:消费者对产品或服务的认知和他们对这些认知的态度。
首先,消费者对产品或服务的认知是指他们对产品或服务的特征、功能和性能的了解程度。
在市场定位分析中,通过调查问卷、访谈或市场调研等方式,可以获取消费者对产品或服务的认知情况。
在Fishbein模型中,这些认知可以从消费者对产品或服务的不同属性进行评价,得出一个对产品或服务的整体认知程度。
其次,消费者对这些认知的态度是指他们对产品或服务的评价和喜好程度。
在Fishbein模型中,消费者对不同属性的态度通过赋予不同的权重来反映。
这些权重可以通过调查问卷中的评分、排名或选择题来获取。
通过将消费者对不同属性的态度与其对产品或服务的认知进行加权得分,可以计算出消费者对产品或服务的整体态度。
基于Fishbein模型的市场定位分析可以从两个层面进行:产品或服务定位和目标市场定位。
在产品或服务定位方面,可以通过评估产品或服务的不同属性,确定其在市场中的特点和竞争优势。
通过比较与竞争对手的差异,可以确定产品或服务的定位策略,如高性能、低价格、豪华体验等。
在目标市场定位方面,可以通过分析不同群体的消费者对产品或服务的认知和态度,了解其需求和偏好。
通过将消费者群体分类,并针对不同群体的特点和需求,开发相应的市场定位策略。
例如,对于年轻消费者,可以强调产品或服务的创新和时尚性;而对于中年消费者,可以强调产品或服务的可靠性和性能。
Measuring relatvie development level of stock market

Measuring relative development level of stock markets:Capacity and effort of countriesNihal Bayraktar *Penn State University e Harrisburg,School of Business Administration,777W.Harrisburg Pike,Middletown,PA 17057,USAAvailable online 21February 2014AbstractOne of the important determinants of economic development is the existence of an effective financial system.Despite widespread need for financial services,the range and depth of financial markets,including stock markets,vary significantly across countries.One question in the literature is how to measure the development level of stock markets across countries for appropriate policy formations.This paper suggests capacity and effort measures of stock market capitalization,which consider country characteristics,as diagnostic tool to assess the gap between the actual level of stock market capitalization and the capacity of countries.It involves a panel study of 104developing and developed countries for the period of 1990e 2012.The analysis can deliver broad guidance for public reforms in countries with various levels of market capitali-zation.Cross-country comparisons with measures considering country characteristics can give a better idea on the state of financial systems.Consequently,countries can be more accurately categorized based on different problems such as unsustainable expansions or shallow financial markets.Copyright Ó2014,Borsa _Istanbul Anonim S ‚irketi.Production and hosting by Elsevier B.V .JELS classification:G1;G2;E44Keywords:Stock market capitalization;Market capitalization capacity;Market capitalization effort1.IntroductionEffective financial markets are considered to be one of themost important factors for economic development and growth both in developing and developed countries.Firms borrow funds and/or sell equities in these markets to finance their investment activities,which in turn promotes growth.Given the obvious importance of financial markets,the link between financial development and economic development as a wholeis extensively investigated in the literature.1In this group of studies stock markets attract special attention as an important source of economic growth and development.Well-developed stock markets are considered essential parts of the financial development of countries.2Despite widespread need for financial services,the range and depth of financial markets,including stock markets,vary*Tel.:þ12404610978.E-mail address:nxb23@ .Peer review under responsibility of Borsa _Istanbul Anonim S ‚irketi1King and Levine (1993),De Gregorio and Guidotti (1995),Levine (1997),and Rajan and Zingales (1998)are some examples of the prominent studies,presenting the link between financial development and growth.Some recent papers include Darrat,Elkhal,Benhabib and Spiegel (2000),Graff (2003),and McCallum (2006).2Baumol (1965)is one of the early examples,studying the link between stock markets and economic growth.Some of the empirical papers implying a positive link between growth performance and stock market development are:Levine and Zervos (1996),Levine and Zervos (1998),Rousseau and Wachtel (2000),Bekaert,Havey,and Lundblad (2001),and Beck and Levine (2004).Available online at Borsa _Istanbul ReviewBorsa _Istanbul Review 14(2014)74e 95/journals/borsa-istanbul-review/2214-8450/10.1016/j.bir.2014.02.0012214-8450/Copyright Ó2014,Borsa _Istanbul Anonim S ‚irketi.Production and hosting by Elsevier B.V .Open access under CC BY-NC-ND license.Open access under CC BY-NC-ND license.significantly across countries.In the literature one of the questions is how to measure the development level of stock markets or otherfinancial markets across countries for appropriate policy formations.Thefirst natural step to un-derstand and compare stock markets is to establish some performance benchmarks and measurements.Measuring per-formance of stock markets is both theoretically and practically challenging.The share of stock market capitalization in gross domestic product(GDP)is generally interpreted as an easy measure of effort for stock market capitalization and used in some studies as the basis for cross-country comparisons.But such a comparison is more meaningful to establish trends across countries with a similar economic structure and a similar level of income.Countries have different stock market characteristics:while developed countries have well-established stock markets,these markets are relatively new for most developing countries and mostly evolved through the globalization andfinancial liberalization process in the1980s and1990s.3The share measure does not consider any hints about the capacity of countries and their effort in the stock market development process.This paper introduces capacity and effort measures of stock market capitalization as diagnostic tools to assess the gap between the actual level of stock market capitalization and the capacity of countries.While constructing these measures,the paper tries to answer the following questions:What is the capacity of a country’s stock market development given their macroeconomic characteristics?What is the level of their effort in this process relative to their capacity?Each country’s characteristics are different,therefore a measure of stock market development that takes into account those country specific characteristics can provide useful in-formation on the development level of stock markets across countries.When cross-country comparisons are based on measures that take into account country specific characteris-tics,such analyses can give a more accurate picture of the state offinancial systems.This in turn helps in the categorization of countries based on the issues of unsustainable expansions (expansion beyond capacity)or shallowfinancial markets(low financial activities relative to the capacity).In this regard,the aim of the paper is to present a tool that might be helpful in identifying jams that stop further deepening of stock markets if the actual level is lower than the capacity,or ease the risk of overheating in stock markets if the actual level is well beyond the capacity of the country.Consecutively,country classifi-cations allow us to analyze how far countries go in facilitating the deepening of stock markets.The answer is important for bothfiscal and monetary policies.The introduction of alternative measures of the develop-ment level offinancial markets,including stock markets,has practical importance.Given thatfinancial development has been seen as an essential tool to promote economic efficiency and growth by governments and multinational agencies,such as the World Bank and the International Monetary Fund,a well-defined set of measures offinancial development is a must for formulating,implementing,and evaluating policies effectively.The alternative measure of stock market develop-ment introduced in this paper can contribute to fulfilling these objectives.The suggested measure can also help to construct a set of performance measures to serve as a useful indicator of relative progress taking into account country specific charac-teristics.The analysis can provide some guidance for countries with various levels of market