XMM-Newton EPIC and RGS observations of LMC X-3
AnEmpiricalSoilLossEquation-tucson.ars.ag.gov

An Empirical Soil Loss EquationLiu Baoyuan, Zhang Keli and Xie YunDepartment of Resources and Environmental Sciences, Beijing Normal University, Key Laboratory of Environmental Change and Natural Disaster, the Ministry of Education of China,Beijing 100875, PR ChinaAbstract: A model was developed for estimating average annual soil loss by water on hillslope for cropland, which is called Chines Soil Loss Equation (CSLE). Six factors causing soil loss were evaluated based on soil loss data collected from experiment stations covering most regions of China and modified to the scale of Chinese unit plot defined. The model uses an empirical multiplicative equation, A=RKLSBET, for predicting interrill erosion from farmland under different soil conservation practices. Rainfall erosivity (R) was the product of rainfall amount and maximum intensity of 10min, and also was estimated by using daily rainfall data. The value of soil erodibility (K), the average soil loss of unit plot per rainfall erosivity, for 6 main soil types was calculated based on the data measured from unit plots and other data modified to the unit plot level. The method of calculating Kfrom soil survey data for regions without measured data was given. The slope length and steepness factors was calculated by using the equations in USLE if slope steepness is less than 11 degree, otherwise the steepness factor was evaluated by using a new seep slope equation based on the analysis of measured soil loss data from steep slope plots within China.According to the soil and water conservation practices in China, the values of bio-control, engineering-control, and tillage factors were estimated.Keywords: Chines soil loss equation, soil loss, unit plot1 IntroductionSoil loss equation is to predict soil loss by using mathematical methods to evaluate factors causing soil erosion. It is an effective tool for assessing soil conservation measures and making land use plans. Universal soil loss equation is an empirical equation developed during 1950’s that had been applied for natural resources inventory successfully in the US and revised in 1990’s. From 1980’s the process-based models for prediction of soil loss have been studied though the world, such as WEPP (Water Erosion Predict Project, Nearing et al., 1989), GUEST (Griffith University Erosion System Template, Misra and Rose, 1996), EUROSEM (the European Soil Erosion Model Morgan etal., 1998) and LISEM (Limburg Soil Erosion Model, De Roo and Wesseling, 1996). There have been many studies on soil erosion models and related experiments since 1940's, but the models were limited in local levels and difficult to expand to broad regions due to data collected without universal standard. So far there is not any soil loss equations that could be applied through China with minor errors. The objective of this study is to develop a soil loss equation used within China based on measured data from Chinese unit plots and data from many plots modified to Chinese unit plot, which is called Chinese soil loss equation (CSLE).2 Model descriptionSoil loss is a process of soil particle detachment by raindrops and then transported by runoff from the rainfall. Many factors like soil physical characteristics slope features, land surface cover etc. will influence soil loss amount, but they have interactions. It is necessary to distinct their effects on soil loss mathematically and to evaluate them on the same scale in order to improve the accuracy of the model. Unit plot is such a good method to solve the problem. The normalized data covering the China by modified to unit plot supported the development of Chines soil loss equation. In addition,22 two features of soil erosion in China are distinctive and should be considered in the equation. One issoil erosion with steep slope, and the other is the systematical practices for soil conservation during thelong history of combating soil erosion, which could be classified as biological-control, engineering-control and tillage measures. So the Chinese soil loss equation was express as followsafter the analysis of data collected from most regions of ChinaA = RKSLBET (1)where A is annual average soil loss (t/ha), R is rainfall erosivity (MJ mm/(h ha y)), K is soilerosibility (t ha h/(ha MJ mm y)), S and L are dimensionless slope steepness and slope lengthfactors, B , E , and T are dimensionless factors of biological-control, engineering-control, and tillagepractices respectively. The dimensionless factors of slope and soil conservation measures were defined as the ratio of soil loss from unit plot to actual plot with aimed factor changed but the samesizes of other factors as unit plot. Chinese soil loss equation is to predict annual average soil lossfrom slope cropland under different soil conservation practices.To evaluated factors in the equation, about 1841 plot-year data were analyzed. Of these, 214plot-year data from 12 plots and 1143 rainfall events from 14 weather stations were used to evaluaterainfall erosivity. The Chinese unit plot was determined by analyzing 384 plot size data, and about200 plot-year data from 12 plots modified to unit plot were used to evaluate erodibility for 6 types ofsoil. About 30 plot-year data from steep plots modified to unit plot was used to establish the steepslope factor equation. Other plot data was used to calculate the values of biological-control, engineering-control and tillage factors.3 Factor calculations for the equation3.1 Rainfall erosivity (R )A threshold for erosive rainfall of 12mm was estimated, close to that suggested by Wischmeierand Smith (1978), 12.7mm. After comprehensive considering the accuracy of rainfall erosivity, thedata availability and calculation simplicity, the rainfall index of a rainfall event for Chinese soil losserosion was defined. It is the product of rainfall amount (P ) and its maximum 10-min intensity (I 10),and the relationship between PI 10 and the universal rainfall index EI 30 was also estimated as follows:EI300.1773PI 10 R 20.902 (2)where E is the total energy for a rainfall event (MJ/ha), I 30 and I 10 are the rainfall maximum 30min and10min intensities respectively (mm/hr), P is the rainfall amount (mm). The annual rainfall erosivityis the sum of PI 10 for total rainfalls through the year. Actually, it is also difficult to get the rainfallevent data. To apply the weather data from weather stations covering the China, an equation functionfor estimating half-month rainfall erosivity by using daily rainfall data was developed.1010.184(n hm d d i i R P ==∑)IR 20.973 (3)where R hm is the rainfall erosivity for half-month (MJ mm/h ha), P d is the daily rainfall amount (mm)and I 10d is the daily maximum 10min rainfall intensivity (mm/h). I =1, …, n is the rainfall days withina half-month. If there is no I 10d available, R hm was also calculated by using only daily rainfall amount.1()n hm d i i R P βα==∑ (4)where α and β are fitted coefficients and other variables had the same meaning as above.Seasonal rainfall erosivity distribution could be estimated by the sum of R hm . To plot Chineseisoerodent map for estimation or interpolation of local values of average annual rainfall erosivity in23 any place, the empirical relationships by using different rainfall available data were estimated (not listed). Users can choose different equations to calculate average annual rainfall erosivity according to the data availability.3.2 Soil erodibility (K)Soil erodibility is defined as soil loss from unit plot with 22.1m long and 9% slope degree per rainfall erosion index unit (Olson and Wischmeier, 1963). Different from the US, much of soil loss was from steep slope in China. So the Chinese unit plot was defined as a 20m long, 5m wide and 15°degree slope plot with continuously in a clean-tilled fallow condition and tillage performed upslope and downslope. The suggestion of Chinese unit plot made data measured be used to evaluate K values as much as possible without large errors. Because 15° is the middle values for most plots in China. Modified data measured from both plots of less than 15° and larger than 15° to a unit plot had the relative minimum errors.Based on the K defination and Chines unit plot, soil erodibility for 6 main soil types in China was estimated. For example, the values of K for loess were 0.61, 0.33, and 0.44 t ha h/(ha MJ mm) in Zizhou, Ansai, and Lishi in Loess Plateau of China.3.3 Slope length (L) and slope steepness (S) factorsTopography is an important factor affecting soil erosion. It is significant to quantitatively evaluate the effects of topography on erosion for predicting soil loss. The effects of topography on erosion includes slope length and steepness in terms of soil-loss estimation. In soil loss equation, factors of slope length and steepenss were cited with dimensionless values. They are values of the ratio of the soil loss from the plot with actual slope steepness or slope length to that from the unit plot.The relationship between slope length and soil loss has been studied from field or lab data for a long time. Many studies showed that the soil loss per area is proportional to some power of slope length except that the values of the exponent are slightly different. For example, Zingg (1940) derived a value of 0.6 for the slope-length exponent. Musgrave (1947) used 0.35. USLE (Universal Soil Loss Equation ) published in 1965 suggested the values of 0.6 and 0.3 respectively for slopes steeper than 10% and very long slopes, and 0.5 for other conditions. In 1978, the USLE (Wischmeier and Smith, 1978) adjusted the exponent values for different cases, 0.5 for 5% slope or more, 0.4 for slopes between 3.5% and 4.5%, 0.3 for slopes between 1% and 3%, and 0.2 for slopes less than 1%. Revised USLE (RUSLE) published in 1997 used a continuous function of slope gradient for calculating slope-length exponent.Soil erosion from the steep slope is serious in China. How slope length influences soil loss on steep slopes needs further studies. For this end, the relationship between slope length and soil loss on steep slopes was examined based on the plot data obtained at Suide, Ansai, and Zizhou on the loess plateau of China and modified to the unit plot. The results indicated that the slope-length equation in the RUSLE could not be used for soil loss prediction under the steep slope conditions. The equation for calculating soil length factor in the USLE published in 1978 could be applied into China:22.13mLλ=(5)where λ is slope-length (m), m is the slope length exponent.Slope gradient is another topographical factor affecting soil erosion. Most studies have shown that the relation of soil loss to gradient may be expressed as some exponential function or quadratic polynomial. Zingg (1940) concluded that soil loss varies as the 1.4 power of percent slope, and Musgrave (1947) recommended the use of 1.35. Based on a substantial number of field data, Wischmeier and Smith (1965) derived a slope-gradient equation expressed as quadratic polynomial function of gradient percent. Having analyzed the data assembled from plots under natural and simulated rainfall, McCool et al., (1987) found that soil loss increased more rapidly from the slopes24 steeper than 5° than that from slopes less than 5°, and he recommended two different slope steepnessfactor equations for different ranges of slopes:S =10.8sin θ + 0.03 θ 5° (6-1)S =16.8 sin θ – 0.5 θ > 5°(6-2) These equations were established based on soil loss data from gentle slopes, and have not beentested for steep slope conditions. We used soil loss plot data from Suide, Ansai, and Tianshui on theloess plateau of China to test the equations. The results showed that great errors were produced whenusing equations suggested by McCool et al ., (1987) for predict soil loss from slopes steeper than 10°. After the slope degree was larger than 10°, soil loss from steep slopes increased ripedly. Based theregression analysis of our data, am equation to calculate slope steepness factor for seep slopes wasdeveloped:S =21.91 sin θ – 0.96 θ10°(6-3)So in Chinese soil loss equation, slope steepness factor could be estimated by using equation (6-1)to (6-3) under different slope conditions3.4 Bilogical-control (B ), engineering-control (E ), and tillage (T ) factorsDuring the development of the historical agriculture traditions in China, the systematical practicesfor soil and water conservation formed. They could be divided into three categories: biological-control, Engineering-control and tillage measures. Biological-control practices include theforest or grass plantation for reducing runoff and soil loss. Engineering-control practices refer to thechanges of topography to reduce runoff and soil loss by engineering construction like terrace, check-dams. Tillage practices are the measures taken by farmland equipment. The difference between engineering and tillage is that the latter does not change the topography and is only applied onthe farmland.Table 1 Estimated values of biological-control factor for cropsSeedbed Establishment Development Maturing crop Growing season Annual averageBuckwheat 0.71 0.54 0.19 0.21 0.74 0.74 Potato 1.00 0.53 0.47 0.30 0.47 0.50 Millet 1.00 0.57 0.52 0.52 0.53 0.55 Soybean 1.00 0.92 0.56 0.46 0.51 0.53 Winter wheat 1.00 0.17 0.23Maize intercropping with soybean1.00 0.40 0.26 0.03 0.28 Hyacinth Dolichos 1.00 0.70 0.46 0.57Table 2 Estimated values for factors of woodland and grassland vegetationSophora Korshinsk Peashrub Seabuckthorn Seabuckthorn & PoplarSeabuckthorn & Chinese Pine Erect Milkvetch Sainfoin Alfalfa First year Sweetclover Second year Sweetclover0.004 0.054 0.083 0.144 0.164 0.067 0.160 0.256 0.377 0.08325Many studies gave the B values for different biologic measures in China, but they were not from the universal calculated methods and could not be used directly in soil loss equation. Based on the defination of B values, the ratio of soil loss from plots with some biological-control practice to that from unit plot, we calculated B values for some types of biological-control practices (Table 1). Some values for typical engineering-control and tillage measures in China were summarized (not listed).References[1] Nearing, M.A., G.R. Foster, L.J. Lane, and S.C. Finkner. A process-based soil erosion modelfor USDA-water erosion prediction project technology. Transactions of The ASAE, 1989, 32(5):1587-1593.[2] Misra R K, Rose C W. Application and sensitivity analysis of process -based erosionmodel—GUEST[J]. European Journal Soil Science. 1996,10:593-604.[3] Morgan R P C, Quinton J N, Smith R E, et al., The European soil erosion model (EUROSEM): Adynamic approach for predicting sediment transport from fields and small catchments[J]. Earth Surface Processes and Landforms, 1998,23:527-544.[4] De Roo A P J. The LISEM project: an introduction. Hydrological Processes. 1996, vol.10:1021-1025.[5] Wischmeier W H, Smith D D. Predicting rainfall erosion losses[R]. USDA AgriculturalHandbook No.537. 1978.[6] Olson,T.C., and Wischmeier,W.H. 1963. Soil erodibility evaluations for soils on the runoff anderosion stations. Soil Science Society of American Proceedings 27(5):590-592.[7] Zingg A W. Degree and length of land slope as it affects soil loss in runoff[J]. AgriculturalEngineering, 1940,21: 59-64.[8] Musgrave G W. The quantitative evaluation of factors in water erosion A first approximation[J].Journal Soil and Water Cons, 1947, 2:133-138.[9] Renard K G,Foster G R,Weesies G A et al., RUSLE―A guide to conservation planning with therevised universal soil loss equation[R]. USDA Agricultural Handbook No.703. 1997.[10] McCool, D. K. Brown, L.C., Foster, G. R., et al., Revised slope steepness factor for theuniversal soil loss equation. TRANSACTIONS of the ASAE, 1987, 30(5): 1387-1396.。
