land surface temperature from Ka band passive microwave observations
热红外遥感在城市区域规划中的应用
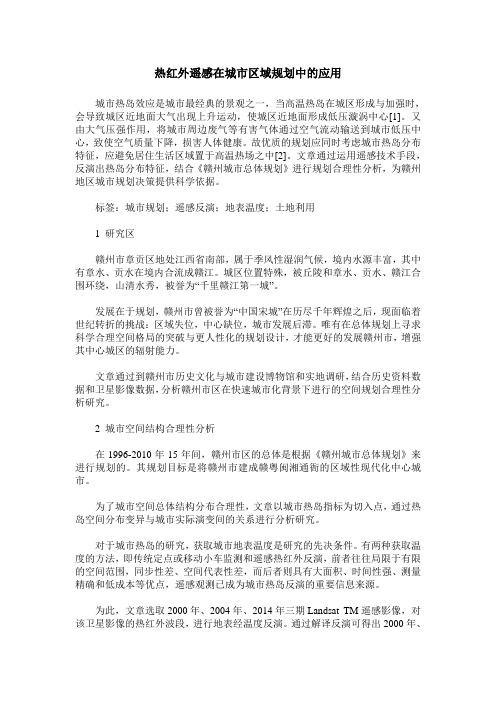
热红外遥感在城市区域规划中的应用城市热岛效应是城市最经典的景观之一,当高温热岛在城区形成与加强时,会导致城区近地面大气出现上升运动,使城区近地面形成低压漩涡中心[1]。
又由大气压强作用,将城市周边废气等有害气体通过空气流动输送到城市低压中心,致使空气质量下降,损害人体健康。
故优质的规划应同时考虑城市热岛分布特征,应避免居住生活区域置于高温热场之中[2]。
文章通过运用遥感技术手段,反演出热岛分布特征,结合《赣州城市总体规划》进行规划合理性分析,为赣州地区城市规划决策提供科学依据。
标签:城市规划;遥感反演;地表温度;土地利用1 研究区赣州市章贡区地处江西省南部,属于季风性湿润气候,境内水源丰富,其中有章水、贡水在境内合流成赣江。
城区位置特殊,被丘陵和章水、贡水、赣江合围环绕,山清水秀,被誉为“千里赣江第一城”。
发展在于规划,赣州市曾被誉为“中国宋城”在历尽千年辉煌之后,现面临着世纪转折的挑战:区域失位,中心缺位,城市发展后滞。
唯有在总体规划上寻求科学合理空间格局的突破与更人性化的规划设计,才能更好的发展赣州市,增强其中心城区的辐射能力。
文章通过到赣州市历史文化与城市建设博物馆和实地调研,结合历史资料数据和卫星影像数据,分析赣州市区在快速城市化背景下进行的空间规划合理性分析研究。
2 城市空间结构合理性分析在1996-2010年15年间,赣州市区的总体是根据《赣州城市总体规划》来进行规划的。
其规划目标是将赣州市建成赣粤闽湘通衙的区域性现代化中心城市。
为了城市空间总体结构分布合理性,文章以城市热岛指标为切入点,通过热岛空间分布变异与城市实际演变间的关系进行分析研究。
对于城市热岛的研究,获取城市地表温度是研究的先决条件。
有两种获取温度的方法,即传统定点或移动小车监测和遥感热红外反演,前者往往局限于有限的空间范围,同步性差、空间代表性差,而后者则具有大面积、时间性强、测量精确和低成本等优点,遥感观测已成为城市热岛反演的重要信息来源。
英文用得到
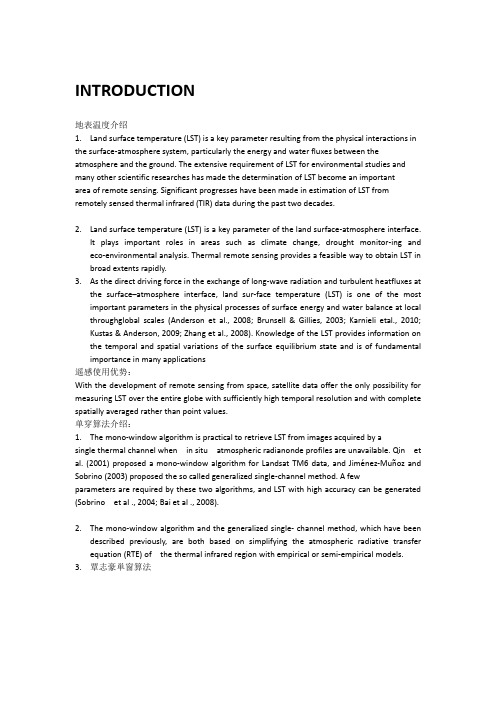
INTRODUCTION地表温度介绍nd surface temperature (LST) is a key parameter resulting from the physical interactions in the surface-atmosphere system, particularly the energy and water fluxes between the atmosphere and the ground. The extensive requirement of LST for environmental studies and many other scientific researches has made the determination of LST become an importantarea of remote sensing. Significant progresses have been made in estimation of LST from remotely sensed thermal infrared (TIR) data during the past two decades.nd surface temperature (LST) is a key parameter of the land surface-atmosphere interface.It plays important roles in areas such as climate change, drought monitor-ing and eco-environmental analysis. Thermal remote sensing provides a feasible way to obtain LST in broad extents rapidly.3.As the direct driving force in the exchange of long-wave radiation and turbulent heatfluxes atthe surface–atmosphere interface, land sur-face temperature (LST) is one of the most important parameters in the physical processes of surface energy and water balance at local throughglobal scales (Anderson et al., 2008; Brunsell & Gillies, 2003; Karnieli etal., 2010;Kustas & Anderson, 2009; Zhang et al., 2008). Knowledge of the LST provides information on the temporal and spatial variations of the surface equilibrium state and is of fundamental importance in many applications遥感使用优势:With the development of remote sensing from space, satellite data offer the only possibility for measuring LST over the entire globe with sufficiently high temporal resolution and with complete spatially averaged rather than point values.单穿算法介绍:1.The mono-window algorithm is practical to retrieve LST from images acquired by asingle thermal channel when in situ atmospheric radianonde profiles are unavailable. Qin et al. (2001) proposed a mono-window algorithm for Landsat TM6 data, and Jiménez-Muñoz and Sobrino (2003) proposed the so called generalized single-channel method. A fewparameters are required by these two algorithms, and LST with high accuracy can be generated (Sobrino et al ., 2004; Bai et al ., 2008).2.The mono-window algorithm and the generalized single- channel method, which have beendescribed previously, are both based on simplifying the atmospheric radiative transfer equation (RTE) of the thermal infrared region with empirical or semi-empirical models.3.覃志豪単窗算法普朗克函数:大气水汽含量Qin et al . (2001) proposed four models for estimating Ta of TM6 channel under four standard at-mospheric profiles with the near-surface air temperature T0, which can be derived from the in situ measurements of meteorological observations. Following the same routine, Duan et al. (2008) proposed four models for calculating Ta of IRS4, and these models have been listed in Table 2.环境卫星介绍1.The HJ-1A/B satellite of China, were launched on September 6, 2008, which are used for disaster and environment monitoring. It is a national project proposed by the Ministry of Environmental Protection and National Committee for Disaster Reduction. The HJ-1A andHJ-1B are on sun-synchronous orbits at the altitude of 650 km. The HJ-1A satellite isequipped with two CCD cameras and a hyper-spectral optical camera, while the HJ-B satellite is equipped with the same CCD cameras and one infrared scanner (IRS). Thespecifications of HJ-1B are shown in Table 12.HJ-1B satellite of China's constellation of small satel-lites for environment and disastermonitoring and fore-casting was launched on September 6, 2008. The infra-red scanner (IRS) on board HJ-1B satellite has a thermal channel (IRS4) with a spectral range of 10.5–12.5 μm.The radiometric resolution of IRS4 is better than 1.0 K. The nadir spatial resolution of IRS4 is 300 m and the swath width is 720 km.方法:METHODOLOGY普朗克函数运用VALIDATION and sensitivity analysis验证:投影坐标统一并重采样All the images are geo-referenced to the Universal Transverse Mercator(UTM) coordinate system and the HJ-1B/IRS LST images have been re-sampled to 1km pixel size to match the MODISLST products.1.验证结果,非常接近:The comparison result indicates that the HJ-1B/IRS LST is basically consistent with the MODIS LST product with areasonable accuracy.2.选取modis验证:In this research, in situ measurements of land surface temperature are unavailable, and validation of the pro- posed improved mono-window algorithm for IRS data is limited. Fortunately, the overpass time of HJ-1B satellite is close to that of Terra satellite (around 10:30 of local solar time in the morning), and the MODIS has excel-lent ability for monitoring the thermal radiation of the ground. The accuracy of the daily MODIS LST product, i.e. MOD11A1 and MYD11A1, isbetter than 1.0 K (Wan et al., 2002; Wan, 2008). Therefore, we use the MOD11A1 product as the "ground truth" to assess the accuracy of LST retrieved with the improved mono-win- dow algorithm.3.与modis对比之后:The spatial distribution patterns of land surface temperature derived by these two meth-ods are similar. LST derived from the MOD11A1 prod-uct is a little higher because the overpass time of Terra satellite is later than that of HJ-1B satellite. The mean absolute bias of the temperat ure of the lake surface es-timated based on the improved mono-window algorithm from that of MOD11A1 product is 0.7 K, indicating that the improved algorithm obtains a good accuracy for re-trieving land surface temperature from IRS4 data.影响因子分析的敏感性因子分析:CONCLUTIONAcknowledgementsREFERENCES。