capitalization and effort.At this stage,it should be noted that even if market capitalization is an important dimension offinancial development for all coun-tries,we do not intend to make country specific advice.Our study focuses on market capitalization performance and de-livers broad directions for reforms in different countries, especially in developing ones.The analyses in this paper are based on a panel dataset from a sample of58developing and46high-income countries,30 of which can be classified as developed countries,for the period of1990e2012.For a robustness check the analyses are repeated for the period of1990e2007,corresponding to the pre-crisis period of2008e2009global economic andfinancial problems.The empirical methodology used in this paper has already been applied in the publicfinance literature to estimate the tax effort and capacity of developing and developed countries.For example,Tanzi and Davoodi(1997),Bird, Vazquez,and Torgler(2004),and Le,Moreno-Dodson,and Bayraktar(2012)introduce such methodologies.This empir-ical methodology requires using regression estimations to construct benchmarks to compare capacity and efforts for market capitalization in different countries.Market capitali-zation capacity is defined as the predicted value of stock market capitalization which is estimated through panel regression analyses,considering a country’s specific macro-economic,financial,and institutional characteristics.Market capitalization effort refers to an index of the ratio of actual market capitalization to a country’s capacity for market capitalization.The index for stock market capitalization effort and coun-tries’actual market capitalization lets us sort countries into four different groups:(i)low market capitalization,low effort for market capitalization;(ii)high market capitalization,high effort for market capitalization;(iii)low market capitalization, high effort for market capitalization;and(iv)high market capitalization,low effort for market capitalization.The clas-sification is based on whether a country’s actual market capitalization is above or below the median value of market capitalization of the countries in our dataset and also whether or not the effort index of a country is above1.The index is equal to1for a country when actual market capitalization is exactly the same as its estimated capacity.Section2gives information on papers focusing on mea-surement issues offinancial development in the literature. Section3summarizes trends in market capitalization.Section 4highlights alternative measures of the market capitalization performance of countries and generates empirical estimations3Some examples of studies focusing on stock markets in developing countries are:Singh(1971),Tirole(1991),El-Erian and Kumar(1995),Nar-ayan et al.(2011),and Udoka and Anyingang(2013).75N.Bayraktar/Borsa I_stanbul Review14(2014)74e95of market capitalization capacity and efforts.This section also reports the trends in market capitalization capacity and effort across countries,calculated based on their market capitaliza-tion and the index measuring their effort.Section5includes some robustness checks.Section6gives detailed information on the stock market structure in Turkey,which is one of the emerging market economies with high growth rates.Section7 concludes.2.Studies on measurement issues infinancial marketsIn the literature there are studies focusing on measurement issues related tofinancial development in general and devel-opment of stock markets in particular.Beck,Demirguc-Kunt, and Levine(2000)list commonly used indicators of the size of stock markets as the ratio of stock market capitalization in percent of GDP,indicators of activity as the ratio of stock market total value traded in percent of GDP,and indicators of efficiency as the stock market turnover ratio.In this group of suggested measures of stock market development,market capitalization in percent of GDP is the most commonly used one.Some examples of studies using this measure for cross country comparisons are:Atje and Jovanovic(1993), Demirguc-Kunt and Levine(1996),Levine and Zervos (1998),Garcia and Liu(1999),Arestis,Demetriadis,and Luintel(2001),Naceur,Ghazouani,and Omran(2007),Bill-meier and Massa(2009),Beck and Demirgu¨c¸-Kunt(2009), Beck,Demirguc-Kunt,and Levine(2010),Narayan,Mishra, and Narayan(2011),Wang,Medianu,and Whally(2011), Cihak,Demirguc-Kunt,Feyen,and Levine(2012),Barajas, Chami,Yousefi(2013),and Barajas,Beck,Dabla-Norris,and Yousefi(2013).Most of these studies compare the size of market capitali-zation in percent of GDP across countries as one indicator of financial deepening and identify countries with high shares as countries with strongfinancial deepening.But this measure-ment ignores how well these countries are doing given coun-tries’financial,macroeconomic,or institutional characteristics.The effort dimension of stock market capital-ization across countries,the main topic investigated in this paper,should not be ignored.There are already some studies in the literature suggesting alternative measures offinancial development.Even though he does not specifically suggest an alternative measure,Levine (1997)states that the size offinancial intermediaries (liquidity liabilities of thefinancial system in percent of GDP, credit allocation by the central bank versus commercial banks, the ratio of credit allocated to private enterprises to total do-mestic credit,credit to private enterprises divided by GDP) may not accurately measure the level offinancial development in cross-country studies.He specifies that country specific features play an important role in determining the level of financial development.Similarly,Lynch(1996)suggests that the size of monetary aggregates or credits is not enough to understand the development level offinancial markets.He adds that alternative measures are required to improve the evaluation of levels offinancial development.He suggests some measures that take into account country characteristics such as structural measures,financial prices,product range, and transaction costs.In a prominent paper Levine and Zervos (1996)suggest alternative ways of measuring stock market development.They use a multifaceted measure of overall stock market development that combines the different indi-vidual characteristics of the functioning of stock markets,such as size,liquidity,and risk diversification.They construct an index combining market capitalization ratio,the total value traded ratio,the turnover ratio and pricing error measure of stock market rger values of the index indicate a higher development level of stock markets.But this study does not consider any country characteristics.In recent years more comprehensive solutions are offered in the literature.Beck(2012)and Beck and Feyen(2013) introduce a gap measure for the distance between the actual level offinancial development and benchmark cases to un-derstand how well countries are doing in terms offinancial development.Beck,Feyen,Ize,and Moizeszowicz(2008) give detailed information on initial steps of their study. These studies are highly related to what this paper is trying to accomplish.One of the questions that they try to answer is: how far and should countries go in facilitatingfinancial deepening?To answer this question they define the“financial possibility frontier”in their studies.The frontier is con-structed using variables,such as socio-economic factors(in-come,market size,population density,age dependency ratio, conflict),macroeconomic management and credibility,avail-able technology and infrastructure.They classify countries based onfinancial systems below frontier and beyond frontier or countries with too low frontier.They also list possible reasons behind these classifications and state that over-shooting a frontier is associated