Video-aware opportunistic network coding over wireless networks
基于深度学习的视频目标检测与跟踪算法研究
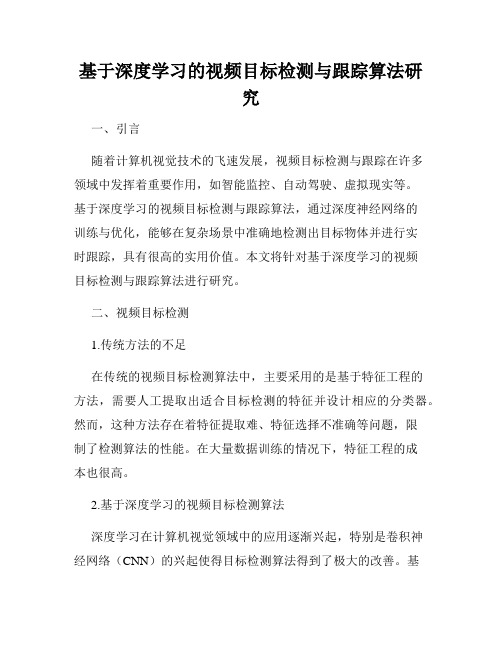
基于深度学习的视频目标检测与跟踪算法研究一、引言随着计算机视觉技术的飞速发展,视频目标检测与跟踪在许多领域中发挥着重要作用,如智能监控、自动驾驶、虚拟现实等。
基于深度学习的视频目标检测与跟踪算法,通过深度神经网络的训练与优化,能够在复杂场景中准确地检测出目标物体并进行实时跟踪,具有很高的实用价值。
本文将针对基于深度学习的视频目标检测与跟踪算法进行研究。
二、视频目标检测1.传统方法的不足在传统的视频目标检测算法中,主要采用的是基于特征工程的方法,需要人工提取出适合目标检测的特征并设计相应的分类器。
然而,这种方法存在着特征提取难、特征选择不准确等问题,限制了检测算法的性能。
在大量数据训练的情况下,特征工程的成本也很高。
2.基于深度学习的视频目标检测算法深度学习在计算机视觉领域中的应用逐渐兴起,特别是卷积神经网络(CNN)的兴起使得目标检测算法得到了极大的改善。
基于深度学习的视频目标检测算法主要通过训练一个深度神经网络,使其能够自动学习出目标物体的特征表示,并通过后续的分类器对目标进行识别与定位。
首先,深度神经网络通常包含多个卷积层和池化层,用于提取图像中不同层次的特征。
然后,通过全连接层将提取到的特征与标签进行匹配,训练网络模型。
最后,通过对新的图像样本进行前向传播,得到目标物体的识别和定位结果。
基于深度学习的视频目标检测算法相较于传统方法具有很多优势。
首先,深度神经网络能够自动学习出适用于目标检测的特征表达,避免了人工特征工程的复杂性。
其次,通过大规模数据的训练,深度学习模型能够提高目标检测的准确性和泛化能力。
此外,深度学习算法还能够处理大规模数据集,使得算法具备更好的实时性。
三、视频目标跟踪1.传统方法的不足传统的视频目标跟踪算法通常基于目标的运动模型或者外观模型,但是它们在面对复杂场景时往往表现不佳。
这是因为传统方法无法有效地应对目标形变、光照变化、遮挡等问题,且对复杂背景下的目标无法准确跟踪。
基于深度学习的视频人工智能分析与应用研究

基于深度学习的视频人工智能分析与应用研究摘要:随着深度学习技术的迅速发展,视频人工智能分析与应用领域也取得了显著的进展。
本文以深度学习为基础,探讨了视频人工智能分析的相关方法和应用研究,包括视频目标检测、视频行为识别、视频内容理解和视频编码等方面。
分析了深度学习在视频人工智能分析中的优势和挑战,并展望了未来的研究方向。
关键词:深度学习、视频人工智能、目标检测、行为识别、内容理解、编码1. 引言视频作为一种丰富多样的媒介形式,蕴含着大量的信息和价值。
然而,要从海量的视频数据中提取和理解有用的信息是一项复杂而具有挑战性的任务。
传统的视频分析方法在处理复杂场景和长时间序列视频时面临诸多限制。
而深度学习作为一种强大的模式识别和数据建模技术,已经在视频人工智能分析领域取得了长足的进展。
2. 深度学习在视频人工智能分析中的应用2.1 视频目标检测视频目标检测是指从视频中准确地识别和定位目标物体。
传统方法对于目标的数量、尺度和姿态变化有一定的限制,而基于深度学习的目标检测方法能够在大规模数据集上进行训练,提取更为丰富的特征,并利用卷积神经网络(CNN)等模型实现高精度的目标检测。
2.2 视频行为识别视频行为识别是指从视频中识别和分析人类的动作和行为。
深度学习模型能够通过学习大规模标注的视频数据,自动提取时空特征,实现对多种复杂行为的识别和分类。
2.3 视频内容理解视频内容理解是指对视频中的场景、物体和语义进行理解和表示。
深度学习模型具有强大的特征提取和表示学习能力,可以从视频数据中学习到抽象的表示,实现对视频内容的高层次理解。
2.4 视频编码视频编码是指通过压缩技术将视频信号编码为较低码率的码流。
基于深度学习的视频编码方法可以通过学习特定的视频场景和内容特征,并能够对视频数据进行高效的压缩和重建,实现更好的视频编码效果。
3. 深度学习在视频人工智能分析中的优势和挑战3.1 优势深度学习模型具有强大的表达能力和特征学习能力,能够自动学习和提取视频数据的抽象特征,减少了对传统手工设计特征的需求。
基于深度学习的视频画面质量评估研究

基于深度学习的视频画面质量评估研究随着互联网的飞速发展,视频平台如雨后春笋般涌现,人们已经习惯了在网上观看视频。
尤其是在疫情期间,更多的人习惯通过视频学习、工作、娱乐,这进一步推动了视频技术的发展。
然而,视频画面质量的评估一直是一个难点问题。
传统的质量评估方法面临很多局限性。
近年来,基于深度学习的视频画面质量评估方法备受研究者们关注,受到了广泛应用。
一、传统方法的不足传统的视频画面质量评估方法基于图像和视频处理技术,通过算法计算图像或视频的像素、码率、信噪比等指标,进行评估。
传统方法通常只能评估一张图片或一段视频某个特定的片段,而难以对整个视频进行全面、准确、客观的评估。
此外,由于现今智能设备的应用广泛,媒体数据的呈现形式变得越来越复杂和多样化,传统算法已经无法满足对不同数据的评估需求。
传统方法对于复杂的画面内容和不同的视频类型,无法用简单的评估指标来评估质量,很容易出现误判,同时对于细节方面的评估也存在一定局限性,而这些问题可以通过深度学习得到较好的解决。
二、基于深度学习的视频画面质量评估方法近年来,随着深度学习技术的应用和发展,基于深度学习的视频画面质量评估方法越来越受到关注。
深度学习作为一种强大的机器学习技术,可以有效利用数据进行模型的训练,并基于模型的结果实现对视频画面质量的自适应和不断优化,实现更加准确、全面、客观的评估。
基于深度学习的视频画面质量评估方法主要分为两大类 --- 无参和有参两种。
无参方法直接对视频片段的像素值进行学习,从而避免了传统方法中过于复杂的完整视频处理流程。
有参方法则首先提取关键信息进行特征描述,再基于描述向量进行学习评估。
两种方法各有优缺点,但无论是哪一种方法,都能够有效解决传统评估方法中的一些问题。
三、未来的发展趋势可以预见的是,基于深度学习的视频画面质量评估将是未来视频技术发展的重点和趋势。
通过融合图像和视频信号处理技术、传统的计算机视觉技术和深度学习技术,可以有效提高对不同视频类型、画质和故障的准确评估,同时也为更高水平和更广泛范围的应用提供更好的支持。
基于深度学习的视频目标识别与跟踪
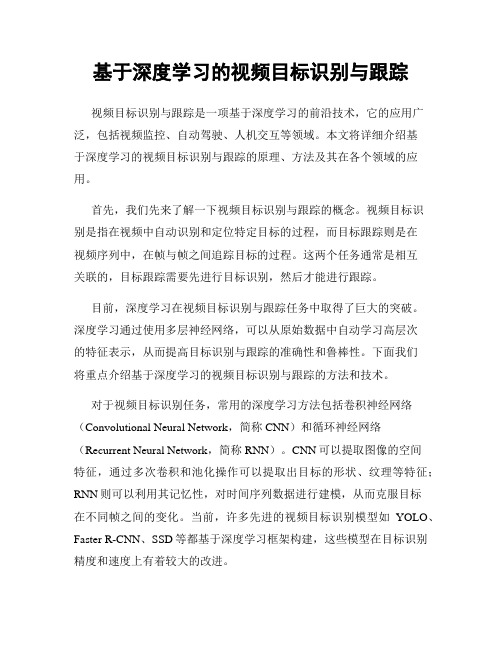
基于深度学习的视频目标识别与跟踪视频目标识别与跟踪是一项基于深度学习的前沿技术,它的应用广泛,包括视频监控、自动驾驶、人机交互等领域。
本文将详细介绍基于深度学习的视频目标识别与跟踪的原理、方法及其在各个领域的应用。
首先,我们先来了解一下视频目标识别与跟踪的概念。
视频目标识别是指在视频中自动识别和定位特定目标的过程,而目标跟踪则是在视频序列中,在帧与帧之间追踪目标的过程。
这两个任务通常是相互关联的,目标跟踪需要先进行目标识别,然后才能进行跟踪。
目前,深度学习在视频目标识别与跟踪任务中取得了巨大的突破。
深度学习通过使用多层神经网络,可以从原始数据中自动学习高层次的特征表示,从而提高目标识别与跟踪的准确性和鲁棒性。
下面我们将重点介绍基于深度学习的视频目标识别与跟踪的方法和技术。
对于视频目标识别任务,常用的深度学习方法包括卷积神经网络(Convolutional Neural Network,简称CNN)和循环神经网络(Recurrent Neural Network,简称RNN)。
CNN可以提取图像的空间特征,通过多次卷积和池化操作可以提取出目标的形状、纹理等特征;RNN则可以利用其记忆性,对时间序列数据进行建模,从而克服目标在不同帧之间的变化。
当前,许多先进的视频目标识别模型如YOLO、Faster R-CNN、SSD等都基于深度学习框架构建,这些模型在目标识别精度和速度上有着较大的改进。
对于视频目标跟踪任务,常用的深度学习方法包括基于卷积神经网络的目标跟踪器和基于循环神经网络的目标跟踪器。
基于卷积神经网络的目标跟踪器通过在两个帧之间匹配特征来实现目标的跟踪,例如Siamese网络、DaSiamRPN等;基于循环神经网络的目标跟踪器则通过对目标在时间序列上进行建模,从而进行目标跟踪,例如LSTM-based目标跟踪器。
这些跟踪器通过不断学习和更新模型,能够在复杂的背景和目标变化的情况下实现准确的跟踪。
基于深度学习的视频目标检测与跟踪算法研究
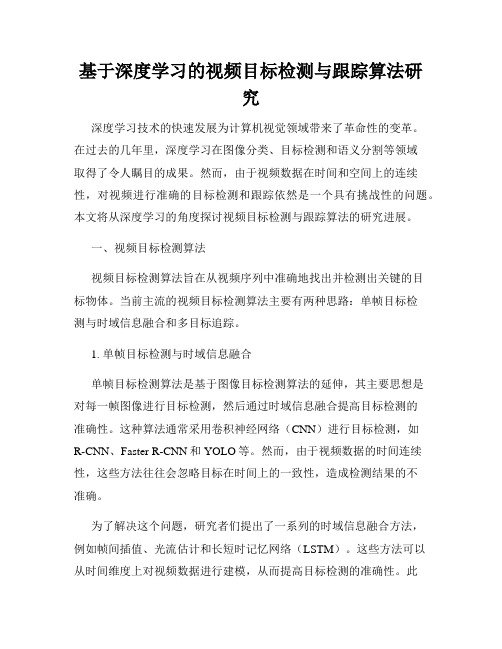
基于深度学习的视频目标检测与跟踪算法研究深度学习技术的快速发展为计算机视觉领域带来了革命性的变革。
在过去的几年里,深度学习在图像分类、目标检测和语义分割等领域取得了令人瞩目的成果。
然而,由于视频数据在时间和空间上的连续性,对视频进行准确的目标检测和跟踪依然是一个具有挑战性的问题。
本文将从深度学习的角度探讨视频目标检测与跟踪算法的研究进展。
一、视频目标检测算法视频目标检测算法旨在从视频序列中准确地找出并检测出关键的目标物体。
当前主流的视频目标检测算法主要有两种思路:单帧目标检测与时域信息融合和多目标追踪。
1. 单帧目标检测与时域信息融合单帧目标检测算法是基于图像目标检测算法的延伸,其主要思想是对每一帧图像进行目标检测,然后通过时域信息融合提高目标检测的准确性。
这种算法通常采用卷积神经网络(CNN)进行目标检测,如R-CNN、Faster R-CNN和YOLO等。
然而,由于视频数据的时间连续性,这些方法往往会忽略目标在时间上的一致性,造成检测结果的不准确。
为了解决这个问题,研究者们提出了一系列的时域信息融合方法,例如帧间插值、光流估计和长短时记忆网络(LSTM)。
这些方法可以从时间维度上对视频数据进行建模,从而提高目标检测的准确性。
此外,还有一些基于光流的方法,通过利用目标的运动信息提高目标检测的性能。
这些方法在许多基准数据集上取得了很好的效果,但是它们的计算复杂度较高,对硬件设备的要求也较高。
2. 多目标追踪多目标追踪算法旨在持续跟踪视频序列中的多个目标,并保持目标的标识信息不变。
当前主流的多目标追踪算法主要有两种思路:基于检测与跟踪的方法和基于在线学习与在线推断的方法。
基于检测与跟踪的方法将目标检测和目标跟踪视为两个独立的任务,首先通过目标检测算法找出视频序列中的目标,然后通过目标跟踪算法对目标进行跟踪。
这种方法的优点是可以利用目标检测算法的准确性,但是由于两个任务的相互独立性,容易导致检测错误和跟踪失败。
一种监控摄像机干扰检测模型
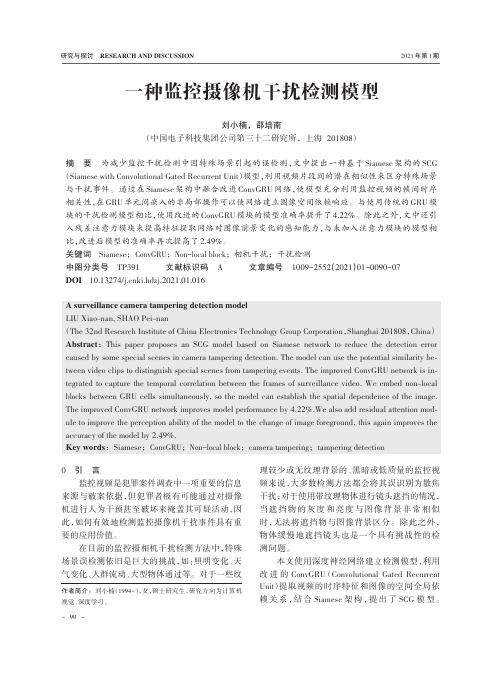
引言监控视频是犯罪案件调查中一项重要的信息来源与破案依据,但犯罪者极有可能通过对摄像机进行人为干预甚至破坏来掩盖其可疑活动,因此,如何有效地检测监控摄像机干扰事件具有重要的应用价值。
在目前的监控摄相机干扰检测方法中,特殊场景误检测依旧是巨大的挑战,如:照明变化、天气变化、人群流动、大型物体通过等。
对于一些纹理较少或无纹理背景的、黑暗或低质量的监控视频来说,大多数检测方法都会将其误识别为散焦干扰;对于使用带纹理物体进行镜头遮挡的情况,当遮挡物的灰度和亮度与图像背景非常相似时,无法将遮挡物与图像背景区分。
除此之外,物体缓慢地遮挡镜头也是一个具有挑战性的检测问题。
本文使用深度神经网络建立检测模型,利用改进的ConvGRU (Convolutional Gated Recurrent Unit )提取视频的时序特征和图像的空间全局依赖关系,结合Siamese 架构,提出了SCG 模型。
一种监控摄像机干扰检测模型刘小楠,邵培南(中国电子科技集团公司第三十二研究所,上海201808)摘要为减少监控干扰检测中因特殊场景引起的误检测,文中提出一种基于Siamese 架构的SCG(Siamese with Convolutional Gated Recurrent Unit )模型,利用视频片段间的潜在相似性来区分特殊场景与干扰事件。
通过在Siamese 架构中融合改进ConvGRU 网络,使模型充分利用监控视频的帧间时序相关性,在GRU 单元间嵌入的非局部操作可以使网络建立图像空间依赖响应。
与使用传统的GRU 模块的干扰检测模型相比,使用改进的ConvGRU 模块的模型准确率提升了4.22%。
除此之外,文中还引入残差注意力模块来提高特征提取网络对图像前景变化的感知能力,与未加入注意力模块的模型相比,改进后模型的准确率再次提高了2.49%。
关键词Siamese ;ConvGRU ;Non-local block ;相机干扰;干扰检测中图分类号TP391文献标识码A文章编号1009-2552(2021)01-0090-07DOI 10.13274/ki.hdzj.2021.01.016A surveillance camera tampering detection modelLIU Xiao -nan,SHAO Pei -nan(The 32nd Research Institute of China Electronics Technology Group Corporation ,Shanghai 201808,China )Abstract :This paper proposes an SCG model based on Siamese network to reduce the detection error caused by some special scenes in camera tampering detection.The model can use the potential similarity be⁃tween video clips to distinguish special scenes from tampering events.The improved ConvGRU network is in⁃tegrated to capture the temporal correlation between the frames of surveillance video.We embed non -local block s between GRU cells simultaneously,so the model can establish the spatial dependence of the image.The improved ConvGRU network improves model performance by 4.22%.We also add residual attention mod⁃ule to improve the perception ability of the model to the change of image foreground,this again improves the accuracy of the model by 2.49%.Key words :Siamese ;ConvGRU ;Non-local block ;camera tampering ;tampering detection作者简介:刘小楠(1994-),女,硕士研究生,研究方向为计算机视觉、深度学习。
基于帧间连续性的在线视频多目标跟踪算法
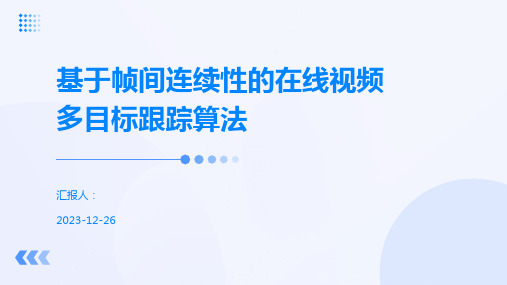
算法实时性改进
• 算法优化:通过算法优化,如减 少冗余计算和优化数据结构,以 降低算法的时间复杂度。- 并行 处理:利用并行计算技术,如 GPU加速,实现算法的高效执行 ,提高处理速度。- 帧率控制: 根据视频帧率动态调整算法的执 行频率,以保持实时跟踪效果。
06
总结与展望
工作总结
算法原理
该算法基于帧间连续性,利用目标在连续帧之间的运动信息,实现了多目标跟踪。通过建 立目标运动轨迹和特征模型,有效地解决了目标遮挡、运动快速变化等复杂场景下的跟踪 问题。
基于帧间连续性的在线视频 多目标跟踪算法
汇报人: 2023-12-26
目录
• 引言 • 算法原理 • 算法实现 • 实验结果与分析 • 算法优化与改进 • 总结与展望
01
引言
研究背景与意义
应用价值