土体邓肯—张非线性弹性模型参数反演分析全

可编辑修改精选全文完整版土体邓肯—张非线性弹性模型参数反演分析近年来,随着科学技术的发展,经过精心设计的弹性模型和参数反演算法技术开始被广泛应用于土体力学中。
英国科学家邓肯(Duncan)和张(Zhang)的非线性弹性模型参数反演分析方法为土体力学研究奠定了坚实的理论基础。
线性弹性模型参数反演分析旨在研究土体的弹性本构模型,决土体的动态参数反演问题,从而更好地控制和解释土体力学行为。
首先,非线性弹性模型是一种普遍适用的土体力学模型,描述了土体的应力应变关系,其中包括受力弹性部分,恢复弹性部分和弹性非线性部分.述应力应变关系的函数可以用地质、浅层力学等参数表示。
其中包括材料参数,比如弹性模量、泊松比、抗拉强度极限等;空间参数,比如等效平面应力变化率等;时间参数,比如历史负荷重复次数等。
然后,非线性弹性参数反演分析是一种专门用于研究土体动态参数变化特性和土体弹性本构模型确定的非线性优化算法。
主要包括反演算法和参数估计算法。
演算法可以从提供的土体动态应力应变数据中恢复弹性本构参数的值,而参数估计算法则可以从实验测量数据中精确估计土体实际弹性参数的值。
此外,非线性弹性模型参数反演分析具有许多优点,到的结果有助于深入理解土体动态变化特性,有助于开发新的土体力学理论,有助于实现高精度的土体力学分析及模拟,为现有土体力学分析方法提供了更为准确的理论支撑。
最后,非线性弹性模型参数反演分析技术对土体力学研究有重要意义。
管技术刚刚起步,但有望在解决实际问题上发挥重要作用。
此,有必要加强相关技术的研究,加强详细计算,改进参数反演算法,并在非线性弹性本构分析的理论和实验研究方面进行深入挖掘,以及在实际工程中对该技术的实际应用。
综上所述,非线性弹性模型参数反演分析是一种新的、有效的土体力学分析方法,从理论和实践上都有重要意义,为土体力学研究和工程实践提供了有用的理论和技术支持。
Optimal Designs for Mixed-Effects Models with Random Nested Factors

Optimal Designs for Mixed-Effects Models with Random Nested FactorsB RUCE E. A NKENMAN, ankenman@Phone: (847) 491-5674; Fax: (847) 491-8005A NA I VELISSE A VILES, ivelisse@Northwestern UniversityDepartment of Industrial Engineering and Management Sciences2145 Sheridan Rd. MEAS C210, Evanston, IL 60208J OSE C. P INHEIRO, jcp@Bell Laboratories, Lucent Technologies600 Mountain Ave. Room 2C-258, Murray Hill, NJ 07974ABSTRACTThe problem of experimental design for the purpose of estimating the fixed effects and the variance components corresponding to random nested factors is a widely applicable problem in industry. Random nested factors arise from quantity designations such as lot or batch and from sampling and measurement procedures. We introduce a new class of designs, called assembled designs, where all the nested factors are nested under the treatment combinations of the crossed factors. We provide parameters and notation for describing and enumerating assembled designs. Using maximum likelihood estimation and the D-optimality criterion, we show that, for most practical situations, designs that are as balanced as possible are optimal for estimating both fixed effects and variance components in a mixed-effects model.KEYWORDS: Assembled Designs, Crossed and Nested Factors, D-Optimality, Experimental Design, Fixed and Random Effects, Hierarchical Nested Design, Maximum Likelihood, Nested Factorials, Variance Components.1. IntroductionIn many experimental settings, different types of factors affect the measured response. The factors of primary interest can usually be set independently of each other and thus are called crossed factors. For example, crossed factors are factors like temperature and pressure where each level of temperature can be applied independently of the level of pressure. These effects are often modeled as fixed effects. Nested factors cannot be set independently because the level of one factor takes on a different meaning when other factors are changed. Random nested factors arise from quantity designations such as lot or batch and from sampling and measurement procedures that are often inherent in the experimentation. The variances of the random effects associated with nested factors are called variance components since they are components of the random variation of the response. Batch-to-batch variation and sample-to-sample variation are examples of variance components.The nested or hierarchical nested design (HND), which is used in many sampling and testing situations, is a design where the levels of each factor are nested within the levels of another factor. Balanced HNDs for estimating variance components have an equal number of observations for each branch at a given level of nesting. Not only do these designs tend to require a large number of observations, but they also tend to produce precise estimates of certain variance components and poor estimates of others. Some articles that address these issues are Bainbridge (1965) and, more recently, Smith and Beverly (1981) and Naik and Khattree (1998). These articles use unbalanced HNDs, called staggered nested designs, to spread the information in the experiment more equally among the variance components. A staggered nested design only branches once at eachlevel of nesting and, thus, there is only one degree of freedom for estimating each variance component. Of course, if a staggered nested design is replicated n times then each variance component will have n degrees of freedom for estimation. Goldsmith and Gaylor (1970) address the optimality of unbalanced HNDs. Delgado and Iyer (1999) extend this work to obtain nearly optimal HNDs for the estimation of variance components using a limit argument combined with numerical optimization.When the fixed effects of crossed factors and variance components from nested random factors appear in the same model, there are many analysis techniques available for estimation and inference (see Searle, Casella, and McCulloch, 1992; Khuri, Matthew, and Sinha, 1998; or Pinheiro and Bates, 2000). However, only limited work has been done to determine what experimental designs should be used when both crossed factors and variance components occur in a single experiment. Smith and Beverly (1981) introduced the idea of a nested factorial, which is an experimental design where some factors appear in factorial relationships and others in nested relationships. They propose placing staggered nested designs at each treatment combination of a crossed factorial design and called the resulting designs staggered nested factorials.Ankenman, Liu, Karr, and Picka (1998) introduced what they call split factorials, which split a fractional factorial design into subexperiments. A different nested design is used for each subexperiment, but within a subexperiment all design points have the same nested design. The nested designs in a split factorial only branch at a single level and thus, the effect is to study a different variance component in each subexperiment.The general problem of experimental design for the purpose of estimating both crossed factor effects and variance components is a quite broad and widely applicable problem in industry. Some examples could be:1)Chemical or pharmaceutical production where certain reactor settings or catalysts are the crossed factors and raw material lot-to-lot variation, reactor batch-to-batch variation, and sample-to-sample variation are the variance components. In this case, knowing the size of the variance components may help to determine the most economical size for a batch.2)A molding process where machine settings such as mold zone temperatures or mold timings are the crossed factors and shift-to-shift variation, part-to-part variation, and measurement-to-measurement variation are the variance components. Knowing which variation source is largest could help to focus quality improvement efforts.3)Concrete mixing where recipe factors such as the size of the aggregate used in the concrete and the ratio of water to cement powder are crossed factors and batch-to-batch variation and sample-to-sample variation are the variance components. Knowing about the variance components can help engineers to understand how variation in the properties of the concrete will change throughout large concrete structures.The purpose of this paper is to provide experimental design procedures for the estimation of the fixed effects of crossed factors as well as variance components associated with nested factors that arise from sampling and measurement procedures. We introduce a special class of nested factorials, called assembled designs, where all the nested factors are random and nested under the treatment combinations of the crossed factors. The class of assembled designs includes both the split factorials and thestaggered nested factorial designs. In Section 2, we provide parameters and notation for describing and enumerating assembled designs. In Section 3, we describe a linear mixed-effects model, which can be used to analyze assembled designs. The fixed effects and the variance components are estimated using maximum likelihood (ML). We present expressions for the information matrix for the fixed effects and the variance components in assembled designs with two variance components in Section 4. In Section 5, we provide theorems which show that under most practical situations, the design which is the most balanced (i.e., spreading the observations as uniformly as possible between the branches in the nested designs) is D-optimal for estimating both fixed effects and two variance components. In Section 6, we show with examples how to obtain the D-optimal design for the requirements of an experiment. Conclusions and discussion are then presented in Section 7.2. Assembled DesignsAn assembled design is a crossed factor design that has an HND placed at each design point of the crossed factor design. If the assembled design has the same number of observations at each design point, it can be described by the following parameters: r , the number of design points in the crossed factor design; n , the number of observations at each design point; q , the number of variance components; and s , the number of different HNDs used. For the special case of only two variance components (q =2), we will use the terms batch and sample to refer to the higher and lower level of random effects,respectively. Also for this case, we define B T as the total number of batches; B j as the number of batches in the j th HND; and r j as the number of design points that contain the j th HND. Thus, T sj j j B B r =∑=1We will use a simplified version of the concrete experiment in Jaiswal, Picka, Igusa,Karr, Shah, Ankenman, and Styer (2000) to illustrate the concepts of and introduce the notation for assembled designs. The objective of the concrete experiment is to determine the effects of certain crossed factors on the permeability of concrete and the variability of the permeability from batch-to-batch and from sample-to-sample. The design has three two-level factors (Aggregate Grade, Water to Cement (W/C) Ratio, and Max Size of Aggregate) and two variance components (q=2), Batch and Sample. The design (Figure1) has a total of 20 batches (B T =20).In Figure 1, each vertex of the cube represents one of the eight possible concrete recipes or design points (r=8) that can be made using the two levels of Grade, W/C Ratio,and Max Size. Thus, the front upper left-hand vertex represents a concrete recipe with the low level of Grade, the low level of W/C Ratio, and the high level of Max Size. The branching structure coming from each vertex represents batches and test samples to be made from that recipe. There are four samples per recipe (n=4).In the context of an assembled design, HNDs that are attached to the crossed factor design points will be referred to as structures . In the concrete experiment, there are twoFigure 1: An Assembled Design (B T = 20, r = 8, q = 2, n = 4, s = 2).Grade W/C RatioMax SizeLevel 2Level 2Level 2Level 1Level 1Level 1Guide to StructuresStructure 1Structure 2different structures (s=2). Structure 1 consists of three batches (B 1=3) where two samples are cast from one of the batches and one sample is cast from each of the other two batches. Structure 1 appears at four of the design points, so r 1=4. Structure 2appears at the other four design points (r 2=4) and consists of two batches (B 2=2) and two samples cast from each batch.In order to compare all possible assembled designs with the same parameter values,we will begin by discussing what structures are available for building the assembled designs. Let N represent the number of structures available for a given n and let k be the number of batches. The number of structures available for given n and k , with two variance components (q =2) is∑∑∑∑∑ −−++−=++−= = −−= −−−=−−−−−−=)3()(2)(11231322121111221231k k i i n i i i i n i i k n i k i n i i k i i n i i k k k k k k k N ,where x called floor[x ], gives the greatest integer less than or equal to x . The total number of structures is then, ∑==nk k N N 1. Figure 2 shows the number of possible structures that are available for use in assembled designs for various values of n and q =2and provides some examples.In a structure, the total number of degrees of freedom is equal to the number of observations and each degree of freedom can be thought of as a piece of information with a cost equal to that of a single observation. For a single structure, the degrees of freedom available for estimating the first variance component equals its number of branches minus one. More generally, the degrees of freedom associated with the i th nested random factor equals the number of branches at the i th level of nesting minus the number of branches at the (i -1)st level of nesting. Figure 3 shows an example of calculations for degrees offreedom for an HND with q =4. (Note that an HND can also be thought of as an assembled design with one structure and one design point.)The notation for a single q -level structure consists of q –1 different sets of parentheses/brackets. The i th nested random factor in a q-stage hierarchical design (i =1,…,q -1) is represented by a specific set of parentheses including as many elements as the number of i th nested random factor branches. For the q th variance component, the number of observations at the last level of nesting is specified. For uniqueness of equivalent nested designs, the elements at the last level of nesting as well as the number of elements at the i th level of nesting, i =1,…,q -1, are specified in descending order,starting from the q th level of nesting and ending with the first level of nesting.Notation can be understood easier by examples. Figure 4 shows the notation for the two structures in the concrete experiment. Structure 1 has three batches and thus has notation (2,1,1), where each element refers to the number of samples cast from eachFigure 3: Degrees of Freedom for an HND (q =4).22334557611n# of Structures Structures 715All available structures Some examplesFigure 2: The Number of Possible Structures (HNDs) for n =[2,7] and q =2. EUDQFKHV/ / / /batch. Structure 2 consists of two batches and two samples cast from each batch and thus has notation (2,2). Note that for q=2, there is just one set of parenthesis. Figure 5 shows an example of notation for a structure with four variance components.Figure 2 shows that as n increases, there are more available structures and it follows that, for a given number of design points, r , there are more potential assembled designs which have the same number of observations at each design point (n ).Building on the notation used for individual structures, the notation for an assembled design is ,} structure with points @{design structure 1∑=sj j j where the design points need to be ordered in some way. For assembled designs, we order the design points so that all rows with the same structure are in adjacent rows. This order is called design order .Recall that r j is the number of design points with structure