with higher crisis probability and more severe bust periods.Their main focus is on banking industries and private credits.Similarly,in a paper concentrating onfinancial market frictions,De la Torre,Feyen,and Ize(2013)use estimated coefficients of panel regressions to construct benchmark cases for different banking and insurance variables.Then, based on estimated coefficients of regression analyses,they try to identify typologies offinancial activities such as early developers,middle developers,and late developers.In the paper they neither analyze stock markets nor classify countries.In a paper trying to answer the question of whetherfinan-cial development beyond some limit can help growth(they name it as“too muchfinance”),Arcand,Berkes,and Panizza (2012)again use estimated coefficients of panel regressions to determine the marginal effect offinancial development on growth and whether there is a threshold above whichfinancial development no longer has a positive effect on economic growth.They show that the positive effect offinancial devel-opment may actually vanish as the size offinancial activities get larger.In the paper,the authors focus on private credit and do not include any stock market activities.The main contributions of this paper to the literature are a specific focus on stock markets;the introduction of country76N.Bayraktar/Borsa I_stanbul Review14(2014)74e95specific characteristics to determine the capacity of countries for stock market capitalization;calculation of the effort index for each country which is a gap measure between actual market capitalization and the capacity of a country;and categorization of countries based on the actual level of market capitalization and their effort.3.Trends in stock market capitalizationThe graphical and tabular analyses in this section are based on data collected from the World Bank’s World Development Indicators.All countries with continuous data points for market capitalization are included in the dataset.The list of countries is given in Table1.As indicated in the previous section,stock market capital-ization is one of the most commonly used indicators of financial market development.With the increasing trend to-wards globalization,stock market capitalization has improved significantly after the1990s,especially in developing coun-tries.For comparison purposes,Fig.1shows the time trend for stock market capitalization in percent of GDP for developing and developed countries.4The series are calculated as the average value of market capitalization for74developing countries and30developed countries between1990and2012. Despite continued growth,the share of market capitalization in GDP is lower in developing countries.The overall average market capitalization in percent of GDP was67percent in developed countries between1990and2012,while it was only 29percent for developing countries.Fig.2shows the share of stock market capitalization in percent of GDP for each country included in the paper. Country averages are calculated for the period of1990e2012. Despite the fact that stock markets are getting larger in most countries,they are still not large enough to be a significant source of funds tofinancefirm-level activities in most coun-tries.The share of market capitalization in GDP is less than50 percent for70countries out of104and only5of those are developed countries.Hong Kong has the largest stock market size relative to its GDP(335percent of GDP).In the group of developing countries South Africa has largest stock market capitalization relative to its GDP(172percent).In the top10 group,the share of Asian countries is significant:Hong Kong, Singapore,Malaysia,and Taiwan.Jordan is the only Middle Eastern country in the top group with108percent of GDP. While the market capitalization share is around115percent in the United States,it is only42percent in China.Chile is the top Latin American country with a95percent stock market capitalization share.Even though it is not in the top group, Brazil has a relatively large market capitalization(close to40 percent).In addition to South Africa,Mauritius is another Sub-Saharan African country with a relatively large stock market (40percent).The share of stock market capitalization in percent of GDP is not the only indicator measuring the development level of stock markets in the literature.Table1reports country aver-ages for three more indicators and countries’per capita real income.Countries with higher-income levels tend to have a larger share of market capitalization in percent of GDP.The simple correlation coefficient between GDP per capita and the share of stock market capitalization is45percent.Luxemburg is the richest country in the group with more than$70,000real GDP per capita.Its share of stock market capitalization is150 percent.However,some other developed countries with high GDP per capita income,such as Norway,Denmark,Iceland, and Ireland,have relatively lower market capitalization.Japan is the richest Asian country in the group and their market size is74percent on average.In the Latin America group Chile is the richest country with the highest share of market capitali-zation(96percent).The cases of Malaysia and South Africa are very similar to Chile:They have162percent and172 percent market capitalization,respectively.The United States has the largest number of domesticfirms traded,followed by India.China,where markets are less competitive,has a rela-tively lower number of listed domesticfirms.Romania also has a large number of listed domesticfirms in the stock market,but the time trend analysis shows that thisfigure has dropped significantly in recent years.Despite its high market capitalization,South Africa has a relatively lower number of listed domesticfirms in the market.Most African countries tend to have a very limited number of listed domesticfirms in their stock markets,while Asian and Latin American countries tend to have morefirms traded.When a country’s number of listed domestic companies is compared to its per capita in-come,it can be seen that there is no clear relationship between the number offirms and income(the simple correlation co-efficient is only16percent).This is because the link can depend on many different factors such as competitiveness of markets.Only one country(the United States)within the top 10per-capita income group have more than the overall average number of listed domesticfirms(428),which is calculated taking into account all the countries included in the study.World Bank(2013)defines the total value of stocks traded (in percent of GDP)as the total value of shares traded during the period.They also add that this indicator complements the market capitalization ratio by showing whether market size is matched by trading.In the literature it is also used to mea-sure the market depth,in terms of its liquidity or the easiness to buy and sell shares.Table1shows that most countries with a high share of market capitalization also have a higher value of stocks traded in percent of GDP.The simple cor-relation coefficient between those two variables is46 percent.The top two developed countries in terms of the share of stocks traded are Switzerland and the United States with171percent and176percent,respectively.The table also reports that the value of stocks traded in percent of GDP is relatively low for most developing countries.4In the World Development Indicators Database,market capitalization(or market value)is defined as the share price times the number of shares outstanding.Listed domestic companies are the domestically incorporated companies listed on the country’s stock exchanges at the end of the year.The group of listed companies does not include investment companies,mutual funds,or other collective investment vehicles.77N.Bayraktar/Borsa I_stanbul Review14(2014)74e95Table1Indicators