多目标跟踪是计算机视觉领域的重要研究方向,在视频监控 、人机交互、自动驾驶等领域具有广泛的应用价值。随着在 线视频的普及,实时、准确的多目标跟踪算法成为研究的热 点。
在轨迹后处理阶段,算法对目标 的运动轨迹进行优化,去除错误 的数据关联,提高跟踪的准确性 。
03
算法实现
目标检测
01
目标检测是算法的第一步,用于在视频帧中识别和定位目标。
02
常用的目标检测算法包括:基于特征的方法(如Haar特征、
LBP等)、深度学习方法(如CNN、YOLO、SSD等)。
目标检测的准确性对后续的目标跟踪至关重要,因此需要选择
技术挑战
在线视频多目标跟踪面临诸多技术挑战,如目标遮挡、相似 背景干扰、动态环境变化等,需要算法具备鲁棒性和适应性 。
相关工作概述
传统算法
传统的多目标跟踪算法主要包括基于特征的方法和基于滤波的方法。基于特征的方法利用目标的颜色、纹理等特 征进行跟踪,但易受光照变化和目标遮挡影响。基于滤波的方法利用滤波器对目标进行跟踪,但计算量大且对模 型参数敏感。
一种视频图像改进卡尔曼滤波定位技术

量 。Kama l n滤 波 对控 制理 论 贡 献 很大 ,最早 被 用
21视频图像跟踪 . 视 频 跟踪 系统 输 入 的 是 图 像 序 列 ,图 像 目标
在航天领域, 现在在视觉 领域、 像处理领域中 图
也 广 泛 应 用 。现 实 中一般 利 用 选择 状 态变 量 体 现
系统 的 特 征 以及 状 况 的变 化 。卡 尔 曼 滤 波 的模 型 主 要 包 括 两 个状 态模 型 和 观 测 模型 。状 态 空 间模 型 是 反 映 状 态变 化 的规 律 ,用 状 态 方程 描 述 相 邻 时刻 的状 态 转 移 它 的变 化 规 律 ;观 测 模 型 是反 映 实 际观 测量 值 和状 态 变量 它 们 的关 系。Ka n滤 l ma 波是 将 观 测 信 息 和状 态 转 移 规 律相 结 合 最 后 得 到 系统 整体状 态的最 优估 计 。 卡尔曼 滤波 的计算 流程如 下 : 计算状 态估计 值 :
+1
:
f )
性 束 呈 究者对视 频理 日 誊 与 竺 约 运 了研 堡 解的兴 寸 趣 ,视 频跟 激发
,
计新 : ’ 算息 … … 一。
计算 卡尔 曼滤波增 益 :
。 。
踪 技 术作 为 必不 可 少 的技 术其 作用 显得 日益重 要 。
△ += .+ 口1 ( . 1 西 1 l 1 ) I 一 +十 一 △
3 实验
本 文 处 理 的 原 始 图 像 分 辨 率 为 2 0×3 0 4 2, C U 为 2 G , 内存 为 5 2 ,视 频 采 集 卡 的 采 P . Hz 4 1M 样 频 率为 每秒 2 5帧 。图 2卡尔 曼滤 波定位 实验 图
基于深度学习的视频动作识别算法研究

基于深度学习的视频动作识别算法研究深度学习技术在计算机视觉领域取得了显著的突破,尤其在视频动作识别方面具有广泛的应用前景。
本文旨在探讨基于深度学习的视频动作识别算法研究,并对其应用进行深入分析。
一、引言随着计算机视觉技术的快速发展,视频动作识别成为了一个备受关注的研究领域。
传统的视频动作识别方法受限于特征提取和模式匹配等问题,难以实现准确、高效和鲁棒性强的动作识别。
而基于深度学习的视频动作识别算法则通过自动学习特征和模式,能够更好地解决这些问题。
二、基于深度学习的视频动作识别算法1. 卷积神经网络(CNN)卷积神经网络是一种广泛应用于图像处理任务中的深度学习模型。
通过卷积层和汇聚层等操作,CNN能够自动提取图像中具有判别性意义的特征。
在视频动作识别中,CNN可以通过对每一帧图像进行处理,并利用时序信息进行动作分类。
2. 循环神经网络(RNN)循环神经网络是一种能够处理序列数据的深度学习模型。
在视频动作识别中,RNN可以利用其记忆能力,对动作序列进行建模和分类。
通过引入长短期记忆(LSTM)单元,RNN能够有效解决长序列建模中的梯度消失和梯度爆炸问题。
3. 时空卷积神经网络(3D CNN)时空卷积神经网络是一种专门用于处理视频数据的深度学习模型。
3D CNN通过在时间维度上引入卷积操作,能够同时利用空间和时间信息进行特征提取。
相比于传统的2D CNN,3D CNN在视频动作识别中具有更好的性能。
三、基于深度学习的视频动作识别算法研究进展1. 特征表示学习基于深度学习的视频动作识别算法中,特征表示学习是一个关键问题。
传统方法通常采用手工设计的特征表示方法,而基于深度学习的方法则通过自动学习特征表示,避免了手工设计特征所带来的局限性。
2. 时序建模在视频动作识别中,时序建模是一个重要的任务。
通过引入循环神经网络等模型,可以对动作序列进行建模,从而更好地捕捉动作的时序信息。
此外,还可以通过引入注意力机制等方法,提升对关键帧或关键时间段的重要性建模能力。
计算机视觉中的视频识别算法综述
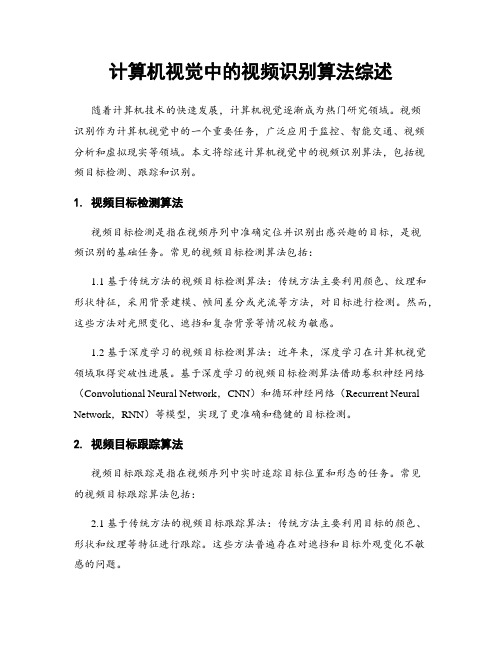
计算机视觉中的视频识别算法综述随着计算机技术的快速发展,计算机视觉逐渐成为热门研究领域。
视频识别作为计算机视觉中的一个重要任务,广泛应用于监控、智能交通、视频分析和虚拟现实等领域。
本文将综述计算机视觉中的视频识别算法,包括视频目标检测、跟踪和识别。
1. 视频目标检测算法视频目标检测是指在视频序列中准确定位并识别出感兴趣的目标,是视频识别的基础任务。
常见的视频目标检测算法包括:1.1 基于传统方法的视频目标检测算法:传统方法主要利用颜色、纹理和形状特征,采用背景建模、帧间差分或光流等方法,对目标进行检测。
然而,这些方法对光照变化、遮挡和复杂背景等情况较为敏感。
1.2 基于深度学习的视频目标检测算法:近年来,深度学习在计算机视觉领域取得突破性进展。
基于深度学习的视频目标检测算法借助卷积神经网络(Convolutional Neural Network,CNN)和循环神经网络(Recurrent Neural Network,RNN)等模型,实现了更准确和稳健的目标检测。
2. 视频目标跟踪算法视频目标跟踪是指在视频序列中实时追踪目标位置和形态的任务。
常见的视频目标跟踪算法包括:2.1 基于传统方法的视频目标跟踪算法:传统方法主要利用目标的颜色、形状和纹理等特征进行跟踪。
这些方法普遍存在对遮挡和目标外观变化不敏感的问题。
2.2 基于深度学习的视频目标跟踪算法:基于深度学习的视频目标跟踪算法利用卷积神经网络(CNN)和循环神经网络(RNN)等模型,可以自动学习目标的外观和运动特征,实现更准确和鲁棒的目标跟踪。
3. 视频目标识别算法视频目标识别是指从视频序列中识别出目标的类别或身份信息。
常见的视频目标识别算法包括:3.1 基于传统方法的视频目标识别算法:传统方法主要利用目标的形状、颜色和纹理等特征进行识别。
这些方法通常需要手动设计特征和分类器,对目标变化较大和背景干扰较多的场景效果不佳。
3.2 基于深度学习的视频目标识别算法:基于深度学习的视频目标识别算法充分利用深度卷积神经网络(Deep Convolutional Neural Network,DCNN)等模型,从大量标注数据中自动学习目标的特征表示。
开放世界目标检测 原理
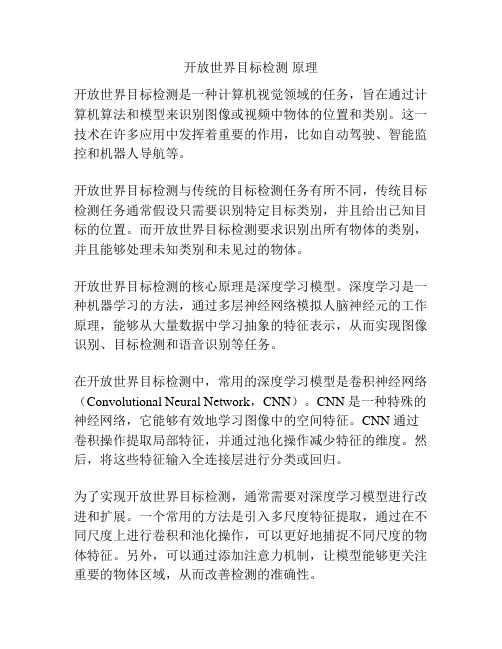
开放世界目标检测原理开放世界目标检测是一种计算机视觉领域的任务,旨在通过计算机算法和模型来识别图像或视频中物体的位置和类别。
这一技术在许多应用中发挥着重要的作用,比如自动驾驶、智能监控和机器人导航等。
开放世界目标检测与传统的目标检测任务有所不同,传统目标检测任务通常假设只需要识别特定目标类别,并且给出已知目标的位置。
而开放世界目标检测要求识别出所有物体的类别,并且能够处理未知类别和未见过的物体。
开放世界目标检测的核心原理是深度学习模型。
深度学习是一种机器学习的方法,通过多层神经网络模拟人脑神经元的工作原理,能够从大量数据中学习抽象的特征表示,从而实现图像识别、目标检测和语音识别等任务。
在开放世界目标检测中,常用的深度学习模型是卷积神经网络(Convolutional Neural Network,CNN)。
CNN 是一种特殊的神经网络,它能够有效地学习图像中的空间特征。
CNN 通过卷积操作提取局部特征,并通过池化操作减少特征的维度。
然后,将这些特征输入全连接层进行分类或回归。
为了实现开放世界目标检测,通常需要对深度学习模型进行改进和扩展。
一个常用的方法是引入多尺度特征提取,通过在不同尺度上进行卷积和池化操作,可以更好地捕捉不同尺度的物体特征。
另外,可以通过添加注意力机制,让模型能够更关注重要的物体区域,从而改善检测的准确性。
对于未知类别和未见过的物体,开放世界目标检测通常使用迁移学习和元学习的方法。
迁移学习是指将一个领域的知识应用到另一个领域,通过将已有的模型和数据迁移到未知类别上,可以快速适应新的物体。
而元学习则是通过在有限的样本上学习到一种“学习规则”,从而在遇到新的任务时,能够快速学习和适应。
这些方法能够提高模型的泛化能力,使其能够识别和检测未知类别。
此外,开放世界目标检测还需要配备大规模的数据集来训练和评估模型。
大规模的数据集包含了许多不同类别和物体的图像和标注信息,它们可以有效地提高模型的泛化能力和准确性。
基于金字塔池化与注意力机制的视频去模糊算法
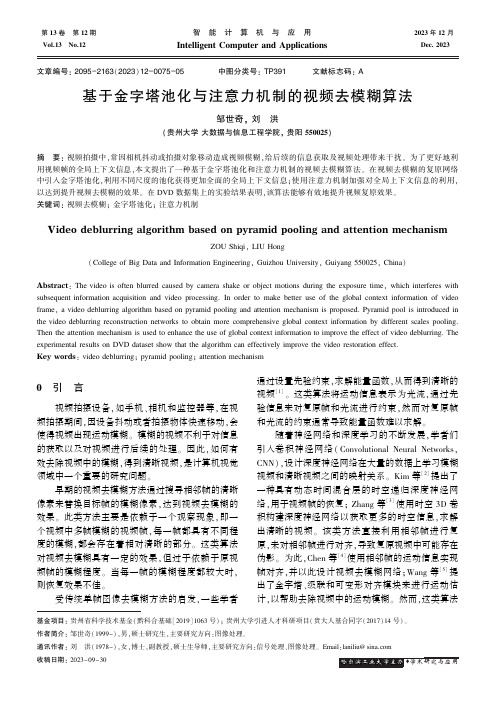
第13卷㊀第12期Vol.13No.12㊀㊀智㊀能㊀计㊀算㊀机㊀与㊀应㊀用IntelligentComputerandApplications㊀㊀2023年12月㊀Dec.2023㊀㊀㊀㊀㊀㊀文章编号:2095-2163(2023)12-0075-05中图分类号:TP391文献标志码:A基于金字塔池化与注意力机制的视频去模糊算法邹世奇,刘㊀洪(贵州大学大数据与信息工程学院,贵阳550025)摘㊀要:视频拍摄中,常因相机抖动或拍摄对象移动造成视频模糊,给后续的信息获取及视频处理带来干扰㊂为了更好地利用视频帧的全局上下文信息,本文提出了一种基于金字塔池化和注意力机制的视频去模糊算法㊂在视频去模糊的复原网络中引入金字塔池化,利用不同尺度的池化获得更加全面的全局上下文信息;使用注意力机制加强对全局上下文信息的利用,以达到提升视频去模糊的效果㊂在DVD数据集上的实验结果表明,该算法能够有效地提升视频复原效果㊂关键词:视频去模糊;金字塔池化;注意力机制VideodeblurringalgorithmbasedonpyramidpoolingandattentionmechanismZOUShiqi,LIUHong(CollegeofBigDataandInformationEngineering,GuizhouUniversity,Guiyang550025,China)Abstract:Thevideoisoftenblurredcausedbycamerashakeorobjectmotionsduringtheexposuretime,whichinterfereswithsubsequentinformationacquisitionandvideoprocessing.Inordertomakebetteruseoftheglobalcontextinformationofvideoframe,avideodeblurringalgorithmbasedonpyramidpoolingandattentionmechanismisproposed.Pyramidpoolisintroducedinthevideodeblurringreconstructionnetworkstoobtainmorecomprehensiveglobalcontextinformationbydifferentscalespooling.Thentheattentionmechanismisusedtoenhancetheuseofglobalcontextinformationtoimprovetheeffectofvideodeblurring.TheexperimentalresultsonDVDdatasetshowthatthealgorithmcaneffectivelyimprovethevideorestorationeffect.Keywords:videodeblurring;pyramidpooling;attentionmechanism基金项目:贵州省科学技术基金(黔科合基础[2019]1063号);贵州大学引进人才科研项目(贵大人基合同字(2017)14号)㊂作者简介:邹世奇(1999-),男,硕士研究生,主要研究方向:图像处理㊂通讯作者:刘㊀洪(1978-),女,博士,副教授,硕士生导师,主要研究方向:信号处理㊁图像处理㊂Email:laniliu@sina.com收稿日期:2023-09-300㊀引㊀言视频拍摄设备,如手机㊁相机和监控器等,在视频拍摄期间,因设备抖动或者拍摄物体快速移动,会使得视频出现运动模糊㊂模糊的视频不利于对信息的获取以及对视频进行后续的处理㊂因此,如何有效去除视频中的模糊,得到清晰视频,是计算机视觉领域中一个重要的研究问题㊂早期的视频去模糊方法通过搜寻相邻帧的清晰像素来替换目标帧的模糊像素,达到视频去模糊的效果㊂此类方法主要是依赖于一个观察现象,即一个视频中多帧模糊的视频帧,每一帧都具有不同程度的模糊,都会存在着相对清晰的部分㊂这类算法对视频去模糊具有一定的效果,但过于依赖于原视频帧的模糊程度㊂当每一帧的模糊程度都较大时,则恢复效果不佳㊂受传统单帧图像去模糊方法的启发,一些学者通过设置先验约束,求解能量函数,从而得到清晰的视频[1]㊂这类算法将运动信息表示为光流,通过先验信息来对复原帧和光流进行约束,然而对复原帧和光流的约束通常导致能量函数难以求解㊂随着神经网络和深度学习的不断发展,学者们引入卷积神经网络(ConvolutionalNeuralNetworks,CNN),设计深度神经网络在大量的数据上学习模糊视频和清晰视频之间的映射关系㊂Kim等[2]提出了一种具有动态时间混合层的时空递归深度神经网络,用于视频帧的恢复;Zhang等[3]使用时空3D卷积构建深度神经网络以获取更多的时空信息,求解出清晰的视频㊂该类方法直接利用相邻帧进行复原,未对相邻帧进行对齐,导致复原视频中可能存在伪影㊂为此,Chen等[4]使用相邻帧的运动信息实现帧对齐,并以此设计视频去模糊网络;Wang等[5]提出了金字塔㊁级联和可变形对齐模块来进行运动估计,以帮助去除视频中的运动模糊㊂然而,这类算法并没有充分地利用视频帧的上下文信息㊂金字塔池化通过多个尺度的池化获得更大的感受野,从而提取到更多的上下文信息来帮助视频去模糊,注意力机制能够引导网络关注学习视频去模糊中的重要信息㊂本文将金字塔池化与注意力机制相结合,利用金字塔池化对提取得到的特征进行4个尺度的池化,将池化得到的特征进行卷积㊁上采样,输出为4个具有上下文信息的全局特征,并与原来的特征进行特征融合;再引入注意力机制,对融合的特征赋予权重;最终经过重构网络恢复得到清晰的视频㊂1㊀算法模型本文通过一个基于金字塔池化与注意力机制的深度网络学习模糊帧与清晰帧之间端到端的映射关系,利用当前模糊帧与相邻模糊帧之间的有用信息来复原当前模糊帧㊂本文视频去模糊算法框架如图1所示㊂输入连续的三帧模糊视频帧,通过光流估计网络提取中间帧与相邻两帧之间的光流信息,利用所提取到的光流对相邻视频帧进行变换,实现帧对齐;将帧对齐后的三帧视频帧输入进复原网络中,复原得到中间视频帧的清晰帧㊂光流估计网络帧对齐复原网络图1㊀本文视频去模糊算法框架Fig.1㊀Theframeworkoftheproposedvideodeblurringalgorithm1.1㊀光流估计网络光流反映了相邻图像帧之间像素间的相对运动信息㊂光流估计是指利用图像序列中像素在时间域上的变化及相邻帧之间的相关性来找到两相邻帧像素间的对应关系㊂本文通过光流估计网络来估计输入相邻视频帧的光流信息,以提供视频帧复原所需的运动信息㊂光流估计网络采用的是PWC网络(CNNsforOpticalFlowUsingPyramid,Warping,andCostVolume)[6]㊂给定任意两帧相邻视频帧Ii和Ii+1,Ii到Ii+1的光流fiңi+1的求解公式(1):fiңi+1=P(Ii,Ii+1)(1)㊀㊀其中,P表示PWC光流估计网络㊂1.2㊀复原网络复原网络结构如图2所示㊂复原网络由特征提取网络㊁金字塔池化模块[7](PyramidPoolingModule,PPM)㊁卷积块注意力模块[8](ConvolutionalBlockAttentionModule,CBAM)以及重构网络构成㊂S