j . The notation in design order would be ∑=−−++s j j j j R ,R R j 111},,21{@ structure , where ∑==jh h j r R 1 and .00=R Figure 6 shows that structures in the concrete experiment were assigned to the design points using the interaction ABC and it also shows the comparison of design order and standard order (see Myers and Montgomery 1995, p. 84) for a two-level factorial. TheseFigure 5: Notation for HND (q = 4).Figure 4: Concrete Experiment; Notation for HND (q =2).Notation for this Structure:{[(2,2,1),(3),(1)],[(3,2,2),(2,1)]}n =19lot batch samplemeasurement q =4(2,2)(2,1,1)batch ; sampleStructure 1Structure 2orderings are for convenience in describing the experiment and in manipulating the expressions of the model and analysis. When conducting the experiment, the order of observations should be randomly determined whenever possible.In an assembled design, the number of degrees of freedom is equal to the total number of observations. Thus, an assembled design with nr observations has a total of nr degrees of freedom. There are r degrees of freedom for estimating the fixed effects including the constant. There are nr -r degrees of freedom left for estimating variance components. We will designate the number of degrees of freedom for estimating the i th variance component as d i . When q=2, ()r B r B r B r d T sj j s j j j s j j j −=−=−=∑∑∑===11111 and d 2=nr-d 1-r=nr-B T .3. Analysis of Assembled Designs3.1. Model and Variance StructureThe linear mixed-effects model used to represent the response in an assembled design with nr observations and q variance components is,∑=+=q i i i 1u Z X y ,(1)Figure 6: Concrete Experiment (A=Grade, B=W/C Ratio, and C=Max Size);Comparison of Standard Order and Design Order.D esignO rder S ta ndard O rderA B C A B C S tructure11----24++--36+-+-47-++-52+--+63-+-+75--++88++++,5,8}(2,2)@{2,3,4,6,7}(2,1,1)@{1 :Order Standard in Notation ,7,8}(2,2)@{5,6,2,3,4}(2,1,1)@{1 :Order Design in Notation ++where y is a vector of nr observations, X is the fixed-effects design matrix, is a vector of r unknown coefficients including the constant term, Z i is an indicator matrix associated with the i th variance component, u i is a vector of normally distributed independent random effects associated with the i th variance component such that ()I 0u 2 i i N a . Let V be the nr ×nr variance-covariance matrix of the observations.Assume that the variance components do not depend on the crossed factor levels. Then,∑=′==qi i i i Var 12 Z Z y V .(2)Let X D be the full rank r r × design matrix (including the constant column) for a single replicate of a crossed factor design, where rows are ordered in design order. Also let the observations in X be ordered such that X =X D §1n , where 1n is an n -length vector of ones and § represents the Kronecker product. This ordering in X gives rise to Z-matrices that have the form ,1it rt i Z Z ⊕== where ⊕ refers to the Kronecker sum and Z it isthe indicator matrix related to the observations associated with variance component i for the treatment combination t . For the fixed effects, X t is the portion of the X matrix associated with the t th treatment combination. X t is a n ×r matrix where all n rows are identical. Let t D X ′ represent the row of X D corresponding to the t th treatment combination, then X t = t D X ′§1n . Let V t be the n ×n variance-covariance matrix associated with the treatment combination t , then , 12∑=′=qi it it i t Z Z V which relates to (2). Thus, Vcan be written as: .1t rt V V ⊕==Consider the case of two variance components (q =2) and denote by 21σ the batchvariance and by 22σ the sample variance. Z 2, the sample indicator matrix, is the identity matrix of order nr . Z 1t has n rows and as many columns as the number of batches used with treatment combination t . Z 1, the batch indicator matrix for an assembled design, has as many rows as total number of samples (nr ) and as many columns as total number of batches (B T ). As an example, recall that in the concrete experiment (introduced in Section 2) there are eight design points (r =8), two structures (s =2), and four observations at each design point (n =4). Recall that Structure 1 is (2,1,1) and Structure 2 is (2,2).Thus, based on treatment combinations: Z 2=I 32, Z 2t =I 4, t =1,2, (8). and ,10100101,100010001001181716151413121111817161514131211=========Z 00Z 00000000Z 00000000Z 0000000Z 0000000Z 00000000Z 00000000Z Z Z Z Z Z Z Z Z Z 3.2. Analysis TechniquesDifferent estimation methods have been proposed for the analysis of linear mixed-effects models. Currently, the two most commonly used methods are maximum likelihood (ML) and restricted maximum likelihood (REML). The method of maximum likelihood prescribes the maximization of the likelihood function over the parameter space, conditional on the observed data. Such optimization involves iterative numerical methods, which only became widely applicable with recent advances in computer technology. REML estimation is effectively ML estimation based on the likelihood of the ordinary least-squares residuals of the response vector y regressed on the X matrix ofthe fixed effects. Because it takes into account the loss of degrees of freedom due to the estimation of the fixed effects, REML estimates of variance components tend to be less biased than the corresponding ML estimates. In this paper, we will use ML estimation for the fixed effects and variance components, because of the greater simplicity of the associated asymptotic variance-covariance matrices (which are used to determine the D-optimal designs). Since ML and REML estimators are asymptotically equivalent, we expect that the D-optimal designs for ML will be at least close to optimal for REML.Conditional on the variance components, the estimation of the fixed effects is a generalized least squares (GLS) problem, with solution ()y V X X V X111ˆ−−−′′= (see Thiel, 1971, p. 238 for further details). In general, the variance components need to be estimated from the data, in which case the GLS methodology becomes equivalent to ML.It follows from the model assumptions that, conditional on the variance components,the variance-covariance matrix of the fixed-effects estimators is ().11−−′X V X In practice,the unknown variance components are replaced by their ML estimates. In this case,under the assumption of normality, the variance-covariance matrix ofˆ is the inverse of the information matrix corresponding to (see Searle, Casella, and McCulloch, 1992, p.252-254). Because of the independence of the observations at different treatmentcombinations, ,)()(11∑=−=′=rt t Inf Inf X V X where t t t t Inf X V X 1)(−′=. It thenfollows that ()()()()∑∑=−=−′⊗′=⊗′⊗′⊗=rt n t n t t rt n ttn t Inf 11111)(1V 1X X 1X V 1X D D D D .Since n t n 1V 11−′ is a scalar,.)(11∑=−′′=rt t t n t n Inf D D X X 1V 1 (3)There are only asymptotic results available for the variance-covariance matrix of the variance component estimators. Denoting the vector of ML estimators by 2ˆσ, the asymptotic variance-covariance matrix of q variance-components estimators for an assembled design is (see Searle, Casella, and McCulloch, 1992, p. 253):()()()()()111111111111111112222122ˆˆˆˆ−−−−−−−−−′′′′′′′′≈=qq q q q q q q q tr tr tr tr Var Var Z Z V Z Z V Z Z V Z Z V Z Z V Z Z V Z Z V Z Z Vσσσ,where tr () indicates the trace function of a matrix. The information matrix of the variance components for treatment combination t is()()()()′′′′′′′′=−−−−−−−−qt qt t qt qt t t t t qt qt t qt qt t t t t t t t t t t t tr tr tr tr Inf Z Z V Z Z V Z Z V Z Z V Z Z V Z Z V Z Z V Z Z V 1111111111111111221)( .The information matrix of variance-components estimators is ∑==rt t Inf Inf 122)()( .4. Information Matrix for Two Variance ComponentsAssembled designs are a very large class of designs, many of which are too complicated for practical use. Since the most likely assembled designs to be used in practice are the most simple, we will study assembled designs for two variance components (q =2) in detail. Detailed study of assembled designs with more than two variance components is left for future research.The simplest assembled design with r =1 design point and s =1 structure is equivalent to an HND. Hence, it is fundamental to study a single HND or the j th structure. For notational simplification, we will use B instead of j B to represent the number of batches in a structure for the case of q =2, r =1, and s =1. Using the notation established in Section3 for HNDs, any structure with B batches and q =2 levels of nesting can be represented by()B B m m m m ,,,,121− , where i m is the number of samples in batch i and 1+≥i im m .To develop the information matrix for fixed effects at a single design point, the expressions in terms of m i ’s for a treatment combination t are:[]i i m m Bi nt t t t t t t I J I Z Z Z Z Z Z V 2221122112122221121+=+′=′+′=⊕=,where J n is an n ×n square matrix with all elements equal to one. Note that for q =2,n t t I Z Z =′22. Also,++−=⊕=−i i m m i Bi tm I J V 2221222221111) ( and from (3), it can be shown that the information for fixed-effects estimator (in this case just the mean) of a structure at a design point given n , B , and q =2 is∑∑==−−+=++−=′′=′=B i i i Bi i i i tt n t n t t t t m mm m m Inf 1212212221222222111. ) ( ) (D D X X 1V 1X V X (4)Note that for a single design point (r =1), X D t =1, thus and ) (t Inf are scalars.The asymptotic results for the variance-covariance matrix of the variance-components estimators for ML (q =2) are:()()()()()()()()1211121112121112)(2ˆ−−−−−′′′≈tt t t t t tt tt t tr tr tr tr Var V Z Z V Z Z V Z Z V σand()()()()()()()()()+−+−++++=′′′=∑∑∑∑====−−−−Bi i i i i B i i i Bi iiB i i i t t t t t t t t t t t m m m m m m m m m m tr tr tr tr Inf 1222121212242211121112121112)1()1())1(1()1()1()1(21)(21ττττττσV Z Z V Z Z V Z Z V σ(5)where τ is defined as the variance ratio 2221σσ. Expressions in terms of the i m ’s in (5)are derived in Appendix 1.The information matrix for []′2 is block diagonal (see Searle, Casella, andMcCulloch, 1992, p. 239) and, therefore, the estimators for the fixed effects and the variance components are asymptotically uncorrelated.5. Optimal Assembled DesignsAs n increases, there become a large number of assembled designs that are essentially equivalent according to their parameters. In Section 4, expressions were provided for the asymptotic variance-covariance matrices of the estimates from assembled designs. In this section, designs are compared in terms of their ability to accurately estimate the fixed effects and the variance components. There are many criteria that have been used to compare experimental designs. These optimality criteria are based on minimizing in some sense the variance of the estimates of the fixed effects and variance components.The D-optimality criterion is possibly the best known and most widely accepted (see Myers and Montgomery, 1995, p. 364 and Pukelsheim, 1993, p. 136). A design is D-optimal if it minimizes the determinant of the variance-covariance matrix of the estimates, often called the generalized variance of the estimates. Because no closed form expressions are available for the variance-covariance matrix of the maximum likelihood estimates in a linear mixed-effects model, we rely on the asymptotic results of Section 4and investigate (approximate) D-optimal assembled designs using the asymptotic variance-covariance matrices. Equivalently, we will then seek the assembled design that maximizes the determinant of the information matrix of the fixed effects and the variance components. Because the information matrix is block diagonal, its determinant is theproduct of the determinant of the fixed-effects information matrix and the determinant of the variance-components information matrix. It follows that if the same design that maximizes the determinant of fixed-effects information matrix also maximizes the determinant of the variance-components information matrix, then that design is D-optimal.Recall that for q =2, any HND with B batches can be represented by ()B B m m m m ,,,,121−= m , where i m is the number of samples in batch i, i=1,2,…,B and 1+≥i i m m . Define M B as the set of all feasible and non-trivial HNDs,{}1;,,2,1;,,,,M 1121B −<=≥∈=++−n B B i m m m m m m m i i i B B ),(6)where )+ denotes the set of positive integers (i.e., at least a sample is taken per produced batch). Note that, by definition, n m Bi i =∑=1. We consider the HNDs where n B = or1−=n B to be trivial, since there is only one HND for each of these cases and thus theymust be optimal.5.1. Fixed-Effects OptimalityFor a single HND, the D-optimality criterion for the fixed effects is the determinant of the matrix defined in (4). Since (4) is a scalar, it is the D-optimality criterion. The D-optimal HND for fixed effects can be found for any choice of n and B by solving Problem I : n m m m Bi i Bi i i=+∑∑==∈112122M subject to max Bσσm .Problem I is non-linear and has implicit integer constraints. A solution to Problem I is found by comparing any given HND with another that subtracts one observation from 1m and adds one observation to B m . By the definition of M B in (6), 1+≥i i m m , 1m and B m are respectively the maximum and minimum number of samples per batch in m . Theorem。
Empirical processes of dependent random variables
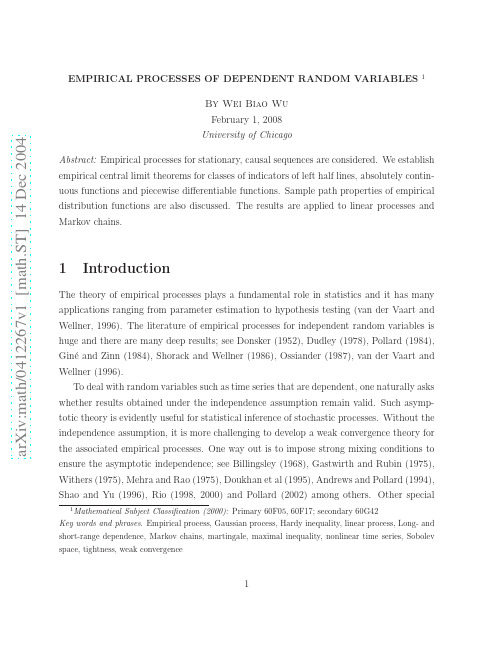
2
Preliminaries
n i=1
from R to R. The centered G -indexed empirical process is given by (P n − P )g = 1 n
n
the marginal and empirical distribution functions. Let G be a class of measurabrocesses that have been discussed include linear processes and Gaussian processes; see Dehling and Taqqu (1989) and Cs¨ org˝ o and Mielniczuk (1996) for long and short-range dependent subordinated Gaussian processes and Ho and Hsing (1996) and Wu (2003a) for long-range dependent linear processes. A collection of recent results is presented in Dehling, Mikosch and Sorensen (2002). In that collection Dedecker and Louhichi (2002) made an important generalization of Ossiander’s (1987) result. Here we investigate the empirical central limit problem for dependent random variables from another angle that avoids strong mixing conditions. In particular, we apply a martingale method and establish a weak convergence theory for stationary, causal processes. Our results are comparable with the theory for independent random variables in that the imposed moment conditions are optimal or almost optimal. We show that, if the process is short-range dependent in a certain sense, then the limiting behavior is similar to that of iid random variables in that the limiting distribution is a Gaussian process and the norming √ sequence is n. For long-range dependent linear processes, one needs to apply asymptotic √ expansions to obtain n-norming limit theorems (Section 6.2.2). The paper is structured as follows. In Section 2 we introduce some mathematical preliminaries necessary for the weak convergence theory and illustrate the essence of our approach. Two types of empirical central limit theorems are established. Empirical processes indexed by indicators of left half lines, absolutely continuous functions, and piecewise differentiable functions are discussed in Sections 3, 4 and 5 respectively. Applications to linear processes and iterated random functions are made in Section 6. Section 7 presents some integral and maximal inequalities that may be of independent interest. Some proofs are given in Sections 8 and 9.