英文版SEBS-ILWIS-help
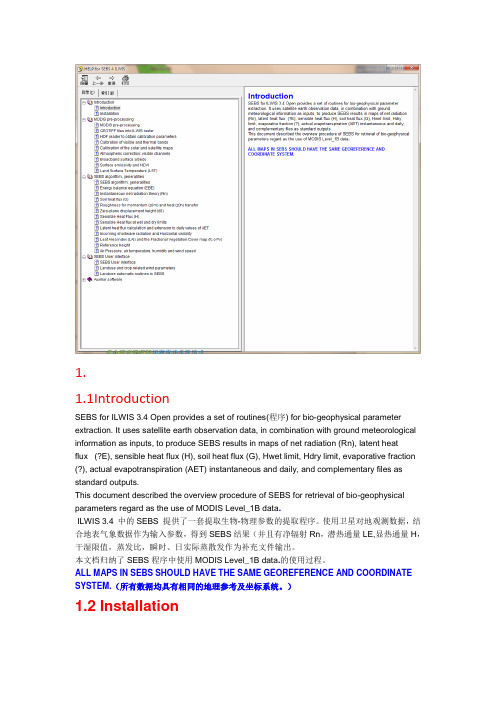
1.1.1IntroductionSEBS for ILWIS 3.4 Open provides a set of routines(程序) for bio-geophysical parameter extraction. It uses satellite earth observation data, in combination with ground meteorological information as inputs, to produce SEBS results in maps of net radiation (Rn), latent heatflux (?E), sensible heat flux (H), soil heat flux (G), Hwet limit, Hdry limit, evaporative fraction (?), actual evapotranspiration (AET) instantaneous and daily, and complementary files as standard outputs.This document described the overview procedure of SEBS for retrieval of bio-geophysical parameters regard as the use of MODIS Level_1B data.ILWIS 3.4 中的SEBS 提供了一套提取生物-物理参数的提取程序。
使用卫星对地观测数据,结合地表气象数据作为输入参数,得到SEBS结果(并且有净辐射Rn,潜热通量LE,显热通量H,干湿限值,蒸发比,瞬时、日实际蒸散发作为补充文件输出。
本文档归纳了SEBS程序中使用MODIS Level_1B data.的使用过程。
ALL MAPS IN SEBS SHOULD HAVE THE SAME GEOREFERENCE AND COORDINATE SYSTEM.(所有数据均具有相同的地理参考及坐标系统。
实习7、地表反射率、温度的反演以及植被指数的计算
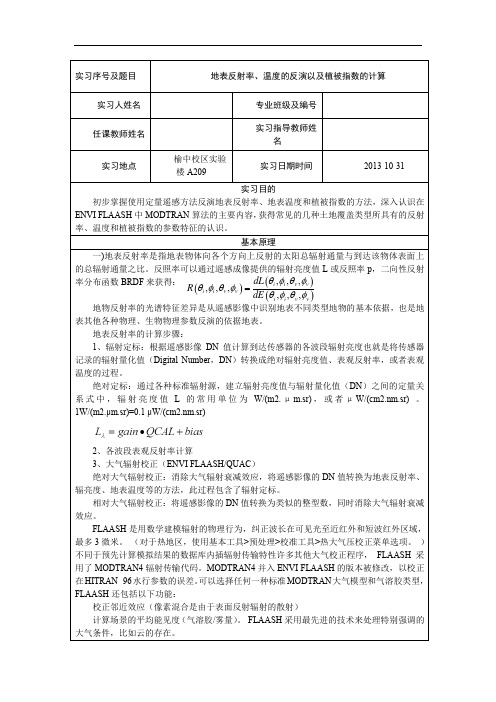
基本原理一)地表反射率是指地表物体向各个方向上反射的太阳总辐射通量与到达该物体表面上的总辐射通量之比。
反照率可以通过遥感成像提供的辐射亮度值L 或反照率p ,二向性反射率分布函数BRDF 来获得:地物反射率的光谱特征差异是从遥感影像中识别地表不同类型地物的基本依据,也是地表其他各种物理、生物物理参数反演的依据地表。
地表反射率的计算步骤:1、辐射定标:根据遥感影像DN 值计算到达传感器的各波段辐射亮度也就是将传感器记录的辐射量化值(Digital Number ,DN )转换成绝对辐射亮度值、表观反射率,或者表观温度的过程。
绝对定标:通过各种标准辐射源,建立辐射亮度值与辐射量化值(DN )之间的定量关系式中,辐射亮度值L 的常用单位为W/(m2.μm.sr),或者μW/(cm2.nm.sr) 。
1W/(m2.μm.sr)=0.1 μW/(cm2.nm.sr)2、各波段表观反射率计算3、大气辐射校正(ENVI FLAASH/QUAC )绝对大气辐射校正:消除大气辐射衰减效应,将遥感影像的DN 值转换为地表反射率、辐亮度、地表温度等的方法,此过程包含了辐射定标。
相对大气辐射校正:将遥感影像的DN 值转换为类似的整型数,同时消除大气辐射衰减效应。
FLAASH 是用数学建模辐射的物理行为,纠正波长在可见光至近红外和短波红外区域,最多3微米。
(对于热地区,使用基本工具>预处理>校准工具>热大气压校正菜单选项。
)不同于预先计算模拟结果的数据库内插辐射传输特性许多其他大气校正程序, FLAASH 采用了MODTRAN4辐射传输代码。
MODTRAN4并入ENVI FLAASH 的版本被修改,以校正在HITRAN -96水行参数的误差。
可以选择任何一种标准MODTRAN 大气模型和气溶胶类型,FLAASH 还包括以下功能:校正邻近效应(像素混合是由于表面反射辐射的散射) 计算场景的平均能见度(气溶胶/雾量)。
MODIS数据说明
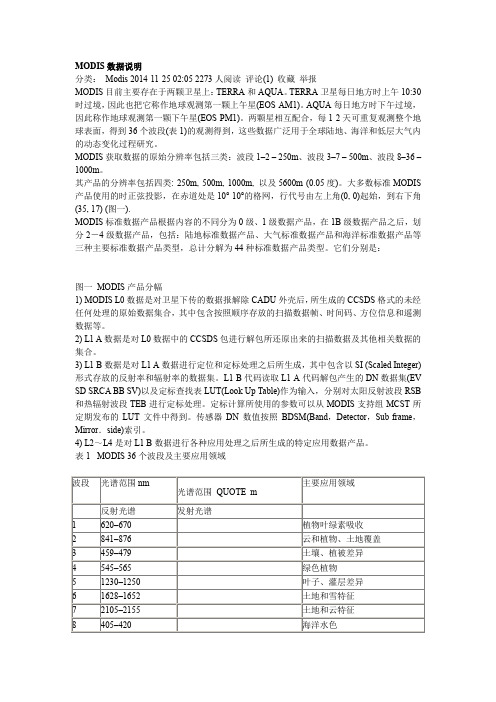
MODIS数据说明分类:Modis 2014-11-25 02:05 2273人阅读评论(1) 收藏举报MODIS目前主要存在于两颗卫星上:TERRA和AQUA。
TERRA卫星每日地方时上午10:30时过境,因此也把它称作地球观测第一颗上午星(EOS-AM1)。
AQUA每日地方时下午过境,因此称作地球观测第一颗下午星(EOS-PM1)。
两颗星相互配合,每1-2天可重复观测整个地球表面,得到36个波段(表1)的观测得到,这些数据广泛用于全球陆地、海洋和低层大气内的动态变化过程研究。
MODIS获取数据的原始分辨率包括三类:波段1–2 – 250m、波段3–7 – 500m、波段8–36 –1000m。
其产品的分辨率包括四类: 250m, 500m, 1000m, 以及5600m (0.05度)。
大多数标准MODIS 产品使用的时正弦投影,在赤道处是10° 10°的格网,行代号由左上角(0, 0)起始,到右下角(35, 17) (图一).MODIS标准数据产品根据内容的不同分为0级、1级数据产品,在1B级数据产品之后,划分2-4级数据产品,包括:陆地标准数据产品、大气标准数据产品和海洋标准数据产品等三种主要标准数据产品类型,总计分解为44种标准数据产品类型。
它们分别是:图一MODIS产品分幅1) MODIS L0数据是对卫星下传的数据报解除CADU外壳后,所生成的CCSDS格式的未经任何处理的原始数据集合,其中包含按照顺序存放的扫描数据帧、时间码、方位信息和遥测数据等。
2) L1 A数据是对L0数据中的CCSDS包进行解包所还原出来的扫描数据及其他相关数据的集合。
3) L1 B数据是对L1 A数据进行定位和定标处理之后所生成,其中包含以SI (Scaled Integer)形式存放的反射率和辐射率的数据集。
L1 B代码读取L1 A代码解包产生的DN数据集(EV SD SRCA BB SV)以及定标查找表LUT(Look Up Table)作为输入,分别对太阳反射波段RSB 和热辐射波段TEB进行定标处理。
作者姓名:阿布都瓦斯提·吾拉木
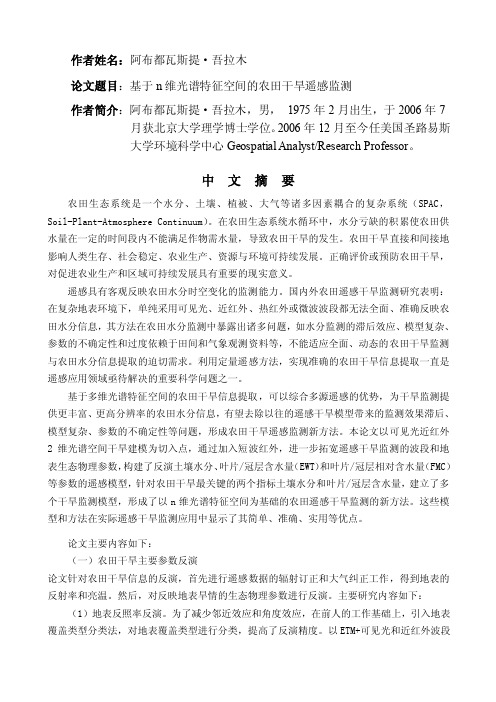
作者姓名:阿布都瓦斯提·吾拉木论文题目:基于n维光谱特征空间的农田干旱遥感监测作者简介:阿布都瓦斯提·吾拉木,男,1975年2月出生,于2006年7月获北京大学理学博士学位。
2006年12月至今任美国圣路易斯大学环境科学中心Geospatial Analyst/Research Professor。
中文摘要农田生态系统是一个水分、土壤、植被、大气等诸多因素耦合的复杂系统(SPAC,Soil-Plant-Atmosphere Continuum)。
在农田生态系统水循环中,水分亏缺的积累使农田供水量在一定的时间段内不能满足作物需水量,导致农田干旱的发生。
农田干旱直接和间接地影响人类生存、社会稳定、农业生产、资源与环境可持续发展。
正确评价或预防农田干旱,对促进农业生产和区域可持续发展具有重要的现实意义。
遥感具有客观反映农田水分时空变化的监测能力。
国内外农田遥感干旱监测研究表明:在复杂地表环境下,单纯采用可见光、近红外、热红外或微波波段都无法全面、准确反映农田水分信息,其方法在农田水分监测中暴露出诸多问题,如水分监测的滞后效应、模型复杂、参数的不确定性和过度依赖于田间和气象观测资料等,不能适应全面、动态的农田干旱监测与农田水分信息提取的迫切需求。
利用定量遥感方法,实现准确的农田干旱信息提取一直是遥感应用领域亟待解决的重要科学问题之一。
基于多维光谱特征空间的农田干旱信息提取,可以综合多源遥感的优势,为干旱监测提供更丰富、更高分辨率的农田水分信息,有望去除以往的遥感干旱模型带来的监测效果滞后、模型复杂、参数的不确定性等问题,形成农田干旱遥感监测新方法。
本论文以可见光近红外2维光谱空间干旱建模为切入点,通过加入短波红外,进一步拓宽遥感干旱监测的波段和地表生态物理参数,构建了反演土壤水分、叶片/冠层含水量(EWT)和叶片/冠层相对含水量(FMC)等参数的遥感模型,针对农田干旱最关键的两个指标土壤水分和叶片/冠层含水量,建立了多个干旱监测模型,形成了以n维光谱特征空间为基础的农田遥感干旱监测的新方法。
南京市冷热岛格局的尺度效应研究

文章编号:2096-3424(2021)01-0075-08DOI:10.3969/j.issn.2096-3424.20015南京市冷/热岛格局的尺度效应研究郭 宇, 唐 明, 王宏伟, 侯梅芳, 刘信杉, 林 毅(上海应用技术大学 生态技术与工程学院,上海 201418)摘 要:城市地表覆被类型变化影响城市热环境的空间格局,研究城市地表覆被类型与城市冷/热岛格局的相关关系,对调节城市微气候,改善人居环境,提高城市韧性具有重要的意义。
基于landsat8影像数据,提取南京市4种主要地表覆被类型(水体、植被、不透水面、建筑)的参数;同时利用热红外波段反演地表温度,分别从像元(小)和市、区级行政区划(大)2个尺度,研究冷/热岛空间分布与主要地表覆被类型之间的关系并就其尺度效应进行了深入探讨。
研究结果如下:基于像元尺度的空间分析表明,植被和水体的分布与冷岛空间格局具有相关性;不同地表覆被类型的降温效应顺序为:水体>植被>不透水面>建筑;建筑和不透水面的分布与热岛空间格局具有相关性。
基于市、区级行政区划尺度的空间分析表明,4种地表覆被类型与冷岛效应相关性较低;建筑与不透水面与热岛效应相关性较高,其中建筑与热岛效应相关性最高(R2=0.95)。
总体而言,基于小尺度的研究能反映地表覆被类型与冷/热岛空间分布的相关关系,而基于大尺度的研究则从数据统计的角度反映城市热环境的综合特征。
研究有助于城市规划者理解城市冷/热岛形成的尺度特征,为应对全球气候变化,合理规划城市布局,促进城市可持续发展提供参考。
关键词:城市热环境;冷/热岛;尺度特征;土地覆被类型中图分类号:TP79 文献标志码:AStudy on the Scale Effect of the Cold/Heat Islands Pattern in NanjingGUO Yu, TANG Ming, WANG Hongwei, HOU Meifang, LIU Xinshan, LIN Yi (School of Ecological Technique and Engineering, Shanghai Institute of Technology,Shanghai 201418, China)Abstract:Changes in urban land cover types affect the spatial pattern of urban thermal environments, and the relationship between urban land cover types and urban cold/heat island patterns is of great significance for regulating urban microclimate, improving human settlements and improving urban resilience. Based on the landsat8 Satellite imaging data, the parameters of the four main types of land cover (water, vegetation, impervious surface, building) in Nanjing were extracted, at the same time, the surface temperature was retrieved using the thermal infrared band. The relationship between the spatial distribution of cold/heat islands and the main land cover types was studied from two scales: pixel (small) and city and district administrative division (middle) , and the scale effect was discussed in depth. The results are as follows:收稿日期:2020-03-05基金项目:国家自然科学基金(41171250);上海高等学校一流研究生教育引领计划(沪教委高[2019]22号-24)资助作者简介:郭 宇(1995-),男,硕士研究生,主要研究方向为城市热环境。
专业英语气象科技英语第三课 气象预报

Serve as 充当,作为
P1①National Meteorological Services perform a variety of activities in order to provide weather forecasts. ②The principal ones are data collection, the preparation of basic analyses and prognostic charts of short-and long-term forecasts for the public as well as special services for aviation, shipping , agricultural and other commercial and industrial users, and the issuance of severe weather warnings.