k i p c o n n e c t i o n卷积层(5?5,步长:1)卷积层(5?5,步长:2)残差块P P M C B A M反卷积层(5?5,步长:2)图2㊀复原网络结构Fig.2㊀Thestructureofrestorationnetwork.1.2.1㊀特征提取与重构网络尺度递归网络(Scale-RecurrentNetwork,SRN)是基于多尺度递归的图像去模糊网络㊂该网络对图像进行降采样,将不同尺度的图像作为输入, 从粗到细 逐步递归恢复出清晰的图像,SRN中的编码器解码器残差网络是恢复出清晰图像的关键[9]㊂在复原网络中引入了SRN中的编码器解码器残差网络作为本文算法中的特征提取网络和重构网络,对视频帧进行特征提取和特征重构㊂由于本文算法是单尺度的视频去模糊,所以没有选用原SRN网络中ConvLSTM模块㊂特征提取网络负责将视频帧转换为具有较小空间大小和更多通道的特征图F㊂特征提取网络由3个卷积块构成,第一个卷积块由一个步长为1的卷积层和3个残差块构成,并以LeakyReLU作为激活函数;另外两个卷积块由步长为2的卷积层和3个残差67智㊀能㊀计㊀算㊀机㊀与㊀应㊀用㊀㊀㊀㊀㊀㊀㊀㊀㊀㊀㊀㊀㊀㊀第13卷㊀块构成,以LeakyReLU作为激活函数㊂残差块由两个步长为1的卷积层和LeakyReLU激活函数构成㊂重构网络负责对经由特征提取㊁金字塔池化以及注意力加权得到的特征Fs进行复原,得到清晰帧㊂重构网络由3个卷积块组成,其中两个卷积块是由3个残差块和一个步长为2的反卷积层以及LeakyReLU激活函数构成,最后一个卷积块是由3个残差块和一个步长为1的卷积层以及LeakyReLU激活函数构成㊂1.2.2㊀金字塔池化模块受感受野大小的限制,卷积层对特征实现的是局部感知处理,得到的是局部信息,缺少了全局上下文信息㊂为了提取到更多的全局上下文信息,本文在编码器与解码器之间引入了金字塔池化,以获得更大的感受野来提高对视频帧上下文全局信息的获取㊂金字塔池化模块结构如图3所示,金字塔池化模块的输入为特征提取网络提取到的特征F,对F进行4个尺度的平均池化,分别得到1ˑ1㊁2ˑ2㊁3ˑ3㊁6ˑ64个尺度大小的特征㊂将池化后的4个特征使用1ˑ1卷积将其通道数减少到1/4,再通过双线性插值进行上采样,将特征上采样至特征F大小,最后将4个上采样得到的特征和特征F在通道方向上拼接起来,得到一个新的特征FP,公式(2):Fp=Np(F)(2)㊀㊀其中,NP表示金字塔池化模块㊂C O N V C O N VC O N VC O N VU P S A M P L E输入特征输出特征P O O LC O N C A T图3㊀金字塔池化模块结构Fig.3㊀Thestructureofpyramidpoolingmodule1.2.3㊀卷积块注意力模块本文采用CBAM引导对特征的通道和空间的学习,CBAM由通道注意力模块和空间注意力模块构成,通道注意力模块引导网络关注学习 什么 更重要,空间注意力模块引导网络关注学习 哪里 更重要㊂卷积注意力模块结构如图4所示㊂在注意力模块中,由金字塔池化模块得到的特征FP(长㊁宽以及通道数分别为H㊁W㊁C)输入到通道注意力模块,对FP的每个通道进行最大池化和平均池化,得到两个Cˑ1ˑ1大小的特征;再通过多层感知机,实现非线性变换;将两个特征对应相加,再经由激活函数输出得到每个通道的权重Mc㊂将Mc与FP相乘得到特征Fc;将特征Fc输入进空间注意力模块中,在通道方向上求平均池化和最大池化,得到两个1ˑHˑW大小的特征;将两个特征在通道方向上进行拼接,再经由激活函数输出得到每个像素的权重Ms,将Ms与Fc相乘得到最终的特征Fs㊂过程如式(3) 式(6):Mc=Nc(Fp)(3)Fc=Mc∗Fp(4)Ms=Ns(Fc)(5)Fs=Ms∗Fc(6)㊀㊀其中,Nc表示通道注意力模块,Ns表示空间注意力模块㊂1.3㊀损失函数损失函数是机器学习和深度学习中用来衡量模型预测值和真实值之间误差的数值评估指标,本文使用L1loss来度量复原帧和真实视频帧之间的误差,损失函数定义如式(7)所示:L=ðNVn=1ðMi=1Ini-Ingt,i1(7)㊀㊀其中,NV表示视频的数量;M代表视频帧的数量;I表示复原帧;Igt表示真实视频帧㊂输入特征输出特征空间权重空间注意力模块通道权重空间注意力模块图4㊀卷积块注意力模块结构Fig.4㊀Thestructureofconvolutionalblockattentionmodule77第12期邹世奇,等:基于金字塔池化与注意力机制的视频去模糊算法2㊀实验结果及分析2.1㊀实验准备2.1.1㊀数据集本文采用DVD数据集来进行训练和评估㊂该数据集有71个视频,每个视频的平均运行时间为3-5s,61个训练视频和10个测试视频㊂本文通过对训练视频帧随机裁剪以扩充数据集,随机裁剪的区域大小为256ˑ256㊂2.1.2㊀实验环境及参数设置本文使用预训练模型对PWC网络初始化,使用Adam优化器,设置参数β1=0.9,β2=0.999,训练批次为8,初始学习率为0.000001,复原网络的初始学习率为0.0001,训练的总周期为300,且在前200个训练周期结束之后,学习率呈线性衰减,设置为0.000001㊂硬件环境为:PG500-216GPU和E5-2678v3CPU㊂2.2㊀实验结果分析本文采用峰值信噪比(PeakSignal-to-NoiseRatio,PSNR)和结构相似度(StructuralSimilarityIndexMeasurement,SSIM)来对复原结果进行客观评价㊂峰值信噪比是基于对应像素点之间的误差,即基于误差敏感的图像质量评价,给定复原视频帧X和真实视频帧Y,其峰值信噪比如式(8)所示:PSNR(X,Y)=10lg2552NðNn=1(xn-yn)2æèççöø÷÷(8)㊀㊀其中,N表示图像的总像素数;xn表示X的第n个像素值;yn表示Y的第n个像素值㊂结构相似性分别从亮度㊁对比度㊁结构三方面度量图像相似性,式(9):SSIM(X,Y)=(2μxμy+C1)(2σxy+C2)(μx2+μy2+C1)(σx2+σy2+C2)(9)㊀㊀其中,μx,μy,σx,σy,σxy分别是X的平均值,Y的平均值,X的标准差,Y的标准差,以及X和Y的协方差;C1,C2是用于保持稳定的常数㊂本文方法参考了文献[9]的编码器解码器残差网络,所以将文献[9]中的SRN与本文方法进行了定量对比㊂为了验证加入的金字塔池化模块和卷积块注意力模块的有效性,同时做了消融实验,实验结果见表1㊂表1㊀算法在DVD数据集上有效性对比Table1㊀ValiditycomparisonofthealgorithmonDVDdataset方法SRN无PPM㊁CBAM只加入PPM只加入CBAM本文方法PSNR29.4230.4230.5130.5430.61SSIM0.87560.89800.89950.89990.9005㊀㊀根据表1所示,本文的方法PSNR和SSIM更高,分别加入PPM和CBAM的视频去模糊算法,在PSNR和SSIM上均有提高,证明了PPM和CABM的有效性㊂此外,还将本文方法与SRN以及未加入PPM和CBAM的视频去模糊方法的去模糊效果进行了对比(如图5所示),为了便于观察细节,将原始模糊帧以及对比方法的去模糊结果的局部细节进行了放大㊂从图5可以看出使用文中方法复原后的视频帧,其纹理细节以及轮廓更加清晰,并且能够有效地抑制伪影㊂㊀㊀将本文算法同当前主流的视频去模糊算法:结合动态时间混合层的时空递归网络(STRCNN+DTB)[2]㊁增强型可变形卷积视频恢复网络(EDVR)[5]㊁结合光流的去模糊网络(DBN+FLOW)[10]进行定量比较,对比结果见表2,实验结果表明,文中方法拥有更高的PSNR和SSIM,证明了本文算法的有效性㊂表2㊀不同算法在DVD数据集上的定量评估Table2㊀QuantitativeevaluationsontheDVDdatasetfordifferentalgorithms方法原始视频STRCNN+DTBEDVRDBN+FLOW本文方法PSNR27.2029.9528.5130.0130.61SSIM0.81230.86920.86370.88770.900587智㊀能㊀计㊀算㊀机㊀与㊀应㊀用㊀㊀㊀㊀㊀㊀㊀㊀㊀㊀㊀㊀㊀㊀第13卷㊀㊀㊀㊀㊀㊀㊀㊀㊀㊀㊀(a)模糊帧㊀㊀㊀㊀㊀(b)SRN方法结果㊀(c)无PPM和CBAM方法结果㊀(d)文本方法结果图5㊀在DVD数据集上的去模糊结果Fig.5㊀DeblurredresultsontheDVDdataset3㊀结束语本文提出了一种有效的视频去模糊算法,在较好地复原出视频帧的轮廓和细节的同时,也有效地抑制了伪影㊂使用金字塔池化为视频复原提供了更多的全局上下文信息;还加入了注意力机制引导网络关注学习视频复原所需的重要信息,加强了对视频复原有用信息的利用㊂将金字塔池化同注意力机制相结合,进一步提高模糊视频复原的效果㊂参考文献[1]PANJ,BAIH,TANGJ.Cascadeddeepvideodeblurringusingtemporalsharpnessprior[C]//ProceedingsoftheIEEE/CVFConferenceonComputerVisionandPatternRecognition.2020:3043-3051.[2]HYUNKIMT,MULEEK,SCHOLKOPFB,etal.Onlinevideodeblurringviadynamictemporalblendingnetwork[C]//ProceedingsoftheIEEEInternationalConferenceonComputerVision.2017:4038-4047.[3]ZHANGK,LUOW,ZHONGY,etal.Adversarialspatio-temporallearningforvideodeblurring[J].IEEETransactionsonImageProcessing,2018,28(1):291-301.[4]CHENH,GUJ,GALLOO,etal.Reblur2deblur:Deblurringvideosviaself-supervisedlearning[C]//Proceedingsofthe2018IEEEInternationalConferenceonComputationalPhotography(ICCP).IEEE,2018:1-9.[5]WANGX,CHANKCK,YUK,etal.Edvr:Videorestorationwithenhanceddeformableconvolutionalnetworks[C]//ProceedingsoftheIEEE/CVFConferenceonComputerVisionandPatternRecognitionWorkshops.2019:1954-1963.[6]SUND,YANGX,LIUMY,etal.Pwc-net:Cnnsforopticalflowusingpyramid,warping,andcostvolume[C]//ProceedingsoftheIEEEConferenceonComputerVisionandPatternRecognition.2018:8934-8943.[7]ZHAOH,SHIJ,QIX,etal.Pyramidsceneparsingnetwork[C]//ProceedingsoftheIEEEConferenceonComputerVisionandPatternRecognition.2017:2881-2890.[8]WOOS,PARKJ,LEEJY,etal.Cbam:Convolutionalblockattentionmodule[C]//ProceedingsoftheEuropeanConferenceonComputerVision(ECCV).2018:3-19.[9]TAOX,GAOH,SHENX,etal.Scale-recurrentnetworkfordeepimagedeblurring[C]//ProceedingsoftheIEEEConferenceonComputerVisionandPatternRecognition.2018:8174-8182.[10]SUS,DELBRACIOM,WANGJ,etal.Deepvideodeblurringforhand-heldcameras[C]//ProceedingsoftheIEEEConferenceonComputerVisionandPatternRecognition.2017:1279-1288.97第12期邹世奇,等:基于金字塔池化与注意力机制的视频去模糊算法。
计算机视觉技术在动作捕捉中的优化方法
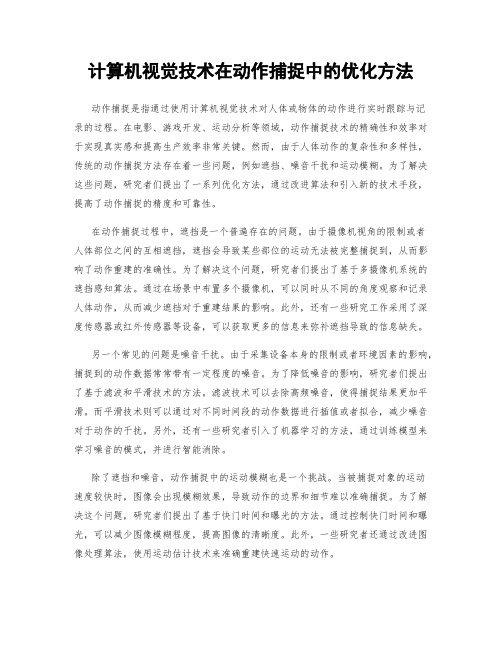
计算机视觉技术在动作捕捉中的优化方法动作捕捉是指通过使用计算机视觉技术对人体或物体的动作进行实时跟踪与记录的过程。
在电影、游戏开发、运动分析等领域,动作捕捉技术的精确性和效率对于实现真实感和提高生产效率非常关键。
然而,由于人体动作的复杂性和多样性,传统的动作捕捉方法存在着一些问题,例如遮挡、噪音干扰和运动模糊。
为了解决这些问题,研究者们提出了一系列优化方法,通过改进算法和引入新的技术手段,提高了动作捕捉的精度和可靠性。
在动作捕捉过程中,遮挡是一个普遍存在的问题。
由于摄像机视角的限制或者人体部位之间的互相遮挡,遮挡会导致某些部位的运动无法被完整捕捉到,从而影响了动作重建的准确性。
为了解决这个问题,研究者们提出了基于多摄像机系统的遮挡感知算法。
通过在场景中布置多个摄像机,可以同时从不同的角度观察和记录人体动作,从而减少遮挡对于重建结果的影响。
此外,还有一些研究工作采用了深度传感器或红外传感器等设备,可以获取更多的信息来弥补遮挡导致的信息缺失。
另一个常见的问题是噪音干扰。
由于采集设备本身的限制或者环境因素的影响,捕捉到的动作数据常常带有一定程度的噪音。
为了降低噪音的影响,研究者们提出了基于滤波和平滑技术的方法。
滤波技术可以去除高频噪音,使得捕捉结果更加平滑。
而平滑技术则可以通过对不同时间段的动作数据进行插值或者拟合,减少噪音对于动作的干扰。
另外,还有一些研究者引入了机器学习的方法,通过训练模型来学习噪音的模式,并进行智能消除。
除了遮挡和噪音,动作捕捉中的运动模糊也是一个挑战。
当被捕捉对象的运动速度较快时,图像会出现模糊效果,导致动作的边界和细节难以准确捕捉。
为了解决这个问题,研究者们提出了基于快门时间和曝光的方法。
通过控制快门时间和曝光,可以减少图像模糊程度,提高图像的清晰度。
此外,一些研究者还通过改进图像处理算法,使用运动估计技术来准确重建快速运动的动作。
总结来说,计算机视觉技术在动作捕捉中的优化方法主要包括遮挡感知、噪音干扰的滤波和消除、以及运动模糊的控制。
利用计算机视觉技术进行视频监控画面清晰度提升的技巧
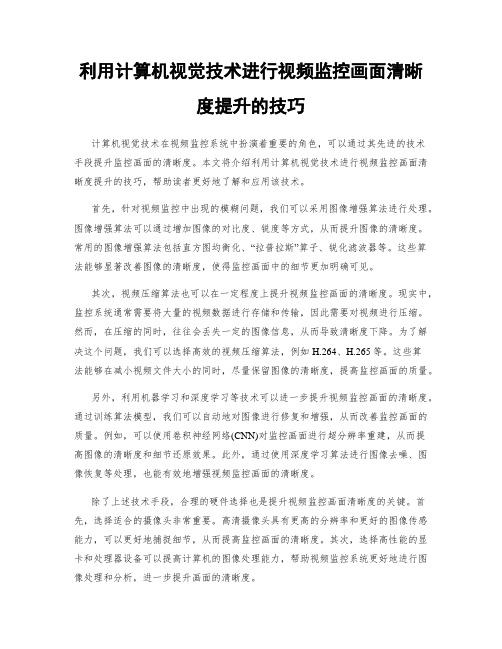
利用计算机视觉技术进行视频监控画面清晰度提升的技巧计算机视觉技术在视频监控系统中扮演着重要的角色,可以通过其先进的技术手段提升监控画面的清晰度。
本文将介绍利用计算机视觉技术进行视频监控画面清晰度提升的技巧,帮助读者更好地了解和应用该技术。
首先,针对视频监控中出现的模糊问题,我们可以采用图像增强算法进行处理。
图像增强算法可以通过增加图像的对比度、锐度等方式,从而提升图像的清晰度。
常用的图像增强算法包括直方图均衡化、“拉普拉斯”算子、锐化滤波器等。
这些算法能够显著改善图像的清晰度,使得监控画面中的细节更加明确可见。
其次,视频压缩算法也可以在一定程度上提升视频监控画面的清晰度。
现实中,监控系统通常需要将大量的视频数据进行存储和传输,因此需要对视频进行压缩。
然而,在压缩的同时,往往会丢失一定的图像信息,从而导致清晰度下降。
为了解决这个问题,我们可以选择高效的视频压缩算法,例如H.264、H.265等。
这些算法能够在减小视频文件大小的同时,尽量保留图像的清晰度,提高监控画面的质量。