牛顿-拉夫逊潮流计算中检测雅可比矩阵奇异性和网络孤岛的新方法
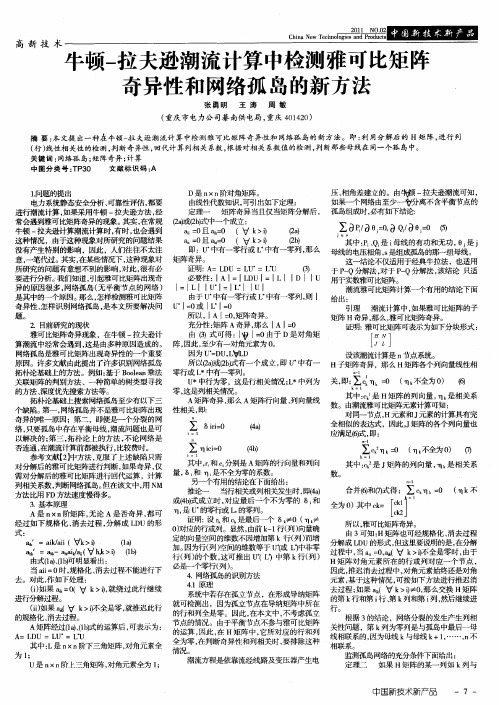
由 ( 式可得:I 【 0由于 D是对角矩 3 ) = 阵, , 因此 至少有一对角元 素为 0 。 因为 U= UL D D ,VL 设该潮流计算 是 n 节点 系统 。 所以( ) 2) 2 或( 工 a b弋有一个成立 , U 中有一 H子矩阵奇异 ,那 么 H矩阵各 个列向量线 性相 即 n 一1 零行 或 中有一零列 。 u 中行为零 , 是行相关 隋况 ;丰中列 为 关 , : 这 L 即 - = ( 不全为 0 q 0 ) 零, 这是列相关 隋况。 其 中: 是 H矩 阵的列 向量 ,1是相关 系 c T A矩 阵奇异 , 那么 A矩 阵行 向量 、 向量线 列 数 。由潮流雅可 比矩阵元素计算可知 : 性相关 , 即: 对 同一节点 , 素和 J 素的计 算具 有完 H元 元 全相似 的表达式 ,因此 ,矩 阵的各个列 向量也 J (a 4) 应满足( , 即:
中国新技术新产 品
一7
!
C ia N w T c n l ge n r d cs h n e e h oo isa d P o u t
高 新 技 术
新型停 水 自动关 闭阀结构 、 点及操作要 点 特
张金龙 曹 艳
( 西安航 空技 术高等专科学校机械 工程 系, 陕西 西安 7 0 7 ) 10 7
中图分 类 号 : 4 . 文献标 识 码 : G6 45 A
。
I 言 。在 日常生 活 中 , 前 由于停 水时 忘记 关 闭 阀门 , 水 时 也没 能及 时 关 闭 阀门 , 来 造成 水 资源 浪 费甚 至形 成安 全 隐 患 的情况 屡 见不 鲜 。 着全 民节 水 概念 不 断深入 人 心 , 一 问 随 这 题 引起 各方 关 注 。 因此 急 需设 计 一 款可 以在 停 水 时 自动关 闭 的水 阀 ,它 能够 在停 水 后 即 使 人们 忘记 关 闭 水 龙 头 也 能实 现 自动 关 闭 , 而再 次 来水 时 不 至于 出 现水 患 的情 况 ,能够 有 效 的节 约水 资源 。 要 实 现 自动 关 闭 功 能首 先 要 有 动 力 , 这 方 面可 以借 助 磁性 元件 的磁 力 、弹性 元 件 的 弹力 、 力 等外 力 , 时考 虑供 水 和停 水 时 的 重 同 水 压变 化 , 通过 联 动机 构实 现 。 2停 水 自动关 闭 阀 的结 构 及 特点 。利用 水 压 、 力 等 力 学 特 性 , 过 一 系 列 的实 验 、 重 经 改 进 , 发 出一 种 简单 、 行 的带 有 停水 自锁 研 可 机 构 的水 阀 。 款 水 阀为纯 机 械构 造 , 阀体 这 以 为 主体 框 架 , 有 阀 芯 、 封 圈 、 心 轮 以及 配 密 偏 手柄 , 无弹 性元 件 , 作状 况 不 受环 境 和时 间 工 的 限制 , 构 简 单 , 价 低 廉 并 方 便拆 换 , 结 造 整 体 可靠 性 高 。 停 水 自动关 闭 阀结 构 原 理 如 图 1 示 , 所 实 物 如 图 2所示 。序号 l 水 阀 的偏 心轮 , 为 2 为 0 型密 封 圈 , 为 V型 密封 圈 , 阀体 , 3 4为 5 为 阀芯 , 销 轴 , 手 柄 。 阀体 4是 主 框 6为 7为 架 , 来装 配其 它 元 件 , 进 水 口和 出 水 口; 用 有 阀芯 5的顶 端 与末 端分 别 装有 V 型密 封 圈 3 和 0 型 密 封 圈 2v 型 密 封 圈 3利 用 其 锥 面 , 与 阀体 4内部 锥 面 配合 实 现 停 水 时 密 封 , 而 0型密 封 圈 2与 阀体 4内壁 的接 触 实 现来 水 时对 水 阀末 端 的密 封 ,在 阀 芯 5的 中部 开两
基于Neumann级数预处理的Newton-GMRES潮流计算方法

Email: wangwb0802@, lxbctgu@
Abstract: This paper presents a new preconditioning technique, which is used to improve the convergence performance of Newton-GMRES power flow computation. By using of four basic types of matrix splitting algorithms and the matrix inversion technique based on Neumann series, a new kind of preconditioner based on Neumann series is derived. This kind of preconditioning technique used for power flow computation of Newton-GMRES method can significantly improve the convergence performance and the efficiency of power flow computation. Finally, Power flow computation results of IEEE 118-bus system verify the effectiveness of the proposed method. Keywords: Matrix splitting; Neumann series; Preconditioning; Newton-GMRES; Convergence performance
A New Approach for Filtering Nonlinear Systems
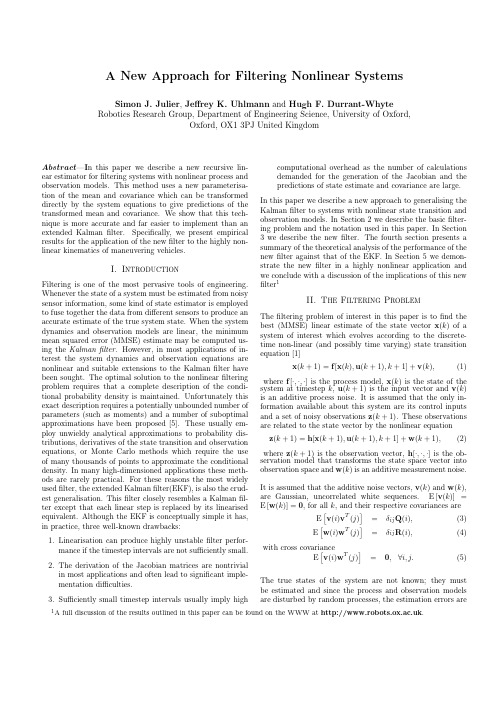
computational overhead as the number of calculations demanded for the generation of the Jacobian and the predictions of state estimate and covariance are large. In this paper we describe a new approach to generalising the Kalman filter to systems with nonlinear state transition and observation models. In Section 2 we describe the basic filtering problem and the notation used in this paper. In Section 3 we describe the new filter. The fourth section presents a summary of the theoretical analysis of the performance of the new filter against that of the EKF. In Section 5 we demonstrate the new filter in a highly nonlinear application and we conclude with a discussion of the implications of this new filter1
Tቤተ መጻሕፍቲ ባይዱ
= = =
δij Q(i), δij R(i), 0, ∀i, j.
(3) (4) (5)
Online Stochastic Modelling for Network-Based GPS Real-Time Kinematic Positioning
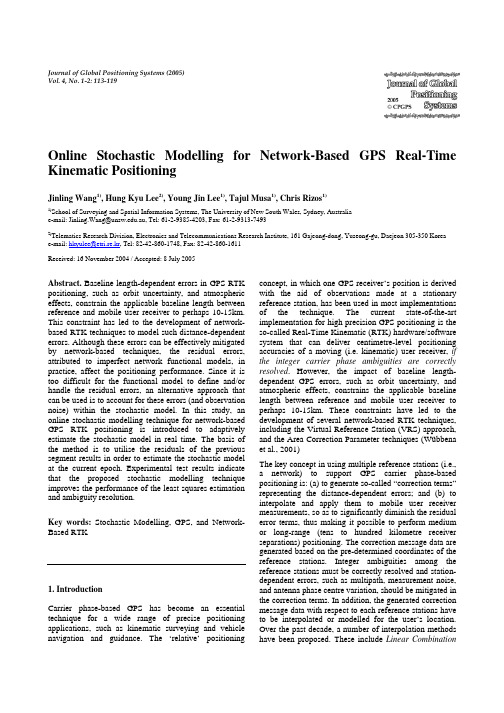
114
Journal of Global Positioning Systems
Model, Distance-Based Linear Interpolation Method, Linear Interpolation Method, Lower-Order Surface Model and Least-Square Collocation (Fotopoulos & Cannon, 2001). However, Dai et al (2001) demonstrated that the performances of all of these methods are similar.
Received: 16 November 2004 / Accepted: 8 July 2005
Abstract. Baseline length-dependent errors in GPS RTK positioning, such as orbit uncertainty, and atmospheric effects, constrain the applicable baseline length between reference and mobile user receiver to perhaps 10-15km. This constraint has led to the development of networkbased RTK techniques to model such distance-dependent errors. Although these errors can be effectively mitigated by network-based techniques, the residual errors, attributed to imperfect network functional models, in practice, affect the positioning performance. Since it is too difficult for the functional model to define and/or handle the residual errors, an alternative approach that can be used is to account for these errors (and observation noise) within the stochastic model. In this study, an online stochastic modelling technique for network-based GPS RTK positioning is introduced to adaptively estimate the stochastic model in real time. The basis of the method is to utilise the residuals of the previous segment results in order to estimate the stochastic model at the current epoch. Experimental test results indicate that the proposed stochastic modelling technique improves the performance of the least squares estimation and ambiguity resolution.