stations for severe weather . ②Under the World
Weather Watch (WWW) program, synoptic reports are made at some 4,000 land stations and by 7,000
ships. ③There are about 700 stations making
P7: During the first half of the century, short-range forecasts were based on synoptic principles, empirical rules and extrapolation of pressure changes.
热红外遥感-DSB心得

热红外遥感心得体会-DSB热红外遥感是利用热红外波段研究地球物质特性的技术手段,可以获取地球表面温度,在城市热岛效应、林火监测、旱灾监测等领域有很好的应用价值。
由于热红外遥感涉及知识多而且深,特别是地表温度反演,需要大气传输、几个定律等方面的知识,本文用通俗语言总结了热红外遥感基本原理和方法,能知道热红外遥感怎么回事及简单的应用。
本文主要包括:●基本定义和原理●常见名词●简单应用与温度反演●ENVI下地表温度反演1、基本定义和原理热红外遥感(infrared remote sensing )是指传感器工作波段限于红外波段范围之内的遥感。
这是一个狭义的定义,只是说明的数据的获取。
另外一个广义的定义是:利用星载或机载传感器收集、记录地物的热红外信息,并利用这种热红外信息来识别地物和反演地表参数如温度、湿度和热惯量等。
热红外遥感的信息源来自物体本身,其基础是:只要其温度超过绝对零度,就会不断发射红外能量,即地表热红外辐射特性。
如下图为黑体的辐射光谱曲线(不同温度下物体辐射能量随波长变化的曲线),常温的地表物体(300K左右)发射的红外能量主要在大于3μm的中远红外区,即地表热辐射。
热辐射不仅与物质温度的表面状态有关,物质内部组成和温度对热辐射也有影响。
在大气传输过程中,地表热辐射能通过3-5μm和8-14μm两个窗口,这也是大多数传感器的设计波段范围。
热红外遥感在地表温度反演、城市热岛效应、林火监测、旱灾监测、探矿、探地热,岩溶区探水等领域都有很广的应用前景。
2、常见名词热红外遥感涉及的知识多而且深,下面来了解热红外遥感中几个基本的名词。
●辐射出射度单位时间内,从单位面积上辐射出的辐射能量称为辐射出射度,单位是wm-2●辐射亮度辐射源在某一方向上单位投影表面、单位立体角内的辐射通量,称为辐射亮度(Radiance),单位是瓦/平方米*微米*球面度( )。
很多地方会将辐射亮度和辐射强度区分,我这里理解的是一个概念。
科技英语11Speaking Practice
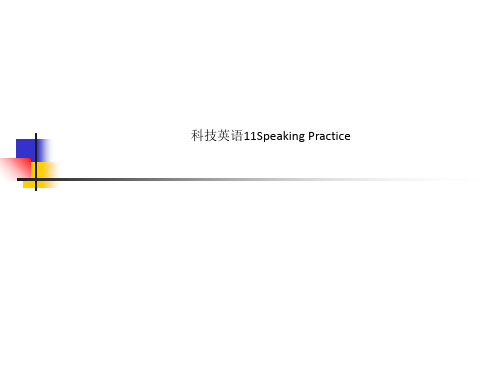
(4)Friendship is like a plant of slow growth. (友谊象生长着的 植物,是慢慢地建立起来的.)
M: No, only a letter written to you by a friend or family member
can really be called "snail mail". L: 噢,人们只有把亲友写的信叫做snail mail。说起写信,我真是应 该给我爸爸妈妈写信了。
M: I'm just too lazy to write snail mail. I think I'll go write some e-
After you. —— 您先。 About when? —— 大约何时? All set? —— 一切妥当? Allow me! —— 让我来!
Baloney! —— 胡扯!荒谬! Behave! —— 放尊重点! Bingo! —— 中了! Boring! —— 真无聊! Bravo! —— 太棒了! Bullshit! —— 胡说!
Yum... —— 恩...(好吃!)
Imagine! —— 想想看! Impossible! —— 不可能吧! Impressive! —— 很感人,永生难忘!
(1)A bosom friend afar brings a distant land near.(海内存知己, 天涯若比邻.)
Landsat 8版本1.1.0地表表面温度检索说明书
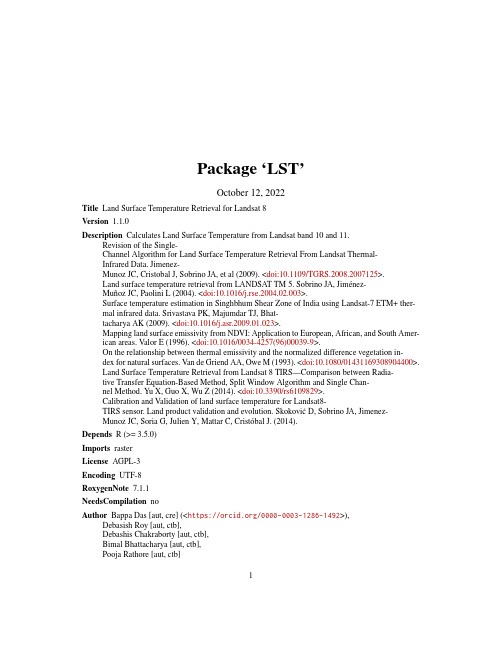
Package‘LST’October12,2022Title Land Surface Temperature Retrieval for Landsat8Version1.1.0Description Calculates Land Surface Temperature from Landsat band10and11.Revision of the Single-Channel Algorithm for Land Surface Temperature Retrieval From Landsat Thermal-Infrared Data.Jimenez-Munoz JC,Cristobal J,Sobrino JA,et al(2009).<doi:10.1109/TGRS.2008.2007125>.Land surface temperature retrieval from LANDSAT TM5.Sobrino JA,Jiménez-Muñoz JC,Paolini L(2004).<doi:10.1016/j.rse.2004.02.003>.Surface temperature estimation in Singhbhum Shear Zone of India using Landsat-7ETM+ther-mal infrared data.Srivastava PK,Majumdar TJ,Bhat-tacharya AK(2009).<doi:10.1016/j.asr.2009.01.023>.Mapping land surface emissivity from NDVI:Application to European,African,and South Amer-ican areas.Valor E(1996).<doi:10.1016/0034-4257(96)00039-9>.On the relationship between thermal emissivity and the normalized difference vegetation in-dex for natural surfaces.Van de Griend AA,Owe M(1993).<doi:10.1080/01431169308904400>.Land Surface Temperature Retrieval from Landsat8TIRS—Comparison between Radia-tive Transfer Equation-Based Method,Split Window Algorithm and Single Chan-nel Method.Yu X,Guo X,Wu Z(2014).<doi:10.3390/rs6109829>.Calibration and Validation of land surface temperature for Landsat8-TIRS nd product validation and evolution.Skokovi´c D,Sobrino JA,Jimenez-Munoz JC,Soria G,Julien Y,Mattar C,Cristóbal J.(2014).Depends R(>=3.5.0)Imports rasterLicense AGPL-3Encoding UTF-8RoxygenNote7.1.1NeedsCompilation noAuthor Bappa Das[aut,cre](<https:///0000-0003-1286-1492>),Debasish Roy[aut,ctb],Debashis Chakraborty[aut,ctb],Bimal Bhattacharya[aut,ctb],Pooja Rathore[aut,ctb]12BTMaintainer Bappa Das<*************************>Repository CRANDate/Publication2021-05-1707:30:23UTCR topics documented:BT (2)E_Skokovic (3)E_Sobrino (4)E_Valor (4)E_VandeGriend (5)E_Yu (6)MWA (6)NDVI (7)Pv (8)RTE (9)SCA (10)SW A (11)Ta (12)tau (12)Index14 BT At-Sensor Temperature or brightness temperatureDescriptionThis function calculates at-Sensor Temperature or brightness temperatureUsageBT(Landsat_10=Landsat_10,Landsat_11=Landsat_10)ArgumentsLandsat_10Raster*object,Landsat band10Landsat_11Raster*object,Landsat band11ValueA list containing brightness temperature corresponding to Landsat band10and Landsat band11E_Skokovic3 Examplesa<-raster::raster(ncol=100,nrow=100)set.seed(2)raster::values(a)=runif(10000,min=27791,max=30878)b<-raster::raster(ncol=100,nrow=100)set.seed(2)raster::values(b)=runif(10000,min=25686,max=28069)BT(Landsat_10=a,Landsat_11=b)E_Skokovic Land Surface Emissivity according to Skokovic et al.2014DescriptionThis function calculates Land Surface Emissivity according to Skokovic et al.2014UsageE_Skokovic(red=red,NDVI=NDVI,band=band)Argumentsred Raster*object,red band of remote sensing imageryNDVI Raster*object,NDVI calculated from remote sensing imageryband A string specifying which Landsat8thermal band to use.It can be"band10"or "band11"ValueRasterLayerExamplesred<-raster::raster(ncol=100,nrow=100)set.seed(2)raster::values(red)=runif(10000,min=0.1,max=0.4)NDVI<-raster::raster(ncol=100,nrow=100)set.seed(2)raster::values(NDVI)=runif(10000,min=0.02,max=0.8)E_Skokovic(red=red,NDVI=NDVI,band="band11")4E_Valor E_Sobrino Land Surface Emissivity according to Sobrino et al.2008DescriptionThis function calculates Land Surface Emissivity according to Sobrino et al.2008UsageE_Sobrino(red=red,NDVI=NDVI)Argumentsred Raster*object,red band of remote sensing imageryNDVI Raster*object,NDVI calculated from remote sensing imageryValueRasterLayerExamplesred<-raster::raster(ncol=100,nrow=100)set.seed(2)raster::values(red)=runif(10000,min=0.1,max=0.4)NDVI<-raster::raster(ncol=100,nrow=100)set.seed(2)raster::values(NDVI)=runif(10000,min=0.02,max=0.8)E_Sobrino(red=red,NDVI=NDVI)E_Valor Land Surface Emissivity according to Valor and Caselles1996DescriptionThis function calculates Land Surface Emissivity according to Valor and Caselles1996UsageE_Valor(NDVI)ArgumentsNDVI Raster*object,NDVI calculated from remote sensing imageryE_VandeGriend5 ValueRasterLayerExamplesNDVI<-raster::raster(ncol=100,nrow=100)set.seed(2)raster::values(NDVI)=runif(10000,min=0.02,max=0.8)E_Valor(NDVI)E_VandeGriend Land Surface Emissivity according to Van de Griend and Owe1993DescriptionThis function calculates Land Surface Emissivity according to Van de Griend and Owe1993UsageE_VandeGriend(NDVI)ArgumentsNDVI Raster*object,NDVI calculated from remote sensing imageryValueRasterLayerExamplesNDVI<-raster::raster(ncol=100,nrow=100)set.seed(2)raster::values(NDVI)=runif(10000,min=0.02,max=0.8)E_VandeGriend(NDVI)6MW A E_Yu Land Surface Emissivity according to Yu et al.2014DescriptionThis function calculates Land Surface Emissivity according to Yu et al.2014UsageE_Yu(red=red,NDVI=NDVI,band=band)Argumentsred Raster*object,red band of remote sensing imageryNDVI Raster*object,NDVI calculated from remote sensing imageryband A string specifying which Landsat8thermal band to use.It can be"band10"or "band11"ValueRasterLayerExamplesred<-raster::raster(ncol=100,nrow=100)set.seed(2)raster::values(red)=runif(10000,min=0.1,max=0.4)NDVI<-raster::raster(ncol=100,nrow=100)set.seed(2)raster::values(NDVI)=runif(10000,min=0.02,max=0.8)E_Yu(red=red,NDVI=NDVI,band="band11")MWA Mono window algorithmDescriptionThis function calculates Land Surface Temperature using mono window algorithmUsageMWA(BT=BT,tau=tau,E=E,Ta=Ta)NDVI7 ArgumentsBT Raster*object,brightness temperaturetau Atmospheric transmittanceE Raster*object,Land Surface Emissivity calculated according to Van de Griendand Owe1993or Valor and Caselles1996or Sobrino et al.2008 Ta Mean atmospheric temperature(K)of the date when Landsat passed over the study areaValueRasterLayerExamplesBTemp<-raster::raster(ncol=100,nrow=100)set.seed(2)raster::values(BTemp)=runif(10000,min=298,max=305)E<-raster::raster(ncol=100,nrow=100)set.seed(2)raster::values(E)=runif(10000,min=0.96,max=0.99)MWA(BT=BTemp,tau=0.86,E=E,Ta=26)NDVI NDVIDescriptionFunction for NDVI calculationUsageNDVI(Red,NIR)ArgumentsRed Raster*object,red band of remote sensing imageryNIR Raster*object,NIR band of remote sensing imageryValueRasterLayer8Pv Examplesred<-raster::raster(ncol=100,nrow=100)set.seed(2)raster::values(red)=runif(10000,min=0.1,max=0.4)NIR<-raster::raster(ncol=100,nrow=100)set.seed(2)raster::values(NIR)=runif(10000,min=0.1,max=0.6)NDVI(Red=red,NIR=NIR)Pv Proportion of vegetation or fractional vegetation coverDescriptionCalculation of the proportion of vegetation or fractional