另外,利用机器学习和深度学习等技术可以进一步提升视频监控画面的清晰度。
通过训练算法模型,我们可以自动地对图像进行修复和增强,从而改善监控画面的质量。
例如,可以使用卷积神经网络(CNN)对监控画面进行超分辨率重建,从而提高图像的清晰度和细节还原效果。
此外,通过使用深度学习算法进行图像去噪、图像恢复等处理,也能有效地增强视频监控画面的清晰度。
除了上述技术手段,合理的硬件选择也是提升视频监控画面清晰度的关键。
首先,选择适合的摄像头非常重要。
高清摄像头具有更高的分辨率和更好的图像传感能力,可以更好地捕捉细节,从而提高监控画面的清晰度。
其次,选择高性能的显卡和处理器设备可以提高计算机的图像处理能力,帮助视频监控系统更好地进行图像处理和分析,进一步提升画面的清晰度。
此外,视频监控画面清晰度提升还需要考虑到光线环境和环境干扰等因素。
在设计和安装监控系统时,应尽量避免背光问题和光线干扰,如合理安排摄像头的角度和位置,进行光源照明设置等。
如何使用计算机视觉技术进行视频分析

如何使用计算机视觉技术进行视频分析计算机视觉技术在视频分析中扮演着重要的角色,它可以对视频进行实时的监测、分析和理解。
通过计算机视觉技术,我们可以从视频中提取关键信息,识别物体,追踪运动,分析行为等。
本文将探讨如何使用计算机视觉技术进行视频分析,并介绍一些常用的技术和工具。
首先,视频分析的第一步是从视频中提取图像帧。
视频是由一系列连续的图像帧组成的,因此首先我们需要将视频转换为图像序列。
这可以通过使用视频处理库,如OpenCV来实现。
OpenCV是一个功能强大的开源计算机视觉库,它支持多种编程语言,如Python和C++。
视频中的每个图像帧都可以被视为一张图片,我们可以对每个图像帧应用计算机视觉算法进行分析。
一个常见的应用是目标检测,就是在视频中自动识别和定位感兴趣的物体。
目标检测算法有很多种,其中最常用的是基于深度学习的算法,如YOLO(You Only Look Once)和Faster R-CNN(Region-based Convolutional Neural Networks)。
使用这些算法,我们可以实现实时目标检测,而且在处理复杂场景和大量物体时表现良好。
通过目标检测,我们可以在视频中标记出每个物体的位置和边界框,从而对视频中的物体进行跟踪和分析。
除了目标检测,计算机视觉技术还可以用于行为分析。
行为分析旨在对视频中的人或物体的动态行为进行建模和识别。
例如,我们可以使用姿态估计算法来识别人体的动作,如行走、跑步、举手等。
另一个例子是行为识别,它可以识别交通摄像头中的交通违规行为,如闯红灯、逆行等。
为了进一步提高视频分析的精度和效果,我们还可以结合其他计算机视觉技术,如光流估计、立体视觉和光学字符识别。
光流估计可以用于分析视频中的运动模式,如运动的方向和速度。
立体视觉可以从多个相机角度获取图像数据,并进行立体匹配以获得三维物体的深度信息。
光学字符识别可以识别视频中的文字,如车牌号码等,用于行人、车辆的识别和跟踪。
手机高清视频实时稳像算法研究与实现
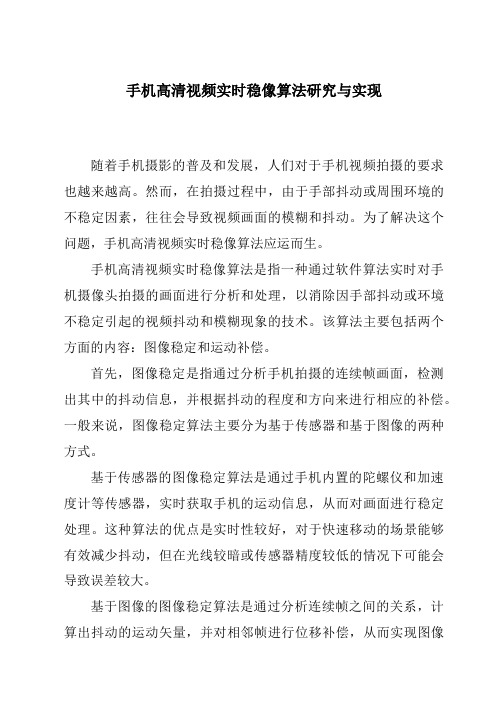
手机高清视频实时稳像算法研究与实现随着手机摄影的普及和发展,人们对于手机视频拍摄的要求也越来越高。
然而,在拍摄过程中,由于手部抖动或周围环境的不稳定因素,往往会导致视频画面的模糊和抖动。
为了解决这个问题,手机高清视频实时稳像算法应运而生。
手机高清视频实时稳像算法是指一种通过软件算法实时对手机摄像头拍摄的画面进行分析和处理,以消除因手部抖动或环境不稳定引起的视频抖动和模糊现象的技术。
该算法主要包括两个方面的内容:图像稳定和运动补偿。
首先,图像稳定是指通过分析手机拍摄的连续帧画面,检测出其中的抖动信息,并根据抖动的程度和方向来进行相应的补偿。
一般来说,图像稳定算法主要分为基于传感器和基于图像的两种方式。
基于传感器的图像稳定算法是通过手机内置的陀螺仪和加速度计等传感器,实时获取手机的运动信息,从而对画面进行稳定处理。
这种算法的优点是实时性较好,对于快速移动的场景能够有效减少抖动,但在光线较暗或传感器精度较低的情况下可能会导致误差较大。
基于图像的图像稳定算法是通过分析连续帧之间的关系,计算出抖动的运动矢量,并对相邻帧进行位移补偿,从而实现图像的稳定。
这种算法的优点是适用于各种光线条件下的拍摄,但对计算能力要求较高,可能存在处理延迟的问题。
其次,运动补偿是指通过对图像的位移进行补偿,使得画面中的运动物体能够保持相对静止。
运动补偿算法是基于视频序列之间的运动估计和补偿原理进行实现的。
这些算法主要包括全局运动估计和局部运动估计两种方式。
全局运动估计是指通过分析整个视频序列的运动信息,计算出全局的运动参数,并将其应用于每一帧的位移补偿中。
这种算法适用于大范围的运动场景,但对计算量较大。
局部运动估计是指通过分析相邻帧之间的差异,计算出局部的运动参数,并将其应用于相邻帧之间的位移补偿中。
这种算法适用于小范围的运动场景,计算量相对较小。
综上所述,手机高清视频实时稳像算法通过图像稳定和运动补偿两个方面的处理,能够有效减少手机视频拍摄中的抖动和模糊现象,提高用户的拍摄体验。
基于深度学习的电视画面质量评测技术研究
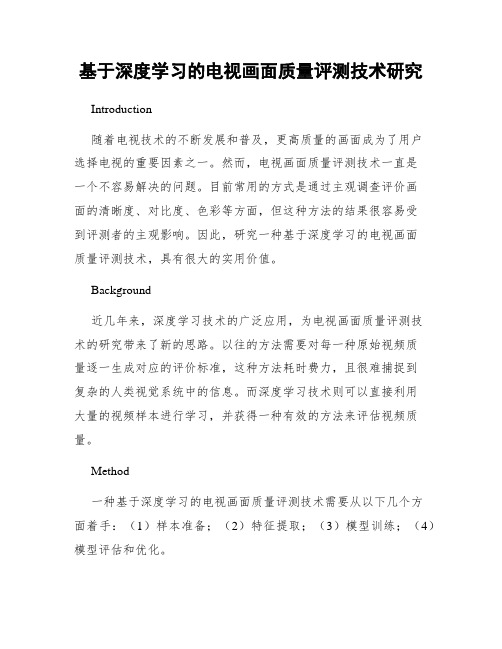
基于深度学习的电视画面质量评测技术研究Introduction随着电视技术的不断发展和普及,更高质量的画面成为了用户选择电视的重要因素之一。
然而,电视画面质量评测技术一直是一个不容易解决的问题。
目前常用的方式是通过主观调查评价画面的清晰度、对比度、色彩等方面,但这种方法的结果很容易受到评测者的主观影响。
因此,研究一种基于深度学习的电视画面质量评测技术,具有很大的实用价值。
Background近几年来,深度学习技术的广泛应用,为电视画面质量评测技术的研究带来了新的思路。
以往的方法需要对每一种原始视频质量逐一生成对应的评价标准,这种方法耗时费力,且很难捕捉到复杂的人类视觉系统中的信息。
而深度学习技术则可以直接利用大量的视频样本进行学习,并获得一种有效的方法来评估视频质量。
Method一种基于深度学习的电视画面质量评测技术需要从以下几个方面着手:(1)样本准备;(2)特征提取;(3)模型训练;(4)模型评估和优化。
在样本准备方面,需要充分利用各种类型的视频样本。
比如,可以选择一些有代表性的视频,包括高清电影、体育赛事、电视剧等,以及一些模糊、有噪声或失真的视频。
这些视频样本可以通过网络爬取或主动收集的方式获取,以保证样本的多样性和数量。
同时还需配备一些人工评测的结果,作为样本评估的标准。
在特征提取方面,可以选择一些比较常见的视频质量相关特征。
比如,可以利用人类感知系统中对于颜色、亮度、对比度等不同方面的特征进行提取。
此外还可以利用根据视频压缩标准得到的一些像素、块信息,以及基于帧间预测的一些运动信息等特征。
在模型训练方面,可以尝试使用一些已经被证明有效的算法,比如深度神经网络(DNN)、深度置信网络(DBN)等。
需要对网络进行有效的训练,以便得到最佳的精度和泛化能力。
此外,在训练过程中使用正则化技术、批量归一化等技术,可以进一步提高模型的性能。
在模型评估和优化方面,需要采用一些评价指标来度量模型的性能,比如均方误差(MSE)、峰值信噪比(PSNR)、结构相似性指数(SSIM)等。
基于人工智能的高清视频超分辨率重建算法
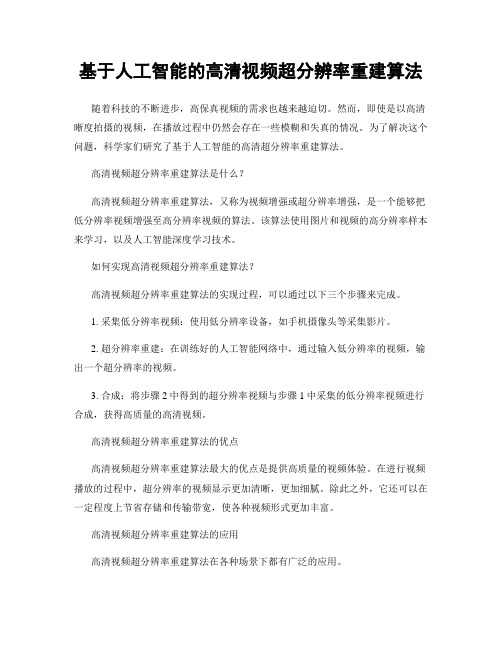
基于人工智能的高清视频超分辨率重建算法随着科技的不断进步,高保真视频的需求也越来越迫切。
然而,即使是以高清晰度拍摄的视频,在播放过程中仍然会存在一些模糊和失真的情况。
为了解决这个问题,科学家们研究了基于人工智能的高清超分辨率重建算法。
高清视频超分辨率重建算法是什么?高清视频超分辨率重建算法,又称为视频增强或超分辨率增强,是一个能够把低分辨率视频增强至高分辨率视频的算法。
该算法使用图片和视频的高分辨率样本来学习,以及人工智能深度学习技术。
如何实现高清视频超分辨率重建算法?高清视频超分辨率重建算法的实现过程,可以通过以下三个步骤来完成。
1. 采集低分辨率视频:使用低分辨率设备,如手机摄像头等采集影片。
2. 超分辨率重建:在训练好的人工智能网络中,通过输入低分辨率的视频,输出一个超分辨率的视频。
3. 合成:将步骤2中得到的超分辨率视频与步骤1中采集的低分辨率视频进行合成,获得高质量的高清视频。
高清视频超分辨率重建算法的优点高清视频超分辨率重建算法最大的优点是提供高质量的视频体验。
在进行视频播放的过程中,超分辨率的视频显示更加清晰,更加细腻。
除此之外,它还可以在一定程度上节省存储和传输带宽,使各种视频形式更加丰富。
高清视频超分辨率重建算法的应用高清视频超分辨率重建算法在各种场景下都有广泛的应用。
1. 游戏动画:在游戏动画领域,高清视频超分辨率重建算法可以为游戏制作人员提供更完美的游戏画面,使游戏更加细腻传神。
2. 影视制作:在影视制作领域,高清视频超分辨率重建算法可以为电影和电视节目等制作人员提供更高质量的视频素材。
3. 视频监控:在视频监控领域,高清视频超分辨率重建算法可以通过提升图像细节和清晰度来帮助安全监控。
4. 医学图像:在医学图像领域,高清视频超分辨率重建算法可以帮助医生和科学家观察所检测的疾病,从而提高临床诊断的准确性。
总结高清视频超分辨率重建算法是一种能够使低分辨率视频转化为高分辨率的算法。
基于模糊神经网络的视频图像目标精准识别研究

基于模糊神经网络的视频图像目标精准识别研究
王学忠
【期刊名称】《信息与电脑》
【年(卷),期】2022(34)16
【摘要】由于对特征的分析结果存在误差,导致对视频图像目标识别的精度较低,因此提出基于模糊神经网络的视频图像目标精准识别方法。
首先,利用Relief算法选择目标特征,根据特征与同类样本和不同类别样本之间距离的关系,对特征进行个性化赋权;其次,将权重最高的m个特征作为模糊神经网络识别的特征。
最后,在计算模糊化层各个节点的高斯隶属度函数参数后,采用误差反向传播算法计算模糊神经网络各层级之间的梯度,并反向逐层计算特征的累积误差,实现对视频图像目标的识别。
测试结果表明,设计方法的识别精度最大值可以达到96.44%,最小值达到88.62%,具有良好的识别效果。
【总页数】3页(P188-190)
【作者】王学忠
【作者单位】安徽三联学院电子电气工程学院
【正文语种】中文
【中图分类】TP391
【相关文献】
1.基于神经网络-模糊推理的目标识别融合研究
2.基于模糊神经网络的地下小目标识别方法研究
3.基于模糊神经网络的舰船目标识别的方法研究
4.基于模糊神经网络的海面目标战术意图识别
5.基于神经网络的运动视频图像分类和识别研究
因版权原因,仅展示原文概要,查看原文内容请购买。
视频目标检测
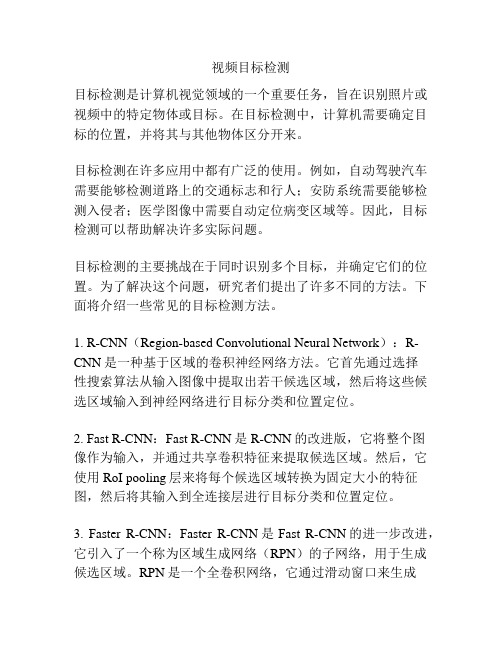
视频目标检测目标检测是计算机视觉领域的一个重要任务,旨在识别照片或视频中的特定物体或目标。
在目标检测中,计算机需要确定目标的位置,并将其与其他物体区分开来。
目标检测在许多应用中都有广泛的使用。
例如,自动驾驶汽车需要能够检测道路上的交通标志和行人;安防系统需要能够检测入侵者;医学图像中需要自动定位病变区域等。
因此,目标检测可以帮助解决许多实际问题。
目标检测的主要挑战在于同时识别多个目标,并确定它们的位置。
为了解决这个问题,研究者们提出了许多不同的方法。
下面将介绍一些常见的目标检测方法。
1. R-CNN(Region-based Convolutional Neural Network):R-CNN是一种基于区域的卷积神经网络方法。
它首先通过选择性搜索算法从输入图像中提取出若干候选区域,然后将这些候选区域输入到神经网络进行目标分类和位置定位。
2. Fast R-CNN:Fast R-CNN是R-CNN的改进版,它将整个图像作为输入,并通过共享卷积特征来提取候选区域。
然后,它使用RoI pooling层来将每个候选区域转换为固定大小的特征图,然后将其输入到全连接层进行目标分类和位置定位。
3. Faster R-CNN:Faster R-CNN是Fast R-CNN的进一步改进,它引入了一个称为区域生成网络(RPN)的子网络,用于生成候选区域。
RPN是一个全卷积网络,它通过滑动窗口来生成候选区域,并使用候选区域与真实目标框之间的IoU (Intersection over Union)来训练网络。
4. YOLO(You Only Look Once):YOLO是一种实时目标检测方法,它将目标检测问题转化为一个回归问题。
它通过将输入图像分为网格,每个网格预测一组边界框和类别概率来定位目标。
然后,通过非最大抑制来剔除重叠的边界框。
以上只是目标检测方法中的一小部分,随着研究的深入,还会有新的方法被提出。
尽管目标检测仍然存在许多挑战,如小目标检测、遮挡目标的检测等,但随着计算机硬件的不断发展和深度学习的进步,目标检测在实际应用中的性能和效果正在不断提高。
- 1、下载文档前请自行甄别文档内容的完整性,平台不提供额外的编辑、内容补充、找答案等附加服务。
- 2、"仅部分预览"的文档,不可在线预览部分如存在完整性等问题,可反馈申请退款(可完整预览的文档不适用该条件!)。
- 3、如文档侵犯您的权益,请联系客服反馈,我们会尽快为您处理(人工客服工作时间:9:00-18:30)。
Video-Aware Opportunistic Network Coding overWireless NetworksHulya Seferoglu,IEEE Student Member,Athina Markopoulou,IEEE MemberAbstract—In this paper,we study video streaming over wireless networks with network coding capabilities.We build upon recent work,which demonstrated that network coding can increase throughput over a broadcast medium,by mixing packets from differentflows into a single packet,thus increasing the in-formation content per transmission.Our key insight is that, when the transmittedflows are video streams,network codes should be selected so as to maximize not only the network throughput but also the video quality.We propose video-aware opportunistic network coding schemes that take into account both the decodability of network codes by several receivers and the importance and deadlines of video packets.Simulation results show that our schemes significantly improve both video quality and throughput.This work is afirst step towards content-aware network coding.Index Terms—Network coding,video streaming,prioritized transmission,wireless networks,cross-layer optimization.I.I NTRODUCTIONP ROVIDING high quality video over wireless networks is a challenging problem,due to both the erratic and