From Data Mining to Knowledge Discovery in Databases
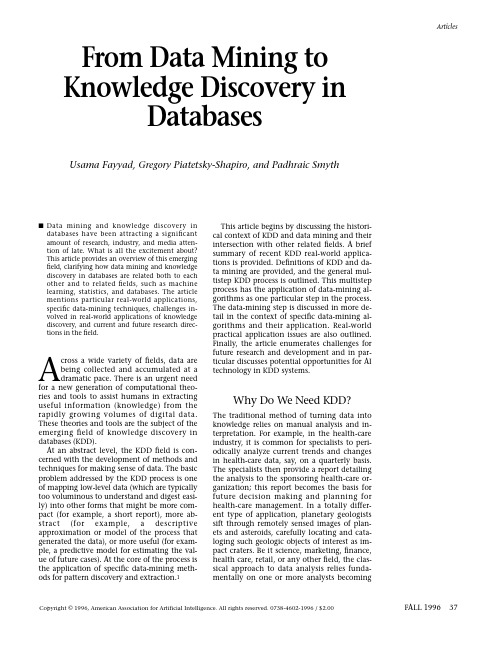
s Data mining and knowledge discovery in databases have been attracting a significant amount of research, industry, and media atten-tion of late. What is all the excitement about?This article provides an overview of this emerging field, clarifying how data mining and knowledge discovery in databases are related both to each other and to related fields, such as machine learning, statistics, and databases. The article mentions particular real-world applications, specific data-mining techniques, challenges in-volved in real-world applications of knowledge discovery, and current and future research direc-tions in the field.A cross a wide variety of fields, data arebeing collected and accumulated at adramatic pace. There is an urgent need for a new generation of computational theo-ries and tools to assist humans in extracting useful information (knowledge) from the rapidly growing volumes of digital data. These theories and tools are the subject of the emerging field of knowledge discovery in databases (KDD).At an abstract level, the KDD field is con-cerned with the development of methods and techniques for making sense of data. The basic problem addressed by the KDD process is one of mapping low-level data (which are typically too voluminous to understand and digest easi-ly) into other forms that might be more com-pact (for example, a short report), more ab-stract (for example, a descriptive approximation or model of the process that generated the data), or more useful (for exam-ple, a predictive model for estimating the val-ue of future cases). At the core of the process is the application of specific data-mining meth-ods for pattern discovery and extraction.1This article begins by discussing the histori-cal context of KDD and data mining and theirintersection with other related fields. A briefsummary of recent KDD real-world applica-tions is provided. Definitions of KDD and da-ta mining are provided, and the general mul-tistep KDD process is outlined. This multistepprocess has the application of data-mining al-gorithms as one particular step in the process.The data-mining step is discussed in more de-tail in the context of specific data-mining al-gorithms and their application. Real-worldpractical application issues are also outlined.Finally, the article enumerates challenges forfuture research and development and in par-ticular discusses potential opportunities for AItechnology in KDD systems.Why Do We Need KDD?The traditional method of turning data intoknowledge relies on manual analysis and in-terpretation. For example, in the health-careindustry, it is common for specialists to peri-odically analyze current trends and changesin health-care data, say, on a quarterly basis.The specialists then provide a report detailingthe analysis to the sponsoring health-care or-ganization; this report becomes the basis forfuture decision making and planning forhealth-care management. In a totally differ-ent type of application, planetary geologistssift through remotely sensed images of plan-ets and asteroids, carefully locating and cata-loging such geologic objects of interest as im-pact craters. Be it science, marketing, finance,health care, retail, or any other field, the clas-sical approach to data analysis relies funda-mentally on one or more analysts becomingArticlesFALL 1996 37From Data Mining to Knowledge Discovery inDatabasesUsama Fayyad, Gregory Piatetsky-Shapiro, and Padhraic Smyth Copyright © 1996, American Association for Artificial Intelligence. All rights reserved. 0738-4602-1996 / $2.00areas is astronomy. Here, a notable success was achieved by SKICAT ,a system used by as-tronomers to perform image analysis,classification, and cataloging of sky objects from sky-survey images (Fayyad, Djorgovski,and Weir 1996). In its first application, the system was used to process the 3 terabytes (1012bytes) of image data resulting from the Second Palomar Observatory Sky Survey,where it is estimated that on the order of 109sky objects are detectable. SKICAT can outper-form humans and traditional computational techniques in classifying faint sky objects. See Fayyad, Haussler, and Stolorz (1996) for a sur-vey of scientific applications.In business, main KDD application areas includes marketing, finance (especially in-vestment), fraud detection, manufacturing,telecommunications, and Internet agents.Marketing:In marketing, the primary ap-plication is database marketing systems,which analyze customer databases to identify different customer groups and forecast their behavior. Business Week (Berry 1994) estimat-ed that over half of all retailers are using or planning to use database marketing, and those who do use it have good results; for ex-ample, American Express reports a 10- to 15-percent increase in credit-card use. Another notable marketing application is market-bas-ket analysis (Agrawal et al. 1996) systems,which find patterns such as, “If customer bought X, he/she is also likely to buy Y and Z.” Such patterns are valuable to retailers.Investment: Numerous companies use da-ta mining for investment, but most do not describe their systems. One exception is LBS Capital Management. Its system uses expert systems, neural nets, and genetic algorithms to manage portfolios totaling $600 million;since its start in 1993, the system has outper-formed the broad stock market (Hall, Mani,and Barr 1996).Fraud detection: HNC Falcon and Nestor PRISM systems are used for monitoring credit-card fraud, watching over millions of ac-counts. The FAIS system (Senator et al. 1995),from the U.S. Treasury Financial Crimes En-forcement Network, is used to identify finan-cial transactions that might indicate money-laundering activity.Manufacturing: The CASSIOPEE trou-bleshooting system, developed as part of a joint venture between General Electric and SNECMA, was applied by three major Euro-pean airlines to diagnose and predict prob-lems for the Boeing 737. To derive families of faults, clustering methods are used. CASSIOPEE received the European first prize for innova-intimately familiar with the data and serving as an interface between the data and the users and products.For these (and many other) applications,this form of manual probing of a data set is slow, expensive, and highly subjective. In fact, as data volumes grow dramatically, this type of manual data analysis is becoming completely impractical in many domains.Databases are increasing in size in two ways:(1) the number N of records or objects in the database and (2) the number d of fields or at-tributes to an object. Databases containing on the order of N = 109objects are becoming in-creasingly common, for example, in the as-tronomical sciences. Similarly, the number of fields d can easily be on the order of 102or even 103, for example, in medical diagnostic applications. Who could be expected to di-gest millions of records, each having tens or hundreds of fields? We believe that this job is certainly not one for humans; hence, analysis work needs to be automated, at least partially.The need to scale up human analysis capa-bilities to handling the large number of bytes that we can collect is both economic and sci-entific. Businesses use data to gain competi-tive advantage, increase efficiency, and pro-vide more valuable services to customers.Data we capture about our environment are the basic evidence we use to build theories and models of the universe we live in. Be-cause computers have enabled humans to gather more data than we can digest, it is on-ly natural to turn to computational tech-niques to help us unearth meaningful pat-terns and structures from the massive volumes of data. Hence, KDD is an attempt to address a problem that the digital informa-tion era made a fact of life for all of us: data overload.Data Mining and Knowledge Discovery in the Real WorldA large degree of the current interest in KDD is the result of the media interest surrounding successful KDD applications, for example, the focus articles within the last two years in Business Week , Newsweek , Byte , PC Week , and other large-circulation periodicals. Unfortu-nately, it is not always easy to separate fact from media hype. Nonetheless, several well-documented examples of successful systems can rightly be referred to as KDD applications and have been deployed in operational use on large-scale real-world problems in science and in business.In science, one of the primary applicationThere is an urgent need for a new generation of computation-al theories and tools toassist humans in extractinguseful information (knowledge)from the rapidly growing volumes ofdigital data.Articles38AI MAGAZINEtive applications (Manago and Auriol 1996).Telecommunications: The telecommuni-cations alarm-sequence analyzer (TASA) wasbuilt in cooperation with a manufacturer oftelecommunications equipment and threetelephone networks (Mannila, Toivonen, andVerkamo 1995). The system uses a novelframework for locating frequently occurringalarm episodes from the alarm stream andpresenting them as rules. Large sets of discov-ered rules can be explored with flexible infor-mation-retrieval tools supporting interactivityand iteration. In this way, TASA offers pruning,grouping, and ordering tools to refine the re-sults of a basic brute-force search for rules.Data cleaning: The MERGE-PURGE systemwas applied to the identification of duplicatewelfare claims (Hernandez and Stolfo 1995).It was used successfully on data from the Wel-fare Department of the State of Washington.In other areas, a well-publicized system isIBM’s ADVANCED SCOUT,a specialized data-min-ing system that helps National Basketball As-sociation (NBA) coaches organize and inter-pret data from NBA games (U.S. News 1995). ADVANCED SCOUT was used by several of the NBA teams in 1996, including the Seattle Su-personics, which reached the NBA finals.Finally, a novel and increasingly importanttype of discovery is one based on the use of in-telligent agents to navigate through an infor-mation-rich environment. Although the ideaof active triggers has long been analyzed in thedatabase field, really successful applications ofthis idea appeared only with the advent of theInternet. These systems ask the user to specifya profile of interest and search for related in-formation among a wide variety of public-do-main and proprietary sources. For example, FIREFLY is a personal music-recommendation agent: It asks a user his/her opinion of several music pieces and then suggests other music that the user might like (<http:// www.ffl/>). CRAYON(/>) allows users to create their own free newspaper (supported by ads); NEWSHOUND(<http://www. /hound/>) from the San Jose Mercury News and FARCAST(</> automatically search information from a wide variety of sources, including newspapers and wire services, and e-mail rele-vant documents directly to the user.These are just a few of the numerous suchsystems that use KDD techniques to automat-ically produce useful information from largemasses of raw data. See Piatetsky-Shapiro etal. (1996) for an overview of issues in devel-oping industrial KDD applications.Data Mining and KDDHistorically, the notion of finding useful pat-terns in data has been given a variety ofnames, including data mining, knowledge ex-traction, information discovery, informationharvesting, data archaeology, and data patternprocessing. The term data mining has mostlybeen used by statisticians, data analysts, andthe management information systems (MIS)communities. It has also gained popularity inthe database field. The phrase knowledge dis-covery in databases was coined at the first KDDworkshop in 1989 (Piatetsky-Shapiro 1991) toemphasize that knowledge is the end productof a data-driven discovery. It has been popular-ized in the AI and machine-learning fields.In our view, KDD refers to the overall pro-cess of discovering useful knowledge from da-ta, and data mining refers to a particular stepin this process. Data mining is the applicationof specific algorithms for extracting patternsfrom data. The distinction between the KDDprocess and the data-mining step (within theprocess) is a central point of this article. Theadditional steps in the KDD process, such asdata preparation, data selection, data cleaning,incorporation of appropriate prior knowledge,and proper interpretation of the results ofmining, are essential to ensure that usefulknowledge is derived from the data. Blind ap-plication of data-mining methods (rightly crit-icized as data dredging in the statistical litera-ture) can be a dangerous activity, easilyleading to the discovery of meaningless andinvalid patterns.The Interdisciplinary Nature of KDDKDD has evolved, and continues to evolve,from the intersection of research fields such asmachine learning, pattern recognition,databases, statistics, AI, knowledge acquisitionfor expert systems, data visualization, andhigh-performance computing. The unifyinggoal is extracting high-level knowledge fromlow-level data in the context of large data sets.The data-mining component of KDD cur-rently relies heavily on known techniquesfrom machine learning, pattern recognition,and statistics to find patterns from data in thedata-mining step of the KDD process. A natu-ral question is, How is KDD different from pat-tern recognition or machine learning (and re-lated fields)? The answer is that these fieldsprovide some of the data-mining methodsthat are used in the data-mining step of theKDD process. KDD focuses on the overall pro-cess of knowledge discovery from data, includ-ing how the data are stored and accessed, howalgorithms can be scaled to massive data setsThe basicproblemaddressed bythe KDDprocess isone ofmappinglow-leveldata intoother formsthat might bemorecompact,moreabstract,or moreuseful.ArticlesFALL 1996 39A driving force behind KDD is the database field (the second D in KDD). Indeed, the problem of effective data manipulation when data cannot fit in the main memory is of fun-damental importance to KDD. Database tech-niques for gaining efficient data access,grouping and ordering operations when ac-cessing data, and optimizing queries consti-tute the basics for scaling algorithms to larger data sets. Most data-mining algorithms from statistics, pattern recognition, and machine learning