vegetation cover from NDVIUsagePv(NDVI,minNDVI,maxNDVI)ArgumentsNDVI Raster*object,NDVI calculated from remote sensing imageryminNDVI=0.2(Ref.Sobrino et al.2004)maxNDVI=0.5(Ref.Sobrino et al.2004)ValueRasterLayerExamplesNDVI<-raster::raster(ncol=100,nrow=100)set.seed(2)raster::values(NDVI)=runif(10000,min=0.02,max=0.8)Pv(NDVI=NDVI,minNDVI=0.2,maxNDVI=0.5)RTE9 RTE Radiative transfer equation methodDescriptionThis function calculates Land Surface Temperature using radiative transfer equation methodUsageRTE(TIR=TIR,tau=tau,E=E,dlrad=dlrad,ulrad=ulrad,band=band)ArgumentsTIR Raster*object,Landsat band10or11tau Atmospheric transmittanceE Raster*object,Land Surface Emissivity calculated according to Van de Griendand Owe1993or Valor and Caselles1996or Sobrino et al.2008 dlrad Downwelling radiance calculated from https:///ulrad upwelling radiance calculated from https:///band A string specifying which Landsat8thermal band to use.It can be"band10"or "band11"ValueRasterLayerExamplesTIR<-raster::raster(ncol=100,nrow=100)set.seed(2)raster::values(TIR)=runif(10000,min=27791,max=30878)BT<-raster::raster(ncol=100,nrow=100)set.seed(2)raster::values(BT)=runif(10000,min=298,max=305)E<-raster::raster(ncol=100,nrow=100)set.seed(2)raster::values(E)=runif(10000,min=0.96,max=0.99)Ts_RTE<-RTE(TIR=TIR,tau=0.86,E=E,dlrad=2.17,ulrad=1.30,band="band11")10SCA SCA Single channel algorithmDescriptionThis function calculates Land Surface Temperature using single channel algorithmUsageSCA(TIR=TIR,BT=BT,tau=tau,E=E,dlrad=dlrad,ulrad=ulrad,band=band)ArgumentsTIR Raster*object,Landsat band10or11BT Raster*object,brightness temperaturetau Atmospheric transmittanceE Raster*object,Land Surface Emissivity calculated according to Van de Griendand Owe1993or Valor and Caselles1996or Sobrino et al.2008 dlrad Downwelling radiance calculated from https:///ulrad upwelling radiance calculated from https:///band A string specifying which Landsat8thermal band to use.It can be"band10"or "band11"ValueRasterLayerExamplesTIR<-raster::raster(ncol=100,nrow=100)set.seed(2)raster::values(TIR)=runif(10000,min=27791,max=30878)BT<-raster::raster(ncol=100,nrow=100)set.seed(2)raster::values(BT)=runif(10000,min=298,max=305)E<-raster::raster(ncol=100,nrow=100)set.seed(2)raster::values(E)=runif(10000,min=0.96,max=0.99)Ts_SCA<-SCA(TIR=TIR,BT=BT,tau=0.86,E=E,dlrad=2.17,ulrad=1.30,band="band11")SWA11 SWA Split-window algorithmDescriptionThis function calculates Land Surface Temperature using split-window algorithmUsageSWA(TIR_10=TIR_10,TIR_11=TIR_11,tau_10=tau_10,tau_11=tau_11,E_10=E_10,E_11=E_11)ArgumentsTIR_10Raster*object,Landsat band10TIR_11Raster*object,Landsat band11tau_10Atmospheric transmittance for Landsat band10tau_11Atmospheric transmittance for Landsat band11E_10Raster*object,Land Surface Emissivity for Landsat band10calculated accord-ing to Skokovic et al.2014or Yu et al.2014E_11Raster*object,Land Surface Emissivity for Landsat band11calculated accord-ing to Skokovic et al.2014or Yu et al.2014ValueRasterLayerExamplesTIR_10<-raster::raster(ncol=100,nrow=100)set.seed(2)raster::values(TIR_10)=runif(10000,min=27791,max=30878)TIR_11<-raster::raster(ncol=100,nrow=100)set.seed(2)raster::values(TIR_11)=runif(10000,min=25686,max=28069)E_10<-raster::raster(ncol=100,nrow=100)set.seed(1)raster::values(E_10)=runif(10000,min=0.96,max=0.99)E_11<-raster::raster(ncol=100,nrow=100)set.seed(2)raster::values(E_11)=runif(10000,min=0.96,max=0.99)12tau Ts_SWA<-SWA(TIR_10=TIR_10,TIR_11=TIR_11,tau_10=0.86,tau_11=0.87,E_10=E_10,E_11=E_11)Ta Mean atmospheric temperatureDescriptionThis function calculates mean atmospheric temperature(Ta)using near-surface air temperature(To)UsageTa(To=To,mod=mod)ArgumentsTo Near-surface air temperature(°C)of the date when Landsat passed over the study areamod A string specifying which model to use.It can be anyone of"USA1976Stan-dard"or"Tropical Region"or"Mid-latitude Summer Region"or"Mid-latitudeWinter Region"ValueMean atmospheric temperature(K)ExamplesTa(To=26,mod="Mid-latitude Winter Region")tau Atmospheric transmittance calculationDescriptionThis function calculates Atmospheric transmittance from near-surface air temperature(To,°C)and relative humidity(RH,%)of the date when Landsat passed over the study areaUsagetau(To=To,RH=To,band=band)tau13ArgumentsTo Near-surface air temperature(°C)of the date when Landsat passed over the study areaRH relative humidity(%)of the date when Landsat passed over the study area band A string specifying which Landsat8thermal band to use.It can be"band10"or "band11"ValueAtmospheric transmittanceExamplestau(To=26,RH=42,band="band11")IndexBT,2E_Skokovic,3E_Sobrino,4E_Valor,4E_VandeGriend,5E_Yu,6MWA,6NDVI,7Pv,8RTE,9SCA,10SWA,11Ta,12tau,1214。
基于Landsat TM 武汉市地表温度反演研究
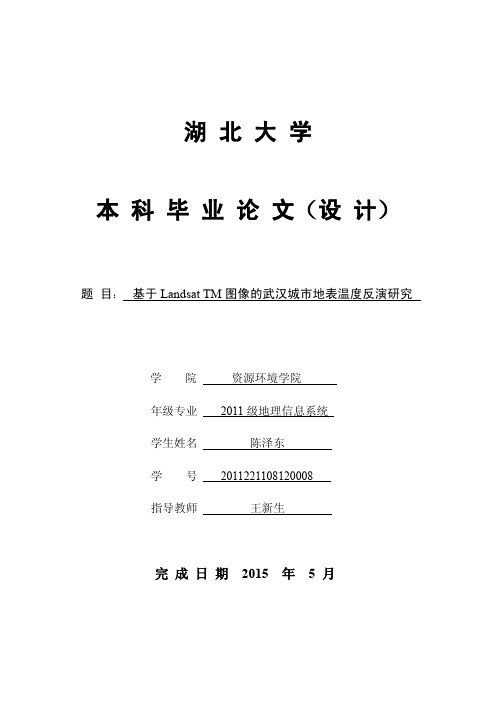
1.1 研究背景
地表能量交换信息的获取是监测区域资源环境变化的一个重要环节。 地表温度是地表能量 平衡的决定因素之一。因此,获取区域地表温度空间差异,并进而分析其对区域资源环境变 化的影响, 是区域资源环境动态监测的重要内容。 传统的的地表温度测量是根据有限的观测 点的数据来推论分析区域的地表温度差异。近20 年来,热红外遥感技术的飞速发展为快速 地获取区域地表温度空间差异信息提供了新的途径。 地表温度在区域资源环境研究中的重要 性已经使热红外遥感成为遥感研究的一个重要领域, 目前已经开发了很多实用的地表温度遥 感反演方法,如热辐射传输方程法、劈窗算法、单窗算法和多通道算法。许多算法是针对具 体的传感器开发的,例如劈窗算法是用来从具有两个热红外波段的NOAA/AVHRR 数据中反演 地表温度,而单窗算法则主要是用于只有一个热红外波段的LandsatTMTM 图像的武汉城市地表温度反演研究
摘 要
利用武汉市 LandsatTM 热红外波段数据, 采用覃志豪等人提出的单窗算法反演得到武汉市 地表温度分布图。从反演结果可以看出,武汉市城区地表温度比郊区温度高,水域的温度最 低。同时分别对 1991 年和 2005 年的影像数据进行地表温度反演,通过比分析,随着城区的 建设,武汉市温度分布模式发生较大变化。
I
湖北大学本科毕业论文(设计)
1 绪论
地表温度是很多环境模型的一个重要参数, 在大气与地表的能量与物质交换, 天气预报, 全球洋流循环, 气候变化等研究领域有重要的应用。 利用热红外遥感可以得到大范围的地表 温度面状信息, 与传统的地表温度测量方式相比, 具有快速、便捷、测量范围大、信息连续 等特点, 因此利用热红外遥感数据反演地面温度得到了广泛的应用。
目 录
1 绪论……………………………………………………………………………… 1 1.1 研究背景…………………………………………………………………… 1 1.2 研究目标和内容…………………………………………………………… 1 1.3 现阶段的研究进展………………………………………………………… 1 2 比辐射率与地表温度的基本概念……………………………………………… 2 2.1 普朗克公式与黑体辐射…………………………………………………… 2 2.2 比辐射率…………………………………………………………………… 2 2.3 地表温度…………………………………………………………………… 3 2.4 辐射温度…………………………………………………………………… 3 2.5 亮度温度…………………………………………………………………… 3 2.6 地表温度反演的常见方法模型…………………………………………… 3 3 单窗算法地表温度反演模型分析……………………………………………… 4 3.1 陆地卫星 TM6 波段范围内地表比辐射率的估计………………………… 4 3.1.1 地表比辐射率的估计方法………………………………………… 3.1.2 地表构成比例的确定……………………………………………… 5 5
基于MODIS和TM数据的陆面温度反演
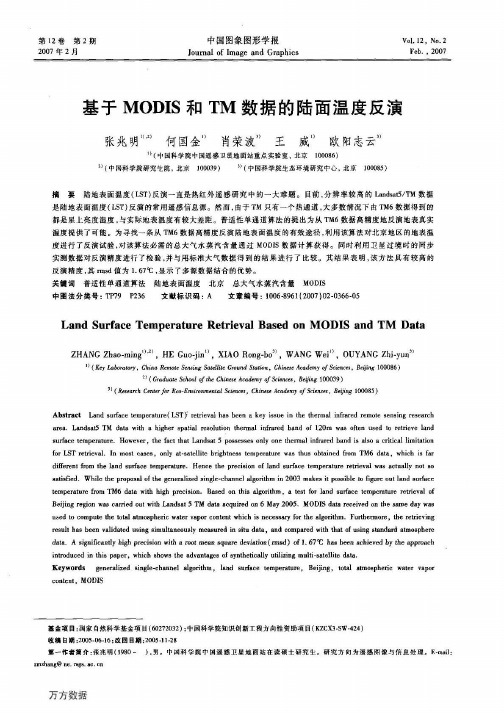
2007 年 2 月
中国图象图形学报 Journal of Image and Graphics
Vol. 12, No. 2 Feb., 2007
基于 MODIS 和 TM 数据的陆面温度反演
张兆明 吟,2)
I)
何国金川
100039)
肖荣波”
王
威 I)
欧阳志云3)
100086) 100085)
(I) <2) (3)
已经在资源环境等领域得到了广泛应用。 TM6 为 热红外波段,空间分辨率为 120m ,与 NOAA/AVHRR
γ -{一→一1-L - rc2L ••••。,fλ‘. , 1-· + λI ~ t r ....。, i c, r ,. JJ
…
/j
= - γL.....曹 , + r.....,
content, MODIS
基金项目 :随家自然科学基金项目( 60272032 ):中国科学院知识创新工程方向性资助项目( KZCX3 -SW-424 )
收稿日期:2005-06-16 ;放回日摘自:2005-l 1-28
’自-f官司旨简介 t 张兆明 (1980 向
),男。’和国科学院小国遥感卫星地商站在读硕士研究生。研究方向为遥感图像与信息处理。 F.-mail:
atm。sphere
data. A significantly high precision with a root mean square deviation ( rmsd) of l. 67 ℃ has been achieved by the approach generalized single-channel algorithm, land surface temperature, Beiji吨, total atmospheric water vapor
利用envi反演地表温度

1.遥感数据预处理影像数据均经过精确地理校正,并具有相同的投影坐标系统(WGS84),Landsat TM5数据中TM l~5波段为多光谱波段,空间分辨率为30m,第6波段为热红外波段,空间分辨率为120米,经过重采样统一为30米。
利用ENVI5.0SP3软件自带Radiometric Calibration 工具对多光谱波段进行辐射定标(同时以区域矢量边界为辅助数据,对经过辐射定标的影像进行裁剪)。
2.地表温度反演Landsat TM5影像第6波段为热红外辐射波段,接收的热红外强度与地表温度高低正相关,可转化为地表的实际温度。
按照以下步骤对地表温度进行反演:○1黑体辐射亮度值(Tb:Brightness Temperature):利用ENVI5.0SP3软件平台Band Math工具,通过公式(3-1)将TM热红外波段(第六波段)像元值D N值转变为黑体的辐射亮温值:Lλ=L MIN+L MAX−L MIN255∗D N(3-1)式中,D N为像元灰度值;Lλ为地物在光谱λ处(单位μm,文中λ取波段中间值11.45μm)处的热辐射值(单位:W·m-2·sr-1·μm-1),L MIN表示TM热红外波段D N为0时的热辐射亮度值,L MAX表示TM 热红外波段D N为最大值时的热辐射值。
L MIN =1.2378 W·m-2·sr-1·μm-1,L MAX=15.303W·m-2·sr-1·μm-1。
假设热红外波段在不同下垫面的地表发射率相同,利用普朗克公式反函数公式(3-2)将计算得出的热辐射值Lλ转换成传感器端的有效亮温(Tb):T b=K2ln(1+K1Lλ)(3-2)式中,Tb为有效亮温值,K1、K2为定标系数,其中K1=607.76 W·m-2·sr-1·μm-1,K2=1260.56K ○2地表比辐射率(ε):利用ENVI5.0SP3软件平台快速大气校正工具(QUAC)对经过裁剪的辐射定标后的多光谱波段数据进行大气校正,通过公式(3-3)获得归一化植被指数(NDVI:Normalized Difference Vegetation Index)NDVI=NIR−RNIR+R(3-3)式中,NIR和R分别是TM影像的近红外波段(波段4)和红光波段(波段3)的灰度值(D N)在获取的归一化植被指数(NDVI)基础上,采用混合像元分解法计算植被覆盖度(Pv),依据经验值,当NDVI>0.5时,下垫面为植被,植被覆盖度(Pv)取1;当NDVI<0.2时,下垫面为裸露土地,植被覆盖度(Pv)取0;当0.2≤NDVI≤0.5时,依照公式(3-4)计算植被覆盖度(Pv)。
基于单窗算法反演地表温度的ENVI操作教程

单窗算法反演地表温度教程1.1 算法原理1.1.1 单窗算法单窗算法(MW 算法)是覃志豪于2001年提出的针对TM 数据只有一个热红外波段的地面温度反演算法。
经过众多学者验证,单窗算法具有很高的反演精度,且同样适用于ETM+和landsat 8数据。
公式如下:式中,LST 为地表温度(K ),T sensor 是传感器上的亮度温度(K ),T a 是大气平均温度(K );a 、b 为参考系数,当地表温度为0-70℃时,a = -67.355351,b = 0.458606;C 、D 为中间变量,计算公式为:式中,为地表比辐射率,为地面到传感器的大气总透射率。
因此单窗算法反演地表温度的关键是计算得到亮度温度T senso 、地表比辐射率、大气透射率和大气平均作用温度T a 。
1.1.2 参数计算1.1.2.1 辐射亮温计算利用Planck 公式将图像像元对应传感器辐射强度值转换为对应的亮度温度值。