time-varying nature of a wireless channel and the stringent delivery requirements of media traffic.Developments in video compression and streaming,wireless networking,and cross-layer design,are continuously advancing the state-of-the art in wireless video[1],[2].In this paper,we propose a novel technique for video streaming in a wireless environment inspired by the emerging paradigm of network coding[3]–[6].Our work builds on recent work in[7],[8]that used network coding to improve throughput in a wireless mesh network. In particular,[7],[8]proposed that wireless routers mix packets from differentflows,so as to increase the information content of each-broadcast-transmission and therefore the throughput for data applications.In this paper,we build on this idea,and propose a network coding and scheduling scheme for transmitting several video streams over a wireless mesh network.Our key insight is that the transmission of video streams in a network coding-capable wireless network should be optimized not only for network throughput but also,and more importantly,for video quality.The fact that video packets have unequal importance is well understood and extensively studied in the video streaming community,e.g.for rate-distortion optimized streaming[9]–[12].The fact that mixing different informationflows can increase throughput in multicast net-works is well understood in the network coding community Manuscript received1August2008;revised20February2009.This work has been supported by the NSF CAREER grant0747110.The authors are with the Department of Electrical Engineering and Com-puter Science at the University of California,Irvine.Digital Object Identifier10.1109/JSAC.2009.090612.[3],[4],[13],[14].Our work bridges the gap between the two approaches,and proposes a new video-aware scheme for network coding and packet scheduling that improves both aspects,namely video quality and throughput.More generally, this idea can be extended from video-aware to content-aware network coding to take into account packet-,flow-,or class-based prioritization of packets in the network code selection. In this paper,we consider a wireless mesh network,in which routers can mix different incomingflows/streams,using simple network coding operations(XOR).The resulting network code is broadcasted to the neighborhood of the router.Nodes in the same neighborhood listen to each other’s transmission and store overheard packets;these are used later to decode received coded packets and also to construct new coded packets.The core question in this architecture is how to select the best-according to an appropriate metric-network code for transmission among all possible codes.In[7],[8],a transmitting node chooses a network code that can be decoded by several neighbors at the same time slot;this policy increases the information per packet transmission thus the throughput. However,when the transmittedflows are video streams,this is not necessarily the best choice.Video quality can be improved by intelligently selecting network codes that combine those video packets that are decodable by several neighbors but also contribute the most to video quality.In other words,when video streams are transmitted,it is not only the quantity but also the quality/content of information transferred that should be taken into account in the selection of network codes.In this paper,we develop schemes for network code selection and packet scheduling that take into account both(i)the importance and deadlines of video packets and(ii)the network state and the received/overheard packets in the neighborhood. The paper is organized as follows.Section II discusses related work.Section III gives an overview of the system model.Section IV presents the algorithms for network coding. Section V presents simulation results that demonstrate the benefits of the proposed algorithms over baseline schemes, in terms of video quality and application-level throughput. Section VI concludes the paper.II.R ELATED W ORKThis work combines ideas and techniques from two bodies of work:video streaming and network coding.Several network-adaptive techniques have been proposed to support streaming media over unreliable and/or time-varying networks[15].Supporting video over wireless is particularly challenging due to the limited,time-varying resources of the wireless channel[1],[2].There is a large body of work on cross-layer design for video over wireless,such as[16]–[20],0733-8716/09/$25.00c 2009IEEEexploiting the fact that packets in a video stream have different importance and therefore should be treated differently by net-work mechanisms.Packet scheduling is an important control at the medium access control layer.The problem of rate-distortion optimized packet scheduling has been studied in the RaDiO family of techniques[9]–[12]:in every transmission opportunity,media units are selected for transmission so as to maximize the expected quality of received video subject to a constraint in the transmission rate,and taking into account transmission errors,delays and decoding dependencies. Independently,the network coding paradigm has emerged from the pioneering work in[13],[14],which showed that,in multicast networks where intermediate nodes do simple linear operations on incoming packets,one can achieve the min-cut throughput of the network to each receiver.The linearly combined packets can be utilized at the receivers to recover the original packets by solving a set of linear equations over a finitefield.This breakthrough idea inspired significant effort in several directions[3]–[6],including practical application of network coding,studying topologies beyond multicast,such as unicast[21]–[23]and broadcast scenarios.The broadcast nature of the wireless medium offers an opportunity for exploiting the throughput benefits of network coding[24], [25].The recent work in[7],[8]applied these ideas from the network coding community in the context of wireless mesh networks.