of stock market development(averages over1990e2012).GDP per capita (constant2005US$)Listed domesticcompanies,totalMarket capitalization of listedcompanies(%of GDP)Stocks traded,totalvalue(%of GDP)Stocks traded,turnover ratio(%)Argentina428612626.58 3.2019.34 Armenia128576 1.030.03 4.41 Australia30,864143891.9562.6965.84 Austria34,4909820.9110.0149.62 Bahrain14,45444101.56 5.27 4.50 Bangladesh387216 5.88 4.0854.73 Barbados10,3901990.17 4.79 4.02 Belgium33,31917157.0319.1331.77 Bermuda67,3772045.12 2.35 4.02 Bolivia9992713.800.110.94 Botswana47961621.980.81 5.25 Brazil463246039.3120.4253.84 Bulgaria327036112.33 1.8912.65 Canada31,721246093.9160.3562.70 Chile679025196.1312.3012.80 China1491112243.0157.96153.34 Colombia333711627.02 2.849.99 Costa Rica4317498.760.54 4.99 Cote d’Ivoire9853415.720.36 2.26 Croatia926313428.49 1.72 6.05 Cyprus20,5529837.8513.7829.01 Czech Republic11,31329222.9511.7451.96 Denmark43,75521852.8936.2266.15 Ecuador290247 6.730.36 5.06 Egypt117667034.3512.0927.57 El Salvador25494318.850.42 2.90 Estonia88911823.887.3826.26 Fiji33291211.960.17 1.55 Finland32,98511386.0276.4080.07 France31,68975764.1753.0477.16 Georgia1373211 5.650.25 5.55 Germany32,61365339.6345.92117.63 Ghana4832513.770.44 3.21 Greece18,71326042.1524.2049.18 Guyana10351116.230.130.97 Hong Kong SAR,China24,263856329.47257.2971.69 Hungary93194519.2014.5565.74 Iceland47,3383364.3946.2145.22 India662486048.6043.64101.00 Indonesia121930127.4711.6746.82 Iran236028115.08 2.6917.94 Ireland46,8006454.0623.2844.75 Israel18,58357160.8532.4059.90 Italy28,71026431.7334.54101.61 Jamaica41794355.78 2.61 5.46 Japan34,355282374.3359.4777.14 Jordan2168172108.3538.3828.22 Kazakhstan32125020.24 1.809.79 Kenya5315524.19 1.63 5.78 Korea,Rep.15,218129058.54109.26194.56 Kuwait31,20211986.6454.7462.55 Kyrgyz Republic48425 1.63 1.43174.36 Latvia5541457.220.8313.26 Lebanon56621120.21 2.4710.78 Lithuania647211115.35 1.7711.92 Luxembourg70,16347149.95 1.99 1.42 Macedonia2836347.16 1.43213.27 Malaysia4998756162.7367.4539.10 Malta13,7541236.57 1.65 5.53 Mauritius46464939.90 2.27 5.84 Mexico725516630.599.4732.64 Mongolia935394 6.210.5011.78 Morocco18246138.827.8017.08 78N.Bayraktar/Borsa I_stanbul Review14(2014)74e95In Sub-Saharan Africa,the share of stocks traded in percent of GDP is highest in South Africa (61percent).Among Asian countries,Malaysia,Thailand,China,and India have relatively larger stocks traded ratios.It should be noted that this ratio is also high in Turkey (33percent).The turnover ratio (another measure of market depth from the literature)is defined as the total value of shares traded during the period divided by the average market capitaliza-tion for the period.Table 1shows that nine developing countries’have a turnover ratio larger than 100percent.These are Kyrgyz Republic,Pakistan,India,China,Macedonia,Korea,Taiwan,Macedonia,and Turkey.The United States,Spain,the Netherlands,Italy,and Germany are the only developed countries with the share higher than 100percent.For the whole group,the average turnover ratio is 45percent.Table 1(continued )GDP per capita(constant 2005US$)Listed domestic companies,total Market capitalization of listed companies (%of GDP)Stocks traded,total value (%of GDP)Stocks traded,turnover ratio (%)Namibia 3317108.370.32 3.90Nepal30412517.000.72 4.53Netherlands 36,39719791.8697.15101.41New Zealand 24,56013941.9514.5236.71Nigeria 76518914.46 1.728.54Norway 58,99017242.4039.2986.32Oman 11,85010126.87 6.9624.76Pakistan 64267919.6730.92161.57Panama45312224.260.52 2.66Papua New Guinea 889994.620.350.37Peru277422532.71 3.5515.90Philippines 114122153.8512.3323.21Poland 711625519.048.0060.80Portugal 16,93410532.0519.5054.67Romania 4133265610.15 1.3620.60Russia490421136.5623.5163.94Saudi Arabia 13,5108959.6670.6086.90Serbia 316469324.18 1.7113.62Singapore25,089376163.7293.5960.25Slovak Republic 10,331314 5.77 1.9938.75Slovenia 15,5215918.53 2.5523.26South Africa 5035526172.4561.2233.77Spain 23,292187464.1192.24129.31Sri Lanka 113723018.39 2.8115.80Swaziland 2228510.670.080.94Sweden 36,70927291.7586.2188.09Switzerland 50,269238187.47171.2787.59Taiwan,China 14,104626113.13236.71229.03Tanzania 3469 4.650.14 4.26Thailand244342859.6944.9183.87Trinidad and Tobago 10,1183154.13 1.81 5.10Tunisia 28644013.05 1.6712.31Turkey 641526724.6132.64135.93Ukraine173318217.950.887.08United Arab Emirates 40,6156825.6423.1059.58United Kingdom 33,5022158128.55114.6290.86United States 38,4256267114.51176.20150.01Uruguay 5290150.710.02 2.78Venezuela 5617718.20 1.5313.94Vietnam 54615613.387.2553.05Zambia6361312.280.433.94Fig.1.Trend in market capitalization of listed companies for developed versus developing countries (1990e 2012,%of GDP).Source:World Bank World Development Indicators.79N.Bayraktar /Borsa I _stanbul Review 14(2014)74e 95Fig.2.Market capitalization of listed companies(averages over1990e2012,%of GDP).Source:World Bank World Development Indicators.。
vanna指标

vanna指标Vanna指标是一种金融衡量指标,用于度量期权价格变动对于波动率变动的敏感程度,也就是隐含波动率对于时间的敏感性。
Vanna指标的概念源自于期权交易,期权交易是金融市场上的一种衍生品合约,给予买方以在未来某个时间点以约定价格买入或卖出一种资产的权利。
Vanna指标主要用于期权交易中,帮助交易者更好地理解和应对市场波动风险,提供了重要的参考依据。
Vanna指标的主要作用是帮助交易者了解波动率和时间对期权价格的影响,及时调整策略以应对市场波动。
在期权交易中,波动率是一个重要的指标,代表了市场上资产价格变动的速度和幅度。
隐含波动率是期权定价模型中的重要参数,它反映了市场对于未来波动率的预期。
Vanna指标通过衡量期权价格对于隐含波动率和时间的敏感程度,帮助交易者判断市场波动情况,选择合适的交易策略。
Vanna指标以希腊字母"V"命名,表示波动率(Vega)和斜度(Gamma)的组合。
斜度(Gamma)是期权价格对于标的资产价格变动的敏感性,而波动率(Vega)是期权价格对于波动率变动的敏感性。
Vanna指标衡量了期权价格对于标的资产价格和波动率同时变动的敏感性,反映了期权价格对于市场的整体变动的综合影响。
Vanna指标的计算方式是通过计算期权价格对于标的资产价格和波动率的二阶偏导数来得到的。
具体计算公式如下:Vanna = (∂V/∂S) * (∂V/∂σ)其中,V是期权价格,S是标的资产价格,σ是波动率。
Vanna指标可以帮助交易者分析市场风险,并做出相应的交易决策。
当市场波动率上升时,隐含波动率也将上升,期权价格可能发生较大的变动。
此时,Vanna指标的值将增大,交易者可以通过增加期权头寸来获得更大的收益机会。
相反,当市场波动率下降时,隐含波动率可能下降,期权价格的变动也会相对较小。
此时,Vanna指标的值较小,交易者可以减少期权头寸以降低风险。
值得注意的是,Vanna指标只能对未来的波动率变动进行预测,并不能准确预测未来的价格变动。
Leveraging Data Science for Business Insights
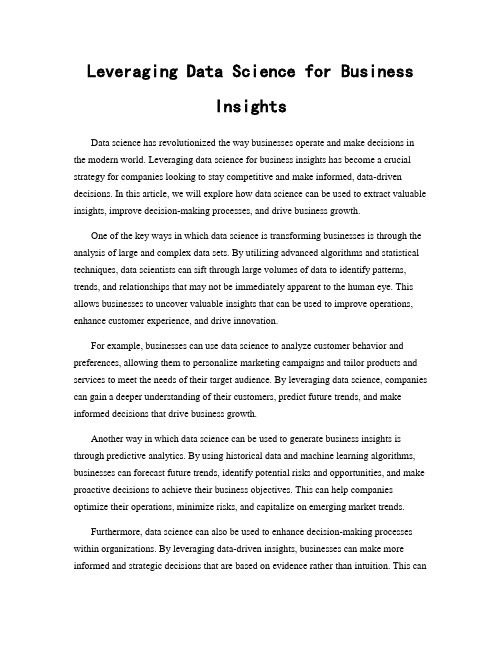
Leveraging Data Science for BusinessInsightsData science has revolutionized the way businesses operate and make decisions in the modern world. Leveraging data science for business insights has become a crucial strategy for companies looking to stay competitive and make informed, data-driven decisions. In this article, we will explore how data science can be used to extract valuable insights, improve decision-making processes, and drive business growth.One of the key ways in which data science is transforming businesses is through the analysis of large and complex data sets. By utilizing advanced algorithms and statistical techniques, data scientists can sift through large volumes of data to identify patterns, trends, and relationships that may not be immediately apparent to the human eye. This allows businesses to uncover valuable insights that can be used to improve operations, enhance customer experience, and drive innovation.For example, businesses can use data science to analyze customer behavior and preferences, allowing them to personalize marketing campaigns and tailor products and services to meet the needs of their target audience. By leveraging data science, companies can gain a deeper understanding of their customers, predict future trends, and make informed decisions that drive business growth.Another way in which data science can be used to generate business insights is through predictive analytics. By using historical data and machine learning algorithms, businesses can forecast future trends, identify potential risks and opportunities, and make proactive decisions to achieve their business objectives. This can help companies optimize their operations, minimize risks, and capitalize on emerging market trends.Furthermore, data science can also be used to enhance decision-making processes within organizations. By leveraging data-driven insights, businesses can make more informed and strategic decisions that are based