公式如下6666666666/)))1(()1((C T D T D C D C b D C a T a sensor s -++--+--=式中,T senso 为亮度温度值;λL 影像预处理后得到的光谱辐射值,单位为)/(2m sr m w μ⋅⋅,K1 、K2为常量,可由数据头文件获取。
计算图像辐射亮温之前,需采用辐射定标参数将像元灰度值DN 转换为热辐射强度值,公式如下:式中,M L 为增益参数,A L 为偏移参数,该参数可直接在影像通文件数据中获取,且ENVI 软件中已经集成,不需要自己在查找。
1.1.2.2 地表比辐射率计算根据覃志豪针对TM 影像提出的混合像元分解法来确定区域地表福辐射率。
对于城市区域,我们简单的将其分为水体、自然表面和建筑表面三种,因此针对混合像元尺度上的地表比辐射率通过下式来估算:式中,为混合像元的地表比辐射率;P V 为植被覆盖率;R V 为植被的温度比率;R M 为建筑表面的温度比率;V 表示植被法地表比辐射率,m 表示建筑表面的地表比辐射率;d 表示辐射校正项。
SRTP结题报告

西南交通大学第五期大学生科研训练计划(SRTP)遥感与气象观测数据耦合的地表干旱状态监测结题报告2010年4月至2011年4月目录目录_______________________________________________________________ 11绪论 ____________________________________________________________ 21. 1项目背景 _________________________________________________________________ 21. 2项目介绍 _________________________________________________________________ 31. 3 开源库GDAL与HDF文件格式介绍________________________________________ 42数据源及GDAL库的配置 __________________________________________ 72. 1 数据源__________________________________________________________________ 72. 2 GDAL库的配置 ___________________________________________________________ 93数据处理原理及实现 _____________________________________________ 103. 1 遥感数据处理___________________________________________________________ 103.2 气象数据处理与降水空间插值法比较 _____________________________________ 203. 3 指数耦合 _______________________________________________________________ 263. 4 程序设计 _______________________________________________________________ 27 4实验结果分析 ___________________________________________________ 27 5不足与期望 _____________________________________________________ 30 6项目感想 _______________________________________________________ 31参考文献__________________________________________________________ 32附录1 程序主要源代码 ______________________________________________ 341绪论1.1项目背景我国是一个旱灾非常严重的国家,旱灾给农业、农村和农民造成了巨大的损失。
envi中lst的计算方法
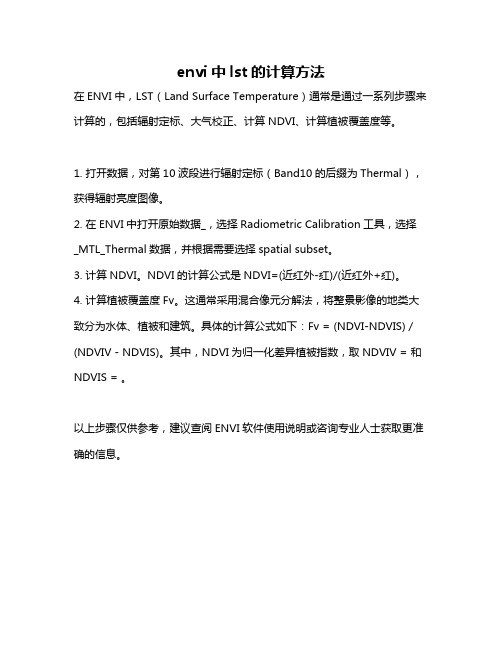
envi中lst的计算方法
在ENVI中,LST(Land Surface Temperature)通常是通过一系列步骤来计算的,包括辐射定标、大气校正、计算NDVI、计算植被覆盖度等。
1. 打开数据,对第10波段进行辐射定标(Band10的后缀为Thermal),获得辐射亮度图像。
2. 在ENVI中打开原始数据_,选择Radiometric Calibration工具,选择_MTL_Thermal数据,并根据需要选择spatial subset。
3. 计算NDVI。
NDVI的计算公式是NDVI=(近红外-红)/(近红外+红)。
4. 计算植被覆盖度Fv。
这通常采用混合像元分解法,将整景影像的地类大致分为水体、植被和建筑。
具体的计算公式如下:Fv = (NDVI-NDVIS)/(NDVIV - NDVIS)。
其中,NDVI为归一化差异植被指数,取NDVIV = 和NDVIS = 。
以上步骤仅供参考,建议查阅ENVI软件使用说明或咨询专业人士获取更准确的信息。
兴安盟区域断裂带与地表温度场变化特征研究

包宝小,席文雅,王志勇,等. 兴安盟区域断裂带与地表温度场变化特征研究[J]. 地震科学进展, 2023, 53(9): 416-422.doi:10.19987/j.dzkxjz.2023-051Bao B X, Xi W Y, Wang Z Y, et al. Study on characteristics of regional fault zone and surface temperature change in Xing’an League[J]. Progress in Earthquake Sciences, 2023, 53(9): 416-422. doi:10.19987/j.dzkxjz.2023-051兴安盟区域断裂带与地表温度场变化特征研究*包宝小※ 席文雅 王志勇 李腾宇 王 慧 贾宝金(内蒙古自治区地震局乌兰浩特地震监测中心站, 内蒙古兴安盟 137400)摘要 本文基于Landsat 8 OLI/TIRS 遥感影像及最新断裂带矢量数据,分析研究兴安盟区域断裂带与地表温度变化的相关特征。
为了提高地表温度反演的准确性,使用最大似然分类算法进行了地表物的分类,然后使用辐射传输方程求解算法分类逆推地表温度。
根据研究区域矢量数据绘制的断裂带构造图与遥感影像反演的相同区域的地表温度图,使用Arcmap 处理软件,将两幅影像叠加在同一图层上,使用等间距分段均值划分方法,研究分析兴安盟区域断裂带构造地表温度场变化特征,绘制等分断裂带内的平均距离与平均地表温度数据的相关性曲线,进一步确认了断裂带与地表温度场变化的相关性特征。
最后,利用同月份不同年份的遥感影像数据进行实验结果的准确性验证。
结果表明:兴安盟区域断裂带与地表温度场之间存在一定的相关性,地表温度会随着断裂带等分带距离远近表现出不同程度的变化,越靠近断裂带,地表温度值越高,越远,地表温度值越低。
关键词 遥感影像;地表温度;断裂带;相关性特征中图分类号:P315.6 文献标识码: A 文章编号: 2096-7780(2023)09-0416-07doi :10.19987/j.dzkxjz.2023-051Study on characteristics of regional fault zone and surfacetemperature change in Xing’an LeagueBao Baoxiao, Xi Wenya, Wang Zhiyong, Li Tengyu, Wang Hui, Jia Baojin (Wulanhot Earthquake Monitoring Center Station, Earthquae Agency of Inner MongoliaAutonomous Region, Inner Mongolia Xing’an League 137400, China )Abstract Based on Landsat 8 OLI/TIRS remote sensing images and the latest fault zone vector data ,this paper analyzes and studies the characteristics of the regional fault zone and land surface temperature changes in the Xing’an League region. For the accuracy of land surface temperature inversion ,the maximum likelihood classification algorithm is used to classify the surface objects ,and then the radiative transfer equation algorithm is used to classify and retrieve the land surface temperature. The surface temperature inversion image and the fault zone structure image are superimposed on the same layer ,and the average surface temperature and the average distance in each isozonal zone are calculated by using the method of equal interval segmented mean division ,the change characteristics of surface temperature field in fault structure are shown by graph ,and the relationship between fault zone and surface temperature field is further* 收稿日期:2023-04-17;采用日期:2023-09-06。
分裂窗算法
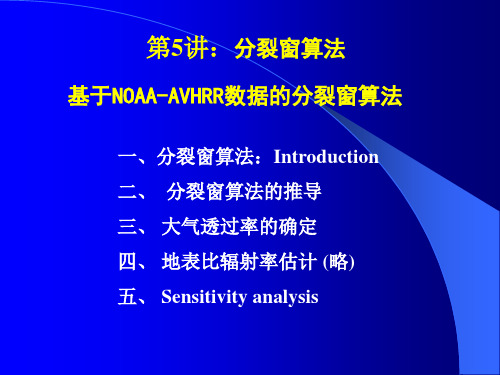
(d) 陆面温度可能大大高于海面温度。因此,针对海表面建立 进来的Planck方程的线性化,可能不适用于陆面温度的变化 范围。此外,AVHRR遥感器在320K(47C)达到饱和,在观测 一些很热环境的LST时,这可能会引起一些问题。 e) 在一个AVHRR像元范围内,LST可能会有很大的变化。这 就会引起所观测到的温度值的确切含义有难以理解和实际意 义问题,因为这种温度观测是在至少1.2km2的面积范围内进 行取值。类似地,“表面”在植被地区可能很难确切地定义。
MODIS数据说明
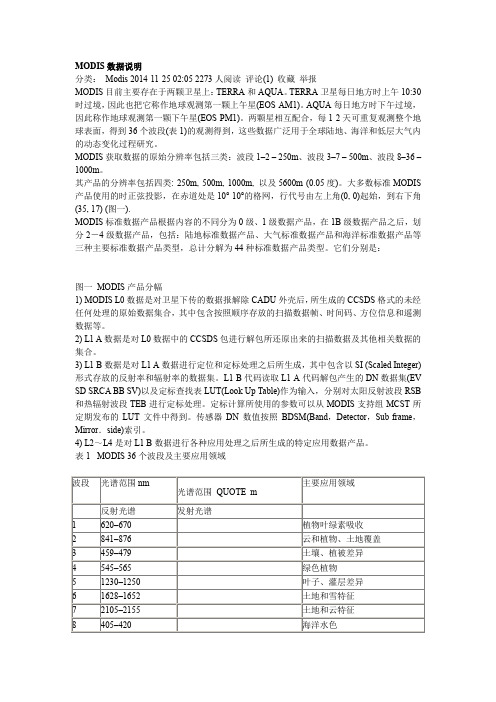
MODIS数据说明分类:Modis 2014-11-25 02:05 2273人阅读评论(1) 收藏举报MODIS目前主要存在于两颗卫星上:TERRA和AQUA。
TERRA卫星每日地方时上午10:30时过境,因此也把它称作地球观测第一颗上午星(EOS-AM1)。
AQUA每日地方时下午过境,因此称作地球观测第一颗下午星(EOS-PM1)。
两颗星相互配合,每1-2天可重复观测整个地球表面,得到36个波段(表1)的观测得到,这些数据广泛用于全球陆地、海洋和低层大气内的动态变化过程研究。
MODIS获取数据的原始分辨率包括三类:波段1–2 – 250m、波段3–7 – 500m、波段8–36 –1000m。
其产品的分辨率包括四类: 250m, 500m, 1000m, 以及5600m (0.05度)。
大多数标准MODIS 产品使用的时正弦投影,在赤道处是10° 10°的格网,行代号由左上角(0, 0)起始,到右下角(35, 17) (图一).MODIS标准数据产品根据内容的不同分为0级、1级数据产品,在1B级数据产品之后,划分2-4级数据产品,包括:陆地标准数据产品、大气标准数据产品和海洋标准数据产品等三种主要标准数据产品类型,总计分解为44种标准数据产品类型。
它们分别是:图一MODIS产品分幅1) MODIS L0数据是对卫星下传的数据报解除CADU外壳后,所生成的CCSDS格式的未经任何处理的原始数据集合,其中包含按照顺序存放的扫描数据帧、时间码、方位信息和遥测数据等。
2) L1 A数据是对L0数据中的CCSDS包进行解包所还原出来的扫描数据及其他相关数据的集合。
3) L1 B数据是对L1 A数据进行定位和定标处理之后所生成,其中包含以SI (Scaled Integer)形式存放的反射率和辐射率的数据集。
L1 B代码读取L1 A代码解包产生的DN数据集(EV SD SRCA BB SV)以及定标查找表LUT(Look Up Table)作为输入,分别对太阳反射波段RSB 和热辐射波段TEB进行定标处理。
- 1、下载文档前请自行甄别文档内容的完整性,平台不提供额外的编辑、内容补充、找答案等附加服务。
- 2、"仅部分预览"的文档,不可在线预览部分如存在完整性等问题,可反馈申请退款(可完整预览的文档不适用该条件!)。
- 3、如文档侵犯您的权益,请联系客服反馈,我们会尽快为您处理(人工客服工作时间:9:00-18:30)。
Land surface temperature from Ka band(37GHz)passivemicrowave observationsT.R.H.Holmes,1R.A.M.De Jeu,1M.Owe,2and A.J.Dolman1Received11April2008;revised21November2008;accepted17December2008;published25February2009.[1]An alternative to thermal infrared satellite sensors for measuring land surfacetemperature(T s)is presented.The37GHz vertical polarized brightness temperature isused to derive T s because it is considered the most appropriate microwave frequency fortemperature retrieval.This channel balances a reduced sensitivity to soil surfacecharacteristics with a relatively high atmospheric transmissivity.It is shown that with asimple linear relationship,accurate values for T s can be obtained from this frequency,witha theoretical bias of within1K for70%of vegetated land areas of the globe.Barren,sparsely vegetated,and open shrublands cannot be accurately described with this singlechannel approach because variable surface conditions become important.The precision ofthe retrieved land surface temperature is expected to be better than2.5K for forests and3.5K for low vegetation.This method can be used to complement existing infraredderived temperature products,especially during clouded conditions.With severalmicrowave radiometers currently in orbit,this method can be used to observe the diurnaltemperature cycles with surprising accuracy.Citation:Holmes,T.R.H.,R.A.M.De Jeu,M.Owe,and A.J.Dolman(2009),Land surface temperature from Ka band(37GHz) passive microwave observations,J.Geophys.Res.,114,D04113,doi:10.1029/2008JD010257.1.Introduction[2]Land Surface Temperature(T s)is defined as the ther-modynamic temperature of the uppermost layer of the Earth’s surface.T s is an important variable in the processes control-ling the energy and water fluxes over the interface between the Earth’s surface and the atmosphere.For continental-to global-scale modeling of these land surface processes there is need for long-term remote sensing–based T s for validation and data assimilation procedures.Furthermore,T s is a key input variable in numerous soil moisture retrieval method-ologies from space observations[e.g.,Kerr et al.,2001;Owe et al.,2001;Njoku et al.,2003;Verstraeten et al.,2006].