[7]implemented a pseudo-broadcast mechanism for802.11together with opportunistic listening and a coding layer between IP and MAC that is used to detect coding opportunities and pack packets from differentflows into a single transmission,thus increasing network throughput. Our paper introduces a novel technique for video streaming over wireless that combines the above two approaches.On one hand,we build on[7],[8]to exploit the broadcast nature of the wireless medium and use network coding to pack several pack-ets from different streams into a single code for transmission, thus increasing throughput.On the other hand,we construct and select network codes taking into account the importance of video packets(in terms of video distortion and playout deadlines)within the same stream,as well as their contribution to the throughput and video quality.This combined approach allows us to improve video quality,pared to[8],while maintaining the same level of throughput.An earlier workshop version of this work appeared in[26], where wefirst introduced the idea of media-aware network coding algorithms(NCV and NCVD).This journal version has a significantly extended evaluation part using a new module we implemented in the GloMoSim environment[28],considering a wide range of traffic scenarios and topologies,compar-ing against a range of baseline algorithms,and discussing complexity issues.In addition,this paper generalizes and formalizes the original idea by introducing NC-RaDiO,an extension of the RaDiO framework to take into account the network coding opportunities,and by showing how our video-aware network coding algorithmsfit within this framework and that they achieve near optimal performance.Looking forward,this idea can be generalized from video-aware to content-aware network coding.In the video-specific application,the importance of the packets reflects the video distortion as determined by content,encoding andplayout Fig.1.A wireless mesh network.I is an intermediate node,A,B,C are receiving(and/or sending)nodes.deadlines.More generally,the importance/priority of packets can be determined by price,policy or other considerations and can express differentiation at various levels of granularity: across packets of the sameflow,across differentflows/users, or across different classes of users.III.S YSTEM O VERVIEWWe consider video streaming over wireless mesh networks where intermediate nodes(wireless mesh routers)are able to forward packets to other intermediate nodes and/or clients, as shown in Fig.1.In this paper,we propose algorithms that can be used at the intermediate node to maximize video quality and throughput.We assume that intermediate nodes can perform simple network coding operations(bit-wise XOR) and combine packets from several incoming streams into a single outgoing packet.This packet is broadcasted to the entire neighborhood,thus reaching several nodes at the same time.We assume that nodes can overhear all transmissions in their neighborhood,whether they are intended for them or not;they can decode a network-coded packet using overheard packets.The idea of combining network coding with broadcast to increase the information content per transmission,is well understood in the network coding community.This idea has been recently applied in802.11-based multi-hop wireless networks and throughput benefits have been demonstrated for data applications[7],[8].Our key observation is that,when the transmittedflows are video streams,this is not necessarily the best choice and video quality must also be considered.The importance and deadlines of video packets must be taken into account to select those codes that contribute the most to the quality of video streams.In this paper,we develop schemes for network coding across differentflows,and packet selection within eachflow, to improve both video quality and throughput.1)Code Selection at an Intermediate Node:Let us consider an intermediate node that receives N packets from different video streams and forwards them to N nodes in its neighbor-hood.The intermediate node maintains a transmission(Tx) queue with incoming video packets.At a given time slot a packet is selected from the Tx queue for transmission.The selected packet is called the primary packet and its destination node is called the target node.The primary packet can be thought as the main packet we try to transmit during a time slot.Depending on the network coding scheme,the primarySEFEROGLU and MARKOPOULOU:VIDEO-AWARE OPPORTUNISTIC NETWORK CODING OVER WIRELESS NETWORKS715TABLE IT ERMINOLOGYTerm DefinitionPrimary Packet The packet selected from the Tx queue beforenetwork coding.It must be included in all net-work codes.It can be thought as the main packetwe try to transmit in a given time-slot.Side Packet Packet in the Tx queue,other than the primary,included in the network code.Network Code One primary and all side packets XOR-ed to-gether into a single packet.Active Packet Packet in the Tx queue that can be considered asprimary.(Not transmitted within the last RTT.) Inactive packet Packet in the Tx queue that cannot be consid-ered as primary.(It has already been transmittedwithin the last RTT,and the acknowledgement isstill pending.)Target Node The intended recipient of the primary packet.Tx Queue The output queue of the transmitting node.Rx Buffer The receive queue of the receiving node.It storesreceived packets,destined to this node.Virtual Buffer Also maintained at a receiving node.It storesoverheard packets,destined to other nodes. packet may be thefirst packet from the head of the queue, or any packet in Tx queue that is marked as active(i.e.,not transmitted within the last round-trip time,as discussed later). In addition to the primary packet,all packets in the queue are considered as candidate side packets,i.e.,candidates for a transmission in the same time slot together with the primary packet;they are useful to nodes other than the target node. The primary and the side packets are all XOR-ed together into a single packet,called the network code.The core question then is:which network code(i.e.XOR of the primary and side packets)to select and transmit so as to maximize the total video quality and throughput.The algorithms addressing this question are the main part of this paper,and will be discussed separately in the next section(IV).In the rest of this section, we describe the remaining components and functions of the system.The terminology is summarized in Table I.2)Receiving,Overhearing and ACKing a Packet(at Re-ceiving Nodes):Once the network code is chosen,it is broadcast to all nodes in the neighborhood.Depending on the channel conditions,some nodes successfully receive it.When the target node receives it,it decodes it(which is guaranteed by the construction of the network code in the next section), stores the primary packet in its receive(Rx)buffer,and sends an acknowledgement(ACK)back to the intermediate node. Nodes,other than the target node,overhear the transmitted packet and try to decode it;if they overhear a new packet destined to them,they store it in their Rx buffer and send an ACK back to the intermediate node;if they obtain a packet destined for another node,they store it in their virtual buffer. An overheard packet stays in the virtual buffer until an ACK from the target is overheard or until its deadline expires.We also assume that the asynchronous ACK mechanism,proposed in[7],is used to combat ACK implosion.3)Active/Inactive Packets(at an Intermediate Node):The intermediate node waits for a mean round-trip time(RTT) from the time it transmits the network code until it receives an ACK.During that period,all packets that were part of the code stay in the Tx queue but are marked as inactive. Inactive packets are not considered for primary transmission (in order to avoid unnecessary duplicate transmissions)but are still considered as candidates for side packets(to increase