on evidence rather than intuition. This canhelp companies reduce biases, minimize errors, and improve overall business performance.In addition to improving decision-making processes, data science can also help businesses optimize their operations and increase efficiency. By analyzing data from various sources, companies can identify bottlenecks, streamline processes, and optimize resource allocation. This can lead to cost savings, increased productivity, and improved business performance.Moreover, data science can also be used to enhance risk management within organizations. By analyzing historical data and identifying potential risks, businesses can develop robust risk management strategies to mitigate threats and protect their assets. This can help companies proactively manage risks, minimize losses, and ensure business continuity.Overall, leveraging data science for business insights is essential for companies looking to thrive in today's competitive business environment. By harnessing the power of data science, businesses can unlock valuable insights, improve decision-making processes, and drive business growth. Whether it's analyzing customer behavior, predicting future trends, or optimizing operations, data science has the potential to transform businesses and drive success in the digital age.。
- 1、下载文档前请自行甄别文档内容的完整性,平台不提供额外的编辑、内容补充、找答案等附加服务。
- 2、"仅部分预览"的文档,不可在线预览部分如存在完整性等问题,可反馈申请退款(可完整预览的文档不适用该条件!)。
- 3、如文档侵犯您的权益,请联系客服反馈,我们会尽快为您处理(人工客服工作时间:9:00-18:30)。
Measuring Data Believability: a Provenance ApproachNicolas PRATESSEC Business SchoolAvenue Bernard HirschBP 50105 - 95021 Cergy Cedex - FRANCEprat@essec.frStuart MADNICKMIT Sloan School of Management 30 Wadsworth Street – Room E53-321Cambridge MA 02142 - USAsmadnick@AbstractData quality is crucial for operational efficiency and sound decision making. This paper focuses on believability, a major aspect of quality, measured along three dimensions: trustworthiness, reasonableness, and temporality. We ground our approach on provenance, i.e. the origin and subsequent processing history of data. We present our provenance model and our approach for computing believability based on provenance metadata. The approach is structured into three increasingly complex building blocks: (1) definition of metrics for assessingthe believability of data sources, (2) definition of metrics for assessing the believability of data resulting from one process run and (3) assessment of believability based on all the sources and processing history of data. We illustrate our approach with a scenario based on Internet data. To our knowledge, this is the first work to develop a precise approach to measuring data believability and making explicit use of provenance-based measurements.1. IntroductionData quality is crucial for operational efficiency and sound decision making. Moreover, this issue is becoming increasingly important as organizations strive to integrate an increasing quantity of external and internal data. This paper addresses the measurement of data believability. Wang and Strong [1] define this concept as “the extent to which data are accepted or regarded as true, real and credible”. Their survey shows that data consumers consider believability as an especially important aspect of data quality. Besides, the authors characterize believabilityas an intrinsic1 (as opposed to context- i.e. task-dependant) data quality dimension.1Although the distinction between intrinsic and contextual dataquality is not always clear-cut and often more a matter of degree, thisFrom the definition of believability, it is clear that the believability of a data value depends on its origin (sources) and subsequent processing history. In other words, it depends on the data provenance (aka lineage), defined in [2] as “information that helps determine the derivation history of a data product, starting from its original sources”. There exists a substantial body of literature on data provenance. Several types of data provenance have been identified, e.g. “why-provenance” versus “where-provenance” [3] [4], and schema-level versus instance-level provenance [5]. Major application areas include e-science (e.g. bioinformatics) [2] [6] [7] [8], data warehousing and business intelligence [9], threat assessment and homeland security [10] [11] [12]. Among the several possible uses of provenance information, data quality assessment is widely mentioned [2] [6] [13] [14]. Ceruti et al. [12] even argue that a computational model of quality (enabling quality computation at various aggregation levels) should be an integral part of a provenance framework. However, in spite of the relationship between data provenance and quality, no computational model of provenance-based data quality (and more specifically believability) can be found in extant data-provenance literature. It should be noted that some papers, including [10], [15] and [16] address knowledge (as opposed to data) provenance. More specifically, [10] and [15] deal with the issue of trust-based belief evaluation. However, those papers deal with the believability of knowledge (represented as logical assertions). In contrast, we focus on data believability.In the literature of data quality, believability has been defined in [1]. Guidelines for measuring this quality dimension may be found in [17] (pp. 57-58). However, these guidelines remain quite general and no formal metrics are proposed. An earlier data quality paper [18] addresses the issue of lineage-based data makes believability more easily amenable to automatic computation than other contextual dimensions like relevancy or timeliness.quality assessment (even if the concept of lineage/provenance is not explicitly mentioned). However, the authors address data quality (defined as the absence of error) in a general and syntactic way. We argue that the different dimensions of quality (and, more particularly, of believability) have different semantics, which should be explicitly considered for quality computation.Summing up the contribution of extant literature, (1) the literature on provenance acknowledges data quality as a key application of provenance, but does not provide an operational, computational model for assessing provenance-based data believability and (2) the literature on data quality has defined believability as an essential dimension of quality, but has provided no specific metrics to assess this dimension. Consequently, the goal of our work is to develop a precise approach to measuring data believability and making explicit use of provenance-based measurements.The rest of the paper is structured as follows. Section 2 presents the dimensions of believability. Section 3 presents our provenance model. This model aims at representing and structuring the data which will then be used for believability computation. The approach for believability measurement is presented in section 4. It is structured into three increasingly complex building blocks: (1) definition of metrics for assessing the believability of data sources, (2) definition of metrics for assessing the believability of data resulting from one process run and (3) global assessment of data believability. Section 5 applies our approach to an example scenario based on Internet data, and section 6 concludes with a discussion and points to further research.2. Dimensions of believabilityBelievability is itself decomposed into sub-dimensions. Lee et al. [17] propose three sub-dimensions, namely believability: (1) of source, (2) compared to internal common-sense standard, and (3) based on temporality of data. Table 1 refines this typology (the notations introduced in the table will be used in section 4).Table 1. Dimensions of believabilityDIMENSION (NOTATION) DEFINITION1. Trustworthiness of source (S i) The extent to which a data