[3]Continental-to global-scale modeling requires T s to be an area averaged value for each model grid square,typically sized between0.5and2.0°.Remote sensing is ideally suited to give area averaged values at this spatial resolution. Commonly used global T s products are derived from thermal infra red(TIR)sensors that are integrated in many satellite systems(e.g.,polar orbiting AQUA,as well as geostationary platforms like GOES and METEOSAT).The spatial resolu-tion of TIR measurements ranges from1to5km for polar orbiting satellites to50km for geostationary platforms. Assuming that the land surface emissivity is known,the actual temperature of the land surface can be derived from TIR measurements under clear skies.The TIR measurements usually need a correction for atmospheric constituents like water vapor,aerosols and particulate matter,and no obser-vations are possible under clouded conditions.The latter is an important limitation because on average50%of the land surface is covered by clouds[Rossow et al.,1993].[4]Passive microwave observations can be an alternative, or an addition to TIR sensors,for measuring T s,in particular at the Ku band(18GHz)or Ka band(37GHz).Observations within these channels are typically divided in horizontal and vertical polarization.The vertical polarized channel is better suited for temperature sensing than the horizontal channel because it is less sensitive to changes in soil moisture at incidence angles of50–55°.Of these two microwave bands, Ka band is the more appropriate frequency to retrieve T s because it balances a reduced sensitivity to soil surface characteristics with a relatively high atmospheric transmis-sivity[Colwell et al.,1983].The sensitivity to soil surface parameters is lower at Ka band than at Ku band because vegetation scatters the surface emission more effectively.As a result,even a thin vegetation cover is opaque to T B,37V emission.The use of T B,37V for deriving T s is limited by snow, frost,and frozen soil,as these conditions have a large effect on the emissivity that cannot easily be parameterized.The atmosphere appears more opaque at Ka band than at Ku band, resulting in an effect of the atmospheric temperature.Also, rain bearing clouds or active precipitation with droplets close to the size of the wavelength(8mm for37GHz)will scatter the microwave emission[Ulaby et al.,1986].T B,37V obser-vations have a spatial resolution of10to25km,which is somewhat higher than the resolution of current global land surface models,but lower than most TIR measurements.JOURNAL OF GEOPHYSICAL RESEARCH,VOL.114,D04113,doi:10.1029/2008JD010257,2009 1Department of Hydrology and Geo-Environmental Sciences,VrijeUniversiteit,Amsterdam,Netherlands.2Hydrological Sciences Branch,NASA Goddard Space Flight Center,Greenbelt,Maryland,USA.Copyright2009by the American Geophysical Union.0148-0227/09/2008JD010257[5]Besides these theoretical considerations,there is an important additional advantage to using either Ku band or Ka band and that is that these channels have been a constant part of satellite microwave missions since the late 1970s.Continuous measurements of T B ,37V are now available from 1978to the present from the Scanning Multichannel Micro-wave Radiometer (SMMR),the Special Sensor Microwave Imager (SSM/I),the TRMM Microwave Imager (TMI)and the Advanced Microwave Scanning Radiometer (AMSR-E),see Figure 1.These radiometers are all on polar orbiting satellites with global coverage,except the TMI that has an equator orbiting path between 40°north and south.In the future,the current missions will be continued and expanded (TRMM by the Global Precipitation Monitoring mission (GPM)Microwave Imager,AMSR-II on the GCOM-W,and a new Microwave Radiometer Imager (MWRI)is planned on the Chinese FY-3).[6]At this point it is important to qualify how T s is defined.The depth of the surface layer that T s refers to depends on the sensor and the composition of the land surface in the sensor footprint.For bare surfaces,T s represents the soil temperature at a shallow depth that depends on view angle,wavelength,and the surface characteristics (e.g.,roughness,wetness,and soil texture).This thermal sampling depth is $50m m for TIR frequencies,and at 37GHz between 1mm for a wet soil and up to 10mm for a dry soil [Ulaby et al.,1986].When the surface is covered with vegetation,T s represents the canopy surface temperature.In this paper,T s refers to the area weighted average of the temperatures of the various land covers within a specific scene.As will be shown,at 37GHz the vegetation is relatively opaque,and for most of the Earth (60%)T s will effectively represent the vegeta-tion canopy temperature.[7]In the past,several authors used the 37GHz signal to derive surface temperature for different well defined study sites and observed a strong linear relationship between T B ,37V and T s [e.g.,Owe et al.,2001;Owe and Van de Griend ,2001;De Jeu and Owe ,2003].Other authors have tried multi-frequency approaches [e.g.,Fily et al.,2003],but these techniques have been more difficult to apply globally.This paper will continue with the single frequency approach and examine the potential of 37GHz passive microwave obser-vations for deriving land surface temperature at global scales.Simulation studies are used to test the theoretical influence of the most important rs that affect the relationshipbetween the T s and the measured T B ,37V at the top of the atmosphere.On the basis of these simulations,a single linear relationship between T s and T B ,37V is derived,that can then be applied parisons to field data are used to validate this approach.2.Materials and Methods2.1.Ground Measurements[8]FLUXNET is a network of meteorological towers spanning the entire globe [Baldocchi et al.,2001].From this database 17sites are selected that have good records of longwave radiation,sensible heat flux and air temperature for the year 2005,with a temporal resolution of a half hour.Secondary variables for this analysis are net radiation and wind speed.These sites represent a variety of vegetation types and climates (see Table 1).The dominant IGBP land cover class for the surrounding 0.15°grid box is based on the MOD12Q1Land Cover Product [Belward et al.,1999].The reader is referred to for a detailed description of all sites.[9]As discussed previously,T s is the integrated temper-ature of all the land surface covers in a given radiometric footprint.It follows then that it cannot be measured at a single point,nor is it easily estimated by multiple observa-tions of the soil and canopy surfaces.One way to address this problem is by comparing T s with the emitted longwave radiation.The longwave flux is a direct function of the physical temperature of the land surface,and like T s ,is representative of all the radiating surfaces in the sensor’s view.The benefit of this approach is that no temperature conversions need to be made to compare temperatures from different depths.Instead,the longwave emissivity (e )must be determined for each site separately to calculate the land surface temperature,now denoted T LW .The procedure to determine e is outlined below.[10]Although the use of the longwave flux makes it easier to compare the satellite derived temperature with ground measurements,the footprint of the flux tower measurement remains much smaller than that of the satellite.Heterogeneity within the satellite footprint can therefore cause a bias between the two measurements,if the location of the flux tower is not representative for the 25Â25km area.For this reason the satellite derived land surface temperature will principally be validated against the ground measurements in terms of correlation R 2and standard error of estimate (SEE),and not in terms of bias.2.2.Longwave Emissivity[11]The relationship between outgoing longwave radia-tion (F LW ,up )and longwave surface temperature,denoted T LW ,is based on the Stefan-Boltzmann law,according to:F LW ;up ¼es T 4LWð1Þwhere e is the broadband emissivity for the entire TIRspectral region and s is the Stefan-Boltzmann’s constant (s =5.6697Â10À8Wm À2K À4).