coding opportunities).When the transmitter receives an ACK, it removes the corresponding packet from the Tx queue.If an RTT expires without receiving an ACK,the packet is marked as active again and the process is repeated.A packet stays in the Tx queue until either it is successfully transmitted or its deadline expires;when either of these occur,the packet is removed from the transmission buffer.14)Requirements:Our system relies on the following ca-pabilities.First,broadcast is needed to harvest the benefits of network coding.Although wireless is inherently a broadcast medium,this may be hidden by some communication proto-cols.We make use of the broadcast capability,implemented as pseudo-broadcast on top of802.11unicast in[7],[8].Second, nodes need to learn the contents of the virtual buffers of all their neighbors,in order to select a code that is decodable in their neighborhood.This can be achieved by explicitly exchanging and/or implicitly learning this information as in [7],[8].Third,nodes must be capable of coding/decoding in real time,which is a realistic assumption for simple(bit-wise XOR)work coding is implemented as a thin layer between IP and MAC,exactly as in the original COPE [8].Nodes are consideredfixed(not mobile)and routing is considered given,i.e.,decided by a routing module orthogonal to the network coding algorithms considered in this paper. 5)Importance of Packets:Nodes make network coding decisions taking into account the importance of video packets. The distortion value(Δ)of every packet can be determined by the source and communicated to the intermediate nodes in order to enable them to take decisions about transmission of video units in a rate-distortion optimized manner[9].This information can be marked on a specialfield of the packet header.Thisfield can be at the application level(e.g.RTP extended headers)or part of the network coding header; alternatively,the typically unused TOS/DiffServ byte in the IP header can be overridden.In addition to the individual importance of packets(Δ)within aflow,our formulation also considers the importance offlows(γ).In general,the overall importance of a packet can be a function of theflow priority and the packet distortion value;in this paper,we use a simple productγ·Δ.6)Handling Video vs.Data Packets:The main focus of this paper is on network coding for video,and most of the discussion is presented in terms of queues that contain only video packets.This could be implemented in practice on top of802.11e,using the differentiation mechanisms to separate real-time traffic(in our case video)from data traffic.Our network coding algorithms could then be applied only on the video queue.Independently,our framework could also handle a mixture of video and data packets in the same queue by assigning them differentflow priorities.1Note that although a transmitted packet remains inactive for an RTT,it does not block the head of the queue:the next active packets in the queue are coded and transmitted during this period.Also note that,although the Tx queue is basically a FIFO,considering any active packet as primary may lead to reordering in packet delivery.Although this may be a concern for TCP, as it was the case in[7],it is clearly better for video that requires timely delivery and can reorder packets at the playout buffer.716IEEE JOURNAL ON SELECTED AREAS IN COMMUNICATIONS,VOL.27,NO.5,JUNE2009IV.C ODING A LGORITHMSThe main questions in this system have to do with theconstruction and selection of network codes.The code con-struction problem is concerned withfinding candidate codesthat guarantee decodability by the target node.The codeselection problem is concerned with selecting the best amongthe candidate codes so as to optimize video quality.Thefirstproposed algorithm,“NCV”,achieves the same throughputgains as in[7]but also intelligently chooses the network codesthat maximize video quality.The second algorithm,“NCVD”,uses NCV as a building block but considers more codingoptions thus further improving video quality and throughput.The third algorithm,“NC-RaDiO”,generalizes these ideas:itextends the rate-distortion optimized(RaDiO)packet schedul-ing framework[9],so as tofind the optimal network codingand transmission policy at every transmission opportunity.A.NCV Algorithm:Network Coding for VideoAssume that there are several video streams coming to anintermediate node.Depending on the content of the virtualbuffers at the clients,there may be several combinationsof these streams,i.e.several network coding opportunities.The main idea behind the Network Coding for Video(NCV)algorithm is to select the best network code to improve videoquality.The following example demonstrates this idea.Example1:Consider the example shown in Fig.1and letus focus on a single-hop shown in more detail in Fig.2.Node I receives three independent video streams,e.g.fromthe Internet through the gateway,destined to its neighborsA,B,C.I maintains a FIFO Tx queue that stores packets{A1,A2,...}destined to node A,{B1,B2,...}destined tonode B,and{C1,C2,...}destined to node C.Fig.2alsoshows the contents of the virtual buffers at each client:nodeA has overheard packets{B1,C1}and nodesB andC haveboth overheard packet A1,from previous transmissions.A1isthefirst active packet from head of the queue and is selectedas the primary packet.Any packet(active or inactive)in theoutput queue,other than A1,can be chosen as a side packet,on the condition that the constructed network code shouldbe decoded at node A,i.e.A1can be retrieved.To satisfythis condition,side packets that will be used in the networkcode should already be available at node A;in other words,the decodability of a network code depends on the overheardpackets at node work codes c1=A1,c2=A1⊕B1,c3=A1⊕C1,and c4=A1⊕B1⊕C1can all be decodedby A and thus are eligible network codes.The Code Construction Problem.More generally,considerthat there are N nodes N={n1,n2,...,n N}in the wirelessnetwork.Consider an intermediate node n∈N,which trans-mits to its neighbor nodes.Letφn be the number of packetsin the Tx queue of node n,and the packets themselves beΦn={p1,p2,...,pφn}.Choose thefirst active packet,p i,fromthe head of the Tx FIFO queue as the primary packet;p i has a target node t(p i)∈N.Node n will construct and broadcast a network code,which consists of the primary packet p i XOR-ed together with some side packets,so that the target node t(p i) can decode and obtain p i.For this to be guaranteed,all side packets must be among the packets that are already overheard Algorithm1The NCV Algorithm1:Initialization:I i max=0,c i max=∅2:Choose thefirst head-of-queue active packet as primary p i.3:Let t(p i)be the target node of packet p i.Let{ν1,...,νΨt(p i)}be the overheard packets at t(p i).4:for k=1...2ψt(p i)do5:c i k={p i}SS t(p i)k6:Calculate I i k with Eq.