assume data are in the main memo-ry and pay no attention to how the algorithm breaks down if only limited views of the data are possible.A related field evolving from databases is data warehousing,which refers to the popular business trend of collecting and cleaning transactional data to make them available for online analysis and decision support. Data warehousing helps set the stage for KDD in two important ways: (1) data cleaning and (2)data access.Data cleaning: As organizations are forced to think about a unified logical view of the wide variety of data and databases they pos-sess, they have to address the issues of map-ping data to a single naming convention,uniformly representing and handling missing data, and handling noise and errors when possible.Data access: Uniform and well-defined methods must be created for accessing the da-ta and providing access paths to data that were historically difficult to get to (for exam-ple, stored offline).Once organizations and individuals have solved the problem of how to store and ac-cess their data, the natural next step is the question, What else do we do with all the da-ta? This is where opportunities for KDD natu-rally arise.A popular approach for analysis of data warehouses is called online analytical processing (OLAP), named for a set of principles pro-posed by Codd (1993). OLAP tools focus on providing multidimensional data analysis,which is superior to SQL in computing sum-maries and breakdowns along many dimen-sions. OLAP tools are targeted toward simpli-fying and supporting interactive data analysis,but the goal of KDD tools is to automate as much of the process as possible. Thus, KDD is a step beyond what is currently supported by most standard database systems.Basic DefinitionsKDD is the nontrivial process of identifying valid, novel, potentially useful, and ultimate-and still run efficiently, how results can be in-terpreted and visualized, and how the overall man-machine interaction can usefully be modeled and supported. The KDD process can be viewed as a multidisciplinary activity that encompasses techniques beyond the scope of any one particular discipline such as machine learning. In this context, there are clear opportunities for other fields of AI (be-sides machine learning) to contribute to KDD. KDD places a special emphasis on find-ing understandable patterns that can be inter-preted as useful or interesting knowledge.Thus, for example, neural networks, although a powerful modeling tool, are relatively difficult to understand compared to decision trees. KDD also emphasizes scaling and ro-bustness properties of modeling algorithms for large noisy data sets.Related AI research fields include machine discovery, which targets the discovery of em-pirical laws from observation and experimen-tation (Shrager and Langley 1990) (see Kloes-gen and Zytkow [1996] for a glossary of terms common to KDD and machine discovery),and causal modeling for the inference of causal models from data (Spirtes, Glymour,and Scheines 1993). Statistics in particular has much in common with KDD (see Elder and Pregibon [1996] and Glymour et al.[1996] for a more detailed discussion of this synergy). Knowledge discovery from data is fundamentally a statistical endeavor. Statistics provides a language and framework for quan-tifying the uncertainty that results when one tries to infer general patterns from a particu-lar sample of an overall population. As men-tioned earlier, the term data mining has had negative connotations in statistics since the 1960s when computer-based data analysis techniques were first introduced. The concern arose because if one searches long enough in any data set (even randomly generated data),one can find patterns that appear to be statis-tically significant but, in fact, are not. Clearly,this issue is of fundamental importance to KDD. Substantial progress has been made in recent years in understanding such issues in statistics. Much of this work is of direct rele-vance to KDD. Thus, data mining is a legiti-mate activity as long as one understands how to do it correctly; data mining carried out poorly (without regard to the statistical as-pects of the problem) is to be avoided. KDD can also be viewed as encompassing a broader view of modeling than statistics. KDD aims to provide tools to automate (to the degree pos-sible) the entire process of data analysis and the statistician’s “art” of hypothesis selection.Data mining is a step in the KDD process that consists of ap-plying data analysis and discovery al-gorithms that produce a par-ticular enu-meration ofpatterns (or models)over the data.Articles40AI MAGAZINEly understandable patterns in data (Fayyad, Piatetsky-Shapiro, and Smyth 1996).Here, data are a set of facts (for example, cases in a database), and pattern is an expres-sion in some language describing a subset of the data or a model applicable to the subset. Hence, in our usage here, extracting a pattern also designates fitting a model to data; find-ing structure from data; or, in general, mak-ing any high-level description of a set of data. The term process implies that KDD comprises many steps, which involve data preparation, search for patterns, knowledge evaluation, and refinement, all repeated in multiple itera-tions. By nontrivial, we mean that some search or inference is involved; that is, it is not a straightforward computation of predefined quantities like computing the av-erage value of a set of numbers.The discovered patterns should be valid on new data with some degree of certainty. We also want patterns to be novel (at least to the system and preferably to the user) and poten-tially useful, that is, lead to some benefit to the user or task. Finally, the patterns should be understandable, if not immediately then after some postprocessing.The previous discussion implies that we can define quantitative measures for evaluating extracted patterns. In many cases, it is possi-ble to define measures of certainty (for exam-ple, estimated prediction accuracy on new data) or utility (for example, gain, perhaps indollars saved because of better predictions orspeedup in response time of a system). No-tions such as novelty and understandabilityare much more subjective. In certain contexts,understandability can be estimated by sim-plicity (for example, the number of bits to de-scribe a pattern). An important notion, calledinterestingness(for example, see Silberschatzand Tuzhilin [1995] and Piatetsky-Shapiro andMatheus [1994]), is usually taken as an overallmeasure of pattern value, combining validity,novelty, usefulness, and simplicity. Interest-ingness functions can be defined explicitly orcan be manifested implicitly through an or-dering placed by the KDD system on the dis-covered patterns or models.Given these notions, we can consider apattern to be knowledge if it exceeds some in-terestingness threshold, which is by nomeans an attempt to define knowledge in thephilosophical or even the popular view. As amatter of fact, knowledge in this definition ispurely user oriented and domain specific andis determined by whatever functions andthresholds the user chooses.Data mining is a step in the KDD processthat consists of applying data analysis anddiscovery algorithms that, under acceptablecomputational efficiency limitations, pro-duce a particular enumeration of patterns (ormodels) over the data. Note that the space ofArticlesFALL 1996 41Figure 1. An Overview of the Steps That Compose the KDD Process.methods, the effective number of variables under consideration can be reduced, or in-variant representations for the data can be found.Fifth is matching the goals of the KDD pro-cess (step 1) to a particular data-mining method. For example, summarization, clas-sification, regression, clustering, and so on,are described later as well as in Fayyad, Piatet-sky-Shapiro, and Smyth (1996).Sixth is exploratory analysis and model and hypothesis selection: choosing the data-mining algorithm(s) and selecting method(s)to be used for searching for data patterns.This process includes deciding which models and parameters might be appropriate (for ex-ample, models of categorical data are differ-ent than models of vectors over the reals) and matching a particular data-mining method with the overall criteria of the KDD process (for example, the end user might be more in-terested in understanding the model than its predictive capabilities).Seventh is data mining: searching for pat-terns of interest in a particular representa-tional form or a set of such representations,including classification rules or trees, regres-sion, and clustering. The user can significant-ly aid the data-mining method by correctly performing the preceding steps.Eighth is interpreting mined patterns, pos-sibly returning to any of steps 1 through 7 for further iteration. This step can also involve visualization of the extracted patterns and models or visualization of the data given the extracted models.Ninth is acting on the discovered knowl-edge: using the knowledge directly, incorpo-rating the knowledge into another system for further action, or simply documenting it and reporting it to interested parties. This process also includes checking for and resolving po-tential conflicts with previously believed (or extracted) knowledge.The KDD process can involve significant iteration and can contain loops between any two steps. The basic flow of steps (al-though not the potential multitude of itera-tions and loops) is illustrated in figure 1.Most previous work on KDD has focused on step 7, the data mining. However, the other steps are as important (and probably more so) for the successful application of KDD in practice. Having defined the basic notions and introduced the KDD process, we now focus on the data-mining component,which has, by far, received the most atten-tion in the literature.patterns is often infinite, and the enumera-tion of patterns involves some form of search in this space. Practical computational constraints place severe limits on the sub-space that can be explored by a data-mining algorithm.The KDD process involves using the database along with any required selection,preprocessing, subsampling, and transforma-tions of it; applying data-mining methods (algorithms) to enumerate patterns from it;and evaluating the products of data mining to identify the subset of the enumerated pat-terns deemed knowledge. The data-mining component of the KDD process is concerned with the algorithmic means by which pat-terns are extracted and enumerated from da-ta. The overall KDD process (figure 1) in-cludes the evaluation and possible interpretation of the mined patterns to de-termine which patterns can be considered new knowledge. The KDD process also in-cludes all the additional steps described in the next section.The notion of an overall user-driven pro-cess is not unique to KDD: analogous propos-als have been put forward both in statistics (Hand 1994) and in machine learning (Brod-ley and Smyth 1996).The KDD ProcessThe KDD process is interactive and iterative,involving numerous steps with many deci-sions made by the user. Brachman and Anand (1996) give a practical view of the KDD pro-cess, emphasizing the interactive nature of the process. Here, we broadly outline some of its basic steps:First is developing an understanding of the application domain and the relevant prior knowledge and identifying the goal of the KDD process from the customer’s viewpoint.Second is creating a target data set: select-ing a data set, or focusing on a subset of vari-ables or data samples, on which discovery is to be performed.Third is data cleaning and preprocessing.Basic operations include removing noise if appropriate, collecting the necessary informa-tion to model or account for noise, deciding on strategies for handling missing data fields,and accounting for time-sequence informa-tion and known changes.Fourth is data reduction and projection:finding useful features to represent the data depending on the goal of the task. With di-mensionality reduction or transformationArticles42AI MAGAZINEThe Data-Mining Stepof the KDD ProcessThe data-mining component of the KDD pro-cess often involves repeated iterative applica-tion of particular data-mining methods. This section presents an overview of the primary goals of data mining, a description of the methods used to address these goals, and a brief description of the data-mining algo-rithms that incorporate these methods.The knowledge discovery goals are defined by the intended use of the system. We can distinguish two types of goals: (1) verification and (2) discovery. With verification,the sys-tem is limited to verifying the user’s hypothe-sis. With discovery,the system autonomously finds new patterns. We further subdivide the discovery goal into prediction,where the sys-tem finds patterns for predicting the future behavior of some entities, and description, where the system finds patterns for presenta-tion to a user in a human-understandableform. In this article, we are primarily con-cerned with discovery-oriented data mining.Data mining involves fitting models to, or determining patterns from, observed data. The fitted models play the role of inferred knowledge: Whether the models reflect useful or interesting knowledge is part of the over-all, interactive KDD process where subjective human judgment is typically required. Two primary mathematical formalisms are used in model fitting: (1) statistical and (2) logical. The statistical approach allows for nondeter-ministic effects in the model, whereas a logi-cal model is purely deterministic. We focus primarily on the statistical approach to data mining, which tends to be the most widely used basis for practical data-mining applica-tions given the typical presence of uncertain-ty in real-world data-generating processes.Most data-mining methods are based on tried and tested techniques from machine learning, pattern recognition, and statistics: classification, clustering, regression, and so on. The array of different algorithms under each of these headings can often be bewilder-ing to both the novice and the experienced data analyst. It should be emphasized that of the many data-mining methods advertised in the literature, there are really only a few fun-damental techniques. The actual underlying model representation being used by a particu-lar method typically comes from a composi-tion of a small number of well-known op-tions: polynomials, splines, kernel and basis functions, threshold-Boolean functions, and so on. Thus, algorithms tend to differ primar-ily in the goodness-of-fit criterion used toevaluate model fit or in the search methodused to find a good fit.In our brief overview of data-mining meth-ods, we try in particular to convey the notionthat most (if not all) methods can be viewedas extensions or hybrids of a few basic tech-niques and principles. We first discuss the pri-mary methods of data mining and then showthat the data- mining methods can be viewedas consisting of three primary algorithmiccomponents: (1) model representation, (2)model evaluation, and (3) search. In the dis-cussion of KDD and data-mining methods,we use a simple example to make some of thenotions more concrete. Figure 2 shows a sim-ple two-dimensional artificial data set consist-ing of 23 cases. Each point on the graph rep-resents a person who has been given a loanby a particular bank at some time in the past.The horizontal axis represents the income ofthe person; the vertical axis represents the to-tal personal debt of the person (mortgage, carpayments, and so on). The data have beenclassified into two classes: (1) the x’s repre-sent persons who have defaulted on theirloans and (2) the o’s represent persons whoseloans are in good status with the bank. Thus,this simple artificial data set could represent ahistorical data set that can contain usefulknowledge from the point of view of thebank making the loans. Note that in actualKDD applications, there are typically manymore dimensions (as many as several hun-dreds) and many more data points (manythousands or even millions).ArticlesFALL 1996 43Figure 2. A Simple Data Set with Two Classes Used for Illustrative Purposes.。