[12]According to Penman [1948],the sensible heat flux (H )can be described as H =C D T ,with C representing vegetation-dependent parameters and boundary conditions.D T is the temperature difference between the landsurfaceFigure 1.Operating years of microwave radiometers in orbit.The SSM/I sensor has been on several overlapping DMSP missions,and the AMSR-E sensor is included in the AQUA and WINDSAT missions.and the air.By this definition,H=0when D T=0.It follows that for a series of measurements the regression line of H against D T goes through zero.The e can now be determined for each field site by optimizing the D T so that the regression(forced through zero)of H against D T has the lowest RMS error.This procedure(A)is applied for every month separately of the2005data and gives robust results when the D T explains a substantial part of the variance in H (we use a minimum R2of0.5).The H values are considered reliable when the net radiation is more than25WmÀ2and the wind speed is more than2msÀ1.Figure2a shows an example of the optimized D T from the cropland site C in Brookings,South Dakota,US,for August2005.The points denoted by pluses are used for the optimization,the dots are the values that have either a low net radiation flux or a low wind speed.[13]The above procedure A does not work when the variation in H cannot be explained by D T.This is the case when the roughness length of the vegetation is high,resulting in only a minimal difference between the vegetation and air temperature(T a).For this situation it is assumed that T s=T a, and that the integrated temperature T s is fully determined by the canopy temperature.The e is now derived by minimizing the RMS error between T s and T a.Figure2b shows an example of the resulting D T according to this procedure B, for the forest site P in North Carolina,US,for August2005. Figure2b illustrates that the variance in H cannot be explained by the temperature difference between the canopy and the air.[14]The retrieved e per month is indicated in Figure3for each site.The emissivity as determined following procedure A is indicated by a dot for R2>0.5.For the months with a lower correlation,the value is rejected and the alternative procedure B is used where T s=T a.The emissivity according to this method is indicated by a circle.If more than4months have a high squared correlation,the average value for the whole year is based on procedure A,otherwise it is based on the alternative method.The resulting average e for each site is listed in the graph and indicated by the horizontal line.It represents the effective longwave emissivity for the footprint of the flux measurements.Note that the emissivity can change during the year,especially if the surface is barren for part of the year(e.g.,sites A and H).[15]The values may be compared to MODIS emissivities for the wavelengths between8and12m m.Snyder[1999] lists them for the same IGBP classes:grasslands(e=0.96); croplands(e=0.97–0.98);deciduous broadleaf forest(e= 0.97);and evergreen needle leaf forest(e=0.99).The emissivities are comparable,although the field values for cropland have a high variability.The high emissivity for the cropland of Cabauw(site F)can be explained by high percentage of water(with e=0.99).Black hills(site O)has a low emissivity compared to the rest of the forest sites and the MODIS emissivity,this is probably because of the open canopy.[16]The year averages of e are subsequently used to calculate T LW for each site.For the comparison with the satellite observations,the ground measurement of T LW is selected that is within15minutes of the satellite observation.2.3.Satellite Observations[17]Vertically polarized brightness temperatures in the Ka band are currently observed by various satellites(see Figure1).In this study,we analyze the brightness tempera-ture as observed by AMSR-E on board the Sun synchronous and polar orbiting AQUA satellite[Ashcroft and Wentz,Table1.Geographical Location,IGBP Vegetation Class,and LW Emissivity for17Field SitesID Site Name Latitude Longitude Vegetation Class at Site Vegetation Class0.15°Emissivity at SiteLow-Vegetation GroupA Arizona,a US31.59°N110.51°W grasslands grasslands0.946B Fort Peck Montana,a US48.31°N105.10°W grasslands cropland/natural vegetation mosaic0.961C Brookings South Dakota,a US44.35°N96.84°W croplands croplands0.971D Bondville Illinois,a US40.01°N88.29°W croplands croplands0.961E Bondville comp.Illinois,a US40.01°N88.29°W croplands croplands0.938F Cabauw,b NL51.97°N 4.93°E croplands croplands0.995G Gebesee,c DE51.10°N10.91°E croplands croplands0.987H Mitra II,d Evora,PT38.54°N8.00°E cropland/natural vegetation mosaic cropland/natural vegetation mosaic0.980High-Vegetation GroupI Ozark Missouri,a US38.74°N92.20°W deciduous broadleaf forest cropland/natural vegetation mosaic0.970J Morgan Monroe Indiana,US39.32°N86.41°W deciduous broadleaf forest deciduous broadleaf forest0.995K Collelongo beech,e IT41.85°N13.59°E deciduous broadleaf forest mixed forest0.960L Mehrstedt1,f DE51.28°N10.65°E mixed forest croplands0.989M Loobos,g NL52.17°N 5.74°E evergreen needleleaf forest croplands0.995N Le Brai,h FR44.72°N0.77°W evergreen needleleaf forest mixed forest0.988O Black Hills South Dakota,a US44.16°N103.65°E evergreen needleleaf forest evergreen needleleaf forest0.971P North Carolina,US35.98°N79.09°W evergreen needleleaf forest mixed forests0.990Q Yatir,i IL31.35°N35.05°E evergreen needleleaf forest barren or sparsely vegetated0.994a Hollinger et al.[2005].b Beljaars and Bosveld[1997].c Anthoni et al.[2004].d David et al.[2004].e DeAngelis et al.[1996].f Scherer-Lorenzen et al.[2007].g Dolman et al.[2002].h Kowalski et al.[2003].i Grunzweig et al.[2003].2003].AMSR-E has a 36.5GHz channel at 55°incidence angle.Equator overpass times are at 1330LT for the ascending path and 0130LT for the descending path.The revisit time at the equator is $3days.For each ground location of Table 1we have extracted a time series of satellite brightness temperature observations for the year 2005based on the Level 2A spatially resampled swath data.This time series includes the nearest points within either the ascending or the descending over passes.[18]For two selected ground sites (North Carolina (P)and Montana (B),US)T B ,37V is extracted for two additional satellites.The first one is the TMI on board the equator orbiting TRMM satellite [Kummerow et al.,1998].The overpass times of TRMM vary through the year.The second radiometer is the SSM/oard the polar orbiting DMSP[Armstrong et al.,1994].Data are extracted from the fl3platform with equator overpass times at 0600and 1800LT and a 4–5day revisit time.2.4.Radiative Transfer Model for Ka Band[19]Brightness temperature as measured by satellite sensors can be simulated by radiative transfer models.A commonly used model that describes the microwave emis-sion above a vegetated surface is the zero-order scattering model,sometimes called the omega-tau model [Mo et al.,1982].In this paper we use this omega-tau model to simulate the 37GHz vertically polarized brightness temperature (T B ,37V )at the top of the atmosphere.[20]The dielectric constant is modeled according to the mixing model by Wang and Schmugge [1980],which is adapted for high frequencies by Calvet et al.[1995].The effect of roughness on the emissivity is corrected with the parameters Q for the cross polarization and h for the roughness height [Wang and Choudhury ,1981].[21]The temperature of the soil surface is considered the same as the canopy temperature (T s =T c ).Published values of single scattering albedo (w )at this frequency are rare,especially for natural vegetation.Pampaloni and Paloscia [1986]found values of w =0.03to w =0.06for crops,while values averaging around w =0.1were found for savannah surfaces [Van de Griend and Owe ,1994].[22]The atmospheric transmissivity is a function of the zenith atmospheric opacity (t a )and incidence angle (G a =e Àt a /cos q ).At 37GHz,the atmospheric opacity varies between t a =0.05and t a =0.20depending on atmospheric water content [Ulaby et al.,1986].2.5.Simulation Experiments[23]The radiative transfer model for T B ,37V is used to test the sensitivity of the T B ,37V /T s relationship to the most important input parameters.For this purpose simulations were conducted that model the T B ,37V for T s =300K and for two scenarios;a typical vegetated surface and an extremely dry,bare surface (see Table 2).The purpose of these simulations is to calibrate the radiative transfer model and to determine the sensitivity to various input parameters.[24]The vegetated scenario has medium volumetric soil water content (W c )of 25%.The soil texture parameters are typical for a silt loam,with a porosity of 50%and a wilting point of 13%.The texture of a silt loam is chosen because the wilting point value is between the values for sand (3%)and clay (27%).At Ka band,even a thin vegetation cover becomes nontransmissive to the emission from the surface.Therefore,the transmissivity will in general be low and a default value of G v =0.2is used.The atmosphere in this scenario is typical for a temperate climate,with 9mm of precipitable water and no liquid water.This corresponds to a t a =0.05(or G a =0.9at an incidence angle of 55°).