value originates from trustworthy sources.2. Reasonableness of data (R i) The extent to which a data value is reasonable (likely).2.1 Possibility (R1i) The extent to which a data value is possible.2.2 Consistency (R2i) The extent to which a data value is consistent with other values of thesame data.2.2.1. Consistency over sources(R21i)The extent to which different sources agree on the data value.2.2.2. Consistency over time (R22i) The extent to which the data value is consistent with past data values.3. Temporality of data (T i) The extent to which a data value is credible based on transaction andvalid times.3.1. Transaction and valid times closeness (T1i) The extent to which a data value is credible based on proximity of transaction time to valid times.3.2. Valid times overlap (T2i) The extent to which a data value is derived from data values withoverlapping valid times.3. Provenance modelSeveral “generic” provenance models have been proposed in the literature. These models are generic in that they may be used for a wide variety of applications. The W7 model is proposed by Ram and Liu [11]. This model represents the semantics of provenance along 7 complementary perspectives: “what” (the events that happen to data), “when” (time), “where” (space), “how” (actions), “who” (actors), “which” (devices) and “why” (reason for events, including goals). The W7 model is expressed with the ER formalism [19]. [8] presents ZOOM, a generic model to capture provenance for scientific workflows. Finally, [20] presents initial ideas concerning the data model of the Trio system. One of the characteristics of Trio is the integration of lineage with accuracy/uncertainty.Figure 1. UML representation of the provenance modelContrary to the above-presented provenance models, which are generic, we have a specific objective in mind, namely the computation of the different dimensions of believability. Therefore, some semantic perspectives need to be developed more thoroughly, while others are of secondary interest. For example, using the terminology of the W7 model, the reasons for events (“why”) are of little interest for computing believability. On the contrary, the “when” is crucial in our case, especially for assessing the third dimension of believability (temporality of data).Figure 1 represents our provenance model in UML notation [21]. Section 4 will illustrate how the elements of the provenance model are used for computing the different dimensions of data believability (introduced in section 2).Since our goal is to assess the believability of data values, they are the central concept of the model. A data value may be atomic or complex (e.g. relational records or tables, XML files…). Our current research is focused on atomic, numeric data values. Other types of values will be explored in further research, and the provenance model will be refined accordingly.A data value (e.g. 25 580 000) is the instance of a data (e.g. “the total population of Malaysia in 2004”).A data value may be a source or resulting data value, where a resulting data value is the output of a process run. We introduce this distinction between source and resulting data values because different believability metrics (presented in section 4) are used for these two types of values. The notion of source data value is relative to the information system under consideration: very often, a “source” data value is itself the result of process runs, but these processes are outside the scope of the information system.A process run is the instantiation (i.e. execution) of a process. This distinction between process runs and processes parallels the distinction between data values and data, respectively. The distinction is similar to the one between steps and step-classes proposed in ZOOM [8]. In our approach, processes may have several inputs but only have one output. This restricted notion of process aims at simplifying believability computation. However, this notion of process is quite general. For example, similarly to the process of data storage [18], the paste operation can be represented as a process whose input is the source and output the target data value.A data value has a transaction time. For a resulting data value, the transaction time is the execution time of the process run that generated the data value. For a source data value, the transaction time is attached directly to the data value. For example, if a source data value comes from a Web page, the transaction time can be defined as the date when the Web page was last updated. In addition to transaction time, we use the notion of valid time, defined as follows in [22] (p. 53): “The valid time of a fact is the time when the fact is true in the modeled reality. A fact may have associated any number of instants and time intervals, with single instants and intervals being important special cases.” Contrary to transaction time which depends on process execution, valid time depends on the semantics of data. For example, for the data “the total population ofMalaysia in 2004”, the start valid time is January 1 and the end valid time is December 31, 2004. The distinction between valid time and transaction time is crucial in our approach. These concepts are used explicitly in the assessment of the two sub-dimensions of temporality. Although transaction time and valid time are standard concepts in temporal databases, we haven’t encountered this distinction in extant provenance models.When computing data believability (more precisely, when assessing the first sub-dimension of the dimension “reasonableness of data”), we will use the concept of possibility defined in possibility theory [23]. Accordingly, a possibility distribution is associated with data. Possibility distributions may be acquired from experts. They take their values between 0 (impossible) and 1 (totally possible) and may be defined on intervals [24]. For example, if one considers that the total population of Malaysia in 2004 is somewhere between 10 000 000 and 40 000 000, this can be expressed by a possibility distribution with a value of 1 in the [10 000 000 ; 40 000 000] interval, and 0 outside. In this case, the possibility distribution is equivalent to an integrity constraint stating that the total population of Malaysia in 2004 should be in the [10 000 000 ; 40 000 000] range. However, possibility distributions allow for a fine-tuned representation of uncertainty, by using possibility values between 0 and 1. The possibility distribution then approaches a bell-shaped curve, with a value of 1 around the center of the interval (e.g. between 20 000 000 and 30 000 000 in our example), and decreasing values as one gets closer to the extremities of the interval. Like our provenance model, Trio combines provenance with uncertainty. However, contrary to Trio, we use the possibility theory instead of probabilities to represent uncertainty. We believe that possibilities provide a more pragmatic approach. In particular, possibility distributions are easier to acquire from experts than probability distributions.Processes are executed by agents (organizations, groups or persons). This concept also represents the providers of the source data values. For example, if a data value comes from the Web site of the Economist magazine, the agent is the Economist (an organization).When computing believability, we are not interested in agents per se, but in the trustworthiness of these agents. The concept of trustworthiness is essential for assessing the dimension “trustworthiness of source”. We use the term “trustworthiness” in a similar way as [25]. Trustworthiness is evaluated for an agent, for a specific knowledge domain [25] [26]. Examples of knowledge domains are “management”, “engineering”… Trustworthiness is closely related to trust and reputation. Reputation