On Sequential Monte Carlo Sampling Methods for Bayesian Filtering
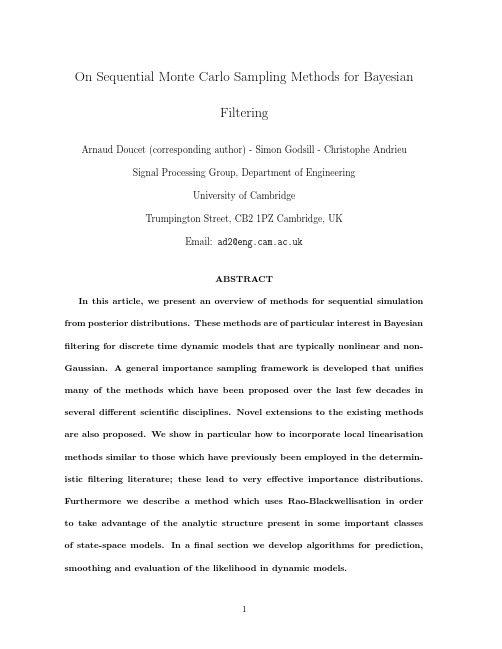
methods, see (Akashi et al., 1975)(Handschin et. al, 1969)(Handschin 1970)(Zaritskii et al., 1975). Possibly owing to the severe computational limitations of the time these Monte Carlo algorithms have been largely neglected until recently. In the late 80’s, massive increases in computational power allowed the rebirth of numerical integration methods for Bayesian filtering (Kitagawa 1987). Current research has now focused on MC integration methods, which have the great advantage of not being subject to the assumption of linearity or Gaussianity in the model, and relevant work includes (M¨ uller 1992)(West, 1993)(Gordon et al., 1993)(Kong et al., 1994)(Liu et al., 1998). The main objective of this article is to include in a unified framework many old and more recent algorithms proposed independently in a number of applied science areas. Both (Liu et al., 1998) and (Doucet, 1997) (Doucet, 1998) underline the central rˆ ole of sequential importance sampling in Bayesian filtering. However, contrary to (Liu et al., 1998) which emphasizes the use of hybrid schemes combining elements of importance sampling with Markov Chain Monte Carlo (MCMC), we focus here on computationally cheaper alternatives. We describe also how it is possible to improve current existing methods via Rao-Blackwellisation for a useful class of dynamic models. Finally, we show how to extend these methods to compute the prediction and fixed-interval smoothing distributions as well as the likelihood. The paper is organised as follows. In section 2, we briefly review the Bayesian filtering problem and classical Bayesian importance sampling is proposed for its solution. We then present a sequential version of this method which allows us to obtain a general recursive MC filter: the sequential importance sampling (SIS) filter. Under a criterion of minimum conditional variance of the importance weights, we obtain the optimal importance function for this method. Unfortunately, for numerous models of applied interest the optimal importance function leads to non-analytic importance weights, and hence we propose several suboptimal distributions and show how to obtain as special cases many of the algorithms presented in the literature. Firstly we consider local linearisation methods of either the state space model 3
SIMATIC Energy Manager PRO V7.2 - Operation Operat
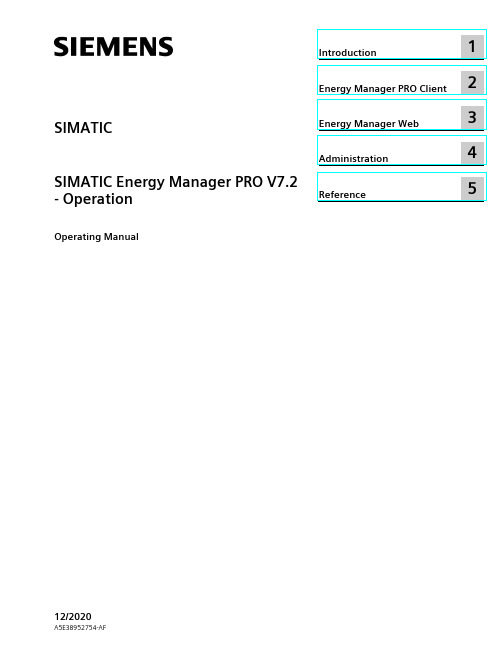
2 Energy Manager PRO Client................................................................................................................. 19
2.1 2.1.1 2.1.2 2.1.3 2.1.4 2.1.5 2.1.5.1 2.1.5.2 2.1.6
Basics ................................................................................................................................ 19 Start Energy Manager ........................................................................................................ 19 Client as navigation tool..................................................................................................... 23 Basic configuration ............................................................................................................ 25 Search for object................................................................................................................ 31 Quicklinks.......................................................................................................................... 33 Create Quicklinks ............................................................................................................... 33 Editing Quicklinks .............................................................................................................. 35 Help .................................................................................................................................. 38
窄线赛弗特I型星系中可能具有吸积盘热辐射起源的软X射线超

窄线赛弗特I型星系中可能具有吸积盘热辐射起源的软X射线超李晔【摘要】理论上,低质量高吸积率活动星系核的标准吸积盘黑体热辐射有可能形成软X射线波段的超出.然而,活动星系核的软X射线超温度为0.1 keV左右,显著高于标准吸积盘理论预言的最高有效温度.只有Yuan等人在2010年报道的RXJ1633+4718是一个例外,其软超温度为32.5±-8.06.0eV,显著小于0.1 keV,并与吸积盘理论预言的温度相符;此外,其光度也符合吸积盘的理论预期.因此该软X射线超很可能起源于吸积盘的热辐射.这一类源对于吸积盘理论和软X射线超的研究都有重要意义.文中使用ROSAT PSPC数据,在所有已知的窄线赛弗特I型星系(Narrow Line Seyfert I galaxies,NLSIs)中寻找类似于RXJ1633+4718的源,即软X射线超可能起源于吸积盘热辐射的源.分析了150个源的245条光谱,其中58个源的90条光谱具有显著的软X射线超.样本中软X射线超温度分布的平均值-Tsoft为0.10 keV,标准偏差为0.03 keV.除了RX J1633+4718外,只有3个源的软X射线超温度小于60 eV,而且温度接近吸积盘最高温度.这个结果表明,类似于RX J1633+4718的具有低温软X射线超的源很稀少.最后,在具有黑洞质量估计的26个源中,软超温度与黑洞质量MBH、爱丁顿比L/LEdd及预期吸积盘最高温度Tmax都没有明显的关联性,与前人的结果一致.%It is theoretically expected that the thermal radiation from accretion disks of Active Galactic Nuclei (AGNs) with low black hole mass would contribute to the soft X-ray band and result in an excess emission. However, the temperatures of the observed soft X-ray excess of AGNs are typically around 0.1 keV, significantly higher than those predicted by the standard disk model. There is only oneexception found so far, R.X J1633+4718, which has a significantly low soft excess temperature of 32.5l+8.0-6.0 eV, consistent with the maximum temperature as well as the luminosity of the predicted disk emission(Yuan et al. 2010).rnIn this paper, we search for RX J1633 like AGNs, whose soft X-ray excess temperature is close to the maximum temperature of accretion disk, in all known Narrow Line Seyfert I galaxies (NLSIs) observed with ROSAT PSPC in the archive. We analyzed the PSPC spectra of 150 NLSIs (245 observations) in total, 58 (90 observations) of which show significantly soft X-ray excess emission. In addition to RX J1633+4718, only 3 objects (4 observations) show soft X-ray excess temperatures less than 60 eV and close to the theoretical prediction of the disk emission. They form a sample of candidates for future follow-up studies. Our result indicates that RX J1633+4718 like objects are very rare. The correlations between the temperature of the soft X-ray excess and black hole mass as well as the Eddington ratio are also investigated, and no correlation is found. This confirms the previous results, and implies that the soft X-ray excess of most AGNs should originate from other mechanisms rather than the thermal emission of the accretion disk.【期刊名称】《天文学进展》【年(卷),期】2013(031)001【总页数】14页(P110-123)【关键词】活动星系核;吸积盘X射线热辐射;软X射线超【作者】李晔【作者单位】中国科学院国家天文台/云南天文台,昆明650011;中国科学院大学,北京100049;中国科学院国家天文台,北京100012【正文语种】中文【中图分类】P157.6活动星系核中心存在超大质量黑洞,物质落入中心黑洞的过程中形成吸积流,并产生辐射[1-3]。
关于非newton渗流方程解的存在性的研究
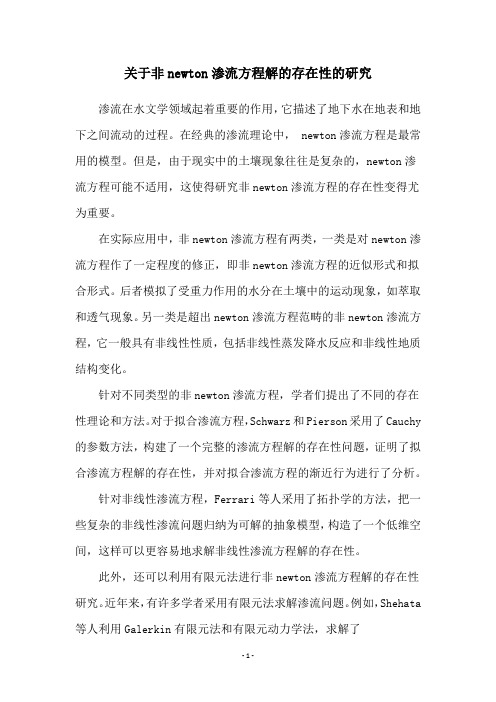
关于非newton渗流方程解的存在性的研究渗流在水文学领域起着重要的作用,它描述了地下水在地表和地下之间流动的过程。
在经典的渗流理论中, newton渗流方程是最常用的模型。
但是,由于现实中的土壤现象往往是复杂的,newton渗流方程可能不适用,这使得研究非newton渗流方程的存在性变得尤为重要。
在实际应用中,非newton渗流方程有两类,一类是对newton渗流方程作了一定程度的修正,即非newton渗流方程的近似形式和拟合形式。
后者模拟了受重力作用的水分在土壤中的运动现象,如萃取和透气现象。
另一类是超出newton渗流方程范畴的非newton渗流方程,它一般具有非线性性质,包括非线性蒸发降水反应和非线性地质结构变化。
针对不同类型的非newton渗流方程,学者们提出了不同的存在性理论和方法。
对于拟合渗流方程,Schwarz和Pierson采用了Cauchy 的参数方法,构建了一个完整的渗流方程解的存在性问题,证明了拟合渗流方程解的存在性,并对拟合渗流方程的渐近行为进行了分析。
针对非线性渗流方程,Ferrari等人采用了拓扑学的方法,把一些复杂的非线性渗流问题归纳为可解的抽象模型,构造了一个低维空间,这样可以更容易地求解非线性渗流方程解的存在性。
此外,还可以利用有限元法进行非newton渗流方程解的存在性研究。
近年来,有许多学者采用有限元法求解渗流问题。
例如,Shehata 等人利用Galerkin有限元法和有限元动力学法,求解了mixed-effects渗流方程解,取得了很好的结果。
最后,学者们还可以利用蒙特卡罗技术来研究非newton渗流方程解的存在性。
蒙特卡罗法可以有效地模拟地下水的流动和扩散,可以找出非newton渗流方程解。
总结而言,存在性研究是解决渗流问题的基本方法,它是一种有效的非newton渗流方程解的求解方法。
虽然存在不同的存在性理论和方法,但它们的目的是一致的,即解决非newton渗流方程解的存在性问题。
土体邓肯—张非线性弹性模型参数反演分析

土体邓肯—张非线性弹性模型参数反演分析近年来,随着土体力学的发展,研究者开始关注土体的非线性弹性以及参数的反演分析,其中土体邓肯张非线性弹性模型参数反演分析已经得到了广泛的应用,成为处理土体非线性弹性问题的一种重要方法。
本文通过对土体邓肯张非线性弹性模型参数反演分析的原理,研究方法及应用进行综述,目的在于为土体非线性弹性问题的研究提供理论参考。
一、体邓肯张非线性弹性模型参数反演分析简介土体邓肯张非线性弹性模型运用反演分析的方法,可以从实验数据中反推出土体的非线性弹性参数。
它是土体力学非线性反演理论的基础模型,可以作为处理土体非线性弹性问题的理论依据。
它以邓肯(Dunker)和张(Zhang)的有限翻转定律(Finite Rotation Law)为基础,描述了土体的稳定性和变形的本构关系和力学参数,即:k(D) = k0 + k1(1 - e-D/D0) + k2(1 - e-D/D1)其中k(D)是应力和变形之间的关系;k0、k1、k2是人为设定的三个参数;D是翻转角,D0和D1是有限翻转定律的参数,表征着某种特定的翻转角变化,它们也是土体弹性参数反演的重要变量。
二、土体邓肯张非线性弹性模型参数反演分析的研究方法土体邓肯张非线性弹性模型参数反演分析的研究方法,就是从实验数据中反推出土体的非线性弹性参数。
实验数据包括土体的曲线拟合数据,以及沿此曲线拟合数据点处的单点变形试验中的翻转角和应力数据。
通过使用标准的数值拟合算法,对上述实验数据进行处理,可以确定三个参数,即k0、k1、k2。
三、体邓肯张非线性弹性模型参数反演分析的应用土体邓肯张非线性弹性模型参数反演分析在土体力学研究中有着重要的应用,包括但不限于:(1)在不完全的数据中,可以运用反演分析的方法得出土体的本构参数。
(2)在岩土受力过程中,利用反演分析的方法可以获得土体的弹性参数,从而得出岩土的变形特性。
(3)在工程设计中,可以采用反演分析的方法来进行岩土弹性参数的确定,从而优化岩土层的设计。
- 1、下载文档前请自行甄别文档内容的完整性,平台不提供额外的编辑、内容补充、找答案等附加服务。
- 2、"仅部分预览"的文档,不可在线预览部分如存在完整性等问题,可反馈申请退款(可完整预览的文档不适用该条件!)。
- 3、如文档侵犯您的权益,请联系客服反馈,我们会尽快为您处理(人工客服工作时间:9:00-18:30)。
a r X i v :a s t r o -p h /0011205v 1 9 N o v 2000A&A manuscript no.(will be inserted by hand later)ASTRONOMYANDASTROPHY SICS1.IntroductionLMC X-3(Leong et al.1971)is a persistent X-ray source in the Large Magellanic Cloud (LMC).It has an orbital period of 1.70d,and a mass function ≃2.3M ⊙(Cowley et al.1983).The optical brightness of V ∼17indicates that the system has a massive companion (van Paradijs et al.1987).The companion is often classified as a B3V star (Cowley et al.1983),although a B5IV spectral type has also been suggested (Soria et al.2001).The non-detection of eclipses in the X-ray curve implies that the orbital incli-nation of the system is <∼70◦(Cowley et al.1983).The inferred mass of the compact star is >∼7M ⊙(Paczynski 1983),thus establishing that the system is a black-hole candidate (BHC).Most BHCs show soft and hard X-ray spectral states.Their X-ray spectrum in the soft state generally consists of both a thermal and a power-law component.The ther-mal component can be fitted by a blackbody spectrum2K.Wu et al.:EPIC and RGS observations of LMC X-3Table1.XMM-Newton EPIC Observation LogRevolution/ID Instrument a Exposure count rate bRev0028/201RGS2(s1)17.6ks 4.79±0.02 Rev0030/501RGS2(tot)12.1ks 4.52±0.03 Rev0045/101RGS1(s1)06.4ks 3.59±0.03RGS2(s1)06.4ks 3.56±0.03 Rev0045/201RGS1(s1)06.7ks 3.62±0.02RGS2(s1)06.8ks 3.57±0.02 Rev0045/301RGS1(s1)03.0ks 3.66±0.04RGS1(s3)03.0ks 3.57±0.04RGS1(s5)03.0ks 3.40±0.04RGS2(s2)00.3ks 3.63±0.17RGS2(s4)03.0ks 3.26±0.04RGS2(s6)00.3ks 3.18±0.17 Rev0066/101RGS1(s7)44.3ks0.0154±0.0012RGS2(s8)44.3ks0.0153±0.0012 Rev0092/101RGS1(s2)51.9ks 1.32±0.01RGS2(s2)51.9ks 1.53±0.01 Rev0092/201RGS1(s4)25.6ks 1.56±0.01RGS2(s5)25.6ks 1.34±0.01a The labels“s#”identifies multiple exposures during the same revolution;for more details see the Preferred Observation Sequencefiles of each revolution.b In photons s−1MOS1/PN and RGS observations used for this study are shown in Tables1and2respectively.The EPIC-MOS2 observations are not presented,as a reliable response ma-trix is not yet available.The exposure times listed in Table 1take into account the counting mode interruptions.In some cases,the fraction of live time during which pho-tons were actually collected is significantly smaller than the total exposure time.All EPIC exposures were taken with the“medium”fil-ter;Some of the data suffer from pile-up when the source was observed in the bright state(before Rev0066).MOS exposures taken in the“full window”mode are worst af-fected,and so are excluded from the analysis.