[25]The dry,bare scenario has a low W c of 10%and high vegetation transmissivity (G v =0.9).The soil texture param-eters are typical for sand,with a porosity of 44%and a wilting point of 3%.Sand is chosen because the persistently dry surfaces do not accumulate clay particles.The atmo-sphere in this scenario is typical for a desert climate with 45mm of precipitable water and no cloud liquid water content.This corresponds to a t a =0.15(or G a =0.74at an incidence angle of 55°).Figure 2.Sensible heat flux against optimized D T for (a)cropland site C and (b)forest site P.The cropland site is an example where procedure A works well because of a high R 2between H and D T for points denoted by pluses.The forest site is an example with no correlation where procedure B has to be applied.Figure3.Monthly longwave emissivities for the ground sites.The letters above each plot refer to the site IDs in Table1.The average value for the whole year is indicated by the horizontal line,and markers indicate retrieval procedure:procedure A(dot)and procedure B(circle).[26]The surface roughness,cross polarization,and single scattering albedo are somewhat difficult to quantify,so they are used to calibrate the radiative transfer model to approx-imate the derived general relation in both scenarios.Further-more,we describe the effective air temperature as a function of the surface temperature[Bevis et al.,1992]:T e=70.2+ 0.72T s(all units in Kelvin).[27]The influence of each of the input parameters on the simulated T B,37V is tested by varying them over a realistic range,while holding the other parameters constant.Varia-tions from the calibrated default scenario will indicate sensitivity.Secondly,the sensitivity of the model to spatial variations in soil moisture and vegetation density is tested with observed global maps of these input variables.3.Results3.1.General Solution[28]The aim of this paper is to test if a single channel approach can be used to derive the land surface temperature globally.For this reason we test a simple linear relation that is derived from the ground observations(Table1)and the AMSR-E37Ghz,vertical polarized channel.Three sites are excluded from this analysis because the flux tower is not representative for the satellite footprint.The excluded sites are sites F,Cabauw,Netherlands,too much open water;Q,Yatir,Israel,small forest in barren surroundings; and H,Mitra2,Portugal,a grass site in open Oak woodland. The resulting sites have a range of different vegetation types and climates.Figure4shows the scatter plot of all the data. The relationship between T B,37V and T s that best describes these observations is:T s¼1:11T B;37VÀ15:2for T B;37V>259:8ð2Þ[29]The corresponding error of4.5K and correlation of R2=0.84are the upper limit of expected error for this method and reflect errors in the model,in the ground measurement and errors due to the heterogeneity of the satellite footprint as compared to the ground site.The lower threshold of suitable brightness temperatures of259.8K marks the divide between frozen and unfrozen conditions at a physical temperature of273.15K.The change in emission is highly nonlinear over this phase change,and is not covered by the regression equation.The errors as caused by this simplification of the radiative transfer model are evaluated in simulation experiments in the following two sections.In section3.4,the ground sites will be analyzed individually to assess the errors resulting from the ground measurements and heterogeneity of the satellite footprint.3.2.Error Simulations[30]The simplification of the radiative transfer model intoa single linear relationship(equation(2))causes a difference in the retrieved T s if the actual parameters deviate from the default scenarios.Assuming that the T s as derived from the radiative transfer model is the‘‘true’’temperature,the devi-ation from this value is regarded as an error.The potential size of these errors is estimated for each input parameter individ-ually.In Figures5–7the most important parameters are tested for their influence on the deviation in T s as compared to the linear relation.In Figures5–7the calibrated value of the evaluated parameter is indicated by a vertical dotted line.[31]Figure5a shows how T s will be affected by soil moisture at different vegetation densities.For a surface with dense vegetation(G v=0.2),the error as introduced by the soil moisture conditions will not exceed1.5K.However, the soil moisture content becomes critical for a vegetation transmissivity greater than0.35.As the vegetation density decreases,the range of W c values that result in a bias within the acceptable limits decreases.At the same time,the W c that minimizes the bias,decreases from the default value of 25%at G v=0.2,to21%for G v=0.35,to15%for a sparsely vegetated surface with G v=0.5.Although the error in the retrieved T s over areas with low vegetation densities can be within the limits for part of the year,it is likely that it exceeds the limit for some parts of the year.For this reason applying the T s retrieval to areas where the G v is higher than 0.5should be done with caution because small variations in W c will result in a high bias.These areas roughly corre-spond to IGBP classes barren and sparsely vegetated and open shrublands.[32]Also shown in Figure5are the effect on T s of the single scattering albedo,roughness,and wilting point at G v=0.2and G v=0.5.The errors for the intermediateTable2.Input Parameters for the Simulation Experiments aVegetated Surface Dry,Bare SurfaceWater content(%)255Soil type Silt loam SandVegetation transmissivity(À)0.20.9Atmospheric transmissivity(À)0.90.74Roughness(À)0.2(0.035)0.2(0.035)Cross polarization(À)0.2(0.039)0.2(0.039)Single scattering albedo(À)0.06(0.01)0.06(0.01)a In parentheses is the standard deviation attributed to theparameter.Figure 4.Regression of satellite observations againstground observations of14FLUXNET sites.vegetation value (G v =0.35)are not shown for these plots,but are understood to be in between the given examples.The corresponding moisture value is chosen that minimizes the error,respectively 25%and 15%(see Figure 5a).The single scattering albedo (Figure 5b)has a strong effect on the error when the vegetation is dense.The roughness (Figure 5c)has a much smaller effect and is not expected to result in errors of more than 1K for vegetation densities with G v <0.5.The texture,and in particular the wilting point,results in errors similar to the roughness,although less linear (Figure 5d).It does not affect the T B ,37V in the most densely vegetated scenario,but for surfaces with less dense vegetation the error can increase to 2K for a silt loam at G v =0.5.[33]The same parameters are tested for a dry,bare surface (see Table 2).The effect of soil moisture under these conditions is extremely strong (see Figure 6a,and note that the axis in Figure 6a is elongated).The moisture value with minimal error is now only 8%and the moisture range with acceptable errors is very small.The single scattering albedo(Figure 6b)has now a weak effect on the error because the vegetation is very sparse.The roughness (Figure 6c)and texture (Figure 6d)have a strong effect in this scenario but are not expected to result in errors of more than 3K.The wilting point in particular will in general be low for desert type scenarios.[34]Not all satellite radiometers have exactly the same frequency channel and incidence angle within the Ka band (see Figure 1).In the simulations an incidence angle of 55°is used,but historically incidence angles between 50°and 55°have been used.For vegetated surfaces this is not expected to make a significant impact,but for bare surfaces this results in a maximum bias of À1K at 50°(Figure 7a).The exact frequency of the Ka band channel for most radio-meters has been 37.0GHz,only for the latest AMSR-E instrument this channel is at 36.5GHz.This small difference is not expected to affect the results (Figure 7b),but it can cause small differences in the atmospherictransmissivity.Figure 5.Deviations in T s (D T (K))from vegetated configuration for selected parameters.Vertical line indicates calibratedvalue.Figure 6.As Figure 5,for bare configuration.。