is similar to our concept of trustworthiness, but we consider this term astoo general i.e. reputation does not depend on a specific domain. Trust, contrary to reputation, is subjective i.e. depends on a particular evaluator, the “trustor” [26]. We avoid introducing this subjectivityin our approach. This is consistent with the finding thatdata consumers consider believability and reputation asan intrinsic part of data quality [1]. However, trust is a function of reputation [27], and a natural extension ofour work would be a more subjective, user-centered assessment of believability.Trustworthiness in an agent for a domain is measured by a trustworthiness value, normalized between 0 and 1. The computation of these values is outside the scope of our work. We assume that these values are obtained from outside sources, e.g. reputation systems [28]. Thus, the trustworthiness ofthe magazine “The Economist” is available from Epinions (). Heuristics may also be used to propagate trustworthiness. For example, [29] shows that an individual belonging to a group inherits a priori reputation based on that group’s reputation.Summing up, our provenance model is specific to believability assessment. Consequently, it integrates allthe concepts that we will need for provenance-based believability assessment. The model was elaborated by integrating concepts from existing models, by specifying these concepts and adding new concepts (e.g. possibility). Our model is represented with an object-oriented formalism (UML), thus enabling a more precise representation of semantics than with the standard ER formalism. Finally, our provenance modelis also guided by pragmatic considerations: several provenance metadata used in our approach (e.g. process execution data like transaction time, input or output values, actors…) are relatively easy to traceand/or readily available in existing tools (e.g. log filesin workflow tools, “history” tab in Wikepedia – –, …).4. Provenance-based believability assessmentBased on the information contained in the provenance model, our approach computes and aggregates the believability of a data value across the different dimensions and sub-dimensions of believability (as presented in Table 1). The approach is structured into three building blocks.4.1. Believability of data sourcesThis section presents the metrics and parts of the associated algorithms for computing the sub-dimensions of the believability of data sources. The metrics are real values ranging from 0 (total absence of quality) to 1 (perfect quality). The algorithms use an object-like notation (for example, for a data value v, v.data is the object of class Data corresponding to the data value v).The trustworthiness of a source data value v (noted S1(v)) is defined as the trustworthiness of the agent which provided the data value (the knowledge domain for which the trustworthiness of the agent is evaluated has to match with the knowledge domain of the data).In order to compute the reasonableness of a source data value v (noted R1(v)), we need to define metrics for possibility (R11(v)), consistency over sources (R211(v)), consistency over time (R221(v)), and aggregate these metrics.The possibility R11(v) of a data value v is retrieved directly from the provenance model, using the possibility distribution of the corresponding data.To compute consistency over sources (R211(v)), the intuition is as follows: we consider the other values of the same data, provided by other sources. For each such value, we determine the distance between this value and the value v (to compute this distance, we use a formula widely used in case-based reasoning [30]). We transform distances into similarities by taking the complement to 1, and compute the average of all similarities. Our approach for computing consistency over sources is similar to the approach described by Tversky [31] for computing the prototypicality of an object with respect to a class (this prototypicality is defined as the average similarity of the object to all members of the class). More formally, based on the UML provenance model represented in Figure 1, the1that values of the same data should not vary too much over time, otherwise they are less believable. The basic principle for computing this metric is similar to the previous metric. However, the specific semantics of time has to be taken into account. Also, this metric assumes that effects of seasonality are absent or may be neglected.The reasonableness R1(v) of a source data value v is computed by aggregating the values of the above-presented metrics. In order to compute the value of a dimension based on the values of its sub-dimensions, the most common aggregation functions are Min, Max, and (weighted) Average [17]. The choice of the appropriate aggregation function depends on the semantics of the dimensions and sub-dimensions, and on the available information. Here, consistency may be defined as the weighted average of the values of its two sub-dimensions (by default, the weights are equal). However, to compute reasonableness from possibility and consistency, the Min operator is more appropriate. Possibility depends solely on the experts’ evaluation, while consistency is strongly correlated with the different data values considered for comparison. Therefore, we make the most cautious choice for aggregating possibility and consistency, namely the Min operator. Alternatively, if the criterion of consistency is considered too much dependant on context or the computation cost too high, the measurement of reasonableness may be based on possibility only. Formally, we have:Let r211 and r221 be the respective weights of consistency over sources and consistency over time (r211 + r221 =1)R1(v)=MIN( R11(v), R21(v))=MIN( R11(v), (r211*R211(v) + r221*R221(v)))To compute the temporal believability of a data value v (T1(v)), we consider two aspects: believability based on transaction and valid times closeness, and believability based on valid times overlap.For believability based on transaction and valid times closeness, the intuition is that a data value computed in advance (estimation) is all the more reliable as the valid time (especially the end valid time) of the data value approaches. To capture this idea, various metrics may be used (e.g. linear, exponential). Here, drawing from the metrics proposed for data currency in [32], we propose an exponential function. The function grows exponentially for transaction times before the end valid time. When transaction time is equal or superior to the end valid time, the value of the metric is 1. A decline coefficient [32] may be used to control the shape of the exponential function. Alternatively, we could use other metrics, e.g. metrics using a different function before and after the start valid time.Believability based on valid times overlap measures the extent to which a data value resulting from a process is derived from data values with “consistent”i.e. overlapping valid times. Thus, this metric isdefined for resulting data values and shall be developed in section 4.2. For source data values, the value of this metric may be defaulted to one (or, alternatively, the weight of the sub-dimension “believability based on valid times overlap” may be set to zero).Consequently, T 1(v) is defined as follows: Let tt:Date such that v.transaction time = tt Let vt:Date such that v.data.end valid time = vt Let t1 be a decline factor (t1>0) T11(v)=)1,))(*1((tt vt t e MIN −−Let t11 and t21 be the weights of the two sub-dimensions of temporality (t11 + t21 =1)T 1(v)= t11*T11(v) + t21*T21(v)= t11*T11(v) + t214.2. Believability of process resultsThe quality of any data value depends on the quality of the source data and on the processes. By combining data, processes may amplify data errors (i.e. quality