“Partial window”MOS exposures and“full window”PN exposures are,however,less affected.In our analysis,we have re-moved the central pixels(which are most affected by pile-up)from the extraction regions,for the partial MOS and full PN exposures that are affected.The corresponding normalisations of thefitted spectral models for these ob-servations are therefore smaller than the true values,and they are put in brackets in Table3.We are aware that the photon index of the power-law component is affected by pile-up.For the Rev0028,0030,0041and0045observa-tions,we estimate that thefitted values are≈0.15–0.20 smaller(harder)than the real value.The“small window”PN exposures are not significantly affected by pile up,and they are more reliable for the determination of the power-law photon indices.The data were processed using the September2000re-lease of the SAS,except for the PN small window mode data,which were processed with the October2000SAS re-lease.All spectralfits presented here were performed with the most up-to-date response matrices available at the end of October2000(mos1all rmf315.rsp for EPIC-MOS1and epn sY9K.Wu et al.:EPIC and RGS observations of LMC X-33et al.2000).The system seemed to be in the process of returning to the soft state in the Rev0092(June9–10) observations.2.3.EPIC MOS and PN dataWe consider a conventional model consisting of an ab-sorbed multi-colour disk blackbody plus a power law, wabs*zvfeabs*(diskbb+powerlaw)in XSPEC,tofit the EPIC data.As thefitting process is limited by the re-liability of the response matrices currently available,more sophisticated models or the inclusion of additional features (such as lines)are not warranted.The generic models used here are therefore sufficient for this preliminary analysis.For the MOS observations,we consider only the chan-nels in the0.3–8.0keV energy range;for the PN obser-vations we extend the energy range to0.3–12.0keV.The data are binned with the FTOOLS task grppha,such that the number of counts in each group of channels is larger than40.This improves the signal-to-noise ratio for ener-gies>∼6keV,while it does not affect low-energy channels. We have also checked that different binning criteria give consistent results.No systematic error has been added to the data.We consider the line-of-sight Galactic absorption to-ward LMC X-3to be3.2×1020cm−2following Wilms et al. (2000),and use it as the value for the(fixed)first absorp-tion component.The second absorption component is then the intrinsic photoelectric absorption within the binary system and the LMC.We alsofix both the iron abundance and the total metal abundance to be0.4times the solar values,to account for the lower metallicity of the LMC (Caputo et al.1999).The bestfit parameters are shown in Table3.Figures2,3and4show the EPIC-PN spectra from the Rev0028/201,Rev0066/101and Rev0092/201 observations respectively(all taken in small window mode, not affected by pile-up).The reducedχ2νare about one in mostfits.The largeχ2νin somefits(e.g.the MOS1observations in Rev0092/201)are probably due to the uncertain response and effective-area calibrations.In particular,the feature seen at≈0.5keV in the PN spectra(Figures2,3,4)is due to uncertainties in the charge transfer efficiency correction for the small window mode.Values ofχ2ν≤1.05are ob-tained when the0.4–0.6keV energy range is excluded from thefit.2.4.RGS dataWe consider the RGS data to search for possible emission lines,and to constrain the value of the column density.The RGS spectra arefitted with the same model used in the analysis of the PN data.As the RGS spectra cover only the 0.35−2.5keV energy range,the thermal and the power-law components cannot be constrained simultaneously.We thereforefixΓto the values determined from the EPICfits (with a correction to the value ofΓfrom Rev0045to take the pile-up into account).Because of the low count rate, the data in the Rev0066observations are binned in groups of100channels each.The error-weighted means of thefit values of T in are0.52keV and0.29keV for observations before and after Rev0066respectively.We do notfind strong evidence of emission lines.The column density n H was generally below1021cm−2,ex-cept for Rev0066,where n H is not well constrained.After the line-of-sight column density to the LMC is subtracted, the value of the column density for the observations be-fore Rev0066is(5.50±0.67)×1020cm−2,and the value after Rev0066is(6.83±0.58)×1020cm−2.(These val-ues are smaller than those obtained from thefits to the EPIC data.)Given that the error may be dominated by systematic effects,the error obtained in thefits may not truly represent the statistical weights of thefit parame-ters.Apart from the conclusion that the spectra do not show strong column absorption,we are unable to deter-mine whether the variations in n H are correlated with the X-ray luminosity.3.Discussion3.1.Soft and hard statesPrevious RXTE/PCA observations(Wilms et al.2000) have shown that when the source was bright(with RXTE/ASM count rates>∼3ct s−1),the disk black-body component was prominent,and itsfit temperature T in≈1.0keV.There was also a power-law tail with Γ≈4in the spectra.The temperature T in appeared to de-crease when the X-ray luminosity decreased,while the nor-malisation parameter A disk remained approximately con-stant.When the RXTE/ASM count rate dropped below ≈0.6ct s−1,T in was reduced to≈0.7keV.The transi-tion was accompanied by the hardening of the power-law component.The photon indexΓbecame∼2.0–3.0.When the RXTE/ASM count rate decreased below the0.3-ct s−1 level,the disk blackbody component was not detected,and the spectrum was a power law withΓ=1.8.The soft-to-hard transition was also seen in our data. The spectra obtained in the Rev0028–Rev0045obser-vations show a disk blackbody component with T in∼0.6–1.0,and a power-law component withΓ≈2.5. The RXTE/ASM count rate was below2ct s−1during the observations.The spectral properties are similar to those observed previously when the system had similar RXTE/ASM count rates.The spectrum obtained in the Rev0066/101observa-tions is dominated by a power law withΓ=1.9±0.1. The photon index is consistent with that observed in the previous hard states(Wilms et al.2000),while a lower value of1.60±0.04was obtained by Boyd et al.(2000)on 2000May7.Theχ2of thefit which includes a thermal disk black-body component(bestfit T in=0.14keV)is4K.Wu et al.:EPIC and RGS observations of LMC X-3Table3.Fits to the EPIC data:absorbed disk blackbody and power lawObservation a n H b T in A diskΓc A plχ2ν(dof)Rev0028/201RGS2(s1)4.37+1.37−0.35×10200.55+0.02−0.02183.8+17.1−15.62.70.00+0.25−0.00×10−2 1.38(485)Rev0045/101RGS1(s1)8.30+2.73−2.85×10200.63+0.07−0.0660.73+25.47−22.182.51.10+0.65−0.58×10−2 1.04(545)RGS2(s1)5.04+3.67−1.07×10200.49+0.03−0.03195.0+5.8−38.42.50.55+7.92−0.55×10−3 1.14(488)Rev0045/201RGS1(s1)8.13+2.63−2.78×10200.67+0.08−0.0651.16+22.14−19.012.51.06+0.62−0.56×10−2 1.08(543)RGS2(s1)7.57+3.71−2.92×10200.50+0.03−0.03178.5+23.7−40.92.54.32+9.3−4.32×10−3 1.05(484)Rev0045/301RGS1(s1)5.20+4.76−1.38×10200.53+0.05−0.05127.4+54.8−41.72.50.45+9.13−0.45×10−3 1.12(543)RGS1(s3)10.05+3.96−4.28×10200.70+0.18−0.1042.99+14.98−24.122.51.07+0.96−0.81×10−20.91(541)RGS1(s5)7.45+4.04−2.24×10200.53+0.06−0.04119.0+53.3−38.52.50.82+7.84−0.82×10−3 1.08(540)RGS2(s4)9.90+5.18−2.73×10200.45+0.03−0.03252.7+91.0−74.72.53.18+13.5−3.18×10−3 1.03(487)Rev0066/101RGS1(s7)0.00+7.77−0.00×10200.07+0.03−0.033311+4171−30561.92.77+0.20−0.20×10−4 1.11(44)Rev0092/101RGS1(s2)5.85+1.03−1.06×10200.33+0.01−0.02211.2+29.6−25.32.050.93+0.26−0.25×10−2 1.27(538)RGS2(s2)8.01+0.95−1.12×10200.27+0.02−0.02478.1+103.8−81.92.051.36+0.28−0.14×10−2 1.25(485)Rev0092/201RGS1(s4)6.40+1.35−1.45×10200.29+0.03−0.03252.0+83.9−52.92.051.06+0.28−0.31×10−2 1.08(537)RGS2(s5)6.84+1.19−1.28×10200.27+0.03−0.03500.0+65.1−52.72.050.99+0.09−0.10×10−2 1.15(485)a We do not showfit parameters for observations without enough counts or without a reliable energy calibration.We assumed a metallicity of0.4times the solar value,i.e.,Z=0.008b The Galactic line-of-sight absorption(=3.2×1020cm−2)has been subtracted.c Fixed.58.486for52degrees of freedom.If the thermal compo-nent is not included,then we obtainχ2=58.493for54 degrees of freedom.The thermal component is therefore insignificant.The system was at the transition from the hard state back to the soft state during the Rev0092observations (see Figure1).The power-law component had steepened, withΓ=2.05±0.02(for the PN data).The disk blackbody component reappeared,with a temperature T in≈0.2keV, significantly lower than that before Rev0066.In summary,between2000February and June LMC X-3underwent a transition from a soft to a hard state,and then in the process of returning to the soft state.During the soft-to-hard transition thefit temperature of the disk blackbody component decreased,and the power law com-ponent became harder.As the system started to return to the soft state,the disk blackbody component becameK.Wu et al.:EPIC and RGS observations of LMC X-35more prominent.The power law appeared to be steeper than that obtained from the RXTE/PCA observations near the middle of the faint state,yet the disk blackbody temperature was still well below the values of the previous soft state.3.2.Mode of mass transferThe RGS data constrain the value of n H within the sys-tem to be<∼1021cm−2,in contrast to the larger intrinsic column density expected for a companion with a strong stellar wind.The non-detection of obvious emission lines in the RGS spectra also indicates the absence of wind mat-ter ejected in previous epochs(cf.the P Cygni lines seen in Cir X-1,Brandt&Schulz2000).Thus,the XMM-Newton spectral data do not support the mass-transfer scenario for LMC X-3in which the black hole accretes matter mainly from a strong stellar wind from a massive companion.The high luminosity observed in the X-ray bands therefore re-quires the companion to overflow its Roche lobe.A similar conclusion is also obtained independently from an analysis of the optical/UV properties of the sys-tem(Soria et al.2001).The OM data obtained in the Rev0066suggest that the companion is a B5subgiant instead of a B3main-sequence star.Roughly3%of the X-rays would be intercepted by the companion,so that the rate at which energy is deposited into its atmosphere can be>∼5×1036erg s−1in the soft state.This rate is larger than the intrinsic luminosity of the companion. If the companion in LMC X-3is indeed a subgiant star its tenuous envelope is susceptible to irradiation heating. The soft-to-hard transitions seen in the RXTE observa-tions in1997/1998and the XMM-Newton observations in 2000may be caused by variations in the rate of mass over-flow from the Roche lobe of the subgiant companion.Wilms et al.(2000)’s interpretation of the decreases in the RXTE/ASM count rate as evidence for transitions from soft to hard state is consistent with our data.We further propose that the decrease in the X-ray luminosity is caused by the decrease in the fraction of the Roche lobefilled by the companion star.When the companion is detached from its critical Roche surface,mass transfer will be dominated by a focused wind.It is worth noting that the three known high-mass BHCs are all persistent X-ray sources which show pref-erential X-ray spectral states.While Cyg X-1tends to be in the hard state,LMC X-1and LMC X-3are more often found in the soft state.Recent studies(e.g.Igumenshchev, Illarionov&Abramowicz1999;Beloborodov&Illarionov 2000)have shown that accretion of matter with low angu-lar momentum will give rise to hard X-rays instead of soft X-rays.The relative angular momentum of the accreting matter is smaller for wind accretion than for Roche-lobe overflow.Cyg X-1has a33-M⊙O-type companion(Giles &Bolton1986),which has a strong stellar wind.The com-panion stars in LMC X-1and LMC X-3are less massive (<∼10M⊙)B stars,whose stellar wind is much weaker. Therefore,we suggest a unified scenario which relates the mode of mass transfer(wind or Roche-lobe overflow)to the spectral state preferentially observed in these three high-mass BHC binaries(hard or soft respectively).The subgiant companion of LMC X-3may occasionally under-fill its Roche lobe because of feedback irradiative processes or instabilities in its envelope.This leads to the residual accretion of the(focused)wind matter which has relatively low angular momentum.4.SummaryThe BHC LMC X-3was observed in2000February–June, with the XMM-Newton EPIC and RGS,throughout a soft-hard transition.The system was apparently in the process of returning to the soft state in2000June.The hard-state spectra are dominated by a power-law component with a photon indexΓ≈1.9.The soft-state spectra consist of a thermal component with an inner-disk temperature T in of≈0.9keV and a power-law tail withΓ≈2.5–2.7. The line-of-sight absorption deduced from the EPIC and RGS data is n H<∼1021cm−2.Our observations therefore do not support the wind accretion model for this system in the soft state.The transition from the soft to the hard state appears to be a smooth process associated with the changes in the mass-transfer rate.Acknowledgements.This work is based on observations ob-tained with XMM-Newton,an ESA science mission with in-struments and contributions directly funded by ESA member states and the USA(NASA).We thank Keith Mason for his comments.KW acknowledges a PPARC visiting fellowship. ReferencesBeloborodov,A.M.,Illarionov,A.,2000,astro-ph/0006351 Boyd,P.T.,Smale,A.P.,2000,IAUC7424Boyd,P.T.,Smale,A.P.,Homan,J.,Jonker,P.G.,van der Klis,M.,Kuulkers,E.,2000,ApJ,in pressBrandt,W.N.,Schulz,N.S.,2000,astro-ph/0007402 Brinkman,A.C.,et al.,2001,A&A,this volumeCaputo,M.,Marconi,G.,Ripepi,V.,1999,ApJ525,784 Cowley,A.P.,Crampton,D.,Hutching,J.B.,Remillard,R., Penfold,J.E.,1983,ApJ,272,118Ebisawa,K.et al.,1993,ApJ,403,684Giles,D.R.,Bolton,C.T.,1986,ApJ,304,371 Igumenshchev,I.G.,Illarionov,A.F.,Abramowicz,M.A., 1999,ApJ,517,L55Leong,C.,Kellogg,E.,Gursky,H.,Tanabaum,H.,Giaccon, R.,1971,ApJ,170,L67Mazeh,T.,van Paradijs,J.,van den Heuvel,E.P.J.,Savonije,G.J.,1986,A&A,157,113Mason,K.O.,et al.,2001,A&A,this volumePaczynski,B.,1983,ApJ,273,L81Paul,B.,Kitamoto,S.,Makino,F.,2000,ApJ,528,410 Soria,R.,Wu,K.,Page,M.,Sakelliou,I.,2001,A&A,this volumeSunyaev,R.A.,Titarchuk,L.G.,1980,A&A,86,1216K.Wu et al.:EPIC and RGS observations of LMC X-3Titarchuk,L.G.,Zannias,T.,1998,ApJ,193,863Turner,M.J.L.,et al.,2001,A&A,this volumevan Paradijs,J.et al.1987,A&A,104,201Wilms,J.,Nowak,M.A.,Pottschmidt,K.,Heindl,W.A.,Dove,J.B.,Begelman,M.C.,2000,astro-ph/0005489Fig.1.(First panel from the top):The XMM-NewtonEPIC observations of LMC X-3,marked by solid verticallines,are shown and compared with the RXTE/ASM lightcurve.The three RXTE/PCA observations that confirmedthe hard state of the system(Boyd et al.2000)are markedby dotted vertical lines.(Second panel):The0.35−2.5keVlight curve from the RGS observations.(Third panel):Theevolution of thefit temperature of the thermal compo-nent in the EPIC-PN and MOS spectra.The open cir-cles are the means of thefit disk-blackbody temperaturesT in error-weighted for the observations during the samerevolution.(Fourth panel):The evolution of the photonindexΓof the power-law tail.The data points(open cir-cles)are error-weighted means.The open squares are thephoton indices obtained by Boyd et al.(2000)from thethree RXTE/PCA observations on May5.76,10.01and13.94UT;a model with a single power law was used.K.Wu et al.:EPIC and RGS observations of LMC X-37Fig.2.The top panel shows the data and fit EPIC-PN spectra of the Rev0028/201observation (2000February 2).An absorbed diskbb+powerlaw model is used in the fit (see Table 3).The residuals are shown in the bottompanel.Fig.3.Same as Fig.2for the Rev0066/101EPIC-PN ob-servation (2000April19).Fig.4.Same as Fig.2for the Rev0092/201EPIC-PN ob-servation (2000June 10).。