(5)7:if I i k>I i max then8:I i max=I i k,c i max=c i k9:end if10:end for11:Choose c i max as the network code.XOR all packets and transmitat the target t(p i).Assume thatψt(pi)packets are overheard at node t(p i)and denoted byΨt(pi)={ν1,ν2,...,νΨt(p i)}. Therefore,the candidate network codes at node n are:c i k={p i}S t(p i)k,k=1,2,...,2ψt(p i)(1)where S t(p i)kis the k th subset ofΨt(pi).Note that,since linear operations are limited to bit-wise XOR,a network code p1⊕p2⊕...⊕p k is completely specified by the set of packets {p1,p2,...,p k}that are XOR-ed together.The next step is to select the best among all candidate codes.Example1Continued.Node A can get packet A1from all four possible network codes.Codes c2and c3improve the video quality at node sets{A,B}and{A,C},respectively. It is clear that c2and c3are better codes than c1and c4both for throughput(they are useful to two instead of one node) and video paring c2to c3,we observe that they are equivalent in terms of throughput but they may contribute differently to video quality depending on the content of video packets A1,B1,C1.Deciding which candidate code to select between c2=A1⊕B1and c3=A1⊕C1should depend on the importance and urgency of the original video packets B1 and C1.NCV exploits this observation.The Code Selection Problem.After constructing all candi-date codes at a node n,we need to choose the best code according to an appropriate metric,which we define here so as to capture the contribution of each candidate code to the video quality improvement.Recall that p i is the primary packettargeted to node t(p i),and{c i k}k=2ψt(p i)k=1are all the candidate codes.Let I i k(nη)be the improvement in video quality at node nηforη=1,2,...,N,when c i k is received and decoded:I i k(nη)=L kl=1(1−P(l))Δ(l)γ(l)g kl(nη)d kl(nη)(2) where each factor in this formula is defined as follows:•L k is the number of original packets included in network code c i k.Notice that at most one out of these L k packets can be useful to a particular node nη,but different packets are useful to different nodes.•d k l(nη)and g k l(nη)are indicator functions that express whether code k is useful for node nη.We defined kl(nη)=1if c ikis decodable at node nη,or0otherwise.We define g k l(nη)=1if packet l is targeted to node nη, or0otherwise.•Δ(l)is the improvement in video quality(PSNR)if packet l is received correctly and on time at client nη.SEFEROGLU and MARKOPOULOU:VIDEO-AWARE OPPORTUNISTIC NETWORK CODING OVER WIRELESS NETWORKS717Fig.2.Example of Network Coding for Video(NCV),for a one-hop downlink scenario with three different streams.To computeΔ(l),we decode the entire video sequence with this packet missing and we compute the resulting distortion.2We assume that this computation is performed at the source offline and that the distortion value is marked on each packet.3•γ(l)is the importance/priority of theflow that packet l belongs to.All packets in the sameflow have the same (flow)importance,but differentflows may have different importance.If someflows are more important,then higher importance should be assigned to them;otherwise they should all be assignedγ=1.If the average quality differs among encoded video sequences and we still want to treatflows equally,theflow importance can be a normalization factor(the inverse of the average PSNR per sequence);this is what we do in the simulations.•P(l)is the probability that packet l is lost due to either channel errors or late arrival for playout:P(l)=P{F T T >t d(l)−t c}(3) where t d(l)is the deadline of packet l,t c is the current time andτ=t d(l)−t c is the remaining time until the playout deadline;F T T is the forward trip time in the presence of delay and loss.The complementary cumula-tive distribution function of F T T can be calculated as follows:P{F T T >τ}=εF+(1−εF)∞τp F(t)dt(4) Thefirst part in Eq.(4)describes the probability that a packet is lost in the forward channel,due to noise,fading, and interference in the wireless.The second part in Eq.(4) describes the probability that a packet,which is not lost, 2This is an approximation as the actual distortion that may also depend on the delivery status of prior and subsequent NALs.The distortion model can be extended to capture these loss correlations[29]–[31].Furthermore,we assume that distortions caused by loss of multiple packets are additive,which is reasonable for sparse losses.These approximations reduce the computational complexity by separating the total distortion function into a set of individual packet distortion functions and optimizing for each one of them.3For real-time traffic,one can still estimate the distortion by performing online analysis with a delay of a few frames.Most distortion occurs in thefirst few frames after a loss and breaks after the next I frame;the error depends on the video content of subsequent frames and on the coding decisions. Another approach is to assign distortion values based solely on the GOP structure,ignoring the video content and coding decisions,or to use a model for dependencies[31].arrives late,i.e.,after its playout deadline;p F(t)is the distribution of the forward-trip time.After defining the contribution of code c i k to the video quality at a single node nη,I i k(nη),we define the total video quality improvement of code c i k as the sum of the video quality improvements at all clientsη=1,...N,due to code c i k:I i k=Nη=1I i k(nη)(5)The NCV algorithm is summarized in Alg.(1).At each time slot,the NCV algorithm chooses the primary packetp i and constructs all candidate network codes{c i k}k=2ψt(p i)k=1. Among all candidate network codes,NCV chooses the code that maximizes the total video quality improvement:maxkI i k(6) Depending on the contents of the virtual buffers,it is possible that no side packets can be used together with a given primary packet p i.In that case,the network code is simply{p i}∪∅= {p i}.B.NCVD Algorithm:looking into the queue in DepthAs described in the previous section,NCV selects the primary packet from the head of the queue but ignoring packets marked as inactive,and then optimally chooses the side packets.However,the fact that NCV does not optimize the primary packet has two implications:(i)the primary packet itself is important for video quality and(ii)the candidate side codes are limited to those that are decodable for this single primary packet.The second algorithm improves over NCV by also optimizing the selection of the primary packet.NVCD looks into the entire Tx queue“in depth”and considers all, not just the head-of-line,packets as candidates for the primary packet,thus increasing the options for candidate codes.A different set of candidate codes can be constructed for each primary packet.We explain NCVD through the following example.Example2:Let us look at Fig.3.The topology is the same as in Fig.2,but the contents of the Tx queue and of the virtual buffers are different.Assume that all packets are active packets,i.e.,they can all be considered as primary.One option is to select the head-of-line packet A1as the primary packet.。