defects), reduce them, or leave them unchanged, depending on the processes; moreover, processes themselves may be error-prone [18].Following the line of [18], we present metrics for assessing the believability of data resulting from one process run, as the next building block of our approach for global believability assessment. More precisely, we consider a process P whose input data values are denoted by v i (i=1...n, where n is the number of input parameters of the process). We want to determine the believability of the data value (noted v) resulting from P, along the different dimensions of believability. Departing from [18], which treats all types of quality errors uniformly, we claim that as data are transformed through processes, the evolution of the different dimensions of believability (and, more generally, quality), depends not only on the data and processes, but also on the dimensions considered and on their semantics. Therefore, as in section 4.1, we distinguish between the different dimensions of believability.For simplicity, this paper assumes that processes are error-free (e.g. a process specified as dividing one number by another makes the division correctly).To compute the source trustworthiness S 2(v) of an output data value v based on the source trustworthiness of the input data values, we use partial derivatives, adapting the general algorithm proposed in [18] for error propagation (in this paper, we consider the particular case of processes for which these partial derivatives are defined). An error caused on v by a lackof trustworthiness of an input value v i has an incidence on v which depends not only on the value of v i itself, but also on the “weight” (influence) of v i in process P, as measured by the derivative. Consequently, to measure the lack of trustworthiness of v, we compute the weighted average of the lack of trustworthiness for the v i (i=1...n). The weight of v i is the value of v i multiplied by the value of the derivative dP/dx i. We normalize the weights such that their sum equals one,P defined by: P(y)= 3*x 1 + 2*x 2 , and suppose that the value of x 1 is 2 with trustworthiness 0.8 while the value of x 2 is 3 with trustworthiness 0.6. In the present case, the derivatives (3 and 2 respectively) are constant. Applying the metric, the trustworthiness of the resulting data value (12) is 0.7, i.e. the average of the trustworthiness of the two input data values. In this case, the input data values equally contribute in the assessment of the trustworthiness of the result. Assuming now that the value of x 2 is 30, the trustworthiness of the resulting data value (66) is 0.62, reflecting a much more significant role of x 2 in the output data value.To compute the reasonableness R 2(v) of an output data value, we need to consider the sub-dimensions of reasonableness. Concerning consistency, since it depends on the data values considered for comparison, it may not easily be derived from the consistency computed for the input values v i . Therefore, if consistency is used to assess reasonableness, it has to be computed again for the data value v, based on the metric presented in section 4.1.In order to compute the possibility of v based on the input values v i , we follow similar lines of reasoning as for combining trustworthiness (i.e. combination based on derivatives, assuming again that all partial derivatives of process P are defined).To compute temporal believability, we consider its two sub-dimensions.Concerning believability based on transaction and valid times closeness, the principle is the same as in section 4.1. (The transaction time is the transaction time of the process run).Believability based on valid times overlap measures the extent to which the valid times of the input values v i of process P are consistent with each other, i.e. their degree of overlap. In order to define the corresponding metric, we assume here, for the sake of simplicity, that there are only two input values v1 and v2; we also assume that the objective of process P is not to compute an evolution (in the later case, it is normal that the input data – e.g. the total sales in fiscal year 2005 and the total sales in fiscal year 2006 – do not have overlapping valid times). Formally, the metric for believability based on valid times overlap is defined as4.3. Global believabilityAt this point, it is clear that to compute the believability of a data value v, we need to consider the provenance/lineage of this data value, i.e. its origin and processing history.Some aspects of believability are transmitted along the transformation chain of data values. Such is the case with trustworthiness, which is transmitted along processes using the derivative-based metric presented in section 4.2. However, some other aspects of believability may not be transmitted as data move along the process chain. This may be the case, for instance, for possibility (a data value may appear completely possible even though it results from highly implausible data values). This can also happen with the sub-dimensions of temporality. For example, a data value v may be computed by a process P after the end valid time of this value (therefore performing well on the sub-dimension “believability based on transaction and valid times closeness”). However, the input values of P may themselves result from processes performing poorly on the sub-dimension “believability based on transaction and valid times closeness”.Since some aspects of believability may not be transmitted as data move across processes, we need metrics accounting for this phenomenon, considering the complete lineage of a data value. For example, if a highly possible data value v results (directly or indirectly) from highly implausible values, this means that v is highly possible “by accident”. We want to reflect this in the believability computation of v.The central idea of global believability assessment is to consider the complete lineage of a data value. Therefore, at this point, we need a more precise definition of data lineage. The lineage of a data value v is a labeled, directed acyclic graph representing the successive data values and processes leading to data value v. Figure 2 illustrates an example lineage, where data value v is computed by process P2 from values v21 and v22; v21 itself is computed by process P1 from values v11 and v12.Based on lineage, the global believability of a data value v is computed as follows:(1) For each of the three dimensions of believability, a global value for this dimension is computed, by considering the data lineage of v. For instance, if the dimension considered is temporal believability, the global temporal believability of v is noted T3(v). This global temporal believability is computed by averaging the temporal believability of all values in v’s lineage. For example, in the example above, T3(v) is computed by averaging T2(v) with T2(v21), T1(v22), T1(v11) and T1(v12). (According to the notation introduced in Table 1, T1 and T2 designate the temporal believability of data sources and of process results respectively). When computing a global value for any of the three believability dimensions, two types of weights are used (i.e. the average is a weighted average). The first weight is a “discount factor” [33], as often proposed in graph-based algorithms. This factor reflects the intuition that the influence of a vertex on another decreases with the length of the path separating the two vertices (the further away a value is in v’s lineage, the less it counts in the global believability of v). The discount factor may be different for the three dimension of believability, depending on the semantics of the dimension. In addition to discount factors, a second type of weight is used, based on derivatives, similarly to the approach。